- Open access
Smart Learning Environments
30k Accesses
22 Citations
11 Altmetric
Metrics details
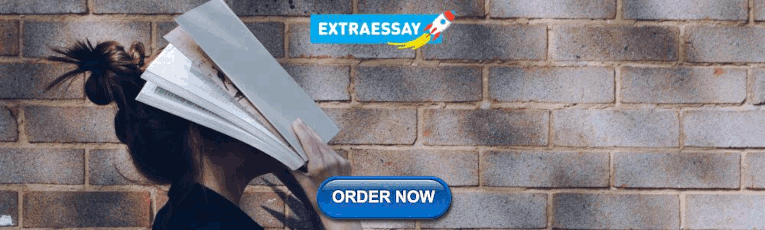
Open Case Studies
What is the open case studies (ocs) project.
The Open Case Studies project is an educational resource of experiential guides that demonstrate how to effectively derive knowledge from data in real-world challenges.
All case studies can be found in our OCS search tool .
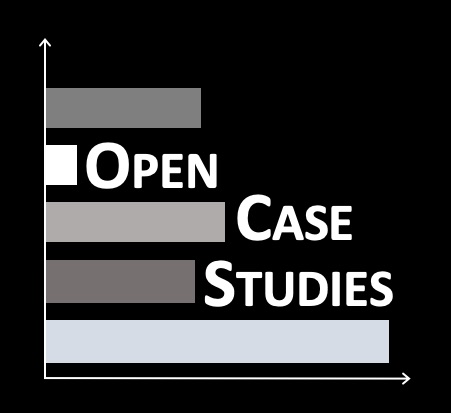
Our case studies can be used:
- by educators to help them teach
- by students to help them with their classes
- by independent learners to help them learn
Find out more about how others are using case studies and what they think of them in this study .
What problem are we addressing?
Despite unprecedented and growing interest in data science on campuses, there are few courses and course materials that provide meaningful opportunity for students to learn about real-world challenges. Most courses frequently fail to frame the lectures around a real-world application and provide unrealistically clean datasets that fit the assumptions of the methods in an unrealistic way. The result is that students are left unable to effectively analyze data and solve real-world challenges outside of the classroom.
Problems with previously suggested solutions
In 1999, Nolan and Speed argued the solution was to teach courses through in-depth case studies derived from interesting problems, with nontrivial solutions that leave room for different analyses. This innovative framework teaches the student to make important connections between the scientific question, data and statistical concepts that only come from hands-on experience analyzing data. However, these case studies based on realistic challenges, not toy examples, are scarce.
What are we proposing as a solution?
To address this, we are developing the Open Case Studies educational resource of case studies, which demonstrate illustrative data analyses that can be used in the classroom to teach students how to effectively derive knowledge from data. This approach has successfully been used to teach data science courses at many universities, including:
- Johns Hopkins Bloomberg School of Public Health
- Harvard University
- Harvard T.H. Chan School of Public Health
- University of California at Berkeley
- Smith College
If you have the time, please fill out our survey (1-10 min).
Recent Posts
Ocs to be featured at the bloomberg american health summit 2020, welcome qier meng and michael breshock to ocs team, ocs work featured in jhu hub, looking for a student to join the ocs team, ocs team awarded delta award.
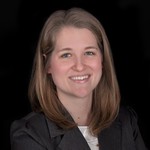
Stephanie Hicks, PhD
Assistant professor (principal investigator).
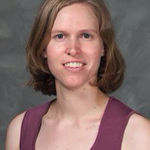
Leah Jager, PhD
Assistant scientist.
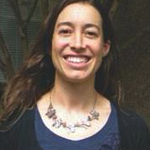
Margaret Taub, PhD
Associate scientist.
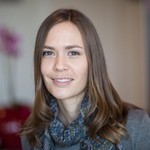
Carrie Wright, PhD
Senior staff scientist.
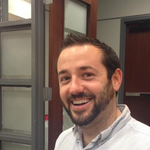
John Muschelli, PhD
Research assistants.
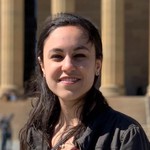
Lyla Atta, BS
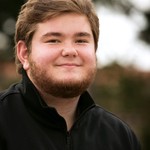
Michael Breshock, MSE
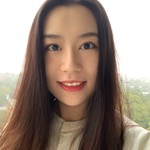
Qier Meng, BS
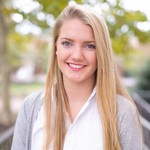
Alexandra Stephens, MSE
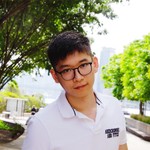
Hanchao (Ted) Zhang, MS
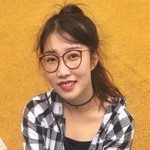
Kexin (Sheena) Wang, MSE
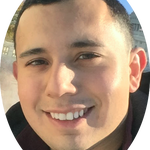
Michael Ontiveros, MHS
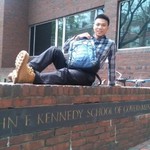
Pei-Lun (Perry) Kuo, MD, MPH
Recent & upcoming talks.
- Exploring initiatives to promote more comprehensive data science and statistical education . The University of Texas at Austin, Depeartment of Statistics and Data Sciences , Austin, Texas, USA.
Problem-forward Statistics and Data Science to Improve Human Health. Myrto Lefkopoulou Distinguished Lectureship. Harvard T. H. Chan School of Public Health. ( https://www.hsph.harvard.edu/biostatistics/myrto-award/ ). Hicks, Stephanie, 2023 Sept 21. Boston, MA. USA.
Reimagining Research Training Through Holistic Data Science Education . University of Washington Department of Biostatistics Seminar . Wright, Carrie. 2023 May 4th. Seattle, WA. USA. (Virtual).
- Case studies to community engagement: Bringing hands-on data science experiences to the classroom . International Chinese Statistical Association (ICSA) . Wright, Carrie. 2022 June 20th. Gainesville, Florida.
Open Case Studies: An Experiential Lesson Guide Delivery Platform Wright, Carrie and Breshock, Michael and Qier, Meng. Johns Hopkins University Provost’s Teaching with Technology DELTA Symposium. 2021 May 4th. Baltimore, MD. USA. (Virtual) See a recording here .
Addressing Open Challenges in Data Science Education . Hicks, Stephanie. Department of Statistics, University of British Columbia. 2021 Feb 9. Vancouver, BC. Canada. (Virtual).
- Open Case Studies Hicks, Stephanie and Wright, Carrie. Bloomberg American Health Summit 2020 2020 Dec 9. Baltimore, MD. USA. (Virtual)
Communicating statistics and data science to the masses via Open Case Studies. Hicks, Stephanie. Joint Statistical Meetings. 2020 Aug 1-6. Philadelphia, PA, USA.(Virtual due to COVID-19).
Addressing Real-World Challenges through Data Science Education .Hicks, Stephanie. Academic Data Science Alliance (ADSA) Data Science Education special interest group (SIG) . 2020 Jul 2.
Addressing Public Health Challenges through Data Science Education . Hicks, Stephanie. American Public Health Association Annual Meeting . 2019 Nov 2-6. Philadelphia, PA, USA.
Motivating Data Science through Case Studies in Public Health. Eastern North American Region (ENAR) Meeting of the International Biometric Society . Jager, Leah. 2019 March 27. Philadelphia, PA, USA.
Open Case Study Search
Find the right open case study for you!
Academia.edu no longer supports Internet Explorer.
To browse Academia.edu and the wider internet faster and more securely, please take a few seconds to upgrade your browser .
Enter the email address you signed up with and we'll email you a reset link.
- We're Hiring!
- Help Center
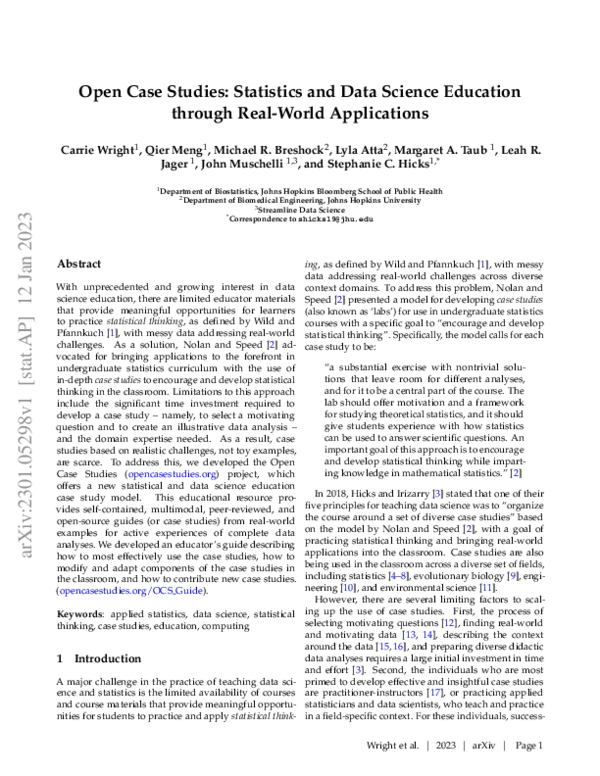
Open Case Studies: Statistics and Data Science Education through Real-World Applications

2023, arXiv (Cornell University)
Related Papers
Duncan Lang
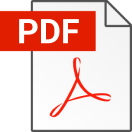
Alison Gibbs
The American Statistician
Nicholas Horton
A growing number of students are completing undergraduate degrees in statistics and entering the workforce as data analysts. In these positions, they are expected to understand how to utilize databases and other data warehouses, scrape data from Internet sources, program solutions to complex problems in multiple languages, and think algorithmically as well as statistically. These data science topics have not traditionally been a major component of undergraduate programs in statistics. Consequently, a curricular shift is needed to address additional learning outcomes. The goal of this paper is to motivate the importance of data science proficiency and to provide examples and resources for instructors to implement data science in their own statistics curricula. We provide case studies from seven institutions. These varied approaches to teaching data science demonstrate curricular innovations to address new needs. Also included here are examples of assignments designed for courses that foster engagement of undergraduates with data and data science.
Springer eBooks
Teaching Statistics
Gunnar Stefansson
Behavior Research Methods, Instruments, & Computers
Thomas Hewett
Journal of Statistics Education
roberto ramirez rivera
… of the Seventh International Conference on …
Aklilu Zeleke
Over the last decade, the use of real world projects in introductory statistics courses has increased in popularity. Real world projects provide students with an opportunity to learn the entire process of a statistical investigation. Such projects fit the principles of active ...
RELATED PAPERS
Arquivos de Neuro-Psiquiatria
Go Lisanawati
Dzineku William
International Journal of Endorsing Health Science Research (IJEHSR)
Kashifuddin Qayoom Soomro
BMC Public Health
Fazlollah Ghofranipour
African journal of reproductive health
Friday Okonofua
International Journal of Surgery
Roberto Klappenbach
Journal of Structural and Construction Engineering (Transactions of AIJ)
Shojiro Motoyui
Panayiotis Vyras
Research, Society and Development
Carlos Alberto Dias
International Journal of Online Engineering (iJOE)
Gustavo Moreira Alves
Materials Sciences and Applications
Javaid Bashir
Physical Review D
Aquacultural Engineering
Steven Summerfelt
Journal of Molecular Neuroscience
Antonio Diego
Johnnie Williams
European Journal of Medicinal Chemistry
Juan Carlo Arenciano Rodrigues
Stefanie Lawrence
alicia meckstroth
Elka Dimitrova
Cecilia Geron
Physical review
Surjan Peter
Injury Epidemiology
Kirsten Bechtel
دار صفحات للنشر
Prof Dr. Qusay Al- Turkey
RELATED TOPICS
- We're Hiring!
- Help Center
- Find new research papers in:
- Health Sciences
- Earth Sciences
- Cognitive Science
- Mathematics
- Computer Science
- Academia ©2024

Data Science for Education
Main navigation.
Educational institutions and learning process entail rich data, and they concern weighty problems of great importance to society and the social good, so education is an especially well-suited domain for data science.
Educational data spans K-12 school and district records, digital archives of instructional materials and gradebooks, as well as student responses on course surveys. Data science of actual classroom interaction is also an increasing interest and reality – there one can capture how classroom management and instruction is accomplished. As video and voice recordings grow more prevalent, it may be a prime data source to analyze via novel computational means. The richness of educational data extends to the higher education realm where an increasing number of online courses are being employed. It even extends to the private sector where online forums, threads, and distributed forms of problem-solving are used to educate employees and resolve task problems.
All these new data sources are replete with information on communication (text), relations(links), and accruing behavioral profiles (careers). All of this information can be mined and analyzed in an effort to understand and solve persistent educational problems.
- Educational data science would train educators - broadly conceived - to study these forms of educational data and to make sense of educational systems, their problems and potential solutions, and to develop a deeper understanding and empirically established forms of solutions.
- Educational data science would empower educators to perform data visualization, data reduction and description, and prediction tasks.
- Data visualization can render information more intuitive and immediately digestible for practitioners.
- Data reduction can be used to make sense of many complex records and fields of data on students (e.g., grade books and assignments, etc).
But even basic descriptions of the educational system like tracking structures, key turning points in careers, etc, also fit. All are feasible with data science of school records.
A variety of educational problems suggest potential modeling and prediction tasks (random forest sort of thing). For example: student attrition & dropout / student attendance; detentions / office referrals / arrests; learning delays / progression problems / failure; assignment completions; bias / prejudice in grading; the diversification bonus; etc.
Looking forward, there are a number of areas where the combination of data sciences and education is particularly promising.
Psychology, education research and the learning sciences often have nuanced theories of human cognition and learning that make general guidelines on the types of pedagogical activities that are effective. However, such guidelines do not always translate to concrete implementable strategies. Data science from prior data (e.g. using counterfactual reasoning methods) can be used to parameterize generic guidelines into grounded strategies that can be used (Chi et al. 2011; Khajah et al. 2016): as a fake but illustrative example, if blocking practice on a particular skill is thought to be beneficial, data science can be used to help determine for this particular school’s seventh grade population, how many practices on this skill is most effective.
Rich contextualization of education -- what works for whom, when and where -- is also an exciting possibility. Much of educational and learning sciences research takes place in laboratory settings or is conducted in a limited set of schools. Sometimes findings of such studies fail to replicate in other environments. Large scale data collection, across MOOCs and classrooms, could help us uncover what are the key features that correlate and cause the effectiveness of an educational intervention. As has long been recognized, online platforms also make it easier to offer personalized interactions to students as we identify which factors are important in the pedagogical activities.
An interesting challenge on the data science methodology side is that many new educational platforms offer mixed autonomy. The learner may have a large amount of flexibility in how she proceeds through the course, and yet the potential for the teaching system to provide recommendations or guide the student’s learning path is considerable. Creating systems that can most effectively navigate this challenge, which may also have significant impacts on student motivation, is an important open issue.
M. Chi, VanLehn, K, Litman, D. & Jordan, P. (2011). Empirically evaluating the application of reinforcement learning to the induction of effective and adaptive pedagogical tactics. User Modeling and User Adapted Instruction (UMUAI), 21, 1-2, pp. 137-180.
Khajah, M., Roads, B. D., Lindsey, R. V., Liu, Y.-E., & Mozer, M. C. (2016). Designing engaging games using Bayesian optimization. In Proceedings of the 2016 CHI Conference on Human Factors in Computing Systems (pp. 5571-5582). New York: ACM.
Contributors
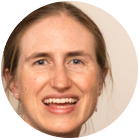
Emma Brunskill
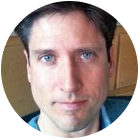
Daniel McFarland
FOR EMPLOYERS
Top 10 real-world data science case studies.
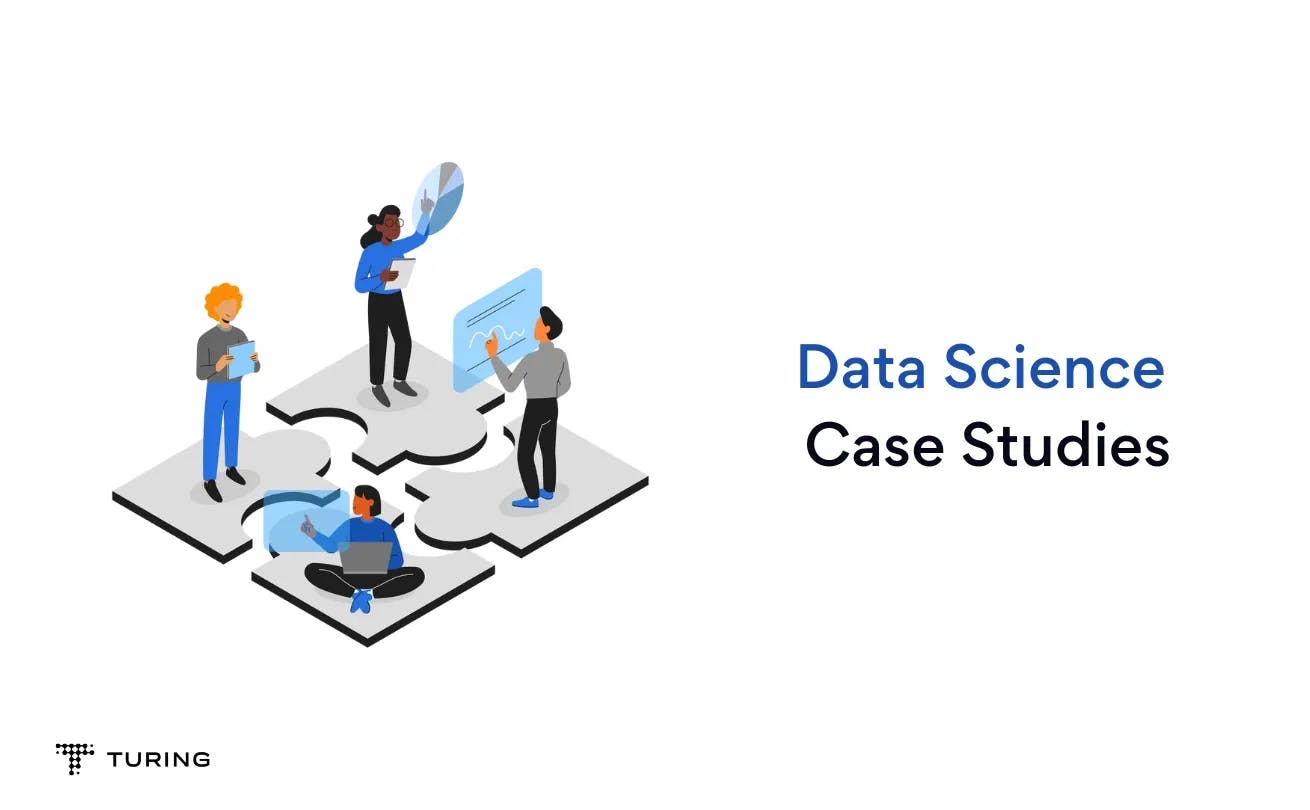
Aditya Sharma
Aditya is a content writer with 5+ years of experience writing for various industries including Marketing, SaaS, B2B, IT, and Edtech among others. You can find him watching anime or playing games when he’s not writing.
Frequently Asked Questions
Real-world data science case studies differ significantly from academic examples. While academic exercises often feature clean, well-structured data and simplified scenarios, real-world projects tackle messy, diverse data sources with practical constraints and genuine business objectives. These case studies reflect the complexities data scientists face when translating data into actionable insights in the corporate world.
Real-world data science projects come with common challenges. Data quality issues, including missing or inaccurate data, can hinder analysis. Domain expertise gaps may result in misinterpretation of results. Resource constraints might limit project scope or access to necessary tools and talent. Ethical considerations, like privacy and bias, demand careful handling.
Lastly, as data and business needs evolve, data science projects must adapt and stay relevant, posing an ongoing challenge.
Real-world data science case studies play a crucial role in helping companies make informed decisions. By analyzing their own data, businesses gain valuable insights into customer behavior, market trends, and operational efficiencies.
These insights empower data-driven strategies, aiding in more effective resource allocation, product development, and marketing efforts. Ultimately, case studies bridge the gap between data science and business decision-making, enhancing a company's ability to thrive in a competitive landscape.
Key takeaways from these case studies for organizations include the importance of cultivating a data-driven culture that values evidence-based decision-making. Investing in robust data infrastructure is essential to support data initiatives. Collaborating closely between data scientists and domain experts ensures that insights align with business goals.
Finally, continuous monitoring and refinement of data solutions are critical for maintaining relevance and effectiveness in a dynamic business environment. Embracing these principles can lead to tangible benefits and sustainable success in real-world data science endeavors.
Data science is a powerful driver of innovation and problem-solving across diverse industries. By harnessing data, organizations can uncover hidden patterns, automate repetitive tasks, optimize operations, and make informed decisions.
In healthcare, for example, data-driven diagnostics and treatment plans improve patient outcomes. In finance, predictive analytics enhances risk management. In transportation, route optimization reduces costs and emissions. Data science empowers industries to innovate and solve complex challenges in ways that were previously unimaginable.
Hire remote developers
Tell us the skills you need and we'll find the best developer for you in days, not weeks.
10 Real World Data Science Case Studies Projects with Example
Top 10 Data Science Case Studies Projects with Examples and Solutions in Python to inspire your data science learning in 2023.
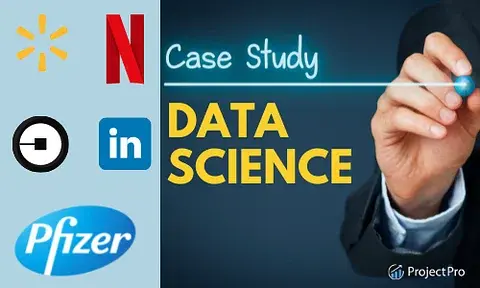
BelData science has been a trending buzzword in recent times. With wide applications in various sectors like healthcare , education, retail, transportation, media, and banking -data science applications are at the core of pretty much every industry out there. The possibilities are endless: analysis of frauds in the finance sector or the personalization of recommendations on eCommerce businesses. We have developed ten exciting data science case studies to explain how data science is leveraged across various industries to make smarter decisions and develop innovative personalized products tailored to specific customers.

Walmart Sales Forecasting Data Science Project
Downloadable solution code | Explanatory videos | Tech Support
Table of Contents
Data science case studies in retail , data science case study examples in entertainment industry , data analytics case study examples in travel industry , case studies for data analytics in social media , real world data science projects in healthcare, data analytics case studies in oil and gas, what is a case study in data science, how do you prepare a data science case study, 10 most interesting data science case studies with examples.
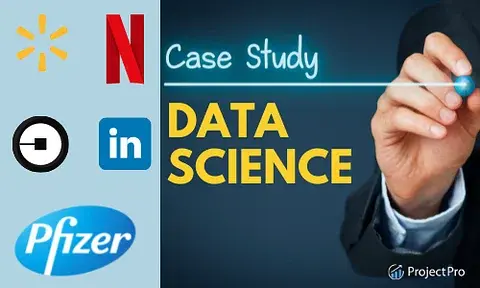
So, without much ado, let's get started with data science business case studies !
With humble beginnings as a simple discount retailer, today, Walmart operates in 10,500 stores and clubs in 24 countries and eCommerce websites, employing around 2.2 million people around the globe. For the fiscal year ended January 31, 2021, Walmart's total revenue was $559 billion showing a growth of $35 billion with the expansion of the eCommerce sector. Walmart is a data-driven company that works on the principle of 'Everyday low cost' for its consumers. To achieve this goal, they heavily depend on the advances of their data science and analytics department for research and development, also known as Walmart Labs. Walmart is home to the world's largest private cloud, which can manage 2.5 petabytes of data every hour! To analyze this humongous amount of data, Walmart has created 'Data Café,' a state-of-the-art analytics hub located within its Bentonville, Arkansas headquarters. The Walmart Labs team heavily invests in building and managing technologies like cloud, data, DevOps , infrastructure, and security.
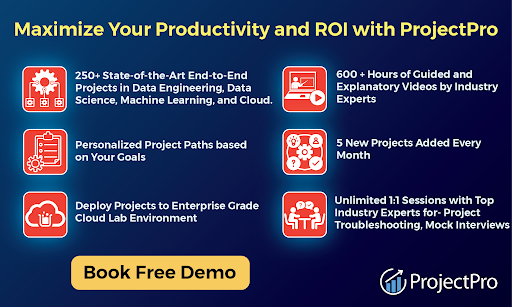
Walmart is experiencing massive digital growth as the world's largest retailer . Walmart has been leveraging Big data and advances in data science to build solutions to enhance, optimize and customize the shopping experience and serve their customers in a better way. At Walmart Labs, data scientists are focused on creating data-driven solutions that power the efficiency and effectiveness of complex supply chain management processes. Here are some of the applications of data science at Walmart:
i) Personalized Customer Shopping Experience
Walmart analyses customer preferences and shopping patterns to optimize the stocking and displaying of merchandise in their stores. Analysis of Big data also helps them understand new item sales, make decisions on discontinuing products, and the performance of brands.
ii) Order Sourcing and On-Time Delivery Promise
Millions of customers view items on Walmart.com, and Walmart provides each customer a real-time estimated delivery date for the items purchased. Walmart runs a backend algorithm that estimates this based on the distance between the customer and the fulfillment center, inventory levels, and shipping methods available. The supply chain management system determines the optimum fulfillment center based on distance and inventory levels for every order. It also has to decide on the shipping method to minimize transportation costs while meeting the promised delivery date.
Here's what valued users are saying about ProjectPro
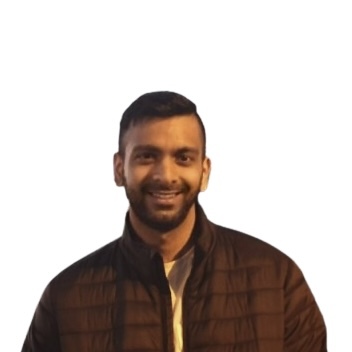
Gautam Vermani
Data Consultant at Confidential
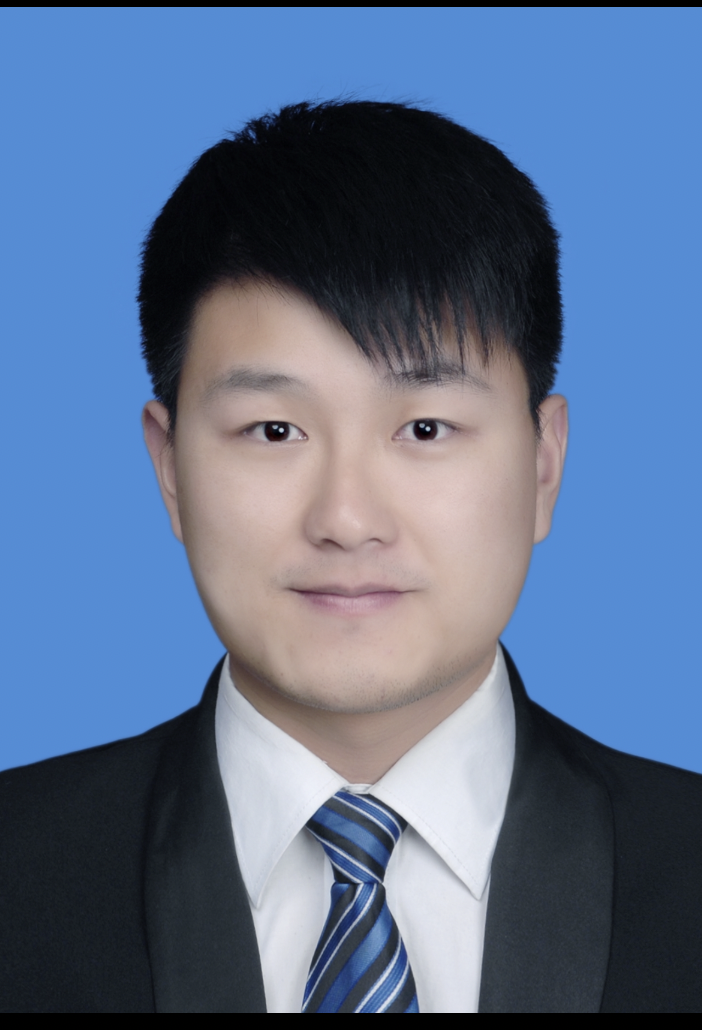
Graduate Research assistance at Stony Brook University
Not sure what you are looking for?
iii) Packing Optimization
Also known as Box recommendation is a daily occurrence in the shipping of items in retail and eCommerce business. When items of an order or multiple orders for the same customer are ready for packing, Walmart has developed a recommender system that picks the best-sized box which holds all the ordered items with the least in-box space wastage within a fixed amount of time. This Bin Packing problem is a classic NP-Hard problem familiar to data scientists .
Whenever items of an order or multiple orders placed by the same customer are picked from the shelf and are ready for packing, the box recommendation system determines the best-sized box to hold all the ordered items with a minimum of in-box space wasted. This problem is known as the Bin Packing Problem, another classic NP-Hard problem familiar to data scientists.
Here is a link to a sales prediction data science case study to help you understand the applications of Data Science in the real world. Walmart Sales Forecasting Project uses historical sales data for 45 Walmart stores located in different regions. Each store contains many departments, and you must build a model to project the sales for each department in each store. This data science case study aims to create a predictive model to predict the sales of each product. You can also try your hands-on Inventory Demand Forecasting Data Science Project to develop a machine learning model to forecast inventory demand accurately based on historical sales data.
Get Closer To Your Dream of Becoming a Data Scientist with 70+ Solved End-to-End ML Projects
Amazon is an American multinational technology-based company based in Seattle, USA. It started as an online bookseller, but today it focuses on eCommerce, cloud computing , digital streaming, and artificial intelligence . It hosts an estimate of 1,000,000,000 gigabytes of data across more than 1,400,000 servers. Through its constant innovation in data science and big data Amazon is always ahead in understanding its customers. Here are a few data analytics case study examples at Amazon:
i) Recommendation Systems
Data science models help amazon understand the customers' needs and recommend them to them before the customer searches for a product; this model uses collaborative filtering. Amazon uses 152 million customer purchases data to help users to decide on products to be purchased. The company generates 35% of its annual sales using the Recommendation based systems (RBS) method.
Here is a Recommender System Project to help you build a recommendation system using collaborative filtering.
ii) Retail Price Optimization
Amazon product prices are optimized based on a predictive model that determines the best price so that the users do not refuse to buy it based on price. The model carefully determines the optimal prices considering the customers' likelihood of purchasing the product and thinks the price will affect the customers' future buying patterns. Price for a product is determined according to your activity on the website, competitors' pricing, product availability, item preferences, order history, expected profit margin, and other factors.
Check Out this Retail Price Optimization Project to build a Dynamic Pricing Model.
iii) Fraud Detection
Being a significant eCommerce business, Amazon remains at high risk of retail fraud. As a preemptive measure, the company collects historical and real-time data for every order. It uses Machine learning algorithms to find transactions with a higher probability of being fraudulent. This proactive measure has helped the company restrict clients with an excessive number of returns of products.
You can look at this Credit Card Fraud Detection Project to implement a fraud detection model to classify fraudulent credit card transactions.
New Projects
Let us explore data analytics case study examples in the entertainment indusry.
Ace Your Next Job Interview with Mock Interviews from Experts to Improve Your Skills and Boost Confidence!
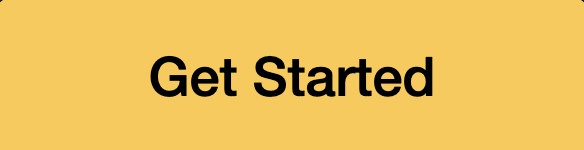
Netflix started as a DVD rental service in 1997 and then has expanded into the streaming business. Headquartered in Los Gatos, California, Netflix is the largest content streaming company in the world. Currently, Netflix has over 208 million paid subscribers worldwide, and with thousands of smart devices which are presently streaming supported, Netflix has around 3 billion hours watched every month. The secret to this massive growth and popularity of Netflix is its advanced use of data analytics and recommendation systems to provide personalized and relevant content recommendations to its users. The data is collected over 100 billion events every day. Here are a few examples of data analysis case studies applied at Netflix :
i) Personalized Recommendation System
Netflix uses over 1300 recommendation clusters based on consumer viewing preferences to provide a personalized experience. Some of the data that Netflix collects from its users include Viewing time, platform searches for keywords, Metadata related to content abandonment, such as content pause time, rewind, rewatched. Using this data, Netflix can predict what a viewer is likely to watch and give a personalized watchlist to a user. Some of the algorithms used by the Netflix recommendation system are Personalized video Ranking, Trending now ranker, and the Continue watching now ranker.
ii) Content Development using Data Analytics
Netflix uses data science to analyze the behavior and patterns of its user to recognize themes and categories that the masses prefer to watch. This data is used to produce shows like The umbrella academy, and Orange Is the New Black, and the Queen's Gambit. These shows seem like a huge risk but are significantly based on data analytics using parameters, which assured Netflix that they would succeed with its audience. Data analytics is helping Netflix come up with content that their viewers want to watch even before they know they want to watch it.
iii) Marketing Analytics for Campaigns
Netflix uses data analytics to find the right time to launch shows and ad campaigns to have maximum impact on the target audience. Marketing analytics helps come up with different trailers and thumbnails for other groups of viewers. For example, the House of Cards Season 5 trailer with a giant American flag was launched during the American presidential elections, as it would resonate well with the audience.
Here is a Customer Segmentation Project using association rule mining to understand the primary grouping of customers based on various parameters.
Get FREE Access to Machine Learning Example Codes for Data Cleaning , Data Munging, and Data Visualization
In a world where Purchasing music is a thing of the past and streaming music is a current trend, Spotify has emerged as one of the most popular streaming platforms. With 320 million monthly users, around 4 billion playlists, and approximately 2 million podcasts, Spotify leads the pack among well-known streaming platforms like Apple Music, Wynk, Songza, amazon music, etc. The success of Spotify has mainly depended on data analytics. By analyzing massive volumes of listener data, Spotify provides real-time and personalized services to its listeners. Most of Spotify's revenue comes from paid premium subscriptions. Here are some of the examples of case study on data analytics used by Spotify to provide enhanced services to its listeners:
i) Personalization of Content using Recommendation Systems
Spotify uses Bart or Bayesian Additive Regression Trees to generate music recommendations to its listeners in real-time. Bart ignores any song a user listens to for less than 30 seconds. The model is retrained every day to provide updated recommendations. A new Patent granted to Spotify for an AI application is used to identify a user's musical tastes based on audio signals, gender, age, accent to make better music recommendations.
Spotify creates daily playlists for its listeners, based on the taste profiles called 'Daily Mixes,' which have songs the user has added to their playlists or created by the artists that the user has included in their playlists. It also includes new artists and songs that the user might be unfamiliar with but might improve the playlist. Similar to it is the weekly 'Release Radar' playlists that have newly released artists' songs that the listener follows or has liked before.
ii) Targetted marketing through Customer Segmentation
With user data for enhancing personalized song recommendations, Spotify uses this massive dataset for targeted ad campaigns and personalized service recommendations for its users. Spotify uses ML models to analyze the listener's behavior and group them based on music preferences, age, gender, ethnicity, etc. These insights help them create ad campaigns for a specific target audience. One of their well-known ad campaigns was the meme-inspired ads for potential target customers, which was a huge success globally.
iii) CNN's for Classification of Songs and Audio Tracks
Spotify builds audio models to evaluate the songs and tracks, which helps develop better playlists and recommendations for its users. These allow Spotify to filter new tracks based on their lyrics and rhythms and recommend them to users like similar tracks ( collaborative filtering). Spotify also uses NLP ( Natural language processing) to scan articles and blogs to analyze the words used to describe songs and artists. These analytical insights can help group and identify similar artists and songs and leverage them to build playlists.
Here is a Music Recommender System Project for you to start learning. We have listed another music recommendations dataset for you to use for your projects: Dataset1 . You can use this dataset of Spotify metadata to classify songs based on artists, mood, liveliness. Plot histograms, heatmaps to get a better understanding of the dataset. Use classification algorithms like logistic regression, SVM, and Principal component analysis to generate valuable insights from the dataset.
Explore Categories
Below you will find case studies for data analytics in the travel and tourism industry.
Airbnb was born in 2007 in San Francisco and has since grown to 4 million Hosts and 5.6 million listings worldwide who have welcomed more than 1 billion guest arrivals in almost every country across the globe. Airbnb is active in every country on the planet except for Iran, Sudan, Syria, and North Korea. That is around 97.95% of the world. Using data as a voice of their customers, Airbnb uses the large volume of customer reviews, host inputs to understand trends across communities, rate user experiences, and uses these analytics to make informed decisions to build a better business model. The data scientists at Airbnb are developing exciting new solutions to boost the business and find the best mapping for its customers and hosts. Airbnb data servers serve approximately 10 million requests a day and process around one million search queries. Data is the voice of customers at AirBnB and offers personalized services by creating a perfect match between the guests and hosts for a supreme customer experience.
i) Recommendation Systems and Search Ranking Algorithms
Airbnb helps people find 'local experiences' in a place with the help of search algorithms that make searches and listings precise. Airbnb uses a 'listing quality score' to find homes based on the proximity to the searched location and uses previous guest reviews. Airbnb uses deep neural networks to build models that take the guest's earlier stays into account and area information to find a perfect match. The search algorithms are optimized based on guest and host preferences, rankings, pricing, and availability to understand users’ needs and provide the best match possible.
ii) Natural Language Processing for Review Analysis
Airbnb characterizes data as the voice of its customers. The customer and host reviews give a direct insight into the experience. The star ratings alone cannot be an excellent way to understand it quantitatively. Hence Airbnb uses natural language processing to understand reviews and the sentiments behind them. The NLP models are developed using Convolutional neural networks .
Practice this Sentiment Analysis Project for analyzing product reviews to understand the basic concepts of natural language processing.
iii) Smart Pricing using Predictive Analytics
The Airbnb hosts community uses the service as a supplementary income. The vacation homes and guest houses rented to customers provide for rising local community earnings as Airbnb guests stay 2.4 times longer and spend approximately 2.3 times the money compared to a hotel guest. The profits are a significant positive impact on the local neighborhood community. Airbnb uses predictive analytics to predict the prices of the listings and help the hosts set a competitive and optimal price. The overall profitability of the Airbnb host depends on factors like the time invested by the host and responsiveness to changing demands for different seasons. The factors that impact the real-time smart pricing are the location of the listing, proximity to transport options, season, and amenities available in the neighborhood of the listing.
Here is a Price Prediction Project to help you understand the concept of predictive analysis which is widely common in case studies for data analytics.
Uber is the biggest global taxi service provider. As of December 2018, Uber has 91 million monthly active consumers and 3.8 million drivers. Uber completes 14 million trips each day. Uber uses data analytics and big data-driven technologies to optimize their business processes and provide enhanced customer service. The Data Science team at uber has been exploring futuristic technologies to provide better service constantly. Machine learning and data analytics help Uber make data-driven decisions that enable benefits like ride-sharing, dynamic price surges, better customer support, and demand forecasting. Here are some of the real world data science projects used by uber:
i) Dynamic Pricing for Price Surges and Demand Forecasting
Uber prices change at peak hours based on demand. Uber uses surge pricing to encourage more cab drivers to sign up with the company, to meet the demand from the passengers. When the prices increase, the driver and the passenger are both informed about the surge in price. Uber uses a predictive model for price surging called the 'Geosurge' ( patented). It is based on the demand for the ride and the location.
ii) One-Click Chat
Uber has developed a Machine learning and natural language processing solution called one-click chat or OCC for coordination between drivers and users. This feature anticipates responses for commonly asked questions, making it easy for the drivers to respond to customer messages. Drivers can reply with the clock of just one button. One-Click chat is developed on Uber's machine learning platform Michelangelo to perform NLP on rider chat messages and generate appropriate responses to them.
iii) Customer Retention
Failure to meet the customer demand for cabs could lead to users opting for other services. Uber uses machine learning models to bridge this demand-supply gap. By using prediction models to predict the demand in any location, uber retains its customers. Uber also uses a tier-based reward system, which segments customers into different levels based on usage. The higher level the user achieves, the better are the perks. Uber also provides personalized destination suggestions based on the history of the user and their frequently traveled destinations.
You can take a look at this Python Chatbot Project and build a simple chatbot application to understand better the techniques used for natural language processing. You can also practice the working of a demand forecasting model with this project using time series analysis. You can look at this project which uses time series forecasting and clustering on a dataset containing geospatial data for forecasting customer demand for ola rides.
Explore More Data Science and Machine Learning Projects for Practice. Fast-Track Your Career Transition with ProjectPro
7) LinkedIn
LinkedIn is the largest professional social networking site with nearly 800 million members in more than 200 countries worldwide. Almost 40% of the users access LinkedIn daily, clocking around 1 billion interactions per month. The data science team at LinkedIn works with this massive pool of data to generate insights to build strategies, apply algorithms and statistical inferences to optimize engineering solutions, and help the company achieve its goals. Here are some of the real world data science projects at LinkedIn:
i) LinkedIn Recruiter Implement Search Algorithms and Recommendation Systems
LinkedIn Recruiter helps recruiters build and manage a talent pool to optimize the chances of hiring candidates successfully. This sophisticated product works on search and recommendation engines. The LinkedIn recruiter handles complex queries and filters on a constantly growing large dataset. The results delivered have to be relevant and specific. The initial search model was based on linear regression but was eventually upgraded to Gradient Boosted decision trees to include non-linear correlations in the dataset. In addition to these models, the LinkedIn recruiter also uses the Generalized Linear Mix model to improve the results of prediction problems to give personalized results.
ii) Recommendation Systems Personalized for News Feed
The LinkedIn news feed is the heart and soul of the professional community. A member's newsfeed is a place to discover conversations among connections, career news, posts, suggestions, photos, and videos. Every time a member visits LinkedIn, machine learning algorithms identify the best exchanges to be displayed on the feed by sorting through posts and ranking the most relevant results on top. The algorithms help LinkedIn understand member preferences and help provide personalized news feeds. The algorithms used include logistic regression, gradient boosted decision trees and neural networks for recommendation systems.
iii) CNN's to Detect Inappropriate Content
To provide a professional space where people can trust and express themselves professionally in a safe community has been a critical goal at LinkedIn. LinkedIn has heavily invested in building solutions to detect fake accounts and abusive behavior on their platform. Any form of spam, harassment, inappropriate content is immediately flagged and taken down. These can range from profanity to advertisements for illegal services. LinkedIn uses a Convolutional neural networks based machine learning model. This classifier trains on a training dataset containing accounts labeled as either "inappropriate" or "appropriate." The inappropriate list consists of accounts having content from "blocklisted" phrases or words and a small portion of manually reviewed accounts reported by the user community.
Here is a Text Classification Project to help you understand NLP basics for text classification. You can find a news recommendation system dataset to help you build a personalized news recommender system. You can also use this dataset to build a classifier using logistic regression, Naive Bayes, or Neural networks to classify toxic comments.
Get confident to build end-to-end projects
Access to a curated library of 250+ end-to-end industry projects with solution code, videos and tech support.
Pfizer is a multinational pharmaceutical company headquartered in New York, USA. One of the largest pharmaceutical companies globally known for developing a wide range of medicines and vaccines in disciplines like immunology, oncology, cardiology, and neurology. Pfizer became a household name in 2010 when it was the first to have a COVID-19 vaccine with FDA. In early November 2021, The CDC has approved the Pfizer vaccine for kids aged 5 to 11. Pfizer has been using machine learning and artificial intelligence to develop drugs and streamline trials, which played a massive role in developing and deploying the COVID-19 vaccine. Here are a few data analytics case studies by Pfizer :
i) Identifying Patients for Clinical Trials
Artificial intelligence and machine learning are used to streamline and optimize clinical trials to increase their efficiency. Natural language processing and exploratory data analysis of patient records can help identify suitable patients for clinical trials. These can help identify patients with distinct symptoms. These can help examine interactions of potential trial members' specific biomarkers, predict drug interactions and side effects which can help avoid complications. Pfizer's AI implementation helped rapidly identify signals within the noise of millions of data points across their 44,000-candidate COVID-19 clinical trial.
ii) Supply Chain and Manufacturing
Data science and machine learning techniques help pharmaceutical companies better forecast demand for vaccines and drugs and distribute them efficiently. Machine learning models can help identify efficient supply systems by automating and optimizing the production steps. These will help supply drugs customized to small pools of patients in specific gene pools. Pfizer uses Machine learning to predict the maintenance cost of equipment used. Predictive maintenance using AI is the next big step for Pharmaceutical companies to reduce costs.
iii) Drug Development
Computer simulations of proteins, and tests of their interactions, and yield analysis help researchers develop and test drugs more efficiently. In 2016 Watson Health and Pfizer announced a collaboration to utilize IBM Watson for Drug Discovery to help accelerate Pfizer's research in immuno-oncology, an approach to cancer treatment that uses the body's immune system to help fight cancer. Deep learning models have been used recently for bioactivity and synthesis prediction for drugs and vaccines in addition to molecular design. Deep learning has been a revolutionary technique for drug discovery as it factors everything from new applications of medications to possible toxic reactions which can save millions in drug trials.
You can create a Machine learning model to predict molecular activity to help design medicine using this dataset . You may build a CNN or a Deep neural network for this data analyst case study project.
Access Data Science and Machine Learning Project Code Examples
9) Shell Data Analyst Case Study Project
Shell is a global group of energy and petrochemical companies with over 80,000 employees in around 70 countries. Shell uses advanced technologies and innovations to help build a sustainable energy future. Shell is going through a significant transition as the world needs more and cleaner energy solutions to be a clean energy company by 2050. It requires substantial changes in the way in which energy is used. Digital technologies, including AI and Machine Learning, play an essential role in this transformation. These include efficient exploration and energy production, more reliable manufacturing, more nimble trading, and a personalized customer experience. Using AI in various phases of the organization will help achieve this goal and stay competitive in the market. Here are a few data analytics case studies in the petrochemical industry:
i) Precision Drilling
Shell is involved in the processing mining oil and gas supply, ranging from mining hydrocarbons to refining the fuel to retailing them to customers. Recently Shell has included reinforcement learning to control the drilling equipment used in mining. Reinforcement learning works on a reward-based system based on the outcome of the AI model. The algorithm is designed to guide the drills as they move through the surface, based on the historical data from drilling records. It includes information such as the size of drill bits, temperatures, pressures, and knowledge of the seismic activity. This model helps the human operator understand the environment better, leading to better and faster results will minor damage to machinery used.
ii) Efficient Charging Terminals
Due to climate changes, governments have encouraged people to switch to electric vehicles to reduce carbon dioxide emissions. However, the lack of public charging terminals has deterred people from switching to electric cars. Shell uses AI to monitor and predict the demand for terminals to provide efficient supply. Multiple vehicles charging from a single terminal may create a considerable grid load, and predictions on demand can help make this process more efficient.
iii) Monitoring Service and Charging Stations
Another Shell initiative trialed in Thailand and Singapore is the use of computer vision cameras, which can think and understand to watch out for potentially hazardous activities like lighting cigarettes in the vicinity of the pumps while refueling. The model is built to process the content of the captured images and label and classify it. The algorithm can then alert the staff and hence reduce the risk of fires. You can further train the model to detect rash driving or thefts in the future.
Here is a project to help you understand multiclass image classification. You can use the Hourly Energy Consumption Dataset to build an energy consumption prediction model. You can use time series with XGBoost to develop your model.
10) Zomato Case Study on Data Analytics
Zomato was founded in 2010 and is currently one of the most well-known food tech companies. Zomato offers services like restaurant discovery, home delivery, online table reservation, online payments for dining, etc. Zomato partners with restaurants to provide tools to acquire more customers while also providing delivery services and easy procurement of ingredients and kitchen supplies. Currently, Zomato has over 2 lakh restaurant partners and around 1 lakh delivery partners. Zomato has closed over ten crore delivery orders as of date. Zomato uses ML and AI to boost their business growth, with the massive amount of data collected over the years from food orders and user consumption patterns. Here are a few examples of data analyst case study project developed by the data scientists at Zomato:
i) Personalized Recommendation System for Homepage
Zomato uses data analytics to create personalized homepages for its users. Zomato uses data science to provide order personalization, like giving recommendations to the customers for specific cuisines, locations, prices, brands, etc. Restaurant recommendations are made based on a customer's past purchases, browsing history, and what other similar customers in the vicinity are ordering. This personalized recommendation system has led to a 15% improvement in order conversions and click-through rates for Zomato.
You can use the Restaurant Recommendation Dataset to build a restaurant recommendation system to predict what restaurants customers are most likely to order from, given the customer location, restaurant information, and customer order history.
ii) Analyzing Customer Sentiment
Zomato uses Natural language processing and Machine learning to understand customer sentiments using social media posts and customer reviews. These help the company gauge the inclination of its customer base towards the brand. Deep learning models analyze the sentiments of various brand mentions on social networking sites like Twitter, Instagram, Linked In, and Facebook. These analytics give insights to the company, which helps build the brand and understand the target audience.
iii) Predicting Food Preparation Time (FPT)
Food delivery time is an essential variable in the estimated delivery time of the order placed by the customer using Zomato. The food preparation time depends on numerous factors like the number of dishes ordered, time of the day, footfall in the restaurant, day of the week, etc. Accurate prediction of the food preparation time can help make a better prediction of the Estimated delivery time, which will help delivery partners less likely to breach it. Zomato uses a Bidirectional LSTM-based deep learning model that considers all these features and provides food preparation time for each order in real-time.
Data scientists are companies' secret weapons when analyzing customer sentiments and behavior and leveraging it to drive conversion, loyalty, and profits. These 10 data science case studies projects with examples and solutions show you how various organizations use data science technologies to succeed and be at the top of their field! To summarize, Data Science has not only accelerated the performance of companies but has also made it possible to manage & sustain their performance with ease.
FAQs on Data Analysis Case Studies
A case study in data science is an in-depth analysis of a real-world problem using data-driven approaches. It involves collecting, cleaning, and analyzing data to extract insights and solve challenges, offering practical insights into how data science techniques can address complex issues across various industries.
To create a data science case study, identify a relevant problem, define objectives, and gather suitable data. Clean and preprocess data, perform exploratory data analysis, and apply appropriate algorithms for analysis. Summarize findings, visualize results, and provide actionable recommendations, showcasing the problem-solving potential of data science techniques.
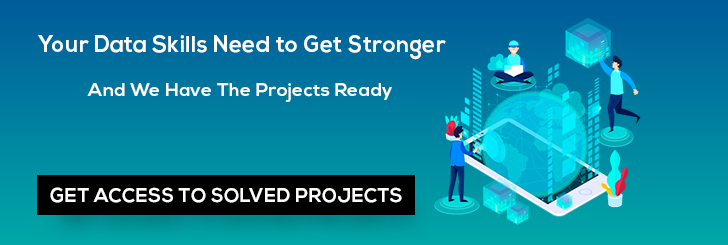
About the Author

ProjectPro is the only online platform designed to help professionals gain practical, hands-on experience in big data, data engineering, data science, and machine learning related technologies. Having over 270+ reusable project templates in data science and big data with step-by-step walkthroughs,

© 2024
© 2024 Iconiq Inc.
Privacy policy
User policy
Write for ProjectPro
For enquiries call:
+1-469-442-0620

- Data Science
Top 12 Data Science Case Studies: Across Various Industries
Home Blog Data Science Top 12 Data Science Case Studies: Across Various Industries

Data science has become popular in the last few years due to its successful application in making business decisions. Data scientists have been using data science techniques to solve challenging real-world issues in healthcare, agriculture, manufacturing, automotive, and many more. For this purpose, a data enthusiast needs to stay updated with the latest technological advancements in AI . An excellent way to achieve this is through reading industry data science case studies. I recommend checking out Data Science With Python course syllabus to start your data science journey. In this discussion, I will present some case studies to you that contain detailed and systematic data analysis of people, objects, or entities focusing on multiple factors present in the dataset. Aspiring and practising data scientists can motivate themselves to learn more about the sector, an alternative way of thinking, or methods to improve their organization based on comparable experiences. Almost every industry uses data science in some way. You can learn more about data science fundamentals in this data science course content . From my standpoint, data scientists may use it to spot fraudulent conduct in insurance claims. Automotive data scientists may use it to improve self-driving cars. In contrast, e-commerce data scientists can use it to add more personalization for their consumers—the possibilities are unlimited and unexplored. Let’s look at the top eight data science case studies in this article so you can understand how businesses from many sectors have benefitted from data science to boost productivity, revenues, and more. Read on to explore more or use the following links to go straight to the case study of your choice.

Examples of Data Science Case Studies
- Hospitality: Airbnb focuses on growth by analyzing customer voice using data science. Qantas uses predictive analytics to mitigate losses
- Healthcare: Novo Nordisk is Driving innovation with NLP. AstraZeneca harnesses data for innovation in medicine
- Covid 19: Johnson and Johnson use s d ata science to fight the Pandemic
- E-commerce: Amazon uses data science to personalize shop p ing experiences and improve customer satisfaction
- Supply chain management : UPS optimizes supp l y chain with big data analytics
- Meteorology: IMD leveraged data science to achieve a rec o rd 1.2m evacuation before cyclone ''Fani''
- Entertainment Industry: Netflix u ses data science to personalize the content and improve recommendations. Spotify uses big data to deliver a rich user experience for online music streaming
- Banking and Finance: HDFC utilizes Big D ata Analytics to increase income and enhance the banking experience
Top 8 Data Science Case Studies [For Various Industries]
1. data science in hospitality industry.
In the hospitality sector, data analytics assists hotels in better pricing strategies, customer analysis, brand marketing , tracking market trends, and many more.
Airbnb focuses on growth by analyzing customer voice using data science. A famous example in this sector is the unicorn '' Airbnb '', a startup that focussed on data science early to grow and adapt to the market faster. This company witnessed a 43000 percent hypergrowth in as little as five years using data science. They included data science techniques to process the data, translate this data for better understanding the voice of the customer, and use the insights for decision making. They also scaled the approach to cover all aspects of the organization. Airbnb uses statistics to analyze and aggregate individual experiences to establish trends throughout the community. These analyzed trends using data science techniques impact their business choices while helping them grow further.
Travel industry and data science
Predictive analytics benefits many parameters in the travel industry. These companies can use recommendation engines with data science to achieve higher personalization and improved user interactions. They can study and cross-sell products by recommending relevant products to drive sales and increase revenue. Data science is also employed in analyzing social media posts for sentiment analysis, bringing invaluable travel-related insights. Whether these views are positive, negative, or neutral can help these agencies understand the user demographics, the expected experiences by their target audiences, and so on. These insights are essential for developing aggressive pricing strategies to draw customers and provide better customization to customers in the travel packages and allied services. Travel agencies like Expedia and Booking.com use predictive analytics to create personalized recommendations, product development, and effective marketing of their products. Not just travel agencies but airlines also benefit from the same approach. Airlines frequently face losses due to flight cancellations, disruptions, and delays. Data science helps them identify patterns and predict possible bottlenecks, thereby effectively mitigating the losses and improving the overall customer traveling experience.
How Qantas uses predictive analytics to mitigate losses
Qantas , one of Australia's largest airlines, leverages data science to reduce losses caused due to flight delays, disruptions, and cancellations. They also use it to provide a better traveling experience for their customers by reducing the number and length of delays caused due to huge air traffic, weather conditions, or difficulties arising in operations. Back in 2016, when heavy storms badly struck Australia's east coast, only 15 out of 436 Qantas flights were cancelled due to their predictive analytics-based system against their competitor Virgin Australia, which witnessed 70 cancelled flights out of 320.
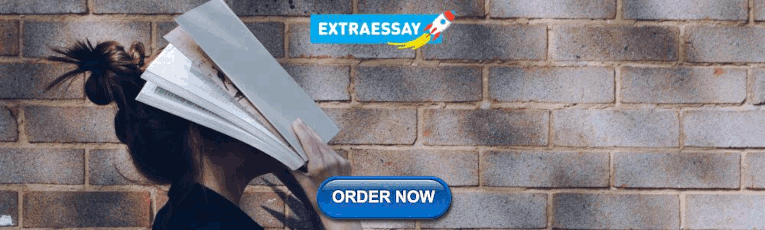
2. Data Science in Healthcare
The Healthcare sector is immensely benefiting from the advancements in AI. Data science, especially in medical imaging, has been helping healthcare professionals come up with better diagnoses and effective treatments for patients. Similarly, several advanced healthcare analytics tools have been developed to generate clinical insights for improving patient care. These tools also assist in defining personalized medications for patients reducing operating costs for clinics and hospitals. Apart from medical imaging or computer vision, Natural Language Processing (NLP) is frequently used in the healthcare domain to study the published textual research data.
A. Pharmaceutical
Driving innovation with NLP: Novo Nordisk. Novo Nordisk uses the Linguamatics NLP platform from internal and external data sources for text mining purposes that include scientific abstracts, patents, grants, news, tech transfer offices from universities worldwide, and more. These NLP queries run across sources for the key therapeutic areas of interest to the Novo Nordisk R&D community. Several NLP algorithms have been developed for the topics of safety, efficacy, randomized controlled trials, patient populations, dosing, and devices. Novo Nordisk employs a data pipeline to capitalize the tools' success on real-world data and uses interactive dashboards and cloud services to visualize this standardized structured information from the queries for exploring commercial effectiveness, market situations, potential, and gaps in the product documentation. Through data science, they are able to automate the process of generating insights, save time and provide better insights for evidence-based decision making.
How AstraZeneca harnesses data for innovation in medicine. AstraZeneca is a globally known biotech company that leverages data using AI technology to discover and deliver newer effective medicines faster. Within their R&D teams, they are using AI to decode the big data to understand better diseases like cancer, respiratory disease, and heart, kidney, and metabolic diseases to be effectively treated. Using data science, they can identify new targets for innovative medications. In 2021, they selected the first two AI-generated drug targets collaborating with BenevolentAI in Chronic Kidney Disease and Idiopathic Pulmonary Fibrosis.
Data science is also helping AstraZeneca redesign better clinical trials, achieve personalized medication strategies, and innovate the process of developing new medicines. Their Center for Genomics Research uses data science and AI to analyze around two million genomes by 2026. Apart from this, they are training their AI systems to check these images for disease and biomarkers for effective medicines for imaging purposes. This approach helps them analyze samples accurately and more effortlessly. Moreover, it can cut the analysis time by around 30%.
AstraZeneca also utilizes AI and machine learning to optimize the process at different stages and minimize the overall time for the clinical trials by analyzing the clinical trial data. Summing up, they use data science to design smarter clinical trials, develop innovative medicines, improve drug development and patient care strategies, and many more.
C. Wearable Technology
Wearable technology is a multi-billion-dollar industry. With an increasing awareness about fitness and nutrition, more individuals now prefer using fitness wearables to track their routines and lifestyle choices.
Fitness wearables are convenient to use, assist users in tracking their health, and encourage them to lead a healthier lifestyle. The medical devices in this domain are beneficial since they help monitor the patient's condition and communicate in an emergency situation. The regularly used fitness trackers and smartwatches from renowned companies like Garmin, Apple, FitBit, etc., continuously collect physiological data of the individuals wearing them. These wearable providers offer user-friendly dashboards to their customers for analyzing and tracking progress in their fitness journey.
3. Covid 19 and Data Science
In the past two years of the Pandemic, the power of data science has been more evident than ever. Different pharmaceutical companies across the globe could synthesize Covid 19 vaccines by analyzing the data to understand the trends and patterns of the outbreak. Data science made it possible to track the virus in real-time, predict patterns, devise effective strategies to fight the Pandemic, and many more.
How Johnson and Johnson uses data science to fight the Pandemic
The data science team at Johnson and Johnson leverages real-time data to track the spread of the virus. They built a global surveillance dashboard (granulated to county level) that helps them track the Pandemic's progress, predict potential hotspots of the virus, and narrow down the likely place where they should test its investigational COVID-19 vaccine candidate. The team works with in-country experts to determine whether official numbers are accurate and find the most valid information about case numbers, hospitalizations, mortality and testing rates, social compliance, and local policies to populate this dashboard. The team also studies the data to build models that help the company identify groups of individuals at risk of getting affected by the virus and explore effective treatments to improve patient outcomes.
4. Data Science in E-commerce
In the e-commerce sector , big data analytics can assist in customer analysis, reduce operational costs, forecast trends for better sales, provide personalized shopping experiences to customers, and many more.
Amazon uses data science to personalize shopping experiences and improve customer satisfaction. Amazon is a globally leading eCommerce platform that offers a wide range of online shopping services. Due to this, Amazon generates a massive amount of data that can be leveraged to understand consumer behavior and generate insights on competitors' strategies. Amazon uses its data to provide recommendations to its users on different products and services. With this approach, Amazon is able to persuade its consumers into buying and making additional sales. This approach works well for Amazon as it earns 35% of the revenue yearly with this technique. Additionally, Amazon collects consumer data for faster order tracking and better deliveries.
Similarly, Amazon's virtual assistant, Alexa, can converse in different languages; uses speakers and a camera to interact with the users. Amazon utilizes the audio commands from users to improve Alexa and deliver a better user experience.
5. Data Science in Supply Chain Management
Predictive analytics and big data are driving innovation in the Supply chain domain. They offer greater visibility into the company operations, reduce costs and overheads, forecasting demands, predictive maintenance, product pricing, minimize supply chain interruptions, route optimization, fleet management , drive better performance, and more.
Optimizing supply chain with big data analytics: UPS
UPS is a renowned package delivery and supply chain management company. With thousands of packages being delivered every day, on average, a UPS driver makes about 100 deliveries each business day. On-time and safe package delivery are crucial to UPS's success. Hence, UPS offers an optimized navigation tool ''ORION'' (On-Road Integrated Optimization and Navigation), which uses highly advanced big data processing algorithms. This tool for UPS drivers provides route optimization concerning fuel, distance, and time. UPS utilizes supply chain data analysis in all aspects of its shipping process. Data about packages and deliveries are captured through radars and sensors. The deliveries and routes are optimized using big data systems. Overall, this approach has helped UPS save 1.6 million gallons of gasoline in transportation every year, significantly reducing delivery costs.
6. Data Science in Meteorology
Weather prediction is an interesting application of data science . Businesses like aviation, agriculture and farming, construction, consumer goods, sporting events, and many more are dependent on climatic conditions. The success of these businesses is closely tied to the weather, as decisions are made after considering the weather predictions from the meteorological department.
Besides, weather forecasts are extremely helpful for individuals to manage their allergic conditions. One crucial application of weather forecasting is natural disaster prediction and risk management.
Weather forecasts begin with a large amount of data collection related to the current environmental conditions (wind speed, temperature, humidity, clouds captured at a specific location and time) using sensors on IoT (Internet of Things) devices and satellite imagery. This gathered data is then analyzed using the understanding of atmospheric processes, and machine learning models are built to make predictions on upcoming weather conditions like rainfall or snow prediction. Although data science cannot help avoid natural calamities like floods, hurricanes, or forest fires. Tracking these natural phenomena well ahead of their arrival is beneficial. Such predictions allow governments sufficient time to take necessary steps and measures to ensure the safety of the population.
IMD leveraged data science to achieve a record 1.2m evacuation before cyclone ''Fani''
Most d ata scientist’s responsibilities rely on satellite images to make short-term forecasts, decide whether a forecast is correct, and validate models. Machine Learning is also used for pattern matching in this case. It can forecast future weather conditions if it recognizes a past pattern. When employing dependable equipment, sensor data is helpful to produce local forecasts about actual weather models. IMD used satellite pictures to study the low-pressure zones forming off the Odisha coast (India). In April 2019, thirteen days before cyclone ''Fani'' reached the area, IMD (India Meteorological Department) warned that a massive storm was underway, and the authorities began preparing for safety measures.
It was one of the most powerful cyclones to strike India in the recent 20 years, and a record 1.2 million people were evacuated in less than 48 hours, thanks to the power of data science.
7. Data Science in the Entertainment Industry
Due to the Pandemic, demand for OTT (Over-the-top) media platforms has grown significantly. People prefer watching movies and web series or listening to the music of their choice at leisure in the convenience of their homes. This sudden growth in demand has given rise to stiff competition. Every platform now uses data analytics in different capacities to provide better-personalized recommendations to its subscribers and improve user experience.
How Netflix uses data science to personalize the content and improve recommendations
Netflix is an extremely popular internet television platform with streamable content offered in several languages and caters to various audiences. In 2006, when Netflix entered this media streaming market, they were interested in increasing the efficiency of their existing ''Cinematch'' platform by 10% and hence, offered a prize of $1 million to the winning team. This approach was successful as they found a solution developed by the BellKor team at the end of the competition that increased prediction accuracy by 10.06%. Over 200 work hours and an ensemble of 107 algorithms provided this result. These winning algorithms are now a part of the Netflix recommendation system.
Netflix also employs Ranking Algorithms to generate personalized recommendations of movies and TV Shows appealing to its users.
Spotify uses big data to deliver a rich user experience for online music streaming
Personalized online music streaming is another area where data science is being used. Spotify is a well-known on-demand music service provider launched in 2008, which effectively leveraged big data to create personalized experiences for each user. It is a huge platform with more than 24 million subscribers and hosts a database of nearly 20million songs; they use the big data to offer a rich experience to its users. Spotify uses this big data and various algorithms to train machine learning models to provide personalized content. Spotify offers a "Discover Weekly" feature that generates a personalized playlist of fresh unheard songs matching the user's taste every week. Using the Spotify "Wrapped" feature, users get an overview of their most favorite or frequently listened songs during the entire year in December. Spotify also leverages the data to run targeted ads to grow its business. Thus, Spotify utilizes the user data, which is big data and some external data, to deliver a high-quality user experience.
8. Data Science in Banking and Finance
Data science is extremely valuable in the Banking and Finance industry . Several high priority aspects of Banking and Finance like credit risk modeling (possibility of repayment of a loan), fraud detection (detection of malicious or irregularities in transactional patterns using machine learning), identifying customer lifetime value (prediction of bank performance based on existing and potential customers), customer segmentation (customer profiling based on behavior and characteristics for personalization of offers and services). Finally, data science is also used in real-time predictive analytics (computational techniques to predict future events).
How HDFC utilizes Big Data Analytics to increase revenues and enhance the banking experience
One of the major private banks in India, HDFC Bank , was an early adopter of AI. It started with Big Data analytics in 2004, intending to grow its revenue and understand its customers and markets better than its competitors. Back then, they were trendsetters by setting up an enterprise data warehouse in the bank to be able to track the differentiation to be given to customers based on their relationship value with HDFC Bank. Data science and analytics have been crucial in helping HDFC bank segregate its customers and offer customized personal or commercial banking services. The analytics engine and SaaS use have been assisting the HDFC bank in cross-selling relevant offers to its customers. Apart from the regular fraud prevention, it assists in keeping track of customer credit histories and has also been the reason for the speedy loan approvals offered by the bank.
9. Data Science in Urban Planning and Smart Cities
Data Science can help the dream of smart cities come true! Everything, from traffic flow to energy usage, can get optimized using data science techniques. You can use the data fetched from multiple sources to understand trends and plan urban living in a sorted manner.
The significant data science case study is traffic management in Pune city. The city controls and modifies its traffic signals dynamically, tracking the traffic flow. Real-time data gets fetched from the signals through cameras or sensors installed. Based on this information, they do the traffic management. With this proactive approach, the traffic and congestion situation in the city gets managed, and the traffic flow becomes sorted. A similar case study is from Bhubaneswar, where the municipality has platforms for the people to give suggestions and actively participate in decision-making. The government goes through all the inputs provided before making any decisions, making rules or arranging things that their residents actually need.
10. Data Science in Agricultural Yield Prediction
Have you ever wondered how helpful it can be if you can predict your agricultural yield? That is exactly what data science is helping farmers with. They can get information about the number of crops they can produce in a given area based on different environmental factors and soil types. Using this information, the farmers can make informed decisions about their yield and benefit the buyers and themselves in multiple ways.

Farmers across the globe and overseas use various data science techniques to understand multiple aspects of their farms and crops. A famous example of data science in the agricultural industry is the work done by Farmers Edge. It is a company in Canada that takes real-time images of farms across the globe and combines them with related data. The farmers use this data to make decisions relevant to their yield and improve their produce. Similarly, farmers in countries like Ireland use satellite-based information to ditch traditional methods and multiply their yield strategically.
11. Data Science in the Transportation Industry
Transportation keeps the world moving around. People and goods commute from one place to another for various purposes, and it is fair to say that the world will come to a standstill without efficient transportation. That is why it is crucial to keep the transportation industry in the most smoothly working pattern, and data science helps a lot in this. In the realm of technological progress, various devices such as traffic sensors, monitoring display systems, mobility management devices, and numerous others have emerged.
Many cities have already adapted to the multi-modal transportation system. They use GPS trackers, geo-locations and CCTV cameras to monitor and manage their transportation system. Uber is the perfect case study to understand the use of data science in the transportation industry. They optimize their ride-sharing feature and track the delivery routes through data analysis. Their data science approach enabled them to serve more than 100 million users, making transportation easy and convenient. Moreover, they also use the data they fetch from users daily to offer cost-effective and quickly available rides.
12. Data Science in the Environmental Industry
Increasing pollution, global warming, climate changes and other poor environmental impacts have forced the world to pay attention to environmental industry. Multiple initiatives are being taken across the globe to preserve the environment and make the world a better place. Though the industry recognition and the efforts are in the initial stages, the impact is significant, and the growth is fast.
The popular use of data science in the environmental industry is by NASA and other research organizations worldwide. NASA gets data related to the current climate conditions, and this data gets used to create remedial policies that can make a difference. Another way in which data science is actually helping researchers is they can predict natural disasters well before time and save or at least reduce the potential damage considerably. A similar case study is with the World Wildlife Fund. They use data science to track data related to deforestation and help reduce the illegal cutting of trees. Hence, it helps preserve the environment.
Where to Find Full Data Science Case Studies?
Data science is a highly evolving domain with many practical applications and a huge open community. Hence, the best way to keep updated with the latest trends in this domain is by reading case studies and technical articles. Usually, companies share their success stories of how data science helped them achieve their goals to showcase their potential and benefit the greater good. Such case studies are available online on the respective company websites and dedicated technology forums like Towards Data Science or Medium.
Additionally, we can get some practical examples in recently published research papers and textbooks in data science.
What Are the Skills Required for Data Scientists?
Data scientists play an important role in the data science process as they are the ones who work on the data end to end. To be able to work on a data science case study, there are several skills required for data scientists like a good grasp of the fundamentals of data science, deep knowledge of statistics, excellent programming skills in Python or R, exposure to data manipulation and data analysis, ability to generate creative and compelling data visualizations, good knowledge of big data, machine learning and deep learning concepts for model building & deployment. Apart from these technical skills, data scientists also need to be good storytellers and should have an analytical mind with strong communication skills.
Opt for the best business analyst training elevating your expertise. Take the leap towards becoming a distinguished business analysis professional
Conclusion
These were some interesting data science case studies across different industries. There are many more domains where data science has exciting applications, like in the Education domain, where data can be utilized to monitor student and instructor performance, develop an innovative curriculum that is in sync with the industry expectations, etc.
Almost all the companies looking to leverage the power of big data begin with a swot analysis to narrow down the problems they intend to solve with data science. Further, they need to assess their competitors to develop relevant data science tools and strategies to address the challenging issue. This approach allows them to differentiate themselves from their competitors and offer something unique to their customers.
With data science, the companies have become smarter and more data-driven to bring about tremendous growth. Moreover, data science has made these organizations more sustainable. Thus, the utility of data science in several sectors is clearly visible, a lot is left to be explored, and more is yet to come. Nonetheless, data science will continue to boost the performance of organizations in this age of big data.
Frequently Asked Questions (FAQs)
A case study in data science requires a systematic and organized approach for solving the problem. Generally, four main steps are needed to tackle every data science case study:
- Defining the problem statement and strategy to solve it
- Gather and pre-process the data by making relevant assumptions
- Select tool and appropriate algorithms to build machine learning /deep learning models
- Make predictions, accept the solutions based on evaluation metrics, and improve the model if necessary.
Getting data for a case study starts with a reasonable understanding of the problem. This gives us clarity about what we expect the dataset to include. Finding relevant data for a case study requires some effort. Although it is possible to collect relevant data using traditional techniques like surveys and questionnaires, we can also find good quality data sets online on different platforms like Kaggle, UCI Machine Learning repository, Azure open data sets, Government open datasets, Google Public Datasets, Data World and so on.
Data science projects involve multiple steps to process the data and bring valuable insights. A data science project includes different steps - defining the problem statement, gathering relevant data required to solve the problem, data pre-processing, data exploration & data analysis, algorithm selection, model building, model prediction, model optimization, and communicating the results through dashboards and reports.

Devashree Madhugiri
Devashree holds an M.Eng degree in Information Technology from Germany and a background in Data Science. She likes working with statistics and discovering hidden insights in varied datasets to create stunning dashboards. She enjoys sharing her knowledge in AI by writing technical articles on various technological platforms. She loves traveling, reading fiction, solving Sudoku puzzles, and participating in coding competitions in her leisure time.
Avail your free 1:1 mentorship session.
Something went wrong
Upcoming Data Science Batches & Dates

- Publications
- Conferences & Events
- Professional Learning
- Science Standards
- Awards & Competitions
- Instructional Materials
- Free Resources
- American Rescue Plan
- For Preservice Teachers
NCCSTS Case Collection
- Partner Jobs in Education
- Interactive eBooks+
- Digital Catalog
- Regional Product Representatives
- e-Newsletters
- Bestselling Books
- Latest Books
- Popular Book Series
- Prospective Authors
- Web Seminars
- Exhibits & Sponsorship
- Conference Reviewers
- National Conference • Denver 24
- Leaders Institute 2024
- National Conference • New Orleans 24
- Submit a Proposal
- Latest Resources
- Professional Learning Units & Courses
- For Districts
- Online Course Providers
- Schools & Districts
- College Professors & Students
- The Standards
- Teachers and Admin
- eCYBERMISSION
- Toshiba/NSTA ExploraVision
- Junior Science & Humanities Symposium
- Teaching Awards
- Climate Change
- Earth & Space Science
- New Science Teachers
- Early Childhood
- Middle School
- High School
- Postsecondary
- Informal Education
- Journal Articles
- Lesson Plans
- e-newsletters
- Science & Children
- Science Scope
- The Science Teacher
- Journal of College Sci. Teaching
- Connected Science Learning
- NSTA Reports
- Next-Gen Navigator
- Science Update
- Teacher Tip Tuesday
- Trans. Sci. Learning
MyNSTA Community
- My Collections
Case Study Listserv
Permissions & Guidelines
Submit a Case Study
Resources & Publications
Enrich your students’ educational experience with case-based teaching
The NCCSTS Case Collection, created and curated by the National Center for Case Study Teaching in Science, on behalf of the University at Buffalo, contains over a thousand peer-reviewed case studies on a variety of topics in all areas of science.
Cases (only) are freely accessible; subscription is required for access to teaching notes and answer keys.
Subscribe Today
Browse Case Studies
Latest Case Studies

Development of the NCCSTS Case Collection was originally funded by major grants to the University at Buffalo from the National Science Foundation , The Pew Charitable Trusts , and the U.S. Department of Education .
Data Science Capstone Team Collaborates with NASA
Three NASA scientists joined the team to study phytoplankton, a vital part of Earth's ecosystem.
Each Harvard Extension School data science master’s degree candidate completes a a capstone project by collaborating with an industry, government, or academic partner to investigate a real-world topic. Previous partnerships include Microsoft, Wildtrack, FEMA, MGH, IEEE, and Canadian Health.
When six students of the 2024 cohort came together for their capstone, they each shared a passion for leveraging their data science skills to affect positive change.
During the three-week, on-campus precapstone residency, the HES students contacted their partner of choice: NASA.
Three NASA scientists from the Ocean Ecology Laboratory agreed to collaborate on the capstone project: Carlos Del Castillo, chief of the Ocean Ecology Laboratory; Bridget Seegers, lead of the Cyanobacteria Assessment Network; and Cecile Rousseaux, research scientist. Later, research scientist Lionel Arteaga also joined the team.
Their project focused on analyzing data to better understand the natural phenomenon of phytoplankton, a crucial part of the Earth’s ecosystem. Castillo and Rousseaux had been working on the topic before the students’ introduction but needed the data science component to round out the research.
Bruce Huang , director of information technology programs, and Stephen Elston , data science instructor, approved the project proposal and the team got to work.
Meet the Data Science Capstone Team
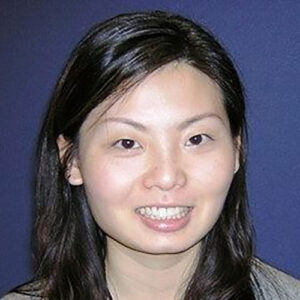
Doris Wong Location: Toronto, Canada Profession: Director of risk modeling
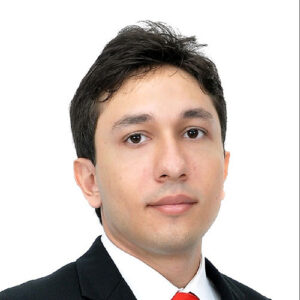
Ronan Fonseca Location: Vitoria, Brazil Profession: Economics
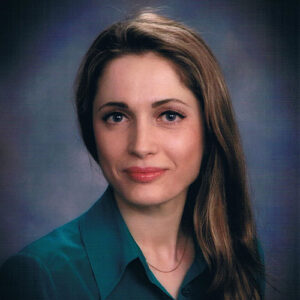
Marina Engelhardt Location: New York, U.S. Profession: Data analytics manager
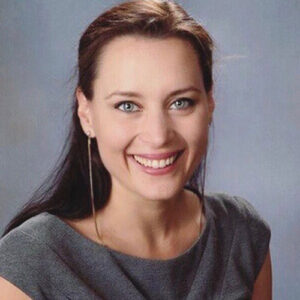
Vivien Kocsis Location: Miskolc, Hungary Profession: Entrepreneur
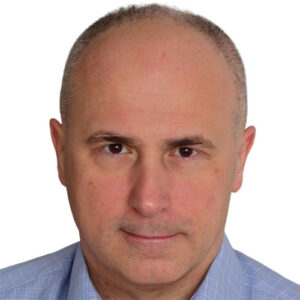
Walter Wojciech Latusek Location: Warsaw, Poland Profession: Data science and marketing analytics consultant
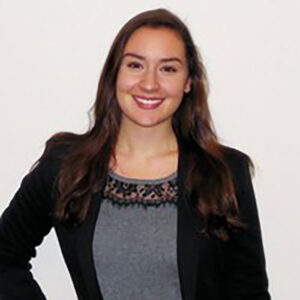
Emily Mocek Location: Toronto, Canada Profession: Data science, ad-tech
The Capstone Research Process
Project research involved evaluating satellite data to estimate phytoplankton levels in the Southern Ocean and in North American Lakes. Phytoplankton is a microscopic organism responsible for producing more than 50 percent of the planet’s oxygen, as well as for maintaining aquatic food webs.
The Southern Ocean is estimated to store a considerable proportion of excess heat and carbon from the atmosphere. Due to its extreme conditions, it is a particularly challenging environment to study and from which to collect data.
The capstone team used satellite data and Earth system models to gain complete coverage of the Southern Ocean.
Harvard professors and NASA scientists were there to help us with their technical expertise and domain-specific knowledge. Vivien Kocsis
Rousseux, one of the NASA scientists, explained, “By using both models and satellite data, this project was able to look at how much of the information is lost and how much the data analysis is biased because of gaps in the data.”
During the second part of the project, the team focused on identifying the drivers of phytoplankton in the area. Because pytoplankton decreases carbon dioxide stored in the atmosphere and feeds higher tropic levels, understanding the drivers can help control phytoplankton levels in the various ocean regions.
This research directly supports four of 17 of the United Nations Sustainable Development Goals : good health and wellbeing, clean water and sanitation, life below water, and climate action.
Collaboration with NASA
The student team worked closely with the NASA scientists, meeting almost every week. The students worked collaboratively, but independently from one another, as each was responsible for their own scope of work.
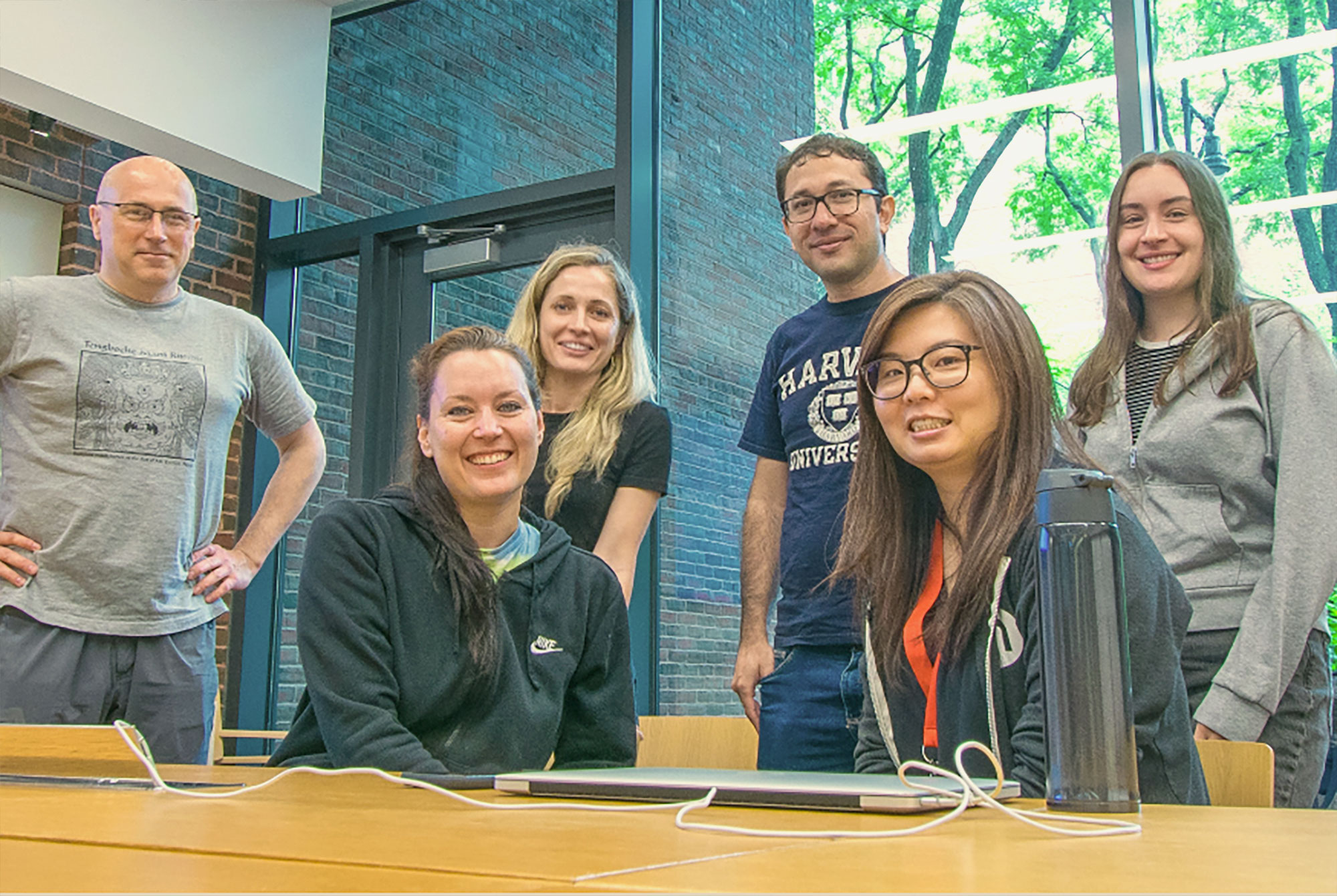
“It has truly been a positive experience to work with them. They were extremely organized, hard-working and appreciative and this made a world of a difference,” said Rousseaux. “I am always looking forward to their weekly updates because it’s polished and professional.”
On both sides of the collaboration, the project went smoothly, despite encountering a few time zone challenges across five different countries.
Kocsis, one of the capstone students, said navigating the rigorous demands of the master’s program while also juggling full-time employment and other responsibilities was daunting at times.
“The flexibility that Harvard afforded us throughout our master’s degree was probably the most valuable support we received,” she said. “This flexibility allowed us to tailor our study schedules to accommodate our professional and personal obligations.”
In the beginning, building the knowledge needed to conduct their research was also challenging.
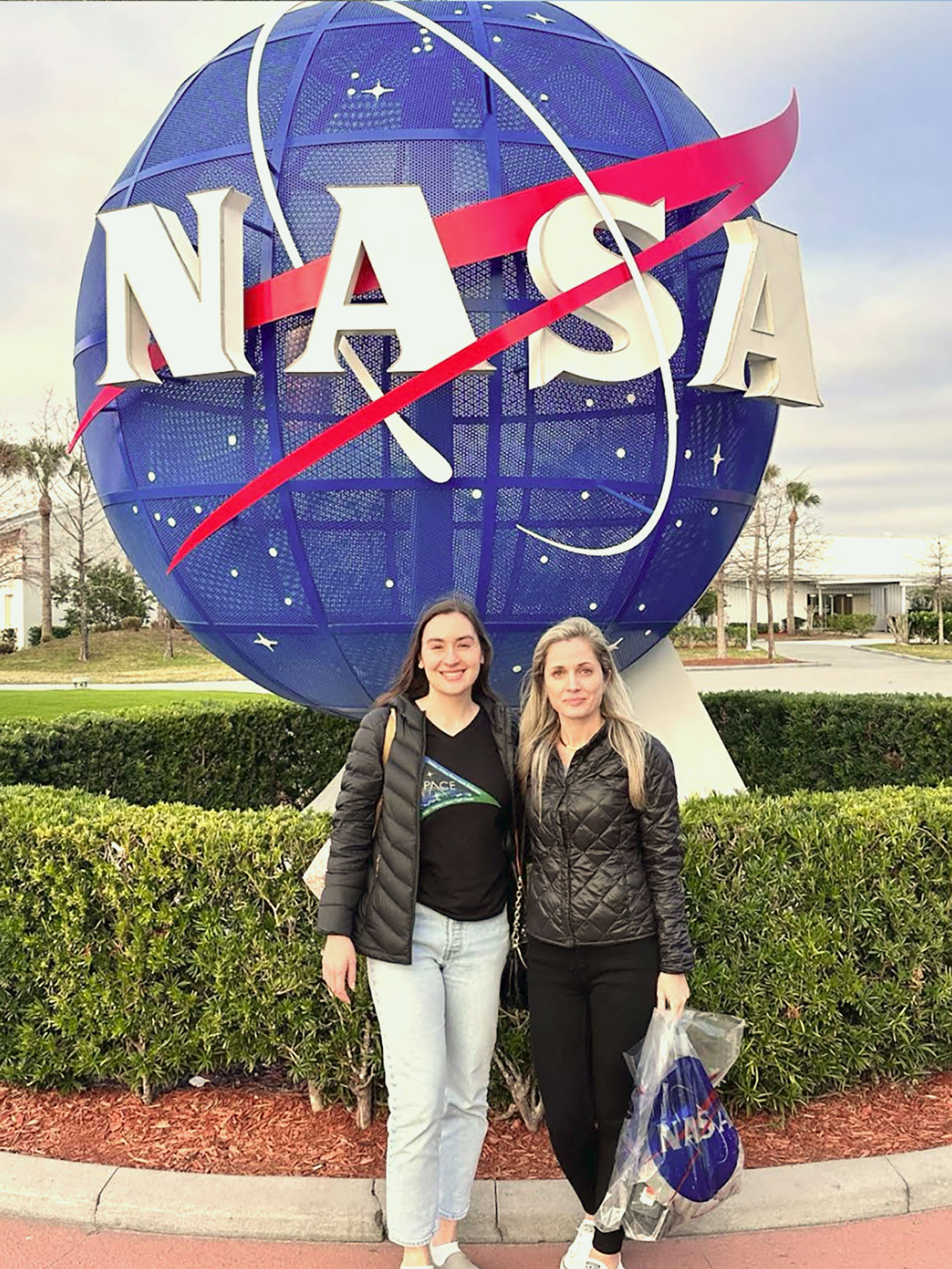
“At first, we encountered a steep learning curve as we built an understanding of natural processes, including the impact of ice, clouds, and seasons on phytoplankton levels,” said Kocsis. “We were also challenged by developing complex data science models. In both cases, Harvard professors and NASA scientists were there to help us with their technical expertise and domain-specific knowledge.”
It wasn’t just the capstone research that left an impression; the team had the opportunity to visit NASA facilities, including a behind-the-scenes tour at the Goddard Space Flight Center, as well as the PACE satellite launch at the NASA Kennedy Space Center in Florida.
On-Campus Experience
Data science precapstone & capstone.
Precapstone Course
Near the end of the master’s degree program, students come together for a 3-week course on campus (during the summer or January). Student teams work with an industry partner on the research design/protocol for their final capstone project.
Capstone Course
In the final online course of the program, students complete the team-based capstone project. It is taken as the sole remaining degree requirement in the semester immediately following the precapstone.
For the precapstone experience, data science students spent three weeks on Harvard’s campus. In addition to taking classes in Harvard Hall and studying in Widener Library, Huang treated the students to what he called “cheap noodles,” which Kocsis recalls as “a fabulous bonding experience.”
Along the way, the students received support and guidance from Huang and Elston, as well as from their fellow students. Additionally, access to campus resources during the on-campus experience facilitated collaboration.
“We felt a strong sense of community among students, which bolstered our collective academic success,” said Kocsis.
Data science students at Harvard Hall; Marina Engelhardt, Bruce Huang, and Emily Mocek in the Brattle Studio ; Huang students enjoy “cheap noodles.”
Opportunity & Impact
The capstone project brought many other opportunities:
- Presenting at an AI for Good Fireside Chat at the Division of Continuing Education’s Brattle Square Studio
- Giving two seminars at NASA
- Co-authoring two scientific papers with the chief of the Ocean Ecology Laboratory
- Facilitating a partnership for the current cohort of data science students to work with the National Oceanic and Atmospheric Administration
- Giving a talk at the upcoming United Nations AI For Good conference in Geneva, Switzerland
The impact of the data science capstone project and real-world applications is broad.
“Their projects answered several questions that we had for quite some time related to hotspots (regions of high phytoplankton concentration) in the Southern Ocean and their drivers,” said Rousseaux. “The level of quality of the work they did was to the point that we decided to publish these results and we are hoping to submit the paper some time this year to a peer-reviewed journal.”
Learn more about the data science master’s degree program
Web Application Development: 7 Essential Skills You Need to Get Started
Web application development skills are in high demand. Learn the seven skills you need to become a successful web developer.
Harvard Division of Continuing Education
The Division of Continuing Education (DCE) at Harvard University is dedicated to bringing rigorous academics and innovative teaching capabilities to those seeking to improve their lives through education. We make Harvard education accessible to lifelong learners from high school to retirement.
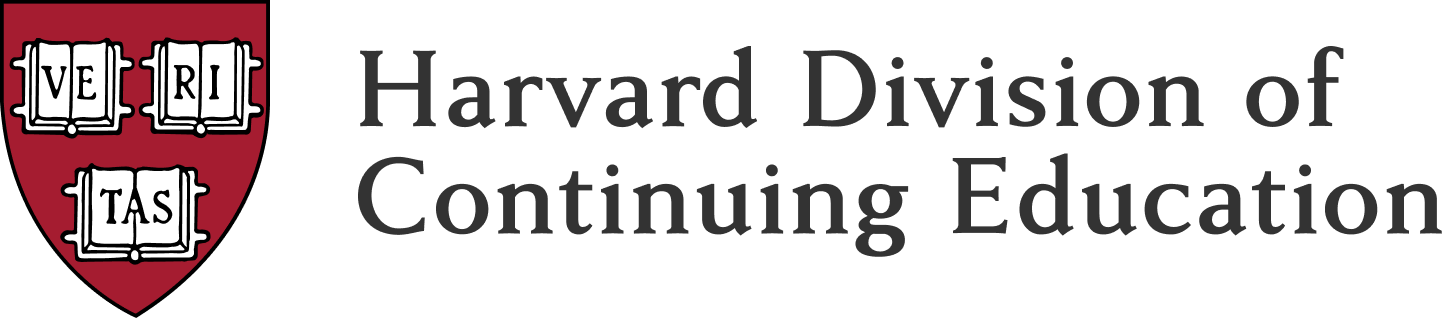
A Mixed Methods Pilot Study to Evaluate User Engagement with MedMicroMaps : A Novel Interactive E -learning Tool for Medical Microbiology
- Short Communication
- Open access
- Published: 01 May 2024
Cite this article
You have full access to this open access article
- Jane Harrington ORCID: orcid.org/0000-0002-4487-0257 1
Educators are witnessing the unfolding of the era of artificial intelligence, raising the question of how to transfer the benefits of yesterday’s pedagogy to the future of education. An interactive digital mind map of infectious diseases was developed for second-year medical students ( n = 865). Analysis of user engagement showed global distribution with 498 QR scans on a single day. Student responses ( n = 79, 9.1% response rate) indicated positive feedback on the resources of Extremely Satisfied (range 65–75%, n = 59–51). The findings of the study support further expansion of MedMicroMaps to cross-platform interfaces with adaptations for diverse audiences within allied health fields.
Avoid common mistakes on your manuscript.
The transformation in preclinical medical education from predominantly didactic in-person lectures to reliance on e -learning modalities became apparent in 2020 in context of the COVID-19 pandemic [ 1 ]. Once students returned to on-campus learning, medical educators faced the quandary of how to best leverage an effective balance of digital content with traditional oral content delivery to meet the needs of a younger generation of learners. Ranges of learning preferences for diverse populations of medical student necessitate development of e -learning modalities that allow flexibility of student-led inquiry which is adaptable to approaching evidence-based medicine with both basic sciences and clinical reasoning [ 2 ]. Visual learning in e -learning study guides with interactive elements confers a benefit over strictly text course content for non-traditional learners including non-native English learners and neurodiverse individuals [ 3 ]. Mind maps and the Method of Loci aka Memory Palace are pedagogical strategies that use spatial positioning, color-coding, and repeated symbolism to create pattern associations for more efficient memorization and are readily adaptable to e -learning modalities including web-based resources and immersive technologies [ 4 , 5 ]. A comprehensive interactive mind map for infectious diseases aka MedMicroMaps was developed in response to global virtual learning during the COVID-19 pandemic to guide medical students through differential diagnoses with options to approach case-based scenarios with clinical, epidemiological, and biological organization algorithms.
The initial impetus for developing the MedMicroMaps system was to create lecture time in the respiratory infections lecture series to accommodate coverage of the novel SARS-CoV-2 in Fall 2020 by organizing content methodically with consistent themes and paradigm patterns. The MedMicroMaps system uses an arching orientation of cardinal directions north/south/west/east, starting with clinical presentation organized by onset, reading left-to-right/west-to-east for acute (less than 1 week) to chronic (greater than 3 weeks) (Fig. 1 , MedMicroMaps compass). The coordinates of top-to-bottom/north-to-south are guides for anatomical location, corresponding to proximal to distal or superficial to deeper organs. The microbe biological classifications are arranged with smallest on top to the largest on the bottom, with viruses on top-left/north-west and helminths on bottom-right/south-east. Furthermore, the microbial colors correspond to staining methods when applicable with Gram-positive bacteria marked as Purple for crystal violet stain and Unicellular Fungi as Blue for lactophenol blue stain. The position of the microbes correlates to disease onset, representing that the microbes in the western quadrants (viruses, typical bacteria) mostly cause acute infections and the microbes in the eastern quadrants (atypical bacteria, fungi, parasites) mostly cause chronic infections.
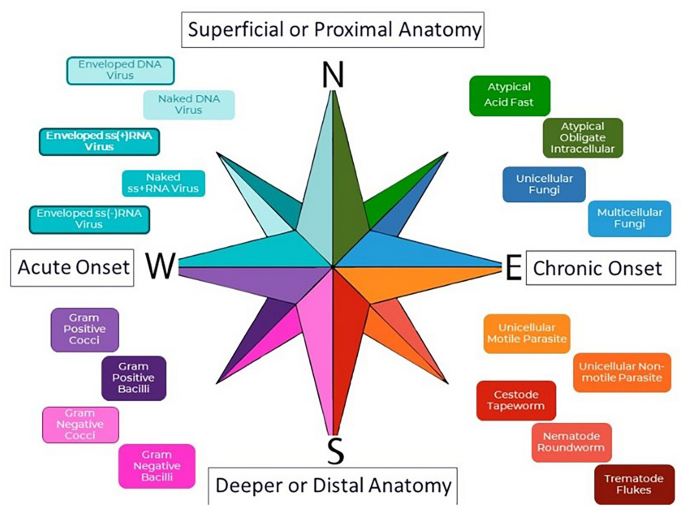
MedMicroMaps compass. An interactive guide of infectious diseases on PowerPoint platform was developed for preclinical medical students, using the principles of mind maps and Method of Loci to create a consistent color-coding and spatial patterns arranged on a compass with cardinal directions. North-to-south corresponds to anatomical location and west-to-east corresponds onset of clinical presentation. Color-coded microbes are arranged north-to-south based on smaller to larger, with subset patterns of classification (viruses: DNA on top and RNA on bottom, with border to indicate enveloped and no border for non-enveloped)
The MedMicroMaps sub-sections are organized by organ system and each module starts with a disease presentation for that respective organ system. The key clinical findings align with the process of decision tree logic of clinical reasoning for physicians-in-training, frequently encountered with board-style multiple-choice questions with a clinical vignette [ 6 ]. The specific category of disease, ex., acute, upper respiratory infection, has an arrow hyperlink that routes a user to an epidemiological map, listing causative agents of respiratory infections, ranked by severity or incidence, and further clustered by epidemiological factors, ex., elderly versus pediatric, exposure risk, occupation, or medical history (see Supplemental Material, Respiratory MedMicroMaps ). The next feature of MedMicroMaps module links the user to the clinical microbiology diagnostic algorithms arranged by biological classifications, based on common patterns for e -learning resources [ 7 ]. The Microbe Biology maps use consistent legends with color-coding microbes and pattern organization, ex., DNA on top and RNA on bottom for viruses, Cocci on top and Bacilli on bottom for typical bacteria, Unicellular on top and Multicellular on bottom for fungi and parasites. Lastly, each organ system map has a complete Overview slide to provide consistent outlines for all diseases and a broad guide of the range of infectious agents for a system. The hyperlinks allow the user to toggle back and forth to consider differential diagnoses to include or exclude microbes based on clues provided in a clinical scenario with follow-up laboratory findings.
Students in term 4 Spring 2022 ( n = 865) at St. George’s University, School of Medicine on the Caribbean Island of Grenada in hybrid delivery format were provided a link to the Microbiology Digital Media Resources website hosted on the Digication web server via QR code announcement during the first synchronous lecture of infectious disease system module. An office hour session delivery via Zoom was offered in the Respiratory module, which consisted of Case-Based Guide to the MedMicroMaps to integrate the decision tree logic through a case series. The office hour recording was posted to Sakai LMS. A link to Feedback Survey in Qualtrics with IRB-approval was provided to students enrolled in term 5 via course email announcement on the LMS Sakai server. Results of single-day engagement showed 498 QR code scans, tracked with QR Tiger, with global distribution of scans covering 4 continents and 16 countries (Fig. 2 , user engagement QR scans). User views on the website indicated 1000 + views per module per month across 2 cohorts over 10 months, with increased viewing the weekend spiking prior to the module exam. A limitation of the study was encountered with viewer statistics reported as 2 K, 3 K, etc. without daily count granularity. After the final infectious disease lecture in term 5, 79 students (9.1% response rate) completed the Qualtrics survey (Fig. 3 , student feedback). Most of the responses indicated Extremely Satisfied to “Rate your satisfaction with the Microbiology digital study resources” with the highest rating for Microbe Biology MedMicroMaps (75%, n = 59), followed by Respiratory MedMicroMaps (68%, n = 54), Overall Resources (66%, n = 52), and lastly Skin and Soft Tissue Infections (65%, n = 51). When prompted for specific utilization of the MedMicroMaps , students ranked Exam Preparation most frequently (63.2%, n = 50), followed by used with Practice Questions (54.4%, n = 43). User engagement of Case-Based Guide to MedMicroMaps indicated a higher usage of Zoom-recorded sessions the weekend after posting (44.3%, n = 35) as compared to attending live Zoom sessions (34.1%, n = 27) (data not shown).
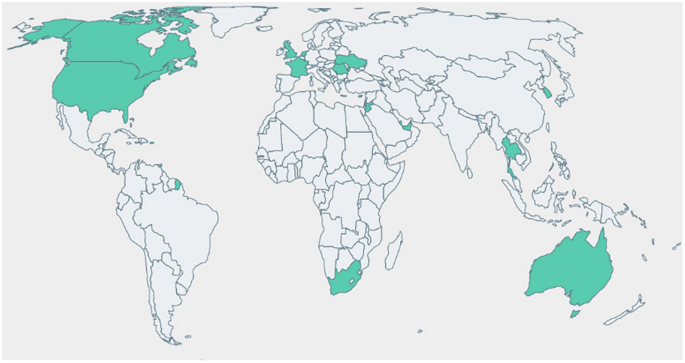
User engagement assessed with QR code scans for Microbiology Digital Media Resources website. Supplemental e -learning MedMicroMaps resources were provided on the Digication website server, with subpages for organ system modules. The resource was announced for second-year medical students ( n = 865 during hybrid synchronous lecture in March 2022 with a QR code provided in lecture PowerPoint. Website viewing on the day of announcement was tracked with QR Tiger scans and follow-up website views were determined with monthly summaries from Digication with estimates represented as 1 K = 1000, with 16 K views acquired over 14 months
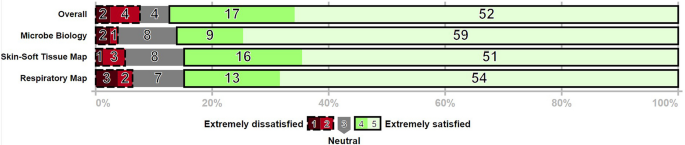
Student feedback survey on digital media resources. A link to the Qualtrics Student Feedback survey was provided via email announcement on Sakai LMS after the last infectious disease module. A 5-point Likert scale was used for student responses ( n = 79) to prompts: “Rate your level of satisfaction with the Microbiology digital study resources (Overall, Microbial Biology Classification MedMicroMaps, Skin/Soft Tissue Infections MedMicroMaps and Respiratory Infections MedMicroMaps)”
The findings of the pilot study support utilization of MedMicroMaps as a supplemental e -learning tool for medical students. The early development and delivery of the resource relied on posting individual system PowerPoint files in the Sakai LMS in subfolders based on lecture dates, starting with Microbe Biology in term 3 and Skin and Soft Tissue in term 4. The Digication website was created to host the comprehensive collection of system-based MedMicroMaps with extra digital media resources, including animations, office hour recordings, and fill-in-the-blank study guides. The data from the QR code tracking (Fig. 2 ) validates the scalability of e -learning resources for global audiences. Challenges encountered during the pilot study were lack of familiarity for the resource availability and lack of understanding how to integrate the system with practice questions or exam study. As the students progressed through the systems in terms 4 and 5, the author of the study reinforced the decision tree logic with the MedMicroMaps-embedded hyperlinks in the Respiratory Infections module lectures, which greatly increased awareness of the resource and engagement with the Digication website for future system modules in terms 4 and 5. The project is ongoing at the Montana College of Osteopathic Medicine, a new branch campus of Rocky Vista University, with curriculum design for all infectious disease content based on MedMicroMaps organization by organ system and microbial classification. To expand broader student access, the digital media resources have been moved to the Google domain website www.medmicromaps.com as the Digication server restricts to institutional login. A longitudinal study is warranted to determine the impact of MedMicroMaps on learning outcomes for short-term recall for module exams and for long-term recall for board exam preparation.
The MedMicroMaps system was created with hyperlinking features on 2-D PowerPoint format and was designed with expansion to cross-platform digital media interfaces, which is an expanding field in biomedical education [ 8 ]. The format of algorithm decision trees is compatible with a large language model in machine learning which can be utilized to develop novel immersive learning formats including virtual reality (VR), augmented reality (AR), metaverse (collectively referred as XR Extended Reality), e -book, and mobile/tablet app with course-tailored study guides and clinical cases. Digital representations of microbes can be accurately modeled by size and shape, utilizing computational power of artificial intelligence to generate a future microbial world in immersive learning platforms. MedMicroMaps in cross-platform format will be expanded for story-line experiences of all infectious diseases and can be adapted for utilization for diverse audiences within the field of biomedical education including allopathic and osteopathic medicine, nursing, pharmacy, and veterinary sciences and can be further modified to academic levels from undergraduate studies to practicing clinicians.
Data Availability
The author confirms that the data supporting the findings of this study are available within the article and its supplementary materials with original data sets from institutional servers (Qualtrics, Sakai, Panopto) accessible upon request.
Wilcha RJ. Effectiveness of virtual medical teaching during the COVID-19 crisis: systematic review. JMIR Med Educ. 2020;6(2):e20963. https://doi.org/10.2196/20963 . PMID: 33106227; PMCID: PMC7682786.
Article Google Scholar
Southwick F, Katona P, Kauffman C, Monroe S, Pirofski LA, del Rio C, Gallis H, Dismukes W, Infectious Diseases Society of America Preclinical Curriculum Committee. Commentary: IDSA guidelines for improving the teaching of preclinical medical microbiology and infectious diseases. Acad Med. 2010;85(1):19–22. https://doi.org/10.1097/ACM.0b013e3181c485c5 . PMID: 20042815.
Dosher B, Lu ZL. Visual perceptual learning and models. Annu Rev Vis Sci. 2017;3:343–63. https://doi.org/10.1146/annurev-vision-102016-061249 . Epub 2017 Jul 19. PMID: 28723311; PMCID: PMC669149.
Twomey C, Kroneisen M. The effectiveness of the loci method as a mnemonic device: meta-analysis. Q J Exp Psychol (Hove). 2021;74(8):1317–26. https://doi.org/10.1177/1747021821993457 . Epub 2021 Feb 18 PMID: 33535926.
Moll B, Sykes E. Optimized virtual reality-based Method of Loci memorization techniques through increased immersion and effective memory palace designs: a feasibility study. Virtual Real. 2023;27(2):941–66. https://doi.org/10.1007/s10055-022-00700-z . Epub 2022 Oct 6 PMID: 36248722.
Podgorelec V, Kokol P, Stiglic B, Rozman I. Decision trees: an overview and their use in medicine. J Med Syst. 2002;26(5):445–63. https://doi.org/10.1023/a:1016409317640 . PMID: 12182209.
Sayıner AA, Ergönül E. E-learning in clinical microbiology and infectious diseases. Clin Microbiol Infect. 2021;27(11):1589–94. https://doi.org/10.1016/j.cmi.2021.05.010 . Epub 2021 May 28 PMID: 34058378.
Venkatesan M, Mohan H, Ryan JR, Schürch CM, Nolan GP, Frakes DH, Coskun AF. Virtual and augmented reality for biomedical applications. Cell Rep Med. 2021;2(7):100348. https://doi.org/10.1016/j.xcrm.2021.100348 . PMID: 34337564; PMCID: PMC8324499.
Download references
Acknowledgements
The author of the study acknowledges Lucia Garces-Torres for her assistance with original designs for the MedMicroMaps and contributions to the PowerPoint build.
Author information
Authors and affiliations.
Montana College of Osteopathic Medicine, Rocky Vista University, Billings, MT, USA
Jane Harrington
You can also search for this author in PubMed Google Scholar
Corresponding author
Correspondence to Jane Harrington .
Ethics declarations
Competing interests.
The author of the article had no conflicting established partnerships of financial or non-financial support during the pilot study and held an academic position at St. George’s University. No funding agencies contributed to the pilot study. The author of the article currently holds an academic role at Rocky Vista University and has recently established a start-up company MedMicroMaps, LLC. to apply for grant funding with the National Science Foundation, Small Business Innovation Research program. MedMicroMaps, LLC. has no established financial relationships with external funding partners.
Additional information
Publisher's note.
Springer Nature remains neutral with regard to jurisdictional claims in published maps and institutional affiliations.
Supplementary Information
Below is the link to the electronic supplementary material.
Supplementary file1 (PDF 397 KB)
Rights and permissions.
Open Access This article is licensed under a Creative Commons Attribution 4.0 International License, which permits use, sharing, adaptation, distribution and reproduction in any medium or format, as long as you give appropriate credit to the original author(s) and the source, provide a link to the Creative Commons licence, and indicate if changes were made. The images or other third party material in this article are included in the article's Creative Commons licence, unless indicated otherwise in a credit line to the material. If material is not included in the article's Creative Commons licence and your intended use is not permitted by statutory regulation or exceeds the permitted use, you will need to obtain permission directly from the copyright holder. To view a copy of this licence, visit http://creativecommons.org/licenses/by/4.0/ .
Reprints and permissions
About this article
Harrington, J. A Mixed Methods Pilot Study to Evaluate User Engagement with MedMicroMaps : A Novel Interactive E -learning Tool for Medical Microbiology. Med.Sci.Educ. (2024). https://doi.org/10.1007/s40670-024-02047-3
Download citation
Accepted : 12 April 2024
Published : 01 May 2024
DOI : https://doi.org/10.1007/s40670-024-02047-3
Share this article
Anyone you share the following link with will be able to read this content:
Sorry, a shareable link is not currently available for this article.
Provided by the Springer Nature SharedIt content-sharing initiative
- Infectious diseases
- Microbiology
- Method of loci
- Spatial memory
- E -learning
- Virtual reality
- Augmented reality
- Extended reality
- Artificial intelligence
- Machine learning
- Find a journal
- Publish with us
- Track your research
First-of-its-kind study definitively shows that conservation actions are effective at halting and reversing biodiversity loss
Download images
A new study published online today, April 25, in the scientific journal Science provides the strongest evidence to date that not only is nature conservation successful, but that scaling conservation interventions up would be transformational for halting and reversing biodiversity loss—a crisis that can lead to ecosystem collapses and a planet less able to support life—and reducing the effects of climate change.
The findings of this first-ever comprehensive meta-analysis of the impact of conservation action are crucial as more than 44,000 species are documented as being at risk of extinction , with tremendous consequences for the ecosystems that stabilize the climate and that provide billions of people around the world with clean water, livelihoods, homes, and cultural preservation, among other ecosystem services. Governments recently adopted new global targets to halt and reverse biodiversity loss, making it even more critical to understand whether conservation interventions are working.
“If you look only at the trend of species declines, it would be easy to think that we’re failing to protect biodiversity, but you would not be looking at the full picture,” said Penny Langhammer, lead author of the study and executive vice president of Re:wild. “What we show with this paper is that conservation is, in fact, working to halt and reverse biodiversity loss. It is clear that conservation must be prioritized and receive significant additional resources and political support globally, while we simultaneously address the systemic drivers of biodiversity loss, such as unsustainable consumption and production.”
Although many studies look at individual conservation projects and interventions and their impact compared with no action taken, these papers have never been pulled into a single analysis to see how and whether conservation action is working overall. The co-authors conducted the first-ever meta-analysis of 186 studies, including 665 trials, that looked at the impact of a wide range of conservation interventions globally, and over time, compared to what would have happened without those interventions. The studies covered over a century of conservation action and evaluated actions targeting different levels of biodiversity—species, ecosystems, and genetic diversity.
The meta-analysis found that conservation actions—including the establishment and management of protected areas, the eradication and control of invasive species, the sustainable management of ecosystems, habitat loss reduction, and restoration—improved the state of biodiversity or slowed its decline in the majority of cases (66%) compared with no action taken at all. And when conservation interventions work, the paper’s co-authors found that they are highly effective .
For example:
- Management of invasive and problematic native predators on two of Florida’s barrier islands, Cayo Costa and North Captiva, resulted in an immediate and substantial improvement in nesting success by loggerhead turtles and least terns, especially compared with other barrier islands where no predator management was applied.
- In the Congo Basin, deforestation was 74% lower in logging concessions under a Forest Management Plan (FMP) compared with concessions without an FMP.
- Protected areas and Indigenous lands were shown to significantly reduce both deforestation rate and fire density in the Brazilian Amazon. Deforestation was 1.7 to 20 times higher and human-caused fires occurred four to nine times more frequently outside the reserve perimeters compared with inside.
- Captive breeding and release boosted the natural population of Chinook salmon in the Salmon River basin of central Idaho with minimal negative impacts on the wild population. On average, fish taken into the hatchery produced 4.7 times more adult offspring and 1.3 times more adult second generation offspring than naturally reproducing fish.
“Our study shows that when conservation actions work, they really work. In other words, they often lead to outcomes for biodiversity that are not just a little bit better than doing nothing at all, but many times greater,” said Jake Bicknell, co-author of the paper and a conservation scientist at DICE, University of Kent. “For instance, putting measures in place to boost the population size of an endangered species has often seen their numbers increase substantially. This effect has been mirrored across a large proportion of the case studies we looked at.”
Even in the minority of cases where conservation actions did not succeed in recovering or slowing the decline of the species or ecosystems that they were targeting compared with taking no action, conservationists benefited from the knowledge gained and were able to refine their methods. For example, in India the physical removal of invasive algae caused the spread of the algae elsewhere because the process broke the algae into many pieces, enabling their dispersal. Conservationists could now implement a different strategy to remove the algae that is more likely to be successful.
This might also explain why the co-authors found a correlation between more recent conservation interventions and positive outcomes for biodiversity— conservation is likely getting more effective over time . Other potential reasons for this correlation include an increase in funding and more targeted interventions.
In some other cases where the conservation action did not succeed in benefiting the target biodiversity compared with no action at all, other native species benefitted unintentionally instead. For example, seahorse abundance was lower in protected sites because marine protected areas increase the abundance of seahorse predators, including octopus.
“It would be too easy to lose any sense of optimism in the face of ongoing biodiversity declines,” said study co-author and Associate Professor Joseph Bull , from the University of Oxford’s Department of Biology. “However, our results clearly show that there is room for hope. Conservation interventions seemed to be an improvement on inaction most of the time; and when they were not, the losses were comparatively limited."
More than half of the world’s GDP, almost $44 trillion , is moderately or highly dependent on nature. According to previous studies, a comprehensive global conservation program would require an investment of between US$178 billion and US$524 billion , focused primarily in countries with particularly high levels of biodiversity. To put this in perspective, in 2022, global fossil fuel handouts--which are destructive to nature—were US$7 trillion . This is 13 times the highest amount needed annually to protect and restore the planet. Today more than US$121 billion is invested annually into conservation worldwide , and previous studies have found the cost-benefit ratio of an effective global program for the conservation of the wild is at least 1:100 .
“Conservation action works—this is what the science clearly shows us,” said Claude Gascon, co-author and director of strategy and operations at the Global Environment Facility. “It is also evident that to ensure that positive effects last, we need to invest more in nature and continue doing so in a sustained way. This study comes at a critical time where the world has agreed on ambitious and needed global biodiversity targets that will require conservation action at an entirely new scale. Achieving this is not only possible, it is well within our grasp as long as it is appropriately prioritized.”
The paper also argues that there must be more investment specifically in the effective management of protected areas, which remain the cornerstone for many conservation actions. Consistent with other studies, this study finds that protected areas work very well on the whole . And what other studies have shown is that when protected areas are not working, it is typically the result of a lack of effective management and adequate resourcing. Protected areas will be even more effective at reducing biodiversity loss if they are well-resourced and well-managed.
Moving forward, the study’s co-authors call for more and rigorous studies that look at the impact of conservation action versus inaction for a wider range of conservation interventions, such as those that look at the effectiveness of pollution control, climate change adaptation, and the sustainable use of species, and in more countries.
“For more than 75 years, IUCN has advanced the importance of sharing conservation practice globally,” said Grethel Aguilar, IUCN director general. “This paper has analyzed conservation outcomes at a level as rigorous as in applied disciplines like medicine and engineering—showing genuine impact and thus guiding the transformative change needed to safeguard nature at scale around the world. It shows that nature conservation truly works, from the species to the ecosystem levels across all continents. This analysis, led by Re:wild in collaboration with many IUCN Members, Commission experts, and staff, stands to usher in a new era in conservation practice.”
This work was conceived and funded through the International Union for Conservation of Nature (IUCN) by the Global Environment Facility.
Lindsay Renick Mayer
[email protected]
+1 512-686-6225
Devin Murphy
+1 512-686-6188
The paper ‘The positive impact of conservation action’ has been published in Science: https://www.science.org/doi/full/10.1126/science.adj6598
Additional quotes
Thomas Brooks, co-author and chief scientist, IUCN
“This paper is not only extremely important in providing robust evidence of the impact of
conservation actions. It is also extremely timely in informing crucial international policy processes, including the establishment of a 20-year vision for IUCN, the development of an IPBES assessment of biodiversity monitoring, and the delivery of the action targets toward the outcome goals of the new Kunming-Montreal Global Biodiversity Framework.”
Stuart Butchart, co-author and chief scientist, BirdLife International
“Recognising that the loss and degradation of nature is having consequences for societies worldwide, governments recently adopted a suite of goals and targets for biodiversity conservation. This new analysis is the best evidence to date that conservation interventions make a difference, slowing the loss of species’ populations and habitats and enabling them to recover. It provides strong support for scaling up investments in nature in order to meet the commitments that countries have signed up to.”
Jamie Carr, co-author and researcher in climate change and biodiversity governance, Leverhulme Centre for Anthropocene Biodiversity, University of York, UK “This work represents a huge effort on the part of many conservation professionals, all of whom are committed to reversing the loss of the world's biodiversity. It is encouraging to find that the past work of other conservationists has had a positive impact on nature, and I sincerely hope that our findings inspire those working now and in the future to ramp up their efforts."
Piero Genovesi, ISPRA, co-author and chair, IUCN SSC Invasive Species Specialist Group
“Species and ecosystems are facing a dramatic crisis, and the Biodiversity Plan of the United Nations is an urgent global call to action. This paper shows that eradication, control and management of invasive alien species have the largest impact in terms of conservation, and can help reverse the current trends of biodiversity loss, potentially saving hundreds of species from extinction. It is essential that governments and donors support the struggle against invasive alien species if we want to meet the agreed biodiversity targets by 2030.”
Mike Hoffmann, co-author and head of wildlife recovery, Zoological Society of London
“The major advance of this study is its sheer weight of evidence. We can point to specific examples, such as how captive breeding and reintroductions have facilitated the return of scimitar-horned oryx to the wild in Chad, but these can feel a bit exceptional. This study draws on more than 650 published cases to show that conservation wins are not rare. Conservation mostly works—unfortunately, it is also mostly significantly under-resourced.”
Madhu Rao, chair, IUCN World Commission on Protected Areas “With less than six years remaining to achieve ambitious biodiversity targets by 2030, there is a great sense of urgency for effective conservation action. We can take proven methods to conserve nature, such as protected areas, and scale them up for real conservation impact. This research clearly demonstrates that conservation actions are successful. We just need to take them to scale.”
Jon Paul Rodriguez, chair of the IUCN Species Survival Commission
“Anyone involved in the field of conservation will have witnessed the power of nature to regenerate and grow, given a chance to do so. From fishery exclusion zones, to ecological restoration on land, and animal, fungi and plant recovery efforts, there are numerous examples of halting and reversing biodiversity declines. Langhammer and colleagues synthesize knowledge on the impact of conservation action, and demonstrate that evidence-based conservation efforts indeed work in the majority of cases, not just in a few hand-picked examples. Much more money is spent on destroying nature than on protection and recovery. The authors show that tipping the balance in favor of nature is likely to help us deliver the world's ambitious biodiversity conservation targets.”
Gernot Segelbacher, co-author, professor and co-chair of Conservation Genetic Specialist Group, University Freiburg
“Conservation matters! While we so often hear about species declining or going extinct, this study shows that we can make a difference.”
Stephen Woodley, co-author, ecologist and vice chair for science and biodiversity, IUCN World Commission on Protected Areas
“The world needs hope that conservation action can work to halt and reverse biodiversity loss. This paper demonstrates that a range of conservation actions are highly effective. We just need to do more of them.”
Re:wild protects and restores the wild. We have a singular and powerful focus: the wild as the most effective solution to the interconnected climate, biodiversity and human wellbeing crises. Founded by a group of renowned conservation scientists together with Leonardo DiCaprio, Re:wild is a force multiplier that brings together Indigenous peoples, local communities, influential leaders, nongovernmental organizations, governments, companies and the public to protect and rewild at the scale and speed we need. Learn more at rewild.org .
University of Oxford
Oxford University has been placed number 1 in the Times Higher Education World University Rankings for the eighth year running, and number 3 in the QS World Rankings 2024. At the heart of this success are the twin-pillars of our ground-breaking research and innovation and our distinctive educational offer. Oxford is world-famous for research and teaching excellence and home to some of the most talented people from across the globe. Our work helps the lives of millions, solving real-world problems through a huge network of partnerships and collaborations. The breadth and interdisciplinary nature of our research alongside our personalised approach to teaching sparks imaginative and inventive insights and solutions.
Durrell Institute of Conservation and Ecology (DICE)
The Durrell Institute of Conservation and Ecology (DICE) is a research centre at the University of Kent. Its teaching and research is designed to break down the barriers between the natural and social sciences and produce real-world impact. Its mission is to conserve biodiversity and the ecological processes that support ecosystems and people, by developing capacity and improving conservation management and policy through high-impact research.
University of Kent
The University of Kent in England is renowned internationally for the quality of its teaching and research, with many of its academic schools and centres being among the best in their disciplines across the arts and humanities, sciences, and social sciences. Its campuses at Canterbury and Medway welcome more than 17,000 students from over 150 countries. The University of Kent is individually and collectively in the pursuit of progress, with a student-focused approach which is supportive, challenging and rewarding, and interdisciplinary research driven by collaboration to create positive impact. We are proud to be a values-driven university and work hard to ensure that our students are at the heart of all we do. We are committed to offering one of the best education and student experiences in the UK, undertaking research and innovation of the highest standard, and being a civic university that serves and contributes to our communities.
International Union for Conservation of Nature (IUCN)
IUCN is a membership Union composed of both government and civil society organisations. It harnesses the experience, resources and reach of its more than 1,400 Member organisations and the input of more than 16,000 experts. IUCN is the global authority on the status of the natural world and the measures needed to safeguard it.
IUCN World Commission on Protected Areas (WCPA)
The World Commission on Protected Areas (WCPA) is the world's premier network of protected and conserved areas expertise. The Commission has over 2500 members spanning 140 countries who provide strategic advice to policymakers and work to strengthen capacity and investment for protected areas establishment and management.
Arizona State University
Arizona State University has developed a new model for the American Research University, creating an institution that is committed to access, excellence and impact. ASU measures itself by those it includes, not by those it excludes. As the prototype for a New American University, ASU pursues research that contributes to the public good, and ASU assumes major responsibility for the economic, social and cultural vitality of the communities that surround it. www.asu.edu
BirdLife International
BirdLife International is the world's largest nature conservation Partnership: a global family of 122 national NGOs covering all continents, landscapes and seascapes. BirdLife is driven by its belief that local people, working for nature in their own places but connected nationally and internationally through the global Partnership, are the key to sustaining all life on this planet. This unique local-to-global approach delivers high impact and long-term conservation for the benefit of nature and people.
Global Environment Facility (GEF)
The Global Environment Facility (GEF) is a multilateral family of funds dedicated to confronting biodiversity loss, climate change, and pollution, and supporting land and ocean health. Its financing enables developing countries to address complex challenges and work towards international environmental goals. The partnership includes 186 member governments as well as civil society, Indigenous Peoples, women, and youth, with a focus on integration and inclusivity. Over the past three decades, the GEF has provided nearly $25 billion in financing and mobilized another $138 billion for thousands of priority projects and programs. The family of funds includes the Global Environment Facility Trust Fund, Global Biodiversity Framework Fund (GBFF), Least Developed Countries Fund (LDCF), Special Climate Change Fund (SCCF), Nagoya Protocol Implementation Fund (NPIF), and Capacity-building Initiative for Transparency Trust Fund (CBIT).
Zoological Society of London (ZSL)
Founded in 1826, ZSL is an international conservation charity, driven by science, working to restore wildlife in the UK and around the world; by protecting critical species, restoring ecosystems, helping people and wildlife live together and inspiring support for nature. Through our leading conservation zoos, London and Whipsnade, we bring people closer to nature and use our expertise to protect wildlife today, while inspiring a lifelong love of animals in the conservationists of tomorrow. Visit www.zsl.org for more information.

DISCOVER MORE
- Support Oxford's research
- Partner with Oxford on research
- Study at Oxford
- Research jobs at Oxford
You can view all news or browse by category
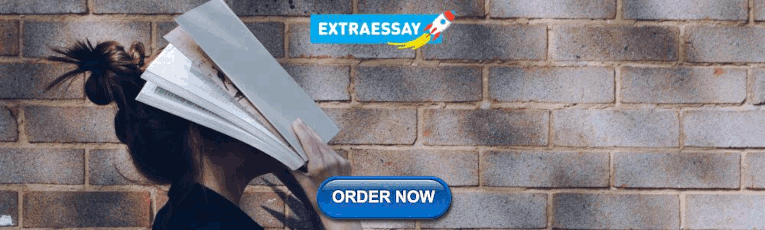
COMMENTS
Keywords: applied statistics, data science, statistical thinking, case studies, education, computing 1Introduction A major challenge in the practice of teaching data sci-ence and statistics is the limited availability of courses and course materials that provide meaningful opportu-nities for students to practice and apply statistical think-
The broad availability of educational data has led to an interest in analyzing useful knowledge to inform policy and practice with regard to education. A data science research methodology is becoming even more important in an educational context. More specifically, this field urgently requires more studies, especially related to outcome measurement and prediction and linking these to specific ...
Case Studies ( opencasestudies.org) project, which. offers a new statistical and data science education. case study model. This educational r esource pro-. vides self-contained, multimodal, peer ...
Several papers use the COVID-19 pandemic as a case study for discussing many of the above-listed issues. Papers in Cluster 5.2 (data science as a skill) discuss the importance of bringing data science education to everyone, since it is nowadays considered to be an important skill for any profession. ... Strengthening data science education ...
A Project-Based Case Study of Data Science Education. February 2016. Data Science Journal 15 (1) DOI: 10.5334/dsj-2016-005. Authors: D. Turek. A. Suen. D. Clark. To read the full-text of this ...
In this study, we present a method for teaching collaboration, a framework for identifying elements of effective collaboration, and provide a comparative case study (Yin 2017) of two collaboration meetings. The purpose of this case study is to model a way to evaluate collaboration on data science projects, provide emergent evidence of the ...
Academic Data Science Alliance (ADSA) Data Science Education special interest group (SIG). 2020 Jul 2. 2019. Addressing Public Health Challenges through Data Science Education. Hicks, Stephanie. American Public Health Association Annual Meeting. 2019 Nov 2-6. Philadelphia, PA, USA. Motivating Data Science through Case Studies in Public Health.
Data Science Curriculum Design: A Case Study. Pages 529-534. Previous Chapter Next Chapter. ... and C. Servin. ACM task force on data science education: Draft report and opportunity for feedback. In E. K. Hawthorne, M. A. Pérez-Quiñones, S. Heckman, and J. Zhang, editors, Proceedings of the 50th ACM Technical Symposium on Computer Science ...
To address this, we developed the Open Case Studies (opencasestudies.org) project, which offers a new statistical and data science education case study model. This educational resource provides self-contained, multimodal, peer-reviewed, and open-source guides (or case studies) from real-world examples for active experiences of complete data ...
What implications did this rise of data science as a transdisciplinary methodological toolkit have for the field of education?One means of illustrating the salience of data science in education research is to study its emergence in the Education Resources Information Center's (ERIC) publication corpus. 1 In the corpus, the growth of data science in education can be identified by the adoption ...
A Project-Based Case Study of Data Science Education. Daniel Turek, Anthony Suen, Dav Clark. Published in Data Science Journal 25 February 2016. Education, Computer Science. The discipline of data science has emerged over the past decade as a convergence of high-power computing, data visualization and analysis, and data-driven application domains.
A data science framework has emerged and is presented in the remainder of this article along with a case study to illustrate the steps. This data science framework warrants refining scientific practices around data ethics and data acumen (literacy). A short discussion of these topics concludes the article. 2.
Case studies are a central component of the data science education body of literature, often exploring a single institution's approach to teaching data science. One such example in The American Statistician described "SDS 292: Data Science" at Smith College, a course that covers data visualization, data wrangling, statistical modeling ...
The development of data science curricula has gained attention in academia and industry. Yet, less is known about the pedagogical practices and tools employed in data science education. Through a systematic literature review, we summarize prior pedagogical practices and tools used in data science initiatives at the higher education level. Following the Technological Pedagogical Content ...
Data Science for Education. Educational institutions and learning process entail rich data, and they concern weighty problems of great importance to society and the social good, so education is an especially well-suited domain for data science. Educational data spans K-12 school and district records, digital archives of instructional materials ...
In this paper, we present a programmatic approach to incorporating ethics into an undergraduate major in statistical and data sciences. In Section 2, we review and delineate notions of ethics in data science. We discuss departmental-level initiatives designed to meet the NAS recommendation for integrating ethics into the curriculum from top-to ...
Real-world data science case studies differ significantly from academic examples. While academic exercises often feature clean, well-structured data and simplified scenarios, real-world projects tackle messy, diverse data sources with practical constraints and genuine business objectives. These case studies reflect the complexities data ...
Data in Action: 7 Data Science Case Studies Worth Reading. The field of data science is rapidly growing and evolving. And in the next decade, new ways of automating data collection processes and deriving insights from data will boost workflow efficiencies like never before. There's no better way to understand the changing nature of data ...
An overview of success factors for using the Case Method in teaching Applied Data Science education is given, showing that close analysis provides a deeper understanding of implications, connects theory to practice, and classes unfold without a detailed script when successful instructors simultaneously manage content and process. Abstract In a world where everything involves data, an ...
BelData science has been a trending buzzword in recent times. With wide applications in various sectors like healthcare, education, retail, transportation, media, and banking -data science applications are at the core of pretty much every industry out there. The possibilities are endless: analysis of frauds in the finance sector or the personalization of recommendations on eCommerce businesses.
Examples of Data Science Case Studies. Hospitality: Airbnb focuses on growth by analyzing customer voice using data science. Qantas uses predictive analytics to mitigate losses. Healthcare: Novo Nordisk is Driving innovation with NLP. AstraZeneca harnesses data for innovation in medicine. Covid 19: Johnson and Johnson uses data science to fight ...
The NCCSTS Case Collection, created and curated by the National Center for Case Study Teaching in Science, on behalf of the University at Buffalo, contains over a thousand peer-reviewed case studies on a variety of topics in all areas of science. Cases (only) are freely accessible; subscription is required for access to teaching notes and ...
Each Harvard Extension School data science master's degree candidate completesa a capstone project by collaborating with an industry, government, or academic partner to investigate a real-world topic. Previous partnerships include Microsoft, Wildtrack, FEMA, MGH, IEEE, and Canadian Health. When six students of the 2024 cohort came together for their capstone, they each shared a passion for ...
Educators are witnessing the unfolding of the era of artificial intelligence, raising the question of how to transfer the benefits of yesterday's pedagogy to the future of education. An interactive digital mind map of infectious diseases was developed for second-year medical students (n = 865). Analysis of user engagement showed global distribution with 498 QR scans on a single day. Student ...
Download images. A new study published online today, April 25, in the scientific journal Science provides the strongest evidence to date that not only is nature conservation successful, but that scaling conservation interventions up would be transformational for halting and reversing biodiversity loss—a crisis that can lead to ecosystem collapses and a planet less able to support life—and ...
The study investigated the effect of a CR shift on the performance of professional NBA athletes. Data was analyzed from 25,016 regular games across 21 consecutive seasons between 2000 to 2021.