Causes and effects of road traffic accidents in Jimma town
- Institutional Repository Home
- Jimma Institute of Technology
- Civil Engineering
Show simple item record
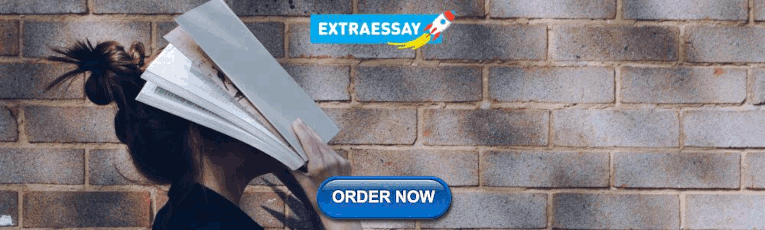
Files in this item
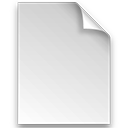
This item appears in the following Collection(s)
- Civil Engineering [853]
- Communities & Collections
- By Issue Date
This Collection

An official website of the United States government
The .gov means it’s official. Federal government websites often end in .gov or .mil. Before sharing sensitive information, make sure you’re on a federal government site.
The site is secure. The https:// ensures that you are connecting to the official website and that any information you provide is encrypted and transmitted securely.
- Publications
- Account settings
Preview improvements coming to the PMC website in October 2024. Learn More or Try it out now .
- Advanced Search
- Journal List
- v.10(2); 2020

Original research
Magnitude and determinants of road traffic accidents in northern ethiopia: a cross-sectional study, awtachew berhe woldu.
1 School of Public Health, Ayder College of Health Sciences, Mekelle University, Mekelle, Ethiopia
Abraham Aregay Desta
2 Directorate of Public Health Research and Emergency Management, Tigray Health Research Institute, Mekelle, Tigray, Ethiopia
Tewolde Wubayehu Woldearegay
3 Tigray Health Research Institute, Mekelle, Tigray, Ethiopia
Associated Data
This study aimed to assess the magnitude and determinants of road traffic accidents (RTAs) in Mekelle city, Northern Ethiopia.
A cross-sectional study was done using a simple random sampling technique.
The study was done in Mekelle city from February to June 2015.
Participants
The study was done among drivers settled in Mekelle city.
Main outcome measures
The main outcome measure was occurrence of RTA within 2 years. A binary logistic regression was used to identify factors associated with RTA.
The magnitude of RTA was found to be 23.17%. According to the drivers’ perceived cause of the accident, 22 (38.60%) of the accident was due to violation of traffic rules and regulations. The majority of the victims were pedestrians, 19 (33.33%). Drivers who were driving a governmental vehicle were 4.16 (adjusted OR (AOR) 4.16; 95% CI 1.48 to 11.70) times more likely to have RTA compared with those who drive private vehicles. Drivers who used alcohol were 2.29 (AOR 2.29; 95% CI 1.08 to 4.85) times more likely to have RTA compared with those drivers who did not consume alcohol.
Magnitude of reported road traffic accident was high. Violation of traffic laws, lack of vehicle maintenance and lack of general safety awareness on pedestrians were the dominant reported causes of RTAs. Driving a governmental vehicle and alcohol consumption were the factors associated with RTA. Monitoring blood alcohol level of drivers and regular awareness to the drivers should be in place. Holistic study should be done to identify the causes of RTAs.
Strengths and limitations of this study
- Data quality was assured under close supervision of the principal investigators.
- Appropriate statistical methods were used to present the findings of the study.
- Cross-sectional study design does not allow establishing causality.
- The analysis of this study misses some important variables like quality of the vehicles and road safety.
- There may be recall bias on the road traffic accidents occurrences.
Introduction
Road traffic accident (RTA) is an accident, which occurs or originates on a way or street open to public traffic; resulting in one or more persons being killed or injured, and at least one moving vehicle is involved. RTA includes collisions between vehicles, vehicles and pedestrians and vehicles and animals or fixed obstacles. 1 RTA contributes to poverty by causing loss of productivity, material damage, injuries, disabilities, grief and deaths. 2 Deaths and injuries resulting from road traffic crashes remain a serious problem globally and current trends suggest that this will continue to be the case in the foreseeable future. 3 4 RTA is the major cause of economic loss globally. The total costs to public services identified as follows: older drivers, £63 million (£10 000 per fatality); people driving for work, £702 million (£700 000 per fatality); motorcyclists, £1.1 billion (£800 000 per fatality) and young drivers, £1.3 billion (£1.1 million per fatality). 5
Approximately 1.3 million people die each year in traffic-related accidents worldwide. 6 Road traffic injury is now the leading cause of death for children and young adults aged 5–29 years, signalling a need for a shift in the current child health agenda. It is the eighth-leading cause of death for all age groups exceeding HIV/AIDS, tuberculosis and diarrhoeal diseases 7 and the deaths due to RTAs are predicted to become the fifth-leading cause of death by the year 2020. 6
The burden of road traffic injuries and deaths is disproportionately borne by vulnerable road users and those living in low-income and middle-income countries, where the growing number of deaths is fuelled by transport that is increasingly motorised. Between 2013 and 2016, no reductions in the number of road traffic deaths were observed in any low-income country. 2 Although road infrastructures have a significant role in the occurrence of RTA, the human factor is the most prevalent contributing factor of RTAs. This includes both driving behaviour (eg, drinking and driving, speeding, traffic law violations) and impaired skills (eg, lack of attention, exhaustion, physical disabilities and so on). 8
Poor conditions of quality of vehicles and less road safety are determinant factors for RTA in Africa 9 including Ethiopia. 10 WHO in 2011 reported that RTA in Ethiopia reached 22 786 which accounted for 2.77% of all the deaths. The report showed that RTA is the ninth killer health problem in the country. RTA makes Ethiopia 12th and 9th in the world and in Africa, respectively. 11 Mekelle is a fast growing regional city, which has a heavy traffic flow, especially during peak hours. 12 In Mekelle city, it was reported that RTA is increasing from year to year and it was shown that 96% of the causes were related to human risky behaviour whereas 4% was due to vehicle-related factors. 12 13 However, despite the growing magnitude of RTAs in the city, there is paucity of data on determinants of RTAs among drivers. In addition, to that the study can have significant role to fill the lack of data as there is lack of reliable data although it is a serious problem in most of the low-income and middle-income countries. 14 Hence, this study was conducted to assess the magnitude and determinants of RTAs among drivers in Mekelle city, Tigray, Ethiopia. This study can have a significant role in supplementing and informing the current status in achieving the United Nations (UN) Sustainable Development Goals (SDG) 3.6 for a reduction in the number of deaths by half by 2020. 15
Study setting
The study was conducted among drivers in Mekelle city, Tigray, Northern Ethiopia from February to June 2015. Mekelle is the capital city of the Tigray regional state which is found at 783 Km north of the capital city of Ethiopia, Addis Ababa. Regarding road infrastructure: Mekelle city has 55 km asphalted, 23 km cobble stone and 152 km gravel road. 16
Study design
A cross-sectional study design was used.
All drivers who were based in Mekelle city with a legal driving licence and who were driving taxi, Bajaj (three wheel taxi), private owned car and governmental car in Mekelle city were included in the study. Heavy truck drivers, drivers who were not working and sick during the study period, those who drive more than two vehicle types and those who came from other areas to Mekelle city were excluded from the study.
The sample size was calculated from a previous study, where the prevalence of RTA was reported, p=22% in Mekelle city. 12 Using 5% marginal error and 95% CI by the following formula: n= (Zα/2) 2 P (P-1) / D 2
Where n=minimum sample size required
Z=standard score corresponding to 95% CI
p=assumed proportion of drivers
D=margin of error (precision) 5%
n=3.84×0.1716/0.0025=263
Since the source population was less than 10 000(ie, 1500), sample size correction formula was used: nf=n/1+ (n/N)
Where nf=desired sample size
n=calculated sample size
n=total population
nf=n/1+ (n/N)=263/1+ (263/1500)=263/1.175=223.8~224
By adding 10% contingency for non-response, the sample size was 224+22=246.
Sampling procedures
A sampling frame was constructed by a vehicle plate number, which was obtained from Mekelle city transport office. The frame was subcategorised based on the type of the vehicle as a taxi, Bajaj, governmental vehicles and private/house vehicles. Subsamples were calculated for each category of vehicles proportional to the number of vehicles in the respective categories. Then, study subjects were selected using simple random sampling method (see figure 1 ).

Sampling procedure. Schematic presentation of the sampling procedure.
Data collection procedures and tools
The study subjects (drivers) were traced and interviewed for data collection. The drivers were traced at their destination for taxi and Bajaj, house cars in their working area and governmental cars at their offices using the car plate number. A structured interviewer administered questionnaire, adapted from different literatures, was used. The questionnaire was initially prepared in English and was translated into the local language Tigrigna. The instrument included: sociodemographic characteristics of drivers, risky behaviours factors and other variables which has a bearing on RTA. Trained data collectors and supervisors handled the data collection process.
Patient and public involvement
Drivers in Mekelle city were involved in the study.
Data quality control
Pre-est was done on 5% of the sample at Adigrat town, Tigray region. Based on the pretest findings, necessary corrections were made to the questionnaire. Adequate supervision was undertaken by the supervisors and principal investigator during the data collection. Daily spot-checking of the filled questionnaires for errors or any incompleteness was done by the supervisors and the principal investigator.
Data management and analysis
The collected data were entered and cleaned in Microsoft excel 2007. Then, the data were exported and analysed using STATA V.12. Values of categorical variables were presented as frequencies and percentages. All statistical tests were performed at the 5% significance level.
The dependent variable was a occurrence RTA within 2 years which was dichotomised into yes (labelled ‘1’) and no (labelled ‘0’). To prevent recall bias respondents were reinforced to remember the occurrence of RTA in the previous 2 years. Each independent variable was cross-tabulated and further evaluated for association in the bivariate binary logistic regression. Finally, variables significant in the bivariate analysis were entered into multivariable binary logistic regression analysis to identify determinants of RTA. Variables on risky behaviours, traffic safety rules and some other personal characteristics were used to interpret the adjusted OR (AOR) in the multivariate analysis under the adjustment of the sociodemographic variables. The final model was developed using a stepwise logistic regression.
The confounding effect of the explanatory variables was checked using forward and backward elimination techniques and any variable above 20% change of coefficient was considered as a confounder. Multicollinearity was checked using variance inflation factor (VIF) at a cut-off value of 10. Variables with greater than 10 VIF value were handled by removing the most intercorrelated variable(s) from the model and substitute their cross product as an interaction term. Final model fitness was checked using the Hosmer-Lemeshow method. Receiver operating characteristic (ROC) curve was used to show how much the independent variables in the final model predicted the dependent variable.
Sociodemographic characteristics of the respondents
The response rate was 100%. The median (IQR) age of the respondents was 30 (10) years. The majority of study participants (98.37%) were males. Regarding the marital status of the respondents, 102 (41.46%), 101 (41.06%), 30 (12.20%) and 13 (5.28%) were divorced, married, single and widower, respectively. The majority of the drivers, 170 (69.11%) were Christian Orthodox, followed by Muslims, 54 (21.95%). With regard to their educational status, 225 (91.46%) had attained at least grade 5. The median (IQR) monthly income (in Birr) of the study participants was 1000 (1200) ( table 1 ).
Sociodemographic and economic characteristics of drivers in Mekelle city, Northern Ethiopia, 2015
RTA, road traffic accident.
Magnitude of RTAs
Among all the drivers, 57 (23.17%) had encountered RTA in the past 2 years from the time of the current study. Most of the accidents happened on Monday, 22 (38.60%) and Friday, 13 (22.81%) even though accidents were reported in all the 7 days. About 22/57 (38.60%), 13/57 (22.81%), 10/57 (17.54%) and 9/57 (15.79%) of the reported causes of RTAs were due to violation of traffic laws, lack of vehicle maintenance, lack of general safety awareness on pedestrians and violation of speed limit. A significant number of the accidents, 25/57 (43.86%) happened at dawn. Pedestrians and cyclists constituted the major share of the RTA victims, 31/57 (54.40%). About three-fourths of the accidents, 43/57 (75.44%) happened at either T-junction road or cross road ( table 2 ).
Characteristics and setting of RTA in Mekelle city, Northern Ethiopia, 2015
Risky driving behaviours, infrastructure setup and practices
Concerning risky driving behaviours, 92 (37.40) of the drivers drunk alcohol before driving. About 43 (17.48%) of the drivers were chat chewers and 30 (12.20%) were smokers. More than one-third of the drivers, 96 (39.02%) ever reported that they used cell phone for communication while driving. The prevalence of RTA among drivers was 3.29%, 32.6%, 36.7%, 18.5% and 21.6% among cell phone users, alcohol consumers, chat chewers, cigarette smokers and seat belt users while driving respectively. However, the prevalence of RTA among the drivers who do not use cell phone and seat belt were 17.33% and 30.9% respectively ( table 3 ).
Risky driving behaviours, infrastructure setup and practices among drivers in Mekelle city, Northern Ethiopia, 2015
Factors associated with RTAs
In the bivariate analysis age, being married, being single, driving governmental vehicle, alcohol use, other substances other than alcohol use, cell phone use during driving, drivers’ years of experience and vehicle service were significantly associated with RTAs at 95% CI. Multivariable binary logistic regression analysis showed that drivers who drove after consuming alcohol were 2.29 (AOR 2. 29; 95% CI 1.08 to 4.85) times more likely to have RTA compared with drivers who did not consume alcohol. Drivers who drove governmental vehicles were 4.16 (AOR 4. 16; 95% CI 1.48 to 11.70) times more likely to have RTA compared with drivers of privately owned vehicles. As the driver’s experience increased by 1 year, the probability of RTA decreased by 26% (AOR 0. 74; 95% CI 0.60 to 0.90) ( table 4 ).
Bivariate and multivariable regression analysis of RTA with the predictors in Mekelle city, Northern Ethiopia, 2015
*P<0.05.
The residuals were checked for influential outlier observations and the result showed that there were no suspicious influential outlier observations. Hosmer-Lemeshow test showed a χ 2 value of 9.41 (p=0.3085) which is greater than 0.05. The null hypothesis is not to be rejected, which implies that the model estimates adequately to fit the data at an acceptable level. The area under ROC curve was 0.7536 (see figure 2 ). The predicting power of the independent variables for the dependent variable was 75.36%. Therefore, it can be concluded that the model fits the data reasonably well. No confounding factor was found.

ROC curve predicting power of the independent variables for the dependent variable. ROC, receiver operating characteristic.
The main aim of the study was to assess the magnitude and determinants of RTAs among drivers in Mekelle city, Tigray, Northern Ethiopia. This study showed 23.17% of the drivers have reported having RTA in the previous 2 years. Ownership of the vehicles, driving after taking alcohol, driver’s experience, used cell phone while driving were the determinants for RTAs among the drivers.
The study revealed that the magnitude of self-reported RTA in Mekelle city was 23.17%. There was a slight increment of accidents in this study compared with the previous study done in Mekelle city, which showed that the prevalence of RTA was 22%. 12 However, it is lower when compared with a similar study conducted in the same city among taxi drivers with four wheels, of which 26.4% of them reported RTA encounter within the past 3 years. 17 This variation might be due to the fact that the city is expanding where the population size is increasing. Or it might be due to the differences in the RTAs report period where the current study included reports of RTA in the past 2 years from the time of the study. About three-fourths (75.44%) of the accidents of this study, happened at either T-junction or cross roads. The finding of this study is higher as compared with recent statistics from USA and India which showed, approximately 55% of the total traffic crashes and 23% of crashes with fatalities in urban areas in the USA occur at intersections and approximately 32% of urban traffic crashes take place at intersections in India. 18 This difference might be due to infrastructure differences like traffic lights in the intersections of the roads. Because traffic signals do help to prevent collisions if obeying for traffic rules by the drivers. 19 In this study about 22/57 (38.60%), 13/57 (22.81%), 10/57 (17.54%) and 9/57 (15.79%) of the reported causes of RTAs were due to violation of traffic laws, lack of vehicle maintenance, lack of general safety awareness on pedestrians and violation of speed limit. This finding is similar with the study on the comparative analysis of literature concerning road safety, which showed that the causes include: lack of control and enforcement concerning implementation of traffic regulation (primarily driving at excessive speed, driving under the influence of alcohol and not respecting the rights of other road users (mainly pedestrians and cyclists), lack of appropriate infrastructure and unroadworthy vehicles. 20 This is because, obeying traffic laws are designed to protect the drivers and other people, animals or from destruction of properties around the road and it self the road. In other words by knowing the rules of the road, practising good driving skills and generally taking care as a road user can help a vital role in preventing a crash.
This study identified that ownership of the vehicles was found to be predictor of RTA. RTA was 3.78 times more likely among those who drove governmental vehicles. A study on Arab gulf countries as compared with other countries showed that vehicle ownership levels and safety parameters in both developed and low-income and middle-income countries is presented to highlight the relative seriousness of the road safety situation in different countries. 14 The possible justification for this to be happen might be due to the fact that governmental drivers might violate the traffic rules and speed up to arrive timely at workplace especially at the peak hours.
This study revealed that driving after taking alcohol was found to be an aggravating factor for RTA. Drivers who drove after consuming alcohol were 2.29 more likely to have RTA compared with those who do not consume alcohol. This finding is similar to a similar study which showed that individuals who drank alcohol were 3.2 times more likely to encounter RTA. 21 It was also supported by the Great Britain department for Transport provisional estimates for 2013 which showed that between 230 and 290 people were killed in accidents in Great Britain where at least one driver was over the drink drive limit. 22 Another study also showed that impairments from alcohol was associated with traffic accident of crashes and deaths. 23 24 This might be due to the nature of alcohol that has a range of psychomotor and cognitive effects, including attitude, judgement, vigilance, perception, reaction and controlling. 25 This can increase accident risk by lowering cognitive processing, coordination, attention, vision and hearing.
This study has also revealed that as driver’s experience increases by 1 year, the probability of getting RTA decreased by 26 percent. This finding was similar to the finding of a study in 2003 which showed that as the drive miles and experience increases, the probability of self-reported crash decreased. 26 This might be due to the anticipation of potentially hazardous traffic situations, which require years of practice.
The likelihood of RTA was 1.8 times higher among drivers who used cell phone while driving compared with these who do not use. This study is consistent with a previously done study in Mekelle city. 17 Other studies have also reported that drivers distracted by mobile devices such as smartphones and/or other in-vehicle devices are at risk for a serious negative outcomes. 27–29 A similar study indicated that telephone use while driving increases the likelihood of RTA/crash by a factor of four, while texting by around 23 times. 30 This is because of loss of attention to surroundings while driving.
The findings of this study showed visual impairment was not found to be a predictor variable for RTA. But a study done in Ibadan town Nigeria showed that drivers who had visual impairment were 1.6 times more likely to encounter RTA. 31 Therefore, this needs further investigation.
The strength of this study is that data quality was assured under close supervision of the principal investigators during both data entry and data collection time. Appropriate statistical methods were used to present the findings of the study. Despite this strength, the study has certain limitations. Due to cross-sectional study design nature, establishing causality is not possible. In addition to that, there may be recall bias and the analysis of this study misses some important variables like quality of the vehicles and road safety.
The magnitude of RTA was high. The intersections of the roads were the main cause of RTAs. Violation of traffic laws, lack of vehicle maintenance and lack of general safety awareness on pedestrians were the dominant reported causes of RTAs. Driving a governmental vehicle and alcohol consumption were the factors associated with RTA. Monitoring blood alcohol level of drivers should be in place. Education on traffic laws and regulations should be given to drivers on regular basis. In addition to that a holistic study should be done to identify the causes of RTA. Due to the similarities of the cities in North Ethiopia, this study can represent to other cities in Northern Ethiopia.
Supplementary Material
Acknowledgments.
We are glad to extend our gratitude to the data collectors and participants of the study. We would like to extend our gratitude to Mekelle University as well for funding the research.
Contributors: ABW conceptualised and designed the study, involved in data analyses, acquisition of data, tabulating the data, interpretation of data, preparing tables and figures, and critically revising the manuscript. AAD and TWW have involved in interpretation of data, Supervision, administration, drafting the initial manuscript and critically revising the manuscript. AAD has primary responsibility for final content and involved in final review. All authors read and approved the final manuscript.
Funding: This study was funded by Mekelle University for research and community services.
Competing interests: None declared.
Patient consent for publication: Not required.
Ethics approval: Ethical clearance and approval was given by Mekelle University, school of public health Ethical Review Committee with the approval number of ERC 0017/2014.
Provenance and peer review: Not commissioned; externally peer reviewed.
Data availability statement: Data are available on reasonable request. The dataset of the study findings is available from the corresponding author on reasonable request.
Advertisement
Time series modeling of road traffic accidents in Amhara Region
- Open access
- Published: 19 July 2021
- Volume 8 , article number 102 , ( 2021 )
Cite this article
You have full access to this open access article
- Kidane Alemtsega Getahun ORCID: orcid.org/0000-0002-6179-3126 1
5397 Accesses
6 Citations
Explore all metrics
Road traffic accidents (RTA) are commonly encountered incidents that can cause injuries, death, and property damage to members of society. Ethiopia is one of the highest incident rates of road traffic accidents. Report of Transport and Communication from 2012 to 2014, shows an increment in the number of traffic accidents in Ethiopia. Amhara region accounted for 27.3% of the total road traffic accident-related deaths in Ethiopia during the year 2008/9, which is the highest share among all regions in Ethiopia. The current research aims to model the trend of injury, fatal and total road traffic accidents in the Amhara region from September 2013 to May 2017. Monthly reported traffic accidents were obtained from the traffic department of the Amhara region police commission. The most universal class of models for forecasting time series data called Auto-regressive Integrated Moving Averages (ARIMA) models were applied to model the trends and patterns of road traffic accident cases in the Amhara region. The average number of observed injury RTA, fatal RTA, and total RTA were 27.2, 14, and 78.2 per month respectively. It was observed that a relatively large number of RTA’s are reported on Tuesday, Thursday, and Saturday relative to other days of the week. The data also reveals that more than 60% of accidents involve drivers between the ages of 18–30 years. ARIMA (2,0,0) (1,0,0) ARIMA (2,0,0) and ARIMA (2,0,0) (1,1,0) were fitted as the best model for total injury accidents, fatal RTA and total RTA data respectively. A 48 months forecast was made based on the fitted models and it can be concluded that road traffic accident cases would continue at the non-decreasing rate in the Amhara region for the predicted periods. Therefore, the findings of this study draw attention to the importance of implementing improved better policies and close monitoring of road trafficking to change the existing non-decreasing trend of road traffic accidents in the region .
Similar content being viewed by others
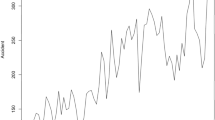
Factors affecting the number of road traffic accidents in Kerman province, southeastern Iran (2015–2021)
Assessment and prediction of road accident injuries trend using time-series models in kurdistan.
Proposal and Validation of a Model for Predicting Number of Injuries Due to Road Accidents in Casablanca City
Introduction.
According to Farag, Hashim [ 1 ], a road traffic accident is a random event involving a road user that results in property damage, death, or injury. The Global status report on road safety 2018, launched by WHO in December 2018, highlights that the number of annual road traffic deaths has reached 1.35 million, and road traffic injuries are now the leading killer of people aged 5–29 years [ 2 ]. Road traffic accidents (RTAs) affect populations all over the world; different local factors influence the causes of RTA in specific regions. The causes of RTAs among others include human or driver errors, vehicle characteristics, traffic infrastructures including engineering design, road maintenance, and traffic regulation [ 3 ]. Driver attitude including road courtesy and behavior, driving under the influence of drugs especially alcohol, gender, use of seat belts, driver age (teenage drivers and elderly drivers) are among the recognized human factors associated with RTAs [ 3 , 4 ]. It is thought that globally, about 20 million to 50 million people are injured or disabled from the effect of RTAs. Unchecked, by the year 2020, RTAs will rank third of all causes of morbidity and mortality globally [ 5 ]. Of worry, though is that RTA-related fatalities seem to increase with the gross domestic product (GDP) per capita in lower-income countries and decrease with GDP per capita in wealthy countries [ 6 ].
Road traffic accidents are the most frequent causes of injury-related deaths worldwide [ 7 ]. According to Peden [ 5 ], traffic accidents account for about 3000 daily fatalities worldwide. Nowadays, RTAs are becoming a major public safety problem and development obstacle in the world. The problem is threatening especially for developing countries like Ethiopia. According to World Health Organization [ 8 ], more than 90% of road traffic deaths occur in low- and middle-income countries. The burden is disproportionately borne by pedestrians, cyclists, and motorcyclists, in particular those living in developing countries.Besides, the victims are mainly public transport travelers in the working-age group (18–30 years). This alarming statistic underpins the importance of updating and improving accident data records and subsequently the methods of analyzing traffic data, as this will help policymakers concerning road safety to formulate evidenced-based regulations and measures.
Statistical projections show that during the period between 2000 and 2020, fatalities related to traffic accidents will decrease by about 30% in high-income countries. the alternative pattern is predicted in developing countries, where traffic accidents are expected to extend at a quick rate within the years to return. Fatalities due to traffic accidents in Ethiopia are reported to be among the highest in the world. According to a global status report on road safety, the road crash fatality rate in Ethiopia was at least 114 deaths per 10,000 vehicles per year, compared to only 10 in the UK and Ireland and 60 across 39 sub-Saharan African countries [ 9 ]. Furthermore, it is sad to note that fatalities due to road traffic accidents are higher among pedestrians in countries like Ethiopia than in developed countries. For instance, 60% of the fatalities in the US account for car drivers, while in Ethiopia only about 5% account for drivers [ 10 ]. This is also supported by a recent study where the majority of fatalities were pedestrians (87%) followed by passengers (9%) and drivers (4%), among a total of 25,110 accidents and 3415 fatalities during the period 2000–2009 in Addis Ababa.
Various studies have indicated that Ethiopia has one of the highest fatality rates per vehicle in the world. According to the estimate of the World Health Organization (WHO), in 2013 the prevalence of road traffic fatality in Ethiopia was 25.3 per 100,000 population and the rate is among the highest in the world [ 11 ]. According to various studies, the major cause of road traffic accidents in Ethiopia is driver-related problems. Driving without a license, cars' technical problems, pedestrians’ mistakes, road quality are among the major causes of road traffic accidents in Ethiopia this day. Although the situation of RTAs is getting worse from time to time, in many developing countries including Ethiopia, evidence is scarce regarding the incidence of RTA-related injuries and fatalities.
Amhara, one of the regions in Ethiopia accounted for 27.3% of the total road traffic accident-related deaths in the country during the year 2008/9, which is the highest share among all regions [ 12 ]. This entails the need to examine the overall situation and trend of road traffic accidents in the region. To date, there have been little types of research on road traffic accidents using pooled data in Amhara National Regional State (ANRS). Therefore, the current study aims to model the trend of road traffic accidents in the Amhara region using time series modeling and forecast for the future.
Materials and methods
Description of study area and source of data.
Amhara region is one of the regions of Ethiopia, containing the homeland of the Amhara people and its capital is Bahir Dar. Amhara region is bordered by the nation of Sudan to the west, and the Ethiopian regions of Tigray to the north, Afar to the east, Benishangul-Gumuz to the west and southwest, and Oromia to the south.
Data used for the analyses were obtained from Amhara region police commission traffic departments, from September 2013 to May 2017. The data collected mainly included a monthly recorded number of traffic accidents in the region and other related information about the cause of accidents. Data used in the current study was the overall regional data on road traffic accidents. The time-series analysis was applied to model the observed RTAs in the study area and to predict the future incidence. Auto-regressive Integrated Moving Averages (ARIMA) method was applied to derive models for forecasting the observed RTA data. This method is preferred because of its high accuracy in forecasting data, especially within a short to medium-term period [ 13 ].
Variables of the study
The major variables utilized in the current study were the number of injuries, the number of fatal RTAs, and the total number of RTAs observed during the study period, i.e. September 2013 to May 2017 in the Amhara region. The current study aimed at modeling all the above variables using the appropriate time series model.
Statistical analysis
Along with descriptive statistics, time series analysis was conducted for data analysis purposes. The data analysis was carried out using the R-Statistical software package. One of the main objectives of statistics is to forecast the future levels of different processes by studying the behavior of the data in the past. The most important techniques of making inferences about the future based on what has happened in the past are the analysis of time series, which may be defined as a set of observations taking at specified times, usually at equal intervals.
Components of time series
In analyzing time series, we may take the observed composite series as a whole for study or study one by one respectively the components in their own right. The components are secular trends, seasonal trends, cyclic variations, and random or irregular variations.
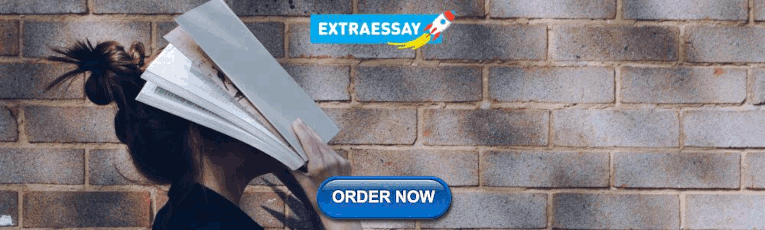
Mathematical model of time series
There are two types of models in time series which are generally accepted as good approximations to the time relationship among the components of the observed data. They are the additive and multiplicative models and are the most commonly assumed relationship between time series and its elements.
Additive model
This assumes that the value of composite series is the sum of the four components, that is
where Y—original, T—Values of the secular trend, S—the value of the seasonal component, C—the value of the irregular component, and I—the value of the irregular component.
Multiplicative model
assumes that the value of composite series is the product of four component values, that is \(E({Y}_{t })\) = constant for all t and \(Var({Y}_{t })\) = constant for all t.
Due to the non-stationary nature of most business and economic time series, it is required that stationarity be achieved before building any model. We can differentiate the data.
Generally, the multiplicative model has been considered the standard conventional model for the analysis of time series.
Measurement of trend
The following are the three methods that are generally used for the study and measurement of the trend component in a time series. That is the Freehand method, the Moving average method, and the Method of least square. In this research work, the method of least-squares was used to estimate the trend value of time series by determining the equation for the best time of fit and also use it to determine whether there will be an increase or decrease in the observed data by predicting for the future occurrence. The study used the Box-Jenkins method to derive models for forecasting these data. This method is preferred because of its high accuracy in forecasting data, especially within a short to medium-term period. Its model simplicity gives it an advantage of cost and response time because the high cost is required to run and set up complex models [ 14 ].
Model building
In theory, Auto-regressive Integrated Moving Averages ARIMA Models (Box Jenkins) are the most universal class of models for forecasting time series data. As proposed by Box and Jenkins, that in general, forecasting based on ARIMA models comprises three different steps: Model Identification, Parameter estimation, and Diagnostic checking. Until a desirable model for the data is identified, the three steps have been repeated [ 15 ].
Stationarity
A time series is stationary if there is no systematic change in mean (no trend), variances and strictly periodic variations have been removed. In other words, a time series is said to be stationary if:
differenced data will contain one less point than the original. For non-Constance variance, taking the logarithm or square root will stabilize the variance. For non-seasonal data, first-order differencing is usually sufficient to attain apparently \(\left({\mathrm{x}}_{1 }\right), \dots \dots ,\left({\mathrm{x}}_{\mathrm{n }}\right)\) by
Occasionally, second-order differencing is required using the operator Δ 2 where
Hence the number of times that the original series is differenced to achieve stationarity is the order of homogeneity.
Differencing
This is a special type of filtering which is particularly useful moving a trend. This is achieved by subtracting each data in a series from its predecessor. For non-seasonal data, first-order differencing is sufficient to obtain apparent stationarity. The concept of backshift operator helps to understand and express differenced ARIMA models.
Auto-regressive integrated moving average (ARIMA(p,d,q))
ARIMA ( \(p, d, q\) ) model was first introduced by Box and Jenkin in 1976, it can be used for forecasting the non-seasonal stationary time-series data [ 16 ]. An ARIMA model is characterized by 3 terms: \(p, d, q\) where \(p\) is the order of the Auto-Regression (AR) term, \(q\) is the order of the Moving Average (MA) term, \(d\) is the order of differencing required to make the time-series stationery. Auto-Regression is nothing but the regression of the variable against itself to forecast the variable of interest. It correlates the pattern of the one-time period to its previous periods. MA is a regression-like model that uses the errors associated with the forecast at a previous time-step to forecast a variable at a later time step. The following are the generalized equations of \({p}\text{th}\) order AR model and \({q}\text{th}\) order MA model.
ARIMA models are built upon incorporating the AR model, integration (I), and the MA model. The integration (I) is the reverse process of differencing to generate the forecast. The generalized ARIMA model is mathematically represented as
where C is an intercept, \({\mathrm{\varphi }}_{\mathrm{i}}\) (i = 1, 2... p) is auto-regressive model parameters, \({\uptheta }_{\mathrm{i}}\) (i = 1, 2... p) is moving average model parameters, \({y}_{t}\) is current time-series value, \({y}_{t-1}\) , \({y}_{t-2}\) , …, \({y}_{t-p}\) is past values and \({E}_{t}\) is a random error or residual term for the \({t}\text{th}\) day and it is given by the following equation:
Box and Jenkins method
The Box-Jenkins model is efficient for analyzing data and forecasting by many authors [ 17 ]. This method of forecasting implements knowledge of autocorrelation analysis based on autoregressive integrated moving average models. The procedure is of four main stages namely: Identification, Estimation, Diagnostics Checking, and Forecasting.
Identification
The first step in developing an ARIMA model is to determine if the series is stationary. If the model is found to be non-stationary, stationarity could be achieved mostly by differencing the series or going for the dickey fuller test. Stationarity could also be achieved by some mode of transformation like the log transformation. Once stationarity has been achieved, the next step is to determine the orders of the autoregressive (AR) and moving average (MA) terms using the Auto Correlation Function (ACF) and Partial Auto Correlation Function (PACF). stationarity so that the new series \(({Y}_{1 }, \dots \dots , {Y}_{n })\) .
Once the preliminary model is chosen, the estimation stage begins. The purpose of estimation is to find the parameter estimates that minimize the mean square error. Two approaches are used and these include the nonlinear least squares and maximum likelihood estimates. In this method, the R statistical package was used in the estimation.
Diagnostics checking
Residuals from the model are examined to ensure that the model is adequate (random). The following diagnostics are made: Time plot of the residuals Plot of the residual ACF Normal Quantile Quantile (QQ) Plot.
Forecasting
When a satisfactory ARIMA model is adequate, then we proceed to forecast or predict for a period or several periods ahead. However, chances of forecast errors are inevitable as the period advances.
The current study applied time series analysis to model the patterns of the total number of road traffic accidents that lead to injury, total fatal road traffic accidents, and the total number of traffic accidents that occurred from September 2013 to May 2017 in the Amhara region, Ethiopia.
Descriptive statistics
Over the 45 months observation (from Sept. 3013 to May 2017), a total of 3385 road traffic accidents were reported. The summary statistics for counts of Total Injuries, Fatal accidents, and Total number of RTA during the study period are presented below.
As it is shown in Table 1 above, the average number of total accidents per month was around 76. The maximum was 108 and the minimum was 43 which were recorded in October 2014 and March 2013 respectively. It was also shown that an average of 14 fatal accidents has been observed per month over the study period.
Table 2 above cross-tabulated the total number of road traffic accidents based on age groups for drivers, pedestrians, and passengers. Among drivers, a considerably large number of accidents were caused by drivers between the age group 18–30 years. Regarding pedestrians and passengers, although the number of victims was relatively high for the age group 18–30, the problem is common to individuals of all age groups.
As shown in Table 3 , it was tried to present the observed road traffic accidents by days of the week during the study period. According to the observed data road traffic accidents are more prevalent on Saturday, Thursday, and Tuesday relative to other days of the week in the Amhara region. It may be related to the market days which are common in the region. So, attention should be given to the mentioned days of the week so that a significant number of accidents can be minimized.
Exploratory analysis
Time series plots of the injury cases, fatal RTA cases, and total RTA cases are shown below.
We can’t infer from the time series plot shown in Fig. 1 that the trend of the data since the graphs didn’t show clear patterns. There is a need for further analysis to find out the exact non-stationary and seasonality in the data. As shown from the time series decomposition plots (Figs. 2 , 3 , 4 ), the observed injury RTA cases show an overall decreasing trend whereas fatal RTA cases and total RTA cases have an overall increasing trend.
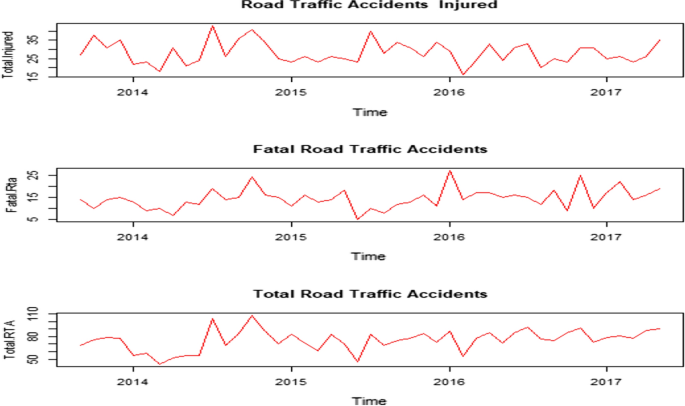
Time series plot of monthly reported Injury, fatal and total road traffic accidents in Amhara Region (September 2013 to May 2017)
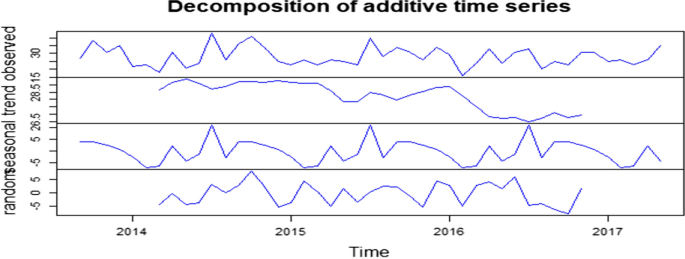
Time series decomposition of injury RTA data
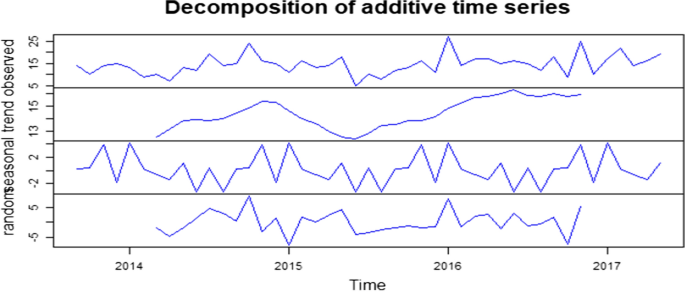
Time series decomposition of fatal RTA data
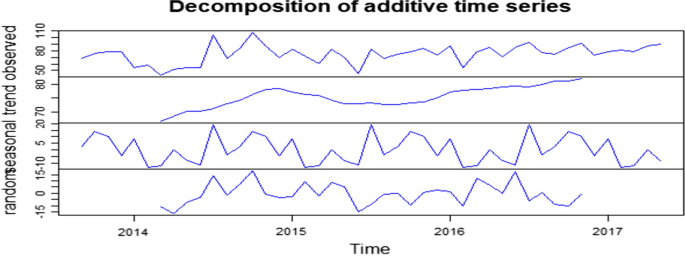
Time series decomposition of Total RTA data
Test of stationarity
To test the stationarity of the data Augmented Dickey-Fuller test for stationarity was used. According to the ADF-test output in Table 4 , fatal RTA and Total RTA data are not stationary. Only the injury RTA data is shown to be stationary since it has a p-value less than 0.05. Therefore, according to the Adf-tets statistics shown in Table 5 the fatal RTA and Total RTA data should be differenced to achieve stationarity.
After applying the first-order differencing on fatal RTA and total RTA cases, it can be shown that both p-values of Adf-Test are small (less than 0.05) which suggests the data is stationary and doesn’t need further differencing.
Fitting time series models
Once the data is ready and satisfies all the assumptions of modeling, the next step is to select the appropriate ARIMA model i.e., to determine the order of the model to be fitted. This task can be done by examining two important plots called autocorrelation function (ACF) and partial autocorrelation (PACF) plots of the stationary time series. For the current data, the PACF and ACF plots are shown below.
Model selection
The model selection was done by examining the ACF and PACF plots (Fig. 5 ). The AIC and BIC values are also used to choose the best model. These criteria are closely related and can be interpreted as an estimate of how much information would be lost if a given model is chosen. ARIMA [2] (1,0,0) [12], ARIMA (2,0,0) and ARIMA (2,0,0) (1,1,0) [12] are chosen as the best models for Total injury, Fatal RTAs and Total RTAs series respectively. As it is shown below in Table 6 , the models chosen above had the lowest AIC and BIC values compared with the other possible models.
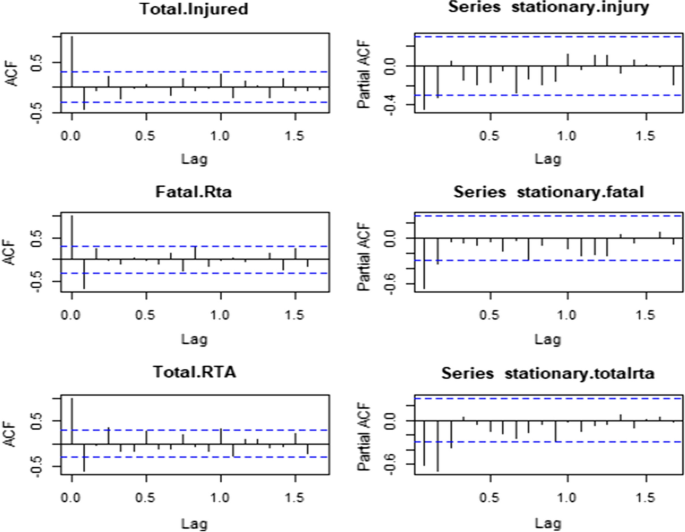
Autocorrelation and Partial autocorrelation function plots of Injury, Fatal and Total RTA
Model diagnostics
Now before using the fitted model for forecasting, one should examine the adequacy of the models. This can be commonly checked by examining ACF and PACF plots for model residuals. If model order parameters and structure are correctly specified, we would expect no significant autocorrelations present.
All standardized residuals plot shows no obvious trend and pattern and looks like an independent and identical distribution. Similarly, all plots of ACF residuals of the diagnostics show no evidence of significant correlation in the residuals. Most of the residuals are located on the straight line except for a few outliers deviating from the normality. In conclusion, the residuals ACF (Fig. 6 ) and normal Q-Q plots (Fig. 7 ) both exhibited a white noise pattern and the goodness of the fitted model.
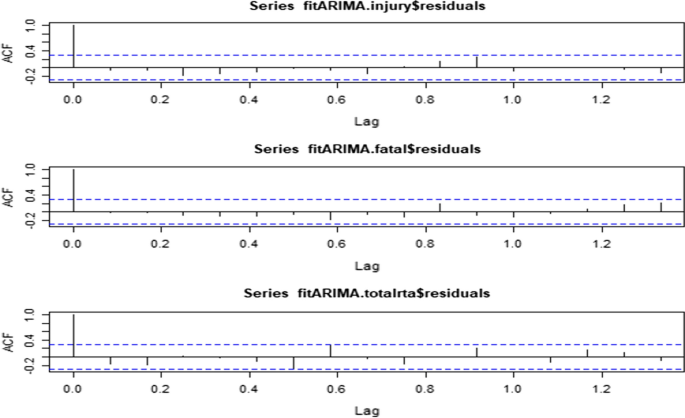
Diagnostics of residuals for ARIMA of injury, fatal, and total RTA data
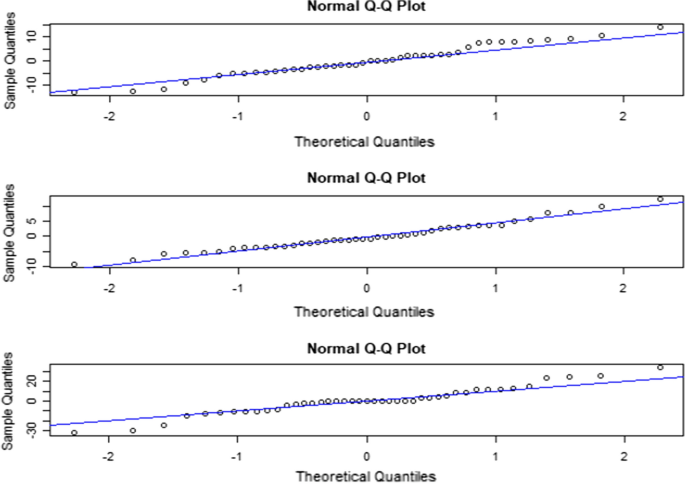
Normal Q-Q plot of residuals for ARIMA of injury, fatal and total RTA data
The model was fitted for 48 months period after the diagnostic test. The predicted counts of injury and total road traffic accidents for the mentiond forecast period are presented in Table 7 . In addition, graph which gives a pictorial view of the observed series, its forecast, and confidence intervals of the forecasts are provided below.
As it can be shown from the forecast plots (Fig. 8 ) as well as the table of predicted road traffic accidents, the trends of injury road traffic cases and total traffic cases generally exhibit a similar pattern with the observed data i.e., the situation keeps what it was before. This result signifies the cases will remain unchanged for the coming 48 months unless new or improved road safety measures are taken. It is in line with what we observe in our day-to-day life that road traffic accidents are common cases here and there.
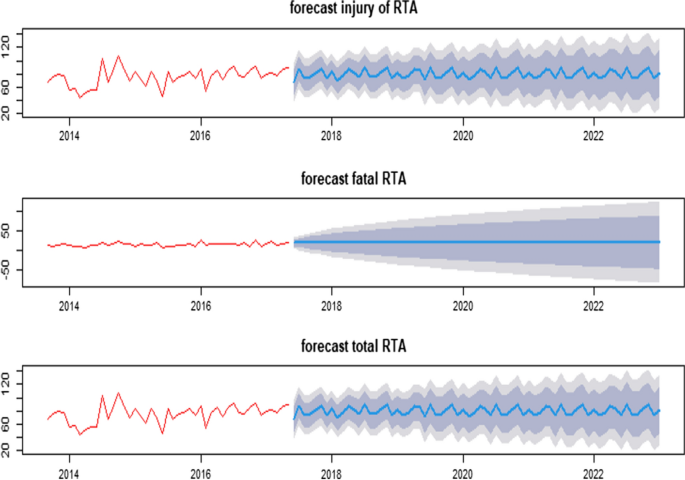
Graph of injury, fatal, and total RTA cases, its forecasts, and confidence intervals
Nowadays, the increment of a road traffic accident at a disturbing rate is a major concern in Ethiopia. The current study focuses on the Amhara region which has the highest share of road traffic accidents in the country. The most widely used conventional method of time series is known as Autoregressive Integrated Moving Average (ARIMA) model, also known as the Box-Jenkins method was applied to monthly reported road traffic accident data in four randomly selected zones of the Amhara region from September 2013 to May 2017 to determine patterns of road traffic accident cases in the region. After identifying various tentative models, the appropriate models for the accident cases (Total injury, fatal and total road traffic accidents) are as follows. ARIMA (2,0,0)(1,0,0)[12], ARIMA(2,0,0) and ARIMA(2,0,0)(1,1,0)[12] are chosen models to model the total injury cases, fatal cases and total RTA cases using the data from September 2013 to May 2017. The adequacies of the model were tested by analyzing standard residuals in different forms. 48 months of forecasts were provided for injury cases, fatal cases and total cases would continue in a non-decreasing trend. This study provides reliable and genuine information that could be useful for determining road accident rates in the region supported by [ 18 ]. This study would also be used for providing important information to increase the level of awareness among stakeholders concerning road safety since the problem has become a growing issue in the country as a whole. Most importantly, this study would provide expected benefit to the road users, Road Safety Authority, researchers, and other stakeholders in understanding the rate of the cases of a road accident.
Based on the results of this study, the rate of road accidents is expected to remain constant for at least the next 4 years. It was found that the incidence of road accidents in the Amhara region can be fitted ARIMA(2,0,0)(1,0,0)12 and ARIMA(2,0,0)(1,1,0) 12 model. The findings of this study draw attention to the importance of implementing key road safety measures to change the existing threat of road accidents in the Amhara region. Therefore, improved and better policies of the National road safety authority should be introduced with much emphasis on publication and education to ensure a maximum reduction in Road accident crashes.
Availability of data and materials
Not applicable.
Abbreviations
Partial Auto Correlation Function
Road Traffic Accidents
Gross Domestic Product
Amhara National Regional State
Auto-Regressive Integrated Moving Average
Auto Correlation Function
Auto-Regressive
Moving Average
Akaike information criterion
Bayesian information criterion
Seasonal Auto-Regressive
Farag SG, Hashim IH, El-Hamrawy SAJIJTTE. Analysis and assessment of accident characteristics: Case study of Dhofar Governorate, Sultanate of Oman. Int J Traffic Trans Eng. 2014;3:189–98.
Google Scholar
Global status report on road safety. Accident: WHO; 2018. https://knoema.com/WHOGSRS2019Jan/global-status-report-on-road-safety-2018?country=1001720-ethiopia .
Bjerre J, Kirkebjerg PG, Larsen LB. Prevention of traffic deaths in accidents involving motor vehicles. Ugeskr Laeger. 2006;168(18):1764–8.
Smart RG, Mann RE. Deaths and injuries from road rage: cases in Canadian newspapers. CMAJ. 2002;167(7):761–2.
Peden M. Global collaboration on road traffic injury prevention. Int J Injury Control Safety Promotion. 2005;12(2):85–91.
Article Google Scholar
Bishai D, Quresh A, James P, Ghaffar A. National road casualties and economic development. Health Econ. 2006;15(1):65–81.
Astrom J, Kent M, Jovin R. Signatures of four generations of road safety planning in Nairobi City, Kenya. J Eastern Afr Res Dev. 2006;20:186–201.
World Health Organization. Road traffic injuries. 2020. https://www.who.int/news-room/fact-sheets/detail/road-traffic-injuries .
Mariam DH. Road traffic accident: a major public health problem in Ethiopia. Ethiopian J Health Dev. 2014;28(1):1–2.
Persson A. Road traffic accidents in Ethiopia: magnitude, causes, and possible interventions. Adv Transportation Studies. 2008;15:5–16.
Organization WH. Global status report on road safety 2015. World Health Organization; 2015.
Association EE. Energy, safety, and environment and transport services in Ethiopia. Research Brief. 2012(2).
Johansson P. Speed limitation and motorway casualties: a time series count data regression approach. Accid Anal Prev. 1996;28(1):73–87.
Nihan NL, Holmesland KO. Use of the Box and Jenkins time series technique in traffic forecasting. Transportation. 1980;9(2):125–43.
Box GE, Jenkins GM, MacGregor JF. Some recent advances in forecasting and control. J R Stat Soc Ser C. 1974;23(2):158–79.
MathSciNet Google Scholar
Box GE, Jenkins GM. Time series analysis: forecasting and control San Francisco. Calif: Holden-Day; 1976.
MATH Google Scholar
Twenefour FB, Ayitey E, Kangah J, Brew L. Time series analysis of road traffic accidents in Ghana. Asian J Probab Stat. 2021. https://doi.org/10.9734/AJPAS/2021/v11i230262 .
Al-Ghamdi AS. Time series forecasts for traffic accidents, injuries, and fatalities in Saudi Arabia. J King Saud Univ Eng Sci. 1995;7(2):199–217.
Download references
Acknowledgements
The author would like to acknowledge the Amhara region police commission traffic departments, transport, and communication bureau to conduct this study and to publish this paper.
No funding was received for this study.
Author information
Authors and affiliations.
Bahir Dar Institute of Technology, Bahir Dar University, Bahir Dar, Ethiopia
Kidane Alemtsega Getahun
You can also search for this author in PubMed Google Scholar
Contributions
KAG was responsible for the formulation of the methodology. KAG made substantial contributions to the conception and design of the study, analyzed and interpreted the data, and was a major contributor in writing the manuscript. KAG drafted the manuscript. All authors read and approved the final manuscript.
Corresponding author
Correspondence to Kidane Alemtsega Getahun .
Ethics declarations
Ethics approval and consent to participate, consent for publication, competing interests.
The authors declare that they have no competing interests.
Additional information
Publisher's note.
Springer Nature remains neutral with regard to jurisdictional claims in published maps and institutional affiliations.
Rights and permissions
Open Access This article is licensed under a Creative Commons Attribution 4.0 International License, which permits use, sharing, adaptation, distribution and reproduction in any medium or format, as long as you give appropriate credit to the original author(s) and the source, provide a link to the Creative Commons licence, and indicate if changes were made. The images or other third party material in this article are included in the article's Creative Commons licence, unless indicated otherwise in a credit line to the material. If material is not included in the article's Creative Commons licence and your intended use is not permitted by statutory regulation or exceeds the permitted use, you will need to obtain permission directly from the copyright holder. To view a copy of this licence, visit http://creativecommons.org/licenses/by/4.0/ .
Reprints and permissions
About this article
Getahun, K.A. Time series modeling of road traffic accidents in Amhara Region. J Big Data 8 , 102 (2021). https://doi.org/10.1186/s40537-021-00493-z
Download citation
Received : 04 March 2021
Accepted : 03 July 2021
Published : 19 July 2021
DOI : https://doi.org/10.1186/s40537-021-00493-z
Share this article
Anyone you share the following link with will be able to read this content:
Sorry, a shareable link is not currently available for this article.
Provided by the Springer Nature SharedIt content-sharing initiative
- Road traffic
- Fatal injury
- Time series
- Amhara Region
- Find a journal
- Publish with us
- Track your research
Academia.edu no longer supports Internet Explorer.
To browse Academia.edu and the wider internet faster and more securely, please take a few seconds to upgrade your browser .
Enter the email address you signed up with and we'll email you a reset link.
- We're Hiring!
- Help Center
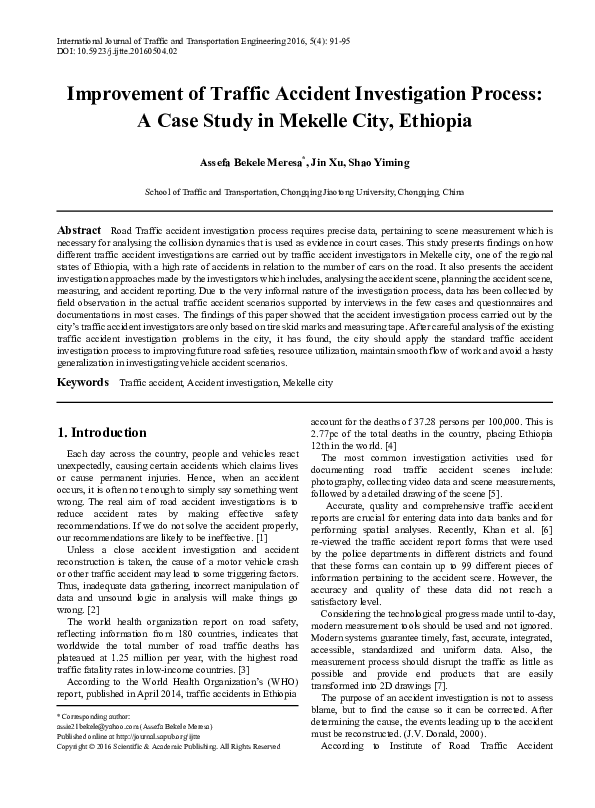
Improvement of Traffic Accident Investigation Process: A Case Study in Mekelle City, Ethiopia

Road Traffic accident investigation process requires precise data, pertaining to scene measurement which is necessary for analysing the collision dynamics that is used as evidence in court cases. This study presents findings on how different traffic accident investigations are carried out by traffic accident investigators in Mekelle city, one of the regional states of Ethiopia, with a high rate of accidents in relation to the number of cars on the road. It also presents the accident investigation approaches made by the investigators which includes, analysing the accident scene, planning the accident scene, measuring, and accident reporting. Due to the very informal nature of the investigation process, data has been collected by field observation in the actual traffic accident scenarios supported by interviews in the few cases and questionnaires and documentations in most cases. The findings of this paper showed that the accident investigation process carried out by the city's traffic accident investigators are only based on tire skid marks and measuring tape. After careful analysis of the existing traffic accident investigation problems in the city, it has found, the city should apply the standard traffic accident investigation process to improving future road safeties, resource utilization, maintain smooth flow of work and avoid a hasty generalization in investigating vehicle accident scenarios.
Related Papers
Amit Vashisth
Traffic Accidents are significantly increasing on Indian roads so as in Panipat District of Haryana state. It is one of the 22 districts of Haryana in north India. As per the census data 2011 it has a population of more than 1 million which is close to the population of Bahrain or New Hampshire State. In this article, we try to identify the traffic accident investigation process of the Panipat district and find out what are the loopholes and suggested possible solutions to the problems. Traffic policemen who are actually on duty as an accident Investigator in the district were interviewed revealed some surprising facts such as involvement of bribes in the traffic accident investigation process and different questions were answered such as, Are they actually experienced in this field or not?, Do they have enough educational background to analyze different aspect and causes of accidents?, how frequently they use accident data form prescribed by IRC (Indian Roads Congress) i.e. IRC:53-2012. I. INTRODUCTION Organize traffic accident investigation was not known in the past but introduction of automobile on the roads results in high death rate on the roads which leads to motivate concerned authority to take desired steps to minimize danger of traffic accidents [6]. There is a loss of indirect costs due to congestion and delays results from blockage of lane while investigating road crashes. In most of the cases vehicles involved in the crash can be moved and roads can be cleared for traffic movement but this does not happen because there is a need to conduct detailed crash investigation [5]. Accident variables such as different road characters, accident severity and composition of road surface were reported less seriously by the police personnel. Reports provided by the police personnel regarding human factors, vehicle and road defects and environmental conditions were not recorded [2]. On-site data collection in the form of photography, videography, measurements, and detailed drawing are considered as the most common documenting method of road traffic accident investigation [3]. Traffic accident investigation generally starts with Inspection of accident site followed by the recording of pieces of evidence left by the vehicle after an accident such as impact point, the final position of the vehicle skid, scrub and gouge marks [4]. Different solutions were suggested by [1] such as minimum qualification of the investigator should be set high also proper training should be given to police personnel collecting data, specifically for traffic accident investigation to update their knowledge and skill to latest technologies. Every accident shows a different pattern and needs to be addressed differently each time during an investigation. Traffic accident investigation process needs to be efficient so as to gather actual information about road accidents and how it affects the nearby environment. Accidents need not just to be investigated properly but measures to reduce accident must be specified on the basis of accident data collected in past. The major problem here is not just the inadequacy of the resources during data collection for road accidents but untrained data collecting personnel also. As per the accident report 2015 released by MORTH (Ministry of road transport and Highways), the major factor responsible for almost 80% of the road accidents is drivers' fault. But this is not possible each and every time that we make drivers responsible for a road accident. Lack of detailed knowledge and proper training specifically for accident data collection techniques for the policemen leads to the prejudice concluded reason for the accident. Data collection system need to be revamping time to time and proper training should be there for traffic accident data collection system in the states. Factors other than drunk and drive, over speeding, traffic lights violations etc. such as the defect in geometric design, proper lighting, road condition etc. also need to be addressed in the traffic accident investigation process.
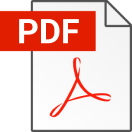
G. Andemichael
Revue Européenne de Psychologie Appliquée/European Review of Applied Psychology
Kai Hakkarainen
Damian Schofield
Court environments, which have been one of the last bastions of the oral tradition, are slowly transforming into cinematic display environments. The persuasive oral rhetoric of lawyers is increasingly being replaced by compelling visual media displays presenting a range of digital evidence in a convincing and credible manner. Advances in media formats and devices have made available new mechanisms for presenting evidence in court. Digital visual evidence presentation systems (including digital displays, computer-generated graphical presentations, animated graphics and immersive virtual environment technology) have already been used in many jurisdictions. There are a number of fundamental implications inherent in the shift from oral to visual mediation, and a number of facets of this modern evidence presentation technology need to be investigated and analysed. At first glance, these computer-generated graphical reconstructions may be seen as potentially useful in any court, and they are often treated like any other form of digital evidence regarding their admissibility. However, perhaps this specific form of digital media warrants special care and attention due to its inherently persuasive nature, and the undue reliance that the viewer may place on evidence presented through a (potentially photorealistic) visualisation medium.
Jesper Sandin
There is a need for increased knowledge about causes of motor-vehicle crashes and their prevention. Multidisciplinary in-depth case studies can provide detailed causation data that is otherwise unattainable. Such data might allow the formulation of hypotheses of causes and causal relationships for further study. By converting the data into causation charts that are aggregated, common causation patterns would give greater weight to such hypotheses. However the charts must first be compiled by means of a systematic analysis method, which requires three parts; a model, a classification scheme and a classification method. Four general accident models were evaluated and found inadequate to form the basis for a causation analysis method. This was primarily because the models in practice treat road-users, vehicles and traffic environment as separate components, but also due to the focus on events immediately prior to the crash and either static, sequential, or absent modelling of interacti...
Abstract Courtroom environments, which have been one of the last bastions of the oral tradition, are slowly morphing into cinematic display environments (Heintz, 2002). The persuasive oral rhetoric of lawyers is increasingly being replaced by compelling visual media displays presenting a range of digital evidence in a convincing and credible manner.
Visualisation is becoming increasingly important for understanding information, such as investigative data (for example: computing, medical and crime scene evidence) and analysis (for example: network capability assessment, data file reconstruction and planning scenarios). Investigative data visualisation is used to reconstruct a scene or item and is used to assist the viewer (who may well be a member of the general public with little or no understanding of the subject matter) to understand what is being presented. Analysis visualisations, on the other hand, are usually developed to review data, information and assess competing scenario hypotheses for those who usually have an understanding of the subject matter. Visualisation represents information that has been digitally recorded (for example: pictures, video and sound), hand written and/or spoken data, to show what may have, could have, did happen or is believed to have happened. That is why visualising data is an important development in the analysis and investigation realms, as visualisation explores the accuracies, inconsistencies and discrepancies of the collected data and information. This paper presents introduces some of the various graphical techniques and technology used to display digital information in a courtroom. The advantages and disadvantages involved in the implementation of this technology are also discussed. This paper is part one of a two part series that aims to describe the use of, and provide guidelines for, the use of graphical displays in courtrooms.
Investigation and Reporting of Incidents and …
Henning Boje Andersen
Transportation Research Part F: Traffic Psychology and Behaviour
Wondwesen Girma Mamo
RELATED PAPERS
Electronic Evidence (3rd Edition)
Jesper Sandin , Henriette Warner
Assessment of Road Traffic Crash Data Collection and Management System of Ethiopia
Zoe Sofoulis , Sarah Redshaw
Bogdan Chivoiu
Prehospital and Disaster Medicine
Julia Irwin , Ian Faulks
Kübra Mercan
Accident Analysis & Prevention
Caroline DECK , Yong Peng , Jikuang Yang
Jikuang Yang
Daniela M Romano
Danielle Tyson
Sylvia Schick
Ilit Oppenheim , Frank Lai , Simon Enjalbert , Yvonne Barnard
Guy Stanford
NANCY ABIRA
clare Lawton
Mostapha Al-Dah
Jez M Noond , Damian Schofield , Martin Evison
Mahdi Pour-Rouholamin
Mike Dilich
Shakil Rifaat , Md. Mosabbir Pasha , Hasan Shovon
Jennie Gallimore
Taimi Immanuel
FORUM FOR ENVIRONMENT ADDIS ABABA, …
Mellese Damtie
Steven Trawley
John Richardson
… Research in Road …
Michael Gormley
Emebet Hailemichael
Workshop on the Investigation and Reporting of Incidents and Accidents
Damian Schofield , Jez M Noond
BMC Public Health
fekede asefa
Consuelo Rodriguez , Pier Paolo Mariani
Ed McCarron
- We're Hiring!
- Help Center
- Find new research papers in:
- Health Sciences
- Earth Sciences
- Cognitive Science
- Mathematics
- Computer Science
- Academia ©2024
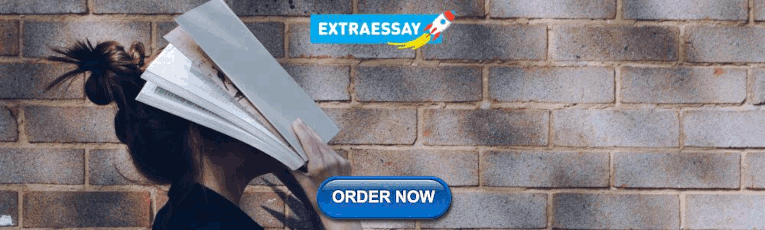
COMMENTS
Ta ble-1.8: V ehicle Factors Th at Causes to Traffic Accidents Years <1 Year 1-2 Years 2-5 Years 5-10 Years >10 Years Unknown 2005 183 424 2292 3323 2417 1904
Investigation on Road Traffic Accident Factors and Possible ...
As thesis research advisors, we her by certify that we have read the thesis prepared by Hirko ... According to WHO, 1.35 million people die each year as a result of road traffic accidents globally. Ethiopia is one of the developing countries and at least 114 people die for every 10,000 vehicle accidents annually. Moreover, road traffic accident ...
The study was conducted to identify the major causes of road traffic accident specifically focusing on Addis-Adama expresssway, to project causes of traffic accident on the expressway and propose ...
Jimma University
The variation on road traffic accident, road network coverage in km and motorized vehicle between commencement of study period (2007/08) or Ethiopia millennium (2000E.C) with end of the study ...
This research concerns on Analyzing Road Traffic Accident in Ethiopia from 2007/08-2017/18. The main objective of the study was to investigate the growth rate of road traffic accident, road network coverage and motorized vehicle, and relationship between them ... Road Traffic Accident in Ethiopia from 2007/08-2017/18 (Since Ethiopia Millennium)
The traffic police report indicate that Ethiopia's road traffic accident meets criteria number one and two that spontaneously classifies its road accidents as disaster risk. The Global status report on road safety reveals that the number of annual road traffic deaths reached 1.35 Million in 2016 (WHO, 2018).
There were a total of 157,326 reported road traffic accidents across Ethiopia in the 2014/2015 fiscal year, and this number increased to 183,472 in the 2018/2019 fiscal year ... Section 2 presents a review of previous research on road traffic accident safety and its spatial analysis techniques. In Section 3, the authors discuss the data used in ...
2023-5301-AJS-MAT - 27 APR 2023 4 1 Objective of the Study 2 3 General Objective 4 The main objective of this research work is to model road traffic accident by 5 using time series techniques. 6 7 Specific Objective 8 The specific objectives of this study include: 9 Assessing the trend of road traffic accident in West Arsi Zone, Ethiopia. 10 Forecasting future Number of road traffic accident ...
road traffic accident every year. Road traffic accident is the 8th leading cause of death among people of all ages while it is the leading cause of death for age group of 5-29 years. This disastrous problem is worsening with the increasing number of vehicles. It is estimated that road traffic accident will be a cause for 13 million deaths and
In the last Ethiopian fiscal year (2007/8), for example, police reported 15,086 accidents which caused the losses of 2,161 lives. Up to 2005/6, traffic accidents and fatalities increased at 17 % and 10 % per year respectively, but in the recent couple of years there is a sudden drop.
The research was based on the collected data from Traffic Police Office in year 2010/11 to year 2014/15. The data from the questionnaire survey and field observations has been carried out to realize the data performed from traffic police, which was used as complement to the traffic accidents analysis for the purpose of this research work.
A study of the effects of road and traffic factors on traffic accidents in Ethiopia is presented in this thesis. Accident data indicate that road safety is Ethiopia is a serious problem. Traffic accident fatalities have progressively increased with growth in the population and the number of vehicles. Pedestrian fatalities comprise 56% of the ...
From the 2007/08 to the 2017/18 fiscal year in Ethiopia, in the past eleven years, the growth of road traffic accidents was 9.16% annually, on average [10]. Respectively; the growth rate of road ...
Magnitude and determinants of road traffic accidents in Northern Ethiopia: a cross-sectional study ... Road traffic accident (RTA) is an accident, which occurs or originates on a way or street open to public traffic; resulting in one or more persons being killed or injured, and at least one moving vehicle is involved. ... Clifford J, THEOBALD C ...
Road traffic accidents (RTA) are commonly encountered incidents that can cause injuries, death, and property damage to members of society. Ethiopia is one of the highest incident rates of road traffic accidents. Report of Transport and Communication from 2012 to 2014, shows an increment in the number of traffic accidents in Ethiopia. Amhara region accounted for 27.3% of the total road traffic ...
The variation on road traffic accident, road network coverage in km and motorized vehicle between commencement of study period (2007/08) or Ethiopia millennium (2000E.C) with end of the study ...
This is a research project conducted in 2011 by a group of graduate students at the University of College London, Development Planning Unit. It includes primary research in Mek'ele Ethiopia, and secondary research in London, England. It focuses on the links between transport infrastructure and poverty reduction in the peri-urban interface of ...
Homepage | UNECE
DOI: 10.11604/pamj.2021.38.190.11650 Corpus ID: 233915718; Road traffic accident and management outcome among in Adama Hospital Medical College, Central Ethiopia @article{Deresse2021RoadTA, title={Road traffic accident and management outcome among in Adama Hospital Medical College, Central Ethiopia}, author={Eskindir Deresse and Meyrema Abdo Komicha and Tesfaye Lema and Shemsedin Abdulkadir ...
In the present study, road traffic accident data of three years (2015-2017) were obtained from Hosanna Town Traffic Police Department, and Hosanna Town Transport Authority in Ethiopia.
Rate of Road Traffic Accident From the year 2007-2015 over 1,675 road traffic accidents occurred. Out of which, over 315 people were died, over 780 people were suffered with easy and series non-fatal accidents and a property lose which accounts over 10,876,512 Birr had occurred. Graph 1. reveals the road traffic accidents occurred from 2007-2015.