- Money Museum
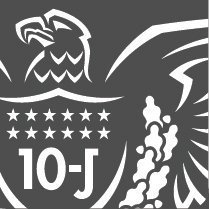
- Community Development
- TEN Magazine
Stay up-to-date on the latest briefings from the Kansas City Fed by subscribing to e-alerts.
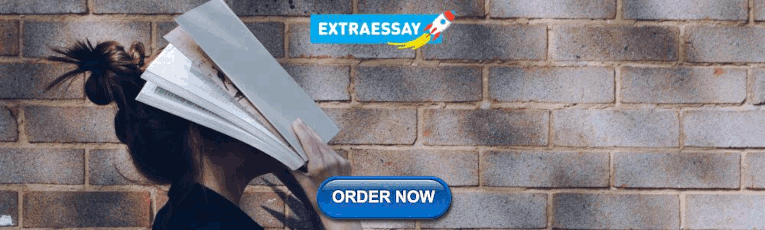
Payments System Research Briefings
Briefings cover a range of topics, including payments methods, developments in payments networks, and various participants' roles in the payments system.
Recent Briefings
Observations from the retail cbdcs of the caribbean.
The retail CBDCs launched in the Caribbean are not yet widely used but may offer insight on rCBDC adoption.
Market Structure of Core Banking Services Providers
Three core providers dominate the market for core banking systems, which may affect banks’ ability to offer new services.
Core Banking Systems and Options for Modernization
Many depository institutions need to update their back-end IT “core systems” to offer modern banking services.
How Much Do Nonbank Transaction Accounts Improve Access to Digital Payments for Unbanked Households?
About 40 percent of unbanked households had a nonbank transaction account in 2021, allowing them access to digital payments.
Social Media for Personal Finances: A New Trend for Millennials and Gen Z
Social media platforms are increasingly where younger generations make payments, shop, raise money, and learn about finance.
The Controversial Business of Cash-to-Crypto Bitcoin ATMs
The Bitcoin ATM industry continues to grow in the United States amid concerns about illegal or financially predatory behavior.
To receive email alerts for Payments System Research Briefings and other research publications, subscribe here .

An official website of the United States government
The .gov means it’s official. Federal government websites often end in .gov or .mil. Before sharing sensitive information, make sure you’re on a federal government site.
The site is secure. The https:// ensures that you are connecting to the official website and that any information you provide is encrypted and transmitted securely.
- Publications
- Account settings
Preview improvements coming to the PMC website in October 2024. Learn More or Try it out now .
- Advanced Search
- Journal List

The ethical anatomy of payment for research participants
Joanna Różyńska
Center for Bioethics and Biolaw, Faculty of Philosophy, University of Warsaw, Krakowskie Przedmiescie 3, 00-047 Warsaw, Poland
Associated Data
Not applicable.
In contrast to most publications on the ethics of paying research subjects, which start by identifying and analyzing major ethical concerns raised by the practice (in particular, risks of undue inducement and exploitation) and end with a set of—more or less well-justified—ethical recommendations for using payment schemes immune to these problems, this paper offers a systematic, principle-based ethical analysis of the practice. It argues that researchers have a prima facie moral obligation to offer payment to research subjects, which stems from the principle of social beneficence. This principle constitutes an ethical “spine” of the practice. Other ethical principles of research ethics (respect for autonomy, individual beneficence, and justice/fairness) make up an ethical “skeleton” of morally sound payment schemes by providing additional moral reasons for offering participants (1) recompense for reasonable expenses; and (2a) remuneration conceptualized as a reward for their valuable contribution, provided (i) it meets standards of equality, adequacy and non-exploitation, and (ii) it is not overly attractive (i.e., it does not constitute undue inducement for participation or retention, and does not encourage deceptive behaviors); or (2b) remuneration conceptualized as a market-driven price, provided (i) it is necessary and designed to help the study achieve its social and scientific goals, (ii) it does not reinforce wider social injustices and inequalities; (iii) it meets the requirement of non-exploitation; and (iv) it is not overly attractive. The principle of justice provides a strong ethical reason for not offering recompenses for lost wages (or loss of other reasonably expected profits).
What are the ethical principles or values which constitute an ethical rationale for paying research subjects? Do those create a moral obligation to pay individuals for participation in biomedical research, or rather a mere justification for its acceptability? What other ethical principles and values shape the payment practice, and how? Surprisingly, these questions have been rarely the subject of in-depth discussions in the literature. Instead of exploring the ethical foundations of payments systematically, scholars and public-policy makers rather focus on payment-related ethical concerns, in particular of undue inducement and exploitation, and—from this perspective—recommend or discourage certain forms, schedules and timings of payment commonly used in research practice (e.g., Macklin 1981 ; Dickert and Grady 1999 ; Grady 2001 , 2005 ; Gelinas et al. 2018 ; Resnik 2015 , 2019 ; Largent and Lynch 2017a , 2017b ). As a consequence—while there is a growing consensus that an ethically sound payment scheme should avoid both excessive payment and underpayment, and it should include, at least, reimbursement of reasonable expenses and compensation for some contributions made by research subjects—there is no generally accepted view on whether a payment to research subjects (as such or of a certain kind) is a moral obligation (Council for International Organizations of Medical Sciences 2016 ), merely an “acceptable practice” (Food and Drug Administration 2018 ), “ethically discretionary” activity (Persad et al. 2019 , p. 319), or just a “necessary evil”. Equally, there is no common view on what constitutes an ethical source of this purported obligation or acceptability of payment (as such or of a certain kind), and which ethical reasons lie behind different payment categories and schemes.
This paper aims at clarifying these issues. It presumes that any discussion on ethically sound payment practice should be preceded by a clear statement of ethical reasons for paying research participants, their deontic nature and mutual relations. Without full understanding of the ethical anatomy of payment, it is impossible to determine what we owe, if anything, to research subjects—what for, and how much research participants should be paid.
Preliminary terminological remarks
Paying research subjects for their participation in biomedical studies is an increasingly common practice across different types of research involving healthy volunteers and patients (Grady et al. 2005 ; Largent and Lynch 2017a ). Nevertheless, the payment continues to raise numerous conceptual, ethical and practical controversies among bioethicists, investigators, research ethics committees/institutional review boards (RECs/IRBs), and other members of the research community. Although prominent international guidelines and national regulations call attention to the crucial moral issues that payment raises (in particular, the risk of undue influence), they offer little substantive guidance on how to pay research subjects in an ethical way, and if they do so, they often provide contradictory advice. For instance, the World Medical Association Declaration of Helsinki ( 2013 ) does not address payment for research participation directly. It only mentions that information on “incentives for subjects” must be contained in the study protocol (par. 22). Also, the U.S. “Common rule” (Department of Health and Human Services 2018 ) and the European Union “Clinical Trial Regulation” ( 2014 ) offer very limited guidance on payment for participants. All these regulatory lacunas and contradictions are reflected in considerable variation in local payment policies and practices around the world (Dickert et al. 2002 ; Grady et al. 2005 ; Fry et al. 2005 ; Ndebele et al. 2008 ; Pasqualetti et al. 2010 ; Roche et al. 2013 ; Largent and Lynch 2017a ).
One of the factors which adds to this confusion is the diversity and ambiguity of terminology used in the literature and guidelines on research payment. For example, the International ethical guidelines for health-related research involving humans of the Council for International Organizations of Medical Sciences (CIOMS) make a distinction between “reimbursement” for reasonable direct costs incurred by research subjects and “compensation” for the time spent and other inconveniences resulting from study participation ( 2016 , Guideline 13 and Commentary). The Guideline for good clinical practice issued by the International Conference on Harmonisation of Technical Requirements for Registration of Pharmaceuticals for Human Use speaks of “payments and compensation” ( 2016 , par. 3.1.2., 3.1.8., 3.1.9.) with no further explanation. The U.S. Food and Drug Administration Guidance for institutional review board and clinical investigators: payment and reimbursement to research subjects ( 2018 ) makes a distinction between “reimbursement” for direct costs and other “payment” for participation, which it considered to be more ethically challenging as in some cases it may constitute an undue influence to prospective subjects. The Council of Europe’s Additional Protocol to the Convention on human rights and biomedicine, concerning biomedical research mentions „payments and rewards” without any additional differentiations, in the Appendix containing a template of an information sheet for the ethics committee ( 2005a ). The term is “unpacked” in the Explanatory Report to Article 12 of the Additional Protocol dealing with undue inducement which refers to “compensation” for burdens and inconveniences and “reimbursement” for expenses and financial losses (Council of Europe 2005b , sec. 64). Australian guidelines on Payment of participation in research: information for researchers, HRECs and other ethics review bodies issued by the National Health and Medical Research Council ( 2019 ) divide “payment” for participants in four analytical categories: “reimbursement” for any research-related expenses; “compensation” for any documented financial losses resulting from participation in research, including loss of wages, or from an injury suffered as a direct consequence of participation; “remuneration” paid to participants in recognition of their service for the time spent and other inconveniences resulting from participation; and “incentive or inducement” provided to individuals simply to encourage their enrolment or continuation in research. In the United Kingdom, the NHS Health Research Authority guidance ( 2014 ) follows terminology developed by the Nuffield Council on Bioethics. The Council, in its report Human bodies: donation for medicine and research ( 2011 ) distinguishes three forms of payment: (i) “recompense” offered in recognition of losses incurred which may take a form of “reimbursement” of direct financial expenses, lost earnings, or “compensation” for non-financial losses, such as time, inconvenience and discomfort; (ii) “reward” defined as a “material advantaged gained by a person …that goes beyond ‘decompensating’ the person for losses they incurred”; “reward” becomes “remuneration” when calculated as a wage or equivalent”; and (iii) “purchase”—money given in exchange of a “thing” (e.g., biological material for research) (Nuffield Council on Bioethics 2011 , p. 2).
In order to bring an end to this terminological (and conceptual) chaos, in this analysis, “payment” is used as an overarching term that encompasses all forms of financial and in-kind support provided to research participants. 1 It covers two sub-categories, which will be referred to as “recompense” and “remuneration”. These categories are distinguished by a different impact each of them has on the participant’s economic position as evaluated ex post .
The term “recompense” stands for any payment that entails no net benefit to recipients. Recompense amends to research participants for financial and non-financial losses or injuries resulting from their participation in research. Thus, this type of payment does not constitute a gain or profit, but merely covers—understood in broad terms—costs of the participation. Recompense may include three sub-categories of payments: (i) reimbursement of direct financial expenses incurred as a result of participation in research (e.g. costs of travel, accommodation, meals, childcare); (ii) compensation for indirect financial expenses, i.e., losses, that arise from participation in research (e.g. loss of wage in result of taking unpaid leave from work); and (iii) compensation for financial and non-financial losses resulting from injuries suffered as a direct consequence of participation in research.
“Remuneration” refers to any payment provided to individuals for their service as research participants, which exceeds expenses, losses or injuries experienced by participants as a result of their participation, and brings net benefit (gain, profit) for recipients. The remuneration for research participation (same as for “regular work”) may be understood either as a reward given in recognition of and as an appreciation for participants’ valuable contribution; or as a price—money given in direct, market-driven exchange for a service provided by participants (cf. Moriarty 2020 ; Różyńska 2021 ). If the remuneration is understood as a reward, the payment should be adequate to the desert, i.e., to the value of participants’ contribution. The latter value necessary depends on various factors, including (i) time allotted to research; (ii) efforts or types of services rendered (e.g., performing psychological or physical tests, taking drugs or using device as instructed, conducting self-monitoring or gathering other research-relevant information); (iii) discomfort, burdens or inconveniences associated with participation (e.g., stress, pain, suffering, but also burdens related to sticking to a dietary regime or inconveniences caused by the expected lifestyle changes); (iv) the level of risk involved in research, and (v) special/unique value of the input of the specific subject (e.g. due to rarity of a disease suffered by the person or her genetic make-up). If remuneration is considered as a price for participation, the amount of payment is determined by market forces—supply and demand, and it does not have to be proportionate to the value of the subjects’ contribution. In literature and guidelines, the latter way of thinking about payment if often concealed under the concept of “incentive” or “inducement”, although—as it will be explained later—these labels are far from being adequate and should be abandoned.
The above typology of payments for research participation is preferable over other schemes proposed in the literature and regulatory documents at least for three reasons. First, it is built upon one clearly defined, objective and disjoint divisional criterion, thereby avoiding a mistake of mixing entirely different criteria in one scheme. Such a mistake affects all typologies of research payments which, alongside recompenses and remunerations, distinguish “incentives” or “inducements” payments. This mistake originates from the fact that the former two categories of payments are defined by the payment impact on the subject baseline economic position, the latter is based on the researcher’s intention. Secondly, by rejecting the term”compensation”, the proposed typology avoids conceptual and normative confusion stemming from ambiguity of this notion in legal terminology, where it stands for both money received in return for services rendered, especially salaries or wages, and for payment of damages for loss or injury (Różyńska 2021 ). The ambiguity undermines the conceptual and normative value of highly popular payment scheme advocated by Gelinas, Largent, Lynch, and collaborators (Gelinas et al. 2018 ; Persad et al. 2019 ; Lynch et al. 2021 ; Bierer et al. 2021 ). Following Christine Grady’s terminology ( 2005 ), the authors separate “reimbursement”, “compensation”, and “incentive”. Trapped by the ambiguous language of “compensation”, they frame remuneration as recompense for losses, arguing that “participants' time, as well as their assumption of research-related burden and inconvenience … are critical contributions experienced as losses by participants; they are giving up or accepting unfavorable as a result of participation. Thus, the same justifications applicable to reimbursement applied here” (Lynch et al. 2021 , p. 16). At the same time, applying the logic of remuneration, they reject compensating participants based on their opportunity costs, noting that “rather, research compensation is an acknowledgement of participants’ contributions to research, which leads to the conclusion that there generally should be equal pay for equal work” (Lynch et al. 2021 , p. 16).
Finally, the advocated typology allows for separating payments form small gifts given at the conclusion of a study (such as chocolates, T-shirts, cups, pens, cinema-tickets) that have minimal market value, serve only as token of appreciation, and have likely zero impact on recruitment (Grady 2005 ).
Having clarified basic terminological and conceptual issues, we can move on and explore the ethical anatomy of payment for research participants. It would be useful, however, to indicate briefly—from the outset—the scope of the forthcoming analyses.
The paper deals with the widespread practice of paying research participants in exchange for their valuable service without determining whether the service should be treated as an unskilled labor, “regular” work, body renting, or a unique sui generis endeavor (cf. Lemmens and Elliott 1999 , 2001 ; King Reame 2001 ; Anderson and Weijer 2002 ; Elliott 2008 ; Abadie 2015 ; Phillips 2011b ; Lynch 2014 ; Różyńska 2018 ). For the sake of the analysis to come, it is assumed that paid participation in biomedical research is a form of paid bodily services, and it should be “no more worrisome to commodify a person’s labor [bodily service— JR ] as a research subject than to commodify a person’s labor in other contexts, which happens all the time” (Lynch 2014 , p. 159). Therefore, commodification concerns against research payment, raised by some commentators (Macklin 1989 ; Chambers 2001 ; Abadie 2010 , 2015 ; Cooper and Waldby 2014 ; Walker and Fisher 2019 ) will not be explored here.
Since the paper focuses on the most fundamental ethical basis of payment practice, it is limited to monetary offers (paid in cash, cheques or pre-paid credit cards) as a paradigmatic case of payment, leaving aside all forms of in-kind support. This paper also leaves aside payments to participants unable to give consent, because those raise additional, substantive issues, as well as recompenses for research-related injuries as they would require in-depth legal analysis. The presented analysis applies to research which RECs/IRBs would consider scientifically and ethically sound thus presenting arguments that payment is offered to overshadow or mask some ethical deficiencies of a study project, especially those regarding its risk–benefit profile or criteria of subjects selection. In other words, it considers “research which ethics committee would allow to proceed, were the subject not paid” (Wilkinson and Moore 1997 , p. 375).
Ethical rationale for paying research participants
The main “pragmatic” reason (Largent and Lynch 2017a , p. 77 fn. 22) for offering payment to research participants is to boost recruitment and retention rates. This “efficiency”-driven (Phillips 2011a ) rationale is widely acknowledged. It is, however, rarely recognized as having not only practical, but also an ethical facet. Since progress in biomedical science and healthcare is not ethically discretionary, this section argues that neither efficient enrolment nor payment for research subjects, being conductive to the latter, are value-neutral practices. On the contrary, they are ethically grounded in and governed by the principle of social beneficence that calls for maximization of a common good, i.e. good which has two characteristics: it is non-exhaustible (one person’s use does not diminish another’s use) and is beneficial for all or almost all members of a society. 2
Biomedical research is social practice aimed at generating such a common good—generalizable scientific knowledge that may contribute to the improvement of healthcare interventions and public health measures, thus leading to the advancement of human health (cf. Schaefer et al. 2009 ; Rhodes 2010 ; London et al. 2010 ). Health is highly valued by all individuals and societies primarily “because of what it enables us to do” (Duncan 2010 , p. 321). A minimum threshold of physical and mental capacities is necessary (though not sufficient) for an individual to be able to pursue her particular life goals, plans, and projects that express her vision of a good life. Since all liberal and democratic societies share a fundamental moral (and political) commitment to protecting and respecting each person’s right to lead her life in accordance with her personal views of what is valuable in human life, they also share a moral obligation to encourage forms of social collaboration useful in fulfilling basic health needs of their members (London 2003 , 2006 ; Rawls 1971 ; Nussbaum 2013 ). This includes the practice of healthcare as well as biomedical research, because the capacity of a society to satisfy its members’ basic health interests is conditional upon progress in biomedical sciences, which in turn is crucially dependent on various types of research, including human research. Thus, societies have a prima facie moral obligation to promote the conduct of biomedical research, including research involving human subjects.
Human biomedical research is a complex, collaborative social “enterprise”, involving various institutional and individual stakeholders with various, often conflicting, interests (London et al. 2010 ; Różyńska 2015 ). Therefore, in order to fulfill their obligation to promote biomedical research, societies should develop, implement and support normative and institutional mechanisms aimed ensuring the existence, stability and effectiveness of research practice in attaining the common good (London et al. 2010 ; Resnik 2011 ; Różyńska 2015 ). These mechanisms should enhance all stakeholders’ trust and willingness to support and invest in the research enterprise, including prospective research participants without whom biomedical research would not be able to achieve its goals.
Socially valuable human biomedical research is critically dependent on successful enrolment and retention of a sufficient number of appropriate participants, and on their willingness to comply with study procedures and conditions. Failure to recruit or retain participants may lead to invalid or inconclusive research data, it may result in premature termination of a study, increase research costs, and—what is the most important—delay or even hinder scientific progress and the anticipated development of safer and more effective diagnostic, prophylactic and therapeutic interventions (Altman 1980 ; Halpern et al. 2002 ; Gul and Ali 2010 ; Williams et al. 2015 ; Carlisle et al. 2015 ). Insufficient or slow recruitment and poor retention rates are, however, a common problem within biomedical research, especially in randomized controlled trials (Salman et al. 2014 ). A systematic review shows that less than half of trials achieve their original recruitment target goal in time (Campbell et al. 2007 ; cf. Sully Ben et al. 2013 ; Parkinson et al. 2019 ). Additionally, only very small portion of eligible adult oncological patients choose to enroll into trials—depending on a source the portion range from 3 to 5% or 8% (Bell and Balneaves 2015 ; American Cancer Society Cancer Action Network 2018 )—leading to a conclusion that “if the proportion of patients with cancer who agree to participate in clinical trials were to increase from its current 5% to 10%, the usual study completion rate would decrease from around 4 years to 1 year. Another estimate suggests that at least 16 million more individuals are needed to participate in research trials each year” (Schaefer et al. 2009 , p. 70).
Many different factors contribute to the low participation and retention rates. Although barriers of structural and clinical character, such as access to trials and eligibility requirements, seems to be the most important, concerns regarding financial costs and benefits linked to research participation also play a significant role in prospective subjects’ decision-making (Friedman et al. 2015 ; Hamel et al. 2016 ; American Cancer Society Cancer Action Network 2018 ; Nipp et al. 2019a , Unger et al. 2013 , 2019 ). Recent statistics published by the Center for Information and Study on Clinical Research Participation show that information about potential costs and their reimbursement as well as information about compensation for time off from work are among the most important factors influencing a decision to participate in research for—respectively—58% and 40% potential subjects ( 2019 ). And the prospect of receiving monetary compensation is one of three top reasons (34% mentioning) impacting a decision to enroll into a study (Center for Information and Study on Clinical Research Participation 2019 ). These data are consistent with results of numerous empirical studies on subjects’ motivations for volunteering, conducted among patients and healthy volunteers. They all indicate that although the payment is not the only reason why people agree to participate in biomedical research, it is definitely one of the top motivates for enrollment, especially among healthy volunteers (Tishler and Bartholomae 2002 ; Almeida et al. 2007 ; Abadie 2010 ; McCann et al. 2010 ; Stunkel and Grady 2011 ; Grady et al. 2017 ; Fisher et al. 2018 ; Manton et al. 2019 ).
Thus, although it is rightly stressed in the literature that the exact impact of paying for participation on the rates of research enrollment and retention remains still under-investigated, it is reasonable to assume that payment can make a positive difference in this respect (Dunn and Gordon 2005 ; Watson and Torgerson 2006 ; Caldwell et al. 2010 ; Probstfield and Frye 2011 ; Treweek et al. 2013 ; Nipp et al. 2019b ; Parkinson et al. 2019 ). Money may remove participation barriers for those individuals who are unable or unwilling to cover direct costs associated with research, such as costs of traveling, lodging or hiring a babysitter. The payment may attract people who would be otherwise discouraged from the participation by the necessity of taking unpaid leave from work and resulting loss of wages. It may help to make a positive enrollment decision for those, who feel forced by their low social-economic status (SES) to dedicate time and efforts for searching for a job or earning their living, instead of altruistically contributing to the development of science. Additionally, an offer of payment could convince to participation persons who believe that their private or professional time is particularly valuable (for example due to their unique responsibilities, competences, or skills), and, therefore, they should not allot it to alternative causes without an adequate remuneration. In all these cases, payment may enhance recruitment, provided it is designed in a way that targets the underlying barriers, needs, or expectations.
Insofar as these factual assumptions about inducing potential of money are true—and we concede them for the sake of the argument—the principle of social beneficence provides fundamental ethical rationale for paying research subjects. Offering payment to participants is an ethically right and prima facie obligatory practice because it increases research recruitment and retention, thereby contributing to the common good produced by the research enterprise. From a broader perspective, “it is useful in fulfilling society’s obligation to meet the essential [health-related] needs of its members” (Ackerman 1989 , p. 1). The strength of the obligation to offer payment for participation grows in relation to studies which are urgently needed, e.g., to address acute public-health emergencies (such as a dire pandemic), or when there is strong evidence that without payment recruitment, retention and completion of socially valuable studies would be doomed or severely compromised. The strength of the obligation weakens when—due to study-specific features—payment offers are not needed to secure an adequate number of participants, i.e., where it is reasonable to assume that people will be willing to join the study for non-economic and social reasons, e.g., the prospect of direct or ancillary medical benefits, the wish to make a contribution to medical progress or the health of others, scientific curiosity, interest in the goals of the study, the prospect of making friends or having new experiences (Stunkel and Grady 2011 ; Grady et al. 2017 ; Fisher et al. 2018 ; Manton et al. 2019 ). Additionally, the obligation to pay research participants may be overridden by justified research budget constraints. If investigators have no money to pay for participation in a socially valuable research project, it is better to allow them to proceed without payment, than obliging them to pay, thereby forcing them to resign from conducting the study (Gelinas et al. 2018 ).
To sum-up, the payment for research subjects is an incentive or inducement for participation grounded in the principle of social beneficence. This is expressive verbis acknowledged by the U.S. Food and Drug Administration ( 2018 ) guidance which argues that payment to research subjects is “a recruitment incentive” and by the European Union’s Clinical Trial Regulation ( 2014 ) which refers to any payment offered to trial participants as “incentives or financial inducements” (art. 31.1(d); art. 32.1(d), 33(d)). Thus, payment is not, as some scholars and guidelines suggest, a demand of justice or fairness (Gelinas et al. 2018 , 2020 ; Persad et al. 2019 ; Lynch et al. 2021 ) or requirement of non-maleficence and beneficence for an individual subject (Bierer et al. 2021 ). These principles, supplemented by the principle of respect for autonomy and other considerations regarding non-exploitation, are nevertheless very important. While they do not provide an ethical rationale for offering payment for research participations by themselves, they set contours for an ethically sound payment practice.
Ethical contours of an ethically sound payment practice
Offering payment for research participants is an incentive prima facie required by the principle of the social beneficence. This does not imply, however, that it is ethical to set payment at whatever level necessary and sufficient to attract an adequate number of proper subjects in a timely fashion. On the contrary, no matter how payment is important and effective as an incentive for participation, not every amount, method and timing of payment is acceptable. The reason being the fact that the consequentialistic principle of social beneficence does not exhaust the reining normative framework for human biomedical research. The ethics of biomedical research is built upon a matrix of principles and values which strives to find an adequate balance between the imperative to advance interests of science and society (“research imperative”) and obligations of all societies to protect other important interests of their members, especially interests of research participants and/or involved communities. These latter obligations may be viewed as constituting a general “imperative of non-exploitation” that sets boundaries for the practice of human biomedical research, in general, and for the practice of paying participant, in specific.
Autonomy and payment
One of the core values behind the imperative of non-exploitation in research is the value of human autonomy. The principle of respect for autonomy is a cornerstone of modern research ethics (National Commission for the Protection of Human Subjects of Biomedical and Behavioral Research 1979 ; Faden and Beauchamp 1986 ; Emanuel et al. 2000 ; Beauchamp and Childress 2001 ). It is also a bedrock of a fundamental ethical requirement for research, namely informed consent that serves to “ensure not only that individuals control whether or not they enroll in clinical studies”, but also that “they participate only when doing so is consistent with their values and interests” (Emanuel et al. 2000 , p. 2706).
The principle of respect for autonomy has a high relevance for the practice of payment for research subjects as one of the most commonly expressed concerns is that payment can be coercive or constitute an undue inducement (undue influence), thus compromising the validity of informed consent (e.g., Macklin 1981 ; Faden and Beauchamp 1986 ; McNeill 1997 ; Dickert and Grady 1999 , 2008 ; Largent et al. 2013 ; Gelinas et al. 2018 , 2020 ). It is claimed that very high or (for other reasons) overly attractive payment may undermine the capacity of individuals to make autonomous decisions regarding study participation, by compromising their voluntariness, ability to adequate understand and assess research risks and benefits, or by “forcing” them to make choices against their “better judgment or deeply held beliefs” (Council for International Organizations of Medical Sciences 2016 , p. 54).
Although there is still a substantial disagreement in the literature about whether an offer of payment may be perceived as coercion, what exactly constitutes undue inducement, and whether money can distort or compromise autonomous decision-making (Macklin 1981 , 1989 ; Wilkinson and Moore 1997 ; Grady 1999, 2001 , 2005 ; Grant and Sugarman 2004 ; Emanuel 2004 , 2005 ; Wertheimer and Miller 2008 ; Klitzman 2013 ; Largent et al. 2013 ; Resnik 2015 , 2019 ; Belfrage 2016 ; Largent and Lynch 2017a , 2017b ; Millum and Garnett 2019 ), existing guidelines and regulatory documents disallow payment, which may unduly seduce people to consent for participation (Largent and Lynch 2017a ). Thus, even though overly attractive payment offers could enhance timely recruitment by “alluring” a sufficient number of adequate individuals to join and stay in a study even against their better judgment, there is a regulatory consensus that such payment should not be offered.
The ethical unacceptability of such an overly attractive payment stems from the respect for autonomy. However, it also finds support in the principle of social beneficence. Common sense and empirical evidence, although still limited, suggest that attractive payments may have a negative impact on scientific value of research, as it may encourage potential and/or actual participants to withhold or misrepresent information, which are critical for their recruitment eligibility or continuation in research (Bentley and Thacker 2004 ; Dresser 2013 ; Dickert 2013 ; Devine et al. 2013 , 2015 ; Largent and Lynch 2017a ; McManus and Fisher 2018 ; Lynch et al. 2019 ) . Such a concealment, fabrication or falsification by participants create risks for participants, but also for research resources and the integrity of research data as it can bias the results and undermine the validity of a study (Lee et al. 2018 ). And—if it occurs frequently enough—it may jeopardize the whole research enterprise. Therefore, assuming—what stills needs to be explored empirically—a positive correlation between the prevalence of deceptive behaviors and the attractiveness of payment (which depends not only on the amount, but also on payment method and timing), the principle of social beneficence provides ethical reasons for employing payment strategies which do not involve overly attractive payment schemes.
The principle of respect for autonomy has fueled much of scholarly discussion on unethical nature of overly attractive payment. However, little attention has been paid to that principle in the context of no-payment and underpayment. Undoubtedly, the lack of resources to cover direct or indirect costs associated with the participation may constitute a barrier for individuals, who otherwise would be willing to take part in research (American Cancer Society Cancer Action Network 2018 ; Nipp et al. 2019a ; Largent and Lynch 2018 ; Bierer et al. 2021 ). The removal of these barriers by covering the relevant expenses is important for promoting potential subjects’ autonomy, because it enables individuals to exercise their free will to contribute to the development of science by serving as research subjects. Thus, the principle of respect for autonomy supports paying subjects recompenses, which make the participation in research a cost-free and revenue-neutral activity.
Finally, what is rarely observed, the principle of respect for autonomy provides a general support for public policies which allow remuneration of research subjects for their contributions—both in the form of reward and price—as it calls for respecting people’s right to decide freely in what practices and activates they what to engage in for the sake of earning their living. As Wilkinson and Moore note, “denying people the option of taking inducements reduces their freedom, since it removes an option that they prefer to the alternatives” (Wilkinson and Moore 1997 , p. 377). Admittedly, biomedical research enterprise does not aim at broadening the scope of subjects’ freedom or autonomy. Nevertheless, it should not restrict people’s choices without a good reason. Participation in socially valuable biomedical research, whether or not one is paid for it, is neither morally wrong nor bad for the person concerned. On the contrary, it is considered at least as good as engaging in any other socially valuable and risky service or work. Therefore, there are no ethical grounds (either paternalistic or non-paternalistic) for depriving competent individuals an opportunity to serve as research subjects in exchange for money, provided that their decision to participate in a given study is autonomous (i.e., based on comprehensive and adequately understood information, and free from unduly controlling influences).
Moreover, some studies suggest that remuneration can in fact play a positive role in prospective subjects’ consent process by reducing the therapeutic misconception and highlighting research risks. Money might send a message to participants that they take risks and burdens for the sake of the benefit of science and society and “should be compensated for it, which would not occur if they were … expected to benefit from it.” (Glannon 2006 , p. 252; also Dickert and Grady 1999 ; Grady 2001 , 2005 ; Menikoff 2001 ; Largent and Lynch 2017a ). Additionally, some studies suggest that payment can enhance autonomous decision-making by drawing prospective subjects’ attention to research risks and inconveniences (Cryder et al. 2010 ; Largent and Lynch 2017a ; Fisher et al. 2019 ).
Justice, fairness and payment
Another ethical principle that has a direct relevance for the practice of paying research subjects is the principle of justice, both in its distributive and commutative dimensions. Many scholars worry that payment may be more attractive to individuals of lower SES, and thus offering payment for participation may result in unfair distribution of research benefits and burdens across the general population (e.g., Maclin 1981 , 1989 ; Faden and Beauchamp 1986 ; Ackerman 1989 ; McNeill 1997 ; Grady 2005 ; Dickert and Grady 2008 ; Gelinas et al. 2018 ). The worse-off will shoulder a disproportionate share of burdens of research, while the benefits will accrue primarily to the better-off. A different, yet linked, concern relates to the risk of exploitation of research participants, especially those of low SES (Lemmens and Elliott 1999 ; Shamoo and Resnik 2006 ; Elliott 2008 ; Elliott and Abadie 2008 ; Abadie 2010 , 2015 ; Stones and McMillan 2010 ; Phillips 2011a ; Resnik 2015 , 2019 ; Largent and Lynch 2017b ; Gelinas et al. 2020 ; Bierer et al. 2021 ; MacKay and Walker 2021 ). Exploitation in research is about the unfair distribution of goods that arise from interaction between researchers and participants (Wertheimer 2008 ). “One party gets too little, while the other gets too much. Often, but not always, the unfair distribution arises because one party to the interaction is in a weak position, due to poverty, ignorance, or extreme urgency, which the other party can take advantage of, offering few benefits” (Emanuel 2004 , p. 101). Economically disadvantaged individuals are in need of money and they tend to value a specific amount of payment more. For many of them accepting an offer of unfairly low but still en bloc beneficial payment for participation might be a reasonable choice. After all, even small payment is better than no payment at all, although it constitutes a (mutually beneficial and consensual) exploitation.
These concerns are highly important, but—as it is shown below—they do not exhaust the role of justice/fairness in shaping an ethically sound payment practice. Moreover, in order to fully understand their consequences for ethics of paying research subjects, it is essential to note two things.
Firstly, biomedical research is a social practice embedded in specific social reality shaped by its historical and cultural roots, reigning power- and economic relations, and normative fabrics. This social reality is marked by profound social and economic inequities (within concrete societies as well as at the level of international community), which should be mitigated, and ideally eliminated by broad social reforms. Research enterprise should not entrench or exacerbate these background social injustices. However, it is neither designed for nor capable of actively fighting them, especially against participants’ poverty, unemployment or lack of access to high-quality healthcare (Fisher 2019 ). Justice in research requires treating all research participants fairly and equitably, also when it comes to payment for their participation. But it does not require paying participants of low SES in order to alleviate or remove hardships of their position. Such a positive impact of research payment on subjects’ social or economic condition is laudable, and even desirable, but it is not a demand of justice.
Moreover, contrary to views of some commentators, the commutative justice per se does not require offering payments to research subjects. Largent, Emanuel and Lynch claim that “when goods and services are not indented as gifts, failure to pay for them is a problem: we call it theft” ( 2019 , p. 1), thus suggesting that this is exactly what happens when participants are not fairly paid for their contribution to the common good. Despite its rhetorical attractiveness, this claim cannot be accepted as it rests on two mistakes. Firstly, it ignores the power of consent. Valid consent of a prospective subject for using her body for research purposes transforms theft into gift, lease, rent, work or other consensual relation with a researcher. Second, it forgets that it takes two willing parties to change provision of goods or services into transaction, i.e., exchange of goods and services in return for money. Thus, when a researcher is unable (e.g., due to budgetary constraints) or uninterested in offering payment for prospective participants (e.g., due to availability of sufficient number of unpaid subjects), or when a prospective participant is genuinely willing to contribute to the development of science without any remuneration and reimbursement, there is no ground for transaction. And there is nothing essentially unfair in allowing researchers and altruistically motivated participants to engage in scientifically and socially valuable biomedical research.
Payment is a recruitment incentive justified and prima facie required by social beneficence, not by justice or fairness. However, when it is to be offered , the offer should satisfy the requirement of distributive and commutative justice.
It is commonly accepted that the principle of justice requires distributing burdens and benefits of study participation in such a way that no segment of the population is unduly burdened by research or denied its potential or actual benefits. Recruitment criteria should reflect the scientific purpose of the study, not target populations which are considered “easy to recruit” “simply because of their easy availability, their compromised position, or their manipulability” (National Commission for the Protection of Human Subjects of Biomedical and Behavioral Research. 1979 ). Thus, researchers must neither exploit the vulnerable, in particular economically disadvantaged, nor exclude without good reason those who stand to benefit from study participation. They should strive to recruit an adequate cross-section of the population in order to spread research burdens and benefits fairly across the population. In other words, an adequate diversity of gender, race, ethnicity, age and SES in research should be sought (Geller et al. 2011 ; Kwiatkowski et al. 2013 ; Heller et al. 2014 ; Winter et al. 2018 ; cf. Dickert 2009 ; Bierer et al. 2021 ). It is worth noting that the last postulate finds an additional support in the principle of social beneficence as it enables the generalization of knowledge to be gained in research, thereby enhancing research scientific and social value (Wilkinson and Moore 1997 ; Grady 2005 ; Resnik 2015 ; Largent and Lynch 2017b ). Thus, justice encourages payment schemes which have a potential of making distribution of research burdens fairer. And justice is against schemes that can deepen social inequalities, lead to unfair social distribution of research burdens and benefits or exploitation.
What should research subjects be paid for and in which amount to make payment consistent with demands of justice? Firstly, justice-related considerations provide an ethical justification for paying research subjects a recompense for direct costs related to research participation. By making participation in research a cost-free activity, recompense removes—at least some—economic barriers for participation, thus equalizing opportunities for all willing individuals to contribute to the development of science, no matter their SES. Moreover, as studies already referred to suggest (Unger et al. 2013 , 2016 , 2019 ; American Cancer Society Cancer Action Network 2018 ; Nipp et al. 2019b ; Chino and Zafar 2019 ), reimbursement of direct expenses may improve access to potentially beneficial studies for patients, who otherwise would not be able to participate due to their low SES or disease-caused greater sensitivity to economic burdens associated with research. Thus, the reimbursement of direct costs has a potential to enhance justice in research not only by reducing inequities in access to research for those who otherwise could not afford it, but also by contributing to the fair distribution of clinical benefits associated with participation.
Secondly, the principle of justice provides a strong ethical reason for disallowing recompenses for the loss of reasonably expected profits, especially lost wages. Such recompenses lead to differential payments between participants—individuals who receive higher wages get higher recompense; those who are lower paid by employees—lower recompense, and those who are unemployed—no recompense at all. This is consistent with a norm of equity, as it makes participation a revenue-neutral activity for all, no matter how much they earn. Nevertheless, it is very likely to reinforce unfair distribution of research risks and benefits between different social strata by prompting researchers to make savings by drawing research participants from “cheaper” populations, especially from the unemployed and the low-paid (Dickert and Grady 1999 ; Resnik 2015 ).
Thirdly, justice supports remuneration for participants’ contributions to the development of science and society, provided it is fair, i.e., equitable, adequate, and non-exploitive. Remuneration is equitable when it does not violate the norm “equal pay for equal work” (Dickert and Grady 1999 ; Resnik 2015 ; Gelinas et al. 2018 , 2020 ; Persad et al. 2019 ). Equal time, efforts, burdens and risks associated with participation deserve equal remuneration (measured in market value, rather than in nominal value in case of multi-site studies conducted in different settings). However, when different groups of participants in a study are expected to make different contributions, equitable remuneration should reflect differences in their input (Persad et al. 2019 ). This is consistent with equal respect and concern for each and every individual and it prevents discrimination.
Remuneration is adequate when it is proportionate in value to the value of participants’ contribution to the study. The latter necessarily depends on various factors which determine how time- and effort-consuming, burdensome and risky give research project is. For example, when a study involves 2 visits, each lasting 60-min and requires filling a questionnaire and giving a blood sample for further analysis, and another project involves 4 visits of the same length and level of associated risks and burdens, participants of the latter study— ceteris paribus —should be offered remuneration of double the remuneration offered to the participant of the first project.
All guidelines and regulatory documents referred to above follow this normative logic by recommending or permitting paying research participants an “appropriate” or “proportionate” or “just and fair” remuneration for the time spent and other inconveniences resulting from the study participation (Council of Europe 2005b , sec. 64; Health Research Authority 2014 , par. 3.4; Council for International Organizations of Medical Sciences 2016 , p. 53; Food and Drug Administration 2018 ; National Health and Medical Research Council 2019 , par. 1.1). Only a few, however, mention the level of research risks among factors which should be taken into consideration when determining an adequate amount of payment. Moreover, those that do so provide conflicting instructions on this matter. For example, the CIOMS Guidelines expressis verbis state that “the level of compensation should not be related to the level of risk that participants agree to undertake” ( 2016 , Commentary on Guideline 13). In contract, the Australian National Health and Medical Research Council’s guideline reads: “In cases where risk may be considered as a factor in determining payment, payment of participants based on the degree of risk associated with the research is not prohibited, so long as there is evidence that a participant’s ability to provide valid consent is not likely to be compromised” ( 2019 , par. 1.3.). Also the UK Health Research Authority “sympathises with the view that not to allow payments on the basis of risk would be unduly paternalistic in the absence of evidence that the participants’ ability to provide valid consent would be compromised” ( 2014 , par. 3.1.).
These regulatory variations mirror the lack of consensus regarding risk-based payments among research ethicists (Grady 2001 ; Menikoff 2001 ; Jones and Liddell 2009 ; Saunders 2009 ; Grimwade et al. 2020 ; Lynch and Largent 2020 ; Jamrozik and Selgelid 2020 ). Although a detailed analysis of this issue goes beyond the scope of this analysis, three following arguments provide strong reasons for arguing that adequate remuneration for research subjects should be proportionate also to the level of risk involved in participation: (i) an argument from consistency, which notices that risk-based remuneration is accepted in many non-research contexts, e.g., in high-risk professions (Menikoff 2001 ; Jones and Liddell 2009 ); (ii) an argument from the nature of human “guinea pigging” which claims that the assumption of risk is often an essential contribution of research participants (Menikoff 2001 ; Różyńska 2018 ; Malmqvist 2019 ); (iii) an argument for public trust, which argues that paying proportionally to the incurred risk meets expectations regarding the fair treatment of a significant portion of prospective subjects as well as researchers, REC/IRB members, and other members of the research community (Czarny et al. 2010 ; Ripley et al. 2010 ; Largent et al. 2012 ; Grimwade et al. 2020 ), thereby enhances public trust in research at large.
Finally, remuneration is non-exploitive when it is not lower than a socially accepted payment which is (or would be) offered for a similarly time- and effort-consuming, burdensome and risky activity, outside research context in the same setting (Gelinas et al. 2018 ; Largent and Lynch 2017b ). Although space does not permit discussing in details how a proper amount of such defined payment should be calculated, a proposal widely advocated in the literature should be mentioned here, namely a minimum hourly wage benchmark. Numerous authors and guidelines suggest that the amount should be based on the minimum hourly wage in the region or country as a point of reference (Council for International Organizations of Medical Sciences 2016 , Commentary to Guideline 15, 53; National Health and Medical Research Council 2019 , Appendix 1, 7) with augmentations for particularly burdensome procedures (Ackerman 1989 ; Dickert and Grady 1999 ; Grady 2005 ; Gelinas et al. 2020 ), risks involved (Menikoff 2001 ), and even other additional benefits (cf. Anderson and Weijer 2002 ). This proposal has three advantages. It is relatively easy to implement as it provides a clear method of setting the baseline amount of reimbursement for research participants. It allows for keeping payments sensitive to specific features of the project and subjects’ contributions by accepting relevant payment augmentations. And—since it sets the reference value of an non-exploitive remuneration relatively low—“more researchers could afford to pay a fair wage and fewer would be inconvenienced by a prohibition on unfairly low wages” (Phillips 2011a , p. 219). However, it also faces challenges. Offers of payment calculated in such a way would be attractive for prospective subjects with low SES, who have no reasonable alternatives to get engaged in better paid activities. But these offers would likely have no impact on recruitment of individuals who are better-off, i.e., who have better paid jobs or capacity to make more money outside research context, thus potentially biasing the subjects’ recruitment. In contrast, the average-wage benchmark for non-exploitive remuneration, mentioned by some authors (Phillips 2011a ) would seem fairer as it would make payment offers reasonably attractive to both individuals of SES and to those better-off, thus promoting a fair distribution of research risks and benefits between different social strata.
Fourthly, the principle of justice does not prohibit remunerations going beyond what constitutes equitable, adequate, and minimally non-exploitive payment—i.e., remunerations driven by a market-driven forces. Although such payments are conceptualized as “price” for desired services, in order to avoid exploitation, they should not be lower than remuneration viewed as “reward”. They may, however, be higher than “rewards” and they may vary within the same study on the basis of salient characteristics of particular groups of participants (i.e., their age, sex, race, ethnicity, rare clinical status etc.). Offering disproportionally high and/or differential remuneration does not violate requirements of justice insofar as the payment is designed to help the study to meet its social and scientific goals by enhancing recruitment and retention of the necessary category of subjects, and it does not reproduce or reinforce wider social inequities and injustices, e.g., racial biases or class differences (Persad et al. 2019 ).
Individual beneficence and payment
The principle of individual beneficence is rarely invoked in the discussion on ethical payment practices. Most probably, it is because research is about advancing the interests of science and society, not the interests of individual participants (Emanuel et al. 2000 ; Miller and Brody 2003 ).While there are studies which have a potential of direct therapeutic benefits for the participants, it is neither the goal of research practice, nor a requirement that research should be beneficial for participants. Moreover, all canonical guidelines on human research ethics exclude non-direct and non-medical benefits to research subjects, such as payment, from the risk–benefit analysis, thereby forbidding IRBs/RECs to take payment into account as a benefit to counterbalance research risks (Emanuel et al. 2010; contra Wertheimer 2013 ).
Nevertheless, despite the above normative premises, in fact, individuals treat money as a benefit when considering an offer to participate, and deciding on entering the study (Abadie 2010 ; Czarny et al., 2010 ; Stunkel and Grady 2011 ; Grady et al. 2017 ; Fisher et al. 2018 ; Manton et al. 2019 ). For an individual prospective participant, the payment is a part of an equation for the overall attractiveness of a research project. Recompenses, when full and adequate, make the research participation cost-free for subjects, and—as shown above—they can remove at least some entrance barriers for patients to potentially clinically beneficial studies. Remunerations—whether calculated as a reward or as a price—may constitute a gain for subjects, thereby making participation in research overall beneficial from their personal perspective.
Thus, the principle of individual beneficence provides additional support for offering money to research subjects. However, the same principle justifies the claim that payment for participation should not be overly attractive. There is some evidence that too attractive remuneration may increase the risk of jeopardizing subjects’ health by encouraging them to conceal or misrepresent information important for their safety in order to ensure recruitment or continued participation in paid research (Bentley and Thacker 2004 ; Devine et al. 2013 , 2015 ; Lee et al. 2018 ; Lynch et al. 2019 ). Such a deceptive behavior by participants may take various forms (e.g., nondisclosure of concurrent enrollment in other studies, concealment of tobacco use, alcohol consumption, or illicit substance abuse, concealment of pre-existing medical conditions, falsification of current health status, over-reporting of a study protocol adherence, etc.), and it may result in severe adverse events or even subjects’ death (Lee et al. 2018 ).
Conclusions
The paper argues that the ethical anatomy of paying research participants is built upon four basic principles of research ethics (and bioethics in general). The ethical “spine” of the practice is the principle of social beneficence, which requires the maximization of the common good—in the case of research practice—socially valuable scientific knowledge. This principle grounds a general prima facie moral obligation of offering payment to research subjects. The remaining ethical principles constitute a “skeleton” of morally sound payment practices by providing additional moral reasons for offering or not offering certain types of payments to research participants. As discussed above and presented in Table Table1, 1 , the principles argue for offering research participants:
- recompense for reasonable expenses, but not for lost wages (or loss of other reasonably expected profits);
- remuneration conceptualized as a reward for their valuable contribution, provided (i) the remuneration meets standards of equality, adequacy and non-exploitation, and (ii) it is not overly attractive, i.e., it does not constitute undue inducement for participation or retention, and does not encourage deceptive behaviors;
- remuneration conceptualized as a market-driven price, provided (i) the remuneration is necessary and designed to help the study achieve its social and scientific goals, (ii) it does not reinforce wider social injustices and inequalities; (iii) it meets the requirement of non-exploitation; (iv) it is not overly attractive (as defined above).

Obviously, a proper application of this ethical “skeleton” into research practice requires investigators and RECs/IRBs to take into account “the nature of the study, the nature of participants contributions and vulnerabilities, institutional and organizational guidelines, and local and cultural norms” (Grady 2005 , p. 1686). Moreover, to make the proposed scheme fully helpful in determining whether any particular offer of payment is not overtly attractive and whether it meets standards of not equality, adequacy and non-exploitation, further detailed analyses of these standards are needed. The proposed scheme should also be tested against and enriched by further empirical studies about payment, especially about how money impacts subjects’ decision-making processes and behaviors.
This analysis was supported by a grant of the National Science Centre, Poland, No. 2015/17/B/HS1/02390.
Data availability
Code availability, declarations.
I have no conflict of interests or competing interests to disclose.
1 This is an improved version of the payment typology presented in Różyńska ( 2021 ).
2 Developing an appropriate understanding of a common good, also referred to as a public, social or collective good, is a challenge that cannot be addressed here. For the sake of this analysis, I use all these terms interchangeably, as is frequently done in the literature. My understanding of a common good is based on the conceptual analysis provided by Hans Radder ( 2017 ). It is also inspired by Alex London’s idea of “the generic interests conception of the common good” by assuming that a social practice or policy is socially valuable when it overall promotes “a subset of interests that are sufficiently basic or fundamental to individuals that they are common to each of the members of the community… interests in being able to develop their intellectual and affective capacities in order to pursue activities that they find meaningful, and to engage in meaningful relationships with others” ( 2003 , p. 21).
Publisher's Note
Springer Nature remains neutral with regard to jurisdictional claims in published maps and institutional affiliations.
- Abadie Roberto. The professional guinea pig: Big pharma and the risky world of human subjects. Durham: Duke University Press; 2010. [ Google Scholar ]
- Abadie Roberto. The “mild-torture economy”: exploring the world of professional research subjects and its ethical implications. Physis: Revista De Saúde Coletiva. 2015; 25 :709–728. doi: 10.1590/S0103-73312015000300003. [ CrossRef ] [ Google Scholar ]
- Ackerman Terrence F. An ethical framework for the practice of paying research subjects. IRB: Ethics & Human Research. 1989; 11 (4):1–4. doi: 10.2307/3564170. [ PubMed ] [ CrossRef ] [ Google Scholar ]
- Almeida Luis, Azevedo Benedita, Nunes Teresa, Vaz-da-Silva Manuel, Soares-da-Silva Patricio. Why healthy subjects volunteer for phase I studies and how they perceive their participation? European Journal of Clinical Pharmacology. 2007; 63 (11):1085–1094. doi: 10.1007/s00228-007-0368-3. [ PubMed ] [ CrossRef ] [ Google Scholar ]
- Altman Douglas G. Statistics and ethics in medical research: III How large a sample? British Medical Journal. 1980; 281 (6251):1336–1338. doi: 10.1136/bmj.281.6251.1336. [ PMC free article ] [ PubMed ] [ CrossRef ] [ Google Scholar ]
- American Cancer Society Cancer Action Network. 2018. Barriers to patient enrollment in therapeutic clinical trials for cancer: a landscape report. https://www.acscan.org/policy-resources/clinical-trial-barriers . Accessed 28 August 2021.
- Anderson James A, Weijer Charles. The research subject as wage earner. Theoretical Medicine and Bioethics. 2002; 23 (4):359–376. doi: 10.1023/A:1021265824313. [ PubMed ] [ CrossRef ] [ Google Scholar ]
- Beauchamp Tom L, Childress James F. The principles of biomedical ethics. 5. Oxford: Oxford University Press; 2001. [ Google Scholar ]
- Belfrage Sara. Exploitative, irresistible, and coercive offers: Why research participants should be paid well or not at all. Journal of Global Ethics. 2016; 12 (1):69–86. doi: 10.1080/17449626.2016.1150318. [ CrossRef ] [ Google Scholar ]
- Bell Jennifer A.H., Balneaves Lynda G. Cancer patient decision making related to clinical trial participation: An integrative review with implications for patients’ relational autonomy. Supportive Care in Cancer. 2015; 23 (4):1169–1196. doi: 10.1007/s00520-014-2581-9. [ PubMed ] [ CrossRef ] [ Google Scholar ]
- Bentley John P, Thacker Paul G. The influence of risk and monetary payment on the research participation decision making process. Journal of Medical Ethics. 2004; 30 (3):293–298. doi: 10.1136/jme.2002.001594. [ PMC free article ] [ PubMed ] [ CrossRef ] [ Google Scholar ]
- Bierer Barbara E, White Sarah A, Gelinas Luke, Strauss David H. Fair payment and just benefits to enhance diversity in clinical research. Journal of Clinical and Translational Science. 2021; 5 (1):e159. doi: 10.1017/cts.2021.816. [ PMC free article ] [ PubMed ] [ CrossRef ] [ Google Scholar ]
- Caldwell Patrina H, Hamilton Sana, Tan Alvin, Craig Jonathan C. Strategies for increasing recruitment to randomised controlled trials: Systematic review. PLoS Medicine. 2010; 7 (11):e1000368. doi: 10.1371/journal.pmed.1000368. [ PMC free article ] [ PubMed ] [ CrossRef ] [ Google Scholar ]
- Campbell, Marion K., Claire Snowdon, David Francis, Diana R. Elbourne, Alison M. McDonald, Resemary C. Knight, Vikki Entwistle, Jo Garcia, Ian Roberts, and STEPS Group. 2007. Recruitment to randomised trials: Strategies for trial enrolment and participation study. The STEPS study. Health Technology Assessment . https://www.journalslibrary.nihr.ac.uk/hta/hta11480/#/full-report . Accessed 28 Aug 2021. [ PubMed ]
- Carlisle Benjamin, Kimmelman Jonathan, Ramsay Tim, MacKinnon Nathalie. Unsuccessful trial accrual and human subjects protections: An empirical analysis of recently closed trials. Clinical Trials. 2015; 12 (1):77–83. doi: 10.1177/1740774514558307. [ PMC free article ] [ PubMed ] [ CrossRef ] [ Google Scholar ]
- Center for Information and Study on Clinical Research Participation. 2019. Charts and statistics. https://www.ciscrp.org/education-center/charts-statistics/ . Accessed 28 Aug 2021.
- Chambers Tod. Participation as commodity, participation as gift. American Journal of Bioethics. 2001; 1 (2):48–48. doi: 10.1162/152651601300169077. [ PubMed ] [ CrossRef ] [ Google Scholar ]
- Chino Fumiko, Zafar S Yousuf. Financial toxicity and equitable access to clinical trials. American Society of Clinical Oncology Educational Book. 2019; 39 :11–18. doi: 10.1200/EDBK_100019. [ PubMed ] [ CrossRef ] [ Google Scholar ]
- Cooper Melinda, Waldby Catherine. Clinical labor: tissue donors and research subjects in the global bioeconomy. Durham: Duke University Press; 2014. [ Google Scholar ]
- Council for International Organizations of Medical Sciences. 2016. International ethical guidelines for health-related research involving humans . Geneva: Council for International Organizations of Medical Sciences. https://cioms.ch/wp-content/uploads/2017/01/WEB-CIOMS-EthicalGuidelines.pdf . Accessed 28 August 2021.
- Council of Europe. 2005a. Additional Protocol to Convention on human rights and biomedicine concerning biomedical research . CETS 195. Strasbourg: Council of Europe. https://rm.coe.int/168008371a . Accessed 28 Aug 2021.
- Council of Europe. 2005b. Explanatory report to Additional Protocol to Convention on human rights and biomedicine concerning biomedical research . Strasbourg: Council of Europe. https://rm.coe.int/16800d3810 . Accessed 28 Aug 2021.
- Cryder Cynthia E, London Alex J, Volpp Kevin G, Loewenstein George. Informative inducement: Study payment as a signal of risk. Social Science & Medicine. 2010; 70 (3):455–464. doi: 10.1016/j.socscimed.2009.10.047. [ PubMed ] [ CrossRef ] [ Google Scholar ]
- Czarny Matthew J, Kass Nancy E, Flexner Charles W, Carson Kathryn A, Myers Rachel K, Fuchs Ephraim J. Payment to healthy volunteers in clinical research: The research subject's perspective. Clinical Pharmacology & Therapeutics. 2010; 87 (3):286–293. doi: 10.1038/clpt.2009.222. [ PMC free article ] [ PubMed ] [ CrossRef ] [ Google Scholar ]
- Department of Health and Human Services. 2018. U.S. Code of Federal Regulations, Title 45: Public Welfare, Part 46: Protection of human subjects. https://www.hhs.gov/ohrp/regulations-and-policy/regulations/45-cfr-46/revised-common-rule-regulatory-text/index.html . Accessed 28 Aug 2021. [ PubMed ]
- Devine Eric G, Waters Megan E, Putnam Megan, Surprise Caitlin, O’Malley Katie, Richambault Courtney, Fishmana Rachel L, Knappa Clifford M, Pattersona Elissa H, Sarid-Segala Ofra, Streetera Chris, Colanaria Laurie, Ciraulo Domenic A. Concealment and fabrication by experienced research subjects. Clinical Trials. 2013; 10 (6):935–948. doi: 10.1177/1740774513492917. [ PubMed ] [ CrossRef ] [ Google Scholar ]
- Devine Eric G, Knapp Clifford M, Sarid-Segal Ofra, O'Keefe Sean M, Wardell Cale, Baskett Morgan, Pecchia Ashley, Ferrell Katie, Ciraulo Domenic A. Payment expectations for research participation among subjects who tell the truth, subjects who conceal information, and subjects who fabricate information. Contemporary Clinical Trials. 2015; 41 :55–61. doi: 10.1016/j.cct.2014.12.004. [ PMC free article ] [ PubMed ] [ CrossRef ] [ Google Scholar ]
- Dickert Neal W. Enrollment of economically disadvantaged participants in clinical research. AMA Journal of Ethics. 2009; 11 (1):54–60. doi: 10.1001/virtualmentor.2009.11.1.pfor1-0901. [ PubMed ] [ CrossRef ] [ Google Scholar ]
- Dickert Neal W. Concealment and fabrication: The hidden price of payment for research participation? Clinical Trials. 2013; 10 (6):840–841. doi: 10.1177/1740774513506619. [ PubMed ] [ CrossRef ] [ Google Scholar ]
- Dickert Neal, Emanuel Ezekiel, Grady Christine. Paying research subjects: An analysis of current policies. Annals of Internal Medicine. 2002; 136 (5):368–373. doi: 10.7326/0003-4819-136-5-200203050-00009. [ PubMed ] [ CrossRef ] [ Google Scholar ]
- Dickert Neal, Grady Christine. What's the price of a research subject? Approaches to payment for research participation. The New England Journal of Medicine. 1999; 341 :198–203. doi: 10.1056/NEJM199907153410312. [ PubMed ] [ CrossRef ] [ Google Scholar ]
- Dickert Neal, Grady Christine. Incentives for participants. In: Emanuel Ezekiel J, Crouch Robert A, Grady Christine C, Lie Reidar K, Miller Franklin G, Wendler David D., editors. The Oxford textbook on the ethics of clinical research. New York: Oxford University Press; 2008. pp. 386–396. [ Google Scholar ]
- Dresser Rebecca. Subversive subjects: Rule-breaking and deception in clinical trials. The Journal of Law, Medicine & Ethics. 2013; 41 (4):829–840. doi: 10.1111/jlme.12093. [ PMC free article ] [ PubMed ] [ CrossRef ] [ Google Scholar ]
- Duncan Peter. Health, health care and the problem of intrinsic value. Journal of Evaluation in Clinical Practice. 2010; 16 (2):318–322. doi: 10.1111/j.1365-2753.2010.01392.x. [ PubMed ] [ CrossRef ] [ Google Scholar ]
- Dunn Laura B, Gordon Nora E. Improving informed consent and enhancing recruitment for research by understanding economic behavior. JAMA. 2005; 293 (5):609–612. doi: 10.1001/jama.293.5.609. [ PubMed ] [ CrossRef ] [ Google Scholar ]
- Elliott, Carl. 2008. Guinea-pigging. The New Yorker , January 7, pp. 36−41. [ PubMed ]
- Elliott Carl, Abadie Roberto. Exploiting a research underclass in phase 1 clinical trials. The New England Journal of Medicine. 2008; 358 (22):2316–2317. doi: 10.1056/NEJMp0801872. [ PubMed ] [ CrossRef ] [ Google Scholar ]
- Emanuel Ezekiel J. Ending concerns about undue inducement. Journal of Law, Medicine & Ethics. 2004; 32 (1):100–105. doi: 10.1111/j.1748-720X.2004.tb00453.x. [ PubMed ] [ CrossRef ] [ Google Scholar ]
- Emanuel Ezekiel J. Undue inducement: Nonsense on stilts? The American Journal of Bioethics. 2005; 5 (5):9–13. doi: 10.1080/15265160500244959. [ PubMed ] [ CrossRef ] [ Google Scholar ]
- Emanuel Ezekiel J, Wendler David, Grady Christine. What makes clinical research ethical? JAMA. 2000; 283 (20):2701–2711. doi: 10.1001/jama.283.20.2701. [ PubMed ] [ CrossRef ] [ Google Scholar ]
- European Union. 2014. Regulations (EU) No 536/2014 of the European Parliament and of the Council of 16 April 2014 on clinical trials on medicinal products for human use, and repealing Directive 2001/20/EC. https://ec.europa.eu/health/sites/default/files/files/eudralex/vol-1/reg_2014_536/reg_2014_536_en.pdf . Accessed 28 Aug 2021.
- Faden Ruth, Beauchamp Tom. A history and theory of informed consent. New York: Oxford University Press; 1986. [ Google Scholar ]
- Fisher Jill A. Research payment and its social justice concerns. The American Journal of Bioethics. 2019; 19 (9):35–36. doi: 10.1080/15265161.2019.1630505. [ PubMed ] [ CrossRef ] [ Google Scholar ]
- Fisher Jill A, McManus Lisa, Wood Megan M, Cottingham Marci D, Kalbaugh Julianne M, Monahan Torin, Walker Rebecca L. Healthy volunteers’ perceptions of the benefits of their participation in phase I clinical trials. Journal of Empirical Research on Human Research Ethics. 2018; 13 (5):494–510. doi: 10.1177/1556264618804962. [ PMC free article ] [ PubMed ] [ CrossRef ] [ Google Scholar ]
- Fisher Jill A, Monahan Torin, Walker Rebecca L. Picking and choosing among phase I trials. Journal of Bioethical Inquiry. 2019; 16 (4):535–549. doi: 10.1007/s11673-019-09946-w. [ PMC free article ] [ PubMed ] [ CrossRef ] [ Google Scholar ]
- Food and Drug Administration. 2018. Payment and reimbursement to research subjects. Guidance for institutional review boards and clinical investigators. U.S. Food and Drug Administration: Office of the Commissioner, Office of Clinical Policy and Programs, Office of Clinical Policy, Office of Good Clinical Practice. https://www.fda.gov/regulatory-information/search-fda-guidance-documents/payment-and-reimbursement-research-subjects . Accessed 28 Aug 2021.
- Friedman Daniela B, Foster Caroline, Bergeron Caroline D, Tanner Andrea, Kim Sei-Hill. A qualitative study of recruitment barriers, motivators, and community-based strategies for increasing clinical trials participation among rural and urban populations. American Journal of Health Promotion. 2015; 29 (5):332–338. doi: 10.4278/ajhp.130514-QUAL-247. [ PubMed ] [ CrossRef ] [ Google Scholar ]
- Fry Craig L, Ritter Alison, Baldwin Simon, Bowen Kathryn J, Paul Gardiner T, Holt Rebecca Jenkinson, Johnston Jennifer. Paying research participants: A study of current practices in Australia. Journal of Medical Ethics. 2005; 31 (9):542–547. doi: 10.1136/jme.2004.009290. [ PMC free article ] [ PubMed ] [ CrossRef ] [ Google Scholar ]
- Gelinas Luke, Largent Emily A, Glenn Cohen I, Kornetsky Susan, Bierer Barbara E, Lynch Holly Fernandez. A framework for ethical payment to research participants. The New England Journal of Medicine. 2018; 378 (8):766–771. doi: 10.1056/NEJMsb1710591. [ PubMed ] [ CrossRef ] [ Google Scholar ]
- Gelinas Luke, White Sarah A, Bierer Barbara E. Economic vulnerability and payment for research participation. Clinical Trials. 2020; 17 (3):264–272. doi: 10.1177/1740774520905596. [ PubMed ] [ CrossRef ] [ Google Scholar ]
- Geller Stacie E, Koch Abby, Pellettieri Beth, Carnes Molly. Inclusion, analysis, and reporting of sex and race/ethnicity in clinical trials: Have we made progress? Journal of Women's Health. 2011; 20 (3):315–320. doi: 10.1089/jwh.2010.2469. [ PMC free article ] [ PubMed ] [ CrossRef ] [ Google Scholar ]
- Glannon William. Phase I oncology trials: Why the therapeutic misconception will not go away. Journal of Medical Ethics. 2006; 32 :252–255. doi: 10.1136/jme.2005.015685. [ PMC free article ] [ PubMed ] [ CrossRef ] [ Google Scholar ]
- Grady Christine. Money for research participation: Does it jeopardize informed consent? The American Journal of Bioethics. 2001; 1 (2):40–44. doi: 10.1162/152651601300169031. [ PubMed ] [ CrossRef ] [ Google Scholar ]
- Grady Christine. Payment of clinical research subjects. The Journal of Clinical Investigation. 2005; 115 (7):1681–1687. doi: 10.1172/JCI25694. [ PMC free article ] [ PubMed ] [ CrossRef ] [ Google Scholar ]
- Grady Christine, Bedarid Gabriella, Sinaii Ninet, Gregorio Mark A, Emanuel Ezekiel J. Motivations, enrollment decisions, and socio-demographic characteristics of healthy volunteers in phase 1 research. Clinical Trials. 2017; 14 (5):526–536. doi: 10.1177/1740774517722130. [ PMC free article ] [ PubMed ] [ CrossRef ] [ Google Scholar ]
- Grady Christine, Dickert Neal, Jawetz Tom, Gensler Gary, Emanuel Ezekiel J. An analysis of US practices of paying research participants. Contemporary Clinical Trials. 2005; 26 (3):365–375. doi: 10.1016/j.cct.2005.02.003. [ PubMed ] [ CrossRef ] [ Google Scholar ]
- Grant Ruth W, Sugarman Jeremy. Ethics in human subjects research: Do incentives matter? The Journal of Medicine and Philosophy. 2004; 29 (6):717–738. doi: 10.1080/03605310490883046. [ PubMed ] [ CrossRef ] [ Google Scholar ]
- Grimwade Olivia, Savulescu Julian, Giubilini Alberto, Oakley Justin, Osowicki Joshua, Pollard Andrew J, Nussberger Anne-Marie. Payment in challenge studies: Ethics, attitudes and a new payment for risk model. Journal of Medical Ethics. 2020; 46 (12):815–826. doi: 10.1136/medethics-2020-106438. [ PMC free article ] [ PubMed ] [ CrossRef ] [ Google Scholar ]
- Gul Raisa B, Ali Parveen A. Clinical trials: The challenge of recruitment and retention of participants. Journal of Clinical Nursing. 2010; 19 (1–2):227–233. doi: 10.1111/j.1365-2702.2009.03041.x. [ PubMed ] [ CrossRef ] [ Google Scholar ]
- Halpern Scott D, Karlawish Jason H.T., Berlin Jesse A. The continuing unethical conduct of underpowered clinical trials. JAMA. 2002; 288 (3):358–362. doi: 10.1001/jama.288.3.358. [ PubMed ] [ CrossRef ] [ Google Scholar ]
- Hamel Lauren M, Penner Louis A, Albrecht Terrance L, Heath Elisabeth, Gwede Klement C, Eggly Susan. Barriers to clinical trial enrollment in racial and ethnic minority patients with cancer. Cancer Control. 2016; 23 (4):327–337. doi: 10.1177/107327481602300404. [ PMC free article ] [ PubMed ] [ CrossRef ] [ Google Scholar ]
- Health Research Authority. 2014. Ethics guidance: payments and incentives in research UK NHS Health Research Authority. National Research and Ethics Advisors' Panel. https://s3.eu-west-2.amazonaws.com/www.hra.nhs.uk/media/documents/hra-guidance-payments-incentives-research.pdf . Accessed 28 Aug 2021.
- Heller, Caren, Joyce E. Balls-Berry, Jill D. Nery, Patricia J. Erwin, Dawn Littleton, Mimi Kim, and Winston P. Kuo. 2014. Strategies addressing barriers to clinical trial enrollment of underrepresented populations: A systematic review. Contemporary Clinical Trials 39 (2): 169–182. [ PMC free article ] [ PubMed ]
- International Conference on Harmonisation of Technical Requirements for Registration of Pharmaceuticals for Human Use. 2016. ICH Harmonised Guideline Integrated Addendum To ICH E6(R1): Guideline For Good Clinical Practice E6(R2). https://database.ich.org/sites/default/files/E6_R2_Addendum.pdf . Accessed 28 Aug 2021.
- Jamrozik Euzebiusz, Selgelid Michael J. Should practice and policy be revised to allow for risk-proportional payment to human challenge study participants? Journal of Medical Ethics. 2020; 46 (12):835–836. doi: 10.1136/medethics-2020-106900. [ PMC free article ] [ PubMed ] [ CrossRef ] [ Google Scholar ]
- Jones Eleri, Liddell Kathleen. Should healthy volunteers in clinical trials be paid according to risk? Yes. BMJ. 2009 doi: 10.1136/bmj.b4142. [ PubMed ] [ CrossRef ] [ Google Scholar ]
- King Reame Nancy Treating research subjects as unskilled wage earners: A risky business. American Journal of Bioethics. 2001; 1 (2):53–54. doi: 10.1162/152651601300169103. [ PubMed ] [ CrossRef ] [ Google Scholar ]
- Klitzman Robert. How IRBs view and make decisions about coercion and undue influence. Journal of Medical Ethics. 2013; 39 (4):224–229. doi: 10.1136/medethics-2011-100439. [ PMC free article ] [ PubMed ] [ CrossRef ] [ Google Scholar ]
- Kwiatkowski Kat, Coe Kathlyn, Bailar John C, Swanson Marie G. Inclusion of minorities and women in cancer clinical trials, a decade later: Have we improved? Cancer. 2013; 119 (16):2956–2963. doi: 10.1002/cncr.28168. [ PubMed ] [ CrossRef ] [ Google Scholar ]
- Largent Emily A, Emanuel Ezekiel J, Lynch Holly Fernandez. Filthy lucre or fitting offer? Understanding worries about payments to research participants. The American Journal of Bioethics. 2019; 19 (9):1–4. doi: 10.1080/15265161.2019.1631076. [ PubMed ] [ CrossRef ] [ Google Scholar ]
- Largent Emily A, Grady Christine, Miller Franklin G, Wertheimer Allan. Money, coercion, and undue inducement: a survey of attitudes about payments to research participants. IRB. 2012; 34 (1):1–8. [ PMC free article ] [ PubMed ] [ Google Scholar ]
- Largent Emily A, Grady Christine, Miller Franklin G, Wertheimer Allan. Misconceptions about coercion and undue influence: Reflections on the views of IRB members. Bioethics. 2013; 27 (9):500–507. doi: 10.1111/j.1467-8519.2012.01972.x. [ PMC free article ] [ PubMed ] [ CrossRef ] [ Google Scholar ]
- Largent Emily A, Lynch Holly Fernandez. Paying research participants: Regulatory uncertainty, conceptual confusion, and a path forward. Yale Journal of Health Policy, Law, and Ethics. 2017; 17 (1):61–141. [ PMC free article ] [ PubMed ] [ Google Scholar ]
- Largent Emily A, Lynch Holly Fernandez. Paying research participants: The outsized influence of “undue influence” IRB. 2017; 39 (4):1–9. [ PMC free article ] [ PubMed ] [ Google Scholar ]
- Largent Emily A, Lynch Holly Fernandez. Addressing financial barriers to enrollment in clinical trials. JAMA Oncology. 2018; 4 (7):913–914. doi: 10.1001/jamaoncol.2018.0492. [ PubMed ] [ CrossRef ] [ Google Scholar ]
- Lee Chuen P, Holmes Tyson, Neri Eric, Kushida Clete A. Deception in clinical trials and its impact on recruitment and adherence of study participants. Contemporary Clinical Trials. 2018; 72 :146–157. doi: 10.1016/j.cct.2018.08.002. [ PMC free article ] [ PubMed ] [ CrossRef ] [ Google Scholar ]
- Lemmens Trudo, Elliott Carl. Guinea pigs on the payroll: The ethics of paying research subjects. Accountability in Research. 1999; 7 (1):3–20. doi: 10.1080/08989629908573939. [ PubMed ] [ CrossRef ] [ Google Scholar ]
- Lemmens Trudo, Elliott Carl. Justice for the professional guinea pig. American Journal of Bioethics. 2001; 1 (2):51–53. doi: 10.1162/152651601300169095. [ PubMed ] [ CrossRef ] [ Google Scholar ]
- London, Alex J. 2003. Threats to the common good: Biochemical weapons and human subjects research. Hastings Center Report 33 (5): 17–25. [ PubMed ]
- London Alex J. Reasonable risks in clinical research: A critique and a proposal for the Integrative Approach. Statistics in Medicine. 2006; 25 (17):2869–2885. doi: 10.1002/sim.2634. [ PubMed ] [ CrossRef ] [ Google Scholar ]
- London Alex J, Kimmelman Jonathan, Emborg Marina E. Beyond access v. protection in trials of innovative therapies. Science. 2010; 328 :829–830. doi: 10.1126/science.1189369. [ PMC free article ] [ PubMed ] [ CrossRef ] [ Google Scholar ]
- Lynch Holly F. Human research subjects as human research workers. Yale Journal of Health Policy, Law, and Ethics. 2014; 14 :122–193. [ PubMed ] [ Google Scholar ]
- Lynch Holly F, Darton Thomas C, Levy Jae, McCormick Frank, Ogbogu Ubaka, Payne Puth O, Roth Alvin E, Shah Akilah Jefferson, Smiley Thomas, Largent Emily A. Promoting ethical payment in human infection challenge studies. The American Journal of Bioethics. 2021; 21 (3):11–31. doi: 10.1080/15265161.2020.1854368. [ PubMed ] [ CrossRef ] [ Google Scholar ]
- Lynch Holly F, Joffe Steven, Thirumurthy Harsha, Xie Dawei, Largent Emily A. Association between financial incentives and participant deception about study eligibility. JAMA Network Open. 2019; 2 (1):e187355. doi: 10.1001/jamanetworkopen.2018.7355. [ PMC free article ] [ PubMed ] [ CrossRef ] [ Google Scholar ]
- Lynch Holly F, Largent Emily A. Compensating for research risk: Permissible but not obligatory. Journal of Medical Ethics. 2020; 46 (12):827–828. doi: 10.1136/medethics-2020-106829. [ PubMed ] [ CrossRef ] [ Google Scholar ]
- MacKay Douglas, Walker Rebecca L. Paying for fairness? Incentives and fair subject selection. The American Journal of Bioethics. 2021; 21 (3):35–37. doi: 10.1080/15265161.2020.1870766. [ PMC free article ] [ PubMed ] [ CrossRef ] [ Google Scholar ]
- Macklin Ruth. ‘Due’ and ‘undue’ inducements: on paying money to research subjects. IRB: Ethics & Human Research. 1981; 3 (5):1–6. doi: 10.2307/3564136. [ PubMed ] [ CrossRef ] [ Google Scholar ]
- Macklin Ruth. The paradoxical case of payment as benefit to research subjects. IRB: Ethics & Human Research. 1989; 11 (6):1–3. doi: 10.2307/3564182. [ PubMed ] [ CrossRef ] [ Google Scholar ]
- Malmqvist Erik. “Paid to endure”: Paid research participation, passivity, and the goods of work. The American Journal of Bioethics. 2019; 19 (9):11–20. doi: 10.1080/15265161.2019.1630498. [ PubMed ] [ CrossRef ] [ Google Scholar ]
- Manton Kerry J, Gauld Cassandra S, White Katherine M, Griffin Paul M, Elliott Suzanne L. Qualitative study investigating the underlying motivations of healthy participants in phase I clinical trials. British Medical Journal Open. 2019; 9 :e024224. doi: 10.1136/bmjopen-2018-024224. [ PMC free article ] [ PubMed ] [ CrossRef ] [ Google Scholar ]
- McCann Sharon K, Campbell Marion K, Entwistle Vikki A. Reasons for participating in randomised controlled trials: Conditional altruism and considerations for self. Trials. 2010; 11 (1):1–10. doi: 10.1186/1745-6215-11-31. [ PMC free article ] [ PubMed ] [ CrossRef ] [ Google Scholar ]
- McManus Lisa, Fisher Jill A. To report or not to report: Exploring healthy volunteers’ rationales for disclosing adverse events in Phase I drug trials. AJOB Empirical Bioethics. 2018; 9 (2):82–90. doi: 10.1080/23294515.2018.1469552. [ PMC free article ] [ PubMed ] [ CrossRef ] [ Google Scholar ]
- McNeill Paul. Paying people to participate in research: Why not? Bioethics. 1997; 11 (5):390–396. doi: 10.1111/1467-8519.00079. [ PubMed ] [ CrossRef ] [ Google Scholar ]
- Menikoff Jerry. Just compensation: Paying research subjects relative to the risks they bear. The American Journal of Bioethics. 2001; 1 (2):56–58. doi: 10.1162/152651601300169121. [ PubMed ] [ CrossRef ] [ Google Scholar ]
- Miller Franklin G, Brody Howard. A critique of clinical equipoise: Therapeutic misconception in the ethics of clinical trials. The Hastings Center Report. 2003; 33 (3):19–28. doi: 10.2307/3528434. [ PubMed ] [ CrossRef ] [ Google Scholar ]
- Millum Joseph, Garnett Michael. How payment for research participation can be coercive. The American Journal of Bioethics. 2019; 19 (9):21–31. doi: 10.1080/15265161.2019.1630497. [ PubMed ] [ CrossRef ] [ Google Scholar ]
- Moriarty Jeffrey. What’s in a wage? A new approach to the justification of pay. Business Ethics Quarterly. 2020; 30 (1):119–137. doi: 10.1017/beq.2019.42. [ CrossRef ] [ Google Scholar ]
- National Commission for the Protection of Human Subjects of Biomedical and Behavioral Research. 1979. The Belmont report: Ethical principles and guidelines for the protection of human subjects of research . U.S. Department of Health and Human Services. https://www.hhs.gov/ohrp/regulations-and-policy/belmont-report/read-the-belmont-report/index.html . Accessed 28 Aug 2021. [ PubMed ]
- National Health and Medical Research Council. 2019. Payment of participation in research: information for researchers, HRECs and other ethics review bodies. Australian Research Council and Universities Australia. Commonwealth of Australia, Canberra. https://www.nhmrc.gov.au/about-us/publications/payment-participants-research-information-researchers-hrecs-and-other-ethics-review-bodies#block-views-block-file-attachments-content-block-1 . Accessed 28 Aug 2021.
- Ndebele Paul, Mfutso-Bengo Joseph, Mduluza Takafira. Compensating clinical trial participants from limited resource settings in internationally sponsored clinical trials: A proposal. Malawi Medical Journal. 2008; 20 (2):42–45. [ PMC free article ] [ PubMed ] [ Google Scholar ]
- Nipp Ryan D, Hong Kessely, Paskett Electra D. Overcoming barriers to clinical trial enrollment. American Society of Clinical Oncology Educational Book. 2019; 39 :105–114. doi: 10.1200/EDBK_243729. [ PubMed ] [ CrossRef ] [ Google Scholar ]
- Nipp Ryan D, Lee Hang, Gorton Emily, Lichtenstein Morgan, Kuchukhidze Salome, Park Elyse, Chabner Bruce A, Moy Beverly. Addressing the financial burden of cancer clinical trial participation: Longitudinal effects of an equity intervention. The Oncologist. 2019; 24 (8):1048–1055. doi: 10.1634/theoncologist.2019-0146. [ PMC free article ] [ PubMed ] [ CrossRef ] [ Google Scholar ]
- Nuffield Council on Bioethics. 2011. Human bodies: donation for medicine and research. Nuffield Council on Bioethics. https://www.nuffieldbioethics.org/publications/human-bodies-donation-for-medicine-and-research . Accessed 28 Aug 2021.
- Nussbaum Martha C. Creating capabilities: The human development approach. Cambridge: Harvard University Press; 2013. [ Google Scholar ]
- Parkinson Beth, Meacock Rachel, Sutton Matt, Fichera Eleonora, Mills Nicola, Shorter Gilian W, Treweek S, Harman Nicola L, Brown Rebecca P, Gilies Katie, Bower Peter. Designing and using incentives to support recruitment and retention in clinical trials: A scoping review and a checklist for design. Trials. 2019; 20 (1):1–14. doi: 10.1186/s13063-019-3710-z. [ PMC free article ] [ PubMed ] [ CrossRef ] [ Google Scholar ]
- Pasqualetti Giuseppe, Gori Giovanni, Blandizzi Corrado, Del Tacca Mario. Healthy volunteers and early phases of clinical experimentation. European Journal of Clinical Pharmacology. 2010; 66 (7):647–653. doi: 10.1007/s00228-010-0827-0. [ PubMed ] [ CrossRef ] [ Google Scholar ]
- Persad Govind, Lynch Holly Fernandez, Largent Emily. Differential payment to research participants in the same study: An ethical analysis. Journal of Medical Ethics. 2019; 45 (5):318–322. doi: 10.1136/medethics-2018-105140. [ PubMed ] [ CrossRef ] [ Google Scholar ]
- Phillips Trisha. Exploitation in payments to research subjects. Bioethics. 2011; 25 (4):209–219. doi: 10.1111/j.1467-8519.2009.01717.x. [ PubMed ] [ CrossRef ] [ Google Scholar ]
- Phillips, Trisha. 2011b. A living wage for research subjects. The Journal of Law, Medicine & Ethics 3 9(2): 243–253. [ PubMed ]
- Probstfield, Jeffrey L., and Robert L. Frye. 2011. Strategies for recruitment and retention of participants in clinical trials. JAMA 306 (6): 1798–1799. [ PubMed ]
- Radder Hans. Which scientific knowledge is a common good? Social Epistemology. 2017; 31 (5):431–450. doi: 10.1080/02691728.2017.1353656. [ CrossRef ] [ Google Scholar ]
- Rawls John. A theory of justice. Cambridge: Harvard University Press; 1971. [ Google Scholar ]
- Resnik David B. Scientific research and the public trust. Science and Engineering Ethics. 2011; 17 (3):399–409. doi: 10.1007/s11948-010-9210-x. [ PMC free article ] [ PubMed ] [ CrossRef ] [ Google Scholar ]
- Resnik David B. Bioethical issues in providing financial incentives to research articipants. Medicolegal and Bioethics. 2015; 5 :35–41. doi: 10.2147/MB.S70416. [ PMC free article ] [ PubMed ] [ CrossRef ] [ Google Scholar ]
- Resnik David B. Are payments to human research subjects ethically suspect? Journal of Clinical Research Best Practices. 2019; 15 (6):2374. [ PMC free article ] [ PubMed ] [ Google Scholar ]
- Rhodes Rosamond. Rethinking research ethics. The American Journal of Bioethics. 2010; 10 (10):19–36. doi: 10.1080/15265161.2010.519233. [ PubMed ] [ CrossRef ] [ Google Scholar ]
- Ripley Elizabeth, Macrina Francis, Markowitz Monika, Gennings Chris. Why do we pay? A national survey of investigators and IRB chairpersons. Journal of Empirical Research on Human Research Ethics. 2010; 5 (3):43–56. doi: 10.1525/jer.2010.5.3.43. [ PMC free article ] [ PubMed ] [ CrossRef ] [ Google Scholar ]
- Roche Eric, King Romain, Mohan Helen M, Gavin Blanaid, McNicholas Fiona. Payment of research participants: Current practice and policies of Irish research ethics committees. Journal of Medical Ethics. 2013; 39 (9):591–593. doi: 10.1136/medethics-2012-100679. [ PubMed ] [ CrossRef ] [ Google Scholar ]
- Różyńska Joanna. On the alleged right to participate in high-risk research. Bioethics. 2015; 29 (7):451–461. doi: 10.1111/bioe.12146. [ PubMed ] [ CrossRef ] [ Google Scholar ]
- Różyńska Joanna. What makes clinical labour different? The case of human guinea pigging. Journal of Medical Ethics. 2018; 44 (9):638–642. doi: 10.1136/medethics-2017-104267. [ PubMed ] [ CrossRef ] [ Google Scholar ]
- Różyńska Joanna. Research participants should be rewarded rather than “compensated for time and burdens” The American Journal of Bioethics. 2021; 21 (3):53–55. doi: 10.1080/15265161.2020.1870770. [ PubMed ] [ CrossRef ] [ Google Scholar ]
- Salman, Rustam A. S. Elaine Beller, Jonathan Kagan, Elina Hemminki, Robert S. Phillips, Julian Savulescu, Malcolm Macleod, Janet Wisley, and Iain Chalmers. 2014. Increasing value and reducing waste in biomedical research regulation and management. The Lancet 383 (9912): 176–185. [ PMC free article ] [ PubMed ]
- Saunders John. Should healthy volunteers in clinical trials be paid according to risk? BMJ. 2009 doi: 10.1136/bmj.b4145. [ PubMed ] [ CrossRef ] [ Google Scholar ]
- Schaefer G. Owen, Emanuel Ezekiel J, Wertheimer Alan. The obligation to participate in biomedical research. JAMA. 2009; 302 (1):67–72. doi: 10.1001/jama.2009.931. [ PMC free article ] [ PubMed ] [ CrossRef ] [ Google Scholar ]
- Shamoo Adil E, Resnik David B. Strategies to minimize risks and exploitation in phase one trials on healthy subjects. The American Journal of Bioethics. 2006; 6 (3):W1–W13. doi: 10.1080/15265160600686281. [ PMC free article ] [ PubMed ] [ CrossRef ] [ Google Scholar ]
- Stones, Martyn, and John McMillan. 2010. Payment for participation in research: a pursuit for the poor? Journal of Medical Ethics 36 (1): 34–36. [ PubMed ]
- Stunkel Leanne, Grady Christine. More than the money: A review of the literature examining healthy volunteer motivations. Contemporary Clinical Trials. 2011; 32 (3):342–352. doi: 10.1016/j.cct.2010.12.003. [ PMC free article ] [ PubMed ] [ CrossRef ] [ Google Scholar ]
- Sully Ben G, Julious Steven A, Nicholl Jon A. A reinvestigation of recruitment to randomised, controlled, multicenter trials: A review of trials funded by two UK funding agencies. Trials. 2013; 14 :166. doi: 10.1186/1745-6215-14-166. [ PMC free article ] [ PubMed ] [ CrossRef ] [ Google Scholar ]
- Tishler Carl L, Bartholomae Suzanne. The recruitment of normal healthy volunteers: A review of the literature on the use of financial incentives. The Journal of Clinical Pharmacology. 2002; 42 (4):365–375. doi: 10.1177/00912700222011409. [ PubMed ] [ CrossRef ] [ Google Scholar ]
- Treweek Shaun, Lockhart Pauline, Pitkethly Marie, Cook Jonathan A, Kjeldstrøm Monica, Johansen Marit, Taskila Taina K, Sullivan Frank M, Wilson Sue, Jackson Catherine, Jones Ritu, Mitchell Elizabeth D. Methods to improve recruitment to randomised controlled trials: Cochrane systematic review and metaanalysis. British Medical Journal Open. 2013; 3 :e002360. doi: 10.1136/bmjopen-2012-002360. [ PMC free article ] [ PubMed ] [ CrossRef ] [ Google Scholar ]
- Unger Joseph M, Gralow Julie R, Albain Kathy S, Ramsey Scott D, Hershman Dawn L. Patient income level and cancer clinical trial participation: A prospective survey study. JAMA Oncology. 2016; 2 (1):137–139. doi: 10.1001/jamaoncol.2015.3924. [ PMC free article ] [ PubMed ] [ CrossRef ] [ Google Scholar ]
- Unger Joseph M, Hershman Dawn L, Albain Kathy S, Moinpour Carol M, Petersen Judith A, Burg Kenda, Crowley John J. Patient income level and cancer clinical trial participation. Journal of Clinical Oncology. 2013; 31 (5):536–542. doi: 10.1200/JCO.2012.45.4553. [ PMC free article ] [ PubMed ] [ CrossRef ] [ Google Scholar ]
- Unger Joseph M, Vaidya Riha, Hershman Dawn L, Minasian Lori M, Fleury Mark E. Systematic review and meta-analysis of the magnitude of structural, clinical, and physician and patient barriers to cancer clinical trial participation. JNCI: Journal of the National Cancer Institute. 2019; 111 (3):245–255. doi: 10.1093/jnci/djy221. [ PMC free article ] [ PubMed ] [ CrossRef ] [ Google Scholar ]
- Walker Rebecca L, Fisher Jill A. “My body is one of the best commodities”: Exploring the ethics of commodification in phase i healthy volunteer clinical trials. Kennedy Institute of Ethics Journal. 2019; 29 (4):305–331. doi: 10.1353/ken.2019.0028. [ PMC free article ] [ PubMed ] [ CrossRef ] [ Google Scholar ]
- Watson Judith M, Torgerson David J. Increasing recruitment to randomised trials: A review of randomised controlled trials. BMC Medical Research Methodology. 2006; 6 (1):1–9. doi: 10.1186/1471-2288-6-34. [ PMC free article ] [ PubMed ] [ CrossRef ] [ Google Scholar ]
- Wertheimer Alan. Exploitation in clinical research. In: Emanuel Ezekiel J, Crouch Robert A, Grady Christine C, Lie Reidar K, Miller Franklin G, Wendler David D., editors. The Oxford textbook on the ethics of clinical research. New York: Oxford University Press; 2008. pp. 201–210. [ Google Scholar ]
- Wertheimer Alan. Is payment a benefit. Bioethics. 2013; 27 :105–116. doi: 10.1111/j.1467-8519.2011.01892.x. [ PMC free article ] [ PubMed ] [ CrossRef ] [ Google Scholar ]
- Wertheimer Alan, Miller Franklin G. Payment for research participation: A coercive offer? Journal of Medical Ethics. 2008; 34 (5):389–392. doi: 10.1136/jme.2007.021857. [ PubMed ] [ CrossRef ] [ Google Scholar ]
- Wilkinson Martin, Moore Andrew. Inducement in research. Bioethics. 1997; 11 (5):373–389. doi: 10.1111/1467-8519.00078. [ PubMed ] [ CrossRef ] [ Google Scholar ]
- Williams Rebecca J, Tse Tony, DiPiazza Katelyn, Zarin Deborah A. Terminated trials in the ClinicalTrials.gov results database: Evaluation of availability of primary outcome data and reasons for termination. PLoS ONE. 2015; 10 (5):e0127242. doi: 10.1371/journal.pone.0127242. [ PMC free article ] [ PubMed ] [ CrossRef ] [ Google Scholar ]
- Winter, Stuart S., Janet M. Page-Reeves, Kimberly A. Page, Emily Haozous, Angelica Solares, Carla N. Cordova, and Richard S. Larson. 2018. Inclusion of special populations in clinical research: Important considerations and guidelines. Journal of Clinical and Translational Research 4 (1): 56–69. [ PMC free article ] [ PubMed ]
- World Medical Association (WMA). 2013. Declaration of Helsinki. Ethical principles for medical research involving human subjects. Fortaleza, Brazil. https://www.wma.net/policies-post/wma-declaration-of-helsinki-ethical-principles-for-medical-research-involving-human-subjects/ . Accessed 28 Aug 2021.
Understanding the Factors Influencing Street Vendors towards Use of Digital Payment Systems in India
Ieee account.
- Change Username/Password
- Update Address
Purchase Details
- Payment Options
- Order History
- View Purchased Documents
Profile Information
- Communications Preferences
- Profession and Education
- Technical Interests
- US & Canada: +1 800 678 4333
- Worldwide: +1 732 981 0060
- Contact & Support
- About IEEE Xplore
- Accessibility
- Terms of Use
- Nondiscrimination Policy
- Privacy & Opting Out of Cookies
A not-for-profit organization, IEEE is the world's largest technical professional organization dedicated to advancing technology for the benefit of humanity. © Copyright 2024 IEEE - All rights reserved. Use of this web site signifies your agreement to the terms and conditions.
- Open access
- Published: 13 March 2023
Online payment fraud: from anomaly detection to risk management
- Paolo Vanini ORCID: orcid.org/0000-0003-0391-4847 1 ,
- Sebastiano Rossi 2 ,
- Ermin Zvizdic 3 &
- Thomas Domenig 4
Financial Innovation volume 9 , Article number: 66 ( 2023 ) Cite this article
10k Accesses
9 Citations
Metrics details
Online banking fraud occurs whenever a criminal can seize accounts and transfer funds from an individual’s online bank account. Successfully preventing this requires the detection of as many fraudsters as possible, without producing too many false alarms. This is a challenge for machine learning owing to the extremely imbalanced data and complexity of fraud. In addition, classical machine learning methods must be extended, minimizing expected financial losses. Finally, fraud can only be combated systematically and economically if the risks and costs in payment channels are known. We define three models that overcome these challenges: machine learning-based fraud detection, economic optimization of machine learning results, and a risk model to predict the risk of fraud while considering countermeasures. The models were tested utilizing real data. Our machine learning model alone reduces the expected and unexpected losses in the three aggregated payment channels by 15% compared to a benchmark consisting of static if-then rules. Optimizing the machine-learning model further reduces the expected losses by 52%. These results hold with a low false positive rate of 0.4%. Thus, the risk framework of the three models is viable from a business and risk perspective.
Introduction
Fraud arises in the financial industry via numerous channels, such as credit cards, e-commerce, phone banking, checks, and online banking. Juniper Research ( 2020 ) reports that e-commerce, airline ticketing, money transfer, and banking services will cumulatively lose over $ 200 billion due to online payment fraud between 2020 and 2024. The increased sophistication of fraud attempts and the increasing number of attack vectors have driven these results. We focus on online and mobile payment channels and identity theft fraud (i.e., stealing an individual’s personal information to conduct fraud )(Amiri and Hekmat 2021 ). The aim is to identify external fraudsters who intend to initiate payments in their interests. As fraudsters gain access to the payment systems as if they were the owners of the accounts, they cannot be identified based on the account access process. However, the fraudster behaves differently during a payment transaction than the account owner and/or the payment has unusual characteristics, such as an unusually high payment amount or transfer to an account in a jurisdiction that does not fit the life context and payment behavior of the customer. The assumption is that algorithms can detect anomalies in behavior during payment transactions.
West and Bhattacharya ( 2016 ), Abdallah et al. ( 2016 ), Hilal et al. ( 2021 ), and Ali et al. ( 2022 ) reviewed financial fraud. They found a low number of articles regarding online payment fraud. For example, Ali et al. ( 2022 ) cited 20 articles on financial statement fraud and 32 articles on credit card fraud, see Li et al. ( 2021 ) for credit card fraud detection. Online payment fraud was not listed. The reviews also clarified that many articles utilized aggregated characteristics. However, we emphasize that fraud in online payments can only be detected based on individual data, as such fraud can only be detected through the possible different behavior of the fraudster and the account holder during payments. As fraudsters learn how best to behave undetected over time, they adapt their behavior. Therefore, self-learning defense methods are expected to outperform static-rule-based algorithms. The correctness of the expectation was shown by Abdallah et al. ( 2016 ) and Hilal et al. ( 2021 ). Various machine-learning algorithms for fraud detection have been proposed in the literature, including decision trees, support vector machines, and logistic regression to neural networks. Aggarwal and Sathe ( 2017 ) discussed various methods for outlier ensembles, and Chandola et al. ( 2009 ) provided a taxonomy and overview of anomaly detection methods.
A common feature in many studies is imbalanced data (i.e., the low proportion of fraud events in the dataset, see Wei et al. 2013 ; Carminati et al. 2015 ; Zhang et al. 2022a ; Singh et al. 2022 ). Risk detection involves detecting fraudulent transactions and stopping them before execution.
In addition to the efficiency of the algorithms, the data basis is an important reason for the differences in fraud-detection performance. While many studies have utilized either often less rich synthetic or Kaggle data, we were able to work with real data. Log files, which have substantial information content in our work, are hardly expected in Kaggle data. The difference in the data complexity is also reflected in the number of features. Singh et al. ( 2022 ) showed that the feature space consists of 31 features compared to our 147 features. Moreover, the proportion of fraudulent transactions in Singh et al. ( 2022 ) is more than a hundred times higher than in our case. Consequently, our data are much more unbalanced than any other study we know of, and the task of finding efficient fraud detection algorithms is more difficult.
However, limiting risk management to the optimal detection of anomalies does not ensure that losses caused by fraud are minimal. Optimal fraud detection can be economically suboptimal if, for example, it is efficient for small amounts of money but unsuccessful for large amounts. Thus, the machine learning outputs for risk identification must be optimized from an economic perspective. We call this optimization the triage model. Yet, neither fraud detection nor the triage model can provide an answer to the question of how large the losses in a payment channel are. Therefore, we develop a statistical risk model that considers the effects of countermeasures on loss potential. The risk model provides risk transparency and makes it possible to assess which measures in the fight against fraud in various payment channels make sense from an economic and risk perspective.
Literature on fraud risk models often refers to qualitative or assessment models for assessing fraud risk or risk assessment models (Sabu et al. 2021 ). We are not aware of any quantitative fraud risk management framework that explicitly considers the impact of the fraud detection process statistically in risk modelling. For organizational, procedural, and legal risk aspects, we refer to the literature. Montague’s ( 2010 ) book focuses on fraud prevention in online payments but does not consider machine learning and risk management in detail. The Financial Conduct Authority’s Handbook (FCA 2021 ) provides a full listing of the FCA’s legal instruments, particularly those relating to financial crime in financial institutions. Power ( 2013 ) highlights the difference between fraud and fraud risk from historical and business perspectives. Van Liebergen ( 2017 ) looks at “regtech” applications of machine learning in online banking. Fraud risk events in cryptocurrency payment systems are different from the online banking cases under consideration; see Jung et al. ( 2019 ) for fraud acting on a decentralized infrastructure and the review article of Trozze et al. ( 2022 ).
The development and validation of the three linked models are the main contributions of our work. To our knowledge, this is the first study to develop, validate, and link components of the risk management process. The output of the anomaly detection model (i.e., the ROC curves), is the input for the triage model, which provides economically optimized ROC curves. Fraud statistics data were utilized to calibrate the various components in the risk model. With these three models, the fraud risk management process can be qualitatively implemented at the same level as the risk management of market or counterparty risks (see Bessis ( 2011 ) to describe risk management in banks).
The performance of our risk management framework is the second contribution, although the performance comparison of our fraud detection method with the literature is limited and cautious, due the use of synthetic data instead of real data, a consideration of different channels in payments with different behavioral characteristics of bank customers, and the publication of incomplete statistics. Nevertheless, we compared our work with Wei et al. ( 2013 ) and Carminati et al. ( 2015 ), both of which analyze online banking fraud based, in part, on real data. The true positive rate (TPR) at a false positive rate (FPR) of \(1\%\) was \(45\%\) . In Wei et al. the TPR is between \(49\%\) and \(60\%\) , but unfortunately, the FPR is unknown. In the relevant scenario of Carminati et al. ( 2015 ), the TPR is \(70\%\) with an FPR of \(14\%\) . This FPR is not acceptable to any bank. Processing by specialists leads to high costs. We discuss all these statements in detail in the " Validation results " section. Considering all three models, the theoretical and practical importance of our approach becomes clear. The expected losses in a scenario of CHF 2.023 million, which utilizes the results of machine learning without economic optimization in the triage model, common in the literature, are reduced to CHF 0.800 million with the triage model (i.e., a reduction in the loss potential by more than 60% follows). In addition, if fraud detection is implemented without a risk model, fraud risk can be massively overestimated. Applying our models to three different payment channels, the overestimation of risk ranged from 54% to over 700%.
The remainder of this paper is organized as follows. In " Fraud risk management framework " section, the model selection for the fraud risk management framework is motivated and described. In " Online payment fraud anomaly detection " section, we consider the anomaly-detection model. " Fraud detection triage model " section links fraud detection to an economic perspective utilizing the triage model. " Risk model " presents the statistical risk model. " Conclusion " section concludes.
Fraud risk management framework
We provide an overview of the three interrelated quantitative models in the context of risk management: online payment anomaly detection, triage model, and risk model.
Online payment fraud anomaly detection
The goal of anomaly detection is to detect fraudulent activities in e-banking systems and to maintain the number of false alarms at an acceptable level. The implementation of the model consists of three steps: pre-filter, feature extraction, and machine learning.
Non-learning pre-filters ensure that both obvious fraud and normal transactions are sorted early to reduce the false positive rate. Only transactions that pass the pre-filter step are passed on to the machine-learning model. Banks utilize non-customer-based static if-then rules, such as blacklists or whitelists. Pre-filters free the algorithms from obvious cases. The adaptability and flexibility of the machine-learning model is necessary to counter the ever-improving attacks of fraudsters with effective fraud detection.
Our data face the following general challenges in payment fraud detection (per Wei et al. 2013 ): large transaction volume with the need for real-time fraud detection, a highly imbalanced dataset, dynamic fraud behavior, limited forensic information, and varying customer behavior.
Given the extremely imbalanced data, fully supervised algorithms typically struggle. Aggarwal and Sathe ( 2017 ) proposed unsupervised and weakly supervised approaches based on features that encode deviations from normal behavior. For each customer participating in an e-banking session, we assess whether the agent’s behavior is consistent with the account holder’s normal behavior. The key information for behavioral analysis lies in the sequence of the customer’s clicks during the session. We show that, unlike online e-commerce transactions (see Wei et al. 2013 ), transaction data, customer behavior data, account data, and booking data are also important for the performance of the algorithm. More precisely, these features are divided into behavioral, transactional, and customer-related features. Starting with nearly 800 features, 147 were extracted utilizing a Bagged Decision Tree Model (BDT). These numbers are many times higher than those for credit card fraud with one to two dozen features (see Table 8 in Hilal et al. 2022 ). The high dimensionality of the feature space also arises in machine learning corporate default prediction models, where several steps are needed to extract all noisy features (see Kou et al. 2021 ).
Our e-fraud model operates according to the following principles.
The model learns the “normal” behavior of each customer based on historical payment data and online banking log data.
Each new transaction is checked against the learned “normal” behavior to determine if it is an anomaly by extracting the 147 features from the data.
If an anomaly is detected, it is flagged as suspected fraud.
Detected transactions that are not found to be fraudulent after manual review are reported back to the model for learning purposes.
As there are very few known fraud cases, all base learners are trained on fraud-free data only in step one. Fraud cases are only utilized in step two of ensemble aggregation when the base learners are combined to form the final predictive function. The first step is to define base learners who are rich enough to detect a wide range of suspicious transactions or online user sessions. We consider three base learners: the density-based outlier detection model (unsupervised, Local Outlier Factor (LOF)), the isolation-based outlier detection model (unsupervised, Isolation Forest (IF)), and a model for normal customer behavior (supervised, Bagged Decision Trees (BDT)) as base learners (see Breunig et al. ( 2000 ), Chandola et al. ( 2009 ); Zhang et al. ( 2022b ) for LOF, Liu et al. ( 2012 ); Tokovarov and Karczmarek ( 2022 ) for IF). We refer to individual instances of LOF, IF, or BDT as base learners. The BDT model is not only a base model, but it is also utilized for feature selection in the other two base models: LOF and IF. The LOF method is suitable for outlier detection, where each observation is assigned an outlier level based on its distance from the nearest cluster of neighboring observations. The aim is to detect outliers in inhomogeneous data, for which classical global outlier methods typically do not provide satisfactory results. Conversely, IF explicitly isolates anomalies without capturing all normal instances. These two methods consider the heterogeneity in the data.
In the second stage, the base learner’s fraud score was aggregated. We consider two approaches to determine the weights in the ensembles: a simple averaging and a supervised approach, although our model largely consists of unsupervised procedures because of the limited availability of fraud cases for which we can extract all the required features. However, we introduce supervision where we utilize scarce labelled data to adjust the importance of certain base learners in the voting scheme, ultimately deciding whether an observation is fraudulent. The penalized logistic regression chosen for classification allows for a better interpretation of the model, as the weights can be utilized to identify base learners, subsets of features, and subsets of samples that have been particularly useful in detecting a particular type of fraud.
Triage model
The fraud detection model calculates scores and, in comparison with a threshold value, decides whether a transaction is flagged as an anomaly. This process results in the probability of detection for a given investigation effort, as indicated by the ROC curve. By making the threshold dependent on the transaction size, we can ensure that larger transaction amounts are more likely detected than smaller ones. This gives up part of the true positive rate (TPR) to reduce overall economic losses (i.e., the TPR decreases for a given FPR). This economic optimization that leads to adjusted ROC curves defines the triage model.
To minimize expected cumulative losses, the constant fraud anomaly detection threshold becomes a function of the transaction amount. Here, the transaction amounts are random variables whose distributions are estimated. In the optimization problem, the transaction function is chosen to maximize the average cumulative sum of the detected fraudulent transactions, where the expected FPR must not exceed a certain threshold. Utilizing this optimal threshold function, the fitted ROC curves were obtained.
The optimization problem has a unique solution if the ROC curve is a concave function of the false positive rate function of the threshold and if the acceptance set of the expected false positive function constraint is convex. With the chosen piecewise linear false positive constraint function, the assumptions regarding the existence of an optimum are satisfied. The ROC curves that result when fraud anomalies are detected serve as inputs for the optimization. However, because only a vanishingly small number of fraud cases exist, the TPR values for certain FPR levels are subject to considerable uncertainty. Hence, cubic spline functions were utilized for the ROC curve of the optimization.
The UK Finance (2019) report states that the recovery value for online and mobile banking in the UK is 18% of the potential loss. Therefore, we introduced an extension to the optimization program to include recovery.
Losses from transaction fraud are included in operational risk incurred by banks. As for other operational risks, one of the key questions from a risk-management perspective is whether the allocated resources and countermeasures are adequate. To answer this, one needs some way of quantifying the risk incurred, ideally a Value-at-Risk (VaR)-model that fits the general risk framework of the bank. Simply, the model calculates the loss \(L=E(\lambda )\times E(\tau )\) where \(\lambda\) is the expected event frequency (fraud), and \(\tau\) is the expected loss per event. The challenge is to determine the distributions of these variables in a tractable and plausible manner and define a model while having very scarce data on past events. We chose the path of an aggregated simulation of many scenarios per e-channel to account for the inherent uncertainty in the choice of these parameters.
Unlike market or credit risk, fraud risk is borne by comparatively few individuals or groups who utilize very specific strategies and technologies to overcome vulnerability in the payment process. Simultaneously, defenders analyze attack plans and update their countermeasures. In this constantly changing environment, neither the frequency of attacks nor the transaction amounts can be assumed to be statistically regular with great certainty. Therefore, we propose a simple, flexible stochastic model composed of basic building blocks. With such a model, risk managers can quickly adjust the model as needed, perform what-if analyses, or simulate changes in payment infrastructure.
The basic model structure for the e-fraud risk model consists of (i) independent models for the three channels, whose components and parameters can be flexibly assembled and adjusted, (ii) sub-models in each channel based on three model types, and (iii) a recovery model for each channel. The three model types for the three different online payment channels in this study are a Beta model (restricted distribution of transaction amounts), a Generalized Pareto Distribution (GPD, unrestricted distribution of transaction amounts), and a “mass attack model” (many simultaneous Beta-type attacks).
Countermeasures against fraud and recovery measures after fraud events play an essential role in determining risk potential. Therefore, they were integrated into the risk models. Countermeasures against online fraud can be divided into those that strengthen the general infrastructure of the payment process and those that focus on defense against actual attacks. The former is conceptually part of the risk model described above, as it affects the frequency and possibly the transaction size of the attacks. However, the latter is better understood in the context of recovery and is considered in the triage model.
Raw data consisted of transaction data, interaction data between customer and e-banking interface, account, booking, and customer reference data. All users with fewer than 10 logged online sessions were removed as input for ensemble learning. The removed cases were handled separately by utilizing a case-back model.
The transaction history in our dataset consists of 140 million transactions over three years. One hundred fraud cases are reported, but only 11 cases can be linked to the recorded 900’000 online session logs: a \(0.0012\%\) fraud rate. Only 900’000 of the 140 million transactions were possible as the log files were only stored in the bank for three months. This change occurred after the project.
A feature vector is created for each e-banking session based on raw data. The interaction pattern features consist of n -grams constructed from customers’ request sequences and normalized deviations from the expected duration between each pair of consecutive requests sent by a customer in an online session. Particular attention was paid to the typical time required to complete a two-step verification process during enrolment. Payment pattern characteristics were calculated for weekday, weekly, and monthly seasonality. These include normalized deviations from the expected payment amount and remaining account balance. Technical data included the IP address of the online session, the HTML agent, and the number of JavaScript scripts executed. Finally, we utilized historically observed confirmed fraudulent transaction identifiers as the ground truth for the weakly monitored part of the pipeline.
Several quality checks were performed. Consistency tests ensure that the session interaction data and transactions match, for example, that the account exists or that a recipient is listed in the transaction. We also checked for missing or non-parsable values, the latter removed.
The data are extracted from several different data sources within the bank in a two-step Python extract, transform, and load (ETL) process, and converted into features for the algorithm. First, we introduce all raw data into a standard structured format for all data sources. Then, we perform the feature engineering described in the following sections to compute the data input for the ensemble.
Our fraud rate \(0.0012\%\) is much lower than that reported in the literature. The figures in the two online banking fraud papers, Wei et al. ( 2013 ) and Carminati et al. ( 2015 ), are \(0.018\%\) and \(1\%\) , respectively. For credit card fraud, the fraud case number is larger, such as \(2\%\) in Piotr et al. ( 2008 ). Outside the banking fraud sector, anomalies account for up to 40% of the observations (see Pang et al. 2020 ). Similar numbers hold in Zhang et al. ( 2022b ), who tested their ensemble-based outlier detection methods on 35 real datasets from various sectors outside the financial sector, with an average fraud rate of 26%.
Feature extraction
For weak supervision, we utilized historically observed confirmed fraudulent transaction identifiers as the ground truth. For training and inference, we created a feature vector for each e-banking session based on the raw data. Each feature aims to encode deviation from expected (“normal”) customer behavior, as observed in historical interactions with the online banking interface and executed transactions. Three types of features are considered.
Behavioral features
The underlying motivation for utilizing features derived from customers’ online session logs is that a large fraction of online payment fraud involves hijacking, where a foreign agent (human or robot fraudster) takes control of the e-banking session. Consequently, it is expected that the timing and sequence of requests posted by the user in a fraudulent session will be significantly different from those in a non-fraudulent session. We utilize the information about user request types (e.g., “get account balance”, “create payment”) and corresponding timestamps to construct the following features:
Normalized n-gram frequency of user’s requests within the online session. We utilized single, pairs and triplets of consecutive requests (1-, 2- and 3-grams) to derive a fixed-size representation. We performed normalization by dividing the number of occurrences for each request with the total number of requests in each session.
Normalized time between consecutive user request n-grams. For each pair of recorded consecutive n-grams, we transformed absolute time between them into deviations by computing z-scores relative to respective historical observations.
Technical attributes of a session (e.g., IP address of the online session, HTML agent, screen size, and the number of executed Javascript scripts) in binary format - 0 if previously observed, otherwise 1.
Transactional features
Transactional features aim to quantify how anomalous aggregate payments scheduled in an online session are compared with previously executed payments and the remaining balance on the account. They are designed to capture attempts to empty a victim’s account through a single large or many small transactions, while being mindful of seasonal patterns (e.g., holidays, travel expenses, bills, etc.).
Normalized ratio of the payment amount relative to remaining account balance. We normalize by computing z-scores relative to historically observed ratios.
Deviation of the scheduled payment amount from the seasonally expected amount. We compute four deviations per session using z-scores relative to historical payments executed in the same hour of the day, day of the week, week of the month, and month of the year, respectively.
Scheduled time for payment execution in binary format - 0 if immediate, 1 if lagged.
A short payment history and many accounts with relatively infrequent transactions proved detrimental to seasonality modelling, hence, these features were omitted from the final model.
Customer-related features
Customer-related features provide insight into peer groups, relationships with other customers, and the service packages they utilize. These include:
Sociodemographic (e.g., age, nationality, profession, and income)
Seniority of client relationship
Product usage (i.e., savings, investment accounts, and mortgages)
Relationship to other customers (i.e., shared accounts, spouses, and family)
These features were not considered within the scope of our study because of data limitations and time constraints.
Functionality and structure of the fraud model
Base learner: bagged decision tree.
Bagged decision trees (BDT) are trained utilizing the concept of transfer learning, which assumes that distinguishing between the behaviors of different clients within their online sessions is a related problem in distinguishing between fraudulent and non-fraudulent sessions. The underlying motivation considers that a large fraction of online payment fraud involves hijacking or when a foreign agent (human or robot fraudster) takes control of the e-banking session. As fraudulent sessions are rare and non-fraudulent sessions abound, the utilization of transfer learning enables the extraction of custom patterns from a much broader dataset and the use of supervised learning. Transfer learning comprises two phases. A learning phase where one discriminates behavioral characteristics of each customer vs. non-customers and a prediction phase where discrimination between non-fraudulent and fraudulent users sessions were considered in this study. The “non-customer” class label is then attributed to fraudulent behavior.
The decision function in BDT base learners is the probability that an observation, a planned transaction x , is a “non-customer behavior.” This value is equal to the average probability observed for all decision trees in the forest.
where M is the number of trees in the bagged forest, \(c_j\) is the corresponding customer behavior class, and \(P_i( Y\ne c_j|X=x)\) is the probability that observation x is a “non-customer behavior” as predicted by the i th tree. Customer behavior class \(c_j\) consists of the collected sessions and transactions, excluding potential fraud. The model was fitted as follows:
For each customer \(c_j\) , we collect the associated sessions, excluding the potential fraud cases. This set represents the “behavior of customer \(c_j\) .”
From the pool of all customer sessions \(C_{-j}\) (excluding \(c_j\) ) we draw a uniform sample of observations to generate a set representing the class “behavior of customer \(c_j\) not,” \(\not c_j\) for short, and equal in size to the \(c_j\) set. Equal sampling is performed to ensure that none of the other customers are overrepresented in \(\not c_j\) .
Each bagged forest in the ensemble is trained on a matching feature subspace utilizing all these observations. The forests consist of 100 decision trees each.
BDTs provide variable selection as an additional benefit, owing to the large amount of data involved in supervised classification. Therefore, they can be utilized to estimate the importance of variables and adequacy of feature engineering. We achieve this by calculating the Gini impurity decreases at each split point within each tree for each feature. Gini impurity is a measure of the likelihood that a randomly selected observation would be incorrectly classified by a specific node m :
where \(p_{mi}\) is the portion of samples classified as i at node m . These impurity decreases were averaged across all decision trees and outputs to estimate the importance of each input variable. The greater the decrease in impurity, the greater the importance. Utilizing these results, we can identify the relevant subsets of input variables.
Relying on the concept of transfer learning (if the problem described in ?? is sufficiently like fraud detection), we use BDT to select a subset of \(N=147\) features. Particularly important were features measuring the deviation from the typical time required to complete a two-step verification process during login. Features that encode the relative time between n -grams and specific user request sequences are important. The following additional base model was built using the features selected by BDT.
Base learner: local outlier factor
The Local Outlier Factor (LOF) detection method assigns an outlier level to each observation based on its distance from the nearest cluster of neighboring observations (Breunig et al. 2000 ). The general intent of the LOF model is to identify outliers in the interior region of data, for which classical global outlier methods and the other considered algorithm, isolation forest, usually do not provide satisfactory results. The LOF decision function is as follows:
with K a k -neighborhood and LD ( x ) the local reachability distance density from x to its k -th nearest neighbor. We fit the model for each customer by collecting the first associated sessions or transactions, excluding potential fraud. Each LOF in the ensemble is created utilizing all these observations on a subspace of the relevant features selected by BDT and the sampled hyper-parameter. Finally, each time a new observation is available, its decision function value is computed regarding the observations from the training set.
Base learner: isolation forest
The IF algorithm recursively splits the data into two parts based on a random threshold, until each data point is isolated. The algorithm randomly selects a feature at each step, and then randomly selects a division value between its minimum and maximum values. The algorithm filters out data points that require fewer steps to be isolated from the entire dataset. In our case, IF separates one observation from the rest of a randomly selected subsample of the original dataset (Fei Tony et al. 2008 ). Anomalies are instances with short average isolation path lengths. The IF decision function is
where E ( H ( x )) is the average number of edges traversed to isolate the node and C is the average number of edges traversed in an unsuccessful search. To fit the model, we first collected each client’s associated sessions or transactions, excluding potential fraud cases. In step two, each isolation forest in the ensemble was created utilizing all these observations in a matching feature subspace. Each forest consisted of 100 isolated trees. Finally, each time a new observation was available, its decision function value was computed regarding the isolation trees created based on the training set.
Base learner scores combination
The decision functions of the base learners produced by our ensembles must be combined into a single fraud score. As these are in different ranges and scales to render the decision functions comparable, we first replace the original scores by their ranks, regarding the non-fraudulent training scores. Rank normalization is more robust and numerical stable as opposed to z-scores, for example. Therefore, we replaced the original scores with their ranks regarding the non-fraudulent training scores for each base learner:
where V is the set of all \(\delta _{\text {Base}}(p )\) over all observations \(p'\) in the learners’ training subsample, with Base being LOF, IF, or BDT.
Owing to the few fraud cases, our model largely consists of unsupervised procedures. However, we introduced supervision utilizing scarce labelled data to readjust the importance of particular base learners in the voting scheme, ultimately deciding whether an observation is fraudulent.
The following score combination procedure was established. First, a training set comprises all fraud cases in the sample, along with healthy transactions uniformly sampled over customers from the ensemble training data. Second, a logistic regression is trained to classify observations as fraudulent or not utilizing the 6 N normalized decision function features and the known fraud status on past transactions as the label.
The following binary-class penalized cost function is minimized:
where \(y_i\) is the fraud label of transaction i , \(X_i'\) is a row of 6 N decision functions describing transaction i , R is the regularization factor, and ( w , c ) is a set of weights defining the decision boundary between the two classes: fraud and non-fraud. To account for the imbalance between fraud and non-fraud transactions in our sample, we assign asymmetric penalties for fraud misclassification, as opposed to non-fraud classification.
Choosing logistic regression to optimize the weights of the base learners ensures that the final score combination \(x'w\) represents the log-odds of an observation to be fraudulent.
where \(\delta (x)\) is the probability that the observation x is fraudulent. Finally, to assign a fraud label to a session x , we compare the output combined score, or equivalently, the probability \(\delta (x)\) , to a threshold y , which is chosen based on ROC curve analysis such that the defined maximum allowed false positive rate is not exceeded. The decision boundary of logistic regression is linear, where each base learner is assigned a weight \(w_i\) to determine its relative importance for fraud classification. This structure simplifies the interpretation of the model because these weights can be utilized to identify the base learners, feature subsets, and sample subsets, which are particularly useful in detecting a particular type of fraud associated with a high weight \(w_i\) . Appendix A provides a detailed description of the ensemble design.
Normal customer behavior model
In summary, we created an ensemble model for each client, which is re-trained with new data at regular intervals and can be described by the following steps:
We consider the disjoint sets of behavioral features on session observations and transactional features on transaction observations.
For each of the two features/observations-pairs, we define \(N=1000\) learners for each of the three model categories as follows.
We fix N random sub-samples of features from the feature set. Each sub-sample remains fixed for all customers.
For each customer, we fix N random observation samples from the customer-specific sessions or transactions observations.
For each of the three model categories, for each customer, and for \(i=1,...,N\) , a base learner is defined by applying the model algorithm to the i -th features sub-sample and i -th observations sub-sample. Thus, this results in 6 N base learners per customer, 3 N for sessions, and another 3 N for transaction data.
The decisions for the three base learners are aggregated utilizing supervision, where the knowledge obtained from existing fraud cases is utilized to adjust the base learner weights.
Utilizing this representation, we train a model that (i) outputs an indicator of how likely a scheduled transaction is fraudulent, (ii) aggregates the overall provided decision functions to derive the unified hypothesis, while assigning more importance to learners that showcase the capability to better distinguish between fraud and non-fraud, and (iii) deals with a large imbalance in class representation.
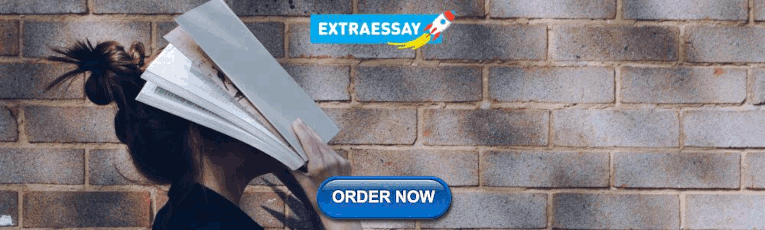
Validation results
The training, test, and validation sets consisted of data collected from July to October 2017, as dictated by the availability of online session log files. Around 900’000 sessions formed the dataset.
Raw data were processed using ETL to derive customer-specific feature representations of each recorded online session. The data were then split into non-fraud and fraud sets. The fraud set was not utilized to train the unsupervised base learners. Non-fraudulent (“healthy”) sessions were separated into training and test sets utilizing a 3-fold cross-validation split. We then sequentially trained the models on each derived training fold and computed the scores for observations in the corresponding test folds. Following, we obtained an out-of-sample decision function value for each healthy session and each base learner. We then assigned base learner scores to each fraudulent session utilizing base learners trained on all healthy data.
The out-of-sample logistic regression decision function values were aggregated by averaging within their respective ensembles (LOFs, IFs, and BDTs). This step yields a 3-dimensional representation of each customer’s online session. Finally, we utilized leave-one-out cross-validation to report the ROC curve measures. Hence, the logistic regression model is consecutively trained on an all-but-one observation, followed by computing the probability of an observation that was left out. Thus, we again obtain an out-of-sample fraud probability for each observation in the sample. We opted for leave-one-out cross-validation to maximize the number of fraudulent observations in each training set, because these are particularly scarce. Once we have obtained the aforementioned out-of-sample probabilities for each observation, we construct an ROC curve to display the FPR and TPR relationship depending on the decision threshold.
Resultantly, when utilizing no transaction data, the detection rate of the machine learning model was in a realistic range of 18% true positives. These primary results can be easily optimized to increase the TPR and simultaneously reduce the FPR utilizing different measures. This led to an increase in true positives by up to 45%, see Table 1 .
Overall, LOF seems to perform best over the entire dataset compared to IF and BDT. However, BDT has a slightly steeper ROC curve at the beginning, thus showing better pure outlier detection capabilities. Furthermore, because BDT seems to detect frauds, as discussed below, involving larger amounts than those detected by LOF, we cannot conclude that LOF outperforms the other approaches considered. Aggregating the decision functions of the ensembles utilizing simple means outperformed supervised aggregation. Through analysis of logistic regression weights assigned to each ensemble of learners, we determined that significantly higher weights were assigned to the LOF ensemble, most likely due to its best performance over the whole dataset. This dampened the input from the other two ensembles. However, this is not the case when the mean for aggregation is utilized. The results were affected by the small number of frauds and the size of the sample analyzed.
Different ensembles detected different types of fraud, and by observing figures depicting money saved per raised alarm, we see that different ensembles (LOF and BDT) detect different types of fraud cases, displayed by a large difference in saved money per trigger. Logistic regression supervision alarms were affected mainly by LOF, thus making it miss large embezzlement detected by the BDT ensemble. This motivates the triage model described in the next section. As of the restriction of the FPR to no greater than 2%, the entire ROC curve is of less interest. The ROC AUC values for the LOF ensemble is 0.93, for the BDT ensemble 0.82, and for the mean decision function ensemble 0.91.
We compared our results with those of Wei et al. ( 2013 ), and Carminati et al. ( 2015 ). These are two of the few studies dealing with online fraud detection that use real-world data, at least in part. Wei et al. ( 2013 ) utilized an unsupervised approach, whereas Carminati et al. ( 2015 ) utilized a semi-supervised approach. Table 2 compares the performance of our model with those of Wei et al. ( 2013 ) and Carminati et al. ( 2015 ). The results of this table should be interpreted with caution. First, different payment channels were considered. Second, the data of Carminati et al. ( 2015 ) were anonymized and did not include fraud cases. These are artificially added to tune \(1\%\) of the data volume, compared to \(0.018\%\) in Wei et al. ( 2013 ) and \(0.0012\%\) in our dataset. Third, Wei et al. ( 2013 ) did not report the FPR. Finally, Carminati et al. ( 2015 ) published an almost perfect error detection for scenarios I + II, but in scenario III, the false positives are too high; they generate too much manual work for the bank. The former scenarios are simple fraud scenarios that would be blacklisted and filtered out in our data before machine learning engages.
Fraud detection triage model
Formalization.
We formalize the triage model and denote by \(\Omega\) the set of all transactions with \(\omega\) as a single transaction, \(T(\omega )\) as the transaction amount function, and \(\chi _F(\omega )\) as the fraud indicator function, where \(\chi _F(\omega )=1\) represents fraud. Space \(\Omega\) is attributed to a probability distribution P with p ( x ) as the density function of the transaction amounts. The threshold value L of the fraud score function S is a function of the transaction amount x . We define:
If we assume stochastic independence of the transaction amount T , score S and fraud indicator \(\chi _F\) , we obtain the following interpretation:
Note that the assumptions of independence are strong, as transaction sizes are utilized as the input of the machine-learning model underlying the score. Conversely, the independence of \(\chi _f\) and T implies that the transaction amounts of fraudulent transactions have the same distribution as those of non-fraudulent transactions. In the context of the value-at-risk model in the next section, we argue that there is little evidence to support this. This considered, the assumption of independence is theoretically difficult to uphold, but in practice quite necessary to obtain our results.
We formulate our optimization problem as follows:
under the constraint of the integrated FPR
The expectation in ( 11 ) is the average cumulated sum of fraudulent transaction amounts detected by the detection model. By letting \(q_0:=E(\chi _F)\) and utilizing ( 10 ), we can rewrite it as
The constant \(q_0\) is irrelevant to the optimization. Setting \(g(x)=\text {FPR}(L(x))\) , we reformulate the optimization problem in terms of the ROC curve as
under the constraint:
To account for the recovery, we introduce a recovery function \(\theta :\Omega \rightarrow [0,1]\) . This function changes the objective function in the optimization problem, as follows:
whereas this constraint does not change.
Optimization
To put our formal model into practice, we need to fix a distribution for transaction amounts. Utilizing approximately 12 million transactions from online banking and 1.2 million from mobile banking, we approximated the distribution for both channels utilizing lognormal distributions. Although this choice does not particularly focus on the distribution’s tails, it will be seen that the optimal model still places strong emphasis on the detection of anomalies with large transaction amounts. Some basic statistics of the fitted distributions are given in Table 3 .
The ROC curve is conceptually the output of the detection model described in the previous section. However, owing to the limited number of actual fraud cases available, the TPR values for the given FPR levels are tainted with considerable uncertainty. The ROC curve utilized in our optimization was obtained by fitting a cubic spline function to the base points, as presented in Table 4 . The support points were adjusted to avoid unwanted spikes in the cubic interpolation.
As the triage model aims to prevent large losses with a higher probability than smaller ones, the optimal FPR will be an increasing function of the transaction size. To avoid possible optimization problems, we choose a simple form for FPR as a function of transaction size, namely, a piecewise linear function satisfying \(g(0) = 0\) , \(g(T_1) = a\) , \(g(T_2) = 1\) for the parameters \(a > 0\) and \(0< T_1 < T_2\) (see Fig. 1 ).
The optimization problem can be simplified by assuming equality in ( 12 ) and solving a as a function of \(T_1\) and \(T_2\) . For a target integrated FPR of 0.4%, we obtained the solutions listed in Table 5 .
Figure 1 illustrates the results for an online banking channel. The concave shape of the FPR curve up to \(T_2\) shows that the optimal solution emphasizes the detection of large transaction fraud cases, accepting, in turn, the less rigorous testing of small and moderate transactions up to \(T_1\) . For transaction amounts larger than \(T_2\) , FPR and TPR are equal to 1 by construction. Hence, all such transactions are automatically flagged as anomalies.
Total Effectiveness
is the average percentage of integrated fraudulent transaction amounts detected as anomalies. In our optimized case, the rate was 39%.
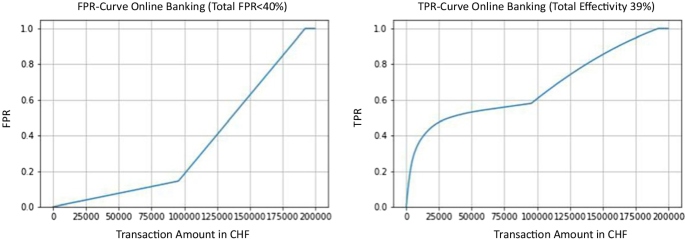
Panel Left: False positive rate as a function of the transaction amount under the constraint that the total false positive rate is smaller than 0.4 percent. Right Panel: True positive rate as a function of the transaction amount. The total effectiveness is 39 percent
Compound Poisson processes were utilized as basic building blocks. We utilize beta marginal distributions for modelling bounded transaction amounts and generalized Pareto marginal distributions (GPD) for unbounded ones. The so-called mass-attack model is formulated as a nested compound Poisson process with a marginal beta distribution. All subprocesses are aggregated independently. Loss statistics, such as value-at-risk or other quantiles of the distribution, are obtained by running Monte Carlo simulations.
Utilizing the limited available fraud data and drawing on discussions with practitioners, we develop the following model for online banking fraud:
Isolated attacks with a moderate transaction size of up to CHF 70’000 are modelled by a compound Poisson process with beta marginal distribution.
Isolated attacks with transaction amounts larger than CHF 70’000 are modelled by a compound Poisson process with GPD marginal.
“Mass attacks” are modelled as a nested compound Poisson process, where the inner Poisson process simulates the individual transactions triggered by the mass attack. The inner process has a beta marginal distribution and generates transaction amounts up to CHF 20’000.
The intensities of the Poisson processes constituting the submodels vary. In our case, isolated attacks of moderate size were by far the most frequent, followed by isolated attacks of large size. Mass attacks were the least frequent.
Mobile banking fraud is modelled analogously, albeit with transaction sizes only up to CHF 20’000, because larger amounts were inadmissible on this channel during our investigation. Hence, there is no Poisson process with GPD marginal in this case. Contrastingly, in the EBICS channel, which is an internet-based payment channel between banks, only the possibility of large fraudulent transactions was of interest. Hence, this model consists of a single compound Poisson process with GPD marginals above CHF 100’000. The details of the parametrization are given in Appendix A .
Countermeasures against fraud and recovery measures after fraud events play an essential role in determining risk potential. Therefore, they were integrated into the risk models. Countermeasures against online fraud fall into two categories: those that strengthen general infrastructure of the payment process to make it harder for attackers to find a weak spot, and those that are geared towards fighting off actual attacks. The first type is conceptually part of the base model described above, as it affects the frequency and possibly the transaction size of attacks. However, the second type is better understood in the context of recovery.
A recovery variable is introduced in the triage model, which accounts for it often being possible to recover money even after it has been transferred to another bank through fraudulent transactions. Conversely, by monitoring transactions utilizing the fraud detection and triage model, a certain percentage of attacks can be identified even before the transactions are released. The ROC curve of the detection model’s ROC curve, in combination with the triage model, allows us to infer the probability of detection from the transaction size: such that this component of the recovery process is readily integrated into the stochastic framework.
Owing to the nonlinearity of the risk statistics, the aggregation of the models was performed at the level of individual scenarios. Thus, for each scenario, \(s_i\) , the loss of the overall model for one-year was calculated from the simulated loss events of the channel models:
For each sub-model, the loss is calculated by pulling the event frequency for the year according to the Poisson intensity, loss magnitude according to the marginal distribution, and stochastic recovery:
where \(\text {Rec}\) denotes recovery function. Simulated loss figures were obtained by simulating the nested overall model, from which the risk statistics could be calculated empirically. Juniper Research ( 2020 ) estimated the recovery rate as \(18\%\) .
The simulation results for online banking are presented in Table 6 . The table shows the simulation results without applying fraud detection utilizing a constant FPR level of 0.4% and the triage model for an integrated FPR of 0.4%, respectively. In this simulation, no additional recovery was applied.
The above table shows the strong mitigation of risk due to fraud detection. The triage model performs better than the constant FPR benchmark in all submodels, particularly for the GPD submodel. Recall that the triage model places strong emphasis on detecting large fraudulent transactions, even flagging all transactions larger than CHF \(192'000\) .
As a second application, we compare the results of this risk model for the three e-channels with the bank’s overall 2019 risk policy. This means that we compare the capital-at-risk (CaR) limits for market and credit risks with operational risk limits, where the e-channel part is now calculated in our model. The following allocation of CaR holds according to the annual report of the bank Footnote 1 : Credit Risk, 69%; operational risk, 11%; market risk trading, 4%; market risk treasury, 11%; market risk real estate, 2%; and investment, 4%.
Approximately 1% of operational risk capital can be attributed to these three channels. Even if we add another 4–5% of the total volume to all payment services, including corporate banking and interbank payments, less than 10% of the operational risk capital is attributed to payment systems. As payment systems account for a significant portion of operational risk, our results confirm serious doubts about the accuracy of the chosen operational risk capital in banks. Without reliable models and data, capital is determined by utilizing dubious business indicators. Our models, which represent a micro-foundation of risk, show that, at least in payment systems, trustworthy risk quantities can be derived by combining machine learning and statistics.
Defense against sophisticated online banking fraud involve several resources and methods. These include risk models, algorithms, human action, knowledge, computer tools, web technology, and online business systems in the context of risk management.
We show that anomaly detection is not only useful per se, identifying a significant proportion of fraud while controlling false alarms, but that linking anomaly detection with statistical risk management methods can significantly reduce risk. A bank equipped with an anomaly detection system will be exposed to orders of magnitude of higher risks in payments than a bank implementing our end-to-end risk management framework with the three components of fraud detection, fraud detection optimization, and risk modelling.
As fraud is part of regulated operational risk, our model allows us to analytically capture these operational risks without crude benchmarking. This also provides a microeconomic foundation for capital adequacy. In the area of operational risk, these results put internal models that are not risk sensitive or difficult to verify on a solid footing.
A complicated problem, such as online payment fraud detection, requires a comprehensive understanding. A prerequisite for this is access to a large dataset. To evaluate our method, we utilized a real dataset from a private bank. Regardless of the chosen algorithm, feature extraction is an essential part of developing an effective fraud detection method. We utilized historically observed and confirmed fraudulent transaction identifiers as the ground truth. Each feature in the feature vectors for each e-banking session aims to encode deviations from normal customer behavior. Thus, behavioral, transactional, and customer-specific features are important.
Our framework opens interesting directions for future research. Roughly speaking, the framework goes in only one direction, from machine learning methods in fraud detection to statistical risk modelling. The feedback process from the risk model to the triage model and from the triage model back to the fraud detection model is a challenging task that can be addressed utilizing reinforcement-learning methods. With such a feedback loop, the entire risk-management framework becomes a learning system. Another research direction is to extend the optimization of fraud detection (triage model) by considering transaction-dependent loss risks and other features such as customer segmentation. More emphasis is placed on segments that are known or suspected to be less alert or more vulnerable to fraudulent attacks. This resulted in a higher-dimensional triage model.
Availability of data and materials
The bank provided real transaction data and data on transactions (“raw data”) of the customers. The legal basis of the Swiss Federal Data Protection Act (2020) prevents the raw data from leaving the bank in any form or being accessible to any party other than the bank.
CaR for credit risk is VaR on the bank’s quantile level and for market risk CaR was in the past chosen on an annual basis and a risk budgeting process was defined to align present risk with the annual risk budget.
Abbreviations
Swiss Franc
Receiver operating curve
Area under the ROC curve
True positive rate
False poistive rate
Bagged decision tree
Local outlier factor
Isolation forest
Value-at-risk
Generalized pareto distribution
Payment channel for corporate banking clients
Extract, transform, load is a three-phase process where data is extracted, transformed and loaded into an output data container
Abdallah A, Maarof MA, Zainal A (2016) Fraud detection system: a survey. J Netw Comput Appl 68:90–113
Article Google Scholar
Ali A, Shukor AR, Siti HO, Abdu S (2022) Financial fraud detection based on machine learning: a systematic literature review. Review Appl Sci 12:9637
Amiri M, Hekmat S (2021) Banking fraud: a customer-side overview of categories and frameworks of detection and prevention. J Appl Intell Syst Inf Sci 2(2):58–68
Google Scholar
Aggarwal CC, Sathe S (2017) Outlier ensembles: an introduction. Springer
Bessis J (2011) Risk management in banking. Wiley, New York
Bolton RJ, Hand DJ (2002) Statistical fraud detection: a review. Stat Sci 17(3):235–249
Bolton RJ, Hand DJ (2001) Unsupervised profiling methods for fraud detection, Credit Scoring and Credit Control VII, pp 235–255
Breunig MM, Kriegel H-P, Ng RT, Sander J (2000) LOF: Identifying density based local outliers. In: Proceedings of the 2000 ACM SIGMOD international conference on management of data, pp 93–104
Carminati M, Caron R, Maggi F, Epifani I, Zanero S (2015) BankSealer: a decision support system for online banking fraud analysis and investigation. Comput Secur 53:175–186
Chandola V, Banerjee A, Kumar V (2009) Anomaly detection: a survey. ACM Comput Surveys 41(3):1–58
Embrechts P, Klüppelberg C, Mikosch T (2013) Modelling extremal events: for insurance and finance (Vol 33). Springer Science & Business Media
FCA (2021) Financial conduct authority handbook. www.handbook.fca.org.uk
Fei Tony L, Kai T, Zhi-Hua Z (2008) Isolation forest. In: 2008 Eighth IEE E International Conference on Data Mining, IEEE, pp. 413-422
Haixiang G, Yijing L, Shang J, Mingyun G, Yuanyue H, Bing G (2017) Learning from class-imbalanced data: review of methods and applications. Expert Syst Appl 73:220–2
Hilal W, Gadsden SA, Yawney J (2021) A review of anomaly detection techniques and applications in financial fraud. Exp Syste Appl 116429.
Hilal W, Gadsden SA, Yawney J (2022) Financial fraud: a review of anomaly detection techniques and recent advances. Expert Syst Appl 193:11
Jung E, Le Tilly M, Gehani A, Ge Y (2019, July) Data mining-based ethereum fraud detection. In: 2019 IEEE international conference on blockchain (Blockchain) (pp 266-273). IEEE
Juniper Research (2020) Online payment fraud: Emerging threats, segment analysis and market forecasts 2020-2024. www.juniperresearch.com
KPMG (2019) Global banking fraud survey, KPMG International
Kou G, Xu Y, Peng Y, Shen F, Chen Y, Chang K, Kou S (2021) Bankruptcy prediction for SMEs using transactional data and two-stage multiobjective feature selection. Decis Support Syst 140:113429
Krawczyk B (2016) Learning from imbalanced data: open challenges and future directions. Prog Artif Intell 5(4):221–232
Li T, Kou G, Peng Y, Philip SY (2021) An integrated cluster detection, optimization, and interpretation approach for financial data. IEEE Trans Cybern 52(12):13848–13861
Liu FT, Ting KM, Zhou ZH (2008) Isolation forest. In: 2008 eighth IEEE international conference on data mining, pp 413-422. IEEE
Liu FT, Ting KM, Zhou ZH (2012) Isolation-based anomaly detection. ACM Trans Knowl Discov Data (TKDD) 6(1):1–39
McNeil AJ, Frey R, Embrechts P (2015) Quantitative risk management: concepts, techniques and tools-revised edition. Princeton University Press, Princeton
Montague DA (2010) Essentials of online payment security and fraud prevention, vol 54. Wiley, New York
Molloy I, Chari S, Finkler U, Wiggerman M, Jonker C, Habeck T, Schaik RV (2016) Graph analytics for real-time scoring of cross-channel transactional fraud. In: International conference on financial cryptography and data security, pp 22–40. Springer, Berlin, Heidelberg
Pang G, Shen C, Cao L, Hengel AVD (2020) Deep learning for anomaly detection: a review. arXiv preprint arXiv:2007.02500
Piotr J, Niall AM, Hand JD, Whitrow C, David J (2008) Off the peg and bespoke classifiers for fraud detection. Comput Stat Data Anal 52:4521–4532
Power M (2013) The apparatus of fraud risk. Account Organ Soc 38(6–7):525–543
Sabu AI, Mare C, Safta IL (2021) A statistical model of fraud risk in financial statements. Case for Romania companies. Risks 9(6):116
Shen H, Kurshan E (2020) Deep Q-network-based adaptive alert threshold selection policy for payment fraud systems in retail banking. arXiv preprint arXiv:2010.11062
Singh A, Ranjan RK, Tiwari A (2022) Credit card fraud detection under extreme imbalanced data: a comparative study of data-level algorithms. J Exper Theor Artif Intell 34(4):571–598
Tokovarov M, Karczmarek P (2022) A probabilistic generalization of isolation forest. Inform Sci 584:433–449
Trozze A, Kamps J, Akartuna EA, Hetzel FJ, Kleinberg B, Davies T, Johnson SD (2022) Cryptocurrencies and future financial crime. Crime Sci 11(1):1–35
Van Liebergen B (2017) Machine learning: a revolution in risk management and compliance? J Financ Trans 45:60–67
Vanini P (2022) Reinforcement Learning in Fraud Detection, Preprint University of Basel
Wei W, Li J, Cao L, Ou Y, Chen J (2013) Effective detection of sophisticated online banking fraud on extremely imbalanced data. World Wide Web 16(4):449–475
West J, Bhattacharya M (2016) Intelligent financial fraud detection: a comprehensive review. Comput Secur 57:47–66
Zhang W, Xie R, Wang Q, Yang Y, Li J (2022a) A novel approach for fraudulent reviewer detection based on weighted topic modelling and nearest neighbors with asymmetric Kullback-Leibler divergence. Decis Support Syst 157:113765
Zhang G, Li Z, Huang J, Wu J, Zhou C, Yang J, Gao J (2022b) efraudcom: An ecommerce fraud detection system via competitive graph neural networks. ACM Trans Inform Syst (TOIS) 40(3):1-29.
Zhou ZH (2012) Ensemble methods: foundations and algorithms. CRC Press, Boca Raton
Book Google Scholar
Download references
Acknowledgements
The authors are thankful to P. Senti, B. Zanella, A. Andreoli and R. Brun all from Zurich Cantonal Bank for the discussions and for providing us with the resources to perform his study. The authors are grateful to P. Embrechts (ETH Zurich) for the model discussions.
This research received no specific grant from any funding agency in the public, commercial, or not-for-profit sectors.
Author information
Authors and affiliations.
University of Basel, Basel, Switzerland
Paolo Vanini
Novartis AG, Basel, Switzerland
Sebastiano Rossi
swissQuant Group, Zurich, Switzerland
Ermin Zvizdic
IT Couture, Zurich, Switzerland
Thomas Domenig
You can also search for this author in PubMed Google Scholar
Contributions
Sebastiano Rossi and Ermin Zvizdic designed the fraud detection model and analysed the data. Thomas Domenig and Paolo Vanini designed the triage model. Thomas Domenig designed the risk model and did the calculations for the triage and risk model. Paolo Vanini wrote the manuscript with contributions from all authors. Paolo Vanini was involved in the analysis of the triage and risk model. Ermin Zvizdic led the project in the bank. All authors read and approved the final manuscript.
Corresponding author
Correspondence to Paolo Vanini .
Ethics declarations
Competing interests.
The authors declare that they have no competing interests.
Additional information
Publisher’s note.
Springer Nature remains neutral with regard to jurisdictional claims in published maps and institutional affiliations.
The structure of our ensemble results from several design decisions based on both insights from the field (online banking) and experience in building machine learning models. Our ensemble model was built according to the following guidelines:
Customer-specific models The features used in our approach encode patterns in customers’ session behaviour and transactions. These patterns vary widely from client to client, which is why we chose to use a client-specific model rather than a global model.
Global feature space Although behaviours vary, we have chosen to use the same feature representation for each session/transaction, which allows us to assign weights to specific (model, feature) pairs based on their performance across all clients. This in turn allows for consistent scoring across all clients and information sharing between clients when fraudulent activity occurs. In other words, our approach makes it easy for learners to adjust their weights.
Separation of models based on feature type We have chosen to form separate ensembles, one based on behavioural features and one based on transactional features, rather than concatenating all features into a single vector and forming a single ensemble based on concatenation. This ensures better interpretability and reduces the likelihood of constructing nonsensical feature subspaces during feature bagging.
Modified Bootstrap aggregation (Bagging) To build an ensemble of weak learners, we use a modification of bootstrap aggregation (bagging). Bagging is a meta-algorithm for ensembles that is used to reduce the variance and improve the stability of the prediction as well as to avoid overfitting.
Bagging Pipeline
Observational sampling (bagging): Bagged ensembles for classification generate additional data for training by resampling with replacement from the initial training data to produce multiple sets of the same size of initial training data, one for each base learner. This is done to reduce the prediction variance. For the two outlier detection ensembles, we used variable subsampling (without replacement) to avoid problems associated with repeated data and to mimic random selection of the neighbourhood count hyperparameter (cf. Aggarwal and Sathe 2017 ).
Feature bagging: An important task in outlier detection is to identify the appropriate features on which to base the analysis. However, these features may differ depending on the fraud mechanism. Therefore, instead of pre-selecting features, a more robust approach is to create an ensemble of models that focus on different feature sets and assign different weights to the models that use different features depending on their performance. The procedure is applied to each base learner \(b_j\) as follows:
Randomly select a number \(r_{b_j }\) in range \([ d/10,d-1]\) , where d denotes the feature dimension.
Sample a subspace of features of size \(r_{b_j }\)
Train the base learner \(b_j\) on the sampled subspace.
No Hyperparameter bagging: Due to limited fraud, tuning the hyperparameters via validation may lead to overfitting. For this reason, similar to feature selection, we could instead randomly select a set of different hyperparameters. In our case, however, IF and BDT are not expected to be sensitive to the choice of hyperparameters, and resampling the hyperparameter from LOF would be redundant to the subsampling of the data performed. We therefore set all hyperparameters to reasonable ones found in the literature.
Sharing bagged features and parameters across customers : Subspaces for sampled features and parameters for local outlier factors are shared between all client models and all types of base models (manifested in the respective weak learners). This allows, for example, the introduction of supervision in the aggregation step and increased interpretability of the model, as it is easier to identify features relevant to the detection of certain types of fraud.
Model aggregation: Each base model provides a decision function \(\delta (x)\) for a given observation x . The base model ensemble directly aggregates (majority voting or averaging) the weak learner results based on different subsamples to form a single hypothesis that determines the class membership of an observation. Usually, this aggregation is directly extended after a normalisation step to include models of different types, parameters or feature groups. In our approach, however, this final aggregation is performed based on a monitoring step that uses knowledge of available frauds to assign different weights to each pair (model, feature set). Essentially, these weights quantify how appropriate each model and feature pair is for fraud detection.
We refer to a composite Poisson process whose marginal distribution corresponds to a beta distribution as a beta model or as a GPD model if the marginal distribution corresponds to a generalised Pareto distribution. The mass attack model is a nested compound Poisson process. The outer Poisson process models the mass attack event, while an inner Poisson process models the number of affected transactions. The extent of damage of the individual affected transactions is modelled with a beta distribution.
Online banking:
Beta model: Intensity 35, \(\alpha =0.42, \beta =2.4\) and \(\text {scale}: 71.000.\) By shifting and scaling, explicitly by the transformation \(x\rightarrow \alpha + (\beta -\alpha )x\) , the beta distribution is shifted from [0, 1] to the interval \([\alpha ,\beta ]\) . The parameter \(\alpha\) is called location, and \(\beta -\alpha\) scale.
GPD model: Intensity 3, \(\text {shape}=0.25, \text {location}=60.000, \text {scale}=100.000\) .
Mass Attack model: Intensity 0.1, intensity nested model 1000, Beta model \(\alpha =0.42, \beta =2.4\) and \(\text {scale}: 20.000.\)
Mobile banking:
Beta model: Intensity 45, \(\alpha =0.42, \beta =2.4\) and \(\text {scale}: 20'000.\)
Mass Attack model: Intensity 0.1, intensity nested model 1000, Beta model \(\alpha =0.42, \beta =2.4\) and \(\text {scale}: 20'000.\)
GPD model: \(\text {shape}=0.25, \text {location}=100'000, \text { scale}=300'000\) .
Recovery model:
The recovery model models the percentage recovery in a fraud case. It has the following form:
With a probability \(p_1=65\%\) , a complete recovery is simulated, i.e. no damage remains. This resulted from the fact that in the 159 fraud cases considered, it was actually possible to reduce the loss amount to zero even in \(80\%\) of the cases.
With a probability \(p_2=18\%\) , a recovery of zero is simulated, i.e. the damage corresponds to the full amount of the offence.
With probability \(1-p_1-p_2\) , a recovery between 0 and 1 is simulated. A beta distribution is chosen as the distribution of these partial recoveries.
The beta distribution parameters for the online banking channel were fitted on the fraud cases recorded from 13/03/2013 to 13/03/2018. These are 159 fraud cases, of which both the initial fraud transaction amounts and the effective loss amount, i.e. the residual amount after recovery, were recorded. Of the 159 cases, 152 have a fraud amount between CHF 0 and 60,000, while the remaining 7 fraud amounts range between CHF 100,000 and 300,000. A beta distribution was fitted on the 152 cases with fraud amounts up to 60,000 CHF, whereby the scaling parameter, i.e. the upper limit of the distribution, was defined as a free parameter of the fitting procedure and estimated by it to be 71,000 CHF. Similar procedures apply to the marginal distribution fits of the GPD and mass attack models.
There exists significant statistical uncertainty and variability in the driving forces of the defined models. Putting the intended flexibility of the model structure into practice, we distinguish between ’easily accessible’ parameters, which should be subject to discussion at any time in the context of risk assessments, and ’deeper’ parameters, whose mode of action is less obvious and whose adjustment is subject to the process of model reviews. Roughly speaking, Poisson intensities, which determine the expected frequency of events, as well as upper and lower boundaries of the marginal distributions belong to the former category, while shape parameters for the Beta and GPD marginal distributions belong to the latter.
Rights and permissions
Open Access This article is licensed under a Creative Commons Attribution 4.0 International License, which permits use, sharing, adaptation, distribution and reproduction in any medium or format, as long as you give appropriate credit to the original author(s) and the source, provide a link to the Creative Commons licence, and indicate if changes were made. The images or other third party material in this article are included in the article's Creative Commons licence, unless indicated otherwise in a credit line to the material. If material is not included in the article's Creative Commons licence and your intended use is not permitted by statutory regulation or exceeds the permitted use, you will need to obtain permission directly from the copyright holder. To view a copy of this licence, visit http://creativecommons.org/licenses/by/4.0/ .
Reprints and permissions
About this article
Cite this article.
Vanini, P., Rossi, S., Zvizdic, E. et al. Online payment fraud: from anomaly detection to risk management. Financ Innov 9 , 66 (2023). https://doi.org/10.1186/s40854-023-00470-w
Download citation
Received : 22 March 2022
Accepted : 18 February 2023
Published : 13 March 2023
DOI : https://doi.org/10.1186/s40854-023-00470-w
Share this article
Anyone you share the following link with will be able to read this content:
Sorry, a shareable link is not currently available for this article.
Provided by the Springer Nature SharedIt content-sharing initiative
- Payment fraud risk management
- Anomaly detection
- Ensemble models
- Integration of machine learning and statistical risk modelling
- Economic optimization machine learning outputs
M-Commerce Offline Payment
- Original Research
- Published: 14 December 2021
- Volume 3 , article number 100 , ( 2022 )
Cite this article
- Yash Kumar Gupta ORCID: orcid.org/0000-0002-3069-6999 1 ,
- Girish Jeswani 1 &
- Olan Pinto 1
4318 Accesses
3 Citations
Explore all metrics
During the ongoing COVID-19 Pandemic we have seen a surge in online transactions. The current wallet payment architecture requires online connectivity during transactions. It is observed that there is a possibility of not getting connected to the Internet due to various reasons. We are working on a solution to enable offline transactions due to any internet failure. We identify three main scenarios where fully offline transaction capability is considered to be beneficial for both merchants and consumers. It includes making purchases in locations without online connectivity, when a reliable connection is not guaranteed, and when it is cheaper to carry out offline transactions due to higher communication/payment processing costs involved in online approvals. Our work aims to address the challenge of providing secure offline transaction capability when there is no online connectivity keeping in mind to not compromise any security features in our M-Commerce offline wallet payment application.
Similar content being viewed by others
SOLDI: Secure Off-Line Disposable CredIts to Secure Mobile Micro Payments
An e-payment Architecture Ensuring a High Level of Privacy Protection
New Security Protocols for Offline Point-of-Sale Machines
Avoid common mistakes on your manuscript.
Introduction
Mobile payment is the root of financial transactions for most of the services or goods. The majority of the payment system currently in use considers online communication with the network and is very infrastructure dependent, which is very different from wireless mobile Adhoc systems. Payment failure is not an uncommon phenomenon these days, due to insufficient balance or just a lack of internet connectivity. Ensure The customers are unable to establish a connection with the payments platform is also a major reason for online payment failure. However, with the proposed application, end-users will be able to make offline payments using their mobile devices(wallet). The RBI proposed a pilot scheme for small value payments in offline mode with built-in features for safeguarding the interest of the users, liability protection, etc. Under the pilot scheme, banks and payment System Operators will be able to provide offline payment solutions using cards, wallets, or mobile devices for remote or proximity payments. This paper looks into a hybrid online-offline wallet payment system, the online system developed enhances the capability of the current system being used. The offline model will enable users in regions of unstable connectivity, devoid of internet, to successfully onboard in this venture to eliminate hard Cash Transactions.
The upcoming Sect. " Literature Survey " discusses the nature of the present work and compares it to our approach. In Sect. " Methodology " we briefly discuss the methods involved in our proposed approach and how we deal with the security of payments. Section " Results and Discussion " depicts the results obtained and reviews the working of the proposed application.
Literature Survey
The author [ 1 ] addresses a challenge in providing secure offline transaction capability when there is no online connectivity on either the mobile or the terminal. It discusses how tokenization can be adopted by the payment industry as a method to prevent Personal Account Number (PAN) and compromise in EMV (Europay MasterCard Visa) transactions, differentiated how the current architecture specified in EMV tokenization that requires online connectivity during transactions. A new privacy-preserving offline mobile payment protocol was designed and was based on NFC. The primary aim was to focus on customer privacy-preserving for mobile payment. This scheme was well designed to accomplish all security and privacy requirements. In this scheme, a group signature is employed to provide the unlinkability of the transactions [ 2 ]. The designed prototype included several security features like anonymity, unlinkability, unforgeability, replay attack prevention, traceability etc.
A novel approach for building secure, scalable, and flexible e-payment systems in offline mode of communication for enhanced security on transactions [ 3 ]. They discussed how the current payment systems are directly dependent on the fixed infrastructure of the network (cellular network), which fails to facilitate an optimal level of security for the payment system. The proposed system is based on a simple public key infrastructure for providing security in payment processes. The performance analysis of the proposed model was analyzed and showed that the system is highly robust and secure ensuring anonymity, privacy, non-repudiation offline payment system over wireless Adhoc network. The author [ 4 ] analyzed the characteristics of online payment, gives a common online payment system model with emphasis on the discussion of the electronic credit card, electronic cash, and electronic check of three online payment modes, and finally points out the respective application environment. Advances in technology make electronic cash, electronic wallets, online banking, electronic exchange and other methods of payment continue to be mature and put into commercial use.
A method was proposed in [ 5 ] based on an e-cash system with multiple denominations that enable e-merchants to give customers change when in an offline environment. They proposed a system that results in convenient transactions regardless of the transaction amount and reduces the number of e-cash users needed to deposit in advance. They used various security measures namely: unlinkability, verifiability, unforgeability, double spending detection, tamper resistance, and nonrepudiation to provide security while performing the transaction. Open service-oriented architecture for secure mobile transactions was described in [ 6 ]. The system was established as a large-scale, secure and complete system comprising several components. It involved mobile network operators, banks, credit card processors, small merchants. The aim was to design a more flexible way to use cash and credit cards securely.
A new prepaid multihop (transferable) cash payment system solution based on hardware technology implementing an electronic wallet (E-wallet) to accommodate digital coins was discussed [ 7 ]. The result is a peer-to-peer (P2P) electronic cash transfer equivalent to a physical cash transfer in public use. This paper also incorporated an offline mode of wallet payment but the drawback is that the system needs still a trusted authority which should act as a catalyst to enhance the trust and possible abuse traceability. The authors in [ 8 ] outlined the digital wallets’ threats and discussed the requirements of digital wallets that technically addressed the fear of security to customers as usual providers while assisting in the successful implementation of digital wallets. They discussed interlocking between digital wallet user threats and requirements. It also discusses the key security requirements that successful wallet payments need to ensure the users’ safety.
The authors discussed how Micro-payment systems have the potential to provide non-intrusive, high-volume, and low-cost pay-as-you-use services for a wide variety of Web-based applications [ 9 ]. It describes a set of extensions, mobile NetPay, optimized for mobile e-commerce. Mobile NetPay provides high performance and security using one-way hashing functions for e-coin encryption. The authors [ 10 ] proposed the importance of offline payment solutions, as people tend to behave carelessly in choosing their passwords to avoid the burden of memorizing complex passwords. Such frail password habits are at severe threats to various services available online, especially electronic banking or e-banking. For eradicating the necessity of creating and managing passwords, a variety of solutions are prevalent, the traditional ones being the usage of One-Time-Password (OTP) that refers to a single session/transaction password.
Paper [ 11 ] discusses the security of electronic payment systems. This paper focuses on either dominant systems or new attempts and innovations to improve the level of security of electronic payment systems. The survey consists of the Card-Present (CP) transactions and a review of its dominant system i.e. EMV. Several pieces of research at Cambridge university show variants of attacks against this standard, which demonstrates the lack of a secure offline authentication method. This paper proposes the use of smart cards instead of magnetic stripe cards which are not able to participate in the authentication process the evaluation of the EMV migration from RSA cryptosystem to ECC based cryptosystem 3.
Mobile payments offer a great wireless network in e-commerce [ 12 ]. This paper discusses the problems such as supporting macro payments, offline transactions, improving the validity of payment in existing e-commerce modes based on mobile payments. This paper puts forward an offline transaction e-commerce system model based on mobile payment which includes the offline POS terminal, a mobile device, and a payment center. In this model, the transaction voucher, a random generated ID on the offline POS terminal is signed by the digital signature technology on the payment center to generate the payment confirmation. The digital signature verification of payment confirmation, which applies the emerging ID-based cryptography for key agreement and authentication, is the guarantee of validity in offline transactions. The offline transaction model based on mobile payment saves the costs of wiring and also makes the transaction process more convenient. They propose a fully offline payment technique which is a great alternative for e-commerce payments. However, it is limited to customer–merchant transactions and requires additional hardware like the POS terminal. Using mobile wallets instead can provide flexibility for day-to-day transactions as well.
The authors in [ 13 ] provide a comprehensive review of secure mobile payment where classification of mobile payment into TPC(third-party payment company)-led mobile payment and Bank-led mobile payment, and based on this, summarize the system structure of mobile payment was performed. The research conducted introduces secure technologies(hardware and software) used in these procedures, discusses and analyzes the security issues that they have been encountered, summarise open issues, and proposes future development directions
This research [ 14 ] considers earlier literature and consumer conduct through ground-level overviews and interviews. They likewise investigate the cryptic challenges looked by clients of various age groups towards a complete acknowledgment of digital payments and modes. As a result of these discoveries, an improvement scope has been talked about wherein a few favorite ideas have been made, including, digital biometric authentication, a coalition of various digital payment platforms, implicit redressal, and shared wallets.
Paper [ 15 ] highlights the previously existing mobile device features like short message services and bluetooth that have the capability to perform digital money transactions in an offline environment. The methodology revolves around the fact that e-paycards can be used to send or receive digital money with the existing functionality of mobile devices like messaging and bluetooth to perform financial transactions without delay even when the e-wallet server is not responsive at a given time. The papers findings suggest that e-paycards can be used by merchants and customers and that customers can send these e-paycards to the merchant through any mobile device messaging functionality or through bluetooth.
This paper [ 16 ] introduces a concept of hard wallet for generating trust. Payment that is issued from the hard wallet can be consumed by a second hard wallet, which will further pay to a third hard wallet thereby creating a payment cycle or ecology of digital money for long periods without the use of a telecommunication channel. The hard wallet approach may be personalized and fitted with ownership security capability.
This paper [ 17 ], implemented a novel protocol where the proposed offline e-payment scheme satisfies all essential security requirements through simplified computations. In the proposed model, blind signatures ensure the anonymity of the customer sensibly and directly. In doing so, the customer is kept anonymous as long as they are not liable for multiple spending. The implemented protocol takes care of multiple-spending detection, untraceability between the e-coin and its customer, no-swindling, fraudster control, unforgeability of the e-coin, no impersonation, no framing attack.
Paper [ 18 ] develops a novel framework which includes features like the lowcost technology, easy implementation and operability, usefulness to local people, and scope for creative appropriation. The aim of the paper was to conduct an in-depth field study through interviews with merchants and consumers to evaluate the framework. The developed framework suggested that along with the features like low-cost, easy installation and operability, customers reported that the mobile payment technology, unlike cash transactions, can also afford creative uses such as reflection on past expenses. The research done on this paper reveals developmental assertions towards the need for greater inclusion of local small-scale vendors.
This paper [ 19 ] conducted a thorough research and explored multiple proposed models of payment systems through mobile devices, their payment methods, security mechanisms, technologies and comparison mechanisms involved in mobile payment systems. The paper also provides an analysis of the encryption technologies, authentication methods and firewall in mobile payment system.
This paper [ 20 ] discusses in detail the different architectures of mobile payment available. Although mobile payment has gained its popularity in many regions due to its convenience, it also faces many threats and security challenges. This paper presents a mobile payment processing model and introduces each type of mobile payment systems. It summarizes the security services desired in mobile payment systems and also the security mechanisms which are currently in place. It further identifies and discusses three security threats, i.e., malware, SSL/TLS vulnerabilities, and data breaches, and four security challenges, i.e., malware detection, multi-factor authentication, data breach prevention, and fraud detection and prevention, in mobile payment systems.
Paper [ 21 ] reviews the security issues involved with electronic payments. The paper focuses its research on online payments as well as E-wallets. This research reviews around 131 research papers concerned with electronic payments in between 2010 ans 2020. It uses a systematic approach to its literature review. The results obtained show that interest in E-wallet and online payment has grown significantly during 2010–2020 period. The paper proposes that the underlying issues with security of online payments should have some protection properties such as authorization, availability, integrity, authentication etc.
This paper [ 22 ] compares existing literature regarding mobile payments to enhance understanding of the current state of knowledge. This research paper discusses in detail the peer reviewed surveys available in the Scopus database between the period of 2005 and 2020. About 56 studies published in 44 international journals were used for a systematic literature search in the Scopus database. The paper also conducts a bibliometric analysis with the VOS viewer to identify thematic clusters. Overall the paper provides a decent understanding of mobile payment architectures and based on the results future avenues were also proposed.
After thorough research on existing work, many methods are available for the secure mobile transactions through online. The usage of OTPs, tokenization, EMVs for the secure transactions were discussed. Using this as a base, we came up with a solution considering security at high level for the mobile payments. The main drawback in the literature was the lack of offline payments implementation. The idea of offline payments is brought up in literature but none of them have created an end to end offline mobile payment system. We considered this concept for the implementation of safe and secure online-offline payment system (Table 1 ).
Methodology
A novel application that incorporates an M-Commerce wallet payment system in an offline environment. This application was developed with a particular goal that is to enhance and improve the current wallet payment system between a customer and a merchant without compromising any security features (Fig. 1 ). The application incorporates online as well as offline payment methods. To carry out this, a well designed M-Commerce payment architecture was developed to prevent any security threats (Fig. 2 ).
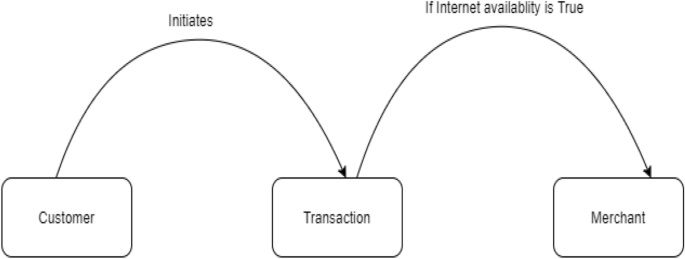
Online scenario flowchart
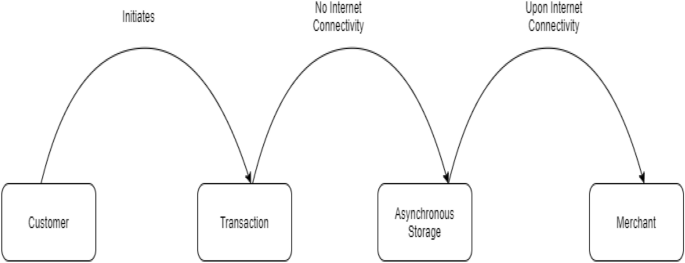
Offline scenario flowchart
In the case of online payments, the first step is a user login page, that has a safe API protocol whereby the end users credentials are hashed and stored in the database. The login page is essential for user identification and to prevent identity theft. As a developer, we need to keep user data as safe as possible even in the situation of a data breach, hence hashing key user information is crucial.
We need a promise-based HTTP client that returns promises rather than accepting callback. Axios is a popular, promise-based HTTP client that supports an easy-to-use API and can be used in both the browser and Node.js. This ensures handling asynchronous calls without creating messy code. Besides that, it wraps the requests using a polyfill for ES6 new promise syntax. We can also intercept and cancel requests, and there is a built-in client-side protection against cross-site request forgery.
Next, there is a homepage that takes care of the basic requirements of the user which are all accessible with a single tap. For a mobile wallet the most important information are the basic details of the user, the balance, associated bank details, and transaction history of the user. All of this information can be easily retrieved using secure API connection’s in an online scenario. For the offline mode, these details are equally important and need to be displayed for the user to continue using the application. These details are stored in an offline environment using asynchronous storage. Asynchronous Storage is a storage system that developers can use and save data in the form of key-value pairs which is very similar to a browsers local storage API. It alleviates the need to rely on heavy duty servers and external databases. This form of storage does not delay the loading of the app and can be quicker than simple data fetching. The key advantage of using asynchronous Storage over cookies and cache is that it limits the amount of data traveling back-and-forth to the server ensuring low latency.
The user can then navigate to the transaction page from the homepage. The transaction between two users is the most important part of the app and security must be a top priority in such payment apps. Given the already existing safety issues of API calls these days, we wanted to create a more secure channel/tunnel where only the concerned client and server can interact. With recent security issues, writing information using API calls can be a risk with hacker’s being able to modify POST requests. The client first tries to establish a Web-Socket connection if possible, and if not, the app initiates an offline mode transaction. In the situation where the customer has no internet connection, identified by NetInfo we make use of the asynchronous storage that react native has to offer to store a logged users information until the customer gets back online. After the customer gets a stable internet connection, the data is picked up from the asynchronous Storage and a secure socket connection is made from the homepage and is connected to the server and the transaction continues as it would similar to the online scenario.
For concurrency, we tried incorporating MongoDB(s) multi-granularity locking that allows operations to lock at the global, database, or collection level and allows for individual storage engines to implement their own concurrency control below the collection level. But for our system implementation, we needed a row-level lock rather than a table-level lock. A table-level/collection level lock would slow down the whole user experience. The pipeline would block off incoming transactions and cause a delay. Hence we have implemented a document level for the application, which locks only a particular user enabling other users to continue with their transactions. Once the connection is established, any kind of communication between the client and the server will be made through this socket connection. After the connection is established, the user can make a transaction by providing the details of the merchant and the amount that has to be transferred.
After the transaction is complete, the user is redirected to a transaction confirmation page, post which they are redirected to the homepage from the payment gateway. From the homepage, the user can either continue making new transactions or can close the application.
To cater to the users needs and at the same time not make the application too complex for common users, we have worked on a system which enhances the capability of the current system being used. The online-offline application developed would ensure that end users will be able to have a full-fledged mobile wallet application at all times irrespective of the strength of their internet connectivity. This is an advancement to the current mobile wallet system being used in the market and provides a suitable replacement for online applications.
Results and Discussion
Based on the above-mentioned methodology an application has been created. The application is fully functional in both online and offline modes and security measures have been applied for both.
The M-Commerce offline payment application opens to a login page that asks the concerned customer or merchant to enter their credentials i.e, username and password. For added security, all passwords are hashed and stored. This initial setup is done when the user is online. Once this is done the details are hashed and stored in the device’s asynchronous storage. The data from the asynchronous storage cannot be tampered with and is safe for the user. This is subsequently used for all offline payments and ensures the safety of user data.
On creating a POST request via the route [/customers] the username is matched with the database and if matched, a response is sent to the client indicating that a customer with those credentials already exists, else a new customer is created and the procedure for it is as follows. First, the password entered by the end-user is hashed using NodeJS’ bcrypt library that takes in two parameters, one, the password to be encrypted and two, the salt to be used to hash the password, and if specified as a number, a salt will be generated with the specified number of rounds. Thereafter the end-users balance, associated bank, and phone number are recorded into the collection.
The first page of the app is the login page as seen in Fig. 3 . Here, the user enters their credentials that have been stored in the database. The entered credentials are matched with the database and the user is allowed to login if they are correct. The login works through the routes set up through Express. The POST request [/customers/login] is created, after which the app moves on to the next page. The way the route works is it first checks if the username exists in the database and then hashes the entered password and matches it with the database. Upon successful verification, the user is allowed to use the App.
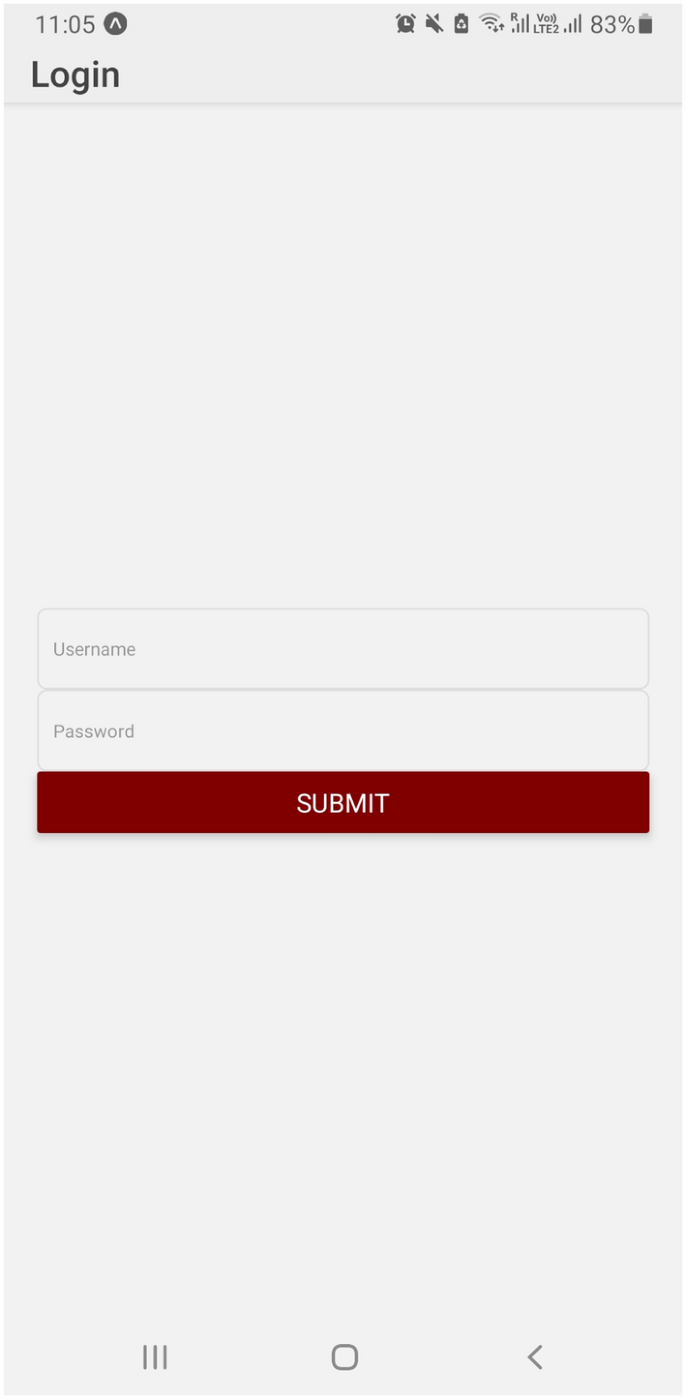
User Login page
Upon successful authentication, the end-user logs in and are redirected to the client’s homepage where the client would be able to see their remaining balance, their associated bank, and a history of the last five transactions made by them via this application. In the case the customer has a stable internet connection, clicking on the make payment button will take the user to the transaction page that is when a socket connection is established with the server, and once this connection is established, any other communication between the client and the server will take place through this socket. We have also coded an additional document level lock for Mongo DB, which ensures that multiple users can make a transaction at a given time without locking the whole collection. It works by locking the rows returned by a selection query, such that other transactions trying to access those rows are forced to wait for the transaction that locked the rows to finish. These other transactions are effectively put into a queue based on when they tried to read the value of the locked rows. The application takes care of concurrency by ensuring that a document/row-level lock is created so that the application does not contain any write/write and read/write conflicts. Once the server-side processing of the transaction is complete, the status of the transaction is sent back to the client through the same socket.
Figure 4 shows the Homepage of the App. The client details such as Username, associated bank account, and the current balance are displayed on this page. Along with such details, the transaction history of the client is displayed as well, which shows the last five transactions of the user, green depicting incoming and red depicting outgoing.
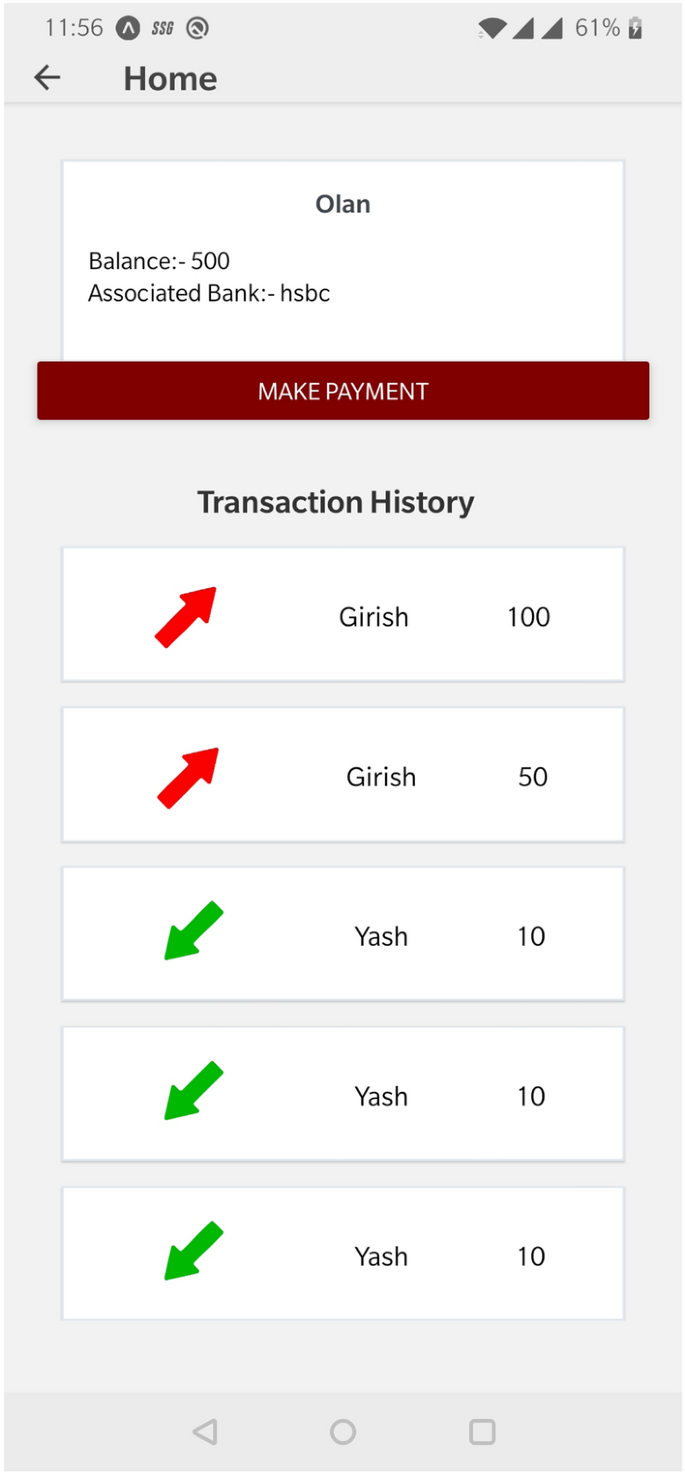
Each component has several “lifecycle methods” that we can override to run code at particular times in the process. We have used the component mounting life cycle method in our project. Mounting is the phase in which our React component will mount on the DOM i.e., is created and inserted into the DOM. This phase comes onto the scene after the initialization phase is completed. In this phase, our component renders for the first time. Once the user has entered valid credentials and login is successful, the App moves onto the homepage. Here, the componentWillMount() method is used which is called just before the component is mounted onto the DOM or the render method is called. Once this method is called, all the client details and the transaction history is queried from the database. React components are stacked on top of the other, for example, the homepage above the login and the payment above the homepage, which makes updating these components difficult as they only get updated with ReactDOM.render. This is why we added an additional listener (div focus) which calls the render method when we come back to our homepage, making sure that only the updated values are displayed. The .focus() method tells the app which screen is being acted on, which is helpful to find out which page the app is currently on. By exploiting that, the app can re-render when a payment is completed.
Moving on to the offline mode payments, we have made use of React Async Storage which is an unencrypted, asynchronous, persistent, and key-value storage system that is global to the app which is better than Local Storage. Here, the associated bank details and the balance amount is stored, which will be modified after a transaction. This data cannot be modified by the user at any time and is completely safe and secure. Hence offline transactions can be achieved without the fear of theft. We have made the use of a community library in react native called ’Netinfo’ which allows us to get information about connection type and connection quality. This helps us when the mobile gets a stable internet connection which the app then checks for using the Netinfo library, after which, the last offline mode transaction can be updated online. This is done from our homepage itself and another socket connection is initiated through this page, which is then used to update the balance info.
Figure 5 is the transaction page of our application. When a customer wants to pay the merchant, the former will enter the transaction amount and the merchant’s phone number and thereafter if sufficient balance is available i.e, post-transaction the wallet value should be greater than zero, a successful transaction will have taken place and the customer will have redirected to the home page where their remaining balance and transaction history will be displayed. All these transactions are taken care of by NodeJS’ socket.io library which enables real-time, bidirectional, and event-based communication between the customer and the merchant. Web Socket is a communication protocol that provides a full-duplex and low-latency channel between the client and the server i.e customer and merchant. Using Web Sockets, a developer can come up with a function that works consistently across all the platforms. Web Socket represents a single TCP socket connection, thus eliminating the problem of connection limitation. In the case of REST architecture, the client and server can be implemented independently, without knowing each other. This client/server paradigm has lots of benefits with it i.e, the client-side code can be altered at any time, without the server getting affected. The different clients having REST interfaces can hit the endpoints at the same time and receive the same response. Also, one other feature is statelessness. A server does not need to know which state the client is in, and the same holds true for the client. All in all, when it comes to security and information assurance it is necessary to ensure that the transactions or any such confidential information are passed through a secure socket only so that the chance of an intrusion attack or a network vulnerability is minimized.
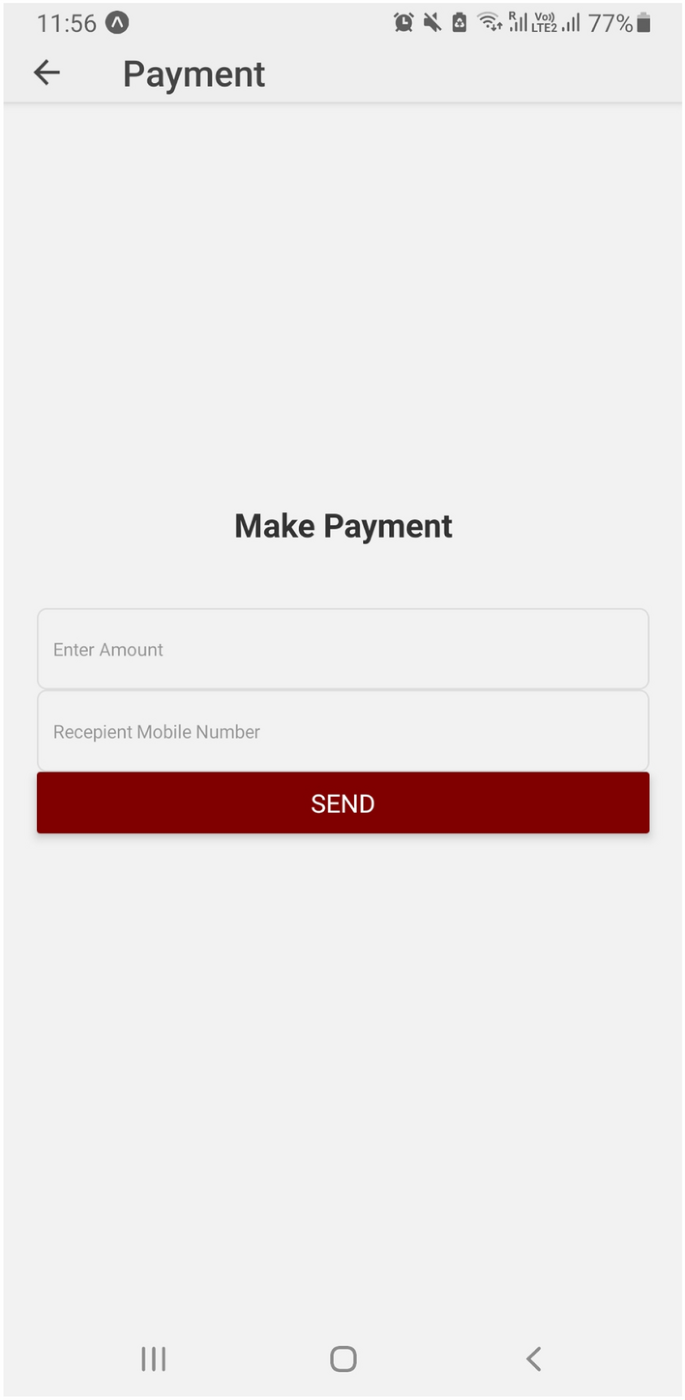
Transaction page
Figure 6 shows the homepage of the application in an offline environment which is very similar to that of Fig. 4 , the key difference being that the client will not be able to see the transaction history in the absence of a stable internet connection. On completion of a successful transaction, the customer is redirected to the ’Transaction Successful’ page as seen in Fig. 7 , and in the case of an unsuccessful transaction, the customer is redirected to the ’Transaction Failed’ page as seen in Fig. 8 . Post a transaction success or failure the customer will be redirected/navigated to the homepage.
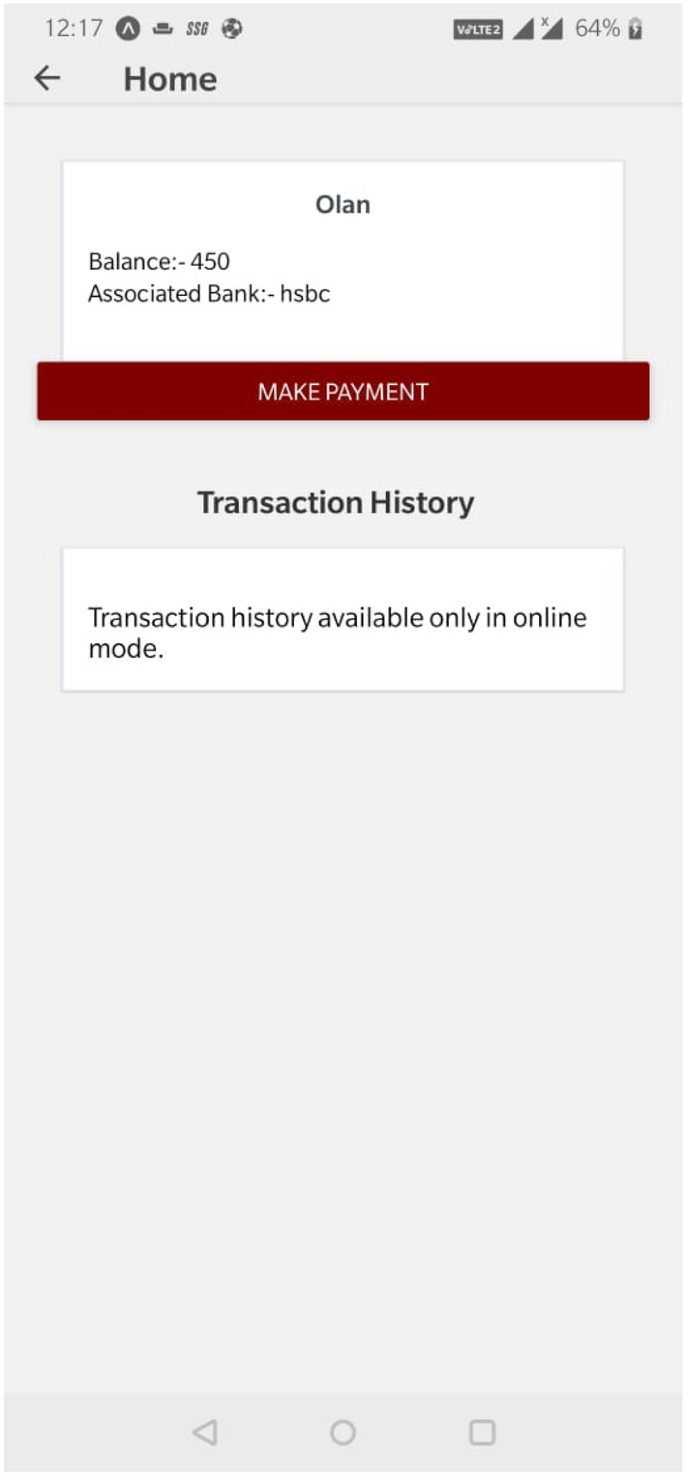
Offline homepage
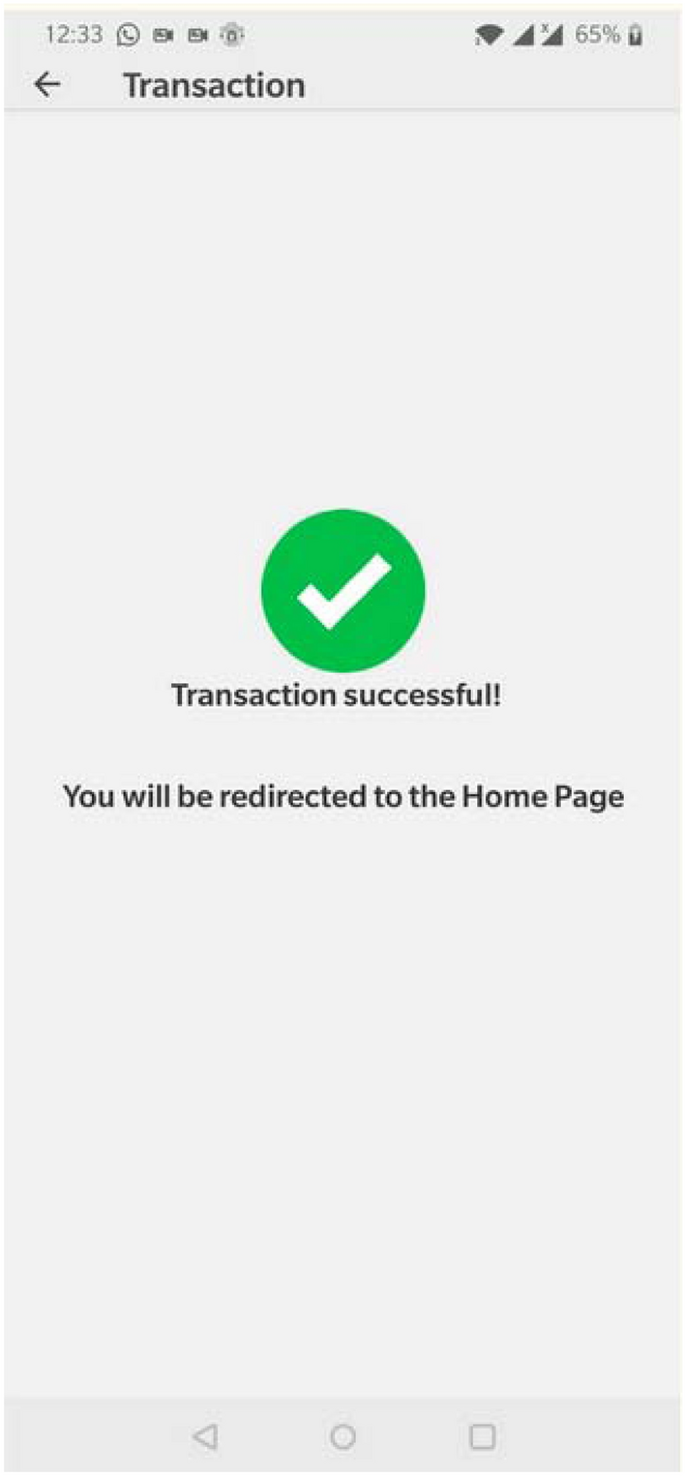
Transaction successful
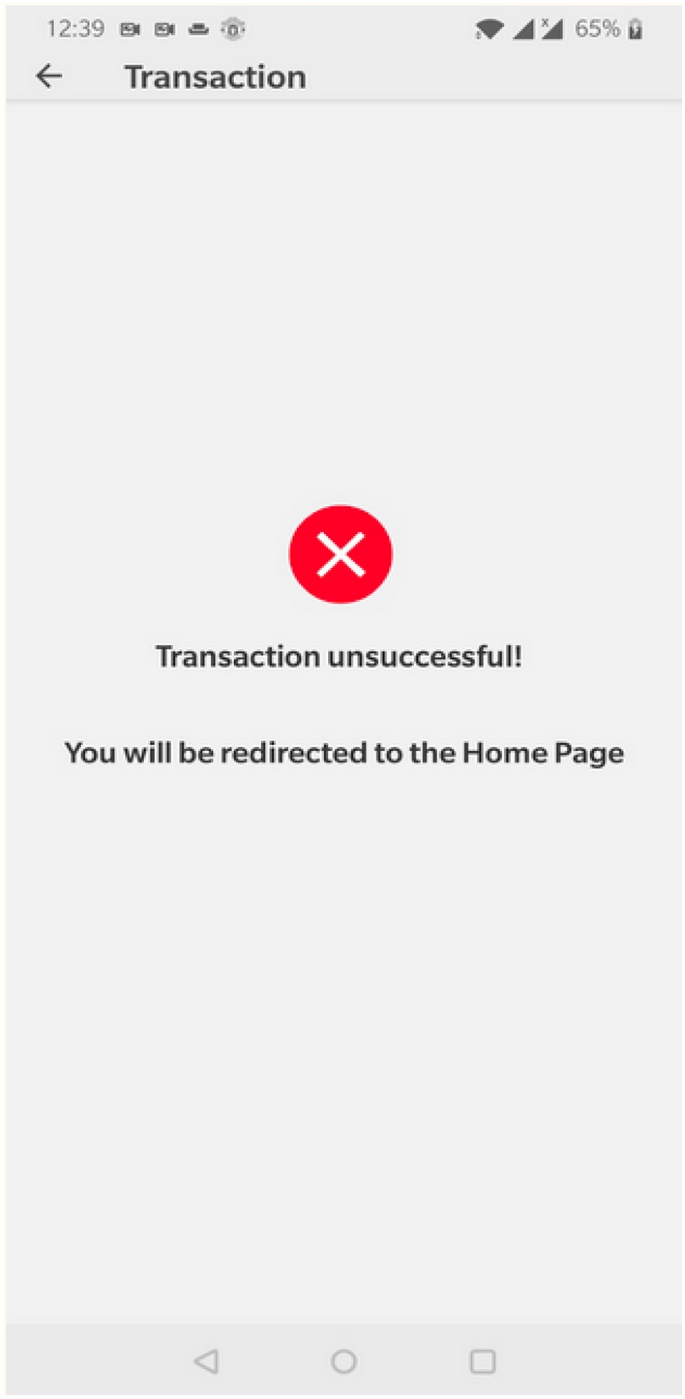
Transaction failed
The evaluation parameters, both in terms of cost and time analysis were extensively performed on our application. Over 1000 transactions between different customer and merchant accounts were done and the results from the analysis performed were better than the other wallet applications available in the market today. The time taken by our application for a given transaction was around 600ms on average which outperforms other benchmark wallet applications which are in the range of 700–800ms, this indicates that our proposed model works positively irrespective of any network-related traffic. The failures encountered so far were only due to business validation errors and not due to any technical faults. Business validation failures are those transactions that were initiated with the customer having insufficient balance and/or those where the customer gave an incorrect recipient for the transaction. The application developed has no technical issues seen thus far and even when a high density of transactions are observed at the same time, the application does not time out. This application is horizontally scalable and for industrial usage, additional nodes can be added for load management.
In this article we present a novel prototype of an offline wallet payment using React Native as our Frontend, NodeJS as our Backend and MongoDB as our database. The main idea of this prototype application was to handle and prevent intrusion attacks and vulnerabilities, to ensure this we used socket.io library and made sure all our API calls were safe. For information assurance and security, we have made use of the bcrypt module, where we hash and store all passwords to our database, thereby reducing the chance of threats to an end user’s wallet. To establish concurrency and to avoid delay we implemented our own document level lock. Furthermore, for the offline mode of our prototype, the application makes use of asynchronous storage which is a global storage system which we used to store the transaction amount and bank details. This idea is very useful in day to day activities where a user may not always get a stable connection and would require to make transactions. This application allows users to continue using mobile payments in any scenario as mobile payments have bloomed in recent years. The internet barrier will allow several merchants and users to start using mobile payments. Security enhancements in the online mode has also been improved to create an absolutely safe environment for a user. At present the M-Commerce wallet application developed is centralised, the future scope of this project would be to make it a decentralised online-offline wallet payment system.
Jayasinghe Danushka, et al. Extending EMV tokenised payments to offline-environments. In: 2016 IEEE Trustcom/BigDataSE/ISPA. 2016;443–50. https://doi.org/10.1109/TrustCom.2016.0095.
San Aye Mi, Sathitwiriyawong Chanboon. ”Privacy-preserving offlinemobile payment protocol based on NFC”. In: 2016 International Computer Science and Engineering Conference (ICSEC). 2016, pp. 1–5. https://doi.org/10.1109/ICSEC.2016.7859939.
Kiran N. Chitra, Narendra Kumar G.”Building robust m-commerce payment system on offline wireless network”. In: 2011 Fifth IEEE Interna-tional Conference on Advanced Telecommunication Systems and Networks (ANTS). 2011, pp. 1–3. https://doi.org/10.1109/ANTS.2011.6163664.
He Yongqiang, Shi Yanrong, He Aixiang. ”Research on online pay-ment mode of e-commerce”. In: 2010 IEEE International Conference on Software Engineering and Service Sciences. 2010, pp. 312–15. https://doi.org/10.1109/ICSESS.2010.5552440.
Luo Jia-Ning, Yang Ming-Hour. ”Offline transferable E-cash mechanism”. In: 2018 IEEE Conference on Dependable and Secure Computing (DSC). 2018, pp. 1–2. https://doi.org/10.1109/DESEC.2018.8625136.
Zhao Hao, Muftic Sead. ”The concept of Secure Mobile Wallet”. In: 2011 World Congress on Internet Security (WorldCIS-2011). 2011, pp. 54–58. https://doi.org/10.1109/WorldCIS17046.2011.5749882.
Kreft Heinz, Adi Wael. ”Wallet based E-cash system for secured multi-hop cash exchange”. In: 2008 3rd International Conference on In-formation and Communication Technologies: From Theory to Applications. 2008, pp. 1–5. https://doi.org/10.1109/ICTTA.2008.4529987.
Hassan Md Arif, Shukur Zarina. ”Review of digital wallet require-ments”. In: 2019 International Conference on Cybersecurity (ICoCSec).2019, pp. 43–48. https://doi.org/10.1109/ICoCSec47621.2019.8970996.
Tso Raylin, Lin Chen-Yi. ”An off-line mobile payment protocol pro-viding double-spending detection”. In: 2017 31st International Confer-ence on Advanced Information Networking and Applications Workshops (WAINA). 2017, pp. 570–75. https://doi.org/10.1109/WAINA.2017.56.
Khan Burhan Ul Islam, et al. ”Offline OTP based solution for SecureInternet Banking Access”. In: 2018 IEEE Conference on e-Learning, e-Management and e-Services (IC3e). 2018, pp. 167–72. https://doi.org/10.1109/IC3e.2018.8632643.
Li Songnong, et al. ”Research on offline transaction model in mobile payment system”. In: Frontier Computing. Ed. by Jason C. Hung, Neil Y. Yen, and Lin Hui. Singapore: Springer Singapore, 2019, pp. 1815–20. ISBN: 978-981-13-3648-5.
Solat Siamak (2017) ”Security of electronic payment systems: A comprehensive survey”. arXiv.1701.04556
Liu Wenzheng, Wang Xiaofeng, Peng Wei. ”State of the Art: Secure Mobile Payment”. In: IEEE Access8 2020, pp. 13898–914. https://doi.org/10.1109/ACCESS.2019.2963480.
Gupta Rahul, Kapoor Cheshtha, Yadav Jayesh. ”Acceptance towards digital payments and improvements in cashless payment ecosystem”. In: 2020 International Conference for Emerging Technology (INCET). 2020, pp. 1–9. https://doi.org/10.1109/INCET49848.2020.9154024.
Singh Jay P. ”Offline transactions functionality in eWallets”. In: Indian journal of science and technology10. 2017, pp. 1–4.
Samid Gideon, Wallet BitMint Hard. ”Digital payment without network communication : No Internet, yet Sustained Payment Regimen between Randomness-Verifiable Hard Wallets”. In: 2020 IEEE International IOT, Electronics and Mechatronics Conference (IEMTRONICS). 2020, pp. 1–7. https://doi.org/10.1109/IEMTRONICS51293.2020.9216456.
Rahat Abdullah, et al. ”An offline electronic payment system based on anuntraceable blind signature scheme”. In: KSII transactions on internetand information systems 11. 2017, pp. 2628–45. https://doi.org/10.3837/tiis.2017.05.018.
Pal Abhipsa, et al. ”Is the convenience worth the risk? An investigation of mobile payment usage”. In: Information systems frontiers 23.4. 2021, pp. 941–61. https://doi.org/10.1007/s10796- 020- 10070.
Ahmed Waqas , et al. ”Security in next generation mobile payment systems: A comprehensive survey”. In: IEEE Access9. 2021, pp. 115932–950.
Wang Yong, Hahn Christen, Sutrave Kruttika. ”Mobile payment security, threats, and challenges”. In: 2016 Second International Conference onMobile and Secure Services (MobiSecServ). 2016, pp. 1–5. https://doi.org/10.1109/MOBISECSERV.2016.7440226.
Hassan Md Arif, et al. ”A review on electronic payments security”. In: Symmetry12.8. 2020; ISSN 2073–8994. https://doi.org/10.3390/sym12081344.
Abdullah, Khan Mohammed Naved. ”Determining mobile payment adoption: A systematic literature search and bibliometric analysis”. In: Cogent Business & Management8.1 (2021). Ed. by Elena Kostadinova, p. 1893245. https://doi.org/10.1080/23311975.2021.1893245.
Canara Bank, Parvathi S. The impact of pandemic on digital payments in India. ResearchGate; 2021.
Download references
Author information
Authors and affiliations.
National Institute of Technology Karnataka Surathkal, Mangalore, India
Yash Kumar Gupta, Girish Jeswani & Olan Pinto
You can also search for this author in PubMed Google Scholar
Corresponding author
Correspondence to Yash Kumar Gupta .
Ethics declarations
There is no funding required for this project.
Conflict of Interest
The authors declare that they have no conflict of interest.
Additional information
Publisher's note.
Springer Nature remains neutral with regard to jurisdictional claims in published maps and institutional affiliations.
This article is part of the topical collection “Enabling Innovative Computational Intelligence Technologies for IOT” guest edited by Omer Rana, Rajiv Misra, Alexander Pfeiffer, Luigi Troiano and Nishtha Kesswani.
Rights and permissions
Reprints and permissions
About this article
Gupta, Y.K., Jeswani, G. & Pinto, O. M-Commerce Offline Payment. SN COMPUT. SCI. 3 , 100 (2022). https://doi.org/10.1007/s42979-021-00978-x
Download citation
Received : 05 September 2021
Accepted : 18 November 2021
Published : 14 December 2021
DOI : https://doi.org/10.1007/s42979-021-00978-x
Share this article
Anyone you share the following link with will be able to read this content:
Sorry, a shareable link is not currently available for this article.
Provided by the Springer Nature SharedIt content-sharing initiative
- Software security
- Web sockets
- Document level locking
- Asynchronous storage
- Information assurance
- Intrusion attack
Advertisement
- Find a journal
- Publish with us
- Track your research
How You Pay Drives What You Choose: Health Savings Accounts versus Cash in Health Insurance Plan Choice
A marked feature of health insurance plan choice is inconsistent choices through the overweighting of premiums relative to out-of-pocket spending. We show that this source of inconsistency disappears when both types of spending come from the same source of designated funds. We focus on the MediSave program in Singapore, whereby residents can pay their health insurance premiums with cash or MediSave funds, but are subject to limits that vary by age and over time. By exploiting variations in those limits, we consistently find that when individuals are able to pay their health insurance premiums with MediSave funds, they are less price sensitive and more willing to enroll in more generous plans—which results in lower spending levels and variance, and lower adverse selection in the market. The results suggest a strong role for mental accounting in insurance decisions.
Lin, Liu, and Yi gratefully acknowledge support from Singapore’s Ministry of Education Academic Research Fund Tier 1 (WBS R-122-000-303- 115). The views expressed herein are those of the authors and do not necessarily reflect the views of the National Bureau of Economic Research.
MARC RIS BibTeΧ
Download Citation Data
- online appendix
Working Groups
More from nber.
In addition to working papers , the NBER disseminates affiliates’ latest findings through a range of free periodicals — the NBER Reporter , the NBER Digest , the Bulletin on Retirement and Disability , the Bulletin on Health , and the Bulletin on Entrepreneurship — as well as online conference reports , video lectures , and interviews .
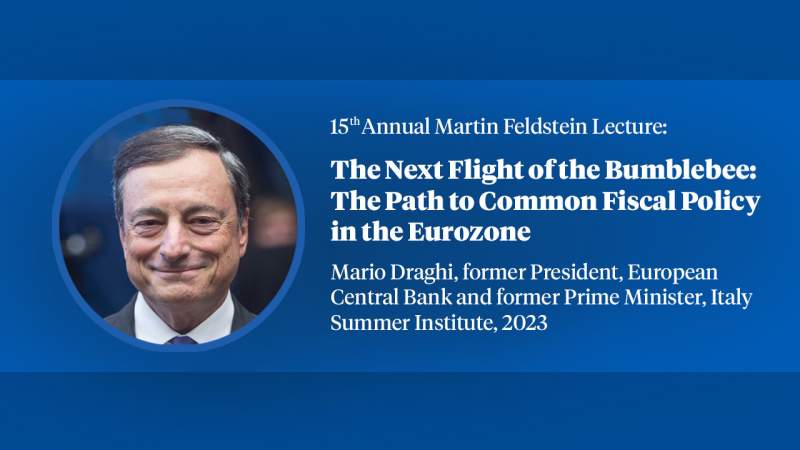
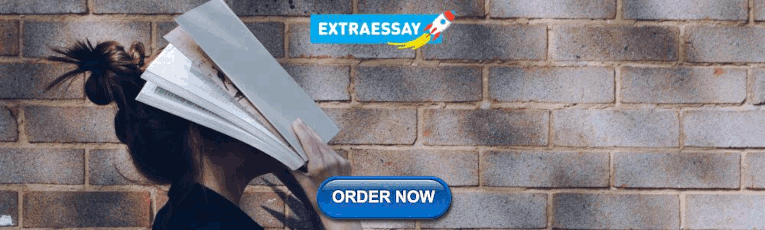
IMAGES
VIDEO
COMMENTS
Highly cited research papers on m-payment. Download CSV Display Table. Analysis of the highly cited papers highlight the fact that Schierz et al. (Citation 2010) significantly contributed towards m-payment research with this seminal work. His contribution laid the foundation for empirical research works in the field of m-payment.
This paper seeks to provide a summary of findings from previous digital payment studies so as to identify potential future research topics and implications. According to Dahlberg et al. (2008) , digital payment service providers and researchers can use.
This paper, therefore, aims to offer the most up-to-date research on digital payments from a customer perspective. Our SLR analysed 193 studies retrieved from Scopus and Web of Science databases. We explored the sample literature by focusing on the research yearly trend, influential studies, regional distribution, theoretical background ...
The adoption of d-payments has been accelerated by the evolution of new technologies (e.g. the Internet of Things, blockchain, near field communication; see Petralia et al., 2019), the presence of country-specific regulation favourable to the adoption of new methods of payment (e.g. Payment Services Directive 2, or PSD2, in the euro area), policies that discourage the use of cash (e.g. Sweden ...
Subsequently, the paper presents the key challenges in digital payment technologies categorized into broad themes: social, economic, technical, awareness and legal.
1. Introduction. Digital wallets provide a method for making payments electronically, enabling users to transfer funds between transaction accounts—either traditional banking accounts or electronic money 1 deposit accounts—and to use other payment instruments. When the digital wallet is based on mobile devices (e.g., smartphones, tablets) it is referred to as a mobile wallet (see Bezhovski ...
Propelled by recent policy initiatives and technological developments, India's digital payment system is a promising success story in the making. At the same time, the data also points towards an increasing usage of cash. While aggregate country-level data can indicate overall preferences of citizens, we use a novel online survey-based dataset to understand how factors such as 'perception ...
The recommendations given will help identify the primary areas requiring advancement in future research on mobile payment systems. ... Following the SLR process, we examined over 350 research papers with a comprehensive and detailed inspection of the mobile payment domain's literature. Based on the analysis, we present the trends, patterns ...
Researchers have increasingly explored various aspects of mobile payment in the past two decades, including different mobile payment systems (MPS), stakeholders, and related research contexts. However, existing literature reviews are limited to user adoption, overlooking the importance of understanding other key stakeholders, such as retailers ...
The range of digital technologies used in financial services is very broad, including household and small business lending, online and mobile payments, insurance, capital market transactions, wealth management and regulatory reporting and compliance. Likewise, a wide range of digital initiatives seek to promote financial inclusion.
The outcomes of the current work revealed useful insights into consumer's usage and satisfaction with four prevailing digital payment methods vis-à-vis demographic factors. This study is significant as it empirically examines the impact of demographic factors on the CU of digital payment systems during the post-demonetization period in India.
A bibliometric analysis is used to summarize large quantities of data to understand the intellectual landscape of a given field ("Subject and Course Guides: Bibliometric Analysis and Visualization: Bibliometrics," 2022). In this paper, the analysis will yield emerging trends in academic documents on blockchain-based payment systems.
This research has undertaken a systematic literature review (SLR) of articles focusing on the acceptance of fintech payment services by identifying 84 peer-reviewed articles published in international scientific journals from 2015 to April 2023. This paper uses the Scientific Procedures and Rationales for Systematic Literature Reviews (SPAR-4-SLR) protocol to gather relevant articles and the ...
E -payment is also defined as. payment by electronic transfer of credit card details, direct credit or other electronic means other than. payment by cheque and cash (Agimo, 2004). Antwi, Hamza ...
This research paper focuses on the impact of electronic payment methods, specifically credit and debit cards, on point-of-sale transactions and the consumer price index.
Digital Payments and Business Resilience: Evidence in the Time of COVID-19. During the COVID-19 pandemic, consumers were encouraged to use contactless payments. An important policy question is whether merchants with contactless payment technology are more resilient to an external health shock than those without. Using a matched difference-in ...
Payments banks came into existence in India in 2015 when the Reserve Bank of India awarded licenses to eleven applicants to set up these banks specifically to further financial inclusion by providing small savings accounts and facilitating payments and remittances to the financially excluded population. As of March 2020, only six of these were operative and not very successful, both from the ...
Empirical research on digital payment system and its continuance intention is very less and to address this research gap, researcher has examined a research model. The result shows that Perceived Reputation, Perceived Security, and Perceived Structural Assurance with digital payment systems has positive impact on trust and Continuance Intention.
Introduction. Payment for daily consumption can be made by physical cash or cashless payment tools. Some frequently used cashless payment tools were credit transfer payments, cheques, direct debits (Tee and Ong 2016), credit cards (Shy 2020), charge cards and e-wallets (Teng and Khong 2021; Alam et al. 2021).Consumers used cashless payments due to their convenience (Jebarajakirthy and Shankar ...
According to McKinsey's latest report 3 on global trade and value chains, in 2017, total global trade stood at $22 trillion, with trade in goods at $17 trillion. Trade in services, though smaller at $5 trillion, has outpaced growth in goods trade by more than 60 percent over the past decade (CAGR of 3.9 percent).
The research has also found that factors such as saves time, cashback and discounts, convenience, detailed records, reduced theft risk, track your spendings, and creating a cashless economy influence consumer's attitudes towards mobile payment applications. The research also indicates some factors as barriers to the consumers towards mobile ...
The Controversial Business of Cash-to-Crypto Bitcoin ATMs. The Bitcoin ATM industry continues to grow in the United States amid concerns about illegal or financially predatory behavior. Briefings cover a range of topics, including payments methods, developments in payments networks, and various participants' roles in the payments system.
In contrast to most publications on the ethics of paying research subjects, which start by identifying and analyzing major ethical concerns raised by the practice (in particular, risks of undue inducement and exploitation) and end with a set of—more or less well-justified—ethical recommendations for using payment schemes immune to these problems, this paper offers a systematic, principle ...
The reviews delve insights into the various challenges and advantages of using digital transactions. The findings reveal that digital transactions are accepted in India and usage is increasing ...
This research paper studies the aspects that impact the use of digital payment systems by street vendors in Mumbai and Thane Region. The responses for the study were composed through a designed questionnaire and informal interaction from 93 street vendors. The respondents were vendors selling a variety of items such as vegetables, fish, milk, coconut, Idli/Dosa, Juice, Vada Pav, Mangoes and ...
Fraud arises in the financial industry via numerous channels, such as credit cards, e-commerce, phone banking, checks, and online banking. Juniper Research reports that e-commerce, airline ticketing, money transfer, and banking services will cumulatively lose over $ 200 billion due to online payment fraud between 2020 and 2024.The increased sophistication of fraud attempts and the increasing ...
Another group of researchers led by Bouwman and de Reuver at TU Delft in the Netherlands has investigated mobile payments lately. In Spain, Sanchez-Fernandez, Munoz-Leiva and Liebana-Cabanillaz from the University of Granada also contributed to mobile payment research with papers on acceptance and adoption, mostly published in 2014.
The paper focuses its research on online payments as well as E-wallets. This research reviews around 131 research papers concerned with electronic payments in between 2010 ans 2020. It uses a systematic approach to its literature review. The results obtained show that interest in E-wallet and online payment has grown significantly during 2010 ...
Founded in 1920, the NBER is a private, non-profit, non-partisan organization dedicated to conducting economic research and to disseminating research findings among academics, public policy makers, and business professionals. ... Research; Working Papers; How You Pay Drives What You Choose:…
digital payments. The purpose of this research paper is to investigate the positive impact on consumers. The current article examines the amount of customer acceptance of different digital payment solutions. While demographic factors such as age, gender, and income are important in determining this choice, we find