- Search Services Directory
- Transformation Leaders
- Innovation Experts
- HR, People & Culture
- Latest Articles
- Search Knowledge Base
- The Case For Digital Transformation
- Strategy & Innovation
- People & Change
- Customer Engagement
- Enabling Technologies
- Data & Analytics
- Cyber Security
- Executive Briefing Book Summaries
- Leading Digital Transformation: Podcast Series
- News In Digital: Archive
- Find new business opportunities
- Publish with us
- Meet The Authors
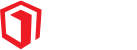
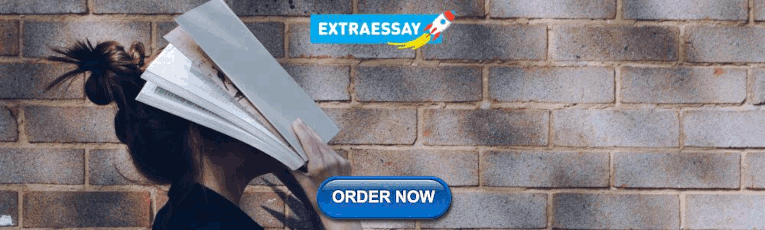
Renault – An industry 4.0 Case Study
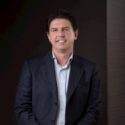
Digital Transformation Practitioner | Innovation Coach | Entrepreneurship | Exponential Technologies | Keynote Speaker
The Digital Economy and the rapid convergence of exponential technologies is forcing many companies to rethink their business models and this is true for automotive companies as well.
Technologies like the Internet of Things , Artificial Intelligence , Blockchain , Robotics or Augmented reality are all converging, and this provides both threats and opportunities for companies like Renault.
We are in an age where autonomous driving is turning from a moonshot a few years back to a reality that will soon hit our streets in most cities. These technology shifts and the convergence of them is also what is shaping the next industrial revolution, i.e. the Industry 4.0.
But not only that, consumer behaviors are changing as well. Vehicle ownership is less on the mind of Millennials that are accustomed to transportation on demand from the likes of Uber or Lyft.
These millennials are also more worried about the impact transportation can have on the environment, they want to see sustainability higher on the agenda for large corporates.
Renault recognizing many of these threats and opportunities decided to embark on a digital transformation journey that is supposed to take the company to new levels.
The Vision: Mobility, Electric Power and Autonomous Driving
It started in 2015, when, with the help of Management Consulting Firm BCG they decided to shape the strategic plan of Renault. The outcome of that effort led to the 2017-2022 strategic plan.
Increase in revenue and productivity are among the usual suspects, when it comes to objectives, but there was also a clear objective to continue strengthening their position in electric vehicles, becoming a more connected and efficient company, leveraging autonomous driving and thinking about new forms of mobility services.
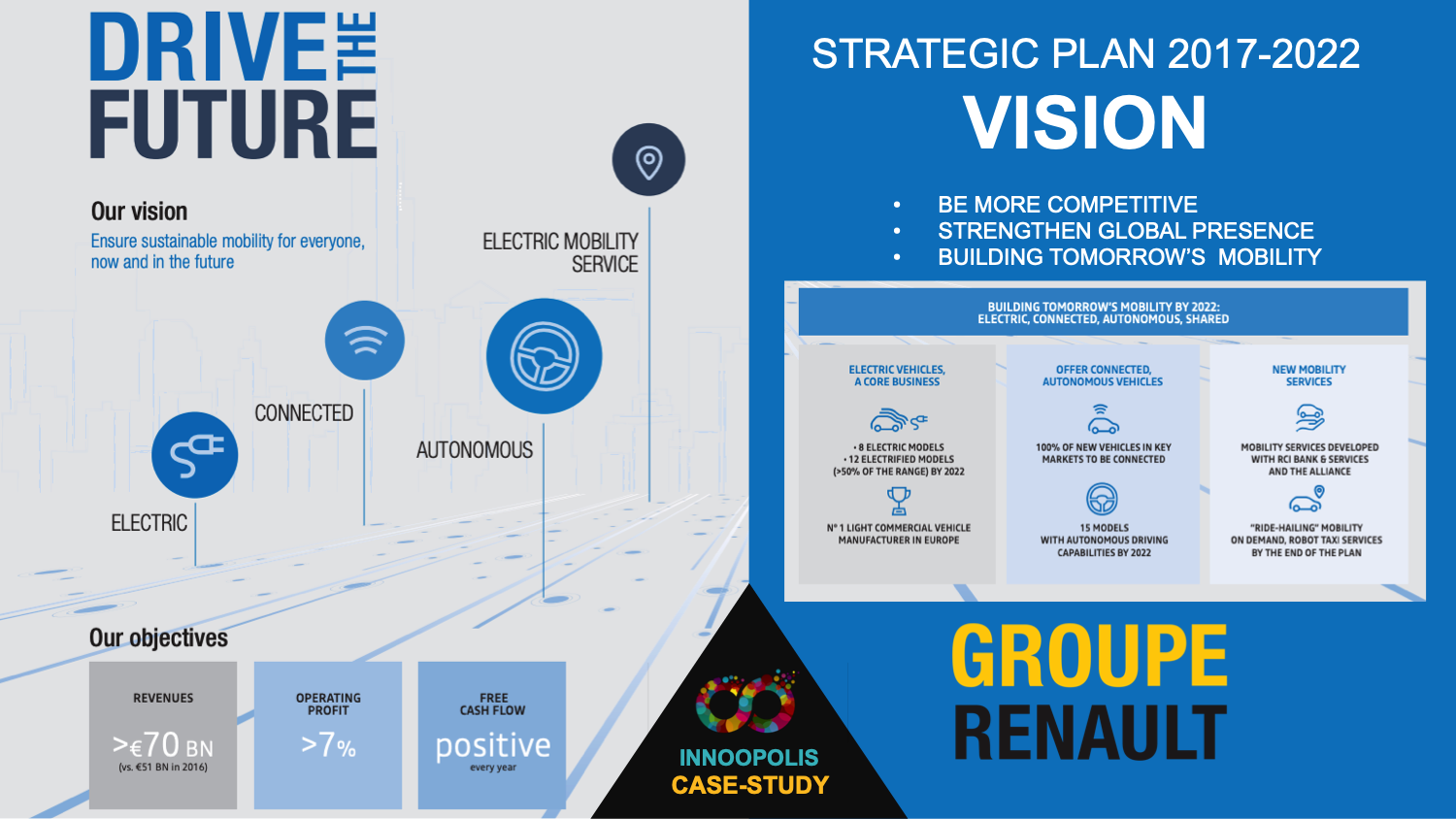
Transforming the company both Upstream and Downstream
In order to execute on this strategy, Renault needed first to understand the impact digital could have on their business. They looked at both upstream and downstream activities to find efficiencies and revenue opportunities.
They found out initially that the cost-saving and optimizations that could be achieved on the design and manufacturing side were close to 300M Euros/Year. Digital and in particular better usage of data, that was still scattered around 2000 legacy applications could provide them with better and faster decisions, increasing productivity.
Getting more of their production equipment connected and getting more of that data to unit managers in real-time was also essential to monetize data. Similarly, they found that becoming more data-driven could also help them to better manage the lifecycle of the customer relationship.
From better managing personalization needs to stronger engagement throughout the lifecycle of the customer relationship, they found out that here also they could make a 300M Euros / Year impact.
Having outlined the vision and developed a long list of use-cases that could impact the business both downstream and upstream, they came up with an overall impact of 600M Euros/Year. They decided then to quickly validate these initial assumptions by going into end-2-end pilots that could measure the real impact.
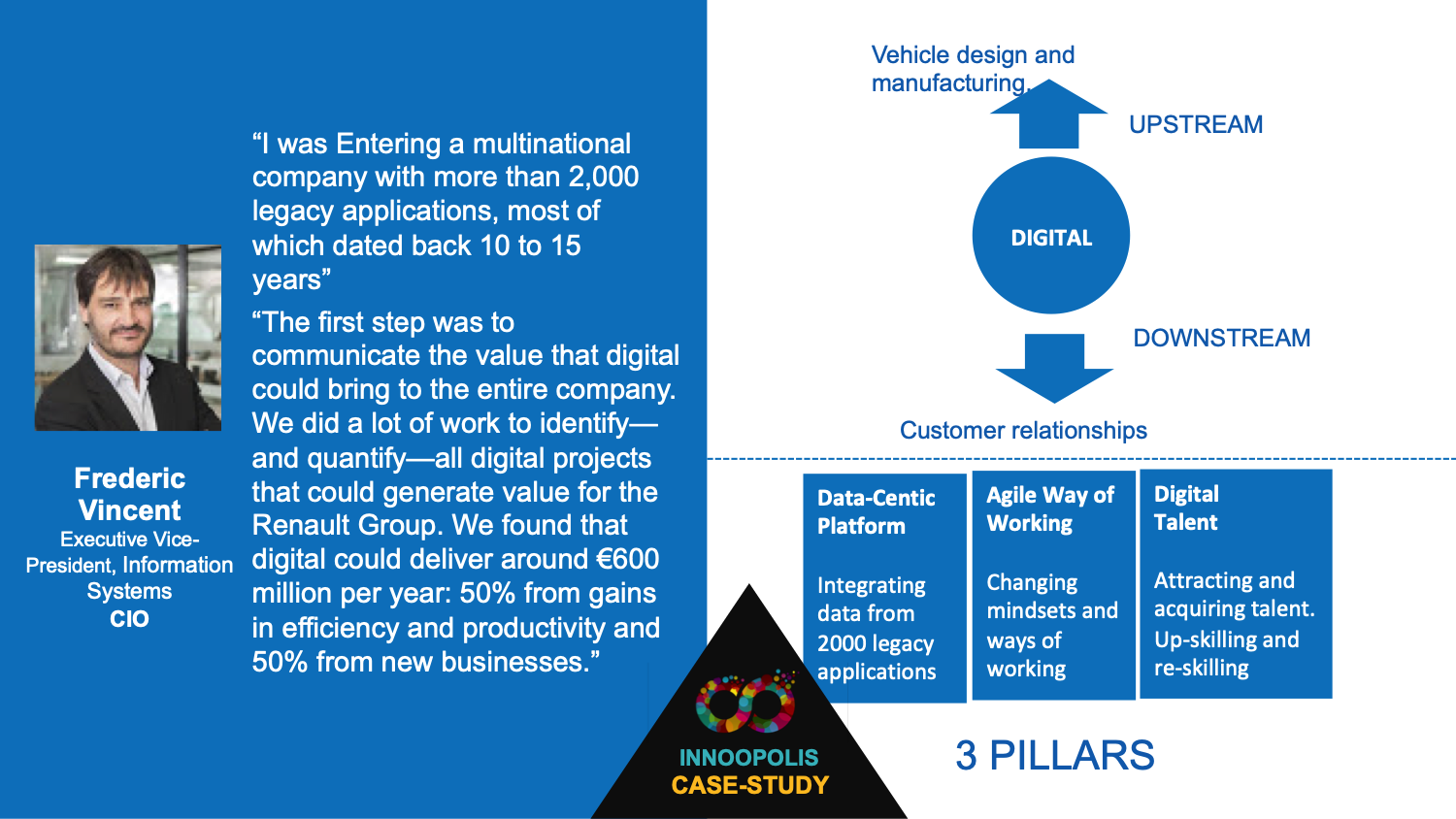
The problem they faced then was a real challenge, they hit a road block. As Frederic Vincent, the “CIO” of the company said in one interview “ the company suffered from lack of internal digital skills, Inconsistent digital methodologies across the company, Heavy governance and processes not adapted to the speed of digital, As well as rigid legacy IT systems .”
Basically for Management, the transformation was not moving fast enough, and the slow down was related to a lack of digital skills and rigid governance. It was essential to change all of this and do it quickly!
Renault Digital, A Digital Center of Excellence
On January 1, 2017, Renault Group decided to set up Renault Digital, an independent, but fully owned subsidiary with the sole objective of supporting the group to achieve its digital transformation objectives.
Renault Digital is the center of excellence that provides all the skills needed to execute digital, agile projects. It consists nowadays of more than 350 employees, with skills and knowledge in Agile methodologies, UX Design, DevOps and Data Science.
Renault Digital works a bit like a startup incubator and that is also the mentality it tries to instil to its staff, but also to the other persons working on their projects, a startup mindset.
For each project request that they get from the business units, they would set up an agile team, that is composed of subject matter experts from the business unit as well as specialists from the centre of excellence.
The projects are then incubated and go-through two distinct phases, an initial framing phase, which aims to narrow down the problem, gather insights and initial hypothesis. This is similar to what you would normally do in initial design thinking workshops, analyzing customer pain points and mapping customer journeys.
In the second phase, they move to an agile development model with the objective to develop the minimum viable product (MVP).
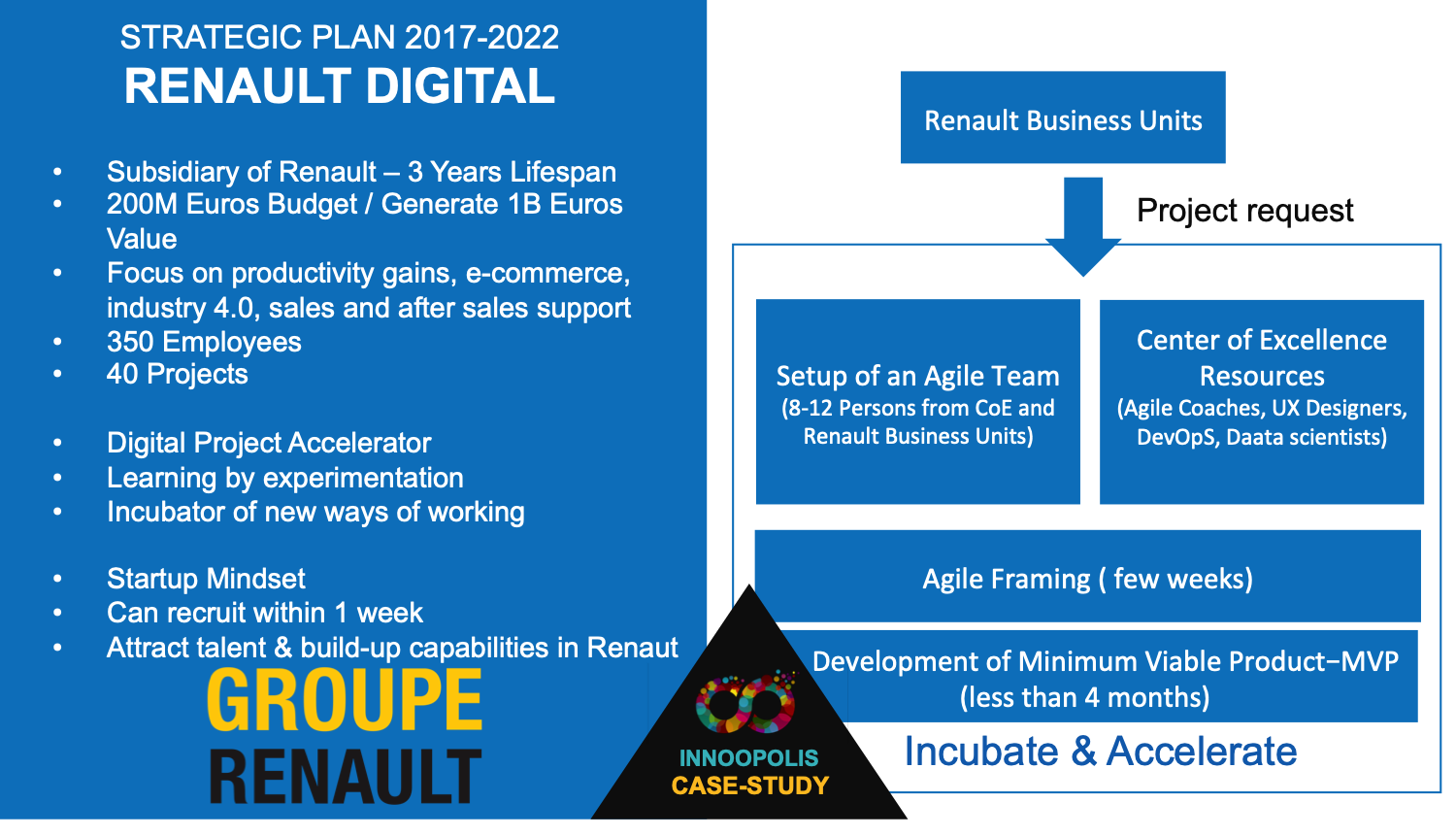
The good thing with this approach is that Renault Digital is really working as a startup and can easily and rapidly recruit new talent when needed.
Secondly, the persons from head office or other business units, that have been incubated for a period of 4-5 months, can now move back to their offices, with a new mindset, they effectively become change agents in the digital transformation.
Industry 4.0 Opportunities
Once Renault set up their Digital Unit, it was important to start building up success stories. Success stories are what helps you set the seeds for a change of culture and since Renault has a lot of staff working in their production plants it was only natural that they tried to find how digital could provide values to their employees.
Project CHUET was this first success story! Basically the challenge plant unit managers were facing back in the days is that they had to manage teams of 10-12 people, but needed to constantly run back and forth to their office computer to get the latest updates on how production had to be tuned, adjusted.
By putting Wi-Fi connectivity in their plants and by developing a bespoke mobile office solution for their unit managers they allowed them to save 45min/Day of ineffective time.
The productivity increase and job satisfaction were notable, now Unit Managers work around with their mobile office tablets and have access to real-time product data whenever they need it.
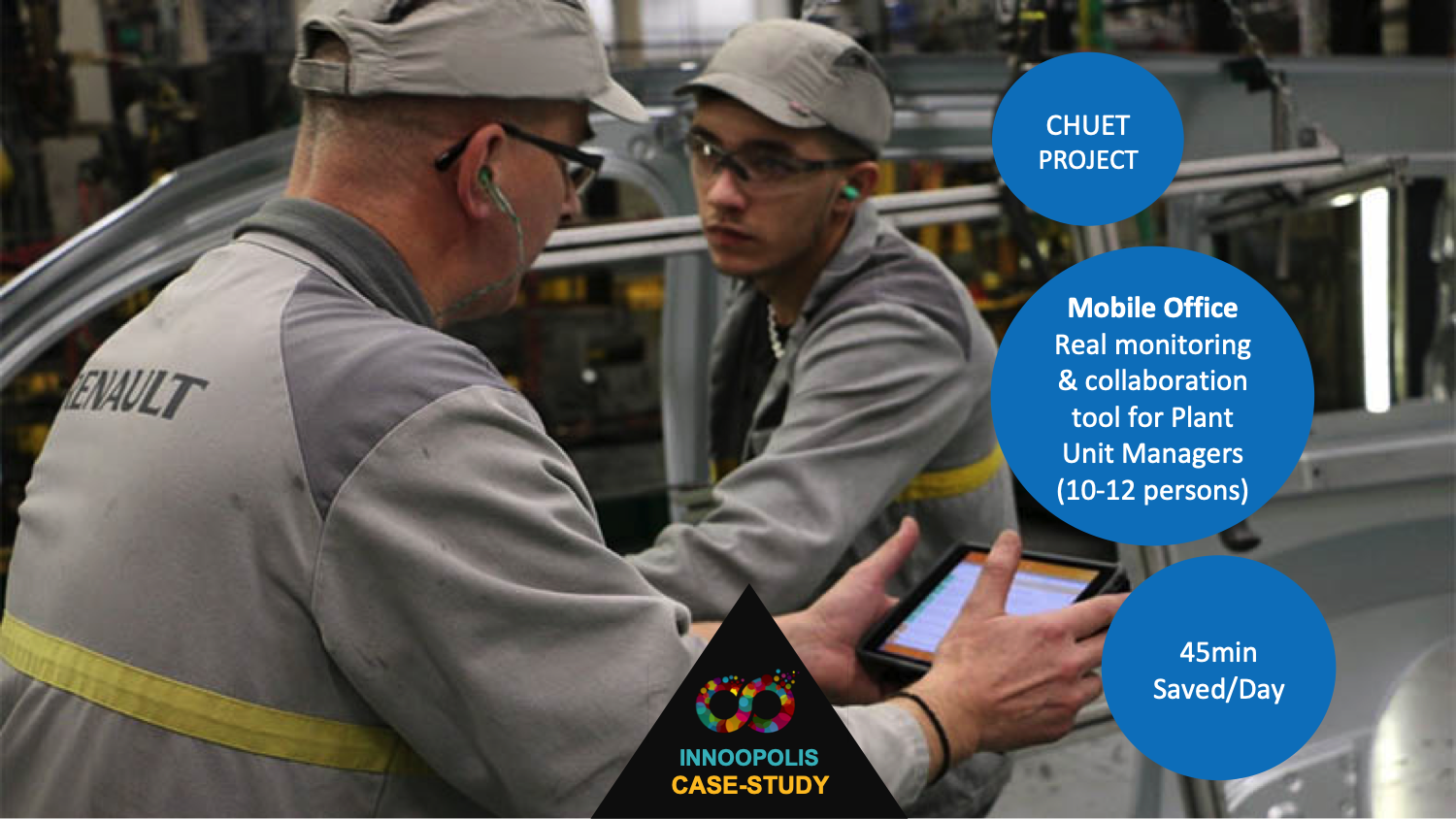
Connecting equipment helped the unit managers, but connecting machines and robots also allowed them to create value in other areas.
Predictive maintenance is one of these areas where expensive downtime due to machine failures can be avoided or at least its probability reduced. Renault has put more than 2000 Lora sensors in place to collect data from their equipment.
In manufacturing plants, robots and automation are of course essential, but with the increasing needs of personalization, with the increasing complexity of the product, some tasks become more complicated and human intervention is often needed to ensure quality.
The collaboration that is needed between robots and humans is such that we are now talking about co-bots (collaborative robots). While the bot takes on heavy lifting and repetitive tasks, the human employee is given more time to perform more complex tasks like quality control.
This new type of collaboration and shift in tasks for plant employees also requires a new type of training, a type of training that is best done with augmented reality and virtual reality tools. Renault is leveraging this type of experiential learning to make sure employees quickly and safely prepared for this new work environment.
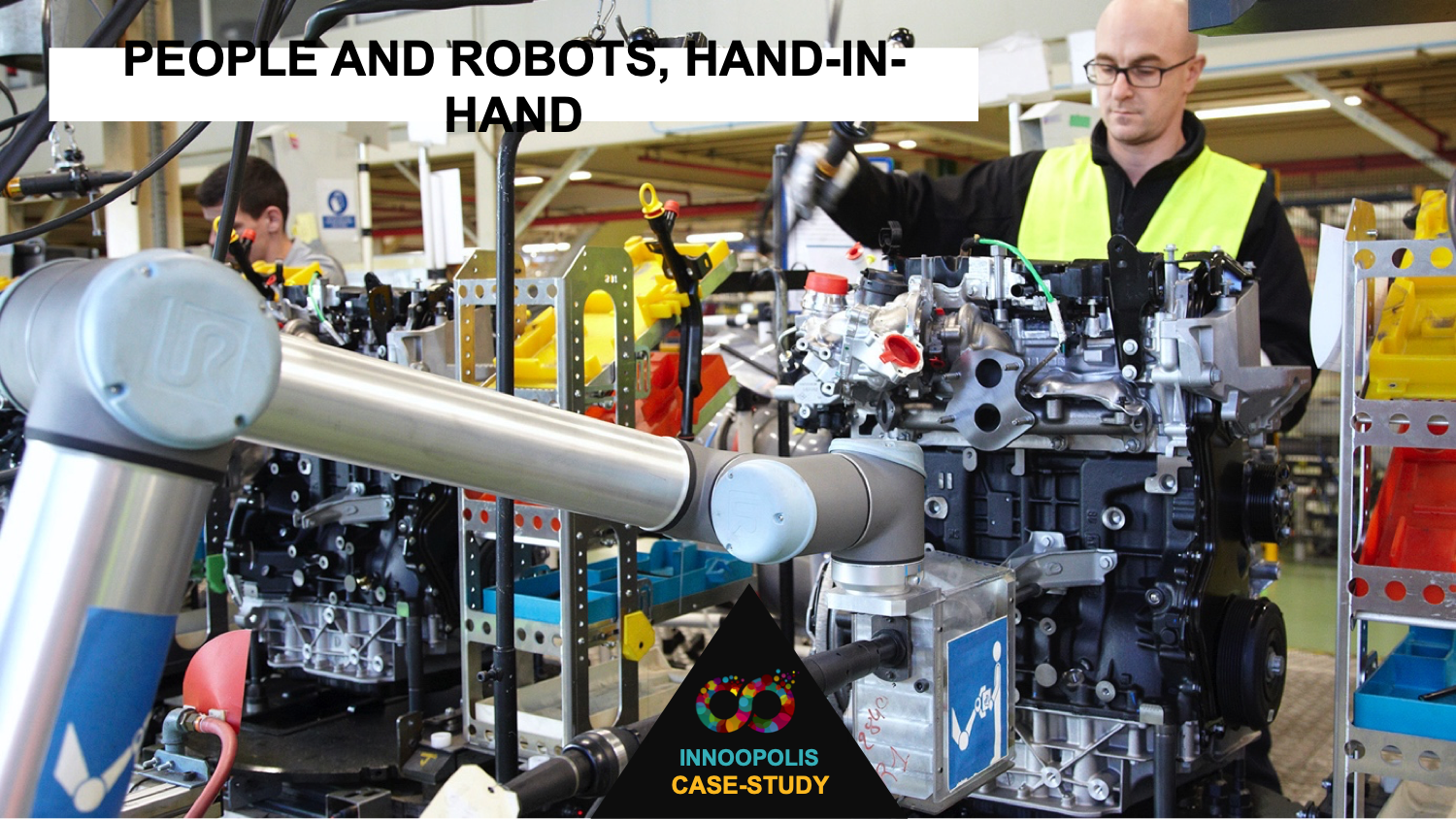
Downstream opportunities
Downstream opportunities are real as well. One of the challenges all auto-makers and dealership face is that they don’t have that long-term permanent relationship with the customer.
Customers typically only come back when a problem arises, and after the warranty is expired they won’t come back until they need a new car… if somebody else didn’t catch their attention first. Connecting cars is the first step, but then it is about building applications that provide engaging experiences and allow to create new value for customers.
By staying connected with its customers Renault expects more sales lead generated through digital, providing better opportunities to achieve its growth ambitions.
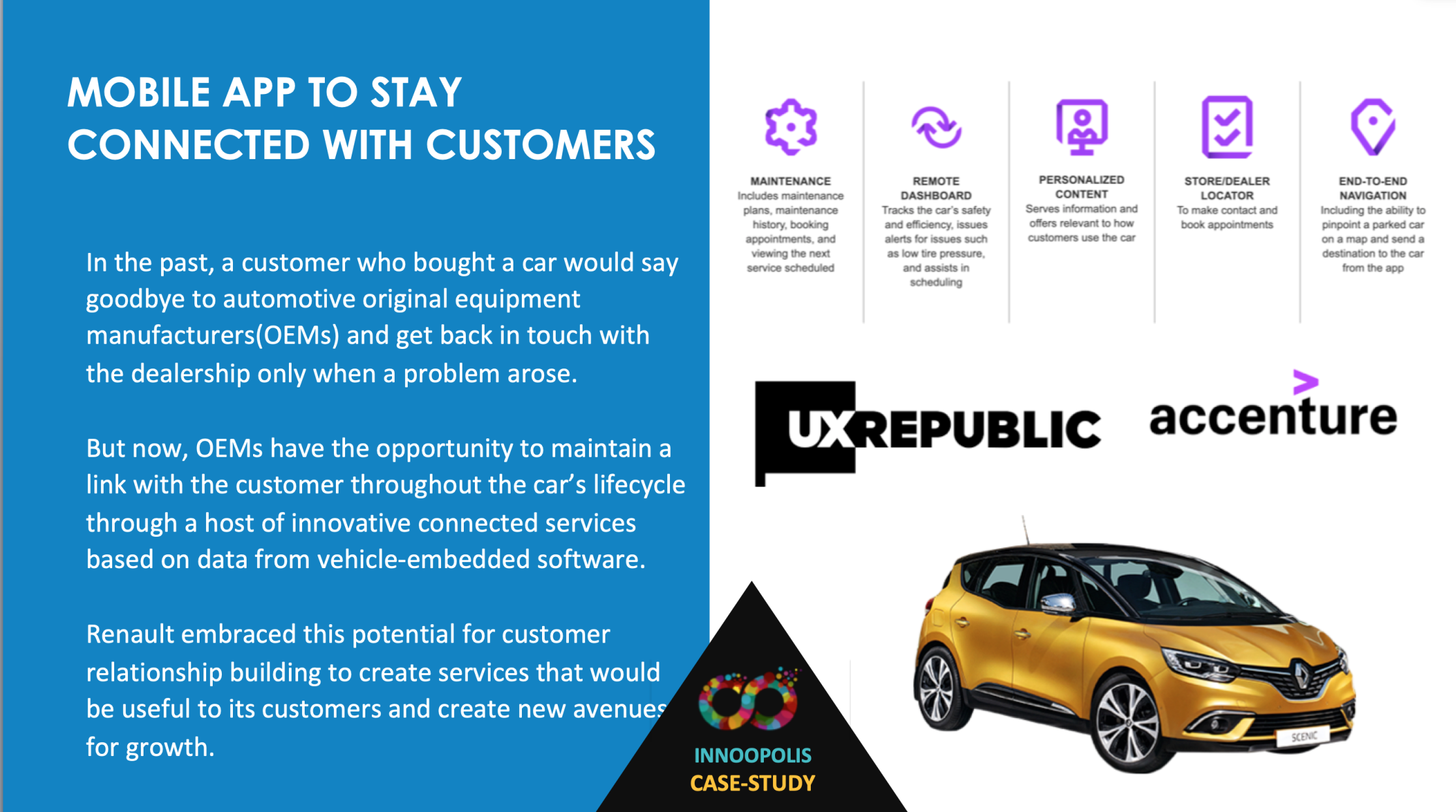
Transformation with a culture change at Heart
Renault Digital has proved to be an immense asset for Renault. Already delivering clear value within 12 months of its inception (through the CHUET project), the ambitions of the group when it comes to the impact of digital have been revised upwards. It is now estimated that the impact will reach 1Billion Euros per year.
But at the end of the day, Renault is aiming to change the entire culture of the company. New leadership mindsets, but also a workforce that becomes increasingly agile.
That focus has also led HR to take a leading role in the transformation of the company. Besides setting various programs to develop digital skills, they also realized that the management style needs to change and that collaboration is becoming more essential to drive agility.
Collaboration and the development of a more aspirational environment are also what is supposed to attract new talent. Ultimately these changes will also lead HR to transform itself. Focus on end-2-end employee journeys, giving more power to employees to manage their careers are all elements that aim to make Renault an attractive employer in a world where digital talent is still scarce.
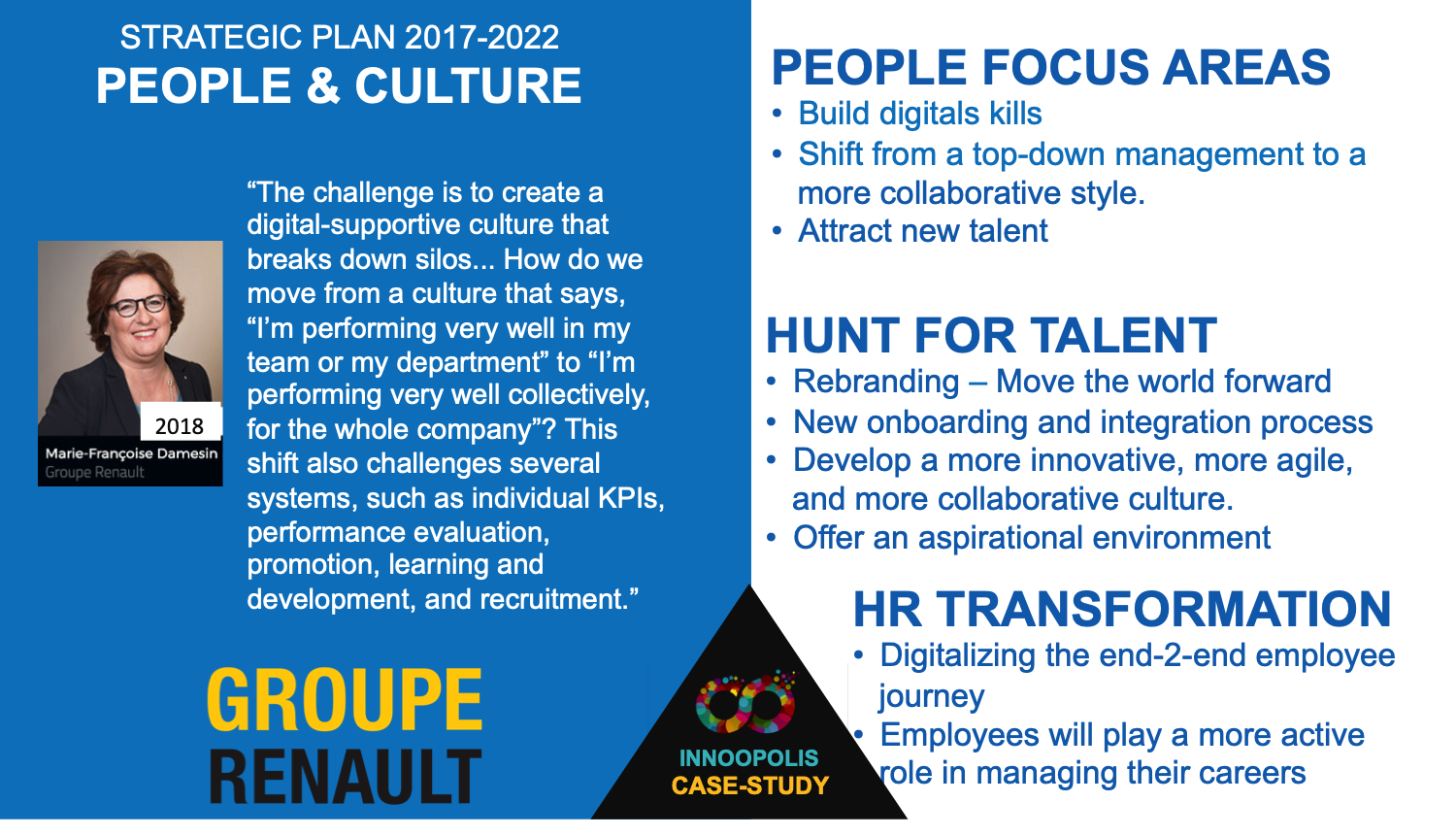
If you have more insights on the Renault Digital Transformation story, then I would love to hear form you to make this story better and more developed.
Do you have similar stories to share? What is the most interesting part of the Renault story?
Disclaimer : This article is based on open public reports, interviews posted in magazines, some of them translated from french. It is an external analysis of the Renault Digital Transformation story to the best of my knowledge. If anything here is inaccurate, please contact me directly and I will update the article.
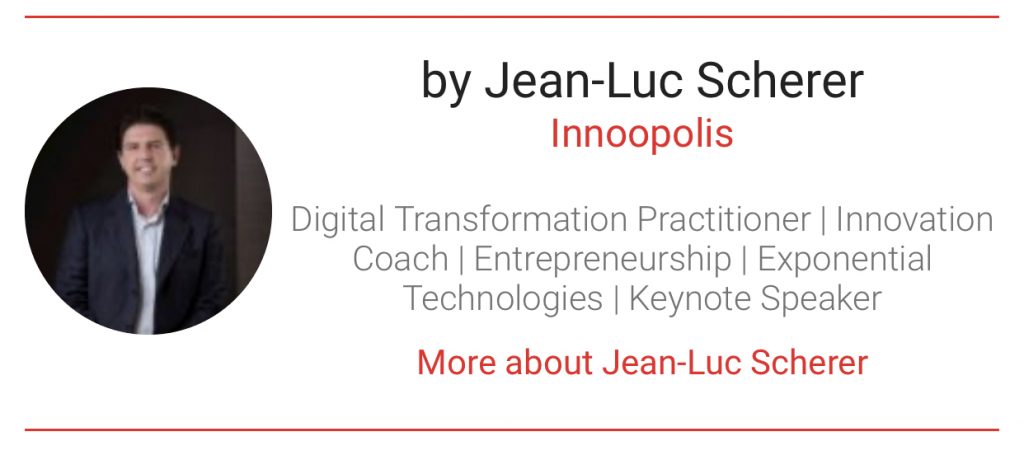
Arrange a Conversation
Article by channel:
Everything you need to know about Digital Transformation
The best articles, news and events direct to your inbox
Read more articles tagged: Featured , Fourth Industrial Revolution
Popular Now
Related articles.
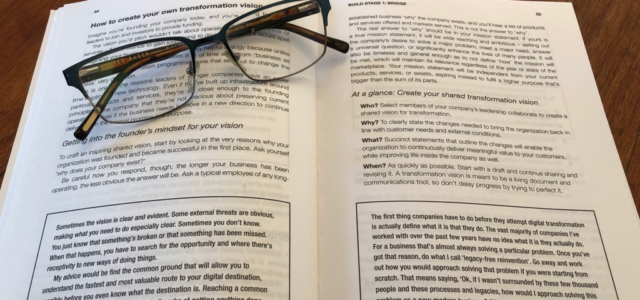
Login to your account
Change password, your password must have 8 characters or more and contain 3 of the following:.
- a lower case character,
- an upper case character,
- a special character
Password Changed Successfully
Your password has been changed
Create a new account
Can't sign in? Forgot your password?
Enter your email address below and we will send you the reset instructions
If the address matches an existing account you will receive an email with instructions to reset your password
Request Username
Can't sign in? Forgot your username?
Enter your email address below and we will send you your username
If the address matches an existing account you will receive an email with instructions to retrieve your username
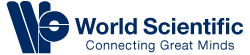
- This Journal
- Institutional Access
Cookies Notification
Our site uses javascript to enchance its usability. you can disable your ad blocker or whitelist our website www.worldscientific.com to view the full content., select your blocker:, adblock plus instructions.
- Click the AdBlock Plus icon in the extension bar
- Click the blue power button
- Click refresh
Adblock Instructions
- Click the AdBlock icon
- Click "Don't run on pages on this site"
uBlock Origin Instructions
- Click on the uBlock Origin icon in the extension bar
- Click on the big, blue power button
- Refresh the web page
uBlock Instructions
- Click on the uBlock icon in the extension bar
Adguard Instructions
- Click on the Adguard icon in the extension bar
- Click on the toggle next to the "Protection on this website" text
Brave Instructions
- Click on the orange lion icon to the right of the address bar
- Click the toggle on the top right, shifting from "Up" to "Down
Adremover Instructions
- Click on the AdRemover icon in the extension bar
- Click the "Don’t run on pages on this domain" button
- Click "Exclude"
Adblock Genesis Instructions
- Click on the Adblock Genesis icon in the extension bar
- Click on the button that says "Whitelist Website"
Super Adblocker Instructions
- Click on the Super Adblocker icon in the extension bar
- Click on the "Don’t run on pages on this domain" button
- Click the "Exclude" button on the pop-up
Ultrablock Instructions
- Click on the UltraBlock icon in the extension bar
- Click on the "Disable UltraBlock for ‘domain name here’" button
Ad Aware Instructions
- Click on the AdAware icon in the extension bar
- Click on the large orange power button
Ghostery Instructions
- Click on the Ghostery icon in the extension bar
- Click on the "Trust Site" button
Firefox Tracking Protection Instructions
- Click on the shield icon on the left side of the address bar
- Click on the toggle that says "Enhanced Tracking protection is ON for this site"
Duck Duck Go Instructions
- Click on the DuckDuckGo icon in the extension bar
- Click on the toggle next to the words "Site Privacy Protection"
Privacy Badger Instructions
- Click on the Privacy Badger icon in the extension bar
- Click on the button that says "Disable Privacy Badger for this site"
Disconnect Instructions
- Click on the Disconnect icon in the extension bar
- Click the button that says "Whitelist Site"
Opera Instructions
- Click on the blue shield icon on the right side of the address bar
- Click the toggle next to "Ads are blocked on this site"

System Upgrade on Tue, May 28th, 2024 at 2am (EDT)
Industry 4.0: the case-study of a global supply chain company.
- Cezar Honorato and
- Francisco Cristóvão Lourenço de Melo
Department of Space, Science and Technologies, Technological Institute of Aeronautics, Brazil
E-mail Address: [email protected]
Corresponding author.
Search for more papers by this author
Department of Aeronautics and Space, Technological Institute of Aeronautics, Brazil
The technological evolution that enterprises are facing is increasingly fast and dynamic, demanding more and more flexibility and agility in the application of new technologies in an increasingly globalized and competitive business environment. This work has a broad view of the industry 4.0 influence in the global supply chain companies, as it is essential for long-term business sustainability and one of the key drivers of profitability and growth. The model proposed in this research effort considers the application in the processes of a global supply chain enterprise, which includes from the beginning of the demand request, also the industrial activities, to the post-sale analysis; finally, an unprecedented indicator was developed to measure the level of application of industry 4.0 concepts in organizations according to the state-of-the-art in the evaluated processes. Then, the model was applied to the real case of a global high-tech supply chain company. The first findings demonstrate the ease of understanding and applicability of the model for companies to analyze the digital transformation in their processes and identify gaps to convert them into real opportunities to leverage their business.
- Advanced manufacturing
- Digital transformation
- Maturity model
- Global enterprise
1. Introduction
In an increasingly competitive scenario with a dynamic and globalized economy, manufacturing companies around the world are facing substantial challenges due to recent environmental, social, economic and technological developments where market conditions tend to change, whether due to new consumer needs, new competitors, changes in the market or economic policies, manufacturing enterprises need to not only become, but also remain competitive to ensure their survival.
Industry 4.0 has revolutionized the traditional production line toward smart factories in a sustainable environment. It represents an intelligent manufacturing network, where machines and products interact without human control. Industry 4.0 technologies enable the seamless information transfer between multiple stakeholders in the supply network ( Gorkhali , 2022 ).
The data generated in many stages of supply chain enterprises from design (or demand start) to after-sales was collected, analyzed and used to support business decisions in many sectors, customer behavior and inventory ( Davenport and Dyché , 2013 ) creating one of the most valuable assets (Knowledge Management) that can define a company’s competitiveness since the modern industry beginning, perhaps even earlier ( Côrte-Real et al . , 2017 ).
Exploiting the full potential of this scenario is supported by technological advances in data collection, new organization forms which are creating the new environment with large data volumes for leading companies to build capacity in new areas knowledge to become more intelligent companies ( Malladi , 2013 ).
In view of recent technological developments and an increasing demand scenario for personalized products, greater complexity, higher quality and reduced costs; the emergence of a new industry model has become essential to maintain the companies’ competitiveness ( Hermann et al. , 2016 ).
The current state-of-the-art in production technology can be described as driven mainly by increased efficiency over manufacturing processes. However, this focus on advances in manufacturing efficiency occurs in the individual company instead of the entire supply chain ( Schumacher et al. , 2016 ).
Advances can also be seen at the organizational-economic level as Lean Management at the manufacturing technology level, i.e., Laser technology, additive manufacturing, robotics integrates with virtual reality ( De Weck et al . , 2014 ) in material level, information technology level, i.e., RFID, embedded systems. All these advances have led to significant gains, but isolated in the process, efficiency and product quality.
Garay-Rondero et al. ( 2020 ) synthesized the main actors that impact supply chain companies being (i) Management Components such as Planning and Control Methods, communication and information (ii) flows such as materials, information, finance (iii) Network structures such as logistics, distribution and (iv) Management process such as managing suppliers, customers, demand which associated with the main industry 4.0 components accelerate a future vision connectivity between different supply chain digitization in order to add value through new cooperation forms and digital integration.
The production processes integration must take place at the horizontal level (across the entire value chain) and at the vertical level (in all automation layers). Fully integrated and factories, machines, processes and networked products will then be able to act intelligently and partially autonomously that requires minimal manual interventions ( Monostori , 2014 ).
Recent concepts like internet of things, cloud-based manufacturing ( Gao et al. , 2015 ) and smart factories meet these requirements in part and are commonly used under the visionary industrial revolution concepts ( Berger , 2014 ).
Industry 4.0 refers to recent technological advances where the internet and supporting technologies (i.e., embedded systems) serve to integrate physical objects, human actors, intelligent machines, production lines and processes across organizational boundaries to form a new kind of intelligence, networked and agile supply chain value.
Experiences from several strategic orientation workshops showed that enterprises have serious problems in understanding the general industry 4.0 idea and apply the concepts in the real business life ( Erol et al. , 2016 ), on the other hand, there is evidence that when consistently implemented Industry 4.0 has a positive impact in operational performance, including cost performance, quality, delivery and flexibility ( Szász et al. , 2020 ).
Enterprises face problems in determining their state of development in relation to the industry 4.0 vision and, therefore, fail to identify specific action fields, programs and projects. Overcome growing uncertainty and dissatisfaction in manufacturing companies in relation to the industry 4.0 idea, new methods and tools are needed to provide guidance and support for aligning business strategies and operations ( Schumacher et al. , 2016 ).
So, we have the research question that most affects companies in the global supply chain: How are global supply chain enterprises getting ready to take advantage of the industry 4.0 environment to generate real value? Our spectrum of view is not concentrated only in assessing industrial activities, but in all operations that involve a global company, therefore, from the beginning of the demand request to the post-sale analysis; based on this question this work purpose is developed.
The objective of this research effort is to analyze a traditional company in the global supply chain manufacturing industry and provide a maturity model with a broad processes analysis, not restricting industrial activities, and through an assessment to identify gaps and provide information that allows discussion to take advantage of the potential of Industry 4.0 in your processes.
This study will be valuable to support the transformation industry’s path in the new technological era, providing the experience and best practices in the preparation of the enterprise and enhancing the knowledge that is important to reduce the response time to the most diverse demands, increase its efficiency and improve your competitiveness.
The paper is structured as follows. Initially, we discuss the concepts that culminate in industry 4.0 as well as demonstrating the evolution in the academic environment. Then, we describe the macro-processes, and respective details, which involve the company considered as well as conceptual bases of the structure of the proposed model. The results presented in the model and in the first findings of the preliminary assessment carried out in the case study are presented. Finally, we conclude with a discussion of the main findings and the linear knowledge continuity is proposed as future research.
2. Literature Review
2.1. the industrial revolutions.
The Industry 4.0 concept originates from a strategic project by the German government with a focus on technology. The term was first used at the Hannover Fair in 2011 ( Drath and Horch , 2014 ). Later, final report on recommendations for implementing Industry 4.0 was published by the German government to capture opportunities and maintain leadership in the manufacturing engineering industry ( Xu et al. , 2018 ).
The term industry 4.0 refers to the fourth industrial revolution when evaluating the most abrupt changes that we had in the sector during the humanity evolution, while the first industrial revolution (1780) was driven mechanical looms design powered by steam engines and resulted in the production centralization process in factories.
After 100 years of steam engines, the second revolution took place, being identified by production lines insertion for the construction of the automotive model Ford T; the third industrial revolution took place in the late 1960s, identified by the presentation of the first programmable logic controller, which allows the programming of digital systems ( Pereira and de Oliveira Simonetto , 2018 ).
Following the evolutionary cycle, the fourth industrial revolution, represented by industry 4.0, has the basic foundation connecting machines, systems and assets having as objectives: to improve products and/or services; reduce costs; manage operations more efficiently thanks to production performance monitoring; to better position yourself in the competition for accessing data and information useful for fasting and better response to market needs ( Moeuf et al. , 2018 ).
In this way, applied to companies, they will be able to create smart grids throughout the supply chain that can control production modules autonomously. In other words, smart factories will have the capacity and autonomy to schedule maintenance, predict process failures and adapt to requirements and unplanned changes in production systems.
2.2. Industry 4.0 principles and pillars
Industry 4.0 has been changing technological reality in manufacturing. The smart industry arises as a new digital systems pattern, information technology and internet solutions ( Oliveira et al. , 2020 ).
The industry 4.0 field study is relatively new (close to ten years), we can say that in the second half of this time there were greater studies contributions, thus evaluating the documents most cited in the literature in the last 5 years (2016–2020).
From these studies, we can highlight the main concepts provided, which are: ability to operate in real time: it consists of the acquisition and data treatment almost instantly, allowing decision making in real time; virtualization: simulations used and also supervisory systems.
Thus, industry 4.0 proposes the smart factories existence that allow tracking and remote monitoring of all processes through the various sensors spread across the industrial plants (i.e., factories, warehouse) and decentralization, in this way decision-making can be made through systems according to production needs and in real time. Furthermore, machines and industrial processes do not receive commands, but are able to provide information about their work cycle and improve their performance through machine learning ( Rüßmann et al. , 2015 ).
In this way, the smart factory modules work decentrally to improve production processes, another relevant concept is flexibility: production according to demand, coupling and decoupling of production modules, i.e., quickly change-over ( Moeuf et al. , 2018 ).
What offers flexibility to easily change machine tasks is system integration which in turn is characterized by three dimensions: (i) vertical integration—takes place within the smart factories, where the people connection, objects and systems in the “smart” factories leads dynamic value networks creation; (ii) horizontal integration—occurs through value networks, starts to use new technologies to exchange and manage information between stakeholders in the process, allowing for closer collaboration between customers, suppliers and business partners and (iii) digital integration—end to end is made possible by vertical and horizontal integrations, which take place from the information collection about the product throughout its life cycle and add value from conception to outbound logistics ( Rüßmann et al. , 2015 ).
Based on the above principles, industry 4.0 is a reality made possible by technological advances in the last decade, combined with technologies in development in the areas of information technology knowledge and engineering ( Xu et al. , 2018 ).
The technological pillars that support Industry 4.0 can be summarized as follows ( Lasi et al. , 2014 ): (i) Internet of things (IoT): Consists in the network connection of physical objects, environments, vehicles and machines by means of embedded electronic devices that allow data collection and exchange, (ii) Cyber-physical Systems (CPS): These are systems that work based on the Internet of Things and are equipped with sensors and actuators and (iii) Big Data analytics: They are very extensive and complex data structures that use new approaches for the capture, analysis and information management.
Applied to industry 4.0, Big Data technology consists of 6Cs to deal with relevant information: Connection (to the industrial network, sensors and PLCs), Cloud (cloud/data on demand), Cyber (model and memory), Content, Community (information sharing) and Customization (personalization and values) and (iv) Security: One of the key challenges for the success of the fourth industrial revolution is the security and information systems robustness. Problems such as transmission failures in machine–machine communication, or even eventual system failures that can cause production problems. With all the connectivity established, systems will also be needed to protect the company’s know-how, contained in the process control files ( Kagermann et al. , 2013 ).
Finally, industry 4.0 is changing the technological enterprises reality. The smart industry emerges as a new standard for digital systems, information technology and internet solutions. The industry 4.0 impacts are multifaceted and complex for all organizations and economies. In addition to the changes, industry 4.0 brings a new organizational dynamic: fewer operational processes implying new job skills and more efficient results ( Rafael et al . , 2020 ).
2.3. Industry 4.0 applied in many fields
The 4.0 industry concepts have been a growing issue in academic publications, Fig. 1 shows the publications evolution since 2011, when we had the first formal document at the Hannover fair, and until end of 2020, when we used the terms “industry 4.0” or “advanced manufacturing”, the most common terms used, the Scopus database was used, the publications growth went from 1,174 documents (2011) to 8,351 (2020).
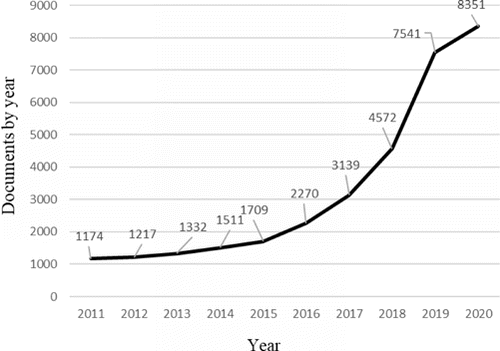
Fig. 1. Number of publications using “industry 4.0” or “advanced manufacturing”.
The industry 4.0 concepts have been the studies subject in several areas and applications in the most economy sector, i.e., technological assistance to workers to maximize the benefit of the varieties that this type of technology has ( Gorecky et al. , 2014 ), blockchain application to facilitate machine-to-machine interaction in the chemical industry ( Sikorski et al . , 2017 ) for creating smart products ( Schmidt et al. , 2015 ), associated with lean manufacturing ( Sanders et al. , 2016 ), application for healthcare ( Pace et al. , 2018 ), in agriculture sector ( Zambon et al. , 2019 ), automotive industry ( Lin et al. , 2018 ), aerospace ( Ceruti et al . , 2019 ) and in higher education ( Li , 2020 ); thus, in addition to the increase in publications involving industry 4.0, there is also greater dissemination in several knowledge areas.
Some maturity models were found that seek to evaluate industry 4.0 in organizations, however, through bibliometrics that uses citation level evaluation in the research bases in the last five years (2016–2020), as can be seen in Table 1 , they were directed to enable the industry 4.0 evaluation or the strategy or even in the evaluation of some specific processes, many studies analyzed do not describe the methodology used in this way were not considered.
Author(s) | Main findings | Author(s) | Main findings |
---|---|---|---|
( ), ( ) | Evaluates the company’s strategy and the level of use of enabling technologies, there is no discrimination by company processes. | ( ) | The model is available to evaluate the use of enabling technologies of industry 4.0 in companies. |
( ) | Evaluation as a quick check and part of a process model for performing gap analysis to overcome barriers through a toolbox; no details about items and development process offered. | ( ) | Model that specifically analyzes the processes of processing, storing and sending orders as to the application and self-approval of these processes. The model has a good detail. |
( ) | Specific model to improve operational performance through industry 4.0. | ( ) | The proposed maturity model is specifically targeted at industrial companies evaluating under five dimensions (strategy, structure and organizational culture; workforce; smart factories; smart processes; smart products and services). |
( ) | The model only provides concepts for diagnosing the company’s vision and assessing the use of enabling tools in industry 4.0, without any description of the processes. | ( ) | In this article a model is developed to assess the level of maturity of industry 4.0, however more directed to the levels of automation, in the production systems specifically of a steel company. |
( ) | Specific model to enable industry 4.0 for manufacturing companies, missing documentation. | ( ) | The developed model aims to assess the maturity level of the technologies of industry 4.0 in the area of logistics in the service industry (eg: construction, transportation, |
( ) | Model directed to application in the most strategic areas of companies such as: process transformation (for enabling technologies), data governance, asset management and organizational alignment areas.There is no detailing, at least in order to exemplify, of processes considered. | ( ) | The framework for evaluating the concepts of industry 4.0 is presented, specifically, in the area of business logistics as well as developing a roadmap for the improvement process. |
( ) | It consists of broadly demonstrating the application of Internet of Things (IoT) by means of criteria. | Lizarralde (2020) | It is a model adapted to small and medium companies (SMEs) in the manufacture of Machine Tool companies (MT) that assesses the technologies of the industry 4.0 in |
( ) | It consists in broadly demonstrating the evaluation of the application of the Internet of Things (IoT) by means of recommended criteria. | ( ) | In this work, an application model of the concepts of Industry 4.0 and Industrial Internet specifically in Heavy Industry. |
From the comparison of the analyzed models and the identification of opportunities for improvement between them, the model proposed in this research effort considers the application in the processes of a global company in the supply chain, not being restricted to industrial facilities or specific areas as the models analyzed, which includes from the beginning of the demand request, also industrial activities, until the post-sale analysis; finally, an unprecedented indicator was developed, as well as its calculation method, to measure the application level of industry 4.0 concepts in organizations according to the state-of-the-art in the evaluated processes.
3. Research Methodology
This work has a broad view on the influence of industry 4.0 in industrial enterprises maintaining competitiveness with sustainable growth in global markets increasingly demanding being one of the key levers for profitability.
Aligned with this concept, we understand that industry 4.0 involves integration with strategy, planning, development, implementation and operation, the latter with regard to direct users in the industry. This involves the strategic business vision with engineering, project management, production systems, supply chain, manufacturing, delivery, research and development, supplier development, sales, operation and after-sales and other actors that are not necessarily within internal companies’ borders.
The research procedure can be divided into four distinct phases. An initial phase creates a complete understanding of all the enterprise processes. The second stage refers to the model bases construction as well as the detailing of the global company process and the respective assessment form and results presentation. The third step is to carry out the evaluation by the experts in each process considered of the global supply chain company and, finally, the fourth and final stages correspond to indicators calculation and results presentation.
3.1. Enterprise process
As the enterprise referring to the case studied in a global company, all its processes were understood, when considering a manufacturing area, the starting point was demand generating and the end point is considered after sales.
The flow that represents all the steps is shown in Fig. 2 , which shows that some processes are sequential and others started in parallel, the process design includes five macro-processes and these are unevenly distributed in 26 processes.
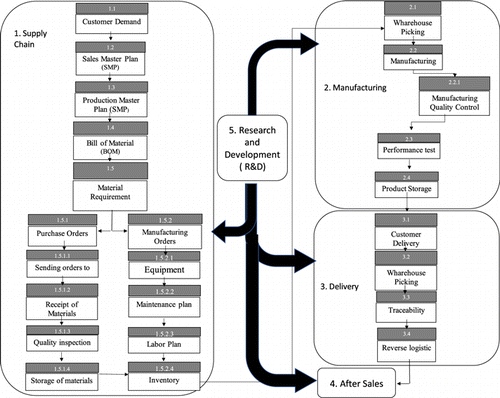
Fig. 2. Enterprise macro-process and its details.
The general diagram (Fig. 4 ) is subdivided into five macro-processes, namely: (1) Supply Chain, (2) Manufacturing, (3) Deliveries, (4) After sales and, (5) Research and Development (R&D), the latter interfaces with all areas. It is possible to check all the details that were made in each macro-process, except after-sales and R&D, due to the particularity of these areas, it was decided to investigate the main inputs, outputs and interfaces with other areas.
3.2. Model bases
As the subject of this research effort is multidisciplinary, as it involves several knowledge areas, the theoretical framework research provided the identification of the conceptual bases to be used that will permeate the entire work.
The recommended model is based on a structure with two evaluation perspectives, the first being designated by levels and the second evaluation perspective are the company areas called macro-processes, which in turn are divided into processes.
Levels are the conceptual references that guide the entire model (Table 2 ), so when developing the criteria for each process, they must necessarily meet these criteria, the scale used (1 to 4) is a reference to how management would be in the four industrial revolutions.
Level | Description |
---|---|
1.0 | The entire process of information, receipt, consolidation, decision-making and starting is documentary and carried out necessarily based and through analysis “Face to Face” - F2F - being with another person or document. |
Industrial production system (without standardization). The entire information system is (inputs, processing and decision making) | |
2.0 | Part of the information processing is as the receiving step, however consolidation is as well as the which also depend |
Mass production, standardization and production system without flexibility. Telephony communication system, manual documentation, decision-making process | |
3.0 | Automation without integration. , however, It does not have the Intelligent Information System. |
Receiving and consolidating information automatically, but not integrated. | |
4.0 | Flexibility and autonomy of the production systems, in an efficient, fast, intelligent and customized process, meeting more specifically the needs of customers in a competitive way. Decision making without manual influence. |
Completely based on the parameters and scenarios of the software (by means of physical and/or virtual robot): receipt, consolidation, decision making and triggering without manual influence and improvements established through machine learning. |
3.3. The model concept
In this phase, it corresponds to the criteria elaboration that define each company process used according to model bases defined in the previous phase.
To facilitate the different analysis of the industry 4.0 concepts application, the 26 processes are divided into 63 assessment items, as shown by the examples in Table 3 .
Macro process | Exemplary of the items |
---|---|
1. Supply Chain | Customer Demand; Sales Master Plan (SMP); Production Master Plan (SMP); Bill of Material (BOM); Material Requirement Planning (MRP), Purchase Orders... |
2. Manufacturing | Wharehouse Picking; Manufacturing; Manufacturing Quality Control; Performance Test, Manufacturing Flexibility, Product Storage, Performance Machines,... |
3. Delivery | Customer Delivery; Delivery Accuracy, Wharehouse Picking; Product Traceability, Reverse Logistic, Life Cycle Product |
4. After Sales | Digitalization of sales/services, Mobile devices utilization; Costumer’s Digital media competence; Receiving customer information.... |
5. P&D | Governance; Customers Relationship, Innovation Process, Open Innovation development; interdepartmental collaboration,... |
Some processes are evaluated by more than one item, in this case, the arithmetic average of all items that make up the result of the evaluation process is considered.
As a next step, the criteria that define each application level in the processes considered were developed according to the bases (Table 2 ) that, as previously mentioned, permeate in the entire model, i.e., the levels which evaluate the 1.5.1 item named Purchase Order process is shown as follows (Fig. 3 ).
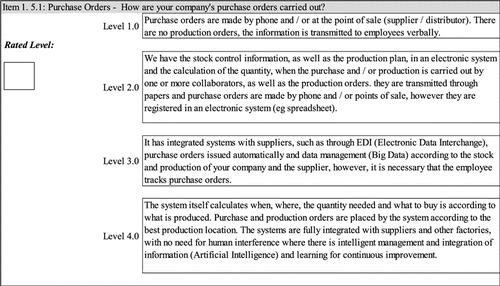
Fig. 3. Assessment checklist example.
The value that represents industry 4.0 application level in each macro-process, is represented by the arithmetic average of the processes that compose it, because it is understood that all have equal importance.
Then, the results achieved are represented by a radar chart where each vertex corresponds to each macro-process evaluated. Through this chart, the indicator called the industry 4.0 application index is calculated.
The indicator is calculated from the polygons area ratio, coming from the company evaluation under the model divided by the maximum possible area, in this situation when the enterprise reaches the maximum grade in all 63 assessment items. The index is evaluated between 0 (lowest possible value) and 100% (maximum possible value), finally a scale is presented which positions the company in relation to the defined state-of-the-art.
4. The Case-Study: Global Company
In this section, we present the model application based on a real case study in enterprise in the global high-tech supply chain in the capital goods sector. We chose an organization that already has applications in its various conceptual processes in industry 4.0, demonstrated by winning the digital transformation award in industry, smart logistics and other global innovation awards and has operation units, or a Research & Development center, in the five continents.
The company received a questionnaire and after explaining it started to fill it, which was carried out together with the application facilitator of the internal program in advanced manufacturing and experts in each macro-process, in this ensured knowledge in the environment of the internal processes as well in the industry 4.0 concepts; in the end, 21 specialists participated in this assessment process.
After the self-assessment, the answers were then inserted in the software tool to calculate application levels and create the report. In Fig. 4 , it is possible to visualize the application level in each enterprise macro-process.
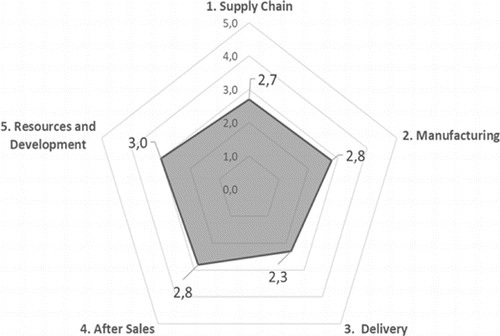
Fig. 4. Radar chart in the macro-process evaluated.
Figure 5 shows the details of the evaluated processes that compose the macro-process “Supply Chain”. It is noticed that the company that obtained the best score in the process was the material receipt (reached 3.2 out of 4.0) and the worst result was quality inspection (reached 2.0 out of 4.0).
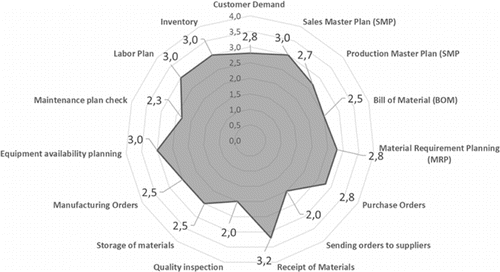
Fig. 5. Radar chart of the entire “supply chain” process.
For example, in Fig. 5 , it is possible to see the item Production Master Plan (PMP) is evaluated by sub-items: (i) How the production schedule management is carried out (input data), (ii) How the production lead time is calculated and monitored and, (iii) How is product to product traceability for customer? The average rating of these three items reached 2.7 out of 4.0.
According to the result shown in Fig. 4 , the industry 4.0 application index is calculated, based on the ratio between the areas of the formed polygons; hatched figure, which is the company assessment result divided by the maximum area formed, in this case, the company achieved the maximum score in all items (level 4).
Figure 6 shows the association between the proposed scale and its respective ranges, which are explained as follows:
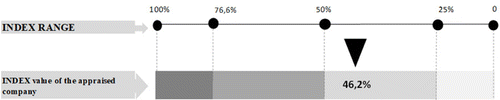
Fig. 6. Illustration of the company result evaluated in the Index scale.
• | Very Low Maturity: The company has the value up to 25% of the Index, for that the chart-built area after assessment done is equivalent to the regular pentagon area which levels is 2 in the five dimensions. This range represents that the enterprise has very low maturity in the industry 4.0 concepts according to the state-of-the-art defined. | ||||
• | Low Maturity: The company has the value between 25% and 50% of the Index, for that the chart-built area after assessment done is equivalent to the regular pentagon area between levels from 2.01 to 2.83 in the five dimensions. This range represents that the enterprise is in an attention situation regarding the industry 4.0 concepts according to state-of-the-art defined. | ||||
• | Relevant Maturity: The company has the value between 50% and 76.6% of the Index, for that the chart-built area after assessment done is equivalent to the regular pentagon area between levels from 2.84 to 3.5 in the five dimensions. This range represents that the enterprise has a significant maturity situation of industry 4.0 concepts according to the state-of-the-art defined. | ||||
• | High Maturity: The company has the value between 76.6% to 100% of the Index, for that the chart-built area after assessment done is equivalent to the regular pentagon area between levels from 3.51 to 4.0 in the five dimensions. This range represents that the enterprise is very close or already reached in terms of industry 4.0 maturity concepts according to state-of-the-art defined. |
5. Conclusion
This research work aimed to develop a model that systematically assesses the application of industry 4.0 concepts in the global supply chain enterprise.
In contrast to other approaches found in the literature, the main contribution of this research effort is to provide a model that specifically assesses the advanced manufacturing concepts in a global supply chain enterprise, not restricted to industrial facilities or specific areas, which included from the beginning of the demand request, also industrial activities, until the after-sales analysis based in five macro-processes and these are unevenly distributed in 26 processes resulting in 63 assessment items.
After conducting the assessment, the data collected from each macro-process are plotted on the Radar chart and from this graph the indicator that measures the application level of advanced industry concepts, described as industry 4.0 application index, it was developed in an unique way of measuring maturity through the area ration of the polygons after carrying out the assessment by the maximum possible area, that is, in this situation when the company reaches the maximum score in all sixty-three items.
The model transformation into a useful tool also allowed its easy application in practice. Early field experiences show that manufacturing enterprises are able to use the results of their self-assessment as a solid reference point for other strategic actions.
The results of the model proposed in this research effort, through the case study carried out, allowed a reflection on the strategies that the company has to address the state-of-the-art of the industry 4.0 concepts. This goes beyond evaluating its execution, or even evaluating isolated processes or proposing solutions without being aligned with the enterprises top management.
During the application and results analysis, it was found that companies do not necessarily intend to implement industry 4.0 in all their processes, due to budget, resource restrictions, or even a certain area is not a priority or because it does not have high economic potential in face of the investments and efforts required in the digital transformation that are required in hardware and software technologies.
Having said that, the proposed model allows for the gap identification for the company to carry out the analysis if at the moment evaluated they are identified as real opportunities for their business in order to prioritize the most relevant for each business.
The possibility of inserting the action plan in the evaluations carried out, associating it to each dimension, in this way, will make it possible to have all the information centralized, avoiding files and documents outside the tool and, finally, it was also understood as relevant when viewing your information in the radar chart that may also have available the result average of the other companies in the sector that represents the ecosystem in which it is inserted, to maintain the companies data confidentiality, whose objective is to make comparisons and assess existing gaps and improvement opportunities in relation to the sector average in which they operate.
Finally, new studies are suggested as a proposal to continue the knowledge evolution, so future research will focus on the application of the same assessment in other units around the world to provide data on the company’s best internal practices; it is also proposed to carry out a company assessment in the electronic components manufacturing sector, because according to the government it is the sector that has the most technology in its processes, which we will have references for other Brazilian industrial sectors.
- Asdecker, B and V Felch [ 2018 ] Development of an Industry 4.0 maturity model for the delivery process in supply chains . Journal of Modelling in Management , 13 , 840–883. https://doi.org/10.1108/JM2–03-2018-0042 . Crossref , Google Scholar
- Berger, R [ 2014 ] Industry 4.0 –The New Industrial Revolution . Alle Publikationen, Medien: Roland Berger. Google Scholar
- Canetta, L, A Barni and E Montini [ 2018 ] Development of a digitalization maturity model for the manufacturing sector . In Proceedings of the 2018 IEEE International Conference on Engineering, Technology and Innovation (ICE/ITMC) , pp. 1–7. https://doi.org/10.1109/ICE.2018.8436292 . Crossref , Google Scholar
- Ceruti, A, P Marzocca, A Liverani and C Bil [ 2019 ] Maintenance in aeronautics in an Industry 4.0 context: The role of Augmented Reality and Additive Manufacturing . Journal of Computational Design and Engineering , 6 (4), 516–526. https://doi.org/10.1016/j.jcde.2019.02.001 . Crossref , Google Scholar
- Côrte-Real, N, T Oliveira and P Ruivo [ 2017 ] Assessing business value of Big Data Analytics in European firms . Journal of Business Research , 70 , 379–390. Available at https://www.iqpc.com/media/7863/11710.pdf . Crossref , Google Scholar
- Davenport, T and J Dyché [ 2013 ] Big data in big companies . International Institute for Analytics , 3 , 1–31. Available at https://www.iqpc.com/media/7863/11710.pdf . Google Scholar
- De Carolis, A, M Macchi, E Negri and S Terzi [ 2017 ] A maturity model for assessing the digital readiness of manufacturing companies . In Proceedings of the IFIP International Conference on Advances in Production Management Systems . pp. 13–20. Cham: Springer. https://doi.org/10.1007/978-3-319-66923-6_2 . Crossref , Google Scholar
- De Weck, O, D Reed, S Sarma and M Schmidt [ 2014 ] Trends in advanced manufacturing technology innovation . Production in the Innovation Economy , 235 . https://doi.org/10.7551/mitpress/9780262019927.003.0009 . Crossref , Google Scholar
- Drath, R and A Horch [ 2014 ] Industry 4.0: Hit or hype?[industry forum] . IEEE Industrial Electronics Magazine , 8 (2), 56–58. https://doi.org/10.1109/MIE.2014.2312079 . Crossref , Google Scholar
- Erol, S, A Schumacher and W Sihn [ 2016 ] Strategic guidance towards Industry 4.0–a three-stage process model . In Proceedings of the International Conference on Competitive Manufacturing , Stellenbosch, South Africa , pp. 495–501. Available at https://www.researchgate.net/profile/Selim-Erol/publication/286937652_Strategic_guidance_towards_Industry_40_-_a_three-stage_process_model/links/5671898308ae90f7843f2d27/Strategic-guidance-towards-Industry-40-a-three-stage-process-model.pdf . Google Scholar
- Facchini, F, J Oleśków-Szłapka, L Ranieri and A Urbinati [ 2020 ] A maturity model for logistics 4.0: An empirical analysis and a roadmap for future research . Sustainability , 12 (1), 86. https://doi.org/10.3390/su12010086 Crossref , Google Scholar
- Gao, R, L Wang, R Teti, D Dornfeld, S Kumara, M Mori and M Helu [ 2015 ] Cloud-enabled prognosis for manufacturing . CIRP Annals , 64 (2), 749–772. https://doi.org/10.1016/j.cirp.2015.05.011 . Crossref , Google Scholar
- Gajsek, B, J Marolt, B Rupnik, T Lerher and M Sternad [ 2019 ] Using maturity model and discrete-event simulation for Industry 4.0 implementation . International Journal of Simulation Modelling , 18 (3), 488–499. https://doi.org/10.2507/IJSIMM18(3)489 . Crossref , Google Scholar
- Ganzarain Epelde, J and N Errasti Lozares (2016). Three stage maturity model in SME’s towards Industry 4.0, https://doi.org/10.3926/jiem.2073. Google Scholar
- Garay-Rondero, C, JL Martinez-Flores, NR Smith, SOC Morales and A Aldrette-Malacara [ 2020 ] Digital supply chain model in Industry 4.0 . Journal of Manufacturing Technology Management. https://doi.org/10.1108/JMTM-08-2018-0280 . Google Scholar
- Gökalp, E, U Şener and P Eren [ 2017 ] Development of an assessment model for industry 4.0: industry 4.0-MM . In Proceedings of the International Conference on Software Process Improvement and Capability Determination . pp. 128–142. Cham: Springer. Available at https://www.researchgate.net/profile/Ebru-Goekalp/publication/319640255_Development_of_an_Assessment_Model_for_Industry_40_Industry_40-MM/links/5a0c6895a6fdcc39e9bf7cca/Development-of-an-Assessment-Model-for-Industry-40-Industry-40-MM.pdf . Crossref , Google Scholar
- Gorecky, D, M Schmitt, M Loskyll and D Zühlke [ 2014 ] Human-machine-interaction in the industry 4.0 era . In Proceedings of the 12th IEEE International Conference on Industrial Informatics , pp. 289–294. Por Alegre, Brazil . https://doi.org/10.1109/INDIN.2014.6945523 Crossref , Google Scholar
- Gorkhali, A [ 2022 ] Industry 4.0 and enabling technologies: Integration framework and challenges . Journal of Industrial Integration and Management , 2250007. https://doi.org/10.1142/S2424862222500075 . Link , Google Scholar
- Hermann, M, T Pentek and B Otto [ 2016 ] Design principles for industrie 4.0 scenarios . In Proceedings of the Annual Hawaii International Conference on System Sciences . https://doi.org/10.1109/HICSS.2016.488 . Google Scholar
- Jæger, B, and L Halse [ 2017 ] The IoT technological maturity assessment scorecard: a case study of norwegian manufacturing companies . In Proceedings of the IFIP International Conference on Advances in Production Management Systems . pp. 143–150. Cham: Springer. https://doi.org/0.1007/978-3-319-66923-6_17 . Crossref , Google Scholar
- Kagermann, H, W Wahlster and J Helbig [ 2013 ] Final report of the industrie 4.0 working group . Federal Ministry of Education and Research , 82. Available at https://www.din.de/blob/76902/e8cac883f42bf28536e7e8165993f1fd/recommendations-for-implementing-industry-4-0-data.pdf . Google Scholar
- Lanza, G, P Nyhuis, S Ansari, T Kuprat and C Liebrecht [ 2016 ] Empowerment and implementation strategies for Industry 4.0 . ZWF Zeitschrift für wirtschaftlichen Fabrikbetrieb 111 (1-2), 76–79. https://doi.org/10.3139/104.111462 . Crossref , Google Scholar
- Lasi, H, P Fettke, HG Kemper, T Feld and M Hoffmann [ 2014 ] Industry 4.0 . Business & Information Systems Engineering , 6 (4), 239–242. https://doi.org/10.1007/s12599-014-0334-4 . Crossref , Google Scholar
- Li, L [ 2020 ] Education supply chain in the era of Industry 4.0 . Systems Research and Behavioral Science , 37 (4), 579–592. https://doi.org/10.1002/sres.2702 . Crossref , Google Scholar
- Lin, D, C Lee, H Lau and Y Yang [ 2018 ] Strategic response to Industry 4.0: an empirical investigation on the Chinese automotive industry . Industrial Management & Data Systems , 118 (3), 589–605. https://doi.org/10.1108/IMDS-09-2017-0403 . Crossref , Google Scholar
- Malladi, S [ 2013 ] Adoption of business intelligence & analytics in organizations–an empirical study of antecedents . Proceedings of the Nineteenth Americas Conference on Information Systems , Chicago, Illinois, USA , pp. 15–17, August, 2013 Google Scholar
- Moeuf, A, R Pellerin, S Lamouri, S Tamayo-Giraldo and R Barbaray [ 2018 ] The industrial management of SMEs in the era of Industry 4.0 . International Journal of Production Research , 56 (3), 1118–1136. https://doi.org/10.1080/00207543.2017.1372647 Crossref , Google Scholar
- Monostori, L [ 2014 ] Cyber-physical production systems: Roots, expectations and R&D challenges . Procedia Cirp , 17 , 9–13. https://doi.org/10.1016/j.procir.2014.03.115 . Crossref , Google Scholar
- Oliveira, B, L Liboni, L Cezarino, N Stefanelli and I Miura [ 2020 ] Industry 4.0 in systems thinking: From a narrow to a broad spectrum . Systems Research and Behavioral Science , 37 (4), 593–606. https://doi.org/10.1002/sres.2703 . Crossref , Google Scholar
- Pace, P, G Aloi, R Gravina, G Caliciuri, G Fortino and A Liotta [ 2018 ] An edge-based architecture to support efficient applications for healthcare industry 4.0 . IEEE Transactions on Industrial Informatics , 15 (1), 481–489. https://doi.org/10.1109/TII.2018.2843169 . Crossref , Google Scholar
- Pereira, A, and E de Oliveira Simonetto [ 2018 ] Indústria 4.0: conceitos e perspectivas para o Brasil . Revista da Universidade Vale do Rio Verde , 16 (1). https://doi.org/10.5892/ruvrd.v16i1.4938 . Crossref , Google Scholar
- Rafael, L, G Jaione, L Cristina and S Ibon [ 2020 ] An Industry 4.0 maturity model for machine tool companies . Technological Forecasting and Social Change , 159 , 120203. https://doi.org/10.1016/j.techfore.2020.120203 Crossref , Google Scholar
- Rüßmann, M, M Lorenz, P Gerbert, M Waldner, J Justus, P Engel and M Harnisch [ 2015 ] Industry 4.0: The future of productivity and growth in manufacturing industries . Boston Consulting Group , 9 (1), 54–89. Google Scholar
- Sanders, A, C Elangeswaran and J Wulfsberg [ 2016 ] Industry 4.0 implies lean manufacturing: Research activities in industry 4.0 function as enablers for lean manufacturing . Journal of Industrial Engineering and Management , 9 (3), 811–833. https://doi.org/10.3926/jiem.1940 Crossref , Google Scholar
- Santos, R and J Martinho [ 2019 ] An Industry 4.0 maturity model proposal . Journal of Manufacturing Technology Management , 32 (3). https://doi.org/10.1108/JMTM-09-2018-0284 . Google Scholar
- Schmidt, R, M Möhring, R Härting, C Reichstein, P Neumaier and P Jozinović [ 2015 ] Industry 4.0-potentials for creating smart products: empirical research results . In Proceedings of the International Conference on Business Information Systems . pp. 16–27. Cham: Springer. https://doi.org/10.1007/978-3-319-19027-3_2 . Crossref , Google Scholar
- Schumacher, A, S Erol and W Sihn [ 2016 ] A maturity model for assessing Industry 4.0 readiness and maturity of manufacturing enterprises . Procedia Cirp , 52 , 161–166. https://doi.org/10.1016/j.procir.2016.07.040 Crossref , Google Scholar
- Schumacher, A, T Nemeth and W Sihn [ 2019 ] Roadmapping towards industrial digitalization based on an Industry 4.0 maturity model for manufacturing enterprises . Procedia Cirp , 79 , 409–414. https://doi.org/10.1016/j.procir.2019.02.110 Crossref , Google Scholar
- Shan, S, X Wen, Y Wei, Z Wang and Y Chen [ 2020 ] Intelligent manufacturing in industry 4.0: A case study of Sany heavy industry . Systems Research and Behavioral Science , 37 (4), 679–690. https://doi.org/10.1002/sres.2709 Crossref , Google Scholar
- Sikorski, JJ, J Haughton and M Kraft [ 2017 ] Blockchain technology in the chemical industry: Machine-to-machine electricity market . Applied Energy , 195 , 234–246. https://doi.org/10.1016/j.apenergy.2017.03.039 Crossref , Google Scholar
- Szász, L, K Demeter, B Rácz and D Losonci [ 2020 ] Industry 4.0: a review and analysis of contingency and performance effects . Journal of Manufacturing Technology Management . https://doi.org/10.1108/JMTM-10-2019-037 . Crossref , Google Scholar
- Tonelli, F, M Demartini, A Loleo and C Testa [ 2016 ] A novel methodology for manufacturing firms value modeling and mapping to improve operational performance in the industry 4.0 era . Procedia CIRP , 57 , 122–127. https://doi.org/10.1016/j.procir.2016.11.022 . Crossref , Google Scholar
- Weber, C, J Königsberger, L Kassner and B Mitschang [ 2017 ] M2DDM–a maturity model for data-driven manufacturing . Procedia CIRP , 63 , 173–178. https://doi.org/10.1016/j.procir.2017.03.309 . Crossref , Google Scholar
- Werner-Lewandowska, K and M Kosacka-Olejnik [ 2019 ] Logistics 4.0 maturity in service industry: Empirical research results . Procedia Manufacturing , 38 , 1058–1065. https://doi.org/10.1016/j.promfg.2020.01.192 . Crossref , Google Scholar
- Xu, L, E Xu and L Li [ 2018 ] Industry 4.0: state of the art and future trends . International Journal of Production Research , 56 (8), 2941–2962. https://doi.org/10.1080/00207543.2018.1444806 . Crossref , Google Scholar
- Zambon, I, M Cecchini, G Egidi, M Saporito and A Colantoni [ 2019 ] Revolution 4.0: Industry vs. agriculture in a future development for SMEs . Processes , 7 (1), 36. https://doi.org/10.3390/pr7010036 . Crossref , Google Scholar
- Anna Strimovskaya
- Research on Intelligent Supply Chain Management Mode under the Background of Industry 4.0 Xu Liu 22 September 2023 | BCP Business & Management, Vol. 50
- Maturity and resilience in supply chains: a systematic review of the literature Luiz Carlos Roque Júnior, Guilherme F. Frederico and Maykon Luiz Nascimento Costa 4 January 2023 | International Journal of Industrial Engineering and Operations Management, Vol. 5, No. 1
Recommended
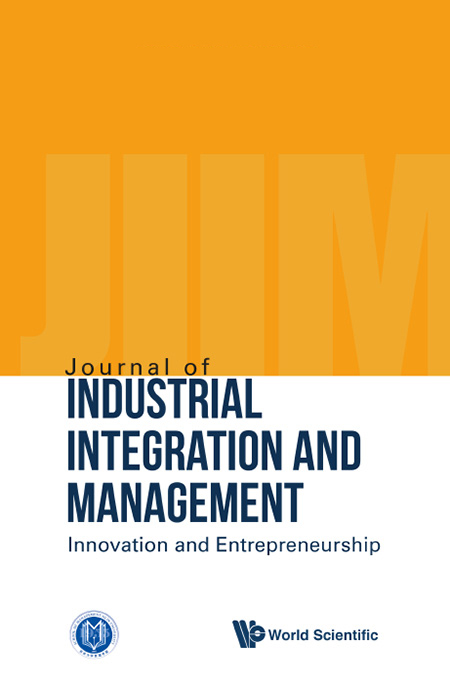
Received 12 August 2021 Revised 17 August 2022 Accepted 22 August 2022 Published: 18 October 2022
Industry 4.0: 7 Real-World Examples of Digital Manufacturing in Action

Industry 4.0, IIoT, and digitisation are currently some of the most-discussed and yet least understood topics within manufacturing today.
With still a lot of confusion surrounding Industry 4.0, today we’ll be exploring the key technologies behind Industry 4.0, as well as real-world applications.
Industry 4.0 in a nutshell
Industry 4.0 is signalling a change in the traditional manufacturing landscape. Also known as the Fourth Industrial Revolution, Industry 4.0 encompasses three technological trends driving this transformation: connectivity, intelligence and flexible automation .
Industry 4.0 converges IT (Information Technology) and OT (Operational Technology), to create a cyber-physical environment.
This convergence has been made possible thanks to the emergence of digital solutions and advanced technologies, which are often associated with Industry 4.0. These include:
- Industrial Internet of Things
- Cloud computing
- Additive manufacturing (AM)
- Advanced robotics
- Augmented and virtual reality (AR/VR)
These technologies are helping to drive manufacturing’s digital transformation through the integration of previously disparate systems and processes through interconnected computer systems across the value and supply chain.
Embracing Industry 4.0, digital manufacturing and the interconnectivity that comes with it opens a myriad of benefits for companies, including greater agility, flexibility and operational performance.
1. Industrial Internet of Things
At the heart of Industry 4.0 is the Internet of Things (IoT).
Put simply, IoT refers to a network of physical devices that are digitally interconnected, facilitating the communication and exchange of data through the Internet. These smart devices could be anything from smartphones and household appliances to cars and even buildings.
Industrial IoT is a subset of the Internet of Things, where various sensors, Radio Frequency Identification (RFID) tags, software and electronics are integrated with industrial machines and systems to collect real-time data about their condition and performance.
IIoT has many use cases, with asset management and tracking being one of the major applications of the technology today.
For example, IIoT can be used is to prevent the overstocking or understocking of inventory.
One way to achieve this is to use shelf-fitted sensors and weighing devices to broadcast inventory information to your warehouse management system. Putting such a system in place allows warehouse managers to monitor inventory levels, thereby gaining real-time visibility and control over the inventory.
Let’s take a look at how BJC HealthCare uses an integrated inventory management solution to achieve cost-savings in its supply chain.

Spotlight: BJC HealthCare adopts IoT for inventory and supply chain management
BJC HealthCare is a healthcare service provider that operates 15 hospitals in Missouri and Illinois.
The company deploys radio frequency identification (RFID) technology to track and manage thousands of medical supplies. RFID technology uses radio waves to read and capture information stored on a tag attached to an object, such as healthcare supplies.
Previously, the process of tracking inventory involved a lot of manual labour. However, monitoring inventory manually can be a challenge, since hospitals purchase a variety of products from suppliers and store a lot of items on site for specific procedures.
In some cases, products’ expiration dates will need to be closely monitored, while the loss of stock can lead to a lot of time spent on conducting inventory checks.
For these reasons, BJC decided to implement RFID tagging technology in 2015.
Since implementing the technology, BJC has been able to reduce the amount of stock kept onsite at each facility by 23 per cent. The company predicts that it will see ongoing savings of roughly $5 million annually, once RFID tagging is fully implemented this year.
As this example demonstrates, IIoT can significantly improve operations, increase efficiency, reduce costs and provide valuable real-time visibility across the supply chain.
2. Big Data and Analytics
Big Data refers to the large and complex data sets generated by IoT devices. This data comes from a wide range of cloud and enterprise applications, websites, computers, sensors, cameras and much more — all coming in different formats and protocols.
In the manufacturing industry, there are many different types of data to take into consideration, including the data coming from production equipment fitted with sensors and databases from ERP, CRM and MES systems.
But how can manufacturers convert the data collected into actionable business insights and tangible benefits?
With data analysis.
When it comes to data, the use of data analytics is essential to convert data to information that can deliver actionable insights.
Machine learning models and data visualisation can aid data analytics processes. Broadly speaking, machine learning techniques apply powerful computational algorithms to process massive data sets, while data visualisation tools enable manufacturers to more easily comprehend the story the data tells.
Ultimately, by taking previously isolated data sets, collecting and analysing them, companies are now able to find new ways to optimise the processes that have the greatest effect on yield.
Spotlight: Big Data decision-making at Bosch Automotive factory in China

Combining IIoT and Big Data is a recipe Bosch is using to drive the digital transformation of its Bosch Automotive Diesel System factory in Wuxi, China.
The company connects its machinery to monitor the overall production process at the core of its plant. This is achieved by embedding sensors into the factory’s machines which are then used to collect data about the machines’ conditions and cycle time.
Once collected, advanced data analytics tools process the data in real time and alert workers when any bottlenecks in the production operations have been identified.
Taking this approach helps to predict equipment failures, enabling the factory to schedule maintenance operations well before any failures occur.
As a result, the factory is able to keep its machinery running and operating for longer stretches of time.
The company states that using data analysis in this way has contributed to more than 10% output increase in certain areas, whilst improving delivery and customer satisfaction.
Ultimately, a greater insight into the plant’s operations supports better and faster decision-making throughout the entire organisation, enabling it to reduce equipment downtime and optimise production processes.
3. Cloud computing

For decades, manufacturers have been collecting and storing data with the goal of improving operations.
However, with the advent of IoT and Industry 4.0, the reality is that data is being generated at a staggering speed and at high volumes, making it impossible to handle manually. This creates a need for an infrastructure that can store and manage this data more efficiently.
This is where cloud computing comes in.
Cloud computing offers a platform for users to store and process vast amounts of data on remote servers. It enables organisations to use computer resources without having to develop a computing infrastructure on premise.
The term cloud computing refers to information being stored in the “cloud”, accessed remotely via the Internet. In itself, cloud computing is not a solution on its own, but enables the implementation of other solutions that once required heavy computing power.
The capability of cloud computing to provide scalable computing resources and storage space enables companies to capture and apply business intelligence through the use of big data analytics, helping them to consolidate and streamline manufacturing and business operations.
Manufacturers’ global spending on cloud computing platforms is predicted to reach $9.2 billion in 2021, according to IDC. A key factor behind this adoption is the benefit of being able to centralise operations, eliminating so that information can be shared across an entire organisation.
According to one IDC survey , Quality Control, Computer-Aided Engineering and Manufacturing Execution Systems (MES) are the three most widely adopted systems in the cloud.
Clearly, cloud computing is transforming virtually every facet of manufacturing, from workflow management to production operations – and even product qualification.
Spotlight: Volkswagen creates Automotive Cloud
Connected cars are a big new trend in the automotive industry, having emerged as an opportunity to offer digital added-value services for customers. One of the first automakers to jump on this trend is Volkswagen, which joined forces with Microsoft to develop a cloud network, the “Volkswagen Automotive Cloud”.
The technology, planned for 2020, will offer a range of features, including smart home connectivity, a personal digital assistant, predictive maintenance service, media streaming and updates.
Volkswagen aims to add over 5 million Volkswagen brand offerings per year to its Internet of Things (IoT) with the help of this cloud service.
As the automotive industry makes impressive strides in developing advanced autonomous and electric vehicles, carmakers need to come up with an effective approach of managing and transmitting large amounts of data to their vehicles. Incorporating cloud-based storage and communication platform emerges as an effective way to overcoming the challenges faced by these automakers.
4. Advanced Robotics
While robotics have been used in manufacturing for decades, Industry 4.0 has given new life to this technology.
With recent advancements in technology, a new generation of advanced robotics is emerging, capable of performing difficult and delicate tasks. Powered by cutting-edge software and sensors, they can recognise, analyse and act upon information they receive from the environment, and even collaborate and learn from humans.
One area of robotics gaining significant traction is collaborative robots (“cobots”), designed to work safely around people, freeing workers from repetitive and dangerous tasks.
Spotlight: Fetch Robotics help DHL improve warehouse operations
![case study on industry 4 0 Fetch_Robotics_in_a_warehouse_[Image_credit_Fetch_robotics]](https://amfg.ai/wp-content/uploads/2019/03/Fetch_Robotics_in_a_warehouse_Image_credit_Fetch_robotics.jpg)
California-based Fetch Robotics has developed collaborative Autonomous Mobile Robots (AMRs) for locating, tracking, and moving inventory in warehouse and logistics facilities.
A DHL distribution centre in the Netherlands is using Fetch AMRs to perform pick and place operations. At DHL, AMRs autonomously move across the facility alongside the workers, automatically learning and sharing the most efficient travel routes. Using self-driving robots in this way can help reduce order cycle time by up to 50% and provide up to twice the picking productivity gain, according to the company.
As robots become more autonomous, flexible and cooperative, they will be able to tackle even more complex assignments, relieving the workers from monotonous tasks and increasing productivity on the factory floor.
5. Additive Manufacturing

Alongside robotics and intelligent systems, additive manufacturing, or 3D printing, is a key technology driving Industry 4.0. Additive manufacturing works by using digital 3D models to create parts with a 3D printer layer by layer.
Within the context of Industry 4.0, 3D printing is emerging as a valuable digital manufacturing technology. Once solely a rapid prototyping technology, today AM offers a huge scope of possibilities for manufacturing from tooling to mass customisation across virtually all industries.
It enables parts to be stored as design files in virtual inventories, so that they can be produced on-demand and closer to the point of need — a model known as distributed manufacturing .
Such a decentralised approach to manufacturing can reduce transportation distances, and hence costs, as well as simplify inventory management by storing digital files instead of physical parts.
Spotlight: Fast Radius’ digital additive manufacturing solutions to enable new business models
There is a multitude of examples of additive manufacturing being put to great use , but a key example is, perhaps, Fast Radius.

The contract manufacturer, which focuses on AM but also offers CNC machining and injection moulding, has production sites in Chicago, Singapore and at the UPS Worldport facility. This makes Fast Radius well-positioned to drive its vision of fast-turnaround and mass customisation of products with the help of advanced manufacturing technologies.
A key factor behind Fast Radius’ agility and flexibility is its proprietary technology platform.
The platform can collect data and findings from every part design that is stored and manufactured in the Fast Radius virtual warehouse. The data helps teams to identify applications suitable for 3D printing and evaluate engineering and economic challenges of producing a component this way.
Furthermore, the company offers supply chain optimisation through its virtual inventory. For example, Fast Radius created a virtual parts warehouse consisting of 3,000 items for a heavy equipment manufacturer. With the high costs involved in storing rarely ordered parts, this approach is an innovative solution for supply chain management.
6. Digital Twins
The concept of a digital twin holds great promise for optimising the performance and maintenance of industrial systems. Global research firm, Gartner, predicts that by 2021, 50% of large industrial companies will be using digital twins to monitor and control their assets and processes.
A digital twin is a digital representation of a real-world product, machine, process, or system, that allows companies to better understand, analyse and optimise their processes through real-time simulation.
While digital twins can be confused with simulation used in engineering, there is much more to this concept.
Unlike engineering simulations, a digital twin runs an online simulation, based on data received from sensors connected to a machine or other device.
As an IIoT device sends data almost in real time, a digital twin is able to collect this data continuously, maintaining its fidelity with the original throughout the lifespan of the product or system.
This enables the digital twin to predict potential issues so that preemptive measures can be taken. For example, an operator can use a digital twin to identify why a part is malfunctioning or to predict the lifetime of a product. This continuous simulation helps to improve designs of products as well as to ensure equipment uptime.
This use of digital twins has long been an important tool in demanding aerospace, heavy machinery and automotive applications. Now, advances in computing technology, machine learning and sensors are expanding the concept of digital twinning across other industries.
Spotlight: Racing to win with digital twins
![case study on industry 4 0 Siemen's_Digital_Twin_technology_can_be_used_to_create_a_virtual_version_of_factory_assets_[Image_Credit_Siemens]](https://amfg.ai/wp-content/uploads/2019/03/Siemens_Digital_Twin_technology_can_be_used_to_create_a_virtual_version_of_factory_assets_Image_Credit_Siemens.jpg)
Motor racing teams face extremely challenging requirements when it comes to product development, and American pro racing squad Team Penske is no exception.
In a bid to speed up the race car development process, Team Penske partnered with Siemens last year, gaining access to advanced digital design and simulation solutions – including digital twins.
Having digital twins provides Team Penske engineers with a virtual test bed for innovating new parts, optimising car performance before they ever touch the physical car.
A race car digital twin is based on sensors fitted onto a real car. These sensors collect data such as tire pressure, engine control and wind speed, which is then converted into a virtual car model. It’s this model that allows engineers to test different design configurations, making effective, data-driven design changes at a very rapid pace.
For Team Penske, this ultimately equates to cheaper, more resource-efficient product testing process and, hopefully, a way towards developing faster vehicles.
7. Augmented reality
Despite its uptake in consumer applications, the manufacturing industry is just beginning to explore the benefits of Augmented Reality (AR) technology. And yet, there is a huge untapped potential for the technology, from helping with assembly processes to helping to maintain manufacturing equipment.
Augmented reality bridges the gap between the digital and physical worlds by superimposing virtual images or data onto a physical object. For this, the technology uses AR-capable devices, such as smartphones, tablets and smart glasses.
Let’s take a medical instance as an example — a surgeon using AR glasses during a surgical operation. The glasses could overlay data from patient’s MRI and CT scans, such as nerves, major blood vessels and ducts, onto the patient, and highlight them in colour. This helps the surgeon to find the safest path into the region that needs invasion, minimising the risk of complications and improving surgeon’s precision.
In the context of manufacturing, AR could enable workers to speed up the assembly process and improve decision-making. For example, AR glasses could be used to project data, such as layouts, assembly guidelines, sites of possible malfunction, or a serial number of components, on the real part, facilitating faster and easier work procedures.
Spotlight: AR increases productivity at GE
General Electric offers a glimpse at how AR technology can empower manufacturing . The company is currently piloting the use of AR glasses at its jet engine manufacturing facility in Cincinnati. Before using these smart glasses, jet engine makers often had to stop what they were doing in order to check their manuals and ensure tasks were being performed correctly.
However, with AR glasses, they can now receive digitised instructions in their field of view. The mechanics can also access training videos or use voice commands to contact experts for immediate assistance.
During the pilot, GE reports that the productivity of workers using smart wearables increased by up to 11%, compared to previously. Ultimately, this approach could offer a tremendous potential to minimise errors, cut down on costs and improve product quality.
Even with this example from GE, we’re still scratching the surface when it comes to implementing AR within the manufacturing context.
Take a closer look at GE’s AR glasses in action:
Riding the Wave of Digital Manufacturing
With new, digital technologies emerging, now is an exciting time for the manufacturing industry. The wave of new technologies opens up opportunities for companies to take steps towards greater flexibility, sustainability and productivity. Industry 4.0 is also facilitating new ways for humans and machines to work together, empowering businesses to achieve greater insights, reduce the risk of error and make better decisions.
Ultimately, Industry 4.0 is set to take root across the manufacturing ecosystem. But only by understanding and harnessing the technologies driving Industry 4.0, will manufacturers stay on the cutting edge of this new digital era.
Subscribe to our newsletter
Get our best content straight to your inbox
Related posts

Thank you for subscribing!
You'll receive our latest content every week, straight to your inbox.
Request sent successfully!
This website uses cookies to improve your user experience and analyse site traffic. Read about how we use cookies and how you can control them by clicking "Privacy Preferences". If you continue to use this site, you consent to our use of cookies.
Privacy Preference Center
Consent management, performance.
When you visit any website, it may store or retrieve information on your browser, mostly in the form of cookies. This information might be about you, your preferences or your device and is mostly used to make the site work as you expect it to. The information does not usually directly identify you, but it can give you a more personalized web experience.
Privacy Policy
Cookies that are necessary for the site to function properly.
Cookies Used
These are used to track user interaction and detect potential problems. These help us improve our services by providing analytical data on how users use this site.
mouseflow.com
Information
- Author Services
Initiatives
You are accessing a machine-readable page. In order to be human-readable, please install an RSS reader.
All articles published by MDPI are made immediately available worldwide under an open access license. No special permission is required to reuse all or part of the article published by MDPI, including figures and tables. For articles published under an open access Creative Common CC BY license, any part of the article may be reused without permission provided that the original article is clearly cited. For more information, please refer to https://www.mdpi.com/openaccess .
Feature papers represent the most advanced research with significant potential for high impact in the field. A Feature Paper should be a substantial original Article that involves several techniques or approaches, provides an outlook for future research directions and describes possible research applications.
Feature papers are submitted upon individual invitation or recommendation by the scientific editors and must receive positive feedback from the reviewers.
Editor’s Choice articles are based on recommendations by the scientific editors of MDPI journals from around the world. Editors select a small number of articles recently published in the journal that they believe will be particularly interesting to readers, or important in the respective research area. The aim is to provide a snapshot of some of the most exciting work published in the various research areas of the journal.
Original Submission Date Received: .
- Active Journals
- Find a Journal
- Proceedings Series
- For Authors
- For Reviewers
- For Editors
- For Librarians
- For Publishers
- For Societies
- For Conference Organizers
- Open Access Policy
- Institutional Open Access Program
- Special Issues Guidelines
- Editorial Process
- Research and Publication Ethics
- Article Processing Charges
- Testimonials
- Preprints.org
- SciProfiles
- Encyclopedia
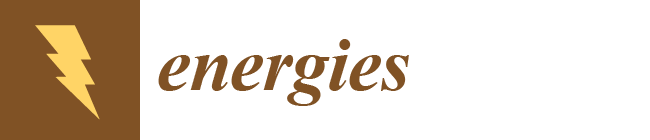
Article Menu
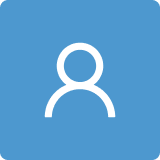
- Subscribe SciFeed
- Recommended Articles
- Google Scholar
- on Google Scholar
- Table of Contents
Find support for a specific problem in the support section of our website.
Please let us know what you think of our products and services.
Visit our dedicated information section to learn more about MDPI.
JSmol Viewer
Implementing industry 4.0: an in-depth case study integrating digitalisation and modelling for decision support system applications.
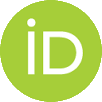
1. Introduction
2. background, 2.1. production process.
- Issue Material: the raw material is allocated to each of the product families. Operators use printers and barcode scanners to enable tracking.
- Saw: The material undergoes processing in machine saws to obtain blocks of the required size for each product family.
- Machine: The individual blocks are processed using Computer Numerical Control (CNC) machines to obtain the finished product.
- Inspect: The products are inspected for compliance and defective ones are scrapped. Various machines are used during this stage.
- Clean: The products are cleaned and sterilised in a multi-stage cleanline. The stages include water-baths as well as air-dryers. The parameters such as temperature, flow-rate, pH, etc., are tightly controlled.
- Label: Each product is assigned labels.
- Package: The products are sealed and packaged.
- Ship: The products are shipped from the facility.
- Production Specific Assets: this category includes all the machine tools and devices that are directly involved in the production process such as electric saws, CNC machines, cleanlines, and sealing and packaging equipment.
- Production Support Assets: this includes the auxiliary assets that are not directly involved in the production process but nevertheless serve other critical aplications. Examples include Air Handling Units (AHUs), chilled water system, and air compressors.
- Renewable Energy Assets: this category includes the wind turbines, photovoltaic panels and other assets that provide the facility with alternate sources of electricity.
2.2. Project Plan
- Project Charter : a project charter was created using the template shown in Figure 4 . This identified all the stakeholders, including, business management, production engineers, utilities technicians, research partners, and representatives within the funding agency. The key tasks were identified and assigned. Additionally, the project management aspects were also included.
- Process Overview : the high-level view of the production process is shown in Figure 2 . The intricate details of the process were discussed through several meetings, some of which were held at the facility. The research partners were also given the opportunity to shadow production engineers to better understand the process.
- Digitalisation : Monitoring assets in real-time as well as equipping them with data-driven decision support systems.
- Energy Efficiency : improving energy efficiency by optimising operation or detecting and addressing faults. This aspect also includes the optimisation of renewable energy sources available at the facility.
- Cost/Energy Savings : The above two will lead to savings in terms of electricity consumption as well as cost.
- Data Catalogue : A data catalogue with a high-level overview of all the data sources was developed as shown in Table 3 . This also identifies the personnel responsible for maintaining the datasets.
- Data Architecture : This step delves into the details of each data source. While some data points correspond to physical assets, others reflect overall aggregates coming from a higher level of abstraction.
- Data Assessment : A detailed data assessment was carried out for each of the asset types identified in the data catalogue. The minimum data requirements for each of the functional blocks were listed. For instance, for the production assets, energy sensors are the minimum requirement. The details of each will be given in the respective sections.
- Connection Plan : from the above six steps, it emerged that the minimum data requirements were not fulfilled for most of the functional blocks. In some cases, the assets were not equipped with sensors, whereas in others, the data were being collected but not stored on the site’s data historian. One of the key steps taken was the installation of energy meters on most of the production assets. As can be seen in Table 2 , having 85% of the production assets equipped with real-time energy monitoring capabilities was one of the concrete targets of this project.
- Stakeholder Engagement: for the success of an Industry 4.0 project, it is important to engage business managers, operators, and research partners from inception to conclusion.
- Business Understanding: Industry 4.0 is not just about upgrading technology; it is crucial to quantify the impact of these advancements on business operations.
- Tasks and Interdependencies: the overall objectives need to be broken down into individual tasks for any project. However, due to the interconnected nature of Industry 4.0 functional blocks, the interdependencies must also be identified.
- Data Assessment: data play a central role in Industry 4.0. However, the initial data seldom meets the requirements to be able to support the project objectives. A connection plan is devised to obtain the necessary additional data as well as establish an appropriate digital architecture.
- Energy modelling and analytics
- Fault detection and diagnosis
- Renewable integration
- Advanced Visualisation Tools
3. Energy Efficiency
- Product code
- Machine code
- Number of units produced/processed
- Number of parts scrapped
3.2. Data Analysis
3.3. idling energy, 3.4. energy models.
- Production Planning: the model aids production planning by providing an estimate of the energy required to produce a given number of units of a certain type of product within a shift
- Anomaly Detection: as can be seen in Figure 7 , the model also flags some anomalous energy consumption patterns that might be caused due to tool wear or faults in the auxiliary machine systems. Thus, the model can also serve as a fault detection tool.
4. Fault Detection And Diagnosis
5. renewable integration.
- Renewable energy sources: there is a wind turbine and a solar plant in this factory.
- Battery bank (B). Store energy and discharge when it is needed.
- Combined Heat and Power (CHP). Produced energy and heat from gas.
- Remaining consumption: all consumption that cannot be manipulated. It is about 90% of the total.
- Manipulable demand as a group of CNCs.
5.1. Renewable Energy Sources Modelling
Neurofuzzy model of res, 5.2. tool architecture.
- Actions that are executed automatically, such as battery operation.
- Operator aids for starting up the various machines in the manufacturing process. These are shown through the visualisation tool below.
5.2.1. Model Predictive Control
5.2.2. data processing module.
- Input data for the models such as wind speed, solar radiation, electricity market prices, and gas prices. These data are obtained from forecasting databases that are updated in real time.
- Feedback from the plant. Data from the plant’s sensors, warnings from the plant’s workers, production carried out, etc.
- Data from other tools. Among these data are the schedule optimised by the Sustainable Production Planning tool. This tool optimises the daily schedule by taking into account the carbon footprint, CO 2 emission reductions, life cycle of the assets, etc. This schedule is incorporated into the MPC as a starting point for optimisation at the beginning of the day.
5.3. Results
- The profile of the manipulable process has been adjusted to times when the market price is lower or renewable energy production is higher.
- The inclusion of the battery means that renewable energy losses are minimised, bearing in mind that at the end of the day there is an excess of renewable energy that cannot be harnessed on this day.
- In some instances, power is produced from CHP to take advantage of the weighted price difference between gas and electricity from the market.
6. User Interface
- Authorisation Controller: facilitates integration with the DENiM authorisation and access control mechanisms to ensure appropriate authorisation and access control to data sources.
- Historical Data Controller: provides a mechanism to the load, query and interrogate of historical data received via the DENiM connectors.
- Site Management Controller: captures a hierarchy of the physical assets, properties and data for a specific site configuration; this is supported by the use of the DENiM semantic model.
- Events Controller: manages any notifications or updates that need to be propagated to the user (e.g., fault alert).
- Data Models: definition of local data models that align and map to visual widgets and components.
6.1. Dashboard Visualisation
6.2. digital twin visualisation, 7. key performance indicators (kpis).
- KPI-1 85% of Production Assets Monitored : Of the assets within scope, the significant energy users (SEUs) were defined to be those with a rated power consumption of more than 3 kW. With the connection plan fully implemented, 95% of the identified assets have been equipped with IoT sensors and meters, providing monitoring of energy consumption of these assets.
- KPI-2 25% of Production Assets equipped with FDD : The production assets include the saws, lathes and cleanlines. Energy models have been developed for the lathes, which also aid in detecting abnormal energy consumption patterns and offer limited but valuable fault detection. Thus, this KPI has also been reached, with approximately 70% of assets being equipped with fault detection.
- KPI-3 50% of Production Support Assets equipped with FDD : Besides the AHUs, the assets within scope include Chillers and air compressors. Due to the lack of data, this KPI has not been met. However, when data are made available, it is possible to extend the developed FDD tools to cover 75% of the assets.
- KPI-4 Reduce Complexity of Data Collection and Energy Audits : The complexity of data generation was reduced with two digital services deployed, which automatically computed the performance indicators for all assets. Additionally, the integration of the visualisation tool on the platform enabled easy access and visualisation of data aggregated from multiple sources. To enhance the accuracy and quickly identify the faults with sensors, a data validation tool was also incorporated that implemented five different kinds of data checks.
- KPI-5 95% Accuracy in Predicting Energy Consumption of Production Assets : This KPI could not be achieved due to the lack of data. For accurate energy prediction, monitoring the process parameters is a must. Nevertheless, the simple models based on linear regression are still helpful in production planning as well as anomaly detection.
- KPI-6 10% Idle Energy Reduction and Schedule Optimisation : As mentioned in Section 3 , the data analysis is based on shift-wise aggregates. In the various scenarios shown, this KPI is met more than satisfactorily. Furthermore, data at a higher resolution will only improve this KPI.
- KPI-7 10% Savings by Optimising Renewable Integration : The renewable integration module was shown to improve renewable integration by approximately 18%. Thus, this KPI is also met.
- KPI-8 15% Savings through FDD : Considering the AHU FDD, and assuming that the scenario for 2022 is generally valid for any year, savings of approximately 16% can be made by quickly repairing faults that consume excessive energy compared to normal operation.
8. Conclusions
Author contributions, data availability statement, conflicts of interest.
- Kagermann, H.; Lukas, W.D.; Wahlster, W. Industrie 4.0: Mit dem Internet der Dinge auf dem Weg zur 4. industriellen Revolution. VDI Nachrichten 2011 , 13 , 2–3. [ Google Scholar ]
- Kagermann, H. Change through digitization—Value creation in the age of Industry 4.0. In Management of Permanent Change ; Springer: Berlin/Heidelberg, Germany, 2014; pp. 23–45. [ Google Scholar ]
- Rivera, J. Gartner’s 2015 Hype Cycle for Emerging Technologies Identifies the Computing Innovations That Organizations Should Monitor. Available online: https://www.gartner.com/en/newsroom/press-releases/2015-08-18-gartners-2015-hype-cycle-for-emerging-technologies-identifies-the-computing-innovations-that-organizations-should-monitor (accessed on 9 November 2023).
- Zhong, R.Y.; Ge, W. Internet of things enabled manufacturing: A review. Int. J. Agil. Syst. Manag. 2018 , 11 , 126–154. [ Google Scholar ] [ CrossRef ]
- Cioffi, R.; Travaglioni, M.; Piscitelli, G.; Petrillo, A.; De Felice, F. Artificial intelligence and machine learning applications in smart production: Progress, trends, and directions. Sustainability 2020 , 12 , 492. [ Google Scholar ] [ CrossRef ]
- Damiani, L.; Demartini, M.; Guizzi, G.; Revetria, R.; Tonelli, F. Augmented and virtual reality applications in industrial systems: A qualitative review towards the Industry 4.0 era. IFAC-Pap. 2018 , 51 , 624–630. [ Google Scholar ] [ CrossRef ]
- Zheng, P.; Wang, H.; Sang, Z.; Zhong, R.Y.; Liu, Y.; Liu, C.; Mubarok, K.; Yu, S.; Xu, X. Smart manufacturing systems for Industry 4.0: Conceptual framework, scenarios, and future perspectives. Front. Mech. Eng. 2018 , 13 , 137–150. [ Google Scholar ] [ CrossRef ]
- Müller, J.M.; Kiel, D.; Voigt, K.I. What drives the implementation of Industry 4.0? The role of opportunities and challenges in the context of sustainability. Sustainability 2018 , 10 , 247. [ Google Scholar ] [ CrossRef ]
- Directorate-General for Research and Innovation, European Commission. Industry 5.0—Towards a Sustainable, Human-Centric and Resilient European Industry. Available online: https://research-and-innovation.ec.europa.eu/knowledge-publications-tools-and-data/publications/all-publications/industry-50-towards-sustainable-human-centric-and-resilient-european-industry_en (accessed on 28 January 2024).
- Bajic, B.; Rikalovic, A.; Suzic, N.; Piuri, V. Industry 4.0 implementation challenges and opportunities: A managerial perspective. IEEE Syst. J. 2020 , 15 , 546–559. [ Google Scholar ] [ CrossRef ]
- Rikalovic, A.; Suzic, N.; Bajic, B.; Piuri, V. Industry 4.0 implementation challenges and opportunities: A technological perspective. IEEE Syst. J. 2021 , 16 , 2797–2810. [ Google Scholar ] [ CrossRef ]
- The DENiM Consortium. DENiM: Unlocking the Energy Saving Potential in Manufacturing Systems. Available online: https://denim-fof.eu/ (accessed on 7 December 2023).
- Buer, S.V.; Semini, M.; Strandhagen, J.O.; Sgarbossa, F. The complementary effect of lean manufacturing and digitalisation on operational performance. Int. J. Prod. Res. 2021 , 59 , 1976–1992. [ Google Scholar ] [ CrossRef ]
- Frank, A.G.; Dalenogare, L.S.; Ayala, N.F. Industry 4.0 technologies: Implementation patterns in manufacturing companies. Int. J. Prod. Econ. 2019 , 210 , 15–26. [ Google Scholar ] [ CrossRef ]
- Pozzi, R.; Rossi, T.; Secchi, R. Industry 4.0 technologies: Critical success factors for implementation and improvements in manufacturing companies. Prod. Plan. Control 2023 , 34 , 139–158. [ Google Scholar ] [ CrossRef ]
- Clancy, R.; Bruton, K.; O’Sullivan, D.T.; Cloonan, A.J. The HyDAPI framework: A versatile tool integrating Lean Six Sigma and digitalisation for improved quality management in Industry 4.0. Int. J. Lean Six Sigma 2022 . [ Google Scholar ] [ CrossRef ]
- Isermann, R. Fault-Diagnosis Applications: Model-Based Condition Monitoring: Actuators, Drives, Machinery, Plants, Sensors, and Fault-Tolerant Systems ; Springer Science & Business Media: Berlin/Heidelberg, Germany, 2011. [ Google Scholar ]
- Roth, K.W.; Westphalen, D.; Feng, M.; Llana, P.; Quartararo, L. Energy Impact of Commercial Building Controls and Performance Diagnostics: Market Characterization. In Energy Impact of Building Faults and Energy Savings Potential ; National Technical Reports Library: Lawrence, KS, USA; U.S. Department of Commerce: Washingtion, DC, USA, 2005; p. 413. [ Google Scholar ]
- Matetić, I.; Štajduhar, I.; Wolf, I.; Ljubic, S. A Review of Data-Driven Approaches and Techniques for Fault Detection and Diagnosis in HVAC Systems. Sensors 2022 , 23 , 1. [ Google Scholar ] [ CrossRef ] [ PubMed ]
- Zhao, Y.; Li, T.; Zhang, X.; Zhang, C. Artificial intelligence-based fault detection and diagnosis methods for building energy systems: Advantages, challenges and the future. Renew. Sustain. Energy Rev. 2019 , 109 , 85–101. [ Google Scholar ] [ CrossRef ]
- Chen, Z.; O’Neill, Z.; Wen, J.; Pradhan, O.; Yang, T.; Lu, X.; Lin, G.; Miyata, S.; Lee, S.; Shen, C.; et al. A review of data-driven fault detection and diagnostics for building HVAC systems. Appl. Energy 2023 , 339 , 121030. [ Google Scholar ] [ CrossRef ]
- Mirnaghi, M.S.; Haghighat, F. Fault detection and diagnosis of large-scale HVAC systems in buildings using data-driven methods: A comprehensive review. Energy Build. 2020 , 229 , 110492. [ Google Scholar ] [ CrossRef ]
- Wen, J.; Shun, L. RP-1312—Tools for Evaluating Fault Detection and Diagnostic Methods for Air-Handling Units. Available online: https://www.techstreet.com/standards/rp-1312-tools-for-evaluating-fault-detection-and-diagnostic-methods-for-air-handling-units?product_id=1833299 (accessed on 28 January 2024).
- Granderson, J.; Lin, G. Inventory of Data Sets for AFDD Evaluation ; Building Technology and Urban Systems Division, Lawrence Berkeley National Laboratory: Berkeley, CA, USA, 2019. [ Google Scholar ]
- House, J.M.; Vaezi-Nejad, H.; Whitcomb, J.M. An expert rule set for fault detection in air-handling units/discussion. Ashrae Trans. 2001 , 107 , 858. [ Google Scholar ]
- Gómez, J.; Chicaiza, W.D.; Escaño, J.M.; Bordons, C. A renewable energy optimisation approach with production planning for a real industrial process: An application of genetic algorithms. Renew. Energy 2023 , 215 , 118933. [ Google Scholar ] [ CrossRef ]
- Jang, J.S. ANFIS: Adaptive-network-based fuzzy inference system. IEEE Trans. Syst. Man Cybern. 1993 , 23 , 665–685. [ Google Scholar ] [ CrossRef ]
- Lindskog, P. Fuzzy Identification from a Grey Box Modeling Point of View. In Fuzzy Model Identification: Selected Approaches ; Hellendoorn, H., Driankov, D., Eds.; Springer: Berlin/Heidelberg, Germany, 1997; pp. 3–50. [ Google Scholar ] [ CrossRef ]
- Chicaiza, W.D.; Sánchez, A.J.; Gallego, A.J.; Escaño, J.M. Neuro-fuzzy Modelling of a Linear Fresnel-type Solar Collector System as a Digital Twin. In Proceedings of the Joint Proceedings of the 19th World Congress of the International Fuzzy Systems Association (IFSA), the 12th Conference of the European Society for Fuzzy Logic and Technology (EUSFLAT), and the 11th International Summer School on Aggregation Operators (AGOP), Bratislava, Slovakia, 19–24 September 2021; Atlantis Press: Amsterdam, The Netherlands, 2021; pp. 242–249. [ Google Scholar ] [ CrossRef ]
- Chicaiza, W.D.; Machado, D.O.; Len, A.J.G.; Gonzalez, J.M.E.; Alba, C.B.; de Andrade, G.A.; Normey-Rico, J.E. Neuro-Fuzzy Digital Twin of a High Temperature Generator. IFAC-Pap. 2022 , 55 , 466–471. [ Google Scholar ] [ CrossRef ]
- Duffe, J.A.; Beckman, W.A. Design of Photovoltaic Systems , 4th ed.; John Wiley & Sons, Inc.: Hoboken, NJ, USA, 2013. [ Google Scholar ]
- Machado, D.; Chicaiza, W.; Escaño, J.; Gallego, A.; de Andrade, G.; Normey-Rico, J.; Bordons, C.; Camacho, E. Digital twin of a Fresnel solar collector for solar cooling. Appl. Energy 2023 , 339 , 120944. [ Google Scholar ] [ CrossRef ]
- Rodríguez, F.; Chicaiza, W.D.; Sánchez, A.; Escaño, J.M. Updating digital twins: Methodology for data accuracy quality control using machine learning techniques. Comput. Ind. 2023 , 151 , 103958. [ Google Scholar ] [ CrossRef ]
- Folgado, F.J.; Calderón, D.; González, I.; Calderón, A.J. Review of Industry 4.0 from the Perspective of Automation and Supervision Systems: Definitions, Architectures and Recent Trends. Electronics 2024 , 13 , 782. [ Google Scholar ] [ CrossRef ]
- Mourtzis, D.; Angelopoulos, J.; Panopoulos, N. The future of the human–machine interface (HMI) in society 5.0. Future Internet 2023 , 15 , 162. [ Google Scholar ] [ CrossRef ]
- Microsoft Hololens; Microsoft Corporation. Available online: https://www.microsoft.com/en-ie/hololens (accessed on 28 January 2024).
- SketchUp: 3D Design Software; Trimble Inc. Available online: https://www.sketchup.com/ (accessed on 15 January 2024).
- Unity Real-Time Development Platform; Unity Technologies. Available online: https://unity.com/ (accessed on 15 January 2024).
- H2020 DT-FOF-09-2020 Project Working Group. Pathways to Energy Efficient Manufacturing through Digitisation, 2023. Available online: https://zenodo.org/records/10039595 (accessed on 15 January 2024).
Click here to enlarge figure
Process | Asset | Power (kW) | No. | Metered? |
---|---|---|---|---|
Issue Material | Printer | <0.5 | N/A | No |
Barcode Scanners | <0.5 | N/A | No | |
Saw | Electric Saw | 16 | 2 | No |
Machine | CNC Mill | 29.2 | 5 | No |
CNC Lathe | 19 | 8 | No | |
Inspect | CMM | 0.7 | 9 | No |
Shadow Graph | <0.5 | 3 | No | |
Surface Tester | <0.5 | 2 | No | |
Laser | <0.3 | 1 | No | |
Clean | Multi-stage Cleanline | 90 | 2 | Yes |
Label | Printer | <0.5 | N/A | No |
Barcode Scanners | <0.5 | N/A | No | |
Package | Sealer | <0.25 | 12 | No |
Digitisation | Energy Efficiency | Cost Efficiency |
---|---|---|
85% of all poly value stream assets monitored | 95% accuracy in prediction of energy consumption | 10% savings through schedule optimisation |
25% of production assets enabled with FDD | 10% reduction in energy by idle time reduction | 15% savings through FDD on support assets |
50% of support assets enabled with FDD | Reduce complexity of energy audits | |
Reduce complexity of data collection |
Asset Type | Data Points | Data Sources | Responsible |
---|---|---|---|
Production | Energy | Data Historian (OSI Pi) | Person A |
Product Logs | Operations Management System (OMS) | Person B | |
Support | Air Handling Units | Building management System | Person C |
Chillers | |||
Compressors | Peak API * | Person D | |
Renewables | Wind | Data Historian (OSI Pi) | Person A |
Enercon API * | Person E | ||
Solar | Data Historian (OSI Pi) | Person A | |
Huawei API * | Person F |
Asset Group | No. | Idle % | Idle 5% | Idle 10 % |
---|---|---|---|---|
All assets | 14 | 3.06 | 18.32 | 26.37 |
Cleanlines | 2 | 0.61 | 0.77 | 51 |
Saws | 2 | 0 | 0 | 0.4 |
Lathes | 10 | 8.24 | 55 | 71.23 |
Mode | Rule No. | Rule Expression |
---|---|---|
1 | ||
2 | For : | |
3 | & | |
4 | ||
5 | ||
6 | ||
7 |
Error Indexes | Model | Model |
---|---|---|
7.424 [kW] | 28.42 [kW] | |
24.587 [kW] | 267.89 [kW] | |
The statements, opinions and data contained in all publications are solely those of the individual author(s) and contributor(s) and not of MDPI and/or the editor(s). MDPI and/or the editor(s) disclaim responsibility for any injury to people or property resulting from any ideas, methods, instructions or products referred to in the content. |
Share and Cite
Ranade, A.; Gómez, J.; de Juan, A.; Chicaiza, W.D.; Ahern, M.; Escaño, J.M.; Hryshchenko, A.; Casey, O.; Cloonan, A.; O’Sullivan, D.; et al. Implementing Industry 4.0: An In-Depth Case Study Integrating Digitalisation and Modelling for Decision Support System Applications. Energies 2024 , 17 , 1818. https://doi.org/10.3390/en17081818
Ranade A, Gómez J, de Juan A, Chicaiza WD, Ahern M, Escaño JM, Hryshchenko A, Casey O, Cloonan A, O’Sullivan D, et al. Implementing Industry 4.0: An In-Depth Case Study Integrating Digitalisation and Modelling for Decision Support System Applications. Energies . 2024; 17(8):1818. https://doi.org/10.3390/en17081818
Ranade, Akshay, Javier Gómez, Andrew de Juan, William D. Chicaiza, Michael Ahern, Juan M. Escaño, Andriy Hryshchenko, Olan Casey, Aidan Cloonan, Dominic O’Sullivan, and et al. 2024. "Implementing Industry 4.0: An In-Depth Case Study Integrating Digitalisation and Modelling for Decision Support System Applications" Energies 17, no. 8: 1818. https://doi.org/10.3390/en17081818
Article Metrics
Article access statistics, further information, mdpi initiatives, follow mdpi.
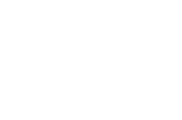
Subscribe to receive issue release notifications and newsletters from MDPI journals
Open Access is an initiative that aims to make scientific research freely available to all. To date our community has made over 100 million downloads. It’s based on principles of collaboration, unobstructed discovery, and, most importantly, scientific progression. As PhD students, we found it difficult to access the research we needed, so we decided to create a new Open Access publisher that levels the playing field for scientists across the world. How? By making research easy to access, and puts the academic needs of the researchers before the business interests of publishers.
We are a community of more than 103,000 authors and editors from 3,291 institutions spanning 160 countries, including Nobel Prize winners and some of the world’s most-cited researchers. Publishing on IntechOpen allows authors to earn citations and find new collaborators, meaning more people see your work not only from your own field of study, but from other related fields too.
Brief introduction to this section that descibes Open Access especially from an IntechOpen perspective
Want to get in touch? Contact our London head office or media team here
Our team is growing all the time, so we’re always on the lookout for smart people who want to help us reshape the world of scientific publishing.
Home > Books > Supply Chain - Recent Advances and New Perspectives in the Industry 4.0 Era
Industry 4.0 and Its Implications: Concept, Opportunities, and Future Directions
Submitted: 22 November 2021 Reviewed: 07 January 2022 Published: 28 February 2022
DOI: 10.5772/intechopen.102520
Cite this chapter
There are two ways to cite this chapter:
From the Edited Volume
Supply Chain - Recent Advances and New Perspectives in the Industry 4.0 Era
Edited by Tamás Bányai, Ágota Bányai and Ireneusz Kaczmar
To purchase hard copies of this book, please contact the representative in India: CBS Publishers & Distributors Pvt. Ltd. www.cbspd.com | [email protected]
Chapter metrics overview
2,065 Chapter Downloads
Impact of this chapter
Total Chapter Downloads on intechopen.com

Total Chapter Views on intechopen.com
This chapter aims to analyze the Industry 4.0 framework, identify the definition and drivers of the Industry 4.0 paradigm, discuss its potential effect, and determine obstacles of the Industry 4.0. For the research methodology, a critical literature review is performed, we relied on the recent studies related to industry 4.0. Findings – This study concluded that Industry 4.0 describes a future production system’s vision; it is an inevitable revolution and radical change, covering a wide range of innovative technologies, and all sectors. Industry 4.0 brings significant advantages to organizations, including real-time data analysis, increased visibility, autonomous monitoring, enhanced productivity, and competitiveness. The key features of Industry 4.0 are collaboration and integration of schemes, both horizontal and vertical. Innovation performs an essential role in organizations, sectors, countries. Industry 4.0 has enormous potential effect in many areas, and its application will have an impact across transforming the work environment. Industry 4.0 leads to potentials in three dimensions of sustainability. The KUKA corporation is an application for industry 4.0, for instance, smart factories, M-2-M, intelligent robots, etc., these technologies help industry 4.0 to separate rapidly. In contrast, there are some barriers, to implementing Industry 4.0 for example financial constraints, technical competency, organizational restraints.
- industrial revolutions
- components of industry 4.0
- impacts of industry 4.0
- industry 4.0 drivers
- barriers of industry 4.0
Author Information
Fathyelsayed youssef abdelmajied *.
- Faculty of Commerce, Benha University, Cairo, Egypt
*Address all correspondence to: [email protected]
1. Introduction
Issues related to Industry 4.0 are constantly discussed among researchers, entrepreneurs, representatives of government agencies, and public organizations. Specifically, the impacts of the Industry 4.0 paradigm in the global and national economies, individual industries, employment, and capital markets are attracting more and more attention from economists. The global industrial environment has transformed dramatically in recent years as a result of technological advances and inventions. Industry 4.0 can be compared to three industrial revolutions that happened in the previous centuries and represent the most significant disruptive shifts in manufacturing as a result of technology advancements [ 1 ].
The advent of the steam engine accelerated the First Industrial Revolution, which began in Britain in the middle of the 18th century. The Second Industrial Revolution arose in Europe and the United States in the second mid-nineteenth century. This revolution had characterized by mass manufacturing and the substitution of chemical and electrical energy for steam. Many technologies and mechanization had been developed to meet the increased demand, allowing productivity to increase [ 2 ]. The Third Industrial Revolution was sparked by the creation of the Integrated Circuit (microchip). Using electronics and information technology to accomplish increased automation in manufacturing is a significant characteristic of this revolution, which arose in many industrialized countries around the world in the later years of the twentieth century [ 1 ].
Every industrial revolution centered around boosting productivity. The first three industrial revolutions had a significant impact on industrial operations, allowing for increased productivity and efficiency by utilizing innovative technological breakthroughs, such as steam engines, electricity, and digital technology [ 3 ]. Industry 4.0, which could ultimately be referred to as the fourth industrial revolution, is a highly complex framework that has been commonly debated and discovered. It has a significant impact on the industrial sector because it introduces relevant improvements related to smart and future factories. This developing Industry 4.0 concept is an umbrella term for a new industrial paradigm that includes Cyber-Physical Systems (CPS), the Internet of Things (IoT), the Internet of Services (IoS), Robotics, Big Data, Cloud Manufacturing, and Augmented Reality, etc. [ 4 ].
The adoption of these technologies, which will bring together the digital and physical worlds through embracing a set of future industrial developments, is essential in the development of further smart industrial processes. This adoption includes devices, machines, production modules, and products that can exchange information and control each other independently, resulting in a smart manufacturing environment [ 5 ]. This new approach will allow the improvement of productivity and efficiency, carrying enormous potential effects, and it will support a set of economic and social opportunities among the companies that are adopting this new manufacturing paradigm [ 1 ].
This chapter intends to provide clear insight into the current developments within Industry 4.0 phenomenon, due to the inconsistency within the existing literature, some stress positive effects of Industry 4.0, while others, negative ones. As a result, the purpose of our research is to provide a full explanation of the Industry 4.0 paradigm, as well as to determine whether or not it is appropriate for businesses, stockholders, and countries to adopt this new approach. This chapter gives a review of Industry 4.0 and definitions in the literature, as well as introduces a brief on Industry 4.0’s main components. Additionally, this chapter’s research methodology was based on papers related to Industry 4.0, which are the most recent and cited references. As well as this study differs from past studies in several aspects, as shown in 1) It conducts a comprehensive survey of all Fourth Industrial Revolution technologies or applications, whereas earlier literature focused on one or a few technologies. 2) It performs a case study of KUKA Corporation, a pioneer company in the manufacturing technologies and applications of the Fourth Industrial Revolution.
Thus, this chapter is structured in seven sections. After this introduction about the Industry 4.0 phenomenon. Section 2 answers the question “What is the industry 4.0?”, presenting two points: an overview or background about Industry 4.0, and provides a comprehensive definition of this concept, its visions. The key Industry 4.0 technology enablers or components of Industry 4.0 characteristics are described in Section 3, which is divided into ten parts. The characteristics of Industry 4.0 state in Section 4. Section 5 provides an analysis of the impacts and influence of this new industrial paradigm: industrial sector, business models and markets, work environment, work skills, economy and sustainability, the value chains, and supply chains. While Section 6 presents the key drivers and obstacles or barriers of the Industry 4.0 concept; also, this part presents a pioneering experience in implementing the applications of the Fourth Industrial Revolution technology “KUKA corporation.” Finally, Section 7 draws the main conclusions and findings of the Industry 4.0 vision and implications.
2. What is industry 4.0?
2.1 an overview of industry 4.0.
There have been three earlier industrial revolutions that have resulted in a transformation in manufacturing patterns: mechanization via water and steam power, mass production in assembly lines, and automating through computer and information technology [ 6 ].
The first industrial revolution (Industry 1.0) was developed in the United Kingdom at the end of 18th century with the advent of water and steam power and mechanization of production. It was the most significant advancement in human productivity, which considerably aided mechanical production and greatly improved agriculture and trade. Where steam engines could be used for power. Developments such as the steamship or the steam-powered locomotive brought about further massive changes because humans and goods could move great distances in fewer hours [ 4 ]. Then, it was followed by the second one ( Industry 2.0 ) at the beginning of 19th century which introduced the electrically powered machines and assembly line production, which is described as the period when mass manufacturing became the dominant style of production in general. The introduction of railways into the industrial system was assisted by steel mass manufacturing, which in turn assisted mass production [ 7 ]. The third industrial revolution ( Industry 3.0 ) took a place in the 1970s by adopting electronics and devices within the machines, which led to developing automation and robots within the manufacturing process. Industry 3.0 developed with the introduction of the Digital Revolution, which is more well-known than Industry 1.0 and 2.0, since most people today are familiar with industries that rely on digital technology in production [ 4 ].
The Fourth Industrial Revolution is presently being implemented. This is also known as “Industry 4.0,” and it is defined by the use of information and communication technology in the industry. It is based on the Third Industrial Revolution’s advancements. Production systems using computer technology are enhanced by a network link and, in a sense, have a digital twin on the Internet. These enable communication with other systems as well as the production of data about themselves. This is the next phase in the automation of production [ 8 ].
All systems are connected, resulting in “cyber-physical production systems” and, as a result, smart factories, in which production systems, components, and people interact through a network and production is almost autonomous. When these enablers are combined, Industry 4.0 has the potential to offer some amazing improvements in manufacturing environments. Machines that can foresee faults and initiate maintenance operations on their own, for example, or self-organized logistics that adapt to unexpected changes in production are examples ( Figure 1 ) [ 9 ].

Represents a graphic illustration of the industrial revolutions overall. Source: Constructed by the author.
It also has the ability to alter people’s working habits. Individuals can be drawn into smarter networks by Industry 4.0, which might lead to more efficient working. The manufacturing environment’s digitization provides for more flexible means of providing the appropriate information to the right person at the right time. Maintenance personnel may now receive equipment documentation and service history more quickly and at the point of use, thanks to the growing usage of digital devices inside factories and out in the field. Maintenance personnel prefer to spend their time addressing issues rather than waste time looking for technical knowledge [ 10 ].
In a summary, Industry 4.0 is a game-changer in the industrial world. Manufacturing will alter as a result of digitization, including how things are manufactured and delivered, as well as how products are maintained and enhanced. As a result, it may legitimately claim to be the start of the fourth industrial revolution. Industry 4.0 is presently taking shape and its supporting technologies, such as the Internet of Things (IoT) and Cloud Manufacturing (CM), are, nevertheless, poorly defined, and under-researched.
2.2 Definition industry 4.0
Industry 4.0 is better known as the fourth industrial revolution and describes a future production system’s vision. The idea of Industry 4.0 was established by a group of professionals from several professions (such as business, politics, and academia) as part of an endeavor to integrate all manufacturing industries systems to achieve sustainability. The German government initially officially approved and implemented industry 4.0 for supporting automation in manufacturing, and for boosting German competitiveness in the manufacturing industry. Essentially, as a result of Industry 4.0, operations and manufactures will become further efficient and less expensive. These are accomplished through the simple interchange of information, integrated control of industrial goods and equipment, which work synchronously and intelligently in interoperability [ 11 ]. However, several researchers have different perceptions of the meaning of industry 4.0.
Kagermann, et al. [ 12 ] stress that industry 4 utilizes the power of communications technology and innovative inventions to boost the development of the manufacturing industry. Corresponding to Kagermann et al., the primary features of the industry 4.0 idea are characterized by three aspects: (1) horizontal integration, (2) vertical integration, and (3) end-to-end digital integration of engineering. Qin, Liu, and Grosvenor [ 13 ] emphasize that industry 4.0 encourages manufacturing efficiency by collecting data, making correct decisions. By using the most advanced technologies, the procedures will be easier. The interoperability operating ability to ensure a stable manufacturing environment. This overall consciousness gives Industry 4.0 the most important aspect of artificial intelligent functions.
The Fourth Industrial Revolution, 4IR, or Industry 4.0 conceptualizes rapid change to technology, industries, and societal patterns and processes in the 21st century due to increasing interconnectivity and smart automation [ 14 ]. Schwab pointed out that Industry 4.0 is one of the most important concepts in the development of global industry and the world economy, he accentuates that, Industry 4.0 is differentiated by a few characteristics of new technologies, the improvement in technologies is bringing significant effects on industries, economies, and governments’ development plans [ 15 ]. Industry 4.0 also denotes a social, political, and economic transformation from the digital age of the late 1990s and early 2000s to an era of embedded connection marked by widespread technological use (e.g., a metaverse). That, in comparison to humans’ inherent senses and industrial ability alone, we have constructed and are entering an augmented social reality [ 16 ].
Wang et al., [ 17 ] defined the fourth industrial revolution as the modern and more sophisticated machines and tools with advanced software and networked sensors that can be used to plan, predict, adjust, and control the societal outcome and business models. Thus, Industry 4.0 is an advantage to stay competitive in any industry. Also, Industry 4.0 can be perceived as a strategy for being competitive in the future. It is focused on the optimization of value chains due to autonomously controlled and dynamic production [ 18 ]. Furthermore, industry 4.0 is possible to indicate three future-relevant themes related to it, such as: dealing with complexity, capacity for innovation, and flexibility [ 19 ].
According to the concepts above, the majority of the researchers considered Cyber-Physical Systems (CPS), Internet of Things (IoT), Industrial Internet, and other topics to be part of Industry 4.0. Numerous authors also emphasized Industry 4.0 on the cost and profitability of recently created high-tech information and intelligent services. According to previous research on Industry 4.0, the early focus was mostly on the industrial manufacturing sector, but many industries are now adopting Industry 4.0, including automotive, engineering, chemical, and electronics. As a result, Industry 4.0 is aggregating existing ideas into a different value chain that leads to an improvement in transforming entire value chains of goods life cycles while developing innovative products in manufacturing, involving the connection of systems and things that create self-organizing and dynamic control within the organization.
Industry 4.0, often referred to as the fourth industrial revolution, is the vision or scenario of a future production process characterized by new levels of controlling, organizing, and transforming the entire value chain with the life cycle of products through three types of effective integration: horizontal, vertical, and end-to-end engineering integration, resulting in increased productivity and flexibility, the industry 4.0 leads to cost optimization and reduction [ 11 ]. The Cyber-Physical Systems (CPS), Internet of Things (IoT), artificial intelligence (AI), additive manufacturing, cloud computing, and other technologies are then combined to construct dynamic, real-time optimized, and self-organizing cross-company value networks. All of these components are necessary and integral to the futuristic Industry 4.0 concept.
3. Components of industry 4.0 and the key enabling technologies
Industry 4.0 is a complicated technical pattern characterized primarily by connection, integration, and industrial digitalization, highlighting the possibilities for integrating all components in a value-adding system. Digital manufacturing technology, network communication technology, computer technology, and automation technology are all included in this approach. Industry 4.0 technology breakthroughs are blurring the lines between the digital and physical worlds by merging human and machine agents, materials, products, production systems, and processes [ 20 ]. Industry 4.0 enables rapid technological advancements in a variety of areas; however, the emerging fourth industrial revolution is being shaped largely by the technical integration of Cyber-Physical Systems into manufacturing processes, as well as the use of the Internet of Things and Services in industrial processes [ 1 ]. As a result, this section gives a brief overview of each significant technology driver for Industry 4.0. It also is providing information on the basic components of Industry 4.0 or key technologies enablers for Industry 4.0, which consists of 10 components.
3.1 Cyber-physical system (CPS)
Cyber-Physical Systems (CPS) is the combination of computational and physical processes, which are essential components of Industry 4.0 implementations. They integrate imaging and control capabilities into the relevant systems. The ability of these systems to respond to any input generated is a key feature. They provide rapid control and verification of process feedback in order to generate predicted outputs. Bergera et al. (2016) defined cyber-physical sensor systems as part of cyberspace, special types of embedded systems, based on powerful software systems, enable integration in digital networks, and generate whole new system features [ 21 ]. Generally speaking, the evolution of a CPS is characterized by three phases. Identification technologies are included in first-generation CPS. Second-generation CPS is equipped with some sensors and actuators with a limited number of functions. In the third-generation CPS, data is kept and analyzed in addition to setting up the equipment. The CPS has many sensors and actuators and is meant to be network compatible. CPSs offer various features [ 19 ].
The CPS has several sensors and actuators and is meant to operate with a network. CPSs have features including quicker information access, preventative maintenance, pre-defined decision-making, and optimization processes. Also, CPS can boost consumers awareness and consciousness. Conversely, the CPS has certain security issues, which means that further usage will definitely result in increased dangers. It was pointed out that CPS equipment might cause disruptive societal changes since intelligent assistive or autonomous environments can cause mental illnesses, which can lead to bias toward new technology adoption and usage [ 21 ]. Cyber-Physical Systems have consisted of two key components: i) A virtual environment built through computer simulation of items and actions in the actual world, and ii) a network of objects and systems interacting with each other over the internet with a designated address [ 4 ].
3.2 Cloud systems (CS)
The term “cloud” is utilized for applications, for instance, remote services, color management, and performance benchmarking applications. It has taken remarkable attention from the IT community, and its role in other business areas will continue to grow. Machines, data management, and functionality will continue to transition away from traditional ways and toward cloud-based solutions as technology improves. The cloud enables significantly faster distribution than standalone systems, as well as quick upgrades, current performance models, and other delivery possibilities [ 19 ].
The industry has found a significant shift toward cloud solutions, which will continue to develop and represent a substantial challenge to traditional data storage methods. Cloud technology is the most basic online storage service that gives operational comfort with web-based apps that do not require any installation. Cloud computing refers to the process of storing all applications, programs, and data on a virtual server. It improves efficiency by guaranteeing those input suppliers, employees, and consumers have access to the same information at the same time [ 22 ]. Cloud Systems lower costs, simplify infrastructure, expand work areas, safeguards data, and allow for instant access to information. There are four types of the system, mainly: i) Public Cloud; ii) Private Cloud; iii) Hybrid Cloud (combination of public and private cloud); 4) Community Cloud (this refers to the co-operation of any service on the cloud with a few companies) [ 9 ].
Cloud systems are an excellent source of Big Data (which might be organized or unstructured) management solutions. Because traditional computers may not be capable of managing large amounts of data, using a cloud system to do the necessary analysis, would be much easier and more efficient. As a result, data analysis and cloud systems should be inescapable components of Industry 4.0. The integration of cloud-connected robots into everyday life, as well as their impact, is considerable [ 4 ].
3.3 Machine to machine (M2M) communication
Machine to machine (M2M), refers to the technology that allows direct communication between devices using any channel, wired or wireless. Machine-to-machine communication can include industrial instrumentation and personal communications [ 23 ]. M2M is also considered to be an essential component of Industry 4.0. Machine to machine (M2M) is a technology that allows devices to communicate directly with one another over any channel, wired or wireless. Machine-to-Machine Communication can include industrial instrumentation and personal networks. M2M is also considered to be an essential component of Industry 4.0. The apps are geared toward adding value to the enterprises by introducing alternative revenue streams and reducing operational costs [ 24 ].
Ackermann (2013) clearly states that M2M operations have to enable aspects with different networked organizations including i) Remote Service and Asset Information Management delivering, which provide information federation and lifecycle support. ii) Connected Vehicles, which creates relationships and interactions. iii) Smart Vending, which includes retail, supply chain, and associated sub-elements [ 4 ]. The M2M vision has raised a number of issues, including establishing smart settings, smart architecture, and a smart grid with wireless sensors, as well as developing a communication language between machines and humans, as well as between humans in different locations [ 23 ].
3.4 Internet of things and internet of services
The Internet of Things (IoT) is an emerging concept that combines various technologies and techniques, based on the interaction between physical things and the Internet. The advancement of technology in recent decades has enabled the Internet to be expanded into a new level known as “smart objects,” which is the foundation of an IoT vision, for this, the novel pattern consists in awarding ordinary things with intelligence, permitting them not only to accumulate information and cooperate with their surroundings, but also to be interrelated with other items, communicating information, and conducted a preliminary via the Internet. The growing interest in this field, which is widely regarded as one of the primary drivers of Industry 4.0, has produced the development of a number of visions and definitions for (IoT) [ 1 ].
The Internet of Things (IoT) refers to the interconnection of physical devices, cars, buildings, and other entities that are equipped with electronics, software, sensors, actuators, and network connections to gather and share data to create a smart manufacturing environment, also known as a smart factory [ 25 ]. Additionally, the concept of “The Internet of Services (IoS)” takes a similar approach to IoT but applies it to services rather than physical assets. The Internet of Services (IoS) idea will open up new prospects for the service sector by providing a commercial and technological foundation for the construction of business networks between service providers and clients [ 4 ].
The expansion of IoT in industrial contexts and value chains will give several opportunities for users, manufacturers, and businesses, having a significant influence in a variety of industries. The Internet of Things is breaking new ground, with a slew of new applications emerging around three key pillars: i) process optimization; ii) resource optimization, and iii) the building of sophisticated autonomous systems. IoT technology will continue to evolve and spread, allowing objects to become smarter, more dependable, and autonomous, allowing for the supply of higher-value products and services [ 1 ]. On the other hand, the effectiveness of Industry 4.0 depends upon existing network infrastructure, the intelligence, and human knowledge embedded into the system [ 22 ].
3.5 Smart factories or smart manufacturing
Smart factories or Smart manufacturing is a type of manufacturing that aims to improve concept creation, production, and product interactions by moving away from traditional methods toward automated and digitized systems. It aims to take advantage of advanced information and manufacturing technologies in order to operate and produce fully flexible production at the highest speed required [ 6 ].
“Dark factories,” “lights off factories,” and “unmanned factories” are all terms used to describe smart factories, this system is integrated with the small intervention of human beings. The individual is entering into these systems mainly in the problem-solving stages. The concept known as Lights out (dark) or unmanned factories nowadays is an automation and autonomy enhanced methodologies including equipment used in factories that actively operate the production [ 4 , 26 ]. The most famous characteristic of dark factories is that they do need no human power. In unmanned factories, there is not enough time to enter the plant from the raw material to the exit from the factory. That is to say that in these factories, production is carried out entirely with robotic systems [ 18 ]. It is self-evident that smart factories will have the characteristics and procedures required by the Fourth Industrial Revolution. And these processes, which are of great importance to our future of production. Furthermore, the essential activity for generating a smart factory running under Industry 4.0 is integrating different other components together, such as big data, CPS, cloud, IoT, M2M, etc. [ 4 ].
There are many challenges that determine the formation of smart factories, such as the availability of energy and its supply, the efficiency of the labor, and the availability of the technological infrastructure necessary to shift toward smart factories. On the other hand, these factories will have a negative impact on existing employment and increase unemployment rates [ 7 ].
3.6 Big data and data mining
Big data is being generated continuously by everything in environments. Every digital process and social media exchange produce data. Systems, sensors, and mobile devices transmit those. Big data is arriving from multiple sources at an alarming velocity, volume, and variety. To extract meaningful value from big data, there is a need for optimal processing power, analytics capabilities, in addition to information management skills [ 4 ]. An abundance of heterogeneous data abounds in the world around us. Without properly applying data mining technology, it appears impossible to make this atmosphere keenly intelligent. With today’s automation, data mining can be supervised, unsupervised, or reinforcement learning. When executed in numerous layers in a hierarchical way, computer-assisted learning becomes more exact. Machine Learning is the process of automatically extracting features through supervised or unsupervised learning in a hierarchical fashion (ML) [ 27 ].
3.7 Intelligent robotics
Every day, new goods and systems emerge as a result of technological advancements. Flying automobiles, holographic television, and hundreds of electrical devices to be implanted into the human body are all possibilities [ 26 ]. Humanoid robots will be a part of everyday life in the not-too-distant future. Recent innovations have brought about skills that empower robots to control their environment. Artificial intelligence will contribute to the development of having robot teams cooperating and collaborating in achieving certain tasks defined for a specific purpose [ 28 ].
Implementing a collaborative robot in a factory will provide several benefits for the company, including i) preventing humans from performing repetitive, non-ergonomic, and dangerous work; ii) producing high-quality products with favorable cost–benefit ratios while also increasing productivity; and iii) increasing competitiveness in comparison to countries with cheap labor [ 29 ]. When a robot is used in a productive process, the benefits of the robot utilization are combined with the effort of an operator. There is no teamwork between the man and the robot on the first level. The workplace is totally shared between the man and the robot at the final level [ 30 ].
3.8 Augmented reality and simulation
Simulation , the data obtained and processed from big data and cloud systems can be used as a feed to a virtual model to evaluate all possible scenarios related to the product design, development, and production. Simulation is used broadly in business models to leverage the available real-time data and simulate the actual working world in a virtual ecosystem. Process testing and optimization through simulation permit people to decrease business changeover, risk, setup time, and enhance quality control for future processes and services, even before the implementation of adjustments in the actual physical world [ 22 ].
Simulation and augmented reality (AR) is a type of enhanced reality in which live direct or indirect views of physical real-world environments are augmented with computer-generated visuals projected on top of them. Industry 4.0 applications rely heavily on this technology. This innovative technology, which is critical to the industrial revolution, was created by combining real operations and simulation industries [ 4 ]. These strategies have a lot of advantages, especially when it comes to creating products and manufacturing processes. One of the cutting-edge technologies included in the Industry 4.0 trend is augmented reality, which is particularly useful in producing smart manufacturing functions [ 28 ].
3.9 Enterprise resource planning (ERP) and business intelligence
Enterprise resource planning (ERP) refers to information systems that are designed to integrate and efficiently employ all of an organization’s resources. An ERP software is a system that supports an organization in bringing together processes and data that are executed all over the processes (suppliers, production, stock, sales). ERP systems are able to provide an integrated approach to information use, to start forecasting and extracting information, which can use in various departments [ 4 ]. There is a connection between big data and Industry 4.0, Manufacturing Executive Systems (MES), cloud systems, and ERP are integrated. It is critical that all procedures in the design stage as well as the customer journey are compatible with the Industry 4.0 approach. The ERP process is also a vital component in this framework [ 28 ].
The idea of Industry 4.0 necessitates connection and collaboration criteria. End-user feedback is critical, as is providing immediate additional value to all interested parties. In order for personalization to be possible, network systems must be intelligent [ 22 ]. A telecom operator may be able to analyze network performance during fluctuations and use preventive scenarios to reduce client dissatisfaction. A well-structured ERP system can enable these characteristic features. ERP systems can help with Industry 4.0 implementations, especially as a result of the following advantages: i) Real-time data may be evaluated and allow for early detection; ii) ERP systems can provide sales and purchasing transparency; iii) ERP data may be used by mobile applications to communicate; iv) Optimum resource utilization may be achieved under varying job descriptions; v) Clients may be able to track their orders online and receive the necessary information quickly [ 4 ].
3.10 Smart virtual product development system (SVPD)
The Smart Virtual Product Development (SVPD) system is a product development decision support technology that saves, uses, and shares the experiential knowledge of previous decisional events in the form of SOEs. It was created to address the requirement for digital knowledge captured in smart manufacturing product design, production planning, and inspection planning. As a result, product quality and development time will be improved, as required by Industry 4.0 concepts [ 31 ].
4. Industry 4.0 characteristics
The core progress from traditional manufacturing toward Industry 4.0 concluded into four key features and characteristics [ 32 ]: (1) vertical networking of smart manufacture schemes; (2) horizontal integration through a new generation of global value chain networks; (3) through-life engineering across the entire value chain; and (4) the impact of exponential technologies.
4.1 Vertical networking of smart production
Industry 4.0′s first main characteristic is the vertical networking of smart manufacturing systems. Vertical integration in Industry 4.0 establishes a connection between the many levels of the industry, from the manufacturing floor up, via production monitoring, control, and supervision, quality management, operations, product management, processing, and so on. This interconnectedness across all corporate levels provides for a fluid, transparent data flow, allowing for data-driven strategic and tactical choices [ 20 ]. Hence, the main objective behind vertical networking is to utilize Cyber-Physical Production Systems (CPPSs), to enable industries to quickly respond to unexpected order changes resulting from demand fluctuations, equipment failure or stock shortage. Vertical networking improves an organization’s capacity to adequately adapt to changes in market requirements and benefit from new possibilities [ 22 ].
Furthermore, it makes it easier to link resources to goods and find supplies and parts at any time. Similarly, processing data, anomalies, and defects from various processing stages of the manufacturing line are automatically captured and registered, allowing for quick responses to order changes, quality variations, and even machinery breakdowns. As a consequence, waste is decreased, and resource efficiency, notably in terms of material usage, energy consumption, and human resources is improved [ 28 ].
4.2 Horizontal integration through a new generation of global value chain networks
In the Industry 4.0 concept, horizontal integration refers to the network of diverse processes, companies, and services that make up a product’s global value chain. This can be viewed at the production level as a total consolidation of all associated manufacturing processes. Vertical integration, on the other hand, refers to a high level of coordination between production and top management layers such as quality management, product management, and production control [ 33 ].
The horizontal integration in an Industry 4.0 enterprise occurs at different levels: production floor, multiple production facilities, and entire value chain. Each connected machine or production unit becomes a node with well-defined properties within the production network. These nodes continuously communicate their status to respond autonomously to dynamic production requirements cost-effectively and reduce system downtime through predictive maintenance . If an enterprise owns several production sites, the horizontal integration enables to share inventory levels and unexpected delays, and possibly redistribute work among owned facilities to respond to market demand fluctuations rapidly or increase the efficiency and speed of the production process. However, the most critical and global horizontal integration remains the integration across the entire value chain [ 12 ].
Industry 4.0 offers a highly automated and transparent collaboration across the complete value chain, using CPPSs, from the inbound assembly, packaging, storing, production, quality control, marketing, and sales, to outbound distribution, logistics, and retail services. The horizontal integration across all these activities creates a transparent value chain that is updated in real-time. Hence, this feature provides a high level of flexibility to respond more rapidly to changing market demands, shortcomings, and problems, facilitates the optimization of the production process, increases its efficiency, and reduces the generated waste [ 17 ]. Additionally, the fact that any part or product’s history is logged and can be accessed at any time ensures constant traceability, also known as “product memory” [ 19 ].
4.3 Through-life engineering across the entire value chain
Among the characteristics of the Fourth Industrial Revolution is also the impact of the ten components of the 4th Industrial Revolution “ten types of innovation,” Efficient management of innovation, and finally, Efficient life cycle management. These are shown below.
The “ten types of innovation” (Components of Industry 4.0). Industry 4.0 will enable integrated and cross-disciplinary engineering throughout the value chain, as well as throughout product and customer life cycles. Industry 4.0 applications are intended to ensure the traditional domain of product innovation. Innovation is not limited, innovation has traditionally been related mainly to product offerings, but it also has significant potential in areas such as company structures, processes, networks, and profit models, as well as customer-facing functions [ 5 ].
Efficient management of innovation. The digital transformation to industry 4.0 will make it possible to improve further the efficiency of innovation management in all the Components of Industry 4.0. Interactive and designed curricula make individualized learning achievable, thereby, speeding up strategic implementation and organizational development [ 34 ]. Industry 4.0 solutions in project portfolio management make it easier to track not only the return on investment (ROI) in innovation, but also to identify risks by utilizing global comparative project data for monitoring and remediation. Information technology can be utilized to speed up R&D in the field of product development [ 5 ].
Efficient life cycle management. The digital transformation industry 4.0 will allow essential data for life cycle management to be provided at any time and from any location. These data will include not just information and reports, but also the outcomes of big data processing, which will be used to develop appropriate early indicators using artificial intelligence (Al). Al will employ global cross-checking to determine the plausibility of developing suitable bases for data-driven decision-making. It will allow businesses to better understand and address the needs of their customers, as well as customize product cycles [ 5 ].
4.4 The impact of exponential technologies
Exponential technologies solutions. Corporate venture capital firms have a strong chance of profiting from disruptive innovation and exponential technology by investing in new trends early on. Corporate venture capital Investing in start-ups allows businesses to participate in the development of new products and services while also ensuring their long-term competitiveness. This type of investment allows for early and convenient access to new technologies. Companies must be given more leeway to “see around the next corner.” Only then can a new business region be formed, which will eventually become the company’s new heart. Companies’ survival may be jeopardized if such possibilities are neglected [ 2 ].
The learning organization . If companies are to fully use the promise of exponential technologies in making the digital transformation to industry 4.0, they must change into learning organizations. Exponential technology adoption and integration must be slow but continuous. Learning is essential for long-term organizational development. It is time to make a change that is not so counterproductive. New ideas, processes, and business sectors are most successful when they begin as a learning niche and eventually migrate to the center of the organization, establishing themselves as a new leading segment [ 5 ].
5. Effects of industry 4.0
Innovation and scientific advancements perform an essential role in businesses, sectors, and countries. However, the digital improvements and the increasing interconnectivity will bring additional challenges and upgrades to societies, since, Industry 4.0 (Ir 4.0) will significantly change the manufacturing systems in terms of design, processes, operations, and services. Industry 4.0 will lead to potential deep changes in a variety of fields outside of the industrial sector. Its influence and effect may be divided into six categories: (1) Industry sector, (2) Products and services, (3) Business models, entrepreneurship, and market competition, (4) Economies of nations, (5) Work environment, and (6) Skills development.
5.1 The impact of industry 4.0 on the industrial sector
The industry sector will be the first to feel the effects of Industry 4.0. This new industrial paradigm will usher in a vision of manufacturing that is decentralized and digitalized, with production elements that can autonomously govern themselves, trigger operations, and adapt to changes in their surroundings. Furthermore, the developing paradigm recommends fully integrating products and processes, altering industrial vision from mass production to mass customization, resulting in increased complexity [ 35 ]. Consequently, advanced technologies and the building of smart factories will have a significant impact on production processes and operations, providing for greater operational flexibility, and more efficient utilization of resources. Industry 4.0 will have a considerable effect on the production systems, supply chains, and industrial activities. This new paradigm is changing the current industrial landscape in three ways: (1) production digitization, (2) automation, and (3) integrating the manufacturing site to a larger supply chain. Industry 4.0, in this sense, entails complete network integration and real-time data sharing [ 1 ]. Productivity growth is at the core of each industrial revolution. The 4th industrial revolution, on the other hand, will influence the entire supply chain, from product creation and manufacturing to outbound logistics, in addition to enhancing productivity [ 36 ].
ROJKO, et al. (2020) used the vector autoregression model forecast for data from the manufacturing sector in the United States over the period (2008−2018) and concluded that, the share of manufacturing output and employment has declined, and that the manufacturing sector has reached a turning point, after which robotization can increase employment and labor productivity of workers while also stimulating further growth of their education levels. They concluded that the shift to Industry 4.0 has a significant impact on the growing demand for new knowledge and skills in order to boost productivity. As a result, anticipated growths of assessed manufacturing indicators imply that the negative effects of robotization in the recent past were only transient, as the Industry 4.0 age has begun. Nonetheless, further policies are needed to enable long-term industry development [ 37 ].
5.2 The impact of industry 4.0 on products and services
This new industrial paradigm has a significant impact on products and services. Rapid changes in the economic landscape and dynamic market demands have resulted in an increased demand for the development of more complicated and intelligent products in recent years [ 36 ]. Products will become increasingly modular and configurable, allowing for mass customization to match individual consumer needs [ 35 ]. As a result, Industry 4.0 is defined by the emergence of new products and services as embedded systems that can become attentive and interactive, be managed, and tracked in real-time, optimize the entire value chain, and provide pertinent information about their status throughout their lifecycle [ 37 ].
5.3 The impact of industry 4.0 on business models and market
In the previous few years, company models and markets have swiftly altered, and new inventive business models will emerge. In the context of Industry 4.0, the introduction of new disruptive technologies has altered the way products and services are sold and delivered, disrupting established enterprises, and introducing new business prospects and models [ 33 ]. As a result, value chains are becoming more responsive, as Industry 4.0 encourages integration between manufacturers and customers, allowing for closer customer connection and business model adaption to market demands. The rising digitalization of industrial production, combined with system integration and complexity, will result in the establishment of increasingly sophisticated and digital market models, boosting competitiveness by removing barriers between information and physical structures [ 1 ].
5.4 The impact of industry 4.0 on the work environment
Because of technological advancements, the workplace environment is changing fast, and Industrial revolution 4.0 is redefining jobs and key competencies. The most significant transition is the human-machine connection, which includes employee contact and a set of new collaborative work approaches [ 18 ]. The number of robots and intelligent technologies is growing, the real and virtual environments are merging, implying the existing work environment is undergoing a considerable transition [ 13 ].
The rising importance of human-machine interfaces will encourage interaction between production elements as well as the necessary communication between smart machines, smart products, and employees, which will be aided by CPS’ vision of IoT and IoS. As a result, ergonomic concerns should be considered in the context of Industry 4.0, and future systems should emphasize the relevance of workers. Job profiles, as well as work management, organization, and planning will be affected by the integration of Industry 4.0 in industrial systems and the rising deployment of new technologies [ 12 ]. In this scenario, the major task is to avoid technological unemployment by reframing present jobs and taking steps to adapt the workforce to the new jobs that will be generated [ 28 ].
5.5 The impact of industry 4.0 on skills development
One of the most significant fundamental factors for a successful acceptance and implementation of the Industry 4.0 framework is skill development, which will lead to demographic and societal changes. New competencies will be required in the future work vision, and it will be vital to provide opportunities for the acquisition of these abilities through high-quality training. This new industrial paradigm will have a significant impact on the labor market and professional roles, and it will be critical to ensure that more jobs are generated than are lost [ 26 ].
Interdisciplinary thinking will be vital, and outstanding abilities in social and technological domains will be desired. The new required competency sectors must be included in schooling. As a result of Industry 4.0’s rising automation of jobs, workers must be prepared to take on new responsibilities [ 28 ]. The same can be said for engineering education, which has a lot of promise in terms of training future professionals and informing them about new technical trends and opportunities, as well as managers who need to adapt their management strategies to meet changing market demands. Furthermore, in order to address Industry 4.0, more qualified personnel will be required in technological sectors [ 1 ].
In summary, Industry 4.0 has enormous potential in many areas, and its implementation will have an impact across the entire value chain, improving production and engineering processes, improving product and service quality, optimizing customer-organization relationships, bringing new business opportunities and economic benefits, changing educational requirements, and transforming the current work environment.
5.6 The impact of industry 4.0 on the economy
An economy can be inspired by the introduction of new models and emerging technological improvements. Digitization involves the convergence between physical and virtual worlds and will have a widespread impact in every economic sector [ 15 ]. This will be the primary driving force behind innovation, which will be crucial to productivity and costs of production, which is reflected in the competitiveness (companies, sectors, and nations) [ 17 ].
Industry 4.0 also, can transform existing relationships in the manufacturing process, allowing the manufacturing sector to join the information age by allowing communication at all stages of the manufacturing process. Some academics anticipate that Industry 4.0 would lead to new economic forms in the industry, agriculture, and services [ 3 ]. The majority of businesses expect a two-year payback on their Industry 4.0 investments, which leads to a considerable rise in investment in this area is likely, it’s reflected in economic growth [ 37 ].
On the other hand, some experts believe that Industry 4.0 will result in increased inequality due to its threat of disrupting labor markets. It is argued that the continuous growth in automation, robots, and computers will take the jobs of workers in many industries with the most worrying factor being the increased danger of the disappearance of low-skill/low-pay jobs which will cause a lot of challenges for the poor, which will lead to a rise in social tensions [ 37 ]. The most concerning fact in Industry 4.0 is that it is not only the transfer of labor from one sector of the economy to another but also the availability of technology that will replace human capital, in other words, taking people’s jobs. The technological revolution will also have an impact on topics such as material or ideological changes brought about by the introduction of new gadgets or systems, all of which will have an impact on redefining humanity’s culture [ 3 ].
In general, digitization and interconnection of industrial processes, lead to potentials in all three dimensions of sustainability. However, achieving long-term benefits of sustainability is accompanied by several challenges respectively, especially in the implementation phase of Industry 4.0 [ 38 ].
Referring to the economic perspective of Industry 4.0 , transparency and interconnection of processes enable process optimization, resulting in increased efficiency, flexibility, quality, and customization. Industry 4.0 allows load balancing between smart manufacturing technologies, innovative value propositions, and increasing demand orientation. All these are enabling smart products, which boost a company’s competitiveness [ 39 ]. In the same regard, increasing process openness in intra- and inter-firm logistics can also be accomplished, lowering logistics costs. On the other hand, such procedures, as well as the adoption of Industry 4.0 in general, represent risks in terms of high investments and uncertain profitability [ 38 ]. Furthermore, manufacturers consider the transition to Industry 4.0 of their current business models to be difficult. Furthermore, Industry 4.0 necessitates the standardization of processes both within and between businesses. Due to their low degree of process standardization, more flexible but less automated manufacturing equipment, and resource limits, among other things, both undertakings, i.e., business model change and standardization, can become particularly problematic for SMEs [ 40 ].
Regarding the ecological dimension of sustainability , Industry 4.0 offers a number of advantages: transparency in demand and process enables for an intelligent task and process scheduling, resulting in lower energy use [ 38 ]. Furthermore, direct data linkage from product consumption back to design can improve manufacturing design, resulting in improved product lifecycle management, including recycling, as a result, Industry 4.0 aids in the identification and reduction of greenhouse gas emissions [ 40 ]. As a result, waste reduction and resource consumption can be improved. Reduced transportation operations and superfluous material flows can also be realized in logistics [ 25 ]. Furthermore, data openness across the entire supply chain can reduce the frequency of incorrect deliveries, wasteful waiting time, and damaged items. Decentralized production close to the point of consumption minimizes both logistics costs and environmental concerns [ 41 ]. Similarly, emerging manufacturing technologies such as additive manufacturing can aid in the reduction of waste in manufacturing and logistics processes, such as replacement parts [ 42 ].
Regarding the social dimension of Industry 4.0, several benefits for employees are named, such as improved human learning through intelligent assistance systems as well as human-machine interfaces that lead to increased employee satisfaction in industrial workplaces [ 8 , 22 ]. However, current literature cannot provide a unified perspective on whether Industry 4.0 will cause an increase or decrease in employee numbers in the industry. In this regard, concrete numbers named differ to a large extent [ 3 , 15 ]. In general, a further replacement of simple tasks is expected, whereas tasks such as monitoring, collaboration, and training will still be required [ 3 ]. Hereby, new job profiles with novel requirements for training and education are expected to emerge, mostly referring to decreasing importance of manual labor in contrast to IT skills. On the other hand, tasks that include planning and monitoring, as well as decision-making, could fall to autonomous systems, therefore, possibly replacing jobs in this area.
Regarding the social dimension of Industry 4.0, Several benefits for employees are mentioned, such as improved human learning through intelligent support systems and human-machine interfaces that lead to increased employee satisfaction in industrial environments [ 38 ]. However, the present research cannot agree on whether Industry 4.0 would result in an increase or decrease in the number of employees in the industry [ 25 ]. In general, easy jobs will be replaced further, while monitoring, collaboration, and training will continue to be required. It is possible that occupations in this field will be replaced [ 38 ]. As a result, implementing Industry 4.0 in an organization necessitates deliberate transformation activities, sometimes known as “digital transformation.” It necessitates new attitudes for dealing with digital transformation difficulties as well as a unified approach for staff qualification and acceptance [ 43 ].
5.7 The impact of industry 4.0 on value chains and supply chains (SC)
The fourth industrial revolution has a significant impact on supply chain interactions, which is mainly due to the exponential growth of sensible data and the widespread of digitalized processes [ 40 ]. To understand the impact of the adoption and exploitation of Industry 4.0 technologies on the value chains and supply chains (SC). Based on the review, the effect of Industry 4.0 implementation on the supply chains (SC) are identified as follows:
Agility and Customization . Industry 4.0 implementation enables real-time planning and control, permitting organizations to be flexible and agile in responding to rapidly changing conditions; for example, by faster reacting to changes in demand, supply, and prices, companies can reduce planning cycles and frozen periods [ 34 ]. Future events and trends, such as consumer behavior, delivery time, and industrial output, can be predicted using business analytics techniques. Real-time delivery routing and tracking also allow logistics operations to be more flexible, efficient, and agile [ 44 ].
Accuracy and Efficiency . Industry 4.0 technologies provide better decision-making by providing real-time, consistent, and accurate data. As a result, next-generation performance management systems will improve end-to-end visibility across the value chain. The data includes everything from key top-level performance metrics like customer service and order fulfillment to detailed process data like a truck position in the logistics network. The automation of physical tasks, planning, control, and information exchange processes improves supply chain (SC) efficiency. Automated technologies are used by a large number of businesses, particularly in their logistics operations [ 44 ]. Companies choose cross-company transportation optimization to optimize truck utilization and boost transport flexibility by cooperating and sharing facilities. The entire SC network design is constantly optimized to ensure that it is a perfect fit for business needs [ 34 ].
6. Key drivers and obstacles or barriers of industry 4.0
6.1 key drivers of industry 4.0.
Despite the rapid rise of Industry 4.0, research related to the identification of potential drivers and hurdles to its implementation are scarce. To better understand the motivations and challenges to the adoption and use of Industry 4.0 technologies, a literature review was conducted. The following are the primary drivers for Industry 4.0 implementation, as determined by the review:
Agility and Customization . Industry 4.0 implementation enables real-time planning and control, permitting organizations to be flexible and agile in responding to rapidly changing conditions; for example, by faster reacting to changes in demand, supply, and prices, companies can reduce planning cycles and frozen periods [ 34 ]. Future events and trends, such as consumer behavior, delivery time, and industrial output, can be predicted using business analytics techniques. Real-time delivery routing and tracking also allow logistics operations to be more flexible, efficient, and agile [ 44 ].
Accuracy and Efficiency . Industry 4.0 technologies provide better decision-making by providing real-time, consistent, and accurate data. As a result, next-generation performance management systems will improve end-to-end visibility across the value chain. The data includes everything from key top-level performance metrics like customer service and order fulfillment to detailed process data like a truck position in the logistics network. The automation of physical tasks, planning, control, and information exchange processes improves SC efficiency. Automated technologies are used by a large number of businesses, particularly in their logistics operations [ 44 ].
6.2 Applications of fourth industrial revolution
In this section, we introduce an overview of some applications of the Fourth Industrial Revolution. Also, we provide a case study for these applications by KUKA Group in many fields. KUKA is an international automation corporation based in Augsburg, Germany. As a world-class provider of intelligent automation solutions. In areas such as automotive, electronics, metal & plastic, consumer products, e-commerce/retail, and healthcare, KUKA provides everything from a single source: from robots and cells to completely automated systems and their networking [ 45 ].
The “ Smart Factories ” are automation solutions from KUKA, which is able to transport aircraft components around the production hangar with millimeter precision. The employees at the Airbus production plant move enormous A380 fuselage sections, weighing 90 tons and measuring 15 meters in length around a building the size of a football stadium. This is made possible by the KUKA omniMove mobile transport platform, a transport vehicle for heavy loads that is equipped with omnidirectional Mecanum wheels [ 46 ].
Similarly, using techniques such as Machine-to-Machine (M-2-M) and intelligent robots as applications from the KUKA company. Robot-based KUKA system technology for machine tool automation is used, among other things, for the loading and unloading of machines and supports elements of Industries 4.0 [ 47 ]. In the KUKA’s site in Augsburg, work 7 robots, which is a typical production environment at an international machine manufacturer [ 48 ].
Another application of industry 4.0 in the medical sector , automation solutions for greater efficiency in hospitals, in areas of diagnosis and surgery to therapy, KUKA robots meet the stringent requirements of the medical sector and are well-suited to a wide range of medical technology applications. For this, KUKA offers a wide range of medical high-tech products, ranging from robot-based help systems for surgery to assistive components for diagnosis or rehabilitation [ 45 ].
There are several applications for industry 4.0, for example, the KUKA corporation which works in the areas, for instance, smart factories, M-2-M, computing cloud, intelligent robots, e-commerce, and so on.
6.3 Key obstacles or barriers of industry 4.0
There are also some intimidating resisting forces, barriers, for implementing Industry 4.0 practices. These obstacles may be classified under the following business dimensions: Firstly, Financial constraints . Financial constraints are a fundamental issue in implementing Industry 4.0 in terms of developing sophisticated contemporary infrastructure and sustainable process improvements [ 28 ]. Secondly, the technical competency of the focal organization is the key focus that influences the scale of investment. The economic perspective, on the other hand, is still in its infancy; a lack of clarity about cost–benefit analysis and monetary rewards on digital investments is a critical issue for deploying Industry 4.0 [ 40 ].
Thirdly, Organizational nature . Other obstacles that businesses aiming to integrate Industry 4.0 technologies confront include insufficient research and development procedures, a lack of infrastructure, poor data quality, a lack of digital culture, and a lack of trust among partners [ 17 ]. Poor infrastructure and internet connectivity are significant impediments to any digital transformation or adoption [ 22 ]. As well as fourthly, Lack of management support and Resistance to change . Industry 4.0 transformative changes are fast-paced and necessitate proper skill development and training, which is difficult to do without a high degree of management support, which is the most important requirement for launching Industry 4.0. Industries are unsure and unfamiliar with the term Industry 4.0 and are ignorant of the benefits of digital transformation due to which there is reluctance in adopting it [ 22 ].
Additionally, Legal Issues . The big data transaction brings cybersecurity risk; therefore, privacy and security concerns must be considered when implementing Industry 4.0 [ 44 ]. Finally, Lack of policies and support from the government . In most nations, governments supply the infrastructure for the digital world (such as the internet and communication networks). However, there is a lack of a roadmap for transforming industrial infrastructure, owing to a lack of clarity (for example, the development of the 5G network and its benefits for Industry 4) about the implications of Industry 4.0 [ 22 ].
Continue reading from the same book
Supply chain.
Edited by Tamás Bányai
Published: 27 July 2022
By Thuso Mphela, Christopher J. Savage and Alejandro ...
398 downloads
By Andre T. Mayounga
302 downloads
By Erica Varese, Maria Chiara Cesarani and Magdalena ...
239 downloads
IntechOpen Author/Editor? To get your discount, log in .
Discounts available on purchase of multiple copies. View rates
Local taxes (VAT) are calculated in later steps, if applicable.
Support: [email protected]
Advertisement
Human-centred design in industry 4.0: case study review and opportunities for future research
- Open access
- Published: 11 June 2021
- Volume 33 , pages 35–76, ( 2022 )
Cite this article
You have full access to this open access article
- Hien Nguyen Ngoc ORCID: orcid.org/0000-0003-4618-9462 1 ,
- Ganix Lasa ORCID: orcid.org/0000-0002-2424-5526 1 &
- Ion Iriarte ORCID: orcid.org/0000-0002-6772-1166 1
19k Accesses
61 Citations
6 Altmetric
Explore all metrics
The transition to industry 4.0 has impacted factories, but it also affects the entire value chain. In this sense, human-centred factors play a core role in transitioning to sustainable manufacturing processes and consumption. The awareness of human roles in Industry 4.0 is increasing, as evidenced by active work in developing methods, exploring influencing factors, and proving the effectiveness of design oriented to humans. However, numerous studies have been brought into existence but then disconnected from other studies. As a consequence, these studies in industry and research alike are not regularly adopted, and the network of studies is seemingly broad and expands without forming a coherent structure. This study is a unique attempt to bridge the gap through the literature characteristics and lessons learnt derived from a collection of case studies regarding human-centred design (HCD) in the context of Industry 4.0. This objective is achieved by a well-rounded systematic literature review whose special unit of analysis is given to the case studies, delivering contributions in three ways: (1) providing an insight into how the literature has evolved through the cross-disciplinary lens; (2) identifying what research themes associated with design methods are emerging in the field; (3) and setting the research agenda in the context of HCD in Industry 4.0, taking into account the lessons learnt, as uncovered by the in-depth review of case studies.
Similar content being viewed by others
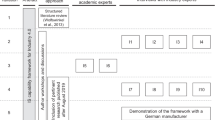
Disentangling Capabilities for Industry 4.0 - an Information Systems Capability Perspective
Principles for Human-Centered System Design in Industry 4.0 – A Systematic Literature Review
Exploring Sustainable Value Creation of Industry 4.0 Technologies Within the Socio-technical Perspective: A Meta-review
Explore related subjects.
- Artificial Intelligence
Avoid common mistakes on your manuscript.
Introduction
A challenge of manufacturing today is adapting to an increasingly fluctuating environment and diverse changes to meet the demands of the market. Product life cycles are getting shorter while production batch sizes are getting smaller with dynamic product variants associated with increasing complexity, which is challenging the traditional production systems (Benabdellah et al., 2019 ; Kuhnle et al., 2021 ; Ma et al., 2017 ; Prinz et al., 2019 ; Windt et al., 2008 ; Zhu et al., 2015 ). To manage these dynamics, the industrial concept of Industry 4.0 has come about and has been accepted in both research and industry, a trend linked to digitalization and smart systems that could enable factories to achieve higher production variety with reduced downtimes while improving yield, quality, safety, and decreasing cost and energy consumption (García-Magro & Soriano-Pinar, 2019 ; Järvenpää et al., 2019 ; Napoleone et al., 2020 ; Oztemel & Gursev, 2020 ; Park & Tran, 2014 ). Although the adoption of Industry 4.0 in manufacturing reveals positive outcomes, the increased complexity as a collateral effect has also brought many challenges (Bednar & Welch, 2020 ; Cohen et al., 2019 ; Fernandez-Carames & Fraga-Lamas, 2018 ; Mourtzis et al., 2018 ; Wittenberg, 2015 ). One of the challenges is to put humans properly at the centre of smart manufacturing design (Grandi et al., 2020 ; Pacaux-Lemoine et al., 2017 ; Paelke et al., 2015 ; Peruzzini et al., 2019 ; Varshney & Alemzadeh, 2017 ). An approach to address this challenge is known as HCD. According to International Organization for Standardization ( 2019 ), HCD is a multidisciplinary approach incorporating human factors and ergonomics knowledge and techniques to make systems usable. However, the design complexity in smart systems can occur in both directions, where in one direction the human must be able to effectively cooperate with other existing physical system components and simultaneously exchange data with system informatics for hybrid decision making (Fernandez-Carames & Fraga-Lamas, 2018 ; Schulze et al., 2005 ; Zheng et al., 2018 ). The reverse direction is that the design of such smart systems must be capable of sensing and responding to the trust levels of humans they interact with in order to result in more productive relationships between the human and other smart components (Chang et al., 2017 ; Rogers et al., 2019 ; Seitz et al., 2021 ; Song et al., 2016 ; Van Acker et al., 2020 ).
Numerous contributions have been written on Industry 4.0 areas; however, the majority of them focus on the technical aspects in which human factors are commonly underestimated (Bhamare et al., 2020 ; Grandi et al., 2020 ; Pacaux-Lemoine et al., 2017 ; Peruzzini et al., 2019 ; Theuer et al., 2013 ). There is an increasing concern about how human factors are barely considered in design for products and/or services and poorly addressed in manufacturing, causing complex problems with often unknown consequences across different industrial contexts: nuclear accidents (Wu et al., 2016 ), market failures in new product development (García-Magro & Soriano-Pinar, 2019 ), robotic-surgery-related adversities (Varshney & Alemzadeh, 2017 ), technological accidents during machine manipulation (Pacaux-Lemoine et al., 2017 ), and interaction issues among humans and smart systems (Jung et al., 2017 ; Rogers et al., 2019 ; Streitz, 2019 ).
The phenomenon of Industry 4.0 reflects contemporary design contexts that frequently contain complex interdependencies of human and non-human actors—internet of thing (IoT) devices, digital and physical environments—shaping the framework of human roles and socio-technical systems (Cimini et al., 2020 ; Coulton & Lindley, 2019 ; Jwo et al., 2021 ; Kong et al., 2019 ; Kymäläinen et al., 2017 ). However, this does not mean that the existing concepts of design—for example, design for manufacturing and assembly (Favi et al., 2021 ), or a traditional design process that considers existing solutions to fulfil the needs of the largest group (Lorentzen & Hedvall, 2018 )—are redundant. They have evolved and enlarged the scope of design: manufacturability fosters the collaboration of design and manufacturing operations, taking the perspectives of efficiency, effectiveness and economics into account (Chen et al., 1995 ; Venkatachalam et al., 1993 ); social sustainability addresses design for quality of human life by considering transdisciplinary relationships with human diversity (Demirel & Duffy, 2013 ; Martin et al., 2013 ; Papetti et al., 2020 ). These new requirements have impacted the factories themselves, but they affect the entire value chain, from the product design and development process through market segmentation to manufacturing and product disposal management (Bauer et al., 2019 ; Kong et al., 2019 ; Pereira Pessôa & Jauregui Becker, 2020 ). In this sense, for transitioning to sustainable manufacturing processes and consumption, human-centred factors play a core role in the achievement of sustainability-oriented operations throughout the supply chain (Bednar & Welch, 2020 ; Ceccacci et al., 2019 ; Grandi et al., 2020 ; Gualtieri et al., 2020 ; Lin, 2018 ; Rossi & Di Nicolantonio, 2020 ).
To address human-related roles in the context of Industry 4.0, there is a constantly growing interest in research and industrial practices where humans are placed at the centre of design across disciplines. This is manifest in the substantial body of literature providing signposts of theoretical frameworks and models, implementation methodologies, and case studies in cross-disciplinary contexts. The scope of the research is extensive: customer-centric business models associated with customer involvement in design (Adrodegari & Saccani, 2020 ; Grieger & Ludwig, 2019 ; Saha et al., 2020 ; Santos et al., 2018 ); smart design engineering in which the users and emotional interactions are empowered (Benabdellah et al., 2019 ; Pereira Pessôa & Jauregui Becker, 2020 ); technology design in which users are centred (Chen & Duh, 2019 ; Rogers et al., 2019 ); interaction designs among operators and smart manufacturing components (Klumpp et al., 2019 ; Rossi & Di Nicolantonio, 2020 ); human-centred designs for product development (Chen et al., 2016 ; Wu et al., 2013 ); data processing by which humans remain the first design consideration of a data-driven approach (Crabtree & Mortier, 2015 ; Victorelli et al., 2020b ); sustainability in social-technical manufacturing contexts, including social robotic interactions with humans (Bednar & Welch, 2020 ; Leng & Jiang, 2017 ; Richert et al., 2018 ; Streitz, 2019 ).
Even though a wide array of studies has been created and published, these studies have become disconnected from other studies after publication. As a consequence, these studies in industry and research alike are not regularly adopted, while the network of studies is scattered and diffused without forming any comprehensive structure. Although numerous review papers portrayed the key developments regarding HCD over recent years, they focused on the reflection of emerging trends based on bibliometric results, debates, and priorities in their own research scope with their defined disciplines. Recently, Zarte et al. ( 2020 ) conducted SLR to structure design principles for HCD while Victorelli et al. ( 2020a ) provided an understanding of human-data integration with bibliometric analysis. Other representative review studies include Benabdellah et al. ( 2019 ), Duque et al. ( 2019 ), Kadir et al. ( 2019 ), Bazzano et al. ( 2017 ). However, the current work does not pay attention to publications whose case studies contain a tremendous source of useful information. The results of a case study can have a very high impact on exploring in-depth conceptual testing and refinement associated with lessons learnt (Kadir et al., 2019 ; Tetnowski, 2015 ; Williams, 2011 ; Yin, 2018 ), something that deserves to be treated as a special unit of analysis in the review process. Moreover, the review papers also pointed out their own methodological limitations, leading to the call for future research priorities in identifying and deepening the research outcomes of HCD through the cross-disciplinary lens.
To take the perspective of HCD under the transition to Industry 4.0 and simultaneously respond to said call, we contribute to the research through a rigorous review of case studies—to capture the lessons learnt—that have been conducted so far in the literature. The objective is to pave the way for the ongoing developments around the concept and also explain its journey in a systematic and well-rounded methodology. To achieve this objective, we review the existing scientific body of knowledge by:
providing insight into how the literature has evolved through the cross-disciplinary lens
identifying what research themes associated with design methods are emerging in the field
setting the research agenda in the context of HCD in Industry 4.0, taking into account the lessons learnt, as uncovered by the in-depth review of case studies
To achieve the above and contribute to the body of knowledge regarding the HCD domain, this article begins with HCD’s fundamental concepts, which indicate for researchers diverse perspectives on HCD across the value chain in the context of Industry 4.0. The next section presents a strict protocol of SLR that ensures a sufficient amount of quality publications for the analysis. " Literature characterization of human-centred design in industry 4.0 " section digs into the literature to unfold the characteristics of HCD. Subsequently, the in-depth review expresses important facts of HCD in the context of Industry 4.0: emerging research schemes among concepts of HCD, diverse design methods and lessons learnt. This article concludes with a comparative discussion of the papers and suggests opportunities for further research.
Human-centred design in industry 4.0
Nowadays, the fourth industrial revolution develops highly connected resources, integrates smart components and enables interoperability in cyber-physical systems (CPSs) in the twenty-first century (Campbell 2021 ; Cruz Salazar et al., 2019 ; Derigent et al., 2020 ; Duque et al., 2019 ; Pereira Pessôa & Jauregui Becker, 2020 ). The changes that trigger Industry 4.0 have impacted different domains throughout the value chain. First, an autonomous system—embedding smart components in CPSs equipped with autonomous capability—achieves a specified goal independently without any human intervention (Gamer et al., 2020 ; Park & Tran, 2014 ). However, human intelligence and intervention remain a key role because of the safety, security, social aspects and uncertainties posed by such autonomous systems (Fosch-Villaronga et al., 2020 ; Gil et al., 2019 ; Nahavandi, 2017 ; Santoni de Sio & van den Hoven 2018 ; Weichhart et al., 2019 ). Along with advanced technologies in such smart systems, the role of humans has changed and shifted from low-level operations—which can be dangerous, dirty, difficult, and dull tasks—to high expertise and safe tasks (Bauer et al., 2019 ; Campbell 2021 ; Nahavandi, 2017 ; Zhang et al., 2017 ). This phenomenon highlights two different concepts of HCD: human-in-the-loop and human-on-the-loop systems (HioTL). The human-in-the-loop system is a system in which a machine executes a task for a specific command and then stops for the human order before continuation. On the other hand, the human-on-the-loop system is an autonomous system that executes a task independently and completely, while the role of humans can provide expertise not available to the system and can respond to issues that the system is unaware of (Kong et al., 2019 ; Nahavandi, 2017 ; Richter et al., 2018 ; Streitz, 2019 ; Vanderhaegen, 2019 ). An autonomous system should not imply the exclusion of the human, but it should allow for a seamless integration of humans in both operational levels of the process monitoring and strategic levels of orchestration in the aggregate plan. This approach enables high levels of human collaboration to achieve the common key performance indicators of manufacturing while meeting internal constraints (Gervasi et al., 2020 ; Pacaux-Lemoine et al., 2017 ).
In addition, the smart robots work safely with humans in collaborative production systems to autonomously and seamlessly perform collaborative tasks working towards common goals (Boschetti et al., 2021 ; Cohen et al., 2019 ; Gervasi et al., 2020 ; Wojtynek et al., 2019 ). These collaborative robots, often called cobots , relieve the factory workers from the low-level tasks to work side-by-side with humans in order to increase the workstation performance: production pace, efficiency, and higher throughput. In this context, design for the collaboration is well known as human–robot collaboration (HRC), which is also interchangeably called human–robot interaction (Cohen et al., 2019 ; Gervasi et al., 2020 ). Beyond the physical interactions, the collaboration design also enables the robots and humans to share knowledge and learn from others, and so work towards social sustainability, i.e., discussions and accommodation with others’ perspectives (Fosch-Villaronga et al., 2020 ; Gualtieri et al., 2020 ; Richert et al., 2018 ; Weichhart et al., 2019 ).
In addition to smart systems and cobots, the industry and research alike pose new requirements and means of interactive interfaces among human and non-human actors (e.g., machines, smart devices) to deal with the new challenges: interdependent interactions with complex information, and natural and intuitive communication (Diegel et al., 2004 ; Haslgrubler et al., 2018 ; Ong et al., 2020 ; Weichhart et al., 2019 ). In the earlier development, the information systems interfaces are usually designed by the technology-oriented approach that adapts humans to the equipment. This lack of consideration of the human results in lower-than-expected manufacturing system performance and an increasing possibility of error rates (Chen & Duh, 2019 ; Oborski, 2004 ; Wu et al., 2016 ). Therefore, putting humans at the centre of interface design is the concept of the human–machine interface (HMI), which allows humans to understand and operate a machine in a digital manufacturing context. Design for HMI requires a transdisciplinary approach that takes various disciplines into account: cognitive psychology, industrial design, information processing graphics, human factors, and ergonomics (Oborski, 2004 ; Ong et al., 2020 ; Wu et al., 2016 ).
Beyond industrial applications, the user-friendly design of HMI is important in various domains—desktop, web engineering, and services—with which its application boundary is very blurred (Chang & Lee, 2013 ; Chang et al., 2017 ; Hoffmann et al., 2019 ). Basically, one of the key measurements to understand the degree to which the design of HMI meets usage requirements is its usability, which focuses on functional indicators: usefulness, efficiency, effectiveness, and the learning curve of the user interface. The deeper concept of user multidimensional experience—which considers users’ emotional and psychological responses—is getting increasing attention and is also known as the core concept of user-centred design (UCD) (Chen, 2016 ; Kymäläinen et al., 2017 ; Lin, 2018 ; Paelke et al., 2015 ; Zheng et al., 2018 ). UCD, also interchangeably called user-centrality , embraces the user’s needs and involvement as the centre of the co-designing development process (Mazali, 2018 ; Wu et al., 2016 ) in order to enhance user acceptability and acceptance. While the former is a prior mental representation that users have before interacting with a product and/or service, the latter is an evaluation after a real interaction with the design has taken place (Van Acker et al., 2020 ).
From the perspective of life-cycle design, the increasing variability of products and varying expectations of customers have impacted development and manufacturing at different stages, requiring new solutions that enhance the value of the customer’s interaction with the product along its life cycle (Benabdellah et al., 2019 ; Chaudhuri et al., 2019 ; Fernandez-Carames & Fraga-Lamas, 2018 ; Pezzotta et al., 2018 ; Zhu et al., 2015 ). In this evolving scenario, manufacturers navigate from product-oriented development to the servitization phenomenon in which the concept of product-service systems (PSS) is a result of product and service integration. PSS is capable of fulfilling the customer’s present requirements while being adaptable to future needs and necessities through all their life-cycle stages (Cheah et al., 2019 ; Haber & Fargnoli, 2019 ; Leoni, 2019 ; Mourtzis et al., 2018 ; Pezzotta et al., 2018 ; Zhu et al., 2015 ). PSS requires a human-centred design thinking process that not only generates the value-in-use to the customer through the identification of the latent requirements, but also manages the stakeholders and the technical feasibility (Cheah et al., 2019 ; Santos et al., 2018 ). The approach of HCD, such as service design, plays an important role in the design of service-oriented value propositions by providing a set of methods to improve customer experience and understand emerging social trends (Iriarte et al., 2018 ).
The value chain itself is being reconfigured because the type of value exchange is shifted from selling products to providing services in order to optimize competitiveness through market segmentation strategies towards customer personalization. Smart PSS allows for a completely new relationship between manufacturers and customers and thus enables new business models towards customer-centricity that facilitate customer-focused and co-creation relationships towards sustainability for business, customers, and stakeholders (Anke, 2019 ; Bednar & Welch, 2020 ; Benabdellah et al., 2019 ; Grieger & Ludwig, 2019 ; Ma et al., 2017 ; Saha et al., 2020 ). This phenomenon is enabled by the ubiquity of digital technologies that allows for a fundamental shift in the business landscape in which the individual customer is at the centre of design activities, at the point of origin, and an active participant across different business processes: innovation, development, management, and production to deliver “smartness” values (Brenner et al., 2014 ; Mazali, 2018 ; Zheng et al., 2018 ).
Smartness is a socio-technical phenomenon—in which the production processes and the products themselves are technical aspects—that impacts society’s awareness of sustainability in terms of the environmental, social, and economic aspects (Bednar & Welch, 2020 ; Fu et al., 2019 ; Gualtieri et al., 2020 ; Pereira Pessôa & Jauregui Becker, 2020 ). There will be a need for a strategic balance between shorter- and longer-term desires, values, and policies, and the interests of different groups of stakeholders. Technology alone cannot give an organization a competitive edge or provide an industry step change, but an organization must be sustainable and have an architecture based on financial, ecological, and socio-technical systems. This context reconfigures the interrelationship among human and non-human actors: people and organizations, technologies and manufacturing systems, and production and consumption. Smartness expresses a new relationship between society and technology in the name of Industry 4.0 (Bauer et al., 2019 ; Bednar & Welch, 2020 ; Mazali, 2018 ; Rogers et al., 2019 ; Rossi & Di Nicolantonio, 2020 ; Yao et al., 2019 ).
The advent of Industry 4.0 has made many changes, and the concepts of design oriented to humans are not exceptional. Some concepts are defined in different contexts, and the boundaries of their application overlap and are often used interchangeably. The similarity among these concepts is a multi-objective approach that aims at designing products and/or services towards human well-being while ensuring sustainable development. In a broader sense, this multi-objective approach addresses not only human factors and ergonomics towards human diversity, but also design for manufacturability: the design process must be efficient; the manufacturing processes must be capable, proactive, and economic (Anderson, 2014 ; Favi et al., 2021 ; Sinclair, 1992 ). This perspective must also take the approach of life-cycle management that aims at managing the activities of products and/or services across the life cycle towards sustainability, such as life-cycle cost analysis for economics (Aurich et al., 2007 ; Jasiulewicz-Kaczmarek et al., 2021 ; Kambanou, 2020 ). This multi-objective approach in HCD is not only consistent with the definition of HCD reported by International Organization for Standardization ( 2019 ) (Fernandez-Carames & Fraga-Lamas, 2018 ; Rossi & Di Nicolantonio, 2020 ) but also provides a broader perspective throughout the value chain in the context of Industry 4.0.
Due to the broader perspective and diverse contexts in which the concepts regarding HCD have emerged and spread across disciplines, it would be difficult for scholars to set a proper research direction. This difficulty motivates us to review and structure lessons learnt in literature via the cross-disciplinary lens to identify coherent research directions for subsequent researchers and industrial practitioners alike. To realize our objective, the following section presents the protocol of SLR that allows the body of knowledge to be gathered in a systematic but objective way.
Research methodology
Figure 1 shows a process flow of SLR whose objective is to sufficiently cover the research topic and provide evidence with minimization of subjectivity and bias (Boell & Cecez-Kecmanovic, 2015 ; Tranfield et al., 2003 ).
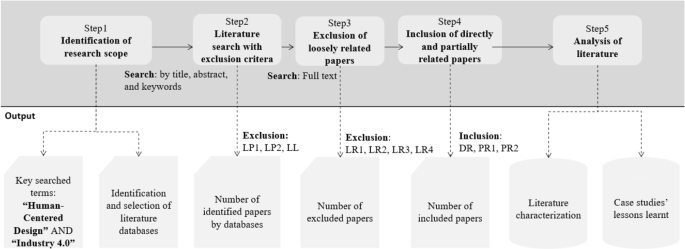
A process flow of systematic literature review
First, there are two fundamental keywords, including “human-centered design” and “industry 4.0”. However, scholars use disparate terms to describe the concepts, and the boundaries of these concepts remain blurred, as analysed in " Human-centred design in industry 4.0 " section. Therefore, a wide range of keywords were identified and combined to discover comprehensively and objectively across a broad range of well-known databases whose description is provided by “ Appendix ” (Table 9 ): Web of Science, Scopus, Science Direct, Emerald, SpringerLink, Engineering Village, SEGA Journals, and EBSCO. Covering a wide range of substantial databases is one of the decisive efforts for overcoming the limitations of a single database, as reported by Saha et al. ( 2020 ). One problem with this breadth of databases is the noticeable difference among their search functionality that requires adjustment according to each database, as detailed by “ Appendix ” (Table 10 ).
As a result, there are 265 identified papers, and nearly 162 of them are found by the database of SpringerLink and Emerald, whose disciplines focus on varying fields—science, technology, engineering, and management—that show the transdisciplinary applications of HCD. Table 1 also shows that the number of papers found across databases decreases while that of duplicate papers among them increases proportionally, which shows that papers relevant to this research have been sufficiently covered and reached a state of saturation.
The next step continues with the review protocol to distinguish two groups of inclusion and three groups of exclusion criteria associated with their corresponding description, described in Table 2 . In addition to the exclusion of duplicate papers (LP2), we also ensure the credibility of published papers by excluding papers that have not undergone a review process and have been published in journals (LP1).
Given our competence in the language, the papers written in non-English language (LL) are not considered for this study. To keep our research focus, we also excluded all irrelevant papers that mention HCD and Industry 4.0 as examples (LR1) instead of their main research subject; mention the research agenda (LR2) instead of research focus; or cite expressions (LR3), keywords and/or references (LR4). For instance, we found the paper published by Ribeiro and Bjorkman ( 2018 ), “Transitioning From Standard Automation Solutions to Cyber-Physical Production Systems: An Assessment of Critical Conceptual and Technical Challenges”, as the search result on the database of Web of Science. However, the paper focuses on the aspects of CPSs instead of HCD, which only appeared as a reference paper. At the end of step 3, we excluded all irrelevant papers across the databases for the following step.
The included papers are analysed in detail and ranked in order according to what extent they are relevant to HCD and Industry 4.0, with a focus on the manufacturing areas. We classified them into three groups of inclusion: (DR) 24 directly related papers dedicated to HCD in the context of manufacturing; (PR1) six partially related papers studying HCD but in different contexts; (PR2) 47 partially related papers providing useful information related to HCD: design concepts, design methods, supporting technologies, human diversity, ergonomics, economics, manufacturability, and sustainability. Based on our presented objectives, the following section starts by presenting the overall characteristics of the literature, followed by an in-depth review of case studies—emerging trends, design methods, lessons learnt—and opportunities for future research.
Literature characterization of human-centred design in industry 4.0
This section provides an overall quantitative picture of the included papers: the trend of research interest associated with the most cited papers, the regions and countries where the papers are made, and, importantly, the transdisciplinary and multidimensional approach in HCD. Subsequently, the in-depth review of case studies presents the emerging trends among the concepts of HCD and design methods, followed by an affinity analysis that categorizes their research outcomes and limitations.
Overall characteristics
Growth rate of research interest.
After excluding the duplicate papers, there are 215 remaining papers whose yearly publication data allow for the extrapolation of two interesting stages from 1997 to the middle of 2020, as portrayed by Fig. 2 . First of all, one notices that the topic has gained momentum and research interest in different aspects of HCD. Secondly, for the period 2015–2019, there has been an almost consistent and healthy growth in the number of publications. Obviously, the 2020 data is still incomplete, which shows a lower number of publications than that of the previous years, because this research was carried out in the middle of the current year. Besides, we applied the Hot’s trend prediction method to exponentially conjecture that the research publications could reach 108 papers by the end of 2020. However, the growth rate could be affected due to the global issue of Covid-19.
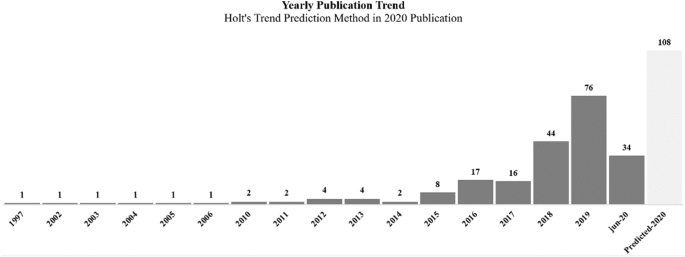
Yearly publication trend with the exclusion of duplicate papers
By examining only 77 included papers, Table 3 presents the most cited papers, accounting for 63% (329 out of 501 total citations). Interestingly, these top-cited papers have almost been published in recent years. This fact shows that the development of HCD has not matured yet, while the scholars have made the references to the recently published papers for new findings instead of citing the previous ones that have not been well generalized in the research community.
The top cited paper of Zheng et al. ( 2018 ) outlines future perspectives of smart manufacturing systems in which user experience is considered as one of development challenges, and transdisciplinary research is called for future research. Beyond the technical perspectives, the scholars also drew attention to social aspects. Specifically, the work of Mazali ( 2018 ) explicitly concluded that one of the key issues for the future is to design a balance between the worker being able to control the process by using their own intelligence and the automation of digital algorithms. This perspective is also agreed upon by the work of Streitz ( 2019 ), who graded the equal importance among humans and technologies in ambient intelligence to achieve the smart paradigm.
Publication origin
By taking a detailed look at 77 included papers, Fig. 3 shows that the most influential countries are accounted for by Germany (18%), followed by Italy (14%), and China (12%). In the regions, European countries have shown strong contributions in the field with 65% publications, which was reflected by several pieces of research— Factories of Future (European Commission, 2013 ) and Platforms for CPSs (Thompson et al., 2018 )—whose recommendation for future research indicates that it has been a long road reaching the systems of HioTL at the matured level together with other emerging technologies. Some specific research programs and priorities in the next three decades are extracted as below:
Human-oriented interfaces for workers: process-oriented simulation and visualization.
Products and work for different types of skilled and aged labour, education and training with IT support.
Regional balance: work conditions in line with the way of life, flexible time-and-wage systems.
Knowledge development, management and capitalisation.
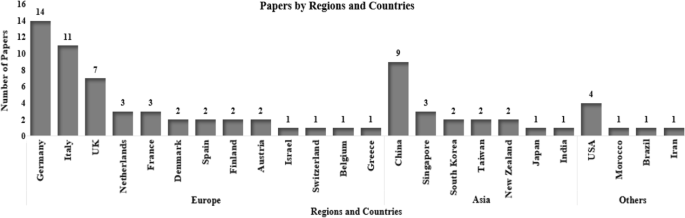
Papers by regions and countries
Transdisciplinary approach
By examining the journals by which the included papers were published, the transdisciplinary approach of HCD is strongly evidenced by the fact that there are no journals significantly overwhelming other journals. Table 4 reveals two interesting facts. First, the top 11 journals out of 54 journals—which publish 77 included papers—range from varying research disciplines: engineering; computer science; business management; social and philosophy, which is specialized by the journals Cognition, Technology & Work and AI & SOCIETY . This transdisciplinarity integrates cross-disciplinary perspectives—philosophy, engineering, computer, business, and social sciences—in the context of HCD and transcends their traditional boundaries. This fact addresses the interest in extending the research boundaries of various dimensions of HCD: human diversity, physical to cognitive ergonomics, economics, manufacturability, and social and human-related sustainability.
This transdisciplinary approach has also brought different studies across various research contexts, as can be seen in Fig. 4 . There are 42 papers out of 77 included papers that clearly indicate their research focuses on specific manufacturing processes and industries: machinery and equipment as the top one, followed by automotive industry and machining process . The adaption of HCD has progressed in more specific fields: adhesive solutions was considered as the case study on which Lee and Abuali ( 2011 ) tested their methodology of innovative and advanced PSS; smart labelling design was developed from the foundation of Industry 4.0 human-centred smart label applications proposed by Fernandez-Carames and Fraga-Lamas ( 2018 ); design for textiles was implanted with interactive technologies to experiment and enhance fashion emotional design by Wang et al. ( 2018 ).
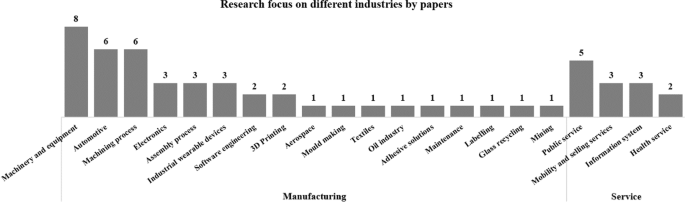
Research focus on different industries by papers
On the other hand, there are 13 papers out of 77 included papers that explicitly adapt HCD in services, for example public service for smart housing services—which seamlessly connect humans and machines—by design for HMI with the application of Bluetooth ubiquitous networks (Diegel et al., 2004 ) or a 3D-based meta-user interface (Mostafazadeh Davani et al., 2018 ). For the healthcare sector , Haber and Fargnoli ( 2019 ) emphasized the understanding of human needs and proposed the approach of PSS—the integration of products (hemodialysis devices) and services (e.g., technical support, response time)—for the offering’s value. In the same sector, Gervasi et al. ( 2020 ) proposed an evaluation framework—which expresses the perspectives of engineering, cognitive, and social science—of HRC to assess the support of robots for elderly people to reach a specific place.
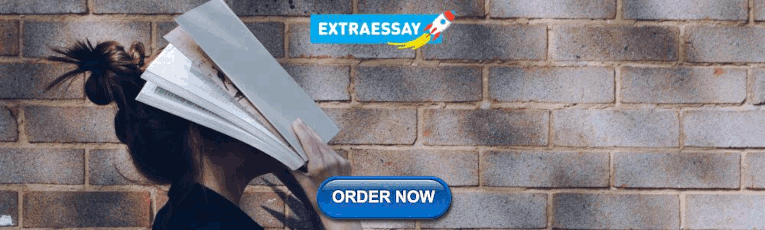
Multidimensional approach
The research methodology is also diverse in both conceptual and empirical research, as evidenced by Table 5 . Fifty-six out of 77 included papers (around 73%) take an empirical approach, while the remaining 21 papers (around 27%) contribute to the conceptual findings. Empirical research uses scientific data or case studies for explorative, descriptive, explanatory, or measurable findings, while conceptual research focuses on abstract ideas, concepts, and theories built on literature reviews (Marczyk et al., 2005 ; Williams, 2011 ). Those conceptual papers are further categorized into SLR, accounting for four papers (around 5%) that differentiate from traditional narrative review papers (around 22%). The strong point of SLR is a replicable, scientific, and transparent process minimizing bias through exhaustive literature searches of studies and simultaneously providing the traceability of results (Boell & Cecez-Kecmanovic, 2015 ; Tranfield et al., 2003 ). Of the 56 empirical articles, 37 papers (around 66%) are qualitative studies and 19 articles (around 34%) are quantitative studies. Those figures explain the current research effort that focuses on describing, explaining, and interpreting HCD is overtaking the research effort on quantification and statistical treatment for supporting or refuting research findings. This fact is reflected by the nature of the social phenomenon being investigated from the human point of view, leading to the difficulty in the generalization of results (Mennell, 1990 ; Walsh et al., 2015 ).
Table 5 also reveals the multidimensional approach of levels of research analysis that range from the level of the product to the levels of the workstation, the company and, finally, society. The research on the level of society and the workstation is still modest in comparison with that of the company or the product, accounting for 12 papers out of 77 included papers (around 16%). The figures show there is reasonable space for further research that deals with HCD at cross-layer levels other than the company and product level, which is also consistent with the future research agenda proposed by the European Commission ( 2013 ).
In a broader sense, by applying the qualitative research methodology, Fosch-Villaronga et al. ( 2020 ) took a step beyond the company level to gather expert opinions addressing social challenges—ethical and legal issues, job availability—due to the use of social robots. They investigated the challenges from both user perspectives—privacy, autonomy, the dehumanization of interactions—and worker perspectives, such as the possible replacement of jobs by robots. Based on the companies’ perspectives with regard to addressing this level of social concerns with the qualitative approach, Mazali ( 2018 ) conducted 40 in-depth interviews with managers of 20 manufacturing companies to accommodate the social needs and organizational contexts that involve multiple stakeholders and new roles of intelligent systems in workflows. In the lower area, the company level is addressed by the business cases and processes. For instance, the work of Hammer et al. ( 2018 ) shows an extension of existing business models for quality of experience that incorporate user needs and motivation as aspects of the individual dimension. Subsequently, the workstation level concerns the design for human-oriented workstations, for instance, addressed by Gualtieri et al. ( 2020 ) who concluded the need to perform an accurate ergonomic assessment at the first phase of workstation design. The last layer of analysis is the product level, whose design object is an artefact or a service solution.
In addition to the transdisciplinary approach—an integration of cross-disciplinary perspectives—in HCD, this multidimensional approach is also evidenced by the cross-layer level—the product and/or service, workstation, company to social level—in which humans are centred.
In-depth review of case studies
There are 43 papers that report case studies out of 77 included papers (around 56%), as detailed by “the Appendix ” (Table 11 ), which provides a useful source for researchers to make references to design for case studies. Those case studies report the design problems associated with the contexts, data collection, and analysis in both quantitative and qualitative approaches. The review objective is to make contributions to the future research agenda by harmonizing the lessons learnt that reveal the research results and limitations of the case studies. In addition, the subsequent section provides the emerging trend of concepts regarding HCD, followed by the structured harmonization of design methods.
Emerging trend
The strategy to categorize the case studies follows the design concepts embraced by the corresponding paper. Those concepts are not always explicitly indicated by the papers that may use the term “human” or “user” and even consider them interchangeable terms. This confusion is also reported by Holeman and Kane ( 2020 ) and Bazzano et al. ( 2017 ). Therefore, Table 6 structures the description of the concepts associated with their common context of use.
The variants of HCD reinforce the findings of the transdisciplinary and multidisciplinary approach—physical to cognitive ergonomics, products and/or services to social-technical systems—towards human diversity, ergonomics, economics, manufacturability, and social and human-related sustainability. Based on the understanding, Table 7 captures the emerging trend that provides insights into six concepts summarized in chronological order.
The top three concepts—namely HCD, PSS and UCD—that account for 35 out of 43 case studies (around 81%) are the most frequently and recently used concepts during the last three years. HCD is the most popular term, although it originated somewhere in the 1400s to systematically improve design for procedures and tools to accomplish the work (Nemeth, 2004 ). HCD has changed dramatically in the context of Industry 4.0, where scholars have expanded the research of physical ergonomics to systems including humans. Specifically, the case studies are designed in various implementation scales in different contexts: the product level by testing the method of individual product innovation design in solving bicycle problems based on ergonomic perspectives (Wu et al., 2013 ); the company level by validating the proposed model of the artificial self-organizing manufacturing control system explicitly putting humans in the centre of the system design (Pacaux-Lemoine et al., 2017 ). Beyond technology, the trend of market personalization has received increasing attention from researchers. The literature witnesses the increasing number of case studies that pertain to the concepts of PSS and UCD. The case studies also distinguish clearly between PSS and UCD by the way that PSS focus on business models at the company level while UCD experiments focus on human experiences about design for product and/or service solutions at the product level in consideration of human diversity and social aspects.
On the other hand, the case studies related to the concepts of HioTL, HMI and HRC are not well accounted for. One of the technical challenges is that the boundaries between technologies and humans are increasingly fuzzy: language processing, social robotics, artificial intelligence, cyber physical systems, virtual reality, and augmented reality. This phenomenon is blurring the limits of where the human ends and technology starts (Frauenberger, 2019 ; Gervasi et al., 2020 ; Weichhart et al., 2019 ; Wojtynek et al., 2019 ). Moreover, recent research tends to focus on technical aspects instead of tackling existing problems related to error-prone interaction between human and non-human actors (Klumpp et al., 2019 ; Song et al., 2016 ).
Another fact shows that the research community has responded in a determined way—35 case studies during the period of 2017–2020, which greatly exceeds other periods—to the call for empirical research in the field (Benabdellah et al., 2019 ; Kadir et al., 2019 ). This effort, which is worthy of emphasis, reveals an increasing interest in empirical studies, which brings research and industrial applications closer together. This trend also aligns with the future research recommendations: Factories of Future (European Commission, 2013 ) and Platforms for CPSs (Thompson et al., 2018 ). The following deep analysis manifests the design methods connected with supporting technologies that the papers embrace in order to realize the effort in question.
Design methods
Norman ( 2016 ) explains that “the human mind is exquisitely tailored to make sense of the world” (p. 2). This ability requires products and/or services that are designed for easy interpretation and understanding. Therefore, methods for design must define procedures, techniques, aids, or tools to discover the minds of humans—users, customers, stakeholders—that serve as key inputs resulting in well-designed solutions. Figure 5 captures the frequency of design methods that are discussed in four generic groups: discovery, clean-up, engineering, and experiment.
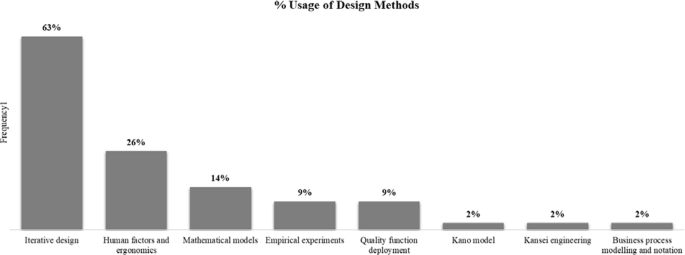
Design methods applied by the reviewed case studies. 1 Frequency divided by the total number of case studies (43 case studies) derived from “ Appendix ” (Table 11 )
Around 63% of case studies make the most of iterative design : knowledge obtained through the discovery is assured by an iterative process of idea exploration, gathering, and assessment. This method contains a bundle of procedures, techniques, and tools—participatory design, interviews, questionnaires, focus groups, scenario observation, field studies, prototyping—for searching and matching design ideas with the human mind. These approaches help designers focus on human diversity to gain critical design inputs and feedback: requirements elicitation acquired from maintenance professionals by field studies (Kaasinen et al., 2018 ), human perception of different stakeholders by focus groups (Turetken et al., 2019 ) and usage difficulties of non-expert users by scenario observation (Song et al., 2016 ). On the basis of questionnaires, Kong et al. ( 2019 ) also studied and called user frustration “the key pain spot” in the context of industrial wearable systems. They also pointed out countermeasures—confinable and reconfigurable modularized hardware sets—that address the usage, cognitional, and operational issues, and reduce the complexity and cost in the design solutions considering various aspects: ergonomics, plug-and-play features, and manufacturability. The modular approach is also comparable to product platform design that tackles the issues regarding manufacturability—product customization, variety, and commonality between products—and brings a competitive advantage: reduction in design effort and time-to-market for future generations of products (Farrell & Simpson, 2003 ; Martin & Ishii, 2002 ; Simpson, 2004 ). This is further evidence to show the necessity of the transdisciplinary and multidimensional approach within which an engineering method can also be applicable in the context of HCD to integrate human and non-human factors: human diversity, ergonomics, economics, manufacturability, and sustainability.
In addition to the acquisition of human needs and requirements, iterative design is also suitable for investigating “what-if” scenarios on design solutions. For instance, Kymäläinen et al. ( 2017 ) and Harwood et al. ( 2019 ) built fiction prototyping—video-illustrated and tangible interaction tools—to facilitate human-centred perception and cognition of the future potentials of products and/or services. This so-called design fiction—an interactive and tangible approach—evaluates alternative design solutions or criticizes existing ones (Knutz et al., 2014 ) before they are manufactured and/or delivered to customers, which enhances the robustness of iterative design by deeply understanding human experience.
Even though an effective understanding of human requirements is vital for well-designed solutions, this task is difficult due to various subjective human ideas: prioritization, complexity, imprecision, and vagueness. Clean-up is significantly more challenging for requirements of services than those of products (Haber & Fargnoli, 2019 ; Song & Sakao, 2016 ). To respond to the challenge, 6 out of 43 case studies (14%) deal with fuzzy inputs and multiple-criteria decision making by applying mathematical models : analytic network process (ANP), Thurstone’s Law of Comparative Judgments (LCJ), fuzzy set theory, and geometric vectors. While Zhu et al. ( 2015 ) took advantage of ANP to determine and prioritize the importance weights of engineering characteristics derived from a set of different customer requirements, Haber and Fargnoli ( 2019 ) prioritized customer requirements by the LCJ that transforms the customer preferences into scale values and then represents the importance of each preference. To quantify the complexity, Mourtzis et al. ( 2018 ) proposed a 2D geometric vector to estimate the product and service’s design complexity, which is defined by information content, quantification of information, and diversity of information. This quantification of complexity supports the decision-making process on alternative design solutions, taking manufacturability into account. To deal with imprecision and vagueness, Chen et al. ( 2016 ) evaluated the users’ perceptual images and feelings about products by the use of the fuzzy membership degree of emotional semantic descriptive words (e.g. traditional-modern, geometrical-organic, romantic-realistic). They also used a statistical method—principal component analysis—to cluster the varying user perceptions and feelings into homogeneous groups of design characteristics. Similarly, Leng and Jiang ( 2017 ) clustered similar individual service design processes into homogeneous bundles of services by applying a granular computing method—fuzzy set theory combined with quotient space theory for classification (or clustering) of uncertain complex problem (Zhang & Zhang, 2010 ). Taking both customer and engineering subjective ideas, Chen ( 2016 ) carried out the fuzzy analytic hierarchy process (AHP) to develop good quality design based on the imprecise relationship between engineering experience (robust design, design optimization, design cognition) and customer experience (requirements management, ergonomics design). Based on that, the author also proposed a linear programming model to optimize the total profit of the product mix-experience portfolio, taking economic considerations into account. This cost–benefit analysis needs to be embraced because its importance is stated by several authors, especially with regard to the entire life-cycle cost analysis (Anke, 2019 ; Heidari et al., 2020 ; Rodriguez et al., 2020 ). These mathematical methods are useful in dealing with the multiple-criteria decision making and fuzziness (uncertainty) under their own assumptions, constraints, and computing capability, requiring practitioners to be transdisciplinary and understand properly the methods in their context of use. For references regarding these methods, refer to the work of Golden et al. ( 1989 ), Kubler et al. ( 2016 ), and Liu et al. ( 2020 ).
In addition to the discover and clean-up, 26% of the case studies apply human factors and ergonomics to understand and evaluate quantitatively the interactions—physical and cognitive ergonomics—among humans and other actors (e.g., design artefacts, virtual objects, system interfaces, industrial workstations) from the engineering perspective. This method is not only for the expected cost saving, but also for the higher process efficiency that can be realized by shedding light on human factors and incorporating human needs and behaviour in a healthy, safe, efficient and enjoyable manner (Labuttis, 2015 ; Soares & Rebelo, 2016 ). In the context of Industry 4.0, this method is also supported by the digital technologies—virtual and mixed reality, eye-tracking systems, digital modelling and simulation for virtual workplaces—to facilitate designers to capture and analyse design data that span from the physical to cognitive level. On the cognitive level, Wu et al. ( 2016 ) studied the relationship between interface complexity and user diversity—novice and expert (human background)—by measuring users’ psycho-physiological data (eye-movement research) combined with questionnaire evaluation methods: NASA-task load index and Questionnaire for User Interface Satisfaction (QUIS) to measure operators’ subjective feelings and workload throughout the experiment. These eye-movement data provide insights into the visual, cognitive, and attentional aspects of human performance (Duchowski, 2002 ). In addition to the psycho-physiological analysis, Richert et al. ( 2018 ) surveyed participants’ personality dimensions—agreeableness, conscientiousness, neuroticism, openness to experience—to measure the performance and human perception of hybrid human–robot collaboration. On the physical level, Caputo et al. ( 2019 ) carried out an appraisal for the human-centred workplace design by reproducing a virtual workplace in which digital human modelling simulates the whole human task towards preventive ergonomics. Peruzzini et al. ( 2019 ) also designed the virtual workstation with preventive ergonomics by the use of digital technologies: virtual and mixed reality. They also used questionnaire methods to quantitatively measure postural comfort: Rapid Upper Limb Assessment (RULA) and Ovako Working Posture Analysis System (OWAS). The case studies apply a wide range of assessment methods regarding human factors and ergonomics: from simple checklists to more complex techniques; from physical ergonomics—for human use and performance (e.g., musculoskeletal symptoms, body posture, low back disorders)—to cognitive ergonomics—for human perception and cognition (e.g., mental stress, emotional stress, situation awareness). In addition, the work of Tillman et al. ( 2016 ), Forsythe et al. ( 2017 ) and Dalle Mura and Dini ( 2019 ) provides a good source of numerous methods for human factors and ergonomics that allow for achieving the various objectives of both manufacturability and social sustainability.
To bridge the gap between human requirements and engineering characteristics, four out of the 43 case studies apply quality function deployment (QFD), which originated in the automotive industry and has been being used with different applications in diverse fields for five decades (Kowalska et al., 2018 ; Zairi & Youssef, 1995 ). This method identifies human-centred requirements, classifies the importance of those requirements, defines engineering characteristics that may meet those requirements, allows for verification of design conflicts among them, and then prioritizes design solutions. In the analysed case studies, this method is also integrated with different methods—application space map and innovation matrix (Lee & Abuali, 2011 ), ANP (Zhu et al., 2015 ); AHP, fuzzy AHP, entropy weight method (Ma et al., 2017 ); LCJ and Kano model (Haber & Fargnoli, 2019 )—to enrich the prioritization and segmentation of the design requirements. The requirements after the cleanup are further converted into the engineering parameters by the QFD. For further reading, the work of Chan and Wu ( 2002 ) and Prasad ( 1998 ) may be of interest to the reader.
Furthermore, other methods also include the Kano model , Kansei engineering , business process modelling, and notation (BPMN). While Haber and Fargnoli ( 2019 ) applied the Kano model to prioritize and classify customer requirements into four different categories—must-be, one-dimensional, attractive, indifferent—for the segmentation of customer value propositions, Wang et al. ( 2018 ) parametrically linked the customer's emotional responses—physical and psychological—to the properties and characteristics of a product and/or service. If these methods focus on a particular process in design (requirement elicitation converted into engineering characteristics), Prinz et al. ( 2019 ) highlighted the use of BPMN to represent workflows—a graphical modelling language for all kinds of business processes. The BPMN is useful for examining a graphical description of design processes to different levels of granularity and discovering inconsistencies and/or differences in sequential steps, conflicting names, or acronyms, to name a few. Even though the methods have only been mentioned one time by the 43 case studies, they have been adapted and applied by different fields for years. Several publications are interesting works that may help readers have a better idea about the Kano model published by Zhao et al. ( 2020 ) and Shahin et al. ( 2013 ), Kansei engineering reviewed by Shiizuka and Hashizume ( 2011 ) and Coronado et al. ( 2020 ), BPMN studied by Ko et al. ( 2009 ) and Chinosi and Trombetta ( 2012 ).
Lastly, another way of gaining knowledge in design is empirical experiments , which account for four out of the 43 case studies. This method is useful for understanding what-if scenarios by different design configurations: an assisted versus collaborative robotic system that supports workers in a plug-and-produce workstation (Wojtynek et al., 2019 ), an automatic speed versus adaptive cruise control system for pedagogical learning supports (Vanderhaegen, 2019 ), delivery of health care services for seniors between a community hospital and social service agency (Hoe, 2019 ), augmented reality that supports trainers versus trainees in phone repairing operations (van Lopik et al., 2020 ). Those empirical experiments allow for designing hypotheses and gaining knowledge by means of direct and indirect experience. However, this method requires knowledge of the experimental setup and validation; it also has limited generalization of results due to controlled settings (Kulyk et al., 2007 ).
In summary, the case studies apply various methods that are categorized in the four generic groups—discovery, clean-up, engineering, experiment—associated with supporting technologies to tackle different problems, which requires the transdisciplinary approach for understanding and applying the methods in their proper context of use. While iterative design is power in discovering the human mind (needs, perception, cognition), mathematical models prioritize and classify those human inputs and support the decision-making process on design alternatives. Furthermore, human factors and ergonomics enrich the understanding of interactions—physical to cognitive ergonomics—among human and non-human actors with the support of digital technologies: virtual and mixed reality, eye-tracking systems, digital modelling and simulation for virtual workplaces. To convert the voice of humans into engineering parameters, the case studies have diverse approaches—QFD, Kano model, Kansei engineering, BPMN—and are used in different combinations. Finally, the empirical experiments gain knowledge based on the investigation of what-if scenarios under the human perspective, which is useful for iteratively improving and testing design solutions. Besides, researchers and practitioners alike also benefit from other relevant engineering methods—product platform design (Simpson et al., 2014 ), design for manufacturability and concurrent manufacturing (Anderson, 2014 ), to name a few—that embrace the transdisciplinary and multidimensional approach to deal with a multi-objective design problem towards human diversity, ergonomics, economics, manufacturability, and sustainability.
These various methods dealing with different problems in diverse contexts of use lead to different lessons learnt in the form of their research results and limitations. The following lessons learnt are useful for subsequent researchers to choose proper research areas and advance research contributions to the field by avoiding the research limitations.
Lessons learnt
One way to organize the case studies sharing mutual facts and document them as the lessons learnt is to use an affinity analysis, which is also known as the KJ method and applied in various fields (Awasthi & Chauhan, 2012 ). The information captured during the analysis is tabulated by “ Appendix ” (Table 11 ), providing researchers useful details about design for case studies. Based on the analysis output, Table 8 categorizes the case studies’ results and limitations into six groups of research results (RR) and four groups of result limitations (RL).
One of the most attractive outcomes those case studies reported is the exploration of the design success factors—which are denoted as RR2 accounting for around 47% of the case studies—revealing how the successful deployment of design oriented to humans can be generalized in various contexts. Figure 6 structures those success factors as a triangular decision-making diagram:
Stakeholder networks : the organizational, social, and environmental contexts—which involve stakeholders (e.g., users, customers, employees, suppliers, distributors, partners, regulators, etc.) through the life-cycle design process—are essential for enhancing the credibility of information and promoting the sharing of transdisciplinary knowledge as valuable design inputs (Chen, 2016 ; Mazali, 2018 ; Schulze et al., 2005 ; Witschel et al., 2019 ). The diversity in interests and expectations of the stakeholders needs to be respected and analysed to comprehend the impact of stakeholder interactions and their features at different life-cycle design phases: design, production, delivery, service, maintenance and end-of-life cycle (Mourtzis et al., 2018 ; Turetken et al., 2019 ; Zhang et al., 2020 ). In this respect, the involvement of the users or customers in the early development stage is well realized (Chen et al., 2016 ; Grieger & Ludwig, 2019 ; Hoe, 2019 ).
Levels of involvement : the engagement modes of stakeholders are depicted by three levels of involvement. These levels include the informative level in which stakeholders only provide and receive design information; the consultative level in which they comment on pre-defined design scenarios; and the participative level in which they make influencing decisions on a design process, which is a higher level of engagement than that of the informative level, which only considers stakeholders as information sources in the design process (Schulze et al., 2005 ; van Lopik et al., 2020 ).
Design practice : the design development—which responds to the extents to which the data about users, customers, and other relevant stakeholders should be properly obtained and analysed—needs to be defined. These data include physical activities, behaviours, opinions, feelings, personalities, and physiological responses (Lin, 2018 ; Peruzzini et al., 2019 ; Richert et al., 2018 ; Wang et al., 2018 ). They are explicitly classified into two groups: physical ergonomics—which emphasizes physical characteristics—and cognitive ergonomics, which reflects the integration of cognition thinking and cultural characteristics—individual aesthetic habits, national, ethnic cultural differences—to address social-technical aspects in the context of Industry 4.0 (Bednar & Welch, 2020 ; Fosch-Villaronga et al., 2020 ; Zhou et al., 2012 ).
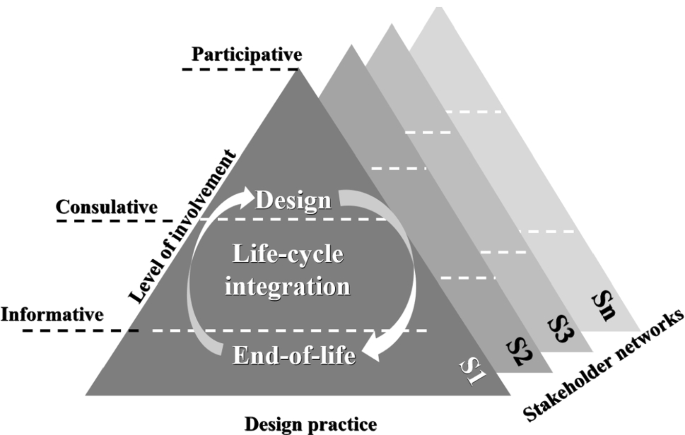
A triangular decision-making diagram in HCD, encompassing design decisions on who in the stakeholder networks (S1, S2, S3, Sn) will be involved, at what levels of involvement, where the involvement will take place in each through-life phase, and what design knowledge should be exploited within the scale of physical to cogitive ergonomics
The knowledge management of these design data is well expressed as an enabling success factor that can be exploited by digital technologies. These technologies facilitate the collection, organization, retrieval, and reuse of design knowledge in an effective manner. While Fu et al. ( 2019 ) took advantage of IoT solutions (sensors) for user data collection—unintentional behaviour, emotion, culture—and artificial intelligence for data processing, Vanderhaegen ( 2019 ) and Grandi et al. ( 2020 ) made use of digital and mixed reality simulation in measuring human factors—physical stress, physiological data—and evaluating their design experiments. Instead of starting from scratch, Zhu et al. ( 2015 ) and Leng and Jiang ( 2017 ) established mathematically a collection of semantic commonalities derived from historical design ontology-based databases—activities, functions, concepts, process sequences—to build a knowledge platform from which a stream of new derivative products and services can be efficiently developed. The objective is to design for variety and custom solutions, enabling designers to not only save time and cost but also make the most of the experience and expertise that were dedicated to the past design activities. The method used to build the knowledge platform is also comparable with product platform design, which has been maturely researched over the last decade (Simpson et al., 2006 , 2014 ) and is a useful source regarding methods and applications for researchers in the field of product and/or service design.
The second group is the engineering objectives of design (RR1) that are converted into key performance indicators to quantify the effectiveness of the proposed models or frameworks. Around 23% of the case studies indicate that their proposed solutions achieve the engineering objectives: avoidance of ergonomic risks (Caputo et al., 2019 ; Ceccacci et al., 2019 ), improvement of productivity and simultaneously biomechanical workloads (Gualtieri et al., 2020 ; Wojtynek et al., 2019 ), production performance in terms of quality and engineering time (Pacaux-Lemoine et al., 2017 ; Prinz et al., 2019 ). Furthermore, Wu et al. ( 2013 ) proposed a multi-function and modular method for design focusing on human anthropometrics—the branch of ergonomics that deals with measurements of the physical characteristics of human beings (Pheasant, 1990 )—and extending products’ service life towards sustainability. Similarity, Chen et al. ( 2016 ) applied a clustering method for product family design based on anthropology—research in understanding human culture, society, and difference (Monaghan & Just, 2000 )—to improve the agility of the design process towards manufacturability. This product family design allows designers to not only utilize existing design methods from the product platform to form a series of products, but also gain inspiration from different ethnic groups—human diversity with distinct cultural traits—to extract ideal design elements. In another aspect, Chen ( 2016 ) emphasized directly the cost–benefit analysis of design quality, taking into account two economic elements: estimated profit; total cost comprising R&D cost, market capital, and design quality for market share. The reported figures prove the robustness and performance of a system—human diversity, ergonomics, economics, manufacturability, sustainability—can be achievable with the approaches of HCD.
The next research interest is to provide supporting design frameworks (RR6) that facilitate the design process by providing systematic thinking—the use of the integrated novel design methods (innovation matrix, application space mapping, QFD) and Lean initiatives (avoidance of valueless reworks and activities)—towards economic sustainability (Lee & Abuali, 2011 ; Pezzotta et al., 2018 ). Other studies focus on design solutions for complexity and uncertainty: incomplete information regarding human requirements (Haber & Fargnoli, 2019 ); the changes in human preferences (Lin, 2018 ); decision making on different design alternatives for mass customization towards manufacturability (Mourtzis et al., 2018 ); interaction requirements among non-human—smart manufacturing devices/tools, core enterprise business systems (ERP, SAP)—and human actors (manufacturers, designers, users) (Mostafazadeh Davani et al., 2018 ; Song et al., 2016 ; Zhang et al., 2020 ); adaptation of design processes to the context of small-and medium-sized enterprises (Adrodegari & Saccani, 2020 ; van Lopik et al., 2020 ). These studies tackle different problems scattered across life-cycle design phases, useful to consider in relation to further research to address the relevant problems in a comprehensive way.
Around 12% of the case studies made an effort to validate the effect of human diversity on the design outcomes (RR3). They concluded with the important inclusions of individual differences—background, age, gender, education, cultural influences, privacy management—in design. Statistically, Wu et al. ( 2016 ) confirmed that information overload in interface design increased cognitive workload for novice operators compared to expert operators and therefore decreased user efficiency. Similarly, Van Acker et al. ( 2020 ) concluded statistically that higher acceptability of wearable mental workload monitoring was associated with being a woman (for trust in the technology), higher technology readiness—the willingness to accept new technologies and security about private data (Victorino et al., 2009 )—and lower educational backgrounds. Besides, lack of considerations regarding specific classes of difference between humans leads to major effects on design outcomes in various design contexts: age with older people (aged 55–75 years) in safe driving (Jung et al., 2017 ) and health sector (Hoe, 2019 ); cultural influences (Russians, a Frenchman, a Chinese) in the experiment of long-term isolation in a limited room space (Boy, 2018 ). These studies address the concern that if design does appreciate individual differences towards the multidimensional approach—considering not only product and/or service design but also social aspects—this could avoid the thwarting of all research efforts and the subsequent lessening of potential benefits.
In addition to the multidimensional approach, four studies also directly address the need for collaborative design frameworks (RR5): the transdisciplinary approach during the life-cycle design phases. Ma et al. ( 2017 ) exploited common expertise of transdisciplinary teams to convert customer requirements into semantic requirement groups that were subsequently transferred into product design specifications through the use of QFD. Based on the perspective of cross-cutting collaboration for advanced business intelligence, Kong et al. ( 2019 ) structured a common platform design of wearable-enabled applications with three aspects of manufacturability: re-configurability, robust architecture, and design scalability. This platform allows standardization by taking advantage of plug-and-play features and modular approaches to integrate human and non-human actors: artificial intelligence, virtual reality, IoT, cloud computing, and cloud-based cyber systems (enterprise resource planning, manufacturing execution systems, warehouse management systems). In addition to manufacturability, Anke ( 2019 ) and Turetken et al. ( 2019 ) addressed directly the aspects of life-cycle cost analysis in the context of smart services. Specifically, Anke ( 2019 ) assessed the profitability of a smart service at an early stage of service design by developing a web-based tool prototype by which project teams from different disciplines collaborate in the design and evaluation process. In a broader sense, Turetken et al. ( 2019 ) promoted the transdisciplinary and iterative approach in which a network of actors—providers, customers, authorities, retailers, event organizers—co-creates the value-in-use for customers and generates benefits—financial and non-financial characters—for all network partners moving towards sustainability. Each study focuses on an important aspect of design—human diversity, ergonomics, economics, manufacturability, sustainability—that needs to be considered together in a transdisciplinary and multidimensional approach for future research.
In the last group of research interest, three studies present experience-driven approaches that visualize design scenarios (RR4) regarding future possibilities to exploit human experience. Based on design fiction, both Kymäläinen et al. ( 2017 ) and Harwood et al. ( 2019 ) demonstrated the usefulness of the video-illustrated prototype in avoiding the difficulty of interpreting abstract verbal descriptions of new design. This method enables designers to interactively envisage a spectrum of “what if” scenarios towards human experience that may then be explored by using the range of other design methods: focus groups, interviews, and questionnaires. Besides, Kaasinen et al. ( 2018 ) made the most of the technologies in Industry 4.0—wearable technologies, virtual and augmented reality—to visualize the human experience of future maintenance work: feeling competent, feeling connected to the work community, feeling a sense of success and achievement by performing better in jobs. These studies go beyond technical design towards the multidimensional approach: they go from the technical to the social aspects.
Even though all case studies reported positive outcomes, four groups of result limitations are also acknowledged. The most frequently reported limitation is the lack of statistical power in result validation (RL1)—accounting for 60% of total analysed case studies—and the rest is undefined due to limited information for making the conclusion. The lack of statistical power shows limitations in experimental set-up conditions: low sample sizes, lack of fitting in target participants, lack of sound statistical studies, and other biased experimental aspects (Pacaux-Lemoine et al., 2017 ; Richert et al., 2018 ; van Lopik et al., 2020 ). This limitation is followed by the lack of generalizability (RL2) showing the insufficient evidence of the extent to which findings from one study in one context can be applied and reproduced to other contexts. Specifically, 56% of the case studies are constrained and required to be tested by further quantitative methods to prove the transferability of their observed results to other usage contexts (Adrodegari & Saccani, 2020 ; Haber & Fargnoli, 2019 ; Kong et al., 2019 ; Witschel et al., 2019 ). The next limitation is categorized as incomplete solutions to implement the proposed models (RL4)—accounting for around 30% of the case studies—claiming the quality of the proposed models will depend on other external factors. These factors include the “manual” processing of the proposed models, resulting in application difficulties (Ceccacci et al., 2019 ; Zhang et al., 2020 ), which requires additional efforts in further development of supplementary methods and applications to achieve model completion in real contexts (Grieger & Ludwig, 2019 ; Leng & Jiang, 2017 ; Lin, 2018 ; Peruzzini et al., 2019 ). Finally, around 23% of the case studies do not explicitly provide the validation of effectiveness of the proposed solutions (RL3), which emphasizes the need for future research for their validation in various contexts of usage; otherwise, the practical effectiveness of the proposed solutions from the studies is limited (Ceccacci et al., 2019 ; Haber & Fargnoli, 2019 ; Witschel et al., 2019 ).
These limitations are explained through the evaluation methods—which are different from the design methods used as procedures or processes for attaining research findings—applied by the case studies to validate their corresponding research findings. Figure 7 , which is visualized from the detailed data of “ Appendix ” (Table 11 ), shows the top four evaluation methods accounted by qualitative methods: questionnaires, interviews, scenario observation, and workshops. These methods validate the effectiveness of the corresponding proposed models by capturing and communicating the participants’ feedback via different means, leading to a potential lack of robustness in research and encompassing subjectivity and bias in research conclusions (Jung et al., 2017 ; Richert et al., 2018 ; Van Acker et al., 2020 ), which is followed by insufficient generalizability, as analysed above.
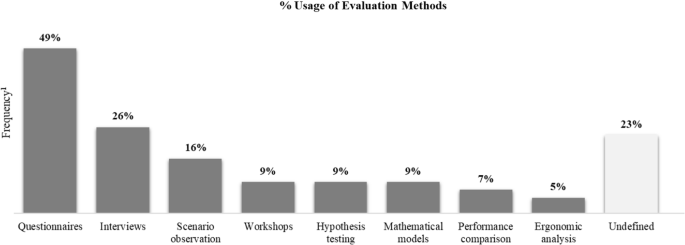
Evaluation methods applied by the case studies reviewed. 1 Frequency divided by the total number of case studies (43 case studies) derived from “ Appendix ” (Table 11 )
Although there is a small portion of case studies applying quantitative methods—hypothesis testing and mathematical models (around 9%), performance comparison (around 7%), and ergonomic analysis (5%)—the validation of the case studies’ findings is still questionable. Specifically, by applying the hypothesis testing, L. Wu et al. ( 2016 ) made an effort to carry out a case study of eye tracking with 38 participants that compared three levels of interface complexity in LED manufacturing systems, resulting in the statistical conclusion of interface complexity and user background affecting the user experience. However, the study failed to prove sufficient statistical power, showing its proper selection of sample size. Moreover, the sampling procedure included only the participants who were all from the same company, leading to biased results and affecting the generalizability of research outcomes. Out of 43 case studies, Ceccacci et al. ( 2019 ) and Gualtieri et al. ( 2020 ) conducted ergonomic analysis to validate the effectiveness of their workstation design—productivity, human postural comfort—with a sample size of only two participants. This small sample size, due to its lack of generalizability, requires further research to validate the studies’ applicability in a real context with human diversity. This problem was further evidenced by Van Acker et al. ( 2020 ) who reported that, statistically speaking, the replication of their case study’s findings found in the first experiment was not successful in the second experiment carried out within the same research context, so leaving the conclusion inconclusive. These limitations lead to a lack of robustness in research findings and reduce applications of these studies in industry and research alike.
In summary, the research efforts contributing to the realisation of human roles in Industry 4.0 span six groups of research results: exploration of design success factors, achievement of engineering objectives, provision of supporting design frameworks, validation of the effect of human diversity on design, provision of transdisciplinary frameworks, and visualization of design scenarios. Each study focuses partially on its own defined aspects, which provides a useful reference for future research that combines the transdisciplinary and multidimensional approach towards human diversity, ergonomics, economics, manufacturability, and sustainability in a comprehensive way. Besides, it is worth realizing the lessons learnt in order to overcome the acknowledged limitations—limited statistical power in result validation, lack of generalizability of research findings, further requirements for the supporting methods, lack of validation of the effectiveness—and enhance the robustness of the research findings. This will inspire research applications to both industry and research. Finally, the following section discusses the results of the in-depth review and ends with future research opportunities.
Discussion and opportunities for future research
The analysis of the overall characteristics of the literature regarding HCD reveals its nature and evolution towards Industry 4.0. Various disciplines have made efforts to integrate human roles into the design process, spreading extensively from artefact and service designs to system designs, taking social manufacturing contexts in Industry 4.0 into account. The topic has gained clear momentum, and interest in different concepts of HCD has increased exponentially. This phenomenon leads to evidence of evolution in HCD, whose characteristics and contextual variants—HCD, PSS, UCD, HMI, HioTL, HRC—have evolved in different disciplines across the value chain to tackle new requirements of Industry 4.0. Specifically, HCD is not only applied for the design of procedures or tools to accomplish a task but is also required to have a transdisciplinary approach. This approach ranges from physical ergonomics—for effective and safe human use—to cognitive ergonomics—for treating personality styles. Another piece of evidence is the multidimensional approach of HCD, whose unit analysis originates from design for the product and/or service level to the workstation and company level, and extends to the level of society: ethical, legal and social concerns have risen along with Industry 4.0. However, concerning the industrial state of the art in this topic, there is a lack of evidence of research with full-scale real implementations that go into any detail on cross-level designs that range from the artefact to the social level from which human issues—privacy, ethnic cultural differences, personality styles—are taken into account within transdisciplinary and multidimensional design thinking. Although an increasing number of studies integrate humans in smart manufacturing, many of them limit research scope to physical ergonomics: human factors and ergonomics on operational levels (Kadir et al., 2019 ; Pacaux-Lemoine et al., 2017 ; Peruzzini et al., 2019 ; Wojtynek et al., 2019 ). Therefore, future research needs to pay attention to the transdisciplinary and multidimensional approach.
Moreover, the changes that trigger Industry 4.0 have impacted throughout the value chain in which the human roles have been shaped in the different phases of the value chain, requiring new approaches to integrate humans in the cycle. This phenomenon also leads to the different variants of HCD as an evolution evidenced by the in-depth review of case studies. Those concepts have been widely studied in recent years, and there is no clear evidence for their maturity, which is further emphasized by the number of conceptual and empirical papers associated with the case studies found in the literature review. In particular, the terms HCD, PSS and UCD have received the most attention in the literature, showing their emerging trend of catching up with the challenges of dynamic environments and diverse changes in the design requirements aimed at personalization and sustainability. To realize the full potential of smart manufacturing, however, the other concepts of HioTL, HMI, and HRC also deserve more attention not only in conceptual research but also in empirical experiments. This is a good indication for both industry and research to pay attention to the numerous research efforts in exploring the various concepts of HCD to tackle the challenging requirements of industry 4.0. In this respect, an interesting consideration for future research would be to try to better unify the relationships between those concepts in order to embed them completely into the cornerstone of Industry 4.0 infrastructure.
In addition, the challenges in Industry 4.0 also call for diverse design methods that tackle different problems across the life-cycle design phases in the transdisciplinary and multidimensional approach. To respond to the call, the in-depth review of case studies captures a wide range of design methods categorized into four generic groups—discovery, clean-up, engineering, and experiment—associated with supporting technologies. While the discovery makes the most of the iterative design—participatory design, interviews, questionnaires, focus groups, scenario observation, field studies, prototyping—to discover human needs and requirements, the clean-up encompasses the mathematical models—ANP, LCJ, fuzzy set theory, geometric vectors—to classify and prioritize the design requirements and make multiple-criteria decisions on design alternatives. Subsequently, the group of engineering methods—human factors and ergonomics, QFD, Kano model, Kansei engineering, BPMN—converts the requirements into engineering characteristics and establishes the design process flow to centre design on humans. Lastly, the case studies carry out the experimental setups for understanding what-if scenarios by different design configurations, which is useful for iteratively improving and testing design solutions from the human perspective. Besides, the support of digital technologies—virtual and mixed reality, eye-tracking systems, digital modelling and simulation for virtual workplaces—enables designers to capture and analyse design data in an efficient way. Due to varying methods in design, it is helpful for researchers and practitioners who are transdisciplinary and understand properly the methods in their context of use. In addition to the design methods, some other engineering methods available in the literature—product design platform (Simpson, 2004 ), mathematical multi-objective models taking human factors and ergonomics into account (Dalle Mura & Dini, 2019 )—are also worthwhile complementing the design toolkit for both products and/or services to acquire multiple design objectives—human diversity, ergonomics, economics, manufacturability, and sustainability—through the transdisciplinary and multidimensional approach in HCD.
Furthermore, the literature review also provides the detailed and useful information extracted from the analysed case studies in the subsection lessons learnt , showing the diverse applications of these concepts in different industrial contexts associated with the insights they provide. These lessons learnt to represent various research results associated with limitations that are captured and harmonized in homogeneous groups: six groups of research results and four groups of research limitations. Given the results, the design success factors—which are again reflected by the transdisciplinary and multidimensional characteristics—are the proper design decisions: the stakeholder networks; levels of involvement of each stakeholder at each design life-cycle phase; how deep analysis of design will take place, ranging from physical ergonomics to cognitive levels in the context of use directed to Industry 4.0. Future research needs to express these success factors that deserve attention and emphasis in a comprehensive way to avoid research limitations and market failures in industry.
Another enabling success factor is the knowledge management of design data. The digital technologies—IoT, artificial intelligent, virtual and mixed reality—facilitate the design knowledge to be collected, organized, retrieved, and reused in an effective manner. This advantage in Industry 4.0 enables designers to facilitate the multidimensional approach in the design knowledge that ranges from physical stress, to physiological data, to social data: culture, human behaviour, emotion, and background. In addition to the technology, a well-established method to construct and manage design knowledge is worth considering in future research. The useful method in this case is to establish a knowledge platform that defines a collection of semantic commonalities derived from historical design ontology-based databases. This platform design enables a new stream of products and/or services to be developed in an efficient manner towards economics and manufacturability: design for variety and customization, the use of the existing design experience, and expertise that reduces design efforts and enhances collaborative working.
In addition to the success factors, 10 out of 43 case studies provide quantifiable outcomes. These results prove that the robustness and performance of the systems can be achieved with the applications of HCD in different aspects: human diversity, ergonomics, economics, manufacturability, and sustainability. A limited array of studies incorporates human diversity—human culture, society, background—to improve robustness and sustainability—which combine the human difference with the extended service life—of design solutions. In contrast, numerous studies enhance the robustness in human performance by ergonomics: avoidance of workplace risks and reduction in biomechanical workloads. This outcome also improves economics and manufacturability in terms of production performance: productivity, engineering time, and quality. Moreover, the engineering methods—design for product platform and family, design for multi-functionality and modularity alike—seek a common design platform that paves the way for manufacturability and economics: reduction in design effort, time-to-market for future generations of products and/or services. Beyond the engineering methods, future research needs to embrace the financial perspective to quantify and evaluate the economics of HCD, such as the cost–benefit analysis that can also be extended to the life-cycle cost analysis. However, each study limits its research scope in one of these aspects, which provides a pivotal research space for subsequent researchers, who should grasp these aspects in their research of HCD within a comprehensive approach. Besides, the rest of the case studies provide limited information about how their design proposals are effective in quantifiable ways, creating a need for future quantitative research rather than the qualitative approach. Regarding this research opportunity, it is also useful to make contributions to the creation of a design evaluation system oriented to the process of HCD. This design evaluation system has the following ultimate objectives: to evaluate how well the decisions and activities that are made during the design phases actually turn out, to monitor the design process, and to facilitate decision making on any potential breakdowns and pitfalls.
Other research efforts provide the design frameworks in different contexts of use: the supporting design frameworks that facilitate the design process in an effective manner and the collaborative design frameworks that promote the transdisciplinary and multidimensional approach. The former provides systematic design thinking—integrated design methods to avoid valueless reworks and activities towards economic sustainability—and possible ways to tackle different challenges—the complexity and uncertainty in the relationship between human and non-human actors—scattered across life-cycle design phases. The latter unfolds the common expertise of transdisciplinary teams to co-create value-in-use for customers and also generate benefits—financial and non-financial measures—for all network partners towards sustainability. These frameworks reflect perspectives of the common platform design and life-cycle cost analysis, which are useful considerations for future research to contribute to multi-objective HCD in a comprehensive way.
The minority of case studies have paid attention to experience-driven design with visualization techniques: design fiction with the video-illustrated prototype, and virtual and augmented reality. These case studies give inspirational examples of how digital technologies enrich the human experience, rather than physical real prototypes that are difficult to produce or interpret in abstract verbal descriptions. This approach examines future possibilities of new design that allow designers to comprehend the human experience and go beyond technical design towards the multidimensional approach, from technical to social aspects. In this respect, another interesting research domain would be exploring the possibility of making the best of the technologies in the age of Industry 4.0 to support the process of HCD. This direction of future research would be beneficial to fulfilling the limitations—namely RL4 in Table 8 —that express different concerns: computational capability (Ceccacci et al., 2019 ; Chen et al., 2016 ; Leng & Jiang, 2017 ), data synchronisation (Lin, 2018 ; Peruzzini et al., 2019 ), and knowledge management (Fu et al., 2019 ; Grandi et al., 2020 ; Vanderhaegen, 2019 ; Zhu et al., 2015 ).
A limited range of studies put the perspective of human diversity towards the multidimensional approach that considers not only design artefacts but also the social aspects—background, age, gender, education, cultural influences, privacy management—in design. Lack of consideration of the difference between humans could thwart all research efforts and lessen potential benefits. This is particularly true in the context of population aging, which makes human diversity an essential consideration across diverse fields (Ahmadpour et al., 2019 ; Dankl, 2017 ; Lee & Coughlin, 2015 ). This phenomenon challenges manufacturing design in Industry 4.0, requiring a multi-objective methodology to capture diverse human factors. For example, Dalle Mura and Dini ( 2019 ) optimized ergonomics in assembly lines by proposing a multi-objective genetic algorithm capturing human factors: age, gender, weight, height, and skill. However, Katiraee et al. ( 2019 ) indicated that human differences regarding age and skill have been well studied in the literature, while few studies investigate other human aspects, including cognitive abilities. Therefore, future research on the topic should be ready to accommodate individualization in accordance with human diversity to encapsulate a new relationship between society and technology in the context of Industry 4.0.
Last but not least, the robustness of the research findings could be jeopardized if the identified limitations could not be alleviated. The majority of identified limitations are assigned to the experimental set-up conditions: low sample sizes, lack of fitting in target participants, lack of sound statistical studies, and other biased experimental aspects. There is also insufficient evidence of the extent to which these findings in one context can be applied and reproduced in other contexts. Future research would be trying to establish and enhance the robustness of research results by satisfying certain criteria for validity, such as the use of multiple sources of evidence, replication logic in multiple-case studies, and the well-established protocol of design for case study (Isaksson et al., 2020 ; Voss et al., 2002 ).
Throughout the value chain, the impact and increasing challenges of the transition to Industry 4.0 mean that integrating the role of humans is a part of the transition. It is going to attract more and more research efforts for the next decade, at least in the following five years. This is an opportunity to look back in a systematic manner on what the literature has achieved and the lessons it’s learnt, as summarized in the following points for the considerations of future research:
Research approach : The fulfilment of the transdisciplinary and multidimensional HCD needs to be achieved through a systematic identification of stakeholder networks, levels of their involvement in each life-cycle design process, and design practice.
Research scalability and robustness : The proposals of a design methodology should provide well-proven empirical results in well-validated case studies in varied contexts in which the individualization towards human diversity is taken into account.
Research performance : A holistic approach is needed to make the best of Industry 4.0 technologies, facilitating the process of HCD in which both human and non-human actors are integrated towards human diversity, ergonomics, economics, manufacturability, and sustainability.
Research framework : A new validated framework of HCD should take the points above into account and incorporate a well-rounded evaluation methodology to quantify the outcome of design activities across the life-cycle design phases. Besides, an interesting consideration in future research is to unify the relationships among the variants of HCD in order to embed them into the complete infrastructure of Industry 4.0.
These research schemes are challenging in a way that requires the increasing involvement of transdisciplinary collaboration in which researchers and industrial experts are brought together. This collaborative research is especially called in the phenomenon in which a transdisciplinary and multidimensional approach is required for a specific scientific topic (Chen & Duh, 2019 ; Hammer et al., 2018 ). This is also an approach for our next contribution.
Active work on developing methods, exploring influencing factors, and proving the effectiveness and efficiency regarding HCD show the increasing awareness of human roles in Industry 4.0. However, numerous studies have been brought into existence, but then subsequently disconnected from other studies. As a consequence, the application of these studies in industry and research alike is not regularly adopted, and the array of studies is broad and expands in different directions without forming a coherent structure. This study is one of the unique attempts to bridge the gap between the literature characteristics and the lessons learnt derived from an expository of case studies of HCD in the context of Industry 4.0. In order to sufficiently cover the research topic and provide evidence with a minimal amount of subjectivity and bias, this research performs SLR in which a special unit of analysis is given to the case studies, delivering the contributions in three ways. First, the approach to HCD claims to be transdisciplinary and multidimensional, which is evidenced by the overall literature characteristics: increasing research interest across disciplines and industries in different levels of analysis—product, workstation, company, and society.
Secondly, the transdisciplinary and multidimensional approach is also reflected by the in-depth review of case studies: the emerging trend, the design methods and lessons learnt. The review of the 43 case studies unfolds the emerging research themes—HCD, PSS, UCD—that deal with the challenges of personalization, servitization, and sustainability in the context of Industry 4.0. This phenomenon also leaves research space for the other concepts—HRC, HioTL, HMI—in smart manufacturing in the form of empirical research. Besides, the in-depth review also captures the wide range of design methods that are categorized in the four generic groups—discovery, clean-up, engineering, experiment—to tackle different problems scattered across different life-cycle design phases. Furthermore, the implementation of these design methods is also facilitated with the support of digital technologies: virtual and mixed reality, eye-tracking systems, digital modelling and simulation for virtual workplaces, IoT solutions, artificial intelligent. The variety in both quantitative and qualitative design methods associated with the supporting technologies expresses the necessity of the transdisciplinary and multidimensional approach for comprehending the methods in their proper context of use towards human diversity, ergonomics, economics, manufacturability, and sustainability. Therefore, for better adaption to the challenges, it is worth having cross-disciplinary collaborative research and/or improving the transdisciplinary skill sets of researchers and practitioners. This fact is further emphasized by the lessons learnt that dig into what the literature has achieved. The “ Appendix ” (Table 11 )—which functions as a useful reference for the design of case studies—expresses the most important facts about the 43 case studies, resulting in the lessons learnt. These lessons learnt encapsulate various research results associated with limitations that are captured and harmonized in homogeneous groups: six groups of research results and four groups of research limitations. The research results are categorized into six groups: exploration of design success factors, achievement of engineering objectives, provision of supporting design frameworks, validation of the effect of human diversity on design, provision of transdisciplinary frameworks, and visualization of design scenarios. Different studies concentrate partially on their own expected results, which highlights a useful reference for future research that expresses both the transdisciplinary and multidimensional approach towards human diversity, ergonomics, economics, manufacturability, and sustainability in a comprehensive way. Besides, it is worth acknowledging the limitations—limited statistical power in result validation, lack of generalizability of research findings, further requirements of the supporting methods, lack of validation of the effectiveness—to enhance the robustness of the research findings. This will inspire research applications to both industry and research.
Third, the opportunities for future research regarding HCD in the context of Industry 4.0 are also provided to advance the research contributions in the coming years through the adoption of the lessons learnt from the previous works. Despite the rigor, relevance and expanse of this study, there are acknowledged limitations. Primarily, we applied the strict protocol of SLR with which some relevant papers might be overlooked. To minimize this, we searched eight databases to ensure a sufficient number of papers relevant to this topic to compensate for the missed papers—missed due to less relevance—by supplementing more relevant papers. Furthermore, we limited the papers to only peer-reviewed journal articles as a means to guarantee the quality of the publications. We also acknowledge that the selection of the topic, definition of search terms, and interpretation of the results are inseparable from our previous knowledge on the topic. Lastly, we assume that considerable knowledge resides among practitioners’ experience and the grey literature.
The particular interest in this topic is the question of how to take advantage of literature, overcome its own acknowledged limitations, and advance research contributions in the body of knowledge. The first two questions are provided in this study, and the last one can be achieved by collaborative research in which transdisciplinary and cross-sectorial research centres and industrial partners join forces to contribute to a comprehensive common understanding of HCD in the transdisciplinary and multidimensional approach towards human diversity, ergonomics, economics, manufacturability and sustainability. This is also the approach for our next contribution to the field of HCD.
Availability of data and materials
Not applicable.
Code availability
Adrodegari, F., & Saccani, N. (2020). A maturity model for the servitization of product-centric companies. Journal of Manufacturing Technology Management, 31 (4), 775–797.
Google Scholar
Ahmadpour, N., Pedell, S., Mayasari, A., & Beh, J. (2019). Co-creating and assessing future wellbeing technology using design fiction. She Ji: THe Journal of Design, Economics, and Innovation, 5 (3), 209–230.
Anderson, D. M. (2014). Design for manufacturability: How to use concurrent engineering to rapidly develop low-cost, high-quality products for lean production . Apple Academic Press.
Anke, J. (2019). Design-integrated financial assessment of smart services. Electronic Markets, 29 (1), 19–35.
Aurich, J. C., Schweitzer, E., & Fuchs, C. (2007). Life cycle management of industrial product-service systems. In S. Takata & Y. Umeda (Eds.), Advances in life cycle engineering for sustainable manufacturing businesses (pp. 171–176). Springer.
Awasthi, A., & Chauhan, S. S. (2012). A hybrid approach integrating affinity diagram, AHP and fuzzy TOPSIS for sustainable city logistics planning. Applied Mathematical Modelling, 36 (2), 573–584.
Bauer, D., Schumacher, S., Gust, A., Seidelmann, J., & Bauernhansl, T. (2019). Characterization of autonomous production by a stage model. Procedia CIRP, 81 , 192–197.
Bazzano, A. N., Martin, J., Hicks, E., Faughnan, M., & Murphy, L. (2017). Human-centred design in global health: A scoping review of applications and contexts. PLoS ONE, 12 (11), e0186744.
Bednar, P. M., & Welch, C. (2020). Socio-technical perspectives on smart working: Creating meaningful and sustainable systems. Information Systems Frontiers, 22 (2), 281–298.
Benabdellah, A. C., Bouhaddou, I., Benghabrit, A., & Benghabrit, O. (2019). A systematic review of design for X techniques from 1980 to 2018: Concepts, applications, and perspectives. International Journal of Advanced Manufacturing Technology, 102 (9–12), 3473–3502.
Bhamare, D., Zolanvari, M., Erbad, A., Jain, R., Khan, K., & Meskin, N. (2020). Cybersecurity for industrial control systems: A survey. Computers & Security, 89 , 101677.
Boell, S. K., & Cecez-Kecmanovic, D. (2015). On being ‘Systematic’ in literature reviews in IS. Journal of Information Technology, 30 (2), 161–173.
Boschetti, G., Bottin, M., Faccio, M., & Minto, R. (2021). Multi-robot multi-operator collaborative assembly systems: A performance evaluation model. Journal of Intelligent Manufacturing, 32 (5), 1455–1470.
Boy, G. A. (2018). Human-centered design of upcoming human missions to Mars. Journal of Space Safety Engineering, 5 (1), 9–13.
Brenner, W., Karagiannis, D., Kolbe, L., Krüger, J., Leifer, L., Lamberti, H. J., Leimeister, J. M., Österle, H., Petrie, C., Plattner, H., Schwabe, G., Uebernickel, F., Winter, R., & Zarnekow, R. (2014). User, use & utility research: The digital user as new design perspective in business and information systems engineering. Business and Information Systems Engineering, 6 (1), 55–61.
Campbell, F. J. (2021). Human factors: The impact on industry and the environment. In E. R. Rhodes & H. Naser (Eds.), Natural resources management and biological sciences (pp. 1–14). IntechOpen.
Caputo, F., Greco, A., Fera, M., & Macchiaroli, R. (2019). Workplace design ergonomic validation based on multiple human factors assessment methods and simulation. Production and Manufacturing Research, 7 (1), 195–222.
Ceccacci, S., Matteucci, M., Peruzzini, M., & Mengoni, M. (2019). A multipath methodology to promote ergonomics, safety and efficiency in agile factories. International Journal of Agile Systems and Management, 12 (4), 407–436.
Chan, L.-K., & Wu, M.-L. (2002). Quality function deployment: A literature review. European Journal of Operational Research, 143 (3), 463–497.
Chang, Y. W., & Lee, S. H. (2013). A development of a web-based and user-centered process analysis system for quality improvement. International Journal of Precision Engineering and Manufacturing, 14 (12), 2165–2170.
Chang, Y. W., Lee, S. H., & Cha, H. M. (2017). Development of a customizable web-based process analysis system for continuous process management. Journal of Mechanical Science and Technology, 31 (7), 3481–3487.
Chaudhuri, A., Rogers, H., Soberg, P., & Pawar, K. S. (2019). The role of service providers in 3D printing adoption. Industrial Management and Data Systems, 119 (6), 1189–1205.
Cheah, S. L. Y., Yang, Y., & Saritas, O. (2019). Reinventing product-service systems: The case of Singapore. Foresight, 21 (3), 332–361.
Chen, D., Ding, J., Gao, M., Ma, D., & Liu, D. (2016). Form gene clustering method about pan-ethnic-group products based on emotional semantic. Chinese Journal of Mechanical Engineering (english Edition), 29 (6), 1134–1144.
Chen, R. Y. (2016). Fuzzy dual experience-based design evaluation model for integrating engineering design into customer responses. International Journal on Interactive Design and Manufacturing, 10 (4), 439–458.
Chen, S.S.-C., & Duh, H. (2019). Interface of mixed reality: From the past to the future. CCF Transactions on Pervasive Computing and Interaction, 1 (1), 69–87.
Chen, Y.-M., Miller, R. A., & Sevenler, K. (1995). Knowledge-based manufacturability assessment: An object-oriented approach. Journal of Intelligent Manufacturing, 6 (5), 321–337.
Chinosi, M., & Trombetta, A. (2012). BPMN: An introduction to the standard. Computer Standards & Interfaces, 34 (1), 124–134.
Cimini, C., Pirola, F., Pinto, R., & Cavalieri, S. (2020). A human-in-the-loop manufacturing control architecture for the next generation of production systems. Journal of Manufacturing Systems, 54 , 258–271.
Cohen, Y., Naseraldin, H., Chaudhuri, A., & Pilati, F. (2019). Assembly systems in Industry 4.0 era: A road map to understand Assembly 4.0. International Journal of Advanced Manufacturing Technology, 105 (9), 4037–4054.
Commission, E. (2013). Factories of the future: Multi-annual Roadmap for the contractual PPP under Horizon 2020 . Publications Office of the European Union.
Coronado, E., Venture, G., & Yamanobe, N. (2020). Applying kansei/affective engineering methodologies in the design of social and service robots: A systematic review. International Journal of Social Robotics . https://doi.org/10.1007/s12369-020-00709-x
Article Google Scholar
Coulton, P., & Lindley, J. G. (2019). More-than human centred design: Considering other things. The Design Journal, 22 (4), 463–481.
Crabtree, A., & Mortier, R. (2015). Human data interaction: Historical lessons from social studies and CSCW. In N. Boulus-Rødje, G. Ellingsen, T. Bratteteig, M. Aanestad, & P. Bjørn (Eds.), ECSCW 2015: Proceedings of the 14th European conference on computer supported cooperative work, 19–23 September 2015, Oslo, Norway (pp. 3–21). Springer.
Cruz Salazar, L. A., Ryashentseva, D., Lüder, A., & Vogel-Heuser, B. (2019). Cyber-physical production systems architecture based on multi-agent’s design pattern—Comparison of selected approaches mapping four agent patterns. International Journal of Advanced Manufacturing Technology, 105 (9), 4005–4034.
Dalle Mura, M., & Dini, G. (2019). Optimizing ergonomics in assembly lines: A multi objective genetic algorithm. CIRP Journal of Manufacturing Science and Technology, 27 , 31–45.
Dankl, K. (2017). Design age: Towards a participatory transformation of images of ageing. Design Studies, 48 , 30–42.
Demirel, H. O., & Duffy, V. G. (2013). A sustainable human centered design framework based on human factors. In G. Duffy Vincent (Ed.), Digital human modeling and applications in health, safety, ergonomics, and risk management. Healthcare and safety of the environment and transport . DHM 2013. Lecture Notes in Computer Science (Vol. 8025, pp. 307–315). Springer.
Derigent, W., Cardin, O., & Trentesaux, D. (2020). Industry 4.0: Contributions of holonic manufacturing control architectures and future challenges. Journal of Intelligent Manufacturing . https://doi.org/10.1007/s10845-020-01532-x
Diegel, O., Bright, G., & Potgieter, J. (2004). Bluetooth ubiquitous networks: Seamlessly integrating humans and machines. Assembly Automation, 24 (2), 168–176.
dos Santos, R., Bueno, E. V., Kato, H. T., & Corrêa, R. O. (2018). Design management as dynamic capabilities: A historiographical analysis. European Business Review, 30 (6), 707–719.
Duchowski, A. T. (2002). A breadth-first survey of eye-tracking applications. Behavior Research Methods, Instruments, & Computers, 34 (4), 455–470.
Duque, E., Fonseca, G., Vieira, H., Gontijo, G., & Ishitani, L. (2019). A systematic literature review on user centered design and participatory design with older people. In Proceedings of the 18th Brazilian symposium on human factors in computing systems (pp. 1–11).
Farrell, R. S., & Simpson, T. W. (2003). Product platform design to improve commonality in custom products. Journal of Intelligent Manufacturing, 14 (6), 541–556.
Favi, C., Garziera, R., & Campi, F. (2021). A rule-based system to promote design for manufacturing and assembly in the development of welded structure: Method and tool proposition. Applied Sciences, 11 (5), 2326.
Fernandez-Carames, T. M., & Fraga-Lamas, P. (2018). A review on human-centered IoT-connected smart labels for the industry 4.0. IEEE Access, 6 , 25939–25957.
Forsythe, C., Liao, H., & S., T. M. C., & Cardona-Rivera, R. E. . (2017). Cognitive neuroscience of human systems: Work and everyday life . CRC Press.
Fosch-Villaronga, E., Lutz, C., & Tamò-Larrieux, A. (2020). Gathering expert opinions for social robots’ ethical, legal, and societal concerns: Findings from four international workshops. International Journal of Social Robotics, 12 (2), 441–458.
Frauenberger, C. (2019). Entanglement HCI the next wave? ACM Transactions on Computer-Human Interaction, 27 (1), 1–27.
Fu, Z., Chao, C., Wang, H., & Wang, Y. (2019). Toward the participatory human-centred community an exploration of cyber-physical public design for urban experience. IET Cyber-Physical Systems: Theory and Applications, 4 (3), 209–213.
Gamer, T., Hoernicke, M., Kloepper, B., Bauer, R., & Isaksson, A. J. (2020). The autonomous industrial plant: Future of process engineering, operations and maintenance. Journal of Process Control, 88 , 101–110.
García-Magro, C., & Soriano-Pinar, I. (2019). Design of services in servitized firms: Gamification as an adequate tool. Journal of Business and Industrial Marketing, 35 (3), 575–585.
Gervasi, R., Mastrogiacomo, L., & Franceschini, F. (2020). A conceptual framework to evaluate human–robot collaboration. International Journal of Advanced Manufacturing Technology, 108 (3), 841–865.
Gil, M., Albert, M., Fons, J., & Pelechano, V. (2019). Designing human-in-the-loop autonomous cyber-physical systems. International Journal of Human-Computer Studies, 130 , 21–39.
Golden, B. L., Wasil, E. A., & Harker, P. T. (Eds.). (1989). The analytic hierarchy process . Springer.
Grandi, F., Zanni, L., Peruzzini, M., Pellicciari, M., & Campanella, C. E. (2020). A transdisciplinary digital approach for tractor’s human-centred design. International Journal of Computer Integrated Manufacturing, 33 (4), 377–397.
Grieger, M., & Ludwig, A. (2019). On the move towards customer-centric business models in the automotive industry: A conceptual reference framework of shared automotive service systems. Electronic Markets, 29 (3), 473–500.
Gualtieri, L., Palomba, I., Merati, F. A., Rauch, E., & Vidoni, R. (2020). Design of human-centered collaborative assembly workstations for the improvement of operators’ physical ergonomics and production efficiency: A case study. Sustainability, 12 (9), 3606.
Haber, N., & Fargnoli, M. (2019). Prioritizing customer requirements in a product-service system (PSS) context. TQM Journal, 31 (2), 257–273.
Hammer, F., Egger-Lampl, S., & Möller, S. (2018). Quality-of-user-experience: A position paper. Quality and User Experience, 3 (1), 1–15.
Harwood, T., Garry, T., & Belk, R. (2019). Design fiction diegetic prototyping: A research framework for visualizing service innovations. Journal of Services Marketing, 34 (1), 59–73.
Haslgrubler, M., Gollan, B., & Ferscha, A. (2018). A cognitive assistance framework for supporting human workers in industrial tasks. IT Professional, 20 (5), 48–56.
Heidari, M. R., Heravi, G., & Esmaeeli, A. N. (2020). Integrating life-cycle assessment and life-cycle cost analysis to select sustainable pavement: A probabilistic model using managerial flexibilities. Journal of Cleaner Production, 254 , 120046.
Hoe, S. L. (2019). Digitalization in practice: The fifth discipline advantage. Learning Organization, 27 (1), 54–64.
Hoffmann, S., de Carvalho, A. F. P., Abele, D., Schweitzer, M., Tolmie, P., & Wulf, V. (2019). Cyber-physical systems for knowledge and expertise sharing in manufacturing contexts: Towards a model enabling design. Computer Supported Cooperative Work: CSCW: An International Journal, 28 (3–4), 469–509.
Holeman, I., & Kane, D. (2020). Human-centered design for global health equity. Information Technology for Development, 26 (3), 477–505.
International Organization for Standardization. (2019). Ergonomics of human-system interaction—Part 210: Human-centred design for interactive systems (ISO Standard No. 9241-210:2019) (2nd ed.). https://www.iso.org/standard/77520.html .
Iriarte, I., Hoveskog, M., Justel, D., Val, E., & Halila, F. (2018). Service design visualization tools for supporting servitization in a machine tool manufacturer. Industrial Marketing Management, 71 , 189–202.
Isaksson, O., Eckert, C., Panarotto, M., & Malmqvist, J. (2020). You need to focus to validate. Proceedings of the Design Society: DESIGN Conference, 1 , 31–40.
Järvenpää, E., Siltala, N., Hylli, O., & Lanz, M. (2019). The development of an ontology for describing the capabilities of manufacturing resources. Journal of Intelligent Manufacturing, 30 (2), 959–978.
Jasiulewicz-Kaczmarek, M., Żywica, P., & Gola, A. (2021). Fuzzy set theory driven maintenance sustainability performance assessment model: A multiple criteria approach. Journal of Intelligent Manufacturing, 32 (5), 1497–1515.
Jung, S., Meng, H. Y., & Qin, S. F. (2017). Exploring challenges in developing a smart and effective assistive system for improving the experience of the elderly drivers. Chinese Journal of Mechanical Engineering (english Edition), 30 (5), 1133–1149.
Jwo, J.-S., Lin, C.-S., & Lee, C.-H. (2021). Smart technology–driven aspects for human-in-the-loop smart manufacturing. The International Journal of Advanced Manufacturing Technology, 114 , 1741–1752.
Kaasinen, E., Susanna, A., Väätänen, A., Mäkelä, V., Hakulinen, J., Keskinen, T., Elo, J., Siltanen, S., Rauhala, V., Aaltonen, I., Hella, J., Honkamaa, P., Leppä, M., Niemelä, A., Parviainen, J., Saarinen, S., Turunen, M., Törnqvist, J., Valtonen, J., & Woodward, C. (2018). Mobile service technician 4.0—Knowledge-sharing solutions for industrial field maintenance. Interaction Design and Architecture(s), 38 , 6–27.
Kadir, B. A., Broberg, O., & da Conceição, C. S. (2019). Current research and future perspectives on human factors and ergonomics in Industry 40. Computers & Industrial Engineering, 137 , 106004.
Kambanou, M. L. (2020). Additional uses for life cycle costing in life cycle management. Procedia CIRP, 90 , 718–723.
Katiraee, N., Battini, D., Battaia, O., & Calzavara, M. (2019). Human diversity factors in production system modelling and design: STATE of the art and future researches. IFAC-PapersOnLine, 52 (13), 2544–2549.
Klumpp, M., Hesenius, M., Meyer, O., Ruiner, C., & Gruhn, V. (2019). Production logistics and human-computer interaction—state-of-the-art, challenges and requirements for the future. International Journal of Advanced Manufacturing Technology, 105 (9), 3691–3709.
Knutz, E., Markussen, T., & Christensen, P. R. (2014). The role of fiction in experiments within design, art and architecture—towards a new typology of design fiction. Artifact, 3 (2), 8.
Ko, R. K. L., Lee, S. S. G., & Wah Lee, E. (2009). Business process management (BPM) standards: A survey. Business Process Management Journal, 15 (5), 744–791.
Kong, X. T. R., Luo, H., Huang, G. Q., & Yang, X. (2019). Industrial wearable system: The human-centric empowering technology in Industry 4.0. Journal of Intelligent Manufacturing, 30 (8), 2853–2869.
Kowalska, M., Pazdzior, M., & Krzton-Maziopa, A. (2018). Implementation of QFD method in quality analysis of confectionery products. Journal of Intelligent Manufacturing, 29 (2), 439–447.
Kubler, S., Robert, J., Derigent, W., Voisin, A., & Le Traon, Y. (2016). A state-of the-art survey & testbed of fuzzy AHP (FAHP) applications. Expert Systems with Applications, 65 , 398–422.
Kuhnle, A., Kaiser, J.-P., Theiß, F., Stricker, N., & Lanza, G. (2021). Designing an adaptive production control system using reinforcement learning. Journal of Intelligent Manufacturing, 32 (3), 855–876.
Kulyk, O., Kosara, R., Urquiza, J., & Wassink, I. (2007). Human-centered aspects. In A. Kerren, A. Ebert, & J. Meyer (Eds.), Human-centered visualization environments. Lecture notes in computer science (Vol. 4417, pp. 13–75). Berlin: Springer.
Kymäläinen, T., Kaasinen, E., Hakulinen, J., Heimonen, T., Mannonen, P., Aikala, M., Paunonen, H., Ruotsalainen, J., & Lehtikunnas, L. (2017). A creative prototype illustrating the ambient user experience of an intelligent future factory. Journal of Ambient Intelligence and Smart Environments, 9 (1), 41–57.
Labuttis, J. (2015). Ergonomics as element of process and production optimization. Procedia Manufacturing, 3 , 4168–4172.
Lee, C., & Coughlin, J. F. (2015). PERSPECTIVE: Older adults’ adoption of technology: An integrated approach to identifying determinants and barriers. Journal of Product Innovation Management, 32 (5), 747–759.
Lee, J., & Abuali, M. (2011). Innovative Product Advanced Service Systems (I-PASS): Methodology, tools, and applications for dominant service design. International Journal of Advanced Manufacturing Technology, 52 (9–12), 1161–1173.
Leng, J., & Jiang, P. (2017). Granular computing–based development of service process reference models in social manufacturing contexts. Concurrent Engineering Research and Applications, 25 (2), 95–107.
Leoni, L. (2019). Servitization strategy adoption: Evidence from Italian manufacturing firms. EuroMed Journal of Business, 14 (2), 123–136.
Lepratti, R. (2006). Advanced human-machine system for intelligent manufacturing : Some issues in employing ontologies for natural language processing. Journal of Intelligent Manufacturing, 17 (6), 653–666.
Lin, K. Y. (2018). User experience-based product design for smart production to empower industry 4.0 in the glass recycling circular economy. Computers and Industrial Engineering, 125 , 729–738.
Liu, Y., Eckert, C. M., & Earl, C. (2020). A review of fuzzy AHP methods for decision-making with subjective judgements. Expert Systems with Applications, 161 , 113738.
Lorentzen, L., & Hedvall, P. O. (2018). Bringing human diversity into design processes through empathic modelling. Studies in Health Technology and Informatics, 256 , 128–136.
Ma, X. J., Ding, G. F., Qin, S. F., Li, R., Yan, K. Y., Xiao, S. N., & Yang, G. W. (2017). Transforming multidisciplinary customer requirements to product design specifications. Chinese Journal of Mechanical Engineering (english Edition), 30 (5), 1069–1080.
Marczyk, G. R., DeMatteo, D., & Festinger, D. (2005). Essentials of research design and methodology . John Wiley & Sons.
Martin, K., Legg, S., & Brown, C. (2013). Designing for sustainability: Ergonomics – carpe diem. Ergonomics, 56 (3), 365–388.
Martin, M. V., & Ishii, K. (2002). Design for variety: Developing standardized and modularized product platform architectures. Research in Engineering Design, 13 (4), 213–235.
Mazali, T. (2018). From industry 4.0 to society 4.0, there and back. AI and Society, 33 (3), 405–411.
Mennell, S. (1990). Decivilising processes: Theoretical significance and some lines of research. International Sociology, 5 (2), 205–223.
Monaghan, J., & Just, P. (2000). Social and cultural anthropology: A very short introduction . Oxford University Press.
Mostafazadeh Davani, A., Nazari Shirehjini, A. A., & Daraei, S. (2018). Towards interacting with smarter systems. Journal of Ambient Intelligence and Humanized Computing, 9 (1), 187–209.
Mourtzis, D., Fotia, S., Boli, N., & Pittaro, P. (2018). Product-service system (PSS) complexity metrics within mass customization and Industry 4.0 environment. International Journal of Advanced Manufacturing Technology, 97 (1–4), 91–103.
Nahavandi, S. (2017). Trusted autonomy between humans and robots: Toward human-on-the-loop in robotics and autonomous systems. IEEE Systems, Man, and Cybernetics Magazine, 3 (1), 10–17.
Napoleone, A., Macchi, M., & Pozzetti, A. (2020). A review on the characteristics of cyber-physical systems for the future smart factories. Journal of Manufacturing Systems, 54 , 305–335.
Nemeth, C. P. (2004). Human factors methods for design: Making systems human-centered . CRC Press.
Norman, D. (2016). The design of everyday things . MIT Press.
Oborski, P. (2004). Man-machine interactions in advanced manufacturing systems. The International Journal of Advanced Manufacturing Technology, 23 (3–4), 227–232.
Ong, S. K., Wang, X., & Nee, A. Y. C. (2020). 3D bare-hand interactions enabling ubiquitous interactions with smart objects. Advances in Manufacturing, 8 (2), 133–143.
Oztemel, E., & Gursev, S. (2020). Literature review of Industry 4.0 and related technologies. Journal of Intelligent Manufacturing, 31 (1), 127–182.
Pacaux-Lemoine, M.-P., Trentesaux, D., Zambrano Rey, G., & Millot, P. (2017). Designing intelligent manufacturing systems through human–machine cooperation principles: A human-centered approach. Computers & Industrial Engineering, 111 , 581–595.
Paelke, V., Röcker, C., Koch, N., Flatt, H., & Büttner, S. (2015). User interfaces for cyber-physical systems: Expanding the designer’s toolbox. At-Automatisierungstechnik, 63 (10), 833–843.
Papetti, A., Rossi, M., Menghi, R., & Germani, M. (2020). Human-centered design for improving the workplace in the footwear sector. Procedia CIRP, 91 , 295–300.
Park, H.-S., & Tran, N.-H. (2014). Autonomy for smart manufacturing. Journal of the Korean Society for Precision Engineering, 31 (4), 287–295.
Pereira Pessôa, M. V., & Jauregui Becker, J. M. (2020). Smart design engineering: A literature review of the impact of the 4th industrial revolution on product design and development. Research in Engineering Design, 31 (2), 175–195.
Peruzzini, M., Pellicciari, M., Grandi, F., & Andrisano, A. O. (2019). A multimodal virtual reality set-up for human-centered design of industrial workstations. Dyna (spain), 94 (2), 182–188.
Pezzotta, G., Sassanelli, C., Pirola, F., Sala, R., Rossi, M., Fotia, S., Koutoupes, A., Terzi, S., & Mourtzis, D. (2018). The Product Service System Lean Design Methodology (PSSLDM): Integrating product and service components along the whole PSS lifecycle. Journal of Manufacturing Technology Management, 29 (8), 1270–1295.
Pheasant, S. (1990). Anthropometrics: An introduction . BSI Standards.
Prasad, B. (1998). Review of QFD and related deployment techniques. Journal of Manufacturing Systems, 17 (3), 221–234.
Prinz, F., Schoeffler, M., Lechler, A., & Verl, A. (2019). A novel I4.0-enabled engineering method and its evaluation. International Journal of Advanced Manufacturing Technology, 102 (5–8), 2245–2263.
Qin, S., Van Der Velde, D., Chatzakis, E., McStea, T., & Smith, N. (2016). Exploring barriers and opportunities in adopting crowdsourcing based new product development in manufacturing SMEs. Chinese Journal of Mechanical Engineering (english Edition), 29 (6), 1052–1066.
Ribeiro, L., & Bjorkman, M. (2018). Transitioning from standard automation solutions to cyber-physical production systems: An assessment of critical conceptual and technical challenges. IEEE Systems Journal, 12 (4), 3816–3827.
Richert, A., Müller, S., Schröder, S., & Jeschke, S. (2018). Anthropomorphism in social robotics: Empirical results on human–robot interaction in hybrid production workplaces. AI and Society, 33 (3), 413–424.
Richter, A., Heinrich, P., Stocker, A., & Schwabe, G. (2018). Digital work design: The interplay of human and computer in future work practices as an interdisciplinary (grand) challenge. Business and Information Systems Engineering, 60 (3), 259–264.
Rodriguez, L. J., Peças, P., Carvalho, H., & Orrego, C. E. (2020). A literature review on life cycle tools fostering holistic sustainability assessment: An application in biocomposite materials. Journal of Environmental Management, 262 , 110308.
Rogers, W. P., Kahraman, M. M., Drews, F. A., Powell, K., Haight, J. M., Wang, Y., Baxla, K., & Sobalkar, M. (2019). Automation in the mining industry: Review of technology, systems, human factors, and political risk. Mining, Metallurgy and Exploration, 36 (4), 607–631.
Rossi, E., & Di Nicolantonio, M. (2020). Integrating human-centred design approach into sustainable-oriented 3D printing systems. Human-Intelligent Systems Integration, 2 (1–4), 57–73.
Saha, V., Mani, V., & Goyal, P. (2020). Emerging trends in the literature of value co-creation: A bibliometric analysis. Benchmarking, 27 (3), 981–1002.
Santoni de Sio, F., & van den Hoven, J. (2018). Meaningful human control over autonomous systems: A philosophical account. Frontiers in Robotics and AI, 5 , 15.
Schulze, H., Brau, H., Haasis, S., Weyrich, M., & Rhatje, T. (2005). Human-centered design of engineering applications: Success factors from a case study in the automotive industry. Human Factors and Ergonomics in Manufacturing, 15 (4), 421–443.
Seitz, M., Gehlhoff, F., Cruz Salazar, L. A., Fay, A., & Vogel-Heuser, B. (2021). Automation platform independent multi-agent system for robust networks of production resources in industry 4.0. Journal of Intelligent Manufacturing . https://doi.org/10.1007/s10845-021-01759-2
Shahin, A., Pourhamidi, M., Antony, J., & Hyun Park, S. (2013). Typology of Kano models: A critical review of literature and proposition of a revised model. International Journal of Quality & Reliability Management, 30 (3), 341–358.
Shiizuka, H., & Hashizume, A. (2011). The role of kansei/affective engineering and its expected in aging society. In J. Watada, G. Phillips-Wren, L. C. Jain, & R. J. Howlett (Eds.), Intelligent decision technologies: Smart innovation, systems and technologies (Vol. 10, pp. 329–339). Springer.
Simpson, T. W. (2004). Product platform design and customization: Status and promise. Artificial Intelligence for Engineering Design, Analysis and Manufacturing, 18 (1), 3–20.
Simpson, T. W., Jiao, J., Siddique, Z., & Hölttä-Otto, K. (Eds.). (2014). Advances in product family and product platform design . Springer.
Simpson, T. W., Siddique, Z., & Jiao, J. R. (Eds.). (2006). Product platform and product family design . Springer.
Sinclair, M. A. (1992). Human factors, design for manufacturability and the computer-integrated manufacturing enterprise. In M. Helander & M. Nagamachi (Eds.), Design for manufacturability: A systems approach to concurrent engineering in ergonomics (pp. 127–146). Taylor & Francis.
Soares, M. M., & Rebelo, F. (Eds.). (2016). Ergonomics in design: Methods and techniques . Productivity Press.
Song, J. W., Norman, D., Nam, T. J., & Qin, S. (2016). Wireless device connection problems and design solutions. Chinese Journal of Mechanical Engineering (english Edition), 29 (6), 1145–1155.
Song, W., & Sakao, T. (2016). Service conflict identification and resolution for design of product–service offerings. Computers & Industrial Engineering, 98 , 91–101.
Streitz, N. (2019). Beyond ‘smart-only’ cities: Redefining the ‘smart-everything’ paradigm. Journal of Ambient Intelligence and Humanized Computing, 10 (2), 791–812.
Tetnowski, J. (2015). Qualitative case study research design. Perspectives on Fluency and Fluency Disorders, 25 (1), 39–45.
Theuer, H., Gronau, N., & Lass, S. (2013). The impact of autonomy on lean manufacturing systems. In A. Azevedo (Ed.), Advances in sustainable and competitive manufacturing systems. Lecture notes in mechanical engineering (pp. 1413–1423). Heidelberg: Springer.
Thompson, H., Reimann, M., Ramos-Hernandez, D., Bageritz, S., Brunet, A., Robinson, C., Sautter, B., Linzbach, J., Pfeifer, H., Aravantinos, V., Törngren, M., Asplund, F., Zhang, X., & de Sutter, I. (2018). Platforms4CPS: Key outcomes and recommendations (1st ed.). Steinbeis-Edition.
Tillman, B., Fitts, D. J., Rose-Sundholm, R., & Tillman, P. (2016). Human factors and ergonomics design handbook (3rd ed.). McGraw-Hill Professional.
Tranfield, D., Denyer, D., & Smart, P. (2003). Towards a methodology for developing evidence-informed management knowledge by means of systematic review. British Journal of Management, 14 (3), 207–222.
Turetken, O., Grefen, P., Gilsing, R., & Adali, O. E. (2019). Service-dominant business model design for digital innovation in smart mobility. Business and Information Systems Engineering, 61 (1), 9–29.
Van Acker, B. B., Conradie, P. D., Vlerick, P., & Saldien, J. (2020). Employee acceptability of wearable mental workload monitoring: Exploring effects of framing the goal and context in corporate communication. Cognition, Technology & Work , 0123456789 .
van Lopik, K., Sinclair, M., Sharpe, R., Conway, P., & West, A. (2020). Developing augmented reality capabilities for industry 4.0 small enterprises: Lessons learnt from a content authoring case study. Computers in Industry, 117 , 103208.
Vanderhaegen, F. (2019). Pedagogical learning supports based on human–systems inclusion applied to rail flow control. Cognition, Technology & Work , 0123456789 .
Varshney, K. R., & Alemzadeh, H. (2017). On the safety of machine learning: Cyber-physical systems, decision sciences, and data products. Big Data, 5 (3), 246–255.
Venkatachalam, A. R., Mellichamp, J. M., & Miller, D. M. (1993). A knowledge-based approach to design for manufacturability. Journal of Intelligent Manufacturing, 4 (5), 355–366.
Victorelli, E. Z., Dos Reis, J. C., Hornung, H., & Prado, A. B. (2020a). Understanding human–data interaction: Literature review and recommendations for design. International Journal of Human-Computer Studies, 134 , 13–32.
Victorelli, E. Z., Dos Reis, J. C., Santos, A. A. S., & Schiozer, D. J. (2020b). A design process integrating human-data interaction guidelines and semio-participatory design. In J. Filipe, M. Śmiałek, A. Brodsky, & S. Hammoudi (Eds.), Enterprise information systems (pp. 334–360). Springer.
Victorino, L., Karniouchina, E., & Verma, R. (2009). Exploring the use of the abbreviated technology readiness index for hotel customer segmentation. Cornell Hospitality Quarterly, 50 (3), 342–359.
Voss, C., Tsikriktsis, N., & Frohlich, M. (2002). Case research in operations management. International Journal of Operations & Production Management, 22 (2), 195–219.
Walsh, I., Holton, J. A., Bailyn, L., Fernandez, W., Levina, N., & Glaser, B. (2015). What grounded theory is…A critically reflective conversation among scholars. Organizational Research Methods, 18 (4), 581–599.
Wang, W., Nagai, Y., Fang, Y., & Maekawa, M. (2018). Interactive technology embedded in fashion emotional design: Case study on interactive clothing for couples. International Journal of Clothing Science and Technology, 30 (3), 302–319.
Weichhart, G., Ferscha, A., Mutlu, B., Brillinger, M., Diwold, K., Lindstaedt, S., Schreck, T., & Mayr-Dorn, C. (2019). Human/machine/roboter: Technologies for cognitive processes. Elektrotechnik Und Informationstechnik, 136 (7), 313–317.
Williams, C. (2011). Research methods. Journal of Business & Economics Research (JBER) , 5 (3).
Windt, K., Böse, F., & Philipp, T. (2008). Autonomy in production logistics: Identification, characterisation and application. Robotics and Computer-Integrated Manufacturing, 24 (4), 572–578.
Witschel, D., Döhla, A., Kaiser, M., Voigt, K.-I., & Pfletschinger, T. (2019). Riding on the wave of digitization: Insights how and under what settings dynamic capabilities facilitate digital-driven business model change. Journal of Business Economics, 89 (8–9), 1023–1095.
Wittenberg, C. (2015). Cause the trend industry 4.0 in the automated industry to new requirements on user interfaces? In Kurosu M. (Ed.), Human–computer interaction: Users and contexts. Lecture notes in computer science . HCI 2015 (Vol. 9171, pp. 238–245). Springer.
Wojtynek, M., Steil, J. J., & Wrede, S. (2019). Plug, plan and produce as enabler for easy workcell setup and collaborative robot programming in smart factories. KI - Künstliche Intelligenz, 33 (2), 151–161.
Wu, L., Zhu, Z., Cao, H., & Li, B. (2016). Influence of information overload on operator’s user experience of human–machine interface in LED manufacturing systems. Cognition, Technology and Work, 18 (1), 161–173.
Wu, X., Qiu, T., & Chen, H. (2013). Function combined method for design innovation of children’s bike. Chinese Journal of Mechanical Engineering (english Edition), 26 (2), 242–247.
Yao, X., Zhou, J., Lin, Y., Li, Y., Yu, H., & Liu, Y. (2019). Smart manufacturing based on cyber-physical systems and beyond. Journal of Intelligent Manufacturing, 30 (8), 2805–2817.
Yin, R. K. (2018). Case study research and applications: Design and methods (6th ed.). SAGE Publications Inc.
Zairi, M., & Youssef, M. A. (1995). Quality function deployment. International Journal of Quality & Reliability Management, 12 (6), 9–23.
Zarte, M., Pechmann, A., & Nunes, I. L. (2020). Principles for human-centered system design in industry 4.0—a systematic literature review. In I. L. Nunes (Ed.), Advances in human factors and systems interaction (AHFE) . Advances in intelligent systems and computing (Vol. 1207, pp. 140–147). Springer.
Zhang, H., Qin, S., Li, R., Zou, Y., & Ding, G. (2020). Environment interaction model-driven smart products through-life design framework. International Journal of Computer Integrated Manufacturing, 33 (4), 360–376.
Zhang, L., & Zhang, B. (2010). Fuzzy tolerance quotient spaces and fuzzy subsets. Science China Information Sciences, 53 (4), 704–714.
Zhang, T., Li, Q., Zhang, C., Liang, H., Li, P., Wang, T., Li, S., Zhu, Y., & Wu, C. (2017). Current trends in the development of intelligent unmanned autonomous systems. Frontiers of Information Technology & Electronic Engineering, 18 (1), 68–85.
Zhao, S., Zhang, Q., Peng, Z., & Fan, Y. (2020). Integrating customer requirements into customized product configuration design based on Kano’s model. Journal of Intelligent Manufacturing, 31 (3), 597–613.
Zheng, P., Wang, H., Sang, Z., Zhong, R. Y., Liu, Y., Liu, C., Mubarok, K., Yu, S., & Xu, X. (2018). Smart manufacturing systems for Industry 40: Conceptual framework, scenarios, and future perspectives. Frontiers of Mechanical Engineering, 13 (2), 137–150.
Zhou, Z., Xie, S., & Chen, D. (2012). Fundamentals of digital manufacturing science . Springer.
Zhu, H., Gao, J., & Cai, Q. (2015). A product-service system using requirement analysis and knowledge management technologies. Kybernetes, 44 (5), 823–842.
Download references
This project has received funding from the European Union’s Horizon 2020 research and innovation programme under the Marie Skłodowska-Curie Grant No. 814078.
Author information
Authors and affiliations.
Design Innovation Center (DBZ), Mondragon Unibertsitatea - Faculty of Engineering, Loramendi, 4, 20500, Arrasate-Mondragón, Gipuzkoa, Spain
Hien Nguyen Ngoc, Ganix Lasa & Ion Iriarte
You can also search for this author in PubMed Google Scholar
Contributions
All authors contributed to this study whose the methodology was proposed by GLE, IIA. Material preparation and data collection were performed by NNH. The subsequent analysis was carried out by NNH, GLE, IIA. A first draft of the manuscript was written by NNH and all authors improved previous versions of the manuscript. All authors read and approved the final manuscript.
Corresponding author
Correspondence to Hien Nguyen Ngoc .
Ethics declarations
Conflict of interest.
The authors have no conflicts of interest to declare that are relevant to the content of this article.
Additional information
Publisher's note.
Springer Nature remains neutral with regard to jurisdictional claims in published maps and institutional affiliations.
See Tables 9 , 10 and 11 .
Rights and permissions
Open Access This article is licensed under a Creative Commons Attribution 4.0 International License, which permits use, sharing, adaptation, distribution and reproduction in any medium or format, as long as you give appropriate credit to the original author(s) and the source, provide a link to the Creative Commons licence, and indicate if changes were made. The images or other third party material in this article are included in the article's Creative Commons licence, unless indicated otherwise in a credit line to the material. If material is not included in the article's Creative Commons licence and your intended use is not permitted by statutory regulation or exceeds the permitted use, you will need to obtain permission directly from the copyright holder. To view a copy of this licence, visit http://creativecommons.org/licenses/by/4.0/ .
Reprints and permissions
About this article
Nguyen Ngoc, H., Lasa, G. & Iriarte, I. Human-centred design in industry 4.0: case study review and opportunities for future research. J Intell Manuf 33 , 35–76 (2022). https://doi.org/10.1007/s10845-021-01796-x
Download citation
Received : 01 December 2020
Accepted : 29 May 2021
Published : 11 June 2021
Issue Date : January 2022
DOI : https://doi.org/10.1007/s10845-021-01796-x
Share this article
Anyone you share the following link with will be able to read this content:
Sorry, a shareable link is not currently available for this article.
Provided by the Springer Nature SharedIt content-sharing initiative
- Human-centred design
- Industry 4.0
- Case study review
- Research opportunities
- Find a journal
- Publish with us
- Track your research
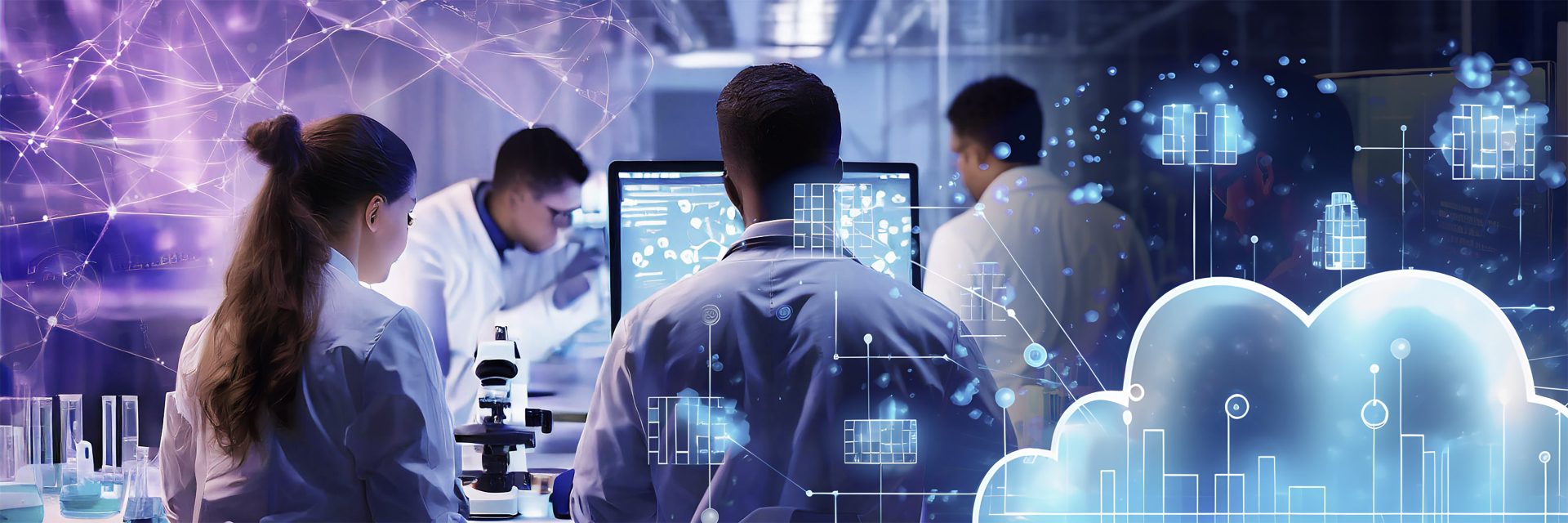
- Share on LinkedIn
- Share via Email
Industry 4.0: Examples and benefits for manufacturing
Home » Insights » Consulting » Industry 4.0: Examples and benefits for manufacturing
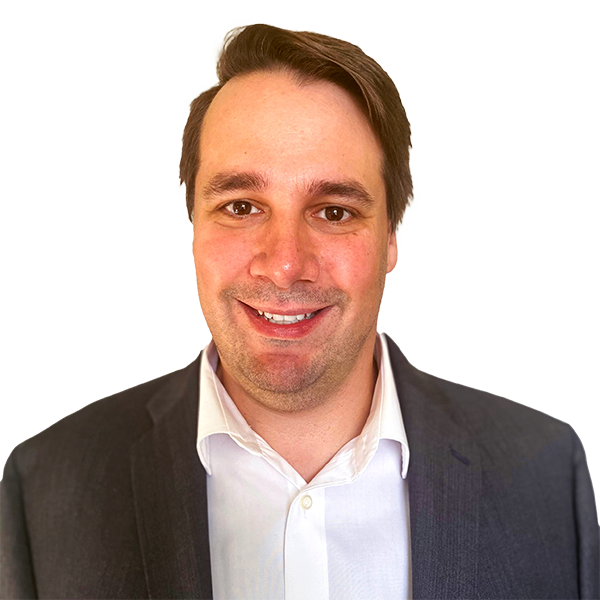
Ryan Thompson
Senior specialist, industry 4.0.
Industry 4.0 may be a buzzword but it’s also a force driving the evolution of the manufacturing industry. It takes advantage of data, connectivity, and potent computational power to digitally transform manufacturing operations. It’s not about any specific technology, like AI or robots, or even a set of technologies like the Internet of Things (IoT). Rather, Industry 4.0 and facility digitalization is the synergy of these technologies working together to produce order-of-magnitude increases in efficiency, reliability, quality, and agility that we expect from an industrial revolution. In a connected facility, data can flow from sensors inside your equipment directly to your ERP systems, triggering real-time action and unleashing new levels of operational productivity and business success.
Manufacturers who have leaned into the benefits of a connected facility have reduced unplanned equipment downtime, successfully navigated worker shortages, reduced expensive errors, and unlocked flexibility in their operations to pivot quickly when consumer preferences change. Manufacturers who drag their feet are exposing their business to costly and growing risks.
Here, we’ll use tangible, detailed Industry 4.0 examples with proven technologies to demonstrate the value it can bring to your manufacturing operations. Skip ahead to examples here.
What is Industry 4.0?
On-demand webinar:
Applying Industry 4.0: Use Cases for Biopharma Efficiency
How does Industry 4.0 differ from digital transformation?
Digital transformation applies to all businesses, not just manufacturing. In fact, the retail and banking sectors are far ahead of manufacturing in terms of digitalization. This is great for manufacturing companies because the technology to drive this change is already available and proven, greatly reducing the risk of adoption. Becoming an Industry 4.0 factory, though, is about more than just technology—we must consider people and processes as well.
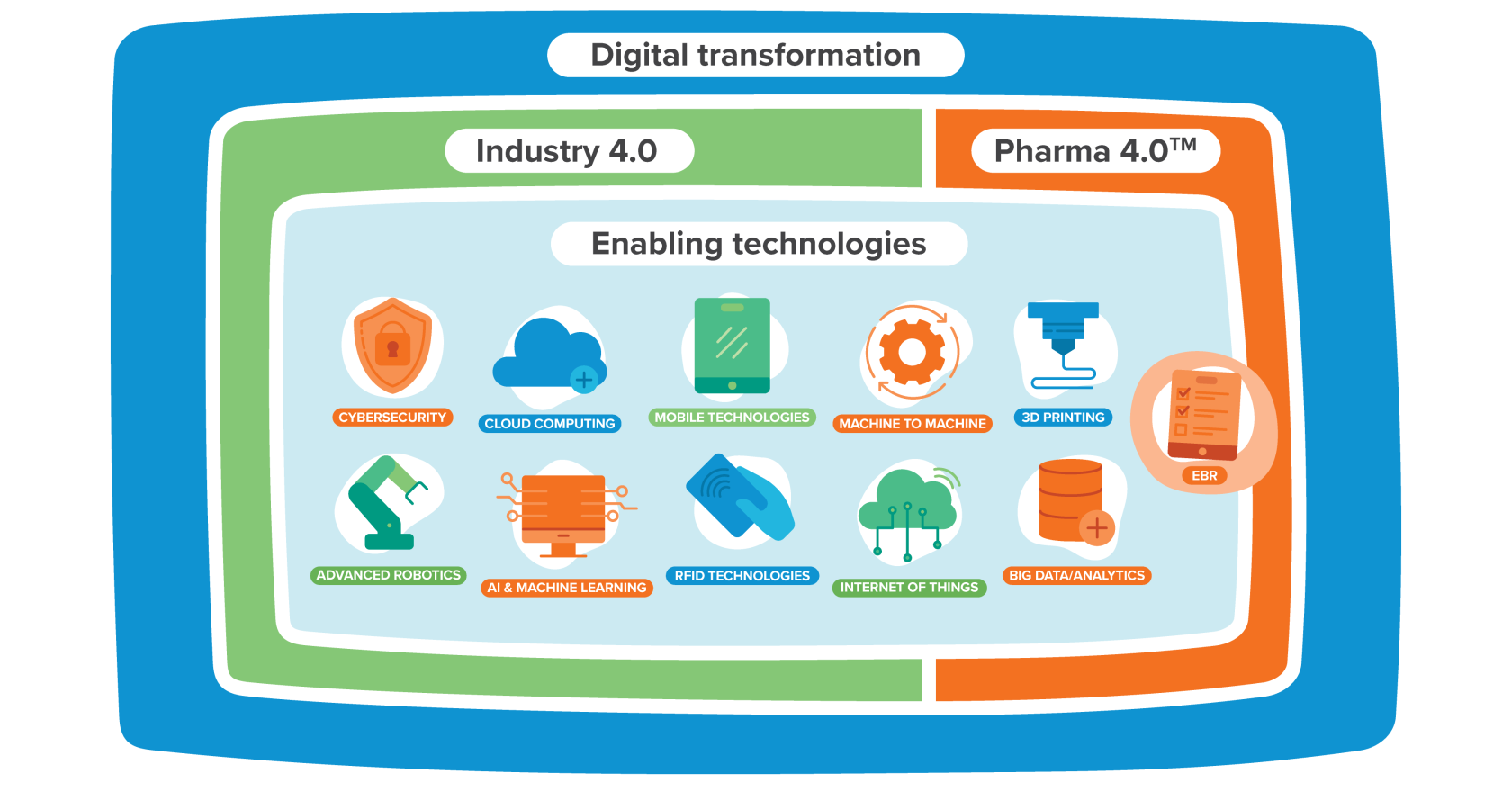
What are some proven Industry 4.0 technologies?
New, expensive technology can be intimidating, and nobody wants their neck on the line for a questionable project that hasn’t been properly tested. This is why many of our pharma and food manufacturing clients ask if there are proven technologies to help optimize their operational technology (OT) on the factory floor. The short answer is yes, and we recommend using proven IT tools to solve OT problems all the time. Take the numerous companies already leveraging Cloud computing platforms. These established technologies power advanced warehouses and banking systems, and are used to seamlessly communicate with both internal and external applications. Not only that, adopting these platforms where a use case demands it gives companies access to tens of millions of skilled people who are certified for developing on these platforms compared to only tens or hundreds of thousands available to develop some niche pieces of software.
Another example of a validated technology ripe for integration is MQTT, which is a machine-to-machine messaging protocol relied on by many IoT sensors, wearables, and devices with resource constraints or limited bandwidth. MQTT was designed to monitor oil pipelines more than 20 years ago and today is being used in ‘smart cities’ to track traffic flow and water use, by Facebook Messenger for its lightweight publishing, and by some manufacturers to connect machines and increase uptime. It’s actively used by billions of people globally. Adopting something like MQTT to develop a Unified Namespace for manufacturing operations can significantly reduce system integration costs as well as provide a company with a single place to know the current state of its business.
The technology itself is not the issue—there are plenty of proven technologies that are primed for manufacturing operations and efficiency.
Manufacturing tends to be slower
Industry 4.0 solutions that integrate IT and OT involve people, processes, and technology. We have to make sure our processes and people are aligned to reap the rewards of technology. Manufacturing needs to develop operating procedures and training programs designed for Industry 4.0 technologies. This is of greater importance for life sciences companies as their procedures are necessarily risk averse. It’s important to think critically and take a risk-based approach.
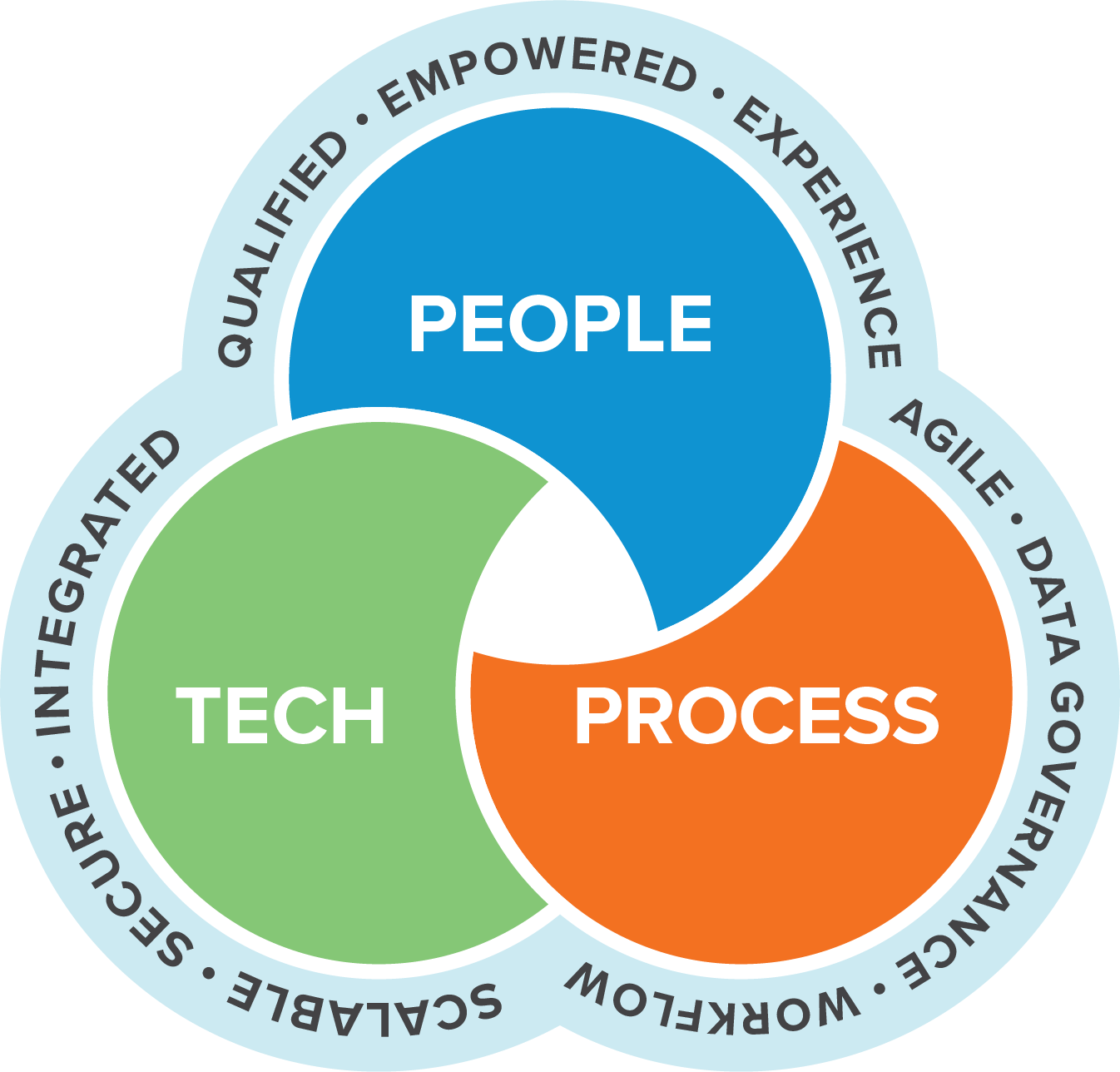
What does a connected manufacturing facility look like?
A connected plant has a high level of automation, as well as integrated and standardized systems. All systems are able to exchange information with all other systems. It ensures all the data in your plant is FAIR—findable, accessible, interoperable, and reusable . To reach this level of maturity requires investment in infrastructure that does not pay dividends by itself. Fortunately, at this point the hard work has been completed and a factory can start taking advantage of this infrastructure to unlock the value found in Industry 4.0.
Industry 4.0 manufacturing examples and their benefits
Generally speaking, we all know what Industry 4.0 is, but how do you implement it and what does it look like in action? Using real-life case studies we will show how to apply Industry 4.0 to your manufacturing operations.
Industry 4.0 example #1: Performance management using Big Data analytics, AI, and machine learning
There are a lot of great metrics to track packaging equipment, especially overall equipment effectiveness (OEE). Measuring OEE is especially important for high-volume manufacturing, like food & beverage or oral solid dose medications for which knowing how your equipment is operating is critical for packaging. A surprising number of companies don’t track this metric.
On the contrary, we don’t really have a lot of good metrics to track manufacturing processes, such as processes within a mixing tank, heating or cooling a recipe, or pumping it through another system for processing. Those metrics aren’t tracked nearly as carefully, and often they are measured as batch cycle time. Tools like computational fluid dynamics can help develop optimized processing times to measure against.
Getting to the Golden Batch
Performance management allows us to use data to measure effectiveness and support how batch tanks operate. Metrics might include the cycle time and temperature profile that lead to the ideal product that is within specifications, the so-called Golden Batch.
But it’s a difficult problem to solve, especially when you don’t have contextualized process data. A tank, for instance, is composed of equipment such as an agitator, pump, temperature transmitter, and heat exchanger. Each of these have subcomponents, such as a setpoint, an actual value, and alarm limits. Without digitally integrated systems, you need to organize data to identify which information is coming from that tank.
A user-defined data type within a unified namespace contextualizes all of the information from the tank and its subcomponents. Now, whenever you retrieve information from your data historian, you know the precise context of the tank that ran a Golden Batch. You have all the field information to know what a future Golden Batch should look like—the specific tank, the recipe it was running, the process value and setpoint for each analog device, and any alarm conditions. Then you can compare it, in real time, to the status of your current batch. What’s different? Is the tank not heating up? Is the agitator too low? If the tank isn’t heating up is that due to a problem with the heat exchanger or the steam supply?
Scaling asset management becomes much easier
Contextualizing your data to enable performance management works especially well for high-volume manufacturing since it improves asset utilization at scale. Not only does it empower the tank operator, it allows a supervisor to follow what’s going on in 10 different tanks simultaneously. Once you’ve taken the time to structure your data like this, you can consider using AI or machine learning to do advanced analytics, supplementing the experience of your operators.
But to do this effectively at scale, you can’t be custom designing each piece of equipment. It’s important to ensure you’ve structured data for multiple pieces of equipment. Then you can plug it in across 10 different tanks or 10 different unit operations.
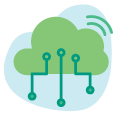
Industry 4.0 example #2: Cloud computing
Accessing Cloud computing and storage offers a short path to value and can be effective for startups with minimal infrastructure on site, such as servers, computers, spare parts, and people to maintain it. Even a large manufacturing company with these resources in its business-wide enterprise data center may choose to operate a manufacturing plant without its own local data center.
Cloud services can be turned on or off as needed
Consider a startup company building a new facility that orders $100,000 worth of computing hardware. While the facility is being built the hardware is delivering no value. Instead, they could consider outsourcing computing resources to a Cloud service provider, eliminating the need for on-site equipment and the staff to maintain it. And this could be turned on only once the facility is up and running. This can also work for a brownfield facility that needs to add a new computer or service. They can turn on a new Cloud instance and have it running almost immediately.
- Reduce costs: Pay only for what you need and avoid maintenance costs.
- Scale quickly as needed: Quickly pivot as project demands change.
- Protect against outages: Engage multiple Cloud providers to prevent downtime.
- Smooth coordination with suppliers: Most suppliers have Cloud-based systems.
A quick example of the benefits of Cloud: 6 months vs. 2 weeks
A CRB client wanted to add an OEE system to tie in equipment performance evaluation. Their IT team estimated it would take six months of work to get servers on site. We used a Cloud solution, turning it on within two weeks instead.
Eliminates on-site storage challenges
Storing data requires servers and the infrastructure to maintain them, including IT maintenance staff and a dedicated air-conditioned server room. We’ve seen many factories where the server sits in a backroom and has four flashing warning lights that nobody ever looks at.
But what happens if your Internet connection goes down? Most companies that rely on the Cloud choose to have duplicate Internet service providers for just this concern. You could also add a 5G cellular network as long as you make sure your data is structured in such a way that, when the 5G network is activated, only the important data gets out, turning off things like video conferencing to save bandwidth.
Security concerns
The International Electrotechnical Commission (IEC) has created IEC 62443 , which are international standards dealing with cybersecurity for automation and control systems. The infrastructure of Cloud service providers tends to be more secure than what’s traditionally provided at any factory. Then it’s a matter of securing connections between the factory and the Cloud provider. This just means installing at least one firewall going into your site.
Industry 4.0 example #3: Electronic batch records
In a paper-driven biopharma facility ( Digital plant maturity model, or DPMM, Level 1 ), a batch record will have thousands of pieces of paper operators have checked, dated, and signed ensuring everything has been executed per the operating procedure. A supervisor reviews all of these records, then again for the batch release. It’s a really time-consuming process.
Read more on Pharma 4.0™ (Industry 4.0 for biotech and pharmaceuticals) here.
How electronic batch records can provide you with an easy Industry 4.0 win.
An electronic batch record (EBR) is a digital version of this. Converting paper record-keeping to inputs on mobile tablets can decrease human errors, reduce administrative work, add visibility to your operations, and quickly provide alerts when a process is running slower than it should.
3 levels to digitizing paper records
While doing a full EBR implementation is time-consuming and expensive, there are incremental steps that deliver a lot of this value quickly.
1. Paper-on-glass transformation
Digitizing paper records is a low-risk, high-reward activity that often yields a quick ROI. A PDF is made to record information that had been recorded on paper. It has the benefit of including timestamps, which allow you to know how much time it takes for an operator to perform each process step. This level doesn’t always include automatic error checking—records still need to be reviewed. It can eliminate entry errors and the time consuming review of legibility issues.
2. Automating workflow reduces costs quickly
Automating the workflow is the next level. It includes PDF files with dropdown menus, real-time error checking, and ensuring steps are executed in the correct order. Workflow management can integrate with your company’s active directory system so you know who’s executing this, and uses electronic signatures.
- Reduction of errors: It eliminates transcription errors and allows automatic error checking. EBRs make manufacturing errors obvious a lot sooner.
- Review by exception: Unlike with paper records, operators and quality staff are notified immediately when a process step is out of specification, such as having an incorrect temperature. Value-added steps could then be stopped, avoiding the cost of having to scrap or rework material. At this level equipment is not directly integrated.
- Cost savings: In our experience, digitizing a single page of a record costs on the order of $100–500, including licensing and the engineering work that ensures error checking. This is easily recouped from the savings you get from quality and workflow improvements.
This automated workflow level provides the quickest and best return on investment (ROI). You’re probably spending 60 percent of the budget on a complete EBR and getting 70–80 percent of the value. Every life sciences manufacturer should be aiming for at least this level.
3. Fully-implemented EBRs are expensive, but needed to scale
To have automatic data collection and record-keeping, without having a human entering any of this information at all, requires connecting directly to the sources of digital information. For example, this could mean having an automation controller that reads the temperature of a step automatically, and enters it into the batch record. Eliminating the human point of contact makes this data collection and recording faster and more accurate.
While fully integrated EBRs require significant investment compared to the first two levels, they allow scaling due to reduced overhead activities requiring operators to write down data for every batch. This won’t matter as much when you’re running a handful of batches each week. But it makes a big difference when the number of batches increases, as it does for personalized medicines that require hundreds of batches weekly. At this rate, the odds of making mistakes also increase. However, this level is not always possible due to equipment limitations. Working with equipment manufacturers to establish data requirements is a critical step in this process.
The ROI for this level can take two to four years, but is a good long-term investment. A personalized medicine facility with an aspiration to supply 15,000 patients per year must have this technology to scale effectively.
How to begin your Industry 4.0 journey
Conduct a digital plant maturity assessment.
A digital maturity assessment gives a snapshot of your facility’s state of digital readiness, compares that to where your peers are, and highlights the major infrastructure investments you need to make. These need to be aligned with your business goals, including revenue, flexibility, sustainability, time to market, or other key performance indicators.
The assessment can be used to choose some small projects to start off, designing standards for equipment or technology you’re procuring to align with your long-term goals. This is an easy place to start and delivers results quickly. You can complete the assessment in as little as four weeks at a cost of roughly $15,000.
Be sure to avoid technical debt.
What is technical debt? Technical debt results from beginning a digitalization project without a big-picture strategy. An example would be a company that has separate projects to put in a new manufacturing line, make changes to the warehouse, and upgrade a quality lab. Yet it’s doing each project in isolation without a long-term strategic plan. The company brought in three different technology sets, some of which align with its goals, some do not. Some have people within its facility who know how to maintain them, others don’t. Technical debt is the cost, time, and related setbacks that ensue due to these short-sighted projects.
Technical debt problems include:
- Rework to match standards because a team proceeded without clarifying standards, or proceeded without knowing its company had standards.
- Needing to hire external consultants because the company doesn’t have anybody who was trained on the technology.
- Investing in technology that will be obsolete in a few years in an ever-changing landscape.
Understanding where you want to go is critical to avoid technical debt on your way there. While you may not know exactly which technologies you will be deploying, it’s still important to lean on your digital roadmap for guidance at the earliest stages of your project.
Identify infrastructure requirements
First, identify what you’ll need to digitally transform the factory floor. A good example is a plant network, which will incur significant capital cost. Once you have this list, choose potential pilot projects to focus on, referring to your digital plant maturity assessment for ideas. You may choose to leverage your strengths or try to improve your weaknesses. An example is a proof of concept on connecting a piece of equipment to a data historian.
Referencing your data strategy and its connectivity standards early will influence your equipment selection process, which is especially helpful for long lead-time items and avoiding costly change orders. Using this information, you can include the appropriate computing and connectivity specs in your proposal requests.
You need to invest in connectivity and data infrastructure early. By investing in Industry 4.0 infrastructure, facilities can adapt and expand with less cost, as well as adopting new technology as it becomes available. For both new builds and retrofits, your most important decisions are those with the longest lifespan that will be difficult, disruptive, and expensive to modify in the future.
This step takes one–three months.
Create a value-led roadmap
This will outline how to get where you want to be—whether tactically in a year or with a strategic five-year plan. You add potential opportunities to a calendar or a Gantt chart in a logical order. This ensures development work and testing is done in the correct order to avoid doing things twice or having one project block another.
Success begets success.
This step provides clarity on the total timeline, estimates investment, and benefits you expect to achieve. Getting some wins builds confidence that your team is getting value from the investments and adds a level of accountability. This can take about one to three months to plan and cost depending on the level of detail and duration of the initiative.
Implement and iterate
Each step can’t be done in a vacuum. The maturity assessment should be reviewed once a year and the roadmap every six to 12 months, depending on the pace of implementation, to make sure you’re achieving what you want to achieve. What progress did you make? What benefits did you gain?
It’s important to know before you start that you won’t get everything right the first time. During proof-of-concept projects it’s particularly important to identify high-risk functions so you can prove or disprove hypotheses, and fail fast.
Navigating the Industry 4.0 revolution
Every vertical, company, and facility is different, and Industry 4.0 will take on a different look depending on where you sit. But one thing rings true despite where you are: there’s a tectonic shift actively changing the manufacturing landscape. The value of Industry 4.0 continues to evolve, with new use cases emerging. AI may entirely reshape the drug discovery process, reducing timelines and the cost of goods and, despite questions about the actual ROI , industry leaders continue to move their vision forward.
It’s important to know where you are currently so you can get where you want to be, unlocking the benefits and developing new use cases that weren’t available before. This is not a technology project. It’s a continuous transformation of a business to ensure your people are empowered and your business processes are aligned to support digital transformations.
Ready for help with your Industry 4.0 initiatives? Our team can help.
Related Content
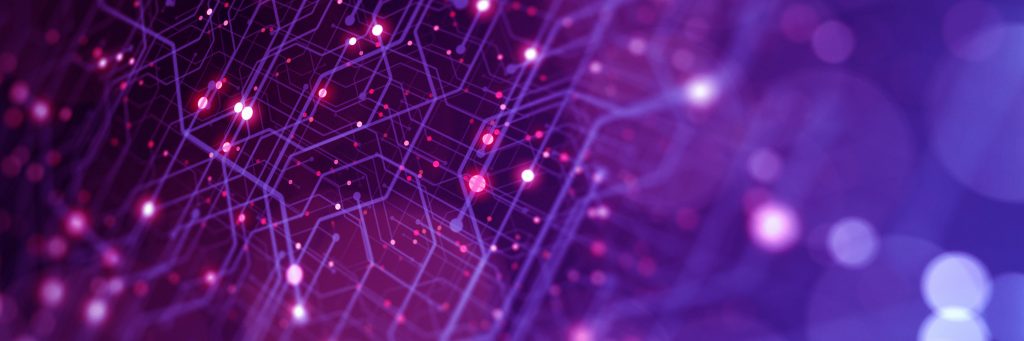
A user-friendly roadmap to Pharma 4.0™ technologies and applications and webinar filled with Pharma 4.0 examples.
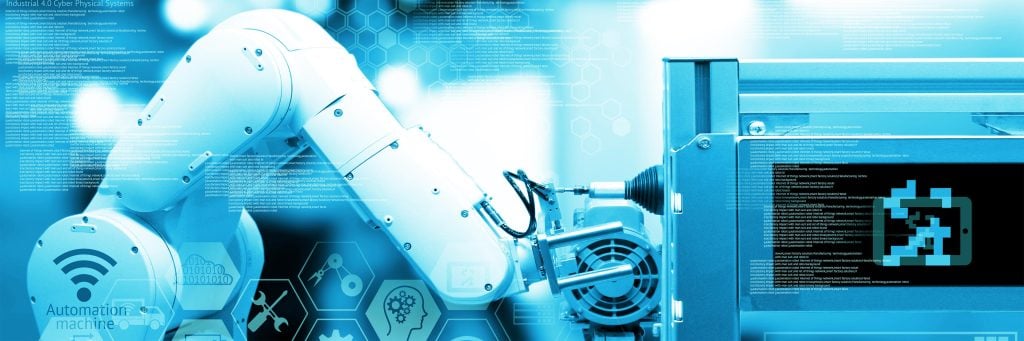
How to leverage Industry 4.0 to reduce manufacturing costs in your pharma and biotech operations.
- Career Opportunities
- Supplier Pre-Qualifications
- Privacy Policy
- Copyright © 2024 CRB • All Rights Reserved.
Industry 4.0 Case Studies (curated)
Curated Set of Case Studies demonstrating problem, solution, and results of implementing Industry 4.0.
- https://www.machinemetrics.com/avalign-case-study requires free login, 10 page pdf article download
- Who – Avalign Technologies medical device company utilized machine metrics for machine and operational data
- Problem – Needed to decrease downtime and increase throughput.
- Solution – deployed measuring, monitoring, dashboards for equipment like grinding, and lathes across 132+ machines at 4 facilities
- Results – 40% increase in OEE, 9.9% increase in throughput, $4.5M capacity utilization increase, 14k hours saved, across 9 months
- Who - BJC healthcare, service provider that operates at multiple hospitals in Missouri and Illinois
- Problem - Inventory tracking is labor intensive and inefficient.
- Solution - RFID tagging to track and manage thousands of medical supplies. Reduced manual labor to maintain inventory.
- Results - Reduce onsite stock by 23% at facilities.
- Who - Bosch Automotive Diesel System factory in Wuxi, China
- Problem - Bottlenecks and production efficiency problems
- Solution - Collect machine monitor sensors' data, combine data sources, and use machine learning analytics to predict equipment failures, thus schedule maintenance before failure.
- Results - Higher uptime durations, contributing to > 10% production output increase in some areas
- Who - Fetch Robotics (California) and DHL distribution center (Netherlands)
- Problem - Improve efficiencies for locating, tracking, and moving inventory in warehouse and logistics facilities
- Solution - Used collaborative Autonomous Mobile Robots (AMRs) to pick and place alongside the workers. AMRs autonomously learning and sharing most efficient travel routes.
- Results - Reduce order cycle time up to 50%, provide up to 2x picking productivity gain.
- Who - Fast Radius Chicago, contract manufacturer with multiple locations.
- Problem - Improve efficiencies for fast turnaround and mass customization of products.
- Solution - Collect data for every part design and identify applications suitable for 3D printing.
- Results - Reduce costs in storing parts through virtual inventory, decrease cycle time.
- Who - Siemens and American pro racing squad Team Penske
- Problem - Speed up the race car development process
- Solution - Create a digital twin, digital representation of a real-world product, machine, process, or system, that allows companies to better understand, analyze and optimize their processes through real-time simulation based on machine learning. Sensors were fitted to a real car and collected data real-time continuously, such as tire pressure, engine control and wind speed, which is then converted into a virtual car model. It’s this model that allows engineers to test different design configurations.
- Results - Reduce testing costs, and time, and ultimately a faster vehicle.
- Who - IBM manufacturing of mainframes, servers, storage, and IT appliances, in Canada, Hungar, mExico and US.
- Problem - Increase efficiencies of visual inspection and product quality. Manual inspections lead to missed defects. Traditional automated inspections also provide too many false positive results.
- Solution - Used IBM Maximo Visual Inspection, an AI-powered computer vision solution to automatically detect quality defects in manufacturing. System was trained using deep learning.
- Results - Up to 5x efficiency gains, 20% reduction in false positives.
- Explained in 7 minute video and implementation of plex.
- Who - Summer Garden Food Manufacturing
- Problem - Not enough productivity and low OEE, no visibility.
- Who - Best Maid food product provider
- Problem - No ERP system - legacy database and excel spreadsheets. Difficulty managing inventory (5 manual audit days/month), Production planning (schedule written day before), challenging for Safe Quality Food Certification.
- Solution - Plex implementation - training, implementation, within 8 months.
- Inventory Management - reduction in raw material inventory of $0.5M, eliminate $50k mislabeled items, eliminate lost 1% due to spoilage.
- Streamline Order to Cash - deliver within 3 days window to avoid penalties (was 1% of orders delayed w/penalties and 50% of Best Maid's revenue)
- Financial management - financial close process now 25% of previously required - was 4 months behind, now closes within 4 weeks of month end.
- Areas with videos, BBC mostly, Logistics, Construction, Public Transport, Manufacturing, Food production, Fieldwork
Please provide the following information to access the course, or click here to learn more about the Industry 4.0 Academy
IEEE Account
- Change Username/Password
- Update Address
Purchase Details
- Payment Options
- Order History
- View Purchased Documents
Profile Information
- Communications Preferences
- Profession and Education
- Technical Interests
- US & Canada: +1 800 678 4333
- Worldwide: +1 732 981 0060
- Contact & Support
- About IEEE Xplore
- Accessibility
- Terms of Use
- Nondiscrimination Policy
- Privacy & Opting Out of Cookies
A not-for-profit organization, IEEE is the world's largest technical professional organization dedicated to advancing technology for the benefit of humanity. © Copyright 2024 IEEE - All rights reserved. Use of this web site signifies your agreement to the terms and conditions.
- Documentation
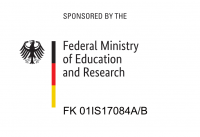
The Smart Factory and the Internet of Things – An Industry 4.0 Case Study
- By Uwe Glockmann
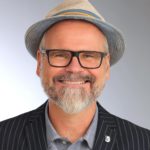
The smart factory, with its connection of many third-party systems to the central business process, has evolved in recent years into a data hub close to the plant. (Image: public domain/ Pixabay)
What is Industry 4.0 actually (for us)?
The term Industry 4.0 was first introduced to the public in 2011, exactly 10 years ago at the Hannover Messe. A working group ultimately gave rise to the Industrie 4.0 platform, an alliance of the industry associations Bitkom , VDMA , and ZVEI . Since then, the Industry 4.0 platform has been further expanded and the goal is to further develop the content associated with the term Industry 4.0 in dialog with unions, trade associations, companies, science, and politics.
Industry 4.0 – making relevant information available
For us, Industry 4.0 is a new level of organization and control of the entire value chain over the life cycle of products – from the idea to recycling. Individual customer requirements arise in discrete manufacturing and a study by International Data Corporation (IDC) from 2019 shows that 42% of Industrial IoT projects in the German industry are implemented precisely where a high degree of product customization (up to batch size 1) is required in industrial production.
The basis is the availability of all relevant information in real-time through the networking of all the instances involved. The challenge here is the ability to derive the optimal value creation flow from the data – at any time. The connectivity of people, objects, and systems results in value-adding networks. They are dynamic, real-time optimized, self-organizing, and cross-company.
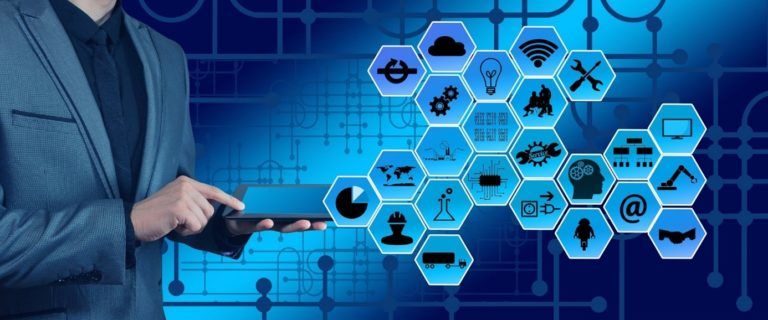
A crucial task here is to be able to produce meaningful information from a multitude of data. ( Image: public domain / Pixabay)
What are the major challenges in the Industrial Internet of Things (IIoT)?
In contrast to the Internet of Things (IoT), the Industrial IoT does not focus on consumers and users, but on industrial processes and workflows. The goals of Industrial IoT are to improve operational efficiency, reduce costs in production, speed up processes and realize new business models.
Back in Rittal’s new digital factory. Driverless transport systems approach me, drive past me under sensor control and automatically take over the transports in the factory. I feel like an extra from a Star Wars movie. I start talking to an engineer from process automation. He is totally enthusiastic, somehow also proud to be working on this 24,000 square meters digital stage.
Formerly analog, now digital
“Previously, we had a large number of isolated solutions of systems, machines, and plants. We lacked transparency about orders, processes, and production status due to a lack of data availability. And then an incredible amount of paper, paper-based process steps, and inevitable media disruptions,” the engineer said. “There was a lot of ad hoc planning and communication instead of structured planning. And lastly, it’s about more precise control of how much electrical power, water, and other resources are being used, when machines are running, and how much they’re producing.”
Connectivity – connecting people and machines
I agree with him, and where the individual manufacturing processes of cutting, edging, welding and painting used to be carried out transactionally, sequentially, and independently of one another, in the smart factory, all people and machines are increasingly integrated into the manufacturing execution system (MES). The individual assemblies are automatically brought together at the end to form the finished product. In the process, the machines and handling systems, the automated guided vehicles communicate with each other and with the higher-level process control systems. In this way, the machinery in the production process automatically adjusts to the workpieces and assemblies to be produced.
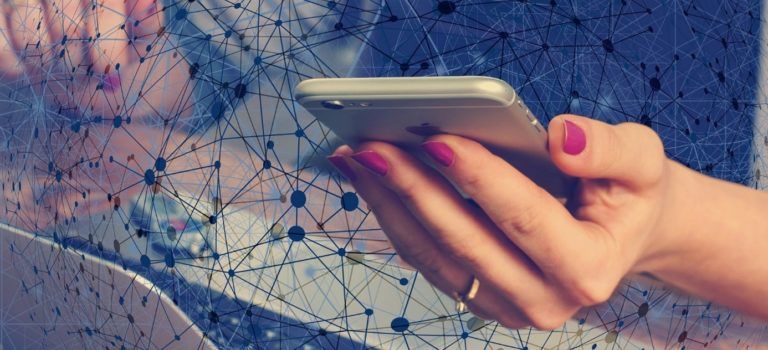
People, objects, and systems are constantly exchanging information. ( Image: public domain / Pixabay)
5G, Edge Computing and AI/ML – new technologies in Industry 4.0
I meet a young man from IT. He tells me a lot about Big Data and new technologies that will be used in the future. The entire machine park constantly supplies data about the control system and the current operating states. In the future, this data will be collected in an edge data center at the plant and analyzed with applications from a sister company. This will form the basis for industrial analytics. With the help of knowledge-based systems based on artificial intelligence (AI) that continuously learn – machine learning (ML) – downtimes are reduced, maintenance is planned in advance (predictive maintenance), and disruptions to the sophisticated production process are minimized.
According to IDC estimates, these new technologies such as 5G, edge computing, and AI/ML should be used and deployed to lay the foundation for new business models and services in the long term, which can either be provided independently or integrated into ecosystems. Developing these ecosystems, establishing corresponding partnerships, and determining generally accepted standards should and will be one of the central tasks for industrial and industry-related companies in Germany in the coming years in order to remain competitive.
5G – data processing at 10 gigabits per second
In the meantime, the control cabinet specialist Rittal has become one of the first industrial companies in Germany to receive a 5G frequency allocation. A private 5G mobile network was already installed at the new plant in Haiger in 2020. The first pilot projects, for example in production monitoring and analysis, are already being planned.
Rittal wants to install and commission the 5G mobile network in its Smart Factory in Haiger in a real production environment as quickly as possible. The new 5G technology is expected to significantly accelerate and simplify data traffic – for example, in the video-based comparison of unit numbers with stored order data and in the step-by-step implementation of data analytics for preventive maintenance. With the new 5G mobile communications standard, data can be processed at a speed of 10 gigabits per second. 5G is regarded as the technology of the future that will enable industrial manufacturing processes to be networked and controlled even better – and thus further exploit the potential of Industry 4.0 .
Edge Computing in the industrial IoT
Current cloud computing trends present edge computing as a concept that can revolutionize industrial operations. This is due to its ability to enable unconnected devices, manufacturing tools, IIoT devices, and workstations to collect and process data without having to work with a central database system. With Industry 4.0 and the millions of sensors and IIoT devices powering it, edge computing for industrial IoT will reach new heights.
Edge computing can be defined as providing data handling activities and operations at the source without having to go through centralized network segments. This computing process optimizes (IoT) devices and applications by bringing computing closer to the network edge of these data-producing devices. Depending on the device or system, the network edge may refer to the area where the device communicates with the Internet.
This is also the case at Rittal. In the future, data generated and required on sensors, components, machines or robots can be collected and evaluated faster than before in an edge or cloud data center. The necessary IT requirements are already in place at the new plant in Haiger.
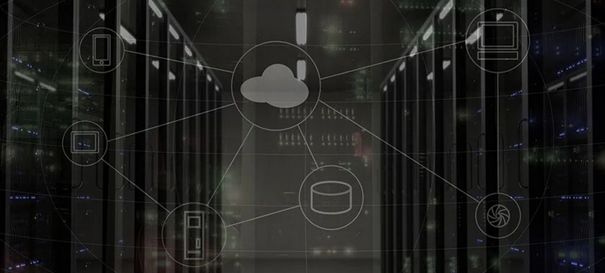
New technologies such as 5G, edge computing, and AI/ML are on the rise. ( Image: public domain / Pixabay)
What is the difference between artificial intelligence (AI) and machine learning (ML)?
In Rittal’s Smart Factory, data is generated by over 100 machines. That’s up to 18 terabytes a day. The data can only be evaluated if it is analyzed by artificial intelligence (AI) after it has been collected. And humans play a decisive role in this: Only with humans as systematic trainers do the plants become learning systems – machine learning (ML) – and later intelligent systems.
Computer programs that played checkers
They are one of the earliest examples of Artificial Intelligence. At the Dartmouth Conference in the summer of 1956, it was the idea of AI pioneers to construct a complex machine that would have the same intelligence as a human with the help of emerging computers. This concept is referred to as the “General Artificial Intelligence (AI)”: Machines that have all our senses, our reason, and think like us .
What can already be implemented today is a concept of “limited AI”. Technologies that are able to solve specific tasks as well or better than humans. An example of this is Facebook’s facial recognition or Pinterest’s image classification. The technologies show aspects of human intelligence. But how does it work and where does the intelligence come from? This is where machine learning (ML) comes into play.
“Machine learning” refers to a cross-cutting discipline of statistics, computer science, and mathematics that aims to construct software systems that can automatically learn from data. A variety of generic learning algorithms have been developed to solve a variety of learning tasks or to gain new insights from data. Machines thus learn complex relationships that humans are no longer capable of in terms of speed and accuracy.
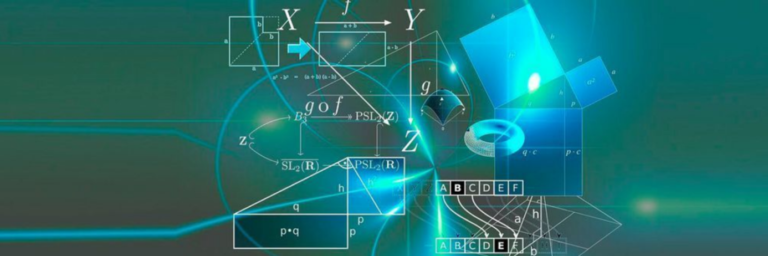
Machine learning combines methods from statistics, computer science, and mathematics with the aim of constructing software systems that can learn automatically from data. (Image: Pixabay / CC0 )
To make the growing flood of data in industrial production manageable, machine learning helps to decipher complex relationships from an immense amount of sensor and process data, to recognize patterns and to generate new knowledge.
Source Code or rather Low-Code / No-Code?
My factory tour of the Smart Factory at Rittal is nearing its end. Almost at the exit, I look over the shoulder of a technician who is making some programming adjustments to the source code on a process computer. What used to be a familiar concept to IT professionals is now indispensable in the field of software and application development. From the automotive industry to pharmaceutical companies – from medium-sized companies to global players. They all use it and the application possibilities are almost limitless.
We are talking about Low-Code / No-Code
Digital processes and applications have to be faster, more powerful, and more (cost)-effective. The premise of digitalization applies to all companies because, in times of rising requirement profiles, an increasing shortage of skilled workers and overburdened IT teams, a Low-Code or No-Code automation platform is often an effective means of saving development time, reducing costs, and always keeping one’s own applications up to date . For IT and business departments, in particular, this offers the opportunity to meet the high demand for applications and other activities.
Where is it going now, the future journey for Low-Code / No-Code?
IT analyst Gartner predicts that the creation of low-code applications that include No-Code will account for more than 65% of all app development functions by 2024. By 2024, 75% of all large corporations will use at least four low-code development tools for activities in both IT application development and Citizen Development. Forrester Research predicts that Low-Code’s market share in business applications will multiply from the current eleven percent to more than 30 percent within the next few years. In a white paper on the subject of low-code, IDC sees greatly shortened development cycles and advantages through productivity increases of up to 25 percent.
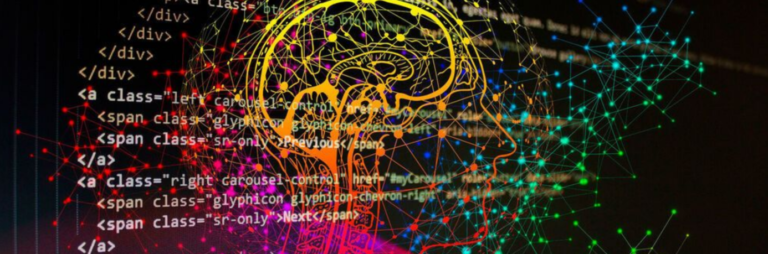
The sometimes tedious coding of individual programming lines is a thing of the past with the modular low-code approach. (Image: public domain / Pixabay )
Individual software solutions are in demand
In just a few years, low-code and no-code development technology has become the hallmark of development efficiency and a clear competitive advantage for companies. Instead of the tedious coding of individual programming lines, low-code works with the help of a modular principle. Prefabricated building blocks replace text-based coding. This saves companies a lot of time and money – and relieves the tight resources of in-house IT teams. The modular building block principle of low-code development uses application designers, graphical methods, and libraries to create individual software solutions and business process applications. In this way, new functions with increasingly complex requirement profiles can be implemented in a short time.
COVID-19 and Industry 4.0
Today, I still often think back to this impressive Industrial IoT experience in Rittal’s Smart Factory. Rittal is a prime example of Industry 4.0 in action, and even today in COVID-19 times, the Industrial Internet of Things is more than present. Currently, many companies see key advantages in the Industrial IoT to overcome short-term challenges. According to a recent IDC study, 59 percent of respondents are planning new IIoT projects. COVID-19 is certainly slowing down some companies at the moment, but for others, it is the moment when their investments in new technologies and especially in Industrial IoT pay off. As the results of the study show, the crisis is also a wake-up call for many to carry out long-needed modernization.
Because one thing is clear. The importance of Industry 4.0 for Germany cannot be overestimated. Industry 4.0 is the prerequisite for maintaining the competitiveness of the German industry and thus securing jobs.
The opportunities are huge, and nothing better can happen to Germany, a location that is poor in resources and rich in know-how, than digitalization in the industry sector.
https://www.rittal.com/ch-de/content/de/unternehmen/presse/pressemeldungen/pressemeldung_detail_57152.jsp
https://en.wikipedia.org/wiki/Fourth_Industrial_Revolution
https://www.rittal.com/at-de/content/de/unternehmen/presse/pressemeldungen/pressemeldung_detail_96576.jsp
https://www.bitkom.org/Themen/Digitale-Transformation-Branchen/Industrie-40/Was-ist-Industrie-40-2.html
https://automationspraxis.industrie.de/industrie-4-0/rittal-smart-factory-fertigt-schaltschraenke/
https://automationspraxis.industrie.de/news/rittal-pionier-beim-5g-mobilfunk-in-der-produktion/
https://www.industry-of-things.de/iot-basics-machine-learning-in-der-smart-factory-a-698047/
https://www.twt.de/news/detail/wo-liegt-der-unterschied-zwischen-artificial-intelligence-machine-learning-und-deep-learning.html
https://www.industry-of-things.de/low-code-ist-integrativ-automatisiert-und-smart-a-960267/
https://www.mendix.com/de/resources/gartner-magic-quadrant-for-low-code-application-platforms/
https://www.idc.com/getdoc.jsp?containerId=prEUR147017420
https://www.bitkom.org/Themen/Digitale-Transformation-Branchen/Industrie-40/Vision-Industrie-40-2.html
- Technology Consulting
- AI Development
- Data Engineering
- Software Engineering
- Data Science
- ML Development
- Manufacturing
- Digital Marketing
- Renewable Energy
- Success Stories
- White Papers
- Company Overview
Manufacturing 4.0: Explanation & Real-World Examples
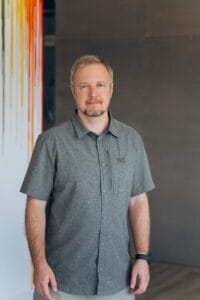
If you step inside any factory floor today, you’re unlikely to see any similarity with the leading factory of ten or even five years ago. Today’s factories are equipped with IoT devices and integrated with emerging technologies, including cloud computing, AI, and ML.
This is how industry 4.0 is paving its way for the manufacturing industry, enabling factories to collect, analyze, and make decisions based on immense stockpiles of data. But what’s the value for business owners? Increased automation, a new level of process efficiencies, and improved quality at lower costs, to name a few.
In this post, we tell about the biggest shift that hit global manufacturing since automation — the phenomenon of manufacturing 4.0, technologies, its characteristics, effects, and real-life examples of industry 4.0.
What is industry 4.0 in manufacturing?
Industry 4.0, also known as manufacturing 4.0 , mixes the latest manufacturing technologies with IT, such as IoT, big data and analytics , artificial intelligence (AI), and machine learning (ML), in order to improve business processes and allow for better decision-making.
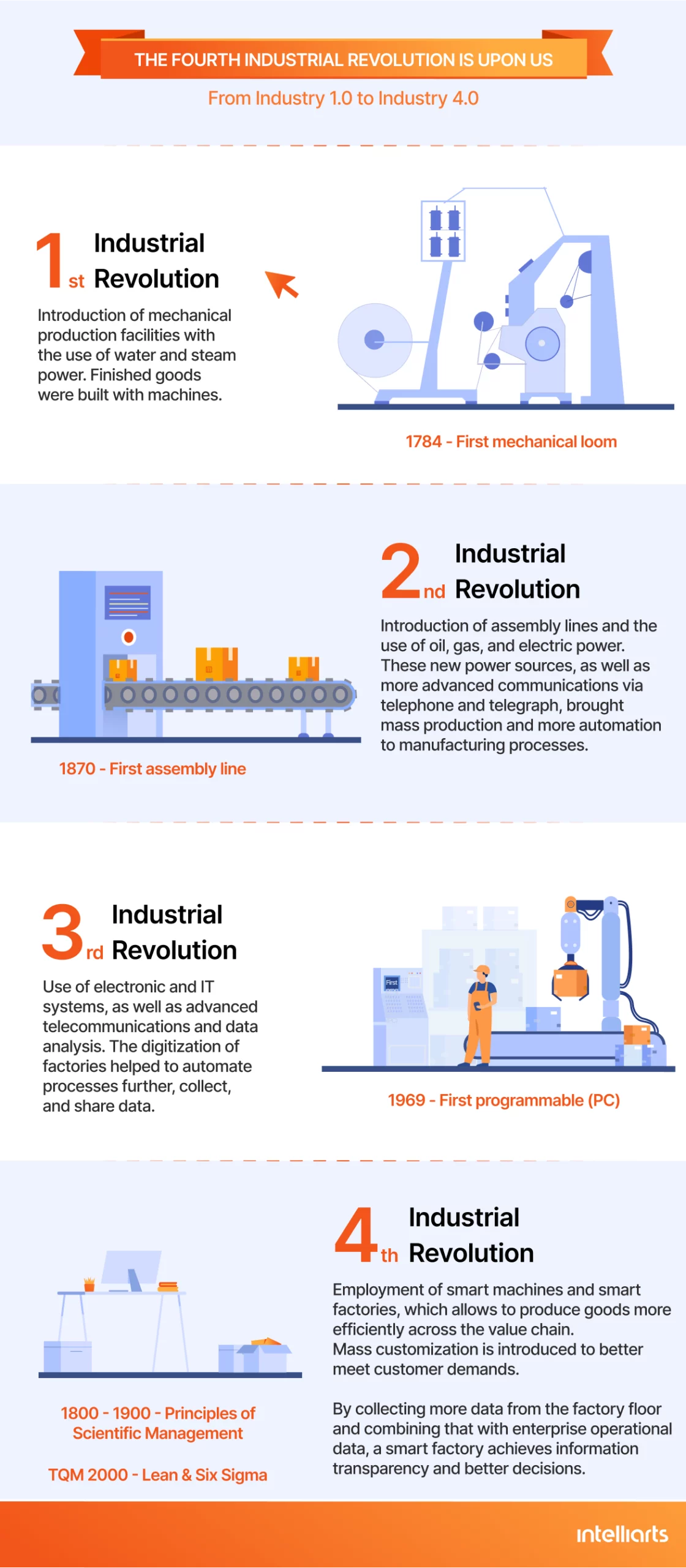
Characteristics of industry 4.0 in manufacturing
The definition of industry 4.0 is rather vague, and it’s often used interchangeably with other notions, including manufacturing 4.0, smart manufacturing, and a smart factory . It’s easier for us to define its main features to be sure you understand it right.
So, industry 4.0 in manufacturing is usually characterized by:
The bridging of the physical and digital world through the use of cyber-physical systems (CPS). CPS refer to intelligent physical components, objects, and software systems with embedded computing and storage capabilities. These systems form the basis of industry 4.0 and are enabled by Industrial IoT (IIoT).
Enhanced decision-making thanks to embedded sensors and interconnected machinery. IoT and IIoT produce lots of big data, which manufacturers can then analyze to explore historical trends, identify patterns, and use in production decision-making. A company can even build a complete virtual representation of the industrial processes and create a so-called digital twin .
Interconnectivity achieved by sensors, devices, and machines fully integrated into manufacturing. A smart factory means integrated operation technology with IT and, thus, smooth cooperation between the two departments.
Real-time monitoring , enabled by the use of IoT and IIoT again. This ensures a timely response to any changes on the factory floor and minimizes the waste of resources.
Decentralized manufacturing as CPS are able to decide on their own and help to perform tasks autonomously. Manufacturing 4.0 doesn’t rely on central computers or pass the decision hierarchically. Instead, it provides more flexibility to local operators and allows them to respond to changes in place.
Improved information transparency due to collected data from sensors. This provides a better understanding of the manufacturing processes and, thus, contributes to data-driven decisions.
Product customization that meets individual customer needs, yet remains cost-effective. With advanced technologies such as 3D printing, smart factories stand aside from a one-fits-all model. Instead, they could easily create more diversified goods for customers.
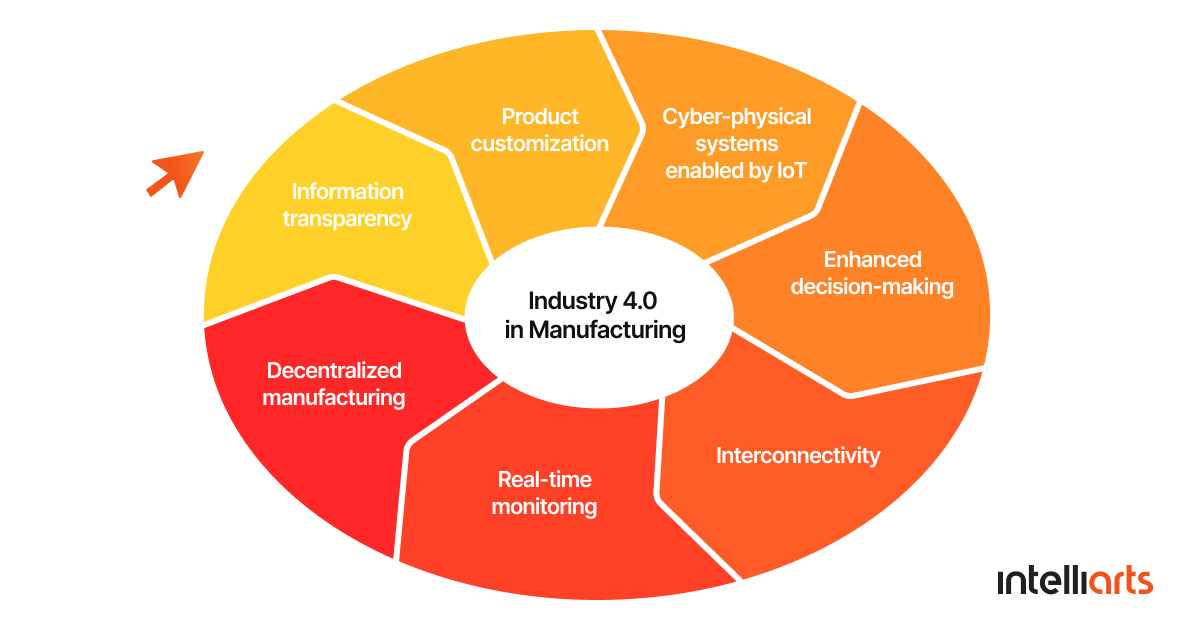
Every business is still unique, and even the most prominent smart manufacturing examples may have not all the characteristics described.
What technologies are driving Industry 4.0?
Let’s also take a closer look at the most important technologies that make a difference in manufacturing 4.0. Together, the five technologies from the list form a high-tech ecosystem on the factory floor and help to increase process efficiencies.
1. IoT and IIoT
IoT/ IIoT sensors are probably the most critical component of smart factories. These connect machines be
tween themselves and to the networks and provide monitoring of equipment, its components, processes, and products.
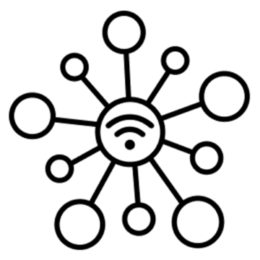
IoT/IIoT sensors are probably the most critical component of smart factories. These connect machines between themselves and to the networks and provide monitoring of equipment, its components, processes, and products.
The technology makes it possible to capture large amounts of data in real-time related to the manufacturing process. Later, this data can be analyzed to understand the behavior of the industrial environment to streamline business processes and optimize supply and demand.
2. AI and ML
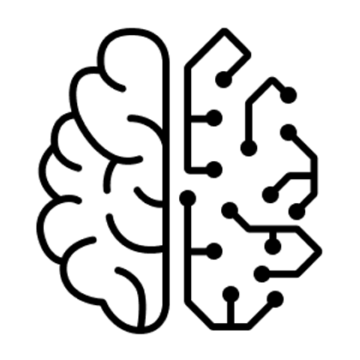
Although valuable, the data received from IoT/IIoT devices is too vast for people to analyze manually. Fortunately, AI and ML algorithms are skillful enough to contextualize this info, find anomalies, and make recommendations based on this.
The most explicit smart factory example is the use of ML in predictive maintenance in manufacturing in order to detect early warning signs of equipment breakage or degradation. An ML-powered model can track and warn about temperature anomalies on the factory floor (or any other deviations). The manufacturer will then interfere timely before the real issue occurs.
3. Cloud computing
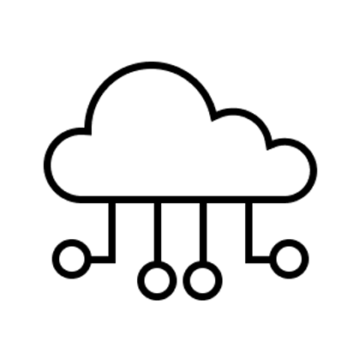
Smart manufacturing is impossible without cloud computing, which provides connectivity and integration of machines, equipment, and components. Another advantage of the cloud includes infrastructure, storage, platforms, and software applications. Cloud providers deliver them to store and manage the data received from IoT. Still, businesses don’t need to buy all this at once. They can scale their operations gradually, paying only for those resources and services they’re using.
4. Edge computing
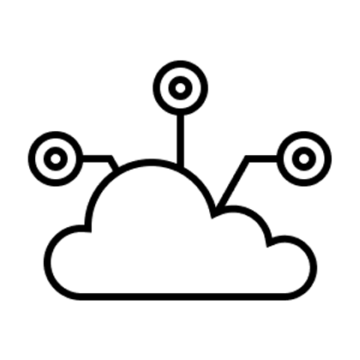
Some issues, such as those related to safety or quality, need to be resolved immediately. So, data analysis should take place at the “edge” — where the data is created. This is possible with the use of edge computing which aims to bring computation and storage closer to the source of data.
While edge computing minimizes security risks, the manufacturer also saves itself from too much dependence on a reliable network.
5. Digital twin
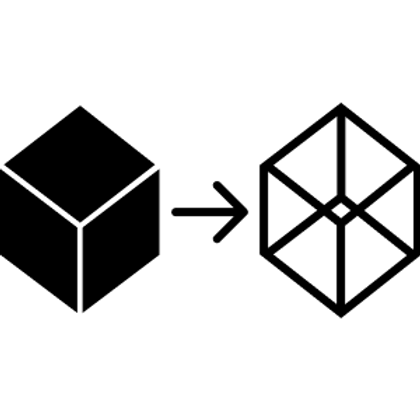
Digital transformation provided by manufacturing 4.0 also gives way to digital twins — digital replicas of processes, production lines, or even the entire plant. This technology helps to improve performance and workflows on the factory floor, yet also test changes in a cost-effective manner. For instance, a manufacturer can test a new product design without actual investment in production.
What effect will industry 4.0 have on manufacturing?
Industry 4.0 can deliver irresistible returns to manufacturers if implemented and maintained properly. Connected, smart manufacturing, enhanced by a fully automated production process, is a major return on investment.
Still, not every manufacturer is ready to venture into the upfront investment needed for launching a pilot smart factory project. If you need a couple of more reasons why a smart factory is worth investing in, here are the meaningful returns:
1. Increase in productivity
Enhanced productivity is the primary advantage of industry 4.0 in manufacturing. It’s also the major goal of smart factory projects. Manufacturers want to get rid of repetitive and tiresome tasks and use smart machines for this type of work. Meanwhile, human operators spend their time monitoring and maintaining systems only.
Altogether, this means faster, more efficient production around the clock and an increase in the production rate.
2. Quality improvements
While performance is the first and most important for manufacturers, product quality comes second. Fortunately, the 4.0 era has all chances to help you deliver better quality products. Processes get more automated while less human engagement means fewer errors. Besides, real-time monitoring, smart sensors, and AI/ML algorithms allow manufacturers to detect any quality issues and react to them quickly.
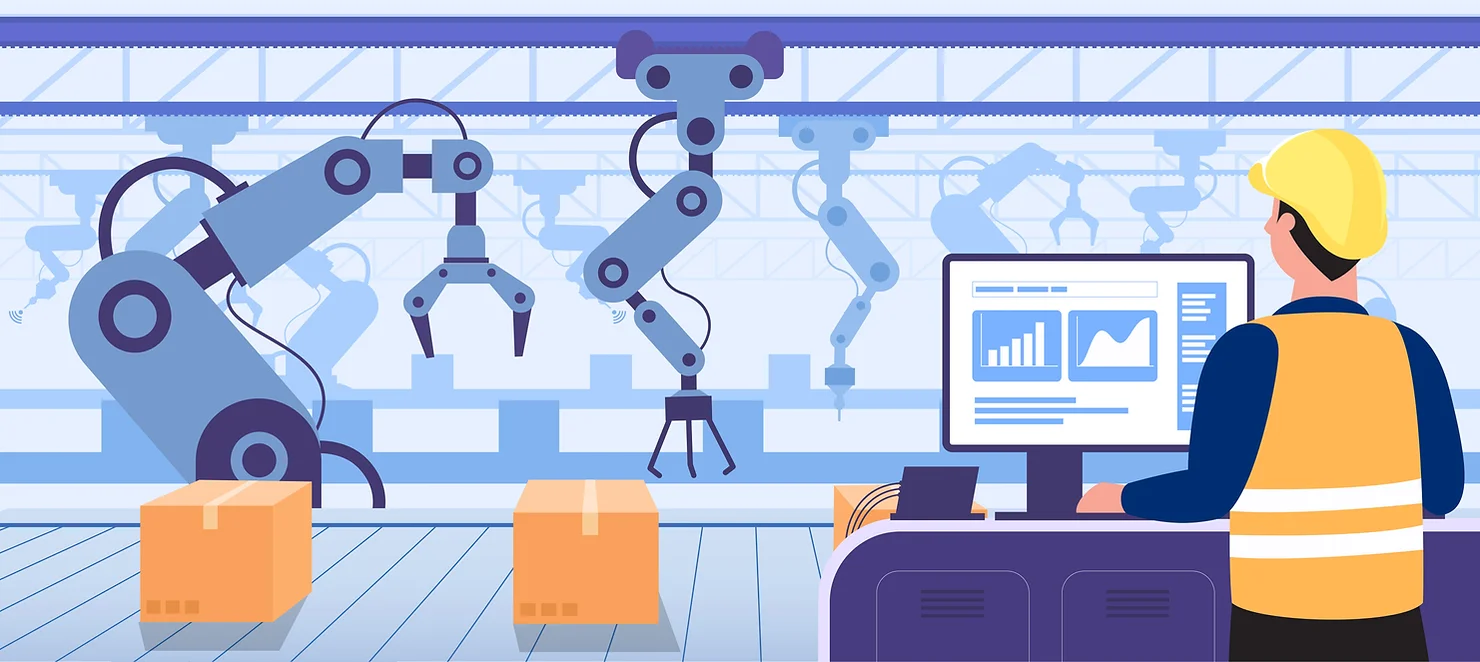
3. Enhanced customer experience
The improvements in product quality logically lead to a better customer experience. Moreover, the use of smart machines and automation technologies means that the manufacturer can produce goods faster and in more quantity. This increases the satisfaction level of customers, as they no longer face issues when the product is unavailable in the market.
Finally, manufacturing 4.0 contributes to products becoming more customized. Thanks to innovative technologies, a smart factory can mass-produce lines of personalized products. It can experiment with more designs and test them via digital twins, shorten routes between production and delivery, co-create, etc.
4. Cost-effectiveness
Another benefit of industry 4.0 that we can’t ignore is its cost-efficiency. Manufacturers should expect lower expenses with automated tasks and fewer production errors. Labor costs also decrease since manufacturers need fewer workers for everyday, repetitive tasks.
And one more thing to mention here. A more efficient production process, especially if a manufacturer enables predictive maintenance, means lower downtime and maintenance costs.
5. Better business continuity
When the equipment or its component breaks down, manufacturers need time and costs to replace or fix it. Now imagine this is a piece of very critical machinery that is experiencing issues — some industrial equipment that your plant cannot work without. So, add here the effects of downtime costs and damaged reputation as orders are canceled or delayed.
With industry 4.0, your business continuity won’t be affected this much. Industrial assets are connected and monitored in real time. So, any equipment issues are addressed immediately or, better, prevented. Advanced maintenance and monitoring capabilities can also help businesses notice patterns and insights in production, which you can then use to optimize the processes further.
6. Flexibility and agility
Automation, machine learning, big data and AI , also bring lots of flexibility to production. Now manufacturers can easily scale their production up or down depending on the seasonal demand and market fluctuations. They can also predict those chances and include or exclude new product lines, avoiding wasted resources in the future.
7. Higher competitiveness
Manufacturing 4.0 also brings more opportunities for companies to stay competitive in the market. By implementing a smart factory, a company can increase its production rates while also minimizing the wastage of materials and valuable resources. Earlier, outsourcing to cheaper regions was the only way for manufacturers to remain competitive. Now businesses can compensate for this with investments in technology.
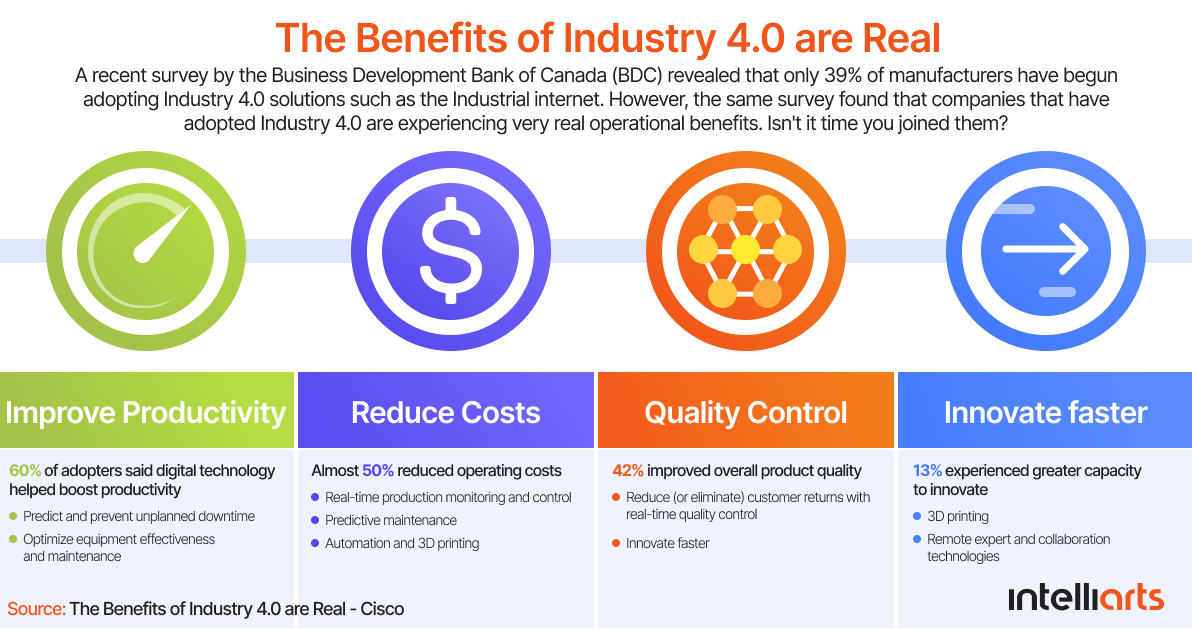
Applications of industry 4.0 in manufacturing
As you can see, industry 4.0 is already transforming the manufacturing sector, especially when it comes to:
1. Asset tracking and optimization
With industry 4.0, manufacturers can locate and monitor equipment, its components, different machines, and work in process. IoT and IIoT devices at place help to increase visibility on the factory floor and keep track of every single stage of production. Based on the data collected, the factory can optimize its operations and improve productivity in the long run.
2. Quality management
Another important use case of industry 4.0 in manufacturing includes quality control and management. With the help of AI and ML, you can identify the parameters relevant to product quality and then monitor deviations, if any. For example, in the case study , we tell how we helped to keep track of quality issues for an appliance manufacturer and resolved repeated equipment failures.
3. Predictive maintenance
Partly mentioned, predictive maintenance allows manufacturers to predict and prevent potential equipment problems. Manufacturers do their best to maximize production efficiency. And it’s not really efficient if you perform maintenance at scheduled intervals. The equipment might not need any intervention at this stage. Still, it’s also a poor strategy to wait until the equipment fully degrades and breaks down, and a manufacturer has to spend a fortune for its replacement or repair.
From this perspective, predictive maintenance seems an optimized solution, with systems being able to know about the business problem arising before it occurs. So, you could fix it early, avoid unplanned downtime, and improve equipment uptime.
Learn more about predictive maintenance in manufacturing by reading our White Paper “ Turn Predictive Maintenance into a Success Story for Your Manufacturing Company ”.
Real-world examples of manufacturing 4.0
Now let’s learn about industry 4.0 examples in today’s business environment and how manufacturers are reaping advantages from this.
A truly great example of today’s smart factory company is Audi. The smart manufacturing company is combining modular assembly (producing pre-assembled modules rather than individual components) with industry 4.0 in its car production. The factory relies a lot on big data, trying to make its production as data-driven and flexible as possible. It’s also implementing many other manufacturing 4.0 projects, from the application of virtual reality to AI/ML to 5G and 3D printing.
What differentiates Audi from other industry 4.0 companies is its focus on employees. While most companies see innovation and profitability as the main motivations behind emerging technology adoption, Audi pays much attention to the value that intelligent machines bring to the workplace. In particular, the company refers to better working conditions, especially when it comes to safety and security, improved reliability and ergonomics. As Henning Löser, Head of Production Labs, puts it :
“At the end of the day, vehicle construction remains craftsmanship. In the future, too, it will always be a question of cooperation between man and machine.”
The car manufacturer is definitely transitioning to the production of the future. Just check its fascinating use of technology in the video:
The appliance manufacturer, Whirpool, introduced a powerful analytics system a couple of years ago. The company was planning to eliminate waste that was sent to landfills. In this case, ML technology helped the smart manufacturing company to monitor the amount of wastage produced by its factories, as well as the usage of resources like electricity or water.
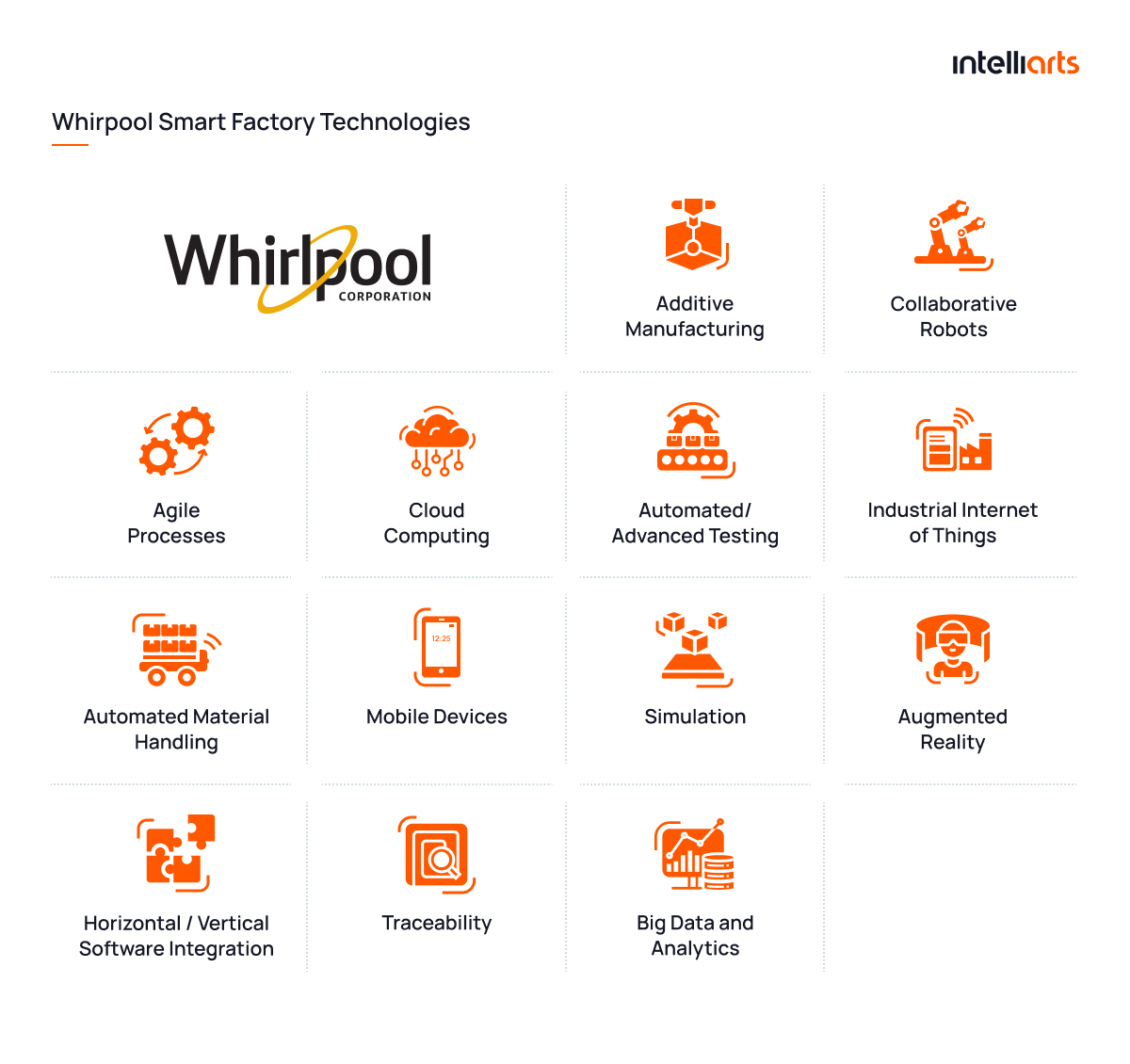
Whirpool’s smart factory is also a vivid example of how to connect plants distributed across the globe. IoT technology and analytics help the manufacturer monitor its sustainability performance in every plant, aiming at some unity in its zero-wastage policy.By 2023, the company has already achieved some impressive results :
- Three Brazilian plants have reached the zero-wastage goal, and what’s more, it happened two years earlier than planned. A few other facilities are also close to this goal.
- By projections, smart factories should help Whirlpool save around $1 million over the next three years,
- In the long run, the company expects to achieve its sustainability strategy with the aid of emerging technologies.
Appliance Manufacturer
In our portfolio, we have a compelling case story when we collaborated with a global appliance manufacturer also transitioning to industry 4.0. The company reached out to us for a technological solution for repeated and costly equipment breakdowns, which caused inconsistent work in the production line, delays in shipping, and overall low plant productivity.
After implementing an ML-based predictive maintenance solution, the Intelliarts team helped the company predict repeated system failures ahead of time with 90% accuracy. More importantly, this allowed the manufacturer to cut maintenance costs by 5% .
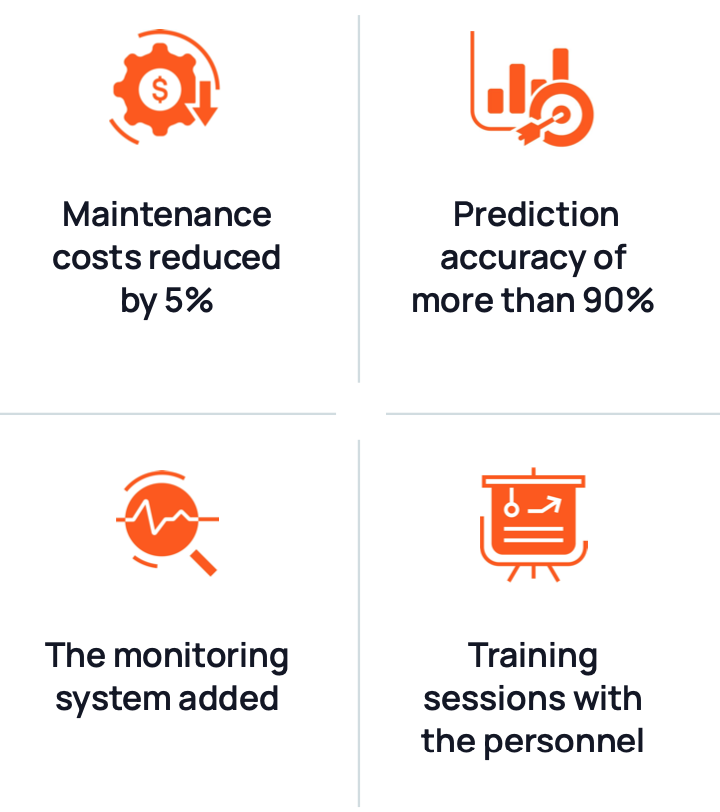
As part of the full-cycle project, we also set up the monitoring system so the customer was able to track the ML model performance and retrain it in case of any data changes. We also joined efforts with the company’s management to organize workshops for the manufacturing operators and teach them how to use the predictive maintenance solution to its full potential.
In 2018, Siemens received the industry 4.0 award for its manufacturing 4.0 solutions implemented in Elektronikwerk in Amberg (EWA), the smart factory in Germany. Equipped with AI/ML technology, lightweight robots, digital twins for production and quality control, and 3D printing, the electronics plant has people, machines, and equipment working side by side as a single mechanism.
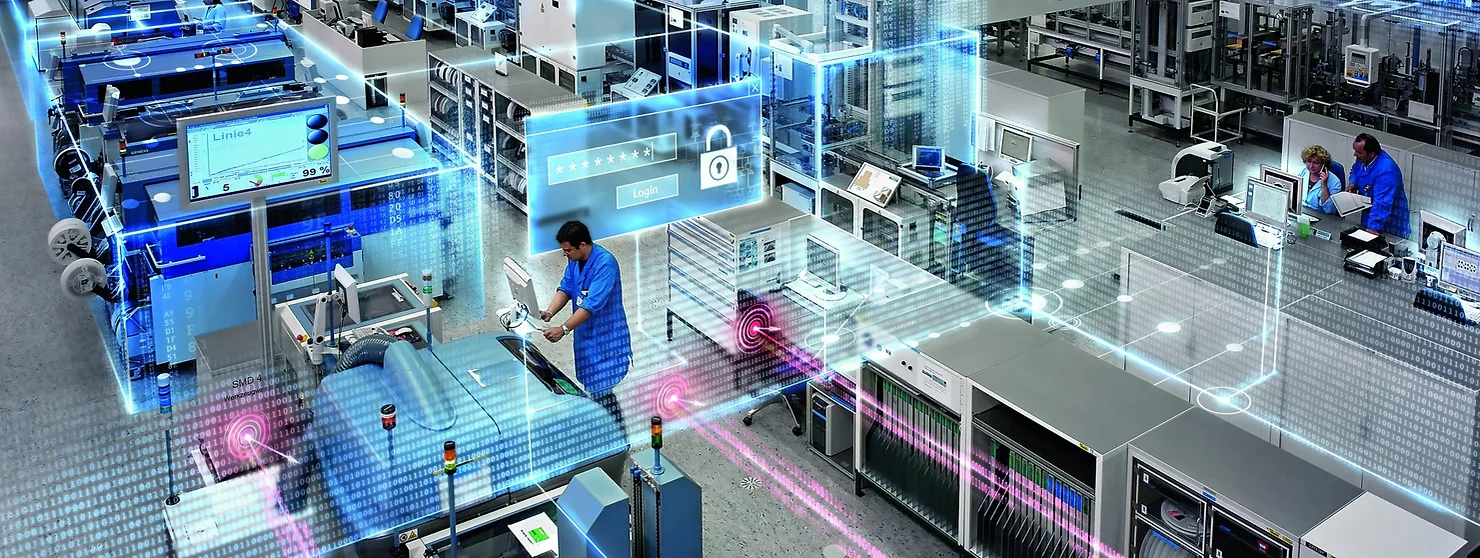
The smart factory uses dashboards to track machine performance and product quality in real-time, so the workers could react quickly to any deviations. Overall, different industry 4.0 components help the company increase productivity, achieve a higher degree of flexibility, and reliability.
Johnson & Johnson DePuy Synthes
Among other smart factory companies, Johnson & Johnson DePuy Synthes amazes with flexibility. While it’s challenging enough to build a factory of the future from scratch, it’s close to impossible to transform an old-fashioned factory into smart manufacturing. But this is exactly what the Irish medical device manufacturer was able to do.
By adopting IIoT and other popular technologies, DePuy Synthes created a visual factory solution, which provides real-time updates on production goals and allows factory personnel to monitor production levels. As the company’s leadership describes it , they allowed old machines to talk to each other and, as a consequence, were able to achieve a 10% reduction in operation costs and a 5% drop in downtime.
IoT and equipment monitoring aren’t the only implementations on DePuy Synthes’ factory floor. The company also benefits from 3D printing, digital twins, and AI to get advanced machine insights and optimize production processes.
Offering technology services itself, Amazon as one of the largest retailers in the US knows how to stay at the forefront of digital technologies and built a smart factory. The introduction of warehouse robots was among its boldest innovations. The company bought thousands of robots and delegated a large part of repeated tasks to them, from carrying pods to lifting totes to packing orders for shipment.
Another interesting example is the use of predictive maintenance on Amazon’s factory floor. With the help of IIoT and machine learning, the company monitors temperature and vibration levels every hour, knowing about and eliminating potential failures almost immediately. By estimations , it has reduced unplanned downtime by 69% and saved $22.75 million since implementing this technology.
Smart factories are especially popular among car manufacturers, but Tesla’s Gigafactory stands out even in this case. Ignoring its huge sizes (wait a minute, Nevada’s factory is 5.4 million square feet), Tesla impresses with its use of innovative technologies. Let’s take some of the most interesting examples:
- Model 3 production infrastructure has cars that can self-diagnose themselves, search for internal issues, and order replacement parts.
- Instead of a conveyor belt, Tesla uses its own invention “smart carts”. These are cart-like robots that carry individual cars and move them inside the factory.
- Shanghai’s version of Gigafactory benefits from 300 different types of robots used to automate various assembly line tasks, including narrow and deep laser welding. With this approach, factory workers don’t suffer from any harmful X-ray, while Tesla wins on welds of high-level quality.
- Tesla takes full advantage of cloud computing to store, process, and back up volumes of data collected. The company has also introduced its own Tesla Cloud. Although still under-developed and under-researched, it makes Tesla’s cars beat the competition by, for example, allowing seats and mirrors to be automatically adjusted while car sharing.
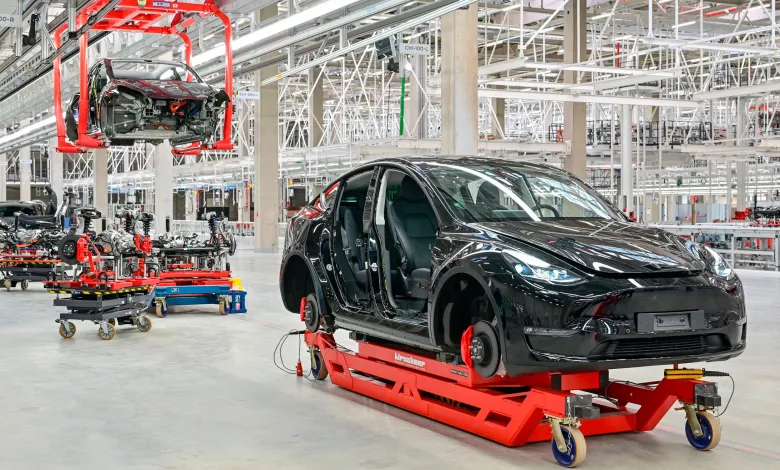
Tesla’s Smart Cart. Image taken from: US Today News
Manufacturing company
A large manufacturing company (under NDA) that we cooperated with has contacted us to solve the problem of repeated malfunctioning of the specific components of its hydraulic system. Of course, the industry 4.0 approach fitted best here.
The Intelliarts team has built an ML-based solution to predict the equipment failure of the components of the hydraulic system, prevent it from happening, and provide the proper maintenance to the facility. The customer was totally satisfied with the outcomes, with the ML models being almost 100% accurate in their results and predicting failure right. This helped the manufacturer to achieve a lower probability of unexpected downtime by the hydraulic system and, thus improve maintenance.
Read the full case study on predictive maintenance to get to know how the solution was built.
Why does industry 4.0 define the future of manufacturing?
Although some manufacturers are still hesitating to adopt innovative technologies, it’s obvious industry 4.0 and smart factories are the new normal in manufacturing. And those who transfer to manufacturing 4.0 earlier have a high chance to be ahead of the competition, let alone leveraging multiple benefits that industry 4.0 brings to manufacturing:
Better productivity
Improved product quality
Reduced costs
Increased resource utilization
If you want to give it a try, but still don’t know where to start, our advice is to start small by equipping your factory with IoT devices and introducing basic automation. In case you already have some IoT in place and would like to use it to your full advantage, we’d recommend scaling up with data analytics and AI/ML technology. Reach us to our Intelliarts team to discover how to move to data-driven decision-making in the most effective way.

According to McKinsey , the expected growth of Industry 4.0 is $3.7 trillion by 2025. So, the tendency is projected to gain momentum even further, bringing intensive growth and development to those manufacturers that adopt emerging technologies.
Sure. Manufacturing 4.0 has no limits when it comes to company size. By contrast, it’ll bring an extra competitive advantage to small and medium-sized businesses, helping them with more acknowledged use of resources, lower maintenance and operational costs, and quicker tempos of production.
The challenges of Manufacturing 4.0 include concerns around cybersecurity and data privacy, lack of appropriate digital infrastructure, the skill gap and labor shortages, and scalability issues, especially related to data growth.
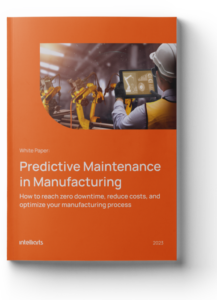
Take our Data & AI Strategy Readiness Assessment— for data leaders in manufacturing.
- Industrial IoT Platform
- Digital Twins
- Industrial AI & Analytics
- Production Visualization
Solutions for
- Engineering
- Executive Teams
- Request a Quote
Our Industries
- Auto, Mass Production and Consumers Goods
- Building Materials and Glass
- Chemical, Oil and Gas
- Food and Beverage
- Mines and Metals
- Paper, Packaging and Forest Products
- Pharmaceutical
- Case Studies
- White Papers
- Cloud / Big Data
- Edge Analytics
- Sustainability
- Continuous Improvement
- Industry 4.0
By Industry
- Aeronautics and Mass Transport
- Consumer Products (CPG)
- Oil and Gas
- Pulp, Paper, and Packaging
- Tires, Elastomer, and Plastic Extrusion
Company Info
- mybraincube.com
Featured Resource
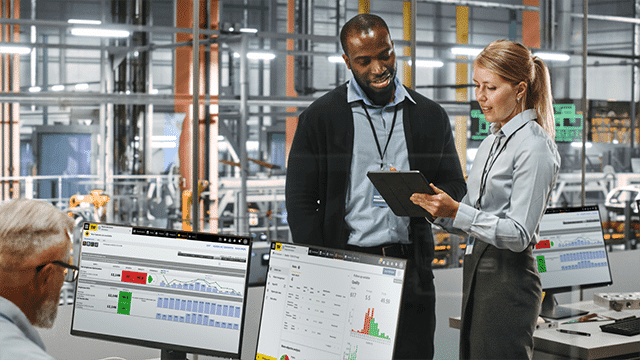
AI-powered automated operations have revolutionized various industries. However, to truly reap the benefits for both people and the environment, it is crucial to put these changes into practice. These practical implementations can unlock the full potential of autonomous manufacturing.
- Why Braincube?
- Our Solutions
- Request a Demo
technologies
Company info, 5 things you should know about the autonomous factory.
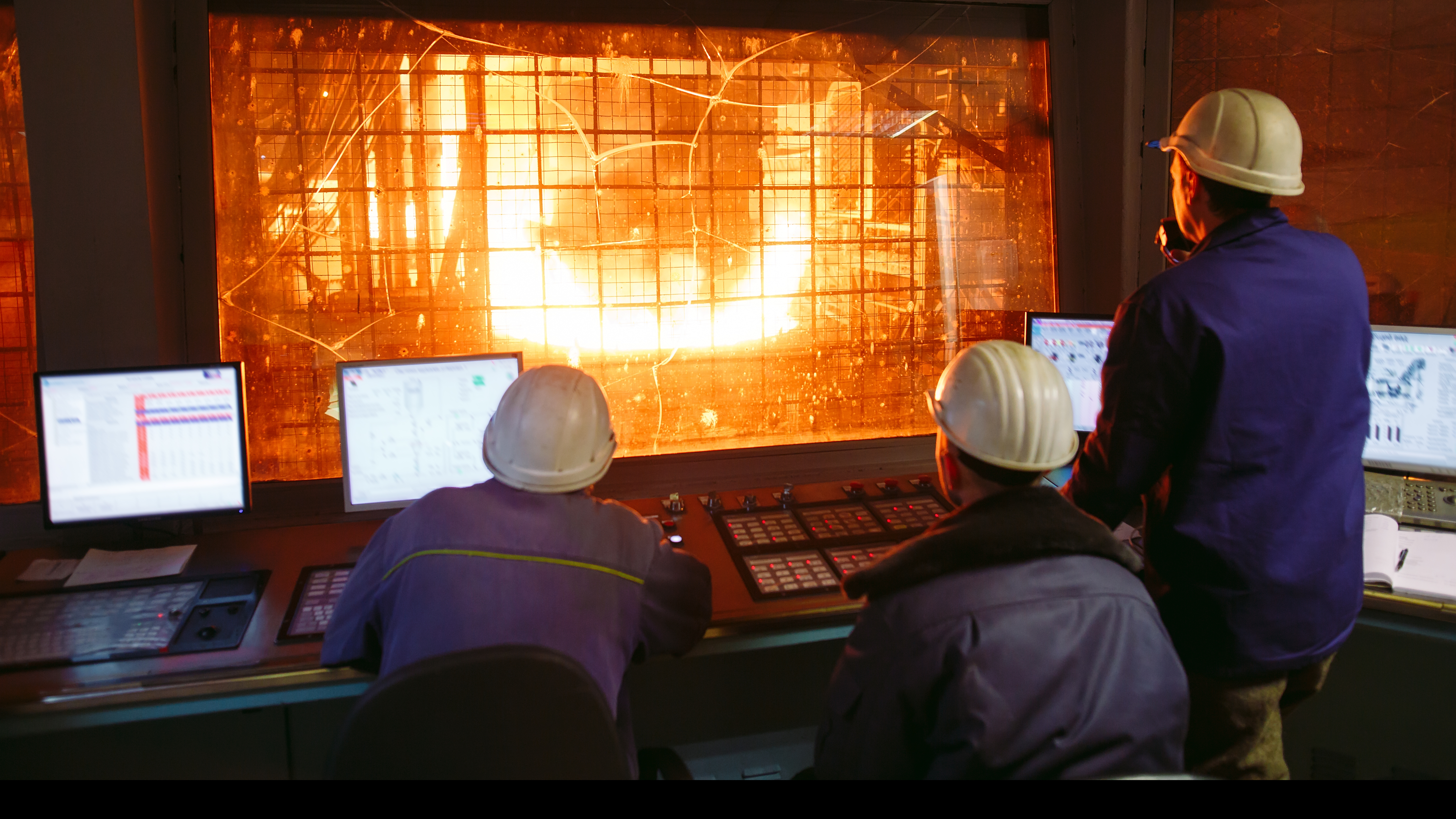
Industry 4.0 for Steel Production: Use Cases and Advantages of Using an Industrial IoT (IIoT) Platform
Rafael Barros (Operations Manager) and Benjamim Canato (Account Manager) have spent years implementing Industry 4.0 tools for Brazilian steel producers. In this exclusive interview, they share insights, use cases, and industry-specific examples for how Braincube’s IIoT Platform can benefit steel manufacturers.
What makes the steel industry different from other industries that Braincube specializes in?
Rafael Barros: Many of our other customers specialize in continuous process manufacturing, which brings its own unique challenges. Steel production takes a long time, sometimes spanning months in order to produce the final product. For our steel customers, we work with them to integrate data from long processes. This is part of our global rollout training and implementation program. Engineers can understand what happens to a single steel product throughout the entire process with their contextualized Digital Twin. The Digital Twin is built in tandem with Braincube manufacturing experts, data scientists, and the local SMEs who provide valuable context and insights into the build process. (Want to see more use cases for Digital Twins in steel manufacturing?)
Benjamim Canato: Steel manufacturers make hundreds—sometimes thousands—of different products simultaneously at the same mill. Braincube can help steel producers track these products individually and analyze each specific product. Many steel producers already use sensors in their production processes, but most IIoT companies only aggregate this data. Braincube transforms this data into powerful information.
Based on what you’ve seen over the past few years, what is one of the biggest challenges steel manufacturers face today?
Benjamim: Steel manufacturers have access to a lot of data but they don’t always have all of the answers they need to make additional improvements. Additionally, their data often live in different, disconnected sources. There is not an easy way to bring it together for analysis.
Why is disconnected data problematic in steel?
Benjamim: Let’s say a product starts in the melt shop in January and exits the lamination stage in March. If you want to analyze data from the entire process, you may not know exactly when the product was in each phase or for how long. You might know the material was in the casting process sometime in late February but you don’t know the exact day hour or length of time. This limits your ability to find key optimizations in your process that could save you time, efficiency, and money.
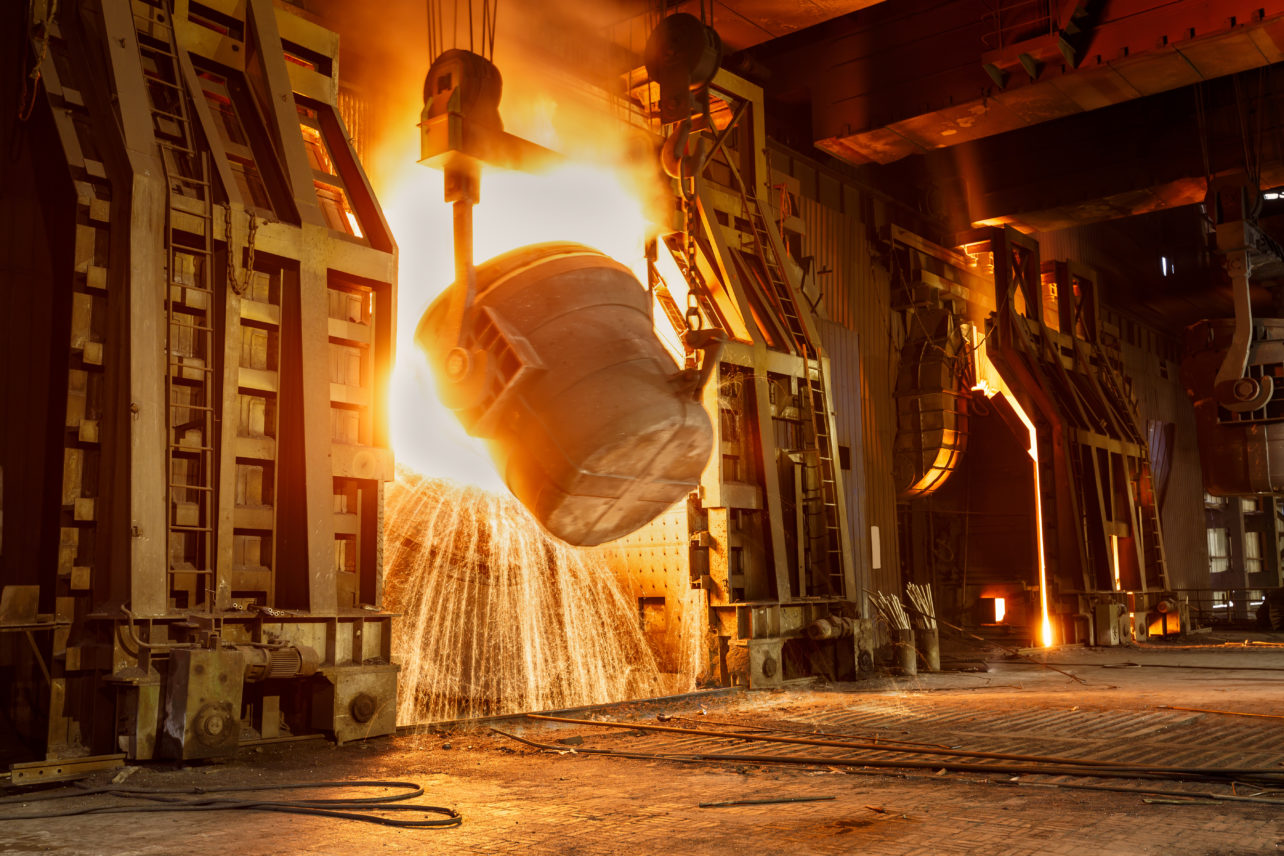
Rafael: In short, Braincube’s IIoT Platform solves the problem of disconnected data sources. It enables companies to track an individual product from raw material to end product in one flow, always making sure the correct data is being used for each analysis. With this type of IIoT Platform, teams have readily accessible, cleansed, accurate data, which helps a lot of company initiatives.
Steel manufacturing is a particularly complicated industry when it comes to data aggregation. Why should steel producers choose an IIoT platform over other data solutions?
Benjamim : Depending on a steel company’s needs, they likely use two platforms for their data. One platform connects and delivers data. The other platform is used to analyze data. They may also have additional platforms for other steps, such as building charts or monitoring production. This means employees must learn multiple programs or tools in order to perform their job.
Braincube was designed as a global data solution, with the ultimate vision of empowering companies to be autonomous power users of their data. – Benjamim Canato, Braincube Account Manager in Brazil
An IIoT platform minimizes the need to learn so many different programs. It centralizes data from multiple sources, structures the data for analysis, and some vendors even provide analysis tools —all within the same platform. Employees can use the same platform to analyze data from different sources, make graphs and tables, and implement changes to the production process. IT teams can fully manage the system, ensuring that proprietary data is safely secured .
In a recent meeting with a Braincube customer, the CIO asked for a global solution that their IT team could manage internally. They currently have different solutions in Africa and South America but wanted one solution that the IT team could manage and that everyone could learn across the world.
We often see companies that want this capability. Braincube was designed as a global data solution, with the ultimate vision of empowering companies to be autonomous power users of their data.
Can you share some examples of how centralized, structured data benefited steel customers?
Benjamim: Sure! If a customer discovers a defect during lamination, it’s too late to go back and fix the issue. They have to decide to scrap the product or, possibly, find a different buyer who would accept the defective product at a lower price.
With Braincube’s IIoT Platform , teams can go beyond understanding what went wrong in production. They can actually uncover the exact settings to have better control or quality for future runs. Companies can improve future production by quickly identifying what went wrong earlier in the process by leveraging the Braincube App Suite , which is included in our IIoT Platform solution.
Getting more specific, Braincube’s CrossRank helped one company identify that changing their furnace temperature by 10 degrees celsius would minimize their waste. They implemented this change in the furnace and achieved the results that Braincube predicted, saving them material costs and reducing waste.
In another example, a company wanted to look at the impact of changing the amount and types of chemicals used in production. They struggled to get an answer because the data was in different systems. They didn’t know how changing these chemicals during production would impact the lamination process. With Braincube’s Digital Twin, they could analyze historical data from different sources. They saw what happened in the past, found the ideal recipe, and made a global standard procedure change across their mills.
With Braincube’s IIoT Platform, teams can go beyond understanding what went wrong in production. They can actually uncover the exact settings to have better control or quality for future runs. — Benjamim Canato, Braincube Account Manager in Brazil
Are there other interesting use cases for centralized data?
Rafael: We have also seen steel companies use Braincube to quickly understand customer issues. Let’s say a customer calls with a complaint about a product they received. Without Braincube, if a customer complains about a defective product, the engineers must dig through raw data to find an answer. They need to try piecing together information from each step of the process. Not only is this a timely project, but it is difficult for a human to analyze the hundreds of factors that went into making a specific batch of each product.
With Braincube, the steel company can use the batch number from their Digital Twin to analyze what happened and report back to the customer about what changes they are going to make so future products remain in-spec. This reassures the customer’s trust in the company and plays into ongoing quality and optimization efforts. If they uncover a wider defect that impacts other customers, they can be proactive and reach out to these customers as well. Financially, it also saves the steel company by avoiding downtime due to preventable issues.
You’ve shared a lot of “big picture” benefits for centralizing process data and using Digital Twins for manufacturing. How can these things help the individual teams working on specific steps of the steel production process?
Rafael : The production process begins with raw materials in the melt shop. A smart sensor combines few data sources to generate a large amount of information. By combining data from multiple sources and systems, steel manufacturers can track specific product batches from the very start of the production process.
For example, a temperature sensor combined with a belt rotation sensor can be structured to generate valuable information about the process. Process specialists can know how long the product spent in the hottest part of the heating curve, if there were temperature spikes (and at what stage of the process), and which batches were affected by these findings. This means there is no guessing about what raw materials went into the final product.
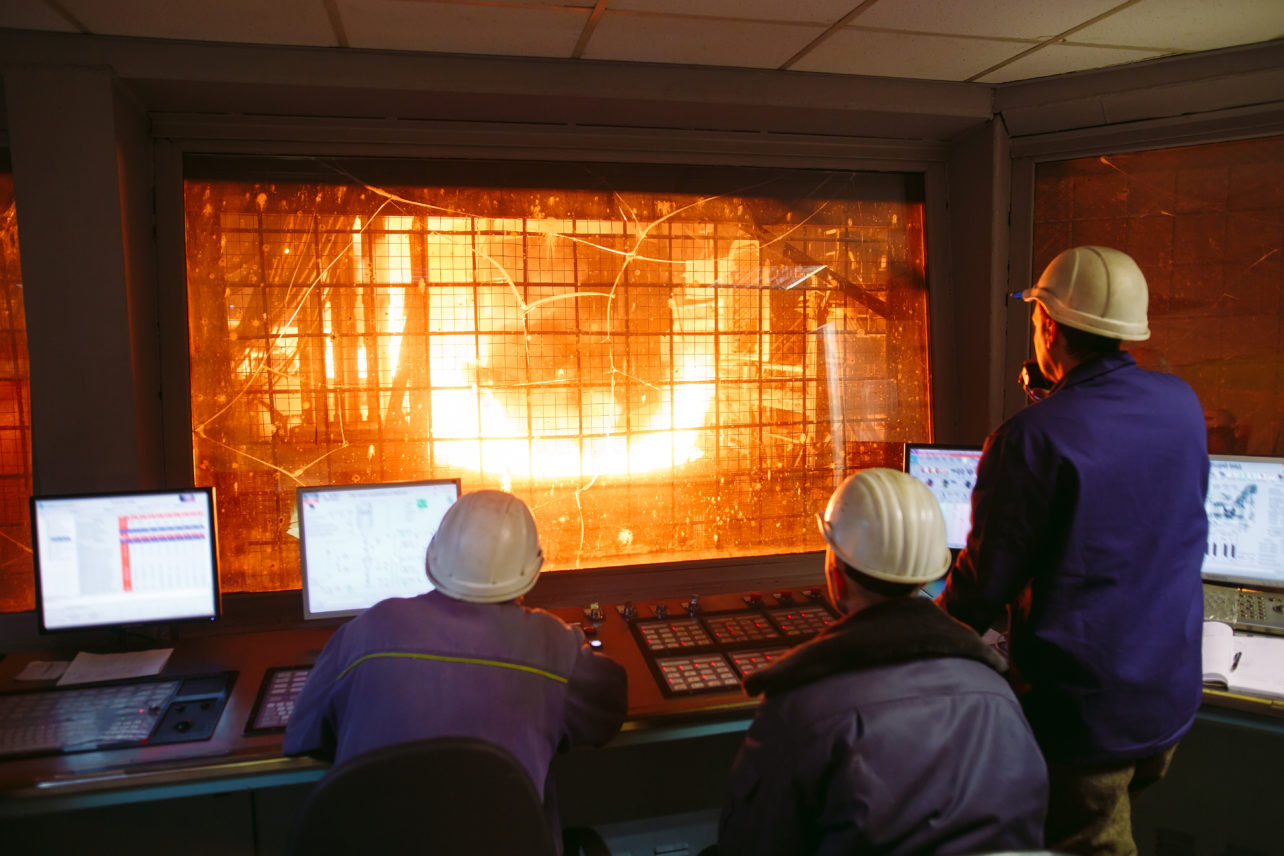
Batch tracking is useful in the casting phase as well. As different batches make their way into different casting lines, you know the source of each final product. You can also perform multiple variable analyses to improve your process.
Benjamim : Teams working at the end of the production process also benefit. Parts of the lamination process take milliseconds, and there are a lot of different things happening at the same line. Many rolls are being produced and each roll has differing speed, pressure, and temperature requirements. It can be hard to understand why there is a problem with the end product. Braincube makes it easy to find the answers.
What is one thing you wish steel companies knew about IIoT?
Rafael : We’ve talked a lot about how IIoT can help with the operational aspects of manufacturing, such as improving processes or helping engineers uncover answers. But IIoT is also a strategic IT advantage for steel manufacturers.
The whole goal of IT teams is to provide operations teams with data so they can perform analyses. An IIoT platform centralizes everything. The platform connects different data sources, transforms raw sensor data into smart sensor data, and then helps IT teams easily distribute this data to end-users to be used in other applications. It can be an infrastructure tool for data and set the global data strategy for the company.
When IT teams can provide better data to their end-users, it makes it possible for OT teams to uncover insights that move the company forward: it’s a win-win.
How can Digital Twins help steel manufacturers?
Digital Twins are a trendy topic in the manufacturing world. But can Digital Twins really help your steel manufacturing company? Our IIoT specialists break down the perks.
See Braincube in action
Ready to see Braincube in action? Schedule a one-on-one demo of our IIoT Platform.
The power of centralized data
Finding connections in your production process begins with centralizing data—from any system, in any format.
Industry 4.0 in your Inbox
Privacy overview.
Necessary cookies are absolutely essential for the website to function properly. This category only includes cookies that ensures basic functionalities and security features of the website. These cookies do not store any personal information.
Analytical cookies are used to understand how visitors interact with the website. These cookies help provide information on metrics, themetrics the number of visitors, bounce rate, traffic source, etc.
Google Analytics Privacy Policy Pardot Privacy Policy
Performance cookies are used to understand and analyze the key performance indexes of the website which helps in delivering a better user experience for the visitors.
Preference cookies are used to store user preferences to provide content that is customized and convenient for the users, like the language of the website or the location of the visitor.
Advertisement cookies are used to provide visitors with relevant ads and marketing campaigns. These cookies track visitors across websites and collect information to provide customized ads.
LinkedIn Privacy Policy Google Ads Privacy Policy YouTube Privacy Policy
Undefined cookies are those that are being analyzed and have not been classified into a category as yet.
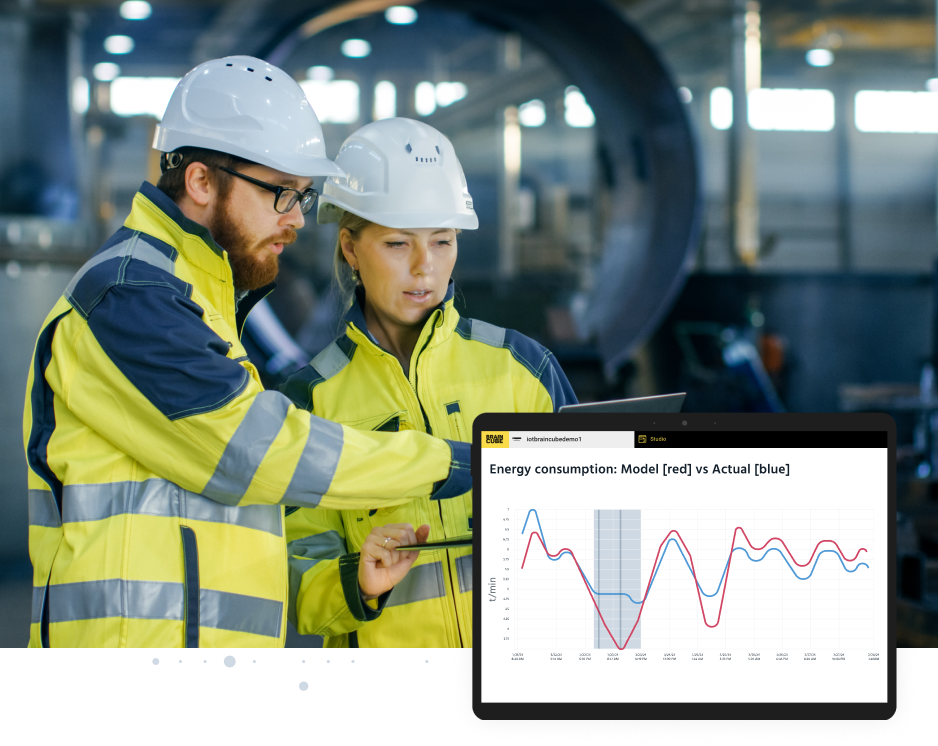
Introducing predictive analytics
Dive deep into your processes to uncover hidden improvement opportunities. Detect patterns, prevent downtime, and reduce costs.
More From Forbes
The revolutionary impact of industry 4.0.
- Share to Facebook
- Share to Twitter
- Share to Linkedin
Vinod Jain is an expert in global and digital business, former business professor, consultant, speaker, and author of Global Meets Digital.
We are currently in the early stages of the Fourth Industrial Revolution, also known as Industry 4.0. Klaus Schwab, the founder and executive chairman of the World Economic Forum, introduced the term Industry 4.0 at the annual 2016 WEF conference . Schwab suggests that the Fourth Industrial Revolution is different in scale, scope and complexity from the prior three industrial revolutions.
I would like to highlight some of the core technologies underlying Industry 4.0, including the Internet of Things (IoT), artificial intelligence (AI), cloud computing and digital twins. With these tools, I’d also like to discuss examples of how you can take advantage of the changes within Industry 4.0.
The Internet Of Things
According to Oracle , the IoT refers to the network of physical devices ("things") with built-in sensors and software and connected to the internet. There are billions of such devices in the world today, including smartphones, smart watches, autonomous vehicles and home security systems.
In 2012, General Electric introduced the term Industrial Internet of Things (IIoT) to refer to industrial equipment and vehicles embedded with software and sensors and connected to the internet. The IIoT, a subset of IoT, includes high-stakes industrial equipment like machinery and power generation, where unplanned equipment failure can be very costly, even life-threatening.
UFC 305 Results: Fighter Goes Viral With ‘Outrageous’ Head Knot
10 great movies leaving netflix at the end of august, samsung warns millions of galaxy users—you will miss update deadline.
A key feature of the IoT and IIoT is that the sensors in the equipment generate vast amounts of data, which can be used to improve operations in many ways.
Artificial Intelligence
AI is a machine’s ability to perform cognitive functions generally associated with the human mind. From the perspective of Industry 4.0, AI helps industrial companies take advantage of the data generated by sensors—thus providing visibility of and insights about operations and processes.
Cloud Computing
Cloud computing is the on-demand processing and analysis of data stored in a cloud. It enables industrial companies to integrate diverse functions like engineering, production, supply chain, sales, distribution and service efficiently and cost-effectively.
Digital Twin
A digital twin is a virtual, digital representation of a machine or system designed to simulate its actual operation. The idea is to simulate the workings of the machine or system using a digital model to identify potential problems and create enhancements.
With the introduction of these technologies, operational efficiencies are improving like never before through better visibility and control of operations.
Below are some examples of how you can take advantage of Industry 4.0:
Preventive Maintenance
Preventive maintenance involves scheduling regular maintenance of industrial equipment to reduce the possibility of unexpected breakdowns, expensive repairs and loss of production. In the age of IIoT, sensors embedded in the equipment monitor its performance remotely and act autonomously if required, thus enabling predictive maintenance. A 2021 study by McKinsey & Co. found that predictive maintenance using IoT cut unplanned downtime at a BMW plant by 25%.
For example, Colgate-Palmolive Company installed sensors in thousands of machines to collect real-time operational data. The sensors monitor machine vibration and detect mechanical problems by comparing their data with identical machines at other locations. This saved the company significantly in downtime costs.
Remote Monitoring And Diagnostics
The sensors in IIoT equipment can monitor temperature, pressure, vibration and other parameters continuously and even change a setting or initiate action as needed. These diagnostic steps and actions occur remotely and autonomously through IIoT gateways.
For example, Schlumberger, a large U.S. oilfield services company, installs sensors in downhole tools and surface equipment at oil rigs. The sensors continuously monitor and control factors such as pressure and temperature, thus improving production optimization. Such measurements are taken remotely without the need for employees to go to field locations, some of which could be dangerous, even offshore.
Remote Software Updates
With billions of IoT devices worldwide, manufacturers need to be able to make automatic, remote software updates to fix bugs, add new features and improve device functionality.
Luxury cars and, increasingly, other upscale cars have hundreds of sensors embedded in them; car manufacturers regularly update the embedded software remotely. The same is true for millions of sensors in other IoT devices.
Asset Tracking
IIoT-based asset tracking systems include identifying the location of an asset and its status using RFID, GPS or other technologies, thus reducing the time and cost of tracking the assets manually to identify its location and operating status.
Lineas, a private rail freight operator in Europe, uses an IIoT-based Track & Trace cross-border fleet management system from Bosch on its wagons. It offers Lineas information on where its wagons are located at any time. It can then communicate ETAs to clients so that they may better plan their loading/unloading and other operations.
Automatic Fulfillment
With asset tracking via IIoT, many supply chain processes are being automated to improve efficiency and reduce costs. One such application is the automation of inventory fulfillment centers and reordering processes. Sensors in warehouse equipment monitor stock levels and initiate a reorder when inventory levels become low or reach a certain level.
Many printers have sensors installed in them, which monitor ink consumption. HP and other companies offer printer owners automatic ink cartridge replacement service for a monthly subscription.
Compliance Monitoring
Industrial manufacturers must comply with the relevant occupational health and safety regulations and data protection regulations, among others. In food industries, regulatory agencies always require food safety compliance. During the Covid-19 pandemic, some employees were tracked through wearable trackers or smartphones to ensure compliance with social-distancing requirements. IIoT-based systems can make such compliance easy and consistent with reporting requirements.
Industry 4.0 is much more than a technological leap; it is reshaping the way we approach production, management and even societal challenges.
However, this journey is not without its challenges. Cybersecurity, ethical concerns and the need for a skilled workforce are critical issues that require ongoing attention. In a digital manufacturing environment, workers should have the ability to work with computers, CAD/CAM tools and digital literacy in areas like IoT and data analysis.
Forbes Business Council is the foremost growth and networking organization for business owners and leaders. Do I qualify?
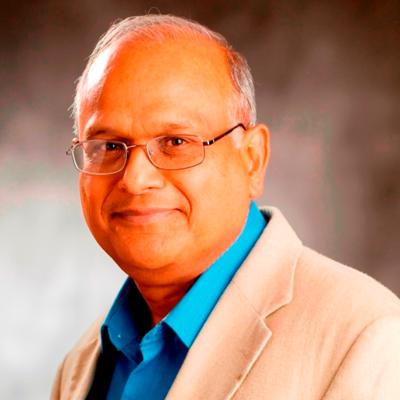
- Editorial Standards
- Reprints & Permissions
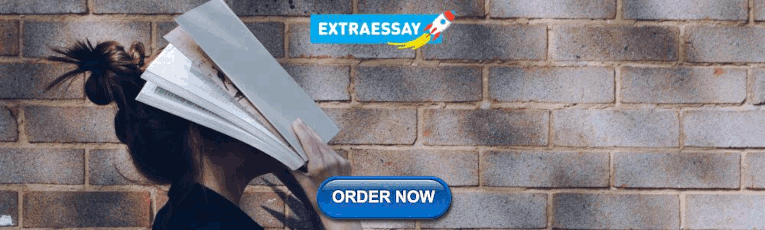
COMMENTS
In the past five years, a select group of companies have started pulling ahead in their efforts to implement Industry 4.0 across their manufacturing networks. Leading manufacturers are now realizing significant value from data and analytics, AI, and machine learning (ML). However, a large majority remain stuck in pilot purgatory, struggling to capture the full potential of their transformation ...
Autonomous driving is turning from a moonshot to a reality. These technology shifts are shaping the next industrial revolution, i.e. the Industry 4.0.
This work has a broad view of the industry 4.0 influence in the global supply chain companies, as it is essential for long-term business sustainability and one of the key drivers of profitability and growth.
To address these disruptions, successful advanced industry (AI) companies are leveraging Industry 4.0 to achieve faster, more sustainable change, shown most dramatically at "lighthouse" manufacturers that have led the way in Industry 4.0 implementation. Through the Global Lighthouse Network (GLN), a research collaboration between the World Economic Forum and McKinsey on the future of ...
Industry 4.0, IIoT, and digitisation are currently some of the most-discussed and yet least understood topics within manufacturing today. With still a lot of confusion surrounding Industry 4.0, today we'll be exploring the key technologies behind Industry 4.0, as well as real-world applications.
The scientific community has shown considerable interest in Industry 4.0 due to its capacity to revolutionise the manufacturing sector through digitalisation and data-driven decision-making. However, the actual implementation of Industry 4.0 within complex industrial settings presents obstacles that are typically beyond the scope of mainstream research articles. In this paper, a comprehensive ...
Our Industry 4.0 Maturity Model looks holistically across six categories and twenty-eight attributes to identify the current situation, and then with our expertise helps to define the actions to move forward and generate business improvement.
To this end, Industry 4.0 value is in closed control loops (enabled by sensor-based, in-line quality inspection), flexible routing, scheduling, load balancing and performance management, and the extension of automation to final assembly.
The Industry 4.0 is becoming a hot topic. In this paper, we revisit Industry 4.0 from the perspectives of its purposes, features, and key performance indicators. We present a reference roadmap for advancing an Industry 4.0 project from plan to implementation. We present the case study of steel industry 4.0 and lessons. In addition, we give suggestions directing the advancement of Industry 4.0.
This chapter aims to analyze the Industry 4.0 framework, identify the definition and drivers of the Industry 4.0 paradigm, discuss its potential effect, and determine obstacles of the Industry 4.0. For the research methodology, a critical literature review is performed, we relied on the recent studies related to industry 4.0. Findings - This study concluded that Industry 4.0 describes a ...
The transition to industry 4.0 has impacted factories, but it also affects the entire value chain. In this sense, human-centred factors play a core role in transitioning to sustainable manufacturing processes and consumption. The awareness of human roles in Industry 4.0 is increasing, as evidenced by active work in developing methods, exploring influencing factors, and proving the ...
Industry 4.0 example #1: Performance management using Big Data analytics, AI, and machine learning. There are a lot of great metrics to track packaging equipment, especially overall equipment effectiveness (OEE).
Curated Set of Case Studies demonstrating problem, solution, and results of implementing Industry 4.0.
The purpose of this paper is to provide an analysis of diverse case studies of Industry 4.0. Industry 4.0 represents the fourth Industrial revolution which illustrates the structure of the organization that controls the production of the products in the entire life cycle. Industry 4.0 is a term that can be given to the combination of humans and technology, when is mixed give a digital way of ...
The concepts of sustainable supply chains and Industry 4.0 are progressively getting attention in different domains. Companies have started developing and implementing these practices in their busi...
Industry 4.0—which includes connectivity, advanced analytics, automation, and advanced-manufacturing technologies (Exhibit 1)—was gaining momentum before COVID-19, helping companies transform their operations in everything from production efficiency to product customization, with improvements in speed to market, service effectiveness, and ...
The Smart Factory and the Internet of Things - An Industry 4.0 Case Study. By Uwe Glockmann. On a snowy December morning in 2019, I find myself at the gates of Rittal's new Smart Factory in Haiger, in Middle Hesse. The world's leading system provider for control cabinets, power distribution, climate control, IT infrastructure and software ...
This is how industry 4.0 is paving its way for the manufacturing industry, enabling factories to collect, analyze, and make decisions based on immense stockpiles of data. But what's the value for business owners? Increased automation, a new level of process efficiencies, and improved quality at lower costs, to name a few.
How a Food and Bev manufacturer reduces scrap and improves product consistency. Learn more in this Food and Beverage Industry 4.0 case study.
Case study results and future plans are summarized in conclusion. Transforming into a Smart Factory and implementing Industry 4.0 technology is an extensive and long-term process.
In this interview with Braincube steel specialists, steel producers can learn how to implement Industry 4.0 tools and strategies.
While optimism prevails, companies have achieved varying degrees of success in implementing Industry 4.0 We conducted the second edition of our Industry 4.0 Global Expert Survey in January 2016. The survey engaged a panel of 300 industry experts in Germany, the US, and Japan and focused on potential changes in attitudes towards Industry 4.0, progress made implementing Industry 4.0, drivers of ...
Here are some examples of how you can take advantage of the changes within Industry 4.0.
Embracing Industry 4.0 and digital manufacturing: How Siemens helped Electrolux improve time-to-market and reduce production costs.