We will keep fighting for all libraries - stand with us!
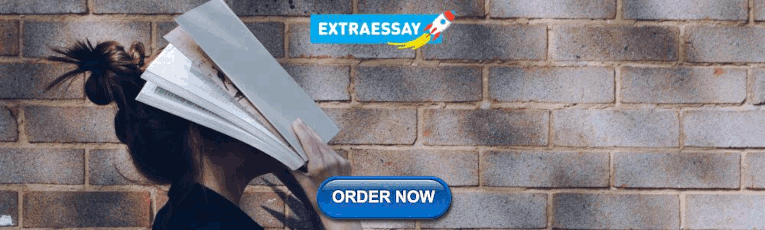
Internet Archive Audio
- This Just In
- Grateful Dead
- Old Time Radio
- 78 RPMs and Cylinder Recordings
- Audio Books & Poetry
- Computers, Technology and Science
- Music, Arts & Culture
- News & Public Affairs
- Spirituality & Religion
- Radio News Archive
- Flickr Commons
- Occupy Wall Street Flickr
- NASA Images
- Solar System Collection
- Ames Research Center
- All Software
- Old School Emulation
- MS-DOS Games
- Historical Software
- Classic PC Games
- Software Library
- Kodi Archive and Support File
- Vintage Software
- CD-ROM Software
- CD-ROM Software Library
- Software Sites
- Tucows Software Library
- Shareware CD-ROMs
- Software Capsules Compilation
- CD-ROM Images
- ZX Spectrum
- DOOM Level CD

- Smithsonian Libraries
- FEDLINK (US)
- Lincoln Collection
- American Libraries
- Canadian Libraries
- Universal Library
- Project Gutenberg
- Children's Library
- Biodiversity Heritage Library
- Books by Language
- Additional Collections
- Prelinger Archives
- Democracy Now!
- Occupy Wall Street
- TV NSA Clip Library
- Animation & Cartoons
- Arts & Music
- Computers & Technology
- Cultural & Academic Films
- Ephemeral Films
- Sports Videos
- Videogame Videos
- Youth Media
Search the history of over 866 billion web pages on the Internet.
Mobile Apps
- Wayback Machine (iOS)
- Wayback Machine (Android)
Browser Extensions
Archive-it subscription.
- Explore the Collections
- Build Collections
Save Page Now
Capture a web page as it appears now for use as a trusted citation in the future.
Please enter a valid web address
- Donate Donate icon An illustration of a heart shape
Bharatiya Bhasha Jyoti : Kannada through Hindi
Bookreader item preview, share or embed this item, flag this item for.
- Graphic Violence
- Explicit Sexual Content
- Hate Speech
- Misinformation/Disinformation
- Marketing/Phishing/Advertising
- Misleading/Inaccurate/Missing Metadata

This library of books, audio, video, and other materials from and about India is curated and maintained by Public Resource. The purpose of this library is to assist the students and the lifelong learners of India in their pursuit of an education so that they may better their status and their opportunities and to secure for themselves and for others justice, social, economic and political.
This library has been posted for non-commercial purposes and facilitates fair dealing usage of academic and research materials for private use including research, for criticism and review of the work or of other works and reproduction by teachers and students in the course of instruction. Many of these materials are either unavailable or inaccessible in libraries in India, especially in some of the poorer states and this collection seeks to fill a major gap that exists in access to knowledge.
For other collections we curate and more information, please visit the Bharat Ek Khoj page. Jai Gyan!
plus-circle Add Review comment Reviews
8,027 Views
9 Favorites
DOWNLOAD OPTIONS
In collections.
Uploaded by Public Resource on August 20, 2019
SIMILAR ITEMS (based on metadata)
Download language pack for speech
For some languages, you might need to manually download a speech pack.
Go to Start and open Settings .
Select Time & language > Language .
Select the language you want to add speech to, and then select the Next button.
Select the speech options you want included with the language.
Sign out and then sign back in for the new speech pack to be added to speech options.
Go back to Settings > Time & language > Language , select your new language, and then use the up-arrow button to move it to the top of the list and make it the default.
Go to Speech , and make sure the Speech language setting is aligned with the previous settings.
Sign out and then sign back in for the new settings to take effect.
Open language setting

Need more help?
Want more options.
Explore subscription benefits, browse training courses, learn how to secure your device, and more.

Microsoft 365 subscription benefits
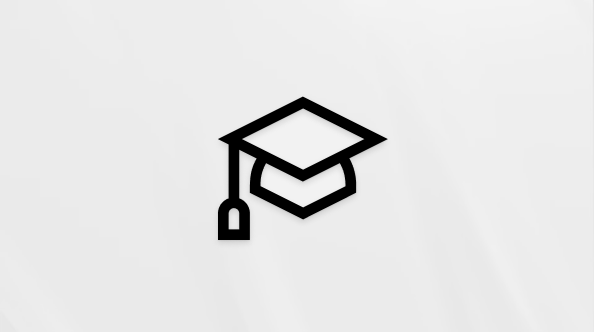
Microsoft 365 training
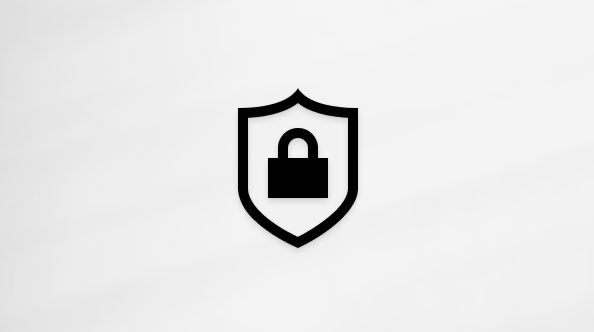
Microsoft security

Accessibility center
Communities help you ask and answer questions, give feedback, and hear from experts with rich knowledge.
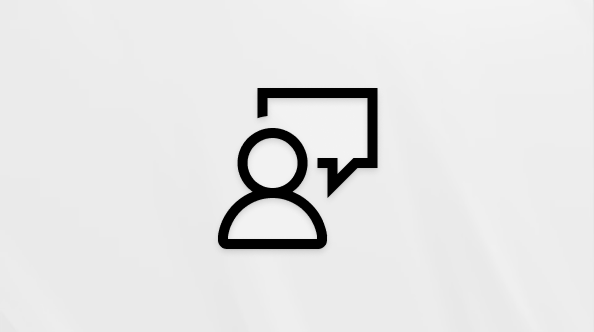
Ask the Microsoft Community
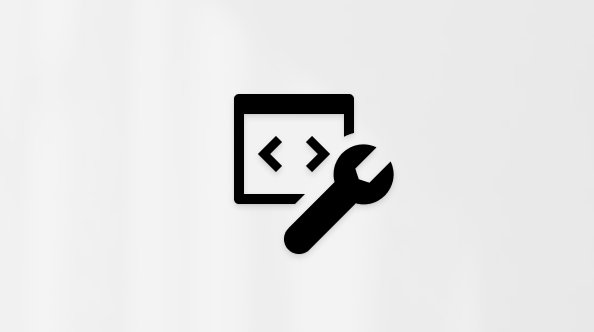
Microsoft Tech Community

Windows Insiders
Microsoft 365 Insiders
Was this information helpful?
Thank you for your feedback.
Voice speed
Text translation, source text, translation results, document translation, drag and drop.
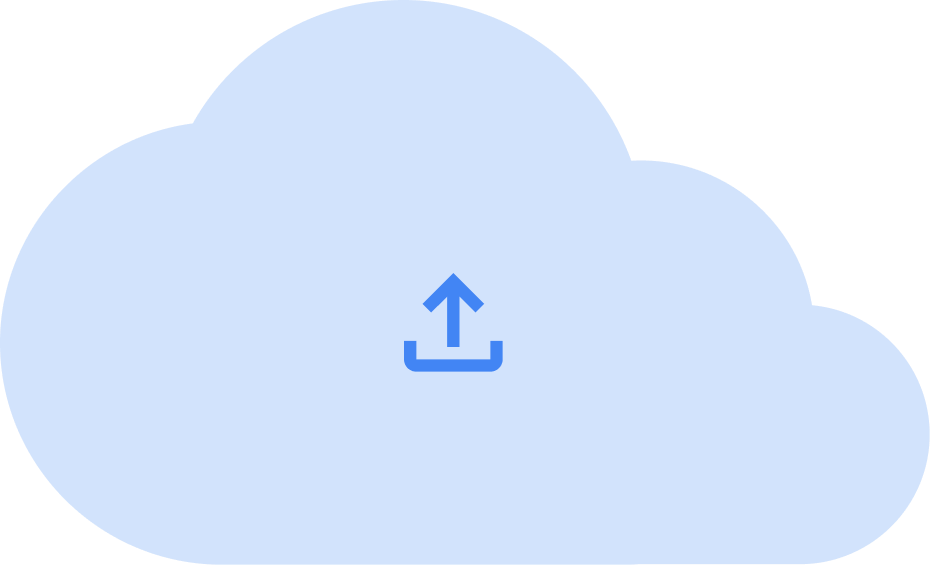
Website translation
Enter a URL
Image translation
Kannada Speech to Text
Accurately transcribe speech to text in Kannada with AI. Save time and money from transcription services
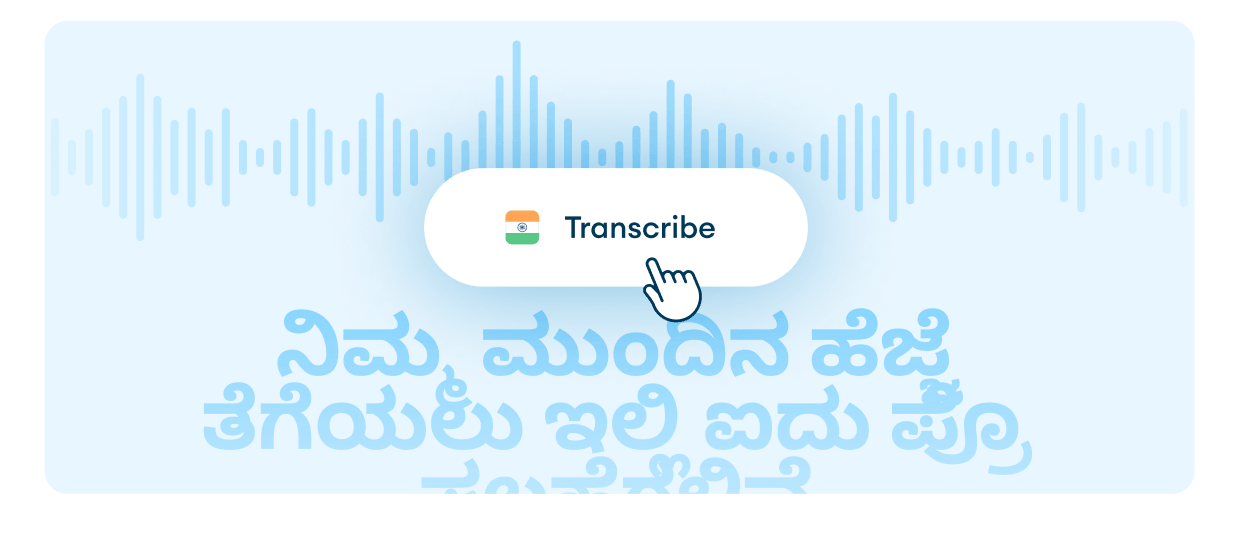
319 reviews
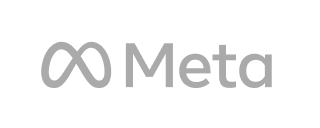
Convert speech to text in Kannada accurately
VEED automatically converts voice to text in Kannada and 100 languages straight from your Google Chrome and other browsers. Transcribe audio and video accurately in one click. Transform Kannada voice recordings into editable text, streamlining communication and saving you valuable time. You can also auto-generate subtitles !
How to convert speech to text in Kannada with AI:
Upload or record
Upload your audio or video to VEED or record one using our online audio recorder.
Auto-transcribe and translate
Auto-transcribe your video from the Subtitles menu. You can also translate your transcript to over 120 languages. Select a language and translate the transcript instantly.
Review and export
Review and edit the transcription if necessary. Just click on a line of text and start typing. Download your transcript in VTT, SRT, or TXT format.
Learn more about our Kannada speech-to-text tool:
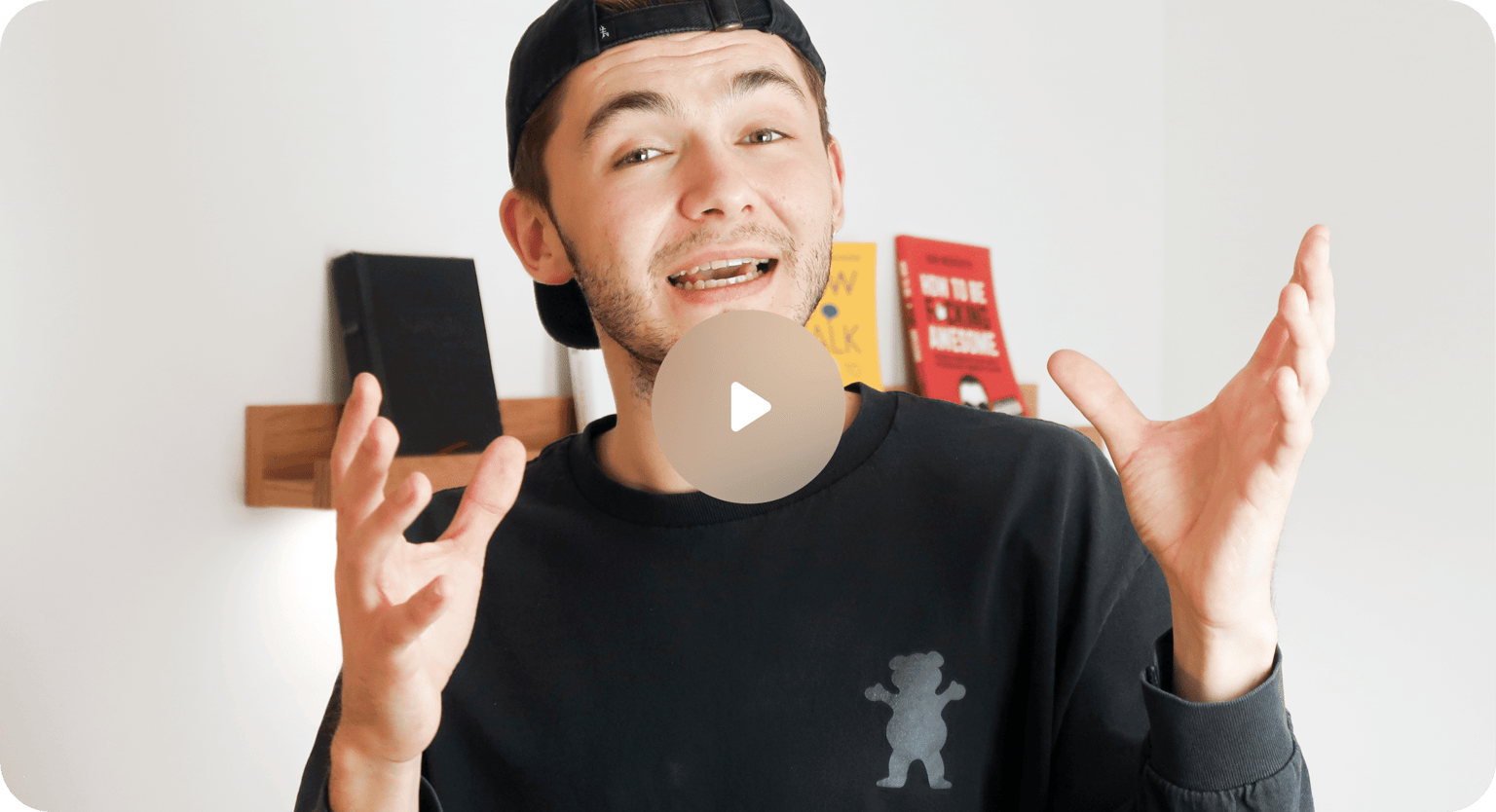
Accurate AI voice-to-text conversion in Kannada
Save time, money, and effort from manual transcription and embrace the ease of effortless speech-to-text conversion. Our cutting-edge speech recognition lets you record and watch your words magically transform into text in real-time. Our premium subscribers get unlimited transcription downloads. Check our pricing page for more info.
Kannada audio-to-text tool and AI-powered translations
Transcribe your audio and video recordings in Kannada or any spoken audio with a Kannada accent—and translate your content from English to 100 other languages. You can even use VEED to record your content. Record your audio, transcribe, and host your videos in one place.
Your one-stop online AI video editing app
Our speech recognition and voice typing app are just some of VEED’s state-of-the-art AI features. With VEED, you have access to a powerful AI video generator. Create professional videos with the help of AI - automatically add subtitles, remove background noise from your recordings, and a full range of other AI video editing tools.
How do I use the Kannada speech-to-text software?
You can transcribe your Kannada audio files to text instantly with VEED. Upload your audio or video file to VEED and our software will transcribe the original file which you can download as a TXT, VTT, or SRT file.
How do I type in Kannada by voice?
You can do it automatically with VEED! No need to type manually. VEED is your go-to app if you want to convert voice to text in Kannada—or instantly generate a Kannada voiceover in various male and female AI voice profiles. VEED’s AI can also convert text to speech!
How do I edit the Kannada transcription and translation generated by VEED?
Depending on how the speech or recording is spaced out through the video, VEED will separate the transcriptions into different boxes. Just click on each box and start typing or editing the text.
Can I change the text’s color and font of the subtitles?
Yes—but only the subtitles appearing on the video and not the TXT file. You can choose from a wide range of fonts and styles. Change its size, color, and opacity.
Discover more
- Assamese Speech to Text
- Audio Transcription
- Bengali Speech to Text
- Cantonese Speech to Text
- Chinese Speech to Text
- Dictation Transcription
- German Speech to Text
- Japanese Speech to Text
- Korean Speech to Text
- M4A to Text
- MP3 to Text
- Music Transcription
- Persian Speech to Text
- Sinhala Speech to Text
- Speech to Text Arabic
- Speech to Text Bulgarian
- Speech to Text Danish
- Speech to Text Dutch
- Speech to Text Finnish
- Speech to Text in Marathi
- Speech to Text Italian
- Speech to Text Portuguese
- Speech to Text Russian
- Speech to Text Serbian
- Speech to Text Slovak
- Speech to Text Swedish
- Speech to Text Thai
- Speech to Text Turkish
- Speech to Text Vietnamese
- Tamil Audio to Text
- Telugu Audio to Text Converter
- Transcribe Recordings to Text
- Verbatim Transcription
- Voice Memo Transcription
- Voice Message to Text
- WAV to Text
Loved by creators.
Loved by the Fortune 500
VEED has been game-changing. It's allowed us to create gorgeous content for social promotion and ad units with ease.
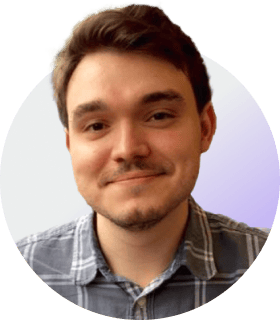
Max Alter Director of Audience Development, NBCUniversal
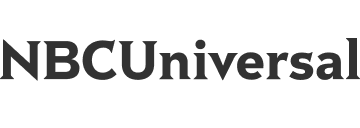
I love using VEED. The subtitles are the most accurate I've seen on the market. It's helped take my content to the next level.
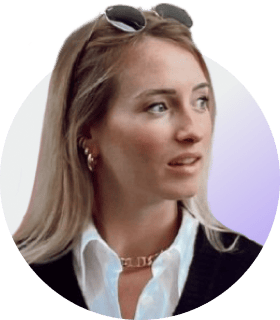
Laura Haleydt Brand Marketing Manager, Carlsberg Importers
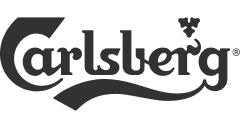
I used Loom to record, Rev for captions, Google for storing and Youtube to get a share link. I can now do this all in one spot with VEED.
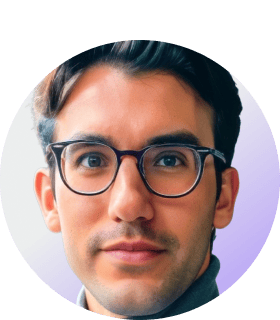
Cedric Gustavo Ravache Enterprise Account Executive, Cloud Software Group
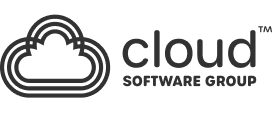
VEED is my one-stop video editing shop! It's cut my editing time by around 60% , freeing me to focus on my online career coaching business.
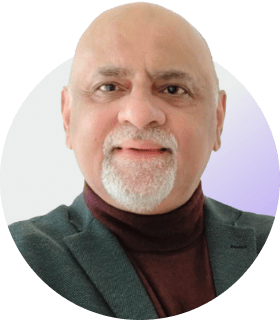
Nadeem L Entrepreneur and Owner, TheCareerCEO.com
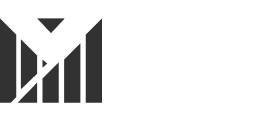
When it comes to amazing videos, all you need is VEED
Transcribe audio now
No credit card required
More than a Kannada speech-to-text software
VEED is a comprehensive and intuitive AI video editing software that allows you to do much more than just transcribing Kannada audio files to text. You can also transcribe the original recording of a video. Add subtitles to your videos to make them more accessible for everyone. It also has all the video editing tools you need. All tools are accessible online so you don’t need to install any software. Try our transcription and video editing app today!
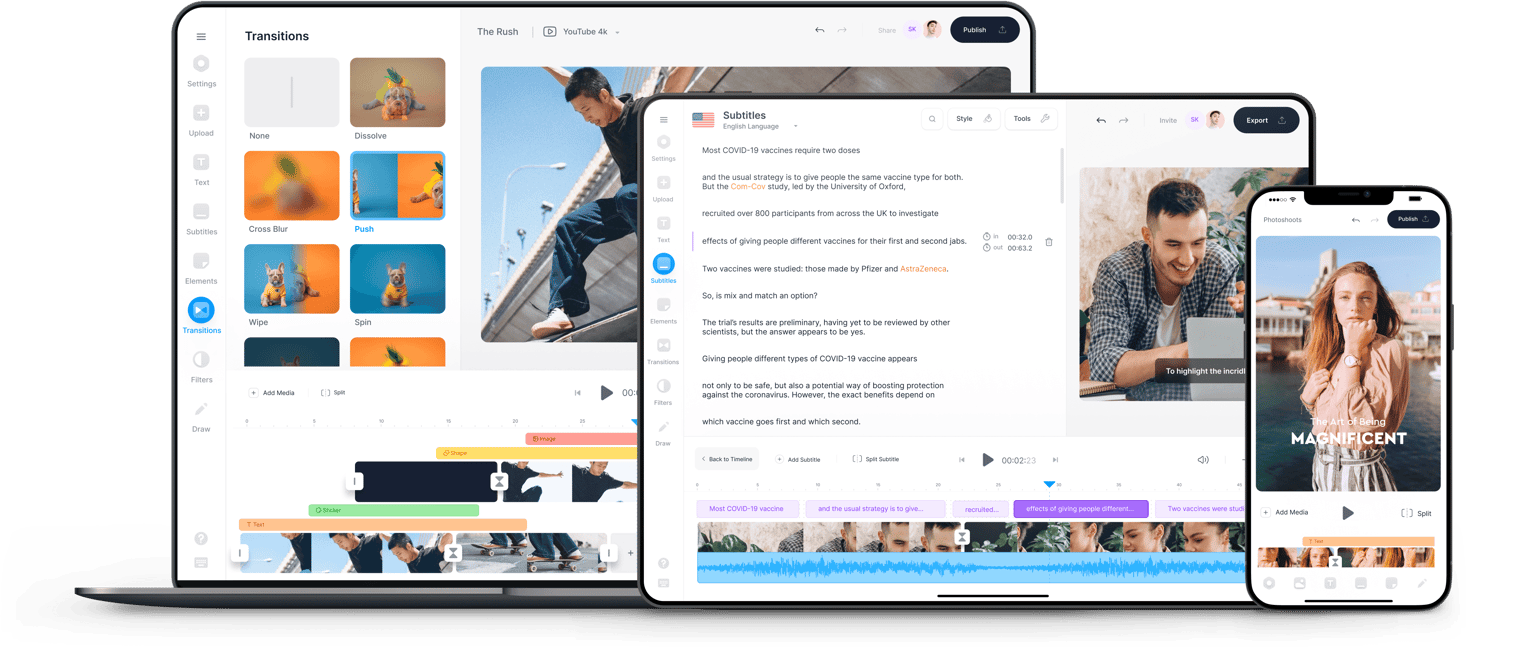
- Culture Shauk
How A Speech In Hindi Inspired Karnataka Icon Narasimhaiah
Jan 10, 2020, 7:49 PM IST
A 100-year-old high school in the heart of Bengaluru was instrumental in encouraging a young freedom fighter to fight against the tyrannical British rule. Interestingly, Hanumanthappa Narasimhaiah's journey began with a Hindi speech which needed to be translated in Kannada! In this episode of Culture ‘Shauk’ with Sunita Iyer, we revisit the story of how the Hindi language became a yardstick of inspiration for an icon of Karnataka to fight for freedom and scientific temper.
- Entertainment
![speech on kannada in hindi Salman Khan sets stage on fire for Anant Ambani, Radhika Merchant pre-wedding festivities [WATCH] ATG](https://static-ai.asianetnews.com/images/01hr1hh8y86gvb4kbqgnyhc0w0/whatsapp-image-2024-03-03-at-12-24-37-pm_254x142xt.jpg)
Salman Khan sets stage on fire for Anant Ambani, Radhika Merchant pre-wedding festivities [WATCH]
Mar 3, 2024, 12:58 PM IST
![speech on kannada in hindi Pregnant Deepika Padukone dances with Ranveer Singh at Anant Ambani, Radhika Merchant pre-wedding bash [WATCH] ATG](https://static-ai.asianetnews.com/images/01hr1ffyd3nzqzgm6ba0k87vr8/whatsapp-image-2024-03-03-at-11-45-35-am_254x142xt.jpg)
Pregnant Deepika Padukone dances with Ranveer Singh at Anant Ambani, Radhika Merchant pre-wedding bash [WATCH]
Mar 3, 2024, 12:06 PM IST
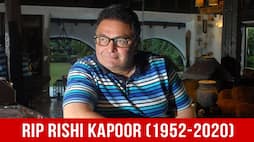
Rishi Kapoor's Life & Career: Remembering Bollywood's Evergreen Heartthrob
Apr 30, 2020, 7:56 PM IST
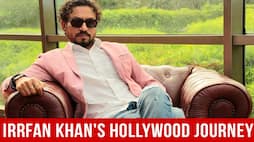
RIP Irrfan Khan: How The Versatile Actor Swept Hollywood Off Its Feet
Apr 29, 2020, 10:52 PM IST
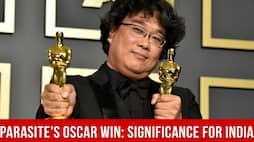
Oscars 2020: Significance Of South Korean Film Parasite's Win For India
Feb 13, 2020, 2:32 PM IST
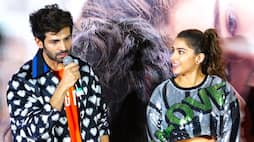
Kartik Aaryan reveals his crush on Sara Ali Khan
Jan 18, 2020, 12:51 PM IST
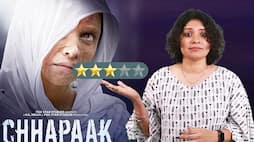
Chhapaak review: Does Deepika Padukone succeed in giving public rude wake up call to acid attack cases?
Jan 10, 2020, 4:41 PM IST
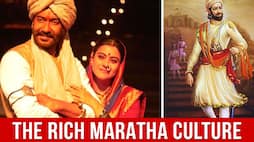
Ajay Devgn's Tanhaji Brings Focus Back On The Rich Maratha Culture
Jan 8, 2020, 8:14 PM IST
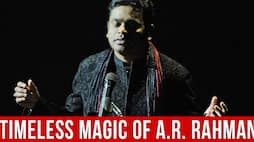
What Made AR Rahman A Musical Sensation World Over
Jan 6, 2020, 6:11 PM IST
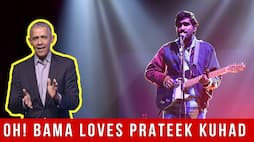
Indian Musician Prateek Kuhad's 'Cold/Mess' in Barack Obama's Favourite Music of 2019 List
Jan 2, 2020, 4:53 PM IST
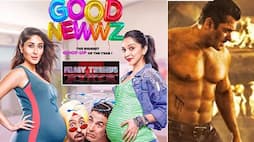
Film Trends: From Dabangg 3's box-office numbers to Good Newwz's amazing response
Dec 28, 2019, 5:58 PM IST
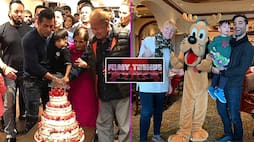
Filmy Trends: From Salman Khan's 54th birthday to Kushal Punjabi's shocking demise
Dec 27, 2019, 6:55 PM IST
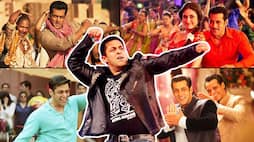
Salman Khan birthday surprise: 10 best dance moves from Sallu's movies that will stay forever
Dec 27, 2019, 1:54 PM IST
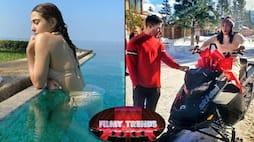
Filmy Trends: From Sara Ali Khan's Kerala vacation to Priyanka Chopra's Christmas celebrations
Dec 26, 2019, 6:40 PM IST
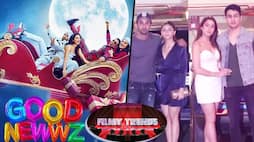
Filmy Trends: Kareena Kapoor’s Christmas party to B-town celebs’ wishes
Dec 25, 2019, 6:20 PM IST
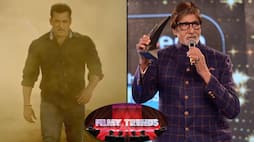
Filmy Trends: From Dabangg 3's collections to Dadasaheb Phalke for Amitabh Bachchan
Dec 23, 2019, 6:26 PM IST
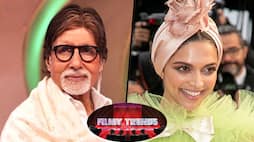
Filmy Trends: From Amitabh Bachchan's message for granddaughter to Deepika Padukone's e-taxi service
Dec 21, 2019, 5:43 PM IST
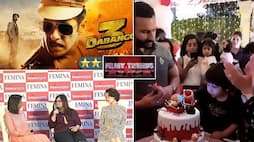
Filmy trends: From Dabangg 3's review to Taimur Ali Khan's birthday cute wishes
Dec 20, 2019, 7:00 PM IST
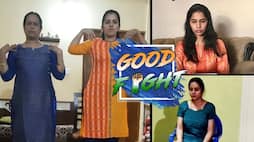
The Good Fight: Bengaluru homemakers keep families positive during coronavirus lockdown
Apr 5, 2020, 3:33 PM IST
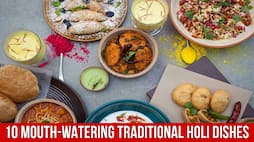
10 Mouth-Watering Traditional Holi Dishes You Must Try This Year
Mar 9, 2020, 5:53 PM IST
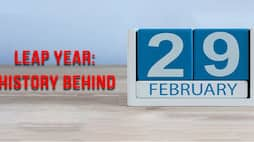
Leap Year 2020: The History Behind February 29
Feb 28, 2020, 6:14 PM IST
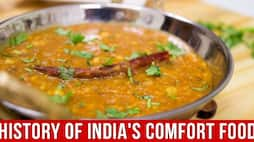
The Fascinating History of India's Favourite Comfort Food - The Humble Dal
Feb 10, 2020, 3:48 PM IST
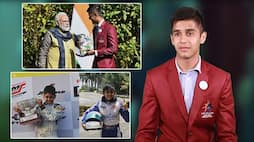
Meeting PM Modi was a dream come true, says Bal Puraskar awardee Yash Aradhya
Jan 31, 2020, 2:08 PM IST
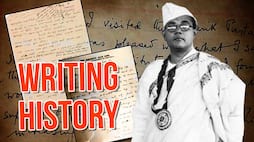
Letters of Netaji Subhas Chandra Bose reveal how he changed over time
Jan 23, 2020, 8:20 AM IST
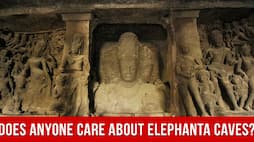
Does anyone care about Mumbai's Elephanta Caves?
Jan 7, 2020, 9:11 PM IST
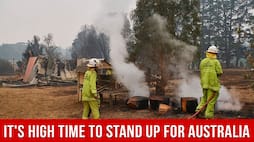
DePress Room | Australia is choking and needs immediate attention
Jan 7, 2020, 9:59 AM IST
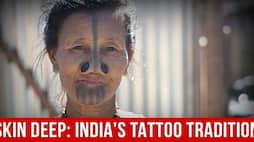
India's Tribes And Its Tradition Of Tattoos
Jan 3, 2020, 6:35 PM IST
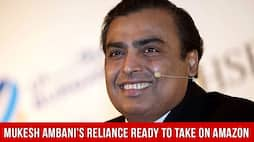
DePress Room | Reliance is ready to give amazon a run for its money
Jan 2, 2020, 9:22 PM IST
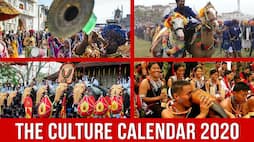
Jaipur Literature Festival, Kala Ghoda Festival & More: The 2020 Culture Calendar
Jan 2, 2020, 6:31 PM IST
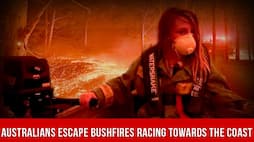
DePress Room | With each passing day fire is engulfing Australia
Jan 1, 2020, 9:37 PM IST
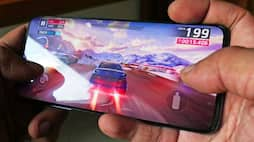
From Devil May Cry V to Resident Evil 2 Remake, here are top 5 games of 2019
Dec 30, 2019, 7:14 PM IST
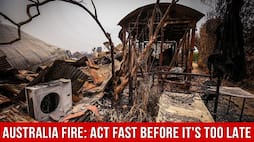
DePress Room | Australia is burning and we have to act fast
Dec 30, 2019, 6:34 PM IST
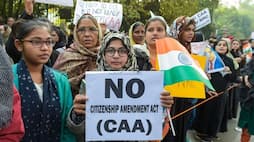
Tourists Staying Away From Taj Mahal Because Of CAA Protests
Dec 30, 2019, 4:48 PM IST
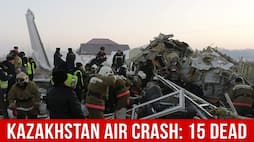
DePress Room | After A Few Minutes Of Taking-Off, Plane Crashes In Kazakhstan, 15 Dead
Dec 28, 2019, 2:16 PM IST
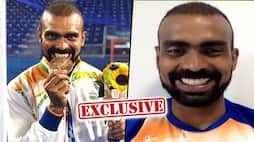
PR Sreejesh Exclusive: 'Sportsmen and their achievements are now getting much more importance'
Aug 10, 2021, 8:34 AM IST
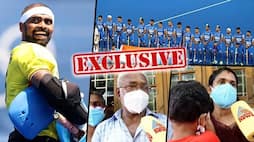
Exclusive: 'For us, this Olympic Bronze medal is like Gold'
Aug 5, 2021, 9:04 PM IST
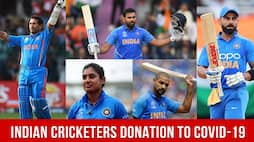
Check out Indian Cricketers Donations to help India fight COVID-19 Pandemic
Apr 1, 2020, 6:12 PM IST
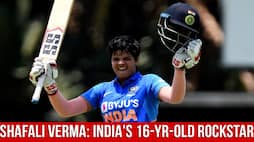
India's Shafali Verma Becomes World No.1 T20 International Batswoman
Mar 5, 2020, 6:47 PM IST
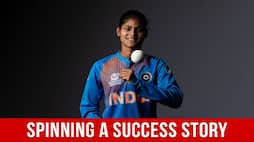
Radha Yadav: Vegetable Vendor's Daughter Is Spinning India's T20 World Cup Success Story
Mar 2, 2020, 7:14 PM IST
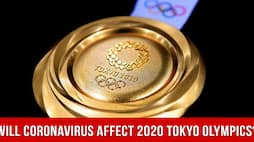
Coronavirus Outbreak: India's Sports Minister Expects 2020 Tokyo Olympics To Go Ahead As Scheduled
Feb 27, 2020, 6:55 PM IST
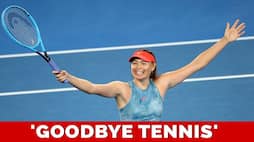
Maria Sharapova Retires: A Look Back At The 5-time Grand Slam Winner's Career
Feb 27, 2020, 6:28 PM IST
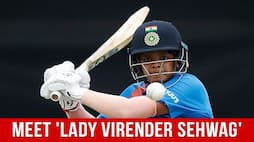
India's 16-Year-Old Shafali Verma Now Has Highest Strike-Rate In Women's T20Is
Feb 27, 2020, 5:56 PM IST
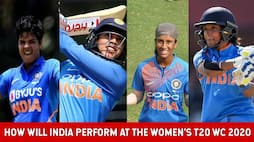
Will The Indian Team Win The ICC Women's T20 World Cup 2020?
Feb 21, 2020, 12:09 PM IST
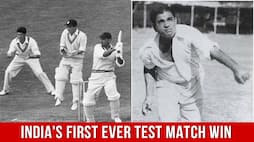
India vs England 1952 Madras Test: First Ever Test Match Win By India
Feb 20, 2020, 12:25 PM IST
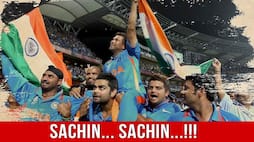
As Sachin Tendulkar Wins Laureus Sporting Moment, Relive How The 2011 WC Final Went Down!
Feb 18, 2020, 5:53 PM IST
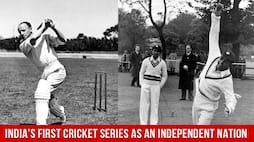
Indian Cricket Highlights: India's First Cricket Series As An Independent Nation
Feb 17, 2020, 7:28 PM IST
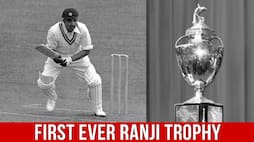
Indian Cricket Highlights: The First Ever Ranji Trophy 1934/35
Feb 11, 2020, 5:56 PM IST
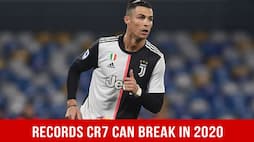
As Cristiano Ronaldo turns 35, here's a look at all possible records he can break in 2020
Feb 5, 2020, 5:02 PM IST
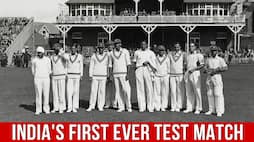
Indian Cricket Highlights: India's First Ever Test Match
Feb 4, 2020, 7:03 PM IST
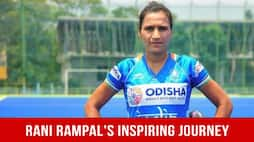
The Inspiring Life Of Indian Women's Hockey Captain Rani Rampal
Feb 2, 2020, 3:11 PM IST
- About Skkannada.com
About Director Satishkumar
- Advertise Here
- Privacy Policy and Disclaimer
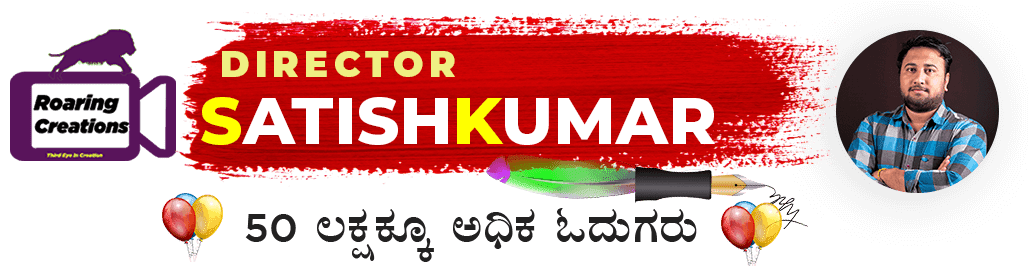
ಹೆದರದೇ ಮುಂದೆ ಸಾಗಿ - One Minute Motivation in Kannada - Motivational Speech in Kannada
.jpg)
-: ನೀವು ಓದಲೇಬೇಕಾದ 7 ಪುಸ್ತಕಗಳು - Books You Should in Kannada :-
1) ರೀಚ ಡ್ಯಾಡ ಪೂರ ಡ್ಯಾಡ ಪುಸ್ತಕ - Rich Dad Poor Dad in Kannada - By Robert Kiyosaki Book Link - Click Here
2) ದಿ ಮ್ಯಾಜಿಕ್ ಆಫ್ ಥಿಂಕಿಂಗ ಬಿಗ ಪುಸ್ತಕ – The Magic of Thinking Big Book in Kannada Book Link :- Click Here
3) ನಿಮ್ಮ ಸಬ್ ಕಾನ್ಸಿಯಸ್ ಮೈಂಡ್ ಪುಸ್ತಕ Power of Your Subconscious Mind Book in Kannada Book By Dr Joseph Murphy Link :- Click Here
4) ಯೋಚಿಸಿ ಮತ್ತು ಶ್ರೀಮಂತರಾಗಿ - Think and Grow Rich Book in Kannada Book Link :- Click Here
5) ದಿ ಸೀಕ್ರೆಟ್ ರಹಸ್ಯ ಪುಸ್ತಕ - The Secret Book in Kannada Book Link :- Click Here
6) ದಿ ಪವರ ಆಫ ಪೋಜಿಟಿವ ಥಿಂಕಿಂಗ ಪುಸ್ತಕ - The Power of Positive Thinking Book Link :- Click Here
7) ಹಣದ ಮನೋವಿಜ್ಞಾನ ಪುಸ್ತಕ :- The Psychology of Money Book in Kannada Book Link :- Click Here
ಈ ಅಂಕಣ (Article) ನಿಮಗೆ ಇಷ್ಟವಾಗಿದ್ದರೆ ನಿಮ್ಮೆಲ್ಲ ಗೆಳೆಯರೊಡನೆ ಮತ್ತು ನೀವಿರುವ ಎಲ್ಲ ಕನ್ನಡ ಫೇಸ್ಬುಕ್ ಗ್ರೂಪಗಳಲ್ಲಿ ಇದನ್ನು ತಪ್ಪದೇ ಶೇರ್ ( Share ) ಮಾಡಿ. ಜೊತೆಗೆ ಪ್ರತಿದಿನ ಹೊಸಹೊಸ ಅಂಕಣಗಳನ್ನು, ಪ್ರೇಮಕಥೆಗಳನ್ನು, ಕವನಗಳನ್ನು ಉಚಿತವಾಗಿ ಓದಲು ತಪ್ಪದೆ ನನ್ನ ಫೇಸ್ಬುಕ್ ಪೇಜನ್ನು (Director Satishkumar) ಲೈಕ್ ಮಾಡಿ.
ಪ್ರತಿದಿನ ಹೊಸಹೊಸ ಅಂಕಣಗಳನ್ನು,ಪ್ರೇಮಕಥೆಗಳನ್ನು, ಕವನಗಳನ್ನು, ಮೋಟಿವೇಶನಲ ಅಂಕಣಗಳನ್ನು ಉಚಿತವಾಗಿ ಓದಲು ತಪ್ಪದೆ www.skkannada.com ಗೆ ವಿಸಿಟ್ ಮಾಡಿ.
To Read New Stories in Kannada, Books in Kannada, Love Stories in Kannada, Kannada Kavanagalu, Kannada Quotes Visit www.skkannada.com
-: Copyright Warning and Trademark Alert :-
All Rights of all Stories, Books, Poems, Articles, Logos, Brand Images, Videos, Films published in our www.skkannada.com are fully Reserved by Director Satishkumar and Roaring Creations Private Limited®, India. All Commercial Rights of our content are registered and protected under Indian Copyright and Trademark Laws. Re-publishing our content in Google or any other social media sites is a copyright and Trademark violation crime. If such copy cats are found to us, then we legally punish them badly without showing any mercy and we also recover happened loss by such copy cats only.. .
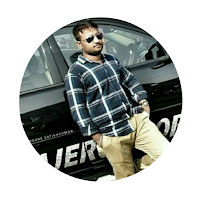
Related posts
Read By Categories
- Life Changing Articles
- Kannada Books
- Kannada love stories
- Business Lessons
- Kannada Kavanagalu - Love Poems
- Premigala Pisumatugalu
- Kannada Stories
- Spiritual Articles
- Motivational Quotes in Kannada
- Festivals & Special Days
- Kannada Life Stories
- Mythological Love Stories Kannada
- Kannada Health Articles
- Historical Love Stories Kannada
- Kannada Stories for Kids
- Comment Box
- Chanakya Niti in Kannada
- Kannada Online Courses
- Kannada Tech Articles
- Car Reviews Kannada
Today's Quote
Trademark and copyright alert, ಕಥೆ ಕವನ ಕಳ್ಳರಿಗೆ ಎಚ್ಚರಿಕೆ : strict warning to copy cats by director satishkumar.
ಈ ನಮ್ಮ ವೆಬಸೈಟನಿಂದ ಕಥೆ, ಕವನ, ಅಂಕಣಗಳನ್ನು ಕದ್ದು ಬೇರೆಡೆಗೆ ಪಬ್ಲಿಷ್ ಮಾಡಿ ಛಿಮಾರಿ ಹಾಕಿಸಿಕೊಳ್ಳುವ ಮುಂಚೆ ಇದನ್ನೊಮ್ಮೆ ಓದಿ... ...
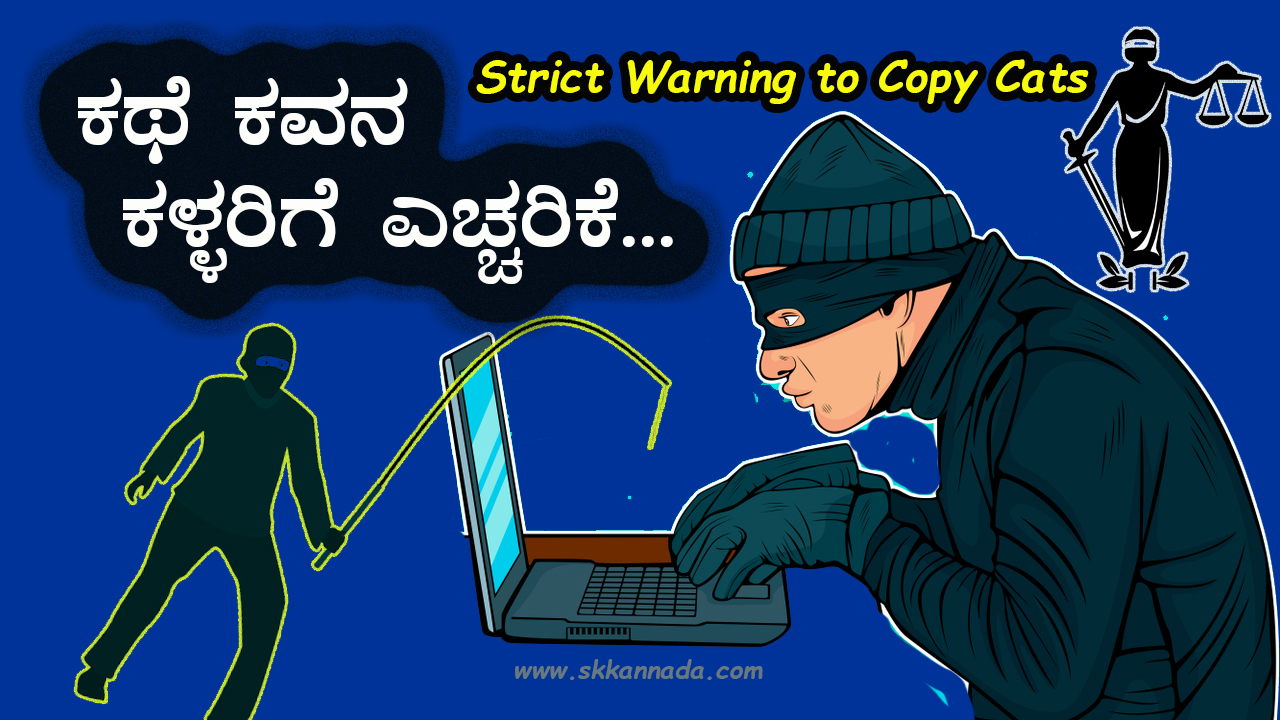
new stories
Trending stories, popular stories.

All Rights of the Content is Reserved
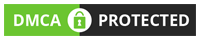
- Sustainability
- Agriculture
- Brand Campaigns
- Watch inspiring videos
- Advertise With Us
- Press Coverage
Follow Us On
Download App
How a Speech in Hindi Inspired This Karnataka Icon to Fight for Scientific Temper!
"My teachers sent me forward, and Gandhi asked me: 'Naam kya hai?' I told him. Then he asked me, 'Hindi aata hai?' and I said, 'Thada-Thoda' and he laughed."
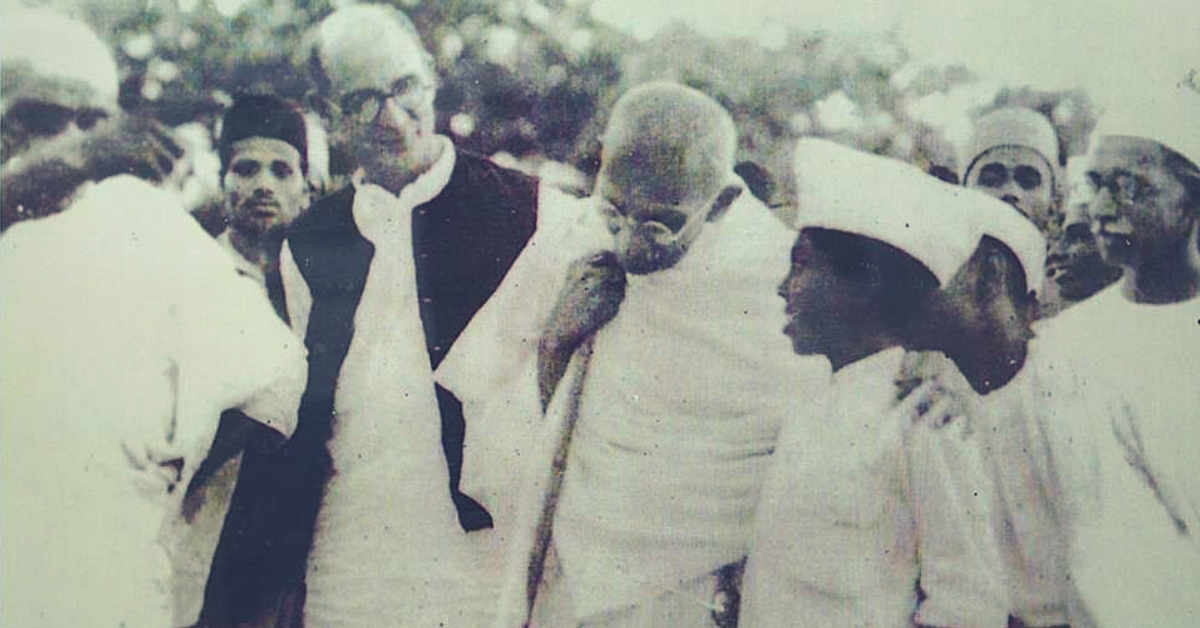
A 100-year-old high school in the heart of Bengaluru was instrumental in encouraging a young freedom fighter to fight against the tyrannical British rule.
Interestingly, his journey began with a Hindi speech which needed to be translated in Kannada!
The National High School (NHS) in Basavanagudi, Bengaluru (then Bangalore), was founded by Annie Besant in 1917—the year she became the president of the Indian National Congress. Besant wished to set up similar educational institutes across the country that would instil the values of patriotism and self-reliance among the young students and the next generation of freedom fighters.
In 1936, Mahatma Gandhi visited the school to gather support for the freedom movement. It is quite possible that his speech, originally in Hindi, did not generate much enthusiasm, so the school selected 16-year-old Hanumanthappa Narasimhaiah to translate the speech into Kannada.
This seemingly simple task would set the course of Narasimhaiah’s (fondly known as Hosur Narasimhaiah or simply, HN) life.
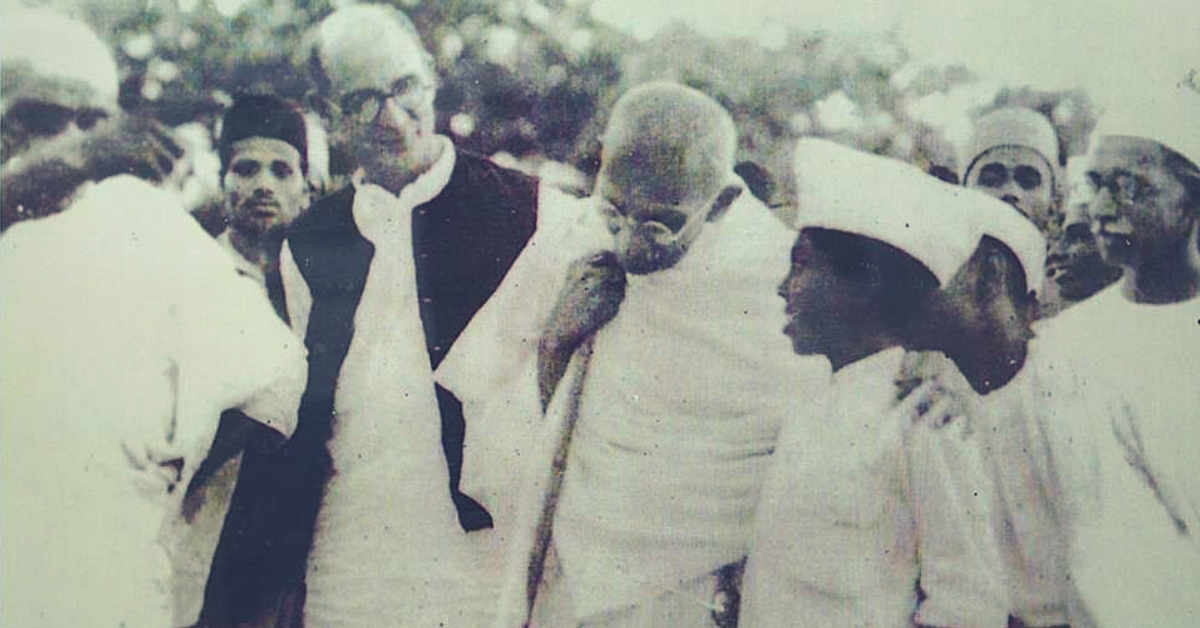
HN was a born in Hosur in Karnataka. The village had no formal education system, and so he was forced to attend a government school in the Gauribidanur town of the Kolar district. However, the school did not have the facility to provide education to children after Class 8, so he was forced to take a one-year hiatus. In the following year, the principal of the Gauribidanur school, S Narayana Raio who was transferred to the NHS in Bengaluru, invited HN to continue his studies there.
Eager to finish his formal education but with limited financial resources, HN walked 85 kms to Bengaluru on foot.
Once he reached the city, he stayed with Raio till he could get a room at the school hostel. He joined as a student in 1935, and the following year, Gandhi paid a visit. HN, a sharp and enthusiastic student, was chosen to translate his Hindi speech for the Kannada-speaking audience.
“I met Gandhi in 1936 when he came to Bangalore. We students of NHS were waiting in the shade of a tree in Kumara Krupa to meet him. He asked to speak to someone who knew Hindi and translate his speech into Kannada. My teachers sent me forward, and Gandhi asked me: ‘Naam kya hai?’ I told him. Then he asked me, ‘Hindi aata hai?’ and I said, ‘Thada-Thoda’ and he laughed. How could a 9th standard student like me translate his Hindi speech?” he wrote .
From that day onward, HN became a dedicated follower of Gandhian values.
According to Good News India , he cherished this task so much that “it was a story he was to tell all his life to his students, they, in turn, basking in reflected glory.”
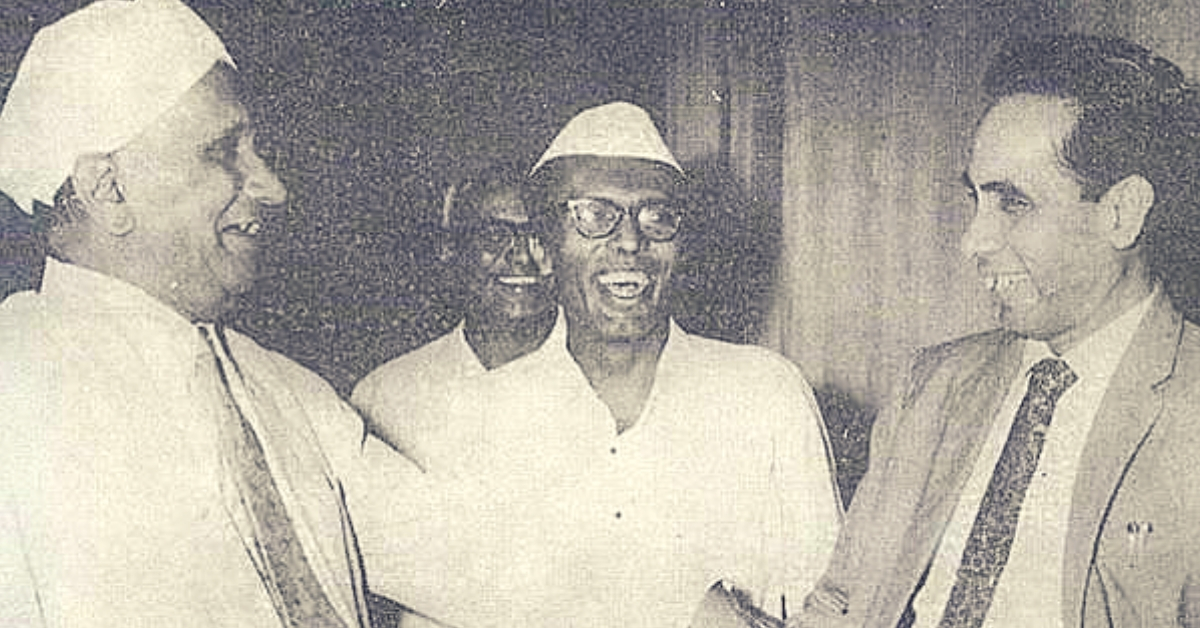
Six years later, when Gandhi launched the Quit India Movement, HN took a break from his undergraduate studies and joined Gandhi, describing this as “the most momentous decision in my life. Like hundreds of other protestors, HN too was arrested for his participation in the movement. “I spent nearly nine months in jails in Yeravada, Mysore and the Central Jail in Bangalore during the Quit India Movement. Throughout my student days, I stayed in free hostels. So when I was in Central Jail, which was just opposite my Central College hostel, I found no difference between them both gave me free boarding and lodging,” HN had once declared .
You may also like: A PM, a Pickle Tree & the Tale of India’s First State-Owned 5 Star Hotel!
HN decided to continue his studies after his release, and studied Physics for his graduation and post graduation. He maintained that he developed a scientific temper in school and that the habit of questioning led him to this path.
Following this, HN taught Physics at the National College in Bengaluru till 1957 before going to the USA for his doctoral research in “The Radioactive Decay of Hafnium and Thulium Isotopes.”
“In school, I was considered a good and an earnest student. And I liked teaching. In middle school, I used to help other students. I have dedicated my life to service, influenced by Gandhiji.
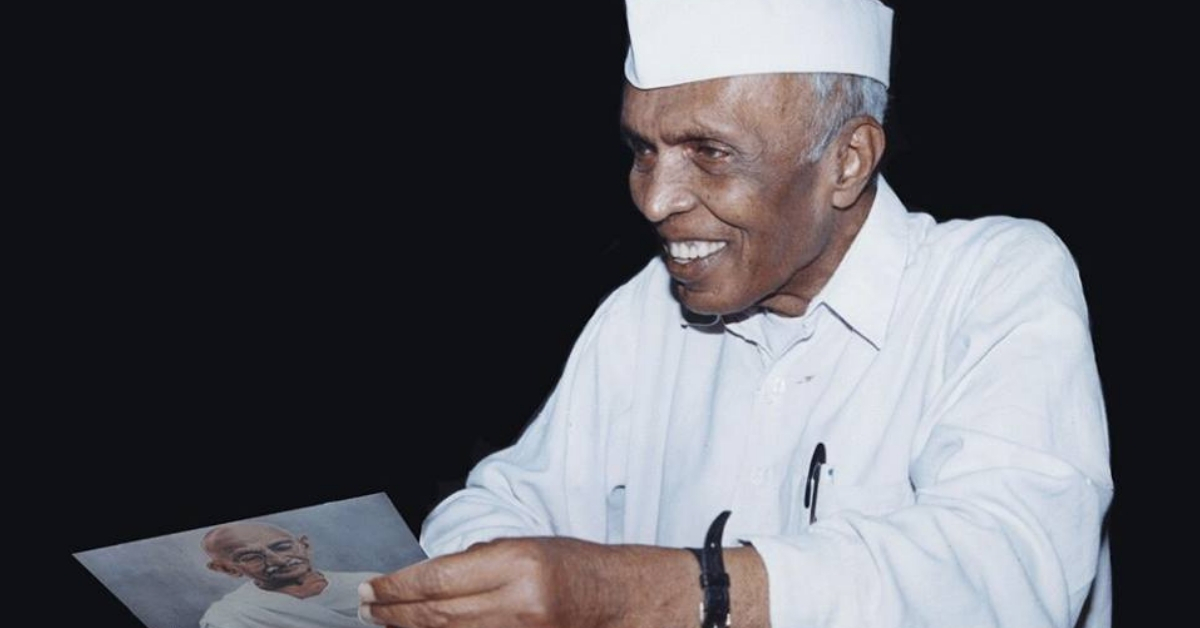
I have worked with missionary zeal to collect crores of rupees to set up numerous educational institutions all over the state. I have that same zeal even today. My willpower and determination have seen me through life. How else do you explain my survival on uppittu, rice and yoghurt for four years in the US?” HN wrote .
He obtained a PhD from the Ohio State University and continued teaching. After serving as the principal of the National College in Bengaluru, he was appointed as the Vice-Chancellor of Bangalore University in 1972. Throughout his life, HN strived to work for science and against superstitions and black magic.
You may also like: Gandhi in Bengaluru: When a ‘Sabarmati Farmer’ Persuaded Women to Donate ‘Streedhan’
In 1962, HN founded an NGO called the Bangalore Science Forum which organised weekly lectures on various scientific topics. He even established the Bangalore Lalithakala Parishat and BV Jagadeesh Science Centre.
HN remained a bachelor, staunch Gandhian, and teacher till the end of his days. He strongly voiced his criticism against self-styled Godmen like Sathya Sai Baba who claimed to perform miracles. He was also strictly against the practice of accepting donations or using political affiliations for admission in colleges.
Till date, HN remains the only Indian to be elected as a fellow of the Committee for the Scientific Investigation of Claims of the Paranormal. In 1984, he received the Padma Bhushan award for his contributions to literature and education.
From religiously following Gandhian principles to questioning unfair religious practices, HN never deviated from the path of reason. When he passed away at the age of 84 due to prolonged septicaemia, HN was still the president of the National Education Society. What could be a better record of his values than his life itself?
(Edited by Gayatri Mishra)
Like this story? Or have something to share? Write to us: [email protected] Connect with us on Facebook and Twitter .
Be a part of the better india community, check what other members are talking about -.
Good evening to all. Sir, I am looking for guidance regarding apiculture.
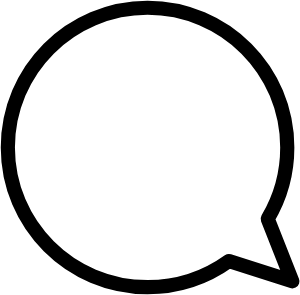
I wanna mixi Mo. No. 9518770848 Please call me
krishi samandhi jankari,,modelling in 57 yrs age,job as chief security officer,administrator in any psu or any best company ltd.l am retd as deputy commandant from border security force(BSf).As on date my age is 57 yrs.l got VRS fm service in April,2021 due to than some domestic reasons.
If you found our stories insightful, informative, or even just enjoyable, we invite you to consider making a voluntary payment to support the work we do at The Better India. Your contribution helps us continue producing quality content that educates, inspires, and drives positive change.
Choose one of the payment options below for your contribution-
By paying for the stories you value, you directly contribute to sustaining our efforts focused on making a difference in the world. Together, let's ensure that impactful stories continue to be told and shared, enriching lives and communities alike.
Thank you for your support. Here are some frequently asked questions you might find helpful to know why you are contributing?

Sounds Interesting? Share it now!

Kannada Text to speech with (human-like) voices
AI-powered Kannada text-to-speech with Dubverse is accurate, real, and fast! Type, paste, or upload a document & convert text to speech for free.
- Free to Start
- No Credit Card
- No lock-ins

Automate Kannada Text-to-speech
It's just like you would have said it, but without saying it....
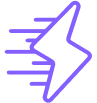
Speed Up Content Creation Journey
Save time, money, and effort with accurate voiceovers for your scripts for as many languages as you want in one go.
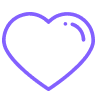
Get Human-Like, Ultra-Realistic Voices
AI-powered, engaging voices with intonation, tones, and accents that sound just like humans.
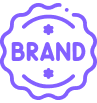
Be Consistent With Neodub Speakers
Same voices for multiple languages to build a strong, credible, and consistent brand voice throughout.
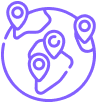
Connect With Global Audiences
Boost visibility and reach a wider audience across the globe who resonates with you with 30+ languages.
Put Kannada Text to speech into action
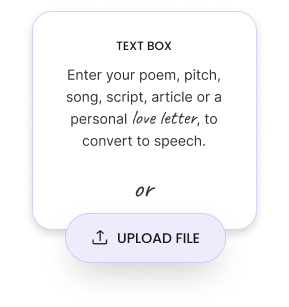
Enter your Kannada text
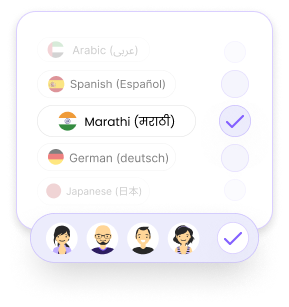
Select Language & Kannada Speaker
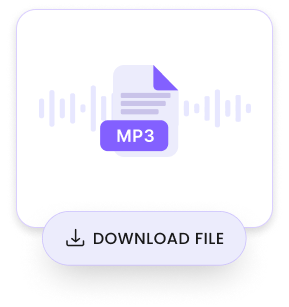
Download Kannada audio
Transform your kannada ai text-to-speech effortlessly with dubverse.
We have versatile speakers within a smooth editing platform.
Preview mode to check as many times as you want before publishing your video
Dubverse SAY is a magic tool for everything
Share your important stories with a wider audience and make your content accessible to people globally. Dubverse creates human-like, engaging voiceovers for your documentary films in multiple languages.
Whether you’re sharing information about your business or providing educational content, make your content accessible to a global audience and provide valuable information to viewers in their native language.
Dubverse is the ideal platform for dubbing your how-to videos. Help viewers learn new skills and techniques no matter where they are in the world by providing accurate dubbing in multiple languages.
Technology is a universal language, and with Dubverse, you can make sure your tech tutorials reach a global audience. Provide accurate translations and realistic voiceovers to help viewers understand complex concepts.
Stay on top of breaking news stories by dubbing your news segments. Dubverse can quickly and accurately translate and dub your content so you can provide up-to-date information to viewers across the world.
Informational
And anything else you want it to be...., minimize cost, maximize returns.
Scale up your Kannada content game
Work with teams
Invite your team to share, create and edit files together, and speed up feedback and production.
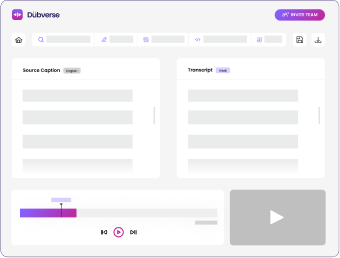
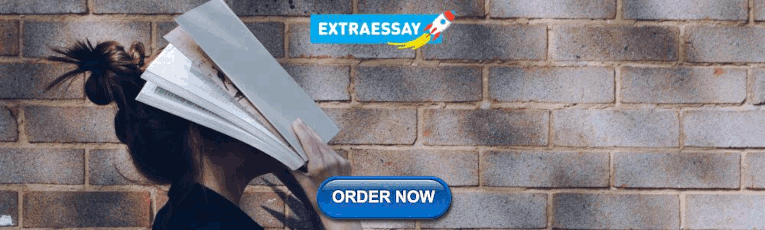
Share on Any Platform
Share your speech directly from the studio to Facebook, Twitter, Whatsapp, LinkedIn, or email.
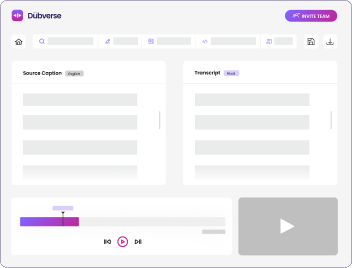
Get Expert Support
Want your speech to be 110% correct? Perfect your voiceover to the T with Dubverse. professionals.
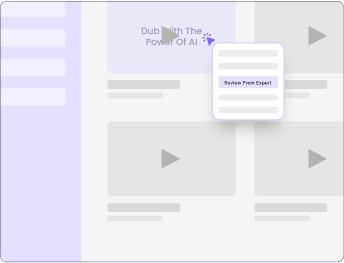
overwhelming,
super-exhausting
extremely-daunting
process of hiring voice artists,
buying recording equipment, and
a never-ending feedback loop.
MAKE DUBVERSE TEXT TO SPEECH

Kannada Text-to-speech is a technology that converts written text into spoken words. It has numerous applications and is used in various contexts, such as accessibility, language learning, and entertainment. text-to-speech technology is becoming increasingly popular as it can improve accessibility and convenience for people with visual impairments or those who prefer audio content.
Kannada Text-to-speech technology works by using advanced algorithms that analyze and understand the context of the input text. This technology enables text-to-speech software to generate natural-sounding voices that are easy to understand, even for people with hearing difficulties. text-to-speech technology has come a long way in recent years, with advancements in artificial intelligence and machine learning enabling the creation of high-quality audio output that rivals human speech.
Some of the significant advantages of Kannada text-to-speech technology are:
- The ability to convert Kannada text to audio in real-time.
Users can input any text, and the software generates the corresponding audio output almost instantly, making text-to-speech software an excellent tool for people with visual impairments or those who prefer to listen to text rather than read it.
- The accuracy and clarity.
The technology analyzes and understands the context of the input text, allowing it to generate natural-sounding voices that are easy to understand.
- SEO value.
By converting written content to audio, businesses and content creators can reach a wider audience and improve user experience. text-to-speech technology can also be used to create audiobooks, podcasts, and other audio content, enabling content creators to expand their reach and diversify their content offerings.
Overall, text-to-speech technology is becoming increasingly popular, with advancements in artificial intelligence and machine learning enabling the creation of high-quality audio output that is easy to understand and customize and can rival human speech. Businesses and content creators can benefit from the SEO value of text-to-speech technology by creating accessible and engaging content. Kannada text-to-speech technology is a must-have tool for anyone looking to expand their content offerings and reach a broader audience.
One of the popular AI apps that provide this feature is Dubverse, which enables users to convert text to audio in a seamless and efficient way.
Dubverse is a Kannada text-to-speech app that uses advanced AI technology to generate high-quality voice output. It has a user-friendly interface that allows users to input any Kannada text and convert it into an audio file. Dubverse supports 30+ Indian and global languages and has a wide range of voices and accents to choose from, enabling users to customize the listening experience.
Dubverse converts Kannada text to audio in real-time, making it an excellent tool for people who prefer to listen to text rather than read it . Users can input any text, and the app generates the corresponding audio output almost instantly. It also makes Dubverse an excellent tool for podcasters and audiobook narrators who need to customize the voice output to match their style and preferences.
Dubverse is an excellent tool for businesses and content creators who want to create engaging and accessible content. By converting written Kannada content to audio, businesses can reach a wider audience and improve user experience. Dubverse can also be used to create audiobooks, podcasts, and other audio content, enabling content creators to expand their reach and diversify their content offerings.
Kannada Text-to-speech technology has revolutionized the way we consume written content, providing an accessible and convenient way to listen to text rather than reading it. From accessibility to language learning, there are many use cases for Kannada text-to-speech technology. In this article, we will explore the top 7 use cases of converting text to audio.
- Accessibility
One of the most important use cases for text-to-speech technology is accessibility. For people with visual impairments, text-to-speech technology provides a way to access written content. By converting text to audio, people with visual impairments can listen to books, articles, and other written content with ease.
2. Language Learning
Kannada Text-to-speech technology is an excellent tool for language learners. By converting text to audio, learners can listen to written content in their target language, improving their listening and comprehension skills. text-to-speech technology can also help learners with pronunciation, as they can listen to native speakers read the text.
3. Productivity
Kannada Text-to-speech technology enables users to multitask. By listening to text rather than reading it, users can do other tasks simultaneously, such as driving or exercising. This makes text-to-speech technology useful for busy professionals or anyone looking to optimize their time and increase productivity.
4. Content Creation
By using text-to-speech technology to convert written content to audio, businesses and content creators can reach a wider audience and improve user experience. Kannada text-to-speech technology can be used to create audiobooks, podcasts, and other audio content, enabling content creators to diversify their content offerings.
5. E-Learning
Text-to-speech technology is an excellent tool for e-learning. By converting written content to audio, learners can access course material in a convenient and accessible way. text-to-speech in Kannada technology can also help learners with special needs, such as dyslexia, by providing an alternative way to access course material.
6. Entertainment
Text-to-speech technology can also be used for entertainment purposes. By converting written content to audio, users can listen to their favorite books or articles while doing other activities. text-to-speech technology can also be used to create engaging podcasts or audio dramas.
7. News and Information
Text-to-speech technology is an excellent tool for news and information. By converting written content to audio, users can listen to news articles or other information while on the go. This makes it easier for users to stay up-to-date with the latest news and information.
Text-to-speech technology has numerous use cases, from accessibility to entertainment., making it an excellent tool for language learners, productivity, content creation, e-learning, entertainment, and news and information. With advancements in artificial intelligence and machine learning, text-to-speech technology is becoming increasingly popular and providing new opportunities for businesses and content creators.
Text-to-speech online is an emerging technology that can benefit businesses in a multitude of ways. It allows businesses to convert written text into spoken words, offering a new channel to engage with customers and employees. Here are some ways businesses can make use of text-to-speech service:
- Enhance customer experience
Businesses can use text-to-speech online to enhance the customer experience. For example, they can use it to create voice-guided tutorials, provide audio instructions or menus for products, or offer audio descriptions for visually rich content such as images and videos. This can make it easier for customers to navigate a website or an app and improve their overall experience.
2. Increase engagement
By using text-to-speech online, businesses can create more engaging content. Audio content can be more emotionally evocative than written content, making it easier to connect with audiences. Businesses can use text-to-speech to create podcasts, audiobooks, or even interactive voice assistants that can provide personalized recommendations to customers.
3. Facilitate language learning
Businesses that operate in multilingual markets can use text-to-speech online to facilitate language learning for employees and customers. They can provide audio content in different languages, allowing users to improve their language skills and learn new vocabulary.
4. Enhance security
Text-to-speech online can also be used to enhance security. For example, businesses can use it to create voice recognition systems that can identify employees or customers based on their unique voiceprint. This can help prevent fraud and unauthorized access to sensitive information.
5. Provide access to information on-the-go
Businesses can use text-to-speech online to create audio versions of their news releases or product updates, enabling users to stay updated even when they cannot read.
6. Improve audio branding
Businesses can use text-to-speech online to improve their audio branding. By creating audio versions of their brand name, tagline, and other important messaging, they can establish a consistent audio identity across different channels and touchpoints. This can help reinforce brand recognition and build brand loyalty.
7. Provide audio feedback
Text-to-speech online can also be used to provide audio feedback to customers or employees. For example, businesses can use it to create personalized audio messages that congratulate customers on completing a task, remind them of upcoming appointments or events, or provide them with feedback on their performance. This can create a more personal and engaging experience for users, while also saving time and resources for businesses.
Kannada Text-to-speech online is a technology that has the potential to benefit a wide range of individuals and organizations. Here are some groups that can benefit from text-to-speech:
Students can use online text-to-speech as a tool for studying and learning. They can convert textbooks, articles, and other written materials into audio files that can be listened to while commuting or doing other activities, which will save time and help students to retain information more effectively, improving their academic performance.
2. People with reading disabilities
By converting written Kannada text into spoken words, people with reading disabilities such as dyslexia, visual impairment, or learning disabilities can turn text-to-speech online to access and process information more easily, improving their literacy skills and overall quality of life.
3. Language learners
Language learners can benefit from text-to-speech online by using it to improve their pronunciation and listening skills. They can listen to audio content in different languages and dialects, improving their comprehension and fluency.
4. Commuters
Commuters can benefit from text-to-speech online by using it to listen to news articles, podcasts, or other audio content while driving, biking, or walking, enabling them to stay informed and entertained while on-the-go, without having to take their eyes off the road or sidewalk.
5. Elderly people
Turning text-to-speech online enables elderly to access important information such as medical prescriptions, bank statements, or news articles easily. As people age, their eyesight and hearing abilities may decline, making it difficult to read small print or listen to audio content. An online text-to-speech tool can bridge this gap and provide a more convenient way to access information.
6. Professionals
Professionals such as lawyers, doctors, or executives can benefit from text-to-speech online by using it to stay up-to-date with the latest news and trends in their industry. They can listen to podcasts, webinars, or conference calls of any language while working on other tasks, improving their productivity and staying informed.
7. Non-native speakers
Non-native speakers can benefit from text-to-speech online by using it to improve their pronunciation and accent. They can listen to audio content in the language they are learning and practice speaking along with it, improving their speaking skills and confidence.
Afrikaans Text to Speech Free
Arabic text to speech free, assamese text to speech free, bangla text to speech free, bengali text to speech free, czech text to speech free, danish text to speech free, dutch text to speech free, english text to speech free, filipino text to speech free, finnish text to speech free, french text to speech free, german text to speech free, greek text to speech free, gujarati text to speech free, hebrew text to speech free, hindi text to speech free, hungarian text to speech free, icelandic text to speech free, indonesian text to speech free, irish text to speech free, italian text to speech free, japanese text to speech free, kannada text to speech free, korean text to speech free, malay text to speech free, malayalam text to speech free, mandarin chinese text to speech free, marathi text to speech free, norwegian text to speech free, oriya text to speech free, persian text to speech free, polish text to speech free, portuguese text to speech free, punjabi text to speech free, romanian text to speech free, russian text to speech free, somali text to speech free, spanish text to speech free, swedish text to speech free, tamil text to speech free, telugu text to speech free, thai text to speech free, turkish text to speech free, ukrainian text to speech free, urdu text to speech free, vietnamese text to speech free, try dubverse for all your content creation needs.
- Get started for free
- No Credit card required
- No contracts, no lock-ins
- Kannada (India) Text to Speech
Text-to-speech Kannada (India) by TTSFree. Online speech synthesis with natural sounds, and lifelike voices. Free mp3 download.
Select language and regions to speech.
- TTS Server 1
- TTS Server 2
Voice Pitch
Audjust voice speed, add background music.
We connect with FreeMusicBG - A collection of free music for commercial or free use, with an attribution license to the author. You can view more and find the Track's ID here: https://freemusicbg.com
- https://www.youtube.com/watch?v=VIDEO_ID
- https://www.youtube.com/watch?v=VIDEO_ID&feature=youtu.be
- https://youtu.be/VIDEO_ID
- https://www.youtube.com/embed/VIDEO_ID
- https://www.youtube.com/watch?v=VIDEO_ID&list=PLAYLIST_ID
- https://www.youtube-nocookie.com/embed/VIDEO_ID
- https://soundcloud.com/username/trackname
- https://soundcloud.com/keysofmoon/infinitely-ambient-music-free-download
Background music
How to convert text to speech in kannada (india) accent, input text-to-speech, select language & voice, convert & download mp3, kannada (india) text to speech voices.

Gagan, Male

Sapna, Female
Wavenet-a, female premium, wavenet-b, male premium, standard-a, female, standard-b, male, text-to-speech kannada (india) additional regional language versions.
To see more other regional Kannada (India) text-to-speech, see the pages below:

Text to speech Kannada (India) Usecases
TTSFree allows you to redistribute your created audio files for free or commercial purposes, no license required.
All intellectual rights belong to you.
Youtube videos
Podcast - broadcasting, e-learning material, sales & social media, call centers & ivr system.
Besides, You can use TTSFree to quickly make text-to-speech Kannada (India) videos and audio files for different purposes without needing a license. You can also see what people usually do with Kannada (India) accents through some of these suggestions:
- Convert Kannada Text to Speech Online
- Make realistic text to speech
- Top Kannada (India) text to speech voices 2024
- Text to speech online Kannada (India) videos
- Kannada (India) text to speech audiobooks
- Kannada (India) voice over
- Kannada (India) voice AI
- TTS Kannada (India) YouTube videos
- Kannada (India) text to speech TikTok videos
- Kannada (India) TTS social media stories
- Kannada (India) text to speech software audio messages
Frequently asked questions when using Kannada (India) Text-to-Speech
Below are some common questions and answers. If you can't find your answer, please email us at [email protected] . We will reply you soon.
What is TTS?
TTS is the abbreviation for Text to Speech, a technology text-to-speech. It has different applications, both free and paid. It can be used to create voiceover for videos, convert text documents into voices or help people with vision problems have can "read" the text.
What is the best free text to speech (software, apps ) ?
Free text to speech apps to convert any text to audio. The best free text to speech software has a lot of use cases in your computing life. The best free text-to-speech program or software can convert your text into voice/speech with just a few seconds. We suggest some listings of the best free text-to-speech that provides natural sound for your project.
- #1 TTSFree.com
- #2 Fromtexttospeech
- #3 Natural Reader
- #4 Google Text-to-Speech
- #5 Microsoft Azure Cognitive
- #6 Notevibes
We use the best AI from Google Cloud, Microsoft, Amazon Polly, Watson IBM Cloud and several other sources
TTSFree.com is a free convert text to voice service?
Yes, Free Text to Speech! Provide the highest quality free TTS service on the Internet. Covert text to speech, MP3 file. You can listen or download it. Supports English, French, German, Japanese, Spanish, Vietnamese... multiple languages. Besides the free plan, we have paid plans with advanced features, increased limits, and best voice quality.
How do Kannada (India) Text to speech programs work?
Most of the text-to-speech tools work similarly. You must type the text you want to convert to voice or copy from the text file into the input box. Then you have to select the voices available and preview the audio. We are talking Kannada (India) here, so you need to choose the language and accent of the Kannada (India). Once you find the most suitable voice, you can generate and download the mp3 file.
Kannada (India) Speech Synthesis Markup Language (SSML) support?
Full SSML support. You can send Speech Synthesis Markup Language (SSML) in your Kannada (India) Text-to-Speech request to allow for more customization in your audio response by providing details on pauses, and audio formatting for acronyms, dates, times, abbreviations, addresses, or text that should be censored. See the Speech-to-Text SSML tutorial for more information and code samples.
Convert text to speech online free unlimited?
With the basic or premium plan, we offer unlimited Kannada (India) Text-to-speech. It includes unlimited number of converted characters, number of conversions. You can create a lot of text-to-speech conversions without any limitations.
The cost of text to speech systems has dropped dramatically in recent years— much faster than most anticipated. As a result, these systems are now accessible to the general public without requiring any financial means or technical expertise. Anyone with an internet connection and an audio device can create their own text to speech system. No technical knowledge is required whatsoever; only an internet connection and web browser.
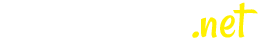
- Voice to Text
- Kannada Voice Typing
Kannada (ಕನ್ನಡ) Voice Typing
Note: Click on the Mic icon and Start Speak.
No speech was detected. You may need to adjust your microphone settings.
No microphone was found. Ensure that a microphone is installed and that microphone settings are configured correctly.
Click the "Allow" button above to enable your microphone.
Permission to use microphone was denied.
Permission to use microphone is blocked. To change, go to chrome://settings/contentExceptions#media-stream
Speech Recognition is not supported by this browser. Upgrade to Chrome version 25 or later.
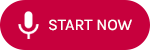
Note : This feature currently works only on Google Chrome browser. You can download and install Google Chrome. Download Google Chrome
Kannada (ಕನ್ನಡ) voice typing is an easy method of typing. This is a very good option for those who want to write Kannada without using any keyboard. All you need is a good mic, set up the mic in your computer and start speaking, the Voice to Text typing tool will recognize your voice and automatically start typing Kannada. After voice typing, you can copy it and use it on MS Word, social media, comments, Kannada articles etc. Please share it on Facebook, Twitter and WhatsApp and help us reach more users.
Instruction
- You must have a good quality mic.
- You have to speak loud and clear.
RELATED LINKS
- 👉 English to Kannada Typing
- 👉 Kannada to English Translation
Navigation Menu
Search code, repositories, users, issues, pull requests..., provide feedback.
We read every piece of feedback, and take your input very seriously.
Saved searches
Use saved searches to filter your results more quickly.
To see all available qualifiers, see our documentation .
- Notifications You must be signed in to change notification settings
Text-to-Speech for languages of India
AI4Bharat/Indic-TTS
Folders and files.
Name | Name | |||
---|---|---|---|---|
53 Commits | ||||
Repository files navigation
Ai4bharat indic-tts, towards building text-to-speech systems for the next billion users.
🎉 Accepted at ICASSP 2023
Deep learning based text-to-speech (TTS) systems have been evolving rapidly with advances in model architectures, training methodologies, and generalization across speakers and languages. However, these advances have not been thoroughly investigated for Indian language speech synthesis. Such investigation is computationally expensive given the number and diversity of Indian languages, relatively lower resource availability, and the diverse set of advances in neural TTS that remain untested. In this paper, we evaluate the choice of acoustic models, vocoders, supplementary loss functions, training schedules, and speaker and language diversity for Dravidian and Indo-Aryan languages. Based on this, we identify monolingual models with FastPitch and HiFi-GAN V1, trained jointly on male and female speakers to perform the best. With this setup, we train and evaluate TTS models for 13 languages and find our models to significantly improve upon existing models in all languages as measured by mean opinion scores. We open-source all models on the Bhashini platform .
TL;DR: We open-source SOTA Text-To-Speech models for 13 Indian languages: Assamese, Bengali, Bodo, Gujarati, Hindi, Kannada, Malayalam, Manipuri, Marathi, Odia, Rajasthani, Tamil and Telugu .
Authors: Gokul Karthik Kumar*, Praveen S V*, Pratyush Kumar, Mitesh M. Khapra, Karthik Nandakumar
[ ArXiv Preprint ] [ Audio Samples ] [ Try It Live ] [ Video ]
Unified architecture of our TTS system

Environment Setup:
Data setup:.
- Format IndicTTS dataset in LJSpeech format using preprocessing/FormatDatasets.ipynb
- Analyze IndicTTS dataset to check TTS suitability using preprocessing/AnalyzeDataset.ipynb
Training Steps:
- Set the configuration with main.py , vocoder.py , configs and run.sh . Make sure to update the CUDA_VISIBLE_DEVICES in all these files.
- Train and test by executing sh run.sh
Trained model weight and config files can be downloaded at this link.
Code Reference: https://github.com/coqui-ai/TTS `
Contributors 4
- Jupyter Notebook 63.4%
- Python 31.6%
- JavaScript 0.5%
- Dockerfile 0.4%
Hindi Text to Speech
Easily convert text to speech in Hindi, and 100 more languages. Try our Hindi text to speech free online. No registration required. Create Audio
Hindi text to voice generators make it easy to create marketing videos, promotional audio materials and language lessons for the Indian market. Use our text to speech Hindi voices to produce voice over script in Hindi much faster than recording it yourself.
Get started with our Hindi voice over online free - no registration required. Check out the instructions below.
Narakeet has 17 Hindi text to speech male and female voices. Play the video below (with sound) for a quick demo.
Making content for the Indian market? In addition to text to voice Hindi, check out our Indian accent English text to speech voices and Indian Bengali text to speech generators and Gujarati speech synthesis and Tamil text to speech online voices and Kannada text to voice generators and Marathi Voice Maker and Punjabi text to speech voice makers and Malayalam text to voice AI and Telugu text reader.
Text to Speech Hindi Voices
In addition to these voices, Narakeet has 700 text-to-speech voices in 100 languages .
For more options (uploading Word documents, voice speed/volume controls, working with Powerpoint files or Markdown scripts), check out our Tools .
How to convert Hindi text to speech?
Upload a Word document with your script, or a Powerpoint document with slides, and Narakeet will use Hindi text to voice converters online with Indian accent to make audio and video materials in minutes.
Hindi text to speech software Indian voices can help you create:
- Text to voice converter Hindi content
- Hindi voice videos
- Convert Hindi text to audio files
- Hindi narration for your existing clips,
- Text to speech in Hindi online MP3 files
- Text to speech online Hindi audio and video content
Narakeet helps you create text to speech voiceovers , turn Powerpoint presentations and Markdown scripts into engaging videos. It is under active development, so things change frequently. Keep up to date: RSS , Slack , Twitter , YouTube , Facebook , Instagram , TikTok
Kannada To Hindi Translation
The most accurate kannada to hindi translation.
- Translate Kannada To Hindi
- Hindi to Kannada Translation
- Kannada to English Translation
- Kannada to Malayalam Translation
- Kannada Alphabet
Special Characters:
Independent vowels:, dependent vowels:, consonants:, additional consonants:, additional vowels for sanskrit:, sign used in sanskrit:, additional consonants:, devanagari digit:, word or two about our translation tool.
Our Kannada to Hindi Translation Tool is powered by Google Translation API. You can start typing in the left-hand text area and then click on the "Translate" button . Our app will then translate your Kannada word, phrase, or sentence into hindi. You can also visit our homepage to type in Kannada.
The translation only takes a few seconds and allows up to 500 characters to be translated in one request. Although this translation may not be 100% accurate, you can still get a basic idea, and with a few modifications, it can be quite accurate. This translation software is evolving day by day, and Google Engineers are continuously working on it to make Kannada to hindi translation more intelligent and accurate . Hopefully, one day it will produce near-perfect translations!
If you have any suggestions, or if you come across a translated sentence that is particularly funny, please share it with us on our Facebook page. And finally, don't forget to give us a like and share our page on Facebook with your loved ones.
Features you should know:
For e.g. typing "ಭಾರತ ಬಹುಸಾಂಸ್ಕೃತಿಕ ದೇಶ" will be translated into "भारत एक बहुसांस्कृतिक देश है"
Powered by Google
High Accuracy Rate
Instant Online Translation
Up to 500 characters can be translated into one request.
This translation tool is FREE
Commonly Spoken Kannada to Hindi Phrases
- - - - - - - - - - - - - - - - - - - - |
- - - - - - - - - - - - - - - - - - |
Frequently Asked Questions (FAQ)
How does kannada to hindi text translation works.
Our translation service either use Google or Microsoft to translate the text you have typed in kannada.
Can we download this translation service?
Once this translation tool is installed, you can highlight and right-click section of text and click on "Translate" icon to translate it to the language of your choice. Furthermore, you can translate entire web page by clicking on the "Translate" icon on the browser toolbar.
It support over 100 languages.
What other tools do you have for kannada typing and Translation?
With this tool you can type in Hindi and Get in kannada. For E.g. typing "Nīvu hēgiddīri" gives you "ನೀವು ಹೇಗಿದ್ದೀರಿ" . Typing kannada is natural, and you don’t need to remember the complex kannada keyboard. Please visit: Easy Kannada typing to use this tool.
This kannada typing is absolutely free and you can email the text you have typed to anyone - including yourself.
Kannada speech translation service is provided by both Microsoft and Google . They both use their own cognitive services to translate spoken words and phrases into a language of your choice. For some languages, you will hear the translation spoken aloud.
Microsoft Translator in particular powers speech translation feature across its products which can be used for Live Presentation, In-Person or Remote Translated Communication (such as Skype), Media Subtitling, Customer support and Business Intelligence.
Is this translation FREE?
Yes. This Kannada to Eng. text translation is absolutely FREE. You can use our translation tool for both personal and commercial use.
However, we have the following restrictions:
- Per Request Limit : At any time you can translate up to maximum of 500 per request. However, there is no restriction on the number of request you can send.
- Daily Limit : While you can make a number of requests for translation, you won’t be able to translate if we run out of a daily quota.
These restrictions are placed to ensure that robots or automated software are not abusing this facilities.
Can I translate from Hindi To Kannada?
Why the translated text is not accurate.
As explained earlier, the machine-language technology is used to perform the translation. This translation software is evolving every day and as a time goes by the translation is going to be pretty accurate - especially for commonly used phrase and sentences.
At a moment, it is not perfect but our translation software is useful for those who needs help framing the sentence and get general idea on what the sentence or phrase is conveying the message.
Welcome to our website, where the past resonates through powerful speeches honoring freedom fighters. Explore imaginative fancy dress concepts, dive into the soul of national festivals, and nurture your creative spirit with engaging art and craft projects. Whether you're a DIY enthusiast or aspiring to learn Kannada, Hindi, Malayalam, or English, our offerings cater to your interests. Join us in the celebration of knowledge and creativity.
Search This Blog
Gandhi jayanti speech in hindi, republic day speech in kannada, republic day speech in kannada, 1. republic day speech in kannada 2021.
2. Republic Day Speech in Kannada 2020
ಭಾರತ ನಮ್ಮ ದೇಶ. ಭಾವೈಕ್ಯತೆಯೇ ಇದರ ಜೀವವಾಗಿದೆ. ನಮ್ಮ ದೇಶಕ್ಕೆ ಸಮೃದ್ಧ ಸಂಸ್ಕೃತಿಯ ಇತಿಹಾಸ ಮತ್ತು ವೀರ ಧೀರರ ಪರಂಪರೆಯ ಹಿನ್ನೆಲೆಯಿದೆ. ಸೌಹಾರ್ದತೆ, ಶಾಂತಿ, ಸಹಿಷ್ಣುತೆ, ಸಮಾನತೆ ಇವು ದೇಶವನ್ನು ಭದ್ರ ಪಡಿಸುವ ಮೌಲ್ಯಗಳಾಗಿವೆ. ನಾವು 07 ದಶಕಗಳಲ್ಲಿ ಬಹಳಷ್ಟು ಸಾಧಿಸಿದ್ದೇವೆ. ಸಾಧಿಸಬೇಕಾಗಿರುವುದು ಇನ್ನೂ ಸಾಕಷ್ಟಿದೆ.
ನಾವೆಲ್ಲರೂ ನಮ್ಮ - ನಮ್ಮ ಜವಾಬ್ದಾರಿಯನ್ನು ಅರಿತು ಕೆಲಸ ಮಾಡುವ ಮೂಲಕ ವೈಯಕ್ತಿಕವಾಗಿ ಮತ್ತು ಸಾಂಘಿಕವಾಗಿ ಪ್ರಯತ್ನಪಟ್ಟರೆ ದೇಶದ ವಿವಿಧ ಕ್ಷೇತ್ರಗಳಲ್ಲಿ ಪ್ರಗತಿ ಸಾಧ್ಯವಾಗುತ್ತದೆ. ಶಿಕ್ಷಣದ ಮೂಲಕ ನಾವು ಈ ಸಾಧನೆಯ ಹಾದಿಯನ್ನು ತಲುಪಲು ಶ್ರಮಪಡಬೇಕಿದೆ ಎಂಬ ಆಶಯದೊಂದಿಗೆ, ನನ್ನ ಮಾತುಗಳನ್ನು ಮುಗಿಸುತ್ತೇನೆ.
ಧನ್ಯವಾದಗಳು-ಜೈ ಹಿಂದ್ ಜೈ ಸಂವಿಧಾನ್
3. Republic Day Speech in Kannada 2019
ಭಾರತದ ಪ್ರಜೆಗಳನ್ನು ಆಳುವ -ಶಾಸಕಾಂಗ, ನ್ಯಾಯ ಒದಗಿಸುವ - ನ್ಯಾಯಾಂಗ. ಹಾಗೂ ಜನರಿಗಾಗಿ ಕೆಲಸ ಮಾಡುವ ಕಾರ್ಯಾಂಗಗಳು ಹೇಗಿರಬೇಕು? ಯಾವೆಲ್ಲ ನೀತಿ ನಿಯಮಗಳನ್ನು ಕಟ್ಟಳೆಗಳನ್ನು ಅವರು ಪಾಲಿಸಬೇಕು ಎಂಬೆಲ್ಲಾ ಸೂಚನೆಗಳನ್ನು ಹಾಕಿಕೊಟ್ಟ ಸಮಗ್ರ ಮಾಹಿತಿಗಳ ಗುಚ್ಛವೇ - ಸಂವಿಧಾನ. ಡಾ. ಬಿ ಆರ್ ಅಂಬೇಡ್ಕರ್ ನೇತೃತ್ವದಲ್ಲಿ ರಚಿಸಲ್ಪಟ್ಟ ಈ ಸಂವಿಧಾನ 1950 ಜನವರಿ 26 ರಂದು ಭಾರತದಲ್ಲಿ ಸಂವಿಧಾನ ಜಾರಿಗೆ ಬಂದ ಮೇಲೆ ಪ್ರಜೆಗಳದ್ದೇ ಸರ್ಕಾರ ಅಸ್ತಿತ್ವಕ್ಕೆ ಬಂದಿತು.
ಭಾರತೀಯರಾದ ನಾವು ಗಣರಾಜ್ಯೋತ್ಸವದ ಸಂದರ್ಭದಲ್ಲಿ " ತಮ್ಮ ತ್ಯಾಗ ಬಲಿದಾನಗಳಿಂದ ದೇಶವನ್ನು ಕಾಪಾಡುವ ಭೂ ಸೇನೆ, ವಾಯು ಸೇನೆ, ಹಾಗೂ ನೌಕಾ ಸೇನೆಯ ಎಲ್ಲಾ ಸೈನಿಕರಿಗೂ ಹಾಗೂ ದೇಶದ ಅಭಿವೃದ್ಧಿಗೆ ಶ್ರಮಿಸುತ್ತಿರುವ ಎಲ್ಲಾ ವಿಜ್ಞಾನಿಗಳು, ತಂತ್ರಜ್ಞರಿಗೆ ಮತ್ತು ದೇಶದ ಬೆನ್ನೆಲುಬಾದ ರೈತ ವರ್ಗಕ್ಕೂ ನಾವು ಈ ಸಂದರ್ಭದಲ್ಲಿ ಚಿರಋಣಿಯಾಗಿರುತ್ತಾ ದೇಶದ ಸರ್ವಾಂಗೀಣ ಅಭಿವೃದ್ಧಿಗೆ ನಮ್ಮ ಸೇವೆ ಸಲ್ಲಿಸೋಣ ಎಂದು ಶಪಥ ಮಾಡೋಣ. ಇಷ್ಟನ್ನು ಹೇಳಿ ನನ್ನ ಮಾತುಗಳನ್ನು ಮುಗಿಸುತ್ತೇನೆ. ಧನ್ಯವಾದಗಳು.
Post a Comment
Popular posts from this blog, rani lakshmi bai fancy dress and speech, bhagat singh fancy dress and speech, jawaharlal nehru fancy dress and speech.
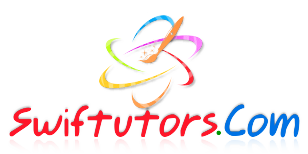
- Search Your Topic

Parts of Speech (शब्द के भेद)
Latest Release
How to write a Letter to the Principal (प्रधानाचार्य को पत्र कैसे लिखें)
Official Letter Writing in Hindi (हिन्दी मे आधिकारिक पत्र लेखन)
Identification of Grammatical Gender (व्याकरणिक लिंग की पहचान)
Hindi Story Writing (कहानी लेखन)
Common Verbs (सामान्य क्रियायें)
Hindi Voice Typing (Speech To Text)
Click on the Mic icon & Start Speaking.
Speak Now !
No speech was detected. You may need to adjust your microphone settings.
No microphone was found. Ensure that a microphone is installed and that settings are configured correctly.
Click the "Allow" button above to enable your microphone.
Permission to use microphone was denied.
Permission to use microphone is blocked.
Voice Typing is not supported by this browser. Please open this page in chrome
Online Hindi Voice Typing (Speech to Text)
This is the best and easy to use free online Hindi voice typing (Hindi Web Speech) software. In Hindi Speech to text recognition system spoken voice of the user is recognized and then translated into Hindi text. That is why it is also known as Hindi Voice Typing.
Convert Hindi Speech into Text (Voice Typing)
Here you have to use desktop Chrome browser with proper internet connection and connect your microphone with the computer. Microphone should be of good quality and placed near to your mouth.
Then just click on the microphone button available above the text box and speak slowly and clearly. You will see whatever you are speaking is automatically get recognized by the software and written in the text box.
Software converts your voice in digital codes which will be matched with the pre-set characters, words and sentences within the system in nano seconds. Hence you get the almost exactly same text output which you had spoken.
That’s why this speech to text is also called voice typing and as well as dictation typing.
This kind of speech recognition software is immensely important to anyone who needs to create a lot of written content without a lot of manual typing. It is also helpful for people with disabilities that make it difficult for them to use a keyboard.
From the technology viewpoint, speech to text recognition has a long history with several major innovations. The field has gained from AI in Big Data & Deep Learning.
You can perform copy, print, delete, download as MS word file or send it to another person via Gmail according to your requirement.
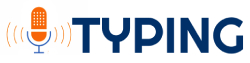
- Kannada Voice Typing
If you want to do typing by speaking (Kannada Voice Typing), you can do typing easily with the help of the software of our voice typing website. This website will help you with voice typing in the Kannada language so that your typing will become very fast. To speak and type in the Kannada language, you just have to turn on your microphone. Our voice typing software will listen to your voice and write in the Kannada language so that your typing work will be finished quickly.
To type with Kannada Voice Typing, you just have to click on the Start Voice Typing button. After which whatever you say in the mic our Voice typing software will start writing in text. When you are finished typing, click on the Stop Voice Typing button after which the typing will stop.
Kannada Voice Typing Facility
The Kannada Voice Typing facility is blown…
- Below the typing box, you will see the total number of words and characters, so you will be able to know how many words you have typed in total and how many characters are there in total.
- On this page of Kannada voice typing, you have been given some more facilities so that your work becomes easier. Below you will first find the download button in Notepad. By clicking on this button, whatever you have typed by speaking will be downloaded in Notepad.
- In the same way, you will also get the button Download as a Word file which allows you to download the paragraph written by you in Microsoft Word. If you want to print, then you can print the words typed by you by clicking on the print button.
This website saves what you say in your browser. Due to this if your internet gets disconnected or the browser is closed by mistake, you will get all your typed paragraphs. When your typing work is done, you can reset it so that the paragraph typed in the box will be deleted.
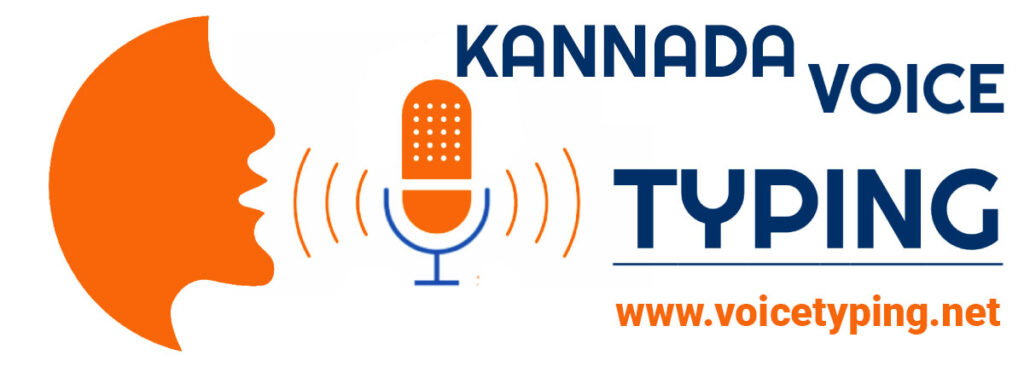
The Kannada language is one of the major spoken languages of India. Kannada comes seventh among the languages spoken in India. This Kannada language is mainly spoken in Karnataka (India). Apart from India, Telugu is also spoken in Maharashtra, Andhra Pradesh/Telangana, Kerala and Goa.
Important points to keep in mind for Kannada Voice Typing Speech To Text software to work Properly:
If you want what you speak should be written correctly and well, then you must keep some things in mind which are as follows.
- Use a good-quality mic.
- Whenever you start speaking into the mic, speak clearly and loudly so that the software can understand and write correctly.
- Do not speak everything too quickly because if you speak too quickly then the software will have a problem to recognizing your voice.
- Pronounce the words clearly and write clearly.
What is Voice Speech To Text Technology?
Voice Speech-to-text (STT) technology is known as automatic speech recognition (ASR). It is a technique that converts spoken language into written text. This type of technology provides the ability to convert phrases or speech into text, allowing it to be understood directly by computers or other devices. The Kannada Voice Typing Speech Text technology provided on our website voicetyping.net will convert your spoken words into digital text.
How does Kannada Voice Typing Speech to Text work?
Kannada Voice Typing Speech to Text technology also known as Speech-to-Text (STT) technology, works by converting spoken language into written text. The process involves several steps:
- Audio Input: The system receives audio input in the form of spoken words from microphones, voice recorders, or other audio recording devices.
- Preprocessing: The incoming audio signal may undergo preprocessing to enhance the quality of the signal. This Preprocessing does noise reduction, filtering, and other techniques to improve the accuracy of the speech recognition process.
- Feature Extraction: The system analyzes the audio signal and extracts relevant features that represent characteristics of the speech, such as frequency, amplitude, and duration of sounds.
- Speech Recognition: The extracted features are then used in a speech recognition algorithm or model. This Speech Recognition model is trained on large datasets of spoken language to recognize patterns and convert them into text. Machine learning techniques, including deep learning models like recurrent neural networks (RNNs) or transformers, are often employed for this task.
- Language Modeling: The system incorporates language models to understand the context and improve accuracy. Language models consider the probability of word sequences occurring together in a given language, helping the system choose the most likely words based on context.
- Text Output: The recognized speech is then transcribed into written text and presented as the final output. This text can be displayed on a screen, saved as a document, or used in various applications as needed.
Kannada Voice Typing FAQs
How does voice typing work.
Just click on the “Start Voice Typing: button and start speaking.
Is Kannada Voice Typing Available for Mobile Devices?
Yes, Our Website gives you the facility for Kannada Voice Typing in Mobile.
What is Kannada Voice Typing?
Kannada Voice typing is also known as speech-to-text or Kannada voice recognition. This technology converts Kannada spoken words into Kannada written text.
Voice typing facility is also available in Bangla , Gujarati , Hindi , Marathi , Tamil , Telugu , Urdu , and English on our website.
More language Voice Typing Tool
- Arabic Speech to Text (Bahrain)
- Arabic Voice Typing (U.A.E.)
- Bangla Voice Typing
- Bhojpuri Voice Typing
- Chinese Speech to Text
- French Speech to text
- French Voice Typing
- Gujarati Voice Typing
- Hindi Voice Typing
- Italian Voice Typing
- Japanese Speech to text
- Korean Voice Typing
- Malayalam voice typing
- Marathi Voice Typing
- Portuguese Speech to text
- Punjabi Voice Typing
- Russian Speech to text
- Spanish Speech To Text
- Tamil Voice Typing
- Telugu Voice Typing
- Turkish Speech to text
- Urdu Voice Typing
- Voice Typing English
- Xhosa Voice Typing
- Yue Chinese Speech to text
- Zulu Voice Typing
Latest Post
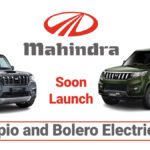
Mahindra अब ला रही है Scorpio और Bolero Electric Car। खरीदने के लिए हो जाओ तैयार
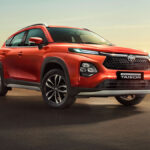
Toyota Taisor Review: Affordable Urban Cruiser with Premium Features 2024
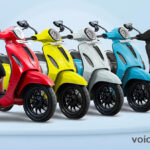
Bajaj Chetak 2901 लॉन्च – नया और सस्ता वेरिएंट में
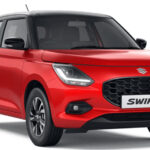
नई पीढ़ी की Maruti Suzuki Swift 2024 आधुनिकता और मस्ती का संगम है
Sign in to your account
Username or Email Address
Remember Me
Indian Republic Day 2018
[kannada] republic day 2019 speech in kannada for students - 26 january kannada speech lines pdf, republic day speech in kannada for students .

26 January Kannada Speech For Kids

No comments
Republic day essay, essay on republic day in hindi, 10 lines on republic day, paragraph on republic day, poem on republic day in hindi, republic day wikipedia, republic day songs for dance, most popular.
![speech on kannada in hindi [#Telugu] Republic Day Speech (రిపబ్లిక్ డే ప్రసంగం) In Telugu For Teachers - 26 January 2019 Telugu Speech PDF](https://blogger.googleusercontent.com/img/b/R29vZ2xl/AVvXsEhzdXyJX4PSYAkkBinkYyCQJCZPBd5RXaXit-SsSdRbRPJiBrm-liQfbA-zsj8rWkovTcsqZcYFEDwcesYY36s90Z2tW6XjmH11lR5fGSjeoHEebYlJ68uGtcLWClYpZo6mpzB59f0FfEhk/s72-c/Republic+Day+HD+Images.jpg)
Blog Archive
- January 2019 (1)
- December 2017 (8)
- November 2017 (14)
- 26 January 2019
- 26 January 2019 Chief Guest Name List
- 26 January 2019 Live Parade
- 26 January 2019 Modi speech
- 26 January 2019 Parade
- 26 January 2019 Parade Online Ticket Booking
- 26 January 2019 Parade Timing
- 26 January 2019 Speech
- 26 January Advance Images
- 26 January Advance Sms
- 26 January Advance Wishes
- 26 January Anchoring Script
- 26 January Anchoring Speech
- 26 January DP
- 26 January Drawing Competition Images
- 26 January Essay
- 26 January Essay In Hindi
- 26 January Images
- 26 January Kavita
- 26 January Mp3 Songs
- 26 January Patriotic Poem
- 26 January Photos
- 26 January Poem
- 26 January Quotes
- 26 January Shayari
- 26 January Sms In Hindi
- 26 January Songs
- 26 January Songs List
- 26 January Speech
- 26 January Speech In Hindi
- 26 January Speech In Kannada
- 26 January Speech In Telugu
- 26 January Status
- 26 January Telugu Speech
- 26 January Tiranga Pictures
- 26 January Wallpaper
- 69th Republic Day Whatsapp DP
- Chief Guest Of Republic Day 2019
- Constitution
- Constitution of India
- indian constitution
- Introduction to Constitution of India
- PM Speech on Republic Day 2019
- Republic Day 2019 Chief Guest
- Republic Day 2019 Essay
- Republic Day 2019 Images
- republic day 2019 modi speech
- Republic Day 2019 Online Ticket Booking
- Republic Day 2019 Parade
- Republic Day 2019 Parade Live
- Republic Day 2019 Speech
- Republic Day 2019 Tickets
- Republic Day 3D Wallpaper
- Republic Day Advance Images
- Republic Day Advance Shayari
- Republic Day Advance Sms
- Republic Day Anchoring Script
- Republic Day Anchoring Script In Hindi
- Republic Day Chief Guest
- Republic Day Drawing Images
- Republic Day Drawing Pictures
- Republic Day Essay
- Republic Day Essay For Students
- Republic Day Essay In English
- Republic Day Essay In Hindi
- Republic Day Essay In Telugu
- Republic Day Essay PDF
- Republic Day FB Status
- Republic Day Images
- Republic Day Indian Flag Images
- Republic Day Kavita
- Republic Day Patriotic Poem
- Republic Day Patriotic Songs
- Republic Day Pictures
- Republic Day Poems
- Republic Day Profile Pics
- Republic Day Quotes
- Republic Day Shayari In Hindi
- Republic Day Short Speech
- Republic Day Songs
- Republic Day Songs List
- Republic Day Speech
- Republic Day Speech For Teachers
- Republic Day Speech In English PDF
- Republic Day Speech In Hindi
- Republic Day Speech In Hindi PDF
- Republic Day Speech In Kannada
- Republic Day Speech In Punjabi
- Republic Day Speech In Tamil
- Republic Day Speech In Telugu
- Republic Day Thoughts
- ► January (1)
- ► December (8)
- 26 January 2019 Profile Pics For FB, Whatsapp DP -...
- [Kannada] Republic Day 2019 Speech In Kannada For ...
- [#Drawing] Indian 69th Republic Day 2019 Drawing P...
- [#Quotes] 26 January (69th) Republic Day 2019 Famo...
- [#Speech] 26 January Republic Day Speech (Essay) I...
- 26 January Republic Day 2019 Advance Images, Sms, ...
- Chief Guest Of Republic Day 2019 - 26 January 2018...
- India Republic Day 2019 Parade Live - 26 January 2...
- India Republic Day 2019 Patriotic Songs List For D...
- Happy Republic Day 2019 Patriotic Poems In English...
- Republic Day 2019 Images HD Free Download - 26 Jan...
- 26 January 2019 Anchoring Script (Speech) In Hindi...
- Republic Day 2019 Essay (Lines) For Children - 26 ...
- Republic Day 2019 Speech In Hindi For Teachers - 2...
Naming in a multilingual context: Norms for the ICMR-Manipal colour picture corpus in Kannada from the Indian context
- Original Manuscript
- Open access
- Published: 24 June 2024
Cite this article
You have full access to this open access article
- Rajath Shenoy ORCID: orcid.org/0000-0001-6288-4196 1 ,
- Lyndsey Nickels ORCID: orcid.org/0000-0002-0311-3524 2 &
- Gopee Krishnan ORCID: orcid.org/0000-0001-9509-5900 1
There have been many published picture corpora. However, more than half of the world’s population speaks more than one language and, as language and culture are intertwined, some of the items from a picture corpus designed for a given language in a particular culture may not fit another culture (with the same or different language). There is also an awareness that language research can gain from the study of bi-/multilingual individuals who are immersed in multilingual contexts that foster inter-language interactions. Consequently, we developed a relatively large corpus of pictures (663 nouns, 96 verbs) and collected normative data from multilingual speakers of Kannada (a southern Indian language) on two picture-related measures (name agreement, image agreement) and three word-related measures (familiarity, subjective frequency, age of acquisition), and report objective visual complexity and syllable count of the words. Naming labels were classified into words from the target language (i.e., Kannada), cognates (borrowed from/shared with another language), translation equivalents, and elaborations. The picture corpus had > 85% mean concept agreement with multiple acceptable names (1–7 naming labels) for each concept. The mean percentage name agreement for the modal name was > 70%, with H -statistics of 0.89 for nouns and 0.52 for verbs. We also analyse the variability of responses highlighting the influence of bi-/multilingualism on (picture) naming. The picture corpus is freely accessible to researchers and clinicians. It may be used for future standardization with other languages of similar cultural contexts, and relevant items can be used in languages from different cultures, following suitable standardization.
Similar content being viewed by others
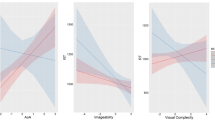
Picture naming in bilingual and monolingual Chinese speakers: Capturing similarity and variability
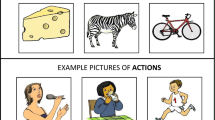
Polish norms for a set of colored drawings of 168 objects and 146 actions with predictors of naming performance
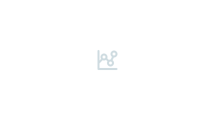
Thai Norms for Name, Image, and Category Agreement, Object Familiarity, Visual Complexity, Manipulability, and Age of Acquisition for 480 Color Photographic Objects
Avoid common mistakes on your manuscript.
Introduction
Pictures are a valuable tool in cognitive science and kindred disciplines. They are employed both in experimental research to understand the nuances of various cognitive processes (e.g., language, attention, memory; for review, see Souza et al., 2020 ) and in various clinical populations (for instance, in dementia: e.g., Cuetos et al., 2005 , 2012 ; Moayedfar et al., 2021 ; Silagi et al., 2015 ; and in aphasia: e.g., Alyahya et al., 2020 ; Bemani et al., 2022 ; Bose & Schafer, 2017 ; Brysbaert & Ellis, 2016 ; Cuetos et al., 2002 ; Nickels, 1995 ; Nickels & Howard, 1995 ). For all such purposes, pictures need to be carefully developed and evaluated in terms of various (psycholinguistic) attributes that are known to influence their processing across tasks.
In the past, several validated corpora of pictures have been made available in many languages and for specific purposes/populations (e.g., Bonin et al., 2003 ; Brodeur et al., 2010 , 2014 ; Dunabeitia et al., 2018 ; Hebart et al., 2019 ; Moreno-Martínez & Montoro, 2012 ; Szekely et al., 2004 ; Szymanska et al., 2019 ). A closer inspection of these corpora shows that they are of two types: specific-purpose and general-purpose. Specific-purpose corpora are developed and validated for specific populations or purposes. For instance, the Besancon Affective Picture Set for Adults (BAPS-Adult) is a corpus of 256 attachment-related pictures (Szymanska et al., 2019 ), and the Image Stimuli for Emotional Elicitation (ISEE: Kim et al., 2018 ) is a set of 158 images that should elicit emotionality. Similarly, the Australian Beverage Picture set (Onie et al., 2020 ) was specifically developed to investigate cognitive bias in the Australian alcoholic population and therefore contains pictures of beverages (9 alcoholic and 10 non-alcoholic) across seven contexts.
General-purpose corpora, on the other hand, usually contain a large set of pictures that have been rated on various psycholinguistic properties such as imageability, familiarity, and visual complexity, and evaluated for name agreement with a group of speakers of the language under inquiry. The pictures in such corpora often include object pictures to elicit nouns that belong to common categories such as vehicles, vegetables, animals, and clothing (e.g., Bonin et al., 2020 ; Brodeur et al., 2010 , 2014 ; Dunabeitia et al., 2018 ; Hebart et al., 2019 ; Snodgrass & Vanderwart, 1980 ; Szekely et al., 2004 ), and, less commonly, action pictures to elicit verbs (e.g., Ahmed et al., 2022 ; Szekely et al., 2004 ). The standardized set of 260 object pictures developed and validated by Snodgrass and Vanderwart ( 1980 ) is one of the most widely used general-purpose corpora for English. The original black and white line drawings have now been supplemented by coloured versions of these pictures (e.g., Rossion & Pourtois, 2004 ) and many more recent corpora have used coloured photographs (Brodeur et al., 2010 , 2014 ; Hebart et al., 2019 ). Colour in particular has been found to improve picture naming accuracy, particularly for items with diagnostic colours and similar shapes, such as fruit and vegetables (Rossion & Pourtois, 2004 ; Bramão et al., 2011 ).
Pictures are visual instantiations of objects, actions, or entities in the world and are thus non-linguistic. Nevertheless, there are a few caveats in using a universal set of pictures around the world and across languages. First, objects depicted in a corpus may not be equally familiar to speakers of different languages, or to speakers of the same language across geographical areas. For instance, concept familiarity ratings for “baseball bat” showed that this item is more familiar among English speakers in the United States of America (Snodgrass & Vanderwart, 1980 ) than Spanish speakers in Spain (Sanfeliu & Fernandez, 1996 ). Second, the name agreement of objects can vary considerably across languages. For instance, for the same set of pictures, Spanish speakers elicited more different unique names per object (thus lower name agreement and higher H -statistic: Sanfeliu & Fernandez, 1996 ) than English speakers (higher name agreement and lower H -statistic: Snodgrass & Vanderwart, 1980 ). It is thus obvious that both item-level (e.g., item familiarity) and language-level (e.g., name agreement) factors can influence the attributes of a corpus. Such between–language differences have fuelled several adaptations of popular picture corpora. For example, adapted (or extended) versions of the Snodgrass and Vanderwart corpus have been published in Spanish (Sanfeliu & Fernandez, 1996 ), French (Alario & Ferrand, 1999 ), Icelandic (Pind et al., 2000 ), Portuguese (Pompéia et al., 2001 ), Japanese (Nishimoto et al., 2005 ), Malayalam (George & Mathuranath, 2007 ), Turkish (Raman et al., 2014 ), Argentinian Spanish (Manoiloff et al., 2010 ), Tunisian-Arabic (Boukadi et al., 2016 ), and Kannada (Bangalore et al., 2022 ). Similarly, Duñabeitia and colleagues (Duñabeitia et al., 2022 ) published a corpus of 500 colour pictures with data for name agreement and familiarity in 32 languages. While such corpora are of enormous importance for helping to understand the differences in language usage across the speakers of various languages, they nevertheless do not take into account the fact that many languages can reside in one speaker. That is, the speakers’ knowledge of more than one language and the interaction among those languages (while engaged in a psycholinguistic task) has not received sufficient research attention. Currently, an abundance of evidence from the bilingualism literature posits that all the languages of a speaker are active while engaged in a linguistic task (e.g., Brysbaert, 1998 ; Moon & Jiang, 2012 ; Van Hell & Dijkstra, 2002 ). In this context, we believe that gathering data in a truly multilingual context is important to inform us not only about the between–language differences among the speakers of various languages but also within speakers of more than one language (i.e., bi-/multilinguals). The current study is an attempt to explore such influence from an arguably ideal multilingual context—India.
India is home to 121 major languages (Census of India, 2011 ). In most geographical states of India, several languages are commonly spoken in addition to the primary language of that state. For instance, Kannada is the primary language spoken in the southern Indian state of Karnataka. However, other languages that are spoken in various parts of Karnataka include Tulu and Konkani (in the south-western sectors), Marathi (in the north-western parts), and Telugu and Tamil along the borders of Telangana and Tamil Nadu states, respectively (Census of India, 2011 ). Moreover, many individuals also speak Hindi and/or English (both official languages, and from the latter other languages have many lexical borrowings). Such linguistic diversity is expected to influence the ratings of psycholinguistic attributes and, more importantly, provide an opportunity to understand the influence of multilingual contexts on norms, something that is seldom considered in earlier studies. Hence, in this study, we developed a corpus of pictures and collected normative data with (multilingual) Kannada speakers in Karnataka.
There are already some published picture corpora in Kannada: United Nations Children's Fund (UNICEF) picture cards (simple line drawings: 361 semantic items, 303 syntactic structures; Karanth, 2007 ); a subset of the coloured version of the Snodgrass and Vanderwart ( 1980 ) picture set ( n = 180, Bangalore et al., 2022 ); and coloured verb pictures ( n = 269 based on their argument structure, Ahmed et al., 2022 ). However, only one of these sets (the UNICEF pictures, Karanth, 2007 ) are original and culturally curated coloured pictures suitable for Kannada speakers (and speakers of other Indian languages), and this set does not have name agreement or rated psycholinguistic attributes.
Duñabeitia et al. ( 2022 ) argue that, in general, to avoid restricting experimental design picture corpora need to be considerably larger than 300 items. Further, several researchers (e.g., Bangalore et al., 2022 ; Duñabeitia et al., 2022 ) have acknowledged the limitations of using black and white images, as they can affect the ease of recognition of the pictures (and thus the concepts). Indeed, only one of the available sets in Kannada (i.e., Bangalore et al., 2022 , using Rossion & Pourtois, 2004 colour pictures) comprises coloured pictures, and it contains only 180 culturally appropriate pictures. Consequently, we aimed to develop an extensive, open-source picture database suitable for cognitive, linguistic, and clinical purposes with ratings on major psycholinguistic attributes in Kannada. We also aimed to explore the nature of naming labels when the normative data are collected from a multilingual population. To capture language-level variability, we use two name agreement measures: percentage name agreement and H -statistics. While percentage name agreement provides an index of agreement on a single name (modal name), the H -statistic captures variability in responses which arises from the interparticipant variability (Snodgrass and Vanderwart, 1980 ). For instance, two pictures could both have the most common name produced 70% of the time; however, one picture only has one alternative name produced, while another has five alternative names. The first picture has less variability in its naming responses, and this is reflected in a low H -statistic ( H = 0.88). The second picture has more variability in naming responses (despite an identical number of participants [70%] producing its modal name), and a higher H -statistic ( H = 1.47).
The study was approved by the Institutional Ethics Committee, Kasturba Medical College, Manipal Academy of Higher Education, Manipal (IEC: 178/2020). All participants recruited to this study read the participant information sheet and provided written informed consent before participating.
Stimulus selection
As the motivation behind the development of this corpus was for a large bank of stimuli to be appropriate for use with adults with aphasia, the selection of concepts was considered with care, to ensure the clinical and functional relevance of the stimuli (e.g., Renvall et al., 2013a , 2013b ). Consequently, we based the list of items on Palmer et al. ( 2017 ) who examined the word lists chosen for therapy by a large number ( N = 100) of English-speaking people with aphasia from the UK and provided lists of the most common topics and words. However, to make the corpus suitable for usage in the Indian multilingual and multicultural context, while some items that were relevant across cultures ( n = 605 nouns) were selected from the Palmer et al. list (e.g., pen, chair), others were more relevant to a UK population (such as specific buildings like Blenheim Palace). These items were replaced with culturally specific items ( n = 64; e.g., monuments: Indian Parliament, Taj Mahal, Mysore Palace; food items: idly , upma ). Of the 669 object pictures, 508 depicted human-made objects and 161 represented natural objects Footnote 1 . In addition to the 669 nouns, 99 picturable verb names Footnote 2 were selected from an existing Kannada word repository (Prarthana, 2015 ). We chose to develop coloured images rather than black and white, because of the facilitating effect of colour on naming accuracy (Rossion & Pourtois, 2004 ).
A hired digital artist developed the pictures corresponding to the nouns and verbs using a professional software program (Adobe Photoshop CS3). We instructed the artist to draw images in a way that was appropriate for both young and older adults as well as a multicultural audience. All pictures were drawn on a white background. However, a few (e.g., coast, star, lightning, moon) needed background colours to enrich the target image and to reduce ambiguity. All pictures had a uniform dimension (height × width: 2480 × 3508 pixels) and were saved in .jpg format (see sample pictures in Appendix Figs. 3 and 4 ). Before they were used for subsequent ratings, the authors inspected them for quality and clarity and requested a revised version from the artist for those pictures that were deemed inappropriate.
Participants
We recruited 120 native adult speakers of Kannada from the Udupi district of Karnataka through a word-of-mouth (snowballing) approach. Participants self-reported that they were fluent speakers of Kannada. Additionally, all participants were multilingual, reporting that they were fluent in a minimum of two languages (mean = 3.6: SD = 0.81, median = 4: IQR = 1, range = 2–5) (see Supplementary Material S1 for the language status of the participants). Participants had a minimum of 7 years of education and were able to read and write Kannada Footnote 3 . None had any current (or history of) cognitive, language, or neurological disorders. The participants were divided into four groups (30 per group, following Snodgrass & Vanderwart, 1980 ) to carry out the following tasks: (1) name agreement for noun pictures (mean age = 22.4: SD = 2.7 years); (2) name agreement for verb pictures, (mean age = 20.5: SD = 0.6 years; (3) image agreement ratings for both nouns and verbs (mean age = 20.3: SD = 0.7 years); and (4) ratings of familiarity, (subjective) frequency, and age of acquisition (mean age = 39.3; SD = 13.3 years).
This study was carried out in a hybrid mode (i.e., online and offline, due to COVID-related restraints). The name and image agreement ratings were conducted online. The rating of psycholinguistic variables was conducted in offline mode (except for five participants who did the rating online). For all the online participants, an orientation session was carried out over video conference to introduce the purpose of the study, and to highlight the importance of adhering to the instructions. The pictures were presented by screen-sharing a Microsoft PowerPoint presentation to all online participants simultaneously. Adequate time was provided to respond to each item in each task (e.g., ~1 minute to respond in the name agreement task, ~30 seconds to respond in the image agreement task). Participants were asked to write their responses on paper and later send a scanned copy to the first author. The entire online rating process lasted for five 2-hour sessions for each task (i.e., name and image agreement). Those participants who missed any online session received additional session(s) to complete the rating of missed items. All participants were asked to note their participant identifier, age, gender, languages known and spoken, and educational background on the response sheet.
Name agreement
As mentioned above, the name agreement task was carried out online. Each picture was displayed individually on a Microsoft PowerPoint slide. Participants were instructed to look at the picture and write down the first name (in Kannada) that came to mind on a sheet of paper (along with the slide number). They were allowed to respond with single, compound, or multiple words. Participants who named noun pictures of objects were required to use a noun, and those who named action pictures were instructed to use a verb in their response. Further, the participants were instructed to write “DKO” (i.e., Do not Know the Object/Verb) if they did not know the object/action, “DKN” (i.e., Don’t Know the Name) if they knew the object/action but not the name, and “TOT” (i.e., Tip-of-the Tongue) if they believed they knew the object/action and its name but were unable to retrieve the name momentarily. Considering the transparency of the Kannada script (highly consistent syllable–grapheme correspondence; Karanth, 2003 ), the participants were requested to write the picture names in the Kannada script (even for borrowed words from other languages). After completing the name agreement task, the participants were requested to scan and send their responses to the first investigator via e-mail or shareable drive.
Image agreement
The modal names (i.e., the most commonly produced correct names) of the pictures from the name agreement data were used for image agreement ratings. The investigator displayed the modal name (using Microsoft PowerPoint) while reading it aloud. Participants were instructed to listen to the spoken name (by the investigator) and read the displayed name, and create a mental image of the concept. After a gap of 5 seconds, the stimulus picture was displayed. Participants were required to rate the agreement between their mental image and the displayed image on a five-point Likert scale (1 – no match, 2 – poor match, 3 – average match, 4 – high match, and 5 – perfect match).
Ratings for other pertinent psycholinguistic variables
Ratings for other relevant psycholinguistic variables (see below) for the nouns and verbs were collected for correct responses (see below for the definition of correct response; total n = 1650 nouns for 669 object pictures, 146 verbs for 99 action pictures) produced in the name agreement rating. The participants were briefed by the first author about the study and the task requirements. They were provided with the stimulus and response booklets (or the link to an online response using Google Forms) and given 15–20 days to complete the tasks. Five participants completed the rating using Google Forms and the rest completed the task offline, returning the booklet to the investigator. Reminder messages to complete the ratings were sent periodically (every ~5 days) to participants’ mobile phones. Incomplete entries were identified and returned to the participants to complete and resend.
The participants were required to rate each stimulus on the following psycholinguistic variables:
Word familiarity: The participants rated each word using a five-point Likert scale (1: unfamiliar, 2: less familiar, 3: moderately familiar, 4: familiar, 5: very familiar) on how commonly they heard that word in their spoken language (Brown & Watson, 1987 ; Gilhooly & Logie, 1980 ).
Subjective frequency: As there are no subjective frequency counts for Kannada words, following Desrochers and Thompson ( 2009 ), we asked the participants to rate each word on its frequency of usage in daily communication using a five-point Likert scale (1: never; 2: rarely; 3: occasionally; 4: frequently; 5: very frequently).
Age of acquisition (AoA): For this task, the participants were instructed to rate the approximate age at which each word was acquired. Following Gilhooly and Logie ( 1980 ), we used a seven-point Likert scale (1: < 2 years; 2: 2–3 years; 3: 3–4 years; 4: 4–5 years; 5: 5–7 years; 6: 7–10 years; and 7: > 10 years).
Additionally, we calculated the number of syllables for all correct names. Note that for loan words, we have provided the syllable counts of both the loan words and their lexicalized forms in Kannada (e.g., syllable counts for pen in English (/peɳ/) is 1, and in Kannada (/pennu/) is 2). Supplementary files S2.xlsx for nouns and S3.xlsx for verbs provide the descriptive statistics for the psycholinguistic variables for every correct response in the corpus.
We also report the objective visual complexity of the images, obtained by retrieving the file size (in KB) of each of the images (see Mirman et al., 2010 ; Székely & Bates, 2000 , for similar measures).

Concept agreement
We first determined whether the concept referred to by the naming responses corresponded to the target depicted. For example, in English, “sofa”, “settee” and “couch” are all correct possible labels for the same concept. In contrast, the label “plastic glass” cannot be used as an adequate label for the concept of “garbage bin” (O’Sullivan et al., 2012 ). Following previous similar studies (e.g., Duñabeitia et al., 2018 ; O’Sullivan et al., 2012 ), when the depicted concepts corresponded with their names, we categorized them as correct (i.e., acceptable labels for the concept) and, when they did not, they were grouped as incorrect (i.e., labels that did not correspond to the concept displayed in the picture). Incorrect responses included semantically related (yet distinct entities: e.g., “harmonium” for “accordion”), and visually similar (yet semantically distinct entities: e.g., “banana” for [crescent] “moon”). The (rare) occasions of uncertainty regarding whether or not a label corresponded to a concept were resolved with the help of two native Kannada speakers considering the colloquial usage of such words (especially for loan words from English).
After eliminating the incorrect responses, the correct name(s) of each concept were rank-ordered. The most common (correct) name provided for a concept was taken as the modal name. Supplementary S2.xlsx and S3.xlsx file provides the raw frequency and percentage of the name(s) produced for each concept.
In contrast to the previous literature that provided name agreement data of the modal names alone, we provide all the correct names produced for each concept and their rate of occurrence. Further, we provide the ratings for word familiarity, subjective frequency, and age of acquisition for every correct name of the concepts.
The first author and three native Kannada speakers (all four being multilingual), classified the correct names into the following categories:

Commonly reported measures of name agreement data include the modal name and the H -statistic (an index of homogeneity of names produced: Snodgrass and Vanderwart, 1980 ). We report these measures calculated for nouns and verbs. We calculated the H -statistic using the following formula H = ∑ i = 1 kpi log 2 ( 1 / pi ), where k refers to the number of different responses to a picture across all participants, and p refers to the proportion of responses given for each name of a concept (Snodgrass & Vanderwart, 1980 ). The lowest value of the H -statistic is 0 (i.e., perfect name agreement: only a single name for a given concept), and a higher H value indicates the multiple names for that concept (Snodgrass & Vanderwart, 1980 ). Snodgrass and Vanderwart ( 1980 ) calculated the H -statistic by including all naming responses, excluding “IDK” and “TOT” responses. There is a lack of clarity in the literature on whether to include incorrect labels referring to a familiar concept (e.g., banana for crescent moon) or an unfamiliar concept (e.g., harmonium for accordion) in the calculation of H -statistics. However, George and Mathuranath ( 2007 ) excluded incorrect naming labels during H -statistic calculation. Hence, we calculated the H -statistic in two ways: (i) including all the names (i.e., correct and incorrect names, but excluding IDK and TOT responses) and (ii) including only the correct names.
Similarly, we calculated two measures of percentage agreement: (i) percentage picture–concept agreement—the percentage of responses that corresponded to the concept depicted by the picture (excluding IDK and TOT responses, similar to Snodgrass & Vanderwart, 1980 ; Singh et al., 2020 ) and (ii) percentage name agreement for all correct names. In line with other studies providing normative ratings (Bangalore et al., 2022 ; Brodeur et al., 2014 ; Duñabeitia et al., 2018 ; George & Mathuranath, 2007 ; Singh et al., 2020 ; Snodgrass & Vanderwart, 1980 ), we also examined the correlations between the psycholinguistic variables. The results of these analyses are provided in the Appendix (see Table 5 for nouns and Table 6 for verbs).
Additionally, we examined the variability of ratings across participants for the subjective ratings (image agreement, familiarity, subjective frequency, age of acquisition). To do this, we analysed the modal names of nouns ( n = 663) and verbs ( n = 93) using two methods. The first method used the intraclass correlation coefficient (ICC; Shrout & Fleiss, 1979 , also see similar methods in Momenian et al., 2021 ) to examine the reliability of participants’ ratings ( n = 30). We considered a two-way mixed model with an average rating selection (see Koo & Li, 2016 ; Shrout & Fleiss, 1979 ). The second method used split-half correlations in which we compared the ratings of the 30 participants divided into two groups (Group 1: even-numbered participants and Group 2: odd-numbered participants; for a similar method see Decuyper et al., 2021 ).
The summary statistics for the nouns and verbs are provided in Tables 1 and 2 , respectively. Supplementary S2.xlsx provides each noun picture’s (1) image agreement data, (2) H -statistic for all names (correct and incorrect), (3) H -statistic using only correct names, (4) concept agreement data, and (5) correct names in the descending order of occurrence with their frequency and the IPA transcription of each name. Supplementary file S3.xlsx provides the same data for the individual verb pictures.
We removed six noun pictures from the 669-item corpus due to poor concept agreement (i.e., burglar alarm, chemist, Horlicks, rug, sailing, tumble dryer), as they did not elicit any names corresponding to the target concept. For the remaining 663 pictures, the mean concept agreement was 90.64% (SD: 21.3%). The incorrect names and IDK responses constituted 4.27%, and names referring to the incorrect concepts comprised 5.09% of responses in the noun corpus. The majority of the items (n = 561; 84.6%) had concept agreement greater than 80%. Of the remaining 102 pictures, 34 had concept agreement between 61–80%, 30 between 41–60%, 22 between 21–40%, and 16 pictures below 20%.
The mean percentage of participants who used the modal (i.e., correct and most frequently produced) name for the concept was 71.24% ( SD = 25.19%). Table 1 provides the summary statistics at the group level for the modal nouns. The H -statistic for the nouns in this corpus was 0.89 (SD: 0.66) for all (correct and incorrect) labels, and remained high at 0.72 ( SD = 0.66) when only correct labels were included. There was high variability in correct naming responses, with up to seven names for a picture. Of the 663 nouns, 25.9 % (i.e., 172 pictures) had single names, 30.01 % (i.e., 199 pictures) had two names, and 23.52 % (i.e., 156 pictures) had three names. Figure 1 depicts the percentage distribution of the modal names for pictures with 1–7 names.
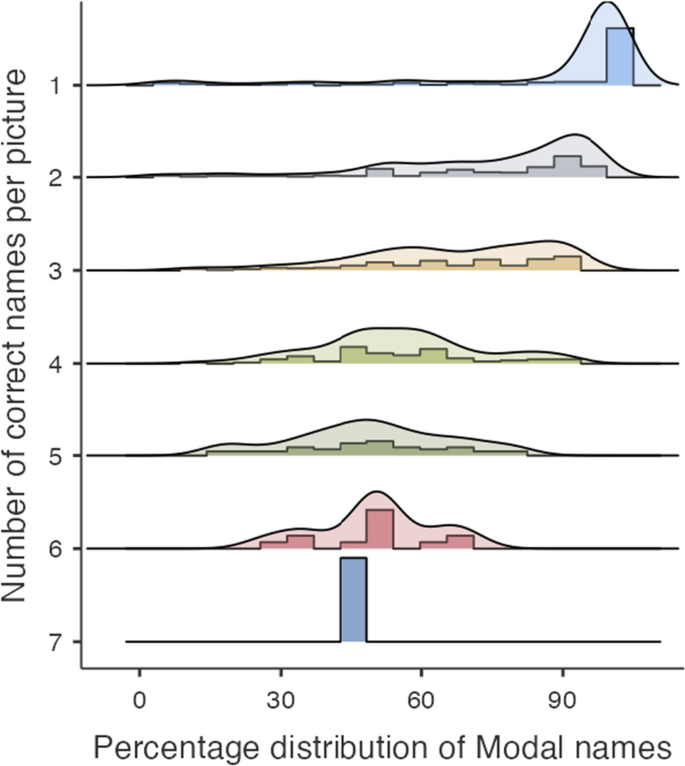
The percentage distribution of the modal names as a function of the number of correct names per picture. Notes: Of the 663 pictures, 172 had a single name, 199 had two names, 156 had three names, 81 had four names, 41 had five names, 13 had six names and one picture had seven names
The mean image agreement rating of the noun corpus was 4.00 ( SD = 0.16), with ratings for all but one noun (accordion: mean = 2.63, SD = 1.38) greater than 3. These relatively high mean image agreement ratings suggest that the noun pictures accurately reflected the stereotypical image of the modal names.
Familiarity, frequency, age of acquisition ratings, and syllable counts
The mean ratings of the modal names for familiarity, frequency, and age of acquisition are provided in Table 1 . Though all modal names were familiar (scale of 1–5: mean = 3.1; SD = 0.4), they had a relatively low mean subjective frequency (scale of 1–5: mean = 2.9; SD = 1.3) and a relatively high age of acquisition (scale of 1–7: mean = 4.85; SD = 1.3). The modal name for the nouns had a mean syllable count of 2.62 ( SD = 1.27).
Correlations between variables (nouns)
For nouns (see Appendix Table 5 ) the percentage of modal names (i.e., name agreement) showed a significant correlation with all other variables except for image agreement: items with higher name agreement and a lower H -statistic were more familiar, more frequent, lower in age of acquisition, had fewer syllables and were less visually complex. Concept agreement showed the same pattern, except that the correlation with the number of syllables was not significant.
In line with previous reports (Alario & Ferrand, 1999 ; Pompéia et al., 2001 ; Pind et al., 2000 ; Sanfeliu & Fernandez, 1996 ; Snodgrass & Vanderwart, 1980 ; Wolna et al., 2022 ), and as expected, we found negative correlations between the H -statistic and the percentage name agreement in the noun corpus. The nouns showed significant correlations between the psycholinguistic variables, except for image agreement which did not show any significant correlation with the remaining variables. This indicates that the extent to which the noun images reflected participants’ stereotypical view of that item was not influenced by any of the other factors. As would be expected, familiarity and frequency showed significant positive correlations with each other, and negative correlations with the AoA and the number of syllables. Similarly, as expected, the AoA and the number of syllables were positively correlated.
From the initial set of 99 verb pictures, we removed three items (remember, spend, fry) that did not elicit the target concept. The mean concept agreement for the remaining 96 verbs was 86.4% ( SD = 24.3: range: 13.3–100%). The mean percentage of “I don’t know” responses was 2.5% ( SD = 6.5). As for nouns, the majority of verb pictures (75%; n = 72) had concept agreement greater than 80%. Of the remaining 24, nine verbs had concept agreement ratings of between 80 and 61%, seven ratings of 60–41%, three of 40–21%, and five verbs of less than 20%.
Compared with nouns, the verbs had slightly higher mean percentage name agreement (modal names: mean = 78.05%; SD = 26.48) and lower mean H -statistic (with all responses: H = 0.52 [ SD = 0.56]; with correct responses: H = 0.28 [ SD = 0.47]). The number of correct names produced for verb pictures ranged between 1 and 4. Sixty-five verb pictures (68%) had only single names. Table 2 provides the summary statistics of the modal verbs.
Image agreement ratings
The mean image agreement of the verb corpus was 3.59 ( SD = 0.95). This relatively high rating suggests that the newly developed verb pictures largely depicted the stereotypical images of the modal names.
All modal verb responses were highly familiar (rated over 4 on a scale of 1–5) and were also of relatively high mean subjective frequency (4.63, scale of 1–5). The mean age of acquisition was 3.93 years (scale of 1–7). Thus, the modal verb names had higher familiarity and subjective frequency, and lower AoA compared with the modal nouns. The modal name for the root verbs had a mean syllable count of 2.52 ( SD = 0.99).
Correlations between variables (verbs)
For verbs (Table 6 ), percentage name agreement (modal name %) and the H -statistic were, as expected, negatively correlated with each other, but not significantly correlated with any other variable except for concept agreement. The only other significant correlations were between the age of acquisition and image agreement, with later-acquired verbs having higher image agreement, and a negative correlation between familiarity and number of syllables (verbs with fewer syllables were more familiar).
Reliability ratings
Appendix Table 8 provides the results of the ICC analysis (Shrout & Fleiss, 1979 ) using Koo and Li’s ( 2016 ) guidelines to interpret the correlation coefficients. Table 9 provides the results of the split-half correlation. For both nouns and verbs, the subjective frequency and age of acquisition ratings showed “excellent” ICC (> 0.90) and high split-half correlations (> .8). In terms of image agreement ratings, the verbs showed high ICC and split-half correlations (> 0.90), whereas the nouns showed poor reliability (ICC < 0.15; split-half < .05). Familiarity ratings for nouns showed moderate reliability (ICC > .6, split-half > .5) though the verbs revealed poor reliability (ICC < .2; split-half < .15).
Variability in the naming labels
Table 3 provides the frequency of occurrence of each response type (i.e., target language word, cognates, and translated equivalent) of modal names which were man-made and natural objects. Figure 2 provides word classifications (target language word, cognates, translated equivalent and elaboration) across acceptable alternatives (i.e., modal name, second name for the picture [CN2], third name of the picture [CN3]). We also provide some descriptive observations and examples in Table 4 . It is of particular note that there were strong cross-linguistic influences, with 110/663 instances (pictures which were modal names) of an English translation equivalent being used even when a Kannada word existed for the target (see Fig. 2 ).
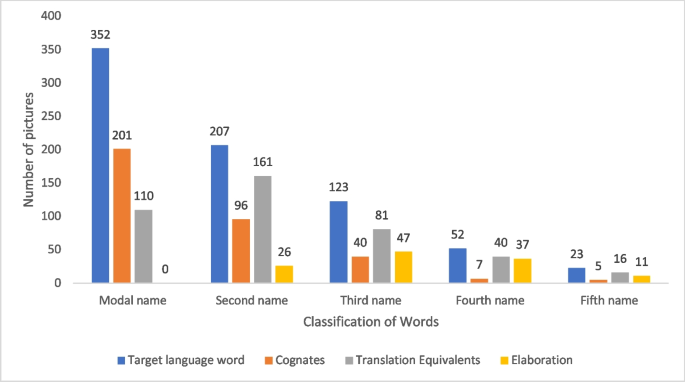
Number of pictures with their distribution of correct response types. Note: 352 pictures had the target language word as the modal name. Similarly, 201 pictures had cognates and 110 pictures had translation-equivalents as the modal names

We have reported on the development of a set of 759 colour pictures (663 noun pictures and 96 verb pictures) that we believe will prove a valuable asset for use in both research and clinical domains. To make the corpus of maximal clinical utility, we based the domains from which target names were drawn on objective data from a study of functionally relevant items (Palmer et al., 2017 ). Subsequently, we included as many picturable nouns and verbs as possible that could be useful across different clinical populations and in experimental work with unimpaired populations.
This corpus helps rectify the issue that, in under-resourced languages, a practical challenge faced by many clinicians and researchers is the lack of availability of culturally curated picture corpora. The use of pictures from other cultures is often inappropriate and unacceptable (Ahmed et al., 2022 ). However, the development of a large picture corpus requires extensive resources including time, professional (including artistic) skills, and budget, and hence is often not feasible. The corpus, the ICMR-Manipal Colour Picture Corpus, provides a culturally curated resource of pictures with norms on name agreement, image agreement and psycholinguistic ratings that can be used directly among speakers of Kannada, a language spoken by nearly 50 million people (Census of India, 2011 ). We believe that the pictures from this corpus may also be appropriate and useful for clinical and research purposes, and, after validation, in other similar sociocultural–linguistic backgrounds.
Beyond the corpus as a resource, we highlight the importance of not only extending corpora to different languages but also examining them in multilingual contexts. The findings from the current study provide several insights into the influence of the multilingual context of the participants on both naming and psycholinguistic variables. Though several well-known picture corpora have been adapted to many languages (e.g., Snodgrass & Vanderwart, 1980 ), the data from such adapted corpora may fail to reflect the cross-linguistic interactions among those languages as participants rated the pictures from multiple monolingual contexts. Below, we discuss these aspects of the current study.
Variability in naming
Substantial variability was found in the names produced by the multilingual speakers recruited here, particularly for nouns. This is reflected in the large number of correct names and the resulting high H -statistic (the H -statistic is an index of naming ambiguity—a picture with several names has a higher H -statistic: Snodgrass & Vanderwart, 1980 ).
The majority of the noun concepts in the corpus had more than one correct name. The mean H -statistic for nouns (all names: 0.89; correct concept names: 0.72) was higher in our corpus relative to previous investigations with monolingual speakers (0.47: Turkish, Raman et al., 2014 ; 0.36: French, Alario & Ferrand, 1999 ; 0.36: Polish, Wolna et al., 2022 ) and multilingual speakers (0.66: Kannada, Bangalore et al., 2022 ; 0.54: Malayalam, George & Mathuranath, 2007 ) except for Hindi speakers (0.90: Hindi, Singh et al., 2020 ). Correspondingly, the mean percentage of the modal names for the nouns (71%) was less than that in previous similar studies with monolingual speakers (83%: Turkish, Raman et al., 2014 ; 85%: French, Alario & Ferrand, 1999 ; 91%: Polish, Wolna et al., 2022 ; 81%: Hindi, Singh et al., 2020 ). Together, the lower mean percentage values of the modal names and the higher H -statistic indicate higher variability in the naming responses. Brodeur et al. ( 2010 ) report that their colour picture corpus received a lower percentage of modal names (64%) and a higher H -statistic (1.65) than the line drawing corpora. They suggest that this could be due to the influence of colour details leading to more alternate names (e.g., “pepper” versus “red pepper”, see Brodeur et al., 2010 , p. 7). The higher number of alternate names (see Fig. 1 ) and higher H statistics in the current corpus may be attributed to the usage of colour pictures like in Brodeur et al. ( 2010 ).
When examining the noun data, we noticed that the nature of the object appeared to influence the category of response. Indeed, when we divided the noun stimuli into those representing man-made concepts and those representing natural kinds, there was a clear difference (see Table 3 ) and there was a significant association between modal name classification and object categorisation (χ 2 = 109, df = 2, p < 0.01). That is, the concepts of human-made objects were predominantly tagged with cross-lingual names, and labels of natural objects were predominantly in the target language. This could plausibly be attributed to the fact that the names of new technology-based devices (e.g., computers) were borrowed from English and later served as independent lexical entities in Kannada. However, for some of such borrowed words, unique target language words were available. For instance, it was evident that many of the household articles (e.g., furniture, utensils) were named with translations despite the availability of their unique names in Kannada. Speakers often seemed to prefer the borrowed words to the translated words. One possibility is that translation equivalents were preferred when they were shorter than the target language names. Indeed, 34% of the translation equivalent modal names were of one syllable, relative to only 4% of the Kannada modal names (see Appendix Table 7 , χ 2 = 83.514, df = 9, p < 0.01). In addition, the frequent use of translation equivalents suggests that, for multilingual speakers who are exposed to English, when the target language word for an object is momentarily unavailable, the knowledge of the English language may provide an alternative means to name the object.
Compared with nouns, the verbs showed higher name agreement (verbs: 78%; nouns: 71%) and a lower H -statistic (all and correct responses of verbs: 0.52 and 0.28; of nouns: 0.89 and 0.72). Approximately a third of the verbs elicited only one name. In addition, the percentage of modal verb names was lower and the H -statistic was higher than those of another recent study in Kannada (Ahmed et al., 2022 ; mean H -statistic = 0.31 [ SD = 0.47]; mean % of modal name = 90.32% [ SD = 15.90], for three-argument verbs). However, the mean H -statistic for verbs in the current corpus was lower than that reported in a recent Polish study (Wolna et al., 2022 ; H -statistic: 0.89, mean % modal verb name: 79.39%).
We found that the cross-linguistic influence was less apparent in verbs compared with nouns. For instance, only 7/96 (7.3%) verbs showed translation equivalent names in contrast to 201/663 (30 %) nouns. While there is a rich body of literature on the differences between nouns and verbs at the conceptual level (e.g., Yang et al., 2017 ), these two grammatical classes seemed to experience different cross-linguistic influences. One possibility is that verbs are more difficult to replace as they undergo linguistic transformations. As the names of actions, verbs need additional morphemes to indicate the state of their occurrence. Especially in inflectional languages like Kannada, morphological and syntactic structures interact together to form complex morphosyntactic constructions. For example, the sentence “she is washing the vessels” in English would translate to “avaLu pa:tregaLu toLijuttida:Le”. Replacement of the same verb “wash” (“toLijuvudu”) with its English counterpart would require an additional morpheme (e.g., “avaLu pa:tragaLu clean ma:duttida:Le ”). Thus, it might be the economy of expression (e.g., Dalrymple et al., 2016 ) that makes the verbs (action words) more resilient to cross-lingual interference than the nouns.
Cross-linguistic influences
As noted earlier, we believe that to better elucidate crosslinguistic influences, data should be collected from people who speak multiple languages rather than from monolingual speakers of multiple languages. In the current study, name agreement norms were collected from native Kannada-speaking university students whose medium of instruction was English. Engaging participants from a multilingual context to rate various attributes in a specific language provided us with the opportunity to capture the natural crosslinguistic interactions including, as discussed above, the use of translation equivalents despite the availability of labels in the target language. The increased variability in noun names in the current study relative to previous studies supports our argument. For instance, a given concept (e.g., table) in a multilingual environment can be acceptably named using either the target language (e.g., me:ju IPA / me:d͡ʒu/) or its translation equivalent/loan word (i.e., table). Poor name agreement with higher concept agreement (that is, multiple names representing a given concept) has been regarded as an index of the richness of languages (Martein, 1995 ). Most Indian languages are lexically rich as multiple names exist for most nouns (e.g., the “sun” has nearly 10 synonyms in Kannada, Shabdkosh® ( 2023 )) although many are rarely used.
Our data showed the influence of English on the target language (Kannada). Though our participants were multilingual, the names were provided predominantly in Kannada and English. Only five names had local geographical influence (e.g. /bɑːlɖɪ/ (Tulu) – bucket, /bəɪɾɑːs̪/ (Konkani) – towel). However, none was the modal name in contrast to 30% of English names enjoying the modal status (see Table 4 and Fig. 2 ). The use of words from English can be attributed in part to the historical colonization in India by the English (Schneider, 2018 ), as well as the increasing dominance of English with globalization (Kalaja & Pitkänen-Huhta, 2020 ) and advances in communication technologies (Chibaka, 2018 ; Kelly-Holmes, 2019 ). When compared with other languages, the use of English as an alternative language has become an essential part of multilingualism (Aronin & Singleton, 2008 , 2012 ; Chengappa, 2001 ; Kalaja & Pitkänen-Huhta, 2020 ).
In India, people are generally aware of basic English vocabulary, such as the labels of household articles (e.g., groceries) as they are tagged with their English names. Such awareness is further augmented, for some concepts, by the lack of equivalents in the native language (e.g., guitar). These words become lexicalized to the native language (e.g., “pen” to /pennu/ in Kannada). The previously published picture corpora from India do acknowledge the influence of borrowed words (Singh et al., 2020 ; Bangalore et al., 2022 ), albeit briefly (i.e., focussing only on the modal names) and ignoring the nature of these words (i.e., whether or not there is a target language word). Thus, we believe the current analysis is more robust than its predecessors.
The psycholinguistic variables (frequency, familiarity, and age of acquisition) of the noun corpus also showed an apparent influence of the multilingual context. For instance, in our study, the mean familiarity and subjective frequency values were centred around 3. That means the modal names were only moderately frequent and familiar to the multilingual participants of the current study. This is likely, at least in part, due to the well-attested fact that because multilinguals speak in more than one language, inevitably the frequency of use of a word in any of those languages will be lower than that of the same word in monolinguals (e.g., Gollan et al., 2008 ). Similarly, the age of acquisition of the names varies across the languages of a multilingual speaker, depending on the context of acquisition of each language and the frequency of its use. Hence, the mean age of acquisition of the items in the noun corpus was relatively high for our participants.
Methodological notes
The H -statistic provides a measure of variability in the name agreement responses. Previously, to calculate the H -statistic, researchers included “dominant and non-dominant words” (which are both correct to a concept) and excluded the “don’t know and tip of the tongue” responses (Snodgrass & Vanderwart, 1980 ). In French norms (Alario & Ferrand, 1999 ) the concept “accordion” had 100% name agreement, with an H -statistic of 0, suggestive of perfect name agreement. In our corpus, “accordion” had 16.67% correct responses, and 80% of responses were incorrect naming using the label “harmonium”. However, as this label refers to a different concept this response could be reasonably excluded and the H -statistic would therefore be 0 (perfect name agreement). However, we believe that for name agreement data, it is important to consider all names (i.e., incorrect and correct labels). As noted above, most previous studies were not explicit regarding whether they had excluded incorrect concept names in H -statistic calculations, although George and Mathuranath ( 2007 ) explicitly note that incorrect responses were excluded for H -statistic calculation. Critically, we have reported the H -statistic both with and without incorrect names for transparency and comparison.
There is also a lack of clarity in the literature regarding whether “non-target” responses should be considered as correct responses. For example, Edmonds & Donovan ( 2014 ) consider “receipt” as a correct and acceptable response for a picture of a ticket, considering that it was the result of “picture ambiguity”. A similar example from our data is the picture of a pen that elicited “pencil” as the response. While it is tempting to conclude that the target name for this picture should, therefore, be “pencil”, this can cause difficulties in the use of such stimuli. For example, in a clinical study, if following intervention, a participant changed their response from “pencil” to “pen”, this improvement would not be apparent if “pencil” was also coded as a correct response.
Finally, we alert the reader to some of the limitations of our normative data. The first of these is also true of other databases: Our ratings were obtained from university students in an English-medium environment and it is unclear the extent to which the data can generalise to the broader population of (Kannada) speakers, particularly older adults, as well as to those with less exposure to English.
Secondly, while our ratings showed good reliability across participants for subjective frequency, age of acquisition ratings, familiarity for nouns, and image agreement for verbs, the image agreement ratings for nouns and familiarity ratings for verbs showed poor reliability. We are aware of only two instances where reliability has been reported for norms in the literature (Decuyper et al., 2021 ; Momenian et al., 2021 ) and neither provides image agreement ratings (or reliability). While Momenian et al. ( 2021 ) do provide familiarity ratings, and the reliability of these ratings is higher than ours (ICC .86–.95 depending on participant language background), they do not provide a breakdown of reliability separately across nouns and verbs.
Critically, however, the poor reliability of ratings for one word type rather than the other in our data was unlikely to be due to any participant-related factors, as the ratings were provided by the same participants for nouns and verbs. Nevertheless, it is possible that as these are multicultural, multilingual participants there is a complex interaction between the language background of the participants and their ratings that results in greater variability between participants for one word class relative to the other.
With regard to the poor reliability of image agreement for nouns relative to verbs, this mirrors the relatively more variable (lower H -statistic) naming responses to nouns, suggesting that the variability between participants in the name that they associated with a picture also influences the likelihood that the image does not agree with their mental image. It is also clearly the case that the mental images invoked by the concept of an object can differ from one participant to another. For example, there can be natural (e.g., physical: colour, size, shape, texture) variability in the real objects belonging to a conceptual category itself: the concept of a “pen” can refer to pens of several subtypes (e.g., ball pen, fountain pen, sketch pen) and within each subtype several variants (colour, precise shape, texture) are possible. Additionally, the individual association with the real objects may also influence this rating (e.g., a personally used pen vs. the depicted image). It is possible that the variability across speakers for concepts of action-related verbs is lower, although this hypothesis would need experimental support (see Kurland et al., 2014 , for a related idea: pictures where different objects are involved [e.g., trousers, shirt] under a particular category (e.g., clothing) elicit the same target verb (e.g., “wear”).
In terms of lower reliability of verb familiarity ratings relative to nouns, it is possible that the limited range of ratings is the likely source of the poor split-half correlations: verb ratings ranged from 4.2 to 4.8 (on a 1–5 scale), whereas noun ratings ranged from ~1.5 to 5.
Nevertheless, given their low reliability across participants, we are reluctant to recommend the use of our image agreement ratings for nouns and familiarity ratings for verbs. While this is clearly less than optimal, it is hard to know the extent to which this is unusual in ratings given that such reliability measures are rarely reported (but see Decuyper et al., 2021 ; Momenian et al., 2021 ). Consequently, we advocate that researchers report these measures routinely in the future to glean a better understanding of the extent to which ratings vary within and across participants. In addition, it is important that, as suggested by Mason et al. ( 2024 ), a clear protocol is developed for exclusion of problematic data sets and data points in the development of norms (e.g., Brysbaert et al., 2014 ; Warriner et al., 2013 ).
Further research that systematically examines the factors influencing the between-subject variability in the ratings (e.g., influence of age, other languages spoken, language of education) would also be valuable. Finally, investigations using these norms to examine the factors that predict picture naming speed in Kannada speakers will be important, although such analyses would be complex given the multiple acceptable responses for each target in a multilingual context.
We have presented the novel ICMR-Manipal Colour Picture Corpus, comprising 663 nouns and 96 verbs with their name agreement data as well as the ratings on the key psycholinguistic variables from a multilingual (Indian) context. This corpus may be used for clinical and research purposes. Unlike the normative data from studies on monolingual populations, the data from the current study are indicative of the apparent influence of the multilingual nature of the participants. As the world is becoming increasingly multilingual, we believe that further similar studies in other multilingual contexts are required.
Data availability
The picture corpus (.jpeg files) and the data set (Excel files) generated from this work are available in the OSF repository: https://osf.io/32m9a/?view_only=6d130363a7cd485890e71b8a74e7a53e .
The subcategories of human-made (e.g., clothing, vehicles) and natural (e.g., fruits, vegetables) stimuli can be viewed in Supplementary Excel file S2. We adapted Palmer et al. ( 2017 ) category list.
The verb classification can be viewed in the Supplementary Excel file S3. We used verb classification based on previous studies (Levin, 1993 ; Vinson & Vigliocco, 2002 ) as cited in Prarthana ( 2015 ).
The participants in the name and image agreement task had English as their medium of instruction at the University, and those who rated the words had had either Kannada or English as the medium of instruction at school/college.
These are words which are used by many speakers of the language, including people who were not exposed to the English language.
d̪uːɾə refers to “tele” and. d̪əɾʃən̪ə indicates “vision”.
Ahmed, W., Giridhar, P. P., & Krishnan, G. (2022). Development and validation of a novel corpus of 269 verb pictures in Kannada based on an argument structure taxonomy. Behavior Research Methods, 54 (3), 1358–1373. https://doi.org/10.3758/s13428-021-01662-x
Article PubMed Google Scholar
Alario, F.-X., & Ferrand, L. (1999). A set of 400 pictures standardized for French: Norms for name agreement, image agreement, familiarity, visual complexity, image variability, and age of acquisition. Behavior Research Methods, Instruments, & Computers, 31 (3), 531–552. https://doi.org/10.3758/BF03200732
Article Google Scholar
Alyahya, R. S. W., Halai, A. D., Conroy, P., & Lambon Ralph, M. A. (2020). Mapping psycholinguistic features to the neuropsychological and lesion profiles in aphasia. Cortex, 124 , 260–273. https://doi.org/10.1016/j.cortex.2019.12.002
Aronin, L., & Singleton, D. (2008). Multilingualism as a New Linguistic Dispensation. International Journal of Multilingualism, 5 (1), 1–16. https://doi.org/10.2167/ijm072.0
Aronin, L., & Singleton, D. (2012). Multilingualism (30th ed.). John Benjamins Publishing Company. https://doi.org/10.1075/impact.30
Book Google Scholar
Balatsou, E., Fischer-Baum, S., & Oppenheim, G. M. (2022). The psychological reality of picture name agreement. Cognition, 218 , 104947.
Bangalore, S., Robson, H., & Astell, A. J. (2022). Standardizing norms for 180 coloured Snodgrass and Vanderwart pictures in Kannada language. PLoS ONE, 17 (4), e0266359. https://doi.org/10.1371/journal.pone.0266359
Article PubMed PubMed Central Google Scholar
Bemani, Z., Moayedfar, S., & Ghasisin, L. (2022). Psycholinguistic variables influencing word retrieval in Persian speaking people with aphasia. Aphasiology, 36 (7), 868–882. https://doi.org/10.1080/02687038.2021.1907292
Bonin, P., Poulin-Charronnat, B., LukowskiDuplessy, H., Bard, P., Vinter, A., Ferrand, L., & Méot, A. (2020). IMABASE: A new set of 313 colourised line drawings standardised in French for name agreement, image agreement, conceptual familiarity, age-of-acquisition, and imageability. Quarterly Journal of Experimental Psychology, 73 (11), 1862–1878. https://doi.org/10.1177/1747021820932822
Bonin, P., Peereman, R., Malardier, N., Méot, A., & Chalard, M. (2003). A new set of 299 pictures for psycholinguistic studies: French norms for name agreement, image agreement, conceptual familiarity, visual complexity, image variability, age of acquisition, and naming latencies. Behavior Research Methods, Instruments, & Computers, 35 , 158–167.
Bose, A., & Schafer, G. (2017). Name agreement in aphasia. Aphasiology, 31 (10), 1143–1165. https://doi.org/10.1080/02687038.2016.1254148
Boukadi, M., Zouaidi, C., & Wilson, M. A. (2016). Norms for name agreement, familiarity, subjective frequency, and imageability for 348 object names in Tunisian Arabic. Behavior Research Methods, 48 (2), 585–599. https://doi.org/10.3758/s13428-015-0602-3
Bramão, I., Reis, A., Petersson, K. M., & Faísca, L. (2011). The role of color information on object recognition: A review and meta-analysis. Acta Psychologica, 138 (1), 244–253.
Brodeur, M. B., Dionne-Dostie, E., Montreuil, T., & Lepage, M. (2010). The Bank of Standardized Stimuli (BOSS), a New Set of 480 normative photos of objects to be used as visual stimuli in cognitive research. PLoS ONE, 5 (5), e10773. https://doi.org/10.1371/journal.pone.0010773
Brodeur, M. B., Guérard, K., & Bouras, M. (2014). Bank of Standardized Stimuli (BOSS) Phase II: 930 new normative photos. PLoS ONE, 9 (9), e106953. https://doi.org/10.1371/journal.pone.0106953
Brown, G. D. A., & Watson, F. L. (1987). First in, first out: Word learning age and spoken word frequency as predictors of word familiarity and word naming latency. Memory & Cognition, 15 (3), 208–216. https://doi.org/10.3758/BF03197718
Brysbaert, M. (1998). Word recognition in bilinguals: Evidence against the existence of two separate lexicons. Psychologica Belgica, 38 (3–4), 163. https://doi.org/10.5334/pb.932
Brysbaert, M., & Ellis, A. W. (2016). Aphasia and age of acquisition: are early-learned words more resilient? Aphasiology, 30 (11), 1240–1263. https://doi.org/10.1080/02687038.2015.1106439
Brysbaert, M., Warriner, A. B., & Kuperman, V. (2014). Concreteness ratings for 40 thousand generally known English word lemmas. Behavior Research Methods, 46 , 904–911.
Census of India 2011. Census of India 2011 - language atlas . Office of the Registrar General & Census Commissioner, India (ORGI) (2022, May 9). Retrieved on September 17, 2023, from https://censusindia.gov.in/nada/index.php/catalog/42561
Chengappa, S. (2001). Speech and language pathology in a multilingual context: Indian experience. Language in India, 3 , 1–8.
Google Scholar
Chibaka, E. F. (2018). Advantages of Bilingualism and Multilingualism: Multidimensional Research Findings. Multilingualism and Bilingualism. InTech. https://doi.org/10.5772/intechopen.74625
Chapter Google Scholar
Cuetos, F., Aguado, G., Izura, C., & Ellis, A. W. (2002). Aphasic naming in Spanish: predictors and errors. Brain and Language, 82 (3), 344–365. https://doi.org/10.1016/S0093-934X(02)00038-X
Cuetos, F., Gonzalez-Nosti, M., & Martínez, C. (2005). The picture-naming task in the analysis of cognitive deterioration in Alzheimer’s disease. Aphasiology, 19 (6), 545–557. https://doi.org/10.1080/02687030544000010
Cuetos, F., Rodríguez-Ferreiro, J., Sage, K., & Ellis, A. W. (2012). A fresh look at the predictors of naming accuracy and errors in Alzheimer’s disease. Journal of Neuropsychology, 6 (2), 242–256. https://doi.org/10.1111/j.1748-6653.2011.02025.x
Dalrymple, M., Kaplan, R. M., & King, T. H. (2016). Economy of Expression as a principle of syntax. Journal of Language Modelling, 3 (2). https://doi.org/10.15398/jlm.v3i2.82
Decuyper, C., Brysbaert, M., Brodeur, M. B., & Meyer, A. S. (2021). Bank of Standardized Stimuli (BOSS): Dutch Names for 1400 Photographs. Journal of Cognition, 4 (1). https://doi.org/10.5334/joc.180
Desrochers, A., & Thompson, G. L. (2009). Subjective frequency and imageability ratings for 3,600 French nouns. Behavior Research Methods, 41 (2), 546–557. https://doi.org/10.3758/BRM.41.2.546
Duñabeitia, J. A., Crepaldi, D., Meyer, A. S., New, B., Pliatsikas, C., Smolka, E., & Brysbaert, M. (2018). MultiPic: A standardized set of 750 drawings with norms for six European languages. Quarterly Journal of Experimental Psychology, 71 (4), 808–816. https://doi.org/10.1080/17470218.2017.1310261
Duñabeitia, J. A., Baciero, A., Antoniou, K., Antoniou, M., Ataman, E., Baus, C., Ben-Shachar, M., Çağlar, O. C., Chromý, J., Comesaña, M., Filip, M., Đurđević, D. F., Dowens, M. G., Hatzidaki, A., Januška, J., Jusoh, Z., Kanj, R., Kim, S. Y., Kırkıcı, B., …, & Pliatsikas, C. (2022). The Multilingual Picture Database. Scientific Data , 9 (1), 431. https://doi.org/10.1038/s41597-022-01552-7
Edmonds, L. A., & Donovan, N. J. (2014). Research applications for An object and action naming battery to assess naming skills in adult Spanish-English bilingual speakers. Behavior Research Methods, 46 (2), 456–471. https://doi.org/10.3758/s13428-013-0381-7
George, A., & Mathuranath, P. (2007). Community-based naming agreement, familiarity, image agreement and visual complexity ratings among adult Indians. Annals of Indian Academy of Neurology, 10 (2), 92. https://doi.org/10.4103/0972-2327.33216
Gilhooly, K. J., & Logie, R. H. (1980). Age-of-acquisition, imagery, concreteness, familiarity, and ambiguity measures for 1,944 words. Behavior Research Methods & Instrumentation, 12 (4), 395–427. https://doi.org/10.3758/BF03201693
Gollan, T. H., Montoya, R. I., Cera, C., & Sandoval, T. C. (2008). More use almost always means a smaller frequency effect: Aging, bilingualism, and the weaker links hypothesis☆. Journal of Memory and Language, 58 (3), 787–814. https://doi.org/10.1016/j.jml.2007.07.001
Hebart, M. N., Dickter, A. H., Kidder, A., Kwok, W. Y., Corriveau, A., Van Wicklin, C., & Baker, C. I. (2019). THINGS: A database of 1,854 object concepts and more than 26,000 naturalistic object images. PLOS ONE, 14 (10), e0223792. https://doi.org/10.1371/journal.pone.0223792
Kalaja, P., & Pitkänen-Huhta, A. (2020). Raising awareness of multilingualism as lived – in the context of teaching English as a foreign language. Language and Intercultural Communication, 20 (4), 340–355. https://doi.org/10.1080/14708477.2020.1786918
Karanth, P. (2007). With a little bit help–An early Language Training Kit. Journal of Rehabilitation Council of India, 3 (1). https://rehabcouncil.nic.in/sites/default/files/journals/jrci_2007.pdf
Karanth, P. (2003). The Alphasyllabaries of India—Kannada as Exemplar. Cross-Linguistic Study of Acquired Reading Disorders (pp. 23–36). Boston: Springer.
Kelly-Holmes, H. (2019). Multilingualism and Technology: A review of developments in digital communication from Monolingualism to Idiolingualism. Annual Review of Applied Linguistics, 39 , 24–39. https://doi.org/10.1017/S0267190519000102
Kim, H., Lu, X., Costa, M., Kandemir, B., Adams, R. B., Li, J., Wang, J. Z., & Newman, M. G. (2018). Development and validation of Image Stimuli for Emotion Elicitation (ISEE): A novel affective pictorial system with test-retest repeatability. Psychiatry Research, 261 , 414–420. https://doi.org/10.1016/j.psychres.2017.12.068
Koo, T. K., & Li, M. Y. (2016). A guideline of selecting and reporting intraclass correlation coefficients for reliability research. Journal of Chiropractic Medicine, 15 (2), 155–163.
Kurland, J., Reber, A., & Stokes, P. (2014). Beyond picture naming: Norms and patient data for a verb-generation task. American Journal of Speech-Language Pathology, 23 (2), S259–S270.
Levin, B. (1993). English verb classes and alternations: A preliminary investigation . University of Chicago press.
Manoiloff, L., Artstein, M., Canavoso, M. B., Fernández, L., & Segui, J. (2010). Expanded norms for 400 experimental pictures in an Argentinean Spanish-speaking population. Behavior Research Methods, 42 (2), 452–460. https://doi.org/10.3758/BRM.42.2.452
Martein, R. (1995). norms for name and concept agreement, familiarity, visual complexity and image agreement on a set of 216 pictures. Psychologica Belgica, 35 (4), 205. https://doi.org/10.5334/pb.886
Mason, C., Hameau, S., & Nickels, L. (2024). What can emotion and abstract words tell us about context availability ratings? Manuscript submitted for publication.
Mirman, D., Strauss, T. J., Brecher, A., Walker, G. M., Sobel, P., Dell, G. S., & Schwartz, M. F. (2010). A large, searchable, web-based database of aphasic performance on picture naming and other tests of cognitive function. Cognitive neuropsychology, 27 (6), 495–504.
Moayedfar, S., Purmohammad, M., Shafa, N., Shafa, N., & Ghasisin, L. (2021). Analysis of naming processing stages in patients with mild Alzheimer. Applied Neuropsychology: Adult, 28 (1), 107–116. https://doi.org/10.1080/23279095.2019.1599894
Momenian, M., Bakhtiar, M., Chan, Y. K., Cheung, S. L., & Weekes, B. S. (2021). Picture naming in bilingual and monolingual Chinese speakers: Capturing similarity and variability. Behavior Research Methods, 53 (4), 1677–1688. https://doi.org/10.3758/s13428-020-01521-1
Moon, J., & Jiang, N. (2012). Non-selective lexical access in different-script bilinguals. Bilingualism: Language and Cognition, 15 (1), 173–180. https://doi.org/10.1017/S1366728911000022
Moreno-Martínez, F. J., & Montoro, P. R. (2012). An ecological alternative to Snodgrass & Vanderwart: 360 high quality colour images with norms for seven psycholinguistic variables. PLoS ONE, 7 (5), 34–42. https://doi.org/10.1371/journal.pone.0037527
Nickels, L. (1995). Getting it right? Using aphasic naming errors to evaluate theoretical models of spoken word recognition. Language and Cognitive Processes, 10 (1), 13–45.
Nickels, L., & Howard, D. (1995). Aphasic naming: What matters? Neuropsychologia, 33 (10), 1281–1303.
Nishimoto, T., Miyawaki, K., Ueda, T., Une, Y., & Takahashi, M. (2005). Japanese normative set of 359 pictures. Behavior Research Methods, 37 (3), 398–416. https://doi.org/10.3758/BF03192709
O’Sullivan, M., Lepage, M., Bouras, M., Montreuil, T., & Brodeur, M. B. (2012). North-American norms for name disagreement: Pictorial stimuli naming discrepancies. PLoS ONE, 7 (10), e47802. https://doi.org/10.1371/journal.pone.0047802
Onie, S., Gong, S., Manwaring, E., Grageda, D., Webb, K., Yuen, W. S., & Most, S. B. (2020). Validation of the Australian beverage picture set: A controlled picture set for cognitive bias measurement and modification paradigms. Australian Journal of Psychology, 72 (2), 223–232. https://doi.org/10.1111/ajpy.12272
Palmer, R., Hughes, H., & Chater, T. (2017). What do people with aphasia want to be able to say? A content analysis of words identified as personally relevant by people with aphasia. PLOS ONE, 12 (3), e0174065. https://doi.org/10.1371/journal.pone.0174065
Pind, J., Jónsdóttir, H., Gissurardóttir, H., & Jónsson, F. (2000). Icelandic Norms for the Snodgrass and Vanderwart (1980) Pictures: Name and image agreement, familiarity, and aage of acquisition. Scandinavian Journal of Psychology, 41 (1), 41–48. https://doi.org/10.1111/1467-9450.00169
Pompéia, S., Miranda, M. C., & Bueno, O. F. A. (2001). A set of 400 pictures standardised for Portuguese: norms for name agreement, familiarity and visual complexity for children and adults. Arquivos de Neuro-Psiquiatria, 59 (2B), 330–337. https://doi.org/10.1590/S0004-282X2001000300004
Prarthana, S. (2015). Mental lexicon of nouns and verbs in adult speakers of Kannada [Unpublished doctoral thesis, University of Mysore].
Raman, I., Raman, E., & Mertan, B. (2014). A standardized set of 260 pictures for Turkish: Norms of name and image agreement, age of acquisition, visual complexity, and conceptual familiarity. Behavior Research Methods, 46 (2), 588–595. https://doi.org/10.3758/s13428-013-0376-4
Renvall, K., Nickels, L., & Davidson, B. (2013a). Functionally relevant items in the treatment of aphasia (part I): Challenges for current practice. Aphasiology, 27 (6), 636–650. https://doi.org/10.1080/02687038.2013.786804
Renvall, K., Nickels, L., & Davidson, B. (2013b). Functionally relevant items in the treatment of aphasia (part II): Further perspectives and specific tools. Aphasiology, 27 (6), 651–677. https://doi.org/10.1080/02687038.2013.796507
Rossion, B., & Pourtois, G. (2004). Revisiting Snodgrass and Vanderwart’s object pictorial set: The role of surface detail in basic-level object recognition. Perception, 33 (2), 217–236. https://doi.org/10.1068/p5117
Sanfeliu, M. C., & Fernandez, A. (1996). A set of 254 Snodgrass-Vanderwart pictures standardized for Spanish: Norms for name agreement, image agreement, familiarity, and visual complexity. Behavior Research Methods, Instruments & Computers, 28 (4), 537–555. https://doi.org/10.3758/BF03200541
Schneider, E. W. (2018). English and colonialism. The Routledge handbook of English language studies (pp. 42–58). Routledge.
Shabdkosh®. (2023). Sun meaning in Kannada: Sun translation in Kannada . https://www.shabdkosh.com/search-dictionary?lc=kn&sl=en&tl=kn&e=sun . Retrieved on September 17, 2023.
Shrout, P. E., & Fleiss, J. L. (1979). Intraclass correlations: Uses in assessing rater reliability. Psychological bulletin, 86 (2), 420.
Silagi, M. L., Bertolucci, P. H. F., & Ortiz, K. Z. (2015). Naming ability in patients with mild to moderate Alzheimer’s disease: What changes occur with the evolution of the disease? Clinics, 70 (6), 423–428. https://doi.org/10.6061/clinics/2015(06)07
Singh, P., Pauranik, N., & Pauranik, A. (2020). Culturally appropriate stimuli for cognitive neuropsychology-based treatment “intensive language action therapy (ILAT).” Annals of Indian Academy of Neurology, 23 (8), 135. https://doi.org/10.4103/aian.AIAN_670_20
Snodgrass, J. G., & Vanderwart, M. (1980). A standardized set of 260 pictures: Norms for name agreement, image agreement, familiarity, and visual complexity. Journal of Experimental Psychology: Human Learning and Memory, 6 (2), 174–215. https://doi.org/10.1037/0278-7393.6.2.174
Souza, C., Garrido, M. V., & Carmo, J. C. (2020). A systematic review of normative studies using images of common objects. Frontiers in Psychology , 11 . https://doi.org/10.3389/fpsyg.2020.573314
Székely, A., & Bates, E. (2000). Objective visual complexity as a variable in studies of picture naming. Center for Research in Language Newsletter, 12 (2), 3–33.
Szekely, A., Jacobsen, T., D’Amico, S., Devescovi, A., Andonova, E., Herron, D., Lu, C. C., Pechmann, T., Pléh, C., Wicha, N., Federmeier, K., Gerdjikova, I., Gutierrez, G., Hung, D., Hsu, J., Iyer, G., Kohnert, K., Mehotcheva, T., Orozco-Figueroa, A., …, & Bates, E. (2004). A new on-line resource for psycholinguistic studies. Journal of Memory and Language , 51 (2), 247–250. https://doi.org/10.1016/j.jml.2004.03.002
Szymanska, M., Comte, A., Tio, G., Vidal, C., Monnin, J., Smith, C. C., Nezelof, S., & Vulliez-Coady, L. (2019). The Besançon affective picture set-adult (BAPS-Adult): Development and validation. Psychiatry Research, 271 , 31–38. https://doi.org/10.1016/j.psychres.2018.11.005
van Hell, J. G., & Dijkstra, T. (2002). Foreign language knowledge can influence native language performance in exclusively native contexts. Psychonomic Bulletin & Review, 9 (4), 780–789. https://doi.org/10.3758/BF03196335
Vinson, D. P., & Vigliocco, G. (2002). A semantic analysis of grammatical class impairments: semantic representations of object nouns, action nouns and action verbs. Journal of Neurolinguistics, 15 (3–5), 317–351.
Warriner, A. B., Kuperman, V., & Brysbaert, M. (2013). Norms of valence, arousal, and dominance for 13,915 English lemmas. Behavior research methods, 45 , 1191–1207.
Wolna, A., Łuniewska, M., Haman, E., & Wodniecka, Z. (2022). Polish norms for a set of colored drawings of 168 objects and 146 actions with predictors of naming performance. Behavior Research Methods, 55 (5), 2706–2732. https://doi.org/10.3758/s13428-022-01923-3
Yang, H., Lin, Q., Han, Z., Li, H., Song, L., Chen, L., He, Y., & Bi, Y. (2017). Dissociable intrinsic functional networks support noun-object and verb-action processing. Brain and Language, 175 , 29–41. https://doi.org/10.1016/j.bandl.2017.08.009
Download references
Acknowledgements
We acknowledge our study participants for their time and effort while providing their ratings We also acknowledge our hired digital artist who made the pictures. We thank Dr Vani Lakshmi (Department of Data Science, Manipal Academy of Higher Education), who helped us with calculation of the H -statistic. We sincerely acknowledge the anonymous reviewers who have reviewed our manuscript and provided valuable suggestions/revisions.
Open access funding provided by Manipal Academy of Higher Education, Manipal. This study received partial financial support from the Indian Council of Medical Research (ICMR), Govt. of India. The picture corpus was developed using financial support from the ICMR project titled Mixed reality in aphasia rehabilitation (MiRAR, Ref: 5/3/8/6/2019-ITR), to the PI (Dr Gopee Krishnan). This work is a part of the doctoral study of the first author. During this study, the first author received a fellowship from ICMR-Senior research fellowship (File no: 3/1/3/10/Dis and Rehab/2022-NCD-II).
Author information
Authors and affiliations.
Department of Speech and Hearing, Manipal College of Health Professions, Manipal Academy of Higher Education, Manipal, India
Rajath Shenoy & Gopee Krishnan
School of Psychological Sciences, Macquarie University, Sydney, Australia
Lyndsey Nickels
You can also search for this author in PubMed Google Scholar
Corresponding author
Correspondence to Gopee Krishnan .
Additional information
Publisher's note.
Springer Nature remains neutral with regard to jurisdictional claims in published maps and institutional affiliations.
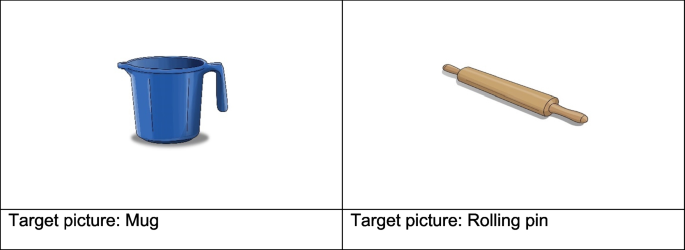
Sample pictures from the noun corpus
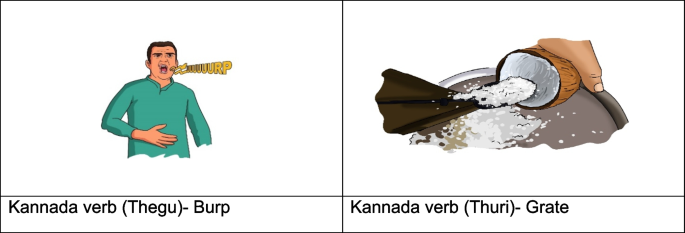
Sample pictures from the verb corpus
Rights and permissions
Open Access This article is licensed under a Creative Commons Attribution 4.0 International License, which permits use, sharing, adaptation, distribution and reproduction in any medium or format, as long as you give appropriate credit to the original author(s) and the source, provide a link to the Creative Commons licence, and indicate if changes were made. The images or other third party material in this article are included in the article's Creative Commons licence, unless indicated otherwise in a credit line to the material. If material is not included in the article's Creative Commons licence and your intended use is not permitted by statutory regulation or exceeds the permitted use, you will need to obtain permission directly from the copyright holder. To view a copy of this licence, visit http://creativecommons.org/licenses/by/4.0/ .
Reprints and permissions
About this article
Shenoy, R., Nickels, L. & Krishnan, G. Naming in a multilingual context: Norms for the ICMR-Manipal colour picture corpus in Kannada from the Indian context. Behav Res (2024). https://doi.org/10.3758/s13428-024-02439-8
Download citation
Accepted : 24 April 2024
Published : 24 June 2024
DOI : https://doi.org/10.3758/s13428-024-02439-8
Share this article
Anyone you share the following link with will be able to read this content:
Sorry, a shareable link is not currently available for this article.
Provided by the Springer Nature SharedIt content-sharing initiative
- Picture corpus
- Multilingual
- Find a journal
- Publish with us
- Track your research
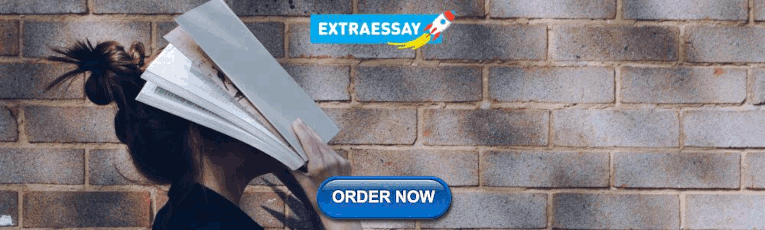
COMMENTS
#motivationalstories #success #successmotivation The Best Motivational StoriesThe Best Motivational speakerDr. Gururaj Karajagi, a doctoral degree holder in...
#kempegowdastatue #kempegowdaspeech #kempegowdajayanti kempegowda speech in Kannada kempegowda Hindi speech in Kannada kempegowda jayanti speech in Kannada k...
Notes. This library of books, audio, video, and other materials from and about India is curated and maintained by Public Resource. The purpose of this library is to assist the students and the lifelong learners of India in their pursuit of an education so that they may better their status and their opportunities and to secure for themselves and for others justice, social, economic and political.
Download language pack for speech. Windows 10 Cortana. For some languages, you might need to manually download a speech pack. Go to Start and open Settings . Select Time & language > Language. Select the language you want to add speech to, and then select the Next button. Select the speech options you want included with the language.
Google's service, offered free of charge, instantly translates words, phrases, and web pages between English and over 100 other languages.
Click here to Subscribe to our channel: https://goo.gl/iLb7RGFor more Kannada Learning Videos.Hello everyone,We got many requests to do a detailed series in ...
VEED automatically converts voice to text in Kannada and 100 languages straight from your Google Chrome and other browsers. Transcribe audio and video accurately in one click. Transform Kannada voice recordings into editable text, streamlining communication and saving you valuable time. You can also auto-generate subtitles!
A 100-year-old high school in the heart of Bengaluru was instrumental in encouraging a young freedom fighter to fight against the tyrannical British rule. Interestingly, Hanumanthappa Narasimhaiah's journey began with a Hindi speech which needed to be translated in Kannada! In this episode of Culture 'Shauk' with Sunita Iyer, we revisit the story of how the Hindi language became a ...
ಹೆದರದೇ ಮುಂದೆ ಸಾಗಿ - One Minute Motivation in Kannada - Motivational Speech in Kannada ... Hindi, Marathi and Kannada), Motivational Speaker, Entrepreneur and independent filmmaker from India. And also he is the co founder and CEO of Roaring Creations Pvt Ltd India.
A 100-year-old high school in the heart of Bengaluru was instrumental in encouraging a young freedom fighter to fight against the tyrannical British rule.. Interestingly, his journey began with a Hindi speech which needed to be translated in Kannada! The National High School (NHS) in Basavanagudi, Bengaluru (then Bangalore), was founded by Annie Besant in 1917—the year she became the ...
Kannada Text-to-speech is a technology that converts written text into spoken words. It has numerous applications and is used in various contexts, such as accessibility, language learning, and entertainment. text-to-speech technology is becoming increasingly popular as it can improve accessibility and convenience for people with visual impairments or those who prefer audio content.
With Narakeet you can generate text to speech in Indian Kannada accent, and many more Indian languages. Just type in script or upload a Word document into our Narakeet text to voice tool, select one of the Kannada voices, and click the "Create Audio" button. Get started with our Kannada text to speech free online, without even registering.
Most of the text-to-speech tools work similarly. You must type the text you want to convert to voice or copy from the text file into the input box. Then you have to select the voices available and preview the audio. We are talking Kannada (India) here, so you need to choose the language and accent of the Kannada (India).
Instruction. You must have a good quality mic. You have to speak loud and clear. It is a Kannada (ಕನ್ನಡ) voice typing tool. Click on the Mic icon and start speaking in Kannada (ಕನ್ನಡ), it will be converted to text. You can write Kannada (ಕನ್ನಡ) by speaking in Kannada here.
Deep learning based text-to-speech (TTS) systems have been evolving rapidly with advances in model architectures, training methodologies, and generalization across speakers and languages. ... Gujarati, Hindi, Kannada, Malayalam, Manipuri, Marathi, Odia, Rajasthani, Tamil and Telugu. Authors: Gokul Karthik Kumar*, Praveen S V*, Pratyush Kumar ...
Narakeet makes it easy to create videos and audio files with life-like audio from text. Get started with Hindi text to speech free. Select from one of our text-to-speech Hindi male and female voices below, and enter some text to create the audio. In addition to these voices, Narakeet has 700 text-to-speech voices in 100 languages.
Our Kannada to Hindi Translation Tool is powered by Google Translation API. You can start typing in the left-hand text area and then click on the "Translate" button.Our app will then translate your Kannada word, phrase, or sentence into hindi. You can also visit our homepage to type in Kannada.. The translation only takes a few seconds and allows up to 500 characters to be translated in one ...
Learn Kannada in 5 days with our list of 600 most common expressions and words. This is a crash course in Kannada / Kanarese / Canarese. Try to memorize all ...
ಬದುಕುವ ಜನ ಒಂದೇ. ನೆಳಲು ಬೆಳಕುಗಳ ರೆಕ್ಕೆಯ ಬಿಚ್ಚುತ. ಹಾರಾಡುವ ಧ್ವಜ ಒಂದೇ. ಒಂದೇ ಒಂದೇ ನಾವೆಲ್ಲರೂ ಒಂದೇ. ಧನ್ಯವಾದಗಳು (Speech written by Aziz) 2. Republic Day Speech in Kannada 2020 ...
Parts of Speech (शब्द के भेद) Just like English, which has its own basic types of words known as part of speech, Hindi on the other hand has them too. Let us learn them one by one. Shabdo ke prakar (Parts of speech): Parts of speech in Hindi are similar to the ones which we have in English. There are eight parts of speech namely:
Convert Hindi Speech into Text (Voice Typing) Here you have to use desktop Chrome browser with proper internet connection and connect your microphone with the computer. Microphone should be of good quality and placed near to your mouth. Then just click on the microphone button available above the text box and speak slowly and clearly.
Kannada Voice typing is also known as speech-to-text or Kannada voice recognition. This technology converts Kannada spoken words into Kannada written text. Voice typing facility is also available in Bangla , Gujarati , Hindi , Marathi , Tamil , Telugu , Urdu , and English on our website.
Give a motivational 26 January Speech In Kannada Language and Download this PDF File For preparing to your school programs. Welcome all of you in this site and as you know Indian Republic Day is the biggest national event and celebrated every year on 26 January.
The summary statistics for the nouns and verbs are provided in Tables 1 and 2, respectively.Supplementary S2.xlsx provides each noun picture's (1) image agreement data, (2) H-statistic for all names (correct and incorrect), (3) H-statistic using only correct names, (4) concept agreement data, and (5) correct names in the descending order of occurrence with their frequency and the IPA ...