
An official website of the United States government
The .gov means it’s official. Federal government websites often end in .gov or .mil. Before sharing sensitive information, make sure you’re on a federal government site.
The site is secure. The https:// ensures that you are connecting to the official website and that any information you provide is encrypted and transmitted securely.
- Publications
- Account settings
Preview improvements coming to the PMC website in October 2024. Learn More or Try it out now .
- Advanced Search
- Journal List
- Elsevier Sponsored Documents
- PMC10028259

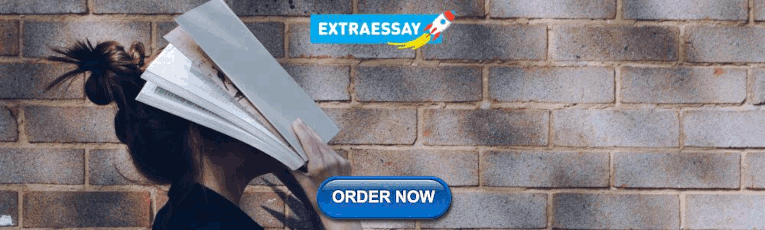
The impact of Covid-19 on student achievement: Evidence from a recent meta-analysis ☆
Giorgio di pietro.
a European Commission- Joint Research Centre 1 , Edificio Expo, Calle Inca Garcilaso, 3, 41092, Seville, Spain
b Institute of Labour Economics (IZA), Schaumburg-Lippe-Straße 5-9, 53113, Bonn, Germany
Associated Data
Data will be made available on request.
This work attempts to synthetize existing research about the impact of Covid-19 school closure on student achievement. It extends previous systematic reviews and meta-analyses by (a) using a more balanced sample in terms of country composition, (b) considering new moderators (type of data and research design), and (c) including studies on tertiary education students in addition to primary and secondary education students. Our meta-analysis findings show that the pandemic had, on average, a detrimental effect on learning. The magnitude of this learning deficit (about 0.19 standard deviations of student achievement) appears to be roughly comparable to that suffered by students who have experienced a significant disruption in their schooling due to a major natural disaster (e.g., Hurricane Katrina). Students are also found to have lost more ground in math/science than in other subjects. Additionally, one year or more after the first lockdown, students seem to have been unable to catch up on unfinished learning from the pandemic. This result suggests that more efforts should be made to ensure students recover their missed learning in order to avoid negative long-term consequences for them and society.
- • We perform a meta-analysis to study the effect of Covid-19 on student achievement.
- • Our dataset includes 239 estimates from 39 studies covering 19 countries.
- • The pandemic had an overall negative effect on learning outcomes.
- • Students lost more ground in math/science than in other subjects.
- • One year or more after Covid-19 students have not recovered from the initial learning loss.
1. Introduction
The Covid-19 pandemic caused a major disruption in the schooling system around the world. In most countries, educational institutions had to close for several weeks or months in an attempt to reduce the spread of the virus ( UNESCO, 2020a ). Students had to continue their schooling from home using different learning tools such as video conferencing, radio and TV. However, the outbreak of Covid-19 was so sudden that there was little or no time for many schools to design and implement learning programs specifically designed to support children's learning while at home. A significant proportion of teachers were unprepared for online learning as they lacked appropriate pedagogical and digital skills ( School Education Gateway, 2020 ). Similarly, many students also struggled to adjust to the new format of learning. In addition to problems in accessing appropriate technology (computers, reliable internet connection, etc.), not all students had a home environment free of disturbances and distractions, hence conducive to learning ( Pokhrel & Chhetri, 2021 ). A large number of parents had serious difficulties in combining their work responsibilities (if not joblessness) with looking after and educating their children ( Soland et al., 2020 ). Moreover, there is evidence showing that Covid-19 and the related containment measures have had a detrimental effect on children's wellbeing ( Xie et al., 2020 ). Longer periods of social isolation might have adversely affected students' mental health (e.g., anxiety and depression) and physical activity ( Vaillancourt et al., 2021 ). This is also likely to have contributed to negatively impact their academic performance given the close association between mental and physical health and educational outcomes ( Joe et al., 2009 ).
While in the literature there is already a relatively large consensus that student learning suffered a setback due to Covid-19, as pointed out by several researchers (e.g., Donnelly & Patrinos, 2022 ; Patrinos et al., 2022 ), more research in this area is still needed. Findings from new studies are important given that, as stated in a recent article published in the World Economic Forum, the full scale of the impact of the pandemic on the education of children is “only just starting to emerge” ( Broom, 2022 ). Not only is a better understanding of the educational impact of Covid-19 needed, but special attention should be paid to investigate the legacy effects of the pandemic. As argued in several papers (e.g., Hanushek & Woessmann, 2020 ; Psacharopoulos et al., 2021 ), there is the risk that the disruption in learning caused by Covid-19 may persist over time, having long-term consequences on students’ knowledge and skills as well as on their labour market prospects. It is therefore very important to determine if and to what extent those children whose schooling was disrupted by Covid-19 subsequently got back on track and reduced their learning deficits. 2 Similarly, it is relevant to gain a more solid understanding of how the educational impact of Covid-19 varies across students and circumstances. This would help educators and policymakers identify those groups of students who may need extra support to recover from the learning deficit caused by the pandemic.
This paper uses meta-analysis in an attempt to synthetize and harmonize evidence about the effect of Covid-19 school closures on student learning outcomes. Meta-analysis, which is widely employed in education as well as in other fields, combines the findings of multiple studies in order to provide a more precise estimate of the relevant effect size and explain the heterogeneity of the results that have been found in individual studies. A total of 239 separate estimates from 39 studies are considered. We extend previous systematic reviews and meta-analyses 3 in four main ways. First, compared to previous meta-analyses, this study covers a larger number of countries (i.e., 19). Not only are several new countries considered in the analysis (e.g., Slovenia, Egypt), but US and UK studies do not dominate the collected empirical evidence. For instance, while in Betthäuser et al. (2023) about 71.1% of the effect sizes are derived from these studies, in our paper the corresponding figure is approximately 33.9%. 4 This makes our results of more general relevance. 5 Second, the current meta-analysis adds to previous meta-analyses by including also studies looking at the impact of Covid-19 among tertiary education students in addition to primary and secondary education students. This is important because, as individuals progress through the education system, academic challenges increase and so does the pressure to perform well. Several studies from various countries (e.g., Bratti et al., 2004 ; Dabalen et al., 2001 ; Koda & Yuki, 2013 ) show that the final grade awarded to students successfully completing university is an important predictor of their labour market prospects. Third, while some relevant moderator variables have already been noted (e.g., subject, level of education, geographical area), the present meta-analysis adds several new ones including type of data and research design. The relevance of these factors in explaining the heterogeneity of results across studies is well-known in the meta-analysis literature. For instance, Havránek et al. (2020) indicate that researchers conducting meta-regression analysis in economics should consider data types. Similarly, Stanley and Jarrell (1989) suggest that variables capturing differences in methodology need to be included among moderators in meta-regression models. More in general, moderators are situational variables as well as characteristics of studies that might influence the effect estimate ( Judd, 2015 ). Fourth, in contrast to previous similar meta-analyses (e.g., König & Frey, 2022 ), we look closely at the issue of the specification of the meta-regression model. As observed by Stanley and Doucouliagos (2012) , this is a more relevant problem in meta-analysis than in primary econometric studies given the higher risk of exhausting degrees of freedom in the former than in the latter. Following recent literature (e.g., Di Pietro, 2022 ), we employ different methods to select the moderator variables to be included in the meta-regression model.
The remainder of the paper is set as follows. Section 2 describes the process of selecting studies and collecting data. It also discusses the empirical approach and the possibility of publication bias. Section 3 reports and discusses the empirical results. Section 4 concludes.
To perform this meta-analysis, we followed the Preferred Reporting Items for Systematic Reviews and Meta-Analysis (PRISMA) ( Moher et al., 2009 ).
2.1. Inclusion criteria
With the purpose of this study in mind, a set of inclusion criteria was defined. They guided the selection of the studies included in this meta-analysis. Specifically, the following four inclusion criteria were used:
- ● the study should quantitatively examine the effect of Covid-19 on student achievement in primary, secondary or tertiary education. This means that the data used in this study were collected before and during the pandemic (or only during the pandemic if, when schools were closed, some students were still receiving in-person teaching thereby simulating pre-pandemic conditions), therefore clearly distinguishing between a control and a treated group, respectively.
- ● the study should use objective indicators (e.g., test scores) to measure student achievement.
- ● the study should be based on real data.
- ● the study should report data on an effect size (or sufficient information to compute it) and its standard error (or t -statistic, or p -value, or sufficient information to calculate it).
2.2. Search ad screening procedures
To identify the relevant studies, we searched in six different electronic databases (i.e., Google Scholar, 6 EconLit, ScienceDirect, Education Resources Information Center, JSTOR and Emerald). The following keywords were used: “Covid-19 (OR coronavirus OR pandemic OR Cov) AND student (OR academic OR scholastic) performance (OR achievement OR learning OR outcome) OR test score”.
This search, which ended on 15 th July 2022, delivered 6,075 hits. 717 duplicates were removed. We kept updated or published versions of any working paper we found. Next, the titles and the abstracts of the remaining 5,358 records were assessed. Following this, 5,205 studies were excluded as they use qualitative approaches (e.g., interviews), report teachers'/parents’ views about the educational impact of Covid-19 (e.g., Kim et al., 2022 ; Lupas et al., 2021 ), or provide a theoretical discussion about how the pandemic is likely to affect education (e.g., Di Pietro et al., 2020 ). Similarly, studies containing predictions and/or projections were also removed (e.g., Kuhfeld et al., 2020a ). After this initial screening, the content of the remaining 153 studies was carefully examined, and only those fulfilling all the inclusion criteria were considered. In this phase, we excluded studies that, although attempting to understand how the pandemic impacted student learning, employ a different outcome measure (e.g., dropout rate) than the one considered in this meta-analysis (e.g., Tsolou et al., 2021 ). In the same vein, we removed studies using student self-reported outcome measures as well as those examining the educational impact of Covid-19 on specific subgroups of students (e.g., Agostinelli et al., 2022 ). Finally, in order to ensure that key sources were not missed, we also screened the references included in previous meta-analyses and systematic reviews. Two more relevant articles were identified through this search. A total of 39 studies was included in this study. Fig. 1 summarizes the literature search and the screening procedure.

Flow chart of the search and screening process.
While all the titles and abstracts were screened only by the author, the next stages of the study selection process were carried out by the author and by another researcher who independently classified the studies as relevant and irrelevant based on the predefined inclusion criteria. While the inter-rater agreement was very high (i.e., 97%), studies on which there was disagreement were discussed in depth until consensus was reached.
2.3. Study coding
All the studies included in this meta-analysis were read in-depth, and relevant information and findings were extracted. Study coding was performed following the same procedure used for the final stages of the study selection process. The inter-rater agreement was again high (i.e., 93%).
In line with the current best practice in meta-analysis ( Polák, 2019 ), we use all relevant estimates included in the selected studies. As argued by Cheung (2019) , not doing so results in missed opportunities to take advantage of all the available data to answer the research question/s under investigation. However, a fundamental issue with this approach lies in the dependence between multiple estimates from the same study given that effect sizes are assumed to be independent in meta-analysis ( Cheung & Vijayakumar, 2016 ). As discussed later in the paper, several methods are used to account for within-study dependence.
2.3.1. Effect size calculation
In order to be able to aggregate the various impact estimates reported in the selected studies, one needs to convert them into a common metric. Consistent with previous relevant systematic reviews and meta-analyses, we use the Cohen's d as a scale-free effect size measure. Cohen's d refers to standardised mean differences and is calculated by dividing the mean difference in student performance between pre-Covid and Covid periods by the pooled standard deviation. While in some cases the Cohen's d was retrieved from the studies, in others it was calculated using information directly available from them. Where the latter was not possible, the studies' author/s was/were contacted to obtain the relevant data. If not reported, the Cohen's d standard error was computed using the formula given in Cooper and Hedges (1994) . In case no information on sample sizes were available from the studies but exact p -values were instead reported, the formula provided by Higgins and Green (2011) was employed to obtain standard errors. In some instances, we also used information on effect sizes contained in the electronic supplement of the meta-analysis article by König and Frey (2022) . For instance, this was the case when a study does not report Cohen's d but this information has been already collected by König and Frey who have contacted the relevant author/s.
2.3.2. Moderator variables
For each effect size, we code several moderator variables, that is, factors potentially influencing the size of the effect of Covid-19 on student achievement. These moderator variables can be divided into two categories: 1) context and 2) characteristics. Regarding the former, we consider:
a) The level of education. Several arguments suggest that remote schooling is more challenging for younger students compared to their older counterparts. To start with, younger learners are less likely to have access, and be able to independently use digital devices. They may be unable to sign into an online class without assistance, may need help or supervision to perform an online task, and may more easily get distracted. Parental engagement therefore plays a crucial role in the success of younger pupils in an online learning environment. However, even though critical, the supervision required for online schooling while younger children are at home may turn out to be unsustainable for many parents who are at the same time engaged with remote working ( Lucas et al., 2020 ). There is also evidence showing that younger students are less likely to have a quiet space to work at home than their older peers. For instance, Andrew et al. (2020) found that in the UK during the first Covid-19 lockdown while the proportion of primary school students reporting not to have a designated space to study at home was about 20%, the corresponding figure for secondary school students was approximately 10%. Furthermore, children in early grades may especially miss in person teaching as they depend on situational learning ( Storey & Zhang, 2021b ). A great emphasis is placed on relationships and interactions with others in order to acquire knowledge. Younger learners are also more likely to need movement and exploration, and these are things that one cannot do while sitting at home and looking at a screen ( Hinton, 2020 ). Finally, some studies ( Domínguez-Álvarez et al., 2020 ; Gómez-Becerra et al., 2020 ) showed that during Covid-19 younger children present more emotional problems than older children. Tomasik et al. (2021) argued that the former group are more likely to have difficulties in coping with socio-emotional stressors associated with the pandemic. Perhaps also as a result of this, there was greater attention to pastoral care than curriculum coverage among primary school students, as opposed to secondary school students ( Julius & Sims, 2020 ).
In an attempt to investigate how the educational impact of the pandemic varies across student age groups, we distinguish between primary, secondary, and tertiary education students.
b) Subject. It is often claimed that the effect of the pandemic on student achievement varies depending on the subject being assessed. Specifically, three main arguments have been advanced to suggest that the pandemic has made students lose more ground in math than in other subjects.
First, while the Covid-19 lockdown has called for increased parental involvement in their children's learning, parents often feel they have difficulties in assisting their children in math. Panaoura (2020) looked at parents' perception of how they have helped their children in math learning during the pandemic in Cyprus. She found that parents' lack of confidence or their low self-efficacy beliefs were enhanced during this period. More teachers' guidance and training would have been needed. Using data on Chinese primary school students during Covid-19, Wang et al. (2022) concluded that parental involvement had a positive impact on children's achievement in Chinese and English, but not in math. While parents are likely to be knowledgeable about the learning content of Chinese and English lessons, this may not be the case for math lessons. In daily life, language practice is more used than math practice. Furthermore, parents may be familiar with math methods different from the ones used by teachers ( Shanley, 2016 ).
Second, teaching math in a fully online context is very challenging. Using data from a survey addressed to math lecturers between May and June 2020, Ní Fhloinn and Fitzmaurice (2021) found that most of the respondents agreed that it is harder to teach math remotely. This is partly due to the idiosyncratic nature of this discipline. It is especially difficult for math instructors to adapt their teaching style to online learning conditions. While many of them used to handwrite the material in real time during their lectures, only a small proportion have the technology to continue doing so online. On the other hand, also students may have problems in communicating math online. Not only do students need to learn and accustom themselves to use technology in order to write mathematical symbols, but this is not always possible in online platforms such as chats ( Mullen et al., 2021 ). Online engagement in math is particularly difficult. Involving students in online discussions around an exact science like math may turn out to be very challenging.
Third, the economic and health problems caused by Covid-19 coupled with the sudden shift to online learning are likely to have increased math anxiety among students. This can be defined as a negative emotional reaction that interferes with the solving of math problems ( Blazer, 2011 , p. 1102). Math anxiety prevents students from learning math because it leads to low self-esteem, frustration, and anger ( Fennema & Sherman, 1976 ). Mamolo (2022) found that the students’ math motivation and self-efficacy decreased during the pandemic. Similarly, Mendoza et al. (2021) and Arnal-Palacián et al. (2022) provided evidence about higher levels of math anxiety experienced by university and primary school students, respectively, during Covid-19.
In light of the above, subjects have been grouped into three different broad categories: math/science, humanities, and a mix category.
c) Timing of student assessment during Covid-19 . As stated earlier, an important question is the extent to which the pandemic has long-lasting effects on learning outcomes. Several arguments suggest that the negative effect of Covid-19 on student achievement may decline as we move to a later stage of the pandemic. To start with, a number of provisions are likely to have been taken in order to help students catch up after the first lockdown and following the re-opening of schools (at least temporarily). An UNESCO, UNICEF, World Bank and OECD report (2021) showed that in the third quarter of 2020 many countries around the world were planning to adopt support programs with the aim of reducing the learning deficit suffered by students earlier in the year. These programs include increased in-person class time, remedial programs, and accelerate learning schemes. Additionally, one would expect students and their parents to have become more used to remote learning during successive school closures and periods of online classes. Finally, many teachers and schools have probably learned important lessons from the first lockdown. These lessons might have helped them design and implement more effective remote learning measures in the subsequent phases of the pandemic.
However, despite the aforementioned considerations, it is possible that it will take some time before students are able to recover from the learning deficit caused by Covid-19. Students may experience problems in re-engaging with education activities following the re-opening of schools. There is evidence showing that, after several months of remote schooling, students have become more passive ad feel disengaged from their learning ( Toth, 2021 ). The stress and anxiety stemming from the pandemic are likely to have caused a fall in student motivation and morale. The uncertainty of the learning environment under Covid-19 could have also contributed to reduce students’ educational aspirations ( OECD, 2020 ). Additionally, during the academic year 2021–2022, as a result of successive waves and different variants of Covid-19, schools had to face several problems including significant staff shortages, high rates of absenteeism and sickness, and rolling school closures ( Kuhfeld & Lewis, 2022 ). Evidence from the US shows that the pandemic has aggravated the problem of teacher shortage ( Schmitt & deCourcy, 2022 ). Following school re-opening, teachers faced new requirements (e.g., hybrid teaching, more administrative tasks) that added to their already full workloads prior to Covid-19 ( Pressley, 2022 ). This increased their stress levels, which made them more likely to leave their job. While many teachers have quit their job during the pandemic, this reduction in staff has not been fully offset by new hires.
In an attempt to look at how the educational impact of Covid-19 changes over time, we distinguish whether the student learning outcome was assessed in 2020 or 2021.
d) The geographical area where the study takes place. We make a distinction between Europe (i.e., Belgium, Czech Republic, Denmark, Germany, Italy, Netherlands, Norway, Spain, Sweden, Slovenia, Switzerland and the UK) and non-Europe (i.e., Australia, Brazil, China, Egypt, Mexico, South Africa and the US).
Coming to 2) characteristics, we code:
e) the type of data . We distinguish between cross-sectional and longitudinal data. As noted by Werner and Woessman (2021), cross-sectional data do not allow to separate the Covid-19 effect from the cohort effects. Using this type of data, the performance of a cohort of students who have been affected by Covid-19 school closures is typically compared to the performance of a previous cohort of students who took the same test in a pre-Covid-19 period. However, this approach does not take into account the possibility that other factors influencing student achievement (e.g., change in education policies) might have changed coincidentally at the same time as Covid-19. Student-level longitudinal (panel) data help to address the cohort effects bias. They allow to look at changes in student performance before and after the lockdown and compare them with the progress made by similar students over the same period of previous years.
f) the type of research design . A number of different methodologies have been used in an attempt to identify the effect of Covid-19 school closures on academic achievement. In this study, we code the type of research design into the following three categories: descriptive, correlational, and quasi experimental/experimental ( Locke et al., 2010 ). Studies using a descriptive research design (e.g., Moliner & Alegre, 2022 ) provide information about the average gap in test scores between the Covid-19 and non-Covid-19 cohorts without accounting for differences between these two cohorts (for example in terms of individual characteristics such as gender and socio-economic background) that could affect academic performances. 7 On the other hand, studies employing a correlational research design (e.g., Ludewig et al., 2022 ) attempt to isolate the effect of Covid-19 from that associated with other factors that could influence student achievement, but their results cannot be given a causal interpretation. Finally, studies using a quasi-experimental or experimental design (e.g., Engzell et al., 2021 ) move closer to a causal interpretation of the relationship between Covid-19 and student performance.
g) the publication year . This study characteristic is a typical moderator variable in meta-analyses. It controls for time-trend effects ( Schütt, 2021 ). In line with the approach followed by several recent meta-analyses (see, for instance, Di Pietro, 2022 ), we consider the year of the first appearance of a draft of the study in Google Scholar. This measure is preferred to publication year on the ground that journals significantly differ with respect to the time between online availability date of an article and the date when the article is given a volume and issue number 8 ( Al & Soydal, 2017 ). Additionally, in our dataset, there are two journal articles that are only available online and it is unclear in which issue of the journal they will be published. The publication years considered are: 2020, 2021, and 2022.
h) the type of publication. This moderator variable is considered in an attempt to control for the quality of the studies included in our sample. We distinguish between journal articles and other publication formats. Articles published in journals are expected to be of higher scientific rigour since they are more likely to have gone through a review process. Additionally, non-journal articles are more likely to contain typos in their regression tables ( Cazachevici et al., 2020 ).
Finally, consistent with the approach taken in several studies (e.g., de Linde Leonard & Stanley, 2020 ), i) the effect size's standard error is also included among our moderator variables.
2.4. Sample characteristics
The dataset used for the meta-analysis includes a total of 239 different impact estimates extracted from 39 separate studies. Each study included in the dataset contains a number of estimates that vary from 1 to 32. Several reasons explain why most studies (i.e., 79%) reported multiple estimates. Many studies (e.g., Bielinski et al., 2021 ; Borgonovi & Ferrara, 2022 ; Feng et al., 2021 ; Gambi & De Witte, 2021 ; Maldonado & De Witte, 2022 ) estimated the effect of Covid-19 on student performance in several subjects. Similarly, a large number of studies (e.g., Ardington et al., 2021 ; Contini et al., 2021 ; Domingue et al., 2021 ; Gore et al., 2021 ) examined the impact of the pandemic on the achievement of students of different levels of education or even of students of different grades within the same level of education. For instance, Meeter (2021) analysed how Covid-19 affected the math performance of primary school children of grades 2–6. Some studies also provided different estimates showing both the short and long-term effects of Covid-19 on student achievement. For example, Kuhfeld et al. (2022) looked at changes in student test scores in fall 2020 and fall 2021 relative to fall 2019.
Table 1 presents the studies included in the dataset. Studies are listed alphabetically. For each study, we report information on the author(s), year of publication, 9 country examined, type of test used to measure student performance, number of the effect sizes collected and their mean value. 10 The studies cover a total of 19 countries. The largest source countries are the US (71 estimates), Germany (39 estimates) and Belgium (33 estimates).
Sources for meta-analysis.
Table 2 shows the descriptive statistics of the moderator variables used in the meta-regressions. While Column (1) displays simple averages (and standard deviations), Column (2) reports averages (and standard deviations) weighted by the inverse of the number of estimates reported in each study. Column (3) reports the number of effect sizes for each moderator variable.
Descriptive statistics.
2.5. Risk of bias assessment
In line with the approach adopted by Betthäuser et al. (2023) and Hammerstein et al. (2021) , the risk of bias in nonrandomized studies was assessed in 38 11 studies using the Risk Of Bias In Non-randomized Studies of Interventions (ROBINS-I) tool ( Sterne et al., 2016 ). Each study was independently evaluated by the author and another researcher, and any disagreements were resolved through discussion to reach a consensus. Studies were scored on six different domains: confounding, participant selection, classification of interventions, missing data, measurement of outcomes, and reporting bias. 12
Table 3 shows the risk of bias ratings for each domain (as well as an overall judgement) for the 38 studies. The lack of appropriate methods to control for confounders, sample selection problems and missing data appear to be the most common sources of potential bias. In several studies, vulnerable students, who have been among the most hardly hit by the pandemic, tend to be under-represented in the Covid-19 sample. This may lead to an underestimation of the pandemic-related learning delays. For example, the study by Gambi and De Witte (2021) relies on a sample where schools participating in the 2021 survey have a more advantaged student population in terms of neighbourhood of residence and mother's education, and have a smaller fraction of students that are considered to be slow learners. Similarly, in the longitudinal data used by Ardington et al., 2021 attrition is significantly higher for the Covid-19 group and attrition is associated with poorer pre-pandemic reading proficiency levels. In Kuhfeld et al. (2022) , between fall 2019 and fall 2021, the number of students testing in a grade dropped significantly more in high-poverty schools compared to their low-poverty counterparts. In other studies, which use non-representative samples including convenience samples (e.g., Moliner & Alegre, 2022 ), the direction of the bias is unclear. One exception is the paper by Meeter (2021) . In his sample the proportion of schools with a more disadvantaged student population appears to be slightly oversampled compared to all schools in the Netherlands, thus potentially biasing upwards the estimated impact of the pandemic on educational achievement. Finally, the question of how the use of non-appropriate methods to control for confounders might affect the estimated relationship between Covid-19 and student performance is addressed later when we discuss the results from the meta-regression analysis. As stated earlier, type of research design is one of our moderator variables.
Risk of bias domain: ROBINS-I.
2.6. Estimators and models
Two approaches frequently used in the meta-analysis literature are: 1) the Fixed Effects (FE) model, and 2) the Random Effects (RE) model. They rely on different assumptions. The FE model assumes that there is one true effect size common to all studies and that all differences in the observed effects can be attributed to within-study sampling error. By contrast, the RE model states that the effect size may vary between studies not only due to within-study sampling error, but also because there is heterogeneity in true effects between studies. Such additional variability is typically modelled employing a between-study variance parameter. Considering the characteristics of the studies included in our sample, it is difficult to assume that there is a common true effect that every study shares. Hence, it is anticipated that the RE model would be more suitable. Specifically, following the approach of Kaiser and Menkhoff (2020) , we estimate the mean of the distribution of true effects using a RE meta-analysis based on a Robust Variance Estimation (RVE). The RVE approach allows to account for the possibility that multiple effect sizes from the same study are not independent from each other. The benefits of this method are that there is no need to drop any effect size (to ensure their statistical independency) and no information is required about the intercorrelation between effect sizes within studies.
In an attempt to investigate factors driving heterogeneity among effect sizes, a meta-regression model is estimated:
where T i denotes the estimated Cohen's d effect size, Z i n is a vector of moderator variables, and ε i is the meta-regression disturbance term. The subscript i stands for the number of effect sizes included in the sample and the subscript n represents the number of moderator variables. In order to deal with the issue of heteroskedasticity in meta-regression analysis, we use Weighted Least Squares (WLS) with weights equal to the inverse of each estimate's standard error. This method is considered to be superior to widely employed RE estimators ( Stanley & Doucouliagos, 2013 ).
A relevant problem in estimating equation (1) lies in the identification of the moderator variables to be included in the model. Selecting incorrect variables leads to misspecification bias and invalid inference ( Xue et al., 2021 ). In line with several recent studies (e.g., Di Pietro, 2022 ; Gregor et al., 2021 ), the “general to specific” approach and the Bayesian Model Averaging (BMA) methodology are used to address model uncertainty. The advantages of the former method are that it addresses the issue of specification-searching bias and minimizes multicollinearity. Moderator variables are removed from the general specification in a stepwise fashion, dropping those with the largest p -value first until all the remaining variables are statistically significant. BMA is a method that runs many regressions containing different combinations of potential explanatory variables and weights them by model fit and complexity. Weighted averages of the estimated coefficients (posterior means) are computed using posterior model probabilities (akin to information criteria in frequentist econometrics). Each coefficient is also given a Posterior Inclusion Probability (PIP), which is the sum of posterior model probabilities of the models including the relevant variable and indicates how likely such a variable is to be contained in the true model ( Havránek et al., 2018 ).
2.7. Publication bias
Publication bias has long been identified as a major problem in meta-analysis ( Dwan et al., 2008 ). Such an issue occurs because editors and scholars tend to prefer publishing papers with statistically significant or non-controversial results. This may lead to distorted conclusions as published findings may end up overstating the true effect. Evidence of publication bias has been found in meta-analyses covering different fields (see, for instance, Begg and Belin (1988) in the case of medical studies).
In line with previous studies (e.g., Di Pietro, 2022 ), we use the Doi plot to graphically evaluate publication bias. Not only does the Doi plot enhance visualization of the asymmetry (in absence of publication bias there is no asymmetry), but it also allows for measuring the asymmetry through the Luis-Furuya-Kanamori (LFK) index. LFK index values within ±1 suggest no asymmetry, LFK index values exceeding ±1 but within ±2 indicate minor asymmetry, while LFK index values exceeding ±2 denote major asymmetry ( Furuya-Kanamori et al., 2018 ). As shown in Fig. 2 , the Doi plot shows no asymmetry (LFK index = 0), indicating that no publication bias is detected.

To further examine the risk of publication bias, we employ the Egger's test ( Egger et al., 1997 ) where the effect size is regressed against its precision (indexed by its standard error). Results indicate that we can safely accept the null hypothesis of no publication bias ( p -value = 0.380).
Our findings are consistent with those in previous relevant meta-analyses. König and Frey (2022) as well as Betthäuser et al. (2023) conclude that the presence of publication bias is unlikely.
3. Results and discussion
This Section is divided into three parts: first, we estimate a summary effect size (Section 3.1 .); second, we investigate potential sources of heterogeneity (Section 3.2 .); and third we provide a discussion of the main results (Section 3.3 .).
3.1. Summary effect size
In order to calculate the overall summary effect, we fit an intercept-only RE RVE model to our set of effect sizes. In such a model, the intercept can be interpreted as the precision-weighted mean effect size adjusted for effect-size dependence ( Friese et al., 2017 ).
The RVE RE mean effect size turns out to be −0.186 13 (SE = 0.0646, p -value = 0.0065, 95% CI [-0.316, −0.055]). It is also important to note that in this model the small-sample corrected degrees of freedom is greater than 4 (i.e., 39), suggesting that the p -value for the associated t -test accurately reflects the type I error ( Tanner-Smith et al., 2016 ).
Next, we compute the I 2 statistic to assess the heterogeneity of the results across studies ( Higgins et al., 2003 ). The appropriateness of the RE model is confirmed as I 2 has a value of 100%. 14 This suggests that all the variability in the effect-size estimates is due to heterogeneity as opposed to sampling error. Additionally, we also look at τ 2 (between-study variance), 15 which denotes the variability in the underlying true effects. Its large value of 1.74 further corroborates the hypothesis of substantial heterogeneity of the effect sizes ( Takase & Yoshida, 2021 ).
One should observe that our findings from the RVE analysis are broadly consistent with those from previous meta-analyses. Storey and Zhang (2021a) concluded that due to Covid-19 students lost, on average, 0.15 standard deviations of learning, König and Frey (2022) found average losses of 0.175 standard deviations, and Betthäuser et al. (2023) estimated average losses at 0.14 standard deviations. 16 Two considerations help put these results into perspective. First, one may notice that the delayed learning suffered by students as a result of Covid-19 school closure is roughly comparable to that experienced by their peers after major natural disasters. For instance, Sacerdote (2012) found that in the spring of 2006 students who were displaced by Katrina and Rita hurricanes saw their test scores fall by between 0.07 and 0.2 standard deviations. A similar result, though of a smaller magnitude, is obtained by Thamtanajit (2020) . He showed that in Thailand floods reduced student test scores by between 0.03 and 0.11 standard deviations, depending on the subject and educational level. Second, following Hanushek and Woessmann (2020) , a learning deficit of about 0.186 standard deviations can be considered to be equivalent to the loss of just over half of a school year. 17
While our results suggest that the pandemic lowered student performance on average by about 0.19 standard deviations, there is a large consensus that it did not affect students equally. For instance, several studies (see, for example, Engzell et al., 2021 ; Hevia et al., 2022 ) showed that Covid-19 had a detrimental effect especially on the achievement of students from less advantaged backgrounds. During school closures, these students are less likely to have had access to a computer, an internet connection, and a space conducive to learning ( Blaskó et al., 2022 ; Di Pietro et al., 2020 ). Moreover, as argued by Ariyo et al. (2022) , one would expect children of less educated parents to have received less parental support while learning at home than children of more educated parents. Greenlee and Reid (2020) provide evidence on this, showing that in Canada during the pandemic the frequency of children's participation in academic activities increased with parental educational levels.
3.2. Heterogeneity
Table 4 shows the results of regressing our standardised measure of student achievement against the moderator variables described above. Column (1) of Table 4 presents estimates from a regression where all potential explanatory variables are included. However, including all 13 variables (in addition to the constant term) in the regression may inflate standard errors and lead to inefficient estimates given that some of the variables may turn out to be redundant. Therefore, the “general-to-specific” approach is employed in an attempt to identify the influential factors. Following this strategy, as shown in Column (2) of Tables 4 , 6 independent variables (in addition to the constant term) are included in the model. To account for the potential dependence of multiple estimates reported by a given study, in Column (3) of Table 4 standard errors are clustered at the study level. Furthermore, since there are relatively few clusters (i.e., 39), following Cameron and Miller (2015) we apply the correction for small number of clusters by employing wild score bootstrapping ( Kline & Santos, 2012 ). Estimates shown in Column (3) indicate that a few moderator variables are robustly important. In line with expectations, students experienced larger learning deficits in math/science. More precisely, other things being equal, student achievement in math/science is on average found to be 0.17 standard deviations smaller than in humanities/subject mix. Our findings indicate also that the negative effect of Covid-19 on student achievement appears to be more pronounced when using experimental/quasi experimental techniques than when using descriptive or correlational research designs. Additionally, studies employing cross-sectional data as well as those focusing on non-European countries tend to suggest greater learning deficits.
Meta-regression results.
Note. Standard errors are in parentheses. Standard errors are clustered at study level (39 clusters) in Columns (3) and (4). In square brackets we report score wild cluster bootstrap p -values ( Kline & Santos, 2012 ) generated using boottest command in Stata with 999 replications ( Roodman, 2016 ). In Columns (1), (2), and (3) the regressions are estimated by weighted least squares where each effect size estimate is weighted by its inverse standard error. In Column (4), the regression is estimated by weighted least squares where each effect size estimate is weighted by its inverse variance.
*, **, and *** denote statistical significance at 10, 5, and 1%, respectively.
As a robustness test, the model depicted in Column (3) of Table 4 is re-estimated but this time each effect size is weighted by its inverse variance. As shown in Column (4) of Table 4 , with the exception of the estimate on longitudinal data, the sign and the magnitude of the other coefficients are broadly in line with those depicted in Column (3).
Next, the BMA approach is employed as an alternative to address the problem of uncertainty in the specification of the meta-regression model. 18 In BMA, following the rule of thumb proposed by Kass and Raftery (1995) , the significance of each explanatory factor is considered not to be weak if the PIP is larger than 0.5. The results, which are reported in Table 5 , show that all the variables that are consistently identified by the BMA methodology as relevant (i.e., Math/Science , Europe and Journal article ) are also included in the specification whose estimates are reported in Columns (2), (3) and (4) of Table 4 . Although the PIP associated with Quasi experimental / experimental does not quite make the relevant threshold, it is relatively close to it.
Bayesian model averaging (BMA).
3.3. Discussion of the main results
Our meta-analysis delivers six main results.
First, we find that, on average, the pandemic depressed student achievement by around 0.19 standard deviations. While this result is in line with the conclusions of earlier meta-analyses and systematic reviews, it should be taken into account that we use a more balanced sample in terms of country composition. This would suggest that our finding is more generalizable than that of previous studies.
Second, the pandemic caused a larger learning deficit in math/science compared to other subjects. This means that extra-support in math/science may be especially needed to help students catch up following the disruption caused by Covid-19.
Third, the effect of Covid-19 on student achievement does not appear to statistically differ across levels of education. Consistent with the findings of Betthäuser et al. (2023) , our results suggest that pandemic-related learning delays are similar across primary and secondary school students. In addition, this research has shown that these learning delays are not statistically different from the learning deficits suffered by tertiary education students. While, as discussed in Subsection 2.3.2 , one would have expected Covid-19 school closures to have had a more negative impact on the achievement of younger students than older students, this effect could have been offset by the greater support in terms of parental involvement received by the former group of students during online learning. Bubb and Jones (2020) found that in Norway, during the peak of the Covid-19 lockdown period, the proportion of parents/carers who reported having gained more information about their children's learning was higher in lower grades than in higher grades. Besides learners' age considerations, one should also observe that the shift towards online learning could have had a detrimental impact on the knowledge and skills of those students, mainly at secondary and tertiary levels, whose curriculum includes experiential learning experiences (e.g., field trips, hands-on activities) that cannot take place virtually ( Tang, 2022 ). However, at the same time, given that our analysis was not conducted at grade level, one cannot rule out the possibility that the pandemic has disproportionately affected the achievement of very young pupils (e.g., grade 1). In other words, there could be heterogeneity within primary school children.
Fourth, our results indicate that in 2021 students were not able to recover from the learning deficits caused by Covid-19 school closures in 2020. There is no statistically significant difference in student performance between assessments that have taken place several months or more than one year after the outbreak of the coronavirus and those that have occurred in the early stages of the pandemic. A similar finding has been obtained by Betthäuser et al. (2023) . It is important to note that, if not addressed, the learning deficits suffered by students may result in significant long-term consequences. Without remedial education upon school re-opening, not only may students who have been disproportionately affected by the pandemic continue to fall behind, but their learning achievements may also suffer a further setback as time goes on ( Angrist et al., 2021 ). Kaffenberger (2021) estimates that if learning in grade 3 is reduced by one-third, the equivalent of about a three-month school closure, learning levels in grade 10 would be a full year lower. Özdemir et al. (2022) forecast that the pandemic could erase decades-long gains in adult skills for affected cohorts unless interventions to alleviate learning deficits are quickly implemented. Additionally, several papers show that there is a relationship between test scores and labour market performance. For instance, Chetty et al. (2014) find that raising student achievement by 0.2 standard deviations is expected, on average, to increase annual lifetime earnings by 2.6%.
Fifth, the extent of the learning deficit seems to be smaller among students in Europe relative to their peers in the rest of the world. Although the reasons behind such a result are unclear, this might be due to several factors. First, one should note that the European countries considered in this study have, on average, a higher gross domestic product per capita than most of the non-European countries included in the analysis (this is not true for the US and Australia). As suggested by Donnelly and Patrinos (2020) , high-income countries are likely to have experienced smaller learning deficits as a result of Covid-19 because of their higher technological capability and the lower share of households living below the poverty line. 19 Second, Schleicher (2020) observes that the impact of the virus on education might have been less severe in many European countries and Southern Hemisphere countries whose 2019–2020 academic calendars had scheduled breaks (up to two weeks) that fell within the school closure period due to Covid-19. Third, there is evidence, but only available at higher education level, that European educational institutions were better prepared to respond to the challenges posed by the pandemic than their counterparts in other parts of the world. A survey carried out by the International Association of Universities immediately after the outbreak of the coronavirus shows that the percentage of higher education institutions where classroom teaching was replaced by distance teaching and learning was higher in Europe than in other continents ( Marinoni et al., 2020 ).
Sixth, our findings seem to suggest that studies using non-causal methods tend to underestimate the negative effect exerted by Covid-19 on student performance. The study by Betthäuser et al. (2023) also hints at the same conclusion, but their meta-analysis does not provide any evidence on this. As pointed out by Engzell et al. (2021) , non-causal methods fail to account for trends in student progress prior to the outbreak of Covid-19 and, hence, by assuming a counterfactual where achievement has stayed flat, they generate estimates of learning deficits that are biased downwards. The underestimation of pandemic-related learning delays may have important policy implications as it could result in under-provision of remedial support to students who are falling behind due to Covid-19.
4. Conclusions
We have assembled and studied a new sample of estimates about the impact of Covid-19 on student achievement. The sample includes 239 estimates from 39 studies covering 19 countries. One of the key findings emerging from our study is that the detrimental effects of Covid-19 school closure on student learning appear to be long-lasting. This calls for more efforts to help students recover from missed learning during the pandemic. As initiatives and programs aimed at learning recovery can be quite costly, several researchers (e.g., Patrinos, 2022 ) stress the importance of protecting the education budget whilst considering the competing financial needs of other sectors such as, for instance, health and social welfare ( UNESCO, 2020b ). Therefore, given the current policy climate where public resources are in high demand by various sectors, it is more important than ever to identify and adopt cost-effective measures.
While there seems to be a relatively large consensus in the literature that small group tutoring programs are a cost-effective way to mitigate the learning deficits caused by the pandemic (see, for instance, Burgess, 2020 ; Gortazar et al., 2022 ), less attention has been paid to a number of time- and cost-effective pedagogical practices ( Carrasco et al., 2021 ). Promoting the development of metacognition skills is, for instance, a powerful way to enhance student learning and performance ( Stanton et al., 2021 ). Metacognition allows students to think about their own learning, and this may increase their self-confidence and motivation. Similarly, increased collaboration and dialogue between students can support learning. Peers may help students clarify study materials and develop critical thinking. Overall, a better understanding is needed about the different types of educational interventions available and their cost-effectiveness. It would be desirable if governments at national, regional and local levels could exchange their experiences in this field and learn from each other.
Funding details
This work has not been supported by any grants.
CRediT authorship contribution statement
Giorgio Di Pietro: Conceptualization, Data curation, Formal analysis, Investigation, Methodology, Software, Writing – review & editing.
Declaration of competing interest
No potential conflict of interest was reported by the author.
☆ The author would like to thank four anonymous referees for their helpful and constructive comments. The usual disclaimer applies.
2 In this study, the term “learning deficit” refers to the lower learning outcomes achieved by students due to the pandemic relative to what would have been expected if the pandemic had not occurred.
3 Previous meta-analyses include König and Frey (2022) who extracted 109 effect sizes nested in 18 studies, Storey and Zhang (2021a) who synthetized 79 effect sizes from 10 studies, and Betthäuser et al. (2023) who considered 291 effect sizes from 42 studies. The reviews by Patrinos et al. (2022) , Moscoviz and Evans (2022) , Donnelly and Patrinos (2022) , Hammerstein et al. (2021) and Zierer (2021) summarised the results of 35 studies, 29 studies, 8 studies, 11 studies and 9 studies, respectively.
4 Similarly, in Storey and Zhang (2021a) 7 out of the 10 studies considered in the meta-analysis are from the US or the UK.
5 One should, however, bear in mind that studies from high-income countries are strongly over-represented.
6 For Google Scholar, in line with the approach of Romanelli et al. (2021) , only the first 100 relevant references at each search were retrieved, as results beyond the first 100 entries were largely irrelevant given the purpose of this study.
7 These studies typically report in a table the mean test scores of the Covid-19 and non-Covid-19 cohorts, together with their corresponding standard deviations and information about the respective sample sizes of the two cohorts. Mean test scores ( X 1 , X 2 ) and their standard deviations ( S 1 , S 2 ) can be used to compute the Cohen's d (i.e., ( X 2 − X 1 ) ( S 1 2 + S 2 2 ) 2 ). Next, Cohen's d standard error can be computed using the formula given in Cooper and Hedges (1994) where information about the sample sizes of the two cohorts and the estimated Cohen's d are used.
8 For instance, in our sample, the journal article by Maldonado and De Witte was available online in 2021 but was published in 2022. On the other hand, the journal article by Ardington et al. was available online and published in 2021.
9 In this table, we report the actual year of publication of the latest version of the study (for journal articles this is the year when they are assigned a volume and issue number) rather than the year of the first appearance of a draft of the study in Google Scholar.
10 All the extracted effect sizes and their standard errors can be found in the supplementary Appendix.
11 One of the studies included in our sample (i.e., Kofoed et al., 2021 ) does use a randomized design.
12 Following Betthäuser et al. (2023) , the domain “deviation from intended interventions” was not considered. As noted by Hammerstein et al. (2021) , information on this domain is very rarely included in the relevant studies because Covid-19 school closures were not intended interventions.
13 The robumeta command in Stata is employed. An intercept-only model is run where the estimate of the meta regression constant is equal to the unconditional mean effect size across studies. With this command, it is possible to specify a value for rho , the expected correlation among dependent effects. Following Tanner-Smith and Tipton (2013) , we use different values of rho ranging from 0 to 1 in intervals of 0.1 in an attempt to check the consistency of results. All models yield the same outcome regardless of the specified value of rho .
14 A value of I 2 greater than 75% is considered large heterogeneity ( Higgins et al., 2003 ).
15 This is calculated using the method-of-moments estimator provided in Hedges et al. (2010) .
16 Relevant systematic reviews have also found similar learning deficits. Donnelly and Patrinos (2022) found average delays of 0.13 standard deviations, Zierer (2021) estimated average losses at 0.14 standard deviations, and Hammerstein et al. (2021) reported average deficits of 0.10 standard deviations.
17 They found that the loss of one third of a school year of learning is equivalent to approximately 11% of a standard deviation of lost test results. This finding is broadly consistent with that obtained by Hill et al. (2008) who conclude that a value of Cohen's d of 0.4 (with a margin of error of ±0.06) corresponds to the average annual reading achievement gains in fourth grade.
18 We treat all moderator variables as auxiliary covariates while the constant is treated as a focus regressor. Each effect size is weighted by its inverse standard error.
19 Results from the meta-analysis by Betthäuser et al. (2023) support this proposition.
Appendix A Supplementary data to this article can be found online at https://doi.org/10.1016/j.edurev.2023.100530 .
Appendix A. Supplementary data
The following is the Supplementary data to this article:
Data availability
- Our Mission
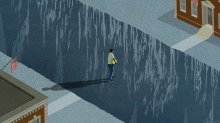
Covid-19’s Impact on Students’ Academic and Mental Well-Being
The pandemic has revealed—and exacerbated—inequities that hold many students back. Here’s how teachers can help.
The pandemic has shone a spotlight on inequality in America: School closures and social isolation have affected all students, but particularly those living in poverty. Adding to the damage to their learning, a mental health crisis is emerging as many students have lost access to services that were offered by schools.
No matter what form school takes when the new year begins—whether students and teachers are back in the school building together or still at home—teachers will face a pressing issue: How can they help students recover and stay on track throughout the year even as their lives are likely to continue to be disrupted by the pandemic?
New research provides insights about the scope of the problem—as well as potential solutions.
The Achievement Gap Is Likely to Widen
A new study suggests that the coronavirus will undo months of academic gains, leaving many students behind. The study authors project that students will start the new school year with an average of 66 percent of the learning gains in reading and 44 percent of the learning gains in math, relative to the gains for a typical school year. But the situation is worse on the reading front, as the researchers also predict that the top third of students will make gains, possibly because they’re likely to continue reading with their families while schools are closed, thus widening the achievement gap.
To make matters worse, “few school systems provide plans to support students who need accommodations or other special populations,” the researchers point out in the study, potentially impacting students with special needs and English language learners.
Of course, the idea that over the summer students forget some of what they learned in school isn’t new. But there’s a big difference between summer learning loss and pandemic-related learning loss: During the summer, formal schooling stops, and learning loss happens at roughly the same rate for all students, the researchers point out. But instruction has been uneven during the pandemic, as some students have been able to participate fully in online learning while others have faced obstacles—such as lack of internet access—that have hindered their progress.
In the study, researchers analyzed a national sample of 5 million students in grades 3–8 who took the MAP Growth test, a tool schools use to assess students’ reading and math growth throughout the school year. The researchers compared typical growth in a standard-length school year to projections based on students being out of school from mid-March on. To make those projections, they looked at research on the summer slide, weather- and disaster-related closures (such as New Orleans after Hurricane Katrina), and absenteeism.
The researchers predict that, on average, students will experience substantial drops in reading and math, losing roughly three months’ worth of gains in reading and five months’ worth of gains in math. For Megan Kuhfeld, the lead author of the study, the biggest takeaway isn’t that learning loss will happen—that’s a given by this point—but that students will come back to school having declined at vastly different rates.
“We might be facing unprecedented levels of variability come fall,” Kuhfeld told me. “Especially in school districts that serve families with lots of different needs and resources. Instead of having students reading at a grade level above or below in their classroom, teachers might have kids who slipped back a lot versus kids who have moved forward.”
Disproportionate Impact on Students Living in Poverty and Students of Color
Horace Mann once referred to schools as the “great equalizers,” yet the pandemic threatens to expose the underlying inequities of remote learning. According to a 2015 Pew Research Center analysis , 17 percent of teenagers have difficulty completing homework assignments because they do not have reliable access to a computer or internet connection. For Black students, the number spikes to 25 percent.
“There are many reasons to believe the Covid-19 impacts might be larger for children in poverty and children of color,” Kuhfeld wrote in the study. Their families suffer higher rates of infection, and the economic burden disproportionately falls on Black and Hispanic parents, who are less likely to be able to work from home during the pandemic.
Although children are less likely to become infected with Covid-19, the adult mortality rates, coupled with the devastating economic consequences of the pandemic, will likely have an indelible impact on their well-being.
Impacts on Students’ Mental Health
That impact on well-being may be magnified by another effect of school closures: Schools are “the de facto mental health system for many children and adolescents,” providing mental health services to 57 percent of adolescents who need care, according to the authors of a recent study published in JAMA Pediatrics . School closures may be especially disruptive for children from lower-income families, who are disproportionately likely to receive mental health services exclusively from schools.
“The Covid-19 pandemic may worsen existing mental health problems and lead to more cases among children and adolescents because of the unique combination of the public health crisis, social isolation, and economic recession,” write the authors of that study.
A major concern the researchers point to: Since most mental health disorders begin in childhood, it is essential that any mental health issues be identified early and treated. Left untreated, they can lead to serious health and emotional problems. In the short term, video conferencing may be an effective way to deliver mental health services to children.
Mental health and academic achievement are linked, research shows. Chronic stress changes the chemical and physical structure of the brain, impairing cognitive skills like attention, concentration, memory, and creativity. “You see deficits in your ability to regulate emotions in adaptive ways as a result of stress,” said Cara Wellman, a professor of neuroscience and psychology at Indiana University in a 2014 interview . In her research, Wellman discovered that chronic stress causes the connections between brain cells to shrink in mice, leading to cognitive deficiencies in the prefrontal cortex.
While trauma-informed practices were widely used before the pandemic, they’re likely to be even more integral as students experience economic hardships and grieve the loss of family and friends. Teachers can look to schools like Fall-Hamilton Elementary in Nashville, Tennessee, as a model for trauma-informed practices .
3 Ways Teachers Can Prepare
When schools reopen, many students may be behind, compared to a typical school year, so teachers will need to be very methodical about checking in on their students—not just academically but also emotionally. Some may feel prepared to tackle the new school year head-on, but others will still be recovering from the pandemic and may still be reeling from trauma, grief, and anxiety.
Here are a few strategies teachers can prioritize when the new school year begins:
- Focus on relationships first. Fear and anxiety about the pandemic—coupled with uncertainty about the future—can be disruptive to a student’s ability to come to school ready to learn. Teachers can act as a powerful buffer against the adverse effects of trauma by helping to establish a safe and supportive environment for learning. From morning meetings to regular check-ins with students, strategies that center around relationship-building will be needed in the fall.
- Strengthen diagnostic testing. Educators should prepare for a greater range of variability in student learning than they would expect in a typical school year. Low-stakes assessments such as exit tickets and quizzes can help teachers gauge how much extra support students will need, how much time should be spent reviewing last year’s material, and what new topics can be covered.
- Differentiate instruction—particularly for vulnerable students. For the vast majority of schools, the abrupt transition to online learning left little time to plan a strategy that could adequately meet every student’s needs—in a recent survey by the Education Trust, only 24 percent of parents said that their child’s school was providing materials and other resources to support students with disabilities, and a quarter of non-English-speaking students were unable to obtain materials in their own language. Teachers can work to ensure that the students on the margins get the support they need by taking stock of students’ knowledge and skills, and differentiating instruction by giving them choices, connecting the curriculum to their interests, and providing them multiple opportunities to demonstrate their learning.
The impact of COVID-19 on student achievement and what it may mean for educators
Subscribe to the brown center on education policy newsletter, jim soland , jim soland assistant professor, school of education and human development - university of virginia, affiliated research fellow - nwea @jsoland megan kuhfeld , megan kuhfeld senior research scientist - nwea @megankuhfeld beth tarasawa , bt beth tarasawa executive vice president of research - nwea @bethtarasawa angela johnson , aj angela johnson research scientist - nwea erik ruzek , and er erik ruzek research assistant professor, curry school of education - university of virginia jing liu jing liu assistant professor of education policy - university of maryland-college park @drjingliu.
May 27, 2020
This Chalkboard post from May 2020 draws on historical data and past research to forecast the possible impact of COVID-19 school closures on student achievement. With actual data from the 2020-21 school year now available, please see this December 2020 Chalkboard post for an updated analysis of this trend.
Virtually all K-12 students in the United States are currently missing face-to-face instruction due to COVID-19. Many parents and educators thus share a common worry: When the pandemic subsides, kids will return to school with lower achievement. There are also concerns that the gap between high- and low-achieving students will become larger. Given the need to address these concerns, we decided to use prior test scores from millions of students and leverage research on summer learning patterns to make informed projections of what learning loss due to the pandemic might look like. Ultimately, we wanted to know: What sort of learning losses could we expect from the shortened 2019-20 school year?
Answering this question is complicated by the unique circumstances of COVID-19. Current school closures have added to the time that most students already spend at home during the summer months without explicit face-to-face instruction from teachers. Meanwhile, teachers are scrambling to adapt content for an online platform and parents are juggling work responsibilities (if not joblessness) with caring for and educating their own children. Students themselves are faced with isolation, anxiety about a deadly virus, and uncertainty about the future. In so many ways, the current situation is unprecedented for most people alive today.
Yet there are parallels between the current situation and other reasons students miss school that can give us insight into how COVID-19 may affect achievement. This includes research on the effects of out-of-school time on learning due to absenteeism , weather-related school closures (e.g., Hurricane Katrina in New Orleans), and summer vacation . Existing evidence can provide a rough sense of how time out of school due to COVID-19 will affect achievement.
We relied heavily on past precedent when trying to understand how COVID-19 might impact achievement in the short and medium term. We used a national sample of over 5 million students in grades 3-8 who took MAP Growth assessments in 2017-2018. These assessments enable such estimates because MAP Growth is administered multiple times per year, which means test scores are available in fall, winter, and spring such that changes in achievement during the year can be understood and anticipated. We compared typical growth for students who completed a standard-length school year to projections under multiple scenarios. These scenarios were directly informed by out-of-school-time research.
The results are deeply concerning.
The two figures below show projected math and reading learning patterns from the beginning of the 2019-20 school year (before COVID-19 school closures) through the start of the 2020-21 school year. The solid lines represent average trajectories in a typical year with typical growth (estimated based on a prior year’s data) followed by normal patterns of learning loss over the summer (generally, student achievement/learning tends to decline during the summer, though this varies greatly by student). Next, we assume an extended summer loss would occur during the period since schools closed. We refer to this scenario as the “COVID Slide” (represented by the dotted lines). These projections give a sense of how much learning students could lose, though we hope they will be overestimations of loss, given the online instruction and home schooling occurring.

These preliminary COVID Slide estimates suggest students could begin fall 2020 with roughly 70% of the learning gains in reading from the prior year relative to a typical school year. In mathematics, students may show even smaller learning gains from the previous year, returning with less than 50% of the gains. In lower grades, students may be nearly a full year behind in math compared to what we would observe in normal conditions.
Though not shown in the figures, we produced similar estimates of learning loss based on research showing the effect of being absent on achievement. That is, we simply assumed students’ learning during COVID-19 school closures would be akin to what occurs when students miss school, a large assumption given the online learning and homeschooling now occurring. Results for absenteeism-based projections were often more dire.
We also examined how much more variable achievement might be in the fall—that is, how wide the range in achievement might be between very high and very low-performing students. This range has implications for whether teachers can provide similar content to all students in their classrooms, or if they might need to further differentiate instruction based on a broader range of needs.

The above figures show our estimate of that variability by subject for 4 th and 6 th grade. The shaded areas display the spread in potential outcomes between students who were in the 25 th percentile of summer learning loss (who showed steep declines) and those in the 75 th percentile (who showed flat lines or even small gains during the summer). In mathematics, we see a fair amount of variability in learning rates, though the majority of students show losses over the extended closure and summer period. However, in reading, there is an even wider spread of potential outcomes, with students who are in the 75 th percentile and above showing sizable learning gains during the summer. Further, the figure below shows that extended time out of school may lead to more variability in achievement when students return in the fall relative to a typical year. A wider range of learning needs like the ones suggested by the figure could create greater challenges for teachers.

The New York Times warns that today’s students could be the “COVID generation.” As we think through our road to recovery, we hope education leaders consider our projections among many data points when preparing to support students returning in the fall. Specifically, our results indicate that:
- Students may be substantially behind, especially in mathematics . Thus, teachers of different grade levels may wish to coordinate in order to determine where to start instruction. Educators will also need to find ways to assess students early, either formally or informally, to understand exactly where students are academically.
- Students are likely to enter school with more variability in their academic skills than under normal circumstances. Therefore, educators may need to consider ways to further differentiate instruction or provide opportunities for individualized learning.
- Students who lose the most during the summer tend to gain the most when back in school, but this may not hold for COVID-19 . Regardless, the ground that students have to make up during the 2020-21 academic year will probably be greater due to COVID-19. Therefore, educators may want to work with students to determine growth rates needed to catch up and set learning goals for the year that are ambitious but obtainable.
Finally, the effects of COVID-19 our study cannot examine may be the ones most worthy of addressing. Prior research on students displaced by Hurricane Katrina indicated that they had difficulty concentrating and often manifested symptoms of depression in the months following the hurricane. Understanding these impacts and how best to support students’ social and emotional needs after the huge disruption of COVID-19 will be essential. Many students may face greater food insecurity, loss of family income, loss of family members to the coronavirus, and fear of catching the virus themselves.
While the scale of the COVID-19 school closures is novel, the inequalities in our school systems are unfortunately anything but new. Our models cannot account for the reality that the crisis is having an unequal impact on our most underserved communities. Nonetheless, we hope these analyses, which synthesize what we know from existing bodies of research, will inform tomorrow’s decision-making.
Related Content
Online only
2:00 pm - 3:00 pm EDT
A. Brooks Bowden, Rebecca Davis
May 26, 2020
Douglas N. Harris
April 24, 2020
Education Policy K-12 Education
Governance Studies
Brown Center on Education Policy
Phillip Levine
April 12, 2024
Hannah C. Kistler, Shaun M. Dougherty
April 9, 2024
Katharine Meyer, Rachel M. Perera, Michael Hansen
Disruptions to School and Home Life Among High School Students During the COVID-19 Pandemic — Adolescent Behaviors and Experiences Survey, United States, January–June 2021
Supplements / April 1, 2022 / 71(3);28–34
Kathleen H. Krause, PhD 1 ; Jorge V. Verlenden, PhD 1 ; Leigh E. Szucs, PhD 1 ; Elizabeth A. Swedo, MD 2 ; Caitlin L. Merlo, MPH 3 ; Phyllis Holditch Niolon, PhD 2 ; Zanie C. Leroy, MD 3 ; Valerie M. Sims, MA 1 ; Xiaoyi Deng, MS 4 ; Sarah Lee, PhD 3 ; Catherine N. Rasberry, PhD 1 ; J. Michael Underwood, PhD 1 ( View author affiliations )
Views: Views equals page views plus PDF downloads
Introduction, limitations, acknowledgment.
- PDF pdf icon [161K]
Youths have experienced disruptions to school and home life since the COVID-19 pandemic began in March 2020. During January–June 2021, CDC conducted the Adolescent Behaviors and Experiences Survey (ABES), an online survey of a probability-based, nationally representative sample of U.S. public- and private-school students in grades 9–12 (N = 7,705). ABES data were used to estimate the prevalence of disruptions and adverse experiences during the pandemic, including parental and personal job loss, homelessness, hunger, emotional or physical abuse by a parent or other adult at home, receipt of telemedicine, and difficulty completing schoolwork. Prevalence estimates are presented for all students and by sex, race and ethnicity, grade, sexual identity, and difficulty completing schoolwork. Since the beginning of the pandemic, more than half of students found it more difficult to complete their schoolwork (66%) and experienced emotional abuse by a parent or other adult in their home (55%). Prevalence of emotional and physical abuse by a parent or other adult in the home was highest among students who identified as gay, lesbian, or bisexual (74% emotional abuse and 20% physical abuse) and those who identified as other or questioning (76% and 13%) compared with students who identified as heterosexual (50% and 10%). Overall, students experienced insecurity via parental job loss (29%), personal job loss (22%), and hunger (24%). Disparities by sex and by race and ethnicity also were noted. Understanding health disparities and student disruptions and adverse experiences as interconnected problems can inform school and community initiatives that promote adolescent health and well-being. With community support to provide coordinated, cross-sector programming, schools can facilitate linkages to services that help students address the adverse experiences that they faced during the ongoing COVID-19 pandemic. Public health and health care professionals, communities, schools, families, and adolescents can use these findings to better understand how students’ lives have been affected during the pandemic and what challenges need to be addressed to promote adolescent health and well-being during and after the pandemic.
Youths have experienced disruptions to school and home life since the COVID-19 pandemic began in March 2020 ( 1 ). The COVID-19 pandemic has disrupted the lives of adolescents by creating or exacerbating economic, food and nutrition, and housing insecurity as well as experiences of abuse, all of which negatively affect health and well-being ( 2 , 3 ). Racial and ethnic discrimination is a social determinant of health ( 4 ), and existing health disparities persisted or worsened during the pandemic. For example, American Indian or Alaska Native, Black, and Hispanic or Latino populations typically experienced higher rates of morbidity and mortality and economic vulnerability compared with the White population before the pandemic and also were more likely than the White population to experience morbidity and mortality from COVID-19 and economic vulnerability during the pandemic ( 5 ). Adolescents experienced disruptions to education and accessing health care, although schools and health care providers shifted rapidly to virtual platforms and telemedicine to continue providing services ( 6 ).
To date, no study has assessed national prevalence of disruptions and adverse experiences experienced by high school students during the COVID-19 pandemic. This study addresses this knowledge gap by estimating the prevalence of disruptions and adverse experiences during the pandemic, overall and by sex, race and ethnicity, grade, and sexual identity. Public health and health care professionals, communities, schools, families, and adolescents can use these findings to better understand how students’ lives have been affected during the pandemic and what challenges need to be addressed to promote adolescent health and well-being during and after the pandemic.
Data Source
This report includes data from the Adolescent Behaviors and Experiences Survey (ABES) conducted by CDC during January–June 2021 to assess student behaviors and experiences during the COVID-19 pandemic. ABES was a one-time, probability-based online survey of U.S. high school students. ABES used a stratified, three-stage cluster sample to obtain a nationally representative sample of public- and private-school students in grades 9–12 in the 50 U.S. states and the District of Columbia (N = 7,705). Participation in ABES was voluntary; each school and teacher decided whether students completed the survey during instructional time or on their own time. Additional information about ABES sampling, data collection, response rates, and processing is available in the overview report of this supplement ( 7 ). The ABES questionnaire, datasets, and documentation are available ( https://www.cdc.gov/healthyyouth/data/abes.htm ).
Students’ self-reported disruptions and adverse experiences were assessed ( Table 1 ), including economic, food and nutrition, and housing insecurity; abuse by a parent or other adult in the home (hereafter referred to as abuse by a parent); receipt of telemedicine; and difficulty completing schoolwork. All questions included the timeframe “During the COVID-19 pandemic,” except for the question about housing insecurity, which asked about experiencing homelessness during the previous 30 days. Demographic variables included sex, race and ethnicity (non-Hispanic American Indian or Alaska Native, non-Hispanic Asian [Asian], non-Hispanic Black [Black], Hispanic or Latino [Hispanic], non-Hispanic persons of multiple races [multiracial], non-Hispanic Native Hawaiian or other Pacific Islander [NH/OPI], and non-Hispanic White [White]), grade (9–12), and sexual identity (gay, lesbian, or bisexual; other or questioning; or heterosexual).
Weighted prevalence estimates and 95% CIs were calculated for disruptions and adverse experiences; estimates were calculated among all students and by demographic characteristics. Bivariate associations between disruptions and adverse experiences and difficulty completing schoolwork are presented. Pairwise t -tests were used to compare prevalence estimates between groups. Estimates were suppressed when n<30; consequently, all results for NH/OPI students were suppressed. Statistical significance was assessed at p<0.05; only significant results are presented. Analyses were completed using SUDAAN (version 11.0.1; RTI International) to account for the complex survey design and weighting.
More than one fourth of adolescents experienced a parent losing a job (28.5%), and nearly one fourth experienced their own job loss (22.3%) or hunger (23.8%) during the COVID-19 pandemic ( Table 2 ). Some experienced homelessness (2.0%). Over half of adolescents experienced emotional abuse by a parent (55.1%), and more than one in 10 experienced physical abuse by a parent (11.3%). Approximately one fourth of students received telemedicine from a doctor or nurse (25.8%), and some received telemedicine for mental health or drug and alcohol counseling (8.5%). Two thirds of students had difficulty completing their schoolwork since the start of the pandemic (66.6%).
Student disruptions and adverse experiences differed by sex and race and ethnicity. Female students experienced a higher prevalence of parental and personal job loss (31.3% and 25.5%), emotional abuse by a parent (62.8%), and difficulty completing schoolwork (69.1%) compared with male students; whereas males experienced homelessness (3.0%) more often. The prevalence of parental job loss was higher among Asian (37.1%) and Hispanic or Latino (38.0%) students compared with all other racial and ethnic groups. Black students experienced the highest prevalence of hunger (32.0%); this estimate is similar to other students of color. White students had the lowest prevalence of experiencing hunger (18.5%), which differed from most other racial and ethnic groups. Multiracial students reported the highest prevalence of emotional abuse by a parent (65.5%), which differed from most other racial and ethnic groups. Black students experienced the highest prevalence of physical abuse by a parent (15.0%); this estimate did not differ from most other students of color, but it was higher than the prevalence of physical abuse experienced by White students (9.8%). Students who reported difficulty completing their schoolwork reported a higher percentage of parental job loss, hunger, or emotional abuse by a parent compared with students who did not have difficulty completing their schoolwork ( Table 3 ).
Students who identified as gay, lesbian, or bisexual and those who identified as other or questioning experienced a higher prevalence of parental job loss (34.9% and 34.9%, respectively), hunger (34.0% and 32.5%, respectively), and emotional abuse by a parent (74.4% and 75.9%, respectively) compared with heterosexual students ( Figure ) (Supplementary Table, https://stacks.cdc.gov/view/cdc/114936 ). Gay, lesbian, or bisexual students experienced a higher prevalence of physical abuse by a parent and difficulty completing their schoolwork (19.7% and 74.4%, respectively) than students who identified as other or questioning (13.4% and 63.8%, respectively) or as heterosexual (9.5% and 65.9%, respectively).
During January–June 2021, approximately half of high school students in the United States reported emotional abuse by a parent or reported difficulty completing their schoolwork since the COVID-19 pandemic began in March 2020. In addition, nearly one in four students reported experiencing hunger or economic insecurity and one in 10 students reported physical abuse by a parent. These findings indicate that adolescents have encountered disruptions and adverse experiences during the pandemic that might impact their immediate and long-term health and well-being.
The finding that more than half of adolescents reported emotional abuse and one in 10 reported physical abuse by a parent or other adult in the home during the pandemic is a public health concern; comparatively, a nationally representative sample from the National Survey of Children’s Exposure to Violence (NSCEV)reported a lower proportion of children aged 14–17 years (13.9% for past-year emotional abuse and 5.5% for past-year physical abuse by a caregiver) ( 8 ). Although these differences might be attributable in part to variations in sampling frame, methodology (e.g., NSCEV is not school based and is administered by telephone) and question wording, the high prevalence of self-reported emotional and physical abuse during the pandemic highlights that increased stress contributes to violence. The situation is further complicated by the fact that school closings because of COVID-19 have resulted in students’ decreased contact with mandated reporters ( 9 ); therefore, the self-reported data in this report are critically important to elucidate the occurrence of child abuse during the pandemic and underscores the need for enhanced violence surveillance and prevention strategies during public health emergencies.
Disparities in experiences of disruption and adversity were observed by sexual identity, race and ethnicity, and sex. Students identifying as gay, lesbian, or bisexual, other or questioning; students of color;,and female students more commonly had disruptions and adverse experiences compared with heterosexual, White, and male students, respectively. Among any demographic grouping, youths who identified as gay, lesbian, or bisexual and other or questioning experienced the highest levels of emotional and physical abuse by a parent. Per analyzed chat transcripts from national online LGBTQ+ support groups for youth, adolescents identifying as lesbian, gay, bisexual, or questioning have been struggling with isolation during the COVID-19 pandemic and coping with family dynamics described as “unsupportive” and “homophobic” ( 10 ). Disparities based on race and ethnicity and sex have been documented throughout the pandemic. Previous research shows that during the pandemic, Black and Hispanic or Latino students were more likely to be in households experiencing food and nutrition insecurity, difficulty paying rent, and difficulty affording household expenses compared with White students ( 11 ), and that approximately two thirds of female adolescents reported an increase in household chores during the pandemic compared with less than half (43%) of the boys, and more girls (20%) compared with boys (10%) reported having too many chores to do to be able to learn ( 12 ).
Many student disruptions and adverse experiences in this report are interconnected with the social determinants of health. Previous research shows that disparities based on race and ethnicity and sex existed among persons who experienced economic, food and nutrition, or housing insecurity before the pandemic, and these persons had a greater likelihood of experiencing these insecurities during the pandemic ( 13 ). In addition, financial and social stressors of the COVID-19 pandemic have been documented as risk factors for increased child abuse ( 9 ). Finally, the bivariate analysis provides evidence that these experiences are interconnected; students who had difficulty completing their schoolwork experienced higher levels of parental job loss, food and nutrition insecurity, and emotional abuse.
One in four students reported using telemedicine to access care from a doctor or nurse and less than one in 10 reported using telemedicine to access mental health or drug and alcohol counseling, with differences by sex and race and ethnicity; White and multiracial students and female students using telemedicine more than other groups. Given the paucity of data on adolescent use of telemedicine, the context for the telemedicine findings of this report remains unclear. Telemedicine might serve as an alternative access point for adolescents seeking essential health services that might address disruptions and adverse experiences, but data describing adolescents’ prepandemic telemedicine use are lacking. A study using data from four major U.S. telehealth providers found that use of telemedicine decreased slightly among youths aged 5–17 years at the start of the pandemic in early 2020 (8.6%) compared with early 2019 (10.0%) ( 6 ), which reflects a lower use than what was found in this report. Future studies could help researchers better understand the range of telemedicine services received and quality of care.
Two thirds of adolescents had difficulty completing their schoolwork since the beginning of the pandemic. These findings are consistent with previous research, which indicates that throughout the COVID-19 pandemic, adolescents have had difficulty transitioning to virtual learning, reporting inconsistencies in school coursework expectations, and confusion about complex and complicated assignments ( 14 ). Students who had difficulty completing their schoolwork experienced higher levels of emotional abuse by a parent, parental job loss, and hunger. These disruptions and adverse experiences threaten adolescents’ health and safety in addition to acting as barriers to learning. Learning is fostered in environments where students’ basic needs are met and where students feel safe, supported, challenged, and engaged ( 15 ). Before the pandemic, schools offered essential health services and social supports, such as school meals, chronic disease management, and mental health counseling; however, the pandemic has challenged the ability of schools to meet students’ evolving academic and health needs ( 16 ).
Schools offer an important pathway to help address the needs of students, but they rely on coordinated efforts across sectors to meet these needs. Prioritization of school health programs and services within schools, in collaboration with families and communities, will be critical to address disruptions to student life and other related effects of the pandemic ( 17 ). For example, during the pandemic, the U.S. Department of Agriculture issued multiple waivers that permitted schools flexibility in distributing free meals to school-aged youths, regardless of family income level, through June 2022 ( 18 ). In addition to traditional meal service in schools, meals are also being distributed in alternative locations, including along school bus routes and in school parking lots and churches ( 18 ). Coordinated, cross-sector programs and services like these are important for providing continued support for students in their lives both inside and outside of school.
General limitations to ABES are outlined in the overview report in this supplement ( 7 ). The findings in this report are subject to at least three specific limitations First, causality or directionality of observed association cannot be determined; although the questions about disruption and adversity ask students about what happened to them during the pandemic (e.g., temporaility associated), it cannot be ascertained that the pandemic caused these student experiences. Second, the telemedicine measures should be interpreted with caution given the unknown context for students’ prepandemic use of telemedicine services. Although most students did not receive telemedicine care from a doctor or nurse since the beginning of the pandemic, students might have accessed in-person health care or might not have needed well-child or other health care visits. In addition, without knowing who provided the care or for which reason, the receipt of telemedicine for mental health or drug and alcohol counseling might not align with students’ needed access to care. Finally, the prevalence of food and nutrition insecurity might have been misclassified because self-reported hunger was used as a proxy measure and this measure has not been validated. In addition, other factors are associated with food and nutrition insecurity (e.g., reducing the size of a meal or the variety of foods consumed) ( 19 ).
Since the beginning of the COVID-19 pandemic in March 2020, many high school students have experienced hunger and economic insecurity. More than half of students have experienced emotional abuse by a parent and have had difficulty completing their schoolwork. Approximately 10% reported physical abuse by a parent. Disparities by sex, race and ethnicity, and sexual identity highlight the importance of strategies to increase health equity in these domains. Understanding health disparities and student experiences of disruptions and adverse experienes as interconnected problems can inform school and community initiatives that promote adolescent health and well-being. With community support to provide coordinated, cross-sector programming, schools can serve as the setting to facilitate linkages to services that help address the adverse experiences that students have faced during the pandemic. Public health and health care professionals, communities, schools, families, and adolescents can use these findings to better understand how students’ lives have been affected during the pandemic and what challenges need to be addressed to promote adolescent health and well-being during and after the pandemic.
Nicole Liddon.
Corresponding author: Kathleen H. Krause, PhD, Division of Adolescent and School Health, National Center for HIV, Viral Hepatitis, STD, and TB Prevention, CDC. Telephone: 404-498-5963; Email: [email protected] .
1 Division of Adolescent and School Health, National Center for HIV, Viral Hepatitis, STD, and TB Prevention, CDC; 2 Division of Violence Prevention, National Center for Injury Prevention and Control, CDC; 3 Divison of Population Health, National Center for Chronic Disease Prevention and Health Promotion, CDC; 4 ICF International, Rockville, Maryland
Conflicts of Interest
All authors have completed and submitted the International Committee of Medical Journal Editors form for disclosure of potential conflicts of interest. No potential conflicts of interest were disclosed.
- World Health Organization. WHO Director-General’s opening remarks at the media briefing on COVID-19–11 March 2020. Geneva, Switzerland: World Health Organization; 2020. https://www.who.int/director-general/speeches/detail/who-director-general-s-opening-remarks-at-the-media-briefing-on-covid-19—11-march-2020 external icon
- US Department of Health and Human Services. Healthy people 2030: food insecurity. Washington, DC: US Department of Health and Human Services; 2021. https://health.gov/healthypeople/objectives-and-data/social-determinants-health/literature-summaries/food-insecurity external icon
- Federal Interagency Forum on Child and Family Statistics. America’s children: key national indicators of well-being, 2017. Washington, DC: Government Printing Office; 2017. https://files.eric.ed.gov/fulltext/ED577338.pdf pdf icon external icon
- American Public Health Association. Structural racism is a public health crisis: impact on the Black community. Washington, DC: American Public Health Association; 2020; Policy LB20–04. https://www.apha.org/policies-and-advocacy/public-health-policy-statements/policy-database/2021/01/13/structural-racism-is-a-public-health-crisis external icon
- McNeely CL, Schintler LA, Stabile B. Social determinants and COVID‐19 disparities: differential pandemic effects and dynamics. World Med Health Policy 2020;12:206–17. https://doi.org/10.1002/wmh3.370 external icon
- Koonin LM, Hoots B, Tsang CA, et al. Trends in the use of telehealth during the emergence of the COVID-19 pandemic—United States, January–March 2020. MMWR Morb Mortal Wkly Rep 2020;69:1595–9. https://doi.org/10.15585/mmwr.mm6943a3 external icon PMID:33119561 external icon
- Rico A, Brener N, Thornton J, et al. Overview and methodology of the Adolescent Behaviors and Experiences Survey—United States, January–June 2021. In: CDC. Adolescent Behaviors and Experiences Survey—United States, January–June 2021. MMWR Suppl 2022;71(No. Suppl 3):1–7.
- Finkelhor D, Turner HA, Shattuck A, Hamby SL. Violence, crime, and abuse exposure in a national sample of children and youth: an update. JAMA Pediatr 2013;167:614–21. https://doi.org/10.1001/jamapediatrics.2013.42 external icon PMID:23700186 external icon
- Campbell AM. An increasing risk of family violence during the COVID-19 pandemic: strengthening community collaborations to save lives. Forensic Sci Int 2020;2:100089. https://www.ncbi.nlm.nih.gov/pmc/articles/PMC7152912/ external icon
- Fish JN, McInroy LB, Paceley MS, et al. “I’m kinda stuck at home with unsupportive parents right now”: LGBTQ youths’ experience with COVID and the importance of online support. J Adolesc Health 2020;67:450–2. https://doi.org/10.1016/j.jadohealth.2020.06.002 external icon PMID:32591304 external icon
- Center on Budget and Policy Priorities. Tracking the COVID-19 recession’s effects on food, housing, and employment hardships. Washington, DC: Center on Budget and Policy Priorities; 2021. https://www.cbpp.org/research/poverty-and-inequality/tracking-the-covid-19-economys-effects-on-food-housing-and external icon
- Ritz D, O’Hare G, Burgess M. The hidden impact of COVID-19 on child protection and wellbeing. London, UK: Save the Children International; 2020. https://resourcecentre.savethechildren.net/pdf/the_hidden_impact_of_covid-19_on_child_protection_and_wellbeing.pdf/ pdf icon external icon
- Perry BL, Aronson B, Pescosolido BA. Pandemic precarity: COVID-19 is exposing and exacerbating inequalities in the American heartland. Proc Natl Acad Sci U S A 2021;118:e20206851118. https://doi.org/10.1073/pnas.2020685118 external icon PMID:33547252 external icon
- Cockerham D, Lin L, Ndolo S, Schwartz M. Voices of the students: adolescent well-being and social interactions during the emergent shift to online learning environments. Educ Inf Technol (Dordr) 2021:1–19. https://link.springer.com/article/10.1007/s10639-021-10601-4 external icon
- CDC. Whole school, whole community, whole child. Atlanta, GA: US Department of Health and Human Services, CDC; 2021. https://www.cdc.gov/healthyschools/wscc/index.htm
- García E, Weiss E. COVID-19 and student performance, equity, and US education policy: lessons from pre-pandemic research to inform relief, recovery, and rebuilding. Washington, DC: Economic Policy Institute; 2020. https://www.epi.org/publication/the-consequences-of-the-covid-19-pandemic-for-education-performance-and-equity-in-the-united-states-what-can-we-learn-from-pre-pandemic-research-to-inform-relief-recovery-and-rebuilding/ external icon
- Kuhfeld M, Tarasawa B, Johnson A, Ruzek E, Lewis K. Learning during COVID-19: initial findings on students’ reading and math achievement and growth. Portland, OR: NWEA; 2020. https://www.nwea.org/content/uploads/2020/11/Collaborative-brief-Learning-during-COVID-19.NOV2020.pdf pdf icon external icon
- Kinsey EW, Hecht AA, Dunn CG, et al. School closures during COVID-19: opportunities for innovation in meal service. Am J Public Health 2020;110:1635–43. https://doi.org/10.2105/AJPH.2020.305875 external icon PMID:32941069 external icon
- Coleman-Jensen A, Rabbitt M, Gregory C, et al. Household food security in the United States in 2020. US Department of Agriculture, Economic Research Service; 2021. https://www.ers.usda.gov/webdocs/publications/102076/err-298.pdf?v=4682.7 pdf icon external icon
*The denominator includes only those who had jobs prior to the beginning of the pandemic.
Abbreviation: AI/AN = American Indian or Alaska Native. * Weighted estimate. † Pairwise t -test significantly different from female students (p<0.05). § Dash indicates that results are suppressed because n<30. ¶ Pairwise t -test significantly different from non-Hispanic AI/AN students (p<0.05). ** Pairwise t -test significantly different from non-Hispanic Asian students (p<0.05). †† Pairwise t -test significantly different from non-Hispanic Black students (p<0.05). §§ Pairwise t -test significantly different from Hispanic or Latino students (p<0.05). ¶¶ Pairwise t -test significantly different from non-Hispanic multiracial students (p<0.05). *** Pairwise t -test significantly different from 9th-grade students (p<0.05). ††† Pairwise t -test significantly different from 10th-grade students (p<0.05). §§§ Pairwise t -test significantly different from 11th-grade students (p<0.05).
* Weighted estimate. † Pairwise t -test significantly different from students who responded “yes” (p<0.05).
FIGURE . Percentage* of parent job loss, †,§ student job loss, hunger, †,§ homelessness, emotional abuse by a parent, †,§ physical abuse by a parent ,†,§,¶ receipt of telemedicine by a nurse or doctor, †,§ receipt of telemedicine for mental health or drug and alcohol counseling, †,§ and schoolwork difficulty †,¶ among high school students during the COVID-19 pandemic, by sexual identity — Adolescent Behaviors and Experiences Survey, United States, January–June 2021
* Weighted estimate.
† Pairwise t -test heterosexual students significantly different from gay, lesbian, or bisexual students (p<0.05).
§ Pairwise t -test heterosexual students significantly different from other or questioning students (p<0.05).
¶ Pairwise t -test other or questioning students significantly different from gay, lesbian, or bisexual students (p<0.05).
Suggested citation for this article: Krause KH, Verlenden JV, Szucs LE, et al. Disruptions to School and Home Life Among High School Students During the COVID-19 Pandemic — Adolescent Behaviors and Experiences Survey, United States, January–June 2021. MMWR Suppl 2022;71(Suppl-3):28–34. DOI: http://dx.doi.org/10.15585/mmwr.su7103a5 external icon .
MMWR and Morbidity and Mortality Weekly Report are service marks of the U.S. Department of Health and Human Services. Use of trade names and commercial sources is for identification only and does not imply endorsement by the U.S. Department of Health and Human Services. References to non-CDC sites on the Internet are provided as a service to MMWR readers and do not constitute or imply endorsement of these organizations or their programs by CDC or the U.S. Department of Health and Human Services. CDC is not responsible for the content of pages found at these sites. URL addresses listed in MMWR were current as of the date of publication.
All HTML versions of MMWR articles are generated from final proofs through an automated process. This conversion might result in character translation or format errors in the HTML version. Users are referred to the electronic PDF version ( https://www.cdc.gov/mmwr ) and/or the original MMWR paper copy for printable versions of official text, figures, and tables.
Exit Notification / Disclaimer Policy
- The Centers for Disease Control and Prevention (CDC) cannot attest to the accuracy of a non-federal website.
- Linking to a non-federal website does not constitute an endorsement by CDC or any of its employees of the sponsors or the information and products presented on the website.
- You will be subject to the destination website's privacy policy when you follow the link.
- CDC is not responsible for Section 508 compliance (accessibility) on other federal or private website.
- Reference Manager
- Simple TEXT file
People also looked at
Original research article, mental health in times of pandemic from the perspective of professors and students.
- 1 Department of Biological Sciences, School of Pharmaceutical Sciences, São Paulo State University (UNESP), Araraquara, Brazil
- 2 Faculty of Medicine and Health Technology, Tampere University, Tampere, Finland
- 3 Tampere University Hospital, Tampere, Finland
- 4 Postgraduate Program in Dental Science, School of Dentistry, São Paulo State University (UNESP), Araraquara, Brazil
- 5 Department of Education, Institute of Biosciences, São Paulo State University (UNESP), Rio Claro, Brazil
- 6 William James Center for Research, University Institute of Psychological, Social, and Life Sciences (ISPA), Lisbon, Portugal
- 7 Flu Pedagogy, Nord University, Bodø, Norway
Introduction: The COVID-19 pandemic brought profound societal changes and disruptions, including in the education system, which underwent swift modifications. It presented unique challenges for both professors and students, contributing to an increase in the prevalence of mental health-related symptoms.
Objective: To determine the prevalence of mental health disorders symptoms, coping strategies, and concerns among students (≥18 years) and professors at different times during the COVID-19 pandemic.
Methods: This was an online cross-sectional study (Student: N = 6,609; Phase 1 = 3,325; Phase 2 = 1,402; Phase 3 = 1,882; Professor: N = 9,096; Phase 1 = 3,924; Phase 2 = 2,223; Phase 3 = 2,949). Depression, Anxiety, and Stress Scale (DASS-21), Impact of Event Scale-revised (IES-R), and BriefCOPE inventory were used. The probability of presenting the symptoms was calculated by multiple logistic regression and odds ratio (OR).
Results: The prevalence of depression, anxiety, stress, and distress symptoms among students and professors was high (≥59.5% and ≥ 33.5%, respectively). Students, women, and those diagnosed with a mental disorder were more likely to have symptoms or distress. In the face of the pandemic, professors used more adaptive coping strategies than students. Health was the focus of professors’ concerns, while for students, future and labor market uncertainty were concerns derived from the health core.
Conclusion: The results point to the need to strengthen psychosocial support for both professors and students.
1 Introduction
Santos (2020) and Harari (2020) argue that the COVID-19 pandemic has taught us some lessons about human behavior and its impact on the world. The authors ( Harari, 2020 ; Santos, 2020 ) point out that the social isolation and quarantine imposed by the health crisis have raised unprecedented social, economic, political, environmental, physical, and emotional issues. As a result, there have been significant changes in several domains of life that have required us to reshape our ways of living.
In Brazil, with the announcement of the pandemic by the World Health Organization (March 11th, 2020), the Ministry of Health decreed lockdowns in several locations across the country, maintaining only essential services, such as hospitals and food establishments. Among the sectors most impacted by the pandemic was the education system, which initially had its activities suspended. However, with the prolongation of the pandemic, it needed to be quickly reorganized into an emergency distance learning system to ensure continuity ( Sousa and Coimbra, 2020 ; Pinho et al., 2021 ; Telyani et al., 2021 ; Tri Sakti et al., 2022 ).
Both professors and students had to adapt to technological and digital resources to make the teaching-learning process viable. This transition demanded proactivity and creativity in implementing synchronous and asynchronous activities. However, there were immediate consequences, such as the expansion of the working hours of professors, the precariousness of working conditions adapted in households, increased spending on work equipment, and a general safety ( Bernardo et al., 2020 ; Azzi et al., 2022 ; Weibenfels et al., 2022 ). Although these consequences were initially focused on the configurations of teaching during the pandemic ( Bernardo et al., 2020 ; Azzi et al., 2022 ; Weibenfels et al., 2022 ), they can be easily extended to students as well.
The surveys conducted with professors ( Bernardo et al., 2020 ; Ozamiz-Etxebarria et al., 2021 ; Pinho et al., 2021 ) show that, regardless of their teaching level and sector (elementary, secondary, or higher education, public or private), many of them encountered an unfamiliar universe, not only in terms of using technology to deliver instruction but also in terms of establishing connections with students and peers in non-face-to-face spaces. Being outside the classroom environment and having family obligations that intersected with course requirements were aggravating elements of the learning process for both professors and students. For students, this experience was perhaps even more remarkable due to the chaotic scenario characterized by a lack of signs of new opportunities and future professional possibilities and chronic inequalities that were constantly and rapidly changing ( Kivunja, 2015 ; Harari, 2018 ). The limited skills and behavioral repertoire that younger people have to cope with so many challenges indicate the complexity of adapting their routine to the pandemic reality ( Campos et al., 2020 ).
In the dynamic of a teaching-learning process where students are the protagonists, the responsibility of the professor extends beyond the mere transmission of information and the construction of knowledge. It should also involve developing the active participation of students in their learning process, giving them autonomy and freedom to carry out their own construction so that learning becomes significant for them. This, in turn, enables the building of educational spaces and relationships in a historical and multicultural context ( Freire, 1996 ). However, the complexity of teaching and the challenges for the unfolding of student protagonism in this unfavorable scenario of the pandemic, especially for those preparing for and approaching the labor market, can lead to overload and physical and mental illness.
In previous studies, a high prevalence of depression, anxiety, stress, and subjective distress symptoms was found among university students ( Chang et al., 2021 ; Li et al., 2021 ; Wang et al., 2021 ; Campos et al., 2021a ) and professors ( Ozamiz-Etxebarria et al., 2021 ; Silva et al., 2021 ). Li et al. (2021) and Silva et al. (2021) indicate that after the onset of the COVID-19 pandemic, this prevalence increased. Some studies also point to a worrisome prevalence of burnout among students ( Azzi et al., 2022 ; Salmela-Aro et al., 2022 ) and professors ( Pressley, 2021 ; Weibenfels et al., 2022 ) given the overload caused not only by the health crisis but also by the rapid and massive transformation of the educational process during COVID-19.
Education in times of pandemic therefore becomes a challenging task, and as we witness the renewal of teaching and learning processes in this scenario, we must have a supportive look at how professors and students have experienced, how they have suffered, how they have resigned, and how these experiences have finally revealed fundamental questions about the life and mental health in this context. We cannot but emphasize that the pandemic severely shook the journey of students in the final year of their program and on their way to professional life, as they were not on school campuses and thus felt even more fragile in their professional identity formation, which in the context of the pandemic seems so far removed from concrete reality.
Thus, this study aimed to gather information on the mental health of Brazilian professors and students (over 18 years old) during the COVID-19 pandemic using a large national sample. Self-reported information was collected to identify the prevalence of mental health disorder symptoms, coping strategies, and main concerns at different times during the pandemic. To the best of our knowledge, this is the first study conducted with a large sample of Brazilian professors and students during different periods of the pandemic, providing relevant information to understand the educational scenario during the pandemic from the perspective of its actors. The evidence presented may also be useful for developing programs and actions aimed at promoting mental health and well-being in the educational environment.
Despite numerous studies conducted during the COVID-19 pandemic, to the best of our knowledge, no research has provided data on coping mechanisms and symptoms across multiple stages of the pandemic or simultaneously collected data from both teachers and students. Thus, the present study is also justified by the scarcity of such information in existing literature. This information could deepen understanding of global transformations in education and their impact on the mental health of teachers and students. This knowledge could better equip practitioners and researchers to develop strategies to handle future crises and support the educational population.
Three main theoretical frameworks were used in the conduction of the present study: one for assessing and interpreting coping strategies; another for identifying symptoms of depression, anxiety, and stress; and a third for recognizing subjective distress in response to the pandemic. To assess coping strategies, the cognitive and behavioral perspective described by Carver et al. (1989) was applied in the present study. These authors developed a model based on the study of Folkman and Lazarus (1980) and on the studies of their own research group, which presented a behavioral model of self-regulation ( Carver and Scheier, 1981 , 1983 , 1985 ; Scheier and Carver, 1988 ). Folkman and Lazarus (1980) proposed a model that divides coping into two functional categories: 1. problem-focused coping and 2. emotion-focused coping. Additionally, it is considered that the coping strategy employed, regardless of its functional category, should not be inherently labeled as good or bad, adaptive or maladaptive ( Folkman and Lazarus, 1980 ; Carver et al., 1989 ). Understanding both the nature of the stressor and the individual and social context involved is necessary. From this perspective, coping refers to cognitive and behavioral efforts, which are deliberate actions individuals undertake to deal with specific demands.
Regarding symptoms of depression, anxiety, and stress, the theoretical model employed in the present study was the one that considers a clinical overlap among these concepts ( Watson et al., 1995 ). This proposal is grounded in the tripartite model, in which symptoms of anxiety and depression are grouped into three basic structures: 1. the presence of negative affects, such as depressed mood, insomnia, discomfort, and irritability; 2. factors representing specific symptoms for depression (anhedonia and absence of positive affect); and 3. specific symptoms of anxiety, including somatic tension and hyperactivity ( Watson et al., 1995 ).
For identifying subjective distress, we used a psychometric scale ( Caiuby et al., 2012 ) developed based on the criteria outlined in the third edition of the Diagnostic and Statistical Manual of Mental Disorders (DSM-III) ( American Psychiatric Association, 1980 ). We clarify that, when conducting the present study, we did not find other simple and self-administered scales for assessing subjective distress that had been developed following the most current edition of DSM (5th ed., DSM-5) ( American Psychiatric Association, 2013 ). Furthermore, given that the present study was conducted online, we understood that using clinical criteria without a clinical assessment would not be feasible. Therefore, the framework adopted for subjective distress was based on the DSM-III ( American Psychiatric Association, 1980 ).
2.1 Study design and participants
This cross-sectional study used online data from a larger project approved by the National Commission for Ethics in Research of the Ministry of Health (CONEP). The larger project is a national survey that has collected data at three-time points, 6 months apart since the beginning of the pandemic, using Google Form or Lime Survey platforms. 1 The link to the survey was sent by email, WhatsApp, or social networks, and remained accessible for about 40 days. The initial contact was made through publicly accessible information on websites of Brazilian higher education institutions (public and private) using non-probability sampling, and the participants were asked to distribute the link to work and personal contacts (snowball technique).
The inclusion criteria were age ≥ 18 years and being a student or professor. In Brazil, students who are 18 years old may be attending the final years of high school/technical school or the first years of undergraduate studies. However, the present study did not differentiate between these levels, as our interest was solely in identifying the participants’ “professional category,” which in this case, was either student or professor. As educational level varies according to age, we chose to control for the effect of age in our statistical analyses rather than specifying educational categories. Information on the teaching level (elementary, high school, or higher education), the type of institution (public or private) of professors, and the education level of the students was also not available. Despite this being a limitation of the study, we understand that its conduction may provide information and trigger relevant reflections that could serve as a starting point for the development of future studies focused on assessing the mental health of these individuals in the peri and post-pandemic context. A total of 12,196, 6,261, and 7,977 people participated in phases 1, 2, and 3, respectively.
2.2 Sample characterization and study variables
Information on age (years), gender (man, woman, non-binary), Brazil macro-region, monthly family income (by income range, in Brazilian reals), pre-existing diagnosis of mental disorder before the pandemic, perceived safety toward the pandemic (very unsafe, unsafe, safe, very safe), and mental health alterations since the beginning of the pandemic (no, yes) was collected. The dependent variables were symptoms of depression, anxiety, stress, subjective distress, and the coping strategies used by the participants. Additionally, participants were asked to provide three open-ended responses to the question: “What are your top 3 concerns at the moment?”
2.3 Sample size calculation
The minimum sample size was calculated based on the number of items of the longest scale (28 items and 14 correlated factors), the number of parameters to be estimated (119), and 5 to 10 participants per parameter. This resulted in a minimum sample size of 595 to 1,190 participants. This sample size was calculated to ensure that the analytical strategies could be conducted, including the verification of the factorial validity of the scales in the samples.
2.4 Measuring scales
The Portuguese version of the Depression, Anxiety and Stress Scale (DASS-21) ( Martins et al., 2019 ), the Impact of Event Scale-revised (IES-R) ( Caiuby et al., 2012 ), and the BriefCOPE Inventory ( Maroco et al., 2014 ) were used in the study.
The DASS-21 has 21 items distributed in 3 factors (Factor/item examples - Depression: “ I felt I wasn’t worth much as a person ,” Anxiety: “ I felt scared without any good reason ” and Stress: “ I felt that I was using a lot of nervous energy ”) and a 4-point Likert-type response scale. The scores of each item are added and multiplied by two. The participants were then grouped according to the degree of involvement, following the proposal of Lovibond and Lovibond (1995) (Depression: Normal - 0 to 9, Mild - 10 to 13, Moderate - 14 to 20, Severe - 21 to 27, and Extremely severe ≥28; Anxiety: Normal - 0 to 7, Mild - 8 to 9, Moderate - 10 to 14, Severe - 15 to 19, and Extremely severe ≥20; Stress: Normal - 0 to 14, Mild - 15 to 18, Moderate - 19 to 25, Severe - 26 to 33, and Extremely severe ≥34).
The IES-R has 22 items distributed in 3 factors (Factor/item examples – Avoidance: “I avoid letting myself get upset when I think about it or I am reminded of it,” Intrusion: “Other things keep making me think about it,” and Hyperarousal: “Reminders of it cause me to have physical reactions, such as sweating, trouble breathing, nausea, or a pounding heart” ), but a general score for subjective distress can be obtained by the sum of the responses (Normal - 0 to 23, Mild - 24 to 32, Moderate - 33 to 36, Severe - ≥37) ( Wang et al., 2020 ). The items have a 5-point Likert-type response scale.
The BriefCOPE has 28 items arranged in 14 factors (Factor/item examples - Active Coping – AC: “I’ve been taking action to try to make the situation better,” Planning – PL: “I’ve been thinking hard about what steps to take,” Instrumental Support – IS: “I’ve been getting help andadvice from other people,” Emotional Support – ES: “I’ve been getting emotional support from others,” Religion – RE: “I’ve been praying or meditating,” Positive Reinterpretation – PR: “I’ve been looking for something good in what is happening,” Self-Blame – SB: “I’ve been criticizing myself,” Acceptance – AT: “I’ve been learning to live with it,” Venting of Emotions – VE: “I’ve been expressing my negative feelings,” Denial – DN: “I’ve been saying to myself “this is not real,” Self-Distraction – SD: “I’ve been turning to work or other activities to take my mind off things,” Behavioral Disengagement – BD: “I’ve been , Substance Use – SU: “I’ve been using alcohol or other drugs to make myself feel better,” and Humor – HU: “I’ve been making jokes about it” ) ( Carver, 1997 ). The scale is answered on a 5-point scale ( Maroco et al., 2014 ) and the participants were grouped considering the average score of each factor into usual strategy (≥3) and non-usual strategy (<3).
2.5 Data validity and reliability
The factorial validity of the data was estimated by confirmatory strategy with a robust estimation of weighted least squares means and variances adjusted (WLSMV). The model fit to the data was evaluated using the following indices: Comparative Fit Index (CFI), Tucker-Lewis Index (TLI), and Root Mean Square Error of Approximation (RMSEA) with a 90% confidence interval ( Marôco, 2021 ; Kline, 2023 ). Data reliability was analyzed to verify the consistency of the obtained information, and it was estimated using the Ordinal Coefficient alpha (α). Values of α ≥ 0.70 were considered indicative of adequate reliability. The analyses were conducted in the R program ( R Core Team, 2022 ) using the “ lavaan ” ( Rosseel, 2012 ) and “ semTools ” ( Jorgensen et al., 2022 ) packages.
The factorial validity of the data was confirmed for each sample and phase of data collection separately (Student – DASS-21: CFI = 0.974–0.976, TLI = 0.971–0.973, RMSEA = 0.059–0.064; BriefCOPE: CFI = 0.949–0.985, TLI = 0.926–0.978, RMSEA = 0.050–0.077; IES-R: CFI = 0.951–0.966, TLI = 0.944–0.961, RMSEA = 0.067–0.080; Professor – DASS-21: CFI = 0.971–0.982, TLI = 0.967–0.979, RMSEA = 0.058–0.066; BriefCOPE: CFI = 0.964–0.984, TLI = 0.948–0.977, RMSEA = 0.064–0.082; IES-R: CFI = 0.968–0.976, TLI = 0.963–0.973, RMSEA = 0.062–0.068). Reliability was also confirmed (Student – DASS-21: α = 0.86–0.94; BriefCOPE: α = 0.70–0.97; IES-R: α = 0.85–0.92; Professor – DASS-21: α = 0.88–0.95; BriefCOPE: α = 0.70–0.96; IES-R: α = 0.88–0.94).
2.6 Similarity analysis
The concerns expressed by professors and students underwent qualitative analysis using similarity analysis, a graph-theoretic method for determining the relationships between the reported concerns of each group about the pandemic. This analysis was conducted for the general sample of each occupational category, without separating the different phases of data collection. With this analysis, it was possible to identify the occurrences/co-occurrences and narrow down the relations between the pandemic-related concerns mentioned by the participants. A static Fruchterman Reingold graph was created to present the results using the Interface de R pour les Analyses Multidimensionnelles de Textes et de Questionnaires - Iramuteq software (version 0.7 alpha 2) ( Ratinaud, 2008/2023 ).
2.7 Statistical analysis
The prevalence of depression, anxiety, stress, and subjective distress symptoms was calculated as a point value with a 95% confidence interval (95%CI) and compared between the data collection phases (within each occupational group) using the z test ( α =5%). The prevalence of usual (mean ≥ 3) coping strategies was also estimated and compared in the same way as described previously.
For each outcome (depression, anxiety, stress, and subjective distress), a multiple logistic regression model was developed: 0 = normal; 1 = mild, moderate, severe, or extremely severe distress taking into account gender [reference category (ref): man], presence/absence of a previous diagnosis of a mental disorder (ref: absent), occupational group [0 = professor (ref); 1 = student], and age. This analysis was performed separately for each phase of data collection. The odds ratio was estimated by point and 95%CI.
2.8 Ethical aspects
Participants voluntarily accessed the link to the survey and signed the informed consent form. The study followed the ethical guidelines of the National Health Council Decision 466/12 and 510/2016 and the guidelines of resolution No. 1/2021-CONEP/SECNS/MS on research in a virtual environment. This study was approved by the National Research Ethics Committee of the Ministry of Health (CONEP) (CAAE 30604220.4.0000.0008).
A total of 6,609 students (Phase 1 = 3,325; Phase 2 = 1,402; Phase 3 = 1,882) and 9,096 professors (Phase 1 = 3,924; Phase 2 = 2,223; Phase 3 = 2,949) participated in the study. Table 1 shows the characteristics of the sample. Of note is the higher prevalence of a previous diagnosis of a mental disorder reported by students compared to professors. There was also a significant difference in the prevalence of mental health changes reported by the two occupational groups since the onset of the pandemic, with students being more affected.
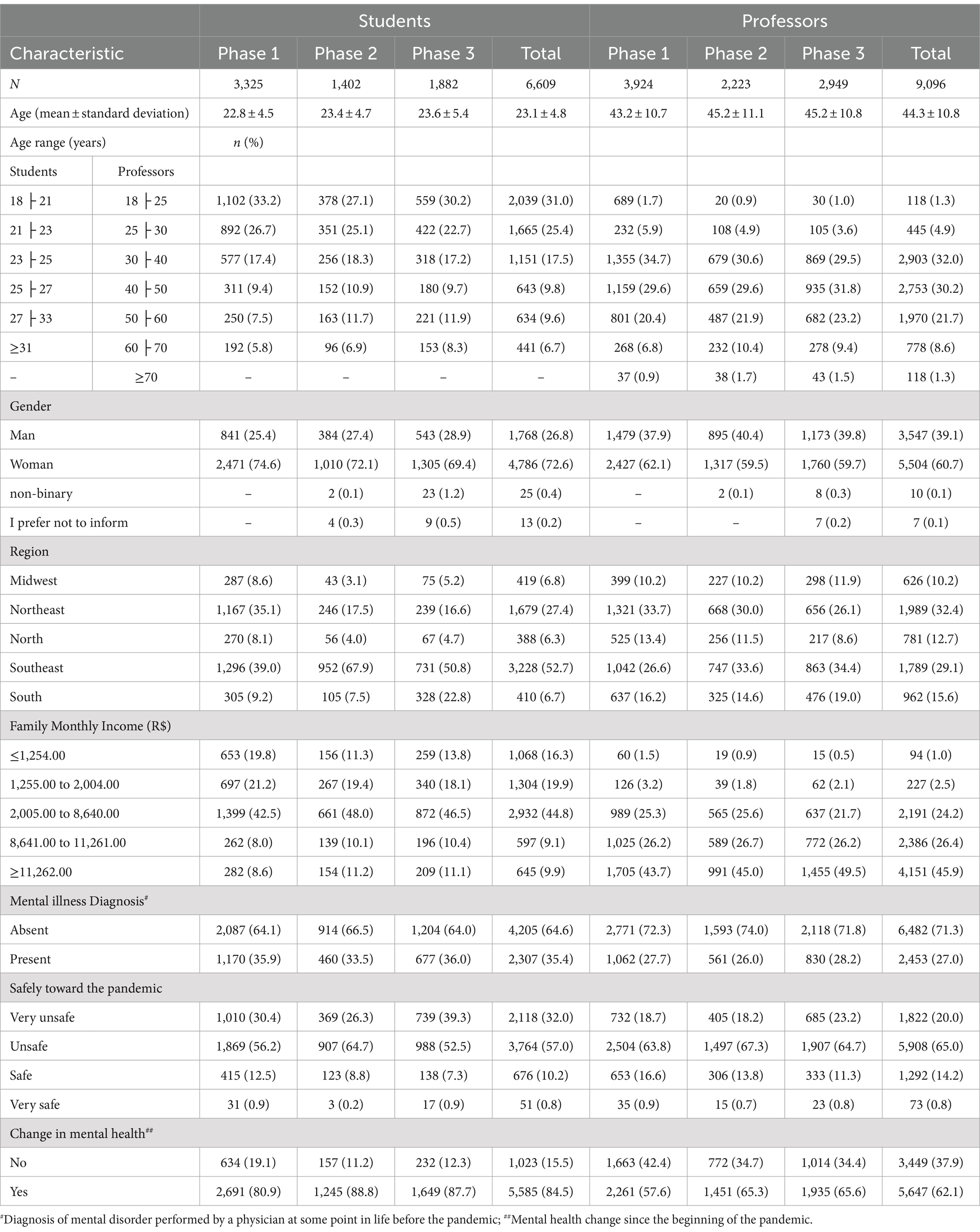
Table 1 . Sample characteristics.
Depression, anxiety, and stress symptoms were significantly higher among professors who participated in Phase 3 than among those who participated in Phases 1 and 2, while subjective distress was lower in Phase 2 of the study ( Table 2 ). Among students, the prevalence of depression and stress symptoms was higher in Phases 2 and 3, while anxiety was higher only in Phase 3. The prevalence of symptoms and distress was high in both occupational categories studied, but these were significantly higher among students (see Table 2 ; Figure 1A ). Professors were more likely to use adaptive coping strategies in the face of the pandemic, such as AC, PL, RE, PR, and AT whereas students were more likely than professors to use maladaptive strategies (SD, BD, SU, DN) ( Table 2 ; Figure 1B ). The use of problem-oriented strategies such as AC, PL, and IS increased from Phase 1 to Phase 3, for both students and professors. Among professors, the prevalence of acceptance was lower and substance use was higher in Phase 3.
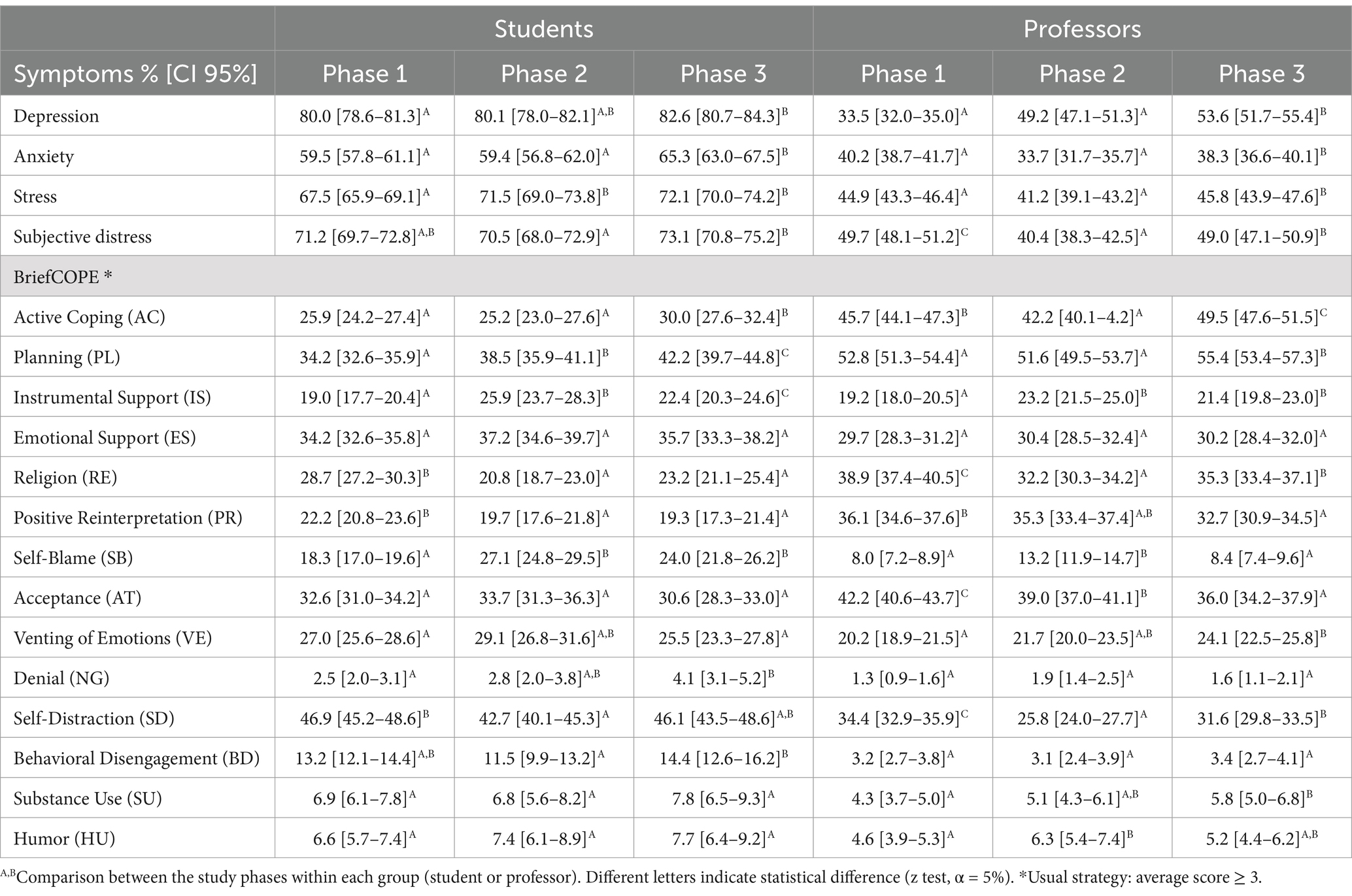
Table 2 . Prevalence [ p 95% (CI)] of depression, anxiety, stress and subjective distress symptoms, and usual coping strategies mentioned in BriefCOPE by students and professors at the different phases of data collection.
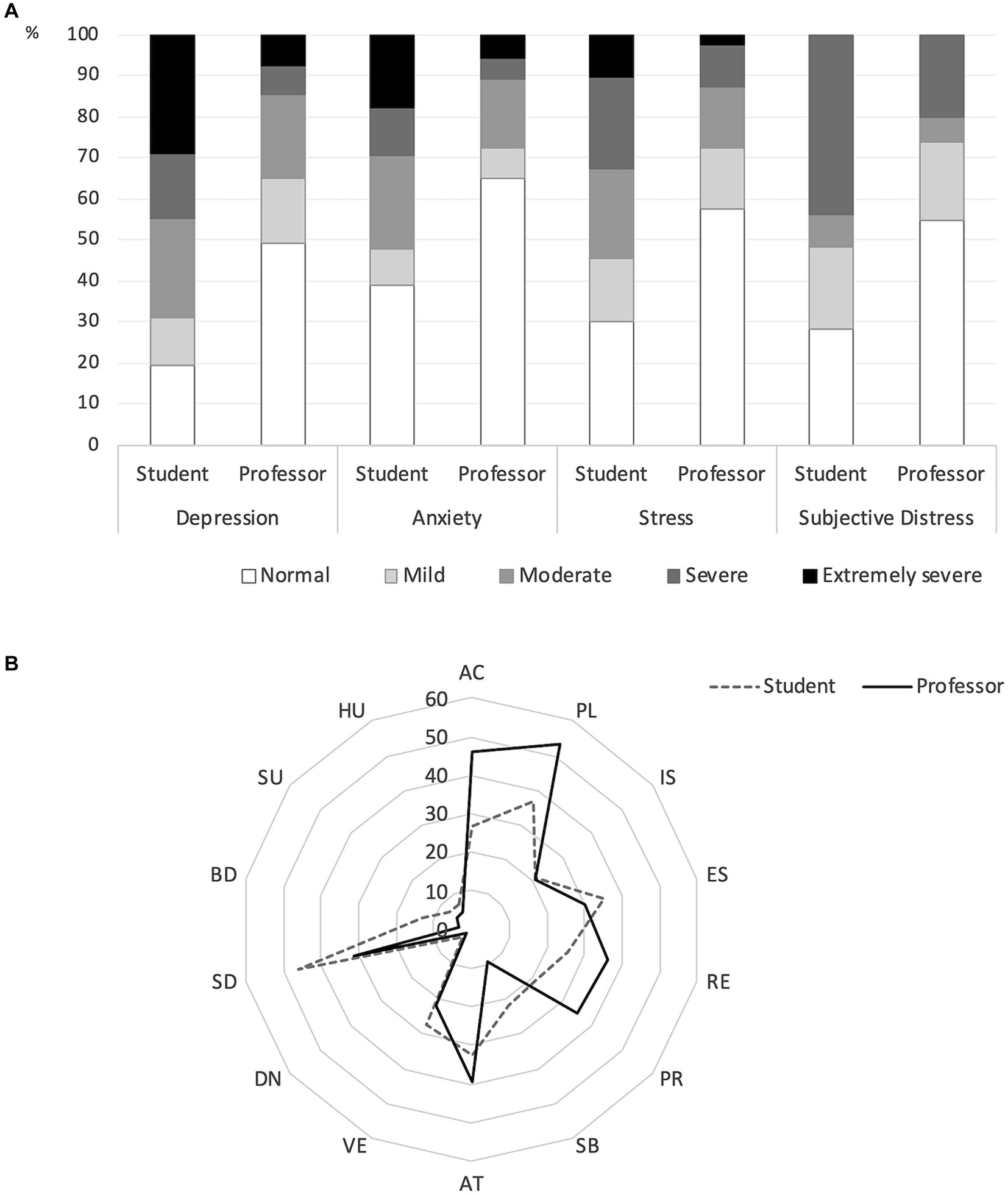
Figure 1 . Distribution of students and professors according to the degree of being affected by symptoms of depression, anxiety, stress, and subjective distress (A) and according to the usual (mean ≥ 3) coping strategy (B) , considering respondents of the three phases of the study (total sample). Radar radius = % of participants using the coping strategy habitually (mean ≥ 3). AC: Active coping; PL: Planning; IS: Instrumental support; ES: Emotional support; RE: Religion; PR: Positive reinterpretation; SB: Self-Blame; AT: Acceptance; VE: Venting of emotions; DN: Denial; SD: Self-Distraction; BD: Behavioral disengagement; SU: Substance use; HU: Humor.
The likelihood of experiencing symptoms of depression, anxiety, and subjective distress was significantly higher among students, women, and those with a prior mental disorder diagnosis. Age was inversely associated with the likelihood of symptoms/distress in the three study phases ( Table 3 ). We clarify that age was included in the model, as it plays an important role in differentiating occupational categories and should therefore be considered.
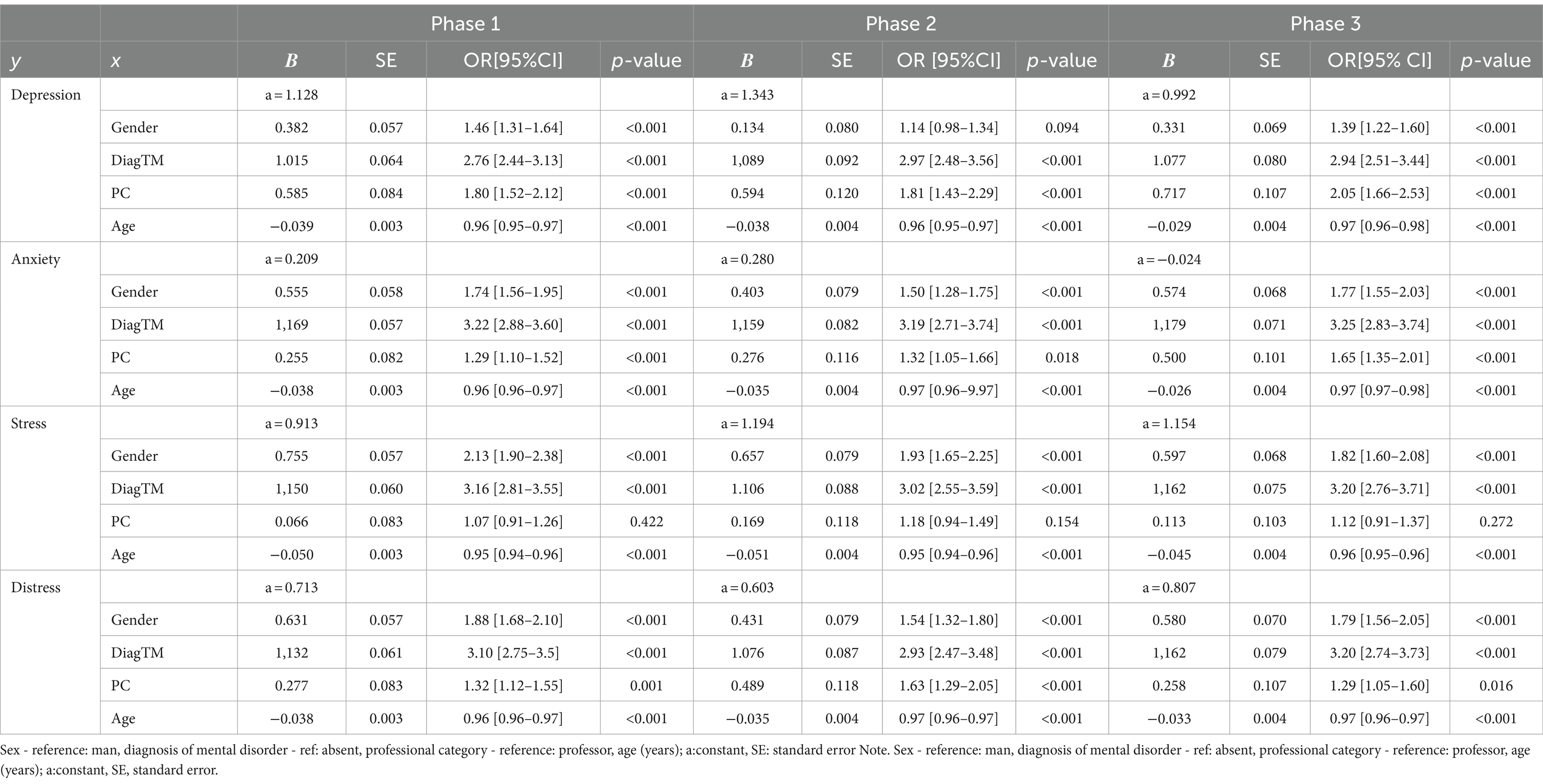
Table 3 . Logistic regression model and odds ratio (OR) of symptom occurrence in relation to mental health according to sex, lifetime diagnosis of mental disorder (DiagTM), professional category (PC), and age.
The main concerns reported by students and professors regarding the pandemic suggest that the two occupational groups had distinct experiences. In the case of professors, the concerns originated from a single core, namely health concerns, from which branched out all other concerns from various spheres of life, particularly family ( Figure 2 ). Conversely, we observed 3 cores among students: health, which branched out and two main groupings (see Figures 2A , B ), family and COVID-19. From the health concern arose group A, which represents the uncertainty and spread of the pandemic (over time), while B is represented by more pragmatic concerns such as unemployment/employment, graduation/education, and money/finances. The family core (group C) is closely related to the health core and is mainly related to worries about the contagion/illness of a family member. The COVID-19 core (Group D) is closer to family than health and also reflects fear of contracting the virus and anticipation of vaccination.
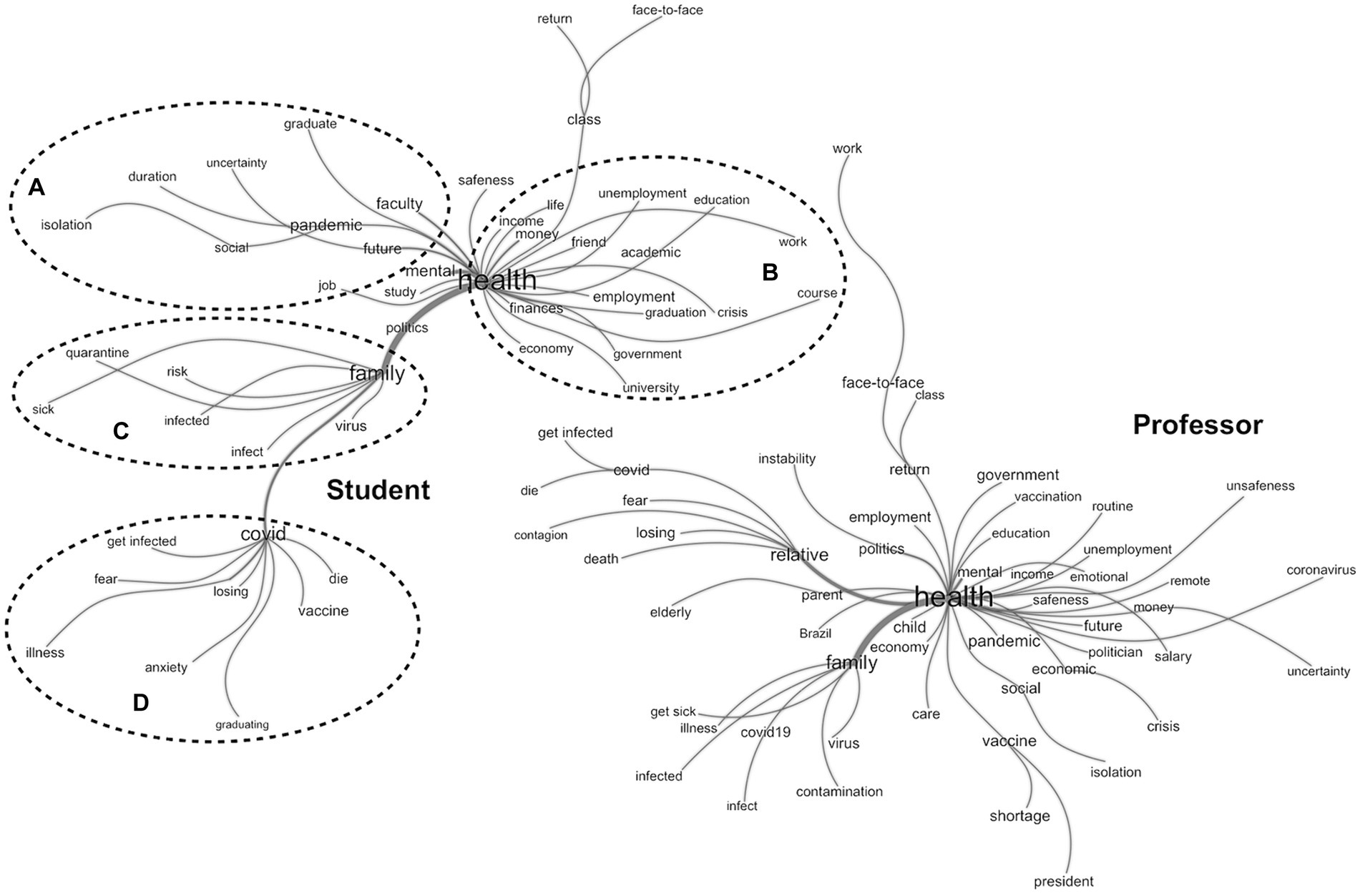
Figure 2 . Similitude analysis of the main concerns of students and professors in the face of the pandemic. Student: 3 cores: health, which branched out and two main grouping (A,B) , family (C) and COVID-19 (D) . Professor: single core: health.
4 Discussion
This study shows that students and professors experienced symptoms of depression (≥33%), anxiety (≥34%), and subjective distress (≥40%), at different times during the pandemic and used various coping strategies. We also found that students (OR = 1.29–2.05; p ≤ 0.001), women (OR = 1.39–2.13, p < 0.001), and those previously diagnosed with a mental health disorder (OR = 2.76–3.25, p < 0.001) were more likely to experience these symptoms. Additionally, our results indicated that professors are teaching young people who have been seriously impacted by the pandemic and require psychological support (e.g., conducting support or assistance actions, engaging in activities that help release tension, or practicing self-care). However, this is complicated by the fact that professors themselves were also experiencing symptoms and distress. Given these challenges, managers and educators need to focus their attention on the need to support mental health in the educational environment. Thus, the main objective of this study was to draw attention to this critical issue.
Tri Sakti et al. (2022) highlighted in a systematic review that the COVID-19 health crisis has had four main consequences on the school population: the major effects on mental health, the teaching-learning process, people’s quality of life, and physical health. The sudden disruption of the school ecosystem required rapid responses to the almost immediate and urgent application of distance education, which resulted in stress, anxiety, and uncertainty that accompanied the health crisis. This situation placed a significant cognitive burden on individuals across education systems and countries ( Keržič et al., 2021 ). This overload led to increasing emotional exhaustion, triggering more or less severe symptoms depending on individual repertoires, social support, and contextual factors ( Hadar et al., 2020 ; Pressley, 2021 ; Salmela-Aro et al., 2022 ).
Students are typically younger and tend to have less life experience and a smaller repertoire of coping strategies than older individuals. Due that, they rely more emotion-focused coping strategies or maladaptive strategies (see Figure 1B ), which can contribute to the maintenance or exacerbation of symptoms ( Restubog et al., 2020 ; Panayiotou et al., 2021 ). In addition, younger individuals were more likely to express their feelings as a coping strategy, which may have contributed to their more frequent reporting of affective symptoms. On the other hand, professors, being older, have a larger cognitive repertoire, which likely contributed to their more frequent use of adaptive coping strategies focused on problem solving, i.e., they used more planning and action skills to eliminate or overcome the daily effects of the pandemic. This leads to better cognitive restructuring of reality, better psychosocial adjustment, and consequently, lower prevalence of symptoms and distress ( Lazarus and Folkman, 1984 ). Better coping and satisfaction with online classes were observed to be strong determinants of academic achievement among students from 10 countries around the world, which serves as a protective factor for health and physical and psychological well-being ( Keržič et al., 2021 ).
The stage of life may be a factor that contributes to the experience of stress and mental health issues for students during the COVID-19 pandemic. The students who participated in the study were over 18 years of age, meaning that they either finished high school or were in various stages of higher education and thus in the process of defining a career and entering the labor market, whereas professors were already in a stable role. Career choice is a complex process and involves expectations and uncertainties. Harari (2018) warned that in the technological era, predictability in the labor market is no longer guaranteed, which increases the need for flexibility and adaptability to constant change. With the advent of the pandemic, the whole scenario of possibilities changed rapidly, and in the context of remote work, uncertainties about their choices and the future increased, which can be observed in our study ( Figure 2 ). While for professors, health was the core of their concerns, which is contextual and to be expected once they were in a stable professional role, for students the core of concerns was divided into the uncertainties about the future on the one hand ( Figure 2A Cluster) and the academic situation and employability on the other hand ( Figure 2B Cluster). This scenario may have contributed to the higher prevalence of symptoms and distress among students, which is supported by the data in the Organization for Economic Cooperation and Development report (2021) .
The sharp decline in spontaneous social interaction and the introduction of distance education are also important factors to be considered. According to Salmela-Aro et al. (2022) and Tonon (2021) , the academic/school environment facilitates contacts and social interactions, providing opportunities to build networks and friendships and space for identity and institutional bonds. With the closure of schools/universities, student engagement in extracurricular activities and peer relationships declined significantly. For young people, this meant a disruption of important socialization rituals, a reduction in personal contact, and increased feelings of loneliness, stress, and burnout ( Salmela-Aro et al., 2022 ). Population studies in the United States and New Zealand present data showing that the prevalence of loneliness among young people (18 to 25 years) has more than doubled since the onset of the pandemic ( Organisation for Economic Co-Operation and Development, 2021 ). It is worth noting that distance education professors also report feelings of loneliness. Telyani et al. (2021) highlighted the relationship between professors’ loneliness and their performance, engagement, job satisfaction, and well-being, and showed that keeping students motivated and engaged in distance education was an arduous task for professors. In general, students and professors differ in terms of their social support network (instrumental and emotional), with professors having a more contextualized cognitive repertoire and coping strategies. Khan and Kadoya (2021) also point to the significant relationship between loneliness and depression and caution the need for interventions that can minimize harm to mental health.
As mentioned earlier, despite the lower prevalence of symptoms in professors than in students, this does not mean that they are low; on the contrary, the prevalence found is alarming and deserves attention ( Table 2 ; 33.5–53.6%). Certainly, COVID-19 has profoundly changed the way education is practiced, requiring intense adaptation from those involved, often without institutional support or sufficient training. In addition, there are complicating factors, such as the enormous social and economic inequalities in the Brazilian population, which are even more evident in the face of distance education ( Santos, 2020 ; Sousa and Coimbra, 2020 ), making the process more difficult. Some studies also suggest that although technological resources have allowed continuity in the communication process, they often create a distance between students and professors that affects social interaction and increases psychological fatigue (technostress: high level of psychophysiological activation; a set of symptoms associated with an excess of information and psychological demands) ( Pinho et al., 2021 ), which can lead to physical and mental illness if not adequately managed. Our results show that the frequency of depression and stress symptoms ( Table 2 ) significantly increased 18 months after the introduction of distance learning compared to the initial phases of the pandemic, both among students (depression: 80.0–82.6%; stress: 67.5–72.1%) and professors (depression: 33.5–53.6%; stress: 44.9–45.8%). Additionally, there was a significant increase in the prevalence of anxiety among students during this period (59.5–65.3%).
Regarding the fact that women (professors or students: OR = 1.39–2.13, p < 0.001) suffer more often from distress, we can support the following arguments: 1. women have more accentuated ruminative reactions than men, which can prolong anxiety and increase the impact of stressors on humor ( Almeida and Kessler, 1998 ; Campos et al., 2020 ); 2. women take on multiple responsibilities and tasks and society expects them to try harder and make fewer mistakes than men ( Foschi, 2000 ); and/or 3. men are encouraged to limit and hide their emotions ( Chaplin, 2015 ). Certainly, other arguments can be used to explain the differences found between men and women; however, we presented only these three because this study was not designed to identify which of these arguments were involved in these results. Thus, these arguments are only speculation, and the reader should consider them cautiously.
Campos et al. (2021b) highlighted the greater vulnerability of people diagnosed with a mental disorder (the observed values in the results of the present study were OR = 2.76–3.25, p < 0.001), in which they reported that these people (1) may have greater emotional instability, (2) may have greater difficulty adapting quickly to changes in their routines, (3) may also have had a disruption in mental health care due to the pandemic, and (4) may have greater difficulty obtaining social support during isolation. Given the pronounced impact of this condition on symptoms and subjective distress, it is suggested that strategic care interventions be developed, such as support, counseling, intervention, and follow-up involving specialized professionals and promoting healthy lifestyles.
Given the data and arguments presented, we understand that educational systems need to strengthen their psychosocial support network and, in this direction, Hadar et al. (2020) recommend that professor education curricula, based on the COVID-19 experience, include the training and development of socio-emotional skills that they can use for themselves and students and others in the educational process for overall well-being. Among the possible techniques for developing these skills, the authors cite conducting case studies and teamwork on problems and challenges encountered in education during the pandemic, training in digital platforms and tools, presentation and familiarization with the topic of crisis management, and stress management techniques.
The first limitation of this study was the fact that it was part of a larger study, which made it impossible to obtain more detailed information about respondents’ job performance (e.g., for professors: number of hours/week worked, work experience, type of institution, and level of education in which they work; for students: course, period and level of the course, full-or part-time studies, screen time used in remote activities, among others), and therefore we recommend conducting future studies. Another limitation is the study design used (non-probability sample and online data collection), which may have limited access to the research for populations with lower levels of education and/or economic status, making it difficult to generalize the data.
A further limitation is that we conducted three independent and anonymous phases in the study, meaning that we cannot determine whether participants took part in just one phase or two or all three. We can also mention the significant decrease in the number of participants from the first to the subsequent phases. This may have occurred due to the increased online demands placed on people during the pandemic, which may have discouraged their participation after 6 and 12 months. However, given the pandemic scenario, this online strategy was feasible to collect information that would directly identify subjective distress in the population. Despite these limitations, we emphasize that there are still few studies in the literature that present data from students and professors at different times during the COVID-19 pandemic and that use large samples.
5 Conclusion
The prevalence of depression, anxiety, stress, and subjective distress symptoms was high among students and professors. Students, women, and those previously diagnosed with a mental disorder were more likely to have mental health symptoms or distress. During the pandemic, professors used more adaptive coping strategies than students. Health was the focus of professors’ concerns, while for students, future and labor market uncertainty were concerns derived from the health core. The results point to the need to strengthen psychosocial support for both professors and students.
Data availability statement
The raw data supporting the conclusions of this article will be made available by the authors, without undue reservation.
Ethics statement
The studies involving humans were approved by the National Research Ethics Committee of the Ministry of Health (CONEP) (CAAE 30604220.4.0000.0008). The studies were conducted in accordance with the local legislation and institutional requirements. The participants provided their written informed consent to participate in this study.
Author contributions
JC: Conceptualization, Formal analysis, Funding acquisition, Investigation, Methodology, Project administration, Software, Supervision, Writing – original draft. LC: Conceptualization, Data curation, Funding acquisition, Investigation, Methodology, Validation, Visualization, Writing – original draft, Writing – review & editing. MA: Conceptualization, Investigation, Methodology, Writing – review & editing. BM: Conceptualization, Data curation, Funding acquisition, Investigation, Methodology, Writing – review & editing. BS: Conceptualization, Data curation, Funding acquisition, Investigation, Methodology, Writing – review & editing. JM: Conceptualization, Investigation, Software, Supervision, Writing – review & editing.
The author(s) declare that financial support was received for the research, authorship, and/or publication of this article. This study was supported by grants #2020/08239-6 and #2021/03775-0, São Paulo Research Foundation (FAPESP); and the National Council for Scientific and Technological Development – CNPQ (#303118/2021-0).
Conflict of interest
The authors declare that the research was conducted in the absence of any commercial or financial relationships that could be construed as a potential conflict of interest.
The author(s) declared that they were an editorial board member of Frontiers, at the time of submission. This had no impact on the peer review process and the final decision.
Publisher’s note
All claims expressed in this article are solely those of the authors and do not necessarily represent those of their affiliated organizations, or those of the publisher, the editors and the reviewers. Any product that may be evaluated in this article, or claim that may be made by its manufacturer, is not guaranteed or endorsed by the publisher.
1. ^ Phase 1: May 18 to June 23, 2020, COVID-19 total (n) cases: 1,228,114, total (n) deaths: 54,971; mean number of cases/day: 25,308.6, mean number of deaths/day: 996.2; phase 2: November 18 to December 23, 2020, n cases: 7,448,560, n deaths: 190,488, mean number of cases/day: 40,442.2, mean number of deaths/day: 626.0; phase 3: May 18 to June 23, 2021, n cases: 18,322,760, n deaths: 511,142, average cases/day: 68,342.8, average deaths/day: 1,912.9.
Almeida, D. M., and Kessler, R. C. (1998). Everyday stressors and gender differences in daily distress. J. Pers. Soc. Psychol. 75, 670–680. doi: 10.1037/0022-3514.75.3.670
PubMed Abstract | Crossref Full Text | Google Scholar
American Psychiatric Association. (1980). Diagnostic and statistical manual of mental disorders: DSM-III . 3rd Edn. Washington, DC: American Psychiatric Publishing.
Google Scholar
American Psychiatric Association. (2013). Diagnostic and statistical manual of mental disorders: DSM-5 . 5th edn. Washington, D.C.: American Psychiatric Publishing.
Azzi, D. V., Melo, J., Neto, A. A. C., Castelo, P. M., Andrade, E. F., and Pereira, L. J. (2022). Quality of life, physical activity and burnout syndrome during online learning period in Brazilian university students during the COVID-19 pandemic: a cluster analysis. Psychol. Health Med. 27, 466–480. doi: 10.1080/13548506.2021.1944656
Bernardo, K. A. S., Maia, F. L., and Bridi, M. A. (2020). As configurações do trabalho remoto da categoria docente no contexto da pandemia covid-19. Novos Rumos Sociol. 8, 8–39. doi: 10.15210/NORUS.V8I14.19908
Crossref Full Text | Google Scholar
Caiuby, A. V. S., Lacerda, S. S., Quintana, M. I., Torii, T. S., and Andreoli, S. B. (2012). Cross-cultural adaptation of the Brazilian version of the impact of events scale-revised (IES-R). Cad Saúde Pública 28, 597–603. doi: 10.1590/S0102-311X2012000300019
Campos, J. A. D., Campos, L. A., Bueno, J. L., and Martins, B. G. (2021a). Emotions and mood swings of pharmacy students in the context of the coronavirus disease of 2019 pandemic. Curr. Pharm. Teach. Learn. 13, 635–642. doi: 10.1016/j.cptl.2021.01.034
Campos, J. A. D., Campos, L. A., Martins, B. G., Valadao Dias, F., Ruano, R., and Maroco, J. (2021b). The psychological impact of COVID-19 on individuals with and without mental health disorders. Psychol. Rep. 125, 2435–2455. doi: 10.1177/00332941211026850
Campos, J. A. D. B., Martins, B. G., Campos, L. A., Marôco, J., Saasiq, R. A., and Ruano, R. (2020). Early psychological impact of the COVID-19 pandemic in Brazil: a national survey. J. Clin. Med. 9:2976. doi: 10.3390/jcm9092976
Carver, C. S. (1997). You want to measure coping but your protocol's too long: consider the brief COPE. Int. J. Behav. Med. 4, 92–100. doi: 10.1207/s15327558ijbm0401_6
Carver, C. S., and Scheier, M. F. (1981). Attention and self-regulation: A control-theory approach to human . New York: Springer-Verlag.
Carver, C. S., and Scheier, M. F. (1983). “A control-theory approach to human behavior, and implications for problems in self-management” in Advances in cognitive-behavioral research and therapy . ed. P. C. Kendal (New York: Academic Press), 127–194.
Carver, C. S., and Scheier, M. F. (1985). “Self-consciousness, expectancies, and the coping process” in Stress and coping . eds. T. Field, P. M. McCabe, N. Schneiderman, and T. M. Field (New York: Psychology Press), 305–330.
Carver, C. S., Scheier, M. F., and Weintraub, J. K. (1989). Assessing coping strategies: a theoretically based approach. J. Pers. Soc. Psychol. 56, 267–283. doi: 10.1037/0022-3514.56.2.267
Chang, J. J., Ji, Y., Li, Y. H., Pan, H. F., and Su, P. Y. (2021). Prevalence of anxiety symptom and depressive symptom among college students during COVID-19 pandemic: a meta-analysis. J. Affect. Disord. 292, 242–254. doi: 10.1016/j.jad.2021.05.109
Chaplin, T. M. (2015). Gender and emotion expression: a developmental contextual perpective. Emot. Rev. 7, 14–21. doi: 10.1177/1754073914544408
Folkman, S., and Lazarus, R. S. (1980). An analysis of coping in a middle-aged community sample. J. Health Soc. Behav. 21, 219–239. doi: 10.2307/2136617
Foschi, M. (2000). Double standards for competence: theory and research. Annu. Rev. Sociol. 26, 21–42. doi: 10.1146/annurev.soc.26.1.21
Freire, P. (1996). Pedagogia da autonomia: saberes necessários à prática . São Paulo: Paz and Terra.
Hadar, L. L., Ergas, O., Alpert, B., and Ariav, T. (2020). Rethinking teacher education in a VUCA world: student teachers’ social-emotional competencies during the Covid-19 crisis. Eur. J. Teach. Educ. 43, 573–586. doi: 10.1080/02619768.2020.1807513
Harari, Y. N. (2018). 21 lessons for the 21st . New York: Random House.
Harari, Y. N. (2020). Notas sobre a pandemia e breves lições para o mundo pós-coronavírus . São Paulo: Companhia das Letras.
Jorgensen, T. D., Pornprasertmanit Schoemann, S. A. M., and Rosseel, Y. (2022). semTools: useful tools for structural equation Modeling . Available at: https://cran.r-project.org/web/packages/semTools/index.html (Accessed February 08, 2022).
Keržič, D., Alex, J. K., Pamela Balbontín Alvarado, R., Bezerra, D. D. S., Cheraghi, M., Dobrowolska, B., et al. (2021). Academic student satisfaction and perceived performance in the e-learning environment during the COVID-19 pandemic: evidence across ten countries. PLoS One 16:e0258807. doi: 10.1371/journal.pone.0258807
Khan, M. S. R., and Kadoya, Y. (2021). Loneliness during the COVID-19 pandemic: a comparison between older and younger people. Int. J. Environ. Res. Public Health 18:7871. doi: 10.3390/ijerph18157871
Kivunja, C. (2015). Teaching students to learn and to work well with 21st century skills: unpacking the career and life skills domain of the new learning paradigm. Int J High Educ 4, 1–12. doi: 10.5430/ijhe.v4n1p1
Kline, R. B. (2023). Principles and practice of structural equation Modeling . 5th Edn New York: The Guilford Press.
Lazarus, R. S., and Folkman, S. (1984). Stress, appraisal and . New York: Springer Publishing Company.
Li, Y., Wang, A., Wu, Y., Han, N., and Huang, H. (2021). Impact of the COVID-19 pandemic on the mental health of college students: a systematic review and meta-analysis. Front. Psychol. 12:669119. doi: 10.3389/fpsyg.2021.669119
Lovibond, S. H., and Lovibond, P. F. (1995). Manual for the depression, anxiety, stress scales . Australia: Psychology Foundation Monograph.
Marôco, J. (2021). Análise de equações estruturais . 3rd Edn. Lisboa: ReportNumber.
Maroco, J., Campos, J. A. D. B., Bonafé, F. S. S., Vinagre, M. G., and Pais-Ribeiro, J. L. (2014). Adaptação transcultural Brasil-Portugal da Escala Brief COPE para estudantes do ensino superior. Psicol. Saúde Doenças 15, 300–313. doi: 10.15309/14psd150201
Martins, B. G., Silva, W. R., Maroco, J., and Campos, J. A. D. B. (2019). Depression, anxiety, and stress scale: psychometric properties and affectivity prevalence. J. Bras. Psiquiatr. 68, 32–41. doi: 10.1590/0047-2085000000222
Organisation for Economic Co-Operation and Development. (2021). Supporting young people’s mental health through the COVID-19 crisis . In: Tackling coronavirus (COVID-19) contributing to a global effort, Paris.
Ozamiz-Etxebarria, N., Idoiaga Mondragon, N., Bueno-Notivol, J., Perez-Moreno, M., and Santabarbara, J. (2021). Prevalence of anxiety, depression, and stress among teachers during the COVID-19 pandemic: a rapid systematic review with meta-analysis. Brain Sci. 11:1172. doi: 10.3390/brainsci11091172
Panayiotou, G., Panteli, M., and Leonidou, C. (2021). Coping with the invisible enemy: the role of emotion regulation and awareness in quality of life during the COVID-19 pandemic. J. Contextual Behav. Sci. 19, 17–27. doi: 10.1016/j.jcbs.2020.11.002
Pinho, P. S., Freitas, A. M. C., Cardoso, M. C. B., Silva, J. S., Reis, L. F., Muniz, C. F. D., et al. (2021). Remote teaching work and health: repercussions of new requirements in the context of the Covid-19 pandemic. Trabalho Educ Saúde 19:e00325157. doi: 10.1590/1981-7746-sol00325
Pressley, T. (2021). Factors contributing to teacher burnout during covid-19. Educ. Res. 50, 325–327. doi: 10.3102/0013189X211004138
R Core Team. (2022). R: A Language and Environment for Statistical Computing. R Foundation for Statistical Computing, Vienna, Austria . Available online at: https://www.R-project.org/ (Accessed March 30, 2024).
Ratinaud, P. (2008/2023). IRAMUTEQ: Interface de R pour les Analyses Multidimensionnelles de Textes et de Questionnaires .
Restubog, S. L., Ocampo, A. C., and Wang, L. (2020). Taking control amidst the chaos: emotion regulation during the COVID-19. J. Vocat. Behav. 119:103440. doi: 10.1016/j.jvb.2020.103440
Rosseel, Y. (2012). Lavaan: an R package for structural equation modeling and more. J. Stat. Softw. 48, 1–36. doi: 10.18637/jss.v048.i02
Salmela-Aro, K., Upadyaya, K., Ronkainen, I., and Hietajarvi, L. (2022). Study burnout and engagement during COVID-19 among university students: the role of demands, resources, and psychological needs. J. Happiness Stud. 23, 2685–2702. doi: 10.1007/s10902-022-00518-1
Santos, B. S. (2020). A cruel pedagogia do . Coimbra: Almedina.
Scheier, M. F., and Carver, C. S. (1988). “A model of behavioral self-regulation: translating intention into action” in Advances in experimental social psychology . ed. L. Berkowits (New York: Academic Press), 303–346.
Silva, D. F. O., Cobucci, R. N., Lima, S., and de Andrade, F. B. (2021). Prevalence of anxiety, depression, and stress among teachers during the COVID-19 pandemic: a PRISMA-compliant systematic review. Medicine (Baltimore) 100:e27684. doi: 10.1097/MD.0000000000027684
Sousa, A. P. R., and Coimbra, L. J. P. (2020). A educação e as novas tecnologias de informação e comunicação no contexto da pandemia do novo coronavírus: o professor "R" e o esvaziamento do ato de ensinar. Rev. Pedag. Cotidiano Ressign. 1, 53–72.
Telyani, A., Farmanesh, P., and Zargar, P. (2021). The impact of covid-19 investigated changes on loneliness of teachers and motivation-engagement of students: a psychological analysis of education sector. Front. Psychol. 12, 1–6. doi: 10.3389/fpsyg.2021.765180
Tonon, G. H. (2021). Student’s quality of life at the university: a qualitative study. Appl. Res. Qual. Life 16, 1517–1535. doi: 10.1007/s11482-020-09827-0
Tri Sakti, A. M., Mohd Ajis, S. Z., Azlan, A. A., Kim, H. J., Wong, E., and Mohamad, E. (2022). Impact of COVID-19 on school populations and associated factors: a systematic review. Int. J. Environ. Res. Public Health 19:4024. doi: 10.3390/ijerph19074024
Wang, C., Pan, R., Wan, X., Tan, Y., Xu, L., Ho, C. S., et al. (2020). Immediate psychological responses and associated factors during the initial stage of the 2019 coronavirus disease (COVID-19) epidemic among the general population in China. Int. J. Environ. Res. Public Health 17:1729. doi: 10.3390/ijerph17051729
Wang, C., Wen, W., Zhang, H., Ni, J., Jiang, J., Cheng, Y., et al. (2021). Anxiety, depression, and stress prevalence among college students during the COVID-19 pandemic: a systematic review and meta-analysis. J. Am. Coll. Heal. 71, 2123–2130. doi: 10.1080/07448481.2021.1960849
Watson, D., Weber, K., Assenheimer, J. S., Clark, L. A., Strauss, M. E., and McCormick, R. A. (1995). Testing a tripartite model: I. Evaluating the convergent and discriminant validity of anxiety and depression symptom scales. J. Abnorm. Psychol. 104, 3–14. doi: 10.1037/0021-843X.104.1.3
Weibenfels, M., Klopp, E., and Perels, F. (2022). Changes in teacher burnout and self-efficacy during the COVID-19 pandemic: interrelations and e-learning variables related to change. Front. Educ. 6, 1–9. doi: 10.3389/feduc.2021.736992
Keywords: mental health, university, students, professors, pandemics
Citation: Campos JADB, Campos LA, Azevedo MAR, Martins BG, Silva BNS and Marôco J (2024) Mental health in times of pandemic from the perspective of professors and students. Front. Educ . 9:1353756. doi: 10.3389/feduc.2024.1353756
Received: 11 December 2023; Accepted: 26 March 2024; Published: 08 April 2024.
Reviewed by:
Copyright © 2024 Campos, Campos, Azevedo, Martins, Silva and Marôco. This is an open-access article distributed under the terms of the Creative Commons Attribution License (CC BY) . The use, distribution or reproduction in other forums is permitted, provided the original author(s) and the copyright owner(s) are credited and that the original publication in this journal is cited, in accordance with accepted academic practice. No use, distribution or reproduction is permitted which does not comply with these terms.
*Correspondence: Lucas Arrais Campos, [email protected]
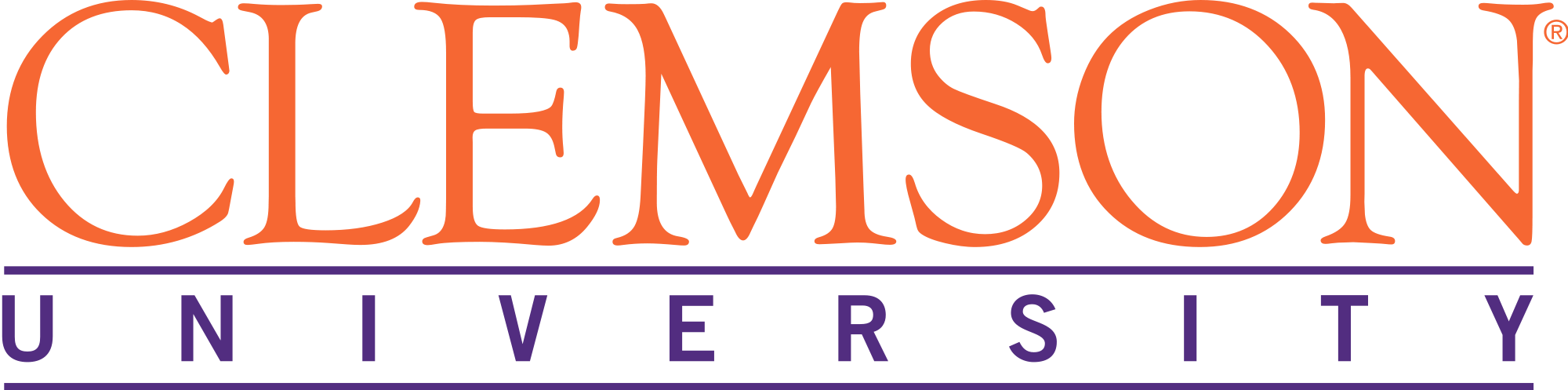
Want to create or adapt books like this? Learn more about how Pressbooks supports open publishing practices.
9 Impact of COVID-19 on K-12 Students
Susan Taylor
Introduction
The novel Coronavirus, also known as COVID-19 took the world by surprise. The United States was not prepared for this pandemic in any aspects and now people are suffering greatly. What at first seemed like a far off disease turned into a global pandemic within a few months and completely changed how our society functioned. One of the areas that was greatly affected was the K-12 educational system. Students around the country are suffering from learning loss, inadequate teaching methods, inability to keep up with online technology while also dealing with many other pandemic related stresses. These developments could have major consequences for students. They are extremely concerning because education is the basis of our society.
Connection to STS Theory
The pandemic will have major consequences for students in the future. This is an example of Path-dependency. The Path-dependency theory occurs when past events affect future outcomes. The negative effects that COVID-19 has had on education could impact students for many years to come. The loss of learning that the pandemic has caused students could lead to a decrease in wages they earn in the future, a lower national GDP , and also make it harder for students to find jobs. Students who are affected by COVID-19 could have a worsened mental health, both in the present day and in the future because they are constantly surrounded by new stressors.
The New Aspect of Technology
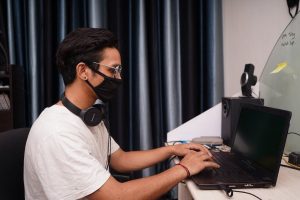
We live in a society where technology is prevalent and commonly used in our everyday lives. However, the education system is in many aspects lagging behind in technology use. Many schools around the country have integrated online technology into their standards or have at least some devices for students to use. But at the same time, many schools do not have the resources to do so. When COVID-19 first hit in spring 2020 schools were taken by surprise and closed. Students received little to no education for the rest of the semester and technology was partially to blame.
Many students do not have the necessary technology needed to survive in this new hybrid and online teaching environment. In a study conducted by EdWeek in May of 2020, only around 59% of schools (elementary to high) surveyed had 1 to 1 student to computer ratios ( Bushweller ). This is an alarmingly low percentage considering schools went virtual for almost all of the spring 2020 semester. Many students have to share devices with family, friends and siblings, and completing school work can be a huge challenge for them. Large numbers of students also do not have access to quality internet at home. Even if they did have a device at home it would be impossible to complete online work. Minority students are more likely to struggle with these new teaching methods than other groups. Many of them do not have a device to complete school work, have to share devices with others or do not have internet connection at their homes. Minority students are also more likely to go to underfunded public schools that can not afford to supply students with devices.
Online learning requires not only the availability of technology, but also the knowledge of how to use it. Students who usually learned with a traditional teaching method might have never had practice using online technology in their school work. They may have used the technology mainly for games or social activities. Without the prior practice and knowledge of how to use their devices in learning, students might find them practically useless ( García & Wiess ).
Education Loss
It is completely normal for students to have a slight learning loss during the summer or long breaks, but the situation with COVID-19 is much different. Students not only have loss from summer break, they also missed many months of educational time during the spring and possibly parts of the fall semesters. This loss needs to be factored in their learning as well. Teaching methods that differ from the traditional ways are expected to grow the already large learning gap even more.
Hybrid and online learning methods are much different than the usual way most students are taught. Students were also thrown into these forms of teaching by surprise in the spring of 2020, resulting in many negative effects on their learning. A study done at Fresno State explains just how bad the learning loss gap will be for students in these new situations. Students who experience average remote learning will lose about 3-4 months of learning. Low quality remote learning will account for about 7-11 months of learning loss and no instruction at all would result in 12-14 months of lost learning ( Dorn et al. ). This time during a student’s life is crucial for building fundamental knowledge that will aid them for the rest of their lives, and this loss is devastating.
The consequences for minority students will be even worse. There is already an educational gap between minority and white students. COVID-19 will likely make it grow larger. Minority students are less likely to have outside resources to support their learning compared to students of higher socioeconomic status because such resources can be very pricey. Parents of minority students are also more likely to work laboring jobs that have long hours, so it is difficult for them to receive extra help outside of the classroom. It is also less likely that these students will have a quiet place to work, without distractions ( Fox ).
Learning Loss equals Earning Loss
This time during a student’s life is crucial for building fundamental knowledge that will aid them for the rest of their lives so this loss is devastating. The loss of education now can lead to a big economic impact in the future. It is estimated that students could lose anywhere from $61,000 to $82,000 in lifetime earnings just from COVID-19 effects so far. When you factor in the current overall dropout rate the average lifetime earning loss goes to about $2.2 billion ( Dorn) . Student dropout rate is higher than ever due to the pandemic and this will lead to a decades long problem for the U.S. and its citizens. There would be a never ending cycle between loss of education and loss of earning.
America’s GDP is nowhere near as high as it could be due to educational gaps between white and minority students. Less education usually means less pay, even in a society without the Coronavirus. Now that COVID-19 is a factor that is affecting the economy, there will be even bigger blows. The loss or lack of education now will lead to an even greater drop in the country’s GDP. This is a problem because the overall wealth of our country and its citizens will decrease.
With the decrease in effectiveness of teaching methods we are creating a less educated society. If this continues then students will not be prepared for their next years of school and their careers that come afterwards. Not only are they losing the teachable aspect of education, they are also missing out on the independently learned side of it as well. K-12 years are crucial for students to learn about problem solving, respect, teamwork and so many more aspects that lead to a successful, sociable person. Many jobs look for these key qualities when hiring employees, but with the loss in learning caused by COVID-19 students with competence in them will be lacking in the future. This could cause many students to not find good jobs or have decreased pay. This development will hurt not only students, but the country as a whole and make it function less effectively.
Pandemic Related Stress
Pandemics are an extremely stressful time for everyone and it is even worse on children, especially students. Pretty much every aspect of a student’s life has been stripped away from them; school, sports, clubs, friends and much more. During this time of unknowing there are so many factors that children have to worry about. These stresses, in turn, will affect their ability to retain information and learn.
Not only do children have to worry about school work and how to teach themselves. They also have many other anxieties during this time as well. Students worry about the Coronavirus and whether they, their friends, families will get this new illness. Sickness can play a huge role in a child’s ability to learn because there is a constant fear and the virus is always being talked about. COVID-19 is also new and everything about it seems uncertain. Sickness is not the only things children have to worry about when it comes to the pandemic, but it is a major factor that can affect their mental status and ability to learn.
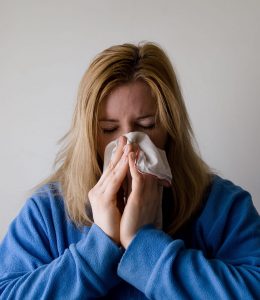
Financial problems play a huge role in a student’s life as well. So many people have lost their jobs during this time due to sickness, quarantine, and economic decline. If their family is greatly struggling students may have to put their education on hold and work in order to help the family. Financial problems can also change a student’s entire way of life, which can be a huge adjustment and make school work seem less important.
Social isolation and being away from peers greatly affects a child’s mental health, which respectively, will affect their ability to learn and retain knowledge. Humans are social creatures meant to be around others, but when the pandemic hit students were separated from their peers for months on end. This increases the chance of mental illnesses in students as well as other problems with their ability to interact socially. Chronic stress changes the chemical and physical structure of the brain, impairing cognitive skills like attention, concentration, memory, and creativity ( Terada ).
Schools account for a majority of mental health services for children so a lot of damage will come from closures. Most children come to their schools for help with mental health and safety issues. Taking away this resource or making it less available could greatly impede a student’s ability to learn and work through personal problems. School is also a safe place for many students, where they can escape problems at home. Now that this resource will not be readily available for them, stress could be overwhelming for many students and their education is almost guaranteed to suffer. A lot of mental illnesses start in childhood and are identified in the school environment. Many of these issues students face will now go unnoticed, possibly for many years.
How Hybrid Learning Might be Beneficial
Hybrid and online learning might be new in a usually traditional schooling method, but these systems have been around for a while and might prove to be beneficial for students. Some believe that these new methods of teaching will allow students to gain new knowledge and skills, such as computer self-efficacy, self-regulation and even boost engagement ( Sun & Rueda ). Some teachers are trying harder to boost class engagement and students can do work at their own pace. Studies have also found that online or hybrid learning methods can have very similar outcomes compared to face-to-face learning when students are engaged and actively being taught ( Kauffman ). Some believe that hybrid learning methods allow students to work around other schedules and be able to do a little work here and there, giving them more time to do what they love. This side of the argument believes that when students have free-reign on how they learn, they learn better.
These teaching methods could be beneficial for students who were prepared for this type of learning, but not all students have the drive and motivation needed to complete all of their work on their own and in their own time. Hybrid learning in this situation could be more beneficial than online or no schooling methods, but many unique challenges have arisen during the pandemic ( Raes ). Studies conducted before the pandemic can not be accurately compared to a student’s educational life now due to so many added factors of stress and unpreparedness. The COVID-19 pandemic brings forth new challenges not discussed in older studies and it is difficult to compare the two at this time.
Every student is different. So are their lives at home. Therefore, the outcome that the COVID-19 pandemic will have on them and their education cannot be set in stone quite yet. However, we can expect major delays in their education just because they were thrown into a situation that no one was prepared for. Schools and students were in many ways unprepared for challenges caused by COVID-19 and new teaching methods. Some issues students face now can affect them and the country for the rest of their lives because education is extremely important in our modern society. All that we can do for now is to support students and their needs for technology, education and assistance, not only in the school place, but in their personal lives as well. If we don’t fix what has been lost, many generations to come could be affected.
Bushweller, Kevin. “How COVID-19 Is Shaping Tech Use. What That Means When Schools Reopen.” Education Week , 2 Jun. 2020, www.edweek.org/ew/articles/2020/06/03/how-covid-19-is-shaping-tech-use-what.html .
Dorn, Emma, et al. COVID-19 and Student Learning in the United States: The Hurt Could Last a Lifetime. Global Editorial Services. McKinsey & Company, 2020, https://fresnostate.edu/kremen/about/centers-projects/weltycenter/documents/COVID-19-and-student-learning-in-the-United-States-FINAL.pdf .
Fox, Michelle. “Coronavirus has suspended school plans. It will also worsen racial and economic inequalities, experts warn.” CNBC, 12 Aug. 2020, https://www.cnbc.com/2020/08/12/impact-of-covid-19-on-schools-will-worsen-racial-inequity-experts-say.html .
García, Emma, and Elaine Weiss. “COVID-19 and student performance, equity, and U.S. education policy.” Economic Policy Institute, 10 Sep. 2020, https://www.epi.org/publication/the-consequences-of-the-covid-19-pandemic-for-education-performance-and-equity-in-the-united-states-what-can-we-learn-from-pre-pandemic-research-to-inform-relief-recovery-and-rebuilding/ .
Kauffman, Heather. “A Review of Predictive Factors of Student Success in and Satisfaction with Online Learning.” Research in Learning Technology , vol. 23, 2015, pp. 1-13, https://doi.org/10.3402/rlt.v23.26507 .
Raes, Annelies, et al. “Learning and Instruction in the Hybrid Virtual Classroom: An Investigation of Students’ Engagement and the Effect of Quizzes.” Computers and Education , vol. 143, 2020, pp. 1-16, https://doi.org/10.1016/j.compedu.2019.103682 .
Sun, Jerry C., and Robert Rueda. “Situational Interest, Computer self‐efficacy and self‐regulation: Their Impact on Student Engagement in Distance Education.” British Journal of Educational Technology , vol. 43, no. 2, 2012, pp. 191-204, https://doi.org/10.1111/j.1467-8535.2010.01157.x .
Terada, Youki. “Covid-19’s Impact on Students’ Academic and Mental Well-Being.” Edutopia , 24 Jun. 2020, www.edutopia.org/article/covid-19s-impact-students-academic-and-mental-well-being .
“woman, covering, nose, white, towel, blow, blowing, hand chief, grey, blond” by Pxfuel is in the Public Domain, CC0
“Mask, student, corona, student, covid-19, class, virus” by Yogen Dras is in the Public Domain, CC0
COVID-19: Success Within Devastation Copyright © 2020 by Susan Taylor is licensed under a Creative Commons Attribution-NonCommercial-ShareAlike 4.0 International License , except where otherwise noted.
Share This Book
COVID-19 and student learning in the United States: The hurt could last a lifetime
The US education system was not built to deal with extended shutdowns like those imposed by the COVID-19 pandemic. Teachers, administrators, and parents have worked hard to keep learning alive; nevertheless, these efforts are not likely to provide the quality of education that’s delivered in the classroom.
Even more troubling is the context: the persistent achievement disparities across income levels and between white students and students of black and Hispanic heritage. School shutdowns could not only cause disproportionate learning losses for these students—compounding existing gaps—but also lead more of them to drop out. This could have long-term effects on these children’s long-term economic well-being and on the US economy as a whole.
Despite the enormous attention devoted to the achievement gap, it has remained a stubborn feature of the US education system. In 2009, we estimated that the gap between white students and black and Hispanic ones deprived the US economy of $310 billion to $525 billion a year in productivity, equivalent to 2 to 4 percent of GDP. The achievement gap between high- and low-income students was even larger, at $400 billion to $670 billion, 3 to 5 percent of GDP. 1 For both 2009 and 2019, we use $25,000 in annual income (in 2009 constant dollars) as the cutoff between low and high income. Although we calculate these two gaps separately, we recognize that black and Hispanic students are also more likely to live in poverty. Yet poverty alone cannot account for the gaps in educational performance . Together, they were the equivalent of a permanent economic recession.
Unfortunately, the past decade has seen little progress in narrowing these disparities. The average black or Hispanic student remains roughly two years behind the average white one, and low-income students continue to be underrepresented among top performers. 2 Erik Hanushek, Paul E. Peterson, Laura M. Talpey, and Ludger Woessmann. Long-run Trends in the U.S. SES-Achievement Gap, NBER Working Paper No. 26764, National Bureau of Economic Research, February 2020; S. F. Reardon, “The widening academic achievement gap between the rich and the poor: New evidence and possible explanations,” in Greg Duncan and Richard Murnane (Eds.), Whither Opportunity? Rising Inequality and the Uncertain Life Chances of Low-Income Children, New York: Russell Sage Foundation, 2011.
We estimate that if the black and Hispanic student-achievement gap had been closed in 2009, today’s US GDP would have been $426 billion to $705 billion higher. 3 The learning gap has remained almost the same between 2007 (the year of the latest data when we published our 2009 report) and 2019. Black students scored, on average, 31 points lower than white students did on eighth-grade National Assessment of Education Progress (NAEP) math assessments in 2007; in 2019 they scored 32 points lower. Hispanic students scored, on average, 26 points lower than white students did on eighth-grade NAEP math assessments in 2007; in 2019 they scored 24 points lower. The increase in dollar values is the result of an increase in proportion of black and Hispanic people in the workforce and higher GDP base value in 2019. If the income-achievement gap had been closed, we estimate that US GDP would have been $332 billion to $550 billion higher (Exhibit 1).
These estimates were made before schools closed and the transition to remote learning began, sometimes chaotically. In this article, we explore the possible long-term damage of COVID-19–related school closures on low-income, black, and Hispanic Americans, and on the US economy.
Learning loss and school closures
To that end, we created statistical models to estimate the potential impact of school closures on learning. The models were based on academic studies of the effectiveness of remote learning relative to traditional classroom instruction for three different kinds of students. We then evaluated this information in the context of three different epidemiological scenarios .
How much learning students lose during school closures varies significantly by access to remote learning, the quality of remote instruction, home support, and the degree of engagement. For simplicity’s sake, we have grouped high-school students into three archetypes. First, there are students who experience average-quality remote learning; this group continues to progress, but at a slower pace than if they had remained in school. 4 High-quality remote-learning programs are typically the result careful planning and deliberate approaches—which were not typical of the COVID-19 transition. Second, some students are getting lower-quality remote learning; they are generally stagnating at their current grade levels. Then there are students who are not getting any instruction at all; they are probably losing significant ground. Finally, some students drop out of high school altogether.
We also modeled three epidemiological scenarios. In the first—“virus contained”—in-class instruction resumes in fall 2020. In the second—“virus resurgence”— school closures and part-time schedules continue intermittently through the 2020–21 school year, and in-school instruction does not fully resume before January 2021. 5 For simplicity’s sake, we have equated this with schools restarting as normal in January 2021, even though the reality is more likely to be a patchwork of different actions. In the third scenario—“pandemic escalation”—the virus is not controlled until vaccines are available, and schools operate remotely for the entire 2020–21 school year.
In our second scenario (in-class instruction does not resume until January 2021), we estimate that students who remain enrolled could lose three to four months of learning if they receive average remote instruction, seven to 11 months with lower-quality remote instruction, and 12 to 14 months if they do not receive any instruction at all (Exhibit 2).

Although students at the best full-time virtual schools can do as well as or better than those at traditional ones, 6 There is evidence from online-learning providers’ internal, peer-reviewed research that some virtual-learning experiences can achieve parity with brick-and-mortar experiences for students, especially those who were struggling academically. most studies have found that full-time online learning does not deliver the academic results of in-class instruction. 7 See, for example the 2015 Online Charter School Study of the Center for Research on Education Outcomes (CREDO), credo.stanford.edu. Moreover, in 28 states, 8 Alaska, Arkansas, Colorado, Connecticut, Georgia, Hawaii, Illinois, Iowa, Kansas, Kentucky, Louisiana, Maine, Maryland, Massachusetts, Missouri, Montana, New Jersey, New York, North Carolina, Ohio, Rhode Island, South Carolina, South Dakota, Tennessee, Utah, Virginia, West Virginia, and Wisconsin. with around 48 percent of K–12 students, distance learning has not been mandated. 9 Politics K–12, “Coronavirus and learnings: What’s happening in each state,” blog entry by Education Week staff, April 3, 2020, blogs.edweek.org. As a result, many students may not receive any instruction until schools reopen. Even in places where distance learning is compulsory, significant numbers of students appear to be unaccounted for. 10 The Curriculum Associates analysis of anonymized data on usage from March to May 2020 of i-Ready software (a personalized learning system typically used as supplemental instruction by classroom teachers), curriculumassociates.com. In short, the hastily assembled online education currently available is likely to be both less effective, in general, than traditional schooling and to reach fewer students as well.
Likely effects on low-income, black, and Hispanic students
Learning loss will probably be greatest among low-income, black, and Hispanic students. Lower-income students are less likely to have access to high-quality remote learning or to a conducive learning environment, such as a quiet space with minimal distractions, devices they do not need to share, high-speed internet, and parental academic supervision. 11 Many parents continue to work full-time outside their homes, so their children may not have an adult at home to supervise their learning; Brooke Auxier and Monica Anderson, “As schools close due to the coronavirus, some U.S. students face a digital ‘homework gap,’” Fact Tank, March 16, 2020, pewreasearch.org. Many white-collar workers, however, are able to work remotely and thus provide at least some supervision. Dana Goldstein, Adam Popescu, and Nikole Hannah-Jones, ”As school moves online, many students stay logged out, New York Times, April 6, 2020, nytimes.com. Also, one in ten public school students in New York City lives in shelter housing, which can mean several children sharing a single room; Anna North, “The shift to online learning could worsen educational inequality,” Vox, April 9, 2020, vox.com. Data from Curriculum Associates, creators of the i-Ready digital-instruction and -assessment software, suggest that only 60 percent of low-income students are regularly logging into online instruction; 90 percent of high-income students do. Engagement rates are also lagging behind in schools serving predominantly black and Hispanic students; just 60 to 70 percent are logging in regularly (Exhibit 3). 12 The Curriculum Associates analysis of anonymized data on usage from March to May 2020 of i-Ready software (a personalized learning system typically used as supplemental instruction by classroom teachers), percentage of log-ins as a portion of pre-closure rates on a weekly basis, curriculumassociates.com.
These variations translate directly into greater learning loss. 13 To gauge the proportion of students that may fall into our three learning archetypes by race or ethnicity and by income level, we integrated multiple sources of information, including national surveys of teachers and data on student log-in patterns by race or ethnicity and income estimates to generate the plausibility of the type of instruction that students may receive given the income quintiles of their families. Specifically, “No instruction” estimates based on Curriculum Associates data and press reporting, including Mark Lieberman, “Taking attendance during Coronavirus closures: Is it even worth it?”, Education Week, May 27, 2020, edweek.org; and Howard Blume and Sonali Kohli, “15,000 LA high-school students are AWOL online, 40,000 fail to check in daily amid coronavirus closures,” Los Angeles Times, March 30, 2020, latimes.com. High- and low-quality instruction estimates are based on US Census income quintiles (Income Data Tables, US Census Bureau, 2019, census.gov), with the assumption that top two income quintiles receive higher-quality instruction. The average loss in our middle epidemiological scenario is seven months. But black students may fall behind by 10.3 months, Hispanic students by 9.2 months, and low-income students by more than a year. We estimate that this would exacerbate existing achievement gaps by 15 to 20 percent.
In addition to learning loss, COVID-19 closures will probably increase high-school drop-out rates (currently 6.5 percent for Hispanic, 5.5 percent for black, and 3.9 percent for white students, respectively). The virus is disrupting many of the supports that can help vulnerable kids stay in school: academic engagement and achievement, strong relationships with caring adults, and supportive home environments. In normal circumstances, students who miss more than ten days of school are 36 percent more likely to drop out. 14 Research brief: Chronic absenteeism, Utah Education Policy Center, University of Utah, 2012, uepc.utah.edu. In the wake of school closures following natural disasters, such as Hurricane Katrina (2005) and Hurricane Maria (2017), 14 to 20 percent of students never returned to school. 15 “Declining Enrollment, Shuttered Schools,” Education Week, September 19, 2018, edweek.org; “Legacy of Katrina: The Impact of a Flawed Recovery on Vulnerable Children of the Gulf Coast,” National Center for Disaster Preparedness, Children’s Health Fund, 2010. We estimate that an additional 2 to 9 percent of high-school students could drop out as a result of the coronavirus and associated school closures—232,000 ninth-to-11th graders (in the mildest scenario) to 1.1 million (in the worst one). 16 To create these estimates, we compared data on the effects on drop-out rates resulting from extended school absences, online-only instruction (which can disrupt engagement and student–teacher relationships), and natural disasters. We focus on grades 9 to 11, as many school districts have relaxed testing and other graduation requirements for current 12th-grade students.
In addition to the negative effects of learning loss and drop-out rates, other, harder to quantify factors could exacerbate the situation: for example, the crisis is likely to cause social and emotional disruption by increasing social isolation and creating anxiety over the possibility that parents may lose jobs and loved ones could fall ill. Milestones such as graduation ceremonies have been canceled, along with sports and other extracurricular events. These challenges can reduce academic motivation and hurt academic performance and general levels of engagement. 17 Leah Lessard and Hannah Schacter, “Why the coronavirus crisis hits teenagers particularly hard: Developmental scientists explain,” Education Week, April 15, 2020, edweek.org.
The loss of learning may also extend beyond the pandemic. Given the economic damage, state budgets are already stressed. Cuts to K–12 education are likely to hit low-income and racial- and ethnic-minority students disproportionately, and that could further widen the achievement gap. 18 During the 2008 recession, annual academic gains in US counties that suffered the largest shocks to employment fell 25 percent from prerecession levels. These districts disproportionately served poor and black Americans. K. Shores, K and M. P. Steinberg, Schooling During the Great Recession: Patterns of School Spending and Student Achievement Using Population Data, 2019.
The economic impact of learning loss and dropping out
These effects—learning loss and higher dropout rates—are not likely to be temporary shocks easily erased in the next academic year. On the contrary, we believe that they may translate into long-term harm for individuals and society.
Using the middle (virus resurgence) epidemiological scenario, in which large-scale in-class instruction does not resume until January 2021, we estimated the economic impact of the learning disruption. (The results would, of course, be worse in the third scenario and better in the first.) All told, we estimate that the average K–12 student in the United States could lose $61,000 to $82,000 in lifetime earnings (in constant 2020 dollars), or the equivalent of a year of full-time work, solely as a result of COVID-19–related learning losses. These costs are significant—and worse for black and Hispanic Americans. While we estimate that white students would earn $1,348 a year less (a 1.6 percent reduction) over a 40-year working life, the figure is $2,186 a year (a 3.3 percent reduction) for black students and $1,809 (3.0 percent) for Hispanic ones.
This translates into an estimated impact of $110 billion annual earnings across the entire current K–12 cohort 19 Using projected learning loss onto the National Assessment of Education Progress and its relationship with the country’s GDP and earnings. In addition, in all calculations below, we have accounted for the effects of an economic recession on academic outcomes. (Exhibit 4). Of that sum, $98.8 billion would be associated with loss of learning and the rest ($11.2 billion) with the increase in the number of high-school dropouts. This is not just an economic issue. Multiple studies have linked greater educational attainment to improved health, reduced crime and incarceration levels, and increased political participation.
The damage to individuals is consequential, but the consequences could go deeper: the United States as a whole could suffer measurable harm. With lower levels of learning and higher numbers of drop-outs, students affected by COVID-19 will probably be less skilled and therefore less productive than students from generations that did not experience a similar gap in learning. 20 Similar effects have been noted for other generations that experienced major learning disruptions. For example, several studies have shown long-term earnings implications for students whose learning was disrupted during World War II. Furthermore, if other countries mitigate the impact of lost learning and the United States does not, this will harm US competitiveness. By 2040, most of the current K–12 cohort will be in the workforce. We estimate a GDP loss of $173 billion to $271 billion a year—a 0.8 to 1.3 percent hit (Exhibit 5). 21 Using Hanushek and Woessman 2008 methodology to map national per capita growth associated with decrease in academic achievement, then adding additional impact of COVID drop-outs on GDP.
A call to action
These numbers are sobering—but they are not inevitable. If the United States acts quickly and effectively, it may avoid the worst possible outcomes. But if there is a delay or a lack of commitment, COVID-19 could end up worsening existing inequities.
It is therefore urgent to intervene immediately to support vulnerable students. Many students will continue to take advantage of free learning resources, but school systems must also think creatively about how to encourage ongoing learning over the summer. Initiatives might include expanding existing summer-school programs, working with agencies that run summer camps and youth programs so that they add academics to their activities, and enlisting corporations to identify and train volunteer tutors. Tennessee, for example, is recruiting 1,000 college students to tutor kids falling behind. New York will be conducting remote summer school for 177,700 students (compared with 44,000 in 2019). Some districts are making digital summer learning available (though optional) to all students.
The necessity of continued remote learning cannot be an excuse for inaction or indifference. There are examples of high-quality online education, and reaching this level should be the general expectation. While no one knows exactly what level of in-class learning will be possible for the 2020–21 school year, many students will probably need to stay home for at least part of it. Educators need to use the summer to learn how to make instruction more effective, whatever the scenario.
Achieving this goal will make it necessary to provide teachers with resources that show them how they can make virtual engagement and instruction effective and to train them in remote-learning best practices. It will also be necessary to work with parents to help create a good learning environment at home, to call upon social and mental-health services so that students can cope with the pandemic’s stresses, and to ensure that all students have the infrastructure (such as laptops, tablets, and good broadband) needed for remote learning.
As a blend of remote and in-classroom learning becomes possible, more flexible staffing models will be required, along with a clear understanding of which activities to prioritize for in-classroom instruction, identification of the students who most need it, and the flexibility to switch between different teaching methods. And all this must be done while school systems keep the most vulnerable students top of mind. That may require investment—something that cannot be taken for granted if state and local government budgets are cut.
The US academic-achievement gap was first identified in 1966. Its persistence is troubling. The possibility that COVID-19 could make it worse deserves focused attention. The achievement gap costs the United States hundreds of billions of dollars—and also exacts a long-term cost in social cohesion. This is a moment—and a challenge—that calls for urgency and energy.
Emma Dorn is the global Education Practice manager in McKinsey’s Silicon Valley office. Bryan Hancock and Jimmy Sarakatsannis are partners in the Washington, DC, office, and Ellen Viruleg is a senior adviser based in Providence, Rhode Island.
The authors wish to thank Priyanka Agrawal, Justice Tention-Palmer, and Josh Williams for their contributions to this article.
This article was edited by Cait Murphy, a senior editor in the New York office.
Explore a career with us
Related articles.

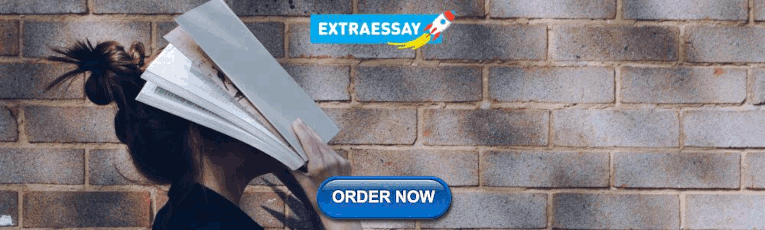
Coronavirus: How should US higher education plan for an uncertain future?
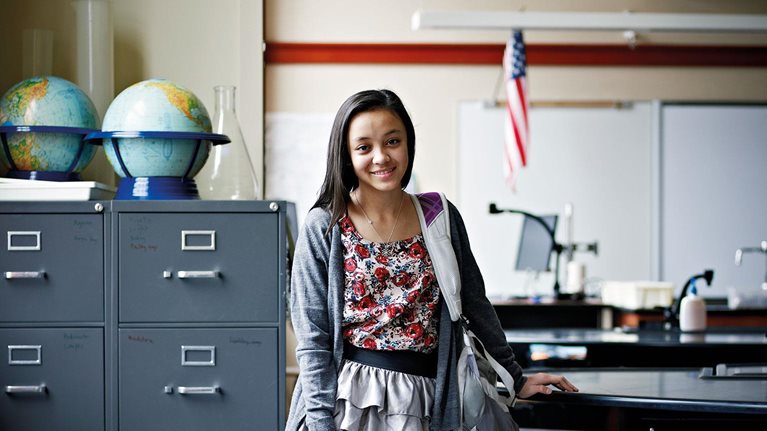
Drivers of student performance: Insights from North America

A transformative moment for philanthropy
Thank you for visiting nature.com. You are using a browser version with limited support for CSS. To obtain the best experience, we recommend you use a more up to date browser (or turn off compatibility mode in Internet Explorer). In the meantime, to ensure continued support, we are displaying the site without styles and JavaScript.
- View all journals
- My Account Login
- Explore content
- About the journal
- Publish with us
- Sign up for alerts
- Open access
- Published: 25 March 2023
The impact of the first wave of COVID-19 on students’ attainment, analysed by IRT modelling method
- Rita Takács ORCID: orcid.org/0000-0002-0314-4179 1 ,
- Szabolcs Takács ORCID: orcid.org/0000-0002-9128-9019 2 , 3 ,
- Judit T. Kárász ORCID: orcid.org/0000-0002-6198-482X 4 , 5 ,
- Attila Oláh 6 , 7 &
- Zoltán Horváth 1
Humanities and Social Sciences Communications volume 10 , Article number: 127 ( 2023 ) Cite this article
3016 Accesses
2 Citations
1 Altmetric
Metrics details
Universities around the world were closed for several months to slow down the spread of the COVID-19 pandemic. During this crisis, a tremendous amount of effort was made to use online education to support the teaching and learning process. The COVID-19 pandemic gave us a profound insight into how online education can radically affect students and how students adapt to new challenges. The question is how switching to online education affected dropout? This study shows the results of a research project clarifying the impact of the transition to online courses on dropouts. The data analysed are from a large public university in Europe where online education was introduced in March 2020. This study compares the academic progress of students newly enroled in 2018 and 2019 using IRT modelling. The results show that (1) this period did not contribute significantly to the increase in dropout, and we managed to retain our students.(2) Subjects became more achievable during online education, and students with less ability were also able to pass their exams. (3) Students who participated in online education reported lower average grade points than those who participated in on-campus education. Consequently, on-campus students could win better scholarships because of better grades than students who participated in online education. Analysing students’ results could help (1) resolve management issues regarding scholarship problems and (2) administrators develop programmes to increase retention in online education.
Similar content being viewed by others
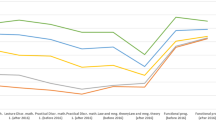
Applying the Rasch model to analyze the effectiveness of education reform in order to decrease computer science students’ dropout
Rita Takács, Judit T. Kárász, … Attila Oláh
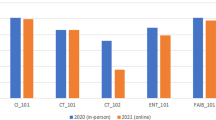
Online vs in-person learning in higher education: effects on student achievement and recommendations for leadership
Bandar N. Alarifi & Steve Song
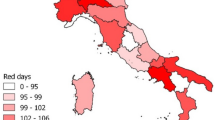
Unraveling the controversial effect of Covid-19 on college students’ performance
Luca Bonacini, Giovanni Gallo & Fabrizio Patriarca
Introduction
During the spread of the COVID-19 pandemic, several countries closed their university buildings and switched to online education. Some opinions suggest that online education had a negative effect on dropouts because of several factors, e.g., lack of social connections, poor contact with teachers. In bachelor’s programmes—like university courses in computer science—where dropout rates were high prior to the pandemic, many questions were raised about the impact of the transition to online education.
This study focuses on the effects of the first wave of the COVID-19 pandemic on students’ dropouts and performance in Hungary. Although the manuscript addresses academic dropout, other issues such as inequality or accessibility were also covered in the research.
Theoretical background
Educational theory about student dropout in higher education.
Tinto ( 1975 ) was the first researcher who analysed the dropout phenomenon and invented the interactional theory of student persistence in higher education. He ( 2012 ) highlighted the interactions between the student and the institution regarding how well they fit in academically and socially. Interactional theories suggest that students’ personal characteristics, traits, experience, and commitment can have an effect on students’ persistence (Pascarella and Terenzini, 1983 ; Terenzini and Reason, 2005 ; Reason, 2009 ). Braxton and Hirschy ( 2004 ) also emphasized the need for community on campus as a help of social integration to develop relationships between peers because interactions with other students and faculty members crucially determine whether students persist and continue their studies or leave.
The student dropout rate has been a crucial issue in higher education in the last two decades. Attrition has serious consequences on the individual (e.g., Nagrecha et al., 2017 ) at both economic (Di Pietro, 2006 ; Belloc et al., 2011 ) and educational (Cabrera et al., 2006 ) levels. As a worldwide phenomenon, it draws the attention of policy-makers, stake-holders and academics to the necessity of seeking solutions. The dropout crisis requires complex intervention programmes for encouraging students in order to complete their studies. Addressing such a dropout crisis requires an actionable interdisciplinary movement based on partnerships among stake-holders and academics.
According to Vision 2030 studies published by the European Union, education is vital for economic development because it has a direct influence on entrepreneurship and productivity growth; at the same time, it increases employment opportunities and women empowerment. Education helps to reduce unemployment and enhance students’ abilities and skills that will be needed in the labour market. Due to students’ high attrition, the economy also suffers because experts with a degree usually contribute more to the GDP than people without (Whittle and Rampton, 2020 ).
A comparative analysis of past studies has been conducted in order to identify various causes of students’ dropout. Students’ performance after the first academic year is a topic of significant interest: the lack of students' engagement in academic life and their unpreparedness are mainly responsible for dropout after the first highly crucial period. However, further studies are necessary to better understand this phenomenon.
The characteristics of online education and its effect on dropout
Online education had already existed before the COVID-19 pandemic and had had a vast literature because online courses had been playing an important role in higher education. Online education has its own benefits, e.g., it enables students to work from the comfort of their homes with more convenient, accessible materials. In recent years, numerous investigations have been performed on how to increase the motivation of students by making them feel engaged during the learning processes (Molins-Ruano et al., 2014 ; Jovanovic et al., 2019 ). The other benefit is “humanizing”, which is an academic strategy that looks for solutions to improve equity gaps by recognizing the fact that learning situations are not the same for everyone. The aim of humanizing education is to remove the affective and cognitive barriers which appear during online learning and to provide a technique in higher education towards a more equitable future in which the success of all students is supported (Pacansky-Brock and Vincent-Layton, 2020 ). Humanizing online STEM courses has specific significance because creating such academic pathways can especially help the graduation of vulnerable, for example, non-traditional students. The definition of a non-traditional student belongs to Bean and Metzner ( 1985 ), who distinguished students by different characteristics. Non-traditional students are not on-campus students (but they can participate in online education), who are usually aged 24 years or older, and dominantly have a job and/or a family. Non-traditional students have less interaction with other participants in education, and they are much more influenced by other factors, e.g., family or other external responsibilities. Financial factors, family attitudes and external incentives can also influence dropout. The dropout model for non-traditional university students highlights that underperforming students are likely to leave the institution. Carr ( 2000 ) (in Rovai, 2003 ) noticed that persistence in online courses is regularly 10–20% lower than in on-campus courses. The dropout rate differs from institution to institution: some reports claim that 80% of students graduated, whereas other findings show that less than 50% of students completed their courses. Humanizing recognizes that engagement and accomplishment are the key factors in students’ success. Engagement and achievement are social constructs created through students’ experience. Teachers can help students to socialize and adapt to the academic environment by using humanizing practices like a liquid syllabus. Stommel ( 2013 ) also considers that hybrid pedagogy is a useful tool in order to support students’ learning because it helps teachers to implement new learning activities and facilitate collaboration among students.
Despite the various benefits that online education has, the success of students depends on the student’s capacity to independently and effectively engage in the learning process (Wang et al., 2013 ). Online learners are required to be more autonomous, as the exceptional nature of online settings relies on self-directed learning (Serdyukov and Hill, 2013 ). It is therefore especially critical that online learners, compared to their conventional classroom peers, have the self-generated capacity to control and manage their learning activities.
Online education also needs extra attention because the dropout rate is high in online university programmes. Students in online courses are more likely to drop out (Patterson and McFadden, 2009 ; in Nistor and Neubauer, 2010 ). Numerous studies reported much higher dropout rates than in the case of on-campus courses (Willging and Johnson, 2019 ; Levy, 2007 ; Morris et al., 2005 ; Patterson and McFadden, 2009 ; in Nistor and Neubauer, 2010 ). Many factors that lead to dropout were examined in the past. During online courses, students are less likely to form communities or study groups and the lack of learning support can lead to isolation. Consequently, demotivated students who were dedicated to their chosen major, in the beginning, may decide to drop out. Fortunately, there are different ways to support students who study in an online setting depending on their various psychological attributes. These psychological attributes that are connected to dropout have already been examined. One of the most noticeable hypothetical models of university persistence in online education was proposed by Rovai ( 2003 ). He claims that dropout depends on students’ characteristics e.g., learning style, socioeconomic status, studying skills, etc. Besides these factors, the method of education also has an impact on students’ decisions on whether they complete the course or drop out.
It is vital to distinguish the online education that was introduced as a consequence of the COVID-19 lockdown, when universities were forced to move their education to fully online platforms because online education had already existed in some educational institutions.
The COVID-19 pandemic and its effect on education: Inequalities in home learning and colleges’ provision of distance teaching during school closure of the COVID-19 lockdown
The lives of millions of college students were affected not only by the health and economic implications of the COVID-19 pandemic but also by the closure of educational institutions. Home and academic environments were interlaced, and most institutions were caught unprepared. In this article, we examine the effects of the transition to online learning in areas such as academic attainment.
There are several debates on the effectiveness of moving to online education. Since currently there is little literature about the COVID-19 pandemic in relation to how it affects dropouts at universities, it is worth discussing it in order to have an overview of recent studies on students’ performance. The learning environment changed radically during the first wave of the pandemic in the spring semester of 2020. The transition to home learning and teaching in such a short time without any warning or preparation raised concerns and became the focus of attention for researchers, teachers, policymakers, and all those interested in the educational welfare of students.
A potential learning loss was anticipated, possibly affecting students’ cognitive gains in the long term (Andrew et al., 2020 ; Bayrakdar and Guveli, 2020 ; Brown et al., 2020 ); in fact, an increasing number of studies suggested that the lockdown might have far-reaching academic consequences (Bol, 2020 ). In general, results suggest that students’ motivation was substantially affected by the COVID-19 pandemic and that academic and relational changes were the most notable sources of stress on both the students’ side (e.g., Rahiem, 2021 ) and the teachers’ side (e.g., Abilleira et al., 2021 ; Daumiller et al., 2021 ). Engzell et al. ( 2021 ) examined nearly 350,000 students’ academic performance before and after the first wave of the pandemic in the Netherlands. Their results suggest that students made very little development while learning from home. Closures also had a substantial effect on students’ sense of belonging and self-efficacy. Academic knowledge loss could be even more severe in countries with less advanced infrastructure or a longer period of college closures (OECD, 2020 ).
Many researchers started to examine the effects of the COVID-19 pandemic on university students’ mental health and academic performance. Clark et al. ( 2021 ) claim that university students are increasingly considered a vulnerable population, as they experience extremely high levels of stress. They draw attention to the fact that students might suffer more from learning difficulties. Daniels et al. ( 2021 ) used a single survey to collect retrospective self-report data from Canadian undergraduate students ( n = 98) about their motivation, engagement and perceptions of success and cheating before COVID-19, which shows that students’ achievements, goals, engagement and perception of success all significantly decreased, while their perception of cheating increased (Daniels et al., 2021 ). Other studies claim that during the COVID-19 pandemic, students were more engaged in studying and had higher perceptions of success. Studies also show that teachers’ strategies changed as well because of the lack of interaction between teachers and students, which led to the fact that students experienced more stress and were more likely to have difficulties in following the material presented and it could be one of the reasons for poor academic performance. Mendoza et al. ( 2021 ) investigated the relationships between anxiety and students’ performance during the first wave of the pandemic among college students. Anxiety regarding learning mathematics was measured among mathematics students studying at the Universidad Nacional de Chimborazo (UNACH) during the autumn semester of the academic year 2020. The total sample contained 120 students, who were studying the subject of mathematics at different levels. The results showed that there were statistically significant differences in the understanding of the contents presented by the teachers in a virtual way. During the COVID-19 pandemic the levels of mathematical anxiety increased. Teaching mathematics at university in an online format requires good quality digital connection and time-limited submission of assignments. This study draws attention to the negative result of the pandemic, i.e. the levels of anxiety might be greater during online education and not only in mathematics education but also in other subjects. Thus it could have an effect on students’ academic performance. However, the results are contradictory to what Said ( 2021 ) found, i.e. there was no difference in students’ performance before and during the COVID-19 pandemic. In their empirical study, they investigated the effect of the shift from face-to-face to online distance learning at one of the universities in Egypt. They compared the grades of 376 business students who participated in a face-to-face course in spring 2019 and those of 372 students who participated in the same course fully online in spring 2020 during the lockdown. A T -test was conducted to compare the grades of quizzes, coursework, and final exams of the two groups. The results suggested that there was no statistically significant difference. Another interesting result was that in some cases students had a better performance during the COVID-19 pandemic. At a large public university in Spain, Iglesias-Pradas et al. ( 2021 ) analysed the following instruction-related variables: class size, synchronous/asynchronous delivery of classes, and the use of digital supporting technologies on students’ academic performance. The research compared the academic results of the students during the COVID-19 pandemic with those of previous years. Using quantitative data from academic records across all ( n = 43) courses of a bachelor’s degree programme, the study showed an increase in students’ academic performance during the sudden shift to online education. Gonzalez et al. ( 2020 ) had similar results. Their research group analysed the effects of COVID-19 on the autonomous learning performance of students. 458 students participated in their studies. In the control group, students started their studies in 2017 and 2018, while in the experimental group, students started in 2019. The results showed that there was a significant positive effect of the COVID-19 lockdown on students’ performance: students had changed their learning strategies and improved their efficiency by studying more continuously. Yu et al. ( 2021 ) found similar results. They used administrative data from students’ grade tracking systems and found that the causal effects of online education on students’ exam performance were positive in a Chinese middle school. Taking a difference-in-differences approach, they found that receiving online education during the COVID-19 lockdown improved students’ academic results by 0.22 of a standard deviation (Yu et al., 2021 ).
Currently, there is little literature about COVID-19 in relation to how it affects students’ performance at universities, so it is worth discussing this aspect as well.
Teachers’ approach to their grading strategies and shift to online education during the COVID-19 lockdown
There is a vast literature on the limits of the capacities and challenges of online education (Davis et al., 2019 ; Dumford and Miller, 2018 ; Palvia et al., 2018 ). The lockdown during the COVID-19 pandemic created new challenges for teachers all over the world and called for innovative teaching techniques (Adedoyin and Soykan, 2020 ; Gamage et al., 2020 ; Paudel, 2020 ; Peimani and Kamalipour, 2021 ; Rapanta et al., 2020 ; Watermeyer et al., 2021 ). These changes had undoubtedly profound impacts on the academic discourse and everyday practices of teaching. Teachers’ motivations for maintaining effective online teaching during the lockdown were diverse and complex, and therefore, learning outcomes were difficult to be guaranteed. Yu et al. ( 2021 ) examined how innovative teaching could be continued during the COVID-19 pandemic, particularly by learning domain-specific knowledge and skills. The results confirmed that during the lockdown teachers who had studied online teaching methods improved their teaching skills and ICT (information and communication technology) efficacy.
Burgess and Sievertsen ( 2020 ) claim that due to the COVID-19 lockdown, educational institutions might cause major interruptions in students’ learning process. Disruption appeared not only in elaborating new knowledge but also in assessment. Given the proof of the significance of exams and tests for learning, educators had to consider postponing rather than renounce assessments. Akar and Coskun ( 2020 ) found that innovative teaching had a slight but positive relationship with creativity. From their point of view, it was not necessarily a consequence of shifting offline teaching to online platforms. Innovative teaching and digital technology were not granted and their impact on student’s performance or teachers’ grading practices is still unclear. The present research aimed to analyse students’ attainment during the COVID-19 pandemic by using student performance data. We focused on the relationship between participation in online courses and dropout decisions, which is connected to teachers’ grading. Examining how grades changed during the lockdown could give us an interesting insight into the educational inequality caused by online education regarding the scholarship system based on student’s grades.
Research questions
We know very little about the effects of transitioning to online education on student dropout and teachers’ grading practices. Even less information is available on the relationship between COVID-19 and dropout, so it is worth a discussion due to the existing controversial and interesting studies on students’ performance. This article gives a suggestion on how the scholarship system could be changed and how we could avoid inequality caused by online education. There is a scholarship system in Hungary that provides financial support to full-time programme students, based on their academic achievement.
Another issue we discuss in this article is dropping out from university programmes, which is a crucial issue worldwide. Between 2010 and 2016 at a large public university in Europe (over 30,000 students) the overall attrition rate is 30%, with the Faculty of Informatics having the worst results (60%) but nowadays these figures are more promising (30|40%). These days at least 800,000 computer scientists may be needed in Europe (Europa.eu, 2015 ), but it seems to be a worldwide issue (Borzovs et al., 2015 ; Ohland et al., 2008 ) to retain students.
This study focuses on the effects of the first wave of the COVID-19 pandemic on students’ dropout and performance in Hungary. Although the manuscript addresses academic dropout, other issues such as inequality or accessibility are also covered in the research. The aim of the paper is therefore to investigate the following questions:
It is inconclusive whether the COVID-19 pandemic had negative effects on students’ performance, which is why we claim that
Hypothesis 1: There is a significant difference in grade point averages between students who participated in online education and those in on-campus education in the second semester of their studies.
Academic achievement (in both traditional and online learning settings) can be measured by accomplishing a specific result in an online assignment and is commonly expressed in terms of a grade point average (GPA; Lounsbury et al., 2005 ; Richardson et al., 2012 ; Wang, 2010 ). According to meta-analyses, GPA is one of the best predictors of dropout (Richardson et al., 2012 ; Broadbent and Poon, 2015 ).
Hypothesis 2: In some subjects (Basic Mathematics practice, Programming, Imperative Programming lecture + practice, Functional Programming, Object-oriented Programming practice + lecture, Algorithms and Data Structures lecture + practice, Discrete Mathematics practice and Analysis practice), it was easier to obtain a passing grade in online education.
Hypothesis 3: More of the students who participated in online education dropped out than those who received on-campus education.
Difficulty and differential analysis of subjects
In the examined higher education system, a BSc programme has six semesters and every subject is graded on a five-point scale, where 1 means fail, and grades from 2 to 5 mean pass, with 5 being the best grade. In the analysis only the final grades were counted in each subject. It is important to see that in order to achieve better grades (or obtain sufficient knowledge), a subject really needs differentiation. It is worth examining the subjects of the various courses because—although there are grades—there is some kind of expected knowledge or skill that the subject should measure. Students are expected to develop these competencies or at least reach an expected level by the end of the semester. To find out whether this kind of competency actually exists (and was developed during online education) and whether the subjects measure this kind of competency, Item Response Theory (IRT) analysis was used to examine the subjects included in the computer science BSc programme. The aim of IRT analysis modelling is to bring the difficulty of the subjects and the ability of the students to the same scale (GRM, Forero and Maydeu-Olivares, 2009 ; Rasch, 1960 ). We had already successfully applied a special IRT model in order to analyse the effects of a student retention programme. In order to prevent student dropout, in a large public university in Europe, a prevention and promotion programme was added to the bachelor’s programme and an education reform was also implemented. In most education systems students have to collect 30 credits per semester by successfully completing 8|10 subjects. We conducted an analysis using data science techniques and the most difficult subjects were identified. As a result, harder subjects were removed, and more introductory courses were built into the curriculum of the first year. A further action—as an intervention—was added to a computer science degree programme: all theoretical lectures became compulsory to attend. According to the results, the dropout level decreased by 28%. The most important benefit of the education reform was that most subjects had become accomplishable (Takács et al., 2021 ). Footnote 1
Hypothesis 1 claims that the online transition due to COVID-19 during the second semester of the 2019 academic year did not result in a change in the requirement system of the subjects. Hypothesis 2 claims that essentially the same expectations were formulated by teachers. In contrast, the way teachers evaluate students necessarily changed. A subject with a given difficulty could be passed by a student with the same ability level with a given probability. Obviously, all subjects that had been less difficult were more likely to be correctly passed than more difficult subjects. The analysis was performed using the IRT, based on the STATA15 software package.
In the study, 862 students were involved in the bachelor’s computer science programme. There were 438 (415) students who started on-campus education in 2018 and 447 students who started on-campus education in 2019, but from March 2020 they participated in online education (Table 1 ). Table 1 shows the result of Hypothesis 1: The grade point average of students who participated in online education (2.5) was lower than that of students who participated in on-campus education (3.3). Table 1 also shows that 447 students participated in online education and only 19 dropped out; 438 students started on-campus education and 50 dropped out. We can conclude that there was no significant difference between students’ dropping out who participated in online education and those who received on-campus education (Hypothesis 3). Note: We can conclude that the grade point average of students who participated in online education (2.5) was lower than that of students who participated in on-campus education (3.3) (Hypothesis 1). On the other hand, there was no significant difference between the drop-out rate of students’ who participated in online education and that of those who received on-campus education (Hypothesis 3). These case numbers make it unnecessary to apply any statistical evidence because the result is obvious.
The subjects were examined by fitting a 2-parameter IRT model to them (scale 1–5 with grades, assuming an ordinal model using the STATA15 programme). ‘Grades’ mean the final grade of the subjects. The STATA15.0 software package was used for the analysis, and the Graded Response Model version of the Ordered item models was chosen from the IRT procedures (GRM; Forero and Maydeu-Olivares, 2009 ).
During the procedure, we examined two parameters: the difficulty of the items and the slope. We took into account those subjects for which the subject matter of the subject remained the same over the years, or the exams did not change substantially (exam grade, according to the same assessment criteria). However, it is important to note that obviously, not the same students completed the assignments each year.
The study involved the following subjects (only professional subjects were considered):
Mathematical Foundations
Programming
Computer Systems lecture+practice
Imperative Programming
Functional Programming
Object-oriented Programming lecture + practice
Algorithms and Data Structures I. lecture
Algorithms and Data Structures I. practice
Discrete Mathematics I. lecture
Discrete Mathematics I. practice
Analysis I. L
Analysis I. P
Examination of slope and difficulty coefficients
In this section, we examine Table 2 . As a first step, it is crucial to understand the slope indices of the given objects in different years, whether they change from one year to another. Table 2 shows the result of Hypothesis 2: In most subjects (Basic Mathematics practice, Programming, Imperative Programming lecture + practice, Functional Programming, Object-oriented Programming practice+lecture, Algorithms and Data Structures lecture + practice, Discrete Mathematics practice, and Analysis practice), it was easier to obtain a passing grade in online education.
Two parametric procedures were applied: each subject has a difficulty index and a slope.
While if the student’s ability falls short of the difficulty, the denominator of the fraction will increase, so the probability that the student will be able to pass the exam will increase—they will earn a good grade (Fig. 1 ).
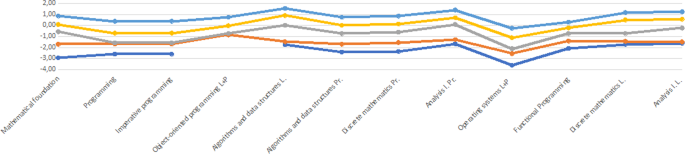
Difficulty levels of the subjects in 2018 and 2019 academic year.
Instead of introducing the whole subject network, we introduce a typical subject that was analysed using the IRT. The analyses of the subject of Discrete Mathematics enable us to adequately illustrate the classic phenomenon that arose. The complete analysis of the subjects can be found in Table 2 .
The period before 2019 and after 2019 are shown separately in the table, as at the beginning of 2020 the lockdown took place when online education was introduced to all students so it had an impact on academic achievement. We presupposed that it had manifested itself in the subjects’ completing difficulty and in their ability to differentiate.
Discrete mathematics I. practice
As far as the Discrete Mathematics subject is regarded, we can observe a slope of high value above 3 (sometimes 4) before and after 2019, which means that the subject had strong differentiating abilities both before and after the COVID-19 pandemic.
There is a debate in the literature on how the performance of students changed during online education. Whereas Said ( 2021 ) found no difference in students’ performance before and during the COVID-19 pandemic, the study by Iglesias-Pradas et al. ( 2021 ) showed an increase in students’ academic performance in distance education. Gonzalez et al. ( 2020 ) predicted better results during online education than in the case of on-campus education. This study partly confirmed their result because more students tried taking the exams. However, they could not perform better as predicted by Gonzalez et al. ( 2020 ) because among computer science students those who participated in online education obtained lower grade point averages than those who participated in on-campus education. According to our results, grade point averages differed substantially between the two examined groups (Hypothesis 1). It can be seen that there are no significant differences in the study groups in terms of dropout after the first year of studies, and the number of students affected was not substantially higher/lower. There are no significant differences in dropout rates between students participating in on-campus or online education (Hypothesis 3).
The result above is crucial; however, the implications and prospective steps based on this result are even more important.
It can be seen that with the introduction of online education, more teaching and learning strategies became available for certain subjects. Teachers’ grading strategies as well as their intentions when giving grades can be assumed as the possible reasons behind the grades. These strategies on both sides (teachers’ and students’) may have appeared during online education.
There were basically two types of changes regarding the grades for the different subjects:
The difficulty associated with the particular grade of the subject in online education decreased for each value on a scale of 1–5 for a given subject (Hypothesis 2). This means that even failing (grade 1) was easier (students preferred to try the exam even if they were unprepared), or even obtaining other passing grades was easier, too. It should be noted that the examined phenomenon cannot have a negative slope (typically not 0), because a slope of 0 means that there is ½ of a probability (regardless of ability) that a student passes a given exam. Fortunately, this is not the case, so we can assume that all slopes are positive.
(a) Behind this strategy, in the case of grade 1, it can be assumed that in online education students’ general strategy was to register for the exam and try it even if unprepared in contrast to the on-campus student who would not take the exam if s/he was unprepared.
(b) It seems that it became easier to obtain a passing grade. Behind this phenomenon, strategies can be assumed from both faculty members' and students’ sides. In case of failing the exam, it makes no sense to talk about the strategy of the teacher, because the teacher was more likely to give a passing grade or even a better grade for less knowledge. In general, the thresholds for obtaining the grade were lower in all cases. This could have been illustrated by the following subjects: Basic Mathematics practice, Programming, Imperative Programming lecture + practice, Functional Programming, Object-oriented Programming practice + lecture, Algorithms and Data Structures lecture + practice, Discrete Mathematics practice and Analysis practice.
Analysing further the subjects by IRT modelling, we saw that it was easier to obtain lower grades (grades 1, 2 and 3). However, in the case grade 4 or 5, it appears that it was more difficult to obtain them due to the prevalence of the higher requirements of the subjects.
(a) The insufficient grades’ (i.e. grade 1) lower level of difficulty (shown by the IRT model) clearly showed that there was no substantial difference in this respect compared to obtaining insufficient grades during the on-campus or online education period.
(b) The results showed that obtaining good grades (4 or 5) became more difficult during online education. It can be assumed that students participating in online education require some kind of help from education management in order to compensate for the disadvantages posed by distance learning because they got worse grades and worse average grade points as compared to on-campus students.
In the following, we examine what strategies faculty members and students may apply considering the difficulty of each grade of the subjects (left column of Table 2 ) showed a decreasing trend.
From the students’ point of view, isolation could result in students being involved in studying more effectively. Consequently, the time spent on the elaboration of the subjects may increase (Wang et al., 2013 ) compared to in-class education and by using available materials, textbooks, practice assignments, students could devote extra energy to subjects, which may result in better exam grades.
From the teachers’ point of view, teachers might want to offer some ‘compensation’ at exams due to non-traditional teaching. In light of this, they are likely to ask a ‘slightly easier’ question, adapt them to the practice tasks, or even lower the exam requirements, e.g., lowering the score limits by 1-2 points more favourable, or accepting answers that would not be accepted in other circumstances.
Note that these two strategies may have been present at the same time: the teacher perceived increased student contribution during the semester, for example, greater activity in online classes, and therefore, provided them with some reward by giving better final grades after taking into consideration their overall performance during the semester.
Please note that both narratives could appear at the same time.
It is also important to see that although grade point averages shifted, the shift was not necessarily drastic, and dropout rates did not improve. It may also be legitimate that there were individual characteristics that caused the difference in the grade point average.
From the student’s point of view, it could also mean that they were prepared in the same way in online education as in in-class education for exams. However, the same strategy did not necessarily result in better grades in the upper segment (obtaining 4 or 5).
The teacher determined the minimum level of requirements, either for mid-term achievements or final assignments and communicated it clearly to the students. How to obtain a passing grade was clear to the students. However, how to obtain good and excellent grades would have required more serious preparation and self-directed learning in online settings.
It is important to see that subjects, where it was more difficult to obtain better grades, were mainly theoretical ones (e.g.: lectures). They were tested mostly by oral exams where it was not possible to use additional materials, they had to answer directly to the questions. In this respect, teachers’ explanations, for example, could lead to very serious shortcomings in the case of knowledge transfer as well as the transfer of the same levels of the previous examination systems. This could result in lower achievement in areas where teachers’ explanations would have been necessary. Students had a harder time bridging the online-offline gap.
Education management issues
In the higher education system analysed, students receive a scholarship according to their grade point average achievement. It is calculated based on the average of the final grades received at the end of the semester and the credits earned. It is worth considering that for online systems, credit-weighted averages will not necessarily show students’ real knowledge. This also results in serious problems when it comes to rewarding students’ performance with a scholarship, where multiple types of educational models may conflict.
This is because whether students can successfully complete a subject differs greatly in an online education system but subjects seem to have become fundamentally easier.
Thus, different education systems (in-class education and online education) can lead to different grading results, so it is not advisable to apply the same scholarship system because it can be fundamentally unfair (some fields can become easier or more difficult).
The results of this study imply that COVID-19 had various effects on the education sector. The results are discussed in connection with the introduction of online education during the COVID-19 pandemic in terms of dropouts. The teachers who were involved in this study were the same during online education and on-campus education. This is the reason why we can conclude that the results also seem to suggest that teachers tried to compensate for the negative effects of the pandemic by bringing in pedagogical strategies aimed at ensuring that students could more easily obtain passing grades in examinations. Similarly, according to Mendoza et al. ( 2021 ), the failures of online education had a direct impact on student’s performance and learning.
This study found that students achieved better results during in-class education, which offers interesting implications for teaching practice. The results suggest that organizational support and flexible structures are needed in order to adapt teaching to the new circumstances set by the crisis. Higher education institutions should pay careful attention to developing students’ skills as well as to seeking ways to quickly respond to environmental changes while sustaining the delivery of high-quality education.
In the literature review, contradictory results were found for students’ performance during online education; therefore, this result contends previous literature and should be further explored.
A substantial difference in grade point averages can be found between the two examined groups. The first hypothesis was confirmed: students who participated in on-campus education obtained better grade point averages than students of online education. The teachers declared the minimum level of requirement and communicated it to the students quite clearly. It is a thought-provoking result that for online education, credit-weighted grade point averages would not necessarily show real knowledge well.
The second hypothesis was also proved because some subjects became easier to pass in online education, at least obtaining a passing grade. Online education facilitated students’ strategies e.g., creating an agenda of studying was essential to maintain effective and continuous learning.
The third hypothesis was not confirmed because significant differences in dropout rates were not found between the students who participated in online education and on-campus education. The dropout rate remained nearly unchanged between students who participated in online education (19 students dropped out), and students who participated in on-campus education (50 students dropped out). Introducing online education was effective or at least not harmful in terms of dropout because the dropout rate remained unchanged, compared to the previous year.
The results suggest that regarding dropout rates, there was no significant difference between online and on-campus education. The result suggests several assumptions: e.g.: the teachers had been more indulgent, as they also found it more difficult to communicate effectively during the COVID-19 period and were less able to apply with traditional methods. The process of knowledge transfer moved to online platforms and a different kind of interaction could be applied to rely on the online education system.
Limitations of the study and future research
This study proposed research clarifying the impact of the transition to online courses on dropout. The results show that this period did not contribute significantly to the increase in dropouts. Subjects became more achievable during online education. Students who participated in online education reported lower average grade points than students who participated in on-campus education. Consequently, on-campus students could win better scholarships than students who participated in online education because of better grades.
Several other factors e.g., whether students have met in person in the past, could affect the dropout and grade point averages which were not taken into consideration in this research. In the future, it is recommended to measure students’ current level of knowledge, how much they can adapt to online education, and how they would react in the next similar crisis.
Even though this study presents interesting results, the authors believe that the conclusions derived from them should be interpreted carefully. It allows both researchers and teachers to develop further methods to examine students’ strategies in online education during the COVID-19 period. Future research should be extended with additional variables. Data analysis techniques should also be taken into consideration in order to evaluate the academic profile of students who dropped out in previous years. Limitations include that analysis does not entirely reflect the true engagement of students in the education system because only the first two semesters were examined.
The results of this study open new lines of similar research. It is hoped that other researchers will consider examining the potential impact of COVID-19 on educational planning and scholarship systems. The results of this study can further be validated by considering a wider study that would collect both quantitative and qualitative data to give a deeper understanding of the effects of this epidemic.
Data availability
The datasets generated during and/or analysed during the current study are available from the corresponding author on reasonable request.
For a detailed explanation of the method see Takács et al. ( 2021 ).
Abilleira MP, Rodicio-García M-L, Ríos-de Deus MP, Mosquera-González MJ (2021) Technostress in Spanish University teachers during the COVID-19 pandemic. Front Psychol 12. https://doi.org/10.3389/fpsyg.2021.617650
Adedoyin OB, Soykan E (2020) Covid-19 pandemic and online learning: the challenges and opportunities. Interact Learn Environ 1–13. https://doi.org/10.1080/10494820.2020.1813180
Akar I, Karabulut Coskun B (2020) Exploring the relationship between creativity and cyberloafing of prospective teachers. Think Skills Creativity 38:100724. https://doi.org/10.1016/j.tsc.2020.100724
Article Google Scholar
Andrew A, Cattan S, Costa-Dias M, Farquharson C, Kraftman L, Krutikova S, Phimister A, Sevilla A (2020) Learning during the lockdown: real-time data on children’s experiences during home learning. Institute for Fiscal Studies, London
Google Scholar
Bean JP, Metzner BS (1985) A conceptual model of nontraditional undergraduate student attrition. Rev Educ Res 55(4):485–540. https://doi.org/10.2307/1170245 . JSTOR
Bayrakdar S, Guveli A (2020) Inequalities in home learning and schools’ provision of distance teaching during school closure of COVID-19 lockdown in the UK. ISER Working Paper Series, No. 2020-09. University of Essex, Institute for Social and Economic Research (ISER), Colchester
Belloc F, Maruotti A, Petrella L (2011) How individual characteristics affect university students drop-out: a semiparametric mixed-effects model for an Italian case study. J Appl Stat 38(10):2225–2239. https://doi.org/10.1080/02664763.2010.545373
Article MathSciNet MATH Google Scholar
Bol T (2020) Inequality in homeschooling during the Corona crisis in the Netherlands. First results from the LISS Panel. https://osf.io/preprints/socarxiv/hf32q . Accessed 10 Nov 2021
Borzovs J, Niedrite L, Solodovnikova D (2015) Factors affecting attrition among first year computer science students: the case of University of Latvia. In: Edmund Teirumnieks (ed) Proceedings of the international scientific and practical conference on environment, technology and resources, Rezekne Academy of Technologies, vol 3. p. 36
Braxton JM, Hirschy AS (2004) Reconceptualizing antecedents of social integration in student departure. In: Yorke M, Longden B (Eds.) Retention and student success in higher education. MPG Books, Bodmin, Great Britain, pp. 89–102
Broadbent J, Poon WL (2015) Self-regulated learning strategies & academic achievement in online higher education learning environments: a systematic review. Internet High Educ 27:1–13. https://doi.org/10.1016/j.iheduc.2015.04.007
Brown N, Te Riele K, Shelley B, Woodroffe J (2020) Learning at home during COVID-19: effects on vulnerable young Australians. Independent rapid response report. University of Tasmania, Peter Underwood Centre for Educational Attainment, Hobart
Burgess S, Sievertsen HH (2020) Schools, skills, and learning: the impact of COVID-19 on education, VoxEu.org. https://voxeu.org/article/impact-covid-19-education . Accessed 11 Nov 2021
Cabrera L, Bethencourt JT, Pérez PA, Afonso MG (2006) El problema del abandono de los estudios universitarios. Rev Electrón Invest Eval Educ 12:171–203
Carr S (2000) As distance education comes of age, the challenge is keeping the students. Chron High Educ 46:23
Clark AE, Nong H, Zhu H, Zhu R (2021) Compensating for academic loss: online learning and student performance during the COVID-19 pandemic. China Econ Rev 68:101629. https://doi.org/10.1016/j.chieco.2021.101629
Article PubMed PubMed Central Google Scholar
Daniels LM, Goegan LD, Parker PC (2021) The impact of COVID-19 triggered changes to instruction and assessment on university students’ self-reported motivation, engagement and perceptions. Soc Psychol Educ 24(1):299–318. https://doi.org/10.1007/s11218-021-09612-3
Daumiller M, Rinas R, Hein J, Janke S, Dickhäuser O, Dresel M (2021) Shifting from face-to-face to online teaching during COVID-19: The role of university faculty achievement goals for attitudes towards this sudden change, and their relevance for burnout/engagement and student evaluations of teaching quality. Comput Hum Behav 118:106677. https://doi.org/10.1016/j.chb.2020.106677
Davis NL, Gough M, Taylor LL (2019) Online teaching: advantages, obstacles and tools for getting it right. J Teach Travel Tour 19(3):256–263. https://doi.org/10.1080/15313220.2019.1612313
Di Pietro G (2006) Regional labour market conditions and university dropout rates: evidence from Italy. Reg Stud 40(6):617–630. https://doi.org/10.1080/00343400600868770
Dumford AD, Miller AL (2018) Online learning in higher education: exploring advantages and disadvantages for engagement. J Comput High Educ 30(3):452–465. https://doi.org/10.1007/s12528-018-9179-z
Engzell P, Frey A, Verhagen MD (2021) Learning loss due to school closures during the COVID-19 pandemic. Proc Natl Acad Sci USA 118(17). https://doi.org/10.1073/pnas.2022376118
Europa.eu. (2015) European Commission—Press release—Commission says yes to first successful European Citizens’ Initiative. Resource document. https://ec.europa.eu/commission/presscorner/detail/en/IP_14_277 . Accessed 5 Nov 2020
Forero CG, Maydeu-Olivares A (2009) Estimation of IRT graded response models: limited versus full information methods. Psychol Methods 14(3):275–299. https://doi.org/10.1037/a0015825
Article PubMed Google Scholar
Gamage KAA, Silva EKde, Gunawardhana N (2020) Online delivery and assessment during COVID-19: safeguarding academic integrity. Educ Sci 10(11):301. https://doi.org/10.3390/educsci10110301
Gonzalez T, de la Rubia MA, Hincz KP, Comas-Lopez M, Subirats L, Fort S, Sacha GM (2020) Influence of COVID-19 confinement on students’ performance in higher education. PLoS ONE 15(10):e0239490. https://doi.org/10.1371/journal.pone.0239490
Article CAS PubMed PubMed Central Google Scholar
Iglesias-Pradas S, Hernández-García Á, Chaparro-Peláez J, Prieto JL (2021) Emergency remote teaching and students’ academic performance in higher education during the COVID-19 pandemic: a case study. Comput Hum Behav 119:106713. https://doi.org/10.1016/j.chb.2021.106713
Jovanovic J, Mirriahi N, Gašević D, Dawson S, Pardo A (2019) Predictive power of regularity of pre-class activities in a flipped classroom. Comput Educ 134:156–168. https://doi.org/10.1016/j.compedu.2019.02.011
Levy Y (2007) Comparing dropouts and persistence in e-learning courses. Comput Educ 48(2):185–204. https://doi.org/10.1016/j.compedu.2004.12.004
Lounsbury JW, Huffstetler BC, Leong FT, Gibson LW (2005) Sense of identity and collegiate academic achievement. J College Student Dev 46(5):501–514. https://doi.org/10.1353/csd.2005.0051
Mendoza D, Cejas M, Rivas G, Varguillas C (2021) Anxiety as a prevailing factor of performance of university mathematics students during the COVID-19 pandemic. Educ Sci J 23(2):94–113. https://doi.org/10.17853/1994-5639-2021-2-94-113
Molins-Ruano P, Sevilla C, Santini S, Haya PA, Rodríguez P, Sacha GM (2014) Designing videogames to improve students’ motivation. Comput Hum Behav 31:571–579. https://doi.org/10.1016/j.chb.2013.06.013
Morris LV, Wu S-S, Finnegan CL (2005) Predicting retention in online general education courses. Am J Distance Educ 19(1):23–36. https://doi.org/10.1207/s15389286ajde1901_3
Nagrecha S, Dillon JZ, Chawla NV (2017) MOOC dropout prediction: lessons learned from making pipelines interpretable. In: Rick Barrett, Rick Cummings (eds) Proceedings of the 26th International Conference on World Wide Web Companion. International World Wide Web Conferences Steering Committee, Perth, WA, Republic and Canton of Genova, pp. 351–359
Nistor N, Neubauer K (2010) From participation to dropout: quantitative participation patterns in online university courses. Comput Educ 55(2):663–672. https://doi.org/10.1016/j.compedu.2010.02.026
Ohland MW, Sheppard SD, Lichtenstein G, Eris O, Chachra D, Layton RA (2008) Persistence, engagement, and migration in engineering programs. J Eng Educ 97(3):259–278. https://doi.org/10.1002/j.2168-9830.2008.tb00978.x
OECD (2020) The impact of COVID-19 on student equity and inclusion: supporting vulnerable students during school closures and school re-openings. https://www.oecd.org/coronavirus/policy-responses/the-impact-of-covid-19-on-student-equity-and-inclusion-supporting-vulnerable-students-during-school-closures-and-school-re-openings-d593b5c8/
Pacansky-Brock M, Vincent-Layton K (2020) Humanizing online teaching to equitize higher ed. https://doi.org/10.13140/RG.2.2.33218.94402
Pascarella ET, Terenzini PT (1983) Predicting voluntary freshman year persistence/withdrawal behavior in a residential university: a path analytic validation of Tinto’s model. J Educ Psychol 75(2):215–226. https://doi.org/10.1037/0022-0663.75.2.215
Palvia S, Aeron P, Gupta P, Mahapatra D, Parida R, Rosner R, Sindhi S (2018) Online education: worldwide status, challenges, trends, and implications. J Global Inf Technol Manag 21(4):233–241. https://doi.org/10.1080/1097198X.2018.1542262
Patterson B, McFadden C (2009) Attrition in online and campus degree programs. Online J Distance Learn Adm 12(2). https://www.westga.edu/~distance/ojdla/summer122/patterson122.html
Paudel P (2020) Online education: benefits, challenges and strategies during and after COVID-19 in higher education. Int J Stud Educ 3(2):70–85. https://doi.org/10.46328/ijonse.32
Peimani N, Kamalipour H (2021) Online education and the COVID-19 outbreak: a case study of online teaching during lockdown. Educ Sci 11(2):72. https://doi.org/10.3390/educsci11020072
Rahiem MDH (2021) Remaining motivated despite the limitations: University students’ learning propensity during the COVID-19 pandemic. Children Youth Serv Rev 120:105802. https://doi.org/10.1016/j.childyouth.2020.105802
Rapanta C, Botturi L, Goodyear P, Guàrdia L, Koole M (2020) Online university teaching during and after the Covid-19 Crisis: refocusing teacher presence and learning activity. Postdigit Sci Educ 2(3):923–945. https://doi.org/10.1007/s42438-020-00155-y
Rasch G (1960) Probabilistic models for some intelligence and achievement tests. Danish Institute for Educational Research, Copenhagen, Denmark
Reason RD (2009) Review of the book evaluating faculty performance: a practical guide to assessing teaching, research, and service. Rev High Educ 32(2):288–289. https://doi.org/10.1353/rhe.0.0043
Richardson M, Abraham C, Bond R (2012) Psychological correlates of university students’ academic performance: a systematic review and meta-analysis. Psychol Bull 138(2):353–387. https://doi.org/10.1037/a0026838
Rovai AP (2003) In search of higher persistence rates in distance education online programs. Internet High Educ 6(1):1–16. https://doi.org/10.1016/S1096-7516(02)00158-6
Said EGR (2021) How did the COVID-19 pandemic affect higher education learning experience? An empirical investigation of learners’ academic performance at a University in a Developing Country. Adv Hum–Comput Interact 2021:1–10. https://doi.org/10.1155/2021/6649524
Serdyukov P, Hill R (2013) Flying with clipped wings: are students independent in online college classes? J Res Innov Teach 6(1):54–67
Stommel J (2013) Decoding digital pedagogy, part. 2: (Un)Mapping the terrain. Hybrid Pedagog. https://hybridpedagogy.org/decoding-digital-pedagogy-pt-2-unmapping-the-terrain/
Takács R, Kárász JT, Takács S, Horváth Z, Oláh A (2021) Applying the Rasch model to analyze the effectiveness of education reform in order to decrease computer science students’ dropout. Humanit Soc Sci Commun 8(1):1–8. https://doi.org/10.1057/s41599-021-00725-w . Springer Nature
Terenzini PT, Reason RD (2005) Parsing the first year of college: rethinking the effects of college on students. The Association for the Study of Higher Education, Philadelphia, p. 630
Tinto V (1975) Dropout from higher education: a theoretical synthesis of recent research. Rev Educ Res 45:89–125
Tinto V (2012) Completing college: rethinking institutional action. The University of Chicago Press, Chicago
Book Google Scholar
Wang C (2010) Students’ characteristics, self-regulated learning, technology self-efficacy, and course outcomes in web-based courses. https://etd.auburn.edu//handle/10415/2256
Wang C-H, Shannon DM, Ross ME (2013) Students’ characteristics, self-regulated learning, technology self-efficacy, and course outcomes in online learning. Distance Educ 34(3):302–323. https://doi.org/10.1080/01587919.2013.835779
Watermeyer R, Crick T, Knight C, Goodall J (2021) COVID-19 and digital disruption in UK universities: Afflictions and affordances of emergency online migration. High Educ 81(3):623–641. https://doi.org/10.1007/s10734-020-00561-y
Whittle M, Rampton J (2020) Towards a 2030 vision on the future of universities in Europe. Publications Office of the European Union. http://op.europa.eu/en/publication-detail/-/publication/a3cde934-12a0-11eb-9a54-01aa75ed71a1/
Willging PA, Johnson SD (2019) Factors that influence students’ decision to dropout of online courses. J Asynchronous Learn Netw 13(3):13
Yu H, Liu P, Huang X, Cao Y (2021) Teacher online informal learning as a means to innovative teaching during home quarantine in the COVID-19 pandemic. Front Psychol 12:2480. https://doi.org/10.3389/fpsyg.2021.596582
Download references
Acknowledgements
The described article was carried out as part of the EFOP 3.4.3-16-2016-00011 project in the framework of the Széchenyi 2020 programme. The realization of these projects is supported by the European Union, co-financed by the European Social Fund.
Open access funding provided by Eötvös Loránd University.
Author information
Authors and affiliations.
Faculty of Informatics, ELTE Eötvös Loránd University, Budapest, Hungary
Rita Takács & Zoltán Horváth
Department of General Psychology and Methodology, Institute of Psychology, Budapest, Hungary
Szabolcs Takács
Károli Gáspár University of the Reformed Church in Hungary, Budapest, Hungary
Doctoral School of Education, ELTE Eötvös Loránd University, Budapest, Hungary
Judit T. Kárász
Institute of Education, ELTE Eötvös Loránd University, Budapest, Hungary
Doctoral School of Psychology, ELTE Eötvös Loránd University, Budapest, Hungary
Attila Oláh
Institute of Psychology, ELTE Eötvös Loránd University, Budapest, Hungary
You can also search for this author in PubMed Google Scholar
Contributions
TR contributed to the design of the study and data interpretation. As principal author, she coordinated the writing process of the manuscript. KJ and TS are researchers that study the dropout phenomenon across higher education, and therefore have participated on each phase of this research. OA and HZ have largely contributed to the analysis and interpretation of data, and consequently to the understanding of the phenomenon. Every author have played a remarkable role in the writing of this article.
Corresponding author
Correspondence to Rita Takács .
Ethics declarations
Ethics approval.
The Ethics Committee of the Institute of Psychology of Károli Gáspár University of the Reformed Church in Hungary approved of the ethical permission, which was registered under the following number: 75/2020 / P / ET. The study protocol was designed and executed in compliance with the code of ethics set out by the university in which the research was conducted, as required by the Helsinki Declaration.
Consent to participate and consent for publication
Written informed consent was obtained from the participants. The data were anonymized before analysis and publication.
Competing interests
The authors declare no competing interests.
Additional information
Publisher’s note Springer Nature remains neutral with regard to jurisdictional claims in published maps and institutional affiliations.
Rights and permissions
Open Access This article is licensed under a Creative Commons Attribution 4.0 International License, which permits use, sharing, adaptation, distribution and reproduction in any medium or format, as long as you give appropriate credit to the original author(s) and the source, provide a link to the Creative Commons license, and indicate if changes were made. The images or other third party material in this article are included in the article’s Creative Commons license, unless indicated otherwise in a credit line to the material. If material is not included in the article’s Creative Commons license and your intended use is not permitted by statutory regulation or exceeds the permitted use, you will need to obtain permission directly from the copyright holder. To view a copy of this license, visit http://creativecommons.org/licenses/by/4.0/ .
Reprints and permissions
About this article
Cite this article.
Takács, R., Takács, S., Kárász, J.T. et al. The impact of the first wave of COVID-19 on students’ attainment, analysed by IRT modelling method. Humanit Soc Sci Commun 10 , 127 (2023). https://doi.org/10.1057/s41599-023-01613-1
Download citation
Received : 13 February 2021
Accepted : 08 March 2023
Published : 25 March 2023
DOI : https://doi.org/10.1057/s41599-023-01613-1
Share this article
Anyone you share the following link with will be able to read this content:
Sorry, a shareable link is not currently available for this article.
Provided by the Springer Nature SharedIt content-sharing initiative
Quick links
- Explore articles by subject
- Guide to authors
- Editorial policies

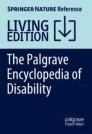
The Palgrave Encyclopedia of Disability pp 1–9 Cite as
Impact of COVID-19 on Teachers’ Quality of Life and Attitude toward Inclusion
- Sara Santilli 3 ,
- Isabella Valbusa 3 &
- Maria Cristina Ginevra 3
- Living reference work entry
- First Online: 26 January 2024
The pandemic outbreak led to economic, health, and social crises, which shaped an ever more complex and challenging context, such as the school environment and specifically in students with disability. Even before this pandemic, students with disabilities or vulnerability are at greater risk for school exclusion than their peers without disabilities. Indeed, students with disability or vulnerability are predominantly left behind during disasters, crises, and emergencies, such as in the case of COVID-19. In particular, teachers’ attitudes are a critical element to make school inclusion successful. Analysis and reflection on teachers’ attitudes in COVID-19 time may constitute the basis for the school’s continuous development to implement inclusion.
This contribution focused on Italian teachers’ attitudes toward students with disabilities or vulnerability as potential social contextual factors favoring or hindering more complex school inclusion processes during COVID-19. Overall, the results of these studies showed as COVID-19 can implement negative teacher attitudes toward inclusion and negatively influence a vision of the more conservative, less cooperative, and supportive future. These results underline the importance of investigating the relationship between environmental and personal variables, such as attitudes toward disability inclusion, with the teacher and all actors that can support inclusive school contexts.
This is a preview of subscription content, log in via an institution .
Ajzen, I. (2005). Attitudes, personality and behaviour . McGraw-Hill Education.
Google Scholar
Avcioglu, H. (2017). Classroom teachers’ behaviors and peers’ acceptance of students in inclusive classrooms. Educational Sciences: Theory and Practice, 17 (2), 463–492. https://doi.org/10.12738/estp.2017.2.0034
Article Google Scholar
Avramidis, E., & Kalyva, E. (2007). The influence of teaching experience and professional development on Greek teachers’ attitudes towards inclusion. European Journal of Special Needs Education, 22 (4), 367–389. https://doi.org/10.1080/08856250701649989
Bernard, A., Weiss, S., Stein, J. D., Ulin, S., D’Souza, C., Salgat, A., ... & Ehrlich, J. (2020). Development of a novel survey to measure the impact of COVID-19 on persons with disabilities. https://doi.org/10.31219/osf.io/2cu4h .
Bešić, E., Paleczek, L., & Gasteiger-Klicpera, B. (2020). Don’t forget about us: Attitudes towards the inclusion of refugee children with (out) disabilities. International Journal of Inclusive Education, 24 (2), 202–217. https://doi.org/10.1080/13603116.2018.1455113
Bohner, G., & Dickel, N. (2011). Attitudes and attitude change. Annual Review of Psychology, 62 , 391–417. https://doi.org/10.1146/annurev.psych.121208.131609
Article PubMed Google Scholar
Brent, A., Chatterjee, A., Burmester, D., & Rayudu, R. (2020). Sustainable microgrids for remote communities: A practical framework for analyzing and designing. In The Palgrave handbook of climate resilient societies (pp. 1–29). Springer International Publishing.
Cassady, J. M. (2011). Teachers’ attitudes toward the inclusion of students with autism and emotional behavioral disorder. Electronic Journal for Inclusive Education, 2 (7), 5.
Castro-Kemp, S., & Mahmud, A. (2021, May). School closures and returning to school: Views of parents of children with disabilities in England during the Covid-19 pandemic. In Frontiers in education (Vol. 6, p. 149). Frontiers. https://doi.org/10.3389/feduc.2021.666574
Chapter Google Scholar
Chimhenga, S. (2016). Resource material barriers: The challenges of implementing inclusive education in primary schools of Zimbabwe. Global Journal of Advanced Research, 3 (6), 526–532. https://d1wqtxts1xzle7.cloudfront.net/47405720/dd496r25-libre.pdf?1469114830=&response-content-disposition=inline%3B+filename%3DRESOURCE
Coelho, M., & Menezes, I. (2021). Universitas: How do students perceive university social responsibility in three European higher education institutions? International Journal of Sustainability in Higher Education . https://doi.org/10.1108/IJSHE-04-2021-0130
Collie, R. J., Shapka, J. D., Perry, N. E., & Martin, A. J. (2016). Teachers’ psychological functioning in the workplace: Exploring the roles of contextual beliefs, need satisfaction, and personal characteristics. Journal of Educational Psychology, 108 (6), 788. https://doi.org/10.1037/edu0000088
De Boer, A., Pijl, S. J., & Minnaert, A. (2011). Regular primary schoolteachers’ attitudes towards inclusive education: A review of the literature. International Journal of Inclusive Education, 15 (3), 331–353. https://doi.org/10.1080/13603110903030089
De Boer, A., Pijl, S. J., & Minnaert, A. (2012). Students’ attitudes towards peers with disabilities: A review of the literature. International Journal of Disability, Development and Education, 59 (4), 379–392. https://doi.org/10.1080/1034912X.2012.723944
de Oliveira Silva, D. F., Cobucci, R. N. O., Lima, S. C. V. C., & de Andrade, F. B. (2021). Prevalence of anxiety, depression, and stress among teachers during the COVID-19 pandemic: Systematic review. medRxiv . https://doi.org/10.1101/2021.05.01.21256442
Di Maggio, I., Ginevra, M. C., Santilli, S., & Nota, L. (under review-a). The impact of COVID-19 on state anxiety, attitudes toward school inclusion, and life satisfaction in Italian teachers . Teachers and Teaching.
Di Maggio, I., Santilli, S., Ginevra, M. C., & Nota, L. (under review-b). State anxiety and personal need of structured as mediator between Covid-19 and teachers’ attitudes toward sustainable and more conservative future . Teachers and Teaching.
Dias, P. C., & Cadime, I. (2016). Effects of personal and professional factors on teachers’ attitudes towards inclusion in preschool. European Journal of Special Needs Education, 31 (1), 111–123. https://doi.org/10.1080/08856257.2015.1108040
Duckworth, A. L., Quinn, P. D., & Seligman, M. E. (2009). Positive predictors of teacher effectiveness. The Journal of Positive Psychology, 4 (6), 540–547. https://doi.org/10.1080/17439760903157232
Education Support. (2019). Teacher wellbeing index. Education Support. https://www.educationsupport.org.uk/media/b1qbtmzl/teacher_wellbeing_index_2019.pdf
Ferguson, K., Frost, L., & Hall, D. (2012). Predicting teacher anxiety, depression, and job satisfaction. Journal of Teaching and Learning, 8 (1). https://doi.org/10.22329/jtl.v8i1.2896
Garbe, A., Ogurlu, U., Logan, N., & Cook, P. (2020). COVID-19 and remote learning: Experiences of parents with children during the pandemic. American Journal of Qualitative Research, 4 (3), 45–65. https://doi.org/10.29333/ajqr/8471
Ginevra, M. C., Di Maggio, I., Valbusa, I., Santilli, S., & Nota, L. (2021). Teachers’ attitudes towards students with disabilities: The role of the type of information provided in the students’ profiles of children with disabilities. European Journal of Special Needs Education , 1–14. https://doi.org/10.1080/08856257.2021.1878658
Hecht, P., Aiello, P., Pace, E. M., & Sibilio, M. (2017). Attitudes and teacher efficacy among Italian and Austrian teachers: A comparative study. Formazione & Insegnamento. Rivista internazionale di Scienze dell’educazione e della formazione, 15 (1), 269–282. https://ojs.pensamultimedia.it/index.php/siref/article/view/2179
Huber, S. G., P. S. Günther, N. Schneider, C. Helm, M. Schwander, J. A. Schneider, & J. Pruitt. 2020. COVID-19 – Aktuelle Herausforderungen in Schule und Bildung. Erste Befunde des Schul-Barometers in Deutschland, Österreich und der Schweiz [COVID-19 – Current challenges in school and education. First findings of the school barometer in Germany, Austria and Switzerland]. Münster.
Hussain, M. A., Shahid, S., & Zaman, A. (2011). Anxiety and attitude of secondary school students towards foreign language learning. Procedia-Social and Behavioral Sciences, 29 , 583–590. https://doi.org/10.1016/j.sbspro.2011.11.279
Ianes, D., Demo, H., & Dell’Anna, S. (2020). Inclusive education in Italy: Historical steps, positive developments, and challenges. Prospects, 49 (3), 249–263. https://doi.org/10.1007/s11125-020-09509-7
Jakubowski, T. D., & Sitko-Dominik, M. M. (2021). Teachers’ mental health during the first two waves of the COVID-19 pandemic in Poland (Vol. 16). https://doi.org/10.1371/journal.pone.0257252
Book Google Scholar
Karwowski, M., Zielińska, A., Jankowska, D. M., Strutyńska, E., Omelańczuk, I., & Lebuda, I. (2021). Creative lockdown? A daily diary study of creative activity during pandemics. Frontiers in psychology, 12 , 600076.
Kast, F., Schwill, M., Stüber, J. C., Pfundstein, S., Nagy-Davidescu, G., Rodríguez, J. M. M., ... & Plückthun, A. (2021). Engineering an anti-HER2 biparatopic antibody with a multimodal mechanism of action. Nature Communications, 12 (1), 1–18. https://doi.org/10.1038/s41467-021-23948-6 .
Kuper, H., Banks, L. M., Bright, T., Davey, C., & Shakespeare, T. (2020). Disability-inclusive COVID-19 response: What it is, why it is important and what we can learn from the United Kingdom’s response. Wellcome Open Research, 5 , 10.12688/wellcomeopenres.15833.1.
Larson, E. A., Bader-Larsen, K. S., & Magkos, F. (2021). The effect of COVID-19-related lockdowns on diet and physical activity in older adults: A systematic review. Aging and Disease, 0 , 10.14336/AD.2021.0606.
Lombardi, D., & Sinatra, G. M. (2013). Emotions about teaching about human-induced climate change. International Journal of Science Education, 35 (1), 167–191. https://doi.org/10.1080/09500693.2012.738372
Madhesh, A. (2021). Full exclusion during COVID-19: Saudi deaf education is an example. Heliyon, 7 (3), e06536. https://doi.org/10.1016/j.heliyon.2021.e06536
Article PubMed PubMed Central Google Scholar
Markava, M., Pit-Ten Cate, I., Krolak-Schwerdt, S., & Glock, S. (2016). Preservice teachers’ attitudes toward inclusion and toward students with special educational needs from different ethnic backgrounds. The Journal of Experimental Education, 84 (3), 554–578. https://doi.org/10.1080/00220973.2015.1055317
Nota, L., Soresi, S., Di Maggio, I., Santilli, S., & Ginevra, M. C. (2020). Sustainable development . Springer.
OECD. (2020). The impact of COVID-19 on student equity and inclusion: Supporting vulnerable students during school closures and school re-openings . OECD Publishing. https://www.oecd.org/education/strength-through-socialty/OECD%20COVID-19%20Brief%20Vulnerable%20Students.pdf
OECD. (2021). Teachers’ wellbeing: A framework for data collection and analysis. OECD Publishing. https://www.oecd.org/officialdocuments/publicdisplaydocumentpdf/?cote=EDU/WKP(2020)1&docLanguage=En
Ojala, M., & Bengtsson, H. (2019). Young people’s coping strategies concerning climate change: Relations to perceived communication with parents and friends and proenvironmental behavior. Environment and Behavior, 51 (8), 907–935. https://doi.org/10.1177/0013916518763894
Parmigiani, D., Benigno, V., Giusto, M., Silvaggio, C., & Sperandio, S. (2020). E-inclusion: Online special education in Italy during the Covid-19 pandemic. Technology, Pedagogy and Education , 1–14. https://doi.org/10.1080/1475939X.2020.1856714
Pit-ten Cate, I. M., & Glock, S. (2019). Teachers’ implicit attitudes toward students from different social groups: A meta-analysis. Frontiers in Psychology, 10 , 2832. https://doi.org/10.1023/A:1020339004125
Santilli, S., di Maggio, I., Ginevra, M. C., Nota, L., & Soresi, S. (2020). Life design, inclusion, and sustainable development: Constructs, dimensions and new instruments to stimulate a quality future design for all. In M. Yuen, W. Beamish, & V. Solberg (Eds.), Careers for students with special educational needs (pp. 301–312). Springer.
Schalock, R. L., & Felce, D. (2004). Quality of life and subjective wellbeing: Conceptual and measurement issues. In E. Emerson, C. Hatton, T. Thompson, & T. Parmenter (Eds.), International handbook of applied research in intellectual disabilities (pp. 261–279). Wiley.
Sermier Dessemontet, R., Benoit, V., & Bless, G. (2011). Schulische Integration von Kindern mit einer geistigen Behinderung. Untersuchung der Entwicklung der Schulleistungen und der adaptiven Fähigkeiten, der Wirkung auf die Lernentwicklung der Mitschüler sowie der Lehrereinstellungen zur Integration. Empirische Sonderpädagogik, 3 (4), 291–307. https://doi.org/10.25656/01:9329
Seubert, C., Hopfgartner, L., & Glaser, J. (2021). Living wages, decent work, and need satisfaction: an integrated perspective. European Journal of Work and Organizational Psychology, 30 (6), 808–823. https://doi.org/10.1080/1359432X.2021.1966094
Shek, D. T. (2021). COVID-19 and quality of life: Twelve reflections. Applied Research in Quality of Life, 16 (1), 1–11. https://doi.org/10.1007/s11482-020-09898-z
Shogren, K. A., Wehmeyer, M. L., Schalock, R. L., & Thompson, J. R. (2016). Reframing educational supports for students with intellectual disability through strengths-based approaches. In Handbook of research-based practices for educating students with intellectual disability (pp. 25–38). Routledge.
Soresi, S., Nota, L., & Wehmeyer, M. L. (2011). Community involvement in promoting inclusion, participation and self-determination. International Journal of Inclusive Education, 15 (1), 15–28. https://doi.org/10.1080/13603116.2010.496189
The Badass Teachers Association. (2017). Teaching in the time of Covid-19. https://static1.squarespace.com/static/5d30f5468f2df10001eae004/t/5f614aa8d6780364661d74b2/1600211626539/Teaching+in+the+Time+of+COVID-19+Exec+Summary.pdf
Thompson, M. M., Naccarato, M. E., Parker, K. C., & Moskowitz, G. B. (2001). The personal need for structure and personal fear of invalidity measures: Historical perspectives, current applications, and future directions. In G. B. Moskowitz (Ed.), Cognitive social psychology: The Princeton symposium on the legacy and future of social cognition (pp. 19–39). Erlbaum.
Trzebiński, J., Cabański, M., & Czarnecka, J. Z. (2020). Reaction to the COVID-19 pandemic: the influence of meaning in life, life satisfaction, and assumptions on world orderliness and positivity. Journal of Loss and Trauma, 25 (6–7), 544–557. https://doi.org/10.1080/15325024.2020.1765098
Tsaridou, H., & Polyzopoulou, K. (2014). Greek teachers’ attitudes toward the inclusion of students. with special educational needs. American . Journal of Educational Research, 2 , 208–218. https://doi.org/10.12691/education-2-4-6
UNESCO. (2020). Education: From disruption to recovery. https://en.unesco.org/covid19/educationresponse/ .
UNESCO & IESALC. (2020, April). COVID-19 and higher education: Today and tomorrow. Impact analysis, policy responses and recommendations. Isaac, 9 , 1–46. http://www.iesalc.unesco.org/en/wp-content/uploads/2020/05/COVID-19-EN130520.pdf
United Nations. (2015). Transforming our world: The agenda 2030 for sustainable development. https://sustainabledevelopment.un.org/content/documents/21252030%20Agenda%20for%20Sustainable%20Development%20web.pdf
Vaz, S., Wilson, N., Falkmer, M., Sim, A., Scott, M., Cordier, R., & Falkmer, T. (2015). Factors associated with primary school teachers’ attitudes toward the inclusion of students with disabilities. PLoS One, 10 (8). https://doi.org/10.1371/journal.pone.0137002
Vogt, C. A., Andereck, K. L., & Pham, K. (2020). Designing for quality of life and sustainability. Annals of Tourism Research, 83 (102), 963. https://doi.org/10.1016/j.annals.2020.102963
Yakar, B., Karakaya, G., Pirincci, E., Onalan, E., Akkoc, R. F., & Aydın, S. (2021). Knowledge, behaviours and anxiety of eastern part of Turkey residents about the current COVID-19 outbreak. Acta Bio Medica: Atenei Parmensis, 92 (3). https://doi.org/10.23750/abm.v92i3.10990
Download references
Author information
Authors and affiliations.
Department of Philosophy, Sociology, Education and Applied Psychology, University of Padova, Padua, Italy
Sara Santilli, Isabella Valbusa & Maria Cristina Ginevra
You can also search for this author in PubMed Google Scholar
Corresponding author
Correspondence to Sara Santilli .
Editor information
Editors and affiliations.
c/o Dr. Matthew Bennett, Klemzig, SA, Australia
Gabriel Bennett
Positive Partnerships, Seaford, SA, Australia
Emma Goodall
Section Editor information
No affiliation provided
Melina Malli
Paul Manuel Aviles Baker
Stephen Meyers
Tanya Marie Breen
Chataika Tsitsi
Rights and permissions
Reprints and permissions
Copyright information
© 2024 The Author(s), under exclusive licence to Springer Nature Switzerland AG
About this entry
Cite this entry.
Santilli, S., Valbusa, I., Ginevra, M.C. (2024). Impact of COVID-19 on Teachers’ Quality of Life and Attitude toward Inclusion. In: Bennett, G., Goodall, E. (eds) The Palgrave Encyclopedia of Disability. Palgrave Macmillan, Cham. https://doi.org/10.1007/978-3-031-40858-8_99-1
Download citation
DOI : https://doi.org/10.1007/978-3-031-40858-8_99-1
Received : 22 December 2023
Accepted : 24 December 2023
Published : 26 January 2024
Publisher Name : Palgrave Macmillan, Cham
Print ISBN : 978-3-031-40858-8
Online ISBN : 978-3-031-40858-8
eBook Packages : Springer Reference Behavioral Science and Psychology Reference Module Humanities and Social Sciences Reference Module Business, Economics and Social Sciences
- Publish with us
Policies and ethics
- Find a journal
- Track your research
- Open access
- Published: 12 April 2024
Dose-response relationship between 15 weeks of running and aerobic fitness: a retrospective analysis based on the fun running program
- Zhixuan Tao ORCID: orcid.org/0000-0001-7619-4989 1 ,
- Xugui Sun 1 ,
- Jun Sun ORCID: orcid.org/0009-0006-7875-0540 1 na1 &
- Ergang Zhu 1 na1
BMC Public Health volume 24 , Article number: 1019 ( 2024 ) Cite this article
Metrics details
Students’ physical fitness, particularly aerobic fitness, has seriously declined during the COVID-19 epidemic. However, in the post-epidemic era, there are few studies on the methods of improving aerobic fitness. Understanding the dose-response relationship between physical activity and aerobic fitness is crucial for developing effective exercise prescriptions.
This retrospective study reviewed the Fun Running program at Wannan Medical College in China. We conducted a pre-post study design to analyze the impact of 15 weeks of Fun Running training on aerobic fitness. Middle and long-distance running pace (MLDR-P) was used as the primary indicator of aerobic fitness. A paired sample T-test was used to analyze the differences between the two MLDR-Ps. Pearson’s correlation was used to examine the correlation between variables. Multiple linear regression was used to determine the extent to which Fun Running components explain the variance in MLDR-P.
A total of 3244 college students participated in this study. 15 weeks of Fun Running training can significantly improve the MLDR-P in both females ( P < 0.001, ES = 0.68) and males ( P < 0.001, ES = 0.72). The MLDR-P was significantly correlated with Fun Running (R 2 = 0.95, p < 0.05, for females; R 2 = 0.96, p < 0.05, for males). The component that had the greatest impact on MLDR-P was pace (β = 1.39, for females; β = 1.09, for males), followed by distance (β = 0.49, for females; β = 0.15, for males), and last frequency (β = -0.03, for all).
This study fills the gap in research on the dose-response relationship between running and aerobic fitness among college students in the post-epidemic era. The results show that 15 weeks of Fun Running training can significantly improve aerobic fitness. Examination of the dose-response relationship between Fun Running and MLDR-P provides practitioners with valuable insights into prescribing aerobic fitness training, allowing them to develop more effective training programs. Future research should focus on how to implement a hierarchical Fun Running program effectively.
Peer Review reports
In the wake of the COVID-19 pandemic, students across the globe have experienced a considerable decrease in their physical activities [ 1 , 2 , 3 ]. Additionally, the widespread adoption of online courses has further exacerbated the sedentary behavior of students during the pandemic [ 4 , 5 ]. Consequently, there has been a significant decline in the physical fitness of students [ 6 , 7 ], particularly in aerobic fitness [ 8 ]. Therefore, it is very urgent and important to conduct in-depth research on how to effectively enhance the aerobic fitness of students in the post-epidemic era.
Aerobic fitness, serving as a critical indicator for assessing an individual’s cardiopulmonary health [ 9 ], has been confirmed by numerous studies to be associated with the risk of cardiovascular diseases [ 10 , 11 , 12 , 13 ]. Specifically, a high level of aerobic fitness is closely linked to a lower risk of cardiovascular diseases [ 10 , 11 ]. Conversely, individuals with inadequate cardiorespiratory function often face a higher overall mortality rate and an increased risk of cardiovascular diseases [ 12 , 13 ]. Therefore, enhancing aerobic fitness is not only crucial for an individual’s overall health but also a key strategy for the prevention of cardiovascular diseases.
There are various methods to improve aerobic fitness, such as swimming, aerobics, and cycling [ 14 ]. However, running stands out as the most cost-effective and accessible form of aerobic exercise [ 15 ]. The positive effects of running on enhancing physical fitness have been widely confirmed. Studies have shown that both high-intensity interval training and continuous endurance training can significantly enhance individual aerobic fitness and improve body composition [ 16 , 17 , 18 ]. Fun Running, as a low-intensity form of endurance training, has become a popular activity for college students [ 19 ]. It not only demonstrates significant effectiveness in enhancing aerobic fitness but also plays a pivotal role in cultivating a positive sports culture on campus [ 20 ]. These findings provide a scientific basis for the diversification of training for running, confirming the positive contribution of various running styles to health. However, it is worth noting that there is a dose-response relationship between different forms of running and health outcomes in aerobic fitness.
The dose-response relationship refers to the physiological response to a given training load [ 21 ]. It should be noted that an individual’s exercise tolerance may vary due to their biological adaptability to exercise, which ultimately determines the appropriate and effective exercise dose [ 22 ]. Therefore, a core issue in developing effective exercise prescriptions is to understand the impact of different physical activity doses on health and health outcomes through empirical evaluation and comparison of actual doses. There is a wealth of studies about aerobic fitness training and loads, as well as many studies documenting dose-response. The World Health Organization has recommended that individuals engage in 150 min of moderate physical activity or 75 min of vigorous-intensity aerobic exercise weekly to attain longevity benefits [ 23 ]. Several other studies [ 24 , 25 ] have also established different levels of physical activity based on the extent of sedentary behavior, considering varying intensities, durations, and frequencies.
However, the debate is ongoing about what exercise loads lead to better aerobic fitness and the lower limits of these loads. Moreover, the evidence regarding dose-response relationships between exercise load and outcomes in aerobic fitness in college students remains very limited. To the best of our knowledge, there are few studies have focused on the dose-response relationship between running and aerobic fitness among college students in the post-epidemic era. Therefore, this study aimed to investigate the dose-response relationship between 15 weeks of running and eaerobic fitness based on the Fun Running program of Wannan Medical College. The purpose of our study is (1) To provide deep insight into the impact of the Fun Running program on aerobic fitness development among college students. (2) To examine the dose-response relationship between the components of Fun Running and aerobic fitness and formulate a more effective Fun Running program.
Participants and ethics
The basic information of the participants is presented in Table 1 . A total of 3224 college students (1694 females, 1530 males) enrolled at Wannan Medical College in 2022 were involved. The average age of participants is 18.5 ± 0.6 years, and the average BMI is 21.8 ± 3.5 kg/m 2 . To ensure that the results of Fun Running programs accurately reflected the natural aerobic fitness levels of the participants, we did not implement any additional physical training for the participants beyond regular physical education classes. Meanwhile, to safeguard the aerobic fitness test and the Fun Running program, participants with health conditions requesting exemption from the physical fitness test were excluded (32 females, 34 males). The study was approved by the Ethics Review Committee of Wannan Medical College, and the consent of all participants was obtained.
Study design
This is a retrospective study conducted by reviewing the Fun Running program at Wannan Medical College in China. A pre-post study design was performed by comparing the changes in MLDR-P before and after a 15-week Fun Running program that was implemented from February to June of 2023. We compared the differences between the two MLDR-Ps and examined the dose-response relationship between the three components of the Fun Running and MLDR-P.
Fun running program and measurement
The Fun Running application (version 3.8.0, Fun Running Sports Internet Co., Ltd., Wuhan) serves as a mobile phone-based tool for recording various data related to Fun Running. This includes information on frequency, pace, and distance. To adhere to the program, certain rules were put in place: (1) The distance covered during each run must meet the standard, with a minimum of 2.5 km for female students and 3.0 km for female students. (2) The pace of each run should range between 3 min/km and 9 min/km. (3) The running route of each run must pass through three designated check-in locations, which are randomly assigned by the app. To encourage students to participate in the Fun Running program, the school stipulates that students can receive 1 point for each Fun Running, with a maximum limit of 30 points, which will be included in the final physical education score (30%).
The measurement of Fun Running involves a series of steps. First, participants are required to initiate the app on their mobile phones before commencing their run. They must grant permission for GPS positioning to be used by their devices. Second, participants are required to press the “complete” button and upload the results at the end of each run. Last, the app automatically evaluates the pace and distance achieved during the run and compares it with the three rules of the Fun Running program, only runs that adhere to these rules will be included in the final results. All Fun Running component data were obtained from the supplier at the end of the Fun Running program.
Aerobic fitness and measurement
According to the Chinese “National Physical Fitness Test Standards”, for college students, the performance of 800-meter running for females and 1000-meter running for males is considered the primary indicator of aerobic fitness [ 26 ]. In this study, we collectively refer to these distances as middle and long-distance running.
At Wannan Medical College, students are required to complete the middle and long-distance running test at the end of each semester unless they have applied for an exemption. It was counted as part of their physical education course grade (10%). For this study, we selected the results of two middle and long-distance running tests, conducted before and after the Fun Running program. Both tests were administered by the same group of physical education teachers at Wannan Medical College. The performance of the 800/1000 meter run was recorded using a stopwatch (YS-810, Yisheng Technology Co., Ltd., Shenzhen, China) and measured in minutes. All middle and long-distance running test results were obtained from the Department of Physical Education of Wannan Medical College. In our study, MLDR-P was used as a measure of aerobic fitness. It was calculated using the following formula:
MLDR- P = Duration (min)/Distance (km).
Statistical analysis
SPSS 27.0(SPSS Inc.) was used for statistical analysis. Descriptive statistics are presented as means and standard deviations (Mean ± SD). Standardized residual plots (histograms, normal probability plots), Durbin-Watson tests, and variability measures were used to examine the variable distributions. A paired samples T test was used to analyze the differences between the two MLDR-Ps, with the effect size (Cohen’s d). The magnitude of change was interpreted based on the following criteria: > 0.2–0.6, small; > 0.6–1.2, moderate; > 1.2, large [ 27 ]. Pearson’s correlation coefficient was used to examine the strength and direction of the dose-response relationship between the Fun Running components and MLDR-P. The magnitude of the relationship was interpreted using the following criteria: < 0.3 low; > 0.3–0.5 moderate; > 0.5–0.7 high; > 0.7 very high [ 27 ]. Multiple regression analysis was used to examine the association between the components of Fun Running and MLDR-P. The coefficient of determination (R 2 ) was calculated via linear regression analysis to determine the level of variance in MLDR-P explained by Fun Running. Standardized regression coefficients were also calculated to understand the extent to which each component of the Fun Running affected the MLDR-P. Each dependent variable was analyzed separately, with the models adjusted for sex. A significance level of P < 0.05 (2-tailed) was considered indicative of statistical significance.
Table 2 shows the results of the three components of Fun Running. For females, the mean and standard deviation for frequency were 30.5 ± 2.5, for distance were 2.20 ± 0.19 km, and for pace were 7.01 ± 0.72 min/km. For males, the corresponding values were 30.3 ± 4.1, 3.07 ± 0.29 km, and 6.30 ± 0.76 min/km, respectively.
Table 3 shows the results of the comparison of MLDR-P before and after the Fun Running program. The changes in MLDR-P were found to be significant for both female and male students. Specifically, the mean MLDR-P improved by 0.11 ( P < 0.001, d = 0.68) for females and 0.09 ( P < 0.001, d = 0.72) for males.
Table 4 shows the correlation between MLDR-P and the three components of the Fun Running. All three components of Fun Running displayed significant intercorrelations with MLDR-P. Specifically, MLDR-P-F showed a high positive correlation with pace ( r = 0.95) and a high negative correlation with distance ( r = -0.78), while showing the lowest correlation with frequency ( r = -0.22). Similar results were apparent with MLDR-P-M as the pace ( r = 0.98), distance ( r = -0.67), and frequency ( r = -0.18). Furthermore, it is worth noting that pace shows a high negative correlation with distance ( r = -0.90, for females, r = -0.75, for males).
Table 5 shows the results of the statistical analysis of the regression model with MLDR-P as the dependent variable. All three components of Fun Running can significantly explain the MLDR-P, be it MLDR-P-F (R 2 = 0.95, p < 0.05) or MLDR-P-M (R 2 = 0.96, p < 0.05). Amongthe components, pace demonstrated the greatest impact on MLDR-P (β = 1.39, for females; β = 1.09, for males), followed by distance (β = 0.49, for females; β = 0.15, for males), and frequency demonstrates the smallest impact on MLDR-P (β = -0.03, for all).
Table 6 shows the minimum frequency, pace, and distance needed for each level of MLDR-P. According to the Chinese “Physical Health Test Standards for College Students“ [ 26 ] and regression equation (Fig. 1 ), we calculated the minimum training load needed to elicit a change in each level of middle and long-distance running performance.
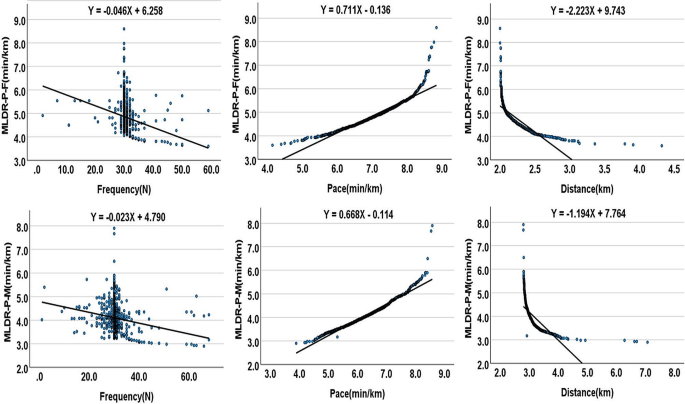
Regression equation between fun running components and MLDR-P
This study fills the gap in research on the dose-response relationship between running and aerobic fitness among college students in the post-epidemic era. A pre-post study design was performed by comparing the changes in MLDR-P before and after a 15-week Fun Running program. The key finding was that 15-week Fun Running can significantly improve the MLDR-P. Furthermore, the pace of Fun Running had the highest correlation with changes in MLDR-P, followed by the distance covered, while frequency displayed the weakest correlation.
Previous studies have shown that long-term regular aerobic exercise, such as swimming, cycling and running can significantly improve aerobic endurance [ 28 , 29 , 30 , 31 , 32 ]. Long-term regular swimming is effective in improving aerobic fitness [ 28 , 29 ], and as a low-impact exercise, it is particularly popular with obese people, the elderly, and people with arthritis because it does not involve weight-bearing [ 33 ]. Similarly, cycling has shown a positive correlation with aerobic fitness, and it can not only improve aerobic fitness but also promote overall health [ 30 , 31 , 32 ]. Consistent with these results, our study indicated that a 15-week Fun Running program had a significant positive impact on the MLDR-P of college students. Long-term aerobic activities can enhance the body’s capacity to inhale, convey, and utilize oxygen [ 34 ], as well as improve the body’s tolerance to lactic acid [ 35 ], thus promoting exercise continuity and improving efficiency [ 18 ]. Exercise performance improves along with improvements in exercise efficiency [ 36 ]. In our study, the MLDR-P was decreased by 0.11 min/km for females and 0.09 min/km for males following the Fun Running program, which implies that female individuals saved 6.8 s and male individuals saved 6.6 s in their middle and long-distance running performance. These findings suggest that the Fun Running program can be used as a means to improve the aerobic fitness of college students in the post-pandemic era.
We also found that all three components of the Fun Running are significantly related to the MLDR-P and can significantly explain it. This is not the same as previous research suggesting that benefits can be achieved by modifying any of the components of exercise [ 37 , 38 , 39 ]. Our results revealed that pace exhibited the highest correlation with changes in MLDR-P, followed by distance, and frequency had the lowest correlation. More importantly, we observed a highly negative correlation between the pace of Fun Running and distance, meaning that engaging in long-distance running may decrease the pace, which may not be conducive to improving the MLDR-P. By comparing the standardized regression coefficients obtained from our regression analysis, we found that for every unit increase in pace, distance, and frequency, the MLDR-P improved by 1.39, 0.49, 0.03 for females and 1.09, 0.15, 0.15 for males. These results suggest that when seeking to develop an effective Fun Running program, focus should be placed on pace, although we acknowledge that more distance and frequency may also be advantageous.
The results also indicated that the minimum Fun Running load needed to elicit a change in middle and long-distance running performance levels does not align with the established rules of the Fun Running program. These results suggest that a hierarchical set of guidelines for the Fun Running program should be developed, taking into account the varying levels of middle and long-distance running achievements among students. This approach will help enhance the aerobic fitness of all students.
This study presents a comprehensive overview of the practical application of aerobic fitness training methods in college during the post-epidemic era. This study is important for improving the theoretical framework of physical training for students. Moreover, it also has important practical significance for the recovery of students’ physical fitness following public health incidents.
There are two main strengths in this study. (1) The sample size of the study is 3244 college students, which is larger than that of previous studies, making the data more powerful and the results more reliable. (2) Our study has established clear guidelines for the Fun Running program designed specifically for Chinese college students, which will help participants develop effective prescriptions. However, there are some limitations in this study. (1) Limitations of the geographic distribution of the sample. By focusing only on specific regions, we failed to capture the variability in the impact of the COVID-19 outbreak on different regions, which may have affected the broad applicability of the assessment of the effects of the Le Running program. (2) Limitations in the selection of the study population. We focused on the medical college student population and failed to include a broader population of different age groups and professional backgrounds, which limits the general applicability of the findings. (3) In analyzing factors contributing to changes in MLDR-P, our study focused primarily on the Fun Running program while assuming that students did not engage in additional physical activity beyond the program and required physical education classes. This assumption ignored other confounding variables that may have an impact on aerobic fitness, such as an individual’s diet, lifestyle habits, and exercise habits. Future studies should be expanded to multiple regions, involve diverse populations, and use a variety of methods including questionnaires and health monitoring in order to more accurately assess the effects of the Fun Running program on aerobic fitness.
This study aims to provide deep insight into the impact of Fun Running on the aerobic fitness development of college students. Results show that 15 weeks of Fun Running can significantly improve aerobic fitness. Examination of the dose-response relationship between Fun Running and MLDR-P provides practitioners with valuable insights into prescribing aerobic fitness training, allowing them to develop more effective training programs. In the future, how to effectively implement the Fun Running program, which is based on hierarchical rules, is worthy of study regarding the recovery of aerobic fitness for all students.
Data availability
The datasets used and/or analyzed during the current study are available from the corresponding author upon reasonable request.
Abbreviations
Middle and long-distance running pace
Middle and long-distance running pace of female students
Middle and long-distance running pace of male students
Effect size
Means and standard deviations
Pearson correlation coefficient
Unstandardized regression coefficients
Standardized regression coefficient
Determination coefficient
The minimum training load required to elicit the middle and long-distance running performance levels change
Dunton GF, Wang SD, Do B, et al. Early effects of the COVID-19 pandemic on physical activity locations and behaviors in adults living in the United States[J]. Prev Med Rep. 2020;20:101241.
Article PubMed PubMed Central Google Scholar
Savage MJ, James R, Magistro D, et al. Mental health and movement behavior during the COVID-19 pandemic in UK university students: prospective cohort study[J]. Ment Health Phys Act. 2020;19:100357.
Article Google Scholar
Yu H, Song Y, Wang X, et al. The impact of COVID-19 restrictions on physical activity among Chinese university students: a retrospectively matched cohort study[J]. Am J Health Behav. 2022;46(3):294–303.
Article PubMed Google Scholar
Hermassi S, Hayes LD, Salman A, et al. Physical activity, sedentary behavior, and satisfaction with life of university students in Qatar: changes during confinement due to the COVID-19 pandemic[J]. Front Psychol. 2021;12:704562.
Hall G, Laddu DR, Phillips SA, et al. A tale of two pandemics: how will COVID-19 and global trends in physical inactivity and sedentary behavior affect one another?[J]. Prog Cardiovasc Dis. 2021;64:108.
Pinho CS, Caria ACI, Aras Júnior R, et al. The effects of the COVID-19 pandemic on levels of physical fitness[J]. Revista Da Associação Médica Brasileira. 2020;66:34–7.
Park AH, Zhong S, Yang H et al. Impact of COVID-19 on physical activity: a rapid review[J]. J Global Health. 2022; 12.
Sun J, Chang J, Zhu E, et al. Comparative research on the development of college students’ physical fitness based on online physical education during the COVID-19 pandemic[J]. BMC Public Health. 2023;23(1):1–7.
Article CAS Google Scholar
Chaddock L, Pontifex MB, Hillman CH, et al. A review of the relation of aerobic fitness and physical activity to brain structure and function in children[J]. J Int Neuropsychol Soc. 2011;17(6):975–85.
Sugamura K, Keaney JF Jr. Reactive oxygen species in cardiovascular disease[J]. Free Radic Biol Med. 2011;51(5):978–92.
Article CAS PubMed PubMed Central Google Scholar
Gu C, Yan J, Zhao L, et al. Regulation of mitochondrial dynamics by aerobic exercise in cardiovascular diseases[J]. Front Cardiovasc Med. 2022;8:788505.
Kaminsky LA, Arena R, Ellingsen Ø, et al. Cardiorespiratory fitness and cardiovascular disease-the past, present, and future[J]. Prog Cardiovasc Dis. 2019;62(2):86–93.
Katzmarzyk PT, Church TS, Blair SN. Cardiorespiratory fitness attenuates the effects of the metabolic syndrome on all-cause and cardiovascular disease mortality in men[J]. Arch Intern Med. 2004;164(10):1092–7.
Pascatello LS. American College of Sports Medicine position stand. Exercise and hypertension[J]. Med Sci Sports Exerc. 2004;36:533–53.
Google Scholar
Jung AP. The impact of resistance training on distance running performance[J]. Sports Med. 2003;33:539–52.
Souissi W, Bouzid MA, Farjallah MA, et al. Effect of different running exercise modalities on post-exercise oxidative stress markers in trained athletes[J]. Int J Environ Res Public Health. 2020;17(10):3729.
Hottenrott K, Ludyga S, Schulze S. Effects of high intensity training and continuous endurance training on aerobic capacity and body composition in recreationally active runners[J]. J Sports Sci Med. 2021;11(3):483.
Baquet G, Van Praagh E, Berthoin S. Endurance training and aerobic fitness in young people[J]. Sports Med. 2003;33:1127–43.
Tan W, Zhang H, Chen G. Creating a running campus culture and promoting the development of School sports [J]. Chin School Sports. 2022;41(11):85–6.
CAS Google Scholar
Tao Z, Sun X, Zhu E. Effects of 15 weeks of low-intensity aerobic endurance training on middle-distance running performance of college students [J]. J Mudanjiang Normal Univ (Natural Sci Edition). 2021; (02): 61–4.
Fitzpatrick JF, Hicks KM, Hayes PR. Dose–response relationship between training load and changes in aerobic fitness in professional youth soccer players[J]. Int J Sports Physiol Perform. 2018;13(10):1365–70.
Bray MS, Hagberg JM, Perusse L, et al. The human gene map for performance and health-related fitness phenotypes: the 2006–2007 update[J]. Med Sci Sports Exerc. 2009;41(1):34–72.
Haskell WL, Lee IM, Pate RR, et al. Physical activity and public health: updated recommendation for adults from the American College of Sports Medicine and the American Heart Association[J]. Circulation. 2007;116(9):1081.
Zubin Maslov P, Schulman A, Lavie CJ, et al. Personalized exercise dose prescription[J]. Eur Heart J. 2018;39(25):2346–55.
Wen CP, Wai JPM, Tsai MK, et al. Minimum amount of physical activity for reduced mortality and extended life expectancy: a prospective cohort study[J]. Lancet. 2011;378(9798):1244–53.
Yang H. Research on the implementation of National Student Physical Health standards [J]. Sports Cult Guide. 2014; (04): 127–30.
Hopkins W, Marshall S, Batterham A, et al. Progressive statistics for studies in sports medicine and exercise science[J]. Med + Sci Sports + Exerc. 2009;41(1):3.
Omar JS, Jaradat N, Qadoumi M et al. Regular swimming exercise improves metabolic syndrome risk factors: a quasi-experimental study[J]. BMC Sports Science, Medicine and Rehabilitation. 2021; 13: 1–7.
Kalkan MK, Daglioglu O. The effects of 8-Week aerobic training program on respiratory and circulatory parameters of female swimmers between 12–14 years Old[J]. J Educ Train Stud. 2018;6(12):202–7.
ARIKAN Ş. The Effect of Bicycle Training Program on aerobic and anaerobic Performance[J]. Turkish J Sport Exerc. 2020;22(1):19–23.
Oja P, Titze S, Bauman A, et al. Health benefits of cycling: a systematic review[J]. Scand J Med Sci Sports. 2011;21(4):496–509.
Article CAS PubMed Google Scholar
Lo GH, Ikpeama UE, Driban JB, et al. Evidence that swimming may be protective of knee osteoarthritis: data from the osteoarthritis initiative[J]. PM&R. 2020;12(6):529–37.
Yázigi F, Espanha M, Vieira F, et al. The PICO project: aquatic exercise for knee osteoarthritis in overweight and obese individuals[J]. BMC Musculoskelet Disord. 2013;14:1–14.
Laurin JL, Reid JJ, Lawrence MM, et al. Long-term aerobic exercise preserves muscle mass and function with age[J]. Curr Opin Physiol. 2019;10:70–4.
Scheer V, Tiller NB, Doutreleau S, et al. Potential long-term health problems associated with ultra-endurance running: a narrative review[J]. Sports Med. 2022;52(4):725–40.
Conley KE. Mitochondria to motion: optimizing oxidative phosphorylation to improve exercise performance[J]. J Exp Biol. 2016;219(2):243–9.
Miller FL, O’Connor DP, Herring MP et al. Exercise dose, exercise adherence, and associated health outcomes in the TIGER study[J]. Med Sci Sports Exerc. 2014, 46(1).
Foulds HJA, Bredin SSD, Charlesworth SA, et al. Exercise volume and intensity: a dose–response relationship with health benefits[J]. Eur J Appl Physiol. 2014;114:1563–71.
Nilsen TS, Scott JM, Michalski M, et al. Novel methods for reporting of exercise dose and adherence: an exploratory analysis[J]. Med Sci Sports Exerc. 2018;50(6):1134.
Download references
Acknowledgements
We sincerely express our gratitude to all participants.
This research was supported by the Youth Fund for Humanities and Social Sciences Research, Ministry of Education (21YJC890052); Key Projects of the Support Program for Outstanding Young Talents in Colleges and Universities (gxyqZD2021019); Teaching Reform Project of the Anhui Provincial Department of Education (Grant Number: 2021jyxm1604); Quality Engineering Teaching and Research Project of Anhui Province (2022jyxm1691); Scientific Research Project of Wannan Medical College (Grant Number: WKS2022Z07); Teaching Reform Project of Wannan Medical College (Grant Number: 2021jyxm20; 2022jbgs01); The funder had no role in the design of the study, collection, analysis, and interpretation of data or in writing the manuscript.
Author information
Jun Sun and Ergang Zhu authors contributed equally to this work.
Authors and Affiliations
Department of Public Foundation, Wannan Medical College, 241000, Wuhu, China
Zhixuan Tao, Xugui Sun, Jun Sun & Ergang Zhu
You can also search for this author in PubMed Google Scholar
Contributions
TXZ and ZEG participated in manuscript drafting and study design. TZX, SJ, and SXG participated in data collection and statistical analysis. All the authors agreed on the final manuscript.
Corresponding authors
Correspondence to Jun Sun or Ergang Zhu .
Ethics declarations
Ethics approval and consent to participate.
All subjects gave their informed consent for inclusion before participating in the study. The study was approved by the Research Ethics Review Committee of Wannan Medical College (Reference Number: 2024-009), 241000, Wuhu, China.
Consent for publication
Not applicable.
Competing interests
The authors declare no competing interests.
Additional information
Publisher’s note.
Springer Nature remains neutral with regard to jurisdictional claims in published maps and institutional affiliations.
Rights and permissions
Open Access This article is licensed under a Creative Commons Attribution 4.0 International License, which permits use, sharing, adaptation, distribution and reproduction in any medium or format, as long as you give appropriate credit to the original author(s) and the source, provide a link to the Creative Commons licence, and indicate if changes were made. The images or other third party material in this article are included in the article’s Creative Commons licence, unless indicated otherwise in a credit line to the material. If material is not included in the article’s Creative Commons licence and your intended use is not permitted by statutory regulation or exceeds the permitted use, you will need to obtain permission directly from the copyright holder. To view a copy of this licence, visit http://creativecommons.org/licenses/by/4.0/ . The Creative Commons Public Domain Dedication waiver ( http://creativecommons.org/publicdomain/zero/1.0/ ) applies to the data made available in this article, unless otherwise stated in a credit line to the data.
Reprints and permissions
About this article
Cite this article.
Tao, Z., Sun, X., Sun, J. et al. Dose-response relationship between 15 weeks of running and aerobic fitness: a retrospective analysis based on the fun running program. BMC Public Health 24 , 1019 (2024). https://doi.org/10.1186/s12889-024-18484-z
Download citation
Received : 30 October 2023
Accepted : 28 March 2024
Published : 12 April 2024
DOI : https://doi.org/10.1186/s12889-024-18484-z
Share this article
Anyone you share the following link with will be able to read this content:
Sorry, a shareable link is not currently available for this article.
Provided by the Springer Nature SharedIt content-sharing initiative
- Dose-response relationship
- Fun running
- Aerobic fitness
- College students
- Post-epidemic era
BMC Public Health
ISSN: 1471-2458
- Submission enquiries: [email protected]
- General enquiries: [email protected]
15 Reasons Teachers Say Social Media Isn’t All Bad for Students

- Share article
Social media is the bane of many educators’ existence.
The platforms distract students from paying attention in class, make it harder for kids to communicate in-person, keep teens feeling isolated, and hurt students’ overall self-confidence, educators say.
But many teachers and school and district leaders do see some positive impact from the apps and platforms that they otherwise disdain.
One big bright spot: social media gives students a chance to pick up knowledge or delve into topics that they aren’t learning about in school. Thirty percent of educators surveyed by the EdWeek Research Center in December and January identified that learning opportunity as a positive outcome of social media use.
“Social media is a place where young people do engage in some self-directed and informal learning,” said Jeff Carpenter, a professor of education at Elon University who studies social media in education, who is also a former high school teacher. “And I think [for] teachers, there’s interest in encouraging students not just to learn within the confines of the school building, not just to learn about the things that teachers tell them they have to learn about, but to realize that you can learn outside of the school context, you can learn [about] topics that aren’t assigned to you. Follow your passions. Explore things.”
Nearly another quarter of educators surveyed said their students have been able to go deeper into topics they are learning about in school through social media, developed positive friendships, improved their creative skills, or received recognition or praise for their accomplishments.
Another quarter of educators surveyed said they couldn’t think of a single positive development arising from students’ social media use. The nationally representative survey of 595 educators was conducted from Dec. 21, 2023 to Jan. 2, 2024.
While Stefanie McKoy, a special education teacher at Branson Middle School in Missouri, understands why some teachers find social media nothing but problematic, her own perspective is more nuanced.
“Social media is a way for students to connect to the world,” said McKoy, who also works as a lecturer at University of Arkansas and studied social media platforms while pursuing her doctorate . “It gives students power, and a sense of choice. … I think what draws students to social media is that they’re like, ‘Oh, hey, you know, I can purposely look at this,’ or, ‘Hey, there’s another student that looks like me, but maybe they’re at a different school.’ And I think that that’s really great.”
Still, McKoy thinks students need help in navigating social media platforms safely and with a critical eye.
“I think that we have to do a better job at preparing our students for social media use,” she said. “And we as educators haven’t necessarily caught up to that yet.”
Sign Up for EdWeek Tech Leader
Edweek top school jobs.

Sign Up & Sign In

Some graduation requirements waived for WA’s class of 2024
Washington school districts will be able to keep waiving certain graduation requirements for high school students in the class of 2024.
School officials asked the State Board of Education to continue allowing them to waive requirements for the state’s “graduation pathways” for the 2023-2024 school year, citing the lasting impact of COVID-19 on students.
The board granted an extension to the emergency waiver Thursday.
State lawmakers established an emergency waiver program in 2021 to prevent delays in graduating for students impacted by “unforeseen disruptions to coursework and assessments that are beyond the student’s control.”
The waiver originally allowed districts to waive up to two credits and the graduation pathway requirement for individual students until the 2022-2023 school year. In the 2023-2024 school year, districts could waive up to one credit.
In 2022, nearly 13% of students used a waiver and 8% used the pathway waiver, according to a state research brief.
In a School Counselor Association survey of members from over 70 districts in the state, 94% said they had students who would not graduate without the pathway requirement waiver.
Board members initially expressed hesitation about extending the waiver, suggesting that it was difficult to figure out the right balance between academic excellence and student well-being.
New limitations developed for the 2024 waiver extension are meant to address some of these concerns by attempting to ensure students show competency in math or English either through earning credits or meeting a graduation pathway requirement.
Under the board’s adopted rules , a student can receive an emergency waiver of up to one math credit only if the student meets a graduation pathway option in math — and vice versa. The same goes for English credits. A student can only have the pathway requirement waived in both English and math if no credits in English or math are waived.
Most Read Local Stories
- Seattle Public Library resorting to rolling branch closures
- Surge in electricity demand spells trouble for PNW, forecasts show VIEW
- Expect Seattle-area I-5 backups this weekend, but there are ways around them
- Wrong-way crash near Lynnwood kills 1, injures 2 after police chase
- Families of 2 killed at Beyond Wonderland sue music festival organizer
The opinions expressed in reader comments are those of the author only and do not reflect the opinions of The Seattle Times.
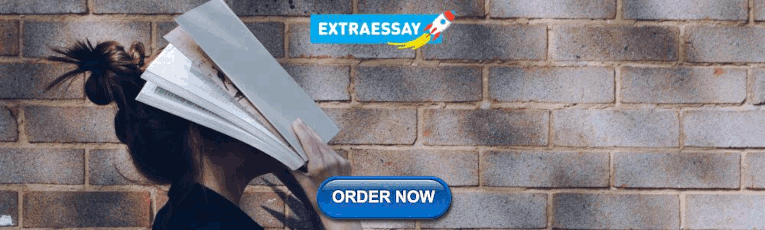
IMAGES
COMMENTS
This work attempts to synthetize existing research about the impact of Covid-19 school closure on student achievement. It extends previous systematic reviews and meta-analyses by (a) using a more balanced sample in terms of country composition, (b) considering new moderators (type of data and research design), and (c) including studies on tertiary education students in addition to primary and ...
As we outline in our new research study released in January, the cumulative impact of the COVID-19 pandemic on students' academic achievement has been large. We tracked changes in math and ...
In almost all grades, the majority of students made some learning gains in both reading and math since the COVID-19 pandemic started, though gains were smaller in math in 2020 relative to the ...
The COVID-19 pandemic has had a notable impact on the landscape of online teaching and learning (Aldowah et al., 2019; Basilaia and Kvavadze, 2020; Khan and Abdou, 2021).Notably, Rameez et al ...
Taken together, although prior research has shown varying levels of impact of the COVID-19 pandemic on student learning, it is evident that student learning was disrupted, leading to short-term and long-term detrimental effects on student achievement and educational attainment. ... Keywords: COVID-19, survey research, elementary school ...
A number of narrative reviews have sought to summarize the emerging research on COVID-19 and ... Fray, L., Miller, A., Harris, J. & Taggart, W. The impact of COVID-19 on student learning in New ...
The research did not rely on any kind of experiments on humans and/or the use of human tissue samples. ... French, J., Araya, M. P. U. & Zafar, B. The impact of COVID-19 on student experiences and ...
For Black students, the number spikes to 25 percent. "There are many reasons to believe the Covid-19 impacts might be larger for children in poverty and children of color," Kuhfeld wrote in the study. Their families suffer higher rates of infection, and the economic burden disproportionately falls on Black and Hispanic parents, who are less ...
NATIONAL BUREAU OF ECONOMIC RESEARCH 1050 Massachusetts Avenue Cambridge, MA 02138 June 2020 Noah Deitrick and Adam Streff provided excellent research assistance. All errors that remain are ... The Impact of COVID-19 on Student Experiences and Expectations: Evidence from a Survey Esteban M. Aucejo, Jacob F. French, Maria Paola Ugalde Araya, and ...
Understanding these impacts and how best to support students' social and emotional needs after the huge disruption of COVID-19 will be essential. Many students may face greater food insecurity ...
Since the outbreak of Covid-19, research has shown the psychological impact of the pandemic on university students and discussed the coping solutions. For instance, disruptions in academic processes due to Covid-19 pandemic have increased student anxiety ( Wang et al., 2020 ), especially for those without adequate social support ( Cao et al ...
Previous research shows that disparities based on race and ethnicity and sex ... O'Hare G, Burgess M. The hidden impact of COVID-19 on child protection and wellbeing. London, UK: Save the Children International; 2020. ... and schoolwork difficulty among high school students during COVID-19 pandemic, by sex, race and ethnicity, and grade ...
IntroductionThe COVID-19 pandemic brought profound societal changes and disruptions, including in the education system, which underwent swift modifications. It presented unique challenges for both professors and students, contributing to an increase in the prevalence of mental health-related symptoms.ObjectiveTo determine the prevalence of mental health disorders symptoms, coping strategies ...
Introduction. The global outbreak of COVID-19 has certainly taken an overwhelming toll on everyone. People have lost their jobs, their homes, and even their lives. There is no getting past the fact that the overall impact on the world has been negative, but it is important to realize that positive aspects of the pandemic have been overshadowed ...
According to some research findings, with the outbreak of COVID-19, senior students were intensely worried about their future careers due to having to postpone their career plans, the increase in competition in the field of business and higher education as a result of the global epidemic, and the decrease in recruitment (David et al., 2021 ...
The negative effects that COVID-19 has had on education could impact students for many years to come. The loss of learning that the pandemic has caused students could lead to a decrease in wages they earn in the future, a lower national GDP, and also make it harder for students to find jobs. Students who are affected by COVID-19 could have a ...
First, there are students who experience average-quality remote learning; this group continues to progress, but at a slower pace than if they had remained in school. 4 High-quality remote-learning programs are typically the result careful planning and deliberate approaches—which were not typical of the COVID-19 transition. Second, some ...
The present research aimed to analyse students' attainment during the COVID-19 pandemic by using student performance data. We focused on the relationship between participation in online courses ...
Past research underscored the pivotal role the attitude displayed by teachers can have when considering school inclusion as a critical element in securing an efficient integration of students with ... The impact of COVID-19 on student equity and inclusion: Supporting vulnerable students during school closures and school re-openings ...
The COVID-19 pandemic has affected a variety of researchers, students and academics. As institutions of higher education have limited in-person activities, research and training have been disrupted. Many graduate students have faced new barriers as a result (Chenneville and Schwartz-Mette, 2020; Thompson, 2020).
In the wake of the COVID-19 pandemic, students across the globe have experienced a considerable decrease in their physical activities [1,2,3].Additionally, the widespread adoption of online courses has further exacerbated the sedentary behavior of students during the pandemic [4, 5].Consequently, there has been a significant decline in the physical fitness of students [6, 7], particularly in ...
The research is based on a mixed-methods design encompassed in wider research, which considers quantitative and qualitative methods. ... There is a Covid-19 impact on the frequency with which teachers used digital tools. ... there are few scientific studies that assess the impact of their use on teachers and students beyond Covid-19 solutions ...
Research addendum: Student mobility to Japan in the age of COVID-19: a matter of degree Lauren Nakasato KAYASHIMA Nobuko Revisiting student mobility to Japan beyond COVID-19 In the four years since the World Health Organization declared a global public health emergency due to the COVID-19 pandemic, the virus has claimed over 7 million lives
Many educators do see some positive impact from social media. For instance, the apps and platforms enable informal learning. 15 Reasons Teachers Say Social Media Isn't All Bad for Students
School officials asked to continue allowing waived requirements for the 2023-2024 school year, citing the lasting impact of COVID-19 on students. ... according to a state research brief.