Research Methods
- Getting Started
- Literature Review Research
- Research Design
- Research Design By Discipline
- SAGE Research Methods
- Teaching with SAGE Research Methods
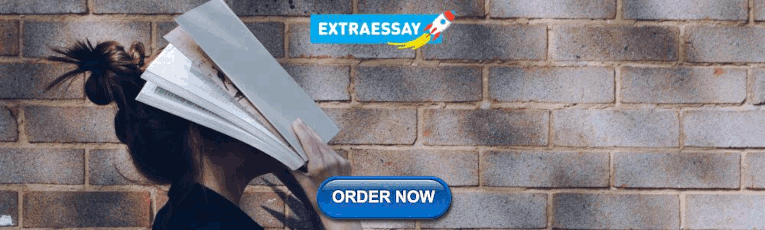
Literature Review
- What is a Literature Review?
- What is NOT a Literature Review?
- Purposes of a Literature Review
- Types of Literature Reviews
- Literature Reviews vs. Systematic Reviews
- Systematic vs. Meta-Analysis
Literature Review is a comprehensive survey of the works published in a particular field of study or line of research, usually over a specific period of time, in the form of an in-depth, critical bibliographic essay or annotated list in which attention is drawn to the most significant works.
Also, we can define a literature review as the collected body of scholarly works related to a topic:
- Summarizes and analyzes previous research relevant to a topic
- Includes scholarly books and articles published in academic journals
- Can be an specific scholarly paper or a section in a research paper
The objective of a Literature Review is to find previous published scholarly works relevant to an specific topic
- Help gather ideas or information
- Keep up to date in current trends and findings
- Help develop new questions
A literature review is important because it:
- Explains the background of research on a topic.
- Demonstrates why a topic is significant to a subject area.
- Helps focus your own research questions or problems
- Discovers relationships between research studies/ideas.
- Suggests unexplored ideas or populations
- Identifies major themes, concepts, and researchers on a topic.
- Tests assumptions; may help counter preconceived ideas and remove unconscious bias.
- Identifies critical gaps, points of disagreement, or potentially flawed methodology or theoretical approaches.
- Indicates potential directions for future research.
All content in this section is from Literature Review Research from Old Dominion University
Keep in mind the following, a literature review is NOT:
Not an essay
Not an annotated bibliography in which you summarize each article that you have reviewed. A literature review goes beyond basic summarizing to focus on the critical analysis of the reviewed works and their relationship to your research question.
Not a research paper where you select resources to support one side of an issue versus another. A lit review should explain and consider all sides of an argument in order to avoid bias, and areas of agreement and disagreement should be highlighted.
A literature review serves several purposes. For example, it
- provides thorough knowledge of previous studies; introduces seminal works.
- helps focus one’s own research topic.
- identifies a conceptual framework for one’s own research questions or problems; indicates potential directions for future research.
- suggests previously unused or underused methodologies, designs, quantitative and qualitative strategies.
- identifies gaps in previous studies; identifies flawed methodologies and/or theoretical approaches; avoids replication of mistakes.
- helps the researcher avoid repetition of earlier research.
- suggests unexplored populations.
- determines whether past studies agree or disagree; identifies controversy in the literature.
- tests assumptions; may help counter preconceived ideas and remove unconscious bias.
As Kennedy (2007) notes*, it is important to think of knowledge in a given field as consisting of three layers. First, there are the primary studies that researchers conduct and publish. Second are the reviews of those studies that summarize and offer new interpretations built from and often extending beyond the original studies. Third, there are the perceptions, conclusions, opinion, and interpretations that are shared informally that become part of the lore of field. In composing a literature review, it is important to note that it is often this third layer of knowledge that is cited as "true" even though it often has only a loose relationship to the primary studies and secondary literature reviews.
Given this, while literature reviews are designed to provide an overview and synthesis of pertinent sources you have explored, there are several approaches to how they can be done, depending upon the type of analysis underpinning your study. Listed below are definitions of types of literature reviews:
Argumentative Review This form examines literature selectively in order to support or refute an argument, deeply imbedded assumption, or philosophical problem already established in the literature. The purpose is to develop a body of literature that establishes a contrarian viewpoint. Given the value-laden nature of some social science research [e.g., educational reform; immigration control], argumentative approaches to analyzing the literature can be a legitimate and important form of discourse. However, note that they can also introduce problems of bias when they are used to to make summary claims of the sort found in systematic reviews.
Integrative Review Considered a form of research that reviews, critiques, and synthesizes representative literature on a topic in an integrated way such that new frameworks and perspectives on the topic are generated. The body of literature includes all studies that address related or identical hypotheses. A well-done integrative review meets the same standards as primary research in regard to clarity, rigor, and replication.
Historical Review Few things rest in isolation from historical precedent. Historical reviews are focused on examining research throughout a period of time, often starting with the first time an issue, concept, theory, phenomena emerged in the literature, then tracing its evolution within the scholarship of a discipline. The purpose is to place research in a historical context to show familiarity with state-of-the-art developments and to identify the likely directions for future research.
Methodological Review A review does not always focus on what someone said [content], but how they said it [method of analysis]. This approach provides a framework of understanding at different levels (i.e. those of theory, substantive fields, research approaches and data collection and analysis techniques), enables researchers to draw on a wide variety of knowledge ranging from the conceptual level to practical documents for use in fieldwork in the areas of ontological and epistemological consideration, quantitative and qualitative integration, sampling, interviewing, data collection and data analysis, and helps highlight many ethical issues which we should be aware of and consider as we go through our study.
Systematic Review This form consists of an overview of existing evidence pertinent to a clearly formulated research question, which uses pre-specified and standardized methods to identify and critically appraise relevant research, and to collect, report, and analyse data from the studies that are included in the review. Typically it focuses on a very specific empirical question, often posed in a cause-and-effect form, such as "To what extent does A contribute to B?"
Theoretical Review The purpose of this form is to concretely examine the corpus of theory that has accumulated in regard to an issue, concept, theory, phenomena. The theoretical literature review help establish what theories already exist, the relationships between them, to what degree the existing theories have been investigated, and to develop new hypotheses to be tested. Often this form is used to help establish a lack of appropriate theories or reveal that current theories are inadequate for explaining new or emerging research problems. The unit of analysis can focus on a theoretical concept or a whole theory or framework.
* Kennedy, Mary M. "Defining a Literature." Educational Researcher 36 (April 2007): 139-147.
All content in this section is from The Literature Review created by Dr. Robert Larabee USC
Robinson, P. and Lowe, J. (2015), Literature reviews vs systematic reviews. Australian and New Zealand Journal of Public Health, 39: 103-103. doi: 10.1111/1753-6405.12393
What's in the name? The difference between a Systematic Review and a Literature Review, and why it matters . By Lynn Kysh from University of Southern California
Systematic review or meta-analysis?
A systematic review answers a defined research question by collecting and summarizing all empirical evidence that fits pre-specified eligibility criteria.
A meta-analysis is the use of statistical methods to summarize the results of these studies.
Systematic reviews, just like other research articles, can be of varying quality. They are a significant piece of work (the Centre for Reviews and Dissemination at York estimates that a team will take 9-24 months), and to be useful to other researchers and practitioners they should have:
- clearly stated objectives with pre-defined eligibility criteria for studies
- explicit, reproducible methodology
- a systematic search that attempts to identify all studies
- assessment of the validity of the findings of the included studies (e.g. risk of bias)
- systematic presentation, and synthesis, of the characteristics and findings of the included studies
Not all systematic reviews contain meta-analysis.
Meta-analysis is the use of statistical methods to summarize the results of independent studies. By combining information from all relevant studies, meta-analysis can provide more precise estimates of the effects of health care than those derived from the individual studies included within a review. More information on meta-analyses can be found in Cochrane Handbook, Chapter 9 .
A meta-analysis goes beyond critique and integration and conducts secondary statistical analysis on the outcomes of similar studies. It is a systematic review that uses quantitative methods to synthesize and summarize the results.
An advantage of a meta-analysis is the ability to be completely objective in evaluating research findings. Not all topics, however, have sufficient research evidence to allow a meta-analysis to be conducted. In that case, an integrative review is an appropriate strategy.
Some of the content in this section is from Systematic reviews and meta-analyses: step by step guide created by Kate McAllister.
- << Previous: Getting Started
- Next: Research Design >>
- Last Updated: Aug 21, 2023 4:07 PM
- URL: https://guides.lib.udel.edu/researchmethods

Research Methods and Design
- Action Research
- Case Study Design
Literature Review
- Quantitative Research Methods
- Qualitative Research Methods
- Mixed Methods Study
- Indigenous Research and Ethics This link opens in a new window
- Identifying Empirical Research Articles This link opens in a new window
- Research Ethics and Quality
- Data Literacy
- Get Help with Writing Assignments
A literature review is a discussion of the literature (aka. the "research" or "scholarship") surrounding a certain topic. A good literature review doesn't simply summarize the existing material, but provides thoughtful synthesis and analysis. The purpose of a literature review is to orient your own work within an existing body of knowledge. A literature review may be written as a standalone piece or be included in a larger body of work.
You can read more about literature reviews, what they entail, and how to write one, using the resources below.
Am I the only one struggling to write a literature review?
Dr. Zina O'Leary explains the misconceptions and struggles students often have with writing a literature review. She also provides step-by-step guidance on writing a persuasive literature review.
An Introduction to Literature Reviews
Dr. Eric Jensen, Professor of Sociology at the University of Warwick, and Dr. Charles Laurie, Director of Research at Verisk Maplecroft, explain how to write a literature review, and why researchers need to do so. Literature reviews can be stand-alone research or part of a larger project. They communicate the state of academic knowledge on a given topic, specifically detailing what is still unknown.
This is the first video in a whole series about literature reviews. You can find the rest of the series in our SAGE database, Research Methods:

Videos covering research methods and statistics
Identify Themes and Gaps in Literature (with real examples) | Scribbr
Finding connections between sources is key to organizing the arguments and structure of a good literature review. In this video, you'll learn how to identify themes, debates, and gaps between sources, using examples from real papers.
4 Tips for Writing a Literature Review's Intro, Body, and Conclusion | Scribbr
While each review will be unique in its structure--based on both the existing body of both literature and the overall goals of your own paper, dissertation, or research--this video from Scribbr does a good job simplifying the goals of writing a literature review for those who are new to the process. In this video, you’ll learn what to include in each section, as well as 4 tips for the main body illustrated with an example.
- Literature Review This chapter in SAGE's Encyclopedia of Research Design describes the types of literature reviews and scientific standards for conducting literature reviews.
- UNC Writing Center: Literature Reviews This handout from the Writing Center at UNC will explain what literature reviews are and offer insights into the form and construction of literature reviews in the humanities, social sciences, and sciences.
- Purdue OWL: Writing a Literature Review The overview of literature reviews comes from Purdue's Online Writing Lab. It explains the basic why, what, and how of writing a literature review.
Organizational Tools for Literature Reviews
One of the most daunting aspects of writing a literature review is organizing your research. There are a variety of strategies that you can use to help you in this task. We've highlighted just a few ways writers keep track of all that information! You can use a combination of these tools or come up with your own organizational process. The key is choosing something that works with your own learning style.
Citation Managers
Citation managers are great tools, in general, for organizing research, but can be especially helpful when writing a literature review. You can keep all of your research in one place, take notes, and organize your materials into different folders or categories. Read more about citations managers here:
- Manage Citations & Sources
Concept Mapping
Some writers use concept mapping (sometimes called flow or bubble charts or "mind maps") to help them visualize the ways in which the research they found connects.

There is no right or wrong way to make a concept map. There are a variety of online tools that can help you create a concept map or you can simply put pen to paper. To read more about concept mapping, take a look at the following help guides:
- Using Concept Maps From Williams College's guide, Literature Review: A Self-guided Tutorial
Synthesis Matrix
A synthesis matrix is is a chart you can use to help you organize your research into thematic categories. By organizing your research into a matrix, like the examples below, can help you visualize the ways in which your sources connect.
- Walden University Writing Center: Literature Review Matrix Find a variety of literature review matrix examples and templates from Walden University.
- Writing A Literature Review and Using a Synthesis Matrix An example synthesis matrix created by NC State University Writing and Speaking Tutorial Service Tutors. If you would like a copy of this synthesis matrix in a different format, like a Word document, please ask a librarian. CC-BY-SA 3.0
- << Previous: Case Study Design
- Next: Quantitative Research Methods >>
- Last Updated: Feb 6, 2024 9:20 AM
CityU Home - CityU Catalog

Have a language expert improve your writing
Run a free plagiarism check in 10 minutes, automatically generate references for free.
- Knowledge Base
- Dissertation
- What Is a Research Methodology? | Steps & Tips
What Is a Research Methodology? | Steps & Tips
Published on 25 February 2019 by Shona McCombes . Revised on 10 October 2022.
Your research methodology discusses and explains the data collection and analysis methods you used in your research. A key part of your thesis, dissertation, or research paper, the methodology chapter explains what you did and how you did it, allowing readers to evaluate the reliability and validity of your research.
It should include:
- The type of research you conducted
- How you collected and analysed your data
- Any tools or materials you used in the research
- Why you chose these methods
- Your methodology section should generally be written in the past tense .
- Academic style guides in your field may provide detailed guidelines on what to include for different types of studies.
- Your citation style might provide guidelines for your methodology section (e.g., an APA Style methods section ).
Instantly correct all language mistakes in your text
Be assured that you'll submit flawless writing. Upload your document to correct all your mistakes.
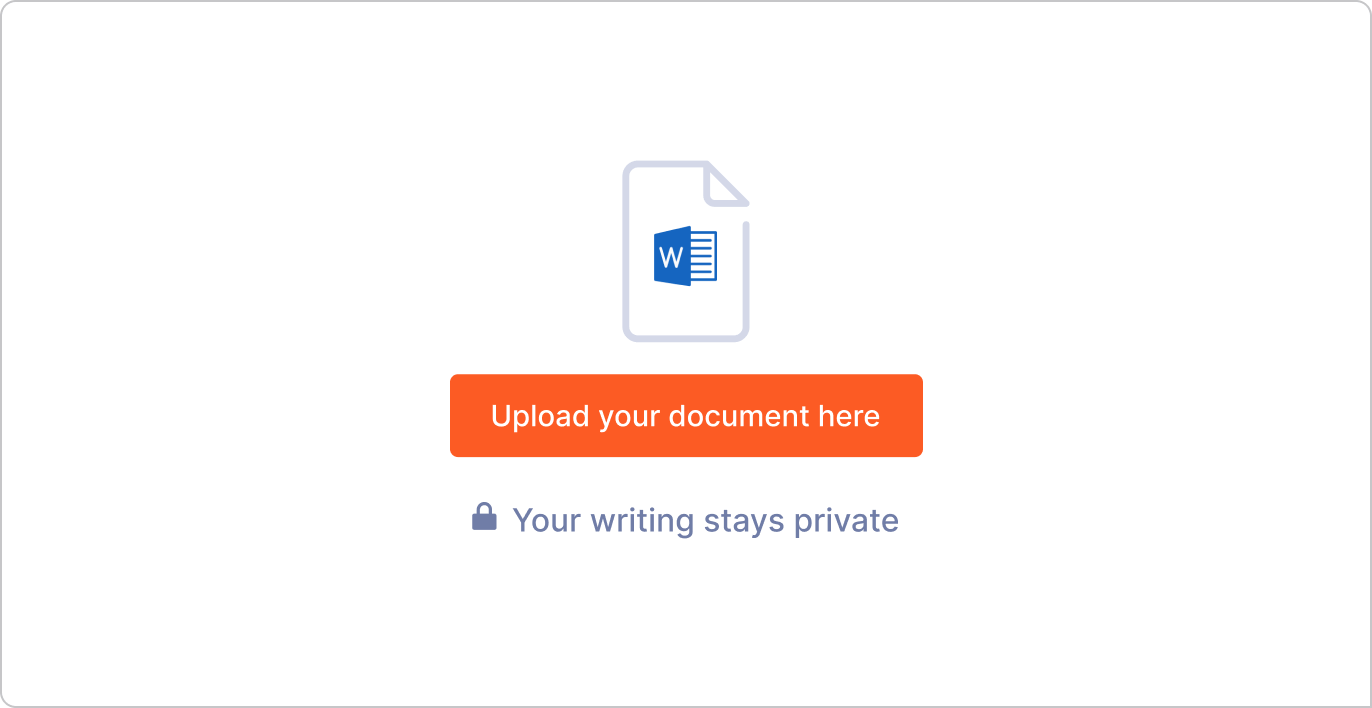
Table of contents
How to write a research methodology, why is a methods section important, step 1: explain your methodological approach, step 2: describe your data collection methods, step 3: describe your analysis method, step 4: evaluate and justify the methodological choices you made, tips for writing a strong methodology chapter, frequently asked questions about methodology.
Prevent plagiarism, run a free check.
Your methods section is your opportunity to share how you conducted your research and why you chose the methods you chose. It’s also the place to show that your research was rigorously conducted and can be replicated .
It gives your research legitimacy and situates it within your field, and also gives your readers a place to refer to if they have any questions or critiques in other sections.
You can start by introducing your overall approach to your research. You have two options here.
Option 1: Start with your “what”
What research problem or question did you investigate?
- Aim to describe the characteristics of something?
- Explore an under-researched topic?
- Establish a causal relationship?
And what type of data did you need to achieve this aim?
- Quantitative data , qualitative data , or a mix of both?
- Primary data collected yourself, or secondary data collected by someone else?
- Experimental data gathered by controlling and manipulating variables, or descriptive data gathered via observations?
Option 2: Start with your “why”
Depending on your discipline, you can also start with a discussion of the rationale and assumptions underpinning your methodology. In other words, why did you choose these methods for your study?
- Why is this the best way to answer your research question?
- Is this a standard methodology in your field, or does it require justification?
- Were there any ethical considerations involved in your choices?
- What are the criteria for validity and reliability in this type of research ?
Once you have introduced your reader to your methodological approach, you should share full details about your data collection methods .
Quantitative methods
In order to be considered generalisable, you should describe quantitative research methods in enough detail for another researcher to replicate your study.
Here, explain how you operationalised your concepts and measured your variables. Discuss your sampling method or inclusion/exclusion criteria, as well as any tools, procedures, and materials you used to gather your data.
Surveys Describe where, when, and how the survey was conducted.
- How did you design the questionnaire?
- What form did your questions take (e.g., multiple choice, Likert scale )?
- Were your surveys conducted in-person or virtually?
- What sampling method did you use to select participants?
- What was your sample size and response rate?
Experiments Share full details of the tools, techniques, and procedures you used to conduct your experiment.
- How did you design the experiment ?
- How did you recruit participants?
- How did you manipulate and measure the variables ?
- What tools did you use?
Existing data Explain how you gathered and selected the material (such as datasets or archival data) that you used in your analysis.
- Where did you source the material?
- How was the data originally produced?
- What criteria did you use to select material (e.g., date range)?
The survey consisted of 5 multiple-choice questions and 10 questions measured on a 7-point Likert scale.
The goal was to collect survey responses from 350 customers visiting the fitness apparel company’s brick-and-mortar location in Boston on 4–8 July 2022, between 11:00 and 15:00.
Here, a customer was defined as a person who had purchased a product from the company on the day they took the survey. Participants were given 5 minutes to fill in the survey anonymously. In total, 408 customers responded, but not all surveys were fully completed. Due to this, 371 survey results were included in the analysis.
Qualitative methods
In qualitative research , methods are often more flexible and subjective. For this reason, it’s crucial to robustly explain the methodology choices you made.
Be sure to discuss the criteria you used to select your data, the context in which your research was conducted, and the role you played in collecting your data (e.g., were you an active participant, or a passive observer?)
Interviews or focus groups Describe where, when, and how the interviews were conducted.
- How did you find and select participants?
- How many participants took part?
- What form did the interviews take ( structured , semi-structured , or unstructured )?
- How long were the interviews?
- How were they recorded?
Participant observation Describe where, when, and how you conducted the observation or ethnography .
- What group or community did you observe? How long did you spend there?
- How did you gain access to this group? What role did you play in the community?
- How long did you spend conducting the research? Where was it located?
- How did you record your data (e.g., audiovisual recordings, note-taking)?
Existing data Explain how you selected case study materials for your analysis.
- What type of materials did you analyse?
- How did you select them?
In order to gain better insight into possibilities for future improvement of the fitness shop’s product range, semi-structured interviews were conducted with 8 returning customers.
Here, a returning customer was defined as someone who usually bought products at least twice a week from the store.
Surveys were used to select participants. Interviews were conducted in a small office next to the cash register and lasted approximately 20 minutes each. Answers were recorded by note-taking, and seven interviews were also filmed with consent. One interviewee preferred not to be filmed.
Mixed methods
Mixed methods research combines quantitative and qualitative approaches. If a standalone quantitative or qualitative study is insufficient to answer your research question, mixed methods may be a good fit for you.
Mixed methods are less common than standalone analyses, largely because they require a great deal of effort to pull off successfully. If you choose to pursue mixed methods, it’s especially important to robustly justify your methods here.
The only proofreading tool specialized in correcting academic writing
The academic proofreading tool has been trained on 1000s of academic texts and by native English editors. Making it the most accurate and reliable proofreading tool for students.
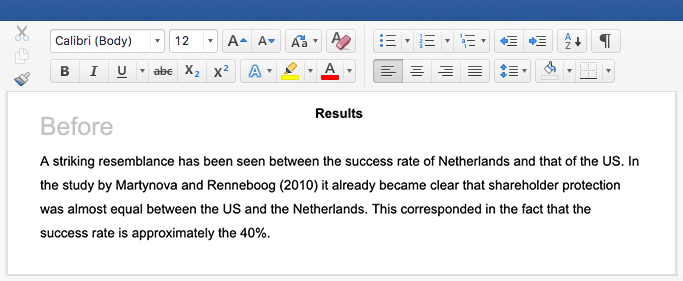
Correct my document today
Next, you should indicate how you processed and analysed your data. Avoid going into too much detail: you should not start introducing or discussing any of your results at this stage.
In quantitative research , your analysis will be based on numbers. In your methods section, you can include:
- How you prepared the data before analysing it (e.g., checking for missing data , removing outliers , transforming variables)
- Which software you used (e.g., SPSS, Stata or R)
- Which statistical tests you used (e.g., two-tailed t test , simple linear regression )
In qualitative research, your analysis will be based on language, images, and observations (often involving some form of textual analysis ).
Specific methods might include:
- Content analysis : Categorising and discussing the meaning of words, phrases and sentences
- Thematic analysis : Coding and closely examining the data to identify broad themes and patterns
- Discourse analysis : Studying communication and meaning in relation to their social context
Mixed methods combine the above two research methods, integrating both qualitative and quantitative approaches into one coherent analytical process.
Above all, your methodology section should clearly make the case for why you chose the methods you did. This is especially true if you did not take the most standard approach to your topic. In this case, discuss why other methods were not suitable for your objectives, and show how this approach contributes new knowledge or understanding.
In any case, it should be overwhelmingly clear to your reader that you set yourself up for success in terms of your methodology’s design. Show how your methods should lead to results that are valid and reliable, while leaving the analysis of the meaning, importance, and relevance of your results for your discussion section .
- Quantitative: Lab-based experiments cannot always accurately simulate real-life situations and behaviours, but they are effective for testing causal relationships between variables .
- Qualitative: Unstructured interviews usually produce results that cannot be generalised beyond the sample group , but they provide a more in-depth understanding of participants’ perceptions, motivations, and emotions.
- Mixed methods: Despite issues systematically comparing differing types of data, a solely quantitative study would not sufficiently incorporate the lived experience of each participant, while a solely qualitative study would be insufficiently generalisable.
Remember that your aim is not just to describe your methods, but to show how and why you applied them. Again, it’s critical to demonstrate that your research was rigorously conducted and can be replicated.
1. Focus on your objectives and research questions
The methodology section should clearly show why your methods suit your objectives and convince the reader that you chose the best possible approach to answering your problem statement and research questions .
2. Cite relevant sources
Your methodology can be strengthened by referencing existing research in your field. This can help you to:
- Show that you followed established practice for your type of research
- Discuss how you decided on your approach by evaluating existing research
- Present a novel methodological approach to address a gap in the literature
3. Write for your audience
Consider how much information you need to give, and avoid getting too lengthy. If you are using methods that are standard for your discipline, you probably don’t need to give a lot of background or justification.
Regardless, your methodology should be a clear, well-structured text that makes an argument for your approach, not just a list of technical details and procedures.
Methodology refers to the overarching strategy and rationale of your research. Developing your methodology involves studying the research methods used in your field and the theories or principles that underpin them, in order to choose the approach that best matches your objectives.
Methods are the specific tools and procedures you use to collect and analyse data (e.g. interviews, experiments , surveys , statistical tests ).
In a dissertation or scientific paper, the methodology chapter or methods section comes after the introduction and before the results , discussion and conclusion .
Depending on the length and type of document, you might also include a literature review or theoretical framework before the methodology.
Quantitative research deals with numbers and statistics, while qualitative research deals with words and meanings.
Quantitative methods allow you to test a hypothesis by systematically collecting and analysing data, while qualitative methods allow you to explore ideas and experiences in depth.
A sample is a subset of individuals from a larger population. Sampling means selecting the group that you will actually collect data from in your research.
For example, if you are researching the opinions of students in your university, you could survey a sample of 100 students.
Statistical sampling allows you to test a hypothesis about the characteristics of a population. There are various sampling methods you can use to ensure that your sample is representative of the population as a whole.
Cite this Scribbr article
If you want to cite this source, you can copy and paste the citation or click the ‘Cite this Scribbr article’ button to automatically add the citation to our free Reference Generator.
McCombes, S. (2022, October 10). What Is a Research Methodology? | Steps & Tips. Scribbr. Retrieved 22 April 2024, from https://www.scribbr.co.uk/thesis-dissertation/methodology/
Is this article helpful?
Shona McCombes
Other students also liked, how to write a dissertation proposal | a step-by-step guide, what is a literature review | guide, template, & examples, what is a theoretical framework | a step-by-step guide.

What Is Research Methodology? A Plain-Language Explanation & Definition (With Examples)
By Derek Jansen (MBA) and Kerryn Warren (PhD) | June 2020 (Last updated April 2023)
If you’re new to formal academic research, it’s quite likely that you’re feeling a little overwhelmed by all the technical lingo that gets thrown around. And who could blame you – “research methodology”, “research methods”, “sampling strategies”… it all seems never-ending!
In this post, we’ll demystify the landscape with plain-language explanations and loads of examples (including easy-to-follow videos), so that you can approach your dissertation, thesis or research project with confidence. Let’s get started.
Research Methodology 101
- What exactly research methodology means
- What qualitative , quantitative and mixed methods are
- What sampling strategy is
- What data collection methods are
- What data analysis methods are
- How to choose your research methodology
- Example of a research methodology
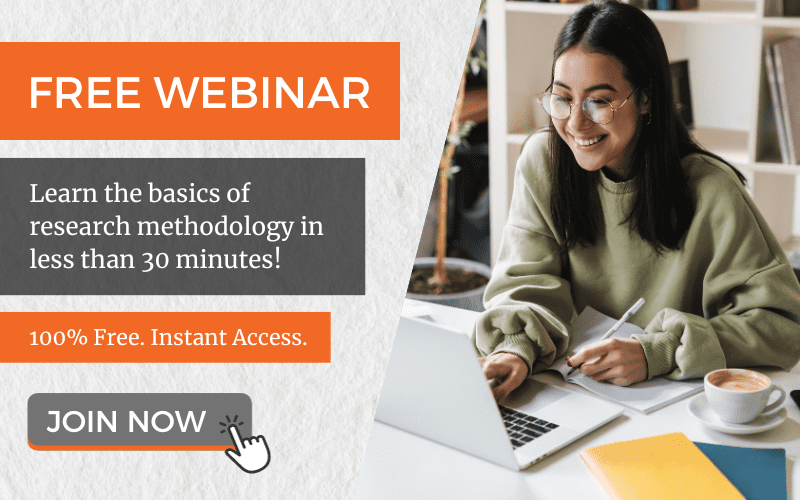
What is research methodology?
Research methodology simply refers to the practical “how” of a research study. More specifically, it’s about how a researcher systematically designs a study to ensure valid and reliable results that address the research aims, objectives and research questions . Specifically, how the researcher went about deciding:
- What type of data to collect (e.g., qualitative or quantitative data )
- Who to collect it from (i.e., the sampling strategy )
- How to collect it (i.e., the data collection method )
- How to analyse it (i.e., the data analysis methods )
Within any formal piece of academic research (be it a dissertation, thesis or journal article), you’ll find a research methodology chapter or section which covers the aspects mentioned above. Importantly, a good methodology chapter explains not just what methodological choices were made, but also explains why they were made. In other words, the methodology chapter should justify the design choices, by showing that the chosen methods and techniques are the best fit for the research aims, objectives and research questions.
So, it’s the same as research design?
Not quite. As we mentioned, research methodology refers to the collection of practical decisions regarding what data you’ll collect, from who, how you’ll collect it and how you’ll analyse it. Research design, on the other hand, is more about the overall strategy you’ll adopt in your study. For example, whether you’ll use an experimental design in which you manipulate one variable while controlling others. You can learn more about research design and the various design types here .
Need a helping hand?
What are qualitative, quantitative and mixed-methods?
Qualitative, quantitative and mixed-methods are different types of methodological approaches, distinguished by their focus on words , numbers or both . This is a bit of an oversimplification, but its a good starting point for understanding.
Let’s take a closer look.
Qualitative research refers to research which focuses on collecting and analysing words (written or spoken) and textual or visual data, whereas quantitative research focuses on measurement and testing using numerical data . Qualitative analysis can also focus on other “softer” data points, such as body language or visual elements.
It’s quite common for a qualitative methodology to be used when the research aims and research questions are exploratory in nature. For example, a qualitative methodology might be used to understand peoples’ perceptions about an event that took place, or a political candidate running for president.
Contrasted to this, a quantitative methodology is typically used when the research aims and research questions are confirmatory in nature. For example, a quantitative methodology might be used to measure the relationship between two variables (e.g. personality type and likelihood to commit a crime) or to test a set of hypotheses .
As you’ve probably guessed, the mixed-method methodology attempts to combine the best of both qualitative and quantitative methodologies to integrate perspectives and create a rich picture. If you’d like to learn more about these three methodological approaches, be sure to watch our explainer video below.
What is sampling strategy?
Simply put, sampling is about deciding who (or where) you’re going to collect your data from . Why does this matter? Well, generally it’s not possible to collect data from every single person in your group of interest (this is called the “population”), so you’ll need to engage a smaller portion of that group that’s accessible and manageable (this is called the “sample”).
How you go about selecting the sample (i.e., your sampling strategy) will have a major impact on your study. There are many different sampling methods you can choose from, but the two overarching categories are probability sampling and non-probability sampling .
Probability sampling involves using a completely random sample from the group of people you’re interested in. This is comparable to throwing the names all potential participants into a hat, shaking it up, and picking out the “winners”. By using a completely random sample, you’ll minimise the risk of selection bias and the results of your study will be more generalisable to the entire population.
Non-probability sampling , on the other hand, doesn’t use a random sample . For example, it might involve using a convenience sample, which means you’d only interview or survey people that you have access to (perhaps your friends, family or work colleagues), rather than a truly random sample. With non-probability sampling, the results are typically not generalisable .
To learn more about sampling methods, be sure to check out the video below.
What are data collection methods?
As the name suggests, data collection methods simply refers to the way in which you go about collecting the data for your study. Some of the most common data collection methods include:
- Interviews (which can be unstructured, semi-structured or structured)
- Focus groups and group interviews
- Surveys (online or physical surveys)
- Observations (watching and recording activities)
- Biophysical measurements (e.g., blood pressure, heart rate, etc.)
- Documents and records (e.g., financial reports, court records, etc.)
The choice of which data collection method to use depends on your overall research aims and research questions , as well as practicalities and resource constraints. For example, if your research is exploratory in nature, qualitative methods such as interviews and focus groups would likely be a good fit. Conversely, if your research aims to measure specific variables or test hypotheses, large-scale surveys that produce large volumes of numerical data would likely be a better fit.
What are data analysis methods?
Data analysis methods refer to the methods and techniques that you’ll use to make sense of your data. These can be grouped according to whether the research is qualitative (words-based) or quantitative (numbers-based).
Popular data analysis methods in qualitative research include:
- Qualitative content analysis
- Thematic analysis
- Discourse analysis
- Narrative analysis
- Interpretative phenomenological analysis (IPA)
- Visual analysis (of photographs, videos, art, etc.)
Qualitative data analysis all begins with data coding , after which an analysis method is applied. In some cases, more than one analysis method is used, depending on the research aims and research questions . In the video below, we explore some common qualitative analysis methods, along with practical examples.
Moving on to the quantitative side of things, popular data analysis methods in this type of research include:
- Descriptive statistics (e.g. means, medians, modes )
- Inferential statistics (e.g. correlation, regression, structural equation modelling)
Again, the choice of which data collection method to use depends on your overall research aims and objectives , as well as practicalities and resource constraints. In the video below, we explain some core concepts central to quantitative analysis.
How do I choose a research methodology?
As you’ve probably picked up by now, your research aims and objectives have a major influence on the research methodology . So, the starting point for developing your research methodology is to take a step back and look at the big picture of your research, before you make methodology decisions. The first question you need to ask yourself is whether your research is exploratory or confirmatory in nature.
If your research aims and objectives are primarily exploratory in nature, your research will likely be qualitative and therefore you might consider qualitative data collection methods (e.g. interviews) and analysis methods (e.g. qualitative content analysis).
Conversely, if your research aims and objective are looking to measure or test something (i.e. they’re confirmatory), then your research will quite likely be quantitative in nature, and you might consider quantitative data collection methods (e.g. surveys) and analyses (e.g. statistical analysis).
Designing your research and working out your methodology is a large topic, which we cover extensively on the blog . For now, however, the key takeaway is that you should always start with your research aims, objectives and research questions (the golden thread). Every methodological choice you make needs align with those three components.
Example of a research methodology chapter
In the video below, we provide a detailed walkthrough of a research methodology from an actual dissertation, as well as an overview of our free methodology template .
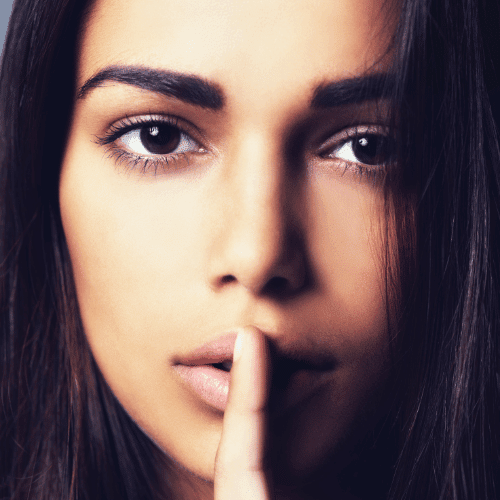
Psst... there’s more!
This post was based on one of our popular Research Bootcamps . If you're working on a research project, you'll definitely want to check this out ...
You Might Also Like:
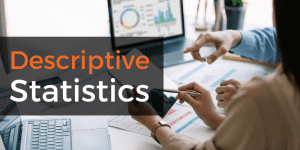
199 Comments
Thank you for this simple yet comprehensive and easy to digest presentation. God Bless!
You’re most welcome, Leo. Best of luck with your research!
I found it very useful. many thanks
This is really directional. A make-easy research knowledge.
Thank you for this, I think will help my research proposal
Thanks for good interpretation,well understood.
Good morning sorry I want to the search topic
Thank u more
Thank you, your explanation is simple and very helpful.
Very educative a.nd exciting platform. A bigger thank you and I’ll like to always be with you
That’s the best analysis
So simple yet so insightful. Thank you.
This really easy to read as it is self-explanatory. Very much appreciated…
Thanks for this. It’s so helpful and explicit. For those elements highlighted in orange, they were good sources of referrals for concepts I didn’t understand. A million thanks for this.
Good morning, I have been reading your research lessons through out a period of times. They are important, impressive and clear. Want to subscribe and be and be active with you.
Thankyou So much Sir Derek…
Good morning thanks so much for the on line lectures am a student of university of Makeni.select a research topic and deliberate on it so that we’ll continue to understand more.sorry that’s a suggestion.
Beautiful presentation. I love it.
please provide a research mehodology example for zoology
It’s very educative and well explained
Thanks for the concise and informative data.
This is really good for students to be safe and well understand that research is all about
Thank you so much Derek sir🖤🙏🤗
Very simple and reliable
This is really helpful. Thanks alot. God bless you.
very useful, Thank you very much..
thanks a lot its really useful
in a nutshell..thank you!
Thanks for updating my understanding on this aspect of my Thesis writing.
thank you so much my through this video am competently going to do a good job my thesis
Thanks a lot. Very simple to understand. I appreciate 🙏
Very simple but yet insightful Thank you
This has been an eye opening experience. Thank you grad coach team.
Very useful message for research scholars
Really very helpful thank you
yes you are right and i’m left
Research methodology with a simplest way i have never seen before this article.
wow thank u so much
Good morning thanks so much for the on line lectures am a student of university of Makeni.select a research topic and deliberate on is so that we will continue to understand more.sorry that’s a suggestion.
Very precise and informative.
Thanks for simplifying these terms for us, really appreciate it.
Thanks this has really helped me. It is very easy to understand.
I found the notes and the presentation assisting and opening my understanding on research methodology
Good presentation
Im so glad you clarified my misconceptions. Im now ready to fry my onions. Thank you so much. God bless
Thank you a lot.
thanks for the easy way of learning and desirable presentation.
Thanks a lot. I am inspired
Well written
I am writing a APA Format paper . I using questionnaire with 120 STDs teacher for my participant. Can you write me mthology for this research. Send it through email sent. Just need a sample as an example please. My topic is ” impacts of overcrowding on students learning
Thanks for your comment.
We can’t write your methodology for you. If you’re looking for samples, you should be able to find some sample methodologies on Google. Alternatively, you can download some previous dissertations from a dissertation directory and have a look at the methodology chapters therein.
All the best with your research.
Thank you so much for this!! God Bless
Thank you. Explicit explanation
Thank you, Derek and Kerryn, for making this simple to understand. I’m currently at the inception stage of my research.
Thnks a lot , this was very usefull on my assignment
excellent explanation
I’m currently working on my master’s thesis, thanks for this! I’m certain that I will use Qualitative methodology.
Thanks a lot for this concise piece, it was quite relieving and helpful. God bless you BIG…
I am currently doing my dissertation proposal and I am sure that I will do quantitative research. Thank you very much it was extremely helpful.
Very interesting and informative yet I would like to know about examples of Research Questions as well, if possible.
I’m about to submit a research presentation, I have come to understand from your simplification on understanding research methodology. My research will be mixed methodology, qualitative as well as quantitative. So aim and objective of mixed method would be both exploratory and confirmatory. Thanks you very much for your guidance.
OMG thanks for that, you’re a life saver. You covered all the points I needed. Thank you so much ❤️ ❤️ ❤️
Thank you immensely for this simple, easy to comprehend explanation of data collection methods. I have been stuck here for months 😩. Glad I found your piece. Super insightful.
I’m going to write synopsis which will be quantitative research method and I don’t know how to frame my topic, can I kindly get some ideas..
Thanks for this, I was really struggling.
This was really informative I was struggling but this helped me.
Thanks a lot for this information, simple and straightforward. I’m a last year student from the University of South Africa UNISA South Africa.
its very much informative and understandable. I have enlightened.
An interesting nice exploration of a topic.
Thank you. Accurate and simple🥰
This article was really helpful, it helped me understanding the basic concepts of the topic Research Methodology. The examples were very clear, and easy to understand. I would like to visit this website again. Thank you so much for such a great explanation of the subject.
Thanks dude
Thank you Doctor Derek for this wonderful piece, please help to provide your details for reference purpose. God bless.
Many compliments to you
Great work , thank you very much for the simple explanation
Thank you. I had to give a presentation on this topic. I have looked everywhere on the internet but this is the best and simple explanation.
thank you, its very informative.
Well explained. Now I know my research methodology will be qualitative and exploratory. Thank you so much, keep up the good work
Well explained, thank you very much.
This is good explanation, I have understood the different methods of research. Thanks a lot.
Great work…very well explanation
Thanks Derek. Kerryn was just fantastic!
Great to hear that, Hyacinth. Best of luck with your research!
Its a good templates very attractive and important to PhD students and lectuter
Thanks for the feedback, Matobela. Good luck with your research methodology.
Thank you. This is really helpful.
You’re very welcome, Elie. Good luck with your research methodology.
Well explained thanks
This is a very helpful site especially for young researchers at college. It provides sufficient information to guide students and equip them with the necessary foundation to ask any other questions aimed at deepening their understanding.
Thanks for the kind words, Edward. Good luck with your research!
Thank you. I have learned a lot.
Great to hear that, Ngwisa. Good luck with your research methodology!
Thank you for keeping your presentation simples and short and covering key information for research methodology. My key takeaway: Start with defining your research objective the other will depend on the aims of your research question.
My name is Zanele I would like to be assisted with my research , and the topic is shortage of nursing staff globally want are the causes , effects on health, patients and community and also globally
Thanks for making it simple and clear. It greatly helped in understanding research methodology. Regards.
This is well simplified and straight to the point
Thank you Dr
I was given an assignment to research 2 publications and describe their research methodology? I don’t know how to start this task can someone help me?
Sure. You’re welcome to book an initial consultation with one of our Research Coaches to discuss how we can assist – https://gradcoach.com/book/new/ .
Thanks a lot I am relieved of a heavy burden.keep up with the good work
I’m very much grateful Dr Derek. I’m planning to pursue one of the careers that really needs one to be very much eager to know. There’s a lot of research to do and everything, but since I’ve gotten this information I will use it to the best of my potential.
Thank you so much, words are not enough to explain how helpful this session has been for me!
Thanks this has thought me alot.
Very concise and helpful. Thanks a lot
Thank Derek. This is very helpful. Your step by step explanation has made it easier for me to understand different concepts. Now i can get on with my research.
I wish i had come across this sooner. So simple but yet insightful
really nice explanation thank you so much
I’m so grateful finding this site, it’s really helpful…….every term well explained and provide accurate understanding especially to student going into an in-depth research for the very first time, even though my lecturer already explained this topic to the class, I think I got the clear and efficient explanation here, much thanks to the author.
It is very helpful material
I would like to be assisted with my research topic : Literature Review and research methodologies. My topic is : what is the relationship between unemployment and economic growth?
Its really nice and good for us.
THANKS SO MUCH FOR EXPLANATION, ITS VERY CLEAR TO ME WHAT I WILL BE DOING FROM NOW .GREAT READS.
Short but sweet.Thank you
Informative article. Thanks for your detailed information.
I’m currently working on my Ph.D. thesis. Thanks a lot, Derek and Kerryn, Well-organized sequences, facilitate the readers’ following.
great article for someone who does not have any background can even understand
I am a bit confused about research design and methodology. Are they the same? If not, what are the differences and how are they related?
Thanks in advance.
concise and informative.
Thank you very much
How can we site this article is Harvard style?
Very well written piece that afforded better understanding of the concept. Thank you!
Am a new researcher trying to learn how best to write a research proposal. I find your article spot on and want to download the free template but finding difficulties. Can u kindly send it to my email, the free download entitled, “Free Download: Research Proposal Template (with Examples)”.
Thank too much
Thank you very much for your comprehensive explanation about research methodology so I like to thank you again for giving us such great things.
Good very well explained.Thanks for sharing it.
Thank u sir, it is really a good guideline.
so helpful thank you very much.
Thanks for the video it was very explanatory and detailed, easy to comprehend and follow up. please, keep it up the good work
It was very helpful, a well-written document with precise information.
how do i reference this?
MLA Jansen, Derek, and Kerryn Warren. “What (Exactly) Is Research Methodology?” Grad Coach, June 2021, gradcoach.com/what-is-research-methodology/.
APA Jansen, D., & Warren, K. (2021, June). What (Exactly) Is Research Methodology? Grad Coach. https://gradcoach.com/what-is-research-methodology/
Your explanation is easily understood. Thank you
Very help article. Now I can go my methodology chapter in my thesis with ease
I feel guided ,Thank you
This simplification is very helpful. It is simple but very educative, thanks ever so much
The write up is informative and educative. It is an academic intellectual representation that every good researcher can find useful. Thanks
Wow, this is wonderful long live.
Nice initiative
thank you the video was helpful to me.
Thank you very much for your simple and clear explanations I’m really satisfied by the way you did it By now, I think I can realize a very good article by following your fastidious indications May God bless you
Thanks very much, it was very concise and informational for a beginner like me to gain an insight into what i am about to undertake. I really appreciate.
very informative sir, it is amazing to understand the meaning of question hidden behind that, and simple language is used other than legislature to understand easily. stay happy.
This one is really amazing. All content in your youtube channel is a very helpful guide for doing research. Thanks, GradCoach.
research methodologies
Please send me more information concerning dissertation research.
Nice piece of knowledge shared….. #Thump_UP
This is amazing, it has said it all. Thanks to Gradcoach
This is wonderful,very elaborate and clear.I hope to reach out for your assistance in my research very soon.
This is the answer I am searching about…
realy thanks a lot
Thank you very much for this awesome, to the point and inclusive article.
Thank you very much I need validity and reliability explanation I have exams
Thank you for a well explained piece. This will help me going forward.
Very simple and well detailed Many thanks
This is so very simple yet so very effective and comprehensive. An Excellent piece of work.
I wish I saw this earlier on! Great insights for a beginner(researcher) like me. Thanks a mil!
Thank you very much, for such a simplified, clear and practical step by step both for academic students and general research work. Holistic, effective to use and easy to read step by step. One can easily apply the steps in practical terms and produce a quality document/up-to standard
Thanks for simplifying these terms for us, really appreciated.
Thanks for a great work. well understood .
This was very helpful. It was simple but profound and very easy to understand. Thank you so much!
Great and amazing research guidelines. Best site for learning research
hello sir/ma’am, i didn’t find yet that what type of research methodology i am using. because i am writing my report on CSR and collect all my data from websites and articles so which type of methodology i should write in dissertation report. please help me. i am from India.
how does this really work?
perfect content, thanks a lot
As a researcher, I commend you for the detailed and simplified information on the topic in question. I would like to remain in touch for the sharing of research ideas on other topics. Thank you
Impressive. Thank you, Grad Coach 😍
Thank you Grad Coach for this piece of information. I have at least learned about the different types of research methodologies.
Very useful content with easy way
Thank you very much for the presentation. I am an MPH student with the Adventist University of Africa. I have successfully completed my theory and starting on my research this July. My topic is “Factors associated with Dental Caries in (one District) in Botswana. I need help on how to go about this quantitative research
I am so grateful to run across something that was sooo helpful. I have been on my doctorate journey for quite some time. Your breakdown on methodology helped me to refresh my intent. Thank you.
thanks so much for this good lecture. student from university of science and technology, Wudil. Kano Nigeria.
It’s profound easy to understand I appreciate
Thanks a lot for sharing superb information in a detailed but concise manner. It was really helpful and helped a lot in getting into my own research methodology.
Comment * thanks very much
This was sooo helpful for me thank you so much i didn’t even know what i had to write thank you!
You’re most welcome 🙂
Simple and good. Very much helpful. Thank you so much.
This is very good work. I have benefited.
Thank you so much for sharing
This is powerful thank you so much guys
I am nkasa lizwi doing my research proposal on honors with the university of Walter Sisulu Komani I m on part 3 now can you assist me.my topic is: transitional challenges faced by educators in intermediate phase in the Alfred Nzo District.
Appreciate the presentation. Very useful step-by-step guidelines to follow.
I appreciate sir
wow! This is super insightful for me. Thank you!
Indeed this material is very helpful! Kudos writers/authors.
I want to say thank you very much, I got a lot of info and knowledge. Be blessed.
I want present a seminar paper on Optimisation of Deep learning-based models on vulnerability detection in digital transactions.
Need assistance
Dear Sir, I want to be assisted on my research on Sanitation and Water management in emergencies areas.
I am deeply grateful for the knowledge gained. I will be getting in touch shortly as I want to be assisted in my ongoing research.
The information shared is informative, crisp and clear. Kudos Team! And thanks a lot!
hello i want to study
Hello!! Grad coach teams. I am extremely happy in your tutorial or consultation. i am really benefited all material and briefing. Thank you very much for your generous helps. Please keep it up. If you add in your briefing, references for further reading, it will be very nice.
All I have to say is, thank u gyz.
Good, l thanks
thank you, it is very useful
Trackbacks/Pingbacks
- What Is A Literature Review (In A Dissertation Or Thesis) - Grad Coach - […] the literature review is to inform the choice of methodology for your own research. As we’ve discussed on the Grad Coach blog,…
- Free Download: Research Proposal Template (With Examples) - Grad Coach - […] Research design (methodology) […]
- Dissertation vs Thesis: What's the difference? - Grad Coach - […] and thesis writing on a daily basis – everything from how to find a good research topic to which…
Submit a Comment Cancel reply
Your email address will not be published. Required fields are marked *
Save my name, email, and website in this browser for the next time I comment.
- Print Friendly

Research Process :: Step by Step
- Introduction
- Select Topic
- Identify Keywords
- Background Information
- Develop Research Questions
- Refine Topic
- Search Strategy
- Popular Databases
- Evaluate Sources
- Types of Periodicals
- Reading Scholarly Articles
- Primary & Secondary Sources
- Organize / Take Notes
- Writing & Grammar Resources
- Annotated Bibliography
- Literature Review
- Citation Styles
- Paraphrasing
- Privacy / Confidentiality
- Research Process
- Selecting Your Topic
- Identifying Keywords
- Gathering Background Info
- Evaluating Sources

Organize the literature review into sections that present themes or identify trends, including relevant theory. You are not trying to list all the material published, but to synthesize and evaluate it according to the guiding concept of your thesis or research question.
What is a literature review?
A literature review is an account of what has been published on a topic by accredited scholars and researchers. Occasionally you will be asked to write one as a separate assignment, but more often it is part of the introduction to an essay, research report, or thesis. In writing the literature review, your purpose is to convey to your reader what knowledge and ideas have been established on a topic, and what their strengths and weaknesses are. As a piece of writing, the literature review must be defined by a guiding concept (e.g., your research objective, the problem or issue you are discussing, or your argumentative thesis). It is not just a descriptive list of the material available, or a set of summaries
A literature review must do these things:
- be organized around and related directly to the thesis or research question you are developing
- synthesize results into a summary of what is and is not known
- identify areas of controversy in the literature
- formulate questions that need further research
Ask yourself questions like these:
- What is the specific thesis, problem, or research question that my literature review helps to define?
- What type of literature review am I conducting? Am I looking at issues of theory? methodology? policy? quantitative research (e.g. on the effectiveness of a new procedure)? qualitative research (e.g., studies of loneliness among migrant workers)?
- What is the scope of my literature review? What types of publications am I using (e.g., journals, books, government documents, popular media)? What discipline am I working in (e.g., nursing psychology, sociology, medicine)?
- How good was my information seeking? Has my search been wide enough to ensure I've found all the relevant material? Has it been narrow enough to exclude irrelevant material? Is the number of sources I've used appropriate for the length of my paper?
- Have I critically analyzed the literature I use? Do I follow through a set of concepts and questions, comparing items to each other in the ways they deal with them? Instead of just listing and summarizing items, do I assess them, discussing strengths and weaknesses?
- Have I cited and discussed studies contrary to my perspective?
- Will the reader find my literature review relevant, appropriate, and useful?
Ask yourself questions like these about each book or article you include:
- Has the author formulated a problem/issue?
- Is it clearly defined? Is its significance (scope, severity, relevance) clearly established?
- Could the problem have been approached more effectively from another perspective?
- What is the author's research orientation (e.g., interpretive, critical science, combination)?
- What is the author's theoretical framework (e.g., psychological, developmental, feminist)?
- What is the relationship between the theoretical and research perspectives?
- Has the author evaluated the literature relevant to the problem/issue? Does the author include literature taking positions she or he does not agree with?
- In a research study, how good are the basic components of the study design (e.g., population, intervention, outcome)? How accurate and valid are the measurements? Is the analysis of the data accurate and relevant to the research question? Are the conclusions validly based upon the data and analysis?
- In material written for a popular readership, does the author use appeals to emotion, one-sided examples, or rhetorically-charged language and tone? Is there an objective basis to the reasoning, or is the author merely "proving" what he or she already believes?
- How does the author structure the argument? Can you "deconstruct" the flow of the argument to see whether or where it breaks down logically (e.g., in establishing cause-effect relationships)?
- In what ways does this book or article contribute to our understanding of the problem under study, and in what ways is it useful for practice? What are the strengths and limitations?
- How does this book or article relate to the specific thesis or question I am developing?
Text written by Dena Taylor, Health Sciences Writing Centre, University of Toronto
http://www.writing.utoronto.ca/advice/specific-types-of-writing/literature-review
- << Previous: Annotated Bibliography
- Next: Step 5: Cite Sources >>
- Last Updated: Apr 19, 2024 12:43 PM
- URL: https://libguides.uta.edu/researchprocess
University of Texas Arlington Libraries 702 Planetarium Place · Arlington, TX 76019 · 817-272-3000
- Internet Privacy
- Accessibility
- Problems with a guide? Contact Us.
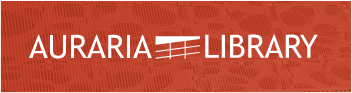
Research Methods: Literature Reviews
- Annotated Bibliographies
- Literature Reviews
- Scoping Reviews
- Systematic Reviews
- Scholarship of Teaching and Learning
- Persuasive Arguments
- Subject Specific Methodology
A literature review involves researching, reading, analyzing, evaluating, and summarizing scholarly literature (typically journals and articles) about a specific topic. The results of a literature review may be an entire report or article OR may be part of a article, thesis, dissertation, or grant proposal. A literature review helps the author learn about the history and nature of their topic, and identify research gaps and problems.
Steps & Elements
Problem formulation
- Determine your topic and its components by asking a question
- Research: locate literature related to your topic to identify the gap(s) that can be addressed
- Read: read the articles or other sources of information
- Analyze: assess the findings for relevancy
- Evaluating: determine how the article are relevant to your research and what are the key findings
- Synthesis: write about the key findings and how it is relevant to your research
Elements of a Literature Review
- Summarize subject, issue or theory under consideration, along with objectives of the review
- Divide works under review into categories (e.g. those in support of a particular position, those against, those offering alternative theories entirely)
- Explain how each work is similar to and how it varies from the others
- Conclude which pieces are best considered in their argument, are most convincing of their opinions, and make the greatest contribution to the understanding and development of an area of research
Writing a Literature Review Resources
- How to Write a Literature Review From the Wesleyan University Library
- Write a Literature Review From the University of California Santa Cruz Library. A Brief overview of a literature review, includes a list of stages for writing a lit review.
- Literature Reviews From the University of North Carolina Writing Center. Detailed information about writing a literature review.
- Undertaking a literature review: a step-by-step approach Cronin, P., Ryan, F., & Coughan, M. (2008). Undertaking a literature review: A step-by-step approach. British Journal of Nursing, 17(1), p.38-43
Literature Review Tutorial
- << Previous: Annotated Bibliographies
- Next: Scoping Reviews >>
- Last Updated: Feb 29, 2024 12:00 PM
- URL: https://guides.auraria.edu/researchmethods
1100 Lawrence Street Denver, CO 80204 303-315-7700 Ask Us Directions
Get science-backed answers as you write with Paperpal's Research feature
What is Research Methodology? Definition, Types, and Examples
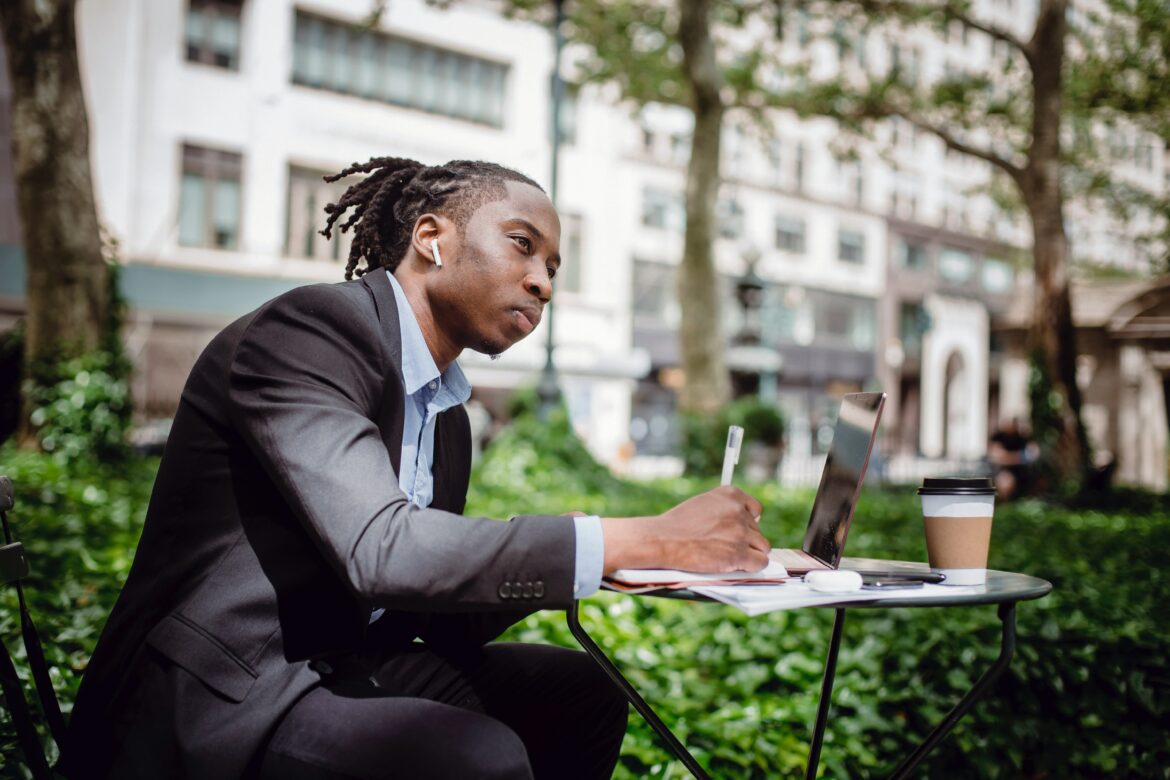
Research methodology 1,2 is a structured and scientific approach used to collect, analyze, and interpret quantitative or qualitative data to answer research questions or test hypotheses. A research methodology is like a plan for carrying out research and helps keep researchers on track by limiting the scope of the research. Several aspects must be considered before selecting an appropriate research methodology, such as research limitations and ethical concerns that may affect your research.
The research methodology section in a scientific paper describes the different methodological choices made, such as the data collection and analysis methods, and why these choices were selected. The reasons should explain why the methods chosen are the most appropriate to answer the research question. A good research methodology also helps ensure the reliability and validity of the research findings. There are three types of research methodology—quantitative, qualitative, and mixed-method, which can be chosen based on the research objectives.
What is research methodology ?
A research methodology describes the techniques and procedures used to identify and analyze information regarding a specific research topic. It is a process by which researchers design their study so that they can achieve their objectives using the selected research instruments. It includes all the important aspects of research, including research design, data collection methods, data analysis methods, and the overall framework within which the research is conducted. While these points can help you understand what is research methodology, you also need to know why it is important to pick the right methodology.
Why is research methodology important?
Having a good research methodology in place has the following advantages: 3
- Helps other researchers who may want to replicate your research; the explanations will be of benefit to them.
- You can easily answer any questions about your research if they arise at a later stage.
- A research methodology provides a framework and guidelines for researchers to clearly define research questions, hypotheses, and objectives.
- It helps researchers identify the most appropriate research design, sampling technique, and data collection and analysis methods.
- A sound research methodology helps researchers ensure that their findings are valid and reliable and free from biases and errors.
- It also helps ensure that ethical guidelines are followed while conducting research.
- A good research methodology helps researchers in planning their research efficiently, by ensuring optimum usage of their time and resources.
Writing the methods section of a research paper? Let Paperpal help you achieve perfection
Types of research methodology.
There are three types of research methodology based on the type of research and the data required. 1
- Quantitative research methodology focuses on measuring and testing numerical data. This approach is good for reaching a large number of people in a short amount of time. This type of research helps in testing the causal relationships between variables, making predictions, and generalizing results to wider populations.
- Qualitative research methodology examines the opinions, behaviors, and experiences of people. It collects and analyzes words and textual data. This research methodology requires fewer participants but is still more time consuming because the time spent per participant is quite large. This method is used in exploratory research where the research problem being investigated is not clearly defined.
- Mixed-method research methodology uses the characteristics of both quantitative and qualitative research methodologies in the same study. This method allows researchers to validate their findings, verify if the results observed using both methods are complementary, and explain any unexpected results obtained from one method by using the other method.
What are the types of sampling designs in research methodology?
Sampling 4 is an important part of a research methodology and involves selecting a representative sample of the population to conduct the study, making statistical inferences about them, and estimating the characteristics of the whole population based on these inferences. There are two types of sampling designs in research methodology—probability and nonprobability.
- Probability sampling
In this type of sampling design, a sample is chosen from a larger population using some form of random selection, that is, every member of the population has an equal chance of being selected. The different types of probability sampling are:
- Systematic —sample members are chosen at regular intervals. It requires selecting a starting point for the sample and sample size determination that can be repeated at regular intervals. This type of sampling method has a predefined range; hence, it is the least time consuming.
- Stratified —researchers divide the population into smaller groups that don’t overlap but represent the entire population. While sampling, these groups can be organized, and then a sample can be drawn from each group separately.
- Cluster —the population is divided into clusters based on demographic parameters like age, sex, location, etc.
- Convenience —selects participants who are most easily accessible to researchers due to geographical proximity, availability at a particular time, etc.
- Purposive —participants are selected at the researcher’s discretion. Researchers consider the purpose of the study and the understanding of the target audience.
- Snowball —already selected participants use their social networks to refer the researcher to other potential participants.
- Quota —while designing the study, the researchers decide how many people with which characteristics to include as participants. The characteristics help in choosing people most likely to provide insights into the subject.
What are data collection methods?
During research, data are collected using various methods depending on the research methodology being followed and the research methods being undertaken. Both qualitative and quantitative research have different data collection methods, as listed below.
Qualitative research 5
- One-on-one interviews: Helps the interviewers understand a respondent’s subjective opinion and experience pertaining to a specific topic or event
- Document study/literature review/record keeping: Researchers’ review of already existing written materials such as archives, annual reports, research articles, guidelines, policy documents, etc.
- Focus groups: Constructive discussions that usually include a small sample of about 6-10 people and a moderator, to understand the participants’ opinion on a given topic.
- Qualitative observation : Researchers collect data using their five senses (sight, smell, touch, taste, and hearing).
Quantitative research 6
- Sampling: The most common type is probability sampling.
- Interviews: Commonly telephonic or done in-person.
- Observations: Structured observations are most commonly used in quantitative research. In this method, researchers make observations about specific behaviors of individuals in a structured setting.
- Document review: Reviewing existing research or documents to collect evidence for supporting the research.
- Surveys and questionnaires. Surveys can be administered both online and offline depending on the requirement and sample size.
Let Paperpal help you write the perfect research methods section. Start now!
What are data analysis methods.
The data collected using the various methods for qualitative and quantitative research need to be analyzed to generate meaningful conclusions. These data analysis methods 7 also differ between quantitative and qualitative research.
Quantitative research involves a deductive method for data analysis where hypotheses are developed at the beginning of the research and precise measurement is required. The methods include statistical analysis applications to analyze numerical data and are grouped into two categories—descriptive and inferential.
Descriptive analysis is used to describe the basic features of different types of data to present it in a way that ensures the patterns become meaningful. The different types of descriptive analysis methods are:
- Measures of frequency (count, percent, frequency)
- Measures of central tendency (mean, median, mode)
- Measures of dispersion or variation (range, variance, standard deviation)
- Measure of position (percentile ranks, quartile ranks)
Inferential analysis is used to make predictions about a larger population based on the analysis of the data collected from a smaller population. This analysis is used to study the relationships between different variables. Some commonly used inferential data analysis methods are:
- Correlation: To understand the relationship between two or more variables.
- Cross-tabulation: Analyze the relationship between multiple variables.
- Regression analysis: Study the impact of independent variables on the dependent variable.
- Frequency tables: To understand the frequency of data.
- Analysis of variance: To test the degree to which two or more variables differ in an experiment.
Qualitative research involves an inductive method for data analysis where hypotheses are developed after data collection. The methods include:
- Content analysis: For analyzing documented information from text and images by determining the presence of certain words or concepts in texts.
- Narrative analysis: For analyzing content obtained from sources such as interviews, field observations, and surveys. The stories and opinions shared by people are used to answer research questions.
- Discourse analysis: For analyzing interactions with people considering the social context, that is, the lifestyle and environment, under which the interaction occurs.
- Grounded theory: Involves hypothesis creation by data collection and analysis to explain why a phenomenon occurred.
- Thematic analysis: To identify important themes or patterns in data and use these to address an issue.
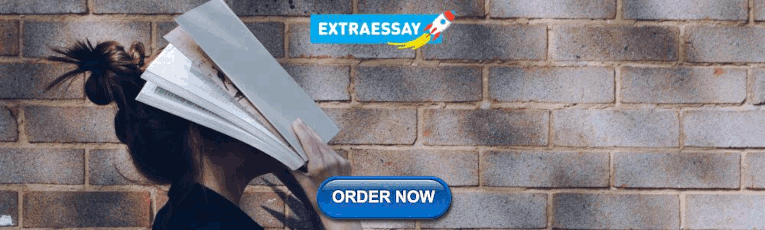
How to choose a research methodology?
Here are some important factors to consider when choosing a research methodology: 8
- Research objectives, aims, and questions —these would help structure the research design.
- Review existing literature to identify any gaps in knowledge.
- Check the statistical requirements —if data-driven or statistical results are needed then quantitative research is the best. If the research questions can be answered based on people’s opinions and perceptions, then qualitative research is most suitable.
- Sample size —sample size can often determine the feasibility of a research methodology. For a large sample, less effort- and time-intensive methods are appropriate.
- Constraints —constraints of time, geography, and resources can help define the appropriate methodology.
Got writer’s block? Kickstart your research paper writing with Paperpal now!
How to write a research methodology .
A research methodology should include the following components: 3,9
- Research design —should be selected based on the research question and the data required. Common research designs include experimental, quasi-experimental, correlational, descriptive, and exploratory.
- Research method —this can be quantitative, qualitative, or mixed-method.
- Reason for selecting a specific methodology —explain why this methodology is the most suitable to answer your research problem.
- Research instruments —explain the research instruments you plan to use, mainly referring to the data collection methods such as interviews, surveys, etc. Here as well, a reason should be mentioned for selecting the particular instrument.
- Sampling —this involves selecting a representative subset of the population being studied.
- Data collection —involves gathering data using several data collection methods, such as surveys, interviews, etc.
- Data analysis —describe the data analysis methods you will use once you’ve collected the data.
- Research limitations —mention any limitations you foresee while conducting your research.
- Validity and reliability —validity helps identify the accuracy and truthfulness of the findings; reliability refers to the consistency and stability of the results over time and across different conditions.
- Ethical considerations —research should be conducted ethically. The considerations include obtaining consent from participants, maintaining confidentiality, and addressing conflicts of interest.
Streamline Your Research Paper Writing Process with Paperpal
The methods section is a critical part of the research papers, allowing researchers to use this to understand your findings and replicate your work when pursuing their own research. However, it is usually also the most difficult section to write. This is where Paperpal can help you overcome the writer’s block and create the first draft in minutes with Paperpal Copilot, its secure generative AI feature suite.
With Paperpal you can get research advice, write and refine your work, rephrase and verify the writing, and ensure submission readiness, all in one place. Here’s how you can use Paperpal to develop the first draft of your methods section.
- Generate an outline: Input some details about your research to instantly generate an outline for your methods section
- Develop the section: Use the outline and suggested sentence templates to expand your ideas and develop the first draft.
- P araph ras e and trim : Get clear, concise academic text with paraphrasing that conveys your work effectively and word reduction to fix redundancies.
- Choose the right words: Enhance text by choosing contextual synonyms based on how the words have been used in previously published work.
- Check and verify text : Make sure the generated text showcases your methods correctly, has all the right citations, and is original and authentic. .
You can repeat this process to develop each section of your research manuscript, including the title, abstract and keywords. Ready to write your research papers faster, better, and without the stress? Sign up for Paperpal and start writing today!
Frequently Asked Questions
Q1. What are the key components of research methodology?
A1. A good research methodology has the following key components:
- Research design
- Data collection procedures
- Data analysis methods
- Ethical considerations
Q2. Why is ethical consideration important in research methodology?
A2. Ethical consideration is important in research methodology to ensure the readers of the reliability and validity of the study. Researchers must clearly mention the ethical norms and standards followed during the conduct of the research and also mention if the research has been cleared by any institutional board. The following 10 points are the important principles related to ethical considerations: 10
- Participants should not be subjected to harm.
- Respect for the dignity of participants should be prioritized.
- Full consent should be obtained from participants before the study.
- Participants’ privacy should be ensured.
- Confidentiality of the research data should be ensured.
- Anonymity of individuals and organizations participating in the research should be maintained.
- The aims and objectives of the research should not be exaggerated.
- Affiliations, sources of funding, and any possible conflicts of interest should be declared.
- Communication in relation to the research should be honest and transparent.
- Misleading information and biased representation of primary data findings should be avoided.
Q3. What is the difference between methodology and method?
A3. Research methodology is different from a research method, although both terms are often confused. Research methods are the tools used to gather data, while the research methodology provides a framework for how research is planned, conducted, and analyzed. The latter guides researchers in making decisions about the most appropriate methods for their research. Research methods refer to the specific techniques, procedures, and tools used by researchers to collect, analyze, and interpret data, for instance surveys, questionnaires, interviews, etc.
Research methodology is, thus, an integral part of a research study. It helps ensure that you stay on track to meet your research objectives and answer your research questions using the most appropriate data collection and analysis tools based on your research design.
Accelerate your research paper writing with Paperpal. Try for free now!
- Research methodologies. Pfeiffer Library website. Accessed August 15, 2023. https://library.tiffin.edu/researchmethodologies/whatareresearchmethodologies
- Types of research methodology. Eduvoice website. Accessed August 16, 2023. https://eduvoice.in/types-research-methodology/
- The basics of research methodology: A key to quality research. Voxco. Accessed August 16, 2023. https://www.voxco.com/blog/what-is-research-methodology/
- Sampling methods: Types with examples. QuestionPro website. Accessed August 16, 2023. https://www.questionpro.com/blog/types-of-sampling-for-social-research/
- What is qualitative research? Methods, types, approaches, examples. Researcher.Life blog. Accessed August 15, 2023. https://researcher.life/blog/article/what-is-qualitative-research-methods-types-examples/
- What is quantitative research? Definition, methods, types, and examples. Researcher.Life blog. Accessed August 15, 2023. https://researcher.life/blog/article/what-is-quantitative-research-types-and-examples/
- Data analysis in research: Types & methods. QuestionPro website. Accessed August 16, 2023. https://www.questionpro.com/blog/data-analysis-in-research/#Data_analysis_in_qualitative_research
- Factors to consider while choosing the right research methodology. PhD Monster website. Accessed August 17, 2023. https://www.phdmonster.com/factors-to-consider-while-choosing-the-right-research-methodology/
- What is research methodology? Research and writing guides. Accessed August 14, 2023. https://paperpile.com/g/what-is-research-methodology/
- Ethical considerations. Business research methodology website. Accessed August 17, 2023. https://research-methodology.net/research-methodology/ethical-considerations/
Paperpal is a comprehensive AI writing toolkit that helps students and researchers achieve 2x the writing in half the time. It leverages 21+ years of STM experience and insights from millions of research articles to provide in-depth academic writing, language editing, and submission readiness support to help you write better, faster.
Get accurate academic translations, rewriting support, grammar checks, vocabulary suggestions, and generative AI assistance that delivers human precision at machine speed. Try for free or upgrade to Paperpal Prime starting at US$19 a month to access premium features, including consistency, plagiarism, and 30+ submission readiness checks to help you succeed.
Experience the future of academic writing – Sign up to Paperpal and start writing for free!
Related Reads:
- Dangling Modifiers and How to Avoid Them in Your Writing
- Webinar: How to Use Generative AI Tools Ethically in Your Academic Writing
- Research Outlines: How to Write An Introduction Section in Minutes with Paperpal Copilot
- How to Paraphrase Research Papers Effectively
Language and Grammar Rules for Academic Writing
Climatic vs. climactic: difference and examples, you may also like, what is academic writing: tips for students, what is hedging in academic writing , how to use ai to enhance your college..., how to use paperpal to generate emails &..., ai in education: it’s time to change the..., is it ethical to use ai-generated abstracts without..., do plagiarism checkers detect ai content, word choice problems: how to use the right..., how to avoid plagiarism when using generative ai..., what are journal guidelines on using generative ai....

An official website of the United States government
The .gov means it's official. Federal government websites often end in .gov or .mil. Before sharing sensitive information, make sure you're on a federal government site.
The site is secure. The https:// ensures that you are connecting to the official website and that any information you provide is encrypted and transmitted securely.
- Publications
- Account settings
- Browse Titles
NCBI Bookshelf. A service of the National Library of Medicine, National Institutes of Health.
Lau F, Kuziemsky C, editors. Handbook of eHealth Evaluation: An Evidence-based Approach [Internet]. Victoria (BC): University of Victoria; 2017 Feb 27.

Handbook of eHealth Evaluation: An Evidence-based Approach [Internet].
Chapter 9 methods for literature reviews.
Guy Paré and Spyros Kitsiou .
9.1. Introduction
Literature reviews play a critical role in scholarship because science remains, first and foremost, a cumulative endeavour ( vom Brocke et al., 2009 ). As in any academic discipline, rigorous knowledge syntheses are becoming indispensable in keeping up with an exponentially growing eHealth literature, assisting practitioners, academics, and graduate students in finding, evaluating, and synthesizing the contents of many empirical and conceptual papers. Among other methods, literature reviews are essential for: (a) identifying what has been written on a subject or topic; (b) determining the extent to which a specific research area reveals any interpretable trends or patterns; (c) aggregating empirical findings related to a narrow research question to support evidence-based practice; (d) generating new frameworks and theories; and (e) identifying topics or questions requiring more investigation ( Paré, Trudel, Jaana, & Kitsiou, 2015 ).
Literature reviews can take two major forms. The most prevalent one is the “literature review” or “background” section within a journal paper or a chapter in a graduate thesis. This section synthesizes the extant literature and usually identifies the gaps in knowledge that the empirical study addresses ( Sylvester, Tate, & Johnstone, 2013 ). It may also provide a theoretical foundation for the proposed study, substantiate the presence of the research problem, justify the research as one that contributes something new to the cumulated knowledge, or validate the methods and approaches for the proposed study ( Hart, 1998 ; Levy & Ellis, 2006 ).
The second form of literature review, which is the focus of this chapter, constitutes an original and valuable work of research in and of itself ( Paré et al., 2015 ). Rather than providing a base for a researcher’s own work, it creates a solid starting point for all members of the community interested in a particular area or topic ( Mulrow, 1987 ). The so-called “review article” is a journal-length paper which has an overarching purpose to synthesize the literature in a field, without collecting or analyzing any primary data ( Green, Johnson, & Adams, 2006 ).
When appropriately conducted, review articles represent powerful information sources for practitioners looking for state-of-the art evidence to guide their decision-making and work practices ( Paré et al., 2015 ). Further, high-quality reviews become frequently cited pieces of work which researchers seek out as a first clear outline of the literature when undertaking empirical studies ( Cooper, 1988 ; Rowe, 2014 ). Scholars who track and gauge the impact of articles have found that review papers are cited and downloaded more often than any other type of published article ( Cronin, Ryan, & Coughlan, 2008 ; Montori, Wilczynski, Morgan, Haynes, & Hedges, 2003 ; Patsopoulos, Analatos, & Ioannidis, 2005 ). The reason for their popularity may be the fact that reading the review enables one to have an overview, if not a detailed knowledge of the area in question, as well as references to the most useful primary sources ( Cronin et al., 2008 ). Although they are not easy to conduct, the commitment to complete a review article provides a tremendous service to one’s academic community ( Paré et al., 2015 ; Petticrew & Roberts, 2006 ). Most, if not all, peer-reviewed journals in the fields of medical informatics publish review articles of some type.
The main objectives of this chapter are fourfold: (a) to provide an overview of the major steps and activities involved in conducting a stand-alone literature review; (b) to describe and contrast the different types of review articles that can contribute to the eHealth knowledge base; (c) to illustrate each review type with one or two examples from the eHealth literature; and (d) to provide a series of recommendations for prospective authors of review articles in this domain.
9.2. Overview of the Literature Review Process and Steps
As explained in Templier and Paré (2015) , there are six generic steps involved in conducting a review article:
- formulating the research question(s) and objective(s),
- searching the extant literature,
- screening for inclusion,
- assessing the quality of primary studies,
- extracting data, and
- analyzing data.
Although these steps are presented here in sequential order, one must keep in mind that the review process can be iterative and that many activities can be initiated during the planning stage and later refined during subsequent phases ( Finfgeld-Connett & Johnson, 2013 ; Kitchenham & Charters, 2007 ).
Formulating the research question(s) and objective(s): As a first step, members of the review team must appropriately justify the need for the review itself ( Petticrew & Roberts, 2006 ), identify the review’s main objective(s) ( Okoli & Schabram, 2010 ), and define the concepts or variables at the heart of their synthesis ( Cooper & Hedges, 2009 ; Webster & Watson, 2002 ). Importantly, they also need to articulate the research question(s) they propose to investigate ( Kitchenham & Charters, 2007 ). In this regard, we concur with Jesson, Matheson, and Lacey (2011) that clearly articulated research questions are key ingredients that guide the entire review methodology; they underscore the type of information that is needed, inform the search for and selection of relevant literature, and guide or orient the subsequent analysis. Searching the extant literature: The next step consists of searching the literature and making decisions about the suitability of material to be considered in the review ( Cooper, 1988 ). There exist three main coverage strategies. First, exhaustive coverage means an effort is made to be as comprehensive as possible in order to ensure that all relevant studies, published and unpublished, are included in the review and, thus, conclusions are based on this all-inclusive knowledge base. The second type of coverage consists of presenting materials that are representative of most other works in a given field or area. Often authors who adopt this strategy will search for relevant articles in a small number of top-tier journals in a field ( Paré et al., 2015 ). In the third strategy, the review team concentrates on prior works that have been central or pivotal to a particular topic. This may include empirical studies or conceptual papers that initiated a line of investigation, changed how problems or questions were framed, introduced new methods or concepts, or engendered important debate ( Cooper, 1988 ). Screening for inclusion: The following step consists of evaluating the applicability of the material identified in the preceding step ( Levy & Ellis, 2006 ; vom Brocke et al., 2009 ). Once a group of potential studies has been identified, members of the review team must screen them to determine their relevance ( Petticrew & Roberts, 2006 ). A set of predetermined rules provides a basis for including or excluding certain studies. This exercise requires a significant investment on the part of researchers, who must ensure enhanced objectivity and avoid biases or mistakes. As discussed later in this chapter, for certain types of reviews there must be at least two independent reviewers involved in the screening process and a procedure to resolve disagreements must also be in place ( Liberati et al., 2009 ; Shea et al., 2009 ). Assessing the quality of primary studies: In addition to screening material for inclusion, members of the review team may need to assess the scientific quality of the selected studies, that is, appraise the rigour of the research design and methods. Such formal assessment, which is usually conducted independently by at least two coders, helps members of the review team refine which studies to include in the final sample, determine whether or not the differences in quality may affect their conclusions, or guide how they analyze the data and interpret the findings ( Petticrew & Roberts, 2006 ). Ascribing quality scores to each primary study or considering through domain-based evaluations which study components have or have not been designed and executed appropriately makes it possible to reflect on the extent to which the selected study addresses possible biases and maximizes validity ( Shea et al., 2009 ). Extracting data: The following step involves gathering or extracting applicable information from each primary study included in the sample and deciding what is relevant to the problem of interest ( Cooper & Hedges, 2009 ). Indeed, the type of data that should be recorded mainly depends on the initial research questions ( Okoli & Schabram, 2010 ). However, important information may also be gathered about how, when, where and by whom the primary study was conducted, the research design and methods, or qualitative/quantitative results ( Cooper & Hedges, 2009 ). Analyzing and synthesizing data : As a final step, members of the review team must collate, summarize, aggregate, organize, and compare the evidence extracted from the included studies. The extracted data must be presented in a meaningful way that suggests a new contribution to the extant literature ( Jesson et al., 2011 ). Webster and Watson (2002) warn researchers that literature reviews should be much more than lists of papers and should provide a coherent lens to make sense of extant knowledge on a given topic. There exist several methods and techniques for synthesizing quantitative (e.g., frequency analysis, meta-analysis) and qualitative (e.g., grounded theory, narrative analysis, meta-ethnography) evidence ( Dixon-Woods, Agarwal, Jones, Young, & Sutton, 2005 ; Thomas & Harden, 2008 ).
9.3. Types of Review Articles and Brief Illustrations
EHealth researchers have at their disposal a number of approaches and methods for making sense out of existing literature, all with the purpose of casting current research findings into historical contexts or explaining contradictions that might exist among a set of primary research studies conducted on a particular topic. Our classification scheme is largely inspired from Paré and colleagues’ (2015) typology. Below we present and illustrate those review types that we feel are central to the growth and development of the eHealth domain.
9.3.1. Narrative Reviews
The narrative review is the “traditional” way of reviewing the extant literature and is skewed towards a qualitative interpretation of prior knowledge ( Sylvester et al., 2013 ). Put simply, a narrative review attempts to summarize or synthesize what has been written on a particular topic but does not seek generalization or cumulative knowledge from what is reviewed ( Davies, 2000 ; Green et al., 2006 ). Instead, the review team often undertakes the task of accumulating and synthesizing the literature to demonstrate the value of a particular point of view ( Baumeister & Leary, 1997 ). As such, reviewers may selectively ignore or limit the attention paid to certain studies in order to make a point. In this rather unsystematic approach, the selection of information from primary articles is subjective, lacks explicit criteria for inclusion and can lead to biased interpretations or inferences ( Green et al., 2006 ). There are several narrative reviews in the particular eHealth domain, as in all fields, which follow such an unstructured approach ( Silva et al., 2015 ; Paul et al., 2015 ).
Despite these criticisms, this type of review can be very useful in gathering together a volume of literature in a specific subject area and synthesizing it. As mentioned above, its primary purpose is to provide the reader with a comprehensive background for understanding current knowledge and highlighting the significance of new research ( Cronin et al., 2008 ). Faculty like to use narrative reviews in the classroom because they are often more up to date than textbooks, provide a single source for students to reference, and expose students to peer-reviewed literature ( Green et al., 2006 ). For researchers, narrative reviews can inspire research ideas by identifying gaps or inconsistencies in a body of knowledge, thus helping researchers to determine research questions or formulate hypotheses. Importantly, narrative reviews can also be used as educational articles to bring practitioners up to date with certain topics of issues ( Green et al., 2006 ).
Recently, there have been several efforts to introduce more rigour in narrative reviews that will elucidate common pitfalls and bring changes into their publication standards. Information systems researchers, among others, have contributed to advancing knowledge on how to structure a “traditional” review. For instance, Levy and Ellis (2006) proposed a generic framework for conducting such reviews. Their model follows the systematic data processing approach comprised of three steps, namely: (a) literature search and screening; (b) data extraction and analysis; and (c) writing the literature review. They provide detailed and very helpful instructions on how to conduct each step of the review process. As another methodological contribution, vom Brocke et al. (2009) offered a series of guidelines for conducting literature reviews, with a particular focus on how to search and extract the relevant body of knowledge. Last, Bandara, Miskon, and Fielt (2011) proposed a structured, predefined and tool-supported method to identify primary studies within a feasible scope, extract relevant content from identified articles, synthesize and analyze the findings, and effectively write and present the results of the literature review. We highly recommend that prospective authors of narrative reviews consult these useful sources before embarking on their work.
Darlow and Wen (2015) provide a good example of a highly structured narrative review in the eHealth field. These authors synthesized published articles that describe the development process of mobile health ( m-health ) interventions for patients’ cancer care self-management. As in most narrative reviews, the scope of the research questions being investigated is broad: (a) how development of these systems are carried out; (b) which methods are used to investigate these systems; and (c) what conclusions can be drawn as a result of the development of these systems. To provide clear answers to these questions, a literature search was conducted on six electronic databases and Google Scholar . The search was performed using several terms and free text words, combining them in an appropriate manner. Four inclusion and three exclusion criteria were utilized during the screening process. Both authors independently reviewed each of the identified articles to determine eligibility and extract study information. A flow diagram shows the number of studies identified, screened, and included or excluded at each stage of study selection. In terms of contributions, this review provides a series of practical recommendations for m-health intervention development.
9.3.2. Descriptive or Mapping Reviews
The primary goal of a descriptive review is to determine the extent to which a body of knowledge in a particular research topic reveals any interpretable pattern or trend with respect to pre-existing propositions, theories, methodologies or findings ( King & He, 2005 ; Paré et al., 2015 ). In contrast with narrative reviews, descriptive reviews follow a systematic and transparent procedure, including searching, screening and classifying studies ( Petersen, Vakkalanka, & Kuzniarz, 2015 ). Indeed, structured search methods are used to form a representative sample of a larger group of published works ( Paré et al., 2015 ). Further, authors of descriptive reviews extract from each study certain characteristics of interest, such as publication year, research methods, data collection techniques, and direction or strength of research outcomes (e.g., positive, negative, or non-significant) in the form of frequency analysis to produce quantitative results ( Sylvester et al., 2013 ). In essence, each study included in a descriptive review is treated as the unit of analysis and the published literature as a whole provides a database from which the authors attempt to identify any interpretable trends or draw overall conclusions about the merits of existing conceptualizations, propositions, methods or findings ( Paré et al., 2015 ). In doing so, a descriptive review may claim that its findings represent the state of the art in a particular domain ( King & He, 2005 ).
In the fields of health sciences and medical informatics, reviews that focus on examining the range, nature and evolution of a topic area are described by Anderson, Allen, Peckham, and Goodwin (2008) as mapping reviews . Like descriptive reviews, the research questions are generic and usually relate to publication patterns and trends. There is no preconceived plan to systematically review all of the literature although this can be done. Instead, researchers often present studies that are representative of most works published in a particular area and they consider a specific time frame to be mapped.
An example of this approach in the eHealth domain is offered by DeShazo, Lavallie, and Wolf (2009). The purpose of this descriptive or mapping review was to characterize publication trends in the medical informatics literature over a 20-year period (1987 to 2006). To achieve this ambitious objective, the authors performed a bibliometric analysis of medical informatics citations indexed in medline using publication trends, journal frequencies, impact factors, Medical Subject Headings (MeSH) term frequencies, and characteristics of citations. Findings revealed that there were over 77,000 medical informatics articles published during the covered period in numerous journals and that the average annual growth rate was 12%. The MeSH term analysis also suggested a strong interdisciplinary trend. Finally, average impact scores increased over time with two notable growth periods. Overall, patterns in research outputs that seem to characterize the historic trends and current components of the field of medical informatics suggest it may be a maturing discipline (DeShazo et al., 2009).
9.3.3. Scoping Reviews
Scoping reviews attempt to provide an initial indication of the potential size and nature of the extant literature on an emergent topic (Arksey & O’Malley, 2005; Daudt, van Mossel, & Scott, 2013 ; Levac, Colquhoun, & O’Brien, 2010). A scoping review may be conducted to examine the extent, range and nature of research activities in a particular area, determine the value of undertaking a full systematic review (discussed next), or identify research gaps in the extant literature ( Paré et al., 2015 ). In line with their main objective, scoping reviews usually conclude with the presentation of a detailed research agenda for future works along with potential implications for both practice and research.
Unlike narrative and descriptive reviews, the whole point of scoping the field is to be as comprehensive as possible, including grey literature (Arksey & O’Malley, 2005). Inclusion and exclusion criteria must be established to help researchers eliminate studies that are not aligned with the research questions. It is also recommended that at least two independent coders review abstracts yielded from the search strategy and then the full articles for study selection ( Daudt et al., 2013 ). The synthesized evidence from content or thematic analysis is relatively easy to present in tabular form (Arksey & O’Malley, 2005; Thomas & Harden, 2008 ).
One of the most highly cited scoping reviews in the eHealth domain was published by Archer, Fevrier-Thomas, Lokker, McKibbon, and Straus (2011) . These authors reviewed the existing literature on personal health record ( phr ) systems including design, functionality, implementation, applications, outcomes, and benefits. Seven databases were searched from 1985 to March 2010. Several search terms relating to phr s were used during this process. Two authors independently screened titles and abstracts to determine inclusion status. A second screen of full-text articles, again by two independent members of the research team, ensured that the studies described phr s. All in all, 130 articles met the criteria and their data were extracted manually into a database. The authors concluded that although there is a large amount of survey, observational, cohort/panel, and anecdotal evidence of phr benefits and satisfaction for patients, more research is needed to evaluate the results of phr implementations. Their in-depth analysis of the literature signalled that there is little solid evidence from randomized controlled trials or other studies through the use of phr s. Hence, they suggested that more research is needed that addresses the current lack of understanding of optimal functionality and usability of these systems, and how they can play a beneficial role in supporting patient self-management ( Archer et al., 2011 ).
9.3.4. Forms of Aggregative Reviews
Healthcare providers, practitioners, and policy-makers are nowadays overwhelmed with large volumes of information, including research-based evidence from numerous clinical trials and evaluation studies, assessing the effectiveness of health information technologies and interventions ( Ammenwerth & de Keizer, 2004 ; Deshazo et al., 2009 ). It is unrealistic to expect that all these disparate actors will have the time, skills, and necessary resources to identify the available evidence in the area of their expertise and consider it when making decisions. Systematic reviews that involve the rigorous application of scientific strategies aimed at limiting subjectivity and bias (i.e., systematic and random errors) can respond to this challenge.
Systematic reviews attempt to aggregate, appraise, and synthesize in a single source all empirical evidence that meet a set of previously specified eligibility criteria in order to answer a clearly formulated and often narrow research question on a particular topic of interest to support evidence-based practice ( Liberati et al., 2009 ). They adhere closely to explicit scientific principles ( Liberati et al., 2009 ) and rigorous methodological guidelines (Higgins & Green, 2008) aimed at reducing random and systematic errors that can lead to deviations from the truth in results or inferences. The use of explicit methods allows systematic reviews to aggregate a large body of research evidence, assess whether effects or relationships are in the same direction and of the same general magnitude, explain possible inconsistencies between study results, and determine the strength of the overall evidence for every outcome of interest based on the quality of included studies and the general consistency among them ( Cook, Mulrow, & Haynes, 1997 ). The main procedures of a systematic review involve:
- Formulating a review question and developing a search strategy based on explicit inclusion criteria for the identification of eligible studies (usually described in the context of a detailed review protocol).
- Searching for eligible studies using multiple databases and information sources, including grey literature sources, without any language restrictions.
- Selecting studies, extracting data, and assessing risk of bias in a duplicate manner using two independent reviewers to avoid random or systematic errors in the process.
- Analyzing data using quantitative or qualitative methods.
- Presenting results in summary of findings tables.
- Interpreting results and drawing conclusions.
Many systematic reviews, but not all, use statistical methods to combine the results of independent studies into a single quantitative estimate or summary effect size. Known as meta-analyses , these reviews use specific data extraction and statistical techniques (e.g., network, frequentist, or Bayesian meta-analyses) to calculate from each study by outcome of interest an effect size along with a confidence interval that reflects the degree of uncertainty behind the point estimate of effect ( Borenstein, Hedges, Higgins, & Rothstein, 2009 ; Deeks, Higgins, & Altman, 2008 ). Subsequently, they use fixed or random-effects analysis models to combine the results of the included studies, assess statistical heterogeneity, and calculate a weighted average of the effect estimates from the different studies, taking into account their sample sizes. The summary effect size is a value that reflects the average magnitude of the intervention effect for a particular outcome of interest or, more generally, the strength of a relationship between two variables across all studies included in the systematic review. By statistically combining data from multiple studies, meta-analyses can create more precise and reliable estimates of intervention effects than those derived from individual studies alone, when these are examined independently as discrete sources of information.
The review by Gurol-Urganci, de Jongh, Vodopivec-Jamsek, Atun, and Car (2013) on the effects of mobile phone messaging reminders for attendance at healthcare appointments is an illustrative example of a high-quality systematic review with meta-analysis. Missed appointments are a major cause of inefficiency in healthcare delivery with substantial monetary costs to health systems. These authors sought to assess whether mobile phone-based appointment reminders delivered through Short Message Service ( sms ) or Multimedia Messaging Service ( mms ) are effective in improving rates of patient attendance and reducing overall costs. To this end, they conducted a comprehensive search on multiple databases using highly sensitive search strategies without language or publication-type restrictions to identify all rct s that are eligible for inclusion. In order to minimize the risk of omitting eligible studies not captured by the original search, they supplemented all electronic searches with manual screening of trial registers and references contained in the included studies. Study selection, data extraction, and risk of bias assessments were performed independently by two coders using standardized methods to ensure consistency and to eliminate potential errors. Findings from eight rct s involving 6,615 participants were pooled into meta-analyses to calculate the magnitude of effects that mobile text message reminders have on the rate of attendance at healthcare appointments compared to no reminders and phone call reminders.
Meta-analyses are regarded as powerful tools for deriving meaningful conclusions. However, there are situations in which it is neither reasonable nor appropriate to pool studies together using meta-analytic methods simply because there is extensive clinical heterogeneity between the included studies or variation in measurement tools, comparisons, or outcomes of interest. In these cases, systematic reviews can use qualitative synthesis methods such as vote counting, content analysis, classification schemes and tabulations, as an alternative approach to narratively synthesize the results of the independent studies included in the review. This form of review is known as qualitative systematic review.
A rigorous example of one such review in the eHealth domain is presented by Mickan, Atherton, Roberts, Heneghan, and Tilson (2014) on the use of handheld computers by healthcare professionals and their impact on access to information and clinical decision-making. In line with the methodological guidelines for systematic reviews, these authors: (a) developed and registered with prospero ( www.crd.york.ac.uk/ prospero / ) an a priori review protocol; (b) conducted comprehensive searches for eligible studies using multiple databases and other supplementary strategies (e.g., forward searches); and (c) subsequently carried out study selection, data extraction, and risk of bias assessments in a duplicate manner to eliminate potential errors in the review process. Heterogeneity between the included studies in terms of reported outcomes and measures precluded the use of meta-analytic methods. To this end, the authors resorted to using narrative analysis and synthesis to describe the effectiveness of handheld computers on accessing information for clinical knowledge, adherence to safety and clinical quality guidelines, and diagnostic decision-making.
In recent years, the number of systematic reviews in the field of health informatics has increased considerably. Systematic reviews with discordant findings can cause great confusion and make it difficult for decision-makers to interpret the review-level evidence ( Moher, 2013 ). Therefore, there is a growing need for appraisal and synthesis of prior systematic reviews to ensure that decision-making is constantly informed by the best available accumulated evidence. Umbrella reviews , also known as overviews of systematic reviews, are tertiary types of evidence synthesis that aim to accomplish this; that is, they aim to compare and contrast findings from multiple systematic reviews and meta-analyses ( Becker & Oxman, 2008 ). Umbrella reviews generally adhere to the same principles and rigorous methodological guidelines used in systematic reviews. However, the unit of analysis in umbrella reviews is the systematic review rather than the primary study ( Becker & Oxman, 2008 ). Unlike systematic reviews that have a narrow focus of inquiry, umbrella reviews focus on broader research topics for which there are several potential interventions ( Smith, Devane, Begley, & Clarke, 2011 ). A recent umbrella review on the effects of home telemonitoring interventions for patients with heart failure critically appraised, compared, and synthesized evidence from 15 systematic reviews to investigate which types of home telemonitoring technologies and forms of interventions are more effective in reducing mortality and hospital admissions ( Kitsiou, Paré, & Jaana, 2015 ).
9.3.5. Realist Reviews
Realist reviews are theory-driven interpretative reviews developed to inform, enhance, or supplement conventional systematic reviews by making sense of heterogeneous evidence about complex interventions applied in diverse contexts in a way that informs policy decision-making ( Greenhalgh, Wong, Westhorp, & Pawson, 2011 ). They originated from criticisms of positivist systematic reviews which centre on their “simplistic” underlying assumptions ( Oates, 2011 ). As explained above, systematic reviews seek to identify causation. Such logic is appropriate for fields like medicine and education where findings of randomized controlled trials can be aggregated to see whether a new treatment or intervention does improve outcomes. However, many argue that it is not possible to establish such direct causal links between interventions and outcomes in fields such as social policy, management, and information systems where for any intervention there is unlikely to be a regular or consistent outcome ( Oates, 2011 ; Pawson, 2006 ; Rousseau, Manning, & Denyer, 2008 ).
To circumvent these limitations, Pawson, Greenhalgh, Harvey, and Walshe (2005) have proposed a new approach for synthesizing knowledge that seeks to unpack the mechanism of how “complex interventions” work in particular contexts. The basic research question — what works? — which is usually associated with systematic reviews changes to: what is it about this intervention that works, for whom, in what circumstances, in what respects and why? Realist reviews have no particular preference for either quantitative or qualitative evidence. As a theory-building approach, a realist review usually starts by articulating likely underlying mechanisms and then scrutinizes available evidence to find out whether and where these mechanisms are applicable ( Shepperd et al., 2009 ). Primary studies found in the extant literature are viewed as case studies which can test and modify the initial theories ( Rousseau et al., 2008 ).
The main objective pursued in the realist review conducted by Otte-Trojel, de Bont, Rundall, and van de Klundert (2014) was to examine how patient portals contribute to health service delivery and patient outcomes. The specific goals were to investigate how outcomes are produced and, most importantly, how variations in outcomes can be explained. The research team started with an exploratory review of background documents and research studies to identify ways in which patient portals may contribute to health service delivery and patient outcomes. The authors identified six main ways which represent “educated guesses” to be tested against the data in the evaluation studies. These studies were identified through a formal and systematic search in four databases between 2003 and 2013. Two members of the research team selected the articles using a pre-established list of inclusion and exclusion criteria and following a two-step procedure. The authors then extracted data from the selected articles and created several tables, one for each outcome category. They organized information to bring forward those mechanisms where patient portals contribute to outcomes and the variation in outcomes across different contexts.
9.3.6. Critical Reviews
Lastly, critical reviews aim to provide a critical evaluation and interpretive analysis of existing literature on a particular topic of interest to reveal strengths, weaknesses, contradictions, controversies, inconsistencies, and/or other important issues with respect to theories, hypotheses, research methods or results ( Baumeister & Leary, 1997 ; Kirkevold, 1997 ). Unlike other review types, critical reviews attempt to take a reflective account of the research that has been done in a particular area of interest, and assess its credibility by using appraisal instruments or critical interpretive methods. In this way, critical reviews attempt to constructively inform other scholars about the weaknesses of prior research and strengthen knowledge development by giving focus and direction to studies for further improvement ( Kirkevold, 1997 ).
Kitsiou, Paré, and Jaana (2013) provide an example of a critical review that assessed the methodological quality of prior systematic reviews of home telemonitoring studies for chronic patients. The authors conducted a comprehensive search on multiple databases to identify eligible reviews and subsequently used a validated instrument to conduct an in-depth quality appraisal. Results indicate that the majority of systematic reviews in this particular area suffer from important methodological flaws and biases that impair their internal validity and limit their usefulness for clinical and decision-making purposes. To this end, they provide a number of recommendations to strengthen knowledge development towards improving the design and execution of future reviews on home telemonitoring.
9.4. Summary
Table 9.1 outlines the main types of literature reviews that were described in the previous sub-sections and summarizes the main characteristics that distinguish one review type from another. It also includes key references to methodological guidelines and useful sources that can be used by eHealth scholars and researchers for planning and developing reviews.
Typology of Literature Reviews (adapted from Paré et al., 2015).
As shown in Table 9.1 , each review type addresses different kinds of research questions or objectives, which subsequently define and dictate the methods and approaches that need to be used to achieve the overarching goal(s) of the review. For example, in the case of narrative reviews, there is greater flexibility in searching and synthesizing articles ( Green et al., 2006 ). Researchers are often relatively free to use a diversity of approaches to search, identify, and select relevant scientific articles, describe their operational characteristics, present how the individual studies fit together, and formulate conclusions. On the other hand, systematic reviews are characterized by their high level of systematicity, rigour, and use of explicit methods, based on an “a priori” review plan that aims to minimize bias in the analysis and synthesis process (Higgins & Green, 2008). Some reviews are exploratory in nature (e.g., scoping/mapping reviews), whereas others may be conducted to discover patterns (e.g., descriptive reviews) or involve a synthesis approach that may include the critical analysis of prior research ( Paré et al., 2015 ). Hence, in order to select the most appropriate type of review, it is critical to know before embarking on a review project, why the research synthesis is conducted and what type of methods are best aligned with the pursued goals.
9.5. Concluding Remarks
In light of the increased use of evidence-based practice and research generating stronger evidence ( Grady et al., 2011 ; Lyden et al., 2013 ), review articles have become essential tools for summarizing, synthesizing, integrating or critically appraising prior knowledge in the eHealth field. As mentioned earlier, when rigorously conducted review articles represent powerful information sources for eHealth scholars and practitioners looking for state-of-the-art evidence. The typology of literature reviews we used herein will allow eHealth researchers, graduate students and practitioners to gain a better understanding of the similarities and differences between review types.
We must stress that this classification scheme does not privilege any specific type of review as being of higher quality than another ( Paré et al., 2015 ). As explained above, each type of review has its own strengths and limitations. Having said that, we realize that the methodological rigour of any review — be it qualitative, quantitative or mixed — is a critical aspect that should be considered seriously by prospective authors. In the present context, the notion of rigour refers to the reliability and validity of the review process described in section 9.2. For one thing, reliability is related to the reproducibility of the review process and steps, which is facilitated by a comprehensive documentation of the literature search process, extraction, coding and analysis performed in the review. Whether the search is comprehensive or not, whether it involves a methodical approach for data extraction and synthesis or not, it is important that the review documents in an explicit and transparent manner the steps and approach that were used in the process of its development. Next, validity characterizes the degree to which the review process was conducted appropriately. It goes beyond documentation and reflects decisions related to the selection of the sources, the search terms used, the period of time covered, the articles selected in the search, and the application of backward and forward searches ( vom Brocke et al., 2009 ). In short, the rigour of any review article is reflected by the explicitness of its methods (i.e., transparency) and the soundness of the approach used. We refer those interested in the concepts of rigour and quality to the work of Templier and Paré (2015) which offers a detailed set of methodological guidelines for conducting and evaluating various types of review articles.
To conclude, our main objective in this chapter was to demystify the various types of literature reviews that are central to the continuous development of the eHealth field. It is our hope that our descriptive account will serve as a valuable source for those conducting, evaluating or using reviews in this important and growing domain.
- Ammenwerth E., de Keizer N. An inventory of evaluation studies of information technology in health care. Trends in evaluation research, 1982-2002. International Journal of Medical Informatics. 2004; 44 (1):44–56. [ PubMed : 15778794 ]
- Anderson S., Allen P., Peckham S., Goodwin N. Asking the right questions: scoping studies in the commissioning of research on the organisation and delivery of health services. Health Research Policy and Systems. 2008; 6 (7):1–12. [ PMC free article : PMC2500008 ] [ PubMed : 18613961 ] [ CrossRef ]
- Archer N., Fevrier-Thomas U., Lokker C., McKibbon K. A., Straus S.E. Personal health records: a scoping review. Journal of American Medical Informatics Association. 2011; 18 (4):515–522. [ PMC free article : PMC3128401 ] [ PubMed : 21672914 ]
- Arksey H., O’Malley L. Scoping studies: towards a methodological framework. International Journal of Social Research Methodology. 2005; 8 (1):19–32.
- A systematic, tool-supported method for conducting literature reviews in information systems. Paper presented at the Proceedings of the 19th European Conference on Information Systems ( ecis 2011); June 9 to 11; Helsinki, Finland. 2011.
- Baumeister R. F., Leary M.R. Writing narrative literature reviews. Review of General Psychology. 1997; 1 (3):311–320.
- Becker L. A., Oxman A.D. In: Cochrane handbook for systematic reviews of interventions. Higgins J. P. T., Green S., editors. Hoboken, nj : John Wiley & Sons, Ltd; 2008. Overviews of reviews; pp. 607–631.
- Borenstein M., Hedges L., Higgins J., Rothstein H. Introduction to meta-analysis. Hoboken, nj : John Wiley & Sons Inc; 2009.
- Cook D. J., Mulrow C. D., Haynes B. Systematic reviews: Synthesis of best evidence for clinical decisions. Annals of Internal Medicine. 1997; 126 (5):376–380. [ PubMed : 9054282 ]
- Cooper H., Hedges L.V. In: The handbook of research synthesis and meta-analysis. 2nd ed. Cooper H., Hedges L. V., Valentine J. C., editors. New York: Russell Sage Foundation; 2009. Research synthesis as a scientific process; pp. 3–17.
- Cooper H. M. Organizing knowledge syntheses: A taxonomy of literature reviews. Knowledge in Society. 1988; 1 (1):104–126.
- Cronin P., Ryan F., Coughlan M. Undertaking a literature review: a step-by-step approach. British Journal of Nursing. 2008; 17 (1):38–43. [ PubMed : 18399395 ]
- Darlow S., Wen K.Y. Development testing of mobile health interventions for cancer patient self-management: A review. Health Informatics Journal. 2015 (online before print). [ PubMed : 25916831 ] [ CrossRef ]
- Daudt H. M., van Mossel C., Scott S.J. Enhancing the scoping study methodology: a large, inter-professional team’s experience with Arksey and O’Malley’s framework. bmc Medical Research Methodology. 2013; 13 :48. [ PMC free article : PMC3614526 ] [ PubMed : 23522333 ] [ CrossRef ]
- Davies P. The relevance of systematic reviews to educational policy and practice. Oxford Review of Education. 2000; 26 (3-4):365–378.
- Deeks J. J., Higgins J. P. T., Altman D.G. In: Cochrane handbook for systematic reviews of interventions. Higgins J. P. T., Green S., editors. Hoboken, nj : John Wiley & Sons, Ltd; 2008. Analysing data and undertaking meta-analyses; pp. 243–296.
- Deshazo J. P., Lavallie D. L., Wolf F.M. Publication trends in the medical informatics literature: 20 years of “Medical Informatics” in mesh . bmc Medical Informatics and Decision Making. 2009; 9 :7. [ PMC free article : PMC2652453 ] [ PubMed : 19159472 ] [ CrossRef ]
- Dixon-Woods M., Agarwal S., Jones D., Young B., Sutton A. Synthesising qualitative and quantitative evidence: a review of possible methods. Journal of Health Services Research and Policy. 2005; 10 (1):45–53. [ PubMed : 15667704 ]
- Finfgeld-Connett D., Johnson E.D. Literature search strategies for conducting knowledge-building and theory-generating qualitative systematic reviews. Journal of Advanced Nursing. 2013; 69 (1):194–204. [ PMC free article : PMC3424349 ] [ PubMed : 22591030 ]
- Grady B., Myers K. M., Nelson E. L., Belz N., Bennett L., Carnahan L. … Guidelines Working Group. Evidence-based practice for telemental health. Telemedicine Journal and E Health. 2011; 17 (2):131–148. [ PubMed : 21385026 ]
- Green B. N., Johnson C. D., Adams A. Writing narrative literature reviews for peer-reviewed journals: secrets of the trade. Journal of Chiropractic Medicine. 2006; 5 (3):101–117. [ PMC free article : PMC2647067 ] [ PubMed : 19674681 ]
- Greenhalgh T., Wong G., Westhorp G., Pawson R. Protocol–realist and meta-narrative evidence synthesis: evolving standards ( rameses ). bmc Medical Research Methodology. 2011; 11 :115. [ PMC free article : PMC3173389 ] [ PubMed : 21843376 ]
- Gurol-Urganci I., de Jongh T., Vodopivec-Jamsek V., Atun R., Car J. Mobile phone messaging reminders for attendance at healthcare appointments. Cochrane Database System Review. 2013; 12 cd 007458. [ PMC free article : PMC6485985 ] [ PubMed : 24310741 ] [ CrossRef ]
- Hart C. Doing a literature review: Releasing the social science research imagination. London: SAGE Publications; 1998.
- Higgins J. P. T., Green S., editors. Cochrane handbook for systematic reviews of interventions: Cochrane book series. Hoboken, nj : Wiley-Blackwell; 2008.
- Jesson J., Matheson L., Lacey F.M. Doing your literature review: traditional and systematic techniques. Los Angeles & London: SAGE Publications; 2011.
- King W. R., He J. Understanding the role and methods of meta-analysis in IS research. Communications of the Association for Information Systems. 2005; 16 :1.
- Kirkevold M. Integrative nursing research — an important strategy to further the development of nursing science and nursing practice. Journal of Advanced Nursing. 1997; 25 (5):977–984. [ PubMed : 9147203 ]
- Kitchenham B., Charters S. ebse Technical Report Version 2.3. Keele & Durham. uk : Keele University & University of Durham; 2007. Guidelines for performing systematic literature reviews in software engineering.
- Kitsiou S., Paré G., Jaana M. Systematic reviews and meta-analyses of home telemonitoring interventions for patients with chronic diseases: a critical assessment of their methodological quality. Journal of Medical Internet Research. 2013; 15 (7):e150. [ PMC free article : PMC3785977 ] [ PubMed : 23880072 ]
- Kitsiou S., Paré G., Jaana M. Effects of home telemonitoring interventions on patients with chronic heart failure: an overview of systematic reviews. Journal of Medical Internet Research. 2015; 17 (3):e63. [ PMC free article : PMC4376138 ] [ PubMed : 25768664 ]
- Levac D., Colquhoun H., O’Brien K. K. Scoping studies: advancing the methodology. Implementation Science. 2010; 5 (1):69. [ PMC free article : PMC2954944 ] [ PubMed : 20854677 ]
- Levy Y., Ellis T.J. A systems approach to conduct an effective literature review in support of information systems research. Informing Science. 2006; 9 :181–211.
- Liberati A., Altman D. G., Tetzlaff J., Mulrow C., Gøtzsche P. C., Ioannidis J. P. A. et al. Moher D. The prisma statement for reporting systematic reviews and meta-analyses of studies that evaluate health care interventions: Explanation and elaboration. Annals of Internal Medicine. 2009; 151 (4):W-65. [ PubMed : 19622512 ]
- Lyden J. R., Zickmund S. L., Bhargava T. D., Bryce C. L., Conroy M. B., Fischer G. S. et al. McTigue K. M. Implementing health information technology in a patient-centered manner: Patient experiences with an online evidence-based lifestyle intervention. Journal for Healthcare Quality. 2013; 35 (5):47–57. [ PubMed : 24004039 ]
- Mickan S., Atherton H., Roberts N. W., Heneghan C., Tilson J.K. Use of handheld computers in clinical practice: a systematic review. bmc Medical Informatics and Decision Making. 2014; 14 :56. [ PMC free article : PMC4099138 ] [ PubMed : 24998515 ]
- Moher D. The problem of duplicate systematic reviews. British Medical Journal. 2013; 347 (5040) [ PubMed : 23945367 ] [ CrossRef ]
- Montori V. M., Wilczynski N. L., Morgan D., Haynes R. B., Hedges T. Systematic reviews: a cross-sectional study of location and citation counts. bmc Medicine. 2003; 1 :2. [ PMC free article : PMC281591 ] [ PubMed : 14633274 ]
- Mulrow C. D. The medical review article: state of the science. Annals of Internal Medicine. 1987; 106 (3):485–488. [ PubMed : 3813259 ] [ CrossRef ]
- Evidence-based information systems: A decade later. Proceedings of the European Conference on Information Systems ; 2011. Retrieved from http://aisel .aisnet.org/cgi/viewcontent .cgi?article =1221&context =ecis2011 .
- Okoli C., Schabram K. A guide to conducting a systematic literature review of information systems research. ssrn Electronic Journal. 2010
- Otte-Trojel T., de Bont A., Rundall T. G., van de Klundert J. How outcomes are achieved through patient portals: a realist review. Journal of American Medical Informatics Association. 2014; 21 (4):751–757. [ PMC free article : PMC4078283 ] [ PubMed : 24503882 ]
- Paré G., Trudel M.-C., Jaana M., Kitsiou S. Synthesizing information systems knowledge: A typology of literature reviews. Information & Management. 2015; 52 (2):183–199.
- Patsopoulos N. A., Analatos A. A., Ioannidis J.P. A. Relative citation impact of various study designs in the health sciences. Journal of the American Medical Association. 2005; 293 (19):2362–2366. [ PubMed : 15900006 ]
- Paul M. M., Greene C. M., Newton-Dame R., Thorpe L. E., Perlman S. E., McVeigh K. H., Gourevitch M.N. The state of population health surveillance using electronic health records: A narrative review. Population Health Management. 2015; 18 (3):209–216. [ PubMed : 25608033 ]
- Pawson R. Evidence-based policy: a realist perspective. London: SAGE Publications; 2006.
- Pawson R., Greenhalgh T., Harvey G., Walshe K. Realist review—a new method of systematic review designed for complex policy interventions. Journal of Health Services Research & Policy. 2005; 10 (Suppl 1):21–34. [ PubMed : 16053581 ]
- Petersen K., Vakkalanka S., Kuzniarz L. Guidelines for conducting systematic mapping studies in software engineering: An update. Information and Software Technology. 2015; 64 :1–18.
- Petticrew M., Roberts H. Systematic reviews in the social sciences: A practical guide. Malden, ma : Blackwell Publishing Co; 2006.
- Rousseau D. M., Manning J., Denyer D. Evidence in management and organizational science: Assembling the field’s full weight of scientific knowledge through syntheses. The Academy of Management Annals. 2008; 2 (1):475–515.
- Rowe F. What literature review is not: diversity, boundaries and recommendations. European Journal of Information Systems. 2014; 23 (3):241–255.
- Shea B. J., Hamel C., Wells G. A., Bouter L. M., Kristjansson E., Grimshaw J. et al. Boers M. amstar is a reliable and valid measurement tool to assess the methodological quality of systematic reviews. Journal of Clinical Epidemiology. 2009; 62 (10):1013–1020. [ PubMed : 19230606 ]
- Shepperd S., Lewin S., Straus S., Clarke M., Eccles M. P., Fitzpatrick R. et al. Sheikh A. Can we systematically review studies that evaluate complex interventions? PLoS Medicine. 2009; 6 (8):e1000086. [ PMC free article : PMC2717209 ] [ PubMed : 19668360 ]
- Silva B. M., Rodrigues J. J., de la Torre Díez I., López-Coronado M., Saleem K. Mobile-health: A review of current state in 2015. Journal of Biomedical Informatics. 2015; 56 :265–272. [ PubMed : 26071682 ]
- Smith V., Devane D., Begley C., Clarke M. Methodology in conducting a systematic review of systematic reviews of healthcare interventions. bmc Medical Research Methodology. 2011; 11 (1):15. [ PMC free article : PMC3039637 ] [ PubMed : 21291558 ]
- Sylvester A., Tate M., Johnstone D. Beyond synthesis: re-presenting heterogeneous research literature. Behaviour & Information Technology. 2013; 32 (12):1199–1215.
- Templier M., Paré G. A framework for guiding and evaluating literature reviews. Communications of the Association for Information Systems. 2015; 37 (6):112–137.
- Thomas J., Harden A. Methods for the thematic synthesis of qualitative research in systematic reviews. bmc Medical Research Methodology. 2008; 8 (1):45. [ PMC free article : PMC2478656 ] [ PubMed : 18616818 ]
- Reconstructing the giant: on the importance of rigour in documenting the literature search process. Paper presented at the Proceedings of the 17th European Conference on Information Systems ( ecis 2009); Verona, Italy. 2009.
- Webster J., Watson R.T. Analyzing the past to prepare for the future: Writing a literature review. Management Information Systems Quarterly. 2002; 26 (2):11.
- Whitlock E. P., Lin J. S., Chou R., Shekelle P., Robinson K.A. Using existing systematic reviews in complex systematic reviews. Annals of Internal Medicine. 2008; 148 (10):776–782. [ PubMed : 18490690 ]
This publication is licensed under a Creative Commons License, Attribution-Noncommercial 4.0 International License (CC BY-NC 4.0): see https://creativecommons.org/licenses/by-nc/4.0/
- Cite this Page Paré G, Kitsiou S. Chapter 9 Methods for Literature Reviews. In: Lau F, Kuziemsky C, editors. Handbook of eHealth Evaluation: An Evidence-based Approach [Internet]. Victoria (BC): University of Victoria; 2017 Feb 27.
- PDF version of this title (4.5M)
- Disable Glossary Links
In this Page
- Introduction
- Overview of the Literature Review Process and Steps
- Types of Review Articles and Brief Illustrations
- Concluding Remarks
Related information
- PMC PubMed Central citations
- PubMed Links to PubMed
Recent Activity
- Chapter 9 Methods for Literature Reviews - Handbook of eHealth Evaluation: An Ev... Chapter 9 Methods for Literature Reviews - Handbook of eHealth Evaluation: An Evidence-based Approach
Your browsing activity is empty.
Activity recording is turned off.
Turn recording back on
Connect with NLM
National Library of Medicine 8600 Rockville Pike Bethesda, MD 20894
Web Policies FOIA HHS Vulnerability Disclosure
Help Accessibility Careers
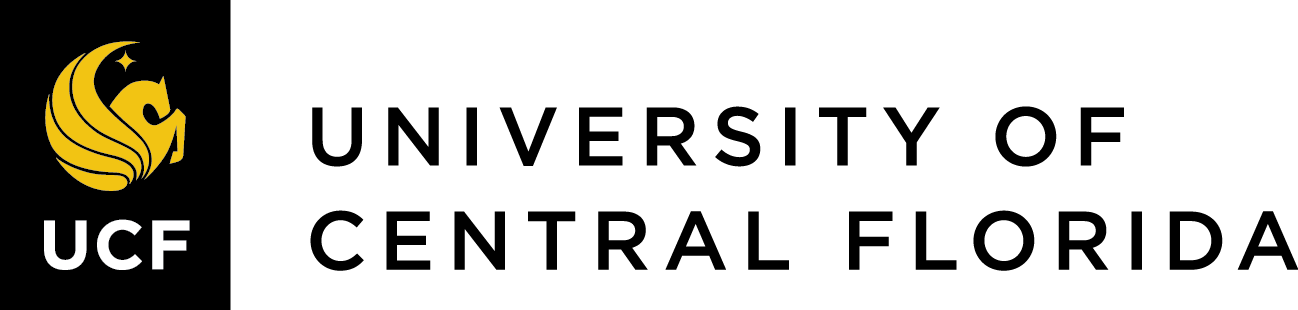
Chapter Four: Theory, Methodologies, Methods, and Evidence
Research Methods
You are viewing the first edition of this textbook. a second edition is available – please visit the latest edition for updated information..
This page discusses the following topics:
Research Goals
Research method types.
Before discussing research methods , we need to distinguish them from methodologies and research skills . Methodologies, linked to literary theories, are tools and lines of investigation: sets of practices and propositions about texts and the world. Researchers using Marxist literary criticism will adopt methodologies that look to material forces like labor, ownership, and technology to understand literature and its relationship to the world. They will also seek to understand authors not as inspired geniuses but as people whose lives and work are shaped by social forces.
Example: Critical Race Theory Methodologies
Critical Race Theory may use a variety of methodologies, including
- Interest convergence: investigating whether marginalized groups only achieve progress when dominant groups benefit as well
- Intersectional theory: investigating how multiple factors of advantage and disadvantage around race, gender, ethnicity, religion, etc. operate together in complex ways
- Radical critique of the law: investigating how the law has historically been used to marginalize particular groups, such as black people, while recognizing that legal efforts are important to achieve emancipation and civil rights
- Social constructivism: investigating how race is socially constructed (rather than biologically grounded)
- Standpoint epistemology: investigating how knowledge relates to social position
- Structural determinism: investigating how structures of thought and of organizations determine social outcomes
To identify appropriate methodologies, you will need to research your chosen theory and gather what methodologies are associated with it. For the most part, we can’t assume that there are “one size fits all” methodologies.
Research skills are about how you handle materials such as library search engines, citation management programs, special collections materials, and so on.
Research methods are about where and how you get answers to your research questions. Are you conducting interviews? Visiting archives? Doing close readings? Reviewing scholarship? You will need to choose which methods are most appropriate to use in your research and you need to gain some knowledge about how to use these methods. In other words, you need to do some research into research methods!
Your choice of research method depends on the kind of questions you are asking. For example, if you want to understand how an author progressed through several drafts to arrive at a final manuscript, you may need to do archival research. If you want to understand why a particular literary work became a bestseller, you may need to do audience research. If you want to know why a contemporary author wrote a particular work, you may need to do interviews. Usually literary research involves a combination of methods such as archival research , discourse analysis , and qualitative research methods.
Literary research methods tend to differ from research methods in the hard sciences (such as physics and chemistry). Science research must present results that are reproducible, while literary research rarely does (though it must still present evidence for its claims). Literary research often deals with questions of meaning, social conventions, representations of lived experience, and aesthetic effects; these are questions that reward dialogue and different perspectives rather than one great experiment that settles the issue. In literary research, we might get many valuable answers even though they are quite different from one another. Also in literary research, we usually have some room to speculate about answers, but our claims have to be plausible (believable) and our argument comprehensive (meaning we don’t overlook evidence that would alter our argument significantly if it were known).
A literary researcher might select the following:
Theory: Critical Race Theory
Methodology: Social Constructivism
Method: Scholarly
Skills: Search engines, citation management
Wendy Belcher, in Writing Your Journal Article in 12 Weeks , identifies two main approaches to understanding literary works: looking at a text by itself (associated with New Criticism ) and looking at texts as they connect to society (associated with Cultural Studies ). The goal of New Criticism is to bring the reader further into the text. The goal of Cultural Studies is to bring the reader into the network of discourses that surround and pass through the text. Other approaches, such as Ecocriticism, relate literary texts to the Sciences (as well as to the Humanities).
The New Critics, starting in the 1940s, focused on meaning within the text itself, using a method they called “ close reading .” The text itself becomes e vidence for a particular reading. Using this approach, you should summarize the literary work briefly and q uote particularly meaningful passages, being sure to introduce quotes and then interpret them (never let them stand alone). Make connections within the work; a sk “why” and “how” the various parts of the text relate to each other.
Cultural Studies critics see all texts as connected to society; the critic therefore has to connect a text to at least one political or social issue. How and why does the text reproduce particular knowledge systems (known as discourses) and how do these knowledge systems relate to issues of power within the society? Who speaks and when? Answering these questions helps your reader understand the text in context. Cultural contexts can include the treatment of gender (Feminist, Queer), class (Marxist), nationality, race, religion, or any other area of human society.
Other approaches, such as psychoanalytic literary criticism , look at literary texts to better understand human psychology. A psychoanalytic reading can focus on a character, the author, the reader, or on society in general. Ecocriticism look at human understandings of nature in literary texts.
We select our research methods based on the kinds of things we want to know. For example, we may be studying the relationship between literature and society, between author and text, or the status of a work in the literary canon. We may want to know about a work’s form, genre, or thematics. We may want to know about the audience’s reading and reception, or about methods for teaching literature in schools.
Below are a few research methods and their descriptions. You may need to consult with your instructor about which ones are most appropriate for your project. The first list covers methods most students use in their work. The second list covers methods more commonly used by advanced researchers. Even if you will not be using methods from this second list in your research project, you may read about these research methods in the scholarship you find.
Most commonly used undergraduate research methods:
- Scholarship Methods: Studies the body of scholarship written about a particular author, literary work, historical period, literary movement, genre, theme, theory, or method.
- Textual Analysis Methods: Used for close readings of literary texts, these methods also rely on literary theory and background information to support the reading.
- Biographical Methods: Used to study the life of the author to better understand their work and times, these methods involve reading biographies and autobiographies about the author, and may also include research into private papers, correspondence, and interviews.
- Discourse Analysis Methods: Studies language patterns to reveal ideology and social relations of power. This research involves the study of institutions, social groups, and social movements to understand how people in various settings use language to represent the world to themselves and others. Literary works may present complex mixtures of discourses which the characters (and readers) have to navigate.
- Creative Writing Methods: A literary re-working of another literary text, creative writing research is used to better understand a literary work by investigating its language, formal structures, composition methods, themes, and so on. For instance, a creative research project may retell a story from a minor character’s perspective to reveal an alternative reading of events. To qualify as research, a creative research project is usually combined with a piece of theoretical writing that explains and justifies the work.
Methods used more often by advanced researchers:
- Archival Methods: Usually involves trips to special collections where original papers are kept. In these archives are many unpublished materials such as diaries, letters, photographs, ledgers, and so on. These materials can offer us invaluable insight into the life of an author, the development of a literary work, or the society in which the author lived. There are at least three major archives of James Baldwin’s papers: The Smithsonian , Yale , and The New York Public Library . Descriptions of such materials are often available online, but the materials themselves are typically stored in boxes at the archive.
- Computational Methods: Used for statistical analysis of texts such as studies of the popularity and meaning of particular words in literature over time.
- Ethnographic Methods: Studies groups of people and their interactions with literary works, for instance in educational institutions, in reading groups (such as book clubs), and in fan networks. This approach may involve interviews and visits to places (including online communities) where people interact with literary works. Note: before you begin such work, you must have Institutional Review Board (IRB) approval “to protect the rights and welfare of human participants involved in research.”
- Visual Methods: Studies the visual qualities of literary works. Some literary works, such as illuminated manuscripts, children’s literature, and graphic novels, present a complex interplay of text and image. Even works without illustrations can be studied for their use of typography, layout, and other visual features.
Regardless of the method(s) you choose, you will need to learn how to apply them to your work and how to carry them out successfully. For example, you should know that many archives do not allow you to bring pens (you can use pencils) and you may not be allowed to bring bags into the archives. You will need to keep a record of which documents you consult and their location (box number, etc.) in the archives. If you are unsure how to use a particular method, please consult a book about it. [1] Also, ask for the advice of trained researchers such as your instructor or a research librarian.
- What research method(s) will you be using for your paper? Why did you make this method selection over other methods? If you haven’t made a selection yet, which methods are you considering?
- What specific methodological approaches are you most interested in exploring in relation to the chosen literary work?
- What is your plan for researching your method(s) and its major approaches?
- What was the most important lesson you learned from this page? What point was confusing or difficult to understand?
Write your answers in a webcourse discussion page.
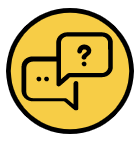
- Introduction to Research Methods: A Practical Guide for Anyone Undertaking a Research Project by Catherine, Dr. Dawson
- Practical Research Methods: A User-Friendly Guide to Mastering Research Techniques and Projects by Catherine Dawson
- Qualitative Inquiry and Research Design: Choosing Among Five Approaches by John W. Creswell Cheryl N. Poth
- Qualitative Research Evaluation Methods: Integrating Theory and Practice by Michael Quinn Patton
- Research Design: Qualitative, Quantitative, and Mixed Methods Approaches by John W. Creswell J. David Creswell
- Research Methodology: A Step-by-Step Guide for Beginners by Ranjit Kumar
- Research Methodology: Methods and Techniques by C.R. Kothari
Strategies for Conducting Literary Research Copyright © 2021 by Barry Mauer & John Venecek is licensed under a Creative Commons Attribution-NonCommercial-ShareAlike 4.0 International License , except where otherwise noted.
Share This Book
Hybrid intelligence failure analysis for industry 4.0: a literature review and future prospective
- Open access
- Published: 22 April 2024
Cite this article
You have full access to this open access article
- Mahdi Mokhtarzadeh ORCID: orcid.org/0000-0002-0348-6718 1 , 2 ,
- Jorge Rodríguez-Echeverría 1 , 2 , 3 ,
- Ivana Semanjski 1 , 2 &
- Sidharta Gautama 1 , 2
Industry 4.0 and advanced technology, such as sensors and human–machine cooperation, provide new possibilities for infusing intelligence into failure analysis. Failure analysis is the process of identifying (potential) failures and determining their causes and effects to enhance reliability and manufacturing quality. Proactive methodologies, such as failure mode and effects analysis (FMEA), and reactive methodologies, such as root cause analysis (RCA) and fault tree analysis (FTA), are used to analyze failures before and after their occurrence. This paper focused on failure analysis methodologies intelligentization literature applied to FMEA, RCA, and FTA to provide insights into expert-driven, data-driven, and hybrid intelligence failure analysis advancements. Types of data to establish an intelligence failure analysis, tools to find a failure’s causes and effects, e.g., Bayesian networks, and managerial insights are discussed. This literature review, along with the analyses within it, assists failure and quality analysts in developing effective hybrid intelligence failure analysis methodologies that leverage the strengths of both proactive and reactive methods.
Avoid common mistakes on your manuscript.
Introduction
Failure analysis entails activities to identify, categorize, and prioritize (potential) failures and determine causes and effects of each failure and failure propagation and interdependencies (Rausand & Øien, 1996 ). Failure analysis significance in manufacturing has grown since Industry 3.0 to mitigate defects and/or failures in production processes, thereby maximizing reliability and quality and minimizing production interruptions, associated risks, and costs (Wu et al., 2021 ; Ebeling, 2019 ).
Failure analysis methodologies have been supported by mathematical, statistical, and graph theories and tools, including MCDM theory, fuzzy theory, six-sigma, SPC, DOE, simulation, Pareto charts, and analysis of mean and variance (Oliveira et al., 2021 ; Huang et al., 2020 ; Tari & Sabater, 2004 ). Industry 4.0 is driven by (real-time) data from sensors, the Internet of Things (IoT), such as Internet-enabled machines and tools, and artificial intelligence (AI). Advances in artificial intelligence theory and technology have brought new tools to strengthen failure analysis methodologies (Oztemel & Gursev, 2020 ). Examples of tools include Bayesian networks (BNs), case-based reasoning (CBR), neural networks, classifications, clusterings algorithms, principal component analysis (PCA), deep learning, decision trees, and ontology-driven methods (Zheng et al., 2021 ). These Industry 4.0 advancments enable more efficient data collection and analysis, enhancing predictive capabilities, increasing efficiency and automation, and improving collaboration and knowledge sharing.
Failure analysis methodologies can be categorized into expert-driven, data-driven, and hybrid ones. Expert-driven failure analysis methods rely on experts’ knowledge and experience (Yucesan et al., 2021 ; Huang et al., 2020 ). This approach is useful when the data is limited or when there is a high degree of uncertainty. Expert-driven methods are also useful when the failure structure is complex and difficult to understand. However, this approach is limited by the availability and expertise of the experts, and is prone to bias and subjective interpretations (Liu et al., 2013 ).
Data-driven failure analysis methods, on the other hand, rely on statistical analysis and machine learning algorithms to identify patterns in the data and predict the causes of the failure (Zhang et al., 2023 ; Mazzoleni et al., 2017 ). This approach is useful when there is a large amount of data available and when the failure structure is well-defined. However, data-driven methods is limited by the quality and completeness of the data (Oliveira et al., 2021 ).
Until recently, most tools have focused on replacing humans with artificial intelligence (Yang et al., 2020 ; Filz et al., 2021b ), which causes them to remove human intellect and capabilities from intelligence systems. Hybrid intelligence creates hybrid human–machine intelligence systems, in which humans and machines collaborate synergistically, proactively, and purposefully to augment human intellect and capabilities rather than replace them with machine intellect and capabilities to achieve shared goals (Akata et al., 2020 ).
Collaboration between humans and machines can enhance the failure analysis process, allowing for analyses that were previously unattainable by either humans or machines alone. Thus, hybrid failure analysis provides a more comprehensive analysis of the failure by incorporating strengths of both expert-driven and data-driven approaches to identify the most likely causes and effects of failures (Dellermann et al., 2019 ; van der Aalst, 2021 ).
Benefits from a smart failure analysis may include reduced costs and production stoppages, improved use of human resources, improved use of knowledge, improved failure, root causes, and effects identification, and real-time failure analysis. Yet, only a few studies specifically addressed hybrid failure analysis (Chhetri et al., 2023 ). A case example of hybrid expert data-driven failure analysis involves using data from similar product assemblies to construct a Bayesian network for proccess failure mode and effects analysis (pFMEA), while also incorporating expert knowledge as constraints based on the specific product being analyzed (Chhetri et al., 2023 ).
Over the past few years, several literature reviews, as reported in Section Literature review , have been accomplished under different outlooks in relation to different failure analysis methodologies including failure mode and effects analysis (FMEA), root cause analysis (RCA), and fault tree analysis (FTA). Currently, most existing literature does not systematically summarize the research status of these failure analysis methodologies from the perspective of Industry 4.0 and (hybrid) intelligence failure analysis with the benefits from new technologies. Therefore, this study aims to review, categorize, and analyze the literature of these three general failure analysis methodologies in production systems. The objective is to provide researchers with a comprehensive overview of these methodologies, with a specific focus on hybrid intelligence, and its benefits for quality issues in production. We address two questions "How can failure analysis methodologies benefit from hybrid intelligence?" and "Which tools are suitable for a good fusion of human and machine intelligence?" Consequently, the main contributions of this study to the failure analysis literature are as follows:
Analysis of 86 papers out of 7113 papers from FMEA, RCA, and FTA with respect to methods and data types that might be useful for a hybrid intelligence failure analysis.
Identification of data and methods to construct and detect multiple failures within different research related to FMEA, RCA, and FTA methodologies.
Identification of the most effective methods for analyzing failures, identifying their sources and effects, and assessing related risks.
Proposal of a categorization of research based on the levels of automation/intelligence, along with the identification of limitations in current research in this regard.
Provision of hybrid intelligent failure analysis future research, along with other future directions such as future research on failure propagation and correlation.
The plan of this paper is as follows. Section Literature review briefly introduces related literature reviews on FMEA, RCA, and FTA. A brief description of other failure analysis methodologies is also provided. Section Research methodology presents our review methodology, including the review scope and protocols, defining both our primary and secondary questions, and the criteria for selecting journals and papers to be reviewed. A bibliography summary of the selected papers is provided. Literature has been categorized in Section Literature categorization based on the four general steps of a failure analysis methodology, involving failure structure detection, failure event probability detection, failure risk analysis, and outputs. Managerial insights, limitations, and future research are discussed in Section Managerial insights, limitations, and future research . This assists researchers with applications and complexity, levels of intelligence, how knowledge is introduced into the failure analysis. A more in-depth discussion of hybrid intelligence, failure propagation and correlation, hybrid methodologies, and other areas of future research is also included. Conclusions are presented in Section Conclusion .
Literature review
General and industry/field-specific failure analysis methodologies have been developed over the last few decades. In this section, we provide useful review papers regarding FMEA, RCA, and FTA, which are the focus of our paper. Additionally, some other general and industry/field-specific failure analysis methodologies are briefly discussed.
FMEA is a most commonly used bottom-up proactive qualitative methodologies for potential quality failure analysis (Huang et al., 2020 ; Stamatis, 2003 ). Among its extensions, process FMEA (pFMEA) proactively identifies potential quality failures in production processes such as assembly lines (Johnson & Khan, 2003 ). Typically, (p)FMEA uses expert knowledge to determine potential failures, effects, and causes, and to prioritize the failures based on the risk priority number (RPN). RPN is a product of severity, occurrence, and detection rates for each failure (Wu et al., 2021 ). Some of the FMEA shortcomings include time-consuming, subjectivity, inability to determine multiple failures, and failure propagation and interdependency (Liu et al., 2013 ).
RCA is a bottom-up reactive quantitative methodology that determines the causal mechanism behind a failure to prevent the recurrence of the failure in manufacturing processes (Oliveira et al., 2023 ). To locate, identify, and/or explain the reasons behind the occurrence of root causes, RCA utilizes statistical analysis tools, such as regression, statistical process control (SPC), design of experiments (DOE), PCA, and cause-effect diagram (Williams, 2001 ). Limited ability to predict future failures and difficulty in identifying complex or systemic issues are among RCA limitations (Yuniarto, 2012 ).
FTA is a top-down reactive graphical method to model failure propagation through a system, i.e., how component failures lead to system failures (Kumar & Kaushik, 2020 ). FTA uses qualitative data to model the structure of a system and quantitative data, including probabilities and graph methods such as minimal cut/path sets, binary decision diagrams, simulation, and BNs, to model failures propagation. Requiring extensive data, limited ability to identify contributing factors, and time-consuming are among the FTA limitations (Ruijters & Stoelinga, 2015 ).
In recent years, several literature reviews have been conducted on failure analysis methodologies, exploring various perspectives and approaches. Liu et al. ( 2013 ) reviewed FMEA risk evaluation tools including rule-based systems, mathematical programming, and multi-criteria decision-making (MCDM). They concluded that artificial intelligence and MCDM tools, particularly fuzzy rule base systems, grey theory, and cost-based models, are the most cited tools to prioritize risks in FMEA. Liu et al. ( 2019a ) and Dabous et al. ( 2021 ) reviewed MCDM tools application for FMEA. Papers with different areas, automotive, electronics, machinery and equipment, and steel manufacturing were considered. The most used MCDM tools, namely technique for order of preference by similarity to ideal solution (TOPSIS), analytic hierarchy process (AHP), decision-making trial and evaluation laboratory (DEMATEL), and grey theory, were identified.
Spreafico et al. ( 2017 ) provided a FMEA/Failure mode, effects, and criticality analysis (FMECA) critical review by classifying FMEA/FMECA limitations and issues and reviewing suggested improvements and solutions for the limitations. FMEA issues were classified into four groups of applicabilities, cause and effect analysis, risk analysis, and problem-solving. Main problems (and solutions) are being time-consuming (integration with design tools, using more structured templates, and automation), lack of secondary effects modeling (integration with other tools such as FTA, BN, and Petri net), being too subjective (using statistical evaluation and cost-based approaches), and lack in evaluating the implementation of a solution (using the improved presentation of the results and integration with other tools such as maintenance management tools), respectively. Huang et al. ( 2020 ) provided a bibliographic analysis of FMEA and its applications in manufacturing, marine, healthcare, aerospace, and electronics. Wu et al. ( 2021 ) sorted out potential failure mode identification approaches such as analyzing entry point for system failure mode identification, failure mode recognition tools, and failure mode specification description. Then a review of FMEA risk assessment tools had been provided.
Oliveira et al. ( 2023 ) reviewed automatic RCA literature in manufacturing. Different data types, location-time, physical, and log-action, that are usually used were identified. Industries with the most use of RCA are ranked, semiconductor, chemical, automotive, and others. Then different tools used to automate RCA, including decision trees, regression models, classification methods, clustering methods, neural networks, BNs, PCA, statistical tests, and control charts, were discussed. Ruijters and Stoelinga ( 2015 ) provided FTA qualitative and quantitative analysis methods. Also, different types of FTA, standard FTA, dynamic FTA, and other extensions, were discussed. Zhu and Zhang ( 2022 ) also reviewed dynamic FTA. Cai et al. ( 2017 ) reviewed the application of BN in fault diagnosis. First, an overview of BN types (static, dynamic, and object-oriented), structure modeling, parameters modeling, and interference has been provided. Then applicability of BN for fault identification in process, energy, structural, manufacturing, and network systems has been discussed. BN verification and validation methods are provided. Future prospects including integration of big data with BN, real-time fault diagnosis BN inference algorithms, and hybrid fault diagnosis methods are finally resulted. More relevant BN reviews include BN application in reliability (Insua et al., 2020 ) and safety and risk assessments (Kabir & Papadopoulos, 2019 ).
The integration of FMEA, RCA, and FTA holds immense potential for quality and production managers to minimize failures and enhance system efficiency. By capitalizing on the unique strengths of each approach, the integration of these failure analysis methodologies enables a more comprehensive and effective examination of failures. However, existing studies and literature reviews have predominantly focused on individual methodologies, leading to a lack of integration and limited familiarity with three approaches among engineers and industry experts. To address this gap and promote the integration of them, this study aims to review the progress of intelligence failure analysis within FMEA, RCA, and FTA.
Other general failure analysis methodologies include, but are not limited to, the following methodologies. Event Tree Analysis, similar to FTA, is a graphical representation that models the progression of events following an initiating event, helping to analyze the potential consequences (Ruijters & Stoelinga, 2015 ). Bow-Tie Analysis, usually used in risk management, visualizes the relationship between different potential causes of a hazard and their possible consequences (Khakzad et al., 2012 ). Human Reliability Analysis focuses on assessing the probability of human error and its potential impact on systems and processes (French et al., 2011 ). The Fishbone Diagram visually represents potential causes of a problem to identify root causes by categorizing them into specific factors like people, process, equipment, materials, etc.
There are also industry-specific methodologies, including but not limited to the following ones. Electrostatic Discharge (ESD) Failure Analysis focuses on identifying failures caused by electrostatic discharge, a common concern in the electronics industry. Hazard and Operability Study is widely used in the chemical industry to examine deviations from the design intent and identify potential hazards and operability issues. Incident Response and Post-Incident Analysis, in the IT industry, is used for analyzing and responding to security incidents, with a focus on preventing future occurrences. Hazard Analysis and Critical Control Points is a systematic preventive approach to food safety that identifies, evaluates, and controls hazards throughout the production process. Maximum credible accident analysis assesses and mitigates the most severe accidents that could occur in high-risk industries. For more information on industry-specific methodologies, an interested reader may consult the paper on that industry, as they are wide and out of the scope of this paper for deep discussion.
Our review focuses on the historical progress of (hybrid) intelligence failure analysis to identify and classify methodologies and tools used within them. In Industry 4.0, (hybrid) intelligence failure analysis can contribute to improve quality management and automate quality through an improved human cyber-physical experience. Different from the abovementioned reviews, the purpose of our study is to provide a rich comprehensive understanding of the recent developments in these methodologies from industry 4.0 and hybrid intelligence, the benefits of making them intelligent, i.e., (augmented) automatic and/or data-driven, and their limitations.
Research methodology
A systematic literature review analyses a particular knowledge domain’s body of literature to provide insights into research and practice and identify research gaps (Thomé et al., 2016 ). This section discusses our review scope and protocols, defining both our primary and secondary questions, and the criteria for selecting journals and papers to be reviewed. A bibliography analysis of the selected papers is also presented, including distributions by year, affiliation, and journals.
Review scope and protocol
We follow Thomé et al. ( 2016 ) 8-step literature review methodology to assure a rigorous literature review of intelligence, automated/data-driven, failure analysis methodology for Industry 4.0.
In Step 1, our (hybrid) intelligence failure analysis problem is planned and formulated by identifying the needs, scope, and questions for this research. Our initial need for this literature review comes from a relevant industrial project entitled "assembly quality management using system intelligence" which aims to reduce the quality failures in assembly lines. The trend towards automated and data-driven methodologies in recent years signifies the need for this systematic literature review. Thus, three general failure analysis methodologies, FMEA, RCA, and FTA, are reviewed with respect to tools to make them intelligent and to derive benefits from hybrid intelligence.
Our primary questions are as follows. (i) What are the failure analysis general methodologies and what tools have been used to make them intelligent? (ii) How these methodologies may benefit from hybrid intelligence? (iii) What are the strengths and weaknesses of these methodologies and tools? Our secondary questions are as follows. (i) How intelligent are these tools? (ii) What types of data do they use? Which tools allow a good fusion of human and machine intelligence? (iii) How well do they identify the root causes of failures? (iv) What are the possible future prospectives?
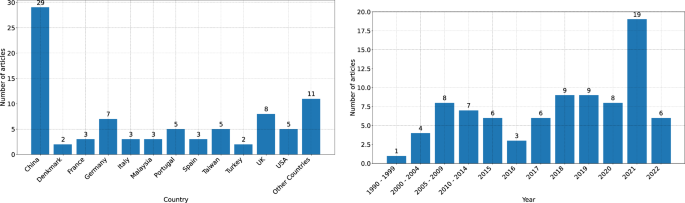
Distribution of papers by year and affliation
Step 2 concerns searching the literature by selecting relevant journals, databases, keywords, and criteria to include or exclude papers. We select the SCOPUS database to scan the relevant paper from 1990 to the first half of 2022. SCOPUS contains all high-quality English publications and covers other databases such as ScienceDirect and IEEE Xplore. A two-level keyword structure is used. The first level retrieves all papers that have either failure mode and effect analysis, FMEA, failure mode and effects and criticality analysis, FMECA, fault tree analysis, FTA, event tree analysis, ETA, root cause analysis, RCA, failure identification, failure analysis, or fault diagnosis in the title, abstract, and/or keywords. The second level limits the retrieved paper by the first level keywords to papers that have either Bayesian network, BN, automated, automatic, automation, smart, intelligence or data-driven in the title, abstract, and/or keywords.
To ensure the scientific rigor of our literature review process, we have removed papers that met at least one of the following criteria: Publications with concise and/or ambiguous information that would make it impossible to re-implement the tools and methodologies described in the paper later on. Publications in low-level journals, i.e., journals in the third quarter (Q3) or lower in the Scimago Journal & Country Rank. Papers with subject areas that are irrelevant to our research topic, such as physics and astronomy.
Steps 3 and 4 involve gathering data and evaluating data quality. We download papers and check their sources according to exclusion criteria. Step 5 concerns data analysis. Step 6 focuses on interpreting the data. The final selected papers are analyzed and interpreted in Section Managerial insights, limitations, and future research . Step 7 involves preparing the results and report. Step 8 requires the review to be updated continuously.
Discussion and statistical analysis
Here is a bibliometric analysis of our literature review. About 15,977 papers were found in our first search. By excluding criteria, we shortened the search to 7113. Then, we checked the titles of 7113 papers including 4359 conference and 2754 journal papers. We downloaded 1,203 papers to read their abstracts and skim their bodies. Then, 1114 low-quality/irrelevant papers were excluded. The remaining 86 high-quality papers were examined for this study.
Distributions of papers by year and affiliation are shown in Fig. 1 . 28 countries have contributed in total. Most affiliations are in advanced countries including China, Germany, and the UK. Surprisingly, we found no publications from Japan and only five from the USA. Only one papers had been published between 1990 and 1999 because of limited data and technology, e.g., sensors and industrial cameras. A slow growth observed between 2000 and 2014 coincides with the technology advancement and Industry 4.0 emergence. The advanced technology and researchers focus on Industry 4.0 have led to significant growth every year since 2015. Worth to note that 2022 information is incomplete because this research has been conducted in the middle of 2022. We expect more publications, at least equal to 2021, for 2022.
Papers distribution by journal is in Fig. 2 . 58 journals and conferences have contributed. Journals with a focus on production and quality, e.g., International Journal of Production Research , have published most papers. Technology-focused journals, e.g., IEEE Access , also have contributed.
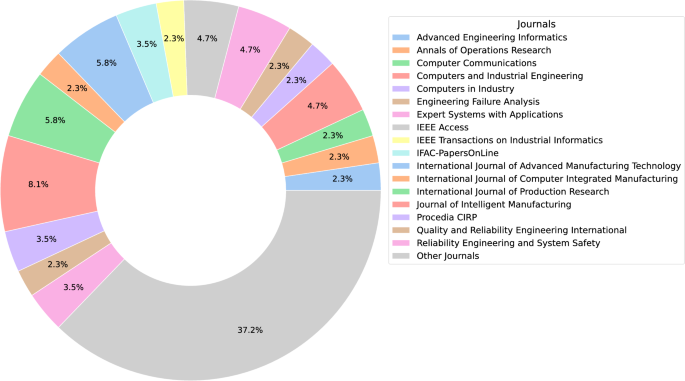
Distribution of papers by journal
Literature categorization
Selected papers are now categorized based on the four general steps of a failure analysis methodology, involving failure structure detection, failure event probabilities detection, failure risk analysis, and outputs. Then, a statistical analysis of these categorizations is provided.
These four steps of a failure analysis methodology are illustrated in Fig. 3 . The first two steps deal with input data. In step 1, the failure structure is identified, encompassing all (possible) failures, the failure propagation structure, failure interdependency, and causes and effects. Step 2 involves detecting event probabilities in a failure structure. For example, classical FMEA scores each failure with severity, occurrence, and detection rates.
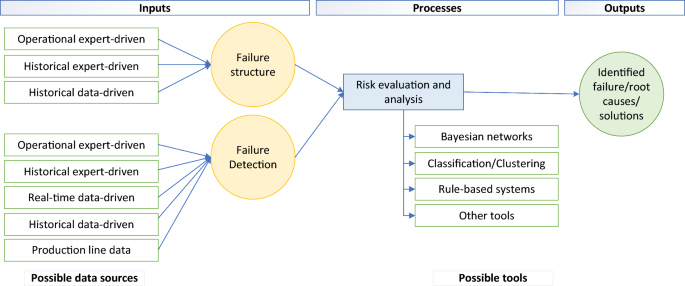
Four general steps of a failure analysis methodology
To analyze failures in a (production) system, data should be collected to identify the failure structure and detect failures. Reactive methodologies, such as RCA, are data-driven and typically gather available data in a system, while proactive methodologies, such as FMEA, are expert-driven and gather data through expert knowledge. However, a (hybrid) intelligence failure analysis methodology should take advantage of both advanced technologies, such as sensors and Internet-enabled machines and tools, and experts to automatically gather required data, combining proactive and reactive approaches, and providing highly reliable analyses and solutions.
In step 3, all input data are processed to determine the associated risk value with each failure, and the most probable causes (usually based on an observed or potential effect). Typically, a main tool, such as Bayesian networks, neural rule-based systems, statistical analysis, or expert analysis, is used to determine root causes, classify failures, and/or rank failures.
Step 4 outputs results that may include failures and sources, reasons behind the sources, and mitigation actions. The output of this tool is post-processed to provide possible solutions and information that is explainable and easy to use for both humans and machines.
Steps 1: failure structure
Failure structure identification is the first step in a failure analysis methodology. (Potential) failures, causes, effects, and/or failure interdependency are identified. We categorize the literature to develop a (hybrid) intelligence failure methodology to identify failure structure, causes, effects, interdependencies, and relationships between failures, failures and causes, and failures and effects.
Traditionally, experts have defined failure structures by analyzing causes, effects, and the interdependency of failures. However, recent studies have explored alternative approaches to identifying failure structures, leveraging available data sources such as problem-solving databases, design forms, and process descriptions. Problem-solving databases include quality issue records, maintenance records, failure analysis records, and CBR databases. These records could be stored in structured databases and sheets, or unstructured texts. Design forms may include design FMEA forms, reliability characteristics, and product quality characteristics. Process descriptions may include operations, stations, and key operational characteristics. Moreover, simulation can be used to generate failures, causes, and effects (Snooke & Price, 2012 ). Design forms and process descriptions are generated by experts, usually for other purposes, and are re-used for failure analysis. Problem-solving databases could be generated by experts, such as previous FMEAs, or by an automated failure analysis methodology, such as automated RCA. Table 1 classifies studies based on the data sources used to identify the failure structure.
Data processing methods
To define failure structure from operational expert-driven data, no specific tool has been used. In the industry, failure structures are typically defined by an expert (or group of experts). When expert-driven or data-driven historical data and/or design forms and process descriptions are available, ontology-driven algorithms, including heuristics (Sayed & Lohse, 2014 ; Zhou et al., 2015 ; Steenwinckel et al., 2018 ; Xu & Dang, 2023 ) and SysML modeling language (Hecht & Baum, 2019 ), process/system decomposition (the operation, the station, and the key characteristics levels) (Zuo et al., 2016 ; Khorshidi et al., 2015 ; Zhou et al., 2015 ), rule-based algorithms that use CBR (Yang et al., 2018 ; Liu & Ke, 2007 ; Xu & Dang, 2023 ; Oliveira et al., 2022 , 2021 ), and FTA/BN modeling from FMEA/expert data (Yang et al., 2022 ; Steenwinckel et al., 2018 ; Palluat et al., 2006 ) and from Perti net (Yang & Liu, 1998 ) have been suggested. Rivera Torres et al. ( 2018 ) divided a system into components and related failures to each of the components to make a tree of components and failures.
Component-failure matrix is generated using unstructured and quality problem texts mining from historical documents such as bills of material and failure analysis. Apriori algorithms were used to find synonyms in the set of failure modes (Xu et al., 2020 ). The 8D method is used to describe a failure. Ontology was used to store and retrieve data in a knowledge base CBR system.
Yang et al. ( 2022 ), Leu and Chang ( 2013 ) and Waghen and Ouali ( 2021 ) have suggested building a BN structure from the FTA model. Wang et al. ( 2018 ) has proposed to use the fault feature diagram, the fault-labeled transition system based on the Kripke structure to describe the system behavior. The MASON (manufacturing semantic ontology) has been used to construct the structure of the failure class by Psarommatis and Kiritsis ( 2022 ). Teoh and Case ( 2005 ) has developed a functional diagram to construct a failure structure between components of a system and to identify causes and effect propagation. Yang et al. ( 2018 ) used an FMEA style CBR to collect failures to search for similarity. They then used CBR to build a BN using a heuristic algorithm.
Step 2: failure detection
Failure detection data are gathered to determine the strength of relationships among failures, causes, and effects.
Failure detection can be based on operational or historical expert-driven data, as well as data-driven historical and/or real-time data obtained from sensors. Such data can come from a variety of sources, including design and control parameters (such as machine age or workpiece geometry), state variables (such as power demand), performance criteria (such as process time or acoustic emission), and internal/external influencing factors (such as environmental conditions) (Filz et al., 2021b ; Dey & Stori, 2005 ). These data are usually used to determine occurrence probability of failures. To determine the severity and detection probabilities of failures, conditional severity utility data/tables may be used (Lee, 2001 ). Simulation can also be used to determine occurrence, severity, and detection (Price & Taylor, 2002 ). Table 2 summarizes types of data that are usually used to detect failures in the literature.
Processing data refers to the transformation of raw data into meaningful information. A data processing tool is needed that provides accurate and complete information about the system and relationships between data and potential failures.
First, data from different sources should be pre-processed. In a data pre-processing step, data is cleaned, edited, reduced, or wrangled to ensure or enhance performance, such as replacing a missing value with the mean value of the entire column (Filz et al., 2021b ; Schuh et al., 2021 ; Zhang et al., 2023 ; Musumeci et al., 2020 ; Jiao et al., 2020 ; Yang et al., 2015 ; Chien et al., 2017 ).
Data then may need to be processed according to the tools used in Step 3. Common data processing methods between all tools include data normalization using the min-max method (Filz et al., 2021b ; Musumeci et al., 2020 ) and other methods (Yang et al., 2018 ; Schuh et al., 2021 ; Jiao et al., 2020 ; Sariyer et al., 2021 ; Chien et al., 2017 ).
Feature selection/extraction algorithms have been used to select the most important features of data (Filz et al., 2021b ; Xu & Dang, 2020 ; Mazzoleni et al., 2017 ; Duan et al., 2020 ; Schuh et al., 2021 ; Zhang et al., 2023 ; Musumeci et al., 2020 ; Yang et al., 2015 ; Sariyer et al., 2021 ).
For BN-based failure analysis, maximum entropy theory is proposed to calculate failure probabilities from expert-based data (Rastayesh et al., 2019 ). Fuzzy methods have also been used to convert linguistic terms to occurrence probabilities (Yucesan et al., 2021 ; Wan et al., 2019 ; Nie et al., 2019 ; Nepal & Yadav, 2015 ; Ma & Wu, 2020 ; Li et al., 2013 ; Duan et al., 2020 ). Euclidean distance-based similarity measure (Chang et al., 2015 ) and fuzzy rule base RPN model (Tay et al., 2015 ), heuristic algorithms (Brahim et al., 2019 ; Dey & Stori, 2005 ; Yang et al., 2022 ), and a fuzzy probability function (Khorshidi et al., 2015 ) have been suggested to build failure probabilities.
Failure analysis data may be incomplete, inaccurate, imprecise, and limited. Therefore, several studies have used tools to deal with uncertainty in data. The most commonly used methods are fuzzy FMEA (Yang et al., 2022 ; Nepal & Yadav, 2015 ; Ma & Wu, 2020 ), fuzzy BN (Yucesan et al., 2021 ; Wan et al., 2019 ; Nie et al., 2019 ), fuzzy MCDM (Yucesan et al., 2021 ; Nie et al., 2019 ; Nepal & Yadav, 2015 ), fuzzy neural network (Tay et al., 2015 ; Palluat et al., 2006 ), and fuzzy evidential reasoning and Petri nets (Shi et al., 2020 ).
Step 3: analysis
A failure analysis tool is essential for conducting any failure analysis. Table 3 categorizes various data-driven tools, such as BNs, Clustering/Classification, Rule-based Reasoning, and other tools used in the literature and the aspects they support.
BNs model probabilistic relationships among failure causes, modes, and effects using directed acyclic graphs and conditional probabilities. Pieces of evidence, i.e., known variables, are propagated through the graph to evaluate unobserved variables (Cai et al., 2017 ). For example, Rastayesh et al. ( 2019 ) applied BNs for FMEA and perform risk analysis of a Proton Exchange Membrane Fuel Cell. Various elements and levels of the system were identified along with possible routes of failure, including failure causes, modes, and effects. A BN was constructed to perform the failure analysis. Some other examples of the BNs application include an assembly system (Sayed & Lohse, 2014 ), kitchen equipment manufacturing (Yucesan et al., 2021 ), and Auxiliary Power Unit (APU) fault isolation (Yang et al., 2015 ).
Classification assigns predefined labels to input data based on learned patterns, Clustering organizes data into groups based on similarities. Neural networks are commonly used for failure classification and have been employed in most studies. Hence, we separated these studies from those that used other clustering/classification tools. Neural networks consist of layers of interconnected nodes, with an input layer receiving data, one or more hidden layers for processing, and an output layer providing the final classification (Jiang et al., 2024 ). For example, Ma and Wu ( 2020 ) applied neural networks to assess the quality of 311 apartments in Shanghai, China, for FMEA. The input includes various APIs collected for the apartments, and the output was the risk rate of each apartment. In another study, Ma et al. ( 2021 ) applied neural networks for RCA to predict the root causes of multiple quality problems in an automobile factory. Some other examples of the neural networks application include industrial valve manufacturing (Pang et al., 2021 ), complex cyber–physical systems (Liu et al., 2021 ), and an electronic module designed for use in a medical device (Psarommatis & Kiritsis, 2022 ).
Other clustering/classification tools include evolving tree (Chang et al., 2015 ), reinforced concrete columns (Mangalathu et al., 2020 ), K-means, random forest algorithms (Xu & Dang, 2020 ; Chien et al., 2017 ; Oliveira et al., 2022 , 2021 ), contrasting clusters (Zhang et al., 2023 ), K-nearest neighbors (Ma et al., 2021 ), self-organizing maps (Gómez-Andrades et al., 2015 ), and Naive Bayes (Schuh et al., 2021 ; Yang et al., 2015 ).
Rule-based reasoning represents knowledge in the form of "if-then" rules. Rule-based reasoning involves a knowledge base containing the rules and a reasoning engine that applies these rules to incoming data or situations. For instance, Jacobo et al. ( 2007 ) utilized rule-based reasoning for analyzing failures in mechanical components. This approach serves as a knowledgeable assistant, offering guidance to less experienced users with foundational knowledge in materials science and related engineering fields throughout the failure analysis process. Also, the application of the rule-based reasoning for wind turbines FMEA is studied by (Zhou et al., 2015 ).
Other tools include gradient-boosted trees, logistic regression (Filz et al., 2021b ), CBR (Tönnes, 2018 ; Camarillo et al., 2018 ; Jacobo et al., 2007 ), analyzing sensitivities of the machining operation by the stream of variations and errors probability distribution determination (Zuo et al., 2016 ), causal reasoning (Teoh & Case, 2005 ), probabilistic Boolean networks with interventions (Rivera Torres et al., 2018 ), principal component analysis (PCA) (Duan et al., 2020 ; Zhang et al., 2023 ; Jiao et al., 2020 ; Sun et al., 2021 ), factor ranking algorithms (Oliveira et al., 2022 , 2021 ), heuristics and/or new frameworks (Camarillo et al., 2018 ; Yang et al., 2009 , 2020 ; Snooke & Price, 2012 ; Xu & Dang, 2023 ; Rokach & Hutter, 2012 ; Wang et al., 2018 ; Hecht & Baum, 2019 ; Yang & Liu, 1998 ; Liu & Ke, 2007 ), and mathematical optimization methods (Khorshidi et al., 2015 ).
These tools may be integrated by other tools including sequential state switching and artificial anomaly association in a neural network (Liu et al., 2021 ), MCDM/optimization (Yucesan et al., 2021 ; Jomthanachai et al., 2021 ; Ma et al., 2021 ; Sun et al., 2021 ), game theory (Mangalathu et al., 2020 ), fuzzy evidential reasoning and Petri nets (Shi et al., 2020 ), and maximum spanning tree, conditional Granger causality, and multivariate time series (Chen et al., 2018 ).
Step 4: output
A data analysis process can benefit not only humans but also machines and tools in a hybrid intelligence failure analysis methodology. Therefore, the output information should be carefully designed. Table 4 ranks the output data, and the list of studies for each output is available in Online Appendix EC.1. Most studies have focused on automatically identifying the root causes of failures, which is the primary objective of a failure analysis methodology. In addition, researchers have also focused on failure occurrence rating, ranking, and classification. While automatically finding the root causes of failures is important, a hybrid intelligence failure analysis process needs to interpret the related data and information and automatically provide mitigation actions for both operators and machines. However, only a few studies have proposed tools to automatically find possible mitigation actions, usually based on CBR databases and only readable for humans. Therefore, future studies may focus on finding possible automated mitigation actions for failures and developing a quality inspection strategy.
Data post-processing
A data post-processing step transforms data from the main tool into readable, actionable, and useful information for both humans and machines. Adapting solutions from similar failures in a database (i.e., CBR) to propose a solution for a detected failure has been proposed by Tönnes ( 2018 ), Camarillo et al. ( 2018 ), Hecht and Baum ( 2019 ), Jacobo et al. ( 2007 ), Liu and Ke ( 2007 ) and Ma et al. ( 2021 ). Simulation to analyze different scenarios (Psarommatis & Kiritsis, 2022 ; Jomthanachai et al., 2021 ; Chien et al., 2017 ; Oliveira et al., 2022 ), mathematical optimization model (Khorshidi et al., 2015 ; Ma et al., 2021 ) and self-organizing map (SOM) neural network (Chang et al., 2017 ) to automatically select the best corrective action have also been proposed. Also, fuzzy rule-based systems to obtain RPN (Nepal & Yadav, 2015 ) and visualisation (Xu & Dang, 2020 ; Yang et al., 2009 ) are discussed.
The statistical analysis of the paper reveals that most FMEA-based studies rely solely on expert-based information to construct failure structures, while RCA-based papers tend to use a hybrid of problem-solving and system-related data. This is depicted in Fig. 4 , which shows the distribution of papers by data used over time. FMEA is used to identify potential failures when there is not enough data available to construct a failure structure based on system-based data. The trend shows some effort to use data, instead of expert knowledge, to construct failure structures, using data from similar products/processes. RCA and FTA are a reactive methodology that analyzes more information than FMEA. Advances in data mining techniques, along with increased data availability, have led to a growing trend of using data to construct failure structures. For a comprehensive and reliable intelligence failure analysis, a combination of all kinds of data is necessary. It is worth noting that Waghen and Ouali ( 2021 ) proposed a heuristic method to augment failure structure identification that uses expert and historical data. They suggested engaging expert knowledge when historical data are insufficient to identify a failure structure and/or the reliability of a failure structure is low. Other studies have solely focused on failure identification through expert knowledge or historical data, without considering the potential benefits of combining different types of data.
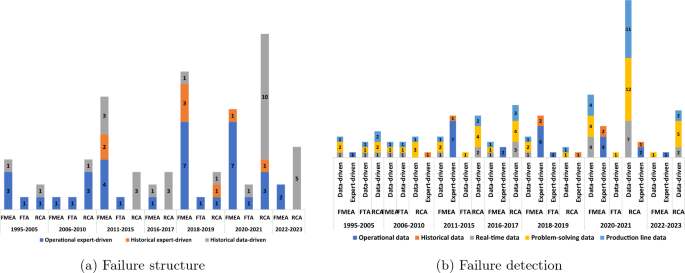
Input data statistical analysis
While most FMEA-based papers use only expert-based data to determine failure probability, there is a significant growth in the utilization of problem-solving data and a hybrid of problem-solving and system-related data, i.e., production line data, over time. RCA and FTA usually tend to use more problem-solving and system-related data. Moreover, this figure and Fig. 5 show that the literature on RCA has been growing in recent years, while the trend for FMEA has remained the same over time. We found that Filz et al. ( 2021b ), Mazzoleni et al. ( 2017 ), Ma and Wu ( 2020 ) and Yang et al. ( 2015 ) improved FMEA to use a combination of expert-based, problem-solving, and system-related data to determine potential failures and their causes. They analyzed these data using deep learning, classification, and neural networks, respectively. Duan et al. ( 2020 ), Ma et al. ( 2021 ) tried to use the benefits of both expert-based data and problem-solving and system-related data in the RCA context. They analyzed the root cause of failures using neural networks.
The distribution of papers by the tools used is shown in Fig. 5 . BNs have been mainly used within the context of FMEA methodologies with a growing trend during the recent years, while RCA researchers have used them less frequently. BNs have the potential to model failure propagation, multi-failure scenarios, and solution analysis to propose potential solutions. However, all of the studies reviewed in this paper only used BNs to identify the root causes of failures. BNs offer a clear graphical representation of failures, their causes, and their effects, which facilitates the interpretation of results by humans. They also provide an easy way for humans to intervene and analyze the sensitivity of results and correct processed data if it appears unrealistic. BNs are well-developed tool and have the ability to work with expert-based, historical, and system-based data, even when data is fuzzy or limited. Developing methodologies that leverage the advantages of BNs seems promising for FMEA, RCA, and FTA.
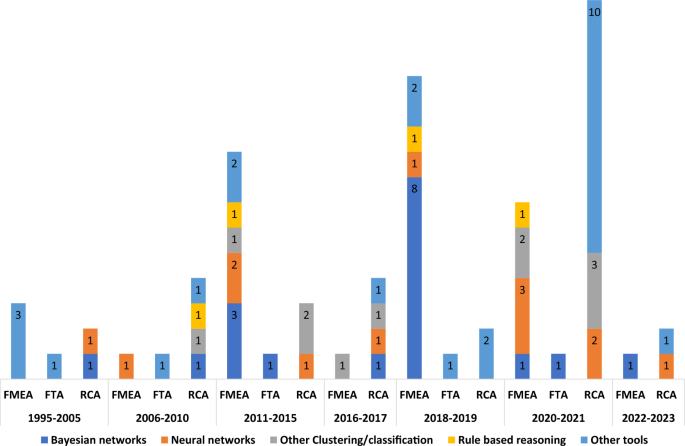
Tools distribution statistical analysis
RCA and FTA are reliant on various tools over time with no trend of using a specific tool, such as PCA and regression, due to their need for a large amount of data. However, these methods have limitations in incorporating both human and machine intelligence and mostly rely on machine intelligence. Although neural networks and classification algorithms have gained attention in both FMEA and RCA during the last few years, they are black boxes and difficult for humans to modify. Also, classification algorithms typically do not address failure propagation or multi-failure modes. BNs offer a promising alternative, as they can model failure propagation, multiple-failures, and provide a clear graphical representation of failures, causes, and effects. Furthermore, BNs can incorporate both expert-based and historical data, making them well-suited for FMEA, RCA, and FTA. Therefore, developing methodologies that fully leverage the benefits of BNs in these domains would be valuable.
Managerial insights, limitations, and future research
In this section, we discuss managerial insights, limitations, and future research related to different aspects of a Hybrid Intelligence failure analysis methodology. The aim is to assist researchers in focusing on relevant recommendations. Section Section Applications and complexity delves into the applications and complexity of each study, and provides examples for each tool. Section Levels of automation/intelligence presents the levels of intelligence for a failure analysis methodology. Section Introducing knowledge into tools discusses how knowledge is introduced into the failure analysis tools for an effective failure analysis. A more in-depth discussion of hybrid intelligence is in Section Hybrid intelligence . The last three sections provide insights into failure propagation and correlation, hybrid methodologies, and other areas of future research.
Applications and complexity
Intelligent FMEA, RCA, and FTA have been applied to various applications, including production quality management, computer systems, reliability and safety, chemical systems, and others. Table 5 presents the distribution of reviewed papers by application. The list of studies per application is available in Online Appendix EC.2. Production quality management has been the most common application of intelligent failure analysis methodologies due to the significant costs associated with quality assurance. Smart failure analysis methodologies have also been impacted by the increased use of sensors and IoT to collect precise data from machines, tools, operators, and stations, as well as powerful computers to analyze the data. Computer systems failure analysis and system reliability and safety rank second, while chemical systems rank third, as these systems often require specific methodologies, such as hazard and operability analysis.
We checked every paper dataset to find information about the complexity of their case-study and reasons behind their good results to help readers select a validated study on a large set of data. An enriched dataset of problem-solving data are used by Xu et al. ( 2020 ), Du et al. ( 2012 ), Oliveira et al. ( 2021 ), Gómez-Andrades et al. ( 2015 ), Leu and Chang ( 2013 ), Price and Taylor ( 2002 ), Sariyer et al. ( 2021 ), Gomez-Andrades et al. ( 2016 ) and Xu and Dang ( 2023 ). An enriched dataset of historical problem-solving and sensors data is used by
Filz et al. ( 2021b ), Sun et al. ( 2021 ), Mazzoleni et al. ( 2017 ), Hireche et al. ( 2018 ), Yanget al. ( 2015 ) Demirbaga et al. ( 2021 ), Waghen and Ouali ( 2021 ), Zhang et al. ( 2023 ), Oliveira et al. ( 2022 ), Sun et al. ( 2021 ). Data from the system and processes are used by Teoh and Case ( 2005 ), Ma et al. ( 2021 ), Schuh et al. ( 2021 ), Waghen and Ouali ( 2021 ). Other studies demonstrated their methodology on a small problem.
Levels of automation/intelligence
Failure analysis intelligence can be divided into five levels based on the data used. Level 1 involves analyzing failures using expert-based data with the use of intelligence tools. This level can be further improved by incorporating fuzzy-based tools, such as fuzzy BNs, fuzzy neural networks, and fuzzy rule-based systems. If the amount of historical data can be increased over time, we suggest using BNs in a heuristic-based algorithm, as they have the capability to work with all possible data, resulting in fewer modifications in the failure analysis methodology over time. Good examples for Level 1 include Yucesan et al. ( 2021 ) and Brahim et al. ( 2019 ).
Level 2 involves analyzing failures using experts to identify failure structures and problem-solving and system-related data to determine failure probabilities. This level can be used by a professional team who can correctly and completely identify failure structure. It can also be used by those who work with variable structures where updating the structure requires a lot of data modification. Identifying failure structures and analyzing failures are both automated at level 3. This level is the most applicable when a good amount of data is available. BNs, classification algorithms, and neural networks are among the best tools to analyze failure within RCA, FMEA, and FTA methodologies. Studies such as Filz et al. ( 2021b ) Zuo et al. ( 2016 ), Dey and Stori ( 2005 ), Mangalathu et al. ( 2020 ), Yang et al. ( 2015 ) and Ma et al. ( 2021 ) are good examples for Levels 2 and 3.
In level 4, mitigation actions are also determined automatically. This level represents a whole automation of failure analysis. BNs are among the few tools that can encompass all steps of failure analysis. As such, we suggest using them. CBR databases can be used by BNs plus system-based data to provide possible corrective actions. Tönnes ( 2018 ), Zuo et al. ( 2016 ) and Hecht and Baum ( 2019 ) are among good studies for Level 4. Chang et al. ( 2017 ) has focused to automate and visualize corrective actions using a self-organizing map (SOM) neural network in an FMEA methodology. Future research should concentrate on the development of an automated FMEA that dynamically updates the current RPN (Risk Priority Number). This can aid in predicting failures in parts or components of a system using a "Live RPN." The predictive capability of such a tool can be utilized to optimize the overall system. It enables the transformation of a manufacturing system into a self-controlling system, allowing adjustments based on current parameters (Filz et al., 2021b ).
Level 5 is a hybrid intelligence approach to failure analysis that encompasses all other levels and can be implemented within FMEA, RCA, and FTA methodologies when a limited amount of historical and system-based data is available until a comprehensive CBR database is built. BNs provide a good graphical representation and can work with all possible data types. The advantages of BNs are significant enough to be suggested for hybrid intelligence failure analysis. However, we did not find any comprehensive study for this level. A combination of studies that proposed methods to use integrated expert-based, problem-solving, and system-based data, such as Waghen and Ouali ( 2021 ); Filz et al. ( 2021b ), is suggested. Nonetheless, this level remains open and needs to be the focus of future research by scholars. To facilitate the implementation of hybrid intelligence failure analysis, a user-friendly interface is crucial for operators to interact with. Several studies have proposed user-interface applications for this purpose, including (Chan & McNaught, 2008 ; Camarillo et al., 2018 ; Li et al., 2013 ; Jacobo et al., 2007 ; Yang et al., 2009 , 2020 ; Demirbaga et al., 2021 ; Snooke & Price, 2012 ; Palluat et al., 2006 ).
Introducing knowledge into tools
In this section, we analyze which types of knowledge, expert-driven, data-driven, or a hybrid of both, are usually used with which tools and what the implications are for providing insights on suitable tools for hybrid intelligence failure analyses.
Figure 6 shows the distribution of literature based on the input data, tools, and outputs (four general steps of a failure analysis methodology in Fig. 3 ). The first column of nodes shows various combinations of types of knowledge, expert-driven, data-driven, or a hybrid of both, that are usually used in the literature to identify the structure of failure and to detect the probability of failures. The second column of nodes shows various tools that are used to analyze the failure. The third column of nodes shows outputs of a failure analysis. The number of studies with each particular focus is shown by the thickness of an arrow. Details are in Appendix EC.1.
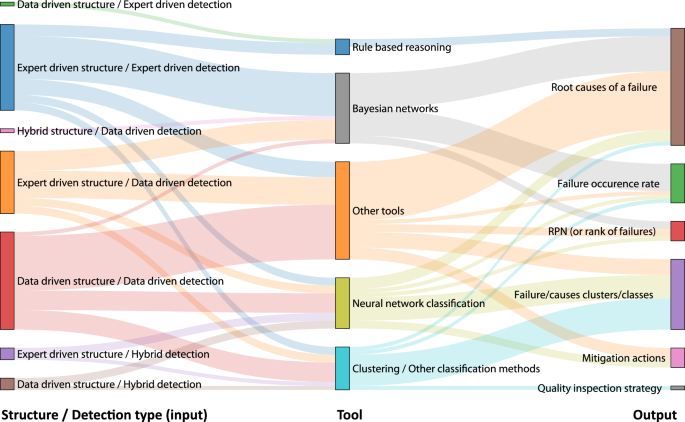
Literature distribution based on inputs, tools, and outputs
The following studies have tried to introduce knowledge and data from expert and data based sources to a failure analysis methodology. Filz et al. ( 2021b ) utilized expert knowledge to identify the structure of failure, the components involved, and the necessary sensors to be used. They then employed sensors to capture data and leveraged problem-solving data from the recorded expert archive to identify failures in a deep learning model. Similarly, Musumeci et al. ( 2020 ) used supervised algorithms to classify failures. Mazzoleni et al. ( 2017 ) they used data from sensors to select the most effective features related to a failure, and subsequently employed sensor data and failure expert data-sets within a gradient boosting tree algorithm to identify the possibility of the failure. Duan et al. ( 2020 ) used data from different sources in a similar way for a neural network to identify the root cause of a failure. Ma and Wu ( 2020 ) utilized expert knowledge to identify failures in construction projects. Subsequently, expert datasets were employed in conjunction with project performance indices to predict the possibility of a failure and determine the root cause of the failure using a neural network tool.
Hireche et al. ( 2018 ), Yang et al. ( 2015 ) gathered data from sensors to determine the conditions of each failure/component node. Then, a BN was used to identify the risks and causes. A multi-level tree is developed by Waghen and Ouali ( 2021 ). Each level contains a solution, pattern, and condition level. Solutions are retrieved from a historical failure database as a combination of certain patterns. The pattern in each problem has been identified and related to the solution using a supervised machine-learning tool. Each level is linked to the next level until the root cause of a failure is correctly identified.
Other usefull tips for introducing knowledge from different sources to a failure analysis methodology can be found in the following studies. Zuo et al. ( 2016 ) divided a multi-operation machining process operation, station, and key characteristics levels. Stream of variations (SoV) was used to evaluate the sensitivities of the machining operations level by level. Results were used to find the sources affecting the quality. Distribution techniques for each quality precision using multi-objective optimization were chosen. Dey and Stori ( 2005 ) used a message-passing method (Pearl, 1988 ) to update a BN using data from sensors to estimate the condition of the system and update the CPTs, when each sensor output is considered as a node in the BN. Chan and McNaught ( 2008 ) also used sensor data to change the probabilities in a BN. A user interface is also developed to make inferences and present the results to operators.
Rokach and Hutter ( 2012 ) used the sequence of machines and a commonality graph of steps and failure causes data to cluster failures to find commonalities between them. A GO methodology is used by Liu et al. ( 2019b ) to model the system and a heuristic is used to construct BN structure and probabilities from the GO methodology model. Teoh and Case ( 2005 ) developed an objective-oriented framework that considers conceptual design information. A hierarchy of components, an assembly tree, and a functional diagram are built to capture data from processes and feed it to FMEA. Bhardwaj et al. ( 2022 ) used historical data from a similar system to estimate failure detection probabilities. Hecht and Baum ( 2019 ) used SysML to describe components and failures.
Zhou et al. ( 2015 ) used a tree of a system. Two classes of knowledge, shallow knowledge and deep knowledge, were gathered to generate rules for failure analysis. The former indicates the experiential knowledge of domain experts, and the latter is the knowledge about the structure and basic principle of the diagnosis system. Liu and Ke ( 2007 ) used CBR to find similar problems and solutions, text mining to find key concepts of the failure in the historical failure record texts, and rule mining to find hidden patterns among system features and failures. Filz et al. ( 2021a ) gathered process parameters after each station using a quality check station. Then a self-organizing Map was used to find failure propagation and cause and effect. Ma et al. ( 2021 ) used data from the system to determine features of problems, products, and operators. Data from problem-solving databases was used to find new failures and classified them using the features and historical data.
Psarommatis and Kiritsis ( 2022 ) developed a methodology that uses data-driven and knowledge-based approaches, an ontology base on the MASON ontology to describe the production domain and enrich the available data. Wang et al. ( 2018 ) developed a data acquisition system including a monitor, sensor, and filter modules. A fault diagram models failure propagation. They extended the Kripke structure by proposing the feature-labeled transition system, which is used to distinguish the behavior of the transition relationship by adding a signature to the transition relationship.
This section highlights that in the realm of failure analysis, a majority of research papers have utilized a hybrid approach, combining expert and data knowledge for tasks such as failure detection, classification, and feature selection. However, to achieve real-time failure analysis, a more effective integration of these two sources is crucial. This integration should enable operators and engineers to provide timely input to the system and observe immediate results. Furthermore, only a limited number of studies have specifically focused on the identification of failure structures using either data or a hybrid of expert and data knowledge.
The use of BNs has emerged as a highly promising approach for achieving real-time input and structure identification in the field of failure analysis. By leveraging both expert knowledge and data sources, BNs have the capability to effectively incorporate expert knowledge as constraints within structure identification algorithms. Unlike traditional classification algorithms that are primarily designed for continuous data, BNs are versatile in handling both discrete and continuous data types. Moreover, BNs possess several strengths that make them particularly suitable for failure analysis. They excel at performing real-time inferences, engaging in counterfactual reasoning, and effectively managing confounding factors. Given these advantages, it is essential to allocate more attention to the application of BNs in hybrid intelligence failure analysis. This involves further exploration of their capabilities and conducting comparative analyses with other tools to assess their effectiveness in various scenarios. By focusing on BNs and conducting comprehensive evaluations, researchers can enhance the understanding and adoption of these powerful tools for improved failure analysis in real-time settings.
Hybrid intelligence
A collaborative failure analysis methodology is needed, in which artificial intelligence tools, machines, and humans can communicate. While hybrid intelligence has gained attention in various fields, literature on the subject for failure analysis is still limited. For example, Piller et al. ( 2022 ) discussed methods to enhance productivity in manufacturing using hybrid intelligence. They explored considerations such as task allocation between humans and machines and the degree of machine intelligence integrated into manufacturing processes. Petrescu and Krishen ( 2023 ) and references within have delved into the benefits and future directions of hybrid intelligence for marketing analytics. Mirbabaie et al. ( 2021 ) has reviewed challenges associated with hybrid intelligence, focusing particularly on conversational agents in hospital settings. Ye et al. ( 2022 ) developed a parallel cognition model. This model draws on both a psychological model and user behavioral data to adaptively learn an individual’s cognitive knowledge. Lee et al. ( 2020 ) combined a data-driven prediction model with a rule-based system to benefit from the combination of human and machine intelligence for personalized rehabilitation assessment.
An artificial intelligence tool should not only provide its final results but also provide its reasoning. A human can analyze the artificial intelligence tool reasoning through a user-interface application and correct possible mistakes instantly and effortlessly. To enable this capability, the use of a white-box artificial tool, such as Bayesian networks, is essential. Explainable AI aids in comprehending and trusting the decision-making process of the hybrid intelligence system by providing the reasoning behind it (Confalonieri et al., 2021 ). Moreover, a machine should be able to interpret and implement an artificial intelligence tool and/or human solutions. Artificial intelligence tools, machines, and humans can learn from mistakes (Correia et al., 2023 ).
To fully exploit the complementarity in human–machine collaborations and effectively utilize the strengths of both, it is important to recognize and understand their roles, limitations, and capabilities in the context of failure analysis. Future research should focus on developing a clear plan for their teamwork and joint actions, including determining the optimal sensor types and locations, quality inspection stations, and human/machine analysis processes. In other words, How to design a decision support system that integrates both human knowledge and machine intelligence with respect to quality management? should be answered. Additionally, tools should be developed to propose possible mitigation actions based on the unique characteristics of the system, environment, humans, and machines. To achieve this, system-related data along with CBR data can be analyzed to find potential mitigation actions.
A general framework for human–machine fusion could involve the following steps: identifying applicable human knowledge and machine data for the problem, determining machine intelligence tools that facilitate the integration of human–machine elements like BNs, identifying the suitable points in the decision-making process to combine human knowledge and machine intelligence effectively, designing the user interface, and incorporating online learning using input from human knowledge (Jarrahi et al., 2022 ). However, human–machine fusion is not an easy task due to the complexity of human–machine interaction, the need for effective and online methods to work with both human and machine data, and the challenge of online learning from human knowledge. For instance, while ChatGPT interacts well with humans, it currently does not update its knowledge using human knowledge input for future cases (Dellermann et al., 2019 ; Correia et al., 2023 ).
Failure propagation and correlation
Most FMEA papers concentrated on analyzing failures in individual products, processes, or machines. It is essential to acknowledge that production processes and machines are interconnected, leading to the correlation and propagation of failures among them. Consequently, it becomes crucial to address the challenge of analyzing failures in multiple machines. To effectively tackle this issue, a holistic approach is necessary. Rather than focusing solely on individual machines, take a broader perspective by considering the entire production system to identify the interdependencies and interactions among different machines, multiple processes, and within the system.
For an intelligence failure analysis, it is necessary to exploit detailed system-related data to carefully and comprehensively identify the relations between different parts of a system, product, and/or process. Some papers have suggested methods to identify failure propagation and correlation (Wang et al., 2021 ; Zhu et al., 2021 ; Chen et al., 2017 ). They usually proposed methods to analyze correlations only between failures or risk criteria using MCDM or statistical methods. However, an intelligence failure analysis should go beyond this and identify failure propagation and correlation among parts of a system.
In the literature, Chen and Jiao ( 2017 ) proposed finite state machine (FSM) theory to model the interactive behaviors between the components, constructing the transition process of fault propagation through the extraction of the state, input, output, and state function of the component. Zuo et al. ( 2016 ) used SoV to model propagation of variations from station to station and operation to operation. A propagation from one station (operation) to the next station (operation) was modeled using a regression like formula. Ament and Goch ( 2001 ) used quality check data after each station to train a neural network for failure progagation and estimate the relationships betweenfailure in stations using a regression model to find patterns in quality check data. Ma et al. ( 2021 ) used patterns in data to classify failures and identify causes.
To conduct an intelligence failure analysis, it is important to identify every part involved, their roles, characteristics, and states. The analysis should include the identification of failure propagation and effects on functions, parts, and other failures. One approach to analyzing failures is through simulation, which can help assess the changes in the characteristics of every part of a system, including humans, machines, and the environment. To analyze the complexity of failure propagation and mutual interactions among different parts of a system, data-driven tools and heuristic algorithms need to be developed. These tools should be capable of managing a large bill of materials and analyzing the failure structure beyond the traditional statistical and MCDM methods. Rule mining can be a useful tool for detecting failure correlation and propagation, especially in situations where there is limited data available, and human interpretation is crucial.
Hybrid methodologies
FMEA, RCA, and FTA methodologies are all complementary and can improve each other’s performance. Furthermore, the availability of data, advanced tools to process data, and the ability to gather online data may lead to a unified FMEA, RCA, and FTA methodology. The reason for this is that while FMEA tries to find potential failures, RCA and FTA try to find root causes of failures, they use similar data and tools to analyze data.
In the literature, FTA has been used as an internal part of FMEA by Steenwinckel et al. ( 2018 ), Palluat et al. ( 2006 )and RCA by Chen et al. ( 2018 ). Using automated mappings from FMEA data to a domain-specific ontology and rules derived from a constructed FTA, Steenwinckel et al. ( 2018 ) annotated and reasoned on sensor observations. Palluat et al. ( 2006 ) used FTA to illustrate the failure structure of a system within an FMEA methodology and developed a neuro-fuzzy network to analyze failures. Chen et al. ( 2018 ) used FTA and graph theory tools, such as the maximum spanning tree, to find the root cause of failures in an RCA methodology. However, studies on the integration of these methodologies regarding the availability of data, tools, and applications should be done to use their advantages within a unified methodology that detects potential failures, finds root causes and effects, and improves the system.
Other future research
Several promising future research directions can be pursued. Cost-based and economic quantification approaches can be integrated into intelligent methodologies to enable more informed decision-making related to failures, their effects, and corrective actions. Additionally, incorporating customer satisfaction criteria, such as using the Kano model, can be useful in situations where there are several costly failures in a system, and budget constraints make it necessary to select the most effective corrective action. This approach has been successfully applied in previous studies (Madzík & Kormanec, 2020 ), and can help optimize decision-making in complex failure scenarios.
Data management is a critical aspect of intelligence methodologies, given the large volume and diverse types of data that need to be processed. Therefore, it is important to design reliable databases that can store and retrieve all necessary data. Ontology can be a valuable tool to help integrate and connect different types of data (Rajpathak & De, 2016 ; Ebrahimipour et al., 2010 ). However, it is also essential to consider issues such as data obsolescence and updates, especially when corrective actions are taken and root causes are removed. Failure to address these issues can lead to incorrect analysis and decision-making.
Traditionally, only single failures were considered in analysis because analyzing a combination of multiple failures was impossible. However, in a system, two or more failures may occur simultaneously or sequentially. It is also possible that a failure occurs as a consequence of another failure. These circumstances are complicated because each failure can have several root causes, and another failure is only one of its causes. Therefore, a clear and powerful tool, such as Bayesian Networks (BNs), should be used to analyze failures and accurately identify possible causes.
The traditional failure analysis methodologies had limitations such as repeatability, subjectivity, and time consumption, which have been addressed by intelligence failure analysis. However, there is a need for more focus on explainability, objective evaluation criteria, and results reliability as some intelligent tools, such as neural networks, act as black boxes. Therefore, suitable tools, such as BNs, should be well-developed and adapted for (hybrid) intelligence failure analysis. Details such as the time and location of the detected failure, possible factors of the causes, such as location, time, conditions, and description of the cause, and reasons behind the causes, such as human fatigue, should be considered within a methodology. These can help to go beyond the CBR and propose intelligence solutions based on the reasons behind a cause. While RCA has implemented these data to a limited extent, FMEA lacks such implementation.
This paper has collected information on both proactive and reactive failure analysis methodologies from 86 papers that focus on FMEA, RCA, or FTA. The goal is to identify areas for improvement, trends, and open problems regarding intelligent failure analysis. This information can help researchers learn the benefits of both methodologies, use their tools, and integrate them to strengthen failure analysis. Each paper has been read and analyzed to extract data and tools used within the paper and their benefits. It was observed that the literature on the three methodologies, FMEA, RCA, and FTA, is diverse. In Industry 4.0, the availability of data, and advances in technology are helping these methodologies benefit from the same tools, such as BNs and neural networks, and make them more integrated.
The literature was classified based on the data needed for a (hybrid) intelligence failure analysis methodology and the tools used for failure analysis to be data-driven and automated. In addition, trends to make these methodologies smart and possible future research in this regard were discussed.
Two main classes of failure structure and failure detection data are usually needed for a failure analysis methodology, each of which can be classified as expert-driven and data-driven. However, a combination of all types of data can lead to more reliable failure analysis. Most papers focused on operational and historical expert-driven and/or data-driven problem-solving data. Among the tools used within FMEA, RCA, and FTA methodologies, BNs have the capability to make a methodology smart and interact with both humans and machines to benefit from hybrid intelligence. BNs not only can analyze failures to identify root causes but also can analyze possible solutions to provide necessary action to prevent failures. A BN’s are also capable of real-time inference, counterfactual reasoning, and managing confounding factors. BNs handle both discrete and continuous data types, unlike traditional classification algorithms. Besides BNs, classification by neural networks, other classification tools, rule-based algorithms, and other tools have been proposed in the literature.
Finally, managerial insights and future research are provided. Most studies have focused on the determination of root causes. It is necessary to automatically find possible mitigation and corrective actions. This step of a failure analysis methodology needs more interaction with humans. Thus, the benefits of hybrid intelligence can be more evident here. It is imperative for humans and machines to work together to properly identify and resolve failures. System-related data should be analyzed to find possible corrective actions. This data is usually available for both proactive and reactive methodologies. Our study showed an effectively tool to integrate knowledge from experts and sensors in needed, enabling operators and engineers to provide timely input and observe immediate results. There is a need to identify failure structures using a hybrid approach that combines expert and data knowledge. Real-time input and structure identification with Bayesian networks can be achieved through the use of Bayesian networks. Further exploration of BNs and comparative analyses with other tools is necessary to enhance understanding and adoption of the best tools for a hybrid intelligence failure analysis in real-time scenarios to prevent failures.
Data availability
There is no data related to this paper.
Agrawal, V., Panigrahi, B. K., & Subbarao, P. (2016). Intelligent decision support system for detection and root cause analysis of faults in coal mills. IEEE Transactions on Fuzzy Systems, 25 (4), 934–944.
Article Google Scholar
Akata, Z., Balliet, D., De Rijke, M., Dignum, F., Dignum, V., Eiben, G., Fokkens, A., Grossi, D., Hindriks, K., Hoos, H., et al. (2020). A research agenda for hybrid intelligence: Augmenting human intellect with collaborative, adaptive, responsible, and explainable artificial intelligence. Computer, 53 (08), 18–28.
Al-Mamory, S. O., & Zhang, H. (2009). Intrusion detection alarms reduction using root cause analysis and clustering. Computer Communications, 32 (2), 419–430.
Ament, C., & Goch, G. (2001). A process oriented approach to automated quality control. CIRP Annals, 50 (1), 251–254.
Bhardwaj, U., Teixeira, A., & Soares, C. G. (2022). Bayesian framework for reliability prediction of subsea processing systems accounting for influencing factors uncertainty. Reliability Engineering & System Safety, 218 , 108143.
Brahim, I. B., Addouche, S. A., El Mhamedi, A., & Boujelbene, Y. (2019). Build a Bayesian network from FMECA in the production of automotive parts: Diagnosis and prediction. IFAC-PapersOnLine, 52 (13), 2572–2577.
Cai, B., Huang, L., & Xie, M. (2017). Bayesian networks in fault diagnosis. IEEE Transactions on Industrial Informatics, 13 (5), 2227–2240.
Camarillo, A., Ríos, J., & Althoff, K. D. (2018). Knowledge-based multi-agent system for manufacturing problem solving process in production plants. Journal of Manufacturing Systems, 47 , 115–127.
Chan, A., & McNaught, K. R. (2008). Using Bayesian networks to improve fault diagnosis during manufacturing tests of mobile telephone infrastructure. Journal of the Operational Research Society, 59 (4), 423–430.
Chang, W. L., Pang, L. M., & Tay, K. M. (2017). Application of self-organizing map to failure modes and effects analysis methodology. Neurocomputing, 249 , 314–320.
Chang, W. L., Tay, K. M., & Lim, C. P. (2015). Clustering and visualization of failure modes using an evolving tree. Expert Systems with Applications, 42 (20), 7235–7244.
Chen, H. S., Yan, Z., Zhang, X., Liu, Y., & Yao, Y. (2018). Root cause diagnosis of process faults using conditional Granger causality analysis and maximum spanning tree. IFAC-PapersOnLine, 51 (18), 381–386.
Chen, L., Jiao, J., Wei, Q., & Zhao, T. (2017). An improved formal failure analysis approach for safety-critical system based on mbsa. Engineering Failure Analysis, 82 , 713–725.
Chen, X., & Jiao, J. (2017). A fault propagation modeling method based on a finite state machine. Annual Reliability and Maintainability Symposium (RAMS), 2017 , 1–7.
Google Scholar
Chhetri, T. R., Aghaei, S., Fensel, A., Göhner, U., Gül-Ficici, S., & Martinez-Gil, J. (2023). Optimising manufacturing process with Bayesian structure learning and knowledge graphs. Computer Aided Systems Theory - EUROCAST, 2022 , 594–602.
Chien, C. F., Liu, C. W., & Chuang, S. C. (2017). Analysing semiconductor manufacturing big data for root cause detection of excursion for yield enhancement. International Journal of Production Research, 55 (17), 5095–5107.
Clancy, R., O’Sullivan, D., & Bruton, K. (2023). Data-driven quality improvement approach to reducing waste in manufacturing. The TQM Journal, 35 (1), 51–72.
Confalonieri, R., Coba, L., Wagner, B., & Besold, T. R. (2021). A historical perspective of explainable artificial intelligence. Wiley Interdisciplinary Reviews: Data Mining and Knowledge Discovery, 11 (1), e1391.
Correia, A., Grover, A., Schneider, D., Pimentel, A. P., Chaves, R., De Almeida, M. A., & Fonseca, B. (2023). Designing for hybrid intelligence: A taxonomy and survey of crowd-machine interaction. Applied Sciences, 13 (4), 2198.
Dabous, S. A., Ibrahim, F., Feroz, S., & Alsyouf, I. (2021). Integration of failure mode, effects, and criticality analysis with multi-criteria decision-making in engineering applications: Part I- manufacturing industry. Engineering Failure Analysis, 122 , 105264.
Dellermann, D., Ebel, P., Söllner, M., & Leimeister, J. M. (2019). Hybrid intelligence. Business & Information Systems Engineering, 61 , 637–643.
Demirbaga, U., Wen, Z., Noor, A., Mitra, K., Alwasel, K., Garg, S., Zomaya, A. Y., & Ranjan, R. (2021). Autodiagn: An automated real-time diagnosis framework for big data systems. IEEE Transactions on Computers, 71 (5), 1035–1048.
Dey, S., & Stori, J. (2005). A Bayesian network approach to root cause diagnosis of process variations. International Journal of Machine Tools and Manufacture, 45 (1), 75–91.
Du, S., Lv, J., & Xi, L. (2012). A robust approach for root causes identification in machining processes using hybrid learning algorithm and engineering knowledge. Journal of Intelligent Manufacturing, 23 (5), 1833–1847.
Duan, P., He, Z., He, Y., Liu, F., Zhang, A., & Zhou, D. (2020). Root cause analysis approach based on reverse cascading decomposition in QFD and fuzzy weight ARM for quality accidents. Computers & Industrial Engineering, 147 , 106643.
Ebeling, C. E. (2019). An introduction to reliability and maintainability engineering . Waveland Press.
Ebrahimipour, V., Rezaie, K., & Shokravi, S. (2010). An ontology approach to support FMEA studies. Expert Systems with Applications, 37 (1), 671–677.
Filz, M. A., Gellrich, S., Lang, F., Zietsch, J., Abraham, T., & Herrmann, C. (2021). Data-driven analysis of product property propagation to support process-integrated quality management in manufacturing systems. Procedia CIRP, 104 , 900–905.
Filz, M. A., Langner, J. E. B., Herrmann, C., & Thiede, S. (2021). Data-driven failure mode and effect analysis (FMEA) to enhance maintenance planning. Computers in Industry, 129 , 103451.
French, S., Bedford, T., Pollard, S. J., & Soane, E. (2011). Human reliability analysis: A critique and review for managers. Safety Science, 49 (6), 753–763.
Gomez-Andrades, A., Barco, R., Serrano, I., Delgado, P., Caro-Oliver, P., & Munoz, P. (2016). Automatic root cause analysis based on traces for LTE self-organizing networks. IEEE Wireless Communications, 23 (3), 20–28.
Gómez-Andrades, A., Munoz, P., Serrano, I., & Barco, R. (2015). Automatic root cause analysis for lte networks based on unsupervised techniques. IEEE Transactions on Vehicular Technology, 65 (4), 2369–2386.
Hecht, M., & Baum, D. (2019). Failure propagation modeling in FMEAs for reliability, safety, and cybersecurity using SysML. Procedia Computer Science, 153 , 370–377.
Hireche, C., Dezan, C., Mocanu, S., Heller, D., & Diguet, J. P. (2018). Context/resource-aware mission planning based on BNs and concurrent MDPs for autonomous UAVs. Sensors, 18 (12), 4266.
Huang, J., You, J. X., Liu, H. C., & Song, M. S. (2020). Failure mode and effect analysis improvement: A systematic literature review and future research agenda. Reliability Engineering & System Safety, 199 , 106885.
Insua, D. R., Ruggeri, F., Soyer, R., & Wilson, S. (2020). Advances in Bayesian decision making in reliability. European Journal of Operational Research, 282 (1), 1–18.
Jacobo, V., Ortiz, A., Cerrud, Y., & Schouwenaars, R. (2007). Hybrid expert system for the failure analysis of mechanical elements. Engineering Failure Analysis, 14 (8), 1435–1443.
Jarrahi, M. H., Lutz, C., & Newlands, G. (2022). Artificial intelligence, human intelligence and hybrid intelligence based on mutual augmentation. Big Data & Society, 9 (2), 20539517221142824.
Jiang, S., Qin, S., Pulsipher, J. L., & Zavala, V. M. (2024). Convolutional neural networks: Basic concepts and applications in manufacturing. Artificial Intelligence in Manufacturing, 8 , 63–102.
Jiao, J., Zhen, W., Zhu, W., & Wang, G. (2020). Quality-related root cause diagnosis based on orthogonal kernel principal component regression and transfer entropy. IEEE Transactions on Industrial Informatics, 17 (9), 6347–6356.
Johnson, K., & Khan, M. K. (2003). A study into the use of the process failure mode and effects analysis (PFMEA) in the automotive industry in the UK. Journal of Materials Processing Technology, 139 (1–3), 348–356.
Jomthanachai, S., Wong, W. P., & Lim, C. P. (2021). An application of data envelopment analysis and machine learning approach to risk management. IEEE Access, 9 , 85978–85994.
Kabir, S., & Papadopoulos, Y. (2019). Applications of Bayesian networks and Petri nets in safety, reliability, and risk assessments: A review. Safety Science, 115 , 154–175.
Khakzad, N., Khan, F., & Amyotte, P. (2012). Dynamic risk analysis using bow-tie approach. Reliability Engineering & System Safety, 104 , 36–44.
Khorshidi, H. A., Gunawan, I., & Ibrahim, M. Y. (2015). Data-driven system reliability and failure behavior modeling using FMECA. IEEE Transactions on Industrial Informatics, 12 (3), 1253–1260.
Kumar, M., & Kaushik, M. (2020). System failure probability evaluation using fault tree analysis and expert opinions in intuitionistic fuzzy environment. Journal of Loss Prevention in the Process Industries, 67 , 104236.
Lee BH (2001) Using Bayes belief networks in industrial FMEA modeling and analysis. Annual Reliability and Maintainability Symposium. 2001 Proceedings. International Symposium on Product Quality and Integrity (Cat. No.01CH37179) , pp. 7–15.
Lee MH, Siewiorek DP, Smailagic A, Bernardino A, Bermúdez i Badia S (2020) Interactive hybrid approach to combine machine and human intelligence for personalized rehabilitation assessment. Proceedings of the ACM Conference on Health, Inference, and Learning , pp. 160–169.
Leu, S. S., & Chang, C. M. (2013). Bayesian-network-based safety risk assessment for steel construction projects. Accident Analysis & Prevention, 54 , 122–133.
Li, B., Han, T., & Kang, F. (2013). Fault diagnosis expert system of semiconductor manufacturing equipment using a Bayesian network. International Journal of Computer Integrated Manufacturing, 26 (12), 1161–1171.
Liu, C., Lore, K. G., Jiang, Z., & Sarkar, S. (2021). Root-cause analysis for time-series anomalies via spatiotemporal graphical modeling in distributed complex systems. Knowledge-Based Systems, 211 , 106527.
Liu, D. R., & Ke, C. K. (2007). Knowledge support for problem-solving in a production process: A hybrid of knowledge discovery and case-based reasoning. Expert Systems with Applications, 33 (1), 147–161.
Liu, H. C., Chen, X. Q., Duan, C. Y., & Wang, Y. M. (2019). Failure mode and effect analysis using multi-criteria decision making methods: A systematic literature review. Computers & Industrial Engineering, 135 , 881–897.
Liu, H. C., Liu, L., & Liu, N. (2013). Risk evaluation approaches in failure mode and effects analysis: A literature review. Expert Systems with Applications, 40 (2), 828–838.
Liu, L., Fan, D., Wang, Z., Yang, D., Cui, J., Ma, X., & Ren, Y. (2019). Enhanced GO methodology to support failure mode, effects and criticality analysis. Journal of Intelligent Manufacturing, 30 (3), 1451–1468.
Ma, G., & Wu, M. (2020). A big data and FMEA-based construction quality risk evaluation model considering project schedule for shanghai apartment projects. International Journal of Quality & Reliability Management, 37 (1), 18–33.
Ma, Q., Li, H., & Thorstenson, A. (2021). A big data-driven root cause analysis system: Application of machine learning in quality problem solving. Computers & Industrial Engineering, 160 , 107580.
Madzík, P., & Kormanec, P. (2020). Developing the integrated approach of Kano model and failure mode and effect analysis. Total Quality Management & Business Excellence, 31 (15–16), 1788–1810.
Mangalathu, S., Hwang, S. H., & Jeon, J. S. (2020). Failure mode and effects analysis of RC members based on machine-learning-based shapley additive explanations (shap) approach. Engineering Structures, 219 , 110927.
Mazzoleni, M., Maccarana, Y., & Previdi, F. (2017). A comparison of data-driven fault detection methods with application to aerospace electro-mechanical actuators. IFAC-PapersOnLine, 50 (1), 12797–12802.
Mirbabaie, M., Stieglitz, S., & Frick, N. R. (2021). Hybrid intelligence in hospitals: Towards a research agenda for collaboration. Electronic Markets, 31 , 365–387.
Musumeci, F., Magni, L., Ayoub, O., Rubino, R., Capacchione, M., Rigamonti, G., Milano, M., Passera, C., & Tornatore, M. (2020). Supervised and semi-supervised learning for failure identification in microwave networks. IEEE Transactions on Network and Service Management, 18 (2), 1934–1945.
Nepal, B., & Yadav, O. P. (2015). Bayesian belief network-based framework for sourcing risk analysis during supplier selection. International Journal of Production Research, 53 (20), 6114–6135.
Nie, W., Liu, W., Wu, Z., Chen, B., & Wu, L. (2019). Failure mode and effects analysis by integrating Bayesian fuzzy assessment number and extended gray relational analysis-technique for order preference by similarity to ideal solution method. Quality and Reliability Engineering International, 35 (6), 1676–1697.
Oliveira, E. E., Miguéis, V. L., & Borges, J. L. (2021). Understanding overlap in automatic root cause analysis in manufacturing using causal inference. IEEE Access, 10 , 191–201.
Oliveira, E. E., Miguéis, V. L., & Borges, J. L. (2022). On the influence of overlap in automatic root cause analysis in manufacturing. International Journal of Production Research, 60 (21), 6491–6507.
Oliveira, E. E., Miguéis, V. L., & Borges, J. L. (2023). Automatic root cause analysis in manufacturing: An overview & conceptualization. Journal of Intelligent Manufacturing, 34 , 2061–2078.
Oztemel, E., & Gursev, S. (2020). Literature review of industry 4.0 and related technologies. Journal of Intelligent Manufacturing, 31 (1), 127–182.
Palluat, N., Racoceanu, D., & Zerhouni, N. (2006). A neuro-fuzzy monitoring system: Application to flexible production systems. Computers in Industry, 57 (6), 528–538.
Pang, J., Zhang, N., Xiao, Q., Qi, F., & Xue, X. (2021). A new intelligent and data-driven product quality control system of industrial valve manufacturing process in CPS. Computer Communications, 175 , 25–34.
Pearl, J. (1988). Probabilistic reasoning in intelligent systems: Networks of plausible inference . Morgan kaufmann.
Petrescu, M., & Krishen, A. S. (2023). Hybrid intelligence: Human-ai collaboration in marketing analytics. Journal of Marketing Analytics, 11 (3), 263–274.
Piller, F. T., Nitsch, V., & van der Aalst, W. (2022). Hybrid intelligence in next generation manufacturing: An outlook on new forms of collaboration between human and algorithmic decision-makers in the factory of the future (pp. 139–158). Forecasting Next Generation Manufacturing: Digital Shadows, Human-Machine Collaboration, and Data-driven Business Models.
Price, C. J., & Taylor, N. S. (2002). Automated multiple failure FMEA. Reliability Engineering & System Safety, 76 (1), 1–10.
Psarommatis, F., & Kiritsis, D. (2022). A hybrid decision support system for automating decision making in the event of defects in the era of zero defect manufacturing. Journal of Industrial Information Integration, 26 , 100263.
Rajpathak, D., & De, S. (2016). A data-and ontology-driven text mining-based construction of reliability model to analyze and predict component failures. Knowledge and Information Systems, 46 (1), 87–113.
Rastayesh, S., Bahrebar, S., Blaabjerg, F., Zhou, D., Wang, H., & Dalsgaard Sørensen, J. (2019). A system engineering approach using FMEA and Bayesian network for risk analysis-a case study. Sustainability, 12 (1), 77.
Rausand, M., & Øien, K. (1996). The basic concepts of failure analysis. Reliability Engineering & System Safety, 53 (1), 73–83.
Rivera Torres, P. J., Serrano Mercado, E. I., Llanes Santiago, O., & Anido Rifón, L. (2018). Modeling preventive maintenance of manufacturing processes with probabilistic Boolean networks with interventions. Journal of Intelligent Manufacturing, 29 (8), 1941–1952.
Rokach, L., & Hutter, D. (2012). Automatic discovery of the root causes for quality drift in high dimensionality manufacturing processes. Journal of Intelligent Manufacturing, 23 (5), 1915–1930.
Ruijters, E., & Stoelinga, M. (2015). Fault tree analysis: A survey of the state-of-the-art in modeling, analysis and tools. Computer Science Review, 15 , 29–62.
Sariyer, G., Mangla, S. K., Kazancoglu, Y., Ocal Tasar, C., & Luthra, S. (2021). Data analytics for quality management in Industry 4.0 from a MSME perspective. Annals of Operations Research, 23 , 1–19.
Sayed, M. S., & Lohse, N. (2014). Ontology-driven generation of Bayesian diagnostic models for assembly systems. The International Journal of Advanced Manufacturing Technology, 74 (5), 1033–1052.
Schuh, G., Gützlaff, A., Thomas, K., & Welsing, M. (2021). Machine learning based defect detection in a low automated assembly environment. Procedia CIRP, 104 , 265–270.
Shi, H., Wang, L., Li, X. Y., & Liu, H. C. (2020). A novel method for failure mode and effects analysis using fuzzy evidential reasoning and fuzzy Petri nets. Journal of Ambient Intelligence and Humanized Computing, 11 (6), 2381–2395.
Snooke, N., & Price, C. (2012). Automated FMEA based diagnostic symptom generation. Advanced Engineering Informatics, 26 (4), 870–888.
Spreafico, C., Russo, D., & Rizzi, C. (2017). A state-of-the-art review of FMEA/FMECA including patents. Computer Science Review, 25 , 19–28.
Stamatis, D. H. (2003). Failure mode and effect analysis: FMEA from theory to execution . ASQ Quality Press.
Steenwinckel B, Heyvaert P, De Paepe D, Janssens O, Vanden Hautte S, Dimou A, De Turck F, Van Hoecke S, Ongenae F (2018) Towards adaptive anomaly detection and root cause analysis by automated extraction of knowledge from risk analyses. 9th International Semantic Sensor Networks Workshop, Co-Located with 17th International Semantic Web Conference (ISWC 2018) , Vol. 2213, pp. 17–31.
Sun, Y., Qin, W., Zhuang, Z., & Xu, H. (2021). An adaptive fault detection and root-cause analysis scheme for complex industrial processes using moving window KPCA and information geometric causal inference. Journal of Intelligent Manufacturing, 32 (7), 2007–2021.
Tari, J. J., & Sabater, V. (2004). Quality tools and techniques: Are they necessary for quality management? International Journal of Production Economics, 92 (3), 267–280.
Tay, K. M., Jong, C. H., & Lim, C. P. (2015). A clustering-based failure mode and effect analysis model and its application to the edible bird nest industry. Neural Computing and Applications, 26 (3), 551–560.
Teoh, P. C., & Case, K. (2004). Failure modes and effects analysis through knowledge modelling. Journal of Materials Processing Technology, 153 , 253–260.
Teoh, P. C., & Case, K. (2005). An evaluation of failure modes and effects analysis generation method for conceptual design. International Journal of Computer Integrated Manufacturing, 18 (4), 279–293.
Thomé, A. M. T., Scavarda, L. F., & Scavarda, A. J. (2016). Conducting systematic literature review in operations management. Production Planning & Control, 27 (5), 408–420.
Tönnes, W. (2018). Applying data of historical defects to increase efficiency of rework in assembly. Procedia CIRP, 72 , 255–260.
van der Aalst, W. M. (2021). Hybrid intelligence: To automate or not to automate, that is the question. International Journal of Information Systems and Project Management, 9 (2), 5–20.
Waghen, K., & Ouali, M. S. (2021). Multi-level interpretable logic tree analysis: A data-driven approach for hierarchical causality analysis. Expert Systems with Applications, 178 , 115035.
Wan, C., Yan, X., Zhang, D., Qu, Z., & Yang, Z. (2019). An advanced fuzzy Bayesian-based FMEA approach for assessing maritime supply chain risks. Transportation Research Part E, 125 , 222–240.
Wang, L., Li, S., Wei, O., Huang, M., & Hu, J. (2018). An automated fault tree generation approach with fault configuration based on model checking. IEEE Access, 6 , 46900–46914.
Wang, Q., Jia, G., Jia, Y., & Song, W. (2021). A new approach for risk assessment of failure modes considering risk interaction and propagation effects. Reliability Engineering & System Safety, 216 , 108044.
Williams, P. M. (2001). Techniques for root cause analysis. Baylor University Medical Center Proceedings, 14 (2), 154–157.
Wu, Z., Liu, W., & Nie, W. (2021). Literature review and prospect of the development and application of FMEA in manufacturing industry. The International Journal of Advanced Manufacturing Technology, 112 (5), 1409–1436.
Xu, Z., & Dang, Y. (2020). Automated digital cause-and-effect diagrams to assist causal analysis in problem-solving: A data-driven approach. International Journal of Production Research, 58 (17), 5359–5379.
Xu, Z., & Dang, Y. (2023). Data-driven causal knowledge graph construction for root cause analysis in quality problem solving. International Journal of Production Research, 61 (10), 3227–3245.
Xu, Z., Dang, Y., Munro, P., & Wang, Y. (2020). A data-driven approach for constructing the component-failure mode matrix for FMEA. Journal of Intelligent Manufacturing, 31 (1), 249–265.
Yang, C., Zou, Y., Lai, P., & Jiang, N. (2015). Data mining-based methods for fault isolation with validated fmea model ranking. Applied Intelligence, 43 (4), 913–923.
Yang, S., Bian, C., Li, X., Tan, L., & Tang, D. (2018). Optimized fault diagnosis based on FMEA-style CBR and BN for embedded software system. The International Journal of Advanced Manufacturing Technology, 94 (9), 3441–3453.
Yang, S., Liu, H., Zhang, Y., Arndt, T., Hofmann, C., Häfner, B., & Lanza, G. (2020). A data-driven approach for quality analytics of screwing processes in a global learning factory. Procedia Manufacturing, 45 , 454–459.
Yang, S., & Liu, T. (1998). A Petri net approach to early failure detection and isolation for preventive maintenance. Quality and Reliability Engineering International, 14 (5), 319-330.
Yang, Y. J., Xiong, Y. L., Zhang, X. Y., Wang, G. H., & Zou, B. (2022). Reliability analysis of continuous emission monitoring system with common cause failure based on fuzzy FMECA and Bayesian networks. Annals of Operations Research, 311 , 451–467.
Yang, Z. X., Zheng, Y. Y., & Xue, J. X. (2009). Development of automatic fault tree synthesis system using decision matrix. International Journal of Production Economics, 121 (1), 49–56.
Ye, P., Wang, X., Zheng, W., Wei, Q., & Wang, F. Y. (2022). Parallel cognition: Hybrid intelligence for human-machine interaction and management. Frontiers of Information Technology & Electronic Engineering, 23 (12), 1765–1779.
Yucesan, M., Gul, M., & Celik, E. (2021). A holistic FMEA approach by fuzzy-based Bayesian network and best-worst method. Complex & Intelligent Systems, 7 (3), 1547–1564.
Yuniarto, H. (2012). The shortcomings of existing root cause analysis tools. Proceedings of the World Congress on Engineering, 3 , 186–191.
Zhang, S., Xie, X., & Qu, H. (2023). A data-driven workflow for evaporation performance degradation analysis: A full-scale case study in the herbal medicine manufacturing industry. Journal of Intelligent Manufacturing, 34 , 651–668.
Zheng, T., Ardolino, M., Bacchetti, A., & Perona, M. (2021). The applications of industry 4.0 technologies in manufacturing context: a systematic literature review. International Journal of Production Research, 59 (6), 1922–1954.
Zhou, A., Yu, D., & Zhang, W. (2015). A research on intelligent fault diagnosis of wind turbines based on ontology and FMECA. Advanced Engineering Informatics, 29 (1), 115–125.
Zhu, C., & Zhang, T. (2022). A review on the realization methods of dynamic fault tree. Quality and Reliability Engineering International, 38 (6), 3233–3251.
Zhu, J. H., Chen, Z. S., Shuai, B., Pedrycz, W., Chin, K. S., & Martínez, L. (2021). Failure mode and effect analysis: A three-way decision approach. Engineering Applications of Artificial Intelligence, 106 , 104505.
Zuo, X., Li, B., & Yang, J. (2016). Error sensitivity analysis and precision distribution for multi-operation machining processes based on error propagation model. The International Journal of Advanced Manufacturing Technology, 86 (1), 269–280.
Download references
This research is funded by Flanders Make under the project AQUME_SBO, project number 2022-0151. Flanders Make is the Flemish strategic research center for the manufacturing industry in Belgium.
Author information
Authors and affiliations.
Department of Industrial Systems Engineering and Product Design, Ghent University, 9052, Ghent, Belgium
Mahdi Mokhtarzadeh, Jorge Rodríguez-Echeverría, Ivana Semanjski & Sidharta Gautama
FlandersMake@UGent–corelab ISyE, Lommel, Belgium
Escuela Superior Politécnica del Litoral, ESPOL, Facultad de Ingeniería en Electricidad y Computación, ESPOL Polytechnic University, Campus Gustavo Galindo, Km 30.5 Vía Perimetral, P.O. Box 09-01-5863, 090112, Guayaquil, Ecuador
Jorge Rodríguez-Echeverría
You can also search for this author in PubMed Google Scholar
Corresponding author
Correspondence to Mahdi Mokhtarzadeh .
Ethics declarations
Competing interest.
The authors report there are no competing interests to declare.
Additional information
Publisher's note.
Springer Nature remains neutral with regard to jurisdictional claims in published maps and institutional affiliations.
Supplementary Information
Below is the link to the electronic supplementary material.
Supplementary file 1 (pdf 137 KB)
Rights and permissions.
Open Access This article is licensed under a Creative Commons Attribution 4.0 International License, which permits use, sharing, adaptation, distribution and reproduction in any medium or format, as long as you give appropriate credit to the original author(s) and the source, provide a link to the Creative Commons licence, and indicate if changes were made. The images or other third party material in this article are included in the article’s Creative Commons licence, unless indicated otherwise in a credit line to the material. If material is not included in the article’s Creative Commons licence and your intended use is not permitted by statutory regulation or exceeds the permitted use, you will need to obtain permission directly from the copyright holder. To view a copy of this licence, visit http://creativecommons.org/licenses/by/4.0/ .
Reprints and permissions
About this article
Mokhtarzadeh, M., Rodríguez-Echeverría, J., Semanjski, I. et al. Hybrid intelligence failure analysis for industry 4.0: a literature review and future prospective. J Intell Manuf (2024). https://doi.org/10.1007/s10845-024-02376-5
Download citation
Received : 27 June 2023
Accepted : 14 March 2024
Published : 22 April 2024
DOI : https://doi.org/10.1007/s10845-024-02376-5
Share this article
Anyone you share the following link with will be able to read this content:
Sorry, a shareable link is not currently available for this article.
Provided by the Springer Nature SharedIt content-sharing initiative
- Automated failure analysis
- Data-driven failure analysis
- Human–machine cooperation
- Find a journal
- Publish with us
- Track your research
- Study Guides
- Homework Questions
Literature Review
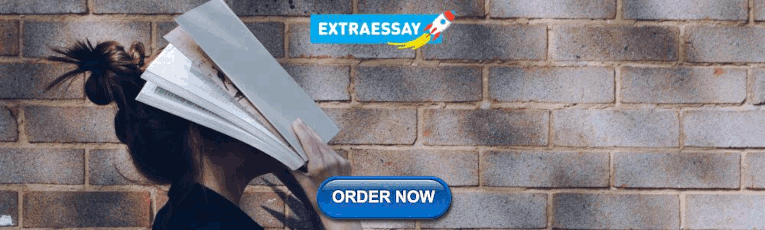
IMAGES
VIDEO
COMMENTS
This is why the literature review as a research method is more relevant than ever. Traditional literature reviews often lack thoroughness and rigor and are conducted ad hoc, rather than following a specific methodology. Therefore, questions can be raised about the quality and trustworthiness of these types of reviews.
What is a literature review? A literature review is a survey of scholarly sources on a specific topic. It provides an overview of current knowledge, allowing you to identify relevant theories, methods, and gaps in the existing research that you can later apply to your paper, thesis, or dissertation topic.
Literature reviews allow scientists to argue that they are expanding current. expertise - improving on what already exists and filling the gaps that remain. This paper demonstrates the literatu ...
A literature review is an integral part of both research and education. It is the first and foremost step in research. There are different types of literature reviews with varying degrees of rigor in methodology, ranging from scoping reviews to systematic reviews.
Literature Review is a comprehensive survey of the works published in a particular field of study or line of research, usually over a specific period of time, in the form of an in-depth, critical bibliographic essay or annotated list in which attention is drawn to the most significant works. Also, we can define a literature review as the ...
Literature review serves as a foundation for all types of research in building knowledge, establishing policy and practice guidelines, and generating new ideas and direction (Snyder, 2019). This ...
A literature review is a discussion of the literature (aka. the "research" or "scholarship") surrounding a certain topic. A good literature review doesn't simply summarize the existing material, but provides thoughtful synthesis and analysis. ... Aimed at undergraduate students in research methods courses or others with a lab or research ...
Your research methodology discusses and explains the data collection and analysis methods you used in your research. A key part of your thesis, dissertation, ... A literature review is a survey of scholarly knowledge on a topic. It is used to identify trends, debates, and gaps in the research. ...
Research methods are specific procedures for collecting and analyzing data. Developing your research methods is an integral part of your research design. When planning your methods, there are two key decisions you will make. First, decide how you will collect data. Your methods depend on what type of data you need to answer your research question:
Step 1: Explain your methodological approach. Step 2: Describe your data collection methods. Step 3: Describe your analysis method. Step 4: Evaluate and justify the methodological choices you made. Tips for writing a strong methodology chapter. Other interesting articles.
What is research methodology? Research methodology simply refers to the practical "how" of a research study. More specifically, it's about how a researcher systematically designs a study to ensure valid and reliable results that address the research aims, objectives and research questions. Specifically, how the researcher went about deciding:
In writing the literature review, your purpose is to convey to your reader what knowledge and ideas have been established on a topic, and what their strengths and weaknesses are. As a piece of writing, the literature review must be defined by a guiding concept (e.g., your research objective, the problem or issue you are discussing, or your ...
A literature review involves researching, reading, analyzing, evaluating, and summarizing scholarly literature (typically journals and articles) about a specific topic. The results of a literature review may be an entire report or article OR may be part of a article, thesis, dissertation, or grant proposal.
The conventional focus of rigorous literature reviews (i.e., review types for which systematic methods have been codified, including the various approaches to quantitative systematic reviews [2-4], and the numerous forms of qualitative and mixed methods literature synthesis [5-10]) is to synthesize empirical research findings from multiple ...
In the field of research, the term method represents the specific approaches and procedures that the researcher systematically utilizes that are manifested in the research design, sampling design, data collec-tion, data analysis, data interpretation, and so forth. The literature review represents a method because the literature reviewer chooses ...
Definition, Types, and Examples. Research methodology 1,2 is a structured and scientific approach used to collect, analyze, and interpret quantitative or qualitative data to answer research questions or test hypotheses. A research methodology is like a plan for carrying out research and helps keep researchers on track by limiting the scope of ...
9.3. Types of Review Articles and Brief Illustrations. EHealth researchers have at their disposal a number of approaches and methods for making sense out of existing literature, all with the purpose of casting current research findings into historical contexts or explaining contradictions that might exist among a set of primary research studies conducted on a particular topic.
This article is organized as follows: The next section presents the methodology adopted by this research, followed by a section that discusses the typology of literature reviews and provides empirical examples; the subsequent section summarizes the process of literature review; and the last section concludes the paper with suggestions on how to improve the quality and rigor of literature ...
Qualitative Research Methodology. This is a research methodology that involves the collection and analysis of non-numerical data such as words, images, and observations. This type of research is often used to explore complex phenomena, to gain an in-depth understanding of a particular topic, and to generate hypotheses.
Sage Research Methods community highlighted this useful collection with a series of interviews and related resources in 2023. Review research is an broad term that describes various types of review articles.Kunisch et al. (2023) define it as . a class of research inquiries that employ scientific methods to analyze and synthesize prior research to develop new knowledge for academia, practice ...
Literary research methods tend to differ from research methods in the hard sciences (such as physics and chemistry). Science research must present results that are reproducible, while literary research rarely does (though it must still present evidence for its claims). Literary research often deals with questions of meaning, social conventions ...
Zhenguo Yuan points out that "literature research methodology" include non-structured qualitative analysis and structured quantitative analysis. They access to and process information contained in literatures from different perspectives. Generally speaking, literatures are descriptions of the nature, functions and characteristics of objects
Keywords: literary research methods or methodologies, literary theories, research skills, oral history, archival, discourse analysis, textual analysis, interviewing, ICT Discover the world's ...
We follow Thomé et al. 8-step literature review methodology to assure a rigorous literature review of intelligence, automated/data-driven, failure analysis methodology for Industry 4.0. In Step 1, our (hybrid) intelligence failure analysis problem is planned and formulated by identifying the needs, scope, and questions for this research.
Research Question/Hypotheses Methodology Analysis and Results Conclusions Implications for Future research Implications for Practice References: Burke, R. E ...