
- SUGGESTED TOPICS
- The Magazine
- Newsletters
- Managing Yourself
- Managing Teams
- Work-life Balance
- The Big Idea
- Data & Visuals
- Reading Lists
- Case Selections
- HBR Learning
- Topic Feeds
- Account Settings
- Email Preferences
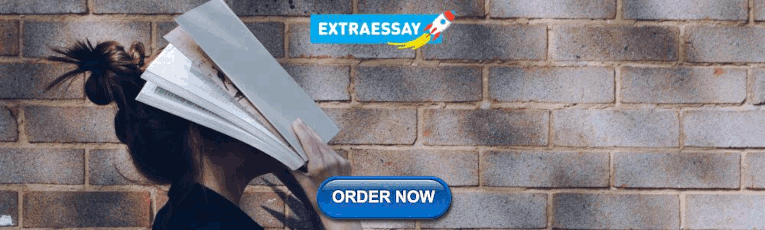
The Dangers of Categorical Thinking
- Bart de Langhe
- Philip Fernbach

Human beings are categorization machines, taking in voluminous amounts of messy data and then simplifying and structuring it. That’s how we make sense of the world and communicate our ideas to others. But according to the authors, categorization comes so naturally to us that we often see categories where none exist. That warps our view of the world and harms our ability to make sound decisions—a phenomenon that should be of special concern to any business that relies on data collection and analysis for decision making. Categorical thinking, the authors argue, creates four dangerous consequences. When we categorize, we compress category members, treating them as more alike than they are; we amplify differences between members of different categories; we discriminate, favoring certain categories over others; and we fossilize, treating the categorical structure we’ve imposed as static. In the years ahead, companies will have to focus attention on how best to mitigate those consequences.
We’re hardwired to sort information into buckets—and that can hamper our ability to make good decisions.
Idea in Brief
The problem.
We all think categorically, and for good reason: It helps us make sense of the world. But in business, categorical thinking can lead to major errors in decision making.
The Fallout
When we categorize, we compress category members, treating them as if they’re more alike than they are; we amplify differences across categories; we discriminate, favoring certain categories over others; and we fossilize, acting as if the structures we’ve imposed are static.
The Solution
Thoughtful leaders can avoid the harm that comes from categorical thinking in four ways: by ensuring that everybody understands the dangers of categorization, by developing ways to analyze data continuously, by questioning decision-making criteria, and by actively “de-fossilizing” categories.
Say ta. Say da. Now repeat the sounds, in each case paying attention to how you’re making them in your mouth. What’s the difference?
- BL Bart de Langhe is an associate professor of marketing at ESADE Business School, Ramon Llull University, in Barcelona.
- PF Philip Fernbach is a marketing professor at the Leeds School of Business, University of Colorado Boulder, and a coauthor of The Knowledge Illusion: Why We Never Think Alone (Riverhead Books, 2017).
Partner Center

An official website of the United States government
The .gov means it’s official. Federal government websites often end in .gov or .mil. Before sharing sensitive information, make sure you’re on a federal government site.
The site is secure. The https:// ensures that you are connecting to the official website and that any information you provide is encrypted and transmitted securely.
- Publications
- Account settings
Preview improvements coming to the PMC website in October 2024. Learn More or Try it out now .
- Advanced Search
- Journal List
- HHS Author Manuscripts

Categories, Concepts, and Conceptual Development
Vladimir m. sloutsky.
a Department of Psychology, The Ohio State University
Wei (Sophia) Deng
b Department of Psychology, University of Macau
Concepts (i.e., lexicalized classes of real or fictitious entities) play a central role in many human intellectual activities, including planning, thinking, reasoning, problem solving, and decision making. How do people acquire concepts in the course of development and learning and use them in their thinking about the world? In this article, we attempt to provide an overview of conceptual development. We suggest that concepts can originate (1) in interactions with the world and get lexicalized later or (2) in the language and get grounded later. The first route is from category learning to a concept, and we discuss this route by focusing on the mechanisms of category learning and developmental changes in these mechanisms. The second route is from a word to a concept, and we discuss this route by focusing on inferring word meanings without visual referents. We then consider proposals of how concepts get organized into networks and hierarchies.
INTRODUCTION
There are many abilities that reflect the remarkable intelligence of humans: people make inferences, develop and use scientific theories, make laws, preserve knowledge and pass it onto new generations, write fiction, reason about past and future, and make counterfactual arguments.
For example, upon learning that all animals are heterotrophs (i.e., they use other organisms as a source of energy) one may conclude that cats are heterotrophs as well. Furthermore, this conclusion follows with logical necessity from knowledge of taxonomy (i.e., cat is a proper subset of animal ) and of the logic of classes (i.e., if class X is properly included in class Y, then whatever is attributable to Y is attributable to X). Therefore, all properties of class Y (i.e., animals) is shared by class X (i.e., cats). One can also entertain a counterfactual (which in this case follows with logical necessity): if cats were not heterotrophs, they would not have been animals .
All these abilities are based on concepts, and, in fact, it is difficult to imagine reasoning (or any intellectual activity) without concepts. Therefore, one of the most interesting challenges in the study of human cognitive development is to understand how people acquire concepts in the course of development and learning and use them in their thinking about the world.
In this article, we attempt to provide a brief overview of conceptual development. Because concepts are classes (rather than individuals) they are more general or abstract . We use these terms interchangeably: abstract or general versus concrete or specific is a dimension reflecting how inclusive the class is. More inclusive classes are also more abstract and general. For example, cats is a more abstract class than an individual (e.g., my cat Fluffy ), but less abstract than a more inclusive class of mammals or living things . More abstract concepts can be formed by the process of abstraction or generalization , whereby some (presumably more important) properties are preserved, whereas other (presumably less important) properties are dropped. For example, it is easy to see that triangle is more abstract than equilateral triangle, whereas polygon (a shape with any number of edges and vertices) is more abstract than triangle .
To distinguish concepts from other classes and sets (such as categories), we define concepts as lexicalized classes of real or fictitious entities. We also suggest that concepts can originate (1) in interactions with the world and get lexicalized later or (2) in the language and get grounded later. For example, it is conceivable that a toddler has enough encounters with dogs to form a category before learning the word for it. In contrast, the concept germ cannot originate in experience and has to originate in language. Therefore, concepts can be acquired in a bottom-up manner (i.e., originating in experience) or in a top-down manner (i.e., originating in language).
Because bottom-up concepts (i.e., those acquired in a bottom-up manner) are tightly linked to experience, there are a number of constraints as to what these concepts might be. In general, many of these are “embodiment” constraints on the concepts (cf., Yu & Smith, 2012 , for ideas on the role of embodiment in the early word learning). Among these constraints are (1) these concepts should primarily include perceptible (and possibly actionable) entities, (2) these concepts should be mostly based on objects, (3) these objects should predominate in a particular child’s experience, (4) these objects’ sizes should be within a certain range, and (5) there should be common and frequently used labels within the child’s native language to denote these objects.
In contrast, the top-down concepts (those acquired in a top-down manner) have a different set of constraints. Because these concepts originate in language, the primary constraints are (1) parents’ education and vocabulary, (2) topics of conversations with and around the child, (3) access to books and media and types of available books and media, and (4) access to formal education. Second language learning may offer an interesting illustration of these latter constraints, as both authors of this paper learned English as a second language. For example, because of the literature we read while learning English, we learned words like pride , prejudice , vanity , expectation , and curiosity long before learning words for eggshell , faucet , fingernail , eyelash , or tire .
In what follows, we will overview concepts and conceptual behaviors, introduce category learning as an important step in conceptual development, discuss both ways of acquiring concepts (i.e., the bottom-up and the top-down), and consider how concepts get organized into coherent networks that promote understanding of the world as well as thinking and reasoning about it.
What are Concepts?
In the simplest possible way, concepts can be defined as lexicalized categories , or equivalence classes . What is an equivalence class? In his chapter focusing on concepts (Chapter XII of the Principles of Psychology ), William James (1983/1890) wrote: “Our principle only lays it down that the mind makes continual use of the notion of sameness, and, if deprived of it, would have a different structure from what it has.” In other words, the mind can treat different things as if they were equivalent in some way. Once equivalence is established, it could be marked by lexicalization: by calling two different dogs d 1 and d 2 a Dog , we can express that as d 1 ≡ d 2 . When such an equivalence class (or category) is lexicalized, it becomes a concept . Lexicalization allows (a) accumulation of new (often unobservable) information about categories and (b) communicating and sharing this information with others. Examples of concepts vary from chairs (obviously, chairs are non-identical, but merely equivalent in some way) to odd numbers to extremely abstract concepts, such as cause or effect . If concepts are lexicalized categories, then development may proceed either from forming a category first to lexicalization or from acquiring a lexical item first to the formation of a category. As we discuss below, both types of progression can be observed in individual development.
We also suggest that regularities in both the world and in language are sources of conceptual development. In the world, members of many categories (e.g., cats or birds) share multiple observable features and therefore these categories can be learned without language. On the other hand, language also provides important input to conceptual development: many related categories (e.g., wolfs and dolphins) share some observable and many unobservable features and knowledge of these unobservable features comes from language. In what follows, we discuss both developments. We first review some principles of category learning – principles that may apply to learning of pre-linguistic categories. We then review how language can be a rich source of conceptual development by contributing to the formation of conceptual hierarchies.
Perceptual Groupings, Categories, Concepts, and Conceptual Networks
If concepts are lexicalized categories (some of which can be learned prior to language acquisition and some cannot be learned without language), then, like to categories to which they refer, conceptual behaviors may vary substantially in levels of complexity ranging from simple perceptual groupings of arbitrary categories, to full blown lexicalized concepts that are linked to other concepts which thereby form conceptual networks. The study of each type of conceptual behavior requires somewhat different research paradigms.
First, people can learn perceptual groupings or equivalence classes that are based on purely perceptual properties. Such groupings may include imposing categorical boundaries on sensory continua (known as categorical perception, e.g., Eimas, 1994 ), learning dot patterns coming from a single prototype and generalizing learning to distortions from the studied prototype, or forming a category based on image properties (see Bhatt & Quinn, 2010 , for a review). Perceptual groupings are the simplest form of categorization because they allow extending category membership on the basis of global familiarity. Therefore, if members of category A share some features, a novel item would be judged as a member of A to the extent that it has these features.
A more complicated variant of conceptual behavior requires one to learn two or more mutually exclusive categories (e.g., cats vs. dogs) at the same time. The categories are mutually exclusive because there are no members common to A and B (i.e., A∩B=⊘). This problem is more difficult than simple perceptual groupings because a decision of whether a novel item belongs to A or to B cannot be made on the basis of global familiarity (i.e., both A and B are equally familiar). The studied categories can be based on multiple correlated features (birds have wings, feathers, and beaks, whereas fish have scales, fins, and gills), few features (e.g., squirrels have a long, fluffy tail, whereas hamsters have a small tail), or relations among features (e.g., rectangles can be grouped into tall if the aspect ratio is less than 1, and wide if the aspect ratio is more than 1). The categories may be also deterministic (such that there is a subset of features that is sufficient to predict category membership with a 100% accuracy) or probabilistic (such that any feature or a combination of features predicts category membership only with a degree of probability). Therefore, to make a categorization decision, at the very minimum, some processing of two category structures is required. This task has been used in some studies with infants and animals, and in many category-learning studies with children and adults.
An even more complicated variant of conceptual behavior is the ability to lexicalize categories and use them in reasoning, inference, prediction, or judgment. Such lexicalized categories can be defined as concepts proper. Lexicalization is critical as it enables acquiring knowledge that may not be directly observable in a given situation (e.g., dogs are friendly pets, they like meat, and are taken to a vet for a physical exam). In other words, having a word for a category allows accumulation of knowledge from sources that are not based on direct observation of category members. These sources include conversations with others, books and other media sources, and formal education. Such concepts proper can be studied in a variety of tasks, including grouping of items, property listing, picture naming, and category judgment among others. A grouping task may require participants to put together items of the same kind (e.g., toys versus animals), whereas an attribute listing task may require a participant to list properties of categories (e.g., of cats, birds, or animals).
Finally, a conceptual network involves not only knowledge of concepts, but also of relations among these concepts. Take, for example, Newton’s second law (F = ma) that acceleration of a body is directly proportional to the net force acting on the body and inversely proportional to the mass of the body. Here, the concepts of mass , force , and acceleration are linked and are part of a broader conceptual network. For example, force is linked to work and power , whereas acceleration is linked to time and space . In other words, all these concepts are linked and each can be derived from others.
Such networks can be organized in a variety of ways; for example, networks of naturally occurring categories often have hierarchical, or taxonomical, organization (e.g., grey hound → dog → mammal → animal → living thing). One way of detecting such hierarchies is a classification task in which a diverse set of items is partitioned into N mutually exclusive and exhaustive sub-sets. These subsets can then be further partitioned into smaller groups or combined into larger groups. Although it has been argued that classification tasks may underestimate children’s concepts (the fact that a child may put together a dog and a bone does not mean that the child considers the two to be the same thing, see Fodor, 1972 ), classification tasks are useful in that they may reveal a limit on the kinds of concepts children may form.
In short, humans exhibit multiplicity of conceptual behaviors, some are universal and shared with other animals, whereas others are uniquely human. Overall, human conceptual repertoire ranges from perceptual groupings (something that can be also achieved by certain non-mammalian species) to conceptual networks that are likely to be unique to humans.
EARLY CONCEPTUAL DEVELOPMENT: FROM CATEGORIES TO EARLY CONCEPTS
Conceptual behaviors come in various forms: they range from more simple, universal, and early emerging forms (i.e., establishing equivalence between non-identical percepts) to rather complex, uniquely human, and late emerging forms (i.e., forming a conceptual network in a knowledge domain).
Category Structure and Category Learning
Are all categories the same? Perhaps not: although there is little doubt that categories differ in content, the most interesting distinctions pertain to category structure. Structural differences identified by researchers include syntactic differences (nouns versus verbs; e.g., Gentner, 1981 ), ontological differences (natural kinds versus artifacts; e.g., Barton & Komatzu, 1989 ), taxonomic differences (i.e., basic-level versus superordinate-level; e.g., Rosch & Mervis, 1975 ), differences in organizational principle (entity categories versus relational categories; e.g., Gentner & Kurtz, 2005 ), differences in concreteness (concrete versus abstract categories; e.g., Barsalou, 1999 ), differences in category coherence and confusability (e.g., Homa et al., 1979 ; Smith & Minda, 2000 ; Rouder & Ratcliff, 2004 ), and some other distinctions (for a review, see Medin, Lynch, & Solomon, 2000 ).
Kloos and Sloutsky (2008) proposed another structural distinction, one that could form the basis for many of the above distinctions. They proposed the idea of statistical density, that is a measure of category structure that (a) can (in principle) be measured independently rather than be inferred from participants’ patterns of response and (b) provides a continuous measure rather than a dichotomous one (which makes it well suited for capturing the graded nature of differences between categories). Conceptually, statistical density is a ratio of variance relevant for category membership to the total variance across members and non-members of the category. Intuitively, statistical density is a measure of how members of a category are separated from non-members (see Kloos & Sloutsky, 2008 , for a detailed discussion). For example, a category of small racing cars is dense (even when contrasted with other categories of vehicles) because there are multiple correlated features that distinguish this category. In contrast, a category of red things is sparse as there is a single feature common to the category members and distinguishing this category from any contrasting category.
The idea of statistical density has important implications for the development of category learning. One possibility is that category learning progresses from spontaneous learning of highly dense categories (i.e., when multiple dimensions are correlated within a category) to less spontaneous (and more guided or supervised) learning of sparser categories (i.e., only few dimensions are relevant; for example, members of a category are all red, but vary on multiple dimensions, such as shape, color, texture, and size).
Category Learning: What is the Mechanism and What Develops?
Category learning is the process by which one or more equivalence classes of discriminable entities are formed. How do people form these classes? One of the first ideas was that category learning is a variant of stimulus generalization: if a new item is sufficiently similar (i.e., exceeds some threshold value) to a member or members of an identified category, it would be included in this category. However, this simple and compelling idea may have difficulty explaining learning of categories based on a single dimension. For example, Shepard, Hovland, & Jenkins (1961) demonstrated that people easily learn single-dimension categories (e.g., black shapes vs. white shapes), even though within-category similarity (measured as stimulus confusability) may be small. Shepard et al. (1961) concluded that categories can be learned by selectively attending to a relevant dimension. These ideas have been captured in several influential models of categorization (e.g., Kruschke, 1992 ; Nosofsky, 1986 ; Love, Medin, & Gureckis, 2004 ).
Similar to Shepard et al. (1961) , these models suggested that categories of the same structure can be learned either by allocating attention to few relevant dimensions or by distributing attention across many dimensions. The way attention is allocated is consequential for how quickly the category will be learned and how the dimensions will be represented in memory. In particular, the former way of category learning is fast and efficient, but it may result in inattention to (and consequently, relatively poor memory for) irrelevant dimensions as these dimensions are ignored. In contrast, the latter way of learning may be slower and less efficient, but may result in attention allocated to all dimensions (and consequently, memory for both relevant and irrelevant dimensions).
Learning of similarity-based categories is a developmental default.
It is hardly controversial that selective attention undergoes protractive development (see Hanania & Smith, 2010; Lane & Pearson, 1982 ; Plude, Enns, & Brodeur, 1994 ; for reviews), with infants and young children tending to distribute attention ( Best, Yim, & Sloutsky, 2013 ; Plebanek & Sloutsky, 2017 ; Deng & Sloutsky, 2015a ). If infants and young children tend to distribute attention rather than to attend selectively, how do they learn categories? One idea is that first categories that infants and young children learn are categories that do not require selective attention – these are sufficiently perceptually distinct and have enough within-category structure to be learned without selective attention ( Sloutsky, 2010 ; Sloutsky & Fisher, 2004a ).
In an attempt to examine the mechanism of category learning and its potential change with development, Deng and Sloutsky (2015b) presented 4-year-olds, 6-year-olds, and adults with a category learning task, in which participants learned two categories. The categories had a rule-plus-similarity structure, such that there was a single deterministic (or rule) feature and multiple probabilistic features (see Figure 1 , for examples of stimuli). Therefore, this category structure allows examining what participants learn spontaneously: given the same set of stimuli, participants could learn either rule-based or similarity-based categories.

Examples of stimuli used in Deng and Sloutsky (2015b) . There were two family resemblance categories, with each training item including a single deterministic feature D (which perfectly distinguished between the two categories) and multiple probabilistic features P (with each providing imperfect probabilistic information about category membership). The body mark (introduced as a body button) was the D feature, and all the other features—the head, body, hands, feet, antennae, and tail—were the P features. Each row depicts items within a category, whereas each column identified an item role (e.g., switch item) and item type (e.g., P jalet D flurp ). The High-Match items were used in training and testing. The switch items, new-D, one-new-P, and all-new-P items were used only in testing. Neither prototype was shown in training or testing.
To establish what specifically was learned by participants, the authors presented them with categorization and memory testing (various test items are presented in Figure 1 ). Categorization trials included High-Match items (these were the items used in training), Switch items (these were the items that had the rule feature from one category and probabilistic features from another category), and All-new-P (these items had an old deterministic feature and all new probabilistic features). The goal of High-Match items was to test whether participants learned the category. The goal of Switch items was to examine what they learned about the category (i.e., whether they learned a rule-based or similarity-based category). Finally, the goal of All-new-P items was to examine whether participants could generalize on the basis of the rule features. There were also memory tests examining memory for the rule feature (i.e., New-D items) and each probabilistic feature (i.e., One-new-P items).
Results of categorization testing (see Figure 2 ) indicated that whereas participants of all age groups ably learned the categories (as evidenced by high performance on High-Match items), only older participants relied in their categorization on rule features (as evidenced by the above-chance performance on Switch items). In contrast, younger participants relied on the overall similarity (as evidenced by the below-chance performance on Switch items). In addition, younger participants were at chance for the All-new-P items, whereas older participants were reliably above chance. These results suggested that whereas 4-year-olds learned similarity-based categories, 6-year-olds and adults learned rule-based categories.

Categorization Performance: Proportion of rule-based responses by trial type and training condition for 4-year-old children, 6-year-old children and adults (After Deng & Sloutsky, 2015b , Experiment 1). Error bars represent standard error of the mean.
Researchers also tested participants’ memory for features. Memory results are important because they reflected what participants had learned about the categories. Whereas older children and adults remembered the rule features better than the probabilistic features, 4-year-olds remembered all the features equally well. Furthermore, follow-up studies indicated that 4-year-olds’ memory for probabilistic features was (at least numerically) better than that of older participants (cf. Sloutsky & Fisher, 2004b ). Taken together, these results indicated that whereas older children and adults spontaneously learned rule-based categories (if the structure supported such learning), young children spontaneously learned similarity-based categories.
To further examine mechanisms of early category learning, Deng & Sloutsky (2015b) attracted their attention to the deterministic feature by pointing to this feature on every training trial and commenting on the importance of this feature. Although in this condition 4-year-olds appeared to have learned a rule-based category (as evidenced by their categorization and generalization responses), they exhibited equivalently good memory for all features. Therefore, their categorization decisions pointed to selective attention, whereas their pattern of memory did not. These findings suggest that category representations and category decisions may be decoupled early in development, but they become coupled in the course of development.
The role of category labels in category learning.
Often times, children learn the category and the label, lexicalizing this category, concurrently. This happens, for example, when a new object is shown to the child and is labeled (e.g., “look, a dax”). In this case, the child needs to figure out what are the other objects that are daxes , or learn the category of dax . In the lab studies, this type of learning is referred to as category learning by classification. First examples of categories are introduced and labeled. Then the participant needs to predict which of the novel items also belong to this category (i.e., have the same category label). Given that participants learn the category and the linguistic label at the same time, it is reasonable to ask: How does the label affect learning? And does this role changes with development?
Several ideas have been proposed. Some have argued that from early in development, the category label is a category marker—an indicator that the items belong to the same category—and guides or supervises category learning ( Gelman & Markman, 1986 ; Gelman, 2003 ; Waxman & Markow, 1995 , Waxman & Gelman, 2009 ; Welder & Graham, 2001 ; Westermann & Mareschal, 2014 ). In contrast, others ( Deng & Sloutsky, 2012 ; 2013 ; 2015a ; Sloutsky & Lo, 1999 ; Sloutsky & Fisher, 2004a ; Sloutsky, Lo, & Fisher, 2001 ) have argued that, at least early in development, labels are akin to other features of items, but their role may change in the course of development. How could these positions be tested and contrasted?
In an attempt to distinguish between labels being features and category markers, Yamauchi and Markman (1998 , 2000 ) developed a paradigm potentially capable of settling the issue. The paradigm is based on the following idea. Imagine two categories A (labeled “A”) and B (labeled “B”), each having five binary dimensions (e.g., Size: large vs. small, Color: black vs. white, Shape: square vs. circle, Luminance: bright vs. dark, and Texture: smooth vs. rough). The prototype of Category A has all values denoted by “1” (i.e., “A”, 1, 1, 1, 1, 1) and the prototype of Category B has all values denoted by “0” (i.e., “B”, 0, 0, 0, 0, 0). There are two inter-related generalization tasks – classification and induction. The goal of the classification task is to infer category membership (and hence the label) on the basis of presented features. For example, participants are presented with all the values for an item (e.g., ?, 0, 1, 1, 1, 1) and have to predict category label “A” or “B”. In contrast, the goal of the induction task is to infer a feature on the basis of category label and other presented features. For example, given an item (e.g., “A”, 1, ?, 1, 0, 1), participants have to predict the value of the missing feature. A critical manipulation that could illuminate the role of labels is the “low-match” condition. For low-match induction, participants were presented with an item “A”, ?, 0, 1, 0, 0 (which had the label of A, but more features in common with the prototype of Category B) and asked to infer the missing feature. For low-match classification, participants were presented with an item “?”, 1, 0, 1, 0, 0 (which again had more features in common with the prototype of Category B) and asked to infer the missing label.
These researchers argued that if the label is just a feature then performance on the classification and the induction tasks should be symmetrical. However, if the label is more than a feature and serve as a category marker, then inferring a label when features are provided (i.e., a classification task) should elicit different performance from a task of inferring a feature when the label is provided (i.e., an induction task). Specifically, category-consistent responding should be more likely in induction tasks (where participants could rely on the category label) than in classification tasks (where participants had to infer the category label). This asymmetry should be particularly evident in the critical low-match condition: in the low-match classification task (when they predict the label and thus cannot rely on it), participants would be likely to identify low-match items (e.g., “?”, 1, 0, 1, 0, 0) as belonging to category B (because these items have more features in common with prototype B), whereas in the low-match induction (when they can rely on the label), participants would be likely to identify low-match items (e.g., “A”, ?, 0, 1, 0, 0) as belonging to category A.
Upon finding predicted asymmetries between the two conditions, these researchers concluded that category labels differed from other features in that adult participants were more likely to treat labels as category markers rather than as features. These findings have been replicated in a series of follow-up studies ( Yamauchi, Kohn, & Yu, 2007 ; Yamauchi & Yu, 2008 ; see also Markman & Ross, 2003 , for a review). However, when Deng and Sloutsky (2013) extended this paradigm to children, they found symmetric performance: regardless of the condition (i.e., classification or induction), young children relied on multiple features rather than on the label (see Figure 3 ). It was concluded, therefore, that early in development category labels may function as features of objects, but they become more than features in the course of development.

Proportion of category-consistent responses by feature match and testing condition (After Deng & Sloutsky, 2013 , Experiment 1). Note. Error bars represent standard error of the mean.
This shift in the role of labels is related to the protractive development of selective attention discussed above: For a label to be used as a category marker, people should be able to selectively attend to relevant information and ignore irrelevant information. They should also have enough experience to realize that labels have higher cue validity than other features: even considering homonyms (which reduce cue validity), the probability that X belongs to category K, given that it has label “K” is very high”.
This transition in the role of label is important because once a label denotes a category, much information about the category can be accumulated through conversations, reading, media, and education. This information can also be merged with what is accumulated through observation. As a result, linguistic labels may become “knowledge hubs” that afford non-trivial inferences that are impossible through observation, such as “cows and dolphins are mammals” or “plants and animals are alive.” Having these “knowledge hubs” in place is critical for semantic development – learning information about categories and using this information for linking the concepts together and forming conceptual networks and hierarchies. We focus on these issues in the next section.
SEMANTIC DEVELOPMENT: FROM EARLY CONCEPTS TO CONCEPTUAL NETWORKS AND TAXONOMIES
Language is not a necessary aspect of category learning: nonhuman animals and preverbal human infants can learn categories ( Lazareva & Wasserman, 2008 ; Smith, et al., 2012 , 2015 ; Eimas & Quinn, 1994 ; Madole & Oakes, 1999 ; Younger & Cohen, 1985 ). However, lexicalization of categories, or learning words for categories, is a critical step in integrating knowledge about objects, people, and events, and this integrated knowledge—semantic memory—is central to our ability to use concepts for planning, prediction, explanation, reasoning, and decision making. Furthermore, as discussed above, words may be a starting point for learning many new categories. For example, unobservable categories (such as germs , heat , or energy ) can be only learned that way.
Importantly, regardless of how a concept is learned (i.e., from a category to lexicalization or from a lexical entry to a category), words for categories eventually become part of category representation and they help connecting what is known about a given category and integrating it with newly learned information. For example, when learning a category (e.g., cat ), some information can be acquired from observing the cats (e.g., shape, texture, and the pattern of locomotion), whereas other information cannot (e.g., the fact that cats have hearts, brains, and other internal organs). This latter information requires a verbal description and lexicalization is critical here: the sentence cats have brains can only be expressed if one knows words for the cat and the brain. For the same reason, words are also important for forming conceptual hierarchies, such as grey hound → dog → mammal → animal → living thing → thing. All this is a product of development and in this section, we discuss how this development may occur.
From Words to Categories: Learning Words from Context
Between birth and adulthood, a typical English-speaking child learns on average about 8–10 words per day: learning new words starts slowly, but accelerates dramatically during the second year of life ( Bloom, 1973 ). Obviously, some of these words are learned by ostension (i.e., someone points to a putative referent and explicitly labels it), but many (if not most) are learned from context, including conversations and reading (see Nagy, Herman, & Anderson, 1985 ; Goodman, McDonough, & Brown, 1998 ), sometimes without a referent being present. When this happens, it is important to ask: How do people infer meanings of words, without having the referent present?
A number of ideas have been proposed to answer this question. Some have argued that the context provides the learner with syntactic, semantic, or social cues to meaning. Another possibility is that the context provides the learner with a rich network of associations that may help figuring out the meaning of the new word. Note that these ideas are not mutually exclusive and different sources may mutually strengthen each other.
One idea is that children use syntactic cues to disambiguate the meaning of a novel word. The idea goes back to Roger Brown (1957) who elegantly demonstrated that when presented with a novel word, 3–4 year-olds used the syntactic frame (e.g., “this is a sib” vs. “this one is sibbing”) to determine whether the word referred to an object, action, or property. These ideas generated much empirical support (see Bloom, 2000 , for an extensive review). For example, Soja (1992) found that participants could use count noun/mass noun syntax to guide their learning of novel nouns. Syntactic cues have also been shown to facilitate learning of new verbs, the process known as syntactic bootstrapping (e.g., Fisher, 1996 ; Gleitman, 1990 ; Landau & Gleitman, 1985 ; Naigles, 1990 ). Another idea is that semantic cues can assist word learning. For example, Goodman et al. (1998) demonstrated that 2-year-olds could learn novel words when given sufficient semantic cues, such as familiar verbs (e.g., “Mommy feeds the ferret”) suggesting possible meanings of a novel noun. However, it is easy to see that syntactic and semantic cues provide only a rough guide as to what the meaning of the new word might be.
The third idea is that word learning could be assisted by the “theory of mind.” In particular, solving a social problem of what the speaker is calling attention to may guide word learning (e.g., Akhtar & Tomasello, 2000 ). This social problem could be solved by focusing on the speaker’s gaze direction, facial expression ( Baldwin, 1991 ; 1993 ), or on some relevant aspects of a social situation ( Akhtar, 2002 ). For example, Akhtar (2002) presented 2-year-olds with a word learning task. In one condition, participants’ attention was attracted to the texture of objects (“this is a smooth one and this is a fuzzy one”), and in another condition their attention was attracted to the shape of the objects. Participants were then shown a triad of novel objects, and one of the novel objects was labeled (“this is a dacky one”). The remaining objects matched the labeled object either in shape or in texture. The results indicated that the context affected inferred meaning of the new adjective – participants were more likely to infer that “dacky” was a shape word in the shape-relevant context. However, while social problem solving may offer some assistance in figuring out the meaning, its assistance is rather limited when entities have multiple feature dimensions. For example, what would be the set of relevant features to communicate that the word cat refers to all cats and only to cats and how could these features be communicated?
Finally, a more recent proposal ( Sloutsky, Yim, Yao, & Dennis, 2017 ) suggests that the context in which words are presented provides associative cues that trigger a candidate meaning of a novel word. Two types of associative cues are of particular importance – syntagmatic and paradigmatic ( Brown & Berko, 1960 ; Dennis, 2005 ; Ervin-Tripp, 1970 ; Nelson, 1977 ).
Syntagmatic associations refer to words that co-occur in close temporal proximity (e.g., The dog was barking at the car). Words associated syntagmatically tend to be thematically related. Paradigmatic associations refer to words playing the same role in sentences and appearing in similar sentential contexts (e.g., “He went home to feed the cat” and “She drove home to feed the dog” ). Words associated paradigmatically also tend to be taxonomically related.
While syntagmatic associations exhibit an early onset, paradigmatic associations tend to emerge later in development appearing around 6-years of age ( McNeill, 1963 ; Nelson, 1977 ). Under this construal, early in development (i.e., before paradigmatic associations come online), only syntagmatic associations provide cues to the meaning of a novel word. At the same time, later in development, both syntagmatic and paradigmatic associations provide cues to novel words. If this is the case, then early in development syntagmatic associates should be better cues than paradigmatic ones.
To illustrate, imagine that the learner is presented by a sequence “..., furry, dax” or by a sequence “..., cat, dax”. When syntagmatic associations predominate (which is believed to be the case for young children), the word furry is associated with the word animal (strengths of such associations can be measured by a free association task ( Nelson, McEvoy, & Schreiber, 2004 ) allowing to calculate the probability that the word animal will be recalled in a free association task, given the word furry ). In this case, furry will activate the word animal , and this may result in dax being interpreted as a kind of animal. At the same time, if paradigmatic associations are not formed yet, the word cat will not be associated with the word animal . As a result, the word furry will be a better cue to the meaning of dax (i.e., suggesting that dax is an animal) than the word cat .
In the case of emergence of paradigmatic associations (which we believe are a product of development), the word furry remains associated with the word animal syntagmatically, whereas the word cat become associated with the word animal paradigmatically. As a result, both words may activate the word animal : furry via the syntagmatic route, whereas cat via the paradigmatic route. Under this construal, the probability that the word dax would be considered as referring to some sort of an animal, would be proportional to the forward associative strength between the words accompanying dax and the word animal (or, perhaps, features of animacy or the set of animals). The initial meaning of a word learned from context is broad and imprecise and additional information would be needed to zero in on a precise meaning of the word. This initially broad meaning may become more precise with additional experience with the word.
If syntagmatic and paradigmatic associations play a role in inferring meanings of novel words, young children whose associative repertoire is limited to syntagmatic associations ( Nelson, 1977 ) should learn words from contexts that involve syntagmatic associations. At the same time, adults, whose repertoire includes both syntagmatic and paradigmatic associations should learn words from contexts that have associations of either type. These predictions have been supported in new research coming out of our lab ( Sloutsky, et al., 2017 ). Specifically, in a series of experiments, Sloutsky et al. presented 4-year-olds and adults with sets of words that included a single nonsense word (e.g., dax ) and asked them to indicate whether the nonsense word was an animal or an artifact. Across experiments, adults reliably identified the appropriate category of the nonsense word when lists contained associatively (all members of the list were semantic associates of the word animal , e.g., zoo , farm , furry , creature , giraffe , hamster , bear , and feeding ) or taxonomically (all members of the list were animals referred to by count nouns, e.g., cat , dog , fish , bird , horse , squirrel , cow , and rabbit ) related items, whereas children could only identify the appropriate category when lists contained associatively related items. Furthermore, a computational model developed by these researchers indicated that only the syntagmatic network initially affected the model performance, which was sufficient to account for the child data. In contrast, to capture the adult data, additional learning in the paradigmatic network was needed, which developed later in training. These results suggest a syntagmatic to paradigmatic shift in development and provide a mechanistic account for the shift: while word co-occurrence (which appears in linguistic environment early in development) gives rise to syntagmatic associations, experience with language (which accumulates with development) gives rise to paradigmatic associations.
Linking Words and Categories: The Development of Semantic Knowledge
With the dramatic growth in language, children start to learn words for both known and unknown categories. There is much evidence that these emerging concepts (as well as fully developed ones) get organized into some form of semantic (or conceptual) network. This evidence includes studies of semantic priming, the development and decline of sematic memory, as well as of property and picture verification tasks (see Rogers & McClelland, 2004 , for a review). In short, language allows the development of a conceptual network (also referred to as semantic knowledge or semantic memory) that represents one’s knowledge about the world.
In subsequent studies, researchers attempted to examine whether priming effects in adults stem from thematic relations (or syntagmatic associations) or from taxonomic relations (or paradigmatic associations). These researchers found evidence for priming effects due taxonomic relations in the absence of thematic relations (e.g., Ferrand & New, 2003 ; Thompson-Schill, Kurtz, & Gabrieli, 1998 ) and for priming effects due thematic relations in the absence of taxonomic relations (e.g., Ferrand & New, 2003 ). However, priming effects were more reliable for words that were both taxonomically and thematically related (e.g., dog and cat) than for words that were related only taxonomically (e.g., dog and cow) or only thematically (e.g., dog and bone) ( McRae & Boisvert, 1998 ; Moss, Ostrin, Tyler, & Marslen-Wilson, 1995 ; Perea & Rosa, 2002 ).
Although considerable progress has been made in characterizing the structure of adults’ semantic knowledge, origins of this knowledge and how it develops remain unclear. Findings from studies of word associations in children are controversial. Some researchers argue that the ability to represent semantic relations transpires very early in development, with infants as young as 24 months of age exhibiting evidence of semantic priming. Others argue for protracted development, presenting evidence of semantic (or taxonomic) sensitivity emerging during elementary school years.
For example, Arias-Trejo and Plunkett (2009) used an intermodal preferential looking paradigm to examine 18- and 21-month-old infants’ responses to related prime-target pairs such as cat-dog, compared with unrelated pairs such as plate-dog. The related pairs were strongly associated according to adult associative norms. Infants first heard a phrase such as “I saw a cat”, followed by a target word (dog). They then concurrently saw two images, one related (e.g., a dog) and one unrelated (e.g., a door). The critical manipulation was whether the initial phrase contained a prime word related to the target word and picture (e.g., “I saw a cat…dog”) or an unrelated word (e.g., “I saw a swing…dog”). The researchers found that 18-month-olds looked significantly longer to the picture named by the target word, regardless of whether it followed a related or an unrelated prime phrase. In contrast, 21-month-olds looked significantly longer to the named picture in the related prime–target condition (“I saw a cat…dog”) but not in the unrelated prime–target condition (“I saw a swing…dog”). The older infants’ sensitivity to the word relatedness provides evidence for lexical organization in infants by 21 months of age.
To further identify the type of lexical relatedness, Arias-Trejo and Plunkett (2013) used the same paradigm with 21- and 24-month-olds, with prime-target word pairs that were either thematically or taxonomically related or were unrelated. Twenty-four-month-olds, but not 21-month-olds, exhibited a priming effect for words that were either thematically or taxonomically related, and the age-related differences suggest that semantic relationships between words developed between 21 and 24 months of age. Similarly, Willits, Wojcik, Seidenberg, & Saffran (2013) also provide convergent evidence showing that, even in the absence of visual referents, infants by 24 months of age are able to represent semantic relations between words when processing language.
However, a recent study by Unger et al. (2016) found that even preschoolers were unable to represent relations that were only taxonomic: these participants appear to recognize only links between concepts that are related along multiple dimensions (both thematic and taxonomic). Older children increasingly recognize links between concepts that are related along one dimension (either thematic or taxonomic), and starting at approximately the age of second grade, taxonomic relations are prioritized over thematic relations. This finding is in contrast to the infant literature where semantic priming is found in 24-month infants, which could be possibly due to the methodological differences between studies with infants and with older children (see also Sloutsky, Deng, Fisher, & Kloos, 2015 , for related evidence).
Although the precise structuring principles of children’s semantic network remains unclear, most researchers would agree that there is a dramatic development in children’s semantic knowledge: children learn links between words and categories and form connections among concepts. Importantly, the development of semantic knowledge is critical and fundamental to the development of conceptual networks and hierarchies.
Development of Conceptual Hierarchies
One critical step in acquiring conceptual significance is establishing a structure for concepts. Among various possible conceptual structures ( Kemp, Shafto, & Tenenbaum, 2012 ), taxonomic hierarchy is the most general and well-studied one. An example of taxonomic hierarchy is grey hound → dog → mammal → animal → living thing → thing. This kind of hierarchy is based on class-inclusion relations, with lower-level categories being exhaustive with respect to a higher-level category, and sub-classes of higher-level category being mutually exclusive, with no common members in it.
In order to form a taxonomic hierarchy of concepts, some researchers ( Inhelder & Piaget, 1964 ) argued that the ability to understand the class-inclusion relations, or the logical constraints, is of critical importance. The idea of the logic of classes is that multidimensional sets of stimuli can be divided into proper subsets focusing on one dimension at a time. As proposed by Inhelder and Piaget (1964) , the development of conceptual hierarchies is a function of the development of the logic of classes. According to this view, fundamental developmental changes occur with respect to understanding of class-inclusion relations, which is closely tied to the ability of understanding quantifiers, such as all , some , some are not , and none . Once these are mastered, a classification scheme based on these relations can be applied to any domain of knowledge. However, logic alone is not sufficient for building the taxonomic hierarchies of concepts. One also needs to know dimensions that distinguish sub-categories, to learn words denoting different level of classes, and to acquire knowledge of a domain in which a taxonomy is to be built. Therefore, most contemporary theories consider domain knowledge as a necessary component of the development of conceptual hierarchies (e.g., Carey, 1985 ; Chi, Hutchinson, & Robin, 1989 ; Inagaki & Hatano, 2002 ; Keil, 1981 ) and argue that a taxonomic hierarchy of concepts may result from knowledge of a domain. According to this view, knowledge of a domain reveals class-inclusion relations, which gives rise to one’s hierarchical representations of concepts. For example, knowledge of mammals may help a child understand that all dogs are mammals, but not all mammals are dogs. In this case, the child does not necessarily need to understand logic and apply class-inclusion relations.
An alternative view, has been proposed by Rogers and McClelland (2004) , according to which conceptual hierarchies can be formed implicitly, on the basis of shared predicates. For example, salmon and trout share most of the predicates (e.g., can swim, had gills, has scales, has skin, has bones, etc.), whereas salmon and eagle share only some of the predicates (e.g., has skin, has bones). Therefore, salmon and eagle are members of a broader class (i.e., vertebrates) than salmon and trout . Although this is a promising approach, it runs into some obvious difficulties. First, in order to bypass the problem of class inclusion, one need to have a representative sample of predicates associated with a given category. However, given vast differences in experience, there is no guarantee that this would happen. As a result, there may be more individual variability in conceptual hierarchies than is currently observed.
Another potential problem is that simply adding the predicates may result in a wrong taxonomy. For example, animals sharing the habitat (e.g., dolphin and tuna or bat and hawk ) may share more predicates with members of a broader class that with members of a narrow class (e.g., dolphin and cow ), yet people eventually learn to classify such animal correctly.
In sum, although people often behave as if they have conceptual hierarchies, it is not fully understood if , when , and how such conceptual hierarchies are developed. Evidence for the early onset of conceptual hierarchies is limited. Even if a child exhibits the ability to classify items at a superordinate level or draws inductive inferences on the basis of a superordinate class, this ability does not necessarily indicate the presence of a conceptual hierarchy—because the child can rely on similarity. Although it is clear that the ability to understand class-inclusion relations (along with the ability to use quantifiers) and the knowledge of how these relations can be applied in a particular domain can greatly contribute to the development of conceptual hierarchies, it remains controversial as to whether the former is really necessary for such development (cf. McClelland & Rogers, 2004 ).
CONCLUSIONS
Conceptual development supports many uniquely human behaviors ranging from accumulation of knowledge that is not directly observable to multiple ways of using this knowledge. Importantly, conceptual development has humble origins – it is based on the ability to form categories, the ability that humans share with many non-human animals. However, this ability is greatly amplified (if not transformed) by language: (a) lexicalization helps turning categories into knowledge hubs as well as to mark to-be-learned categories and (b) language is an important source of knowledge about the concepts. Knowledge acquired through perceptual experience coupled with knowledge acquired through language (including reading) leads to the formation of conceptual networks and hierarchies.
However, much is unknown about each constituent part of conceptual development (i.e., the development of category learning, lexicalization, and sematic organization) as well as about their interaction. Therefore, a challenge for future research is to establish precise details of conceptual development, including the way the constituent components change and interact in the course of development.
Acknowledgments
Writing of this manuscript is supported by IES grant R305A140214 and NIH grants R01HD078545 and P01HD080679 to Vladimir Sloutsky. We thank members of the Cognitive Development Lab for helpful comments.
- Akhtar N (2002). Relevance and early word learning . Journal of Child Language , 29 , 677–686. doi: 10.1017/S0305000902005214 [ PubMed ] [ CrossRef ] [ Google Scholar ]
- Akhtar N & Tomasello M (2000). The social nature of words and word learning In Golinkoff RM & Hirsh-Pasek K (Eds.), Becoming a word learner: a debate on lexical acquisition (pp. 115–135). Oxford: Oxford University Press. [ Google Scholar ]
- Arias-Trejo N, & Plunkett K (2009). Lexical-semantic priming effects during infancy . Philosophical Transactions of the Royal Society B: Biological Sciences , 364 ( 1536 ), 3633–3647. doi: 10.1098/rstb.2009.0146 [ PMC free article ] [ PubMed ] [ CrossRef ] [ Google Scholar ]
- Arias-Trejo N, & Plunkett K (2013). What’s in a link: associative and taxonomic priming effects in the infant lexicon . Cognition , 128 , 214–227. doi: 10.1016/j.cognition.2013.03.008 [ PubMed ] [ CrossRef ] [ Google Scholar ]
- Baldwin DA (1991). Infants’ contribution to the achievement of joint reference . Child Development , 62 , 875–890. doi: 10.1111/j.1467-8624.1991.tb01577.x [ PubMed ] [ CrossRef ] [ Google Scholar ]
- Baldwin DA (1993). Early referential understanding: Infants’ ability to recognize referential acts for what they are . Developmental Psychology , 29 , 832–843. doi: 10.1037/0012-1649.29.5.832 [ CrossRef ] [ Google Scholar ]
- Barsalou LW (1999). Perceptual symbol systems . Behavioral and Brain Sciences , 22 , 577–609. doi: 10.1017/S0140525X99002149 [ PubMed ] [ CrossRef ] [ Google Scholar ]
- Barton ME, & Komatsu LK (1989). Defining features of natural kinds and artifacts . Journal of Psycholinguistic Research , 18 , 433–447. doi: 10.1007/BF01067309 [ CrossRef ] [ Google Scholar ]
- Best CA, Yim H, & Sloutsky VM (2013). The cost of selective attention in category learning: Developmental differences between adults and infants . Journal of Experimental Child Psychology , 116 , 105–119. doi: 10.1016/j.jecp.2013.05.002 [ PMC free article ] [ PubMed ] [ CrossRef ] [ Google Scholar ]
- Bhatt RS, & Quinn PC (2010). How does learning impact development in infancy? The case of perceptual organization . Infancy , 16 , 2–38. doi: 10.1111/j.1532-7078.2010.00048.x [ PMC free article ] [ PubMed ] [ CrossRef ] [ Google Scholar ]
- Bloom L (1973). One word at a time: The use of single word utterances before syntax . The Hague: Mouton. [ Google Scholar ]
- Bloom P (2000). How children learn the meanings of words . Cambridge, MA: MIT Press. [ Google Scholar ]
- Brown R (1957). Linguistic determinism and the part of speech . Journal of Abnormal and Social Psychology , 55 , 1–5. doi: 10.1037/h0041199 [ PubMed ] [ CrossRef ] [ Google Scholar ]
- Brown R, & Berko J (1960). Word association and the acquisition of grammar . Child Development , 31 , 1–14. doi: 10.2307/1126377 [ PubMed ] [ CrossRef ] [ Google Scholar ]
- Carey S (1985). Conceptual change in childhood . Cambridge, MA: MIT Press. [ Google Scholar ]
- Chi MTH, Hutchinson JE, & Robin AF (1989). How inferences about novel domain-related concepts can be constrained by structured knowledge . Merrill-Palmer Quarterly , 35 , 27–62. [ Google Scholar ]
- Deng W, & Sloutsky VM (2012). Carrot eaters or moving heads: Inductive inference is better supported by salient features than by category labels . Psychological Science , 23 , 178–186. doi: 10.1177/0956797611429133 [ PubMed ] [ CrossRef ] [ Google Scholar ]
- Deng W, & Sloutsky VM (2013). The role of linguistic labels in inductive generalization . Journal of Experimental Child Psychology , 114 , 432–455. doi: 10.1016/j.jecp.2012.10.011 [ PMC free article ] [ PubMed ] [ CrossRef ] [ Google Scholar ]
- Deng W, & Sloutsky VM (2015a). Linguistic labels, dynamic visual features, and attention in infant category learning . Journal of Experimental Child Psychology , 134 , 62–77. doi: 10.1016/j.jecp.2015.01.012 [ PMC free article ] [ PubMed ] [ CrossRef ] [ Google Scholar ]
- Deng W, & Sloutsky VM (2015b). The development of categorization: Effects of classification and inference training on category representation . Developmental Psychology , 51 , 392–405. doi: 10.1037/a0038749 [ PMC free article ] [ PubMed ] [ CrossRef ] [ Google Scholar ]
- Dennis S (2005). A memory-based theory of verbal cognition . Cognitive Science , 29 , 145–193. doi: 10.1207/s15516709cog0000_9 [ PubMed ] [ CrossRef ] [ Google Scholar ]
- Eimas PD (1994). Categorization in early infancy and the continuity of development . Cognition , 50 , 83–93. doi: 10.1016/0010-0277(94)90022-1 [ PubMed ] [ CrossRef ] [ Google Scholar ]
- Eimas PD, & Quinn PC (1994). Studies on the formation of perceptually based basic‐level categories in young infants . Child Development , 65 , 903–917. doi: 10.1111/j.1467-8624.1994.tb00792.x [ PubMed ] [ CrossRef ] [ Google Scholar ]
- Ervin-Tripp SM (1970). Substitution, context and association In Postman L & Keppel G (Eds.), Norms of word association (pp. 383–467). New York: Academic Press. [ Google Scholar ]
- Ferrand L, & New B (2003). Semantic and associative priming in the mental lexicon In Bonin P (Ed.), Mental Lexicon: Some Words to Talk about Words (pp. 25–43). Hauppauge, NY: Nova Science Publisher. [ Google Scholar ]
- Fisher C (1996). Structural limits on verb mapping: The role of analogy in children’s interpretations of sentences . Cognitive Psychology , 31 , 41–81. doi: 10.1006/cogp.1996.0012 [ PubMed ] [ CrossRef ] [ Google Scholar ]
- Fodor J (1972). Some reflections on LS Vygotsky’s Thought and Language . Cognition , 1 , 83–95. doi: 10.1016/0010-0277(72)90046-7 [ CrossRef ] [ Google Scholar ]
- Gelman SA (2003). The essential child: Origins of essentialism in everyday thought . New York: Oxford University Press. [ Google Scholar ]
- Gelman SA, & Markman E (1986). Categories and induction in young children . Cognition , 23 , 183–209. doi: 10.1016/0010-0277(86)90034-X [ PubMed ] [ CrossRef ] [ Google Scholar ]
- Gentner D (1981). Some interesting differences between nouns and verbs . Cognition and Brain Theory , 4 , 161–178. [ Google Scholar ]
- Gentner D, & Kurtz KJ (2005). Relational categories In Ahn WK, Goldstone RL, Love BC, Markman AB, & Wolff PW (Eds.), Categorization inside and outside the lab (pp. 151–175). Washington, DC: APA. [ Google Scholar ]
- Gleitman LR (1990). The structural sources of verb meanings . Language Acquisition , 1 , 3–55. doi: 10.1207/s15327817la0101_2 [ CrossRef ] [ Google Scholar ]
- Goodman JC, McDonough L, & Brown NB (1998). The role of semantic context and memory in the acquisition of novel nouns . Child Development , 69 , 1330–1344. doi: 10.1111/j.1467-8624.1998.tb06215.x [ PubMed ] [ CrossRef ] [ Google Scholar ]
- Hanania R, & Smith LB (2009). Selective attention and attention switching: towards a unified developmental approach . Developmental Science , 13 , 622–635. doi: 10.1111/j.1467-7687.2009.00921.x [ PMC free article ] [ PubMed ] [ CrossRef ] [ Google Scholar ]
- Homa D, Rhoades D, & Chambliss D (1979). Evolution of conceptual structure . Journal of Experimental Psychology: Human Learning and Memory , 5 , 11–23. doi: 10.1037/0278-7393.5.1.11 [ CrossRef ] [ Google Scholar ]
- Inagaki K, & Hatano G (2002). Young children’s naive thinking about biological world . New York, NY: Psychology Press. [ Google Scholar ]
- Inhelder B, & Piaget J (1964). The early growth of logic in the child: classification and seriation . New York: Harper and Row. [ Google Scholar ]
- James W (1983/1890). The Principles of Psychology (originally published in 1890) . Cambridge, MA: Harvard University Press. [ Google Scholar ]
- Keil FC (1981). Constraints on knowledge and cognitive development . Psychological Review , 88 , 197–227. doi: 10.1037/0033-295X.88.3.197 [ CrossRef ] [ Google Scholar ]
- Kemp C, Shafto P, & Tenenbaum JB (2012). An integrated account of generalization across objects and features . Cognitive Psychology , 64 , 35–73. doi: 10.1016/j.cogpsych.2011.10.001 [ PubMed ] [ CrossRef ] [ Google Scholar ]
- Kloos H, & Sloutsky VM (2008). What’s behind different kinds of kinds: Effects of statistical density on learning and representation of categories . Journal of Experimental Psychology: General , 137 , 52–72. doi: 10.1037/0096-3445.137.1.52 [ PubMed ] [ CrossRef ] [ Google Scholar ]
- Kruschke JK (1992). ALCOVE: an exemplar-based connectionist model of category learning . Psychological Review , 99 , 22–44. doi: 10.1037/0033-295X.99.1.22 [ PubMed ] [ CrossRef ] [ Google Scholar ]
- Landau B, & Gleitman LR (1985). Language and experience: Evidence from the blind child . Cambridge, MA: Harvard University Press. [ Google Scholar ]
- Lane DM, & Pearson DA (1982). The development of selective attention . Merrill-Palmer Quarterly , 28 , 317–337. [ Google Scholar ]
- Lazareva OF, & Wasserman EA (2008). Categories and concepts in animals In Menzel R (Ed.), Learning Theory and Behavior . Vol. [1] of Learning and Memory: A Comprehensive Reference , 4 vols. (Byrne JEd.), pp. 197–226, Oxford: Elsevier. [ Google Scholar ]
- Love BC, Medin DL, & Gureckis TM (2004). SUSTAIN: A network model of category learning . Psychological Review , 111 , 309–332. doi: 10.1037/0033-295X.111.2.309 [ PubMed ] [ CrossRef ] [ Google Scholar ]
- Madole KL, & Oakes LM (1999). Making sense of infant categorization: Stable processes and changing representations . Developmental Review , 19 , 263–296. doi: 10.1006/drev.1998.0481 [ CrossRef ] [ Google Scholar ]
- Markman AB, & Ross BH (2003). Category use and category learning . Psychological Bulletin , 129 , 592–613. doi: 10.1037/0033-2909.129.4.592 [ PubMed ] [ CrossRef ] [ Google Scholar ]
- McNeill D (1963). The origin of associations within the same grammatical class . Journal of Verbal Learning and Verbal Behavior , 2 , 250–262. doi: 10.1016/S0022-5371(63)80091-2 [ CrossRef ] [ Google Scholar ]
- McRae K, & Boisvert S (1998). Automatic semantic similarity priming . Journal of Experimental Psychology: Learning, Memory, and Cognition , 24 , 558–572. doi: 10.1037/0278-7393.24.3.558 [ CrossRef ] [ Google Scholar ]
- Medin DL, Lynch EB, & Solomon KO (2000). Are there kinds of concepts? Annual Review of Psychology , 51 , 121–147. doi: 10.1146/annurev.psych.51.1.121 [ PubMed ] [ CrossRef ] [ Google Scholar ]
- Moss HE, Ostrin RK, Tyler LK, & Marslen-Wilson WD (1995). Accessing different types of lexical semantic information: Evidence from priming . Journal of Experimental Psychology: Learning, Memory, and Cognition , 21 , 863–883. doi: 10.1037/0278-7393.21.4.863 [ CrossRef ] [ Google Scholar ]
- Nagy WE, Herman PA, & Anderson RC (1985). Learning words from context . Reading Research Quarterly , 20 , 233–253. doi: 10.2307/747758 [ CrossRef ] [ Google Scholar ]
- Naigles L (1990). Children use syntax to learn verb meanings . Journal of Child Language , 17 , 357–374. doi: 10.1017/S0305000900013817 [ PubMed ] [ CrossRef ] [ Google Scholar ]
- Nelson DL, McEvoy CL, & Schreiber TA (2004). The University of South Florida free association, rhyme, and word fragment norms . Behavior Research Methods, Instruments, & Computers , 36 , 402–407. doi: 10.3758/BF03195588 [ PubMed ] [ CrossRef ] [ Google Scholar ]
- Nelson K (1977). The syntagmatic-paradigmatic shift revisited: a review of research and theory . Psychological Bulletin , 84 , 93–116. doi: 10.1037/0033-2909.84.1.93 [ PubMed ] [ CrossRef ] [ Google Scholar ]
- Nosofsky RM (1986). Attention, similarity, and the identification–categorization relationship . Journal of Experimental Psychology: General , 115 , 39–57. doi: 10.1037/0096-3445.115.1.39 [ PubMed ] [ CrossRef ] [ Google Scholar ]
- Perea M, & Rosa E (2002). The effects of associative and semantic priming in the lexical decision task . Psychological Research , 66 , 180–194. doi: 10.1007/s00426-002-0086-5 [ PubMed ] [ CrossRef ] [ Google Scholar ]
- Plebanek DJ, & Sloutsky VM (2017). Costs of selective attention: When children notice what adults miss . Psychological Science , 28 , 723–732. doi: 10.1177/0956797617693005 [ PMC free article ] [ PubMed ] [ CrossRef ] [ Google Scholar ]
- Plude DJ, Enns JT, & Brodeur D (1994). The development of selective attention: A life-span overview . Acta Psychologica , 86 , 227–272. doi: 10.1016/0001-6918(94)90004-3 [ PubMed ] [ CrossRef ] [ Google Scholar ]
- Rogers TT and McClelland JL (2004). Semantic cognition: A parallel distributed processing approach . Cambridge, MA: MIT Press. [ Google Scholar ]
- Rosch E, & Mervis CB (1975). Family resemblances: Studies in the internal structure of categories . Cognitive Psychology , 7 , 573–605. doi: 10.1016/0010-0285(75)90024-9 [ CrossRef ] [ Google Scholar ]
- Rouder JN, & Ratcliff R (2004). Comparing categorization models . Journal of Experimental Psychology: General , 133 , 63–82. doi: 10.1037/0096-3445.133.1.63 [ PMC free article ] [ PubMed ] [ CrossRef ] [ Google Scholar ]
- Shepard RN, Hovland CI, & Jenkins HM (1961). Learning and memorization of classifications . Psychological Monographs: General and Applied , 75 , 1–42. doi: 10.1037/h0093825 [ CrossRef ] [ Google Scholar ]
- Sloutsky VM (2010). From perceptual categories to concepts: What develops? Cognitive Science , 34 , 1244–1286. doi: 10.1111/j.1551-6709.2010.01129.x [ PMC free article ] [ PubMed ] [ CrossRef ] [ Google Scholar ]
- Sloutsky VM, Deng W, Fisher AV, & Kloos H (2015). Conceptual influences on induction: A case for a late onset . Cognitive Psychology , 82 , 1–31. doi: 10.1016/j.cogpsych.2015.08.005 [ PMC free article ] [ PubMed ] [ CrossRef ] [ Google Scholar ]
- Sloutsky VM, & Fisher AV (2004a). Induction and categorization in young children: a similarity-based model . Journal of Experimental Psychology: General , 133 , 166–188. doi: 10.1037/0096-3445.133.2.166 [ PubMed ] [ CrossRef ] [ Google Scholar ]
- Sloutsky VM, & Fisher AV (2004b). When development and learning decrease memory: Evidence against category-based induction in children . Psychological Science , 15 , 553–558. doi: 10.1111/j.0956-7976.2004.00718.x [ PubMed ] [ CrossRef ] [ Google Scholar ]
- Sloutsky VM, & Lo Y-F (1999). How much does a shared name make things similar? Part 1. Linguistic labels and the development of similarity judgment . Developmental Psychology , 35 , 1478–1492. doi: 10.1037/0012-1649.35.6.1478 [ PubMed ] [ CrossRef ] [ Google Scholar ]
- Sloutsky VM, Lo Y-F, & Fisher AV (2001). How much does a shared name make things similar? Linguistic labels, similarity, and the development of inductive inference . Child Development , 72 , 1695–1709. doi: 10.1111/1467-8624.00373 [ PubMed ] [ CrossRef ] [ Google Scholar ]
- Sloutsky VM, Yim H, Yao X, & Dennis S (2017). An associative account of the development of word learning . Cognitive Psychology , 97 , 1–98. doi: 10.1016/j.cogpsych.2017.06.001 [ PMC free article ] [ PubMed ] [ CrossRef ] [ Google Scholar ]
- Smith JD, & Minda JP (2000). Thirty categorization results in search of a model . Journal of Experimental Psychology: Learning, Memory, and Cognition , 26 , 3–27. doi: 10.1037/0278-7393.26.1.3 [ PubMed ] [ CrossRef ] [ Google Scholar ]
- Smith JD, Berg ME, Cook RG, Murphy MS, Crossley MJ, Boomer J, et al. (2012). Implicit and explicit categorization: A tale of four species . Neuroscience and Biobehavioral Reviews , 36 , 2355–2369. doi: 10.1016/j.neubiorev.2012.09.003 [ PMC free article ] [ PubMed ] [ CrossRef ] [ Google Scholar ]
- Smith JD, Zakrzewski AC, Johnston JJR, Roeder JL, Boomer J, Ashby FG, & Church BA (2015). Generalization of category knowledge and dimensional categorization in humans (Homo sapiens) and nonhuman primates (Macaca mulatta) . Journal of Experimental Psychology: Animal Learning and Cognition , 41 , 322–335. doi: 10.1037/xan0000071 [ PMC free article ] [ PubMed ] [ CrossRef ] [ Google Scholar ]
- Soja NN (1992). Inferences about the meanings of nouns: The relationship between perception and syntax . Cognitive Development , 7 , 29–45. doi: 10.1016/0885-2014(92)90003-A [ CrossRef ] [ Google Scholar ]
- Thompson-Schill SL, Kurtz KJ, & Gabrieli JDE (1998). Effects of semantic and associative relatedness on automatic priming . Journal of Memory and Language , 38 , 440–458. doi: 10.1006/jmla.1997.2559 [ CrossRef ] [ Google Scholar ]
- Unger L, Fisher AV, Nugent R, Ventura SL, & MacLellan CJ (2016). Developmental changes in semantic knowledge organization . Journal of Experimental Child Psychology , 146 , 202–222. doi: 10.1016/j.jecp.2016.01.005 [ PubMed ] [ CrossRef ] [ Google Scholar ]
- Waxman SR, & Gelman SA (2009). Early word-learning entails reference, not merely associations . Trends in Cognitive Sciences , 13 , 258–263. doi: 10.1016/j.tics.2009.03.006 [ PMC free article ] [ PubMed ] [ CrossRef ] [ Google Scholar ]
- Waxman SR, & Markow DB (1995). Words as invitations to form categories: Evidence from 12-to 13-month-old infants . Cognitive Psychology , 29 , 257–302. doi: 10.1006/cogp.1995.1016 [ PubMed ] [ CrossRef ] [ Google Scholar ]
- Welder AN, & Graham SA (2001). The influences of shape similarity and shared labels on infants’ inductive inferences about nonobvious object properties . Child Development , 72 , 1653–1673. doi: 10.1111/1467-8624.00371 [ PubMed ] [ CrossRef ] [ Google Scholar ]
- Westermann G, & Mareschal D (2014). From perceptual to language-mediated categorization . Philosophical Transactions of the Royal Society B: Biological Sciences , 369 , 20120391. doi: 10.1098/rstb.2012.0391 [ PMC free article ] [ PubMed ] [ CrossRef ] [ Google Scholar ]
- Willits JA, Wojcik EH, Seidenberg MS, & Saffran JR (2013). Toddlers activate lexical semantic knowledge in the absence of visual referents: Evidence from auditory priming . Infancy , 18 , 1053–1075. doi: 10.1111/infa.12026 [ PMC free article ] [ PubMed ] [ CrossRef ] [ Google Scholar ]
- Yamauchi T, Kohn N, & Yu NY (2007). Tracking mouse movement in feature inference: Category labels are different from feature labels . Memory & Cognition , 35 , 852–863. doi: 10.3758/BF03193460 [ PubMed ] [ CrossRef ] [ Google Scholar ]
- Yamauchi T, & Markman AB (1998). Category learning by inference and classification . Journal of Memory and Language , 39 , 124–148. doi: 10.1006/jmla.1998.2566 [ CrossRef ] [ Google Scholar ]
- Yamauchi T, & Markman AB (2000). Inference using categories . Journal of Experimental Psychology: Learning, Memory, and Cognition , 26 , 776–795. doi: 10.1037/0278-7393.26.3.776 [ PubMed ] [ CrossRef ] [ Google Scholar ]
- Yamauchi T, & Yu N (2008). Category labels versus feature labels: Category labels polarize inferential predictions . Memory & Cognition , 36 , 544–553. doi: 10.3758/MC.36.3.544 [ PubMed ] [ CrossRef ] [ Google Scholar ]
- Younger BA, & Cohen LB (1985). How infants form categories . Psychology of Learning and Motivation , 19 , 211–247. doi: 10.1016/S0079-7421(08)60528-9 [ CrossRef ] [ Google Scholar ]
- Yu C, & Smith LB (2012). Embodied attention and word learning by toddlers . Cognition , 125 , 244–262. doi: 10.1016/j.cognition.2012.06.016 [ PMC free article ] [ PubMed ] [ CrossRef ] [ Google Scholar ]
An IERI – International Educational Research Institute Journal
- Open access
- Published: 10 December 2014
The acquisition of problem solving competence: evidence from 41 countries that math and science education matters
- Ronny Scherer 1 , 2 &
- Jens F Beckmann 3
Large-scale Assessments in Education volume 2 , Article number: 10 ( 2014 ) Cite this article
14k Accesses
16 Citations
1 Altmetric
Metrics details
On the basis of a ‘problem solving as an educational outcome’ point of view, we analyse the contribution of math and science competence to analytical problem-solving competence and link the acquisition of problem solving competence to the coherence between math and science education. We propose the concept of math-science coherence and explore whether society-, curriculum-, and school-related factors confound with its relation to problem solving.
By using the PISA 2003 data set of 41 countries, we apply multilevel regression and confounder analyses to investigate these effects for each country.
Our results show that (1) math and science competence significantly contribute to problem solving across countries; (2) math-science coherence is significantly related to problem solving competence; (3) country-specific characteristics confound this relation; (4) math-science coherence is linked to capability under-utilisation based on science performance but less on math performance.
Conclusions
In sum, low problem solving scores seem a result of an impeded transfer of subjectspecific knowledge and skills (i.e., under-utilisation of science capabilities in the acquisition of problem solving competence), which is characterised by low levels of math-science coherence.
The ability to solve real-world problems and to transfer problem-solving strategies from domain-specific to domain-general contexts and vice versa has been regarded an important competence students should develop during their education in school (Greiff et al. [ 2013 ]; van Merriënboer [ 2013 ]). In the context of large-scale assessments such as the PISA study problem solving competence is defined as the ability to solve cross-disciplinary and real-world problems by applying cognitive skills such as reasoning and logical thinking (Jonassen [ 2011 ]; OECD [ 2004 ]). Since this competence is regarded a desirable educational outcome, especially math and science educators have focused on developing students’ problem solving and reasoning competence in their respective domain-specific contexts (e.g., Kind [ 2013 ]; Kuo et al. [ 2013 ]; Wu and Adams [ 2006 ]). Accordingly, different conceptual frameworks were proposed that describe the cognitive processes of problem solving such as understanding the problem, building adequate representations of the problem, developing hypotheses, conducting experiments, and evaluating the solution (Jonassen [ 2011 ]; OECD [ 2005 ]). In comparing these approaches in math and science, it seems apparent that there is a conceptual overlap between the problem solving models in these two domains. This overlap triggers the question regarding its contribution to the development of students’ cross-curricular problem-solving competence (Abd-El-Khalick et al. [ 2004 ]; Bassok and Holyoak [ 1993 ]; Hiebert et al. [ 1996 ]).
The operationalization and scaling of performance in PISA assessments enables direct contrasting of scores in students’ competences in math and problem solving. Leutner et al. ([ 2012 ]) suggest that discrepancies between math and problem solving scores are indicative of the relative effectiveness of math education (OECD [ 2004 ]). In line with a “Capability-Utilisation Hypothesis”, it is assumed that math scores that negatively deviate from their problem solving counterpart signify an under-utilisation of students’ problem-solving capabilities as indicated by their scores in generic problem solving.
We intend to extend this view in two ways: First, by introducing the concept of math-science coherence we draw the focus on the potential synergistic link between math and science education and its contribution to the acquisition of problem solving competence. Second, the introduction of a Capability Under-Utilisation Index will enable us to extend the focus of the Capability-Utilisation Hypothesis to both, math and science education. The combination of the concept of math-science coherence with the notion of capability-utilisation will help to further explore the facilitating processes involved in the transition of subject-specific knowledge and skills to the acquisition of problem solving competence. These insights are expected to contribute to a better understanding of meaningful strategies to improve and optimize educational systems in different countries.
Theoretical framework
Problem solving as an educational goal.
In the PISA 2003 framework, problem solving is referred to as “an individual’s capacity to use cognitive processes to resolve real, cross-disciplinary situations where the solution path is not immediately obvious” (OECD [ 2004 ], p. 156). This definition is based on the assumption of domain-general skills and strategies that can be employed in various situations and contexts. These skills and strategies involve cognitive processes such as: Understanding and characterizing the problem, representing the problem, solving the problem, reflecting and communicating the problem solution (OECD [ 2003 ]). Problem solving is often regarded a process rather than an educational outcome, particularly in research on the assessment and instruction of problem solving (e.g., Greiff et al. [ 2013 ]; Jonassen [ 2011 ]). This understanding of the construct is based on the assumption that problem solvers need to perform an adaptive sequence of cognitive steps in order to solve a specific problem (Jonassen [ 2011 ]). Although problem solving has also been regarded as a process skill in large-scale assessments such as the PISA 2003 study, these assessments mainly focus on problem solving performance as an outcome that can be used for international comparisons (OECD [ 2004 ]). However, problem solving competence was operationalized as a construct comprised of cognitive processes. In the context of the PISA 2003 study, these processes were referred to as analytical problem solving, which was assessed by static tasks presented in paper-and-pencil format. Analytical problem-solving competence is related to school achievement and the development of higher-order thinking skills (e.g., Baumert et al. [ 2009 ]; OECD [ 2004 ]; Zohar [ 2013 ]). Accordingly, teachers and educators have focused on models of problem solving as guidelines for structuring inquiry-based processes in their subject lessons (Oser and Baeriswyl [ 2001 ]). Van Merriënboer ([ 2013 ]) pointed out that problem solving should not only be regarded a mere instructional method but also as a major educational goal. Recent curricular reforms have therefore shifted towards the development of problem solving abilities in school (Gallagher et al. [ 2012 ]; Koeppen et al. [ 2008 ]). These reforms were coupled with attempts to strengthen the development of transferable skills that can be applied in real-life contexts (Pellegrino and Hilton [ 2012 ]). For instance, in the context of 21 st century skills, researchers and policy makers have agreed on putting emphasis on fostering skills such as critical thinking, digital competence, and problem solving (e.g., Griffin et al. [ 2012 ]). In light of the growing importance of lifelong learning and the increased complexity of work- and real-life problem situations, these skills are now regarded as essential (Griffin et al. [ 2012 ]; OECD [ 2004 ]). Hence, large-scale educational studies such as PISA have shifted towards the assessment and evaluation of problem solving competence as a 21 st century skill.
The PISA frameworks of math and science competence
In large-scale assessments such as the PISA studies, students’ achievement in the domains of science and mathematics play an important role. Moreover, scientific and mathematical literacy are now regarded essential to being a reflective citizen (Bybee [ 2004 ]; OECD [ 2003 ]). Generally, Baumert et al. ([ 2009 ]) have shown that students’ math and science achievements are highly related to domain-general ability constructs such as reasoning or intelligence. In this context, student achievement refers to “the result of domain-specific processes of knowledge acquisition and information processing” (cf. Baumert et al. [ 2009 ], p. 169). This line of argument is reflected in definitions and frameworks of scientific and mathematical literacy, which are conceptualized as domain-specific competences that are hierarchically organized and build upon abilities closely related to problem solving (Brunner et al. [ 2013 ]).
Scientific literacy has been defined within a multidimensional framework, differentiating between three main cognitive processes, namely describing, explaining, and predicting scientific phenomena, understanding scientific investigations, and interpreting scientific evidence and conclusions (OECD [ 2003 ]). In addition, various types of knowledge such as ‘knowledge about the nature of science’ are considered as factors influencing students’ achievements in this domain (Kind [ 2013 ]). We conclude that the concept of scientific literacy encompasses domain-general problem-solving processes, elements of scientific inquiry (Abd-El-Khalick et al. [ 2004 ]; Nentwig et al. [ 2009 ]), and domain-specific knowledge.
The definition of mathematical literacy refers to students’ competence to utilise mathematical modelling and mathematics in problem-solving situations (OECD [ 2003 ]). Here, we can also identify overlaps between cognitive processes involved in mathematical problem solving and problem solving in general: Structuring, mathematizing, processing, interpreting, and validating (Baumert et al. [ 2009 ]; Hiebert et al. [ 1996 ]; Kuo et al. [ 2013 ]; Polya [ 1945 ]). In short, mathematical literacy goes beyond computational skills (Hickendorff [ 2013 ]; Wu and Adams [ 2006 ]) and is conceptually linked to problem solving.
In the PISA 2003 framework, the three constructs of math, science, and problem solving competence overlap conceptually. For instance, solving the math items requires reasoning, which comprises analytical skills and information processing. Given the different dimensions of the scientific literacy framework, the abilities involved in solving the science items are also related to problem solving, since they refer to the application of knowledge and the performance of inquiry processes (OECD [ 2003 ]). This conceptual overlap is empirically supported by high correlations between math and problem solving ( r = .89) and between science and problem solving ( r = .80) obtained for the sample of 41 countries involved in PISA 2003 (OECD [ 2004 ]). The relation between math and science competence was also high ( r = .83). On the one hand, the sizes of the inter-relationships, give rise to the question regarding the uniqueness of each of the competence measures. On the other hand, the high correlations indicate that problem-solving skills are relevant in math and science (Martin et al. [ 2012 ]). Although Baumert et al. ([ 2009 ]) suggest that the domain-specific competences in math and science require skills beyond problem solving (e.g., the application of domain-specific knowledge) we argue from an assessment perspective that the PISA 2003 tests in math, science, and problem solving measure predominantly basic academic skills relatively independent from academic knowledge (see also Bulle [ 2011 ]).
The concept of capability-utilisation
Discrepancies between students’ performance in math/science and problem solving were studied at country level (OECD [ 2004 ]) and were, for example for math and problem solving scores, interpreted in two ways: (1) If students’ perform better in math than in problem solving, they would “have a better grasp of mathematics content […] after accounting for the level of generic problem-solving skills…” (OECD [ 2004 ], p. 55); (2) If students’ estimated problem-solving competence is higher than their estimated math competence, “… this may suggest that students have the potential to achieve better results in mathematics than that reflected in their current performance…” (OECD [ 2004 ], p. 55). Whilst the latter discrepancy constitutes a capability under-utilisation in math, the former suggests challenges in utilising knowledge and skills acquired in domain-specific contexts in domain-unspecific contexts (i.e., transfer problem).
To quantify the degree to which students are able to transfer their problem solving capabilities from domain-specific problems in math or science to cross-curricular problems, we introduce the Capability Under-Utilisation Index (CUUI) as the relative difference between math or science and problem solving scores:
A positive CUUI indicates that the subject-specific education (i.e., math or science) in a country tends to under-utilise its students’ capabilities to problem solve. A negative CUUI indicates that a country’s educational system fails to fully utilise its students’ capabilities to acquire math and science literacy in the development of problem solving. The CUUI reflects the relative discrepancies between the achievement scores in different domains a .
The concept of math-science coherence
In light of the conceptual and empirical discussion on the relationship between math, science, and problem solving competence, we introduce the concept of math-science coherence as follows: First, math-science coherence refers to the set of cognitive processes involved in both subjects and thus represents processes which are related to reasoning and information processing, relatively independent from domain-specific knowledge. Second, math-science coherence reflects the degree to which math and science education is harmonized as a feature of the educational environment in a country. This interpretation is based on the premise that PISA measures students’ competence as educational outcomes (OECD [ 2004 ]). The operationalization of math-science coherence is realized by means of the correlation between math and science scores [ r (M,S)] at the country level. Low math-science coherence indicates that students who are successful in the acquisition of knowledge and skills in math are not necessarily successful in the acquisition of knowledge and skills in science and vice versa.
On the basis of this conceptualization of math-science coherence, we expect a significant and positive relation to problem solving scores, since the conceptual overlap between mathematical and scientific literacy refers to cognitive abilities such as reasoning and information processing that are also required in problem solving (Arts et al. [ 2006 ]; Beckmann [ 2001 ]; Wüstenberg et al. [ 2012 ]). Hence, we assert that math-science coherence facilitates the transfer of knowledge, skills, and insights across subjects resulting in better problem solving performance (OECD [ 2004 ]; Pellegrino and Hilton [ 2012 ]).
We also assume that math-science coherence as well as capability utilisation is linked to characteristics of the educational system of a country. For instance, as Janssen and Geiser ([ 2012 ]) and Blömeke et al. ([ 2011 ]) suggested, the developmental status of a country, measured by the Human Development Index (HDI; UNDP [ 2005 ]), is positively related to students’ academic achievements as well as to teachers’ quality of teaching. Furthermore, the socio-economic status of a country co-determines characteristics of its educational system, which ultimately affects a construct referred to as national intelligence (Lynn and Meisenberg [ 2010 ]). Research also indicated that curricular settings and educational objectives are related to school achievement in general (Bulle [ 2011 ]; Martin et al. [ 2004 ]). Besides these factors, school- and classroom-related characteristics might also confound the relation between math-science coherence and problem solving. For instance, the schools’ autonomy in developing curricula and managing educational resources might facilitate the incorporation of inquiry- and problem-based activities in science lessons (Chiu and Chow [ 2011 ]). These factors have been discussed as being influential to students’ competence development (OECD [ 2004 ], [ 2005 ]). Ewell ([ 2012 ]) implies that cross-national differences in problem solving competence might be related to differences in education and in using appropriate teaching material. These factors potentially confound the relation between math-science coherence and problem solving.
Discrepancies between math and problem solving scores are discussed in relation to quality of education. Although research has found that crossing the borders between STEM subjects positively affects students’ STEM competences (e.g., National Research Council NRC [ 2011 ]), we argue that the PISA analyses have fallen short in explaining cross-country differences in the development of problem solving competence, since they ignored the link between math and science competences and the synergistic effect of learning universally applicable problem-solving skills in diverse subject areas. Hence, we use the concept of math-science coherence to provide a more detailed description of the discrepancies between problem solving and domain-specific competences. In this regard, we argue that the coherence concept indicates the synergistic potential and students’ problem-solving competence the success of transfer.
The present study
The current study is based on the premise that in contrast to math and science competence problem solving competence is not explicitly taught as a subject at school. Problem solving competence, however, is an expected outcome of education (van Merriënboer [ 2013 ]). With the first step in our analyses, we seek to establish whether math and science education are in fact main contributors to the acquisition of problem solving competence. On the basis of this regression hypothesis, we subsequently focus on the question whether there are significant and systematic differences between countries ( Moderation-Hypothesis ). In light of the conceptual overlap due to cognitive processes involved in dealing with math, science and problem solving tasks and the shared item format employed in the assessments, we expect math and science competence scores to substantially predict scores in problem solving competence. Furthermore, since math and science education are differently organized across the 41 countries participating in the PISA 2003 study, differences in the contribution are also expected.
On the basis of these premises, we introduce the concept of math-science coherence, operationalised as the correlation between math and science scores [ r (M,S)], and analyse its relationship to problem solving and the effects of confounders (i.e., country characteristics) as a step of validation. Since math, science, and problem solving competence show a conceptual overlap, we expect problem solving and math-science coherence to be positively related. Countries’ educational systems differ in numerous aspects, their educational structure, and their educational objectives. Countries also differ with regard to the frequency of assessments, the autonomy of schools in setting up curricula and resources, and the educational resources available. Consequently, we expect the relation between math-science coherence and problem solving competence to be confounded by society-, curriculum-, and school-related factors ( Confounding-Hypothesis ).
In a final step, we aim to better understand the mechanisms with which math and science education contributes to the acquisition of problem-solving competence by exploring how math-science coherence, capability utilisation, and problem solving competence are related. We thus provide new insights into factors related to the transfer between students’ domain-specific and cross-curricular knowledge and skills ( Capability-Utilisation Hypothesis ).
In PISA 2003, a total sample of N = 276,165 students (49.4% female) from 41 countries participated. The entire sample was randomly selected by applying a two-step sampling procedure: First, schools were chosen within a country. Second, students were chosen within these schools. This procedure consequently led to a clustered structure of the data set, as students were nested in 10,175 schools. On average, 27 students per school were chosen across schools within countries. Students’ mean age was 15.80 years ( SD = 0.29 years) ranging from 15.17 to 16.25 years.
In the PISA 2003 study, different assessments were used in order to measure students’ competence in math, science, and problem solving. These assessments were administered as paper-and-pencil tests within a multi-matrix design (OECD [ 2005 ]). In this section, the assessments and further constructs are described that served as predictors of the contribution of math and science competence to problem solving at the country level.
Student achievement in math, science, and problem solving
In order to assess students’ competence to solve cross-curricular problems (i.e., analytical problem solving requiring information retrieval and reasoning), students had to work on an analytical problem-solving test. This test comprised a total of 19 items (7 items referred to trouble-shooting, 7 items referred to decision-making, and 5 items referred to system analysis and design; see OECD [ 2004 ]). Items were coded according to the PISA coding scheme, resulting in dichotomous and polytomous scores (OECD [ 2005 ]). Based on these scores, models of item response theory were specified in order to obtain person and item parameters (Leutner et al. [ 2012 ]). The resulting plausible values could be regarded as valid indicators of students’ abilities in problem solving (Wu [ 2005 ]). The problem solving test showed sufficient reliabilities between .71 and .89 for the 41 countries.
To assess mathematical literacy as an indicator of math competence , an 85-items test was administered (for details, refer to OECD [ 2003 ]). Responses were dichotomously or polytomously scored. Again, plausible values were obtained as person ability estimates and reliabilities were good (range: 0.83 – 0.93). In the context of mathematical literacy, students were asked to solve real-world problems by applying appropriate mathematical models. They were prompted to “identify and understand the role mathematics plays in the world, to make well-founded judgements and to use […] mathematics […]” (OECD [ 2003 ], p. 24).
Scientific literacy as a proxy for science competence was assessed by using problems referring to different content areas of science in life, health, and technology. The reliability estimates for the 35 items in this test ranged between .68 and .88. Again, plausible values served as indicators of this competence.
Country-specific characteristics
In our analyses, we incorporated a range of country-specific characteristics that can be subdivided into three main categories. These are: society-related factors, curriculum-related factors, and school-related factors. Country-specific estimates of National Intelligence as derived by Lynn and Meisenberg ([ 2010 ]) as well as the Human Development Index (HDI) were subsumed under society-related factors . The HDI incorporates indicators of a country’s health, education, and living standards (UNDP [ 2005 ]). Both variables are conceptualised as factors that contribute to country-specific differences in academic performance.
Holliday and Holliday ([ 2003 ]) emphasised the role of curricular differences in the understanding of between-country variance in test scores. We incorporated two curriculum-related factors in our analyses. First, we used Bulle’s ([ 2011 ]) classification of curricula into ‘progressive’ and ‘academic’. Bulle ([ 2011 ]) proposed this framework and classified the PISA 2003 countries according to their educational model. In her framework, she distinguishes between ‘academic models’ which are primarily geared towards teaching academic subjects (e.g., Latin, Germanic, and East-Asian countries) and ‘progressive models’ which focus on teaching students’ general competence in diverse contexts (e.g., Anglo-Saxon and Northern countries). In this regard, academic skills refer to the abilities of solving academic-type problems, whereas so called progressive skills are needed in solving real-life problems (Bulle [ 2011 ]). It can be assumed that educational systems that focus on fostering real-life and domain-general competence might be more conducive to successfully tackling the kind of problem solving tasks used in PISA (Kind [ 2013 ]). This classification of educational systems should be seen as the two extreme poles of a continuum rather than as a strict dichotomy. In line with the reflections above, we would argue that academic and progressive skills are not exclusively distinct, since both skills utilise sets of cognitive processes that largely overlap (Klahr and Dunbar [ 1988 ]). The fact that curricular objectives in some countries are shifting (e.g., in Eastern Asia) makes a clear distinction between both models even more difficult. Nonetheless, we will use this form of country-specific categorization based on Bulle’s model in our analyses.
Second, we considered whether countries’ science curricula were ‘integrated’ or ‘not integrated’ (Martin et al. [ 2004 ]). In this context, integration refers to linking multiple science subjects (biology, chemistry, earth science, and physics) to a unifying theme or issue (cf. Drake and Reid [ 2010 ], p. 1).
In terms of school-related factors, we used the PISA 2003 scales of ‘Frequency of assessments in schools’, ‘Schools’ educational resources’, and ‘School autonomy towards resources and curricula’ from the school questionnaire. Based on frequency and rating scales, weighted maximum likelihood estimates (WLE) indicated the degree to which schools performed in these scales (OECD [ 2005 ]).
The country-specific characteristics are summarized in the Table 1 .
The PISA 2003 assessments utilised a randomized incomplete block design to select different test booklets which covered the different content areas of math, science, and problem solving (Brunner et al. [ 2013 ]; OECD [ 2005 ]). The test administration took 120 minutes, and was managed for each participating country separately. It was established that quality standards of the assessment procedure were high.
Statistical analyses
In PISA 2003, different methods of obtaining person estimates with precise standard errors were applied. The most accurate procedure produced five plausible values, which were drawn from a person ability distribution (OECD [ 2005 ]). To avoid missing values in these parameters and to obtain accurate estimates, further background variables were used within the algorithms (Wu [ 2005 ]). The resulting plausible values were subsequently used as indicators of students’ competence in math, science, and problem solving. By applying Rubin’s combination rules (Bouhilila and Sellaouti [ 2013 ]; Enders [ 2010 ]), analyses were replicated with each of the five plausible values and then combined. In this multiple imputation procedure, standard errors were decomposed to the variability across and within the five imputations (Enders [ 2010 ]; OECD [ 2005 ]; Wu [ 2005 ]).
Within the multilevel regression analyses for each country, we specified the student level as level 1 and the school level as level 2. Since PISA 2003 applied a random sampling procedure at the student and the school level, we decided to control for the clustering of data at these two levels (OECD [ 2005 ]). In addition to this two-level procedure, we regarded the 41 countries as multiple groups (fixed effects). This decision was based on our assumption that the countries selected in PISA 2003 did not necessarily represent a sample of a larger population (Martin et al. [ 2012 ]). Moreover, we did not regard the effects of countries as interchangeable, because, given the specific characteristics of education and instruction within countries; we argue that the effects of competences in mathematics and science on problem solving have their own distinct interpretation in each country (Snijders and Bosker [ 2012 ]). The resulting models were compared by taking into account the Akaike’s information criteria ( AIC ), Bayesian information criteria ( BIC ), and the sample-size adjusted BIC . Also, a likelihood ratio test of the log-Likelihood values ( LogL ) was applied (Hox [ 2010 ]).
To test the Moderation-Hypothesis, we first specified a two-level regression model with problem solving scores as outcomes at the student level (level 1), which allowed variance in achievement scores across schools (level 2). In this model, math and science scores predicted problem solving scores at the student level. To account for differences in the probabilities of being selected as a student within the 41 countries and to adjust the standard errors of regression parameters, we used the robust maximum likelihood (MLR) estimator and students’ final weights (see also Brunner et al. [ 2013 ]; OECD [ 2005 ]). All analyses were conducted in Mplus 6.0 by using the TYPE = IMPUTATION option (Muthén and Muthén [ 2010 ]). As Hox ([ 2010 ]) suggested, using multilevel regression models without taking into account the clustering of data in schools often leads to biased estimates, since achievement variables often have substantial variance at the school level. Consequently, we allowed for level-2-variance within the scores.
After having established whether success in math and science education contributes to the development in problem solving competence across the 41 countries, we then tested whether cross-country differences in the unstandardized regression coefficients were statistically significant by using a multi-group regression model, in which the coefficients were constrained to be equal across countries. We compared this model with the freely estimated model.
Finally, we conceptualized the correlation between math and science scores as an indicator of the level of coherence in math and science education in a country. In relation to the Confounding-Hypothesis, we tested country-specific characteristics for their potentially confounding effects on the relation between math-science coherence and problem solving competence. Following the recommendations proposed by (MacKinnon et al. [ 2000 ]), the confounding analysis was conducted in two steps: (1) we estimated two regression equations. In the first equation, problem solving scores across the 41 countries were regressed on math-science coherence. In the second equation, the respective country characteristics were added as further predictors; (2) the difference between the regression coefficients for math-science coherence obtained in either equation represented the magnitude of a potential confounder effect.
Lastly, we tested the Capability-Utilisation Hypothesis by investigating the bivariate correlations among the CUU Indices and math-science coherence.
Regressing problem solving on math and science performance
To test the Moderation-Hypothesis, we specified regression models with students’ problem-solving score as the outcome and math and science scores as predictors for each of the 41 countries. Due to the clustering of data in schools, these models allowed for between-level variance. Intraclass correlations (ICC-1) for math, science, and problem solving performance ranged between .03 and .61 for the school level ( M = .33, SD = .16).
We specified multilevel regression models for each country separately. These results are reported in Table 2 . The regression coefficients for math on problem solving ranged from .53 to .82 with an average of M( β Math ) = .67 ( SD = .06). The average contribution of science towards problem solving was M( β Science ) = .16 ( SD = .09, Min = -.06, Max = .30). The combination of the distributions of both parameters resulted in substantial differences in the variance explanations of the problem solving scores across the 41 countries ( M[R 2 ] = .65, SD = .15, Min = .27, Max = .86). To test whether these differences were statistically significant, we constrained the regression coefficients of math and science competence within the multi-group regression model to be equal across the 41 countries. Compared to the freely estimated model ( LogL = -4,561,273.3, df = 492, AIC = 9,123,530.5, BIC = 9,128,410.7), the restricted model was empirically not preferred LogL = -4,564,877.9, df = 412, AIC = 9,130,579.8, BIC = 9,134,917.6; Δχ 2 [80] = 7,209.2, p < .001. These findings lend evidence for the Moderation-Hypothesis.
From a slightly different perspective, the country-specific amount of variance in problem solving scores that is explained by the variation in math and science performance scores ( R 2 ) is strongly associated with the country’s problem solving score ( r = .77, p < .001), which suggests that the contribution of science and math competence to the acquisition of problem solving competence was significantly lower in low-performing countries.
As shown in Table 2 , the regression weights of math and science were significant for all but two countries. Across countries the regression weight for math tended to be higher than the regression weight for science when predicting problem solving competence. This finding indicates a stronger overlap between students’ competences in mathematics and problem solving on the one hand and similarities between the assessments in both domains on the other hand.
Validating the concept of math-science coherence
In order to validate the concept of math-science coherence, which is operationalised as the correlation between math and science scores [ r (M,S)], we explored its relation to problem solving and country characteristics.
Regarding the regression outcomes shown in Table 2 , it is apparent that math-science coherence varied considerably across countries, ranging from .39 to .88 with an average of M(r) = .70 ( SD = .13). Interestingly, countries’ level of coherence in math-science education was substantially related to their problem solving scores ( r = .76, p < .001). An inspection of Figure 1 reveals that this effect was mainly due to countries that both achieve low problem solving scores and show relatively low levels of math-science coherence (see bottom left quadrant in Figure 1 ), whilst amongst the remaining countries the correlational link between math-science coherence and problem solving score was almost zero ( r = -.08, p = .71) b . This pattern extends the moderation perspective on the presumed dependency of problem solving competence from math and science competences.
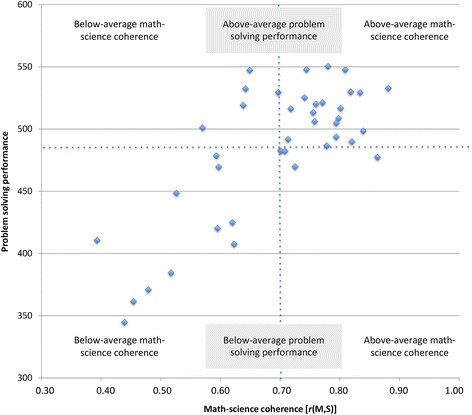
The relation between math-science coherence and problem solving performance across the 41 countries.
As a result of the moderator analysis, we know that countries not only differ in regard to their average problem-solving scores and level of coherence between math and science, countries also differ in the strengths with which math-science coherence predicts problem solving scores. To better understand the conceptual nature of the link between math-science coherence and problem solving, we now attempt to adjust this relationship for potential confounding effects that country-specific characteristics might have. To this end, we employed linear regression and path analysis with students’ problem-solving scores as outcomes, math-science coherence (i.e., r [M,S]) as predictor, and country characteristics as potential confounders.
To establish whether any of the country characteristics had a confounding effect on the link between math-science coherence and problem solving competence, two criteria had to be met: (1) a reduction of the direct effect of math-science coherence on problem solving scores, and (2) testing the difference between the direct effect within the baseline Model M0 and the effect with the confounding Model M1 (Table 3 ).
Regarding the society-related factors, both the countries’ HDI and their national intelligence were confounders with a positive effect. Furthermore, the countries’ integration of the science curriculum was also positively related to the problem solving performance. Finally, the degree of schools’ autonomy towards educational resources and the implementation of curricula and the frequency of assessments were school-related confounders, the former with a positive effect whilst the latter represents a negative confounder. The direct effect of math-science coherence to problem solving decreased and thus indicated that confounding was present (MacKinnon et al. [ 2000 ]).
These findings provide evidence on the Confounding-Hypothesis and support our expectations on the relation between math-science coherence, problem solving, and country characteristics. We regard these results as evidence for the validity of the math-science coherence measure.
Relating math-science coherence to the capability under-utilisation indices
To advance our understanding of the link between math-science coherence and problem solving scores, we tested the Capability-Utilisation Hypothesis. To this end, we explored the relationship between math-science coherence and the CUU Indices for math and science, respectively. For math competence the average Capability Under-Utilisation Index was rather neutral with M CUUI-Math = -0.001 ( SD = 0.02). This suggests that, on average, all countries sufficiently utilise their students’ math capabilities in facilitating the development of problem solving competence (i.e., transfer). It also suggests that math education across participating countries tends to sufficiently utilise generic problem-solving skills (Figure 2 ). The picture is different for science education. Here, the Capability Under-Utilisation Indices and their variation across the participating countries ( M CUUI-Science = -0.01, SD = 0.04) suggest that in a range of countries knowledge and skills taught in science education tend to be under-utilised in the facilitation of the acquisition of problem solving competence (Figure 3 ).
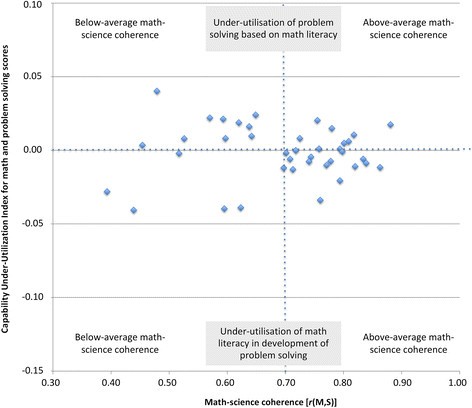
The relation between math-science coherence and the capability under-utilisation index for math and problem solving scores across the 41 countries.
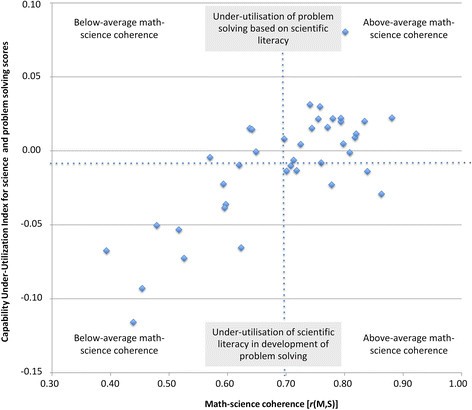
The relation between math-science coherence and the capability under-utilisation index for science and problem solving scores across the 41 countries.
For math competence, the relative difference to problem solving was not related to math-science coherence ( r = .02, p = .89; Figure 2 ). In contrast, the Capability Under-Utilisation Index for science showed a strong positive correlation with math-science coherence ( r = .76, p < .001; Figure 3 ), indicating that low levels of coherence between math and science education were associated with a less effective transfer of domain-specific knowledge and skills to problem solving.
The present study was aimed at investigating the differences in the contribution of math and science competence to problem solving competence across the 41 countries that participated in the PISA 2003 study (Moderation-Hypothesis). To this end, we proposed the concept of math-science coherence and explored its relationship to problem solving competence and how this relationship is confounded by country characteristics (Confounding-Hypothesis). To further extend our understanding of the link between math-science coherence and problem solving, we introduced the concept of capability-utilisation. Testing the Capability-Utilisation Hypothesis enabled us to identify what may contribute to varying levels of math-science coherence and ultimately the development of problem solving competence.
The contribution of math and science competence across countries
Regarding the prediction of problem solving competence, we found that in most countries, math and science competence significantly contributed to students’ performance in analytical problem solving. This finding was expected based on the conceptualizations of mathematical and scientific literacy within the PISA framework referring to shared cognitive processes such as information processing and reasoning (Kind [ 2013 ]; OECD [ 2005 ]), which are regarded as components of problem solving (Bybee [ 2004 ]; Klahr and Dunbar [ 1988 ]; Mayer [ 2010 ]).
It is noteworthy that, for some of the below-average performing countries, science competence did not significantly contribute to the prediction of problem solving competence. It can be speculated that education in these countries is more geared towards math education and modelling processes in mathematical scenarios, whilst the aspect of problem solving in science is less emphasised (Janssen and Geiser [ 2012 ]). The results of multilevel regression analyses supported this interpretation by showing that math competence was a stronger predictor of problem solving competence. On the one hand, this finding could be due to the design of the PISA tests (Adams [ 2005 ]), since math and problem solving items are designed in such a way that modelling real-life problems is required, whereas science items are mostly domain-specific and linked to science knowledge (Nentwig et al. [ 2009 ]; OECD [ 2004 ]). Moreover, one may argue that math and problem solving items allow students to employ different solution strategies, whereas science items offer fewer degrees of freedom for test takers (Nentwig et al. [ 2009 ]). In particular, the shared format of items in math, science, and problem solving may explain an overlap between their cognitive demands. For instance, most of the items were designed in such a way that students had to extract and identify relevant information from given tables or figures in order to solve specific problems. Hence, these items were static and did not require knowledge generation by interaction or exploration but rather the use of given information in problem situations (Wood et al. [ 2009 ]). In contrast to the domain-specific items in math and science, problem solving items did not require the use of prior knowledge in math and science (OECD [ 2004 ]). In addition, some of the math and science items involved cognitive operations that were specific to these domains. For instance, students had to solve a number of math items by applying arithmetic and combinatorial operations (OECD [ 2005 ]). Finally, since items referred to contextual stimuli, which were presented in textual formats, reading ability can be regarded as another, shared demand of solving the items. Furthermore, Rindermann ([ 2007 ]) clearly showed that the shared demands of the achievement tests in large-scale assessments such as PISA were strongly related to students’ general reasoning skills. This finding is in line with the strong relations between math, science, and problem solving competence, found in our study. The interpretation of the overlap between the three competences can also be interpreted from a conceptual point of view. In light of the competence frameworks in PISA, we argue that there are a number of skills that can be found in math, science, and problem solving: information retrieval and processing, knowledge application, and evaluation of results (Griffin et al. [ 2012 ]; OECD [ 2004 ], [ 2005 ]). These skills point out to the importance of reasoning in the three domains (Rindermann [ 2007 ]). Thus, the empirical overlap between math and problem solving can be explained by shared processes of, what Mayer ([ 2010 ]) refers to as, informal reasoning. On the other hand, the stronger effect of math competence could be an effect of the quality of math education. Hiebert et al. ([ 1996 ]) and Kuo et al. ([ 2013 ]) suggested that math education is more based on problem solving skills than other subjects in school (e.g., Polya [ 1945 ]). Science lessons, in contrast, are often not necessarily problem-based, despite the fact that they often start with a set problem. Risch ([ 2010 ]) showed in a cross-national review that science learning was more related to contents and contexts rather than to generic problem-solving skills. These tendencies might lead to a weaker contribution of science education to the development of problem solving competence (Abd-El-Khalick et al. [ 2004 ]).
In sum, we found support on the Moderation-Hypothesis, which assumed systematic differences in the contribution of math and science competence to problem solving competence across the 41 PISA 2003 countries.
The relation to problem solving
In our study, we introduced the concept of math-science coherence, which reflects the degree to which math and science education are harmonized. Since mathematical and scientific literacy show a conceptual overlap, which refers to a set of cognitive processes that are linked to reasoning and information processing (Fensham and Bellocchi [ 2013 ]; Mayer [ 2010 ]), a significant relation between math-science coherence and problem solving was expected. In our analyses, we found a significant and positive effect of math-science coherence on performance scores in problem solving. In this finding we see evidence for the validity of this newly introduced concept of math-science coherence and its focus on the synergistic effect of math and science education on problem solving. The results further suggest that higher levels of coordination between math and science education has beneficial effects on the development of cross-curricular problem-solving competence (as measured within the PISA framework).
Confounding effects of country characteristics
As another step of validating the concept of math-science coherence, we investigated whether country-specific characteristics that are linked to society-, curriculum-, and school-related factors confounded its relation to problem solving. Our results showed that national intelligence, the Human Development Index, the integration of the science curriculum, and schools’ autonomy were positively linked to math-science coherence and problem solving, whilst a schools’ frequency of assessment had a negative confounding effect.
The findings regarding the positive confounders are in line with and also extend a number of studies on cross-country differences in education (e.g., Blömeke et al. [ 2011 ]; Dronkers et al. [ 2014 ]; Janssen and Geiser [ 2012 ]; Risch [ 2010 ]). Ross and Hogaboam-Gray ([ 1998 ]), for instance, found that students benefit from an integrated curriculum, particularly in terms of motivation and the development of their abilities. In the context of our confounder analysis, the integration of the science curriculum as well as the autonomy to allocate resources is expected to positively affect math-science coherence. At the same time, an integrated science curriculum with a coordinated allocation of resources may promote inquiry-based experiments in science courses, which is assumed to be beneficial for the development of problem solving within and across domains. Teaching science as an integrated subject is often regarded a challenge for teachers, particularly when developing conceptual structures in science lessons (Lang and Olson, [ 2000 ]), leading to teaching practices in which cross-curricular competence is rarely taken into account (Mansour [ 2013 ]; van Merriënboer [ 2013 ]).
The negative confounding effect of assessment frequency suggests that high frequencies of assessment, as it presumably applies to both math and science subjects, contribute positively to math-science coherence. However, the intended or unintended engagement in educational activities associated with assessment preparation tends not to be conducive to effectively developing domain-general problem solving competence (see also Neumann et al. [ 2012 ]).
The positive confounder effect of HDI is not surprising as HDI reflects a country’s capability to distribute resources and to enable certain levels of autonomy (Reich et al. [ 2013 ]). To find national intelligence as a positive confounder is also to be expected as the basis for its estimation are often students’ educational outcome measures (e.g., Rindermann [ 2008 ]) and, as discussed earlier, academic achievement measures share the involvement of a set of cognitive processes (Baumert et al. [ 2009 ]; OECD [ 2004 ]).
In summary, the synergistic effect of a coherent math and science education on the development of problem solving competence is substantially linked to characteristics of a country’s educational system with respect to curricula and school organization in the context of its socio-economic capabilities. Math-science coherence, however, also is linked to the extent to which math or science education is able to utilise students’ educational capabilities.
Math-science coherence and capability-utilisation
So far, discrepancies between students’ performance in math and problem solving or science and problem solving have been discussed as indicators of students’ capability utilisation in math or science (Leutner et al. [ 2012 ]; OECD [ 2004 ]). We have extended this perspective by introducing Capability Under-Utilisation Indices for math and science to investigate the effectiveness with which knowledge and skills acquired in the context of math or science education are transferred into cross-curricular problem-solving competence. The Capability Under-Utilisation Indices for math and science reflect a potential quantitative imbalance between math, science, and problem solving performance within a country, whilst the also introduced concept of math-science coherence reflects a potential qualitative imbalance between math and science education.
The results of our analyses suggest that an under-utilisation of problem solving capabilities in the acquisition of science literacy is linked to lower levels of math-science coherence, which ultimately leads to lower scores in problem solving competence. This interpretation finds resonance in Ross and Hogaboam-Gray’s ([ 1998 ]) argumentation for integrating math and science education and supports the attempts of math and science educators to incorporate higher-order thinking skills in teaching STEM subjects (e.g., Gallagher et al. [ 2012 ]; Zohar [ 2013 ]).
In contrast, the CUU Index for math was not related to math-science coherence in our analyses. This might be due to the conceptualizations and assessments of mathematical literacy and problem solving competence. Both constructs share cognitive processes of reasoning and information processing, resulting in quite similar items. Consequently, the transfer from math-related knowledge and skills to cross-curricular problems does not necessarily depend on how math and science education are harmonised, since the conceptual and operational discrepancy between math and problem solving is rather small.
Math and science education do matter to the development of students’ problem-solving skills. This argumentation is based on the assumption that the PISA assessments in math, science, and problem solving are able to measure students’ competence as outcomes, which are directly linked to their education (Bulle [ 2011 ]; Kind [ 2013 ]). In contrast to math and science competence, problem solving competence is not explicitly taught as a subject. Problem solving competence requires the utilisation of knowledge and reasoning skills acquired in specific domains (Pellegrino and Hilton [ 2012 ]). In agreement with Kuhn ([ 2009 ]), we point out that this transfer does not happen automatically but needs to be actively facilitated. In fact, Mayer and Wittrock ([ 2006 ]) stressed that the development of transferable skills such as problem solving competence needs to be fostered within specific domains rather than taught in dedicated, distinct courses. Moreover, they suggested that students should develop a “repertoire of cognitive and metacognitive strategies that can be applied in specific problem-solving situations” (p. 299). Beyond this domain-specific teaching principle, research also proposes to train the transfer of problem solving competence in domains that are closely related (e.g., math and science; Pellegrino and Hilton [ 2012 ]). In light of the effects of aligned curricula (as represented by the concept of math-science coherence), we argue that educational efforts to increase students’ problem solving competence may focus on a coordinated improvement of math and science literacy and fostering problem solving competence within math and science. The emphasis is on coordinated, as the results of our analyses indicated that the coherence between math and science education, as a qualitative characteristic of a country’s educational system, is a strong predictor of problem solving competence. This harmonisation of math and science education may be achieved by better enabling the utilisation of capabilities, especially in science education. Sufficiently high levels of math-science coherence could facilitate the emergence of educational synergisms, which positively affect the development of problem solving competence. In other words, we argue for quantitative changes (i.e., improve science attainment) in order to achieve qualitative changes (i.e., higher levels of curriculum coherence), which are expected to create effective transitions of subject-specific knowledge and skills into subject-unspecific competences to solve real-life problems (Pellegrino and Hilton [ 2012 ]; van Merriënboer [ 2013 ]).
Finally, we encourage research that is concerned with the validation of the proposed indices for different forms of problem solving. In particular, we suggest studying the facilities of the capability-under-utilisation indices for analytical and dynamic problem solving, as assessed in the PISA 2012 study (OECD [ 2014 ]). Due to the different cognitive demands in analytical and dynamic problems (e.g., using existing knowledge vs. generating knowledge; OECD [ 2014 ]), we suspect differences in capability utilisation in math and science. This research could provide further insights into the role of 21 st century skills as educational goals.
a The differences between students’ achievement in mathematics and problem solving, and science and problem solving have to be interpreted relative to the OECD average, since the achievement scales were scaled with a mean of 500 and a standard deviation of 100 for the OECD countries (OECD [ 2004 ], p. 55). Although alternative indices such as country residuals may also be used in cross-country comparisons (e.g., Olsen [ 2005 ]), we decided to use CUU indices, as they reflect the actual differences in achievement scores.
b In addition, we checked whether this result was due to the restricted variances in low-performing countries and found that neither ceiling nor floor effects in the problem solving scores existed. The problem solving scale differentiated sufficiently reliably in the regions below and above the OECD mean of 500.
Abd-El-Khalick F, Boujaoude S, Duschl R, Lederman NG, Mamlok-Naaman R, Hofstein A, Niaz M, Treagust D, Tuan H-L: Inquiry in science education: International perspectives. Science Education 2004, 88: 397–419. doi:10.1002/sce.10118 10.1002/sce.10118
Article Google Scholar
Adams RJ: Reliability as a measurement design effect. Studies in Educational Evaluation 2005, 31: 162–172. doi:10.1016/j.stueduc.2005.05.008 10.1016/j.stueduc.2005.05.008
Arts J, Gijselaers W, Boshuizen H: Understanding managerial problem solving, knowledge use and information processing: Investigating stages from school to the workplace. Contemporary Educational Psychology 2006, 31: 387–410. doi:10.1016/j.cedpsych.2006.05.005 10.1016/j.cedpsych.2006.05.005
Bassok M, Holyoak K: Pragmatic knowledge and conceptual structure: determinants of transfer between quantitative domains. In Transfer on trial: intelligence, cognition, and instruction . Edited by: Detterman DK, Sternberg RJ. Ablex, Norwood, NJ; 1993:68–98.
Google Scholar
Baumert J, Lüdtke O, Trautwein U, Brunner M: Large-scale student assessment studies measure the results of processes of knowledge acquisition: evidence in support of the distinction between intelligence and student achievement. Educational Research Review 2009, 4: 165–176. doi:10.1016/j.edurev.2009.04.002 10.1016/j.edurev.2009.04.002
Beckmann JF: Zur Validierung des Konstrukts des intellektuellen Veränderungspotentials [On the validation of the construct of intellectual change potential] . logos, Berlin; 2001.
Blömeke S, Houang R, Suhl U: TEDS-M: diagnosing teacher knowledge by applying multidimensional item response theory and multiple-group models. IERI Monograph Series: Issues and Methodologies in Large-Scale Assessments 2011, 4: 109–129.
Bouhilila D, Sellaouti F: Multiple imputation using chained equations for missing data in TIMSS: a case study. Large-scale Assessments in Education 2013, 1: 4.
Brunner M, Gogol K, Sonnleitner P, Keller U, Krauss S, Preckel F: Gender differences in the mean level, variability, and profile shape of student achievement: Results from 41 countries. Intelligence 2013, 41: 378–395. doi:10.1016/j.intell.2013.05.009 10.1016/j.intell.2013.05.009
Bulle N: Comparing OECD educational models through the prism of PISA. Comparative Education 2011, 47: 503–521. doi: 10.1080/03050068.2011.555117 10.1080/03050068.2011.555117
Bybee R: Scientific Inquiry and Science Teaching. In Scientific Inquiry and the Nature of Science . Edited by: Flick L, Lederman N. Springer & Kluwers, New York, NY; 2004:1–14. doi:10.1007/978–1-4020–5814–1_1
Chiu M, Chow B: Classroom discipline across forty-One countries: school, economic, and cultural differences. Journal of Cross-Cultural Psychology 2011, 42: 516–533. doi:10.1177/0022022110381115 10.1177/0022022110381115
Drake S, Reid J: Integrated curriculum: Increasing relevance while maintaining accountability. Research into Practice 2010, 28: 1–4.
Dronkers J, Levels M, de Heus M: Migrant pupils’ scientific performance: the influence of educational system features of origin and destination countries. Large-scale Assessments in Education 2014, 2: 3.
Enders C: Applied Missing Data Analysis . The Guilford Press, New York, NY; 2010.
Ewell P: A world of assessment: OECD’s AHELO initiative. Change: The Magazine of Higher Learning 2012, 44: 35–42. doi:10.1080/00091383.2012.706515 10.1080/00091383.2012.706515
Fensham P, Bellocchi A: Higher order thinking in chemistry curriculum and its assessment. Thinking Skills and Creativity 2013, 10: 250–264. doi:10.1016/j.tsc.2013.06.003 10.1016/j.tsc.2013.06.003
Gallagher C, Hipkins R, Zohar A: Positioning thinking within national curriculum and assessment systems: perspectives from Israel, New Zealand and Northern Ireland. Thinking Skills and Creativity 2012, 7: 134–143. doi:10.1016/j.tsc.2012.04.005 10.1016/j.tsc.2012.04.005
Greiff S, Holt D, Funke J: Perspectives on problem solving in educational assessment: analytical, interactive, and collaborative problem solving. The Journal of Problem Solving 2013, 5: 71–91. doi:10.7771/1932–6246.1153 10.7771/1932-6246.1153
Griffin P, Care E, McGaw B: The changing role of education and schools. In Assessment and Teaching of 21st Century Skills . Edited by: Griffin P, McGaw B, Care E. Springer, Dordrecht; 2012:1–15. 10.1007/978-94-007-2324-5_1
Chapter Google Scholar
Hickendorff M: The language factor in elementary mathematics assessments: Computational skills and applied problem solving in a multidimensional IRT framework. Applied Measurement in Education 2013, 26: 253–278. doi:10.1080/08957347.2013.824451 10.1080/08957347.2013.824451
Hiebert J, Carpenter T, Fennema E, Fuson K, Human P, Murray H, Olivier A, Wearne D: Problem Solving as a Basis for Reform in Curriculum and Instruction: The Case of Mathematics. Educational Researcher 1996, 25: 12–21. doi:10.3102/0013189X025004012 10.3102/0013189X025004012
Holliday W, Holliday B: Why using international comparative math and science achievement data from TIMSS is not helpful. The Educational Forum 2003, 67: 250–257. 10.1080/00131720309335038
Hox J: Multilevel Analysis . 2nd edition. Routlegde, New York, NY; 2010.
Janssen A, Geiser C: Cross-cultural differences in spatial abilities and solution strategies: An investigation in Cambodia and Germany. Journal of Cross-Cultural Psychology 2012, 43: 533–557. doi:10.1177/0022022111399646 10.1177/0022022111399646
Jonassen D: Learning to solve problems . Routledge, New York, NY; 2011.
Kind P: Establishing Assessment Scales Using a Novel Disciplinary Rationale for Scientific Reasoning. Journal of Research in Science Teaching 2013, 50: 530–560. doi:10.1002/tea.21086 10.1002/tea.21086
Klahr D, Dunbar K: Dual Space Search during Scientific Reasoning. Cognitive Science 1988, 12: 1–48. doi:10.1207/s15516709cog1201_1 10.1207/s15516709cog1201_1
Koeppen K, Hartig J, Klieme E, Leutner D: Current issues in competence modeling and assessment. Journal of Psychology 2008, 216: 61–73. doi:10.1027/0044–3409.216.2.61
Kuhn D: Do students need to be taught how to reason? Educational Research Review 2009, 4: 1–6. doi:10.1016/j.edurev.2008.11.001 10.1016/j.edurev.2008.11.001
Kuo E, Hull M, Gupta A, Elby A: How Students Blend Conceptual and Formal Mathematical Reasoning in Solving Physics Problems. Science Education 2013, 97: 32–57. doi:10.1002/sce.21043 10.1002/sce.21043
Lang M, Olson J: Integrated science teaching as a challenge for teachers to develop new conceptual structures. Research in Science Education 2000, 30: 213–224. doi:10.1007/BF02461629 10.1007/BF02461629
Leutner D, Fleischer J, Wirth J, Greiff S, Funke J: Analytische und dynamische Problemlösekompetenz im Lichte internationaler Schulleistungsstudien [Analytical and dynamic problem-solvng competence in international large-scale studies]. Psychologische Rundschau 2012, 63: 34–42. doi:10.1026/0033–3042/a000108 10.1026/0033-3042/a000108
Lynn R, Meisenberg G: National IQs calculated and validated for 108 nations. Intelligence 2010, 38: 353–360. doi:10.1016/j.intell.2010.04.007 10.1016/j.intell.2010.04.007
MacKinnon D, Krull J, Lockwood C: Equivalence of the mediation, confounding, and suppression effect. Prevention Science 2000, 1: 173–181. doi:10.1023/A:1026595011371 10.1023/A:1026595011371
Mansour N: Consistencies and inconsistencies between science teachers’ beliefs and practices. International Journal of Science Education 2013, 35: 1230–1275. doi:10.1080/09500693.2012.743196 10.1080/09500693.2012.743196
Martin M, Mullis I, Gonzalez E, Chrostowski S: TIMSS 2003 International Science Report . IEA, Chestnut Hill, MA; 2004.
Martin AJ, Liem GAD, Mok MMC, Xu J: Problem solving and immigrant student mathematics and science achievement: Multination findings from the Programme for International Student Assessment (PISA). Journal of Educational Psychology 2012, 104: 1054–1073. doi:10.1037/a0029152 10.1037/a0029152
Mayer R: Problem solving and reasoning. In International Encyclopedia of Education . 3rd edition. Edited by: Peterson P, Baker E, McGraw B. Elsevier, Oxford; 2010:273–278. doi:10.1016/B978–0-08–044894–7.00487–5 10.1016/B978-0-08-044894-7.00487-5
Mayer R, Wittrock MC: Problem solving. In Handbook of Educational Psychology . 2nd edition. Edited by: Alexander PA, Winne PH. Lawrence Erlbaum, New Jersey; 2006:287–303.
Muthén B, Muthén L: Mplus 6 . Muthén & Muthén, Los Angeles, CA; 2010.
Successful K-12 STEM Education . National Academies Press, Washington, DC; 2011.
Nentwig P, Rönnebeck S, Schöps K, Rumann S, Carstensen C: Performance and levels of contextualization in a selection of OECD countries in PISA 2006. Journal of Research in Science Teaching 2009, 8: 897–908. doi:10.1002/tea.20338 10.1002/tea.20338
Neumann K, Kauertz A, Fischer H: Quality of Instruction in Science Education. In Second International Handbook of Science Education (Part One . Edited by: Fraser B, Tobin K, McRobbie C. Springer, Dordrecht; 2012:247–258.
The PISA 2003 Assessment Frameworks . OECD, Paris; 2003.
Problem solving for tomorrow’s world . OECD, Paris; 2004.
PISA 2003 Technical Report . OECD, Paris; 2005.
PISA 2012 Results : Creative Problem Solving – Students’ Skills in Tackling Real-Life Problems (Vol. V) . OECD, Paris; 2014.
Olsen RV: An exploration of cluster structure in scientific literacy in PISA: Evidence for a Nordic dimension? NorDiNa. ᅟ 2005, 1 (1):81–94.
Oser F, Baeriswyl F: Choreographies of Teaching: Bridging Instruction to Learning. In Handbook of Research on Teaching . 4th edition. Edited by: Richardson V. American Educational Research Association, Washington, DC; 2001:1031–1065.
Pellegrino JW, Hilton ML: Education for Life and Work – Developing Transferable Knowledge and Skills in the 21st Century . The National Academies Press, Washington, DC; 2012.
Polya G: How to solve it: a new aspect of mathematical method . Princeton University Press, Princeton, NJ; 1945.
Reich J, Hein S, Krivulskaya S, Hart L, Gumkowski N, Grigorenko E: Associations between household responsibilities and academic competencies in the context of education accessibility in Zambia. Learning and Individual Differences 2013, 27: 250–257. doi:10.1016/j.lindif.2013.02.005 10.1016/j.lindif.2013.02.005
Rindermann H: The g-factor of international cognitive ability comparisons: The homogeneity of results in PISA, TIMSS, PIRLS and IQ-tests across nations. European Journal of Personality 2007, 21: 661–706. doi:10.1002/per.634
Rindermann H: Relevance of education and intelligence at the national level for the economic welfare of people. Intelligence 2008, 36: 127–142. doi:10.1016/j.intell.2007.02.002 10.1016/j.intell.2007.02.002
Risch B: Teaching Chemistry around the World . Waxmann, Münster; 2010.
Ross J, Hogaboam-Gray A: Integrating mathematics, science, and technology: effects on students. International Journal of Science Education 1998, 20: 1119–1135. doi:10.1080/0950069980200908 10.1080/0950069980200908
Snijders TAB, Bosker RJ: Multilevel Analysis: An Introduction to Basic and Advanced Multilevel Modeling . 2nd edition. Sage Publications, London; 2012.
Human Development Report 2005 . UNDP, New York, NY; 2005.
Van Merriënboer J: Perspectives on problem solving and instruction. Computers & Education 2013, 64: 153–160. doi:10.1016/j.compedu.2012.11.025 10.1016/j.compedu.2012.11.025
Wood RE, Beckmann JF, Birney D: Simulations, learning and real world capabilities. Education Training 2009, 51 (5/6):491–510. doi:10.1108/00400910910987273 10.1108/00400910910987273
Wu M: The role of plausible values in large-scale surveys. Studies in Educational Evaluation 2005, 31: 114–128. doi:10.1016/j.stueduc.2005.05.005 10.1016/j.stueduc.2005.05.005
Wu M, Adams R: Modelling Mathematics Problem Solving Item Responses using a Multidimensional IRT Model. Mathematics Education Research Journal 2006, 18: 93–113. doi:10.1007/BF03217438 10.1007/BF03217438
Wüstenberg S, Greiff S, Funke J: Complex problem solving: More than reasoning? Intelligence 2012, 40: 1–14. doi:10.1016/j.intell.2011.11.003 10.1016/j.intell.2011.11.003
Zohar A: Scaling up higher order thinking in science classrooms: the challenge of bridging the gap between theory, policy and practice. Thinking Skills and Creativity 2013, 10: 168–172. doi:10.1016/j.tsc.2013.08.001 10.1016/j.tsc.2013.08.001
Download references
Author information
Authors and affiliations.
Centre for Educational Measurement, University of Oslo (CEMO), Oslo, Norway
Ronny Scherer
Faculty of Educational Sciences, University of Oslo, Oslo, Norway
School of Education, Durham University, Durham, UK
Jens F Beckmann
You can also search for this author in PubMed Google Scholar
Corresponding author
Correspondence to Ronny Scherer .
Additional information
Competing interests.
The authors declare that they have no competing interests.
Authors’ contributions
RS carried out the analyses, participated in the development of the rationale, and drafted the manuscript. JFB carried out some additional analyses, participated in the development of the rationale, and drafted the manuscript. Both authors read and approved the final manuscript.
Authors’ original submitted files for images
Below are the links to the authors’ original submitted files for images.
Authors’ original file for figure 1
Authors’ original file for figure 2, authors’ original file for figure 3, rights and permissions.
Open Access This article is distributed under the terms of the Creative Commons Attribution 4.0 International License ( https://creativecommons.org/licenses/by/4.0 ), which permits use, duplication, adaptation, distribution, and reproduction in any medium or format, as long as you give appropriate credit to the original author(s) and the source, provide a link to the Creative Commons license, and indicate if changes were made.
Reprints and permissions
About this article
Cite this article.
Scherer, R., Beckmann, J.F. The acquisition of problem solving competence: evidence from 41 countries that math and science education matters. Large-scale Assess Educ 2 , 10 (2014). https://doi.org/10.1186/s40536-014-0010-7
Download citation
Received : 08 June 2014
Accepted : 25 November 2014
Published : 10 December 2014
DOI : https://doi.org/10.1186/s40536-014-0010-7
Share this article
Anyone you share the following link with will be able to read this content:
Sorry, a shareable link is not currently available for this article.
Provided by the Springer Nature SharedIt content-sharing initiative
- Capability under-utilisation
- Math-science coherence
- Math education
- Problem solving competence
- Science education
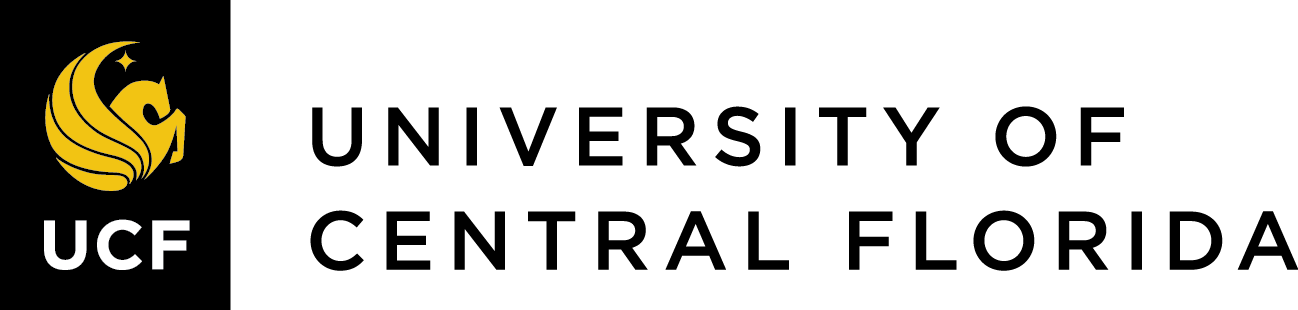
Thinking and Intelligence
Introduction to Thinking and Problem-Solving
What you’ll learn to do: describe cognition and problem-solving strategies.
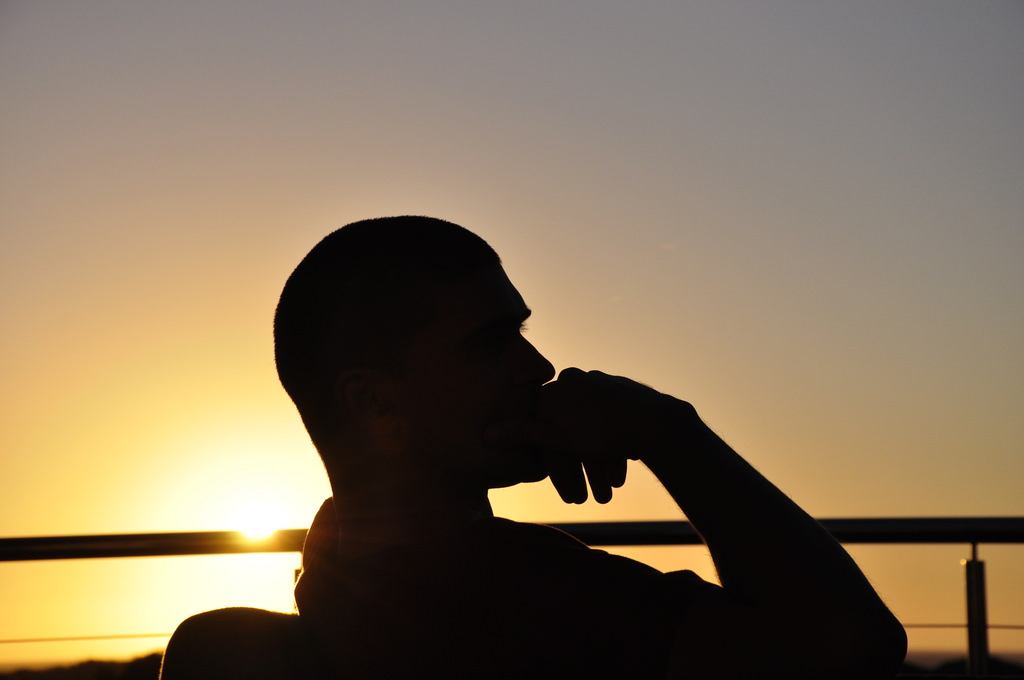
Imagine all of your thoughts as if they were physical entities, swirling rapidly inside your mind. How is it possible that the brain is able to move from one thought to the next in an organized, orderly fashion? The brain is endlessly perceiving, processing, planning, organizing, and remembering—it is always active. Yet, you don’t notice most of your brain’s activity as you move throughout your daily routine. This is only one facet of the complex processes involved in cognition. Simply put, cognition is thinking, and it encompasses the processes associated with perception, knowledge, problem solving, judgment, language, and memory. Scientists who study cognition are searching for ways to understand how we integrate, organize, and utilize our conscious cognitive experiences without being aware of all of the unconscious work that our brains are doing (for example, Kahneman, 2011).
Learning Objectives
- Distinguish between concepts and prototypes
- Explain the difference between natural and artificial concepts
- Describe problem solving strategies, including algorithms and heuristics
- Explain some common roadblocks to effective problem solving
CC licensed content, Original
- Modification, adaptation, and original content. Provided by : Lumen Learning. License : CC BY: Attribution
CC licensed content, Shared previously
- What Is Cognition?. Authored by : OpenStax College. Located at : https://openstax.org/books/psychology-2e/pages/7-1-what-is-cognition . License : CC BY: Attribution . License Terms : Download for free at https://openstax.org/books/psychology-2e/pages/1-introduction
- A Thinking Man Image. Authored by : Wesley Nitsckie. Located at : https://www.flickr.com/photos/nitsckie/5507777269 . License : CC BY-SA: Attribution-ShareAlike
General Psychology Copyright © by OpenStax and Lumen Learning is licensed under a Creative Commons Attribution 4.0 International License , except where otherwise noted.
Share This Book
Jerome Bruner’s Theory Of Learning And Cognitive Development
Saul Mcleod, PhD
Editor-in-Chief for Simply Psychology
BSc (Hons) Psychology, MRes, PhD, University of Manchester
Saul Mcleod, PhD., is a qualified psychology teacher with over 18 years of experience in further and higher education. He has been published in peer-reviewed journals, including the Journal of Clinical Psychology.
Learn about our Editorial Process
Olivia Guy-Evans, MSc
Associate Editor for Simply Psychology
BSc (Hons) Psychology, MSc Psychology of Education
Olivia Guy-Evans is a writer and associate editor for Simply Psychology. She has previously worked in healthcare and educational sectors.
On This Page:
Jerome Bruner believed that children construct knowledge and meaning through active experience with the world around them. He emphasized the role of culture and language in cognitive development, which occurs in a spiral fashion with children revisiting basic concepts at increasing levels of complexity and abstraction.
Bruner’s Ideas
- Like Ausubel (and other cognitive psychologists), Bruner sees the learner as an active agent; emphasizing the importance of existing schemata in guiding learning.
- Bruner argues that students should discern for themselves the structure of subject content – discovering the links and relationships between different facts, concepts and theories (rather than the teacher simply telling them).
- Bruner (1966) hypothesized that the usual course of intellectual development moves through three stages: enactive, iconic, and symbolic, in that order. However, unlike Piaget’s stages, Bruner did not contend that these stages were necessarily age-dependent, or invariant.
- Piaget and, to an extent, Ausubel, contended that the child must be ready, or made ready, for the subject matter. But Bruner contends just the opposite. According to his theory, the fundamental principles of any subject can be taught at any age, provided the material is converted to a form (and stage) appropriate to the child.
- The notion of a “spiral curriculum” embodies Bruner’s ideas by “spiraling” through similar topics at every age, but consistent with the child’s stage of thought.
- His spiral curriculum revisits basic ideas repeatedly, building upon them into more complex, abstract concepts over time in a developmentally appropriate sequence.
- The aim of education should be to create autonomous learners (i.e., learning to learn).
- Cognitive growth involves an interaction between basic human capabilities and “culturally invented technologies that serve as amplifiers of these capabilities.”
- These culturally invented technologies include not just obvious things such as computers and television, but also more abstract notions such as the way a culture categorizes phenomena, and language itself.
- Bruner would likely agree with Vygotsky that language serves to mediate between environmental stimuli and the individual’s response.
Three Modes of Representation
Modes of representation are how information or knowledge is stored and encoded in memory.
Rather than neat age-related stages (like Piaget), the modes of representation are integrated and only loosely sequential as they “translate” into each other.
Bruner (1966) was concerned with how knowledge is represented and organized through different modes of thinking (or representation).
In his research on the cognitive development of children, Jerome Bruner proposed three modes of representation:
- Enactive representation (action-based)
- Iconic representation (image-based)
- Symbolic representation (language-based)
Bruner’s constructivist theory suggests it is effective when faced with new material to follow a progression from enactive to iconic to symbolic representation; this holds true even for adult learners.
Bruner’s work also suggests that a learner even of a very young age is capable of learning any material so long as the instruction is organized appropriately, in sharp contrast to the beliefs of Piaget and other stage theorists.
Enactive Mode (0-1 year)
In the enactive mode , knowledge is stored primarily in the form of motor responses. This mode is used within the first year of life (corresponding with Piaget’s sensorimotor stage ).
Thinking is based entirely on physical actions , and infants learn by doing, rather than by internal representation (or thinking).
It involves encoding physical action-based information and storing it in our memory. For example, in the form of movement as muscle memory, a baby might remember the action of shaking a rattle.
And this is not just limited to children. Many adults can perform a variety of motor tasks (typing, sewing a shirt, operating a lawn mower) that they would find difficult to describe in iconic (picture) or symbolic (word) form.
This mode continues later in many physical activities, such as learning to ride a bike.
Iconic Mode (1-6 years)
Information is stored as sensory images (icons), usually visual ones, like pictures in the mind. For some, this is conscious; others say they don’t experience it.
This may explain why, when we are learning a new subject, it is often helpful to have diagrams or illustrations to accompany the verbal information.
Thinking is also based on using other mental images (icons), such as hearing, smell or touch.
Symbolic Mode (7 years onwards)
This develops last. In the symbolic stage , knowledge is stored primarily as language, mathematical symbols, or in other symbol systems.
This mode is acquired around six to seven years old (corresponding to Piaget’s concrete operational stage ).
In the symbolic stage, knowledge is stored primarily as words, mathematical symbols, or other symbol systems, such as music.
Symbols are flexible in that they can be manipulated, ordered, classified, etc., so the user isn’t constrained by actions or images (which have a fixed relation to that which they represent).
According to Bruner’s taxonomy, these differ from icons in that symbols are “arbitrary.” For example, the word “beauty” is an arbitrary designation for the idea of beauty in that the word itself is no more inherently beautiful than any other word.
The Importance of Language
Language is important for the increased ability to deal with abstract concepts.
Bruner argues that language can code stimuli and free an individual from the constraints of dealing only with appearances, to provide a more complex yet flexible cognition.
The use of words can aid the development of the concepts they represent and can remove the constraints of the “here & now” concept.
Bruner views the infant as an intelligent & active problem solver from birth, with intellectual abilities basically similar to those of the mature adult.
Educational Implications
Education should aim to create autonomous learners (i.e., learning to learn).
For Bruner (1961), the purpose of education is not to impart knowledge, but instead to facilitate a child’s thinking and problem-solving skills which can then be transferred to a range of situations. Specifically, education should also develop symbolic thinking in children.
In 1960 Bruner’s text, The Process of Education was published. The main premise of Bruner’s text was that students are active learners who construct their own knowledge.
Bruner (1960) opposed Piaget’s notion of readiness . He argued that schools waste time trying to match the complexity of subject material to a child’s cognitive stage of development.
This means students are held back by teachers as certain topics are deemed too difficult to understand and must be taught when the teacher believes the child has reached the appropriate stage of cognitive maturity .
The Spiral Curriculum
Bruner (1960) adopts a different view and believes a child (of any age) is capable of understanding complex information:
“We begin with the hypothesis that any subject can be taught effectively in some intellectually honest form to any child at any stage of development.” (p. 33)
Bruner (1960) explained how this was possible through the concept of the spiral curriculum. This involved information being structured so that complex ideas can be taught at a simplified level first, and then re-visited at more complex levels later on.
The underlying principle in this is that the student should review particular concepts at over and over again during their educative experience; each time building and their understanding and requiring more sophisticated cognitive strategies (and thus increase the sophistication of their understanding).
Therefore, subjects would be taught at levels of gradually increasing difficultly (hence the spiral analogy). Ideally, teaching his way should lead to children being able to solve problems by themselves.
Bruner argues that, as children age, they are capable of increasingly complex modes of representation (basically, ways of thinking) – and the spiral curriculum should be sensitive to this development;
- Initially, children learn better using an enactive mode of representation (i.e. they learn better through “doing things” such as physical and manual tasks) – for instance, the concept of addition might be first taught by asking the child to combine piles of beads and counting the results.
- As they grow older – and more familiar with subject content – pupils become more confident in using an iconic mode of representation; they are able to perform tasks by imagining concrete pictures in their heads. To continue the above example; as the child becomes more confident with addition, they should be able to imagine the beads in order to complete additions (without physically needing to manipulate the piles).
- Finally, students become capable of more abstract, symbolic modes of representation; without the need for either physical manipulation or mental imagery. Consequently, at this point, the student should have little problem with completing a series of written calculations; of numbers which are higher than is possible by “imagining beads”.
Discovery Learning Theory
Bruner (1960) developed the concept of Discovery Learning – arguing that students should “not be presented with the subject matter in its final form, but rather are required to organize it themselves…[requiring them] to discover for themselves relationships that exist among items of information”.
Bruner (1961) proposes that learners construct their own knowledge and do this by organizing and categorizing information using a coding system.
Bruner believed that the most effective way to develop a coding system is to discover it rather than being told by the teacher.
The concept of discovery learning implies that students construct their own knowledge for themselves (also known as a constructivist approach ).
The result is an extremely active form of learning, in which the students are always engaged in tasks, finding patterns or solving puzzles – and in which they constantly need to exercise their existing schemata , reorganizing and amending these concepts to address the challenges of the task.
The role of the teacher should not be to teach information by rote learning, but instead to facilitate the learning process. This means that a good teacher will design lessons that help students discover the relationship between bits of information.
To do this a teacher must give students the information they need, but without organizing for them. The use of the spiral curriculum can aid the process of discovery learning .
For example, in teaching a particular concept, the teacher should present the set of instances that will best help learners develop an appropriate model of the concept. The teacher should also model the inquiry process. Bruner would likely not contend that all learning should be through discovery.
For example, it seems pointless to have children “discover” the names of the U.S. Presidents, or important dates in history.
Bruner’s theory is probably clearest when illustrated with practical examples. The instinctive response of a teacher to the task of helping a primary-school child understand the concept of odd and even numbers, for instance, would be to explain the difference to them.
However, Bruner would argue that understanding of this concept would be much more genuine if the child discovered the difference for themselves; for instance, by playing a game in which they had to share various numbers of beads fairly between themselves and their friend.
Discovery is not just an instructional technique, but an important learning outcome in itself. Schools should help learners develop their own ability to find the “recurrent regularities” in their environment.
Bruner would likely not contend that all learning should be through discovery. For example, it seems pointless to have children “discover” the names of the U.S. Presidents, or important dates in history.
Scaffolding Theory
On the surface, Bruner’s emphasis on the learner discovering subject content for themselves seemingly absolves the teacher of a great deal of work.
In practice, however, his model requires the teacher to be actively involved in lessons; providing cognitive scaffolding which will facilitate learning on the part of the student.
On the one hand, this involves the selection and design of appropriate stimulus materials and activities which the student can understand and complete – however Bruner also advocates that the teacher should circulate the classroom and work with individual students, performing six core “functions” (Wood, Bruner and Ross: 1976):
- Recruitment : ensuring that the student is interested in the task, and understands what is required of them.
- Reducing degrees of freedom : helping the student make sense of the material by eliminating irrelevant directions and thus reducing the “trial and error” aspect of learning.
- Direction Maintenance : ensuring that the learner is on-task and interest is maintained – often by breaking the ultimate aim of the task into “sub-aims” which are more readily understood and achieved.
- Marking critical features : highlighting relevant concepts or processes and pointing out errors.
- Frustration Control : stopping students from “giving up” on the task.
- Demonstration : providing models for imitation or possible (partial solution).
In this context, Bruner’s model might be better described as guided discovery learning; as the teacher is vital in ensuring that the acquisition of new concepts and processes is successful.
Bruner and Vygotsky
Both Bruner and Vygotsky emphasize a child’s environment, especially the social environment, more than Piaget did. Both agree that adults should play an active role in assisting the child’s learning.
Bruner, like Vygotsky, emphasized the social nature of learning, citing that other people should help a child develop skills through the process of scaffolding.
“[Scaffolding] refers to the steps taken to reduce the degrees of freedom in carrying out some task so that the child can concentrate on the difficult skill she is in the process of acquiring” (Bruner, 1978, p. 19).
He was especially interested in the characteristics of people whom he considered to have achieved their potential as individuals.
The term scaffolding first appeared in the literature when Wood, Bruner, and Ross described how tutors” interacted with a preschooler to help them solve a block reconstruction problem (Wood et al., 1976).
The concept of scaffolding is very similar to Vygotsky’s notion of the zone of proximal development , and it’s not uncommon for the terms to be used interchangeably.
Scaffolding involves helpful, structured interaction between an adult and a child with the aim of helping the child achieve a specific goal.
The purpose of the support is to allow the child to achieve higher levels of development by:
- Simplifying the task or idea.
- Motivating and encouraging the child.
- Highlighting important task elements or errors.
- Giving models that can be imitated.
Bruner and Piaget
There are similarities between Piaget and Bruner, but a significant difference is that Bruner’s modes are not related in terms of which presuppose the one that precedes it. While sometimes one mode may dominate in usage, they coexist.
Bruner states that the level of intellectual development determines the extent to which the child has been given appropriate instruction together with practice or experience.
So – the right way of presentation and explanation will enable a child to grasp a concept usually only understood by an adult. His theory stresses the role of education and the adult.
Although Bruner proposes stages of cognitive development, he doesn’t see them as representing different separate modes of thought at different points of development (like Piaget).
Instead, he sees a gradual development of cognitive skills and techniques into more integrated “adult” cognitive techniques.
Bruner views symbolic representation as crucial for cognitive development, and since language is our primary means of symbolizing the world, he attaches great importance to language in determining cognitive development.
- Children are innately PRE-ADAPTED to learning
- Children have a NATURAL CURIOSITY
- Children’s COGNITIVE STRUCTURES develop over time
- Children are ACTIVE participants in the learning process
- Cognitive development entails the acquisition of SYMBOLS
- Social factors, particularly language, were important for cognitive growth. These underpin the concept of ‘scaffolding’.
- The development of LANGUAGE is a cause not a consequence of cognitive development
- You can SPEED-UP cognitive development. You don’t have to wait for the child to be ready
- The involvement of ADULTS and MORE KNOWLEDGEABLE PEERS makes a big difference
Bruner, J. S. (1957). Going beyond the information given. New York: Norton.
Bruner, J. S. (1960). The Process of education. Cambridge, Mass.: Harvard University Press.
Bruner, J. S. (1961). The act of discovery. Harvard Educational Review , 31, 21-32.
Bruner, J. S. (1966). Toward a theory of instruction , Cambridge, Mass.: Belkapp Press.
Bruner, J. S. (1973). The relevance of education . New York: Norton.
Bruner, J. S. (1978). The role of dialogue in language acquisition. In A. Sinclair, R., J. Jarvelle, and W. J.M. Levelt (eds.) The Child’s Concept of Language. New York: Springer-Verlag.
Wood, D. J., Bruner, J. S., & Ross, G. (1976). The role of tutoring in problem solving. Journal of Child Psychiatry and Psychology , 17(2), 89-100.
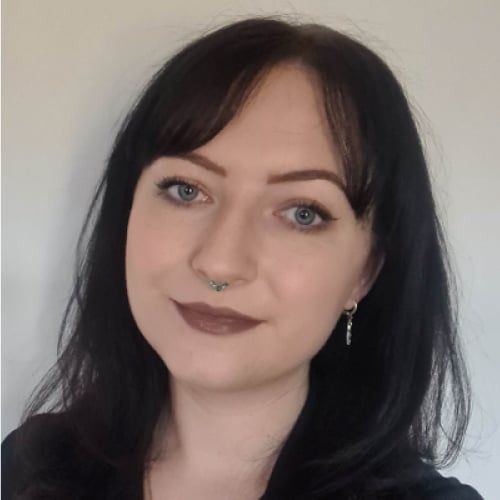
Thinking and Intelligence
Introduction to thinking and problem-solving, what you’ll learn to do: describe cognition and problem-solving strategies.
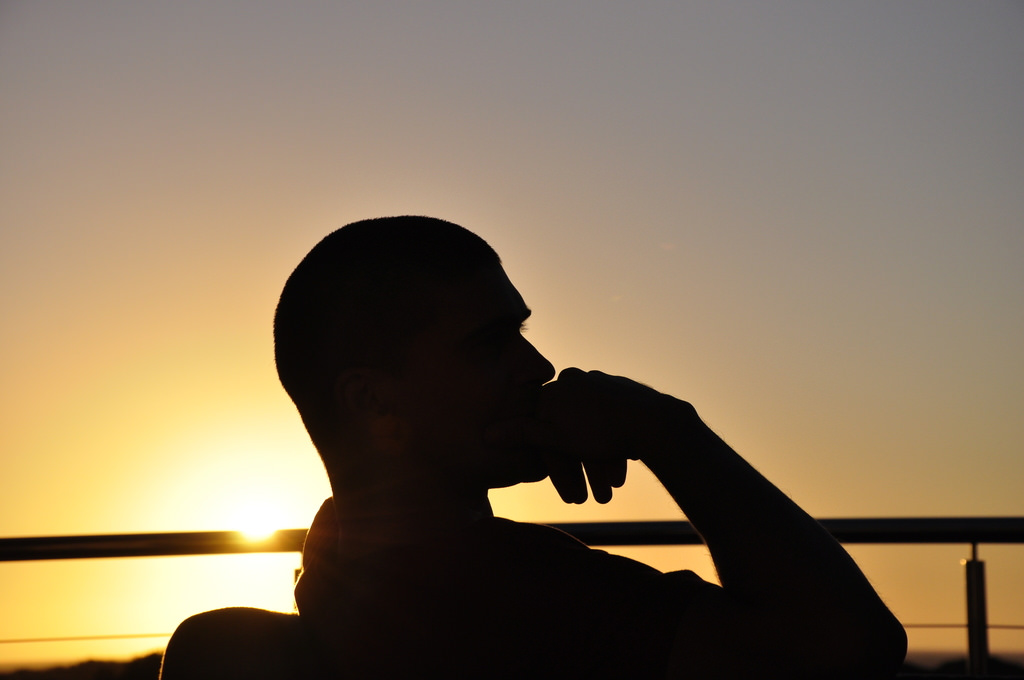
Imagine all of your thoughts as if they were physical entities, swirling rapidly inside your mind. How is it possible that the brain is able to move from one thought to the next in an organized, orderly fashion? The brain is endlessly perceiving, processing, planning, organizing, and remembering—it is always active. Yet, you don’t notice most of your brain’s activity as you move throughout your daily routine. This is only one facet of the complex processes involved in cognition. Simply put, cognition is thinking, and it encompasses the processes associated with perception, knowledge, problem solving, judgment, language, and memory. Scientists who study cognition are searching for ways to understand how we integrate, organize, and utilize our conscious cognitive experiences without being aware of all of the unconscious work that our brains are doing (for example, Kahneman, 2011).
Learning Objectives
- Distinguish between concepts and prototypes
- Explain the difference between natural and artificial concepts
- Describe problem solving strategies, including algorithms and heuristics
- Explain some common roadblocks to effective problem solving
Contribute!
Improve this page Learn More
- Modification, adaptation, and original content. Provided by : Lumen Learning. License : CC BY: Attribution
- What Is Cognition?. Authored by : OpenStax College. Located at : https://openstax.org/books/psychology-2e/pages/7-1-what-is-cognition . License : CC BY: Attribution . License Terms : Download for free at https://openstax.org/books/psychology-2e/pages/1-introduction
- A Thinking Man Image. Authored by : Wesley Nitsckie. Located at : https://www.flickr.com/photos/nitsckie/5507777269 . License : CC BY-SA: Attribution-ShareAlike
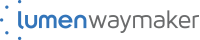
IResearchNet
Categorization
In cognitive psychology, categorization focuses on how knowledge is organized. Objects in the same category are likely to share certain attributes, and category membership allows inferences to be drawn. The term cat egory refers to a set of things (objects, ideas, events) that are grouped together. The term concept often refers to the mental representation of such knowledge.
Categorization has obvious functions for learning, organizing, and storing information. From an evolutionary standpoint, it benefits an individual to be able to amass as large an amount of information with the least amount of effort or time. By grouping known items together, one can better identify novel items as well as predict some of their likely attributes.
Academic Writing, Editing, Proofreading, And Problem Solving Services
Get 10% off with 24start discount code.
Neuroscientific studies support an underlying categorical organization for semantic knowledge in the human brain; subjects with neurological damage can lose the ability to retrieve categorically selective information. For example, a person may be able to name tools or utensils, but not animals or fruits. These specific deficits point to a key organizational role for categorization in cognitive processing.
Categorization is a process that occurs cross-culturally as well. But while some objects tend to be universally grouped together, such as shapes and colors, others may be grouped differently as a function of cultural background. Consider a classification task for the items shovel , hoe , dirt , and hammer . Someone from an industrialized background with formal education might use a taxonomic grouping and thus omit dirt because it is not a tool. Someone from an agrarian background without formal education might use a thematic grouping and thus omit hammer because the other items are used together.
Levels Of Categorization
Many items can be categorized hierarchically, and the following scheme is often used in describing this organization:
Superordinate —The superordinate level is a broad, inclusive level in the hierarchical scale of categories (e.g., animal, vehicle). Often the superordinate level will consist of several basic-level categories.
Basic —The basic-level category (e.g., cat, boat) is often used when one labels an object. Items within a basiclevel category share many features in common and are distinct from items in other basic-level categories. Researcher Eleanor Rosch argued for the primacy of basic-level categorization in early development.
Subordinate —Subordinate-level categories offer a more specific breakdown within a basic level category (e.g., tabby [for cat], canoe [for boat]).
Theories Of Categorization
Researchers continue to debate various theoretical approaches for describing categorization processes. Most theories can be classified as either similarity based or explanation based.
Similarity-Based Categorization
The classical defining-attribute theory identifies members of a category based on certain traits that all items have in common. The set of common attributes is both essential and sufficient for an item to be included in the category. All squares have four equal sides and four 90-degree corners. A shape that does not meet all the standards is not a square. This all-or-none explanation implies that no item within a given category is more representative of that category than another item. This theory has been disputed on the basis that most categories are not clearly marked but rather have fuzzy boundaries. Philosopher Ludwig Wittgenstein used the example of games, pointing out that among all possible games (those with boards, balls, cards, sticks, etc.) no set of features is necessary or sufficient for a particular game’s inclusion or exclusion.
The prototype theory states that because there are no absolute boundaries, some items of a category are indeed more representative than others. The theory explains that a set of characteristic attributes, not defining attributes, is what identifies objects as belonging to a category. A prototype is created by averaging features together to create a typical or central example. Rosch cited one example as the category birds , stating that robin is far more “typical” than penguin and thus closer to the prototype, because it contains more of the characteristic attributes. The research finding that people are quicker to judge a robin as a bird than a penguin as a bird has been used to support this theory.
The exemplar theory states that we categorize items based on examples, rather than on a prototype that becomes abstracted over time. In other words, people are capable of drawing on multiple instances when locating or placing an object into a particular category. The mental representation of the category is presumed to contain information from various specific exemplars.
This theory is consistent with the finding that understanding variability and typicality of instances within a category plays a role in predicting category membership.
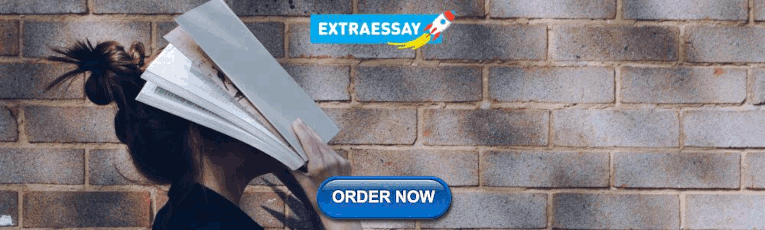
Explanation-Based Categorization
Instead of emphasizing similar features, explanation-based approaches define categories based on theory-driven inferences. For example, categories may be based on nonobvious features, such as the presumed internal characteristics that differentiate animate from inanimate objects. Also, ad hoc categories that ignore perceptual similarity can be created, such as the category things at a yard sale . These categories cannot be explained using a similarity-based view, but instead require fundamental theoretical knowledge.
The Development Of Categorization
Questions about the development of categorization help us characterize how infants and children understand their worlds and provide information about the building blocks of adults’ cognitive skills.
Current research explores some critical questions about how categorization and conceptual processing develop. For example, do infants first recognize basic-level categories, followed by elaboration of the hierarchical organization of superordinate categories? Does development proceed from perceptually based categories to conceptually defined categories?
Psychologist Eleanor Gibson argued that laboratory studies of categorization often focus on static presentation of visual stimuli (e.g., pictures), whereas infants in the real world use dynamic information from various modalities. She emphasized the benefit of a symbiotic connection between perception and action. Acting on an object provides more information about it, and its perceptual characteristics allow for plausible actions. She proposed that perception is not separate from meaning, and thus the distinction between perceptual and conceptual categorization may be unnecessary.
Categorization in Infants
Studying categorization in infants requires methods that do not rely on verbal explanation. In one popular method, infants are shown a series of objects from the same category (e.g., cats) and the time that they look at each item is measured. The subsequent test measures how long the infant looks at a novel member of that same category (cat) compared to how long they look at a novel member of a different category (e.g., rabbit). The inference is that a preference for the novel category demonstrates the infant’s categorization of the initially presented category.
Using this method, researchers have demonstrated that infants as young as 3 months can discriminate categories such as shapes, colors, and animal types. Between 6 months and 1 year, infants attend to categories such as vehicles, tools, facial expressions, and gender.
Researchers propose that early conceptual understanding occurs for distinctions such as animate versus inanimate objects, and that infants begin to categorize based not only on perceptual appearance, but also on function. During the second year, infants can group toys with different attributes into different piles, actively demonstrating their categorization skills. As linguistic skills develop, children’s abilities can be tested with verbal tasks.
Categorization in Childhood
Later in childhood, hierarchical classification skills emerge. Rosch found that preschool-aged children are more adept at sorting objects at the basic level than at the superordinate level. Children’s language learning and adults’ labeling of objects to children follow this same trend. Other researchers suggest an early appreciation of more global characteristics of object categories.
The development of explanation-based categorization has also been studied. For example, researcher Frank Keil tested children with stories about an animal (e.g., raccoon) that was physically transformed to look like a skunk. Preschoolers tended to be fooled by appearance, but second-graders were able to classify the animal using internal characteristics.
Categorization and Language Development
Another area of debate is the developmental connection between categorization and language. This debate reflects the classic distinction between theorists Lev Vygotsky and Jean Piaget on the connection between language and thought. That is, do children’s linguistic processes (e.g., labeling) simply reflect their level of cognitive understanding of categories, or does the acquisition of language influence and facilitate developing processes of categorization?
Researchers have proposed that linguistic development is important in categorization and conceptual development because the use of a common label (e.g., vehicle ) across multiple things (e.g., boats, planes, cars) highlights common features of the category and allows for inferences about internal and functional properties. Many studies reveal young children’s ability to link language and concepts. For example, children’s sensitivity to linguistic distinctions has been revealed through the use of nonsense phrases such as “this is a nax” versus “this is some nax”; they correctly map the first label to the count noun (e.g., a whole object) and the second label to the substance of a mass noun (e.g., sand).
Categorization is a fundamental aspect of cognition, and there is growing interest in the organization and mental representation of categories. Perceptual categorization is evident early in infancy. Preschool-aged children categorize adeptly, use concepts for inductive reasoning, and notice linguistic cues for categorization. During middle childhood, theory-based reasoning continues to unfold and hierarchical classification is evident. The mental representation of categorical knowledge in children and adults continues to be debated, and each of the various theoretical approaches has advantages and disadvantages.
References:
- Gibson, J. (2000). Commentary on perceptual and conceptual processes in infancy. Journal of Cognition and Development , 1 , 43–48.
- Gopnik, , Meltsoff, A. N., & Kuhl, P. K. (2000). The scientist in the crib: What early learning tells us about the mind . New York: Perennial.
- Lakoff, (1987). Women, fire, and dangerous things: What categories reveal about the mind . Chicago: University of Chicago Press.
- Medin, D. , & Atran, S. (Eds.). (1999). Folkbiology .Cambridge: MIT Press.
- Pinker, (1997). How the mind works . New York: W. W.Norton.
- Rakison, H., & Oakes, L. M. (Eds.). (2004). Early category and concept development: Making sense of the blooming, buzzing confusion . New York: Oxford.
Want to create or adapt books like this? Learn more about how Pressbooks supports open publishing practices.
Ch 8: Thinking and Language
Thinking and language.
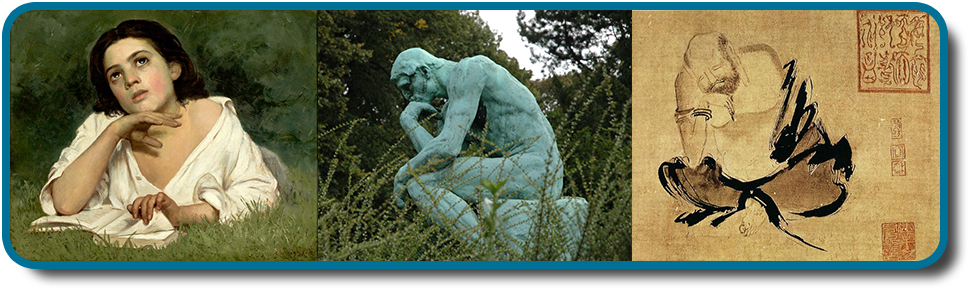
Why is it so difficult to break habits—like reaching for your ringing phone even when you shouldn’t, such as when you’re driving? Why is it hard to pay attention to a conversation when typing out a text message? How does a person who has never seen or touched snow in real life develop an understanding of the concept of snow? How do young children acquire the ability to learn language with no formal instruction? Psychologists who study thinking explore questions like these.
As a part of this discussion, we will consider thinking, and briefly explore the development and use of language. We will also discuss problem solving and creativity. After finishing this chapter, you will have a greater appreciation of the higher-level cognitive processes that contribute to our distinctiveness as a species.
Learning Objectives
- Understand why selective attention is important and how it can be studied.
- Learn about different models of when and how selection can occur.
- Understand how divided attention or multitasking is studied, and implications of multitasking in situations such as distracted driving.
Thinking and Problem-Solving
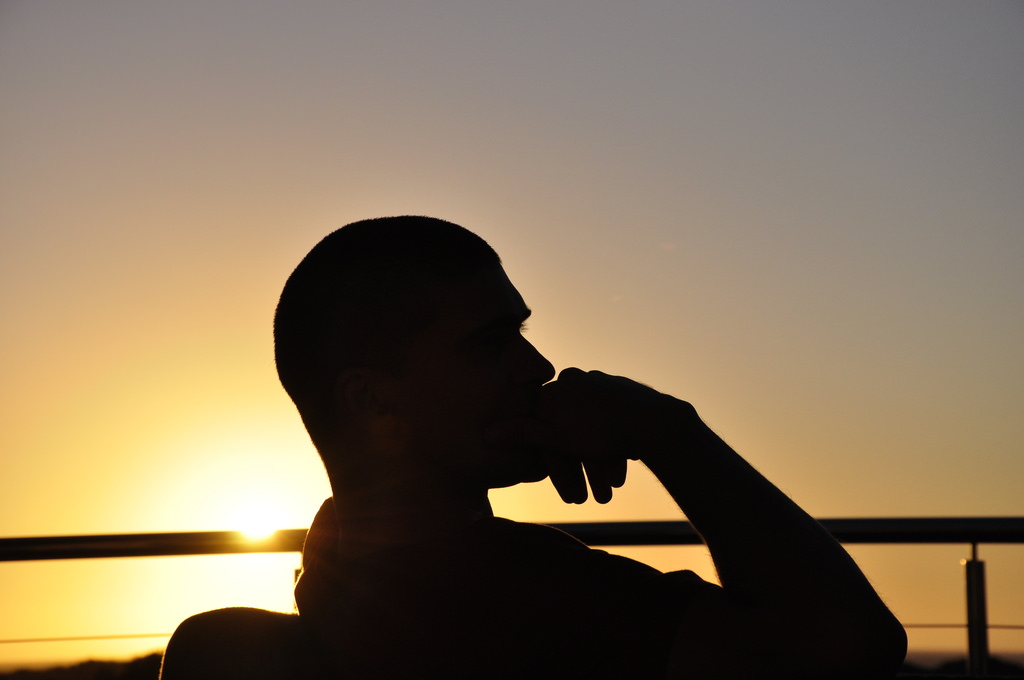
Imagine all of your thoughts as if they were physical entities, swirling rapidly inside your mind. How is it possible that the brain is able to move from one thought to the next in an organized, orderly fashion? The brain is endlessly perceiving, processing, planning, organizing, and remembering—it is always active. Yet, you don’t notice most of your brain’s activity as you move throughout your daily routine. This is only one facet of the complex processes involved in cognition. Simply put, cognition is thinking, and it encompasses the processes associated with perception, knowledge, problem solving, judgment, language, and memory. Scientists who study cognition are searching for ways to understand how we integrate, organize, and utilize our conscious cognitive experiences without being aware of all of the unconscious work that our brains are doing (for example, Kahneman, 2011).
- Distinguish between concepts and prototypes
- Explain the difference between natural and artificial concepts
- Describe problem solving strategies, including algorithms and heuristics
- Explain some common roadblocks to effective problem solving
What is Cognition?
Categories and concepts, concepts and prototypes.
The human nervous system is capable of handling endless streams of information. The senses serve as the interface between the mind and the external environment, receiving stimuli and translating it into nerve impulses that are transmitted to the brain. The brain then processes this information and uses the relevant pieces to create thoughts, which can then be expressed through language or stored in memory for future use. To make this process more complex, the brain does not gather information from external environments only. When thoughts are formed, the brain also pulls information from emotions and memories (Figure 9). Emotion and memory are powerful influences on both our thoughts and behaviors.
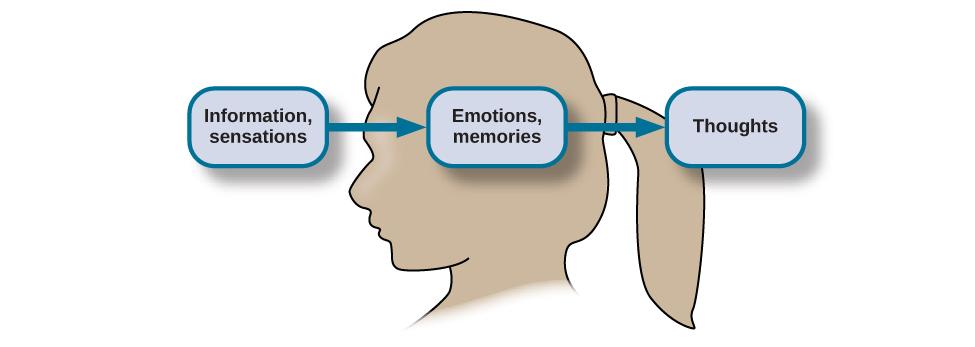
In order to organize this staggering amount of information, the brain has developed a file cabinet of sorts in the mind. The different files stored in the file cabinet are called concepts. Concepts are categories or groupings of linguistic information, images, ideas, or memories, such as life experiences. Concepts are, in many ways, big ideas that are generated by observing details, and categorizing and combining these details into cognitive structures. You use concepts to see the relationships among the different elements of your experiences and to keep the information in your mind organized and accessible.
Concepts are informed by our semantic memory (you will learn more about this concept when you study memory) and are present in every aspect of our lives; however, one of the easiest places to notice concepts is inside a classroom, where they are discussed explicitly. When you study United States history, for example, you learn about more than just individual events that have happened in America’s past. You absorb a large quantity of information by listening to and participating in discussions, examining maps, and reading first-hand accounts of people’s lives. Your brain analyzes these details and develops an overall understanding of American history. In the process, your brain gathers details that inform and refine your understanding of related concepts like democracy, power, and freedom.
Concepts can be complex and abstract, like justice, or more concrete, like types of birds. In psychology, for example, Piaget’s stages of development are abstract concepts. Some concepts, like tolerance, are agreed upon by many people because they have been used in various ways over many years. Other concepts, like the characteristics of your ideal friend or your family’s birthday traditions, are personal and individualized. In this way, concepts touch every aspect of our lives, from our many daily routines to the guiding principles behind the way governments function.
Concepts are at the core of intelligent behavior. We expect people to be able to know what to do in new situations and when confronting new objects. If you go into a new classroom and see chairs, a blackboard, a projector, and a screen, you know what these things are and how they will be used. You’ll sit on one of the chairs and expect the instructor to write on the blackboard or project something onto the screen. You do this even if you have never seen any of these particular objects before , because you have concepts of classrooms, chairs, projectors, and so forth, that tell you what they are and what you’re supposed to do with them. Furthermore, if someone tells you a new fact about the projector—for example, that it has a halogen bulb—you are likely to extend this fact to other projectors you encounter. In short, concepts allow you to extend what you have learned about a limited number of objects to a potentially infinite set of entities.
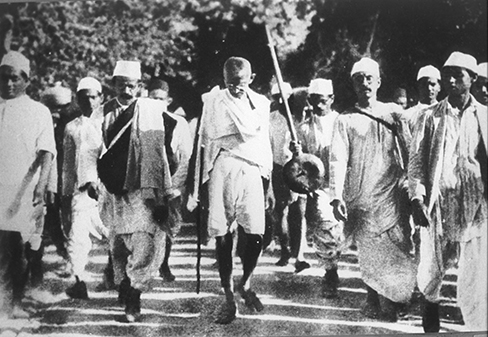
Another technique used by your brain to organize information is the identification of prototypes for the concepts you have developed. A prototype is the best example or representation of a concept. For example, for the category of civil disobedience, your prototype could be Rosa Parks. Her peaceful resistance to segregation on a city bus in Montgomery, Alabama, is a recognizable example of civil disobedience. Or your prototype could be Mohandas Gandhi, sometimes called Mahatma Gandhi (“Mahatma” is an honorific title) (Figure 10).
Mohandas Gandhi served as a nonviolent force for independence for India while simultaneously demanding that Buddhist, Hindu, Muslim, and Christian leaders—both Indian and British—collaborate peacefully. Although he was not always successful in preventing violence around him, his life provides a steadfast example of the civil disobedience prototype (Constitutional Rights Foundation, 2013). Just as concepts can be abstract or concrete, we can make a distinction between concepts that are functions of our direct experience with the world and those that are more artificial in nature.
Link to Learning
Natural and artificial concepts.
In psychology, concepts can be divided into two categories, natural and artificial. Natural concepts are created “naturally” through your experiences and can be developed from either direct or indirect experiences. For example, if you live in Essex Junction, Vermont, you have probably had a lot of direct experience with snow. You’ve watched it fall from the sky, you’ve seen lightly falling snow that barely covers the windshield of your car, and you’ve shoveled out 18 inches of fluffy white snow as you’ve thought, “This is perfect for skiing.” You’ve thrown snowballs at your best friend and gone sledding down the steepest hill in town. In short, you know snow. You know what it looks like, smells like, tastes like, and feels like. If, however, you’ve lived your whole life on the island of Saint Vincent in the Caribbean, you may never have actually seen snow, much less tasted, smelled, or touched it. You know snow from the indirect experience of seeing pictures of falling snow—or from watching films that feature snow as part of the setting. Either way, snow is a natural concept because you can construct an understanding of it through direct observations or experiences of snow (Figure 11).
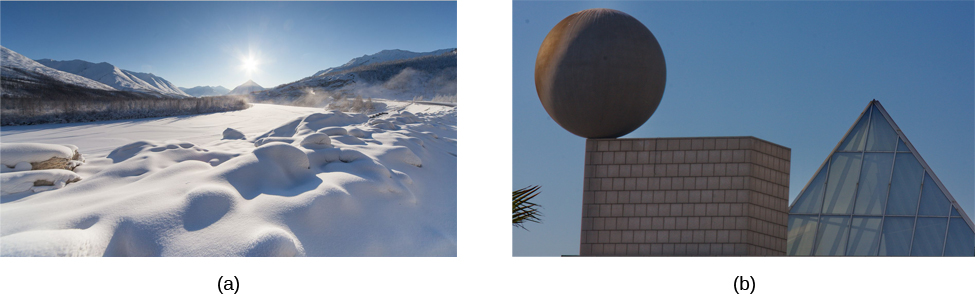
An artificial concept on the other hand, is a concept that is defined by a specific set of characteristics. Various properties of geometric shapes, like squares and triangles, serve as useful examples of artificial concepts. A triangle always has three angles and three sides. A square always has four equal sides and four right angles. Mathematical formulas, like the equation for area (length × width) are artificial concepts defined by specific sets of characteristics that are always the same. Artificial concepts can enhance the understanding of a topic by building on one another. For example, before learning the concept of “area of a square” (and the formula to find it), you must understand what a square is. Once the concept of “area of a square” is understood, an understanding of area for other geometric shapes can be built upon the original understanding of area. The use of artificial concepts to define an idea is crucial to communicating with others and engaging in complex thought. According to Goldstone and Kersten (2003), concepts act as building blocks and can be connected in countless combinations to create complex thoughts.
A schema is a mental construct consisting of a cluster or collection of related concepts (Bartlett, 1932). There are many different types of schemata, and they all have one thing in common: schemata are a method of organizing information that allows the brain to work more efficiently. When a schema is activated, the brain makes immediate assumptions about the person or object being observed.
There are several types of schemata. A role schema makes assumptions about how individuals in certain roles will behave (Callero, 1994). For example, imagine you meet someone who introduces himself as a firefighter. When this happens, your brain automatically activates the “firefighter schema” and begins making assumptions that this person is brave, selfless, and community-oriented. Despite not knowing this person, already you have unknowingly made judgments about him. Schemata also help you fill in gaps in the information you receive from the world around you. While schemata allow for more efficient information processing, there can be problems with schemata, regardless of whether they are accurate: Perhaps this particular firefighter is not brave, he just works as a firefighter to pay the bills while studying to become a children’s librarian.
An event schema , also known as a cognitive script , is a set of behaviors that can feel like a routine. Think about what you do when you walk into an elevator (Figure 12). First, the doors open and you wait to let exiting passengers leave the elevator car. Then, you step into the elevator and turn around to face the doors, looking for the correct button to push. You never face the back of the elevator, do you? And when you’re riding in a crowded elevator and you can’t face the front, it feels uncomfortable, doesn’t it? Interestingly, event schemata can vary widely among different cultures and countries. For example, while it is quite common for people to greet one another with a handshake in the United States, in Tibet, you greet someone by sticking your tongue out at them, and in Belize, you bump fists (Cairns Regional Council, n.d.)
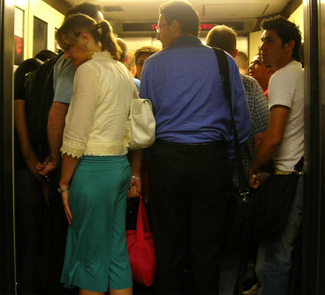
Because event schemata are automatic, they can be difficult to change. Imagine that you are driving home from work or school. This event schema involves getting in the car, shutting the door, and buckling your seatbelt before putting the key in the ignition. You might perform this script two or three times each day. As you drive home, you hear your phone’s ring tone. Typically, the event schema that occurs when you hear your phone ringing involves locating the phone and answering it or responding to your latest text message. So without thinking, you reach for your phone, which could be in your pocket, in your bag, or on the passenger seat of the car. This powerful event schema is informed by your pattern of behavior and the pleasurable stimulation that a phone call or text message gives your brain. Because it is a schema, it is extremely challenging for us to stop reaching for the phone, even though we know that we endanger our own lives and the lives of others while we do it (Neyfakh, 2013) (Figure 13).
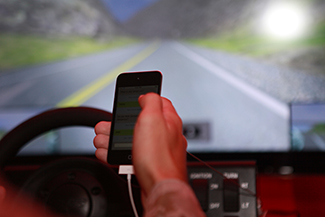
Remember the elevator? It feels almost impossible to walk in and not face the door. Our powerful event schema dictates our behavior in the elevator, and it is no different with our phones. Current research suggests that it is the habit, or event schema, of checking our phones in many different situations that makes refraining from checking them while driving especially difficult (Bayer & Campbell, 2012). Because texting and driving has become a dangerous epidemic in recent years, psychologists are looking at ways to help people interrupt the “phone schema” while driving. Event schemata like these are the reason why many habits are difficult to break once they have been acquired. As we continue to examine thinking, keep in mind how powerful the forces of concepts and schemata are to our understanding of the world.
Watch this CrashCourse video to see more examples of concepts and prototypes. You’ll also get a preview on other key topics in cognition, including problem-solving strategies like algorithms and heuristics.
You can view the transcript for “Cognition – How Your Mind Can Amaze and Betray You: Crash Course Psychology #15” here (opens in new window) .
Think It Over
People face problems every day—usually, multiple problems throughout the day. Sometimes these problems are straightforward: To double a recipe for pizza dough, for example, all that is required is that each ingredient in the recipe be doubled. Sometimes, however, the problems we encounter are more complex. For example, say you have a work deadline, and you must mail a printed copy of a report to your supervisor by the end of the business day. The report is time-sensitive and must be sent overnight. You finished the report last night, but your printer will not work today. What should you do? First, you need to identify the problem and then apply a strategy for solving the problem.
Problem-Solving Strategies
When you are presented with a problem—whether it is a complex mathematical problem or a broken printer, how do you solve it? Before finding a solution to the problem, the problem must first be clearly identified. After that, one of many problem solving strategies can be applied, hopefully resulting in a solution.
A problem-solving strategy is a plan of action used to find a solution. Different strategies have different action plans associated with them. For example, a well-known strategy is trial and error . The old adage, “If at first you don’t succeed, try, try again” describes trial and error. In terms of your broken printer, you could try checking the ink levels, and if that doesn’t work, you could check to make sure the paper tray isn’t jammed. Or maybe the printer isn’t actually connected to your laptop. When using trial and error, you would continue to try different solutions until you solved your problem. Although trial and error is not typically one of the most time-efficient strategies, it is a commonly used one.
Another type of strategy is an algorithm. An algorithm is a problem-solving formula that provides you with step-by-step instructions used to achieve a desired outcome (Kahneman, 2011). You can think of an algorithm as a recipe with highly detailed instructions that produce the same result every time they are performed. Algorithms are used frequently in our everyday lives, especially in computer science. When you run a search on the Internet, search engines like Google use algorithms to decide which entries will appear first in your list of results. Facebook also uses algorithms to decide which posts to display on your newsfeed. Can you identify other situations in which algorithms are used?
A heuristic is another type of problem solving strategy. While an algorithm must be followed exactly to produce a correct result, a heuristic is a general problem-solving framework (Tversky & Kahneman, 1974). You can think of these as mental shortcuts that are used to solve problems. A “rule of thumb” is an example of a heuristic. Such a rule saves the person time and energy when making a decision, but despite its time-saving characteristics, it is not always the best method for making a rational decision. Different types of heuristics are used in different types of situations, but the impulse to use a heuristic occurs when one of five conditions is met (Pratkanis, 1989):
- When one is faced with too much information
- When the time to make a decision is limited
- When the decision to be made is unimportant
- When there is access to very little information to use in making the decision
- When an appropriate heuristic happens to come to mind in the same moment
Working backwards is a useful heuristic in which you begin solving the problem by focusing on the end result. Consider this example: You live in Washington, D.C. and have been invited to a wedding at 4 PM on Saturday in Philadelphia. Knowing that Interstate 95 tends to back up any day of the week, you need to plan your route and time your departure accordingly. If you want to be at the wedding service by 3:30 PM, and it takes 2.5 hours to get to Philadelphia without traffic, what time should you leave your house? You use the working backwards heuristic to plan the events of your day on a regular basis, probably without even thinking about it.
What problem-solving method could you use to solve Einstein’s famous riddle?
You can view the transcript for “Can you solve “Einstein’s Riddle”? – Dan Van der Vieren” here (opens in new window) .
Another useful heuristic is the practice of accomplishing a large goal or task by breaking it into a series of smaller steps. Students often use this common method to complete a large research project or long essay for school. For example, students typically brainstorm, develop a thesis or main topic, research the chosen topic, organize their information into an outline, write a rough draft, revise and edit the rough draft, develop a final draft, organize the references list, and proofread their work before turning in the project. The large task becomes less overwhelming when it is broken down into a series of small steps.
Everyday Connections: Solving Puzzles
Problem-solving abilities can improve with practice. Many people challenge themselves every day with puzzles and other mental exercises to sharpen their problem-solving skills. Sudoku puzzles appear daily in most newspapers. Typically, a sudoku puzzle is a 9×9 grid. The simple sudoku below (Figure 14) is a 4×4 grid. To solve the puzzle, fill in the empty boxes with a single digit: 1, 2, 3, or 4. Here are the rules: The numbers must total 10 in each bolded box, each row, and each column; however, each digit can only appear once in a bolded box, row, and column. Time yourself as you solve this puzzle and compare your time with a classmate.
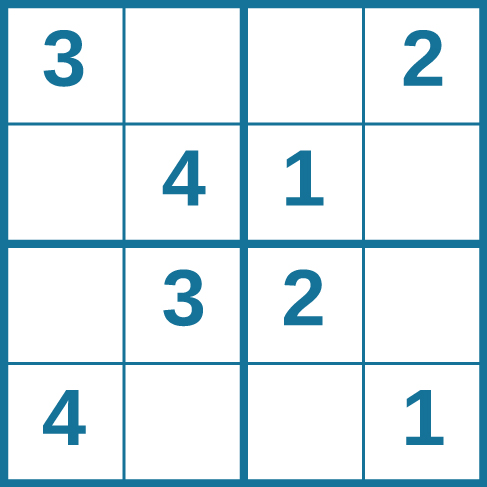
Here is another popular type of puzzle that challenges your spatial reasoning skills. Connect all nine dots with four connecting straight lines without lifting your pencil from the paper:
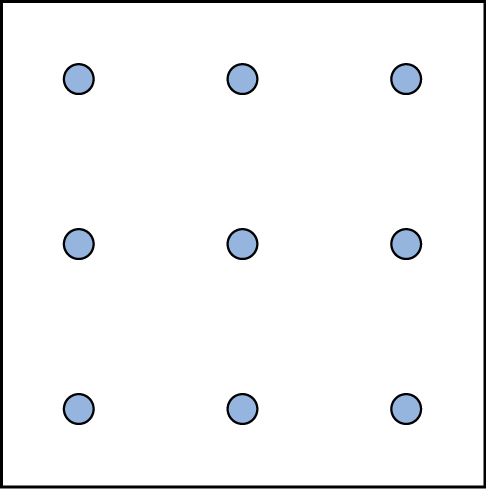
Take a look at the “Puzzling Scales” logic puzzle below (Figure 16). Sam Loyd, a well-known puzzle master, created and refined countless puzzles throughout his lifetime (Cyclopedia of Puzzles, n.d.).
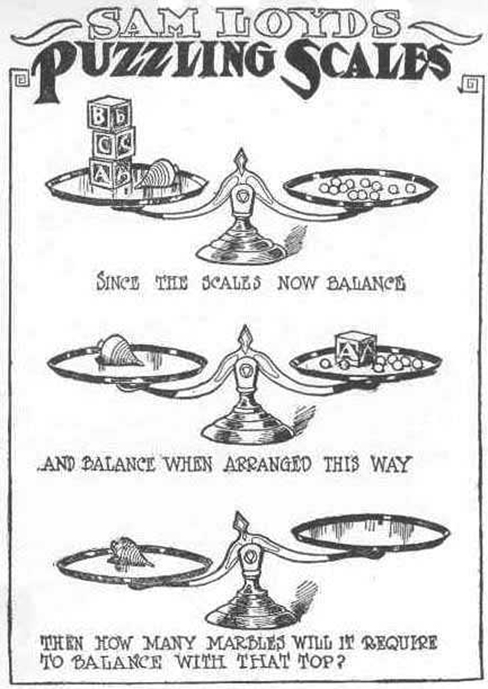
Were you able to determine how many marbles are needed to balance the scales in the Puzzling Scales? You need nine. Were you able to solve the other problems above? Here are the answers:
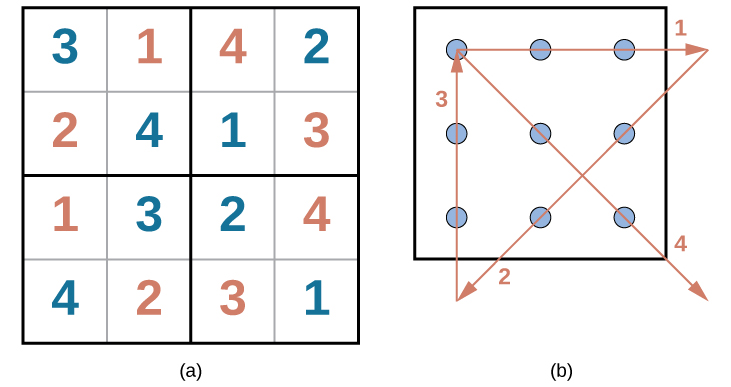
Pitfalls to Problem Solving
Not all problems are successfully solved, however. What challenges stop us from successfully solving a problem? Albert Einstein once said, “Insanity is doing the same thing over and over again and expecting a different result.” Imagine a person in a room that has four doorways. One doorway that has always been open in the past is now locked. The person, accustomed to exiting the room by that particular doorway, keeps trying to get out through the same doorway even though the other three doorways are open. The person is stuck—but she just needs to go to another doorway, instead of trying to get out through the locked doorway. A mental set is where you persist in approaching a problem in a way that has worked in the past but is clearly not working now. Functional fixedness is a type of mental set where you cannot perceive an object being used for something other than what it was designed for. During the Apollo 13 mission to the moon, NASA engineers at Mission Control had to overcome functional fixedness to save the lives of the astronauts aboard the spacecraft. An explosion in a module of the spacecraft damaged multiple systems. The astronauts were in danger of being poisoned by rising levels of carbon dioxide because of problems with the carbon dioxide filters. The engineers found a way for the astronauts to use spare plastic bags, tape, and air hoses to create a makeshift air filter, which saved the lives of the astronauts.
In order to make good decisions, we use our knowledge and our reasoning. Often, this knowledge and reasoning is sound and solid. Sometimes, however, we are swayed by biases or by others manipulating a situation. For example, let’s say you and three friends wanted to rent a house and had a combined target budget of $1,600. The realtor shows you only very run-down houses for $1,600 and then shows you a very nice house for $2,000. Might you ask each person to pay more in rent to get the $2,000 home? Why would the realtor show you the run-down houses and the nice house? The realtor may be challenging your anchoring bias. An anchoring bias occurs when you focus on one piece of information when making a decision or solving a problem. In this case, you’re so focused on the amount of money you are willing to spend that you may not recognize what kinds of houses are available at that price point.
The confirmation bias is the tendency to focus on information that confirms your existing beliefs. For example, if you think that your professor is not very nice, you notice all of the instances of rude behavior exhibited by the professor while ignoring the countless pleasant interactions he is involved in on a daily basis. This bias proves that first impressions do matter and that we tend to look for information to confirm our initial judgments of others.
Watch this video from the Big Think to learn more about the confirmation bias.
You can view the transcript for “Confirmation Bias: Your Brain is So Judgmental” here (opens in new window) .
Hindsight bias leads you to believe that the event you just experienced was predictable, even though it really wasn’t. In other words, you knew all along that things would turn out the way they did. Representative bias describes a faulty way of thinking, in which you unintentionally stereotype someone or something; for example, you may assume that your professors spend their free time reading books and engaging in intellectual conversation, because the idea of them spending their time playing volleyball or visiting an amusement park does not fit in with your stereotypes of professors.
Finally, the availability heuristic is a heuristic in which you make a decision based on an example, information, or recent experience that is that readily available to you, even though it may not be the best example to inform your decision . To use a common example, would you guess there are more murders or more suicides in America each year? When asked, most people would guess there are more murders. In truth, there are twice as many suicides as there are murders each year. However, murders seem more common because we hear a lot more about murders on an average day. Unless someone we know or someone famous takes their own life, it does not make the news. Murders, on the other hand, we see in the news every day. This leads to the erroneous assumption that the easier it is to think of instances of something, the more often that thing occurs.
Watch the following video for an example of the availability heuristic.
You can view the transcript for “Availability Heuristic: Are Planes More Dangerous Than Cars?” here (opens in new window) .
Biases tend to “preserve that which is already established—to maintain our preexisting knowledge, beliefs, attitudes, and hypotheses” (Aronson, 1995; Kahneman, 2011). These biases are summarized in Table 2 below.
Learn more about heuristics and common biases through the article, “ 8 Common Thinking Mistakes Our Brains Make Every Day and How to Prevent Them ” by Belle Beth Cooper.
You can also watch this clever music video explaining these and other cognitive biases.
Which type of bias do you recognize in your own decision making processes? How has this bias affected how you’ve made decisions in the past and how can you use your awareness of it to improve your decisions making skills in the future?
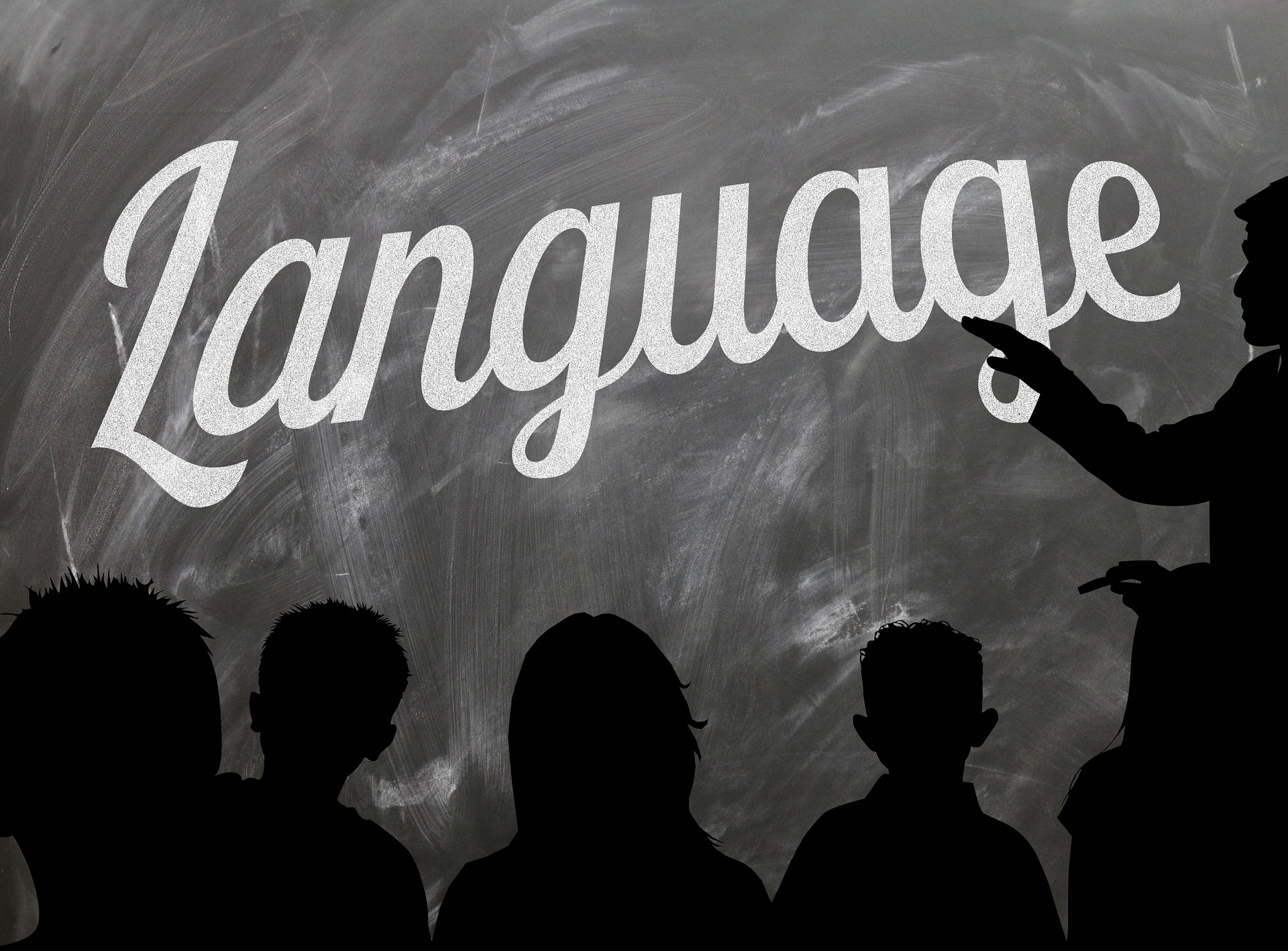
- Understand how the use of language develops
- Explain the relationship between language and thinking
Language Development
Language is a communication system that involves using words and systematic rules to organize those words to transmit information from one individual to another. While language is a form of communication, not all communication is language. Many species communicate with one another through their postures, movements, odors, or vocalizations. This communication is crucial for species that need to interact and develop social relationships with their conspecifics. However, many people have asserted that it is language that makes humans unique among all of the animal species (Corballis & Suddendorf, 2007; Tomasello & Rakoczy, 2003). This section will focus on what distinguishes language as a special form of communication, how the use of language develops, and how language affects the way we think.
Components of Language
Language , be it spoken, signed, or written, has specific components: a lexicon and grammar. Lexicon refers to the words of a given language. Thus, lexicon is a language’s vocabulary. Grammar refers to the set of rules that are used to convey meaning through the use of the lexicon (Fernández & Cairns, 2011). For instance, English grammar dictates that most verbs receive an “-ed” at the end to indicate past tense.
Words are formed by combining the various phonemes that make up the language. A phoneme (e.g., the sounds “ah” vs. “eh”) is a basic sound unit of a given language, and different languages have different sets of phonemes. Phonemes are combined to form morphemes , which are the smallest units of language that convey some type of meaning (e.g., “I” is both a phoneme and a morpheme). Further, a morpheme is not the same as a word. The main difference is that a morpheme sometimes does not stand alone, but a word, by definition, always stands alone.
We use semantics and syntax to construct language. Semantics and syntax are part of a language’s grammar. Semantics refers to the process by which we derive meaning from morphemes and words. Syntax refers to the way words are organized into sentences (Chomsky, 1965; Fernández & Cairns, 2011).
We apply the rules of grammar to organize the lexicon in novel and creative ways, which allow us to communicate information about both concrete and abstract concepts. We can talk about our immediate and observable surroundings as well as the surface of unseen planets. We can share our innermost thoughts, our plans for the future, and debate the value of a college education. We can provide detailed instructions for cooking a meal, fixing a car, or building a fire. The flexibility that language provides to relay vastly different types of information is a property that makes language so distinct as a mode of communication among humans.
Given the remarkable complexity of a language, one might expect that mastering a language would be an especially arduous task; indeed, for those of us trying to learn a second language as adults, this might seem to be true. However, young children master language very quickly with relative ease. B. F. Skinner (1957) proposed that language is learned through reinforcement. Noam Chomsky (1965) criticized this behaviorist approach, asserting instead that the mechanisms underlying language acquisition are biologically determined. The use of language develops in the absence of formal instruction and appears to follow a very similar pattern in children from vastly different cultures and backgrounds. It would seem, therefore, that we are born with a biological predisposition to acquire a language (Chomsky, 1965; Fernández & Cairns, 2011). Moreover, it appears that there is a critical period for language acquisition, such that this proficiency at acquiring language is maximal early in life; generally, as people age, the ease with which they acquire and master new languages diminishes (Johnson & Newport, 1989; Lenneberg, 1967; Singleton, 1995).
Children begin to learn about language from a very early age (Table 1). In fact, it appears that this is occurring even before we are born. Newborns show preference for their mother’s voice and appear to be able to discriminate between the language spoken by their mother and other languages. Babies are also attuned to the languages being used around them and show preferences for videos of faces that are moving in synchrony with the audio of spoken language versus videos that do not synchronize with the audio (Blossom & Morgan, 2006; Pickens, 1994; Spelke & Cortelyou, 1981).
Dig Deeper: The Case of Genie
In the fall of 1970, a social worker in the Los Angeles area found a 13-year-old girl who was being raised in extremely neglectful and abusive conditions. The girl, who came to be known as Genie, had lived most of her life tied to a potty chair or confined to a crib in a small room that was kept closed with the curtains drawn. For a little over a decade, Genie had virtually no social interaction and no access to the outside world. As a result of these conditions, Genie was unable to stand up, chew solid food, or speak (Fromkin, Krashen, Curtiss, Rigler, & Rigler, 1974; Rymer, 1993). The police took Genie into protective custody.
Genie’s abilities improved dramatically following her removal from her abusive environment, and early on, it appeared she was acquiring language—much later than would be predicted by critical period hypotheses that had been posited at the time (Fromkin et al., 1974). Genie managed to amass an impressive vocabulary in a relatively short amount of time. However, she never developed a mastery of the grammatical aspects of language (Curtiss, 1981). Perhaps being deprived of the opportunity to learn language during a critical period impeded Genie’s ability to fully acquire and use language.
You may recall that each language has its own set of phonemes that are used to generate morphemes, words, and so on. Babies can discriminate among the sounds that make up a language (for example, they can tell the difference between the “s” in vision and the “ss” in fission); early on, they can differentiate between the sounds of all human languages, even those that do not occur in the languages that are used in their environments. However, by the time that they are about 1 year old, they can only discriminate among those phonemes that are used in the language or languages in their environments (Jensen, 2011; Werker & Lalonde, 1988; Werker & Tees, 1984).
After the first few months of life, babies enter what is known as the babbling stage, during which time they tend to produce single syllables that are repeated over and over. As time passes, more variations appear in the syllables that they produce. During this time, it is unlikely that the babies are trying to communicate; they are just as likely to babble when they are alone as when they are with their caregivers (Fernández & Cairns, 2011). Interestingly, babies who are raised in environments in which sign language is used will also begin to show babbling in the gestures of their hands during this stage (Petitto, Holowka, Sergio, Levy, & Ostry, 2004).
Generally, a child’s first word is uttered sometime between the ages of 1 year to 18 months, and for the next few months, the child will remain in the “one word” stage of language development. During this time, children know a number of words, but they only produce one-word utterances. The child’s early vocabulary is limited to familiar objects or events, often nouns. Although children in this stage only make one-word utterances, these words often carry larger meaning (Fernández & Cairns, 2011). So, for example, a child saying “cookie” could be identifying a cookie or asking for a cookie.
As a child’s lexicon grows, she begins to utter simple sentences and to acquire new vocabulary at a very rapid pace. In addition, children begin to demonstrate a clear understanding of the specific rules that apply to their language(s). Even the mistakes that children sometimes make provide evidence of just how much they understand about those rules. This is sometimes seen in the form of overgeneralization . In this context, overgeneralization refers to an extension of a language rule to an exception to the rule. For example, in English, it is usually the case that an “s” is added to the end of a word to indicate plurality. For example, we speak of one dog versus two dogs. Young children will overgeneralize this rule to cases that are exceptions to the “add an s to the end of the word” rule and say things like “those two gooses” or “three mouses.” Clearly, the rules of the language are understood, even if the exceptions to the rules are still being learned (Moskowitz, 1978).
Language and Thinking
Think about it: the meaning of language.
Think about what you know of other languages; perhaps you even speak multiple languages. Imagine for a moment that your closest friend fluently speaks more than one language. Do you think that friend thinks differently, depending on which language is being spoken? You may know a few words that are not translatable from their original language into English. For example, the Portuguese word saudade originated during the 15th century, when Portuguese sailors left home to explore the seas and travel to Africa or Asia. Those left behind described the emptiness and fondness they felt as saudade (Figure 20) . The word came to express many meanings, including loss, nostalgia, yearning, warm memories, and hope. There is no single word in English that includes all of those emotions in a single description. Do words such as saudade indicate that different languages produce different patterns of thought in people? What do you think??
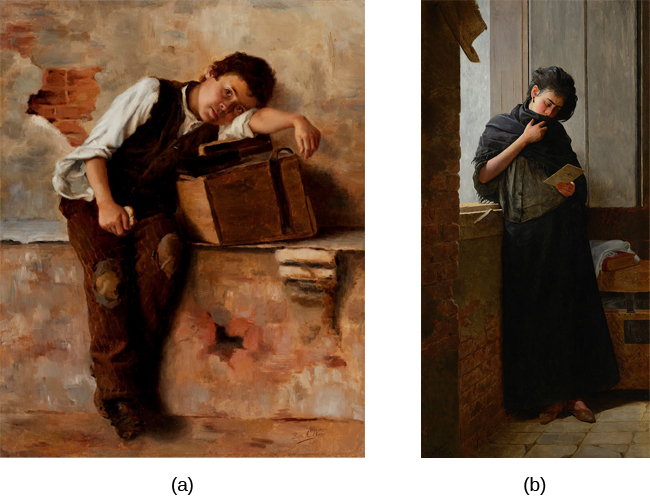
Language may indeed influence the way that we think, an idea known as linguistic determinism. One recent demonstration of this phenomenon involved differences in the way that English and Mandarin Chinese speakers talk and think about time. English speakers tend to talk about time using terms that describe changes along a horizontal dimension, for example, saying something like “I’m running behind schedule” or “Don’t get ahead of yourself.” While Mandarin Chinese speakers also describe time in horizontal terms, it is not uncommon to also use terms associated with a vertical arrangement. For example, the past might be described as being “up” and the future as being “down.” It turns out that these differences in language translate into differences in performance on cognitive tests designed to measure how quickly an individual can recognize temporal relationships. Specifically, when given a series of tasks with vertical priming, Mandarin Chinese speakers were faster at recognizing temporal relationships between months. Indeed, Boroditsky (2001) sees these results as suggesting that “habits in language encourage habits in thought” (p. 12).
Language does not completely determine our thoughts—our thoughts are far too flexible for that—but habitual uses of language can influence our habit of thought and action. For instance, some linguistic practice seems to be associated even with cultural values and social institution. Pronoun drop is the case in point. Pronouns such as “I” and “you” are used to represent the speaker and listener of a speech in English. In an English sentence, these pronouns cannot be dropped if they are used as the subject of a sentence. So, for instance, “I went to the movie last night” is fine, but “Went to the movie last night” is not in standard English. However, in other languages such as Japanese, pronouns can be, and in fact often are, dropped from sentences. It turned out that people living in those countries where pronoun drop languages are spoken tend to have more collectivistic values (e.g., employees having greater loyalty toward their employers) than those who use non–pronoun drop languages such as English (Kashima & Kashima, 1998). It was argued that the explicit reference to “you” and “I” may remind speakers the distinction between the self and other, and the differentiation between individuals. Such a linguistic practice may act as a constant reminder of the cultural value, which, in turn, may encourage people to perform the linguistic practice.
One group of researchers who wanted to investigate how language influences thought compared how English speakers and the Dani people of Papua New Guinea think and speak about color. The Dani have two words for color: one word for light and one word for dark . In contrast, the English language has 11 color words. Researchers hypothesized that the number of color terms could limit the ways that the Dani people conceptualized color. However, the Dani were able to distinguish colors with the same ability as English speakers, despite having fewer words at their disposal (Berlin & Kay, 1969). A recent review of research aimed at determining how language might affect something like color perception suggests that language can influence perceptual phenomena, especially in the left hemisphere of the brain. You may recall from earlier chapters that the left hemisphere is associated with language for most people. However, the right (less linguistic hemisphere) of the brain is less affected by linguistic influences on perception (Regier & Kay, 2009)
Learn more about language, language acquisition, and especially the connection between language and thought in the following CrashCourse video:
You can view the transcript for “Language: Crash Course Psychology #16” here (opens in new window) .
In this chapter, you learned to
- describe attention
- describe cognition and problem-solving strategies
- describe language acquisition and the role language plays in communication and thought
You learned about non-memory cognitive processes in this chapter. Because each of you reading this is using language in some shape or form, we will end with a quick summary and a video on this topic. Language is a communication system that has both a lexicon and a system of grammar. Language acquisition occurs naturally and effortlessly during the early stages of life, and this acquisition occurs in a predictable sequence for individuals around the world. Language has a strong influence on thought, and the concept of how language may influence cognition remains an area of study and debate in psychology.
In this TED talk, Lera Boroditsky summarizes unique ways that language and culture intersect with some basic cognitive processes. How was your language shaped your thinking?
Abler, W. (2013). Sapir, Harris, and Chomsky in the twentieth century. Cognitive Critique, 7, 29–48.
Aronson, E. (Ed.). (1995). Social cognition. In The social animal (p. 151). New York: W.H. Freeman and Company.
Bartlett, F. C. (1932). Remembering: A study in experimental and social psychology. Cambridge, England: Cambridge University Press.
Bayer, J. B., & Campbell, S. W. (2012). Texting while driving on automatic: Considering the frequency-independent side of habit. Computers in Human Behavior, 28, 2083–2090.
Beilock, S. L., & Carr, T. H. (2001). On the fragility of skilled performance: What governs choking under pressure? Journal of Experimental Psychology: General, 130 , 701–725.
Berlin, B., & Kay, P. (1969). Basic color terms: Their universality and evolution. Berkley: University of California Press.
Blossom, M., & Morgan, J. L. (2006). Does the face say what the mouth says? A study of infants’ sensitivity to visual prosody. In the 30th annual Boston University Conference on Language Development, Somerville, MA.
Boroditsky, L. (2001). Does language shape thought? Mandarin and English speakers’ conceptions of time. Cognitive Psychology, 43, 1–22.
Boroditsky, L. (2011, February). How language shapes thought. Scientific American, 63–65.Chomsky, N. (1965). Aspects of the theory of syntax. Cambridge, MA: MIT Press
Broadbent, D. A. (1958). Perception and communication . London, England: Pergamon Press.
Cairns Regional Council. (n.d.). Cultural greetings. Retrieved from http://www.cairns.qld.gov.au/__data/assets/pdf_file/0007/8953/CulturalGreetingExercise.pdf
Callero, P. L. (1994). From role-playing to role-using: Understanding role as resource. Social Psychology Quarterly, 57, 228–243.
Cherry, E. C. (1953). Experiments on the recognition of speech with one and two ears. Journal of the Acoustical Society of America, 25 , 975–979.
Chomsky, N.(1965). Aspects of the theory of syntax. Cambridge, MA: MIT Press
Corballis, M. C., & Suddendorf, T. (2007). Memory, time, and language. In C. Pasternak (Ed.), What makes us human (pp. 17–36). Oxford, UK: Oneworld Publications.
Curtiss, S. (1981). Dissociations between language and cognition: Cases and implications. Journal of Autism and Developmental Disorders, 11(1), 15–30.
Cyclopedia of Puzzles. (n.d.) Retrieved from http://www.mathpuzzle.com/loyd/
Deutsch, J. A., & Deutsch, D. (1963). Attention: some theoretical considerations. Psychological Review, 70 , 80–90.
Fernández, E. M., & Cairns, H. S. (2011). Fundamentals of psycholinguistics. West Sussex, UK: Wiley-Blackwell.
Fromkin, V., Krashen, S., Curtiss, S., Rigler, D., & Rigler, M. (1974). The development of language in Genie: A case of language acquisition beyond the critical period. Brain and Language, 1, 81–107.
German, T. P., & Barrett, H. C. (2005). Functional fixedness in a technologically sparse culture. Psychological Science, 16, 1–5.
Goldstone, R. L., & Kersten, A. (2003). Concepts and categorization. In A. F. Healy, R. W. Proctor, & I.B. Weiner (Eds.), Handbook of psychology (Volume IV, pp. 599–622). Hoboken, New Jersey: John Wiley & Sons, Inc.
Hirst, W. C., Neisser, U., & Spelke, E. S. (1978). Divided attention. Human Nature, 1 , 54–61.
James, W. (1983). The principles of psychology . Cambridge, MA: Harvard University Press. (Original work published 1890)
Jensen, J. (2011). Phoneme acquisition: Infants and second language learners. The Language Teacher, 35(6), 24–28.
Johnson, J. S., & Newport, E. L. (1989). Critical period effects in second language learning: The influence of maturational state on the acquisition of English as a second language. Cognitive Psychology, 21, 60–99.
Johnston, W. A., & Heinz, S. P. (1978). Flexibility and capacity demands of attention. Journal of Experimental Psychology: General, 107 , 420–435.
Kahneman, D. (2011). Thinking, fast and slow. New York: Farrar, Straus, and Giroux.
Lenneberg, E. (1967). Biological foundations of language. New York: Wiley.
Monsell, S. (2003). Task switching. Trends in Cognitive Science, 7 (3), 134–140.
Moray, N. (1959). Attention in dichotic listening: Affective cues and the influence of instructions. Quarterly Journal of Experimental Psychology, 11 , 56–60.
Moskowitz, B. A. (1978). The acquisition of language. Scientific American, 239, 92–108. Petitto, L. A., Holowka, S., Sergio, L. E., Levy, B., & Ostry, D. J. (2004). Baby hands that move to the rhythm of language: Hearing babies acquiring sign languages babble silently on the hands. Cognition, 93, 43–73.
Neyfakh, L. (2013, October 7). “Why you can’t stop checking your phone.” Retrieved from http://www.bostonglobe.com/ideas/2013/10/06/why-you-can-stop-checking-your-phone/rrBJzyBGDAr1YlEH5JQDcM/story.html
Petitto, L. A., Holowka, S., Sergio, L. E., Levy, B., & Ostry, D. J. (2004). Baby hands that move to the rhythm of language: Hearing babies acquiring sign languages babble silently on the hands. Cognition, 93, 43–73.
Pickens, J. (1994). Full-term and preterm infants’ perception of face-voice synchrony. Infant Behavior and Development, 17, 447–455.
Pratkanis, A. (1989). The cognitive representation of attitudes. In A. R. Pratkanis, S. J. Breckler, & A. G. Greenwald (Eds.), Attitude structure and function (pp. 71–98). Hillsdale, NJ: Erlbaum.
Regier, T., & Kay, P. (2009). Language, thought, and color: Whorf was half right. Trends in Cognitive Sciences, 13(10), 439–446.
Rymer, R. (1993). Genie: A Scientific Tragedy. New York: Harper Collins.
Sapir, E. (1964). Culture, language, and personality. Berkley: University of California Press. (Original work published 1941)
Simons, D. J., & Chabris, C. F. (1999). Gorillas in our midst: Sustained inattentional blindness for dynamic events. Perception, 28 , 1059–1074.
Skinner, B. F. (1957). Verbal behavior. Acton, MA: Copley Publishing Group.
Spelke, E. S., & Cortelyou, A. (1981). Perceptual aspects of social knowing: Looking and listening in infancy. In M.E. Lamb & L.R. Sherrod (Eds.), Infant social cognition: Empirical and theoretical considerations (pp. 61–83). Hillsdale, NJ: Erlbaum.
Spelke, E. S., Hirst, W. C., & Neisser, U. (1976). Skills of divided attention. Cognition, 4 , 215–250.
Strayer, D. L., & Drews, F. A. (2007). Cell-phone induced inattention blindness. Current Directions in Psychological Science, 16 , 128–131.
Strayer, D. L., & Johnston, W. A. (2001). Driven to distraction: Dual-task studies of simulated driving and conversing on a cellular telephone. Psychological Science, 12 , 462–466.
Strayer, D. L., Watson, J. M., & Drews, F. A. (2011) Cognitive distraction while multitasking in the automobile. In Brian Ross (Ed.), The Psychology of Learning and Motivation (Vol. 54, pp. 29–58). Burlington, VT: Academic Press.
Tomasello, M., & Rakoczy, H. (2003). What makes human cognition unique? From individual to shared to collective intentionality. Mind & Language, 18(2), 121–147.
Treisman, A. (1960). Contextual cues in selective listening. Quarterly Journal of Experimental Psychology, 12 , 242–248.
Tversky, A., & Kahneman, D. (1974). Judgment under uncertainty: Heuristics and biases. Science, 185(4157), 1124–1131.
van Troyer, G. (1994). Linguistic determinism and mutability: The Sapir-Whorf “hypothesis” and intercultural communication. JALT Journal, 2, 163–178.
Watson, J. M., & Strayer, D. L. (2010). Supertaskers: Profiles in extraordinary multitasking ability. Psychonomic Bulletin & Review, 17 , 479–485.
Werker, J. F., & Lalonde, C. E. (1988). Cross-language speech perception: Initial capabilities and developmental change. Developmental Psychology, 24, 672–683.
Werker, J. F., & Tees, R. C. (1984). Cross-language speech perception: Evidence for perceptual reorganization during the first year of life. Infant Behavior and Development, 7, 49–63.
Whorf, B. L. (1956). Language, thought and relativity. Cambridge, MA: MIT Press.
CC original content.
Attention, Thinking and Language. Authored by: Karenna Malavanti Provided by: PressBooks. License: CC BY-SA: Attribution-ShareAlike
CC licensed content, Shared previously
- Why It Matters: Thinking and Intelligence. Authored by : Lumen Learning License : CC BY: Attribution Located at : https://pressbooks.online.ucf.edu/lumenpsychology/chapter/introduction-10/
- Attention. Authored by: Frances Friedrich. Located at NOBA Psychology. License: CC-BY-NC-SA. Retrieved from: Retrieved from http://noba.to/uv9x8df5
- Introduction to Thinking and Intelligence. Authored by : OpenStax College. License : CC BY: Attribution . License Terms : Download for free at https://openstax.org/books/psychology-2e/pages/1-introduction Located at : https://openstax.org/books/psychology-2e/pages/7-introduction .
- What Is Cognition?. Authored by : OpenStax College. License : CC BY: Attribution . License Terms : Download for free at https://openstax.org/books/psychology-2e/pages/1-introduction Located at : https://openstax.org/books/psychology-2e/pages/7-1-what-is-cognition .
- A Thinking Man Image. Authored by : Wesley Nitsckie. License : CC BY-SA: Attribution-ShareAlike Located at : https://www.flickr.com/photos/nitsckie/5507777269 .
- What Is Cognition?. Authored by : Lumen Learning License : CC BY: Attribution Located at : https://pressbooks.online.ucf.edu/lumenpsychology/chapter/what-is-cognition/
- Categories and Concepts. Authored by : Gregory Murphy. Provided by : New York University. Project : The Noba Project. License : CC BY-NC-SA: Attribution-NonCommercial-ShareAlike Located at : http://nobaproject.com/textbooks/wendy-king-introduction-to-psychology-the-full-noba-collection/modules/categories-and-concepts .
- Solving Problems. Authored by : Lumen Learning License : CC BY: Attribution Located at : https://pressbooks.online.ucf.edu/lumenpsychology/chapter/problem-solving/
- Problem-Solving. Authored by : OpenStax College. License : CC BY: Attribution . License Terms : Download for free at https://openstax.org/books/psychology-2e/pages/1-introduction . Located at : https://openstax.org/books/psychology-2e/pages/7-3-problem-solving .
- Pitfalls to Problem Solving. Authored by : Lumen Learning License : CC BY: Attribution Located at : https://pressbooks.online.ucf.edu/lumenpsychology/chapter/reading-pitfalls-to-problem/
- Introduction to Language. Authored by : Lumen Learning License : CC BY: Attribution Located at : https://pressbooks.online.ucf.edu/lumenpsychology/chapter/outcome-language/
- Language. Authored by : OpenStax College. License : CC BY: Attribution . License Terms : Download for free at https://openstax.org/books/psychology-2e/pages/1-introduction Located at : https://openstax.org/books/psychology-2e/pages/7-2-language .
- Language. Authored by : geralt. Provided by : Pixabay. License : CC0: No Rights Reserved Located at : https://pixabay.com/en/school-board-languages-blackboard-1063556/ .
- Language and Language Use. Authored by : Lumen Learning License : CC BY: Attribution Located at : https://pressbooks.online.ucf.edu/lumenpsychology/chapter/language-and-language-use/
- Language and Language Use. Authored by : Yoshihisa Kashima. Project : The Noba Project. License : CC BY-NC-SA: Attribution-NonCommercial-ShareAlike Located at : http://nobaproject.com/textbooks/introduction-to-psychology-the-full-noba-collection/modules/language-and-language-use .
- Language Development. Authored by : Lumen Learning License : CC BY: Attribution Located at : https://pressbooks.online.ucf.edu/lumenpsychology/chapter/language/
- Morpheme. Provided by : Wikipedia. License : CC BY-SA: Attribution-ShareAlike Located at : https://en.wikipedia.org/wiki/Morpheme .
- Language and Thinking. Authored by : Lumen Learning License : CC BY: Attribution Located at : https://pressbooks.online.ucf.edu/lumenpsychology/chapter/reading-language-and-thought/
- Summary. Authored by : OpenStax College. License : CC BY: Attribution . License Terms : Download for free at https://openstax.org/books/psychology-2e/pages/1-introduction . Located at : https://openstax.org/books/psychology-2e/pages/7-summary .
All rights reserved content
- Cognition: How Your Mind Can Amaze and Betray You – Crash Course Psychology #15. Provided by : CrashCourse. License : All Rights Reserved . License Terms : Standard YouTube License Located at : https://www.youtube.com/watch?v=R-sVnmmw6WY&feature=youtu.be&list=PL8dPuuaLjXtOPRKzVLY0jJY-uHOH9KVU6 .
- Can you solve Einsteinu2019s Riddle? . Authored by : Dan Van der Vieren. Provided by : Ted-Ed. License : Other . License Terms : Standard YouTube License . Located at : https://www.youtube.com/watch?v=1rDVz_Fb6HQ&index=3&list=PLUmyCeox8XCwB8FrEfDQtQZmCc2qYMS5a .
- Language: Crash Course Psychology #16. Authored by : CrashCourse. License : Other . License Terms : Standard YouTube License . Located at : https://www.youtube.com/watch?v=s9shPouRWCs&feature=youtu.be&list=PL8dPuuaLjXtOPRKzVLY0jJY-uHOH9KVU6 .
- How language shapes the way we think Authored by: Lera Boroditsky. Provided by : TED. License : Other . License Terms : Standard YouTube License . Located at : https://youtu.be/RKK7wGAYP6k
thinking, including perception, learning, problem solving, judgment, and memory
field of psychology dedicated to studying every aspect of how people think
a set of objects that can be treated as equivalent in some way
category or grouping of linguistic information, objects, ideas, or life experiences
best representation of a concept
mental groupings that are created “naturally” through your experiences
concept that is defined by a very specific set of characteristics
(plural = schemata) mental construct consisting of a cluster or collection of related concepts
set of expectations that define the behaviors of a person occupying a particular role
set of behaviors that are performed the same way each time; also referred to as a cognitive script
set of behaviors that are performed the same way each time; also referred to as an event schema
method for solving problems
problem-solving strategy in which multiple solutions are attempted until the correct one is found
problem-solving strategy characterized by a specific set of instructions
mental shortcut that saves time when solving a problem
heuristic in which you begin to solve a problem by focusing on the end result
continually using an old solution to a problem without results
inability to see an object as useful for any other use other than the one for which it was intended
faulty heuristic in which you fixate on a single aspect of a problem to find a solution
belief that the event just experienced was predictable, even though it really wasn’t
subset of the population that accurately represents the general population
faulty heuristic in which you make a decision based on information readily available to you
communication system that involves using words to transmit information from one individual to another
Words and expressions.
set of rules that are used to convey meaning through the use of a lexicon
basic sound unit of a given language
smallest unit of language that conveys some type of meaning
process by which we derive meaning from morphemes and words
manner by which words are organized into sentences
extension of a rule that exists in a given language to an exception to the rule
Psychological Science: Understanding Human Behavior Copyright © by Karenna Malavanti is licensed under a Creative Commons Attribution-NonCommercial-ShareAlike 4.0 International License , except where otherwise noted.
Share This Book
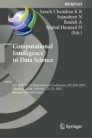
International Conference on Computational Intelligence in Data Science
ICCIDS 2023: Computational Intelligence in Data Science pp 141–152 Cite as
The Use of the Semantic Network for Categorization of Problems Solved by Design Thinking
- Iwona Chomiak-Orsa ORCID: orcid.org/0000-0003-3550-8624 19 ,
- Andrzej Greńczuk ORCID: orcid.org/0000-0002-0464-8555 19 &
- Kamila Łuczak ORCID: orcid.org/0000-0002-7085-5704 19
- Conference paper
- First Online: 22 July 2023
97 Accesses
Part of the book series: IFIP Advances in Information and Communication Technology ((IFIPAICT,volume 673))
Creativity is one of the most awaited professional competences of their employees by modern entrepreneurs. Therefore, more and more importance are attached to the development and application of tools and methods supporting creativity both in the individual and team dimension. This became the main stimulus for the development of methods of creative thinking and problem solving. One of such methods, gaining more and more popularity, is Design Thinking. Despite the numerous advantages of this method, it should be realized that it cannot be used in every case. Therefore, the authors of this article defined the purpose of the article as: the use of semantic networks in the categorization of problems that can be solved with the use of Design Thinking. The research results presented in the article were obtained using triangulation of research methods such as: analytical literature review, computer simulation carried out using the Protégé program and a research experiment consisting in the simulation of selected problem situations.
- Design Thinking (DT)
This is a preview of subscription content, log in via an institution .
Buying options
- Available as PDF
- Read on any device
- Instant download
- Own it forever
- Available as EPUB and PDF
- Durable hardcover edition
- Dispatched in 3 to 5 business days
- Free shipping worldwide - see info
Tax calculation will be finalised at checkout
Purchases are for personal use only
Barsalou, L.W.: Define design thinking. She Ji: The J. Des. Econ. Innovation 3 (2), 102–105 (2017). https://doi.org/10.1016/j.sheji.2017.10.007
Article Google Scholar
Baruk, J.: Innowacje jako czynnik sukcesu organizacji. Zarządzanie i finanse 4 (1), 7–16 (2013)
Google Scholar
Cardoso, J., Pinto, A.M.: The Web Ontology Language (OWL) and its applications. In: Encyclopedia of Information Science and Technology, Information Science Pub (2014). https://doi.org/10.4018/978-1-4666-5888-2.ch756
Cross, N.: Design thinking: Understanding how designers think and work. Bloomsbury Publishing (2023)
den Dekker, T.: Design thinking. Routledge (2020)
Book Google Scholar
Dorst, K.: The core of ‘design thinking’ and its application. Des. Stud. 32 (6), 521–532 (2010). https://doi.org/10.1016/j.destud.2011.07.006
Dunne, D.: Design thinking at Work: How Innovative Organizations are Embracing Design. University of Toronto Press (2018)
Emami, M., Rezaei, S., Valaei, N., Gardener, J.: Creativity mindset as the organizational capability: the role of creativity-relevant processes, domain-relevant skills and intrinsic task motivation. Asia-Pacific J. Bus. Adm. 15 (1), 139–160 (2023)
Krzepicka, A., Tarapata, J.: Innowacje jako czynnik budowania wzrostu wartości przedsiębiorstwa. Zeszyty Naukowe Wyższej Szkoły Humanitas. Zarządzanie 2 , 74–89 (2012)
Lewrick, M., Link, P., Leifer, L.: The Design Thinking Toolbox: A Guide to Mastering the Most Popular and Valuable Innovation Methods. John Wiley & Sons (2020)
Matthews, B., Doherty, S., Worthy, P., Reid, J.: Design thinking, wicked problems and institutioning change: a case study. CoDesign 1–17 (2022)
Odlanicka-Poczobut, M.: Istota sieci semantycznych – pułapki i korzyści zastosowania w sądownictwie. Comp. Legilinguistics 40 , 21–41 (2019). https://doi.org/10.14746/cl.2019.40.2
Parayitam, S., Usman, A.S., Olson, B.J., Shea, T.: Effect of emotional exhaustion and knowledge sharing on depersonalization, work accomplishment, and organizational performance. Int. J. Knowl. Manag. (IJKM) 18 (1), 1–20 (2022). https://doi.org/10.4018/IJKM.291101
Parjanen, S.: Experiencing creativity in the organization: From individual creativity to collective creativity. Interdiscip. J. Inform., Know. Manag. 7 , 109–128 (2012). https://doi.org/10.28945/1580
Reiter-Palmon, R. (ed.): Team creativity and Innovation. Oxford University Press (2017)
Runco, M.A., Pritzker, S.R. (eds.): Encyclopedia of creativity. Academic press (2020)
Torrance, P.: Verbal Tests. Forms A and B-Figural Tests, Forms A and B. The Torrance Tests of Creative Thinking-Norms-Technical Manual Research Edition. Princeton, New Jersey: Personnel Press, p. 6 (1966).
Totawar, A.K., Nambudiri, R.: An overview of depersonalization in the organizational context. Indore Manage. J. 4 (2), 64–72 (2012)
Wolcott, M.D., McLaughlin, J.E., Hubbard, D.K., Rider, T.R., Umstead, K.: Twelve tips to stimulate creative problem-solving with design thinking. Med. Teacher 43 (5), 501–508 (2021)
Wolniak, R.: The design thinking method and its stages. Systemy Wspomagania w Inżynierii Produkcji 6 (6), 247–255 (2017)
Wszołek, M., Moszczyński, K.: Algorytmizacja procesów projektowych. w: Grech, M. (red.) Communication Design: badanie i projektowanie komunikacji 4 Kraków, Libron, 2015, s. 173–195 (2015)
Download references
Author information
Authors and affiliations.
Wroclaw University of Economics and Business, 53-345, Wroclaw, Poland
Iwona Chomiak-Orsa, Andrzej Greńczuk & Kamila Łuczak
You can also search for this author in PubMed Google Scholar
Corresponding author
Correspondence to Andrzej Greńczuk .
Editor information
Editors and affiliations.
Sri Sivasubramaniya Nadar College of Engineering, Chennai, India
Sarath Chandran K R
Sujaudeen N
Shahul Hamead H
Rights and permissions
Reprints and permissions
Copyright information
© 2023 IFIP International Federation for Information Processing
About this paper
Cite this paper.
Chomiak-Orsa, I., Greńczuk, A., Łuczak, K. (2023). The Use of the Semantic Network for Categorization of Problems Solved by Design Thinking. In: Chandran K R, S., N, S., A, B., Hamead H, S. (eds) Computational Intelligence in Data Science. ICCIDS 2023. IFIP Advances in Information and Communication Technology, vol 673. Springer, Cham. https://doi.org/10.1007/978-3-031-38296-3_11
Download citation
DOI : https://doi.org/10.1007/978-3-031-38296-3_11
Published : 22 July 2023
Publisher Name : Springer, Cham
Print ISBN : 978-3-031-38295-6
Online ISBN : 978-3-031-38296-3
eBook Packages : Computer Science Computer Science (R0)
Share this paper
Anyone you share the following link with will be able to read this content:
Sorry, a shareable link is not currently available for this article.
Provided by the Springer Nature SharedIt content-sharing initiative
- Publish with us
Policies and ethics
Societies and partnerships
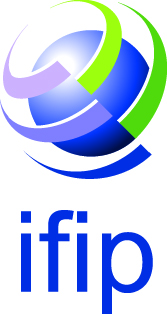
- Find a journal
- Track your research
- Bipolar Disorder
- Therapy Center
- When To See a Therapist
- Types of Therapy
- Best Online Therapy
- Best Couples Therapy
- Best Family Therapy
- Managing Stress
- Sleep and Dreaming
- Understanding Emotions
- Self-Improvement
- Healthy Relationships
- Student Resources
- Personality Types
- Verywell Mind Insights
- 2023 Verywell Mind 25
- Mental Health in the Classroom
- Editorial Process
- Meet Our Review Board
- Crisis Support
Cognition in Psychology
How People Think and What's Involved in This Process
Kendra Cherry, MS, is a psychosocial rehabilitation specialist, psychology educator, and author of the "Everything Psychology Book."
:max_bytes(150000):strip_icc():format(webp)/IMG_9791-89504ab694d54b66bbd72cb84ffb860e.jpg)
Daniel B. Block, MD, is an award-winning, board-certified psychiatrist who operates a private practice in Pennsylvania.
:max_bytes(150000):strip_icc():format(webp)/block-8924ca72ff94426d940e8f7e639e3942.jpg)
Verywell / Laura Porter
Definition of Cognition
- Improvement Tips
Frequently Asked Questions
Cognition is a term referring to the mental processes involved in gaining knowledge and comprehension. Some of the many different cognitive processes include thinking, knowing, remembering, judging, and problem-solving .
These are higher-level functions of the brain and encompass language, imagination, perception, and planning. Cognitive psychology is the field of psychology that investigates how people think and the processes involved in cognition.
What is an example of cognition?
Cognition includes all of the conscious and unconscious processes involved in thinking, perceiving, and reasoning. Examples of cognition include paying attention to something in the environment, learning something new, making decisions, processing language, sensing and perceiving environmental stimuli, solving problems, and using memory.
History of the Study of Cognition
The study of how humans think dates back to the time of ancient Greek philosophers Plato and Aristotle.
Philosophical Origins
Plato's approach to the study of the mind suggested that people understand the world by first identifying basic principles buried deep inside themselves, then using rational thought to create knowledge. This viewpoint was later advocated by philosophers such as Rene Descartes and linguist Noam Chomsky. It is often referred to as rationalism.
Aristotle, on the other hand, believed that people acquire knowledge through their observations of the world around them. Later thinkers such as John Locke and B.F. Skinner also advocated this point of view, which is often referred to as empiricism.
Early Psychology
During the earliest days of psychology—and for the first half of the 20th century—psychology was largely dominated by psychoanalysis , behaviorism , and humanism .
Eventually, a formal field of study devoted solely to the study of cognition emerged as part of the "cognitive revolution" of the 1960s. This field is known as cognitive psychology.
The Emergence of Cognitive Psychology
One of the earliest definitions of cognition was presented in the first textbook on cognitive psychology, which was published in 1967. According to Ulric Neisser, a psychologist and the book's author, cognition is "those processes by which the sensory input is transformed, reduced, elaborated, stored, recovered, and used."
Types of Cognitive Processes
There are many different types of cognitive processes. They include:
- Attention : Attention is a cognitive process that allows people to focus on a specific stimulus in the environment.
- Language : Language and language development are cognitive processes that involve the ability to understand and express thoughts through spoken and written words. This allows us to communicate with others and plays an important role in thought.
- Learning : Learning requires cognitive processes involved in taking in new things, synthesizing information, and integrating it with prior knowledge.
- Memory : Memory is an important cognitive process that allows people to encode, store, and retrieve information. It is a critical component in the learning process and allows people to retain knowledge about the world and their personal histories.
- Perception : Perception is a cognitive process that allows people to take in information through their senses, then utilize this information to respond and interact with the world.
- Thought : Thought is an essential part of every cognitive process. It allows people to engage in decision-making , problem-solving, and higher reasoning.
Hot Cognition vs. Cold Cognition
Some split cognition into two categories: hot and cold. Hot cognition refers to mental processes in which emotion plays a role, such as reward-based learning . Conversely, cold cognition refers to mental processes that don't involve feelings or emotions, such as working memory .
What Can Affect Cognition?
It is important to remember that these cognitive processes are complex and often imperfect. Some of the factors that can affect or influence cognition include:
Research indicates that as we age, our cognitive function tends to decline. Age-related cognitive changes include processing things more slowly, finding it harder to recall past events, and a failure to remember information that was once known (such as how to solve a particular math equation or historical information).
Attention Issues
Selective attention is a limited resource, so there are a number of things that can make it difficult to focus on everything in your environment. Attentional blink , for example, happens when you are so focused on one thing that you completely miss something else happening right in front of you.
Cognitive Biases
Cognitive biases are systematic errors in thinking related to how people process and interpret information about the world. Confirmation bias is one common example that involves only paying attention to information that aligns with your existing beliefs while ignoring evidence that doesn't support your views.
Some studies have connected cognitive function with certain genes. For example, a 2020 study published in Brain Communications found that a person's level of brain-derived neurotrophic factor (BDNF), which is 30% determined by heritability, can impact the rate of brain neurodegeneration, a condition that ultimately impacts cognitive function.
Memory Limitations
Short-term memory is surprisingly brief, typically lasting just 20 to 30 seconds, whereas long-term memory can be stable and enduring, with memories lasting years and even decades. Memory can also be fragile and fallible. Sometimes we forget and other times we are subject to misinformation effects that may even lead to the formation of false memories .
Uses of Cognition
Cognitive processes affect every aspect of life, from school to work to relationships. Some specific uses for these processes include the following.
Learning New Things
Learning requires being able to take in new information, form new memories, and make connections with other things that you already know. Researchers and educators use their knowledge of these cognitive processes to create instructive materials to help people learn new concepts .
Forming Memories
Memory is a major topic of interest in the field of cognitive psychology. How we remember, what we remember, and what we forget reveal a great deal about how cognitive processes operate.
While people often think of memory as being much like a video camera—carefully recording, cataloging, and storing life events away for later recall—research has found that memory is much more complex.
Making Decisions
Whenever people make any type of a decision, it involves making judgments about things they have processed. This might involve comparing new information to prior knowledge, integrating new information into existing ideas, or even replacing old knowledge with new knowledge before making a choice.
Impact of Cognition
Our cognitive processes have a wide-ranging impact that influences everything from our daily life to our overall health.
Perceiving the World
As you take in sensations from the world around you, the information that you see, hear, taste, touch, and smell must first be transformed into signals that the brain can understand. The perceptual process allows you to take in this sensory information and convert it into a signal that your brain can recognize and act upon.
Forming Impressions
The world is full of an endless number of sensory experiences . To make meaning out of all this incoming information, it is important for the brain to be able to capture the fundamentals. Events are reduced to only the critical concepts and ideas that we need.
Filling in the Gaps
In addition to reducing information to make it more memorable and understandable, people also elaborate on these memories as they reconstruct them. In some cases, this elaboration happens when people are struggling to remember something . When the information cannot be recalled, the brain sometimes fills in the missing data with whatever seems to fit.
Interacting With the World
Cognition involves not only the things that go on inside our heads but also how these thoughts and mental processes influence our actions. Our attention to the world around us, memories of past events, understanding of language, judgments about how the world works, and abilities to solve problems all contribute to how we behave and interact with our surrounding environment.
Tips for Improving Cognition
Cognitive processes are influenced by a range of factors, including genetics and experiences. While you cannot change your genes or age, there are things that you can do to protect and maximize your cognitive abilities:
- Stay healthy . Lifestyle factors such as eating a nutritious diet and getting regular exercise can have a positive effect on cognitive functioning.
- Think critically . Question your assumptions and ask questions about your thoughts, beliefs, and conclusions.
- Stay curious and keep learning . A great way to flex your cognitive abilities is to keep challenging yourself to learn more about the world.
- Skip multitasking . While it might seem like doing several things at once would help you get done faster, research has shown it actually decreases both productivity and work quality.
Thinking is an important component, but cognition also encompasses unconscious and perceptual processes as well. In addition to thinking, cognition involves language, attention, learning, memory, and perception.
People utilize cognitive skills to think, learn, recall, and reason. Five important cognitive skills include short-term memory, logic, processing speed, attention, and spatial recognition.
American Psychological Association. Cognition .
Ezebuilo HC. Descartes, Leibniz and Spinoza: A brief survey of rationalism . J App Philos . 2020;18(6):95-118. doi:10.13140/RG.2.2.19692.39043
Sgarbi M. The Aristotelian Tradition and the Rise of British Empiricism: Logic and Epistemology in the British Isles (1570–1689) .
Lachman R, Lachman J L, Butterfield EC. Cognitive psychology and information processing: An introduction .
Neisser U. Cognitive psychology: Classic edition .
Murman D. The impact of age on cognition . Semin Hear . 2015;36(3):111-121. doi:10.1055/s-0035-1555115
Li S, Weinstein G, Zare H, et al. The genetics of circulating BDNF: Towards understanding the role of BDNF in brain structure and function in middle and old ages . Brain Commun . 2020;2(2):fcaa176. doi:10.1093/braincomms/fcaa176
Weinsten Y. How long is short-term memory: Shorter than you might think . Duke Undergraduate Education.
Leding J, Antonio L. Need for cognition and discrepancy detection in the misinformation effect . J Cognitive Psychol . 2019;31(4):409-415. doi:10.1080/20445911.2019.1626400
Scheiter K, Schubert C, Schuler A. Self-regulated learning from illustrated text: Eye movement modelling to support use and regulation of cognitive processes during learning from multimedia . Brit J Educ Psychol . 2017;88(1):80-94. doi:10.1111/bjep.12175
Toppi J, Astolfi L, Risetti M, et al. Different topological properties of EEG-derived networks describe working memory phases as revealed by graph theoretical analysis . Front Hum Neurosci . 2018;11:637. doi:10.3389/fnhum.2017.00637
Mather G. Foundations of sensation and perception .
Sousa D. How the brain learns .
Houben S, Otgaar H, Roelofs J, Merckelbach H. EMDR and false memories: A response to Lee, de Jongh, and Hase (2019) . Clin Psycholog Sci . 2019;7(3):405-6. doi:10.1177/2167702619830392
Schwarzer R. Self-efficacy: Thought control of action .
Imaoka M, Nakao H, Nakamura M, et al. Effect of multicomponent exercise and nutrition support on the cognitive function of older adults: A randomized controlled trial . Clin Interv Aging . 2019;14:2145-53. doi:10.2147/CIA.S229034
Petroutsatou K, Sifiniadis A. Exploring the consequences of human multitasking in industrial automation projects: A tool to mitigate impacts - Part II . Organiz Techn Manage Construct . 2018;10(1):1770-1777. doi:10.2478/otmcj-2016-0031
Mullis CE, Hatfield RC. The effects of multitasking on auditors' judgment quality . Contemp Account Res . 2017;35(1):314-333. doi:10.1111/1911-3846.12392
Revlin R. Cognition: Theory and Practice .
By Kendra Cherry, MSEd Kendra Cherry, MS, is a psychosocial rehabilitation specialist, psychology educator, and author of the "Everything Psychology Book."
[G02] Classifying problems
Module: Strategic reasoning
- G01. Overview
- G03. Solving problems
- G04. Complex systems
- G05. Charts & diagrams
- G06. SWOT analysis
- G07. The 7-S framework
- G08. Making good decisions
- G09. Decision by pros and cons
- G10. Formal decision theory
Quote of the page
Popular pages
- What is critical thinking?
- What is logic?
- Hardest logic puzzle ever
- Free miniguide
- What is an argument?
- Knights and knaves puzzles
- Logic puzzles
- What is a good argument?
- Improving critical thinking
- Analogical arguments
Solving problems require good critical and creative thinking. We need to be able to define the problem, analyse the nature of the problem, and come up with effective solutions. The ability to solve problems is a very important skill in the workplace.
§1. Defining the problem
When we are faced with a difficult problem, how should we go about solving it in an efficient and effective manner? An important starting point is knowing what the problem is. In school students are usually solving problems that are already well-formulated. Most problem sets and exam questions are like that. However, in everyday life and in the workplace, most of the time we have to identify the problem and formulate it correctly. In defining a problem there are these points to consider :
- The formulation of a problem can indirectly influence us in the directions that we take in seeking solutions. So sometimes it might be useful to come up with alternative formulations of the problem, and consider how to best formulate a problem. For example, faced with an unhappy relationship on the verge of breakup, one might think of the problem as "why is she leaving me?", and focus on the faults and reasons of the other person. But thinking of the problem in terms of "what have I been doing wrong" might lead one to focus more on oneself and think about what one might do to rescue the relationship. Similarly, instead of focusing on how a business competitor is taking away business from one's own company, the real problem to focus on might be why one's own company is not doing enough to adapt to the new market.
- If the problem concerns how a goal or target might be achieved, it is important to avoid vagueness and try to be more specific. If we are thinking about how to improve a company's profit, it would be useful to say more precisely how much of an increase we are looking for, in order to know whether the goal is realistic or not.
- It is also important to think about whether the problem so-defined is real or not. What data is available to show that there is a real problem to be solved? For example a university might be concerned that student standards are dropping. It would be useful to have information available confirming that this is not a subjective judgment but an actual declining trend. Gathering more data about the problem can also help us understand how serious it is and which are the most important factors to consider in dealing with it.
§2. Problem classification
Having defined the problem, the next thing to do is to know what type of problem it is. Generally, a problem might be posed in the form of a question, and we might classify these questions into three kinds :
- Empirical questions
- Conceptual questions
- Evaluative questions
Empirical questions are questions concerning empirical facts, particular events or causal processes in the world. Here are some examples :
- Who is the current president of the United States?
- Did Germany participated in the First World War?
- Can AIDS be transmitted through kissing?
- Is the universe expanding?
To answer an empirical question, we need observations or experiments, or solicit the help of experts in the relevant field, such as physics, biology, psychology, economics, history, etc. Very often these questions cannot be answered by sitting in the armchair. For example, consider the question of whether human beings evolved from other forms of animals. This is an empirical question to be answered by careful scientific study. We might have certain convictions or intuitions about the answer, and be inclined to believe one way or another. But these prior beliefs should be evaluated according to empirical data. Pure thinking is not going to help find the answer.
On the other hand, pure thinking can help us answer conceptual questions. Here are some examples of conceptual questions :
- Is rule of law sufficient for democracy?
- Can a woman sexually harass a man?
- Are there any married bachelors?
- Is 981567 divisible by 3?
To answer these questions, we appeal to logic and the meaning of words and concepts to arrive at the answers without engaging in experiments or observations. In other words, mere thinking is enough to answer these questions. Here, "mere thinking" refers to conceptual analysis and reasoning. For example, in answering the first question, we note that "rule of law" means the consistent use of due procedure and legal processes in a society where the legal principles are not arbitrarily applied or withheld. But we might note that the laws in a society where this is the case might nonetheless discriminate against certain social groups and provide them with inadequate political representation in the government. As long as these rules are not abused and are consistently applied, there is rule of law but no democracy. Using pure reasoning and our understanding of the concepts of "rule of law" and "democracy", we conclude that rule of law is not sufficient for democracy.
Similarly, the other conceptual questions are questions which can be answered without empirical observations or scientific study.
Finally, let us consider evaluative questions. Evaluative questions are questions which explicitly or implicitly invoke values and norms. These questions relate to value judgments about moral correctness or aesthetic values. Here are some examples :
- Is abortion immoral?
- Is Beethoven a more profound composer than Mozart?
- Should the amount of unemployment benefits be raised?
To answer this type of questions, we need to understand the distinction between intrinsic and instrumental values. Very briefly, intrinsic value is value that exists on its own. The intrinsic value of an object does not depend on its being used to satisfy some further end. But if something has value only because and in so far as it can be used to satisfy a further end, and would cease to have value if it fails to do so, then the value involved is instrumental value. (See here for further discussion.)
§3. Mixed questions
Conceptual questions might be regarded as the most basic kind of question among the three categories. This is because factual and evaluative questions can only be answered if we understand the relevant concepts invoked by the questions. For example, if we do not know what a black hole is, we cannot answer the question "Can light escape from a black hole?" Similarly, we need to know what abortion is if we want to find out whether abortion is immoral.
Empirical questions are generally independent of evaluative questions. We do not have to consider any evaluative judgements if we want to answer an empirical question. However, the reverse is not true. To answer many evaluative questions, we need to know quite a few empirical facts. For example, to evaluate whether an action is morally right or wrong, we usually have to consider the consequences of the action, or the motives behind the action. Once we know these empirical facts, we can then apply the correct moral standards to judge whether the action is good or bad. Suppose we want to find out whether it was right for the US to drop two atomic bombs onto Japan. We have to consider empirical facts such as Japanese atrocities during the Second World War, the extent of the destruction caused by the atomic bombs, the number of innocent civilians killed as a result, and whether there are other alternative ways of ending the war. These are all empirical matters which are important to consider in answering the questions.
Many disputes and controversies persist because of bad thinking, and bad thinking techniques might take the form of failure to understand the nature and type of the questions that have to be answered. The distinction between three types of questions discussed here is a simple and crucial part of the methodology in problem-solving.
- Suppose "sexual harassment" is defined as any unwelcome sexual behavior or conduct which is offensive, humiliating or intimidating. How would you answer the question of whether telling sexual jokes constitutes sexual harassment?
- Describe how you might answer the question of whether animals have languages.
homepage • top • contact • sitemap
© 2004-2024 Joe Lau & Jonathan Chan
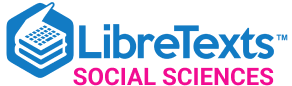
- school Campus Bookshelves
- menu_book Bookshelves
- perm_media Learning Objects
- login Login
- how_to_reg Request Instructor Account
- hub Instructor Commons
- Download Page (PDF)
- Download Full Book (PDF)
- Periodic Table
- Physics Constants
- Scientific Calculator
- Reference & Cite
- Tools expand_more
- Readability
selected template will load here
This action is not available.
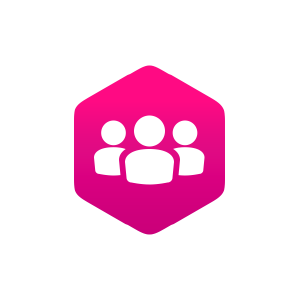
9.5: Pitfalls to Problem Solving
- Last updated
- Save as PDF
- Page ID 59922
Learning Objectives
- Explain some common roadblocks to effective problem solving
Not all problems are successfully solved, however. What challenges stop us from successfully solving a problem? Albert Einstein once said, “Insanity is doing the same thing over and over again and expecting a different result.” Imagine a person in a room that has four doorways. One doorway that has always been open in the past is now locked. The person, accustomed to exiting the room by that particular doorway, keeps trying to get out through the same doorway even though the other three doorways are open. The person is stuck—but she just needs to go to another doorway, instead of trying to get out through the locked doorway. A mental set is where you persist in approaching a problem in a way that has worked in the past but is clearly not working now. Functional fixedness is a type of mental set where you cannot perceive an object being used for something other than what it was designed for. During the Apollo 13 mission to the moon, NASA engineers at Mission Control had to overcome functional fixedness to save the lives of the astronauts aboard the spacecraft. An explosion in a module of the spacecraft damaged multiple systems. The astronauts were in danger of being poisoned by rising levels of carbon dioxide because of problems with the carbon dioxide filters. The engineers found a way for the astronauts to use spare plastic bags, tape, and air hoses to create a makeshift air filter, which saved the lives of the astronauts.
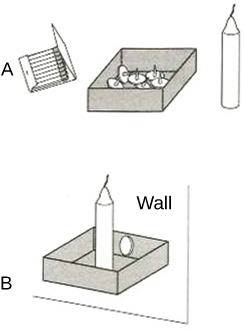
Figure 1 . In Duncker’s classic study, participants were provided the three objects in the top panel and asked to solve the problem. The solution is shown in the bottom portion.
Link to Learning
Check out this Apollo 13 scene where the group of NASA engineers are given the task of overcoming functional fixedness.
Researchers have investigated whether functional fixedness is affected by culture. In one experiment, individuals from the Shuar group in Ecuador were asked to use an object for a purpose other than that for which the object was originally intended. For example, the participants were told a story about a bear and a rabbit that were separated by a river and asked to select among various objects, including a spoon, a cup, erasers, and so on, to help the animals. The spoon was the only object long enough to span the imaginary river, but if the spoon was presented in a way that reflected its normal usage, it took participants longer to choose the spoon to solve the problem. (German & Barrett, 2005). The researchers wanted to know if exposure to highly specialized tools, as occurs with individuals in industrialized nations, affects their ability to transcend functional fixedness. It was determined that functional fixedness is experienced in both industrialized and nonindustrialized cultures (German & Barrett, 2005).
Query \(\PageIndex{1}\)
Query \(\PageIndex{2}\)
Query \(\PageIndex{3}\)
In order to make good decisions, we use our knowledge and our reasoning. Often, this knowledge and reasoning is sound and solid. Sometimes, however, we are swayed by biases or by others manipulating a situation. For example, let’s say you and three friends wanted to rent a house and had a combined target budget of $1,600. The realtor shows you only very run-down houses for $1,600 and then shows you a very nice house for $2,000. Might you ask each person to pay more in rent to get the $2,000 home? Why would the realtor show you the run-down houses and the nice house? The realtor may be challenging your anchoring bias. An anchoring bias occurs when you focus on one piece of information when making a decision or solving a problem. In this case, you’re so focused on the amount of money you are willing to spend that you may not recognize what kinds of houses are available at that price point.
The confirmation bias is the tendency to focus on information that confirms your existing beliefs. For example, if you think that your professor is not very nice, you notice all of the instances of rude behavior exhibited by the professor while ignoring the countless pleasant interactions he is involved in on a daily basis. This bias proves that first impressions do matter and that we tend to look for information to confirm our initial judgments of others.
Watch this video from the Big Think to learn more about the confirmation bias.
You can view the transcript for “Confirmation Bias: Your Brain is So Judgmental” here (opens in new window) .
Hindsight bias leads you to believe that the event you just experienced was predictable, even though it really wasn’t. In other words, you knew all along that things would turn out the way they did. Representative bias describes a faulty way of thinking, in which you unintentionally stereotype someone or something; for example, you may assume that your professors spend their free time reading books and engaging in intellectual conversation, because the idea of them spending their time playing volleyball or visiting an amusement park does not fit in with your stereotypes of professors.
Finally, the availability heuristic is a heuristic in which you make a decision based on an example, information, or recent experience that is that readily available to you, even though it may not be the best example to inform your decision . To use a common example, would you guess there are more murders or more suicides in America each year? When asked, most people would guess there are more murders. In truth, there are twice as many suicides as there are murders each year. However, murders seem more common because we hear a lot more about murders on an average day. Unless someone we know or someone famous takes their own life, it does not make the news. Murders, on the other hand, we see in the news every day. This leads to the erroneous assumption that the easier it is to think of instances of something, the more often that thing occurs.
Watch the following video for an example of the availability heuristic.
You can view the transcript for “Availability Heuristic: Are Planes More Dangerous Than Cars?” here (opens in new window) .
Biases tend to “preserve that which is already established—to maintain our preexisting knowledge, beliefs, attitudes, and hypotheses” (Aronson, 1995; Kahneman, 2011). These biases are summarized in Table 2 below.
Learn more about heuristics and common biases through the article, “ 8 Common Thinking Mistakes Our Brains Make Every Day and How to Prevent Them ” by Belle Beth Cooper.
You can also watch this clever music video explaining these and other cognitive biases.
Query \(\PageIndex{4}\)
Query \(\PageIndex{5}\)
Query \(\PageIndex{6}\)
Think It Over
Which type of bias do you recognize in your own decision making processes? How has this bias affected how you’ve made decisions in the past and how can you use your awareness of it to improve your decisions making skills in the future?
anchoring bias: faulty heuristic in which you fixate on a single aspect of a problem to find a solution
availability heuristic: faulty heuristic in which you make a decision based on information readily available to you
confirmation bias: faulty heuristic in which you focus on information that confirms your beliefs
functional fixedness: inability to see an object as useful for any other use other than the one for which it was intended
hindsight bias: belief that the event just experienced was predictable, even though it really wasn’t
mental set: continually using an old solution to a problem without results
representative bias: faulty heuristic in which you stereotype someone or something without a valid basis for your judgment
Licenses and Attributions
CC licensed content, Original
- Modification, adaptation, and original content. Provided by : Lumen Learning. License : CC BY: Attribution
- Problem Solving. Authored by : OpenStax College. Located at : http://cnx.org/contents/[email protected]:Lk3YnvuC@6/Problem-Solving . License : Public Domain: No Known Copyright . License Terms : Download for free at http://cnx.org/contents/[email protected]
- More information on heuristics. Authored by : Dr. Scott Roberts, Dr. Ryan Curtis, Samantha Levy, and Dr. Dylan Selterman. Provided by : University of Maryland. Located at : http://openpsyc.blogspot.com/2014/07/heuristics.html . Project : OpenPSYC. License : CC BY-NC-SA: Attribution-NonCommercial-ShareAlike
Please log in to save materials. Log in
- Anchoring Bias
- Availability Heuristic
- Confirmation Bias
- Functional Fixedness
- Hindsight Bias
- Problem-solving Strategy
- Representative Bias
- Trial and Error
- Working Backwards
Problem Solving
- Describe problem solving strategies
- Define algorithm and heuristic
- Explain some common roadblocks to effective problem solving
People face problems every day—usually, multiple problems throughout the day. Sometimes these problems are straightforward: To double a recipe for pizza dough, for example, all that is required is that each ingredient in the recipe be doubled. Sometimes, however, the problems we encounter are more complex. For example, say you have a work deadline, and you must mail a printed copy of a report to your supervisor by the end of the business day. The report is time-sensitive and must be sent overnight. You finished the report last night, but your printer will not work today. What should you do? First, you need to identify the problem and then apply a strategy for solving the problem.
PROBLEM-SOLVING STRATEGIES
When you are presented with a problem—whether it is a complex mathematical problem or a broken printer, how do you solve it? Before finding a solution to the problem, the problem must first be clearly identified. After that, one of many problem solving strategies can be applied, hopefully resulting in a solution.
A problem-solving strategy is a plan of action used to find a solution. Different strategies have different action plans associated with them ( Table ). For example, a well-known strategy is trial and error . The old adage, “If at first you don’t succeed, try, try again” describes trial and error. In terms of your broken printer, you could try checking the ink levels, and if that doesn’t work, you could check to make sure the paper tray isn’t jammed. Or maybe the printer isn’t actually connected to your laptop. When using trial and error, you would continue to try different solutions until you solved your problem. Although trial and error is not typically one of the most time-efficient strategies, it is a commonly used one.
Another type of strategy is an algorithm. An algorithm is a problem-solving formula that provides you with step-by-step instructions used to achieve a desired outcome (Kahneman, 2011). You can think of an algorithm as a recipe with highly detailed instructions that produce the same result every time they are performed. Algorithms are used frequently in our everyday lives, especially in computer science. When you run a search on the Internet, search engines like Google use algorithms to decide which entries will appear first in your list of results. Facebook also uses algorithms to decide which posts to display on your newsfeed. Can you identify other situations in which algorithms are used?
A heuristic is another type of problem solving strategy. While an algorithm must be followed exactly to produce a correct result, a heuristic is a general problem-solving framework (Tversky & Kahneman, 1974). You can think of these as mental shortcuts that are used to solve problems. A “rule of thumb” is an example of a heuristic. Such a rule saves the person time and energy when making a decision, but despite its time-saving characteristics, it is not always the best method for making a rational decision. Different types of heuristics are used in different types of situations, but the impulse to use a heuristic occurs when one of five conditions is met (Pratkanis, 1989):
- When one is faced with too much information
- When the time to make a decision is limited
- When the decision to be made is unimportant
- When there is access to very little information to use in making the decision
- When an appropriate heuristic happens to come to mind in the same moment
Working backwards is a useful heuristic in which you begin solving the problem by focusing on the end result. Consider this example: You live in Washington, D.C. and have been invited to a wedding at 4 PM on Saturday in Philadelphia. Knowing that Interstate 95 tends to back up any day of the week, you need to plan your route and time your departure accordingly. If you want to be at the wedding service by 3:30 PM, and it takes 2.5 hours to get to Philadelphia without traffic, what time should you leave your house? You use the working backwards heuristic to plan the events of your day on a regular basis, probably without even thinking about it.
Another useful heuristic is the practice of accomplishing a large goal or task by breaking it into a series of smaller steps. Students often use this common method to complete a large research project or long essay for school. For example, students typically brainstorm, develop a thesis or main topic, research the chosen topic, organize their information into an outline, write a rough draft, revise and edit the rough draft, develop a final draft, organize the references list, and proofread their work before turning in the project. The large task becomes less overwhelming when it is broken down into a series of small steps.
Solving Puzzles
Problem-solving abilities can improve with practice. Many people challenge themselves every day with puzzles and other mental exercises to sharpen their problem-solving skills. Sudoku puzzles appear daily in most newspapers. Typically, a sudoku puzzle is a 9×9 grid. The simple sudoku below ( Figure ) is a 4×4 grid. To solve the puzzle, fill in the empty boxes with a single digit: 1, 2, 3, or 4. Here are the rules: The numbers must total 10 in each bolded box, each row, and each column; however, each digit can only appear once in a bolded box, row, and column. Time yourself as you solve this puzzle and compare your time with a classmate.

Here is another popular type of puzzle ( Figure ) that challenges your spatial reasoning skills. Connect all nine dots with four connecting straight lines without lifting your pencil from the paper:

Take a look at the “Puzzling Scales” logic puzzle below ( Figure ). Sam Loyd, a well-known puzzle master, created and refined countless puzzles throughout his lifetime (Cyclopedia of Puzzles, n.d.).

PITFALLS TO PROBLEM SOLVING
Not all problems are successfully solved, however. What challenges stop us from successfully solving a problem? Albert Einstein once said, “Insanity is doing the same thing over and over again and expecting a different result.” Imagine a person in a room that has four doorways. One doorway that has always been open in the past is now locked. The person, accustomed to exiting the room by that particular doorway, keeps trying to get out through the same doorway even though the other three doorways are open. The person is stuck—but she just needs to go to another doorway, instead of trying to get out through the locked doorway. A mental set is where you persist in approaching a problem in a way that has worked in the past but is clearly not working now.
Functional fixedness is a type of mental set where you cannot perceive an object being used for something other than what it was designed for. During the Apollo 13 mission to the moon, NASA engineers at Mission Control had to overcome functional fixedness to save the lives of the astronauts aboard the spacecraft. An explosion in a module of the spacecraft damaged multiple systems. The astronauts were in danger of being poisoned by rising levels of carbon dioxide because of problems with the carbon dioxide filters. The engineers found a way for the astronauts to use spare plastic bags, tape, and air hoses to create a makeshift air filter, which saved the lives of the astronauts.

Check out this Apollo 13 scene where the group of NASA engineers are given the task of overcoming functional fixedness.
Researchers have investigated whether functional fixedness is affected by culture. In one experiment, individuals from the Shuar group in Ecuador were asked to use an object for a purpose other than that for which the object was originally intended. For example, the participants were told a story about a bear and a rabbit that were separated by a river and asked to select among various objects, including a spoon, a cup, erasers, and so on, to help the animals. The spoon was the only object long enough to span the imaginary river, but if the spoon was presented in a way that reflected its normal usage, it took participants longer to choose the spoon to solve the problem. (German & Barrett, 2005). The researchers wanted to know if exposure to highly specialized tools, as occurs with individuals in industrialized nations, affects their ability to transcend functional fixedness. It was determined that functional fixedness is experienced in both industrialized and nonindustrialized cultures (German & Barrett, 2005).
In order to make good decisions, we use our knowledge and our reasoning. Often, this knowledge and reasoning is sound and solid. Sometimes, however, we are swayed by biases or by others manipulating a situation. For example, let’s say you and three friends wanted to rent a house and had a combined target budget of $1,600. The realtor shows you only very run-down houses for $1,600 and then shows you a very nice house for $2,000. Might you ask each person to pay more in rent to get the $2,000 home? Why would the realtor show you the run-down houses and the nice house? The realtor may be challenging your anchoring bias. An anchoring bias occurs when you focus on one piece of information when making a decision or solving a problem. In this case, you’re so focused on the amount of money you are willing to spend that you may not recognize what kinds of houses are available at that price point.
The confirmation bias is the tendency to focus on information that confirms your existing beliefs. For example, if you think that your professor is not very nice, you notice all of the instances of rude behavior exhibited by the professor while ignoring the countless pleasant interactions he is involved in on a daily basis. Hindsight bias leads you to believe that the event you just experienced was predictable, even though it really wasn’t. In other words, you knew all along that things would turn out the way they did. Representative bias describes a faulty way of thinking, in which you unintentionally stereotype someone or something; for example, you may assume that your professors spend their free time reading books and engaging in intellectual conversation, because the idea of them spending their time playing volleyball or visiting an amusement park does not fit in with your stereotypes of professors.
Finally, the availability heuristic is a heuristic in which you make a decision based on an example, information, or recent experience that is that readily available to you, even though it may not be the best example to inform your decision . Biases tend to “preserve that which is already established—to maintain our preexisting knowledge, beliefs, attitudes, and hypotheses” (Aronson, 1995; Kahneman, 2011). These biases are summarized in Table .
Please visit this site to see a clever music video that a high school teacher made to explain these and other cognitive biases to his AP psychology students.
Were you able to determine how many marbles are needed to balance the scales in Figure ? You need nine. Were you able to solve the problems in Figure and Figure ? Here are the answers ( Figure ).

Many different strategies exist for solving problems. Typical strategies include trial and error, applying algorithms, and using heuristics. To solve a large, complicated problem, it often helps to break the problem into smaller steps that can be accomplished individually, leading to an overall solution. Roadblocks to problem solving include a mental set, functional fixedness, and various biases that can cloud decision making skills.
Review Questions
A specific formula for solving a problem is called ________.
- an algorithm
- a heuristic
- a mental set
- trial and error
A mental shortcut in the form of a general problem-solving framework is called ________.
Which type of bias involves becoming fixated on a single trait of a problem?
- anchoring bias
- confirmation bias
- representative bias
- availability bias
Which type of bias involves relying on a false stereotype to make a decision?
Critical Thinking Questions
What is functional fixedness and how can overcoming it help you solve problems?
Functional fixedness occurs when you cannot see a use for an object other than the use for which it was intended. For example, if you need something to hold up a tarp in the rain, but only have a pitchfork, you must overcome your expectation that a pitchfork can only be used for garden chores before you realize that you could stick it in the ground and drape the tarp on top of it to hold it up.
How does an algorithm save you time and energy when solving a problem?
An algorithm is a proven formula for achieving a desired outcome. It saves time because if you follow it exactly, you will solve the problem without having to figure out how to solve the problem. It is a bit like not reinventing the wheel.
Personal Application Question
Which type of bias do you recognize in your own decision making processes? How has this bias affected how you’ve made decisions in the past and how can you use your awareness of it to improve your decisions making skills in the future?
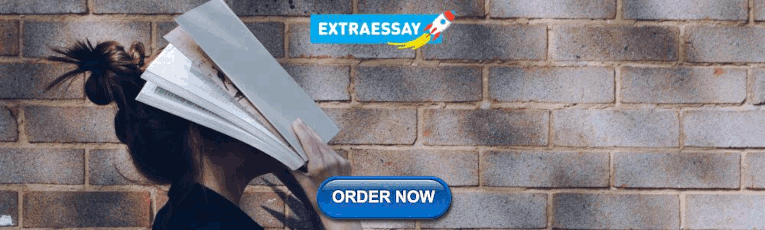
IMAGES
VIDEO
COMMENTS
Categorization and problem-solving are widely studied in psychology and artificial intelligence (AI), but these areas of research have been largely segregated. Several articles in this volume seek to rectify this situation by discussing relationships between categorization and problem-solving and the memory structures that support them (Brewer ...
Thinking and Problem-Solving presents a comprehensive and up-to-date review of literature on cognition, reasoning, intelligence, and other formative areas specific to this field. Written for advanced undergraduates, researchers, and academics, this volume is a necessary reference for beginning and established investigators in cognitive and ...
broad functions: categorization and communication. The conceptual function that most research has targeted is categorization, the process by which mental representations (concepts) determine whether some entity is a member of a category. Categorization enables a wide variety of subordinate functions because classifying something as a category ...
The Problem. We all think categorically, and for good reason: It helps us make sense of the world. But in business, categorical thinking can lead to major errors in decision making.
Concepts (i.e., lexicalized classes of real or fictitious entities) play a central role in many human intellectual activities, including planning, thinking, reasoning, problem solving, and decision making. How do people acquire concepts in the course of development and learning and use them in their thinking about the world? In this article, we ...
A crucial step in problem solving is the retrieval of already learned schemata from long-term memory, a process which may be facilitated by categorization of the problem. The way knowledge is organized affects its availability, and, at the same time, it constitutes the important difference between experts and novices. The present study employed concept maps in a novel way, as a categorization ...
The ability to solve real-world problems and to transfer problem-solving strategies from domain-specific to domain-general contexts and vice versa has been regarded an important competence students should develop during their education in school (Greiff et al. []; van Merriënboer []).In the context of large-scale assessments such as the PISA study problem solving competence is defined as the ...
This is only one facet of the complex processes involved in cognition. Simply put, cognition is thinking, and it encompasses the processes associated with perception, knowledge, problem solving, judgment, language, and memory. Scientists who study cognition are searching for ways to understand how we integrate, organize, and utilize our ...
The Concrete Operational Stage is the third stage in Jean Piaget's theory of cognitive development, typically occurring between the ages of 7 and 11. During this stage, children begin to develop logical thinking skills and can perform operations on concrete objects and events. However, they still struggle with certain cognitive tasks:
For Bruner (1961), the purpose of education is not to impart knowledge, but instead to facilitate a child's thinking and problem-solving skills which can then be transferred to a range of situations. Specifically, education should also develop symbolic thinking in children. In 1960 Bruner's text, The Process of Education was published. The ...
This is only one facet of the complex processes involved in cognition. Simply put, cognition is thinking, and it encompasses the processes associated with perception, knowledge, problem solving, judgment, language, and memory. Scientists who study cognition are searching for ways to understand how we integrate, organize, and utilize our ...
In cognitive psychology, categorization focuses on how knowledge is organized. Objects in the same category are likely to share certain attributes, and category membership allows inferences to be drawn. The term category refers to a set of things (objects, ideas, events) that are grouped together. The term concept often refers to the mental representation of… Continue reading Categorization
Comparison of experts and novices on a similarity judgment task found that the experts predominantly relied on the problems' deep structures in deciding on similarity of solution, although the presence of surface-feature similarity had a clear adverse effect on performance. These investigations were conducted to examine the relationship between problem-solving ability and the criteria used ...
Teaching problem solving, or skillful thinking toward given ends, important as it is, is surely not enough. It does not suffice to be able to bring effective strategies to bear on problems as given; one should be able also to make reasonable judgments about which problems are worth solving and which are not.
Problem-solving abilities can improve with practice. Many people challenge themselves every day with puzzles and other mental exercises to sharpen their problem-solving skills. Sudoku puzzles appear daily in most newspapers. Typically, a sudoku puzzle is a 9×9 grid. The simple sudoku below (Figure 14) is a 4×4 grid.
Creating such a tool can significantly support the decision to use Design Thinking by managers in solving a selected problem. The experiment, presented graphically in Figs. 3 and 4, clearly indicates the legitimacy of using semantic networks to categorize problems that can be solved by using the Design Thinking method. Which indicates that the ...
Cognition is a term referring to the mental processes involved in gaining knowledge and comprehension. Some of the many different cognitive processes include thinking, knowing, remembering, judging, and problem-solving. These are higher-level functions of the brain and encompass language, imagination, perception, and planning.
Solving problems require good critical and creative thinking. We need to be able to define the problem, analyse the nature of the problem, and come up with effective solutions. The ability to solve problems is a very important skill in the workplace. §1. Defining the problem
11.2.2 Barriers to Problem Solving Mental set-When you solve a problem in a one particular way, it becomes a set. These are already tried mental operations or steps. This could lead to success in some situation but could also create a kind of mental rigidity that acts as a hindrance to think in new ways, rules and strategies for problem solving.
Analogical thinking enters into creative discovery, problem-solving, categorization, and learning and transfer. This realization has led to a surge in research activity over the last three decades, resulting in great gains in our understanding of analogical processing.
Try It. Query 9.5.1 9.5. 1. Query 9.5.2 9.5. 2. Query 9.5.3 9.5. 3. In order to make good decisions, we use our knowledge and our reasoning. Often, this knowledge and reasoning is sound and solid. Sometimes, however, we are swayed by biases or by others manipulating a situation.
To better understand analytical thinking, it helps to learn how it's different from other types of thinking. For instance, when solving a problem, analytical thinkers take a methodical approach, breaking up information and analyzing each part until they form a conclusion. Creative thinkers take a less organized approach to problem-solving.
Solving Puzzles. Problem-solving abilities can improve with practice. Many people challenge themselves every day with puzzles and other mental exercises to sharpen their problem-solving skills. Sudoku puzzles appear daily in most newspapers. Typically, a sudoku puzzle is a 9×9 grid. The simple sudoku below ( Figure) is a 4×4 grid.