
An official website of the United States government
The .gov means it’s official. Federal government websites often end in .gov or .mil. Before sharing sensitive information, make sure you’re on a federal government site.
The site is secure. The https:// ensures that you are connecting to the official website and that any information you provide is encrypted and transmitted securely.
- Publications
- Account settings
Preview improvements coming to the PMC website in October 2024. Learn More or Try it out now .
- Advanced Search
- Journal List
- Behav Sci (Basel)

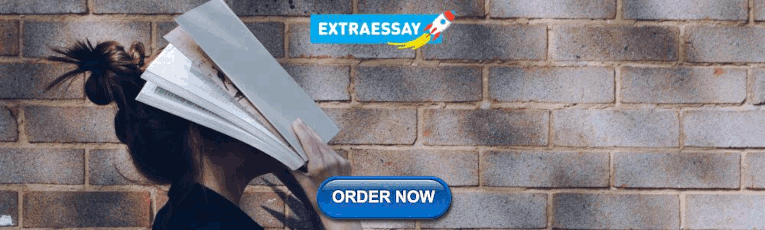
Assessing Cognitive Factors of Modular Distance Learning of K-12 Students Amidst the COVID-19 Pandemic towards Academic Achievements and Satisfaction
Yung-tsan jou.
1 Department of Industrial and Systems Engineering, Chung Yuan Christian University, Taoyuan 320, Taiwan; wt.ude.ucyc@uojty (Y.-T.J.); moc.oohay@enimrahcrolfas (C.S.S.)
Klint Allen Mariñas
2 School of Industrial Engineering and Engineering Management, Mapua University, Manila 1002, Philippines
3 Department of Industrial Engineering, Occidental Mindoro State College, San Jose 5100, Philippines
Charmine Sheena Saflor
Associated data.
Not applicable.
The COVID-19 pandemic brought extraordinary challenges to K-12 students in using modular distance learning. According to Transactional Distance Theory (TDT), which is defined as understanding the effects of distance learning in the cognitive domain, the current study constructs a theoretical framework to measure student satisfaction and Bloom’s Taxonomy Theory (BTT) to measure students’ academic achievements. This study aims to evaluate and identify the possible cognitive capacity influencing K-12 students’ academic achievements and satisfaction with modular distance learning during this new phenomenon. A survey questionnaire was completed through an online form by 252 K-12 students from the different institutions of Occidental Mindoro. Using Structural Equation Modeling (SEM), the researcher analyses the relationship between the dependent and independent variables. The model used in this research illustrates cognitive factors associated with adopting modular distance learning based on students’ academic achievements and satisfaction. The study revealed that students’ background, experience, behavior, and instructor interaction positively affected their satisfaction. While the effects of the students’ performance, understanding, and perceived effectiveness were wholly aligned with their academic achievements. The findings of the model with solid support of the integrative association between TDT and BTT theories could guide decision-makers in institutions to implement, evaluate, and utilize modular distance learning in their education systems.
1. Introduction
The 2019 coronavirus is the latest infectious disease to develop rapidly worldwide [ 1 ], affecting economic stability, global health, and education. Most countries have suspended thee-to-face classes in order to curb the spread of the virus and reduce infections [ 2 ]. One of the sectors impacted has been education, resulting in the suspension of face-to-face classes to avoid spreading the virus. The Department of Education (DepEd) has introduced modular distance learning for K-12 students to ensure continuity of learning during the COVID-19 pandemic. According to Malipot (2020), modular learning is one of the most popular sorts of distance learning alternatives to traditional face-to-face learning [ 3 ]. As per DepEd’s Learner Enrolment and Survey Forms, 7.2 million enrollees preferred “modular” remote learning, TV and radio-based practice, and other modalities, while two million enrollees preferred online learning. It is a method of learning that is currently being used based on the preferred distance learning mode of the students and parents through the survey conducted by the Department of Education (DepEd); this learning method is mainly done through the use of printed and digital modules [ 4 ]. It also concerns first-year students in rural areas; the place net is no longer available for online learning. Supporting the findings of Ambayon (2020), modular teaching within the teach-learn method is more practical than traditional educational methods because students learn at their own pace during this modular approach. This educational platform allows K-12 students to interact in self-paced textual matter or digital copy modules. With these COVID-19 outbreaks, some issues concerned students’ academic, and the factors associated with students’ psychological status during the COVID-19 lockdown [ 5 ].
Additionally, this new learning platform, modular distance learning, seems to have impacted students’ ability to discover and challenged their learning skills. Scholars have also paid close attention to learner satisfaction and academic achievement when it involves distance learning studies and have used a spread of theoretical frameworks to assess learner satisfaction and educational outcomes [ 6 , 7 ]. Because this study aimed to boost academic achievement and satisfaction in K-12 students, the researcher thoroughly applied transactional distance theory (TDT) to understand the consequences of distance in relationships in education. The TDT was utilized since it has the capability to establish the psychological and communication factors between the learners and the instructors in distance education that could eventually help researchers in identifying the variables that might affect students’ academic achievement and satisfaction [ 8 ]. In this view, distance learning is primarily determined by the number of dialogues between student and teacher and the degree of structuring of the course design. It contributes to the core objective of the degree to boost students’ modular learning experiences in terms of satisfaction. On the other hand, Bloom’s Taxonomy Theory (BTT) was applied to investigate the students’ academic achievements through modular distance learning [ 6 ]. Bloom’s theory was employed in addition to TDT during this study to enhance students’ modular educational experiences. Moreover, TDT was utilized to check students’ modular learning experiences in conjuction with enhacing students’ achievements.
This study aimed to detect the impact of modular distance learning on K-12 students during the COVID-19 pandemic and assess the cognitive factors affecting academic achievement and student satisfaction. Despite the challenging status of the COVID-19 outbreak, the researcher anticipated a relevant result of modular distance learning and pedagogical changes in students, including the cognitive factors identified during this paper as latent variables as possible predictors for the utilization of K-12 student academic achievements and satisfaction.
1.1. Theoretical Research Framework
This study used TDT to assess student satisfaction and Bloom’s theory to quantify academic achievement. It aimed to assess the impact of modular distance learning on academic achievement and student satisfaction among K-12 students. The Transactional Distance Theory (TDT) was selected for this study since it refers to student-instructor distance learning. TDT Moore (1993) states that distance education is “the universe of teacher-learner connections when learners and teachers are separated by place and time.” Moore’s (1990) concept of ”Transactional Distance” adopts the distance that occurs in all linkages in education, according to TDT Moore (1993). Transactional distance theory is theoretically critical because it states that the most important distance is transactional in distance education, rather than geographical or temporal [ 9 , 10 ]. According to Garrison (2000), transactional distance theory is essential in directing the complicated experience of a cognitive process such as distance teaching and learning. TDT evaluates the role of each of these factors (student perception, discourse, and class organization), which can help with student satisfaction research [ 11 ]. Bloom’s Taxonomy is a theoretical framework for learning created by Benjamin Bloom that distinguishes three learning domains: Cognitive domain skills center on knowledge, comprehension, and critical thinking on a particular subject. Bloom recognized three components of educational activities: cognitive knowledge (or mental abilities), affective attitude (or emotions), and psychomotor skills (or physical skills), all of which can be used to assess K-12 students’ academic achievement. According to Jung (2001), “Transactional distance theory provides a significant conceptual framework for defining and comprehending distance education in general and a source of research hypotheses in particular,” shown in Figure 1 [ 12 ].

Theoretical Research Framework.
1.2. Hypothesis Developments and Literature Review
This section will discuss the study hypothesis and relate each hypothesis to its related studies from the literature.
There is a significant relationship between students’ background and students’ behavior .
The teacher’s guidance is essential for students’ preparedness and readiness to adapt to a new educational environment. Most students opt for the Department of Education’s “modular” distance learning options [ 3 ]. Analyzing students’ study time is critical for behavioral engagement because it establishes if academic performance is the product of student choice or historical factors [ 13 ].
There is a significant relationship between students’ background and students’ experience .
Modules provide goals, experiences, and educational activities that assist students in gaining self-sufficiency at their speed. It also boosts brain activity, encourages motivation, consolidates self-satisfaction, and enables students to remember what they have learned [ 14 ]. Despite its success, many families face difficulties due to their parents’ lack of skills and time [ 15 ].
There is a significant relationship between students’ behavior and students’ instructor interaction .
Students’ capacity to answer problems reflects their overall information awareness [ 5 ]. Learning outcomes can either cause or result in students and instructors behavior. Students’ reading issues are due to the success of online courses [ 16 ].
There is a significant relationship between students’ experience and students’ instructor interaction .
The words “student experience” relate to classroom participation. They establish a connection between students and their school, teachers, classmates, curriculum, and teaching methods [ 17 ]. The three types of student engagement are behavioral, emotional, and cognitive. Behavioral engagement refers to a student’s enthusiasm for academic and extracurricular activities. On the other hand, emotional participation is linked to how children react to their peers, teachers, and school. Motivational engagement refers to a learner’s desire to learn new abilities [ 18 ].
There is a significant relationship between students’ behavior and students’ understanding .
Individualized learning connections, outstanding training, and learning culture are all priorities at the Institute [ 19 , 20 ]. The modular technique of online learning offers additional flexibility. The use of modules allows students to investigate alternatives to the professor’s session [ 21 ].
There is a significant relationship between students’ experience and students’ performance .
Student conduct is also vital in academic accomplishment since it may affect a student’s capacity to study as well as the learning environment for other students. Students are self-assured because they understand what is expected [ 22 ]. They are more aware of their actions and take greater responsibility for their learning.
There is a significant relationship between students’ instructor interaction and students’ understanding .
Modular learning benefits students by enabling them to absorb and study material independently and on different courses. Students are more likely to give favorable reviews to courses and instructors if they believe their professors communicated effectively and facilitated or supported their learning [ 23 ].
There is a significant relationship between students’ instructor interaction and students’ performance.
Students are more engaged and active in their studies when they feel in command and protected in the classroom. Teachers play an essential role in influencing student academic motivation, school commitment, and disengagement. In studies on K-12 education, teacher-student relationships have been identified [ 24 ]. Positive teacher-student connections improve both teacher attitudes and academic performance.
There is a significant relationship between students’ understanding and students’ satisfaction .
Instructors must create well-structured courses, regularly present in their classes, and encourage student participation. When learning objectives are completed, students better understand the course’s success and learning expectations. “Constructing meaning from verbal, written, and graphic signals by interpreting, exemplifying, classifying, summarizing, inferring, comparing, and explaining” is how understanding is characterized [ 25 ].
There is a significant relationship between students’ performance and student’s academic achievement .
Academic emotions are linked to students’ performance, academic success, personality, and classroom background [ 26 ]. Understanding the elements that may influence student performance has long been a goal for educational institutions, students, and teachers.
There is a significant relationship between students’ understanding and students’ academic achievement .
Modular education views each student as an individual with distinct abilities and interests. To provide an excellent education, a teacher must adapt and individualize the educational curriculum for each student. Individual learning may aid in developing a variety of exceptional and self-reliant attributes [ 27 ]. Academic achievement is the current level of learning in the Philippines [ 28 ].
There is a significant relationship between students’ performance and students’ satisfaction .
Academic success is defined as a student’s intellectual development, including formative and summative assessment data, coursework, teacher observations, student interaction, and time on a task [ 29 ]. Students were happier with course technology, the promptness with which content was shared with the teacher, and their overall wellbeing [ 30 ].
There is a significant relationship between students’ academic achievement and students’ perceived effectiveness .
Student satisfaction is a short-term mindset based on assessing students’ educational experiences [ 29 ]. The link between student satisfaction and academic achievement is crucial in today’s higher education: we discovered that student satisfaction with course technical components was linked to a higher relative performance level [ 31 ].
There is a significant relationship between students’ satisfaction and students’ perceived effectiveness.
There is a strong link between student satisfaction and their overall perception of learning. A satisfied student is a direct effect of a positive learning experience. Perceived learning results had a favorable impact on student satisfaction in the classroom [ 32 ].
2. Materials and Methods
2.1. participants.
The principal area under study was San Jose, Occidental Mindoro, although other locations were also accepted. The survey took place between February and March 2022, with the target population of K-12 students in Junior and Senior High Schools from grades 7 to 12, aged 12 to 20, who are now implementing the Modular Approach in their studies during the COVID-19 pandemic. A 45-item questionnaire was created and circulated online to collect the information. A total of 300 online surveys was sent out and 252 online forms were received, a total of 84% response rate [ 33 ]. According to several experts, the sample size for Structural Equation Modeling (SEM) should be between 200 and 500 [ 34 ].
2.2. Questionnaire
The theoretical framework developed a self-administered test. The researcher created the questionnaire to examine and discover the probable cognitive capacity influencing K-12 students’ academic achievement in different parts of Occidental Mindoro during this pandemic as well as their satisfaction with modular distance learning. The questionnaire was designed through Google drive as people’s interactions are limited due to the effect of the COVID-19 pandemic. The questionnaire’s link was sent via email, Facebook, and other popular social media platforms.
The respondents had to complete two sections of the questionnaire. The first is their demographic information, including their age, gender, and grade level. The second is about their perceptions of modular learning. The questionnaire is divided into 12 variables: (1) Student’s Background, (2) Student’s Experience, (3) Student’s Behavior, (4) Student’s Instructor Interaction, (5) Student’s Performance, (6) Student’s Understanding, (7) Student’s Satisfaction, (8) Student’s Academic Achievement, and (9) Student’s Perceived Effectiveness. A 5-point Likert scale was used to assess all latent components contained in the SEM shown in Table 1 .
The construct and measurement items.
2.3. Structural Equation Modeling (SEM)
All the variables have been adapted from a variety of research in the literature. The observable factors were scored on a Likert scale of 1–5, with one indicating “strongly disagree” and five indicating “strongly agree”, and the data were analyzed using AMOS software. Theoretical model data were confirmed by Structural Equation Modeling (SEM). SEM is more suitable for testing the hypothesis than other methods [ 53 ]. There are many fit indices in the literature, of which the most commonly used are: CMIN/DF, Comparative Fit Index (CFI), AGFI, GFI, and Root Mean Square Error (RMSEA). Table 2 demonstrates the Good Fit Values and Acceptable Fit Values of the fit indices, respectively. AGFI and GFI are based on residuals; when sample size increases, the value of the AGFI also increase. It takes a value between 0 and 1. The fit is good if the value is more significant than 0.80. GFI is a model index that spans from 0 to 1, with values above 0.80 deemed acceptable. An RMSEA of 0.08 or less suggests a good fit [ 54 ], and a value of 0.05 to 0.08 indicates an adequate fit [ 55 ].
Acceptable Fit Values.
3. Results and Discussion
Figure 2 demonstrates the initial SEM for the cognitive factors of Modular Distance learning towards academic achievements and satisfaction of K-12 students during the COVID-19 pandemic. According to the figure below, three hypotheses were not significant: Students’ Behavior to Students’ Instructor Interaction (Hypothesis 3), Students’ Understanding of Students’ Academic Achievement (Hypothesis 11), and Students’ Performance to Students’ Satisfaction (Hypothesis 12). Therefore, a revised SEM was derived by removing this hypothesis in Figure 3 . We modified some indices to enhance the model fit based on previous studies using the SEM approach [ 47 ]. Figure 3 demonstrates the final SEM for evaluating cognitive factors affecting academic achievements and satisfaction and the perceived effectiveness of K-12 students’ response to Modular Learning during COVID-19, shown in Table 3 . Moreover, Table 4 demonstrates the descriptive statistical results of each indicator.

Initial SEM with indicators for evaluating the cognitive factors of modular distance learning towards academic achievements and satisfaction of K-12 students during COVID-19 pandemic.

Revised SEM with indicators for evaluating the cognitive factors of modular distance learning towards academic achievements and satisfaction of K-12 students during the COVID-19 pandemic.
Summary of the Results.
Descriptive statistic results.
The current study was improved by Moore’s transactional distance theory (TDT) and Bloom’s taxonomy theory (BTT) to evaluate cognitive factors affecting academic achievements and satisfaction and the perceived effectiveness of K-12 students’ response toward modular learning during COVID-19. SEM was utilized to analyze the correlation between Student Background (SB), Student Experience (SE), Student Behavior (SBE), Student Instructor Interaction (SI), Student Performance (SP), Student Understanding (SAU), Student Satisfaction (SS), Student’s Academic achievement (SAA), and Student’s Perceived effectiveness (SPE). A total of 252 data samples were acquired through an online questionnaire.
According to the findings of the SEM, the students’ background in modular learning had a favorable and significant direct effect on SE (β: 0.848, p = 0.009). K-12 students should have a background and knowledge in modular systems to better experience this new education platform. Putting the students through such an experience would support them in overcoming all difficulties that arise due to the limitations of the modular platforms. Furthermore, SEM revealed that SE had a significant adverse impact on SI (β: 0.843, p = 0.009). The study shows that students who had previous experience with modular education had more positive perceptions of modular platforms. Additionally, students’ experience with modular distance learning offers various benefits to them and their instructors to enhance students’ learning experiences, particularly for isolated learners.
Regarding the Students’ Interaction—Instructor, it positively impacts SAU (β: 0.873, p = 0.007). Communication helps students experience positive emotions such as comfort, satisfaction, and excitement, which aim to enhance their understanding and help them attain their educational goals [ 62 ]. The results revealed that SP substantially impacted SI (β: 0.765; p = 0.005). A student becomes more academically motivated and engaged by creating and maintaining strong teacher-student connections, which leads to successful academic performance.
Regarding the Students’ Understanding Response, the results revealed that SAA (β: 0.307; p = 0.052) and SS (β: 0.699; p = 0.008) had a substantial impact on SAU. Modular teaching is concerned with each student as an individual and with their specific capability and interest to assist each K-12 student in learning and provide quality education by allowing individuality to each learner. According to the Department of Education, academic achievement is the new level for student learning [ 63 ]. Meanwhile, SAA was significantly affected by the Students’ Performance Response (β: 0.754; p = 0.014). It implies that a positive performance can give positive results in student’s academic achievement, and that a negative performance can also give negative results [ 64 ]. Pekrun et al. (2010) discovered that students’ academic emotions are linked to their performance, academic achievement, personality, and classroom circumstances [ 26 ].
Results showed that students’ academic achievement significantly positively affects SPE (β: 0.237; p = 0.024). Prior knowledge has had an indirect effect on academic accomplishment. It influences the amount and type of current learning system where students must obtain a high degree of mastery [ 65 ]. According to the student’s opinion, modular distance learning is an alternative solution for providing adequate education for all learners and at all levels in the current scenario under the new education policy [ 66 ]. However, the SEM revealed that SS significantly affected SPE (β: 0.868; p = 0.009). Students’ perceptions of learning and satisfaction, when combined, can provide a better knowledge of learning achievement [ 44 ]. Students’ perceptions of learning outcomes are an excellent predictor of student satisfaction.
Since p -values and the indicators in Students’ Behavior are below 0.5, therefore two paths connecting SBE to students’ interaction—instructor (0.155) and students’ understanding (0.212) are not significant; thus, the latent variable Students’ Behavior has no effect on the latent variable Students’ Satisfaction and academic achievement as well as perceived effectiveness on modular distance learning of K12 students. This result is supported by Samsen-Bronsveld et al. (2022), who revealed that the environment has no direct influence on the student’s satisfaction, behavior engagement, and motivation to study [ 67 ]. On the other hand, the results also showed no significant relationship between Students’ Performance and Students’ Satisfaction (0.602) because the correlation p -values are greater than 0.5. Interestingly, this result opposed the other related studies. According to Bossman & Agyei (2022), satisfaction significantly affects performance or learning outcomes [ 68 ]. In addition, it was discovered that the main drivers of the students’ performance are the students’ satisfaction [ 64 , 69 ].
The result of the study implies that the students’ satisfaction serves as the mediator between the students’ performance and the student-instructor interaction in modular distance learning for K-12 students [ 70 ].
Table 5 The reliabilities of the scales used, i.e., Cronbach’s alphas, ranged from 0.568 to 0.745, which were in line with those found in other studies [ 71 ]. As presented in Table 6 , the IFI, TLI, and CFI values were greater than the suggested cutoff of 0.80, indicating that the specified model’s hypothesized construct accurately represented the observed data. In addition, the GFI and AGFI values were 0.828 and 0.801, respectively, indicating that the model was also good. The RMSEA value was 0.074, lower than the recommended value. Finally, the direct, indirect, and total effects are presented in Table 7 .
Construct Validity Model.
Direct effect, indirect effect, and total effect.
Table 6 shows that the five parameters, namely the Incremental Fit Index, Tucker Lewis Index, the Comparative Fit Index, Goodness of Fit Index, and Adjusted Goodness Fit Index, are all acceptable with parameter estimates greater than 0.8, whereas mean square error is excellent with parameter estimates less than 0.08.
4. Conclusions
The education system has been affected by the 2019 coronavirus disease; face-to-face classes are suspended to control and reduce the spread of the virus and infections [ 2 ]. The suspension of face-to-face classes results in the application of modular distance learning for K-12 students according to continuity of learning during the COVID-19 pandemic. With the outbreak of COVID-19, some issues concerning students’ academic Performance and factors associated with students’ psychological status are starting to emerge, which impacted the students’ ability to learn. This study aimed to perceive the impact of Modular Distance learning on the K-12 students amid the COVID-19 pandemic and assess cognitive factors affecting students’ academic achievement and satisfaction.
This study applied Transactional Distance Theory (TDT) and Bloom Taxonomy Theory (BTT) to evaluate cognitive factors affecting students’ academic achievements and satisfaction and evaluate the perceived effectiveness of K-12 students in response to modular learning. This study applied Structural Equation Modeling (SEM) to test hypotheses. The application of SEM analyzed the correlation among students’ background, experience, behavior, instructor interaction, performance, understanding, satisfaction, academic achievement, and student perceived effectiveness.
A total of 252 data samples were gathered through an online questionnaire. Based on findings, this study concludes that students’ background in modular distance learning affects their behavior and experience. Students’ experiences had significant effects on the performance and understanding of students in modular distance learning. Student instructor interaction had a substantial impact on performance and learning; it explains how vital interaction with the instructor is. The student interacting with the instructor shows that the student may receive feedback and guidance from the instructor. Understanding has a significant influence on students’ satisfaction and academic achievement. Student performance has a substantial impact on students’ academic achievement and satisfaction. Perceived effectiveness was significantly influenced by students’ academic achievement and student satisfaction. However, students’ behavior had no considerable effect on students’ instructor interaction, and students’ understanding while student performance equally had no significant impact on student satisfaction. From this study, students are likely to manifest good performance, behavior, and cognition when they have prior knowledge with regard to modular distance learning. This study will help the government, teachers, and students take the necessary steps to improve and enhance modular distance learning that will benefit students for effective learning.
The modular learning system has been in place since its inception. One of its founding metaphoric pillars is student satisfaction with modular learning. The organization demonstrated its dedication to the student’s voice as a component of understanding effective teaching and learning. Student satisfaction research has been transformed by modular learning. It has caused the education research community to rethink long-held assumptions that learning occurs primarily within a metaphorical container known as a “course.” When reviewing studies on student satisfaction from a factor analytic perspective, one thing becomes clear: this is a complex system with little consensus. Even the most recent factor analytical studies have done little to address the lack of understanding of the dimensions underlying satisfaction with modular learning. Items about student satisfaction with modular distance learning correspond to forming a psychological contract in factor analytic studies. The survey responses are reconfigured into a smaller number of latent (non-observable) dimensions that the students never really articulate but are fully expected to satisfy. Of course, instructors have contracts with their students. Studies such as this one identify the student’s psychological contact after the fact, rather than before the class. The most important aspect is the rapid adoption of this teaching and learning mode in Senior High School. Another balancing factor is the growing sense of student agency in the educational process. Students can express their opinions about their educational experiences in formats ranging from end-of-course evaluation protocols to various social networks, making their voices more critical.
Furthermore, they all agreed with latent trait theory, which holds that the critical dimensions that students differentiate when expressing their opinions about modular learning are formed by the combination of the original items that cannot be directly observed—which underpins student satisfaction. As stated in the literature, the relationship between student satisfaction and the characteristic of a psychological contract is illustrated. Each element is translated into how it might be expressed in the student’s voice, and then a contract feature and an assessment strategy are added. The most significant contributor to the factor pattern, engaged learning, indicates that students expect instructors to play a facilitative role in their teaching. This dimension corresponds to the relational contract, in which the learning environment is stable and well organized, with a clear path to success.
5. Limitations and Future Work
This study was focused on the cognitive capacity of modular distance learning towards academic achievements and satisfaction of K-12 students during the COVID-19 pandemic. The sample size in this study was small, at only 252. If this study is repeated with a larger sample size, it will improve the results. The study’s restriction was to the province of Occidental Mindoro; Structural Equation Modeling (SEM) was used to measure all the variables. Thus, this will give an adequate solution to the problem in the study.
The current study underlines that combining TDT and BTT can positively impact the research outcome. The contribution the current study might make to the field of modular distance learning has been discussed and explained. Based on this research model, the nine (9) factors could broadly clarify the students’ adoption of new learning environment platform features. Thus, the current research suggests that more investigation be carried out to examine relationships among the complexity of modular distance learning.
Funding Statement
This research received no external funding.
Author Contributions
Data collection, methodology, writing and editing, K.A.M.; data collection, writing—review and editing, Y.-T.J. and C.S.S. All authors have read and agreed to the published version of the manuscript.
Institutional Review Board Statement
Informed consent statement.
Informed consent was obtained from all subjects involved in the study.
Data Availability Statement
Conflicts of interest.
The authors declare no conflict of interest.
Publisher’s Note: MDPI stays neutral with regard to jurisdictional claims in published maps and institutional affiliations.
Loading metrics
Open Access
Peer-reviewed
Research Article
Neural Modularity Helps Organisms Evolve to Learn New Skills without Forgetting Old Skills
Affiliation Department of Computer and Information Science, Norwegian University of Science and Technology, Trondheim, Norway
Affiliations Sorbonne Université UPMC Univ Paris 06, UMR 7222, ISIR, Paris, France, CNRS, UMR 7222, ISIR, Paris, France
* E-mail: [email protected]
Affiliation Computer Science Department, University of Wyoming, Laramie, Wyoming, United States of America
- Kai Olav Ellefsen,
- Jean-Baptiste Mouret,
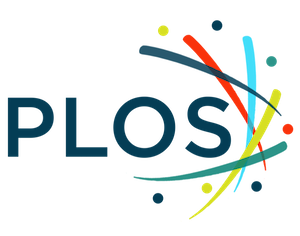
- Published: April 2, 2015
- https://doi.org/10.1371/journal.pcbi.1004128
- Reader Comments
A long-standing goal in artificial intelligence is creating agents that can learn a variety of different skills for different problems. In the artificial intelligence subfield of neural networks, a barrier to that goal is that when agents learn a new skill they typically do so by losing previously acquired skills, a problem called catastrophic forgetting . That occurs because, to learn the new task, neural learning algorithms change connections that encode previously acquired skills. How networks are organized critically affects their learning dynamics. In this paper, we test whether catastrophic forgetting can be reduced by evolving modular neural networks. Modularity intuitively should reduce learning interference between tasks by separating functionality into physically distinct modules in which learning can be selectively turned on or off. Modularity can further improve learning by having a reinforcement learning module separate from sensory processing modules, allowing learning to happen only in response to a positive or negative reward. In this paper, learning takes place via neuromodulation, which allows agents to selectively change the rate of learning for each neural connection based on environmental stimuli (e.g. to alter learning in specific locations based on the task at hand). To produce modularity, we evolve neural networks with a cost for neural connections. We show that this connection cost technique causes modularity, confirming a previous result, and that such sparsely connected, modular networks have higher overall performance because they learn new skills faster while retaining old skills more and because they have a separate reinforcement learning module. Our results suggest (1) that encouraging modularity in neural networks may help us overcome the long-standing barrier of networks that cannot learn new skills without forgetting old ones, and (2) that one benefit of the modularity ubiquitous in the brains of natural animals might be to alleviate the problem of catastrophic forgetting.
Author Summary
A long-standing goal in artificial intelligence (AI) is creating computational brain models (neural networks) that learn what to do in new situations. An obstacle is that agents typically learn new skills only by losing previously acquired skills. Here we test whether such forgetting is reduced by evolving modular neural networks, meaning networks with many distinct subgroups of neurons. Modularity intuitively should help because learning can be selectively turned on only in the module learning the new task. We confirm this hypothesis: modular networks have higher overall performance because they learn new skills faster while retaining old skills more. Our results suggest that one benefit of modularity in natural animal brains may be allowing learning without forgetting.
Citation: Ellefsen KO, Mouret J-B, Clune J (2015) Neural Modularity Helps Organisms Evolve to Learn New Skills without Forgetting Old Skills. PLoS Comput Biol 11(4): e1004128. https://doi.org/10.1371/journal.pcbi.1004128
Editor: Josh C. Bongard, University of Vermont, UNITED STATES
Received: September 17, 2014; Accepted: January 14, 2015; Published: April 2, 2015
Copyright: © 2015 Ellefsen et al. This is an open access article distributed under the terms of the Creative Commons Attribution License , which permits unrestricted use, distribution, and reproduction in any medium, provided the original author and source are credited
Data Availability: The exact source code and experimental configuration files used in our experiments, along with data from all our experiments, are freely available in the online Dryad scientific archive at http://dx.doi.org/10.5061/dryad.s38n5 .
Funding: KOE and JC have no specific financial support for this work. JBM is supported by an ANR young researchers grant (Creadapt, ANR-12-JS03-0009). URL: http://www.agence-nationale-recherche.fr/en/funding-opportunities/documents/aap-en/generic-call-for-proposals-2015-2015/nc/ . The funders had no role in study design, data collection and analysis, decision to publish, or preparation of the manuscript.
Competing interests: The authors have declared that no competing interests exist.
Introduction
A long-standing scientific challenge is to create agents that can learn , meaning they can adapt to novel situations and environments within their lifetime. The world is too complex, dynamic, and unpredictable to program all beneficial strategies ahead of time, which is why robots, like natural animals, need to be able to continuously learn new skills on the fly.
Having robots learn a large set of skills, however, has been an elusive challenge because they need to learn new skills without forgetting previously acquired skills [ 1 – 3 ]. Such forgetting is especially problematic in fields that attempt to create artificial intelligence in brain models called artificial neural networks [ 1 , 4 , 5 ]. To learn new skills, neural network learning algorithms change the weights of neural connections [ 6 – 8 ], but old skills are lost because the weights that encoded old skills are changed to improve performance on new tasks. This problem is known as catastrophic forgetting [ 9 , 10 ] to emphasize that it contrasts with biological animals (including humans), where there is gradual forgetting of old skills as new skills are learned [ 11 ]. While robots and artificially intelligent software agents have the potential to significantly help society [ 12 – 14 ], their benefits will be extremely limited until we can solve the problem of catastrophic forgetting [ 1 , 15 ]. To advance our goal of producing sophisticated, functional artificial intelligence in neural networks and make progress in our long-term quest to create general artificial intelligence with them, we need to develop algorithms that can learn how to handle more than a few different problems. Additionally, the difference between computational brain models and natural brains with respect to catastrophic forgetting limits the usefulness of such models as tools to study neurological pathologies [ 16 ].
In this paper, we investigate the hypothesis that modularity, which is widespread in biological neural networks [ 17 – 21 ], helps reduce catastrophic forgetting in artificial neural networks. Modular networks are those that have many clusters (modules) of highly connected neurons that are only sparsely connected to neurons in other modules [ 19 , 22 , 23 ]. The intuition behind this hypothesis is that modularity could allow learning new skills without forgetting old skills because learning can be selectively turned on only in modules learning a new task ( Fig. 1 , top). Selective regulation of learning occurs in natural brains via neuromodulation [ 24 ], and we incorporate an abstraction of it in our model [ 25 ]. We also investigate a second hypothesis: that modularity can improve skill learning by separating networks into a skill module and a reward module , resulting in more precise control of learning ( Fig. 1 , bottom).
- PPT PowerPoint slide
- PNG larger image
- TIFF original image
Hypothesis 1: Evolving non-modular networks leads to the forgetting of old skills as new skills are learned. Evolving networks with a pressure to minimize connection costs leads to modular solutions that can retain old skills as new skills are learned. Hypothesis 2: Evolving modular networks makes reward-based learning easier, because it allows a clear separation of reward signals and learned skills. We present evidence for both hypotheses in this paper.
https://doi.org/10.1371/journal.pcbi.1004128.g001
To evolve modular networks, we add another natural phenomenon: costs for neural connections. In nature, there are many costs associated with neural connections (e.g. building them, maintaining them, and housing them) [ 26 – 28 ] and it was recently demonstrated that incorporating a cost for such connections encourages the evolution of modularity in networks [ 23 ]. Our results support the hypothesis that modularity does mitigate catastrophic forgetting: modular networks have higher overall performance because they learn new skills faster while retaining old skills more. Additional research into this area, including investigating the generality of our results, will catalyze research on creating artificial intelligence, improve models of neural learning, and shed light on whether one benefit of modularity in natural animal brains is an improved ability to learn without forgetting.
Catastrophic forgetting.
Catastrophic forgetting (also called catastrophic interference) has been identified as a problem for artificial neural networks (ANNs) for over two decades: When learning multiple tasks in a sequence, previous skills are forgotten rapidly as new information is learned [ 9 , 10 ]. The problem occurs because learning algorithms only focus on solving the current problem and change any connections that will help solve that problem, even if those connections encoded skills appropriate to previously encountered problems [ 9 ].
Many attempts have been made to mitigate catastrophic forgetting. Novelty vectors modify the backpropagation learning algorithm [ 7 ] to limit the number of connections that are changed in the network based on how novel, or unexpected, the input pattern is [ 29 ]. This technique is only applicable for auto-encoder networks (networks whose target output is identical to their input), thus limiting its value as a general solution to catastrophic forgetting [ 1 ]. Orthogonalization techniques mitigate interference between tasks by reducing their representational overlap in input neurons (via manually designed preprocessing) and by encouraging sparse hidden-neuron activations [ 30 – 32 ]. Interleaved learning avoids catastrophic forgetting by training on both old and new data when learning [ 10 ], although this method cannot scale and does not work for realistic environments because in the real world not all challenges are faced concurrently [ 33 , 34 ]. This problem with interleaved learning can be reduced with pseudo rehearsal , wherein input-output associations from old tasks are remembered and rehearsed [ 34 ]. However, scaling remains an issue with pseudo rehearsal because such associations still must be stored and choosing which associations to store is an unsolved problem [ 15 ]. These techniques are all engineered approaches to reducing the problem of catastrophic forgetting and are not proposed as methods by which natural evolution solved the problem of catastrophic forgetting [ 1 , 10 , 29 – 32 , 34 ].
Dual-net architectures , on the other hand, present a biologically plausible [ 35 ] mechanism for limiting catastrophic forgetting [ 33 , 36 ]. The technique, inspired by theories on how human brains separate and subsequently integrate old and new knowledge, partitions early processing and long-term storage into different subnetworks. Similar to interleaved learning techniques, dual-net architectures enable both new knowledge and input history (in the form of current network state) to affect learning.
Although these methods have been suggested for reducing catastrophic forgetting, many questions remain about how animals avoid this problem [ 1 ] and which mechanisms can help avoid it in neural networks [ 1 , 15 ]. In this paper, we study a new hypothesis, which is that modularity can help avoid catastrophic forgetting. Unlike the techniques mentioned so far, our solution does not require human design, but is automatically generated by evolution. Evolving our solution under biologically realistic constraints has the added benefit of suggesting how such a mechanism may have originated in nature.
Evolving neural networks that learn.
One method for setting the connection weights of neural networks is to evolve them, meaning that an evolutionary algorithm specifies each weight, and the weight does not change within an organism’s “lifetime” [ 5 , 37 – 39 ]. Evolutionary algorithms abstract Darwinian evolution: in each generation a population of “organisms” is subjected to selection (for high performance) and then mutation (and possibly crossover) [ 5 , 38 ]. These algorithms have shown impressive performance—often outperforming human engineers [ 40 , 41 ]—on a range of tasks, such as measuring properties in quantum physics [ 12 ], dynamic rocket guidance [ 42 ], and robot locomotion [ 43 , 44 ].
Another approach to determining the weights of neural networks is to initialize them randomly and then allow them to change via a learning algorithm [ 5 , 7 , 45 ]. Some learning algorithms, such as backpropagation [ 6 , 7 ], require a correct output (e.g. action) for each input. Other learning algorithms are considered more biologically plausible in that they involve only information local to each neuron (e.g. Hebb’s rule [ 45 ]) or infrequent reward signals [ 8 , 46 , 47 ].
Evolution and learning can be combined, wherein evolution creates an initial neural network and then a learning algorithm modifies its connections within the lifetime of the organism [ 5 , 37 , 47 – 49 ]. Compared to behaviors defined solely by evolution, evolving agents that learn leads to better solutions in fewer generations [ 48 , 50 , 51 ], improved adaptability to changing environments [ 48 , 49 ], and enables evolving solutions for larger neural networks [ 48 ]. Computational studies of evolving agents that learn have also shed light on open biological questions regarding the interactions between evolution and learning [ 50 , 52 , 53 ].
The idea of using evolutionary computation to reduce catastrophic forgetting has not been widely explored. In one relevant paper, evolution optimized certain parameters of a neural network to mitigate catastrophic forgetting [ 15 ]. Such parameters included the number of hidden (internal) neurons, learning rates, patterns of connectivity, initial weights, and output error tolerances. That paper did show that there is a potential for evolution to generate a stronger resistance to catastrophic forgetting, but did not investigate the role of modularity in helping produce such a resistance.
Neuromodulatory learning in neural networks.
Evolutionary experiments on artificial neural networks typically model only the classic excitatory and inhibitory actions of neurons in the brain [ 5 ]. In addition to these processes, biological brains employ a number of different neuromodulators , which are chemical signals that can locally modify learning [ 24 , 54 , 55 ]. By allowing evolution to design neuromodulatory dynamics, learning rates for particular synapses can be upregulated and downregulated in response to certain inputs from the environment. These additional degrees of freedom greatly increase the possible complexity of reward-based learning strategies. This type of plasticity-controlling neuromodulation has been successfully applied when evolving neural networks that solve reinforcement learning problems [ 25 , 46 ], and a comparison found that evolution was able to solve more complex tasks with neuromodulated Hebbian learning than with Hebbian learning alone [ 25 ]. Our experiments include this form of neuromodulation ( Methods ).
Evolved modularity in neural networks.
Modularity is ubiquitous in biological networks, including neural networks, genetic regulatory networks, and protein interaction networks [ 17 – 21 ]. Why modularity evolved in such networks has been a long-standing area of research [ 18 – 20 , 56 – 59 ]. Researchers have also long studied how to encourage the evolution of modularity in artificial neural networks, usually by creating the conditions that are thought to promote modularity in natural evolution [ 19 , 57 – 61 ]. Several different hypotheses have been suggested for the evolutionary origins of modularity.
A leading hypothesis has been that modularity emerges when evolution occurs in rapidly changing environments that have common subproblems, but different overall problems [ 57 ]. These environments are said to have modularly varying goals . While such environments can promote modularity [ 57 ], the effect only appears for certain frequencies of environmental change [ 23 ] and can fail to appear with different types of networks [ 58 , 60 , 61 ]. Moreover, it is unclear how many natural environments change modularly and how to design training problems for artificial neural networks that have modularly varying goals. Other experiments have shown that modularity may arise from gene duplication and differentiation [ 19 ], or that it may evolve to make networks more robust to noise in the genotype-phenotype mapping [ 58 ] or to reduce interference between network activity patterns [ 59 ].
Recently, a different cause of module evolution was documented: that modularity evolves when there are costs for connections in networks [ 23 ]. This explanation for the evolutionary origins of modularity is biologically plausible because biological networks have connection costs (e.g. to build connections, maintain them, and house them) and there is evidence that natural selection optimally arranges neurons to minimize these connection costs [ 26 , 27 ]. Moreover, the modularity-inducing effects of adding a connection cost were shown to occur in a wide range of environments, suggesting that adding a selection pressure to reduce connection costs is a robust, general way to encourage modularity [ 23 ]. We apply this technique in our paper because of its efficacy and because it may be a main reason that modularity evolves in natural networks.
Experimental Setup
To test our hypotheses, we set up an environment in which there is a potential for catastrophic forgetting and where individuals able to avoid this forgetting receive a higher evolutionary fitness , meaning they are more likely to reproduce. The environment is an abstraction of a world in which an organism performs a daily routine of trying to eat nutritious food while avoiding eating poisonous food. Every day the organism observes every food item one time: half of the food items are nutritious and half are poisonous. To achieve maximum fitness, the individual needs to eat all the nutritious items and avoid eating the poisonous ones. After a number of days, the season changes abruptly from a summer season to a winter season. In the new season, there is a new set of food sources, half of them nutritious and half poisonous, and the organism has to learn which is which. After this winter season, the environment changes back to the summer season and the food items and their nutritious/poisonous statuses are the same as in the previous summer. The environment switches back and forth between these two seasons multiple times in the organism’s lifetime. Individuals that remember each season’s food associations perform better by avoiding poisonous items without having to try them first.
We consider each pair of a summer and winter season a year . Every season lasts for five days , and in each day an individual encounters all four food items for that season in a random order. A lifetime is three years ( Fig. 2 ). To ensure that individuals must learn associations within their lifetimes instead of having genetically hardcoded associations [ 47 , 62 ], in each lifetime two food items are randomly assigned as nutritious and the other two food items are assigned as poisonous ( Fig. 3 ). To select for general learners rather than individuals that by chance do well in a specific environment, performance is averaged over four random environments (lifetimes) for each individual during evolution, and over 80 random environments (lifetimes) when assessing the performance of final, end-of-experiment individuals ( Methods ).
A lifetime lasts 3 years. Each year has 2 seasons: winter and summer. Each season consists of 5 days. In each day, each individual sees all food items available in that season (only two are shown) in a random order.
https://doi.org/10.1371/journal.pcbi.1004128.g002
To ensure that agents learn associations within their lifetimes instead of genetically hardcoding associations, whether each food item is nutritious or poisonous is randomized each generation. There are four food items per season (two are depicted).
https://doi.org/10.1371/journal.pcbi.1004128.g003
This environment selects for agents that can avoid forgetting old information as they learn new, unrelated information. For instance, if an agent is able to avoid forgetting the summer associations during the winter season, it will immediately perform well when summer returns, thus outcompeting agents that have to relearn summer associations. Agents that forget, especially catastrophically, are therefore at a selective disadvantage.
Our main results were found to be robust to variations in several of our experimental parameters, including changes to the number of years in the organism’s lifetime, the number of different seasons per year, the number of different edible items, and different representations of the inputs (the presence of items being represented either by a single input or distributed across all inputs for a season). We also observed that our results are robust to lengthening the number of days per season: networks in the experimental treatment (called “P&CC” for reasons described below) significantly outperform the networks in the control (“PA”) treatment ( p < 0.05) even when doubling or quadrupling the number of days per season, although the size of the difference diminished in longer seasons.
Neural network model.
The model of the organism’s brain is a neural network with 10 input neurons (Supp. S1 Fig ). From left to right, inputs 1-4 and 5-8 encode which summer and winter food item is present, respectively. During summer, the winter inputs are never active and vice versa. Catastrophic forgetting may appear in these networks because a non-modular neural network is likely to use the same hidden neurons for both seasons ( Fig. 1 , top). We segmented the summer and winter items into separate input neurons to abstract a neural network responsible for an intermediate phase of cognition, where early visual processing and object recognition have already occurred, but before decisions have been made about what to do in response to the recognized visual stimuli. Such disentangled representations of objects have been identified in animal brains [ 63 ] and are common at intermediate layers of neural network models [ 64 ]. The final two inputs are for reinforcement learning: inputs 9 and 10 are reward and punishment signals that fire when a nutritious or poisonous food item is eaten, respectively. The network has a single output that determines if the agent will eat ( output > 0) or ignore ( output < = 0) the presented food item.
Associations can be learned by properly connecting reward signals through neuromodulatory neurons to non-modulatory neurons that determine which actions to take in response to food items ( Methods ). Evolution determines the neural wiring that produces learning dynamics, as described next.
Evolutionary algorithm.
Evolution begins with a randomly generated population of neural networks. The performance of each network is evaluated as described above. More fit networks tend to have more offspring, with fitness being determined differently in each treatment, as explained below. Offspring are generated by copying a parent genome and mutating it by adding or removing connections, changing the strength of connections, and switching neurons from being modulatory to non-modulatory or vice versa. The process repeats for 20,000 generations.
To evolve modular neural networks, we followed a recently demonstrated procedure where modularity evolves as a byproduct of a selection pressure to reduce neural connectivity [ 23 ]. We compared a treatment where the fitness of individuals was based on performance alone (PA) to one based on both maximizing performance and minimizing connection costs (P&CC). Specifically, evolution proceeds according to a multi-objective evolutionary algorithm with one (PA) or two (P&CC) primary objectives. A network’s connection cost equals its number of connections, following [ 23 ]. More details on the evolutionary algorithm can be found in Methods.
A Connection Cost Increases Performance and Modularity
The addition of a cost for connections (the P&CC treatment) leads to a rapid, sustained, and statistically significant fitness advantage versus not having a connection cost (the PA treatment) ( Fig. 4 ). In addition to overall performance across generations, we looked at the day-to-day performance of final, evolved individuals ( Fig. 5 ). P&CC networks learn associations faster in their first summer and winter, and maintain higher performance over multiple years (pairs of seasons).
Modularity is measured via a widely used approximation of the standard Q modularity score [ 23 , 57 , 65 , 67 ] ( Methods ). For each treatment, the median from 100 independent evolution experiments is shown ± 95% bootstrapped confidence intervals of the median ( Methods ). Asterisks below each plot indicate statistically significant differences at p < 0.01 according to the Mann-Whitney U test, which is the default statistical test throughout this paper unless otherwise specified.
https://doi.org/10.1371/journal.pcbi.1004128.g004
Plotted is median performance per day (± 95% bootstrapped confidence intervals of the median) measured across 100 organisms (the highest-performing organism from each experiment per treatment) tested in 80 new environments (lifetimes) with random associations ( Methods ). P&CC networks significantly outperform PA networks on every day (asterisks). Eating no items or all items produces a score of 0.5; eating all and only nutritious food items achieves the maximum score of 1.0.
https://doi.org/10.1371/journal.pcbi.1004128.g005
The presence of a connection cost also significantly increases network modularity ( Fig. 4 ), confirming the finding of Clune et al. [ 23 ] in this different context of networks with within-life learning. Networks evolved in the P&CC treatment tend to create a separate reinforcement learning module that contains the reward and punishment inputs and most or all neuromodulatory neurons ( Fig. 6 ). One of our hypotheses ( Fig. 1 , bottom) suggested that such a separation could improve the efficiency of learning, by regulating learning (via neuromodulatory neurons) in response to whether the network performed a correct or incorrect action, and applying that learning to downstream neurons that determine which action should be taken in response to input stimuli.
Dark blue nodes are inputs that encode which type of food has been encountered. Light blue nodes indicate internal, non-modulatory neurons. Red nodes are reward or punishment inputs that indicate if a nutritious or poisonous item has been eaten. Orange neurons are neuromodulatory neurons that regulate learning. P&CC networks tend to separate the reward/punishment inputs and neuromodulatory neurons into a separate module that applies learning to downstream neurons that determine which actions to take. For each treatment, the highest-performing network from each of the nine highest-performing evolution experiments are shown (all are shown in the Supporting Information). In each panel, the left number reports performance and the right number reports modularity. We follow the convention from [ 23 ] of placing nodes in the way that minimizes the total connection length.
https://doi.org/10.1371/journal.pcbi.1004128.g006
To quantify whether learning is separated into its own module, we adopted a technique from [ 23 ], which splits a network into the most modular decomposition according to the modularity Q score [ 65 ]. We then measured the frequency with which the reinforcement inputs (reward/punishment signals) were placed into a different module from the remaining food-item inputs. This measure reveals that P&CC networks have a separate module for learning in 31% of evolutionary trials, whereas only 4% of the PA trials do, which is a significant difference ( p = 2.71 × 10 −7 ), in agreement with our hypothesis ( Fig. 1 , bottom). Analyses also reveal that the networks from both treatments that have a separate module for learning perform significantly better than networks without this decomposition (median performance of modular networks in 80 randomly generated environments ( Methods ): 0.87 [95% CI: 0.83, 0.88] vs. non-modular networks: 0.80 [0.71, 0.84], p = 0.02). Even though only 31% of the P&CC networks are deemed modular in this particular way, the remaining P&CC networks are still significantly more modular on average than PA networks (median Q scores are 0.25 [0.23, 0.28] and 0.2 [0.19, 0.22] respectively, p = 4.37 × 10 −6 ), suggesting additional ways in which modularity improves the performance of P&CC networks.
After observing that a connection cost significantly improves performance and modularity, we analyzed whether this increased performance can be explained by the increased modularity, or whether it may better correlate with network sparsity , since P&CC networks also have fewer connections (P&CC median number of connections is 35.5 [95% CI: 31.0, 40.0] vs. PA 82.0 [74.0, 97.1], p = 7.97 × 10 −19 ). Both sparsity and modularity are correlated with the performance of networks ( Fig. 7 ). Sparsity also correlates with modularity ( p = 5.15 × 10 −40 as calculated by a t -test of the hypothesis that the correlation is zero), as previously shown [ 23 , 66 ]. Our interpretation of the data is that the pressure for both functionality and sparsity causes modularity, which in turn helps evolve learners that are more resistant to catastrophic forgetting. However, it cannot be ruled out that sparsity itself mitigates catastrophic forgetting [ 1 ], or that the general learning abilities of the network have been improved due to the separation into a skill module and a learning module. Either way, the data support our hypothesis that a connection cost promotes the evolution of sparsity, modularity, and increased performance on learning tasks.
Black dots represent the highest-performing network from each of the 100 experiments from both the PA and P&CC treatments. Both the sparsity ( p = 1.08 × 10 −16 ) and modularity ( p = 1.19 × 10 −5 ) of networks significantly correlates with their performance. Performance was measured in 80 randomly generated environments ( Methods ). Significance was calculated by a t -test of the hypothesis that the correlation is zero. Notice that many of the lowest-performing networks are close to the maximum of 150 connections.
https://doi.org/10.1371/journal.pcbi.1004128.g007
Modular P&CC Networks Learn More and Forget Less
We next investigated whether the improved performance of P&CC individuals is because they forget less. Measuring the percent of information a network retains can be misleading, because networks that never learn anything are reported as never forgetting anything. In many PA experiments, networks did not learn in one or both seasons, which looks like perfect retention , but for the wrong reason: they do not forget anything because they never knew anything to begin with. To prevent such pathological, non-learning networks from clouding this analysis, we compared only the 50 highest-performing experiments from each treatment, instead of all 100 experiments. For both treatments, we then measured retention and forgetting in the highest-performing network from each of these 50 experiments.
To illuminate how old associations are forgotten and new ones are formed, we performed an experiment from studies of association forgetting in humans [ 11 ]: already evolved individuals learned one task and then began training on a new task, during which we measured how their performance on the original task degraded. Specifically, we allowed individuals to learn for 50 winter days—to allow even poor learners time to learn the winter associations—before exposing them to 20 summer days, during which we measured how rapidly they forgot winter associations and learned summer associations ( Methods ). Notice that individuals were evolved in seasons lasting only 5 days, but we measure learning and forgetting for 20 days in this analysis to study the longer-term consequences of the evolved learning architectures. Thus, the key result relevant to catastrophic forgetting is what occurs during the first five days. We included the remaining 15 days to show that the differences in performance persist if the seasons are extended.
P&CC networks retain higher performance on the original task when learning a new task ( Fig. 8 , left). They also learn the new task better ( Fig. 8 , center). The combined effect significantly improves performance ( Fig. 8 , right), meaning P&CC networks are significantly better at learning associations in a new season while retaining associations from a previous one.
P&CC networks, which are more modular, are better at retaining associations learned on a previous task (winter associations) while learning a new task (summer associations), better at learning new (summer) associations, and significantly better when measuring performance on both the associations for the original task (winter) and the new task (summer). Note that networks were evolved with five days per season, so the results during those first five days are the most informative regarding the evolutionary mitigation of catastrophic forgetting: we show additional days to reveal longer-term consequences of the evolved architectures. Solid lines show median performance and shaded areas indicate 95% bootstrapped confidence intervals of the median. The retention scores (left panel) are normalized relative to the original performance before training on the new task (an unnormalized version is provided as Supp. S6 Fig ). During all performance measurements, learning was disabled to prevent such measurements from changing an individual’s known associations ( Methods ).
https://doi.org/10.1371/journal.pcbi.1004128.g008
To further understand whether the increased performance of the P&CC individuals is because they learn more, retain more, or both, we counted the number of retained and learned associations for individuals in 80 randomly generated environments (lifetimes). If we regard performance in each season as a skill , this experiment measures whether the individuals can retain a previously-learned skill (perfect summer performance) after learning a new skill (perfect winter performance). We tested the knowledge of the individuals in the following way: at the end of each season, we counted the number of sets of associations (summer or winter) that individuals knew perfectly, which required them knowing the correct response for each food item in that season. We formulated four metrics that quantify how well individuals knew and retained associations.
The first metric (“ Perfect ”) measures the number of seasons an individual knew both sets of associations (summer and winter). Doing well on this metric indicates reduced catastrophic forgetting because it requires retaining an old skill even after a new one is learned. P&CC individuals learned significantly more Perfect associations ( Fig. 9 , Perfect).
P&CC individuals learn significantly more associations, whether counting only when the associations for both seasons are known (“Perfect” knowledge) or separately counting knowledge of either season’s association (total “Known”). P&CC networks also forget fewer associations, defined as associations known in one season and then forgotten in the next, which is significant when looking at the percent of known associations forgotten (“% Forgotten”). P&CC networks also retain significantly more associations, meaning they did not forget one season’s association when learning the next season’s association. See text for more information about the “Perfect”, “Known”, “Forgotten,” and “Retained” metrics. During all performance measurements, learning was disabled to prevent such measurements from changing an individual’s known associations ( Methods ). Bars show median performance, whiskers show the 95% bootstrapped confidence interval of the median. Two asterisks indicate p < 0.01, three asterisks indicate p < 0.001.
https://doi.org/10.1371/journal.pcbi.1004128.g009
The second metric (“ Known ”) is the sum of the number of seasons that summer associations were known and the number of seasons that winter associations were known. In other words, it counts knowing either season in a year and doubly counts knowing both. P&CC individuals learned significantly more of these Known associations ( Fig. 9 , Known).
The third metric counts the number of seasons in which an association was “ Forgotten ”, meaning an association was completely known in one season, but was not in the following season. There is no significant difference between treatments on this metric when measured in absolute numbers ( Fig. 9 , Forgotten). However, measured as a percentage of Known items, P&CC individuals forgot significantly fewer associations ( Fig. 9 , % Forgotten). The modular P&CC networks thus learned more and forgot less—leading to a significantly lower percentage of forgotten associations.
The final metric counts the number of seasons in which an association was “ Retained ”, meaning an association was completely known in one season and the following season. P&CC individuals retained significantly more than PA individuals, both in absolute numbers ( Fig. 9 , Retained) and as a percentage of the total number of known items ( Fig. 9 , % Retained).
In each season, an agent can know two associations (summer and winter), leading to a maximum score of 6 × 80 × 2 = 960 for the known metric (6 seasons per lifetime ( Fig. 2 ), 80 random environments). The agent can retain or forget two associations each season except the first, making the maximum score for these metrics 5 × 80 × 2 = 800. However, the agent can only score one perfect association (meaning both summer and winter is known) each season, leading to a maximum score of 6 × 80 = 480 for that metric.
In summary, this analysis reveals that a connection cost caused evolution to find individuals that are better at gaining new knowledge without forgetting old knowledge. In other words, adding a connection cost mitigated catastrophic forgetting. That, in turn, enabled an increase in the total number of associations P&CC individuals learned in their lifetimes.
Removing the Ability of Evolution to Improve Retention
To further test whether the improved performance in the P&CC treatment results from it mitigating catastrophic forgetting, we conducted experiments in a regime where retaining skills between tasks is impossible. Under such a regime, if the P&CC treatment does not outperform the PA treatment, that is evidence for our hypothesis that the ability of P&CC networks to outperform PA networks in the normal regime is because P&CC networks retain previously learned skills more when learning new skills.
To create a regime similar to the original problem, but without the potential to improve performance by minimizing catastrophic forgetting, we forced individuals to forget everything they learned at the end of every season. This forced forgetting was implemented by resetting all neuromodulated weights in the network to random values between each season change. The experimental setup was otherwise identical to the main experiment. In this treatment, evolution cannot evolve individuals to handle forgetting better, and can focus only on evolving good learning abilities for each season. With forced forgetting, the P&CC treatment no longer significantly outperforms the PA treatment ( Fig. 10 ).
With forced forgetting, P&CC does not significantly outperform PA: P&CC 0.91 [95% CI: 0.91, 0.91] vs. PA 0.91 [0.90, 0.91], p > 0.05. In the default treatment where remembering is possible, P&CC significantly outperforms PA: P&CC 0.94 [0.92, 0.94] vs. PA 0.78 [0.78, 0.81], p = 8.08 × 10 −6 .
https://doi.org/10.1371/journal.pcbi.1004128.g010
This result indicates that the connection cost specifically helps evolution in optimizing the parts of learning related to resistance against forgetting old associations while learning new ones.
Interestingly, without the connection cost (the PA treatment), forced forgetting significantly improves performance ( Fig. 10 , p = 2.5 × 10 −5 via bootstrap sampling with randomization [ 68 ]). Forcing forgetting likely removes some of the interference between learning the two separate tasks. With the connection cost, however, forced forgetting leads to worse results, indicating that the modular networks in the P&CC treatment have found solutions that benefit from remembering what they have learned in the past, and thus are worse off when not allowed to remember that information.
The Importance of Neuromodulation
We hypothesized that a key factor that causes modularity to help minimize catastrophic forgetting is neuromodulation , which is the ability for learning to be selectively turned on and off in specific neural connections in specific situations. To test whether neuromodulation is essential to evolving a resistance to forgetting in our experiments, we evolved neural networks with and without neuromodulation. When we evolve without neuromodulation, the Hebbian learning dynamics of each connection are constant throughout the lifetime of the organism: this is accomplished by disallowing neuromodulatory neurons from being included in the networks ( Methods ).
Comparing the performance of networks evolved with and without neuromodulation demonstrates that with purely Hebbian learning (i.e. without neuromodulation) evolution never produces a network that performs even moderately well ( Fig. 11 ). This finding is in line with previous work demonstrating that neuromodulation allows evolution to solve more complex reinforcement learning problems than purely Hebbian learning [ 25 ]. While the non-modulatory P&CC networks perform slightly better than non-modulatory PA networks, the differences, while significant (P&CC performance 0.72 [95% CI: 0.71, 0.72] vs. PA 0.70 [0.69, 0.71], p = 0.003), are small. Because networks in neither treatment learn much, studying whether they suffer from catastrophic forgetting is uninformative. These results reveal that neuromodulation is essential to perform well in these environments, and its presence is effectively a prerequisite for testing the hypothesis that modularity mitigates catastrophic forgetting. Moreover, neuromodulation is ubiquitous in animal brains, justifying its inclusion in our default model. One can think of neuromodulation, like the presence of neurons, as a necessary, but not sufficient, ingredient for learning without forgetting. Including it in the experimental backdrop allows us to isolate whether modularity further improves learning and helps mitigate catastrophic forgetting.
Connection costs and neuromodulatory dynamics interact to evolve forgetting-resistant solutions. Without neuromodulation, neither treatment performs well, suggesting that neuromodulation is a prerequisite for solving these types of problems, a result that is consistent with previous research showing that neuromodulation is required to solve challenging learning tasks [ 25 ]. However, even in the non-neuromodulatory (pure Hebbian) experiments, P&CC is more modular (0.33 [95% CI: 0.33, 0.33] vs PA 0.26 [0.22, 0.31], p = 1.16 × 10 −12 ) and performs significantly better (0.72 [95% CI: 0.71, 0.72] vs. PA 0.70 [0.69, 0.71], p = 0.003). That said, because both treatments perform poorly without neuromodulation, and because natural animal brains contain neuromodulated learning [ 28 ], it is most interesting to see the additional impact of modularity against the backdrop of neuromodulation. Against that backdrop, neural modularity improves performance to a much larger degree (P&CC 0.94 [0.92, 0.94] vs. PA 0.78 [0.78, 0.81], p = 8.08 × 10 −6 ), in part by reducing catastrophic forgetting (see text).
https://doi.org/10.1371/journal.pcbi.1004128.g011
In the experiments we performed, we found evidence that adding a connection cost when evolving neural networks significantly increases modularity and the ability of networks to learn new skills while retaining previously learned skills. The resultant networks have a separate learning module and exhibit significantly higher performance, learning, and retention. We further found three lines of evidence that modularity improves performance and helps prevent catastrophic forgetting: (1) networks with a separate learning module performed significantly better, (2) modularity and performance are significantly correlated, and (3) the performance increase disappeared when the ability to retain skills was artificially eliminated. These findings support the idea that neural modularity can improve learning performance both for tasks with the potential for catastrophic forgetting , by reducing the overlap in how separate skills are stored ( Fig. 1 , top), and in general , by modularly separating learned skills from reward signals ( Fig. 1 , bottom).
We also found evidence supporting the hypothesis that the ability to selectively regulate per-connection learning in specific situations, called neuromodulation, is critical for the benefits of a connection cost to be realized. In the presence of neuromodulatory learning dynamics, which occur in the brains of natural animals [ 24 , 54 ], a connection cost could thus significantly mitigate catastrophic forgetting. This work thus provides a new candidate technique for improving learning and reducing catastrophic forgetting, which is essential for advancing our goal of making sophisticated robots and intelligent software based on neural networks. It also suggests that one benefit of the modularity ubiquitous in natural networks may be improved learning via reduced catastrophic forgetting.
While we found these results hold in the experiments we conducted, much work remains to be done on the interesting question of how catastrophic forgetting is avoided in animal brains. Future work in different types of problems and experimental setups are needed to confirm or deny the hypotheses suggested in this paper. Specific studies that can investigate the generality of our hypothesis include studying whether the connection cost technique still reduces interference when inputs cannot be as easily disentangled (for instance, if certain inputs are shared between several skills), investigating the effect of more complex learning tasks that may not be learned at all if the agent forgets between training episodes, and further exploring the effect of experimental parameters, such as the length of training episodes, number of tasks, and different neural network sizes and architectures.
Additionally, while we focused primarily on evolution specifying modular architectures, those architectures could also emerge via intra-life learning rules that lead to modular neural architectures. In fact, there may have been evolutionary pressure to create learning dynamics that result in neural modularity: whether such “modular plasticity” rules exist, how they mechanistically cause modularity, and the role of evolution in producing them, is a ripe area for future study. More generally, exploring the degree to which evolution encodes learning rules that lead to modular architectures, as opposed to hard coding modular architectures, is an interesting area for future research.
The experiments in this paper are meant to invigorate the conversation about how evolution and learning produce brains that avoid catastrophic forgetting. While the results of these experiments shed light on that question, the importance, magnitude, and complexity of the question will yield fascinating research for decades, if not centuries, to come.
Neural Network Model Details
We utilize a standard network model common in previous studies of the evolution of modularity [ 23 , 57 ], extended with neuromodulatory neurons to add reinforcement learning dynamics [ 25 , 69 ]. The network has five layers (Supp. S1 Fig ) and is feed-forward , meaning each node receives inputs only from nodes in the previous layer and sends outputs only to nodes in the next layer. The number of neurons is 10/4/2 for the three hidden layers. The weights (connection strengths) and biases (activation thresholds) in the network take values in the range [-1, 1]. Following the paper that introduced the connection cost technique [ 23 ], networks are directly encoded [ 70 , 71 ].
Information flows through the network from the input layer towards the output layer, with one layer per time step. The output of each node is a function of its inputs, as described in the next section.
Learning Model
The neuromodulated ANN model in this paper was introduced by Soltoggio et al. [ 25 ], and adapted for the Sferes software package by Tonelli and Mouret [ 69 ]. It differs from standard ANN models by employing two types of neurons: non-modulatory neurons , which are regular, activity-propagating neurons, and modulatory neurons . Inputs into each neuron consist of two types of connections: modulatory connections C m and non-modulatory connections C n (normal neural network connections).
Equation 2 describes how the modulatory input to each neuron is calculated. φ is a sigmoid function that maps its input to the interval [−1, 1] (thus allowing both positive and negative modulation). The sum includes weighted contributions from all modulatory connections.
Equation 3 describes how this modulatory input determines the learning rate of all incoming, non-modulatory connections to neuron i . η is a constant learning rate that is set to 0.04 in our experiments. The a i ⋅ a j component is a regular Hebbian learning term that is high when the activity of the pre- and post-synaptic neurons of a connection are correlated [ 45 ]. The result is a Hebbian learning rule that is regulated by the inputs from neuromodulatory neurons, allowing the learning rate of specific connections to be increased or decreased in specific circumstances .
In control experiments without the potential for neuromodulation, all neurons were non-modulatory. Updates to the weights of their incoming connections were calculated via Equation 3 with m i set to a constant value of 1.
Evolutionary Algorithm
Our experiments feature a multi-objective evolutionary algorithm, which optimizes multiple objectives simultaneously. Specifically, it is a modification of the widely used Non-dominated Sorting Genetic Algorithm (NSGA-II) [ 72 ]. However, NSGA-II does not take into account that one objective may be more important than others. In our case, network performance is essential to survival, and minimizing the sum of connection costs is a secondary priority. To capture this difference, we follow [ 23 ] in having a stochastic version of Pareto dominance, in which the secondary objective (connection cost) only factors into selection for an individual with a given probability p . In the experiments reported here, the value of p was 0.75, but preliminary runs demonstrated that values of p of 0.25 and 0.5 led to qualitatively similar results, indicating that the results are robust to substantial changes to this value. However, a p value of 1 was found to overemphasize connection costs at the expense of performance, leading to pathological solutions that perform worse than the PA networks.
Evolutionary algorithms frequently get stuck in local optima [ 5 ] and, due to computational costs, are limited to small population sizes compared to biological evolution. To better capture the power of larger populations, which contain more diversity and thus are less likely to get trapped on local optima, we adopted the common technique of encouraging phenotypic diversity in the population [ 5 , 73 , 74 ]. Diversity was encouraged by adding a diversity objective to the multi-objective algorithm that selected for organisms whose network outputs were different than others in the population. As with performance, the diversity objective factors into selection 100% of the time (i.e. the probability p for PNSGA was 1). Technically, we register every choice (to eat or not) each individual makes and determine how different its sequence of choices is from the choices of other individuals: differences are calculated via a normalized bitwise XOR of the binary choice vectors of two individuals. For each individual, this difference is measured with regards to all other individuals, summed and normalized, resulting in a value between 0 and 1, which measures how different the behavior of this individual is from that of all other individuals. Preliminary experiments demonstrated that, for the problems in this paper, this diversity-promoting technique is necessary to reliably obtain functional networks in either treatment, and is thus a necessary prerequisite to conduct our study. This finding is in line with previous experiments that have showed that diversity is especially necessary for problems that involve learning, because learning problems are especially laden with local optima [ 74 ].
All experiments were implemented in the Sferes evolutionary algorithm software package [ 75 ]. The exact source code and experimental configuration files used in our experiments, along with data from all our experiments, are freely available in the online Dryad scientific archive at http://dx.doi.org/10.5061/dryad.s38n5 .
Mutational Effects
The variation necessary to drive evolution is supplied via random mutation. In each generation, every new offspring network is a copy of its parent that is randomly mutated. Mutations can add a connection, remove a connection, change the strength of connections, move connections and change the type of neurons (switching between modulatory and non-modulatory). Probabilities and details for each mutational event are given in Supp. S1 Table . We chose these evolutionary parameters, including keeping things simple by not adding crossover, to maintain similarity with related experiments on evolving modularity [ 23 ] and neuromodulated learning [ 76 ].
Fitness Function
The fitness function simulates an organism learning associations in a world that fluctuates periodically between a summer and a winter season. During evolution, each individual is tested in four randomly generated environments (i.e. for four “lifetimes”, Fig. 2 ) that vary in which items are designated as food and poison, and in which order individuals encounter the items. Because there is variance in the difficulty of these random worlds, we test in 4 environments (lifetimes), instead of 1, to increase the sample size. We further increase the sample size to 80 environments (lifetimes) when measuring the performance of final, evolved, end-of-experiment individuals (e.g. Figs. 8 and 9 ). Individuals within the same generation are all subjected to the same four environments, but across generations the environments are randomized to select for learning, rather than genetically hard-coded solutions ( Fig. 3 ). To start each environment (note: not season) from a clean slate, before being inserted in an environment the modulated weights of individuals are randomly initialized, which follows previous work with this neuromodulatory learning model [ 76 ]. Modulatory connections never change, and thus do not need to be altered between environments. In the runs without neuromodulation, all connections are reset to their genetically specified weights.
Throughout its life, an individual encounters different edible items several times ( Fig. 2 ). Fitness is proportional to the number of food items consumed minus the number of poison items consumed across all environments (Supp. S7 Fig ). Individuals that can successfully learn which items to eat and which to avoid are thus rewarded, and the best fitness scores are obtained by individuals that are able to retain this information across the fluctuating seasons (i.e. individuals that do not exhibit catastrophic forgetting).
Modularity Calculations
Our modularity calculations follow those developed by Leicht and Newman for directed networks [ 67 ], which is an extension of the most well-established modularity optimization method [ 65 ]. That modularity optimization method relies on the maximization of a benefit function Q , which measures the difference between the number of connections within each module and the expected fraction of such connections given a “null model”, that is, a statistical model of random networks. High values of Q indicate an “unexpectedly modular” network.
A ij is the connectivity matrix (1 if there is an edge from node i to node j , and 0 otherwise), m is the total number of edges in the network, and δ ci , cj is a function that is 1 if i and j belong to the same module, and 0 otherwise. Our results are qualitatively unchanged when using layered, feed-forward networks as “null model” to compute and optimize Q (Supp. S2 Table ).
Maximizing Q is an NP-hard problem [ 77 ], meaning it is necessary to rely on an approximate optimization algorithm instead of an exact one. Here we applied the spectral optimization method , which gives good results in practice at a low computational cost [ 67 , 78 ]. As suggested by Leicht and Newman [ 67 ], each module is split in two until the next split stops increasing the modularity score.
Experimental Parameters
Each experimental treatment was repeated 100 times with different stochastic events (accomplished by initiating experiments with a different numeric seed to a random number generator). Analyses are based on the highest-performing network from each trial. The experiments lasted 20,000 generations and had a population size of 400.
The environment had 2 different seasons (“summer” and “winter”). Each season lasted 5 days , and cycled through 3 years ( Fig. 2 ). In each season, 2 poisonous items and 2 nutritious items were available, each item encoded by a separate input neuron (i.e. a “one-hot encoding” [ 64 ]).
Considering the fact that visiting objects in a different order may affect learning, the total number of possible different environments is 25,920. Each day we randomize the order in which food items are presented, yielding 4! = 24 different possibilities per day. There are in total 5 days per season, and an individual lives for 6 seasons, resulting in 5 × 6 = 30 days per lifetime ( Fig. 2 ), and thus 24 × 30 = 720 different ways to visit the items in a single lifetime. In addition to randomizing the order items are visited in, the edibility associations agents are supposed to learn are randomized between environments. We randomly designate 2 of the 4 items as nutritious food, giving 4 2 = 6 different possibilities for summer and 6 different possibilities for winter. There are thus a total of 6 × 6 = 36 different ways to organize edibility associations across both seasons. In total, we have 720 × 36 = 25,920 unique environments, reflecting the 720 different ways food items can be presented and the 36 possible edibility associations.
As mentioned in the previous section, four of these environments were seen by each individual during evolution, and 80 of them were seen in the final performance tests. In both cases they were selected at random from the set of 25,920.
Unless otherwise stated, the test of statistical significance is the Mann-Whitney U test. 95% bootstrapped confidence intervals of the median are calculated by re-sampling the data 5,000 times. In Fig. 4 , we smooth the plotted values with a median filter to remove sampling noise. The median filter has a window size of 11, and we plot each 10 generations, meaning the median spans a total of 110 generations.
Measuring Learning and Retention
While measuring the forgetting and retention of evolved individuals (e.g. Figs. 8 and 9 ), further learning was disabled. The process is thus (1) learn food associations, (2) measure what was learned and forgotten without further learning, and (3) repeat. Disabling learning allows measurements of what has been learned without the evaluation changing that learned information.
Supporting Information
S1 fig. the number and layout of the input, hidden, and output neurons..
Inputs provide information about the environment. The output is interpreted as the decision to eat a food item or ignore it.
https://doi.org/10.1371/journal.pcbi.1004128.s001
S2 Fig. The highest-performing networks from all of the 100 experiments in the PA treatment (part 1 of 2).
Dark blue nodes are inputs that encode which type of food has been encountered. Light blue nodes indicate internal, non-modulatory neurons. Red nodes are reward or punishment inputs that indicate if a nutritious or poisonous item has been eaten. Orange nodes are neuromodulatory neurons that regulate learning. In the cases where an input neuron was modulatory, we indicate this with an orange circle around the neuron. In each panel, the left number reports performance and the right number reports modularity. We follow the convention from [ 23 ] of placing nodes in the way that minimizes the total connection length.
https://doi.org/10.1371/journal.pcbi.1004128.s002
S3 Fig. The highest-performing networks from all of the 100 experiments in the PA treatment (part 2 of 2).
See the previous figure caption for more details.
https://doi.org/10.1371/journal.pcbi.1004128.s003
S4 Fig. The highest-performing networks from all of the 100 experiments in the P&CC treatment (part 1 of 2).
https://doi.org/10.1371/journal.pcbi.1004128.s004
S5 Fig. The highest-performing networks from all of the 100 experiments in the P&CC treatment (part 2 of 2).
https://doi.org/10.1371/journal.pcbi.1004128.s005
S6 Fig. Unnormalized values for Fig. 8 (left panel).
Shows how old associations are forgotten as new ones are learned for the two experimental treatments. The treatment with a connection cost (P&CC) was able to learn the associations better and shows a more gradual forgetting in the first timesteps. Together, this leads it to outperform the regular treatment (PA) significantly when measuring how fast individuals forget. Note that networks were evolved with five days per season, so the results during those first five days are the most informative regarding the evolutionary mitigation of catastrophic forgetting: we show additional days to reveal longer-term consequences of the evolved architectures.
https://doi.org/10.1371/journal.pcbi.1004128.s006
S7 Fig. The steps for evaluating the fitness of an individual.
The example describes what happens when an agent encounters a food item during summer. For the winter season, the process is the same, but with winter inputs active instead of summer inputs.
https://doi.org/10.1371/journal.pcbi.1004128.s007
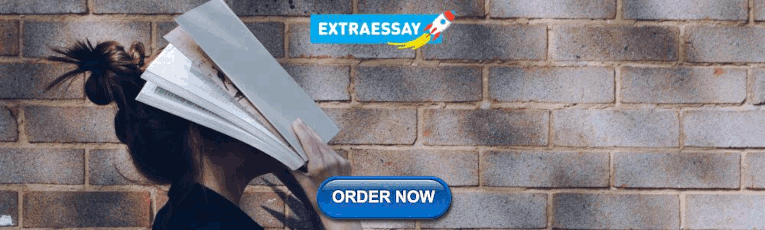
S1 Table. The mutation operators along with their probabilities of affecting an individual.
https://doi.org/10.1371/journal.pcbi.1004128.s008
S2 Table. Two different null models for calculating the modularity score.
The conventional way to calculate modularity is inherently relative: one computes the modularity of network N by searching for the modular decomposition (assigning N’s nodes to different modules) that maximizes the number of edges within the modules compared to the number of expected edges given by a statistical model of random, but similar, networks called the “null model”. There are different ways to model random networks, depending on the type of networks being measured and their topological constraints. Here, we calculated the modularity Q-score with two different null models, one modeling random, directed networks and the other modeling random, layered, feed-forward networks. When calculating modularity with either null model, P&CC networks are significantly more modular than PA networks. A ij is 1 if there is an edge from node i to node j , and 0 otherwise, k i i n and k j o u t are the in- and out-degrees of node i and j , respectively, m is the total number of edges in the network, m ij is the number of edges between the layer containing node i and the layer containing node j , and δ ci , cj is a function that is 1 if i and j belong to the same module, and 0 otherwise.
https://doi.org/10.1371/journal.pcbi.1004128.s009
Acknowledgments
We thank Joost Huizinga, Henok Sahilu Mengistu, Mohammad Sadegh Norouzzadeh, Oliver Coleman, Keith Downing, Roby Velez, Gleb Sizov, Jean-Marc Montanier, Anh Nguyen, Jingyu Li and Boye Annfelt Høverstad for helpful comments. We also thank the editors and anonymous reviewers. The images in Figs. 2 & 3 are from OpenClipArt.org and are in the public domain.
Author Contributions
Conceived and designed the experiments: KOE JC. Performed the experiments: KOE. Analyzed the data: KOE JBM JC. Contributed reagents/materials/analysis tools: KOE JBM. Wrote the paper: KOE JMB JC. Developed the software used in experiments: JBM.
- View Article
- PubMed/NCBI
- Google Scholar
- 4. Haykin SS (2009) Neural networks and learning machines. New York: Prentice Hall, 3 edition.
- 5. Floreano D, Mattiussi C (2008) Bio-inspired artificial intelligence: theories, methods, and technologies. The MIT Press, 659 pp.
- 8. Soltoggio A (2008) Neural Plasticity and Minimal Topologies for Reward-Based Learning. In: Hybrid Intelligent Systems, 2008 HIS’08 Eighth International Conference on. IEEE, pp. 637–642.
- 15. Seipone T, Bullinaria J (2005) The evolution of minimal catastrophic forgetting in neural systems. In: Proc Annu Conf Cogn Sci Soc. Cognitive Science Society, pp. 1991–1996.
- 17. Alon U (2006) An Introduction to Systems Biology: Design Principles of Biological Circuits. CRC press.
- 23. Clune J, Mouret JB, Lipson H (2013) The evolutionary origins of modularity. Proc R Soc London Ser B Biol Sci 280.
- 28. Striedter G (2005) Principles of brain evolution. Sinauer Associates Sunderland, MA.
- 29. Kortge C (1990) Episodic memory in connectionist networks. In: Proc Annu Conf Cogn Sci Soc. pp. 764–771.
- 30. Lewandowsky S, Li SC (1993) Catastrophic interference in neural networks: causes, solutions and data. In: Dempster F, Brainerd C, editors, New Perspectives on Interference and Inhibition in Cognition, Academic Press. pp. 329–361.
- 31. French R (1991) Using semi-distributed representations to overcome catastrophic forgetting in connectionist networks. In: Proceedings of the 13th Annual Congitive Science Society Conference. pp. 173–178.
- 32. French R (1994) Dynamically constraining connectionist networks to produce distributed, orthogonal representations to reduce catastrophic interference. In: Proc Annu Conf Cogn Sci Soc. pp. 335–340.
- 38. Holland JH (1975) Adaptation in natural and artificial systems: An introductory analysis with applications to biology, control, and artificial intelligence. U Michigan Press.
- 40. Koza JR (2003) Genetic programming IV: Routine human-competitive machine intelligence. Kluwer.
- 42. Gomez FJ, Miikkulainen R (2003) Active guidance for a finless rocket using neuroevolution. In: Proc Genet Evol Comput Conf. pp. 2084–2095.
- 43. Clune J, Beckmann BE, Ofria C, Pennock RT (2009) Evolving coordinated quadruped gaits with the HyperNEAT generative encoding. In: Proc Congr Evol Comput. pp. 2764–2771.
- 45. Hebb DO (1949) The Organization of Behavior. New York: Wiley and Sons, 335 pp.
- 47. Ellefsen KO (2013) Balancing the Costs and Benefits of Learning Ability. In: European Conference of Artificial Life. pp. 292–299.
- 49. Blynel J, Floreano D (2002) Levels of dynamics and adaptive behavior in evolutionary neural controllers. In: 7th International Conference on Simulation on Adaptive Behavior (SAB’2002). MIT Press, pp. 272–281.
- 60. Clune J, Beckmann BE, McKinley PK, Ofria C (2010) Investigating whether HyperNEAT produces modular neural networks. In: Genet Evol Comput Conf. ACM, pp. 635–642.
- 61. Verbancsics P, Stanley KO (2011) Constraining connectivity to encourage modularity in HyperNEAT. In: Genet Evol Comput Conf. ACM, pp. 1483–1490.
- 67. Leicht EA, Newman MEJ (2008) Community structure in directed networks. Phys Rev Lett: 118703–118707.
- 68. Cohen PR (1995) Empirical Methods for Artificial Intelligence. Cambridge, MA, USA: MIT Press, 405 pp.
- 69. Tonelli P, Mouret JB (2011) On the relationships between synaptic plasticity and generative systems. In: Genet Evol Comput Conf. pp. 1531–1538.
- 74. Risi S, Vanderbleek SD, Hughes CE, Stanley KO (2009) How novelty search escapes the deceptive trap of learning to learn. In: Genet Evol Comput Conf. pp. 153–160.
- 75. Mouret JB, Doncieux S (2010) SFERES v2: Evolvin’ in the Multi-Core World. In: Proc Congr Evol Comput. IEEE, 2, pp. 4079–4086.
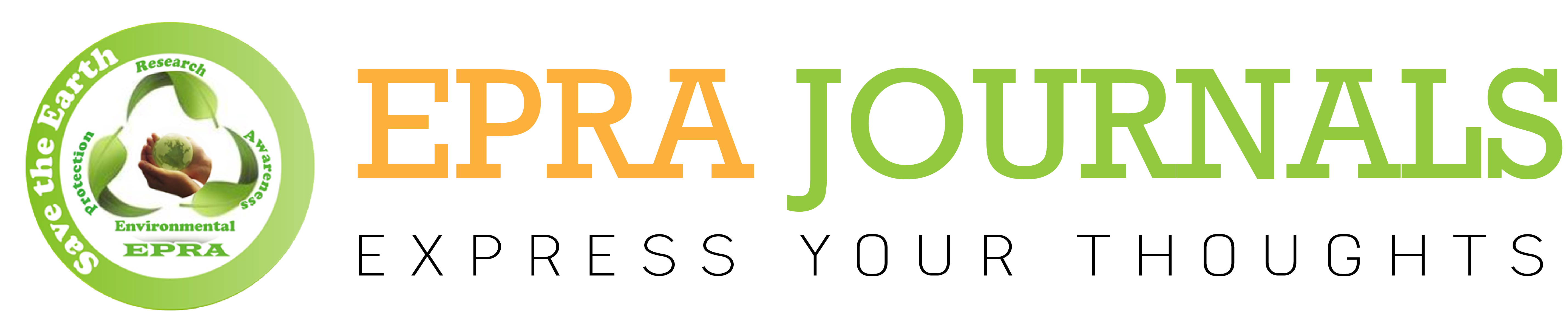
EPRA International Journal of Multidisciplinary Research (IJMR)
- Vol. 7 Issue. 7 (July-2021) EPRA International Journal of Multidisciplinary Research (IJMR)
THE CHALLENGES AND STATUS OF MODULAR LEARNING: ITS EFFECT TO STUDENTS' ACADEMIC BEHAVIOR AND PERFORMANCE
Monica anna ladan agarin, about epra journals, quick links.
- Submit Your Paper
- Track Your Paper Status
- Certificate Download
FOR AUTHORS
- Impact Factor
- Plagiarism Policy
- Retraction Policy
- Publication Policy
- Terms & Conditions
- Refund Policy
- Privacy Policy
- Cancellation Policy
- Shipping Policy
Academia.edu no longer supports Internet Explorer.
To browse Academia.edu and the wider internet faster and more securely, please take a few seconds to upgrade your browser .
- We're Hiring!
- Help Center
Modular Distance Learning
- Most Cited Papers
- Most Downloaded Papers
- Newest Papers
- Save to Library
Enter the email address you signed up with and we'll email you a reset link.
- Academia.edu Publishing
- We're Hiring!
- Help Center
- Find new research papers in:
- Health Sciences
- Earth Sciences
- Cognitive Science
- Mathematics
- Computer Science
- Academia ©2024
Thank you for visiting nature.com. You are using a browser version with limited support for CSS. To obtain the best experience, we recommend you use a more up to date browser (or turn off compatibility mode in Internet Explorer). In the meantime, to ensure continued support, we are displaying the site without styles and JavaScript.
- View all journals
- My Account Login
- Explore content
- About the journal
- Publish with us
- Sign up for alerts
- Open access
- Published: 20 May 2024
Testing theory of mind in large language models and humans
- James W. A. Strachan ORCID: orcid.org/0000-0002-8618-3834 1 ,
- Dalila Albergo ORCID: orcid.org/0000-0002-8039-5414 2 , 3 ,
- Giulia Borghini 2 ,
- Oriana Pansardi ORCID: orcid.org/0000-0001-6092-1889 1 , 2 , 4 ,
- Eugenio Scaliti ORCID: orcid.org/0000-0002-4977-2197 1 , 2 , 5 , 6 ,
- Saurabh Gupta ORCID: orcid.org/0000-0001-6978-4243 7 ,
- Krati Saxena ORCID: orcid.org/0000-0001-7049-9685 7 ,
- Alessandro Rufo ORCID: orcid.org/0009-0003-8565-4192 7 ,
- Stefano Panzeri ORCID: orcid.org/0000-0003-1700-8909 8 ,
- Guido Manzi ORCID: orcid.org/0009-0009-2927-3380 7 ,
- Michael S. A. Graziano 9 &
- Cristina Becchio ORCID: orcid.org/0000-0002-6845-0521 1 , 2
Nature Human Behaviour ( 2024 ) Cite this article
6503 Accesses
228 Altmetric
Metrics details
- Human behaviour
At the core of what defines us as humans is the concept of theory of mind: the ability to track other people’s mental states. The recent development of large language models (LLMs) such as ChatGPT has led to intense debate about the possibility that these models exhibit behaviour that is indistinguishable from human behaviour in theory of mind tasks. Here we compare human and LLM performance on a comprehensive battery of measurements that aim to measure different theory of mind abilities, from understanding false beliefs to interpreting indirect requests and recognizing irony and faux pas. We tested two families of LLMs (GPT and LLaMA2) repeatedly against these measures and compared their performance with those from a sample of 1,907 human participants. Across the battery of theory of mind tests, we found that GPT-4 models performed at, or even sometimes above, human levels at identifying indirect requests, false beliefs and misdirection, but struggled with detecting faux pas. Faux pas, however, was the only test where LLaMA2 outperformed humans. Follow-up manipulations of the belief likelihood revealed that the superiority of LLaMA2 was illusory, possibly reflecting a bias towards attributing ignorance. By contrast, the poor performance of GPT originated from a hyperconservative approach towards committing to conclusions rather than from a genuine failure of inference. These findings not only demonstrate that LLMs exhibit behaviour that is consistent with the outputs of mentalistic inference in humans but also highlight the importance of systematic testing to ensure a non-superficial comparison between human and artificial intelligences.
Similar content being viewed by others
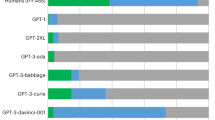
Human-like intuitive behavior and reasoning biases emerged in large language models but disappeared in ChatGPT
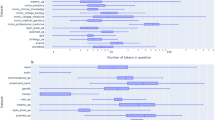
ThoughtSource: A central hub for large language model reasoning data
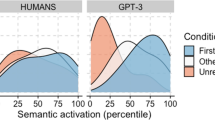
Overlap in meaning is a stronger predictor of semantic activation in GPT-3 than in humans
People care about what other people think and expend a lot of effort thinking about what is going on in other minds. Everyday life is full of social interactions that only make sense when considered in light of our capacity to represent other minds: when you are standing near a closed window and a friend says, ‘It’s a bit hot in here’, it is your ability to think about her beliefs and desires that allows you to recognize that she is not just commenting on the temperature but politely asking you to open the window 1 .
This ability for tracking other people’s mental states is known as theory of mind. Theory of mind is central to human social interactions—from communication to empathy to social decision-making—and has long been of interest to developmental, social and clinical psychologists. Far from being a unitary construct, theory of mind refers to an interconnected set of notions that are combined to explain, predict, and justify the behaviour of others 2 . Since the term ‘theory of mind’ was first introduced in 1978 (ref. 3 ), dozens of tasks have been developed to study it, including indirect measures of belief attribution using reaction times 4 , 5 , 6 and looking or searching behaviour 7 , 8 , 9 , tasks examining the ability to infer mental states from photographs of eyes 10 , and language-based tasks assessing false belief understanding 11 , 12 and pragmatic language comprehension 13 , 14 , 15 , 16 . These measures are proposed to test early, efficient but inflexible implicit processes as well as later-developing, flexible and demanding explicit abilities that are crucial for the generation and comprehension of complex behavioural interactions 17 , 18 involving phenomena such as misdirection, irony, implicature and deception.
The recent rise of large language models (LLMs), such as generative pre-trained transformer (GPT) models, has shown some promise that artificial theory of mind may not be too distant an idea. Generative LLMs exhibit performance that is characteristic of sophisticated decision-making and reasoning abilities 19 , 20 including solving tasks widely used to test theory of mind in humans 21 , 22 , 23 , 24 . However, the mixed success of these models 23 , along with their vulnerability to small perturbations to the provided prompts, including simple changes in characters’ perceptual access 25 , raises concerns about the robustness and interpretability of the observed successes. Even in cases where these models are capable of solving complex tasks 20 that are cognitively demanding even for human adults 17 , it cannot be taken for granted that they will not be tripped up by a simpler task that a human would find trivial 26 . As a result, work in LLMs has begun to question whether these models rely on shallow heuristics rather than robust performance that parallels human theory of mind abilities 27 .
In the service of the broader multidisciplinary study of machine behaviour 28 , there have been recent calls for a ‘machine psychology’ 29 that have argued for using tools and paradigms from experimental psychology to systematically investigate the capacities and limits of LLMs 30 . A systematic experimental approach to studying theory of mind in LLMs involves using a diverse set of theory of mind measures, delivering multiple repetitions of each test, and having clearly defined benchmarks of human performance against which to compare 31 . In this Article, we adopt such an approach to test the performance of LLMs in a wide range of theory of mind tasks. We tested the chat-enabled version of GPT-4, the latest LLM in the GPT family of models, and its predecessor ChatGPT-3.5 (hereafter GPT-3.5) in a comprehensive set of psychological tests spanning different theory of mind abilities, from those that are less cognitively demanding for humans such as understanding indirect requests to more cognitively demanding abilities such as recognizing and articulating complex mental states like misdirection or irony 17 . GPT models are closed, evolving systems. In the interest of reproducibility 32 , we also tested the open-weight LLaMA2-Chat models on the same tests. To understand the variability and boundary limitations of LLMs’ social reasoning capacities, we exposed each model to multiple repetitions of each test across independent sessions and compared their performance with that of a sample of human participants (total N = 1,907). Using variants of the tests considered, we were able to examine the processes behind the models’ successes and failures in these tests.
Theory of mind battery
We selected a set of well-established theory of mind tests spanning different abilities: the hinting task 14 , the false belief task 11 , 33 , the recognition of faux pas 13 , and the strange stories 15 , 16 . We also included a test of irony comprehension using stimuli adapted from a previous study 34 . Each test was administered separately to GPT-4, GPT-3.5 and LLaMA2-70B-Chat (hereafter LLaMA2-70B) across 15 chats. We also tested two other sizes of LLaMA2 model (7B and 13B), the results of which are reported in Supplementary Information section 1 . Because each chat is a separate and independent session, and information about previous sessions is not retained, this allowed us to treat each chat (session) as an independent observation. Responses were scored in accordance with the scoring protocols for each test in humans ( Methods ) and compared with those collected from a sample of 250 human participants. Tests were administered by presenting each item sequentially in a written format that ensured a species-fair comparison 35 ( Methods ) between LLMs and human participants.
Performance across theory of mind tests
Except for the irony test, all other tests in our battery are publicly available tests accessible within open databases and scholarly journal articles. To ensure that models did not merely replicate training set data, we generated novel items for each published test ( Methods ). These novel test items matched the logic of the original test items but used a different semantic content. The text of original and novel items and the coded responses are available on the OSF (methods and resource availability).
Figure 1a compares the performance of LLMs against the performance of human participants across all tests included in the battery. Differences in performance on original items versus novel items, separately for each test and model, are shown in Fig. 1b .
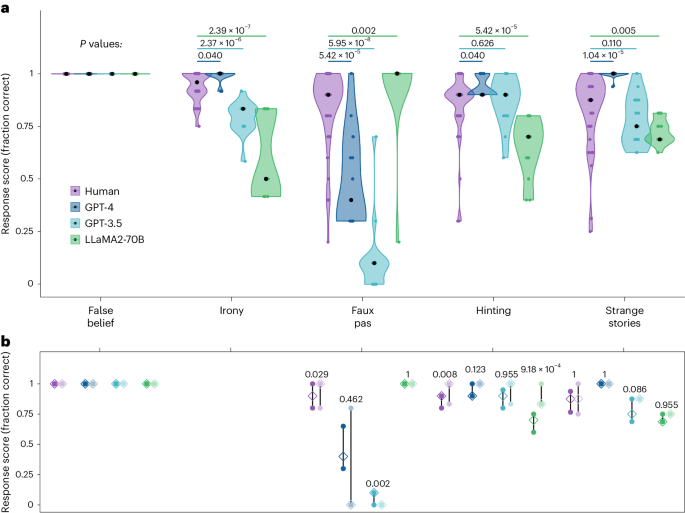
a , Original test items for each test showing the distribution of test scores for individual sessions and participants. Coloured dots show the average response score across all test items for each individual test session (LLMs) or participant (humans). Black dots indicate the median for each condition. P values were computed from Holm-corrected Wilcoxon two-way tests comparing LLM scores ( n = 15 LLM observations) against human scores (irony, N = 50 human participants; faux pas, N = 51 human participants; hinting, N = 48 human participants; strange stories, N = 50 human participants). Tests are ordered in descending order of human performance. b , Interquartile ranges of the average scores on the original published items (dark colours) and novel items (pale colours) across each test (for LLMs, n = 15 LLM observations; for humans, false belief, N = 49 human participants; faux pas, N = 51 human participants; hinting, N = 48 human participants; strange stories, N = 50 human participants). Empty diamonds indicate the median scores, and filled circles indicate the upper and lower bounds of the interquartile range. P values shown are from Holm-corrected Wilcoxon two-way tests comparing performance on original items against the novel items generated as controls for this study.
Source data
False belief.
Both human participants and LLMs performed at ceiling on this test (Fig. 1a ). All LLMs correctly reported that an agent who left the room while the object was moved would later look for the object in the place where they remembered seeing it, even though it no longer matched the current location. Performance on novel items was also near perfect (Fig. 1b ), with only 5 human participants out of 51 making one error, typically by failing to specify one of the two locations (for example, ‘He’ll look in the room’; Supplementary Information section 2 ).
In humans, success on the false belief task requires inhibiting one’s own belief about reality in order to use one’s knowledge about the character’s mental state to derive predictions about their behaviour. However, with LLMs, performance may be explained by lower-level explanations than belief tracking 27 . Supporting this interpretation, LLMs such as ChatGPT have been shown to be susceptible to minor alterations to the false belief formulation 25 , 27 , such as making the containers where the object is hidden transparent or asking about the belief of the character who moved the object rather than the one who was out of the room. Such perturbations of the standard false belief structure are assumed not to matter for humans (who possess a theory of mind) 25 . In a control study using these perturbation variants (Supplementary Information section 4 and Supplementary Appendix 1 ), we replicated the poor performance of GPT models found in previous studies 25 . However, we found that human participants ( N = 757) also failed on half of these perturbations. Understanding these failures and the similarities and differences in how humans and LLMs may arrive at the same outcome requires further systematic investigation. For example, because these perturbations also involve changes in the physical properties of the environment, it is difficult to establish whether LLMs (and humans) failed because they were sticking to the familiar script and were unable to automatically attribute an updated belief, or because they did not consider physical principles (for example, transparency).
GPT-4 performed significantly better than human levels ( Z = 0.00, P = 0.040, r = 0.32, 95% confidence interval (CI) 0.14–0.48). By contrast, both GPT-3.5 ( Z = −0.17, P = 2.37 × 10 −6 , r = 0.64, 95% CI 0.49–0.77) and LLaMA2-70B ( Z = −0.42, P = 2.39 × 10 −7 , r = 0.70, 95% CI 0.55–0.79) performed below human levels (Fig. 1a ). GPT-3.5 performed perfectly at recognizing non-ironic control statements but made errors at recognizing ironic utterances (Supplementary Information section 2 ). Control analysis revealed a significant order effect, whereby GPT-3.5 made more errors on earlier trials than later ones (Supplementary Information section 3 ). LLaMA2-70B made errors when recognizing both ironic and non-ironic control statements, suggesting an overall poor discrimination of irony.
On this test, GPT-4 scored notably lower than human levels ( Z = −0.40, P = 5.42 × 10 −5 , r = 0.55, 95% CI 0.33–0.71) with isolated ceiling effects on specific items (Supplementary Information section 2 ). GPT-3.5 scored even worse, with its performance nearly at floor ( Z = −0.80, P = 5.95 × 10 −8 , r = 0.72, 95% CI 0.58–0.81) on all items except one. By contrast, LLaMA2-70B outperformed humans ( Z = 0.10, P = 0.002, r = 0.44, 95% CI 0.24–0.61) achieving 100% accuracy in all but one run.
The pattern of results for novel items was qualitatively similar (Fig. 1b ). Compared with original items, the novel items proved slightly easier for humans ( Z = −0.10, P = 0.029, r = 0.29, 95% CI 0.10–0.50) and more difficult for GPT-3.5 ( Z = 0.10, P = 0.002, r = 0.69, 95% CI 0.49–0.88), but not for GPT-4 and LLaMA2-70B ( P > 0.462; Bayes factor (BF 10 ) of 0.77 and 0.43, respectively). Given the poor performance of GPT-3.5 of the original test items, this difference was unlikely to be explained by a prior familiarity with the original items. These results were robust to alternative coding schemes (Supplementary Information section 5 ).
On this test, GPT-4 performance was significantly better than humans ( Z = 0.00, P = 0.040, r = 0.32, 95% CI 0.12–0.50). GPT-3.5 performance did not significantly differ from human performance ( Z = 0.00, P = 0.626, r = 0.06, 95% CI 0.01–0.33, BF 10 0.33). Only LLaMA2-70B scored significantly below human levels of performance on this test ( Z = −0.20, P = 5.42 × 10 −5 , r = 0.57, 95% CI 0.41–0.72).
Novel items proved easier than original items for both humans ( Z = −0.10, P = 0.008, r = 0.34, 95% CI 0.14–0.53) and LLaMA2-70B ( Z = −0.20, P = 9.18 × 10 −4 , r = 0.73, 95% CI 0.50–0.87) (Fig. 1b ). Scores on novel items did not differ from the original test items for GPT-3.5 ( Z = −0.03, P = 0.955, r = 0.24, 95% CI 0.02–0.59, BF 10 0.61) or GPT-4 ( Z = −0.10, P = 0.123, r = 0.44, 95% CI 0.07–0.75, BF 10 0.91). Given that better performance on novel items is the opposite of what a prior familiarity explanation would predict, it is likely that this difference for LLaMA2-70B was driven by differences in item difficulty.
Strange stories
GPT-4 significantly outperformed humans on this test ( Z = 0.13, P = 1.04 × 10 −5 , r = 0.60, 95% CI 0.46–0.72). The performance of GPT-3.5 did not significantly differ from humans ( Z = −0.06, P = 0.110, r = 0.24, 95% CI 0.03–0.44, BF 10 0.47), while LLaMA2-70B scored significantly lower than humans ( Z = −0.13, P = 0.005, r = 0.41, 95% CI 0.24–0.60). There were no differences between original and novel items for any model (all P > 0.085; BF 10 : human 0.22, GPT-3.5 1.46, LLaMA2-70B 0.46; the variance for GPT-4 was too low to compute a Bayes factor). As reported in Supplementary Information section 6 , partial successes were infrequent and more likely for LLaMA2-70B than for other models.
Understanding faux pas
In line with previous findings that GPT models struggle with faux pas 36 , in our battery, faux pas was the only test in which GPT-4 did not match or exceed human performance. Surprisingly, faux pas was also the only test in which LLaMA2-70B, which was otherwise the poorest-performing model, scored better than humans (Fig. 1 ).
The faux pas test consists of vignettes describing an interaction where one character (the speaker) says something they should not have said, not knowing or not realizing that they should not say it. To understand that a faux pas has occurred, one must represent two mental states: that the speaker does not know that they should not say what they said, and that the person hearing it (the victim) would feel insulted or hurt by what was said. Consider the following example of a story describing a faux pas:
Jill had just moved into a new house. She went shopping with her Mum and bought some new curtains. When Jill had just put them up, her best friend Lisa came round and said, "Oh, those curtains are horrible, I hope you're going to get some new ones." Jill asked, "Do you like the rest of my bedroom?"
Following the presentation of the story, four comprehension questions are asked. The first question is, ‘In the story, did somebody say something they should not have said?’ to which the correct answer is always yes. The second question asks the respondent to report what the person said that they should not have said, and the third question is a comprehension question specific to the content of the story. The fourth and key question, which was our focus for coding, relates to the speaker’s belief when they made the inappropriate utterance: ‘Did [Lisa] know that [the curtains were new]?’ The correct answer to this final question is always no.
Both GPT-4 and GPT-3.5 correctly identified that the victim would feel insulted or hurt, sometimes going so far as to provide additional details about why the utterance might cause offence. However, when asked whether the speaker was aware of the context that made their statement offensive (such as Lisa knowing that Jill had just bought the curtains), they failed to produce a correct answer. A closer look revealed that the overwhelming majority of the errors on this question reported that there was not enough information provided to be sure, for example:
Did Lisa know the curtains were new? GPT-4: […] It is unclear from the story whether Lisa knew the curtains were new or not.
Only two responses out of 349 reported that, yes, the character did know. We consider three alternative hypotheses for why GPT models, and specifically GPT-4, fail to answer this question correctly.
The first hypothesis, which we term the failure of inference hypothesis, is that models fail to generate inferences about the mental state of the speaker (note that we refer to inference here not in the sense of the processes by which biological organisms infer hidden states from their environment, but rather as any process of reasoning whereby conclusions are derived from a set of propositional premises). Recognizing a faux pas in this test relies on contextual information beyond that encoded within the story (for example, about social norms). For example, in the above example there is no information in the story to indicate that saying that the newly bought curtains are horrible is inappropriate, but this is a necessary proposition that must be accepted in order to accurately infer the mental states of the characters. This inability to use non-embedded information would fundamentally impair the ability of GPT-4 to compute inferences.
The second hypothesis, which we term the Buridan’s ass hypothesis, is that models are capable of inferring mental states but cannot choose between them, as with the eponymous rational agent caught between two equally appetitive bales of hay that starves because it cannot resolve the paradox of making a decision in the absence of a clear preference 37 . Under this hypothesis, GPT models can propose the correct answer (a faux pas) as one among several possible alternatives but do not rank these alternatives in terms of likelihood. In partial support of this hypothesis, responses from both GPT models occasionally indicate that the speaker may not know or remember but present this as one hypothesis among alternatives (Supplementary Information section 5 ).
The third hypothesis, which we term the hyperconservatism hypothesis, is that GPT models are able both to compute inferences about the mental states of characters and recognise a false belief or lack of knowledge as the likeliest explanation among competing alternatives but refrain from committing to a single explanation out of an excess of caution. GPT models are powerful language generators, but they are also subject to inhibitory mitigation processes 38 . It is possible that such processes could lead to an overly conservative stance where GPT models do not commit to the likeliest explanation despite being able to generate it.
To differentiate between these hypotheses, we devised a variant of the faux pas test where the question assessing performance on the faux pas test was formulated in terms of likelihood (hereafter, the faux pas likelihood test). Specifically, rather than ask whether the speaker knew or did not know, we asked whether it was more likely that the speaker knew or did not know. Under the hyperconservatism hypothesis, GPT models should be able to both make the inference that the speaker did not know and identify it as more likely among alternatives, and so we would expect the models to respond accurately that it was more likely that the speaker did not know. In case of uncertainty or incorrect responses, we further prompted models to describe the most likely explanation. Under the Buridan’s ass hypothesis, we expected this question would elicit multiple alternative explanations that would be presented as equally plausible, while under the failure of inference hypothesis, we expected that GPT would not be able to generate the right answer at all as a plausible explanation.
As shown in Fig. 2a , on the faux pas likelihood test GPT-4 demonstrated perfect performance, with all responses identifying without any prompting that it was more likely that the speaker did not know the context. GPT-3.5 also showed improved performance, although it did require prompting in a few instances (~3% of items) and occasionally failed to recognize the faux pas (~9% of items; see Supplementary Information section 7 for a qualitative analysis of response types).
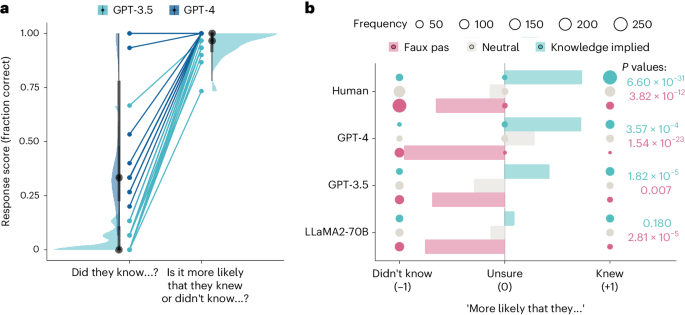
a , Scores of the two GPT models on the original framing of the faux pas question (‘Did they know…?’) and the likelihood framing (‘Is it more likely that they knew or didn’t know…?’). Dots show average score across trials ( n = 15 LLM observations) on particular items to allow comparison between the original faux pas test and the new faux pas likelihood test. Halfeye plots show distributions, medians (black points), 66% (thick grey lines) and 99% quantiles (thin grey lines) of the response scores on different items ( n = 15 different stories involving faux pas). b , Response scores to three variants of the faux pas test: faux pas (pink), neutral (grey) and knowledge-implied variants (teal). Responses were coded as categorical data as ‘didn’t know’, ‘unsure’ or ‘knew’ and assigned a numerical coding of −1, 0 and +1. Filled balloons are shown for each model and variant, and the size of each balloon indicates the count frequency, which was the categorical data used to compute chi-square tests. Bars show the direction bias score computed as the average across responses of the categorical data coded as above. On the right of the plot, P values (one-sided) of Holm-corrected chi-square tests are shown comparing the distribution of response type frequencies in the faux pas and knowledge-implied variants against neutral.
Taken together, these results support the hyperconservatism hypothesis, as they indicate that GPT-4, and to a lesser but still notable extent GPT-3.5, successfully generated inferences about the mental states of the speaker and identified that an unintentional offence was more likely than an intentional insult. Thus, failure to respond correctly to the original phrasing of the question does not reflect a failure of inference, nor indecision among alternatives the model considered equally plausible, but an overly conservative approach that prevented commitment to the most likely explanation.
Testing information integration
A potential confound of the above results is that, as the faux pas test includes only items where a faux pas occurs, any model biased towards attributing ignorance would demonstrate perfect performance without having to integrate the information provided by the story. This potential bias could explain the perfect performance of LLaMA2-70B in the original faux pas test (where the correct answer is always, ‘no’) as well as GPT-4’s perfect and GPT-3.5’s good performance on the faux pas likelihood test (where the correct answer is always ‘more likely that they didn’t know’).
To control for this, we developed a novel set of variants of the faux pas likelihood test manipulating the likelihood that the speaker knew or did not know (hereafter the belief likelihood test). For each test item, all newly generated for this control study, we created three variants: a ‘faux pas’ variant, a ‘neutral’ variant, and a ‘knowledge-implied’ variant ( Methods ). In the faux pas variant, the utterance suggested that the speaker did not know the context. In the neutral variant, the utterance suggested neither that they knew nor did not know. In the knowledge-implied variant, the utterance suggested that the speaker knew (for the full text of all items, see Supplementary Appendix 2 ).
If the models’ responses reflect a true discrimination of the relative likelihood of the two explanations (that the person knew versus that they didn’t know, hereafter ‘knew’ and ‘didn’t know’), then the distribution of ‘knew’ and ‘didn’t know’ responses should be different across variants. Specifically, relative to the neutral variant, ‘didn’t know’ responses should predominate for the faux pas, and ‘knew’ responses should predominate for the knowledge-implied variant. If the responses of the models do not discriminate between the three variants, or discriminate only partially, then it is likely that responses are affected by a bias or heuristic unrelated to the story content.
We adapted the three variants (faux pas, neutral and knowledge implied) for six stories, administering each test item separately to each LLM and a new sample of human participants (total N = 900). Responses were coded using a numeric code to indicate which, if either, of the knew/didn’t know explanations the response endorsed (−1, didn’t know; 0, unsure or impossible to tell; +1, knew). These coded scores were then averaged for each story to give a directional score for each variant such that negative values indicated the model was more likely to endorse the ‘didn’t know’ explanation, while positive values indicated the model was more likely to endorse the ‘knew’ explanation. These results are shown in Fig. 2b . As expected, humans were more likely to report that the speaker did not know for faux pas than for neutral ( χ 2 (2) = 56.20, P = 3.82 × 10 −12 ) and more likely to report that the speaker did know for knowledge implied than for neutral ( χ 2 (2) = 143, P = 6.60 × 10 −31 ). Humans also reported uncertainty on a small proportion of trials, with a higher proportion in the neutral condition (28 out of 303 responses) than in the other variants (11 out of 303 for faux pas, and 0 out of 298 for knowledge implied).
Similarly to humans, GPT-4 was more likely to endorse the ‘didn’t know’ explanation for faux pas than for neutral ( χ 2 (2) = 109, P = 1.54 × 10 −23 ) and more likely to endorse the ‘knew’ explanation for knowledge implied than for neutral ( χ 2 (2) = 18.10, P = 3.57 × 10 −4 ). GPT-4 was also more likely to report uncertainty in the neutral condition than responding randomly (42 out of 90 responses, versus 6 and 17 in the faux pas and knowledge-implied variants, respectively).
The pattern of responses for GPT-3.5 was similar, with the model being more likely to report that the speaker didn’t know for faux pas than for neutral ( χ 2 (1) = 8.44, P = 0.007) and more likely that the character knew for knowledge implied than for neutral ( χ 2 (1) = 21.50, P = 1.82 × 10 −5 ). Unlike GPT-4, GPT-3.5 never reported uncertainty in response to any variants and always selected one of the two explanations as the likelier even in the neutral condition.
LLaMA2-70B was also more likely to report that the speaker didn’t know in response to faux pas than neutral ( χ 2 (1) = 20.20, P = 2.81 × 10 −5 ), which was consistent with this model’s ceiling performance in the original formulation of the test. However, it showed no differentiation between neutral and knowledge implied ( χ 2 (1) = 1.80, P = 0.180, BF 10 0.56). As with GPT-3.5, LLaMA2-70B never reported uncertainty in response to any variants and always selected one of the two explanations as the likelier.
Furthermore, the responses of LLaMA2-70B and, to a lesser extent, GPT-3.5 appeared to be subject to a response bias towards affirming that someone had said something they should not have said. Although the responses to the first question (which involved recognising that there was an offensive remark made) were of secondary interest to our study, it was notable that, although all models could correctly identify that an offensive remark had been made in the faux pas condition (all LLMs 100%, humans 83.61%), only GPT-4 reliably reported that there was no offensive statement in the neutral and knowledge-implied conditions (15.47% and 27.78%, respectively), with similar proportions to human responses (neutral 19.27%, knowledge implied 30.10%). GPT-3.5 was more likely to report that somebody made an offensive remark in all conditions (neutral 71.11%, knowledge implied 87.78%), and LLaMA2-70B always reported that somebody in the story had made an offensive remark.
We collated a battery of tests to comprehensively measure performance in theory of mind tasks in three LLMs (GPT-4, GPT-3.5 and LLaMA2-70B) and compared these against the performance of a large sample of human participants. Our findings validate the methodological approach taken in this study using a battery of multiple tests spanning theory of mind abilities, exposing language models to multiple sessions and variations in both structure and content, and implementing procedures to ensure a fair, non-superficial comparison between humans and machines 35 . This approach enabled us to reveal the existence of specific deviations from human-like behaviour that would have remained hidden using a single theory of mind test, or a single run of each test.
Both GPT models exhibited impressive performance in tasks involving beliefs, intentions and non-literal utterances, with GPT-4 exceeding human levels in the irony, hinting and strange stories. Both GPT-4 and GPT-3.5 failed only on the faux pas test. Conversely, LLaMA2-70B, which was otherwise the poorest-performing model, outperformed humans on the faux pas. Understanding a faux pas involves two aspects: recognizing that one person (the victim) feels insulted or upset and understanding that another person (the speaker) holds a mistaken belief or lacks some relevant knowledge. To examine the nature of models’ successes and failures on this test, we developed and tested new variants of the faux pas test in a set of control experiments.
Our first control experiment using a likelihood framing of the belief question (faux pas likelihood test), showed that GPT-4, and to a lesser extent GPT-3.5, correctly identified the mental state of both the victim and the speaker and selected as the most likely explanation the speaker not knowing or remembering the relevant knowledge that made their statement inappropriate. Despite this, both models consistently provided an incorrect response (at least when compared against human responses) when asked whether the speaker knew or remembered this knowledge, responding that there was insufficient information provided. In line with the hyperconservatism hypothesis, these findings imply that, while GPT models can identify unintentional offence as the most likely explanation, their default responses do not commit to this explanation. This finding is consistent with longitudinal evidence that GPT models have become more reluctant to answer opinion questions over time 39 .
Further supporting that the failures of GPT at recognizing faux pas were due to hyperconservatism in answering the belief question rather than a failure of inference, a second experiment using the belief likelihood test showed that GPT responses integrated information in the story to accurately interpret the speaker’s mental state. When the utterance suggested that the speaker knew, GPT responses acknowledged the higher likelihood of the ‘knew’ explanation. LLaMA2-70B, on the other hand, did not differentiate between scenarios where the speaker was implied to know and when there was no information one way or another, raising the concern that the perfect performance of LLaMA2-70B on this task may be illusory.
The pattern of failures and successes of GPT models on the faux pas test and its variants may be the result of their underlying architecture. In addition to transformers (generative algorithms that produce text output), GPT models also include mitigation measures to improve factuality and avoid users’ overreliance on them as sources 38 . These measures include training to reduce hallucinations, the propensity of GPT models to produce nonsensical content or fabricate details that are not true in relation to the provided content. Failure on the faux pas test may be an exercise of caution driven by these mitigation measures, as passing the test requires committing to an explanation that lacks full evidence. This caution can also explain differences between tasks: both the faux pas and hinting tests require speculation to generate correct answers from incomplete information. However, while the hinting task allows for open-ended generation of text in ways to which LLMs are well suited, answering the faux pas test requires going beyond this speculation in order to commit to a conclusion.
The cautionary epistemic policy guiding the responses of GPT models introduces a fundamental difference in the way that humans and GPT models respond to social uncertainty 40 . In humans, thinking is, first and last, for the sake of doing 41 , 42 . Humans generally find uncertainty in social environments to be aversive and will incur additional costs to reduce it 43 . Theory of mind is crucial in reducing such uncertainty; the ability to reason about mental states—in combination with information about context, past experience and knowledge of social norms—helps individual reduce uncertainty and commit to likely hypotheses, allowing for successful navigation of the social environment as active agents 44 , 45 . GPT models, on the other hand, respond conservatively despite having access to tools to reduce uncertainty. The dissociation we describe between speculative reasoning and commitment mirrors recent evidence that, while GPT models demonstrate sophisticated and accurate performance in reasoning tasks about belief states, they struggle to translate this reasoning into strategic decisions and actions 46 .
These findings highlight a dissociation between competence and performance 35 , suggesting that GPT models may be competent, that is, have the technical sophistication to compute mentalistic-like inferences but perform differently from humans under uncertain circumstances as they do not compute these inferences spontaneously to reduce uncertainty. Such a distinction can be difficult to capture with quantitative approaches that code only for target response features, as machine failures and successes are the result of non-human-like processes 30 (see Supplementary Information section 7 for a preliminary qualitative breakdown of how GPT models’ successes on the new version of the faux pas test may not necessarily reflect perfect or human-like reasoning).
While LLMs are designed to emulate human-like responses, this does not mean that this analogy extends to the underlying cognition giving rise to those responses 47 . In this context, our findings imply a difference in how humans and GPT models trade off the costs associated with social uncertainty against the costs associated with prolonged deliberation 48 . This difference is perhaps not surprising considering that resolving uncertainty is a priority for brains adapted to deal with embodied decisions, such as deciding whether to approach or avoid, fight or flight, or cooperate or defect. GPT models and other LLMs do not operate within an environment and are not subject to the processing constraints that biological agents face to resolve competition between action choices, so may have limited advantages in narrowing the future prediction space 46 , 49 , 50 .
The dis-embodied cognition of GPT models could explain failures in recognizing faux pas, but they may also underlie their success on other tests. One example is the false belief test, one of the most widely used tools so far for testing the performance of LLMs on social cognitive tasks 19 , 21 , 22 , 23 , 25 , 51 , 52 . In this test, participants are presented with a story where a character’s belief about the world (the location of the item) differs from the participant’s own belief. The challenge in these stories is not remembering where the character last saw the item but rather in reconciling the incongruence between conflicting mental states. This is challenging for humans, who have their own perspective, their own sense of self and their own ability to track out-of-sight objects. However, if a machine does not have its own self-perspective because it is not subject to the constraints of navigating a body through an environment, as with GPT 53 , then tracking the belief of a character in a story does not pose the same challenge.
An important direction for future research will be to examine the impact of these non-human decision behaviours on second-person, real-time human–machine interactions 54 , 55 . Failure of commitment by GPT models, for example, may lead to negative affect in human conversational partners. However, it may also foster curiosity 40 . Understanding how GPTs’ performance on mentalistic inferences (or their absences) influences human social cognition in dynamically unfolding social interactions is an open challenge for future work.
The LLM landscape is fast-moving. Our findings highlight the importance of systematic testing and proper validation in human samples as a necessary foundation. As artificial intelligence (AI) continues to evolve, it also becomes increasingly important to heed calls for open science and open access to these models 32 . Direct access to the parameters, data and documentation used to construct models can allow for targeted probing and experimentation into the key parameters affecting social reasoning, informed by and building on comparisons with human data. As such, open models can not only serve to accelerate the development of future AI technologies but also serve as models of human cognition.
Ethical compliance
The research was approved by the local ethical committee (ASL 3 Genovese; protocol no. 192REG2015) and was carried out in accordance with the principles of the revised Helsinki Declaration.
Experimental model details
We tested two versions of OpenAI’s GPT: version 3.5, which was the default model at the time of testing, and version 4, which was the state-of-the-art model with enhanced reasoning, creativity and comprehension relative to previous models ( https://chat.openai.com/ ). Each test was delivered in a separate chat: GPT is capable of learning within a chat session, as it can remember both its own and the user’s previous messages to adapt its responses accordingly, but it does not retain this memory across new chats. As such, each new iteration of a test may be considered a blank slate with a new naive participant. The dates of data collection for the different stages are reported in Table 1 .
Three LLaMA2-Chat models were tested. These models were trained on sets of different sizes: 70, 13 and 7 billion tokens. All LLaMA2-Chat responses were collected using set parameters with the prompt, ‘You are a helpful AI assistant’, a temperature of 0.7, the maximum number of new tokens set at 512, a repetition penalty of 1.1, and a Top P of 0.9. Langchain’s conversation chain was used to create a memory context within individual chat sessions. Responses from all LLaMA2-Chat models were found to include a number of non-codable responses (for example, repeating the question without answering it), and these were regenerated individually and included with the full response set. For the 70B model, these non-responses were rare, but for the 13B and 7B models they were common enough to cause concern about the quality of these data. As such, only the responses of the 70B model are reported in the main manuscript and a comparison of this model against the smaller two is reported in Supplementary Information section 1 . Details and dates of data collection are reported in Table 1 .
For each test, we collected 15 sessions for each LLM. A session involved delivering all items of a single test within the same chat window. GPT-4 was subject to a 25-message limit per 3 h; to minimize interference, a single experimenter delivered all tests for GPT-4, while four other experimenters shared the duty of collecting responses from GPT-3.5.
Human participants were recruited online through the Prolific platform and the study was hosted on SoSci. We recruited native English speakers between the ages of 18 and 70 years with no history of psychiatric conditions and no history of dyslexia in particular. Further demographic data were not collected. We aimed to collect around 50 participants per test (theory of mind battery) or item (belief likelihood test, false belief perturbations). Thirteen participants who appeared to have generated their answers using LLMs or whose responses did not answer the questions were excluded. The final human sample was N = 1,907 (Table 1 ). All participants provided informed consent through the online survey and received monetary compensation in return for their participation at a rate of GBP£12 h −1 .
We selected a series of tests typically used in evaluating theory of mind capacity in human participants.
False belief assess the ability to infer that another person possesses knowledge that differs from the participant’s own (true) knowledge of the world. These tests consist of test items that follow a particular structure: character A and character B are together, character A deposits an item inside a hidden location (for example, a box), character A leaves, character B moves the item to a second hidden location (for example, a cupboard) and then character A returns. The question asked to the participant is: when character A returns, will they look for the item in the new location (where it truly is, matching the participant’s true belief) or the old location (where it was, matching character A’s false belief)?
In addition to the false belief condition, the test also uses a true belief control condition, where rather than move the item that character A hid, character B moves a different item to a new location. This is important for interpreting failures of false belief attribution as they ensure that any failures are not due to a recency effect (referring to the last location reported) but instead reflect an accurate belief tracking.
We adapted four false/true belief scenarios from the sandbox task used by Bernstein 33 and generated three novel items, each with false and true belief versions. These novel items followed the same structure as the original published items but with different details such as names, locations or objects to control for familiarity with the text of published items. Two story lists (false belief A, false belief B) were generated for this test such that each story only appeared once within a testing session and alternated between false and true belief depending on the session. In addition to the standard false/true belief scenarios, two additional catch stories were tested that involved minor alterations to the story structure. The results of these items are not reported here as they go beyond the goals of the current study.
Comprehending an ironic remark requires inferring the true meaning of an utterance (typically the opposite of what is said) and detecting the speaker’s mocking attitude, and this has been raised as a key challenge for AI and LLMs 19 .
Irony comprehension items were adapted from an eye-tracking study 34 in which participants read vignettes where a character made an ironic or non-ironic statement. Twelve items were taken from these stimuli that in the original study were used as comprehension checks. Items were abbreviated to end following the ironic or non-ironic utterance.
Two story lists were generated for this test (irony A, irony B) such that each story only appeared once within a testing session and alternated between ironic and non-ironic depending on the session. Responses were coded as 1 (correct) or 0 (incorrect). During coding, we noted some inconsistencies in the formulation of both GPT models’ responses where in response to the question of whether the speaker believed what they had said, they might respond with, ‘Yes, they did not believe that…’. Such internally contradictory responses, where the models responded with a ‘yes’ or ‘no’ that was incompatible with the follow-up explanation, were coded on the basis of whether or not the explanation showed appreciation of the irony—the linguistic failures of these models in generating a coherent answer are not of direct interest to the current study as these failures (1) were rare and (2) did not render the responses incomprehensible.
The faux pas test 13 presents a context in which one character makes an utterance that is unintentionally offensive to the listener because the speaker does not know or does not remember some key piece of information.
Following the presentation of the scenario, we presented four questions:
‘In the story did someone say something that they should not have said?’ [The correct answer is always ‘yes’]
‘What did they say that they should not have said?’ [Correct answer changes for each item]
A comprehension question to test understanding of story events [Question changes for every item]
A question to test awareness of the speaker’s false belief phrased as, ‘Did [the speaker] know that [what they said was inappropriate]?’ [Question changes for every item. The correct answer is always ‘no’]
These questions were asked at the same time as the story was presented. Under the original coding criteria, participants must answer all four questions correctly for their answer to be considered correct. However, in the current study we were interested primarily in the response to the final question testing whether the responder understood the speaker’s mental state. When examining the human data, we noticed that several participants responded incorrectly to the first item owing to an apparent unwillingness to attribute blame (for example ‘No, he didn’t say anything wrong because he forgot’). To focus on the key aspect of faux pas understanding that was relevant to the current study, we restricted our coding to only the last question (1 (correct if the answer was no) or 0 (for anything else); see Supplementary Information section 5 for an alternative coding that follows the original criteria, as well as a recoding where we coded as correct responses where the correct answer was mentioned as a possible explanation but was not explicitly endorsed).
As well as the 10 original items used in Baron-Cohen et al. 13 , we generated five novel items for this test that followed the same structure and logic as the original items, resulting in 15 items overall.
Hinting task
The hinting task 14 assesses the understanding of indirect speech requests through the presentation of ten vignettes depicting everyday social interactions that are presented sequentially. Each vignette ends with a remark that can be interpreted as a hint.
A correct response identifies both the intended meaning of the remark and the action that it is attempting to elicit. In the original test, if the participant failed to answer the question fully the first time, they were prompted with additional questioning 14 , 56 . In our adapted implementation, we removed this additional questioning and coded responses as a binary (1 (correct) or 0 (incorrect)) using the evaluation criteria listed in Gil et al. 56 . Note that this coding offers more conservative estimates of hint comprehension than in previous studies.
In addition to 10 original items sourced from Corcoran 14 , we generated a further 6 novel hinting test items, resulting in 16 items overall.
The strange stories 15 , 16 offer a means of testing more advanced mentalizing abilities such as reasoning about misdirection, manipulation, lying and misunderstanding, as well as second- or higher-order mental states (for example, A knows that B believes X …). The advanced abilities that these stories measure make them suitable for testing higher-functioning children and adults. In this test, participants are presented with a short vignette and are asked to explain why a character says or does something that is not literally true.
Each question comes with a specific set of coding criteria and responses can be awarded 0, 1 or 2 points depending on how fully it explains the utterance and whether or not it explains it in mentalistic terms 16 . See Supplementary Information section 6 for a description of the frequency of partial successes.
In addition to the 8 original mental stories, we generated 4 novel items, resulting in 12 items overall. The maximum number of points possible was 24, and individual session scores were converted to a proportional score for analysis.
Testing protocol
For the theory of mind battery, the order of items was set for each test, with original items delivered first and novel items delivered last. Each item was preceded by a preamble that remained consistent across all tests. This was then followed by the story description and the relevant question(s). After each item was delivered, the model would respond and then the session advanced to the next item.
For GPT models, items were delivered using the chat web interface. For LLaMA2-Chat models, delivery of items was automated through a custom script. For humans, items were presented with free text response boxes on separate pages of a survey so that participants could write out their responses to each question (with a minimum character count of 2).
Faux pas likelihood test
To test alternative hypotheses of why the tested models performed poorly at the faux pas test, we ran a follow-up study replicating just the faux pas test. This replication followed the same procedure as the main study with one major difference.
The original wording of the question was phrased as a straightforward yes/no question that tested the subject’s awareness of a speaker’s false belief (for example, ‘Did Richard remember James had given him the toy aeroplane for his birthday?’). To test whether the low scores on this question were due to the models’ refusing to commit to a single explanation in the face of ambiguity, we reworded this to ask in terms of likelihood: ‘Is it more likely that Richard remembered or did not remember that James had given him the toy aeroplane for his birthday?’
Another difference from the original study was that we included a follow-up prompt in the rare cases where the model failed to provide clear reasoning on an incorrect response. The coding criteria for this follow-up were in line with coding schemes used in other studies with a prompt system 14 , where an unprompted correct answer was given 2 points, a correct answer following a prompt was given 1 point and incorrect answers following a prompt were given 0 points. These points were then rescaled to a proportional score to allow comparison against the original wording.
During coding by the human experimenters, a qualitative description of different subtypes of response (beyond 0–1–2 points) emerged, particularly noting recurring patterns in responses that were marked as successes. This exploratory qualitative breakdown is reported along with further detail on the prompting protocol in Supplementary Information section 7 .
Belief likelihood test
To manipulate the likelihood that the speaker knew or did not know, we developed a new set of variants of the faux pas likelihood test. For each test item, all newly generated for this control study, we created three variants: a faux pas variant, a neutral variant and a knowledge-implied variant. In the faux pas variant, the utterance suggested that the speaker did not know the context. In the neutral variant, the utterance suggested neither that they knew nor did not know. In the knowledge-implied variant, the utterance suggested that the speaker knew (for the full text of all items, see Supplementary Appendix 2 ). For each variant, the core story remained unchanged, for example:
Michael was a very awkward child when he was at high school. He struggled with making friends and spent his time alone writing poetry. However, after he left he became a lot more confident and sociable. At his ten-year high school reunion he met Amanda, who had been in his English class. Over drinks, she said to him,
followed by the utterance, which varied across conditions:
'I don't know if you remember this guy from school. He was in my English class. He wrote poetry and he was super awkward. I hope he isn't here tonight.'
'Do you know where the bar is?'
Knowledge implied:
'Do you still write poetry?'
The belief likelihood test was administered in the same way as with previous tests with the exception that responses were kept independent so that there was no risk of responses being influenced by other variants. For ChatGPT models, this involved delivering each item within a separate chat session for 15 repetitions of each item. For LLaMA2-70B, this involved removing the Langchain conversation chain allowing for within-session memory context. Human participants were recruited separately to answer a single test item, with at least 50 responses collected for each item (total N = 900). All other details of the protocol were the same.
Quantification and statistical analysis
Response coding.
After each session in the theory of mind battery and faux pas likelihood test, the responses were collated and coded by five human experimenters according to the pre-defined coding criteria for each test. Each experimenter was responsible for coding 100% of sessions for one test and 20% of sessions for another. Inter-coder per cent agreement was calculated on the 20% of shared sessions, and items where coders showed disagreement were evaluated by all raters and recoded. The data available on the OSF are the results of this recoding. Experimenters also flagged individual responses for group evaluation if they were unclear or unusual cases, as and when they arose. Inter-rater agreement was computed by calculating the item-wise agreement between coders as 1 or 0 and using this to calculate a percentage score. Initial agreement across all double-coded items was over 95%. The lowest agreement was for the human and GPT-3.5 responses of strange stories, but even here agreement was over 88%. Committee evaluation by the group of experimenters resolved all remaining ambiguities.
For the belief likelihood test, responses were coded according to whether they endorsed the ‘knew’ explanation or ‘didn’t know’ explanation, or whether they did not endorse either as more likely than the other. Outcomes ‘knew’, ‘unsure’ and ‘didn’t know’ were assigned a numerical coding of +1, 0 and −1, respectively. GPT models adhered closely to the framing of the question in their answer, but humans were more variable and sometimes provided ambiguous responses (for example, ‘yes’, ‘more likely’ and ‘not really’) or did not answer the question at all (‘It doesn’t matter’ and ‘She didn’t care’). These responses were rare, constituting only ~2.5% of responses and were coded as endorsing the ‘knew’ explanation if they were affirmative (‘yes’) and the ‘didn’t know’ explanation if they were negative.
Statistical analysis
Comparing llms against human performance.
Scores for individual responses were scaled and averaged to obtain a proportional score for each test session in order to create a performance metric that could be compared directly across different theory of mind tests. Our goal was to compare LLMs’ performance across different tests against human performance to see how these models performed on theory of mind tests relative to humans. For each test, we compared the performance of each of the three LLMs against human performance using a set of Holm-corrected two-way Wilcoxon tests. Effect sizes for Wilcoxon tests were calculated by dividing the test statistic Z by the square root of the total sample size, and 95% CIs of the effect size were bootstrapped over 1,000 iterations. All non-significant results were further examined using corresponding Bayesian tests represented as a Bayes factor (BF 10 ) under continuous prior distribution (Cauchy prior width r = 0.707). Bayes factors were computed in JASP 0.18.3 with a random seed value of 1. The results of the false belief test were not subjected to inferential statistics owing to the ceiling performance and lack of variance across models.
Novel items
For each publicly available test (all tests except for irony), we generated novel items that followed the same logic as the original text but with different details and text to control for low-level familiarity with the scenarios through inclusion in the LLM training sets. For each of these tests, we compared the performance of all LLMs on these novel items against the validated test items using Holm-corrected two-way Wilcoxon tests. Non-significant results were followed up with corresponding Bayesian tests in JASP. Significantly poorer performance on novel items than original items would indicate a strong likelihood that the good performance of a language model can be attributed to inclusion of these texts in the training set. Note that, while the open-ended format of more complex tasks like hinting and strange stories makes this a convincing control for these tests, they are of limited strength for tasks like false belief and faux pas that use a regular internal structure that make heuristics or ‘Clever Hans’ solutions possible 27 , 36 .
We calculated the count frequency of the different response types (‘didn’t know’, ‘unsure’ and ‘knew’) for each variant and each model. Then, for each model we conducted two chi-square tests that compared the distribution of these categorical responses to the faux pas variant against the neutral, and to the neutral variant against the knowledge implied. A Holm correction was applied to the eight chi-square tests to account for multiple comparisons. The non-significant result was further examined with a Bayesian contingency table in JASP.
Reporting summary
Further information on research design is available in the Nature Portfolio Reporting Summary linked to this article.
Data availability
All resources are available on a repository stored on the Open Science Framework (OSF) under a Creative Commons Attribution Non-Commercial 4.0 International (CC-BY-NC) license at https://osf.io/fwj6v . This repository contains all test items, data and code reported in this study. Test items and data are available in an Excel file that includes the text of every item delivered in each test, the full text responses to each item and the code assigned to each response. This file is available at https://osf.io/dbn92 Source data are provided with this paper.
Code availability
The code used for all analysis in the main manuscript and Supplementary Information is included as a Markdown file at https://osf.io/fwj6v . The data used by the analysis files are available as a number of CSV files under ‘scored_data/’ in the repository, and all materials necessary for replicating the analysis can be downloaded as a single .zip file within the main repository titled ‘Full R Project Code.zip’ at https://osf.io/j3vhq .
Van Ackeren, M. J., Casasanto, D., Bekkering, H., Hagoort, P. & Rueschemeyer, S.-A. Pragmatics in action: indirect requests engage theory of mind areas and the cortical motor network. J. Cogn. Neurosci. 24 , 2237–2247 (2012).
Article PubMed Google Scholar
Apperly, I. A. What is ‘theory of mind’? Concepts, cognitive processes and individual differences. Q. J. Exp. Psychol. 65 , 825–839 (2012).
Article Google Scholar
Premack, D. & Woodruff, G. Does the chimpanzee have a theory of mind? Behav. Brain Sci. 1 , 515–526 (1978).
Apperly, I. A., Riggs, K. J., Simpson, A., Chiavarino, C. & Samson, D. Is belief reasoning automatic? Psychol. Sci. 17 , 841–844 (2006).
Kovács, Á. M., Téglás, E. & Endress, A. D. The social sense: susceptibility to others’ beliefs in human infants and adults. Science 330 , 1830–1834 (2010).
Apperly, I. A., Warren, F., Andrews, B. J., Grant, J. & Todd, S. Developmental continuity in theory of mind: speed and accuracy of belief–desire reasoning in children and adults. Child Dev. 82 , 1691–1703 (2011).
Southgate, V., Senju, A. & Csibra, G. Action anticipation through attribution of false belief by 2-year-olds. Psychol. Sci. 18 , 587–592 (2007).
Article CAS PubMed Google Scholar
Kampis, D., Kármán, P., Csibra, G., Southgate, V. & Hernik, M. A two-lab direct replication attempt of Southgate, Senju and Csibra (2007). R. Soc. Open Sci. 8 , 210190 (2021).
Article CAS PubMed PubMed Central Google Scholar
Kovács, Á. M., Téglás, E. & Csibra, G. Can infants adopt underspecified contents into attributed beliefs? Representational prerequisites of theory of mind. Cognition 213 , 104640 (2021).
Baron-Cohen, S., Wheelwright, S., Hill, J., Raste, Y. & Plumb, I. The ‘Reading the Mind in the Eyes’ Test revised version: a study with normal adults, and adults with Asperger syndrome or high-functioning autism. J. Child Psychol. Psychiatry Allied Discip. 42 , 241–251 (2001).
Article CAS Google Scholar
Wimmer, H. & Perner, J. Beliefs about beliefs: representation and constraining function of wrong beliefs in young children’s understanding of deception. Cognition 13 , 103–128 (1983).
Perner, J., Leekam, S. R. & Wimmer, H. Three-year-olds’ difficulty with false belief: the case for a conceptual deficit. Br. J. Dev. Psychol. 5 , 125–137 (1987).
Baron-Cohen, S., O’Riordan, M., Stone, V., Jones, R. & Plaisted, K. Recognition of faux pas by normally developing children and children with asperger syndrome or high-functioning autism. J. Autism Dev. Disord. 29 , 407–418 (1999).
Corcoran, R. Inductive reasoning and the understanding of intention in schizophrenia. Cogn. Neuropsychiatry 8 , 223–235 (2003).
Happé, F. G. E. An advanced test of theory of mind: understanding of story characters’ thoughts and feelings by able autistic, mentally handicapped, and normal children and adults. J. Autism Dev. Disord. 24 , 129–154 (1994).
White, S., Hill, E., Happé, F. & Frith, U. Revisiting the strange stories: revealing mentalizing impairments in autism. Child Dev. 80 , 1097–1117 (2009).
Apperly, I. A. & Butterfill, S. A. Do humans have two systems to track beliefs and belief-like states? Psychol. Rev. 116 , 953 (2009).
Wiesmann, C. G., Friederici, A. D., Singer, T. & Steinbeis, N. Two systems for thinking about others’ thoughts in the developing brain. Proc. Natl Acad. Sci. USA 117 , 6928–6935 (2020).
Bubeck, S. et al. Sparks of artificial general intelligence: early experiments with GPT-4. Preprint at https://doi.org/10.48550/arXiv.2303.12712 (2023).
Srivastava, A. et al. Beyond the imitation game: quantifying and extrapolating the capabilities of language models. Preprint at https://doi.org/10.48550/arXiv.2206.04615 (2022).
Dou, Z. Exploring GPT-3 model’s capability in passing the Sally-Anne Test A preliminary study in two languages. Preprint at OSF https://doi.org/10.31219/osf.io/8r3ma (2023).
Kosinski, M. Theory of mind may have spontaneously emerged in large language models. Preprint at https://doi.org/10.48550/arXiv.2302.02083 (2023).
Sap, M., LeBras, R., Fried, D. & Choi, Y. Neural theory-of-mind? On the limits of social intelligence in large LMs. In Proc. 2022 Conference on Empirical Methods in Natural Language Processing (EMNLP) 3762–3780 (Association for Computational Linguistics, 2022).
Gandhi, K., Fränken, J.-P., Gerstenberg, T. & Goodman, N. D. Understanding social reasoning in language models with language models. In Advances in Neural Information Processing Systems Vol. 36 (MIT Press, 2023).
Ullman, T. Large language models fail on trivial alterations to theory-of-mind tasks. Preprint at https://doi.org/10.48550/arXiv.2302.08399 (2023).
Marcus, G. & Davis, E. How Not to Test GPT-3. Marcus on AI https://garymarcus.substack.com/p/how-not-to-test-gpt-3 (2023).
Shapira, N. et al. Clever Hans or neural theory of mind? Stress testing social reasoning in large language models. Preprint at https://doi.org/10.48550/arXiv.2305.14763 (2023).
Rahwan, I. et al. Machine behaviour. Nature 568 , 477–486 (2019).
Hagendorff, T. Machine psychology: investigating emergent capabilities and behavior in large language models using psychological methods. Preprint at https://doi.org/10.48550/arXiv.2303.13988 (2023).
Binz, M. & Schulz, E. Using cognitive psychology to understand GPT-3. Proc. Natl Acad. Sci. USA 120 , e2218523120 (2023).
Webb, T., Holyoak, K. J. & Lu, H. Emergent analogical reasoning in large language models. Nat. Hum. Behav. 7 , 1526–1541 (2023).
Frank, M. C. Openly accessible LLMs can help us to understand human cognition. Nat. Hum. Behav. 7 , 1825–1827 (2023).
Bernstein, D. M., Thornton, W. L. & Sommerville, J. A. Theory of mind through the ages: older and middle-aged adults exhibit more errors than do younger adults on a continuous false belief task. Exp. Aging Res. 37 , 481–502 (2011).
Au-Yeung, S. K., Kaakinen, J. K., Liversedge, S. P. & Benson, V. Processing of written irony in autism spectrum disorder: an eye-movement study: processing irony in autism spectrum disorders. Autism Res. 8 , 749–760 (2015).
Firestone, C. Performance vs. competence in human–machine comparisons. Proc. Natl Acad. Sci. USA 117 , 26562–26571 (2020).
Shapira, N., Zwirn, G. & Goldberg, Y. How well do large language models perform on faux pas tests? In Findings of the Association for Computational Linguistics: ACL 2023 10438–10451 (Association for Computational Linguistics, 2023)
Rescher, N. Choice without preference. a study of the history and of the logic of the problem of ‘Buridan’s ass’. Kant Stud. 51 , 142–175 (1960).
OpenAI. GPT-4 technical report. Preprint at https://doi.org/10.48550/arXiv.2303.08774 (2023).
Chen, L., Zaharia, M. & Zou, J. How is ChatGPT’s behavior changing over time? Preprint at https://doi.org/10.48550/arXiv.2307.09009 (2023).
Feldman Hall, O. & Shenhav, A. Resolving uncertainty in a social world. Nat. Hum. Behav. 3 , 426–435 (2019).
James, W. The Principles of Psychology V ol. 2 (Henry Holt & Co, 1890).
Fiske, S. T. Thinking is for doing: portraits of social cognition from daguerreotype to laserphoto. J. Personal. Soc. Psychol. 63 , 877–889 (1992).
Plate, R. C., Ham, H. & Jenkins, A. C. When uncertainty in social contexts increases exploration and decreases obtained rewards. J. Exp. Psychol. Gen. 152 , 2463–2478 (2023).
Frith, C. D. & Frith, U. The neural basis of mentalizing. Neuron 50 , 531–534 (2006).
Koster-Hale, J. & Saxe, R. Theory of mind: a neural prediction problem. Neuron 79 , 836–848 (2013).
Zhou, P. et al. How far are large language models from agents with theory-of-mind? Preprint at https://doi.org/10.48550/arXiv.2310.03051 (2023).
Bonnefon, J.-F. & Rahwan, I. Machine thinking, fast and slow. Trends Cogn. Sci. 24 , 1019–1027 (2020).
Hanks, T. D., Mazurek, M. E., Kiani, R., Hopp, E. & Shadlen, M. N. Elapsed decision time affects the weighting of prior probability in a perceptual decision task. J. Neurosci. 31 , 6339–6352 (2011).
Pezzulo, G., Parr, T., Cisek, P., Clark, A. & Friston, K. Generating meaning: active inference and the scope and limits of passive AI. Trends Cogn. Sci. 28 , 97–112 (2023).
Chemero, A. LLMs differ from human cognition because they are not embodied. Nat. Hum. Behav. 7 , 1828–1829 (2023).
Brunet-Gouet, E., Vidal, N. & Roux, P. In Human and Artificial Rationalities. HAR 2023. Lecture Notes in Computer Science (eds. Baratgin, J. et al.) Vol. 14522, 107–126 (Springer, 2024).
Kim, H. et al. FANToM: a benchmark for stress-testing machine theory of mind in interactions. In Proc. 2023 Conference on Empirical Methods in Natural Language Processing (EMNLP) 14397–14413 (Association for Computational Linguistics, 2023).
Yiu, E., Kosoy, E. & Gopnik, A. Transmission versus truth, imitation versus nnovation: what children can do that large language and language-and-vision models cannot (yet). Perspect. Psychol. Sci. https://doi.org/10.1177/17456916231201401 (2023).
Redcay, E. & Schilbach, L. Using second-person neuroscience to elucidate the mechanisms of social interaction. Nat. Rev. Neurosci. 20 , 495–505 (2019).
Schilbach, L. et al. Toward a second-person neuroscience. Behav. Brain Sci. 36 , 393–414 (2013).
Gil, D., Fernández-Modamio, M., Bengochea, R. & Arrieta, M. Adaptation of the hinting task theory of the mind test to Spanish. Rev. Psiquiatr. Salud Ment. Engl. Ed. 5 , 79–88 (2012).
Download references
Acknowledgements
This work is supported by the European Commission through Project ASTOUND (101071191—HORIZON-EIC-2021-PATHFINDERCHALLENGES-01 to A.R., G.M., C.B. and S.P.). J.W.A.S. was supported by a Humboldt Research Fellowship for Experienced Researchers provided by the Alexander von Humboldt Foundation. The funders had no role in study design, data collection and analysis, decision to publish or preparation of the manuscript.
Open access funding provided by Universitätsklinikum Hamburg-Eppendorf (UKE).
Author information
Authors and affiliations.
Department of Neurology, University Medical Center Hamburg-Eppendorf, Hamburg, Germany
James W. A. Strachan, Oriana Pansardi, Eugenio Scaliti & Cristina Becchio
Cognition, Motion and Neuroscience, Italian Institute of Technology, Genoa, Italy
Dalila Albergo, Giulia Borghini, Oriana Pansardi, Eugenio Scaliti & Cristina Becchio
Center for Mind/Brain Sciences, University of Trento, Rovereto, Italy
Dalila Albergo
Department of Psychology, University of Turin, Turin, Italy
Oriana Pansardi
Department of Management, ‘Valter Cantino’, University of Turin, Turin, Italy
Eugenio Scaliti
Human Science and Technologies, University of Turin, Turin, Italy
Alien Technology Transfer Ltd, London, UK
Saurabh Gupta, Krati Saxena, Alessandro Rufo & Guido Manzi
Institute for Neural Information Processing, Center for Molecular Neurobiology, University Medical Center Hamburg- Eppendorf, Hamburg, Germany
Stefano Panzeri
Princeton Neuroscience Institute, Princeton University, Princeton, NJ, USA
Michael S. A. Graziano
You can also search for this author in PubMed Google Scholar
Contributions
J.W.A.S., A.R., G.M., M.S.A.G. and C.B. conceived the study. J.W.A.S., D.A., G.B., O.P. and E.S. designed the tasks and performed the experiments including data collection with humans and GPT models, response coding and curation of the dataset. S.G., K.S. and G.M. collected data from LLaMA2-Chat models. J.W.A.S. performed the analyses and wrote the manuscript with input from C.B., S.P. and M.S.A.G. All authors contributed to the interpretation and editing of the manuscript. C.B. supervised the work. A.R., G.M., S.P. and C.B. acquired the funding. D.A., G.B., O.P. and E.S. contributed equally to the work.
Corresponding authors
Correspondence to James W. A. Strachan or Cristina Becchio .
Ethics declarations
Competing interests.
The authors declare no competing interests.
Peer review
Peer review information.
Nature Human Behaviour thanks the anonymous reviewers for their contribution to the peer review of this work. Peer reviewer reports are available.
Additional information
Publisher’s note Springer Nature remains neutral with regard to jurisdictional claims in published maps and institutional affiliations.
Supplementary information
Supplementary information.
Supplementary Figs. 1–8, Tables 1–4, additional methodological details, analyses and discussion, Appendix 1 (full text of false belief perturbations adapted from Ullman (2023)) and Appendix 2 (full text of items generated for the belief likelihood test).
Reporting Summary
Peer review file, source data fig. 1.
Raw score data on the full theory of mind battery for all models used to generate Fig. 1a,b.
Source Data Fig. 2
Zip file containing two CSV files used to generate Fig. 2. Fig2A_data.csv: raw score data with GPT models’ performance in the Faux Pas Likelihood test, used to generate Fig. 2a. Fig2B_data.csv: raw score data on the belief likelihood test for all models used to generate Fig. 2b.
Rights and permissions
Open Access This article is licensed under a Creative Commons Attribution 4.0 International License, which permits use, sharing, adaptation, distribution and reproduction in any medium or format, as long as you give appropriate credit to the original author(s) and the source, provide a link to the Creative Commons licence, and indicate if changes were made. The images or other third party material in this article are included in the article’s Creative Commons licence, unless indicated otherwise in a credit line to the material. If material is not included in the article’s Creative Commons licence and your intended use is not permitted by statutory regulation or exceeds the permitted use, you will need to obtain permission directly from the copyright holder. To view a copy of this licence, visit http://creativecommons.org/licenses/by/4.0/ .
Reprints and permissions
About this article
Cite this article.
Strachan, J.W.A., Albergo, D., Borghini, G. et al. Testing theory of mind in large language models and humans. Nat Hum Behav (2024). https://doi.org/10.1038/s41562-024-01882-z
Download citation
Received : 14 August 2023
Accepted : 05 April 2024
Published : 20 May 2024
DOI : https://doi.org/10.1038/s41562-024-01882-z
Share this article
Anyone you share the following link with will be able to read this content:
Sorry, a shareable link is not currently available for this article.
Provided by the Springer Nature SharedIt content-sharing initiative
Quick links
- Explore articles by subject
- Guide to authors
- Editorial policies
Sign up for the Nature Briefing newsletter — what matters in science, free to your inbox daily.

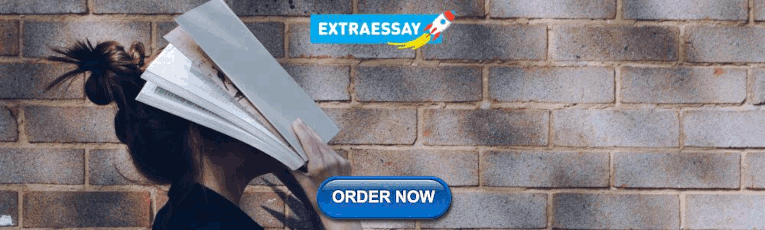
IMAGES
VIDEO
COMMENTS
The term "modular approach" refers to learning that takes the form of individualized instruction and allows students to use Self-Learning Modules (SLMs) in the print or advanced format/electronic ...
The purpose of this descriptive paper was to explore and synthesize literature related to understanding modular learning and how it can be implemented effectively so faculty members embrace its use. An in-depth review of literature addressed topics including, Educational Theories supporting modular learning, the development of modular learning,
The COVID-19 pandemic brought extraordinary challenges to K-12 students in using modular distance learning. According to Transactional Distance Theory (TDT), which is defined as understanding the effects of distance learning in the cognitive domain, the current study constructs a theoretical framework to measure student satisfaction and Bloom's Taxonomy Theory (BTT) to measure students ...
This mode of learning have been used by the learners and teachers during the conduct of Modular Distance Learning [3], [4]. This will attempt to increase their performances of the in the school. ...
Moreover, TDT was utilized to check students' modular learning experiences in conjuction with enhacing students' achievements. This study aimed to detect the impact of modular distance learning on K-12 students during the COVID-19 pandemic and assess the cognitive factors affecting academic achieve-ment and student satisfaction.
At the same time, the neutral results of the self-learning module experience significantly impacted the GWA and rejected the null hypothesis. Thus, for a better result for this study, the researchers recommended that a standardized test be used to get a high positive correlation of modular class experiences to the learners' academic performance.
The coronavirus pandemic (COVID-19) is a global health crisis that has affected educational systems worldwide. North Eastern Mindanao State University (NEMSU), a typical countryside academic institution in the Southern Philippines, did not escape this dilemma. The advent of remote learning to continue the students' learning process has caused difficulties for both the students and the ...
Amidst of the COVID-19 crisis, the education don't stop, it must continue whether with or without physically going to school. Face-to-face learning modality is out, modular distance learning is in. At the present moment of situation; Department of Education made an urgent response to ensure the safety of learners and the teachers. On the other hand, they also ensure the continuity of quality ...
Due to Covid-19 pandemic, schools, particularly in the rural areas employed Modular Distance Learning (MDL) to ensure education continuity. This study seeks to investigate the effects of MDL in the academic performance of learners whether there is a significant difference in their performance before and after the implementation of MDL.
Assessment of the students' level of submission of assigned tasks from printed remote learning modular materials under the College of Teacher Education of NEMSU revealed that the country's digital divide became more apparent as the students navigated this new mode of the remote learning system. The coronavirus pandemic (COVID-19) is a global health crisis that has affected educational ...
Based on the decision-making rule, the research confirms the hypothesis that the modular approach is more effective in forming students' independent creativity in math learning if compared with conventional education. The research results demonstrate that 92% of students in the experimental group set about solving advanced math problems ...
International Journal of Multidisciplinary Research and Development www.allsubjectjournal.com Online ISSN: 2349-4182, Print ISSN: 2349-5979 ... Volume 8, Issue 9, 2021, Page No. 117-119 Learners' needs and challenges in modular distance learning approach Joan T PO Guimaras State College, Graduate School, Guimaras, Philippines Abstract
academic behavior and performance rejected the null hypothesis "The challenges and status of modular learning have no significant effect on learners' academic behavior and performance.". These implies that there is a significant effect between the Challenges and Status of Modular Learning as to learners' academic behavior and performance.
Evolving networks with a pressure to minimize connection costs leads to modular solutions that can retain old skills as new skills are learned. Hypothesis 2: Evolving modular networks makes reward-based learning easier, because it allows a clear separation of reward signals and learned skills. We present evidence for both hypotheses in this paper.
In the Philippine setting [], the coronavirus disease forced schools in the country to stop face-to-face learning activities and abruptly shift to a modular approach.In a practical sense, the modular approach situates Filipino learners to learn in the comfort of their homes. In this picture, the parents or guardians are the ones who aid in the entire homeschooling process.Research extant ...
The mean of the four (4) quarters before the MDL implementation is 88.25% while after the Modular Distance Learning the mean is 86%. This implies that there is a 2.25% difference between the mean ...
The researcher aimed to present the difficulties and experiences faced by the learners on Modular Distance Learning. A descriptive, qualitative research was conducted and used an online survey, interview, and observation as tools to gather data and to find out the problems encountered of the learners on this mode of learning. ... The hypothesis ...
Parents play a vital role as home facilitators. Their primary role in modular learning is to establish a connection and guide the child. (FlipScience, 2020). According to the Department of Education (DepEd), parents and guardians' perform the various roles in Modular Learning such as Module-ator, Bundy-clock, and as Home Innovator. As a
Amidst of the COVID-19 crisis, the education don’t stop, it must continue whether with or without physically going to school. Face-to-face learning modality is out, modular distance learning is in. At the present moment of situation; Department of Education made an urgent response to ensure the safety of learners and the teachers. On the other hand, they also ensure the continuity of ...
This research study concluded that the hypothesis stating that the profile of the respondents has no significant relationship to the students' achievement in TLE 7 is therefore accepted. Similarly, the hypothesis stating that the perceived support to modular distance learning modality has no significant relationship to the students' achievement ...
Large language models (LLMs) have demonstrated remarkable capabilities in natural language processing tasks, including conversation, in-context learning, reasoning, and code generation. This paper explores the potential application of LLMs in radiological information systems (RIS) and assesses the impact of integrating LLMs on RIS development and human-computer interaction. We present ChatUI ...
Abstract. At the core of what defines us as humans is the concept of theory of mind: the ability to track other people's mental states. The recent development of large language models (LLMs ...
Developing accurate machine learning models for oncology requires large-scale, high-quality multimodal datasets. However, creating such datasets remains challenging due to the complexity and heterogeneity of medical data. To address this challenge, we introduce HoneyBee, a scalable modular framework for building multimodal oncology datasets that leverages foundational models to generate ...