
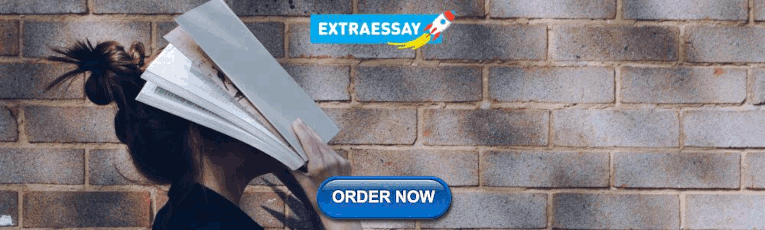
How To Write a Statistical Analysis Essay
Home » Videos » How To Write a Statistical Analysis Essay
Statistical analysis is a powerful tool used to draw meaningful insights from data. It can be applied to almost any field and has been used in everything from natural sciences, economics, and sociology to sports analytics and business decisions. Writing a statistical analysis essay requires an understanding of the concepts behind it as well as proficiency with data manipulation techniques.
In this guide, we’ll look at the steps involved in writing a statistical analysis essay. Experts in research paper writing from https://domypaper.me/write-my-research-paper/ give detailed instructions on how to properly conduct a statistical analysis and make valid conclusions.
Overview of statistical analysis essays
A statistical analysis essay is an academic paper that involves analyzing quantitative data and interpreting the results. It is often used in social sciences, economics and business to draw meaningful conclusions from the data. The objective of a statistical analysis essay is to analyze a specific dataset or multiple datasets in order to answer a question or prove or disprove a hypothesis. To achieve this effectively, the information must be analyzed using appropriate statistical techniques such as descriptive statistics, inferential statistics, regression analysis and correlation analysis.
Researching the subject matter
Before writing your statistical analysis essay it is important to research the subject matter thoroughly so that you have an understanding of what you are dealing with. This can include collecting and organizing any relevant data sets as well as researching different types of statistical techniques available for analyzing them. Furthermore, it is important to become familiar with the terminology associated with statistical analysis such as mean, median and mode.
Structuring your statistical analysis essay
The structure of your essay will depend on the type of data you are analyzing and the research question or hypothesis that you are attempting to answer. Generally speaking, it should include an introduction which introduces the research question or hypothesis; a body section which includes an overview of relevant literature; a description of how the data was collected and analyzed and any conclusions drawn from it; and finally a conclusion which summarizes all findings.
Analyzing data and drawing conclusions from it
After collecting and organizing your data, you must analyze it in order to draw meaningful conclusions from it. This involves using appropriate statistical techniques such as descriptive statistics, inferential statistics, regression analysis and correlation analysis. It is important to understand the assumptions made when using each technique in order to analyze the data correctly and draw accurate conclusions from it. When choosing a statistical technique for your research, it is important to consult with an expert https://typemyessay.me/service/research-paper-writing-service who can guide you on the most appropriate approach for your study.
Interpreting results and writing a conclusion
Once you have analyzed the data successfully, you must interpret the results carefully in order to answer your research question or prove/disprove your hypothesis. This involves making sure that any conclusions drawn are soundly based on the evidence presented. After interpreting the results, you should write a conclusion which summarizes all of your findings.
Using sources in your analysis
In order to back up your claims and provide support for your arguments, it is important to use credible sources within your analysis. This could include peer-reviewed articles, journals and books which can provide evidence to support your conclusion. It is also important to cite all sources used in order to avoid plagiarism.
Proofreading and finalizing your work
Once you have written your essay it is important to proofread it carefully before submitting it. This involves checking for grammar, spelling and punctuation errors as well as ensuring that the flow of the essay makes sense. Additionally, make sure that any references cited are correct and up-to-date. If you find it hard to complete your research statistical paper, you may want to consider buying a research paper for sale . This service can save you time and money, allowing you to focus on other important tasks.
Tips for writing a successful statistical analysis essay
Here are some tips for writing a successful statistical analysis essay:
- Research your subject matter thoroughly before writing your essay.
- Structure your paper according to the type of data you are analyzing.
- Analyze your data using appropriate statistical techniques.
- Interpret and draw meaningful conclusions from your results.
- Use credible sources to back up any claims or arguments made.
- Proofread and finalize your work before submitting it.
These tips will help ensure that your essay is well researched, structured correctly and contains accurate information. Following these tips will help you write a successful statistical analysis essay which can be used to answer research questions or prove/disprove hypotheses.
Sources and links For the articles and videos I use different databases, such as Eurostat, OECD World Bank Open Data, Data Gov and others. You are free to use the video I have made on your site using the link or the embed code. If you have any questions, don’t hesitate to write to me!
Support statistics and data, if you have reached the end and like this project, you can donate a coffee to “statistics and data”..
Copyright © 2022 Statistics and Data
Introductory essay
Written by the educators who created Visualizing Data, a brief look at the key facts, tough questions and big ideas in their field. Begin this TED Study with a fascinating read that gives context and clarity to the material.
The reality of today
All of us now are being blasted by information design. It's being poured into our eyes through the Web, and we're all visualizers now; we're all demanding a visual aspect to our information...And if you're navigating a dense information jungle, coming across a beautiful graphic or a lovely data visualization, it's a relief, it's like coming across a clearing in the jungle. David McCandless
In today's complex 'information jungle,' David McCandless observes that "Data is the new soil." McCandless, a data journalist and information designer, celebrates data as a ubiquitous resource providing a fertile and creative medium from which new ideas and understanding can grow. McCandless's inspiration, statistician Hans Rosling, builds on this idea in his own TEDTalk with his compelling image of flowers growing out of data/soil. These 'flowers' represent the many insights that can be gleaned from effective visualization of data.
We're just learning how to till this soil and make sense of the mountains of data constantly being generated. As Gary King, Director of Harvard's Institute for Quantitative Social Science says in his New York Times article "The Age of Big Data":
It's a revolution. We're really just getting under way. But the march of quantification, made possible by enormous new sources of data, will sweep through academia, business and government. There is no area that is going to be untouched.
How do we deal with all this data without getting information overload? How do we use data to gain real insight into the world? Finding ways to pull interesting information out of data can be very rewarding, both personally and professionally. The managing editor of Financial Times observed on CNN's Your Money : "The people who are able to in a sophisticated and practical way analyze that data are going to have terrific jobs." Those who learn how to present data in effective ways will be valuable in every field.
Many people, when they think of data, think of tables filled with numbers. But this long-held notion is eroding. Today, we're generating streams of data that are often too complex to be presented in a simple "table." In his TEDTalk, Blaise Aguera y Arcas explores images as data, while Deb Roy uses audio, video, and the text messages in social media as data.
Some may also think that only a few specialized professionals can draw insights from data. When we look at data in the right way, however, the results can be fun, insightful, even whimsical — and accessible to everyone! Who knew, for example, that there are more relationship break-ups on Monday than on any other day of the week, or that the most break-ups (at least those discussed on Facebook) occur in mid-December? David McCandless discovered this by analyzing thousands of Facebook status updates.
Data, data, everywhere
There is more data available to us now than we can possibly process. Every minute , Internet users add the following to the big data pool (i):
- 204,166,667 email messages sent
- More than 2,000,000 Google searches
- 684,478 pieces of content added on Facebook
- $272,070 spent by consumers via online shopping
- More than 100,000 tweets on Twitter
- 47,000 app downloads from Apple
- 34,722 "likes" on Facebook for different brands and organizations
- 27,778 new posts on Tumblr blogs
- 3,600 new photos on Instagram
- 3,125 new photos on Flickr
- 2,083 check-ins on Foursquare
- 571 new websites created
- 347 new blog posts published on Wordpress
- 217 new mobile web users
- 48 hours of new video on YouTube
These numbers are almost certainly higher now, as you read this. And this just describes a small piece of the data being generated and stored by humanity. We're all leaving data trails — not just on the Internet, but in everything we do. This includes reams of financial data (from credit cards, businesses, and Wall Street), demographic data on the world's populations, meteorological data on weather and the environment, retail sales data that records everything we buy, nutritional data on food and restaurants, sports data of all types, and so on.
Governments are using data to search for terrorist plots, retailers are using it to maximize marketing strategies, and health organizations are using it to track outbreaks of the flu. But did you ever think of collecting data on every minute of your child's life? That's precisely what Deb Roy did. He recorded 90,000 hours of video and 140,000 hours of audio during his son's first years. That's a lot of data! He and his colleagues are using the data to understand how children learn language, and they're now extending this work to analyze publicly available conversations on social media, allowing them to take "the real-time pulse of a nation."
Data can provide us with new and deeper insight into our world. It can help break stereotypes and build understanding. But the sheer quantity of data, even in just any one small area of interest, is overwhelming. How can we make sense of some of this data in an insightful way?
The power of visualizing data
Visualization can help transform these mountains of data into meaningful information. In his TEDTalk, David McCandless comments that the sense of sight has by far the fastest and biggest bandwidth of any of the five senses. Indeed, about 80% of the information we take in is by eye. Data that seems impenetrable can come alive if presented well in a picture, graph, or even a movie. Hans Rosling tells us that "Students get very excited — and policy-makers and the corporate sector — when they can see the data."
It makes sense that, if we can effectively display data visually, we can make it accessible and understandable to more people. Should we worry, however, that by condensing data into a graph, we are simplifying too much and losing some of the important features of the data? Let's look at a fascinating study conducted by researchers Emre Soyer and Robin Hogarth . The study was conducted on economists, who are certainly no strangers to statistical analysis. Three groups of economists were asked the same question concerning a dataset:
- One group was given the data and a standard statistical analysis of the data; 72% of these economists got the answer wrong.
- Another group was given the data, the statistical analysis, and a graph; still 61% of these economists got the answer wrong.
- A third group was given only the graph, and only 3% got the answer wrong.
Visualizing data can sometimes be less misleading than using the raw numbers and statistics!
What about all the rest of us, who may not be professional economists or statisticians? Nathalie Miebach finds that making art out of data allows people an alternative entry into science. She transforms mountains of weather data into tactile physical structures and musical scores, adding both touch and hearing to the sense of sight to build even greater understanding of data.
Another artist, Chris Jordan, is concerned about our ability to comprehend big numbers. As citizens of an ever-more connected global world, we have an increased need to get useable information from big data — big in terms of the volume of numbers as well as their size. Jordan's art is designed to help us process such numbers, especially numbers that relate to issues of addiction and waste. For example, Jordan notes that the United States has the largest percentage of its population in prison of any country on earth: 2.3 million people in prison in the United States in 2005 and the number continues to rise. Jordan uses art, in this case a super-sized image of 2.3 million prison jumpsuits, to help us see that number and to help us begin to process the societal implications of that single data value. Because our brains can't truly process such a large number, his artwork makes it real.
The role of technology in visualizing data
The TEDTalks in this collection depend to varying degrees on sophisticated technology to gather, store, process, and display data. Handling massive amounts of data (e.g., David McCandless tracking 10,000 changes in Facebook status, Blaise Aguera y Arcas synching thousands of online images of the Notre Dame Cathedral, or Deb Roy searching for individual words in 90,000 hours of video tape) requires cutting-edge computing tools that have been developed specifically to address the challenges of big data. The ability to manipulate color, size, location, motion, and sound to discover and display important features of data in a way that makes it readily accessible to ordinary humans is a challenging task that depends heavily on increasingly sophisticated technology.
The importance of good visualization
There are good ways and bad ways of presenting data. Many examples of outstanding presentations of data are shown in the TEDTalks. However, sometimes visualizations of data can be ineffective or downright misleading. For example, an inappropriate scale might make a relatively small difference look much more substantial than it should be, or an overly complicated display might obfuscate the main relationships in the data. Statistician Kaiser Fung's blog Junk Charts offers many examples of poor representations of data (and some good ones) with descriptions to help the reader understand what makes a graph effective or ineffective. For more examples of both good and bad representations of data, see data visualization architect Andy Kirk's blog at visualisingdata.com . Both consistently have very current examples from up-to-date sources and events.
Creativity, even artistic ability, helps us see data in new ways. Magic happens when interesting data meets effective design: when statistician meets designer (sometimes within the same person). We are fortunate to live in a time when interactive and animated graphs are becoming commonplace, and these tools can be incredibly powerful. Other times, simpler graphs might be more effective. The key is to present data in a way that is visually appealing while allowing the data to speak for itself.
Changing perceptions through data
While graphs and charts can lead to misunderstandings, there is ultimately "truth in numbers." As Steven Levitt and Stephen Dubner say in Freakonomics , "[T]eachers and criminals and real-estate agents may lie, and politicians, and even C.I.A. analysts. But numbers don't." Indeed, consideration of data can often be the easiest way to glean objective insights. Again from Freakonomics : "There is nothing like the sheer power of numbers to scrub away layers of confusion and contradiction."
Data can help us understand the world as it is, not as we believe it to be. As Hans Rosling demonstrates, it's often not ignorance but our preconceived ideas that get in the way of understanding the world as it is. Publicly-available statistics can reshape our world view: Rosling encourages us to "let the dataset change your mindset."
Chris Jordan's powerful images of waste and addiction make us face, rather than deny, the facts. It's easy to hear and then ignore that we use and discard 1 million plastic cups every 6 hours on airline flights alone. When we're confronted with his powerful image, we engage with that fact on an entirely different level (and may never see airline plastic cups in the same way again).
The ability to see data expands our perceptions of the world in ways that we're just beginning to understand. Computer simulations allow us to see how diseases spread, how forest fires might be contained, how terror networks communicate. We gain understanding of these things in ways that were unimaginable only a few decades ago. When Blaise Aguera y Arcas demonstrates Photosynth, we feel as if we're looking at the future. By linking together user-contributed digital images culled from all over the Internet, he creates navigable "immensely rich virtual models of every interesting part of the earth" created from the collective memory of all of us. Deb Roy does somewhat the same thing with language, pulling in publicly available social media feeds to analyze national and global conversation trends.
Roy sums it up with these powerful words: "What's emerging is an ability to see new social structures and dynamics that have previously not been seen. ...The implications here are profound, whether it's for science, for commerce, for government, or perhaps most of all, for us as individuals."
Let's begin with the TEDTalk from David McCandless, a self-described "data detective" who describes how to highlight hidden patterns in data through its artful representation.
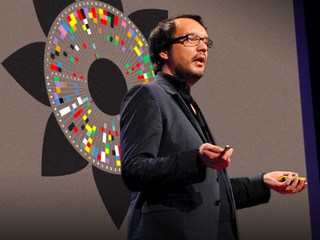
David McCandless
The beauty of data visualization.
i. Data obtained June 2012 from “How Much Data Is Created Every Minute?” on http://mashable.com/2012/06/22/data-created-every-minute/ .
Relevant talks
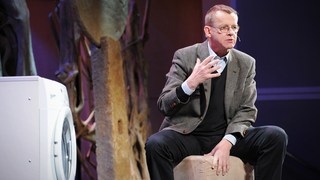
Hans Rosling
The magic washing machine.
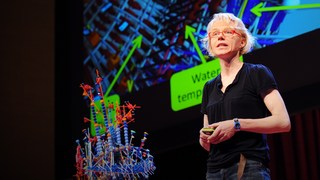
Nathalie Miebach
Art made of storms.
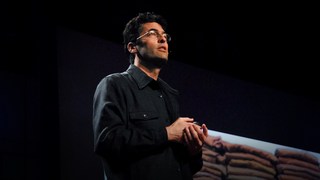
Chris Jordan
Turning powerful stats into art.
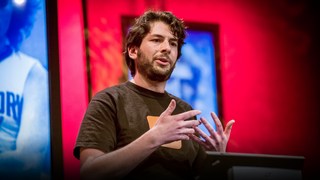
Blaise Agüera y Arcas
How photosynth can connect the world's images.
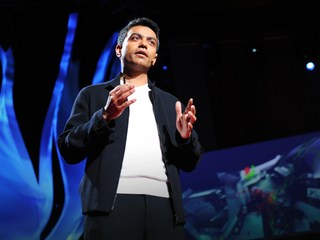
The birth of a word
- How it works
Step-by-Step Guide to Statistical Analysis
It would not be wrong to say that statistics are utilised in almost every aspect of society. You might have also heard the phrase, “you can prove anything with statistics.” Or “facts are stubborn things, but statistics are pliable, which implies the results drawn from statistics can never be trusted.
But what if certain conditions are applied, and you analyse these statistics before getting somewhere? Well, that sounds totally reliable and straight from the horse’s mouth. That is what statistical analysis is.
It is the branch of science responsible for rendering various analytical techniques and tools to deal with big data. In other words, it is the science of identifying, organising, assessing and interpreting data to make interferences about a particular populace.Every statistical dissection follows a specific pattern, which we call the Statistical Analysis Process.
It precisely concerns data collection , interpretation, and presentation. Statistical analyses can be carried out when handling a huge extent of data to solve complex issues. Above all, this process delivers importance to insignificant numbers and data that often fills in the missing gaps in research.
This guide will talk about the statistical data analysis types, the process in detail, and its significance in today’s statistically evolved era.
Types of Statistical Data Analysis
Though there are many types of statistical data analysis, these two are the most common ones:
Descriptive Statistics
Inferential statistics.
Let us discuss each in detail.
It quantitatively summarises the information in a significant way so that whoever is looking at it might detect relevant patterns instantly. Descriptive statistics are divided into measures of variability and measures of central tendency. Measures of variability consist of standard deviation, minimum and maximum variables, skewness, kurtosis, and variance , while measures of central tendency include the mean, median , and mode .
- Descriptive statistics sum up the characteristics of a data set
- It consists of two basic categories of measures: measures of variability and measures of central tendency
- Measures of variability describe the dispersion of data in the data set
- Measures of central tendency define the centre of a data set
With inferential statistics , you can be in a position to draw conclusions extending beyond the immediate data alone. We use this technique to infer from the sample data what the population might think or make judgments of the probability of whether an observed difference between groups is dependable or undependable. Undependable means it has happened by chance.
- Inferential Statistics is used to estimate the likelihood that the collected data occurred by chance or otherwise
- It helps conclude a larger population from which you took samples
- It depends upon the type of measurement scale along with the distribution of data
Other Types Include:
Predictive Analysis: making predictions of future events based on current facts and figures
Prescriptive Analysis: examining data to find out the required actions for a particular situation
Exploratory Data Analysis (EDA): previewing of data and assisting in getting key insights into it
Casual Analysis: determining the reasons behind why things appear in a certain way
Mechanistic Analysis: explaining how and why things happen rather than how they will take place subsequently
Statistical Data Analysis: The Process
The statistical data analysis involves five steps:
- Designing the Study
- Gathering Data
- Describing the Data
- Testing Hypotheses
- Interpreting the Data
Step 1: Designing the Study
The first and most crucial step in a scientific inquiry is stating a research question and looking for hypotheses to support it.
Examples of research questions are:
- Can digital marketing increase a company’s revenue exponentially?
- Can the newly developed COVID-19 vaccines prevent the spreading of the virus?
As students and researchers, you must also be aware of the background situation. Answer the following questions.
What information is there that has already been presented by other researchers?
How can you make your study stand apart from the rest?
What are effective ways to get your findings?
Once you have managed to get answers to all these questions, you are good to move ahead to another important part, which is finding the targeted population .
What population should be under consideration?
What is the data you will need from this population?
But before you start looking for ways to gather all this information, you need to make a hypothesis, or in this case, an educated guess. Hypotheses are statements such as the following:
- Digital marketing can increase the company’s revenue exponentially.
- The new COVID-19 vaccine can prevent the spreading of the virus.
Remember to find the relationship between variables within a population when writing a statistical hypothesis. Every prediction you make can be either null or an alternative hypothesis.
While the former suggests no effect or relationship between two or more variables, the latter states the research prediction of a relationship or effect.
How to Plan your Research Design?
After deducing hypotheses for your research, the next step is planning your research design. It is basically coming up with the overall strategy for data analysis.
There are three ways to design your research:
1. Descriptive Design:
In a descriptive design, you can assess the characteristics of a population by using statistical tests and then construe inferences from sample data.
2. Correlational Design:
As the name suggests, with this design, you can study the relationships between different variables .
3. Experimental Design:
Using statistical tests of regression and comparison, you can evaluate a cause-and-effect relationship.
Step 2: Collecting Data
Collecting data from a population is a challenging task. It not only can get expensive but also take years to come to a proper conclusion. This is why researchers are instead encouraged to collect data from a sample.
Sampling methods in a statistical study refer to how we choose members from the population under consideration or study. If you select a sample for your study randomly, the chances are that it would be biased and probably not the ideal data for representing the population.
This means there are reliable and non-reliable ways to select a sample.
Reliable Methods of Sampling
Simple Random Sampling: a method where each member and set of members have an equal chance of being selected for the sample
Stratified Random Sampling: population here is first split into groups then members are selected from each group
Clutter Random Sampling: the population is divided into groups, and members are randomly chosen from some groups.
Systematic Random Sampling: members are selected in order. The starting point is chosen by chance, and every nth member is set for the sample.
Non-Reliable Methods of Sampling
Voluntary Response Sampling: choosing a sample by sending out a request for members of a population to join. Some might join, and others might not respond
Convenient Sampling: selecting a sample readily available by chance
Here are a few important terms you need to know for conducting samples in statistics:
Population standard deviation: estimated population parameter on the basis of the previous study
Statistical Power: the chances of your study detecting an effect of a certain size
Expected Effect Size: it is an indication of how large the expected findings of your research be
Significance Level (alpha): it is the risk of rejecting a true null hypothesis
Step 3: Describing the Data
Once you are done finalising your samples, you are good to go with their inspection by calculating descriptive statistics , which we discussed above.
There are different ways to inspect your data.
- By using a scatter plot to visualise the relationship between two or more variables
- A bar chart displaying data from key variables to view how the responses have been distributed
- Via frequency distribution where data from each variable can be organised
When you visualise data in the form of charts, bars, and tables, it becomes much easier to assess whether your data follow a normal distribution or skewed distribution. You can also get insights into where the outliers are and how to get them fixed.
Is the Statistics assignment pressure too much to handle?
How about we handle it for you.
Put in the order right now in order to save yourself time, money, and nerves at the last minute.
How is a Skewed Distribution Different from a Normal One?
A normal distribution is where the set of information or data is distributed symmetrically around a centre. This is where most values lie, with the values getting smaller at the tail ends.
On the other hand, if one of the tails is longer or smaller than the other, the distribution would be skewed . They are often called asymmetrical distributions, as you cannot find any sort of symmetry in them.
The skewed distribution can be of two ways: left-skewed distribution and right-skewed distribution . When the left tail is longer than the right one, it is left-stewed distribution, while the right tail is longer in a right-strewed distribution.
Now, let us discuss the calculation of measures of central tendency. You might have heard about this one already.
What do Measures of Central Tendency Do?
Well, it precisely describes where most of the values lie in a data set. Having said that, the three most heard and used measures of central tendency are:
When considered from low to high, this is the value in the exact centre.
Mode is the most wanted or popular response in the data set.
You calculate the mean by simply adding all the values and dividing by the total number.Coming to how you can calculate the , which is equally important.
Measures of variability
Measures of variability give you an idea of how to spread out or dispersed values in a data set.
The four most common ones you must know about are:
Standard Deviation
The average distance between different values in your data set and the mean
Variance is the square of the standard deviation.
The range is the highest value subtracted from the data set's minimum value.
Interquartile Range
Interquartile range is the highest value minus lowest of the data set
Step 4: Testing your Hypotheses
Two terms you need to know in order to learn about testing a hypothesis:
Statistic-a number describing a sample
Parameter-a number describing a population
So, what exactly are hypotheses testing?
It is where an analyst or researcher tests all the assumptions made earlier regarding a population parameter. The methodology opted for by the researcher solely depends on the nature of the data utilised and the reason for its analysis.
The only objective is to evaluate the plausibility of hypotheses with the help of sample data. The data here can either come from a larger population or a sample to represent the whole population .
How it Works?
These four steps will help you understand what exactly happens in hypotheses testing.
- The first thing you need to do is state the two hypotheses made at the beginning.
- The second is formulating an analysis plan that depicts how the data can be assessed.
- Next is physically analysing the sample data about the plan.
- The last and final step is going through the results and assessing whether you need to reject the null hypothesis or move forward with it.
Questions might arise on knowing if the null hypothesis is plausible, and this is where statistical tests come into play.
Statistical tests let you determine where your sample data could lie on an expected distribution if the null hypotheses were plausible. Usually, you get two types of outputs from statistical tests:
- A test statistic : this shows how much your data differs from the null hypothesis
- A p-value: this value assesses the likelihood of getting your results if the null hypothesis is true
Step 5: Interpreting the Data
You have made it to the final step of statistical analysis , where all the data you found useful till now will be interpreted. In order to check the usability of data, researchers compare the p-value to a set significant level, which is 0.05, so that they can know if the results are statistically important or not. That is why this process in hypothesis testing is called statistical significance .
Remember that the results you get here are unlikely to have arisen because of probability. There are lower chances of such findings if the null hypothesis is plausible.
By the end of this process, you must have answers to the following questions:
- Does the interpreted data answer your original question? If yes, how?
- Can you defend against objections with this data?
- Are there limitations to your conclusions?
If the final results cannot help you find clear answers to these questions, you might have to go back, assess and repeat some of the steps again. After all, you want to draw the most accurate conclusions from your data.
You May Also Like
Learn about the steps required to successfully complete their research project. Make sure to follow these steps in their respective order.
Looking for an easy guide to follow to write your essay? Here is our detailed essay guide explaining how to write an essay and examples and types of an essay.
Applying to a university and looking for a guide to write your UCAS personal statement? We have covered all aspects of the UCAS statement to help you get to your dream university.
More Interesting Articles
USEFUL LINKS
LEARNING RESOURCES
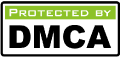
COMPANY DETAILS
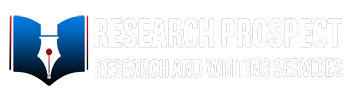
- How It Works

The Best PhD and Masters Consulting Company

Statistical Analysis: A Step-by-Step Guide
Introduction to statistical analysis , step 1: make a list of your hypotheses and make a plan for your study., statistical hypotheses writing, creating a research design.
- Statistical tests of comparison or regression are what you can use in an experimental design to analyze a cause-and-effect connection (e.g., the influence of meditation on test scores).
- With a correlational design, you can use correlation coefficients and significance tests to investigate correlations between variables (for example, parental income and GPA) without making any assumptions about causality.
- Using statistical tests to derive inferences from sample data, you can analyse the features of a population or phenomenon (e.g., the prevalence of anxiety in US college students) in a descriptive design.
- You evaluate the group-level results of individuals who undergo different treatments (e.g., those who undertook a meditation exercise vs. those who did not) in a between-subjects design.
- A within-subjects design compares repeated measures from participants who have completed all of the study’s treatments (e.g., scores from before and after performing a meditation exercise).
- One variable you can change between subjects while another you can change within subjects in a factorial design.
Variables are exact.
- Groupings you can present using categorical data. These can be nominal (for example, gender) or ordinal (for example, age) (e.g. level of language ability).
- Quantitative data is a representation of quantity. These can be on an interval scale (for example, a test score) or a ratio scale (for example, a weighted average) (e.g. age).
Step 2: Collect data from a representative sample
Sample vs. population.
- Probability sampling : every member of the population has a probability of being chosen at random for the study.
- Non-probability sampling : some people are more likely to be chosen for the study than others based on factors like convenience or voluntary self-selection.
- Your sample is representative of the population to whom your findings are being applied.
- Your sample is biased in a systematic way.
Make a suitable sampling procedure.
- Will you have the resources to publicize your research extensively, including outside of your university?
- Will you be able to get a varied sample that represents the entire population?
- Do you have time to reach out to members of hard-to-reach groups and follow up with them?
Calculate an appropriate sample size.
- The risk of rejecting a true null hypothesis that you are ready to incur is called the significance level (alpha). It is commonly set at 5%.
- Statistical power is the likelihood that your study will discover an impact of a specific size if one exists, which is usually around 80% or higher.
- Predicted impact size: a standardized estimate of the size of your study’s expected result, usually based on similar studies.
- The standard deviation of the population: an estimate of the population parameter based on past research or a pilot study of your own.
Step 3: Use descriptive statistics to summarize your data.
Examine your information..
- Using frequency distribution tables to organize data from each variable.
- To see the distribution of replies, use a bar chart to display data from a key variable.
- Using a scatter plot to visualize the relationship between two variables.
Calculate central tendency measures.
- The most prevalent response or value in the data set is the mode.
- When you arrange data set from low to high, the median is the value in the exact middle.
- The sum of all values divided by the number of values is the mean.
Calculate the variability measurements.
- The highest value of data set minus the lowest value is called the range.
- The range of the data set’s middle half is interquartile range.
- The average distance between each value in your data collection and the mean is standard deviation.
- The square of the standard deviation is the variance.
Step 4: Use inferential statistics to test hypotheses or create estimates.
- Estimation is the process of determining population parameters using sample statistics.
- Hypothesis testing is a formal procedure for employing samples to test research assumptions about the population.
- A point estimate is a number that indicates your best approximation of a parameter’s exact value.
- An interval estimate is a set of numbers that represents your best guess as to where the parameter is located.
Testing Hypotheses
- A test statistic indicates how far your data deviates from the test’s null hypothesis.
- A p value indicates how likely it is that you obtain your results if the null hypothesis is true in the population.
- Comparison tests look for differences in outcomes across groups.
- Correlation tests look at how variables are related without assuming causation.
Step 5: Analyze your findings
The importance of statistics, size of the effect, errors in judgement, statistics: frequentist vs. bayesian, leave a comment cancel reply.
Your email address will not be published. Required fields are marked *
Save my name, email, and website in this browser for the next time I comment.
Have a language expert improve your writing
Run a free plagiarism check in 10 minutes, generate accurate citations for free.
- Knowledge Base
An Easy Introduction to Statistical Significance (With Examples)
Published on January 7, 2021 by Pritha Bhandari . Revised on June 22, 2023.
If a result is statistically significant , that means it’s unlikely to be explained solely by chance or random factors. In other words, a statistically significant result has a very low chance of occurring if there were no true effect in a research study.
The p value , or probability value, tells you the statistical significance of a finding. In most studies, a p value of 0.05 or less is considered statistically significant, but this threshold can also be set higher or lower.
Table of contents
How do you test for statistical significance, what is a significance level, problems with relying on statistical significance, other types of significance in research, other interesting articles, frequently asked questions about statistical significance.
In quantitative research , data are analyzed through null hypothesis significance testing, or hypothesis testing. This is a formal procedure for assessing whether a relationship between variables or a difference between groups is statistically significant.
Null and alternative hypotheses
To begin, research predictions are rephrased into two main hypotheses: the null and alternative hypothesis .
- A null hypothesis ( H 0 ) always predicts no true effect, no relationship between variables , or no difference between groups.
- An alternative hypothesis ( H a or H 1 ) states your main prediction of a true effect, a relationship between variables, or a difference between groups.
Hypothesis testin g always starts with the assumption that the null hypothesis is true. Using this procedure, you can assess the likelihood (probability) of obtaining your results under this assumption. Based on the outcome of the test, you can reject or retain the null hypothesis.
- H 0 : There is no difference in happiness between actively smiling and not smiling.
- H a : Actively smiling leads to more happiness than not smiling.
Test statistics and p values
Every statistical test produces:
- A test statistic that indicates how closely your data match the null hypothesis.
- A corresponding p value that tells you the probability of obtaining this result if the null hypothesis is true.
The p value determines statistical significance. An extremely low p value indicates high statistical significance, while a high p value means low or no statistical significance.
Next, you perform a t test to see whether actively smiling leads to more happiness. Using the difference in average happiness between the two groups, you calculate:
- a t value (the test statistic) that tells you how much the sample data differs from the null hypothesis,
- a p value showing the likelihood of finding this result if the null hypothesis is true.
Here's why students love Scribbr's proofreading services
Discover proofreading & editing
The significance level , or alpha (α), is a value that the researcher sets in advance as the threshold for statistical significance. It is the maximum risk of making a false positive conclusion ( Type I error ) that you are willing to accept .
In a hypothesis test, the p value is compared to the significance level to decide whether to reject the null hypothesis.
- If the p value is higher than the significance level, the null hypothesis is not refuted, and the results are not statistically significant .
- If the p value is lower than the significance level, the results are interpreted as refuting the null hypothesis and reported as statistically significant .
Usually, the significance level is set to 0.05 or 5%. That means your results must have a 5% or lower chance of occurring under the null hypothesis to be considered statistically significant.
The significance level can be lowered for a more conservative test. That means an effect has to be larger to be considered statistically significant.
The significance level may also be set higher for significance testing in non-academic marketing or business contexts. This makes the study less rigorous and increases the probability of finding a statistically significant result.
As best practice, you should set a significance level before you begin your study. Otherwise, you can easily manipulate your results to match your research predictions.
It’s important to note that hypothesis testing can only show you whether or not to reject the null hypothesis in favor of the alternative hypothesis. It can never “prove” the null hypothesis, because the lack of a statistically significant effect doesn’t mean that absolutely no effect exists.
When reporting statistical significance, include relevant descriptive statistics about your data (e.g., means and standard deviations ) as well as the test statistic and p value.
There are various critiques of the concept of statistical significance and how it is used in research.
Researchers classify results as statistically significant or non-significant using a conventional threshold that lacks any theoretical or practical basis. This means that even a tiny 0.001 decrease in a p value can convert a research finding from statistically non-significant to significant with almost no real change in the effect.
On its own, statistical significance may also be misleading because it’s affected by sample size. In extremely large samples , you’re more likely to obtain statistically significant results, even if the effect is actually small or negligible in the real world. This means that small effects are often exaggerated if they meet the significance threshold, while interesting results are ignored when they fall short of meeting the threshold.
The strong emphasis on statistical significance has led to a serious publication bias and replication crisis in the social sciences and medicine over the last few decades. Results are usually only published in academic journals if they show statistically significant results—but statistically significant results often can’t be reproduced in high quality replication studies.
As a result, many scientists call for retiring statistical significance as a decision-making tool in favor of more nuanced approaches to interpreting results.
That’s why APA guidelines advise reporting not only p values but also effect sizes and confidence intervals wherever possible to show the real world implications of a research outcome.
Aside from statistical significance, clinical significance and practical significance are also important research outcomes.
Practical significance shows you whether the research outcome is important enough to be meaningful in the real world. It’s indicated by the effect size of the study.
Clinical significance is relevant for intervention and treatment studies. A treatment is considered clinically significant when it tangibly or substantially improves the lives of patients.
If you want to know more about statistics , methodology , or research bias , make sure to check out some of our other articles with explanations and examples.
- Normal distribution
- Descriptive statistics
- Measures of central tendency
- Correlation coefficient
Methodology
- Cluster sampling
- Stratified sampling
- Types of interviews
- Cohort study
- Thematic analysis
Research bias
- Implicit bias
- Cognitive bias
- Survivorship bias
- Availability heuristic
- Nonresponse bias
- Regression to the mean
Statistical significance is a term used by researchers to state that it is unlikely their observations could have occurred under the null hypothesis of a statistical test . Significance is usually denoted by a p -value , or probability value.
Statistical significance is arbitrary – it depends on the threshold, or alpha value, chosen by the researcher. The most common threshold is p < 0.05, which means that the data is likely to occur less than 5% of the time under the null hypothesis .
When the p -value falls below the chosen alpha value, then we say the result of the test is statistically significant.
A p -value , or probability value, is a number describing how likely it is that your data would have occurred under the null hypothesis of your statistical test .
P -values are usually automatically calculated by the program you use to perform your statistical test. They can also be estimated using p -value tables for the relevant test statistic .
P -values are calculated from the null distribution of the test statistic. They tell you how often a test statistic is expected to occur under the null hypothesis of the statistical test, based on where it falls in the null distribution.
If the test statistic is far from the mean of the null distribution, then the p -value will be small, showing that the test statistic is not likely to have occurred under the null hypothesis.
No. The p -value only tells you how likely the data you have observed is to have occurred under the null hypothesis .
If the p -value is below your threshold of significance (typically p < 0.05), then you can reject the null hypothesis, but this does not necessarily mean that your alternative hypothesis is true.
Cite this Scribbr article
If you want to cite this source, you can copy and paste the citation or click the “Cite this Scribbr article” button to automatically add the citation to our free Citation Generator.
Bhandari, P. (2023, June 22). An Easy Introduction to Statistical Significance (With Examples). Scribbr. Retrieved April 2, 2024, from https://www.scribbr.com/statistics/statistical-significance/
Is this article helpful?
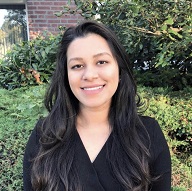
Pritha Bhandari
Other students also liked, understanding p values | definition and examples, what is effect size and why does it matter (examples), hypothesis testing | a step-by-step guide with easy examples, what is your plagiarism score.

Understanding and Using Statistical Methods
Statistics is a set of tools used to organize and analyze data. Data must either be numeric in origin or transformed by researchers into numbers. For instance, statistics could be used to analyze percentage scores English students receive on a grammar test: the percentage scores ranging from 0 to 100 are already in numeric form. Statistics could also be used to analyze grades on an essay by assigning numeric values to the letter grades, e.g., A=4, B=3, C=2, D=1, and F=0.
Employing statistics serves two purposes, (1) description and (2) prediction. Statistics are used to describe the characteristics of groups. These characteristics are referred to as variables . Data is gathered and recorded for each variable. Descriptive statistics can then be used to reveal the distribution of the data in each variable.
Statistics is also frequently used for purposes of prediction. Prediction is based on the concept of generalizability : if enough data is compiled about a particular context (e.g., students studying writing in a specific set of classrooms), the patterns revealed through analysis of the data collected about that context can be generalized (or predicted to occur in) similar contexts. The prediction of what will happen in a similar context is probabilistic . That is, the researcher is not certain that the same things will happen in other contexts; instead, the researcher can only reasonably expect that the same things will happen.
Prediction is a method employed by individuals throughout daily life. For instance, if writing students begin class every day for the first half of the semester with a five-minute freewriting exercise, then they will likely come to class the first day of the second half of the semester prepared to again freewrite for the first five minutes of class. The students will have made a prediction about the class content based on their previous experiences in the class: Because they began all previous class sessions with freewriting, it would be probable that their next class session will begin the same way. Statistics is used to perform the same function; the difference is that precise probabilities are determined in terms of the percentage chance that an outcome will occur, complete with a range of error. Prediction is a primary goal of inferential statistics.
Revealing Patterns Using Descriptive Statistics
Descriptive statistics, not surprisingly, "describe" data that have been collected. Commonly used descriptive statistics include frequency counts, ranges (high and low scores or values), means, modes, median scores, and standard deviations. Two concepts are essential to understanding descriptive statistics: variables and distributions .
Statistics are used to explore numerical data (Levin, 1991). Numerical data are observations which are recorded in the form of numbers (Runyon, 1976). Numbers are variable in nature, which means that quantities vary according to certain factors. For examples, when analyzing the grades on student essays, scores will vary for reasons such as the writing ability of the student, the students' knowledge of the subject, and so on. In statistics, these reasons are called variables. Variables are divided into three basic categories:
Nominal Variables
Nominal variables classify data into categories. This process involves labeling categories and then counting frequencies of occurrence (Runyon, 1991). A researcher might wish to compare essay grades between male and female students. Tabulations would be compiled using the categories "male" and "female." Sex would be a nominal variable. Note that the categories themselves are not quantified. Maleness or femaleness are not numerical in nature, rather the frequencies of each category results in data that is quantified -- 11 males and 9 females.
Ordinal Variables
Ordinal variables order (or rank) data in terms of degree. Ordinal variables do not establish the numeric difference between data points. They indicate only that one data point is ranked higher or lower than another (Runyon, 1991). For instance, a researcher might want to analyze the letter grades given on student essays. An A would be ranked higher than a B, and a B higher than a C. However, the difference between these data points, the precise distance between an A and a B, is not defined. Letter grades are an example of an ordinal variable.
Interval Variables
Interval variables score data. Thus the order of data is known as well as the precise numeric distance between data points (Runyon, 1991). A researcher might analyze the actual percentage scores of the essays, assuming that percentage scores are given by the instructor. A score of 98 (A) ranks higher than a score of 87 (B), which ranks higher than a score of 72 (C). Not only is the order of these three data points known, but so is the exact distance between them -- 11 percentage points between the first two, 15 percentage points between the second two and 26 percentage points between the first and last data points.
Distributions
A distribution is a graphic representation of data. The line formed by connecting data points is called a frequency distribution. This line may take many shapes. The single most important shape is that of the bell-shaped curve, which characterizes the distribution as "normal." A perfectly normal distribution is only a theoretical ideal. This ideal, however, is an essential ingredient in statistical decision-making (Levin, 1991). A perfectly normal distribution is a mathematical construct which carries with it certain mathematical properties helpful in describing the attributes of the distribution. Although frequency distribution based on actual data points seldom, if ever, completely matches a perfectly normal distribution, a frequency distribution often can approach such a normal curve.
The closer a frequency distribution resembles a normal curve, the more probable that the distribution maintains those same mathematical properties as the normal curve. This is an important factor in describing the characteristics of a frequency distribution. As a frequency distribution approaches a normal curve, generalizations about the data set from which the distribution was derived can be made with greater certainty. And it is this notion of generalizability upon which statistics is founded. It is important to remember that not all frequency distributions approach a normal curve. Some are skewed. When a frequency distribution is skewed, the characteristics inherent to a normal curve no longer apply.
Making Predictions Using Inferential Statistics
Inferential statistics are used to draw conclusions and make predictions based on the descriptions of data. In this section, we explore inferential statistics by using an extended example of experimental studies. Key concepts used in our discussion are probability, populations, and sampling.
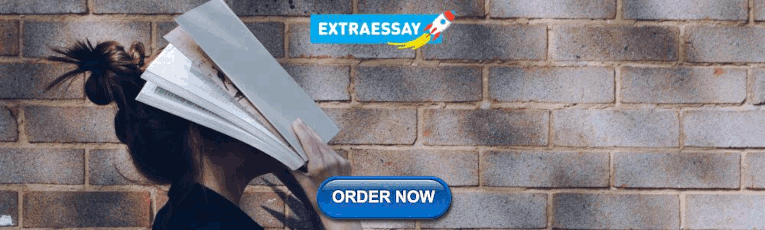
Experiments
A typical experimental study involves collecting data on the behaviors, attitudes, or actions of two or more groups and attempting to answer a research question (often called a hypothesis). Based on the analysis of the data, a researcher might then attempt to develop a causal model that can be generalized to populations.
A question that might be addressed through experimental research might be "Does grammar-based writing instruction produce better writers than process-based writing instruction?" Because it would be impossible and impractical to observe, interview, survey, etc. all first-year writing students and instructors in classes using one or the other of these instructional approaches, a researcher would study a sample – or a subset – of a population. Sampling – or the creation of this subset of a population – is used by many researchers who desire to make sense of some phenomenon.
To analyze differences in the ability of student writers who are taught in each type of classroom, the researcher would compare the writing performance of the two groups of students.
Dependent Variables
In an experimental study, a variable whose score depends on (or is determined or caused by) another variable is called a dependent variable. For instance, an experiment might explore the extent to which the writing quality of final drafts of student papers is affected by the kind of instruction they received. In this case, the dependent variable would be writing quality of final drafts.
Independent Variables
In an experimental study, a variable that determines (or causes) the score of a dependent variable is called an independent variable. For instance, an experiment might explore the extent to which the writing quality of final drafts of student papers is affected by the kind of instruction they received. In this case, the independent variable would be the kind of instruction students received.
Probability
Beginning researchers most often use the word probability to express a subjective judgment about the likelihood, or degree of certainty, that a particular event will occur. People say such things as: "It will probably rain tomorrow." "It is unlikely that we will win the ball game." It is possible to assign a number to the event being predicted, a number between 0 and 1, which represents degree of confidence that the event will occur. For example, a student might say that the likelihood an instructor will give an exam next week is about 90 percent, or .9. Where 100 percent, or 1.00, represents certainty, .9 would mean the student is almost certain the instructor will give an exam. If the student assigned the number .6, the likelihood of an exam would be just slightly greater than the likelihood of no exam. A rating of 0 would indicate complete certainty that no exam would be given(Shoeninger, 1971).
The probability of a particular outcome or set of outcomes is called a p-value . In our discussion, a p-value will be symbolized by a p followed by parentheses enclosing a symbol of the outcome or set of outcomes. For example, p(X) should be read, "the probability of a given X score" (Shoeninger). Thus p(exam) should be read, "the probability an instructor will give an exam next week."
A population is a group which is studied. In educational research, the population is usually a group of people. Researchers seldom are able to study every member of a population. Usually, they instead study a representative sample – or subset – of a population. Researchers then generalize their findings about the sample to the population as a whole.
Sampling is performed so that a population under study can be reduced to a manageable size. This can be accomplished via random sampling, discussed below, or via matching.
Random sampling is a procedure used by researchers in which all samples of a particular size have an equal chance to be chosen for an observation, experiment, etc (Runyon and Haber, 1976). There is no predetermination as to which members are chosen for the sample. This type of sampling is done in order to minimize scientific biases and offers the greatest likelihood that a sample will indeed be representative of the larger population. The aim here is to make the sample as representative of the population as possible. Note that the closer a sample distribution approximates the population distribution, the more generalizable the results of the sample study are to the population. Notions of probability apply here. Random sampling provides the greatest probability that the distribution of scores in a sample will closely approximate the distribution of scores in the overall population.
Matching is a method used by researchers to gain accurate and precise results of a study so that they may be applicable to a larger population. After a population has been examined and a sample has been chosen, a researcher must then consider variables, or extrinsic factors, that might affect the study. Matching methods apply when researchers are aware of extrinsic variables before conducting a study. Two methods used to match groups are:
Precision Matching
In precision matching , there is an experimental group that is matched with a control group. Both groups, in essence, have the same characteristics. Thus, the proposed causal relationship/model being examined allows for the probabilistic assumption that the result is generalizable.
Frequency Distribution
Frequency distribution is more manageable and efficient than precision matching. Instead of one-to-one matching that must be administered in precision matching, frequency distribution allows the comparison of an experimental and control group through relevant variables. If three Communications majors and four English majors are chosen for the control group, then an equal proportion of three Communications major and four English majors should be allotted to the experiment group. Of course, beyond their majors, the characteristics of the matched sets of participants may in fact be vastly different.
Although, in theory, matching tends to produce valid conclusions, a rather obvious difficulty arises in finding subjects which are compatible. Researchers may even believe that experimental and control groups are identical when, in fact, a number of variables have been overlooked. For these reasons, researchers tend to reject matching methods in favor of random sampling.
Statistics can be used to analyze individual variables, relationships among variables, and differences between groups. In this section, we explore a range of statistical methods for conducting these analyses.
Statistics can be used to analyze individual variables, relationships among variables, and differences between groups.
Analyzing Individual Variables
The statistical procedures used to analyze a single variable describing a group (such as a population or representative sample) involve measures of central tendency and measures of variation . To explore these measures, a researcher first needs to consider the distribution , or range of values of a particular variable in a population or sample. Normal distribution occurs if the distribution of a population is completely normal. When graphed, this type of distribution will look like a bell curve; it is symmetrical and most of the scores cluster toward the middle. Skewed Distribution simply means the distribution of a population is not normal. The scores might cluster toward the right or the left side of the curve, for instance. Or there might be two or more clusters of scores, so that the distribution looks like a series of hills.
Once frequency distributions have been determined, researchers can calculate measures of central tendency and measures of variation. Measures of central tendency indicate averages of the distribution, and measures of variation indicate the spread, or range, of the distribution (Hinkle, Wiersma and Jurs 1988).
Measures of Central Tendency
Central tendency is measured in three ways: mean , median and mode . The mean is simply the average score of a distribution. The median is the center, or middle score within a distribution. The mode is the most frequent score within a distribution. In a normal distribution, the mean, median and mode are identical.
Measures of Variation
Measures of variation determine the range of the distribution, relative to the measures of central tendency. Where the measures of central tendency are specific data points, measures of variation are lengths between various points within the distribution. Variation is measured in terms of range, mean deviation, variance, and standard deviation (Hinkle, Wiersma and Jurs 1988).
The range is the distance between the lowest data point and the highest data point. Deviation scores are the distances between each data point and the mean.
Mean deviation is the average of the absolute values of the deviation scores; that is, mean deviation is the average distance between the mean and the data points. Closely related to the measure of mean deviation is the measure of variance .
Variance also indicates a relationship between the mean of a distribution and the data points; it is determined by averaging the sum of the squared deviations. Squaring the differences instead of taking the absolute values allows for greater flexibility in calculating further algebraic manipulations of the data. Another measure of variation is the standard deviation .
Standard deviation is the square root of the variance. This calculation is useful because it allows for the same flexibility as variance regarding further calculations and yet also expresses variation in the same units as the original measurements (Hinkle, Wiersma and Jurs 1988).
Analyzing Differences Between Groups
Statistical tests can be used to analyze differences in the scores of two or more groups. The following statistical tests are commonly used to analyze differences between groups:
A t-test is used to determine if the scores of two groups differ on a single variable. A t-test is designed to test for the differences in mean scores. For instance, you could use a t-test to determine whether writing ability differs among students in two classrooms.
Note: A t-test is appropriate only when looking at paired data. It is useful in analyzing scores of two groups of participants on a particular variable or in analyzing scores of a single group of participants on two variables.
Matched Pairs T-Test
This type of t-test could be used to determine if the scores of the same participants in a study differ under different conditions. For instance, this sort of t-test could be used to determine if people write better essays after taking a writing class than they did before taking the writing class.
Analysis of Variance (ANOVA)
The ANOVA (analysis of variance) is a statistical test which makes a single, overall decision as to whether a significant difference is present among three or more sample means (Levin 484). An ANOVA is similar to a t-test. However, the ANOVA can also test multiple groups to see if they differ on one or more variables. The ANOVA can be used to test between-groups and within-groups differences. There are two types of ANOVAs:
One-Way ANOVA: This tests a group or groups to determine if there are differences on a single set of scores. For instance, a one-way ANOVA could determine whether freshmen, sophomores, juniors, and seniors differed in their reading ability.
Multiple ANOVA (MANOVA): This tests a group or groups to determine if there are differences on two or more variables. For instance, a MANOVA could determine whether freshmen, sophomores, juniors, and seniors differed in reading ability and whether those differences were reflected by gender. In this case, a researcher could determine (1) whether reading ability differed across class levels, (2) whether reading ability differed across gender, and (3) whether there was an interaction between class level and gender.
Analyzing Relationships Among Variables
Statistical relationships between variables rely on notions of correlation and regression. These two concepts aim to describe the ways in which variables relate to one another:
Correlation
Correlation tests are used to determine how strongly the scores of two variables are associated or correlated with each other. A researcher might want to know, for instance, whether a correlation exists between students' writing placement examination scores and their scores on a standardized test such as the ACT or SAT. Correlation is measured using values between +1.0 and -1.0. Correlations close to 0 indicate little or no relationship between two variables, while correlations close to +1.0 (or -1.0) indicate strong positive (or negative) relationships (Hayes et al. 554).
Correlation denotes positive or negative association between variables in a study. Two variables are positively associated when larger values of one tend to be accompanied by larger values of the other. The variables are negatively associated when larger values of one tend to be accompanied by smaller values of the other (Moore 208).
An example of a strong positive correlation would be the correlation between age and job experience. Typically, the longer people are alive, the more job experience they might have.
An example of a strong negative relationship might occur between the strength of people's party affiliations and their willingness to vote for a candidate from different parties. In many elections, Democrats are unlikely to vote for Republicans, and vice versa.
Regression analysis attempts to determine the best "fit" between two or more variables. The independent variable in a regression analysis is a continuous variable, and thus allows you to determine how one or more independent variables predict the values of a dependent variable.
Simple Linear Regression is the simplest form of regression. Like a correlation, it determines the extent to which one independent variables predicts a dependent variable. You can think of a simple linear regression as a correlation line. Regression analysis provides you with more information than correlation does, however. It tells you how well the line "fits" the data. That is, it tells you how closely the line comes to all of your data points. The line in the figure indicates the regression line drawn to find the best fit among a set of data points. Each dot represents a person and the axes indicate the amount of job experience and the age of that person. The dotted lines indicate the distance from the regression line. A smaller total distance indicates a better fit. Some of the information provided in a regression analysis, as a result, indicates the slope of the regression line, the R value (or correlation), and the strength of the fit (an indication of the extent to which the line can account for variations among the data points).
Multiple Linear Regression allows one to determine how well multiple independent variables predict the value of a dependent variable. A researcher might examine, for instance, how well age and experience predict a person's salary. The interesting thing here is that one would no longer be dealing with a regression "line." Instead, since the study deals with three dimensions (age, experience, and salary), it would be dealing with a plane, that is, with a two-dimensional figure. If a fourth variable was added to the equations, one would be dealing with a three-dimensional figure, and so on.
Misuses of Statistics
Statistics consists of tests used to analyze data. These tests provide an analytic framework within which researchers can pursue their research questions. This framework provides one way of working with observable information. Like other analytic frameworks, statistical tests can be misused, resulting in potential misinterpretation and misrepresentation. Researchers decide which research questions to ask, which groups to study, how those groups should be divided, which variables to focus upon, and how best to categorize and measure such variables. The point is that researchers retain the ability to manipulate any study even as they decide what to study and how to study it.
Potential Misuses:
- Manipulating scale to change the appearance of the distribution of data
- Eliminating high/low scores for more coherent presentation
- Inappropriately focusing on certain variables to the exclusion of other variables
- Presenting correlation as causation
Measures Against Potential Misuses:
- Testing for reliability and validity
- Testing for statistical significance
- Critically reading statistics
Annotated Bibliography
Dear, K. (1997, August 28). SurfStat australia . Available: http://surfstat.newcastle.edu.au/surfstat/main/surfstat-main.html
A comprehensive site contain an online textbook, links together statistics sites, exercises, and a hotlist for Java applets.
de Leeuw, J. (1997, May 13 ). Statistics: The study of stability in variation . Available: http://www.stat.ucla.edu/textbook/ [1997, December 8].
An online textbook providing discussions specifically regarding variability.
Ewen, R.B. (1988). The workbook for introductory statistics for the behavioral sciences. Orlando, FL: Harcourt Brace Jovanovich.
A workbook providing sample problems typical of the statistical applications in social sciences.
Glass, G. (1996, August 26). COE 502: Introduction to quantitative methods . Available: http://seamonkey.ed.asu.edu/~gene/502/home.html
Outline of a basic statistics course in the college of education at Arizona State University, including a list of statistic resources on the Internet and access to online programs using forms and PERL to analyze data.
Hartwig, F., Dearing, B.E. (1979). Exploratory data analysis . Newberry Park, CA: Sage Publications, Inc.
Hayes, J. R., Young, R.E., Matchett, M.L., McCaffrey, M., Cochran, C., and Hajduk, T., eds. (1992). Reading empirical research studies: The rhetoric of research . Hillsdale, NJ: Lawrence Erlbaum Associates.
A text focusing on the language of research. Topics vary from "Communicating with Low-Literate Adults" to "Reporting on Journalists."
Hinkle, Dennis E., Wiersma, W. and Jurs, S.G. (1988). Applied statistics for the behavioral sciences . Boston: Houghton.
This is an introductory text book on statistics. Each of 22 chapters includes a summary, sample exercises and highlighted main points. The book also includes an index by subject.
Kleinbaum, David G., Kupper, L.L. and Muller K.E. Applied regression analysis and other multivariable methods 2nd ed . Boston: PWS-KENT Publishing Company.
An introductory text with emphasis on statistical analyses. Chapters contain exercises.
Kolstoe, R.H. (1969). Introduction to statistics for the behavioral sciences . Homewood, ILL: Dorsey.
Though more than 25-years-old, this textbook uses concise chapters to explain many essential statistical concepts. Information is organized in a simple and straightforward manner.
Levin, J., and James, A.F. (1991). Elementary statistics in social research, 5th ed . New York: HarperCollins.
This textbook presents statistics in three major sections: Description, From Description to Decision Making and Decision Making. The first chapter underlies reasons for using statistics in social research. Subsequent chapters detail the process of conducting and presenting statistics.
Liebetrau, A.M. (1983). Measures of association . Newberry Park, CA: Sage Publications, Inc.
Mendenhall, W.(1975). Introduction to probability and statistics, 4th ed. North Scltuate, MA: Duxbury Press.
An introductory textbook. A good overview of statistics. Includes clear definitions and exercises.
Moore, David S. (1979). Statistics: Concepts and controversies , 2nd ed . New York: W. H. Freeman and Company.
Introductory text. Basic overview of statistical concepts. Includes discussions of concrete applications such as opinion polls and Consumer Price Index.
Mosier, C.T. (1997). MG284 Statistics I - notes. Available: http://phoenix.som.clarkson.edu/~cmosier/statistics/main/outline/index.html
Explanations of fundamental statistical concepts.
Newton, H.J., Carrol, J.H., Wang, N., & Whiting, D.(1996, Fall). Statistics 30X class notes. Available: http://stat.tamu.edu/stat30x/trydouble2.html [1997, December 10].
This site contains a hyperlinked list of very comprehensive course notes from and introductory statistics class. A large variety of statistical concepts are covered.
Runyon, R.P., and Haber, A. (1976). Fundamentals of behavioral statistics , 3rd ed . Reading, MA: Addison-Wesley Publishing Company.
This is a textbook that divides statistics into categories of descriptive statistics and inferential statistics. It presents statistical procedures primarily through examples. This book includes sectional reviews, reviews of basic mathematics and also a glossary of symbols common to statistics.
Schoeninger, D.W. and Insko, C.A. (1971). Introductory statistics for the behavioral sciences . Boston: Allyn and Bacon, Inc.
An introductory text including discussions of correlation, probability, distribution, and variance. Includes statistical tables in the appendices.
Stevens, J. (1986). Applied multivariate statistics for the social sciences . Hillsdale, NJ: Lawrence Erlbaum Associates.
Stockberger, D. W. (1996). Introductory statistics: Concepts, models and applications . Available: http://www.psychstat.smsu.edu/ [1997, December 8].
Describes various statistical analyses. Includes statistical tables in the appendix.
Local Resources
If you are a member of the Colorado State University community and seek more in-depth help with analyzing data from your research (e.g., from an undergraduate or graduate research project), please contact CSU's Graybill Statistical Laboratory for statistical consulting assistance at http://www.stat.colostate.edu/statlab.html .
Jackson, Shawna, Karen Marcus, Cara McDonald, Timothy Wehner, & Mike Palmquist. (2005). Statistics: An Introduction. Writing@CSU . Colorado State University. https://writing.colostate.edu/guides/guide.cfm?guideid=67
Statistical Data Analysis in Education Essay (Critical Writing)
Description of the research problem, research methodology: design, approach, and structure, data and research conclusions, critique of the article.
In the area of education, much attention should be paid to the development of statistical reasoning in teachers. Scheaffer and Jacobbe (2014) and Utts (2015) state that the use of statistical data with the focus on its further analysis and interpretation is often a challenge for educators, and they need to concentrate on developing skills in working with statistical methods.
In their article “Hold My Calls: An Activity for Introducing the Statistical Process,” Abel and Poling (2015) described the specific statistical activity that can be used in the teaching-learning process at the secondary level to explain the principles of the statistical process. The article was published in Teaching Statistics , and this descriptive research aimed to discuss aspects of the statistical process with the focus on data analysis and interpretation.
The statistical process is based on a set of concrete phases that should be performed in a series. The data analysis and interpretation are usually regarded as the most challenging stages of this process (Slootmaeckers, Kerremans, & Adriaensen, 2014). Students’ skills need to be improved regarding the use of statistical methods to conduct studies and analyze the data (Dierker, Cooper, Alexander, Selya, & Rose, 2015).
The problem is in the fact that many teachers also do not have enough skills to apply and explain statistical methods appropriately (Ben-Zvi, 2014; Fotache & Strimbei, 2015). Thus, Abel and Poling (2015) focused on describing the particular statistical activity aimed at providing students and educators with clear information regarding the stages of the statistical process.
The article by Abel and Poling (2015) presents the description of the results of implementing the GAISE framework for the statistical process. The researchers recruited practicing teachers to participate in the study and use the four-step GAISE model to examine how the use of mobile phones can influence the person’s reactions on roads.
Four groups of participants were formed, and the practical sessions were held for two days. The structure of the article depends on the number of components in the GAISE framework. Thus, the article includes the following sections: Abstract, Introduction, Context, Statistical Process and Activity, including such tasks as to formulate questions, collect data, analyze data, and interpret results, Discussion, and Conclusion.
Abel and Poling (2015) focused on collecting the narrative data regarding the results of the activity to conclude about the successes related to implementing the GAISE framework. It was found that all groups of teachers discussed the activity as important to be implemented in the classroom settings because the framework allowed for understanding the nature of stages in the statistical process. The participants emphasized the problems associated with the choice of appropriate statistical methods to analyze the data. Also, the participants accentuated the possibility of adapting the framework to students’ needs.
Abel and Poling’s (2015) article has a descriptive title that allows for making conclusions regarding the article’s content, but the abstract is rather short, and it does not provide all the useful information about the work. Still, the authors present the clear purpose of the study in the introduction, and it is impossible to state that this article is appropriate to explain the nature of analyzing and interpreting statistical data in the field of education. The researchers chose to assess the participants’ results at each stage related to the GAISE framework. This approach is effective while paying attention to the nature of the descriptive study.
Thus, Abel and Poling (2015) were able to evaluate successes of the participants regarding the choice of graphs for the statistical analysis, and they determined weaknesses in teachers’ approaches to analyzing the data with the focus on the selection of wrong statistical methods. The section related to the interpretation stage was also effective because the researchers provided a comprehensive analysis of the participants’ activities regarding the challenging task of interpreting the study results.
Still, the detailed information about the procedures for analyzing and interpreting the data was presented only in the section where the activity was described, and the Discussion section needs improvement. It is important to pay more attention to discussing why the procedure of analyzing the statistical data is important, and how mistakes in the analysis can influence the process of interpretation (Ziegler & Garfield, 2013). Therefore, it is possible to expand the Discussion section and provide references to other studies to evaluate the received results.
Despite the determined limitations in the presentation and discussion of the study results, it is possible to state that the authors’ statements are clear, and based on the evidence. Thus, to support their ideas, the authors cited the limited number of studies, but all of them can be discussed as directly related to the study. The purpose of the research was achieved, and the authors’ conclusions are reasonable.
The article by Abel and Poling is effective to accentuate the importance of the data analysis and interpretation stages in the statistical process, as well as to determine possible challenges for educators and students. Still, the article requires improvements in terms of developing its sections. More attention should be paid to the discussion of the activity’s results in the context of prior studies.
Abel, T., & Poling, L. (2015). Hold my calls: An activity for introducing the statistical process. Teaching Statistics , 37 (3), 96-103.
Ben-Zvi, D. (2014). Data handling and statistics teaching and learning. Mathematics Education , 1 (2), 137-140.
Dierker, L., Cooper, J., Alexander, J., Selya, A., & Rose, J. (2015). Evaluating access: A comparison of demographic and disciplinary characteristics of students enrolled in a traditional introductory statistics course vs. a multidisciplinary, project-based course. Journal of Interdisciplinary Studies in Education , 4 (1), 22-37.
Fotache, M., & Strimbei, C. (2015). SQL and data analysis: Some implications for data analysts and higher education. Procedia Economics and Finance , 20 (1), 243-251.
Scheaffer, R. L., & Jacobbe, T. (2014). Statistics education in the K–12 schools of the United States: A brief history. Journal of Statistics Education , 22 (2), 1-10.
Slootmaeckers, K., Kerremans, B., & Adriaensen, J. (2014). Too afraid to learn: Attitudes towards statistics as a barrier to learning statistics and to acquiring quantitative skills. Politics , 34 (2), 191-200.
Utts, J. (2015). The many facets of statistics education: 175 years of common themes. The American Statistician , 69 (2), 100-107.
Ziegler, L., & Garfield, J. (2013). Teaching bits: Statistics education articles from 2012 and 2013. Journal of Statistics Education , 21 (1), 1-18.
- Chicago (A-D)
- Chicago (N-B)
IvyPanda. (2020, August 3). Statistical Data Analysis in Education. https://ivypanda.com/essays/statistical-data-analysis-in-education/
"Statistical Data Analysis in Education." IvyPanda , 3 Aug. 2020, ivypanda.com/essays/statistical-data-analysis-in-education/.
IvyPanda . (2020) 'Statistical Data Analysis in Education'. 3 August.
IvyPanda . 2020. "Statistical Data Analysis in Education." August 3, 2020. https://ivypanda.com/essays/statistical-data-analysis-in-education/.
1. IvyPanda . "Statistical Data Analysis in Education." August 3, 2020. https://ivypanda.com/essays/statistical-data-analysis-in-education/.
Bibliography
IvyPanda . "Statistical Data Analysis in Education." August 3, 2020. https://ivypanda.com/essays/statistical-data-analysis-in-education/.
- Justice and Injustice in Genesis 4: The Story of Cain and Abel
- Network Security Tools: Brutus and Cain and Abel Overview
- Abel Athletics: Equipment Purchase Appraisal
- "Economic Development" by Eleanor M. Fox & Abel M. Mateus
- Abel Athletics Company's Financial Management
- “House Made of Dawn” by N. Scott Momaday
- Summary of the Fourth Chapter of Genesis
- Brothers in Bible: Love & Hatred in Old Testament
- European Maters in 18th Century in England
- Sibling Rivalry in the Bible
- Experience and Completion of Projects Correlation
- Tuberculosis Statistics Among Cigarette Smokers
- Correlation Types and Their Uses
- Statistical Decision-Making in Behavioral Sciences
- Antimalarial Drug Efficacy: Statistical Analysis

An official website of the United States government
The .gov means it’s official. Federal government websites often end in .gov or .mil. Before sharing sensitive information, make sure you’re on a federal government site.
The site is secure. The https:// ensures that you are connecting to the official website and that any information you provide is encrypted and transmitted securely.
- Publications
- Account settings
Preview improvements coming to the PMC website in October 2024. Learn More or Try it out now .
- Advanced Search
- Journal List
- Indian J Anaesth
- v.60(9); 2016 Sep
Basic statistical tools in research and data analysis
Zulfiqar ali.
Department of Anaesthesiology, Division of Neuroanaesthesiology, Sheri Kashmir Institute of Medical Sciences, Soura, Srinagar, Jammu and Kashmir, India
S Bala Bhaskar
1 Department of Anaesthesiology and Critical Care, Vijayanagar Institute of Medical Sciences, Bellary, Karnataka, India
Statistical methods involved in carrying out a study include planning, designing, collecting data, analysing, drawing meaningful interpretation and reporting of the research findings. The statistical analysis gives meaning to the meaningless numbers, thereby breathing life into a lifeless data. The results and inferences are precise only if proper statistical tests are used. This article will try to acquaint the reader with the basic research tools that are utilised while conducting various studies. The article covers a brief outline of the variables, an understanding of quantitative and qualitative variables and the measures of central tendency. An idea of the sample size estimation, power analysis and the statistical errors is given. Finally, there is a summary of parametric and non-parametric tests used for data analysis.
INTRODUCTION
Statistics is a branch of science that deals with the collection, organisation, analysis of data and drawing of inferences from the samples to the whole population.[ 1 ] This requires a proper design of the study, an appropriate selection of the study sample and choice of a suitable statistical test. An adequate knowledge of statistics is necessary for proper designing of an epidemiological study or a clinical trial. Improper statistical methods may result in erroneous conclusions which may lead to unethical practice.[ 2 ]
Variable is a characteristic that varies from one individual member of population to another individual.[ 3 ] Variables such as height and weight are measured by some type of scale, convey quantitative information and are called as quantitative variables. Sex and eye colour give qualitative information and are called as qualitative variables[ 3 ] [ Figure 1 ].

Classification of variables
Quantitative variables
Quantitative or numerical data are subdivided into discrete and continuous measurements. Discrete numerical data are recorded as a whole number such as 0, 1, 2, 3,… (integer), whereas continuous data can assume any value. Observations that can be counted constitute the discrete data and observations that can be measured constitute the continuous data. Examples of discrete data are number of episodes of respiratory arrests or the number of re-intubations in an intensive care unit. Similarly, examples of continuous data are the serial serum glucose levels, partial pressure of oxygen in arterial blood and the oesophageal temperature.
A hierarchical scale of increasing precision can be used for observing and recording the data which is based on categorical, ordinal, interval and ratio scales [ Figure 1 ].
Categorical or nominal variables are unordered. The data are merely classified into categories and cannot be arranged in any particular order. If only two categories exist (as in gender male and female), it is called as a dichotomous (or binary) data. The various causes of re-intubation in an intensive care unit due to upper airway obstruction, impaired clearance of secretions, hypoxemia, hypercapnia, pulmonary oedema and neurological impairment are examples of categorical variables.
Ordinal variables have a clear ordering between the variables. However, the ordered data may not have equal intervals. Examples are the American Society of Anesthesiologists status or Richmond agitation-sedation scale.
Interval variables are similar to an ordinal variable, except that the intervals between the values of the interval variable are equally spaced. A good example of an interval scale is the Fahrenheit degree scale used to measure temperature. With the Fahrenheit scale, the difference between 70° and 75° is equal to the difference between 80° and 85°: The units of measurement are equal throughout the full range of the scale.
Ratio scales are similar to interval scales, in that equal differences between scale values have equal quantitative meaning. However, ratio scales also have a true zero point, which gives them an additional property. For example, the system of centimetres is an example of a ratio scale. There is a true zero point and the value of 0 cm means a complete absence of length. The thyromental distance of 6 cm in an adult may be twice that of a child in whom it may be 3 cm.
STATISTICS: DESCRIPTIVE AND INFERENTIAL STATISTICS
Descriptive statistics[ 4 ] try to describe the relationship between variables in a sample or population. Descriptive statistics provide a summary of data in the form of mean, median and mode. Inferential statistics[ 4 ] use a random sample of data taken from a population to describe and make inferences about the whole population. It is valuable when it is not possible to examine each member of an entire population. The examples if descriptive and inferential statistics are illustrated in Table 1 .
Example of descriptive and inferential statistics

Descriptive statistics
The extent to which the observations cluster around a central location is described by the central tendency and the spread towards the extremes is described by the degree of dispersion.
Measures of central tendency
The measures of central tendency are mean, median and mode.[ 6 ] Mean (or the arithmetic average) is the sum of all the scores divided by the number of scores. Mean may be influenced profoundly by the extreme variables. For example, the average stay of organophosphorus poisoning patients in ICU may be influenced by a single patient who stays in ICU for around 5 months because of septicaemia. The extreme values are called outliers. The formula for the mean is

where x = each observation and n = number of observations. Median[ 6 ] is defined as the middle of a distribution in a ranked data (with half of the variables in the sample above and half below the median value) while mode is the most frequently occurring variable in a distribution. Range defines the spread, or variability, of a sample.[ 7 ] It is described by the minimum and maximum values of the variables. If we rank the data and after ranking, group the observations into percentiles, we can get better information of the pattern of spread of the variables. In percentiles, we rank the observations into 100 equal parts. We can then describe 25%, 50%, 75% or any other percentile amount. The median is the 50 th percentile. The interquartile range will be the observations in the middle 50% of the observations about the median (25 th -75 th percentile). Variance[ 7 ] is a measure of how spread out is the distribution. It gives an indication of how close an individual observation clusters about the mean value. The variance of a population is defined by the following formula:

where σ 2 is the population variance, X is the population mean, X i is the i th element from the population and N is the number of elements in the population. The variance of a sample is defined by slightly different formula:

where s 2 is the sample variance, x is the sample mean, x i is the i th element from the sample and n is the number of elements in the sample. The formula for the variance of a population has the value ‘ n ’ as the denominator. The expression ‘ n −1’ is known as the degrees of freedom and is one less than the number of parameters. Each observation is free to vary, except the last one which must be a defined value. The variance is measured in squared units. To make the interpretation of the data simple and to retain the basic unit of observation, the square root of variance is used. The square root of the variance is the standard deviation (SD).[ 8 ] The SD of a population is defined by the following formula:

where σ is the population SD, X is the population mean, X i is the i th element from the population and N is the number of elements in the population. The SD of a sample is defined by slightly different formula:

where s is the sample SD, x is the sample mean, x i is the i th element from the sample and n is the number of elements in the sample. An example for calculation of variation and SD is illustrated in Table 2 .
Example of mean, variance, standard deviation

Normal distribution or Gaussian distribution
Most of the biological variables usually cluster around a central value, with symmetrical positive and negative deviations about this point.[ 1 ] The standard normal distribution curve is a symmetrical bell-shaped. In a normal distribution curve, about 68% of the scores are within 1 SD of the mean. Around 95% of the scores are within 2 SDs of the mean and 99% within 3 SDs of the mean [ Figure 2 ].

Normal distribution curve
Skewed distribution
It is a distribution with an asymmetry of the variables about its mean. In a negatively skewed distribution [ Figure 3 ], the mass of the distribution is concentrated on the right of Figure 1 . In a positively skewed distribution [ Figure 3 ], the mass of the distribution is concentrated on the left of the figure leading to a longer right tail.

Curves showing negatively skewed and positively skewed distribution
Inferential statistics
In inferential statistics, data are analysed from a sample to make inferences in the larger collection of the population. The purpose is to answer or test the hypotheses. A hypothesis (plural hypotheses) is a proposed explanation for a phenomenon. Hypothesis tests are thus procedures for making rational decisions about the reality of observed effects.
Probability is the measure of the likelihood that an event will occur. Probability is quantified as a number between 0 and 1 (where 0 indicates impossibility and 1 indicates certainty).
In inferential statistics, the term ‘null hypothesis’ ( H 0 ‘ H-naught ,’ ‘ H-null ’) denotes that there is no relationship (difference) between the population variables in question.[ 9 ]
Alternative hypothesis ( H 1 and H a ) denotes that a statement between the variables is expected to be true.[ 9 ]
The P value (or the calculated probability) is the probability of the event occurring by chance if the null hypothesis is true. The P value is a numerical between 0 and 1 and is interpreted by researchers in deciding whether to reject or retain the null hypothesis [ Table 3 ].
P values with interpretation

If P value is less than the arbitrarily chosen value (known as α or the significance level), the null hypothesis (H0) is rejected [ Table 4 ]. However, if null hypotheses (H0) is incorrectly rejected, this is known as a Type I error.[ 11 ] Further details regarding alpha error, beta error and sample size calculation and factors influencing them are dealt with in another section of this issue by Das S et al .[ 12 ]
Illustration for null hypothesis

PARAMETRIC AND NON-PARAMETRIC TESTS
Numerical data (quantitative variables) that are normally distributed are analysed with parametric tests.[ 13 ]
Two most basic prerequisites for parametric statistical analysis are:
- The assumption of normality which specifies that the means of the sample group are normally distributed
- The assumption of equal variance which specifies that the variances of the samples and of their corresponding population are equal.
However, if the distribution of the sample is skewed towards one side or the distribution is unknown due to the small sample size, non-parametric[ 14 ] statistical techniques are used. Non-parametric tests are used to analyse ordinal and categorical data.
Parametric tests
The parametric tests assume that the data are on a quantitative (numerical) scale, with a normal distribution of the underlying population. The samples have the same variance (homogeneity of variances). The samples are randomly drawn from the population, and the observations within a group are independent of each other. The commonly used parametric tests are the Student's t -test, analysis of variance (ANOVA) and repeated measures ANOVA.
Student's t -test
Student's t -test is used to test the null hypothesis that there is no difference between the means of the two groups. It is used in three circumstances:

where X = sample mean, u = population mean and SE = standard error of mean

where X 1 − X 2 is the difference between the means of the two groups and SE denotes the standard error of the difference.
- To test if the population means estimated by two dependent samples differ significantly (the paired t -test). A usual setting for paired t -test is when measurements are made on the same subjects before and after a treatment.
The formula for paired t -test is:

where d is the mean difference and SE denotes the standard error of this difference.
The group variances can be compared using the F -test. The F -test is the ratio of variances (var l/var 2). If F differs significantly from 1.0, then it is concluded that the group variances differ significantly.
Analysis of variance
The Student's t -test cannot be used for comparison of three or more groups. The purpose of ANOVA is to test if there is any significant difference between the means of two or more groups.
In ANOVA, we study two variances – (a) between-group variability and (b) within-group variability. The within-group variability (error variance) is the variation that cannot be accounted for in the study design. It is based on random differences present in our samples.
However, the between-group (or effect variance) is the result of our treatment. These two estimates of variances are compared using the F-test.
A simplified formula for the F statistic is:

where MS b is the mean squares between the groups and MS w is the mean squares within groups.
Repeated measures analysis of variance
As with ANOVA, repeated measures ANOVA analyses the equality of means of three or more groups. However, a repeated measure ANOVA is used when all variables of a sample are measured under different conditions or at different points in time.
As the variables are measured from a sample at different points of time, the measurement of the dependent variable is repeated. Using a standard ANOVA in this case is not appropriate because it fails to model the correlation between the repeated measures: The data violate the ANOVA assumption of independence. Hence, in the measurement of repeated dependent variables, repeated measures ANOVA should be used.
Non-parametric tests
When the assumptions of normality are not met, and the sample means are not normally, distributed parametric tests can lead to erroneous results. Non-parametric tests (distribution-free test) are used in such situation as they do not require the normality assumption.[ 15 ] Non-parametric tests may fail to detect a significant difference when compared with a parametric test. That is, they usually have less power.
As is done for the parametric tests, the test statistic is compared with known values for the sampling distribution of that statistic and the null hypothesis is accepted or rejected. The types of non-parametric analysis techniques and the corresponding parametric analysis techniques are delineated in Table 5 .
Analogue of parametric and non-parametric tests

Median test for one sample: The sign test and Wilcoxon's signed rank test
The sign test and Wilcoxon's signed rank test are used for median tests of one sample. These tests examine whether one instance of sample data is greater or smaller than the median reference value.
This test examines the hypothesis about the median θ0 of a population. It tests the null hypothesis H0 = θ0. When the observed value (Xi) is greater than the reference value (θ0), it is marked as+. If the observed value is smaller than the reference value, it is marked as − sign. If the observed value is equal to the reference value (θ0), it is eliminated from the sample.
If the null hypothesis is true, there will be an equal number of + signs and − signs.
The sign test ignores the actual values of the data and only uses + or − signs. Therefore, it is useful when it is difficult to measure the values.
Wilcoxon's signed rank test
There is a major limitation of sign test as we lose the quantitative information of the given data and merely use the + or – signs. Wilcoxon's signed rank test not only examines the observed values in comparison with θ0 but also takes into consideration the relative sizes, adding more statistical power to the test. As in the sign test, if there is an observed value that is equal to the reference value θ0, this observed value is eliminated from the sample.
Wilcoxon's rank sum test ranks all data points in order, calculates the rank sum of each sample and compares the difference in the rank sums.
Mann-Whitney test
It is used to test the null hypothesis that two samples have the same median or, alternatively, whether observations in one sample tend to be larger than observations in the other.
Mann–Whitney test compares all data (xi) belonging to the X group and all data (yi) belonging to the Y group and calculates the probability of xi being greater than yi: P (xi > yi). The null hypothesis states that P (xi > yi) = P (xi < yi) =1/2 while the alternative hypothesis states that P (xi > yi) ≠1/2.
Kolmogorov-Smirnov test
The two-sample Kolmogorov-Smirnov (KS) test was designed as a generic method to test whether two random samples are drawn from the same distribution. The null hypothesis of the KS test is that both distributions are identical. The statistic of the KS test is a distance between the two empirical distributions, computed as the maximum absolute difference between their cumulative curves.
Kruskal-Wallis test
The Kruskal–Wallis test is a non-parametric test to analyse the variance.[ 14 ] It analyses if there is any difference in the median values of three or more independent samples. The data values are ranked in an increasing order, and the rank sums calculated followed by calculation of the test statistic.
Jonckheere test
In contrast to Kruskal–Wallis test, in Jonckheere test, there is an a priori ordering that gives it a more statistical power than the Kruskal–Wallis test.[ 14 ]
Friedman test
The Friedman test is a non-parametric test for testing the difference between several related samples. The Friedman test is an alternative for repeated measures ANOVAs which is used when the same parameter has been measured under different conditions on the same subjects.[ 13 ]
Tests to analyse the categorical data
Chi-square test, Fischer's exact test and McNemar's test are used to analyse the categorical or nominal variables. The Chi-square test compares the frequencies and tests whether the observed data differ significantly from that of the expected data if there were no differences between groups (i.e., the null hypothesis). It is calculated by the sum of the squared difference between observed ( O ) and the expected ( E ) data (or the deviation, d ) divided by the expected data by the following formula:

A Yates correction factor is used when the sample size is small. Fischer's exact test is used to determine if there are non-random associations between two categorical variables. It does not assume random sampling, and instead of referring a calculated statistic to a sampling distribution, it calculates an exact probability. McNemar's test is used for paired nominal data. It is applied to 2 × 2 table with paired-dependent samples. It is used to determine whether the row and column frequencies are equal (that is, whether there is ‘marginal homogeneity’). The null hypothesis is that the paired proportions are equal. The Mantel-Haenszel Chi-square test is a multivariate test as it analyses multiple grouping variables. It stratifies according to the nominated confounding variables and identifies any that affects the primary outcome variable. If the outcome variable is dichotomous, then logistic regression is used.
SOFTWARES AVAILABLE FOR STATISTICS, SAMPLE SIZE CALCULATION AND POWER ANALYSIS
Numerous statistical software systems are available currently. The commonly used software systems are Statistical Package for the Social Sciences (SPSS – manufactured by IBM corporation), Statistical Analysis System ((SAS – developed by SAS Institute North Carolina, United States of America), R (designed by Ross Ihaka and Robert Gentleman from R core team), Minitab (developed by Minitab Inc), Stata (developed by StataCorp) and the MS Excel (developed by Microsoft).
There are a number of web resources which are related to statistical power analyses. A few are:
- StatPages.net – provides links to a number of online power calculators
- G-Power – provides a downloadable power analysis program that runs under DOS
- Power analysis for ANOVA designs an interactive site that calculates power or sample size needed to attain a given power for one effect in a factorial ANOVA design
- SPSS makes a program called SamplePower. It gives an output of a complete report on the computer screen which can be cut and paste into another document.
It is important that a researcher knows the concepts of the basic statistical methods used for conduct of a research study. This will help to conduct an appropriately well-designed study leading to valid and reliable results. Inappropriate use of statistical techniques may lead to faulty conclusions, inducing errors and undermining the significance of the article. Bad statistics may lead to bad research, and bad research may lead to unethical practice. Hence, an adequate knowledge of statistics and the appropriate use of statistical tests are important. An appropriate knowledge about the basic statistical methods will go a long way in improving the research designs and producing quality medical research which can be utilised for formulating the evidence-based guidelines.
Financial support and sponsorship
Conflicts of interest.
There are no conflicts of interest.
Purdue Online Writing Lab Purdue OWL® College of Liberal Arts
Writing with Descriptive Statistics
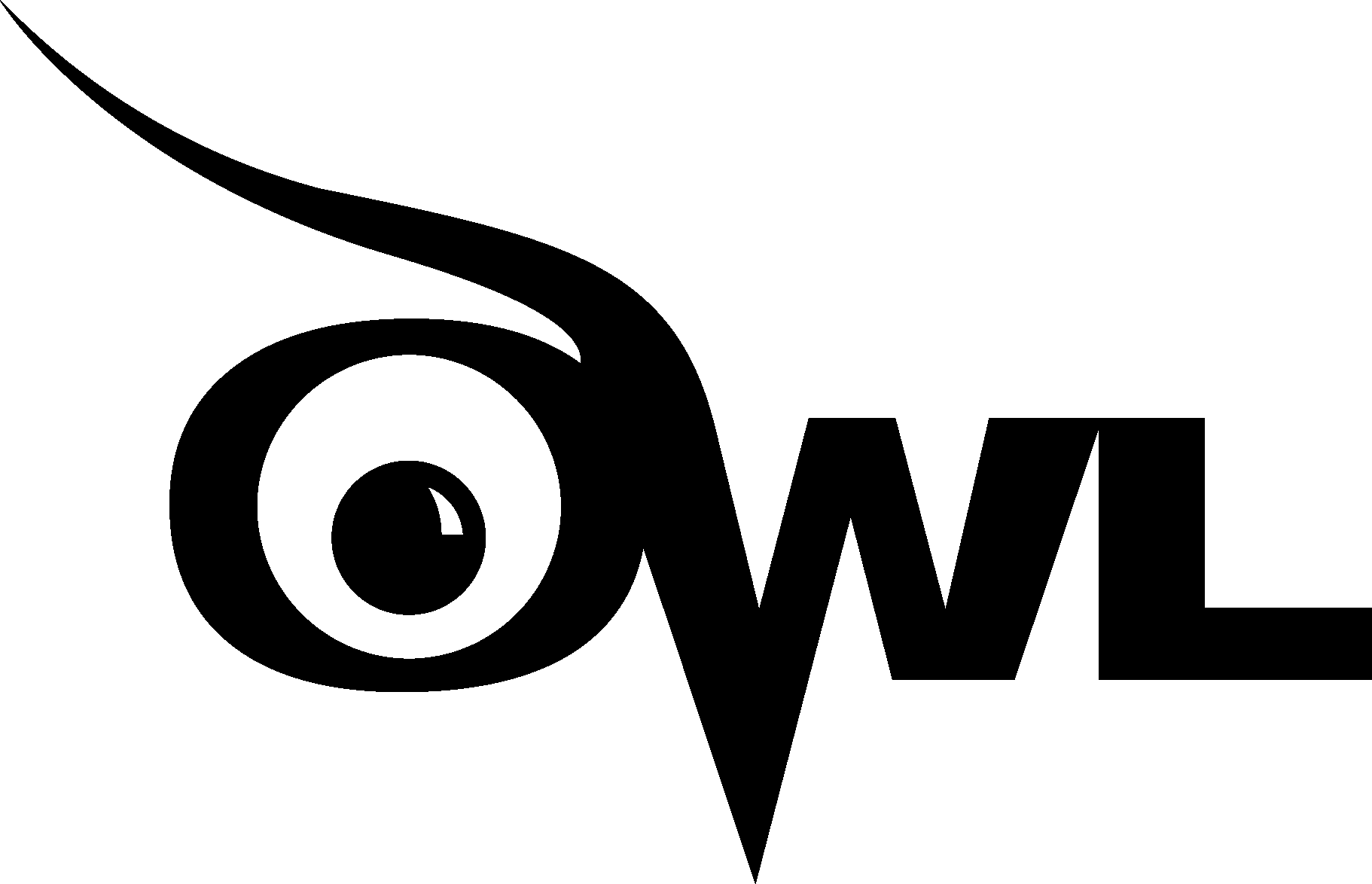
Welcome to the Purdue OWL
This page is brought to you by the OWL at Purdue University. When printing this page, you must include the entire legal notice.
Copyright ©1995-2018 by The Writing Lab & The OWL at Purdue and Purdue University. All rights reserved. This material may not be published, reproduced, broadcast, rewritten, or redistributed without permission. Use of this site constitutes acceptance of our terms and conditions of fair use.
This handout explains how to write with statistics including quick tips, writing descriptive statistics, writing inferential statistics, and using visuals with statistics.
Usually there is no good way to write a statistic. It rarely sounds good, and often interrupts the structure or flow of your writing. Oftentimes the best way to write descriptive statistics is to be direct. If you are citing several statistics about the same topic, it may be best to include them all in the same paragraph or section.
The mean of exam two is 77.7. The median is 75, and the mode is 79. Exam two had a standard deviation of 11.6.
Overall the company had another excellent year. We shipped 14.3 tons of fertilizer for the year, and averaged 1.7 tons of fertilizer during the summer months. This is an increase over last year, where we shipped only 13.1 tons of fertilizer, and averaged only 1.4 tons during the summer months. (Standard deviations were as followed: this summer .3 tons, last summer .4 tons).
Some fields prefer to put means and standard deviations in parentheses like this:
If you have lots of statistics to report, you should strongly consider presenting them in tables or some other visual form. You would then highlight statistics of interest in your text, but would not report all of the statistics. See the section on statistics and visuals for more details.
If you have a data set that you are using (such as all the scores from an exam) it would be unusual to include all of the scores in a paper or article. One of the reasons to use statistics is to condense large amounts of information into more manageable chunks; presenting your entire data set defeats this purpose.
At the bare minimum, if you are presenting statistics on a data set, it should include the mean and probably the standard deviation. This is the minimum information needed to get an idea of what the distribution of your data set might look like. How much additional information you include is entirely up to you. In general, don't include information if it is irrelevant to your argument or purpose. If you include statistics that many of your readers would not understand, consider adding the statistics in a footnote or appendix that explains it in more detail.
Essay on Statistics: Meaning and Definition of Statistics
“Statistics”, that a word is often used, has been derived from the Latin word ‘Status’ that means a group of numbers or figures; those represent some information of our human interest.
We find statistics in everyday life, such as in books or other information papers or TV or newspapers.
Although, in the beginning it was used by Kings only for collecting information about states and other information which was needed about their people, their number, revenue of the state etc.
This was known as the science of the state because it was used only by the Kings. So it got its development as ‘Kings’ subject or ‘Science of Kings’ or we may call it as “Political Arithmetic’s”. It was for the first time, perhaps in Egypt to conduct census of population in 3050 B.C. because the king needed money to erect pyramids. But in India, it is thought, that, it started dating back to Chandra Gupta Maurya’s kingdom under Chankya to collect the data of births and deaths. TM has also been stated in Chankya’s Arthshastra.
ADVERTISEMENTS:
But now-a-days due to its pervading nature, its scope has increased and widened. It is now used in almost in all the fields of human knowledge and skills like Business, Commerce, Economics, Social Sciences, Politics, Planning, Medicine and other sciences, Physical as well as Natural.
Definition :
The term ‘Statistics’ has been defined in two senses, i.e. in Singular and in Plural sense.
“Statistics has two meanings, as in plural sense and in singular sense”.
—Oxford Dictionary
In plural sense, it means a systematic collection of numerical facts and in singular sense; it is the science of collecting, classifying and using statistics.
A. In the Plural Sense :
“Statistics are numerical statements of facts in any department of enquiry placed in relation to each other.” —A.L. Bowley
“The classified facts respecting the condition of the people in a state—especially those facts which can be stated in numbers or in tables of numbers or in any tabular or classified arrangement.” —Webster
These definitions given above give a narrow meaning to the statistics as they do not indicate its various aspects as are witnessed in its practical applications. From the this point of view the definition given by Prof. Horace Sacrist appears to be the most comprehensive and meaningful:
“By statistics we mean aggregates of facts affected to a marked extent by multiplicity of causes, numerically expressed, enumerated or estimated according to reasonable standard of accuracy, collected in a systematic manner for a predetermined purpose, and placed in relation to each other.”—Horace Sacrist
B. In the Singular Sense :
“Statistics refers to the body of technique or methodology, which has been developed for the collection, presentation and analysis of quantitative data and for the use of such data in decision making.” —Ncttor and Washerman
“Statistics may rightly be called the science of averages.” —Bowleg
“Statistics may be defined as the collection, presentation, analysis, and interpretation of numerical data.” —Croxton and Cowden
Stages of Investigations :
1. Collection of Data:
It is the first stage of investigation and is regarding collection of data. It is determined that which method of collection is needed in this problem and then data are collected.
2. Organisation of Data:
It is second stage. The data are simplified and made comparative and are classified according to time and place.
3. Presentation of Data:
In this third stage, organised data are made simple and attractive. These are presented in the form of tables diagrams and graphs.
4. Analysis of Data:
Forth stage of investigation is analysis. To get correct results, analysis is necessary. It is often undertaken using Measures of central tendencies, Measures of dispersion, correlation, regression and interpolation etc.
5. Interpretation of Data:
In this last stage, conclusions are enacted. Use of comparisons is made. On this basis, forecasting is made.
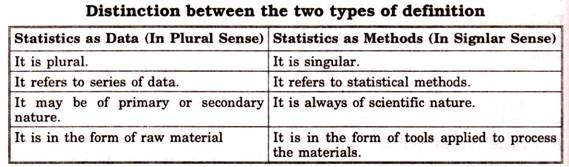
Some Modern Definitions :
From the above two senses of statistics, modem definitions have emerged as given below:
“Statistics is a body of methods for making wise decisions on the face of uncertainty.” —Wallis and Roberts
“Statistics is a body of methods for obtaining and analyzing numerical data in order to make better decisions in an uncertain world.” —Edward N. Dubois
So, from above definitions we find that science of statistics also includes the methods of collecting, organising, presenting, analysing and interpreting numerical facts and decisions are taken on their basis.
The most proper definition of statistics can be given as following after analysing the various definitions of statistics.
“Statistics in the plural sense are numerical statements of facts capable of some meaningful analysis and interpretation, and in singular sense, it relates to the collection, classification, presentation and interpretation of numerical data.”
Related Articles:
- 7 Main Characteristics of Statistics – Explained!
- Use of Statistics in Economics: Origin, Meaning and Other Details
- Nature and Subject Matter of Statistics
- Relation of Statistics with other Sciences
Home — Essay Samples — Science — Statistics — The Importance of Statistics in Daily Life

The Importance of Statistics in Daily Life
- Categories: Mathematics in Everyday Life Statistics
About this sample

Words: 468 |
Published: Jan 29, 2024
Words: 468 | Page: 1 | 3 min read
Table of contents
Utilizing statistics for decision-making, understanding trends and patterns, evaluating claims and evidence, limitations and challenges in statistics.
- Agresti, A. (2015). Foundations of linear and generalized linear models. John Wiley & Sons.
- Coleman, J. S. (1990). Foundations of Social Theory. Cambridge, MA: Belknap Press of Harvard University.
- Kabacoff, R. (2015). The R graphics cookbook. O'Reilly Media, Inc.
- Mendenhall, W., Beaver, R. J., & Beaver, B. M. (2016). Introduction to probability and statistics. Cengage Learning.
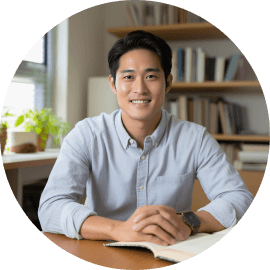
Cite this Essay
Let us write you an essay from scratch
- 450+ experts on 30 subjects ready to help
- Custom essay delivered in as few as 3 hours
Get high-quality help
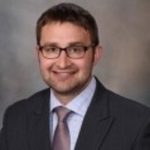
Dr. Heisenberg
Verified writer
- Expert in: Science
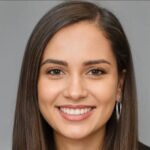
+ 120 experts online
By clicking “Check Writers’ Offers”, you agree to our terms of service and privacy policy . We’ll occasionally send you promo and account related email
No need to pay just yet!
Related Essays
1 pages / 649 words
1 pages / 423 words
1 pages / 513 words
1 pages / 424 words
Remember! This is just a sample.
You can get your custom paper by one of our expert writers.
121 writers online
Still can’t find what you need?
Browse our vast selection of original essay samples, each expertly formatted and styled
Related Essays on Statistics
Statistics play a crucial role in health care research, data collection and analysis, decision making, and policy development. This essay aims to examine the application of statistics in various areas of health care and [...]
Siegel, L. (2015). Criminology: Theories, Patterns, and Typologies (12th ed.). Boston, MA: Cengage Learning.Vulnerable Youth and the Transition to Adulthood. (2009). Retrieved from
Sir David Cox, whose full name Sir David Roxbee Cox, was born on July 15, 1924, in Birmingham Warwickshire England. In 1947, Cox married Joyce Drummond. Their marriage produced four children and two grandchildren. Sir David is [...]
The main objective of the article is to orient the possibilities of using statistical methods both in education and in research in the areas of Social Work. Social Work and related research, such as Psychiatric Social Work, [...]
For this project, Grade 10 Math Project, we have to pick something from real life that I can explain how math affects it. I chose to do my project about the Pharmaceutical industry. The pharmaceutical mathematics curriculum [...]
This paper will be a summary of my findings in answering the questions, “how large can a set with zero ‘length’ be?”. Throughout this paper I will be explaining facts regarding the Cantor set. The Cantor set is the best example [...]
Related Topics
By clicking “Send”, you agree to our Terms of service and Privacy statement . We will occasionally send you account related emails.
Where do you want us to send this sample?
By clicking “Continue”, you agree to our terms of service and privacy policy.
Be careful. This essay is not unique
This essay was donated by a student and is likely to have been used and submitted before
Download this Sample
Free samples may contain mistakes and not unique parts
Sorry, we could not paraphrase this essay. Our professional writers can rewrite it and get you a unique paper.
Please check your inbox.
We can write you a custom essay that will follow your exact instructions and meet the deadlines. Let's fix your grades together!
Get Your Personalized Essay in 3 Hours or Less!
We use cookies to personalyze your web-site experience. By continuing we’ll assume you board with our cookie policy .
- Instructions Followed To The Letter
- Deadlines Met At Every Stage
- Unique And Plagiarism Free
Have a thesis expert improve your writing
Check your thesis for plagiarism in 10 minutes, generate your apa citations for free.
- Knowledge Base
The Beginner's Guide to Statistical Analysis | 5 Steps & Examples
Statistical analysis means investigating trends, patterns, and relationships using quantitative data . It is an important research tool used by scientists, governments, businesses, and other organisations.
To draw valid conclusions, statistical analysis requires careful planning from the very start of the research process . You need to specify your hypotheses and make decisions about your research design, sample size, and sampling procedure.
After collecting data from your sample, you can organise and summarise the data using descriptive statistics . Then, you can use inferential statistics to formally test hypotheses and make estimates about the population. Finally, you can interpret and generalise your findings.
This article is a practical introduction to statistical analysis for students and researchers. We’ll walk you through the steps using two research examples. The first investigates a potential cause-and-effect relationship, while the second investigates a potential correlation between variables.
Table of contents
Step 1: write your hypotheses and plan your research design, step 2: collect data from a sample, step 3: summarise your data with descriptive statistics, step 4: test hypotheses or make estimates with inferential statistics, step 5: interpret your results, frequently asked questions about statistics.
To collect valid data for statistical analysis, you first need to specify your hypotheses and plan out your research design.
Writing statistical hypotheses
The goal of research is often to investigate a relationship between variables within a population . You start with a prediction, and use statistical analysis to test that prediction.
A statistical hypothesis is a formal way of writing a prediction about a population. Every research prediction is rephrased into null and alternative hypotheses that can be tested using sample data.
While the null hypothesis always predicts no effect or no relationship between variables, the alternative hypothesis states your research prediction of an effect or relationship.
- Null hypothesis: A 5-minute meditation exercise will have no effect on math test scores in teenagers.
- Alternative hypothesis: A 5-minute meditation exercise will improve math test scores in teenagers.
- Null hypothesis: Parental income and GPA have no relationship with each other in college students.
- Alternative hypothesis: Parental income and GPA are positively correlated in college students.
Planning your research design
A research design is your overall strategy for data collection and analysis. It determines the statistical tests you can use to test your hypothesis later on.
First, decide whether your research will use a descriptive, correlational, or experimental design. Experiments directly influence variables, whereas descriptive and correlational studies only measure variables.
- In an experimental design , you can assess a cause-and-effect relationship (e.g., the effect of meditation on test scores) using statistical tests of comparison or regression.
- In a correlational design , you can explore relationships between variables (e.g., parental income and GPA) without any assumption of causality using correlation coefficients and significance tests.
- In a descriptive design , you can study the characteristics of a population or phenomenon (e.g., the prevalence of anxiety in U.S. college students) using statistical tests to draw inferences from sample data.
Your research design also concerns whether you’ll compare participants at the group level or individual level, or both.
- In a between-subjects design , you compare the group-level outcomes of participants who have been exposed to different treatments (e.g., those who performed a meditation exercise vs those who didn’t).
- In a within-subjects design , you compare repeated measures from participants who have participated in all treatments of a study (e.g., scores from before and after performing a meditation exercise).
- In a mixed (factorial) design , one variable is altered between subjects and another is altered within subjects (e.g., pretest and posttest scores from participants who either did or didn’t do a meditation exercise).
- Experimental
- Correlational
First, you’ll take baseline test scores from participants. Then, your participants will undergo a 5-minute meditation exercise. Finally, you’ll record participants’ scores from a second math test.
In this experiment, the independent variable is the 5-minute meditation exercise, and the dependent variable is the math test score from before and after the intervention. Example: Correlational research design In a correlational study, you test whether there is a relationship between parental income and GPA in graduating college students. To collect your data, you will ask participants to fill in a survey and self-report their parents’ incomes and their own GPA.
Measuring variables
When planning a research design, you should operationalise your variables and decide exactly how you will measure them.
For statistical analysis, it’s important to consider the level of measurement of your variables, which tells you what kind of data they contain:
- Categorical data represents groupings. These may be nominal (e.g., gender) or ordinal (e.g. level of language ability).
- Quantitative data represents amounts. These may be on an interval scale (e.g. test score) or a ratio scale (e.g. age).
Many variables can be measured at different levels of precision. For example, age data can be quantitative (8 years old) or categorical (young). If a variable is coded numerically (e.g., level of agreement from 1–5), it doesn’t automatically mean that it’s quantitative instead of categorical.
Identifying the measurement level is important for choosing appropriate statistics and hypothesis tests. For example, you can calculate a mean score with quantitative data, but not with categorical data.
In a research study, along with measures of your variables of interest, you’ll often collect data on relevant participant characteristics.
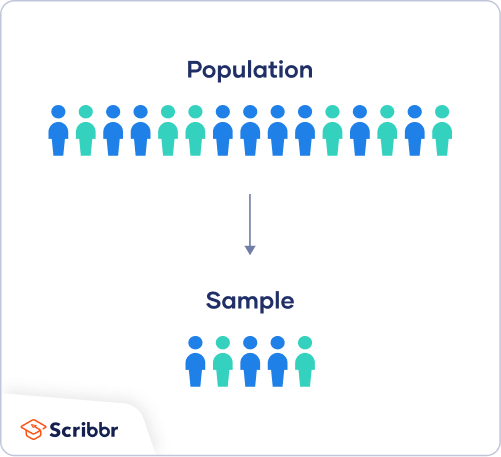
In most cases, it’s too difficult or expensive to collect data from every member of the population you’re interested in studying. Instead, you’ll collect data from a sample.
Statistical analysis allows you to apply your findings beyond your own sample as long as you use appropriate sampling procedures . You should aim for a sample that is representative of the population.
Sampling for statistical analysis
There are two main approaches to selecting a sample.
- Probability sampling: every member of the population has a chance of being selected for the study through random selection.
- Non-probability sampling: some members of the population are more likely than others to be selected for the study because of criteria such as convenience or voluntary self-selection.
In theory, for highly generalisable findings, you should use a probability sampling method. Random selection reduces sampling bias and ensures that data from your sample is actually typical of the population. Parametric tests can be used to make strong statistical inferences when data are collected using probability sampling.
But in practice, it’s rarely possible to gather the ideal sample. While non-probability samples are more likely to be biased, they are much easier to recruit and collect data from. Non-parametric tests are more appropriate for non-probability samples, but they result in weaker inferences about the population.
If you want to use parametric tests for non-probability samples, you have to make the case that:
- your sample is representative of the population you’re generalising your findings to.
- your sample lacks systematic bias.
Keep in mind that external validity means that you can only generalise your conclusions to others who share the characteristics of your sample. For instance, results from Western, Educated, Industrialised, Rich and Democratic samples (e.g., college students in the US) aren’t automatically applicable to all non-WEIRD populations.
If you apply parametric tests to data from non-probability samples, be sure to elaborate on the limitations of how far your results can be generalised in your discussion section .
Create an appropriate sampling procedure
Based on the resources available for your research, decide on how you’ll recruit participants.
- Will you have resources to advertise your study widely, including outside of your university setting?
- Will you have the means to recruit a diverse sample that represents a broad population?
- Do you have time to contact and follow up with members of hard-to-reach groups?
Your participants are self-selected by their schools. Although you’re using a non-probability sample, you aim for a diverse and representative sample. Example: Sampling (correlational study) Your main population of interest is male college students in the US. Using social media advertising, you recruit senior-year male college students from a smaller subpopulation: seven universities in the Boston area.
Calculate sufficient sample size
Before recruiting participants, decide on your sample size either by looking at other studies in your field or using statistics. A sample that’s too small may be unrepresentative of the sample, while a sample that’s too large will be more costly than necessary.
There are many sample size calculators online. Different formulas are used depending on whether you have subgroups or how rigorous your study should be (e.g., in clinical research). As a rule of thumb, a minimum of 30 units or more per subgroup is necessary.
To use these calculators, you have to understand and input these key components:
- Significance level (alpha): the risk of rejecting a true null hypothesis that you are willing to take, usually set at 5%.
- Statistical power : the probability of your study detecting an effect of a certain size if there is one, usually 80% or higher.
- Expected effect size : a standardised indication of how large the expected result of your study will be, usually based on other similar studies.
- Population standard deviation: an estimate of the population parameter based on a previous study or a pilot study of your own.
Once you’ve collected all of your data, you can inspect them and calculate descriptive statistics that summarise them.
Inspect your data
There are various ways to inspect your data, including the following:
- Organising data from each variable in frequency distribution tables .
- Displaying data from a key variable in a bar chart to view the distribution of responses.
- Visualising the relationship between two variables using a scatter plot .
By visualising your data in tables and graphs, you can assess whether your data follow a skewed or normal distribution and whether there are any outliers or missing data.
A normal distribution means that your data are symmetrically distributed around a center where most values lie, with the values tapering off at the tail ends.
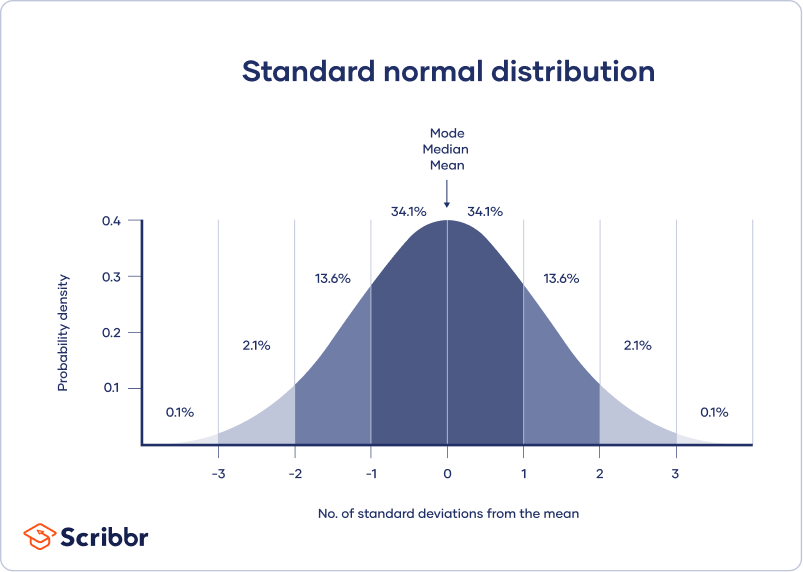
In contrast, a skewed distribution is asymmetric and has more values on one end than the other. The shape of the distribution is important to keep in mind because only some descriptive statistics should be used with skewed distributions.
Extreme outliers can also produce misleading statistics, so you may need a systematic approach to dealing with these values.
Calculate measures of central tendency
Measures of central tendency describe where most of the values in a data set lie. Three main measures of central tendency are often reported:
- Mode : the most popular response or value in the data set.
- Median : the value in the exact middle of the data set when ordered from low to high.
- Mean : the sum of all values divided by the number of values.
However, depending on the shape of the distribution and level of measurement, only one or two of these measures may be appropriate. For example, many demographic characteristics can only be described using the mode or proportions, while a variable like reaction time may not have a mode at all.
Calculate measures of variability
Measures of variability tell you how spread out the values in a data set are. Four main measures of variability are often reported:
- Range : the highest value minus the lowest value of the data set.
- Interquartile range : the range of the middle half of the data set.
- Standard deviation : the average distance between each value in your data set and the mean.
- Variance : the square of the standard deviation.
Once again, the shape of the distribution and level of measurement should guide your choice of variability statistics. The interquartile range is the best measure for skewed distributions, while standard deviation and variance provide the best information for normal distributions.
Using your table, you should check whether the units of the descriptive statistics are comparable for pretest and posttest scores. For example, are the variance levels similar across the groups? Are there any extreme values? If there are, you may need to identify and remove extreme outliers in your data set or transform your data before performing a statistical test.
From this table, we can see that the mean score increased after the meditation exercise, and the variances of the two scores are comparable. Next, we can perform a statistical test to find out if this improvement in test scores is statistically significant in the population. Example: Descriptive statistics (correlational study) After collecting data from 653 students, you tabulate descriptive statistics for annual parental income and GPA.
It’s important to check whether you have a broad range of data points. If you don’t, your data may be skewed towards some groups more than others (e.g., high academic achievers), and only limited inferences can be made about a relationship.
A number that describes a sample is called a statistic , while a number describing a population is called a parameter . Using inferential statistics , you can make conclusions about population parameters based on sample statistics.
Researchers often use two main methods (simultaneously) to make inferences in statistics.
- Estimation: calculating population parameters based on sample statistics.
- Hypothesis testing: a formal process for testing research predictions about the population using samples.
You can make two types of estimates of population parameters from sample statistics:
- A point estimate : a value that represents your best guess of the exact parameter.
- An interval estimate : a range of values that represent your best guess of where the parameter lies.
If your aim is to infer and report population characteristics from sample data, it’s best to use both point and interval estimates in your paper.
You can consider a sample statistic a point estimate for the population parameter when you have a representative sample (e.g., in a wide public opinion poll, the proportion of a sample that supports the current government is taken as the population proportion of government supporters).
There’s always error involved in estimation, so you should also provide a confidence interval as an interval estimate to show the variability around a point estimate.
A confidence interval uses the standard error and the z score from the standard normal distribution to convey where you’d generally expect to find the population parameter most of the time.
Hypothesis testing
Using data from a sample, you can test hypotheses about relationships between variables in the population. Hypothesis testing starts with the assumption that the null hypothesis is true in the population, and you use statistical tests to assess whether the null hypothesis can be rejected or not.
Statistical tests determine where your sample data would lie on an expected distribution of sample data if the null hypothesis were true. These tests give two main outputs:
- A test statistic tells you how much your data differs from the null hypothesis of the test.
- A p value tells you the likelihood of obtaining your results if the null hypothesis is actually true in the population.
Statistical tests come in three main varieties:
- Comparison tests assess group differences in outcomes.
- Regression tests assess cause-and-effect relationships between variables.
- Correlation tests assess relationships between variables without assuming causation.
Your choice of statistical test depends on your research questions, research design, sampling method, and data characteristics.
Parametric tests
Parametric tests make powerful inferences about the population based on sample data. But to use them, some assumptions must be met, and only some types of variables can be used. If your data violate these assumptions, you can perform appropriate data transformations or use alternative non-parametric tests instead.
A regression models the extent to which changes in a predictor variable results in changes in outcome variable(s).
- A simple linear regression includes one predictor variable and one outcome variable.
- A multiple linear regression includes two or more predictor variables and one outcome variable.
Comparison tests usually compare the means of groups. These may be the means of different groups within a sample (e.g., a treatment and control group), the means of one sample group taken at different times (e.g., pretest and posttest scores), or a sample mean and a population mean.
- A t test is for exactly 1 or 2 groups when the sample is small (30 or less).
- A z test is for exactly 1 or 2 groups when the sample is large.
- An ANOVA is for 3 or more groups.
The z and t tests have subtypes based on the number and types of samples and the hypotheses:
- If you have only one sample that you want to compare to a population mean, use a one-sample test .
- If you have paired measurements (within-subjects design), use a dependent (paired) samples test .
- If you have completely separate measurements from two unmatched groups (between-subjects design), use an independent (unpaired) samples test .
- If you expect a difference between groups in a specific direction, use a one-tailed test .
- If you don’t have any expectations for the direction of a difference between groups, use a two-tailed test .
The only parametric correlation test is Pearson’s r . The correlation coefficient ( r ) tells you the strength of a linear relationship between two quantitative variables.
However, to test whether the correlation in the sample is strong enough to be important in the population, you also need to perform a significance test of the correlation coefficient, usually a t test, to obtain a p value. This test uses your sample size to calculate how much the correlation coefficient differs from zero in the population.
You use a dependent-samples, one-tailed t test to assess whether the meditation exercise significantly improved math test scores. The test gives you:
- a t value (test statistic) of 3.00
- a p value of 0.0028
Although Pearson’s r is a test statistic, it doesn’t tell you anything about how significant the correlation is in the population. You also need to test whether this sample correlation coefficient is large enough to demonstrate a correlation in the population.
A t test can also determine how significantly a correlation coefficient differs from zero based on sample size. Since you expect a positive correlation between parental income and GPA, you use a one-sample, one-tailed t test. The t test gives you:
- a t value of 3.08
- a p value of 0.001
The final step of statistical analysis is interpreting your results.
Statistical significance
In hypothesis testing, statistical significance is the main criterion for forming conclusions. You compare your p value to a set significance level (usually 0.05) to decide whether your results are statistically significant or non-significant.
Statistically significant results are considered unlikely to have arisen solely due to chance. There is only a very low chance of such a result occurring if the null hypothesis is true in the population.
This means that you believe the meditation intervention, rather than random factors, directly caused the increase in test scores. Example: Interpret your results (correlational study) You compare your p value of 0.001 to your significance threshold of 0.05. With a p value under this threshold, you can reject the null hypothesis. This indicates a statistically significant correlation between parental income and GPA in male college students.
Note that correlation doesn’t always mean causation, because there are often many underlying factors contributing to a complex variable like GPA. Even if one variable is related to another, this may be because of a third variable influencing both of them, or indirect links between the two variables.
Effect size
A statistically significant result doesn’t necessarily mean that there are important real life applications or clinical outcomes for a finding.
In contrast, the effect size indicates the practical significance of your results. It’s important to report effect sizes along with your inferential statistics for a complete picture of your results. You should also report interval estimates of effect sizes if you’re writing an APA style paper .
With a Cohen’s d of 0.72, there’s medium to high practical significance to your finding that the meditation exercise improved test scores. Example: Effect size (correlational study) To determine the effect size of the correlation coefficient, you compare your Pearson’s r value to Cohen’s effect size criteria.
Decision errors
Type I and Type II errors are mistakes made in research conclusions. A Type I error means rejecting the null hypothesis when it’s actually true, while a Type II error means failing to reject the null hypothesis when it’s false.
You can aim to minimise the risk of these errors by selecting an optimal significance level and ensuring high power . However, there’s a trade-off between the two errors, so a fine balance is necessary.
Frequentist versus Bayesian statistics
Traditionally, frequentist statistics emphasises null hypothesis significance testing and always starts with the assumption of a true null hypothesis.
However, Bayesian statistics has grown in popularity as an alternative approach in the last few decades. In this approach, you use previous research to continually update your hypotheses based on your expectations and observations.
Bayes factor compares the relative strength of evidence for the null versus the alternative hypothesis rather than making a conclusion about rejecting the null hypothesis or not.
Hypothesis testing is a formal procedure for investigating our ideas about the world using statistics. It is used by scientists to test specific predictions, called hypotheses , by calculating how likely it is that a pattern or relationship between variables could have arisen by chance.
The research methods you use depend on the type of data you need to answer your research question .
- If you want to measure something or test a hypothesis , use quantitative methods . If you want to explore ideas, thoughts, and meanings, use qualitative methods .
- If you want to analyse a large amount of readily available data, use secondary data. If you want data specific to your purposes with control over how they are generated, collect primary data.
- If you want to establish cause-and-effect relationships between variables , use experimental methods. If you want to understand the characteristics of a research subject, use descriptive methods.
Statistical analysis is the main method for analyzing quantitative research data . It uses probabilities and models to test predictions about a population from sample data.
Is this article helpful?
Other students also liked, a quick guide to experimental design | 5 steps & examples, controlled experiments | methods & examples of control, between-subjects design | examples, pros & cons, more interesting articles.
- Central Limit Theorem | Formula, Definition & Examples
- Central Tendency | Understanding the Mean, Median & Mode
- Correlation Coefficient | Types, Formulas & Examples
- Descriptive Statistics | Definitions, Types, Examples
- How to Calculate Standard Deviation (Guide) | Calculator & Examples
- How to Calculate Variance | Calculator, Analysis & Examples
- How to Find Degrees of Freedom | Definition & Formula
- How to Find Interquartile Range (IQR) | Calculator & Examples
- How to Find Outliers | Meaning, Formula & Examples
- How to Find the Geometric Mean | Calculator & Formula
- How to Find the Mean | Definition, Examples & Calculator
- How to Find the Median | Definition, Examples & Calculator
- How to Find the Range of a Data Set | Calculator & Formula
- Inferential Statistics | An Easy Introduction & Examples
- Levels of measurement: Nominal, ordinal, interval, ratio
- Missing Data | Types, Explanation, & Imputation
- Normal Distribution | Examples, Formulas, & Uses
- Null and Alternative Hypotheses | Definitions & Examples
- Poisson Distributions | Definition, Formula & Examples
- Skewness | Definition, Examples & Formula
- T-Distribution | What It Is and How To Use It (With Examples)
- The Standard Normal Distribution | Calculator, Examples & Uses
- Type I & Type II Errors | Differences, Examples, Visualizations
- Understanding Confidence Intervals | Easy Examples & Formulas
- Variability | Calculating Range, IQR, Variance, Standard Deviation
- What is Effect Size and Why Does It Matter? (Examples)
- What Is Interval Data? | Examples & Definition
- What Is Nominal Data? | Examples & Definition
- What Is Ordinal Data? | Examples & Definition
- What Is Ratio Data? | Examples & Definition
- What Is the Mode in Statistics? | Definition, Examples & Calculator

An official website of the United States government
Gross Domestic Product by State and Personal Income by State, 4th Quarter 2023 and Preliminary 2023
- News Release
- Related Materials
- Additional Information
Real gross domestic product (GDP) increased in all 50 states and the District of Columbia in the fourth quarter of 2023, with the percent change ranging from 6.7 percent in Nevada to 0.2 percent in Nebraska (table 1), according to statistics released today by the U.S. Bureau of Economic Analysis (BEA). Current-dollar GDP increased in 49 states and the District of Columbia. For the year 2023 , real, or inflation-adjusted, GDP also increased in 49 states and the District of Columbia.
Personal income , in current dollars, increased in all 50 states and the District of Columbia in the fourth quarter of 2023, with the percent change ranging from 6.7 percent in Nevada to 0.8 percent in Iowa and North Dakota (table 4). For the year 2023 , current-dollar personal income also increased in all 50 states and the District of Columbia.
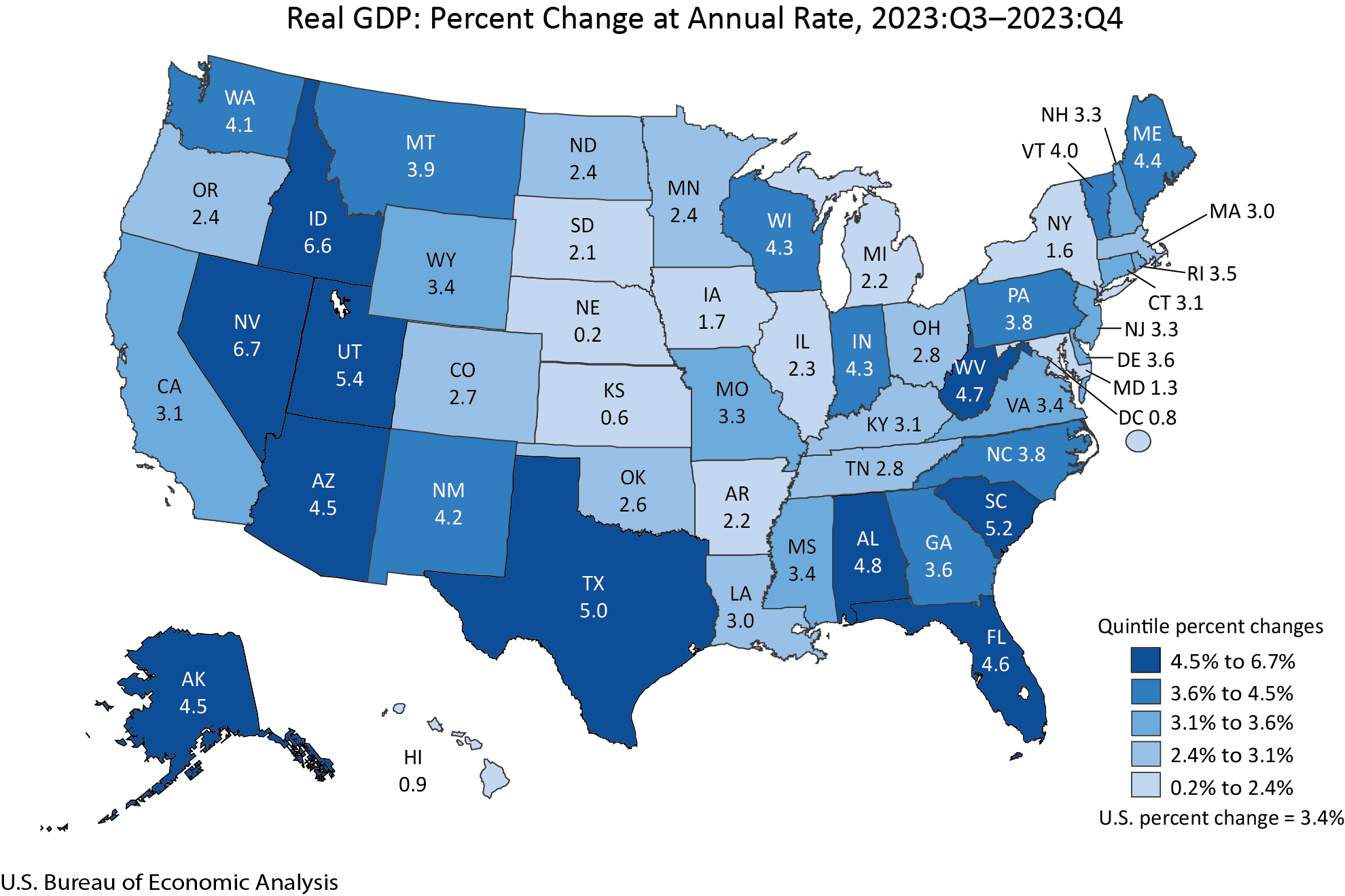
Quarterly GDP
In the fourth quarter of 2023, real GDP for the nation grew at an annual rate of 3.4 percent. Real GDP increased in 18 of the 23 industry groups for which BEA prepares quarterly state estimates (table 2). Nondurable-goods manufacturing, retail trade, and durable-goods manufacturing were the leading contributors to growth in real GDP nationally.
- Construction, which increased in 45 states and the District of Columbia, was the leading contributor to growth in 3 states including Nevada, the state with the largest increase in real GDP.
- Agriculture, forestry, fishing, and hunting, which increased nationally and in 32 states, was the leading contributor to growth in Idaho, the state with the second-largest increase in real GDP. In contrast, this industry was the leading offset to growth in Nebraska and Kansas, the states with the smallest increases in real GDP.
- Retail trade, which increased in all 50 states and the District of Columbia, was the leading contributor to growth in 14 states including Utah, the state with the third-largest increase in real GDP.
In 2023, real GDP for the nation grew at an annual rate of 2.5 percent, with the percent change ranging from 5.9 percent in North Dakota to –1.2 percent in Delaware. Real GDP increased in 17 of the 23 industry groups for which BEA prepares preliminary annual state estimates (table 3). Retail trade; professional, scientific, and technical services; and health care and social assistance were the leading contributors to growth in real GDP nationally.
- Mining increased in 43 states. This industry was the leading contributor to growth in seven states including North Dakota, Texas, Wyoming, Alaska, and Oklahoma, the states with the first-, second-, third-, fourth-, and fifth-largest increases in real GDP, respectively.
- Retail trade increased in all 50 states and the District of Columbia. This industry was the leading contributor to growth in 23 states including Florida, the state with the seventh-largest increase in real GDP.
- Health care and social assistance increased in 49 states and the District of Columbia. This industry was the leading contributor to growth in 6 states.
- Finance and insurance decreased in 43 states and the District of Columbia. The industry was the leading contributor to the decline in Delaware.
Quarterly personal income
In the fourth quarter of 2023, current-dollar personal income increased $229.4 billion, or 4.0 percent at an annual rate (table 4). Increases in earnings and property income (dividends, interest, and rent) were partially offset by a decrease in transfer receipts (chart 1).
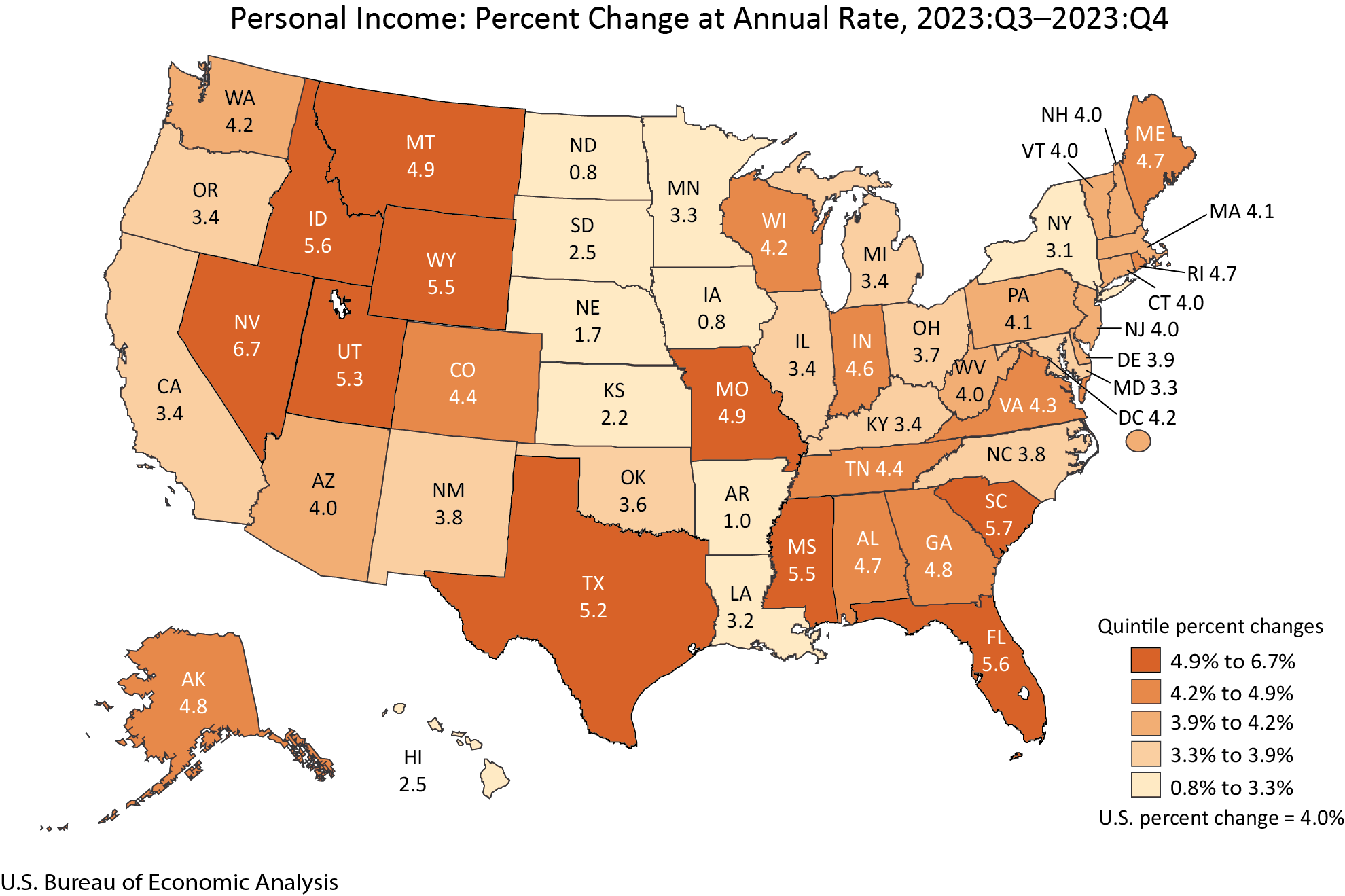
Earnings increased in 48 states and the District of Columbia, while growing 4.6 percent nationally (table 5). The percent change in earnings ranged from 8.5 percent in Nevada to –0.8 percent in North Dakota.
- Earnings increased in 20 of the 24 industries for which BEA prepares quarterly estimates (table 6).
- Construction earnings increased in 48 states and the District of Columbia. This industry was the leading contributor to growth in personal income in Nevada and Idaho, the states with the largest and third-largest increases in personal income, respectively.
- In South Carolina, the state with the second-largest increase in personal income, growth in earnings in the construction and professional, scientific, and technical services industries were the leading contributors to the increase in personal income.
- Decreases in farm earnings were the leading offsets to growth in Iowa and North Dakota, the states with the smallest increases in personal income.
Property income increased in all 50 states and the District of Columbia, while growing 6.7 percent nationally. The percent change ranged from 8.8 percent in Florida to 4.7 percent in Iowa and Mississippi (table 5).
Transfer receipts decreased in 32 states and the District of Columbia, while declining 0.7 percent nationally. The percent change in transfer receipts ranged from 8.1 percent in Mississippi to –5.0 percent in Arizona (table 5).
Annual personal income
In 2023, personal income for the nation increased at an annual rate of 5.2 percent, with the percent change ranging from 7.0 percent in Florida to 3.4 percent in Indiana.
Nationally, increases in earnings, property income, and transfer receipts contributed to the increase in personal income (chart 2).
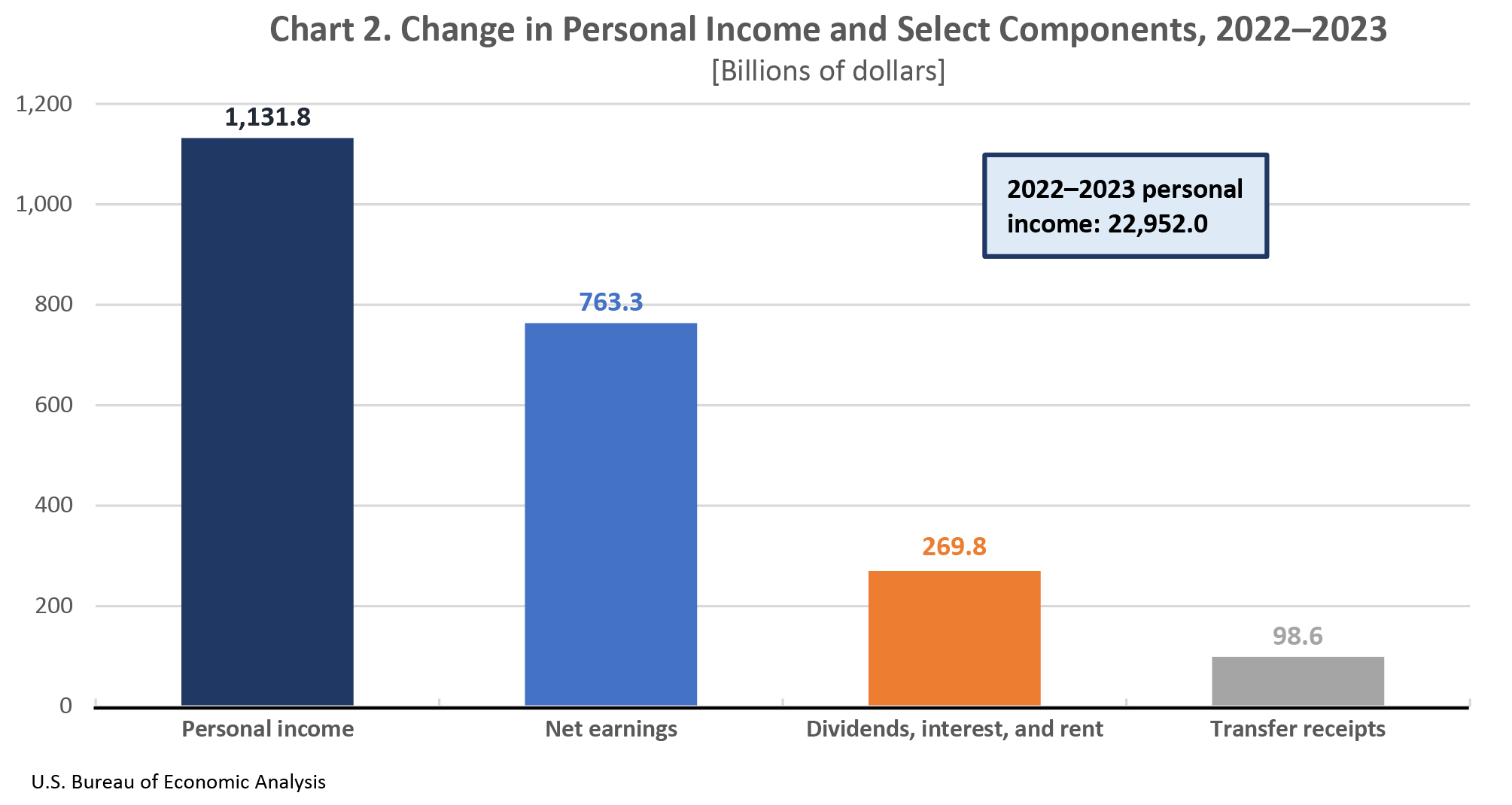
Earnings increased in all 50 states and the District of Columbia, while growing 5.6 percent nationally. The percent change in earnings ranged from 8.5 percent in Alaska to 4.0 percent in Mississippi (table 7).
- Earnings increased in 21 of the 24 industries for which BEA prepares annual estimates (table 8).
- In Florida, the state with the largest increase in personal income, growth in earnings in the professional, scientific, and technical services and in the health care and social assistance industries were the leading contributors to the increase in personal income.
- In Utah and Wyoming, the states with the second- and third-largest increases in personal income, growth in earnings in state and local government was the leading contributor to the increase in personal income.
Property income increased in all 50 states and the District of Columbia, while growing 6.3 percent nationally. The percent change ranged from 9.0 percent in Idaho to 2.7 percent in Iowa (table 7).
Transfer receipts increased in 45 states and the District of Columbia, while growing 2.5 percent nationally. The percent change in transfer receipts ranged from 7.3 percent in the District of Columbia to –8.9 percent in Alaska (table 7).
Update of state statistics
Today, BEA also released revised quarterly estimates of personal income by state for the first quarter of 2023 through the third quarter of 2023. This update incorporates new and revised source data that are more complete and more detailed than previously available and aligns the states with the national estimates from the National Income and Product Accounts released on March 28, 2024.
BEA also released new estimates of per capita personal income for the fourth quarter of 2023, along with revised estimates for the first quarter of 2020 through the third quarter of 2023. BEA used U.S. Census Bureau (Census) population figures to calculate per capita personal income estimates for the first quarter of 2020 through the fourth quarter of 2023. BEA also used new Census population figures to update annual 2020 to 2022 per capita personal income statistics and to produce new per capita personal income statistics for 2023. For earlier estimates, BEA continues to use intercensal population statistics that it developed based on Census methodology. See “ Note on Per Capita Personal Income and Population .”
* * *
Next release: June 28, 2024, at 10:00 a.m. EDT Gross Domestic Product by State and Personal Income by State, 1st Quarter 2024
Full Release & Tables (PDF)
Tables only (excel), quarterly highlights (pdf), annual highlights (pdf), interactive tables.
- Technical (GDP) Clifford Woodruff 301-278-9234 [email protected]
- Technical (Income) Matthew von Kerczek 301-278-9250 [email protected]
- Media (BEA) Connie O'Connell 301-278-9003 [email protected]
- Stay informed about BEA developments by reading The BEA Wire , signing up for BEA's email subscription service , or following @BEA_News on X, formerly known as Twitter.
- Historical time series for these estimates can be accessed in BEA's Interactive Data Application .
- Access BEA data by registering for BEA's Data Application Programming Interface .
- For more on BEA's statistics, see our online journal, the Survey of Current Business .
- For upcoming economic indicators, see BEA's news release schedule .
- BEA Regional Facts ( BEARFACTS ) is a narrative summary of personal income, per capita personal income, components of income, and gross domestic product for counties, metropolitan statistical areas, and states.
- For complete information on the sources and methods used to estimate gross domestic product and personal income by state, see BEA's gross domestic product by state and state personal income and employment methodologies.
Definitions
Gross domestic product (GDP) by state is the market value of goods and services produced by the labor and property located in a state. GDP by state is the state counterpart of the nation's GDP, the Bureau's featured and most comprehensive measure of U.S. economic activity.
Current-dollar statistics are valued in the prices of the period when the transactions occurred—that is, at “market value.” They are also referred to as “nominal GDP” or “current-price GDP.”
Real values are inflation-adjusted statistics—that is, these exclude the effects of price changes.
Contributions to growth are an industry’s contribution to the state’s overall percent change in real GDP. The contributions are additive and can be summed to the state’s overall percent change.
Personal income is the income received by, or on behalf of, all persons from all sources: from participation as laborers in production, from owning a home or business, from the ownership of financial assets, and from government and business in the form of transfers. It includes income from domestic sources as well as the rest of world. It does not include realized or unrealized capital gains or losses.
Personal income is measured before the deduction of personal income taxes and other personal taxes and is reported in current dollars (no adjustment is made for price changes).
State personal income differs slightly from the estimate of U.S. personal income in the National Income and Product Accounts because of differences in coverage, in the methodologies used to prepare the estimates, and in the timing of the availability of source data. In BEA’s state statistics, estimates of personal income for the United States is the sum of the state estimates and the estimate for the District of Columbia.
Per capita personal income is calculated as the total personal income of the residents of a state divided by the population of the state. In computing per capita personal income, BEA uses mid-quarter population estimates based on unpublished U.S. Census Bureau data.
Earnings by place of work is the sum of wages and salaries, supplements to wages and salaries, and proprietors’ income. BEA’s industry estimates are presented on an earnings by place-of-work basis.
Net earnings by place of residence is earnings by place of work less contributions for government social insurance plus an adjustment to convert earnings by place of work to a place-of-residence basis. BEA presents net earnings on an all-industry level.
Property income is rental income of persons, personal dividend income, and personal interest income.
Personal current transfer receipts are benefits received by persons from federal, state, and local governments and from businesses for which no current services are performed. They include retirement and disability insurance benefits (mainly social security), medical benefits (mainly Medicare and Medicaid), income maintenance benefits, unemployment insurance compensation, veterans’ benefits, and federal education and training assistance.
Statistical conventions
Quarter-to-quarter percent changes are calculated from unrounded data and are annualized. Annualized growth rates show the rate of change that would have occurred had the pattern been repeated over four quarters (1 year). Annualized rates of change can be calculated as follows: (((level of later quarter / level of earlier quarter)^4)-1)*100. Quarterly estimates are expressed at seasonally adjusted annual rates unless otherwise specified. Quarter-to-quarter dollar changes are differences between published estimates.
Seasonal adjustment and annual rates . Quarterly values are expressed at seasonally adjusted annual rates. For details, see the FAQ " Why does BEA publish estimates at annual rates? " on the BEA website.
Quantities and prices . Quantities, or “real” measures, are expressed as index numbers with a specified reference year equal to 100 (currently 2017). Quantity indexes are calculated using a Fisher chain-weighted formula that incorporates weights from two adjacent periods (quarters for quarterly data and annuals for annual data). “Real” dollar series are calculated by multiplying the quantity index by the current dollar value in the reference year and then dividing by 100. Percent changes calculated from chained-dollar levels and quantity indexes are conceptually the same; any differences are due to rounding.
Chained-dollar values are not additive, because the relative weights for a given period differ from those of the reference year.
Chained-dollar values of GDP by state are derived by applying national chain-type price indexes to the current dollar values of GDP by state for the 23 North American Industry Classification System-based industry sectors. The chain-type index formula that is used in the national accounts is then used to calculate the values of total real GDP by state and real GDP by state at more aggregated industry levels. Real GDP by state may reflect a substantial volume of output that is sold to other states and countries. To the extent that a state's output is produced and sold in national markets at relatively uniform prices (or sold locally at national prices), real GDP by state captures the differences across states that reflect the relative differences in the mix of goods and services that the states produce. However, real GDP by state does not capture geographic differences in the prices of goods and services that are produced and sold locally.
BEA regions
BEA groups all 50 states and the District of Columbia into 8 distinct regions for purposes of presentation and analysis as follows:
New England (Connecticut, Maine, Massachusetts, New Hampshire, Rhode Island, and Vermont) Mideast (Delaware, District of Columbia, Maryland, New Jersey, New York, and Pennsylvania) Great Lakes (Illinois, Indiana, Michigan, Ohio, and Wisconsin) Plains (Iowa, Kansas, Minnesota, Missouri, Nebraska, North Dakota, and South Dakota) Southeast (Alabama, Arkansas, Florida, Georgia, Kentucky, Louisiana, Mississippi, North Carolina, South Carolina, Tennessee, Virginia, and West Virginia) Southwest (Arizona, New Mexico, Oklahoma, and Texas) Rocky Mountain (Colorado, Idaho, Montana, Utah, and Wyoming) Far West (Alaska, California, Hawaii, Nevada, Oregon, and Washington)
Uses of GDP and personal income by state statistics
GDP and personal income by state statistics provide a framework for analyzing current economic conditions in each state and can serve as a basis for decision-making. For example:
- Federal government agencies use the statistics in forecasting models to project energy and water use. The statistics are also used as a basis for allocating funds and determining matching grants to states.
- State governments use the statistics to project tax revenues and the need for public services.
- Academic regional economists use the statistics for applied research.
- Businesses, trade associations, and labor organizations use the statistics for market research.

Today in Energy
- Recent articles
- liquid fuels
- natural gas
- electricity
- oil/petroleum
- production/supply
- consumption/demand
- exports/imports
- international
- forecasts/projections
- steo (short-term energy outlook)
M&A activity in 2023 furthers consolidation of U.S. crude oil and natural gas firms
In 2023, crude oil and natural gas exploration and production (E&P) companies increased spending on mergers and acquisitions (M&A) to $234 billion, the most in real 2023 dollars since 2012. Recent dealmaking marks a return to the previous trend of consolidation among oil companies in the United States after transactions declined amid significant oil market volatility in 2020 and 2022.
Spending on M&A includes both corporate M&A and asset acquisitions. Corporate M&A involves one company merging or acquiring another company. Asset acquisition is when one owner purchases an asset from another owner. Corporate M&A was 82% of total announced spending, due in large part to two in-progress deals: ExxonMobil’s announced acquisition of Pioneer Natural Resources for $64.5 billion and Chevron’s announced acquisition of Hess Corporation for $60 billion. These deals are the largest by value in real terms since Occidental Petroleum Corporation acquired Anadarko Petroleum Corporation for a total acquisition cost of $55 billion in 2019.
Corporate M&A and asset acquisitions, such as acreage sales, can be attractive for both buyers and sellers. Buyers can purchase proved reserves instead of using capital expenditures on exploration and development that might not generate profitable assets. Some companies may also want to diversify their portfolios or purchase acreage that geographically complements their existing portfolios, which can lead to lower costs and greater production efficiency. Sellers may see selling property or merging with companies as beneficial for shareholders or, in some cases, may seek out buyers as a way to emerge from bankruptcy proceedings and improve balance sheets.
One result of recent consolidation activity is larger companies that own more producing assets. Chevron is currently the largest crude oil and natural gas liquids (NGL) producer in the United States, accounting for 5% of the U.S. total with average production of just over 1.0 million barrels per day (b/d) in the third quarter of 2023 (3Q23). If Chevron successfully acquires Hess Corporation, it could increase Chevron’s share of U.S production to 6% (just over 1.2 million b/d), based on 3Q23 data.
Similarly, ExxonMobil , the fourth-largest crude oil and NGL producer in the United States, has the potential to increase its production to nearly 7% of total U.S. production (from about 750,000 b/d to 1.3 million b/d) if it successfully acquires Pioneer Natural Resources. Barring any other large production or asset ownership changes, recent M&A could make ExxonMobil the largest crude oil and NGL producer in the United States.
ExxonMobil’s goals for the acquisition of Pioneer Natural Resources include increasing the company’s footprint in the Permian Basin in West Texas, realizing advantages from combining ExxonMobil’s and Pioneer’s adjoining acreage, and reducing overall risk in its production portfolio by increasing its domestic crude oil production, based on public statements. ExxonMobil expects that combining its 570,000 net acres in the Delaware and Midland Basins—both sub-basins of the Permian Basin—with Pioneer’s more than 850,000 net acres in the Midland Basin will result in an estimated 16 billion barrels of oil equivalent (BOE) worth of reserves in the Permian Basin. The Permian has been the main source of increasing crude oil production in the United States in recent years, and we expect it to continue to be a major source for production growth in the United States going forward.
Public statements suggest Chevron’s primary goal for acquiring Hess Corporation is to gain access to the Stabroek Block off the coast of Guyana. The Stabroek Block is the world’s largest oil discovery in the last 10 years; Chevron estimates the block has more than 11 billion BOE of recoverable resources. Hess owns a 30% stake in the Stabroek Block, ExxonMobil owns 45%, and China National Offshore Oil Corporation owns 25%. ExxonMobil filed an arbitration claim to block Chevron’s acquisition of Hess’s Stabroek Block stake, claiming a right to first refusal of a sale. The outcome of the claim could affect whether the acquisition is completed or not.
Other recent notable deals include:
- Diamondback Energy announced it will merge with Endeavor Energy for a total transaction cost of $26 billion, which is the total cost for Diamondback Energy to acquire all Endeavor Energy shares, its net debt, and transaction costs from the merger. The combined company has the potential to be the third-largest oil and natural gas producer in the Permian Basin, behind ExxonMobil and Chevron.
- Occidental Petroleum Corporation announced it will acquire CrownRock L.P. for a total acquisition cost of $12 billion. Occidental , which produced about 1.2 million BOE per day (BOE/d) of crude oil, NGL, and natural gas in 3Q23, expects to add 170,000 BOE/d of production in 2024 from CrownRock’s assets in the Midland Basin.
- Chesapeake Energy announced it will merge with Southwestern Energy for a total transaction cost of $11.5 billion, which is the total cost for Chesapeake Energy to acquire all Southwestern Energy shares, its net debt, and transaction costs from the merger. The combined company, which will assume a new name at closing, could become the largest natural gas producer in the United States.
- Chevron acquired PDC Energy, Inc., for a total acquisition cost of $7.6 billion in August 2023. Prior to acquisition, PDC Energy produced 178,000 b/d of crude oil and NGL in 2Q23, mainly from assets in the Denver-Julesburg Basin in Colorado.
- ExxonMobil acquired Denbury, Inc., for a total acquisition cost of $4.9 billion in November 2023. The deal provides ExxonMobil with the largest CO 2 pipeline network in the United States and 10 onshore sequestration sites used for carbon capture and storage .
- APA Corporation announced it will acquire Callon Petroleum Company for a total acquisition cost of $4.5 billion. In 3Q23, APA Corporation produced 150,000 b/d of crude oil and NGL in the United States, and Callon Petroleum Company produced 81,000 b/d. Both companies operate primarily in the Permian Basin in West Texas and New Mexico.
Principal contributor: Alex De Keyserling
Tags: natural gas , financial markets , liquid fuels , crude oil , oil/petroleum , Permian , production/supply
- Share full article
Advertisement
Supported by
New York Takes Crucial Step Toward Making Congestion Pricing a Reality
The board of the Metropolitan Transportation Authority voted to approve a new $15 toll to drive into Manhattan. The plan still faces challenges from six lawsuits before it can begin in June.

By Winnie Hu and Ana Ley
New York City completed a crucial final step on Wednesday in a decades-long effort to become the first American city to roll out a comprehensive congestion pricing program, one that aims to push motorists out of their cars and onto mass transit by charging new tolls to drive into Midtown and Lower Manhattan.
The program could start as early as mid-June after the board of the Metropolitan Transportation Authority, the state agency that will install and manage the program, voted 11-to-1 to approve the final tolling rates, which will charge most passenger cars $15 a day to enter at 60th Street and below in Manhattan. The program is expected to reduce traffic and raise $1 billion annually for public transit improvements.
It was a historic moment for New York’s leaders and transportation advocates after decades of failed attempts to advance congestion pricing even as other gridlocked cities around the world, including London, Stockholm and Singapore, proved that similar programs could reduce traffic and pollution.
While other American cities have introduced related concepts by establishing toll roads or closing streets to traffic, the plan in New York is unmatched in ambition and scale.
Congestion pricing is expected to reduce the number of vehicles that enter Lower Manhattan by about 17 percent, according to a November study by an advisory committee reporting to the M.T.A. The report also said that the total number of miles driven in 28 counties across the region would be reduced.
“This was the right thing to do,” Janno Lieber, the authority’s chairman and chief executive, said after the vote. “New York has more traffic than any place in the United States, and now we’re doing something about it.”
Congestion pricing has long been a hard sell in New York, where many people commute by car from the boroughs outside of Manhattan and the suburbs, in part because some of them do not have access to public transit.
New York State legislators finally approved congestion pricing in 2019 after Gov. Andrew M. Cuomo helped push it through. A series of recent breakdowns in the city’s subway system had underscored the need for billions of dollars to update its aging infrastructure.
It has taken another five years to reach the starting line. Before the tolling program can begin, it must be reviewed by the Federal Highway Administration, which is expected to approve it.
Congestion pricing also faces legal challenges from six lawsuits that have been brought by elected officials and residents from across the New York region. Opponents have increasingly mobilized against the program in recent months, citing the cost of the tolls and the potential environmental effects from shifting traffic and pollution to other areas as drivers avoid the tolls.
A court hearing is scheduled for April 3 and 4 on a lawsuit brought by the State of New Jersey, which is seen as the most serious legal challenge. The mayor of Fort Lee, N.J., Mark J. Sokolich, has filed a related lawsuit.
Four more lawsuits have been brought in New York: by Ed Day, the Rockland County executive; by Vito Fossella, the Staten Island borough president, and the United Federation of Teachers; and by two separate groups of city residents.
Amid the litigation, M.T.A. officials have suspended some capital construction projects that were to be paid for by the program, and they said at a committee meeting on Monday that crucial work to modernize subway signals on the A and C lines had been delayed.
Nearly all the toll readers have been installed, and will automatically charge drivers for entering the designated congestion zone at 60th Street or below. There is no toll for leaving the zone or driving around in it. Through traffic on Franklin D. Roosevelt Drive and the West Side Highway will not be tolled.
Under the final tolling structure, which was based on recommendations by the advisory panel, most passenger vehicles will be charged $15 a day from 5 a.m. to 9 p.m. on weekdays, and from 9 a.m. to 9 p.m. on weekends. The toll will be $24 for small trucks and charter buses, and will rise to $36 for large trucks and tour buses. It will be $7.50 for motorcycles.
Those tolls will be discounted by 75 percent at night, dropping the cost for a passenger vehicle to $3.75.
Fares will go up by $1.25 for taxis and black car services, and by $2.50 for Uber and Lyft. Passengers will be responsible for paying the new fees, and they will be added to every ride that begins, ends or occurs within the congestion zone. There will be no nighttime discounts. (The new fees come on top of an existing congestion surcharge that was imposed on for-hire vehicles in 2019.)
The tolls will mostly be collected using the E-ZPass system. Electronic detection points have been placed at entrances and exits to the tolling zone. Drivers who do not use an E-ZPass will pay significantly higher fees — for instance, $22.50 instead of $15 during peak hours for passenger vehicles.
Emergency vehicles like fire trucks, ambulances and police cars, as well as vehicles carrying people with disabilities, were exempted from the new tolls under the state’s congestion pricing legislation .
As for discounts, low-income drivers who make less than $50,000 annually can apply to receive half off the daytime toll after their first 10 trips in a calendar month. In addition, low-income residents of the congestion zone who make less than $60,000 a year can apply for a state tax credit.
All drivers entering the zone directly from four tolled tunnels — the Lincoln, Holland, Hugh L. Carey and Queens-Midtown — will receive a “crossing credit” that will be applied against the daytime toll. The credit will be $5 round-trip for passenger vehicles, $12 for small trucks and intercity and charter buses, $20 for large trucks and tour buses, and $2.50 for motorcycles. No credits will be offered at night.
Grace Ashford contributed reporting.
Winnie Hu is a Times reporter covering the people and neighborhoods of New York City. More about Winnie Hu
Ana Ley is a Times reporter covering New York City’s mass transit system and the millions of passengers who use it. More about Ana Ley
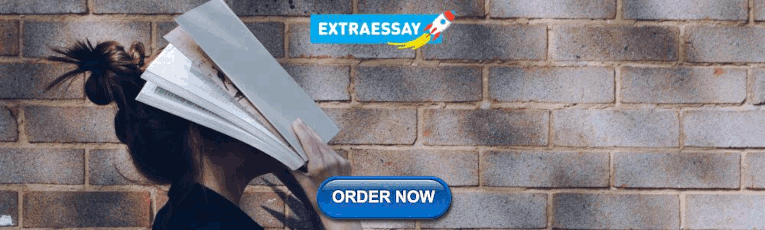
IMAGES
VIDEO
COMMENTS
A statistical analysis essay is an academic paper that involves analyzing quantitative data and interpreting the results. It is often used in social sciences, economics and business to draw meaningful conclusions from the data. The objective of a statistical analysis essay is to analyze a specific dataset or multiple datasets in order to answer ...
Table of contents. Step 1: Write your hypotheses and plan your research design. Step 2: Collect data from a sample. Step 3: Summarize your data with descriptive statistics. Step 4: Test hypotheses or make estimates with inferential statistics.
One group was given the data and a standard statistical analysis of the data; 72% of these economists got the answer wrong. Another group was given the data, the statistical analysis, and a graph; still 61% of these economists got the answer wrong. A third group was given only the graph, and only 3% got the answer wrong.
Step 3: Describing the Data. Once you are done finalising your samples, you are good to go with their inspection by calculating descriptive statistics, which we discussed above. There are different ways to inspect your data. By using a scatter plot to visualise the relationship between two or more variables.
Introduction. Statistical analysis is necessary for any research project seeking to make quantitative conclusions. The following is a primer for research-based statistical analysis. It is intended to be a high-level overview of appropriate statistical testing, while not diving too deep into any specific methodology.
Hypothesis testing is a formal process of statistical analysis using inferential statistics. The goal of hypothesis testing is to compare populations or assess relationships between variables using samples. Hypotheses, or predictions, are tested using statistical tests. Statistical tests also estimate sampling errors so that valid inferences ...
Body paragraphs - they should contain a detailed analysis of the statistical data produced by research; Conclusion - it contains the summary of your work and the conclusions you've come to. Your essay may include these parts without individual headings, but if you want to make your paper easier to navigate you can add them.
The term "statistical analysis" refers to the use of quantitative data to investigate trends, patterns, and relationships. Scientists, governments, businesses, and other organizations use it as a research tool. Statistical analysis necessitates careful planning from the outset of the research process in order to obtain meaningful conclusions.
Levels of measurement tell you how precisely variables are recorded. There are 4 levels of measurement, which can be ranked from low to high: Nominal: the data can only be categorised.; Ordinal: the data can be categorised and ranked.; Interval: the data can be categorised and ranked, and evenly spaced.; Ratio: the data can be categorised, ranked, evenly spaced and has a natural zero.
The use of chi-square test, correlation, regression, etc., are some examples of statistical tools used to predict a survey. Descriptive and inferential statistics are similar procedures because they both use population size and sample to analyze statistical data. The difference between these two procedures can be explained by the pattern of ...
The p value determines statistical significance. An extremely low p value indicates high statistical significance, while a high p value means low or no statistical significance. Example: Hypothesis testing. To test your hypothesis, you first collect data from two groups. The experimental group actively smiles, while the control group does not.
Statistics could also be used to analyze grades on an essay by assigning numeric values to the letter grades, e.g., A=4, B=3, C=2, D=1, and F=0. ... The ANOVA (analysis of variance) is a statistical test which makes a single, overall decision as to whether a significant difference is present among three or more sample means (Levin 484). An ...
Statistical analysis of the data is to be carried out using technique sof analyzing categorical data. In this process a contingency table analysis is employed in order to examine the nature of relationship existing between two categorical variables (financial stability and customer spending).In this process, the McNemer's test will be used in analyzing if the pair is dichotomous (Elliot ...
The second part of the paper (Questions of statistical analysis) should reflect the essence of specific methods of statistical generalization and analysis of indicators considered by the essay. The content of statistical methods, calculation formulas, stages of calculations, the system of the interrelation of indicators are mandatory for this ...
Scheaffer and Jacobbe (2014) and Utts (2015) state that the use of statistical data with the focus on its further analysis and interpretation is often a challenge for educators, and they need to concentrate on developing skills in working with statistical methods. We will write a custom essay on your topic. 809 writers online.
Statistical methods involved in carrying out a study include planning, designing, collecting data, analysing, drawing meaningful interpretation and reporting of the research findings. The statistical analysis gives meaning to the meaningless numbers, thereby breathing life into a lifeless data. The results and inferences are precise only if ...
Additionally, add data sheets, questions, and records of interviews and focus groups to the appendix. The statistical analysis and quotes from interviews, on the other hand, must be included in the dissertation. 4. Validity and Reliability Of Statistical Analysis. The idea that the information provided is self-explanatory is a prevalent one.
Writing with Descriptive Statistics. Usually there is no good way to write a statistic. It rarely sounds good, and often interrupts the structure or flow of your writing. Oftentimes the best way to write descriptive statistics is to be direct. If you are citing several statistics about the same topic, it may be best to include them all in the ...
In the Singular Sense: "Statistics refers to the body of technique or methodology, which has been developed for the collection, presentation and analysis of quantitative data and for the use of such data in decision making." —Ncttor and Washerman. "Statistics may rightly be called the science of averages." —Bowleg.
Conclusion. Statistics are a vital aspect of daily life, enabling us to make more informed decisions, analyze trends, and evaluate claims. Understanding statistics is critical to navigating the world we live in effectively and ensuring that we make sound decisions. Statistical literacy is essential for effective decision-making in both personal ...
Table of contents. Step 1: Write your hypotheses and plan your research design. Step 2: Collect data from a sample. Step 3: Summarise your data with descriptive statistics. Step 4: Test hypotheses or make estimates with inferential statistics.
Personal income increased $66.5 billion (0.3 percent at a monthly rate) in February, according to estimates released today by the Bureau of Economic Analysis (tables 2 and 3). Disposable personal income (DPI), personal income less personal current taxes, increased $50.3 billion (0.2 percent) and personal consumption expenditures (PCE) increased $145.5 billion (0.8 percent).
Real gross domestic product (GDP) increased in all 50 states and the District of Columbia in the fourth quarter of 2023, with the percent change ranging from 6.7 percent in Nevada to 0.2 percent in Nebraska (table 1), according to statistics released today by the U.S. Bureau of Economic Analysis (BEA).Current-dollar GDP increased in 49 states and the District of Columbia.
There are two full-service terminals that receive, store, and load coal onto ocean-going vessels at the Port of Baltimore: the Curtis Bay Coal Piers served by the CSX Railroad and the CONSOL Energy Baltimore Marine terminal served by both the CSX and Norfolk Southern Railroads.
In 2023, crude oil and natural gas exploration and production (E&P) companies increased spending on mergers and acquisitions (M&A) to $234 billion, the most in real 2023 dollars since 2012. Recent dealmaking marks a return to the previous trend of consolidation among oil companies in the United States after transactions declined amid significant oil market volatility in 2020 and 2022.
Congestion pricing is expected to reduce the number of vehicles that enter Lower Manhattan by about 17 percent, according to a November study by an advisory committee reporting to the M.T.A. The ...