Use the
Natural Rate
Median Estimate (%) | Low (%) | High (%) | 1996:Q3 | 62 | 5.65 | 5.00 | 6.00 |
1997:Q3 | 59 | 5.25 | 4.50 | 5.88 |
1998:Q3 | 45 | 5.30 | 4.50 | 5.80 |
1999:Q3 | 43 | 5.00 | 4.13 | 5.60 |
2000:Q3 | 48 | 4.50 | 4.00 | 5.00 |
2001:Q3 | 34 | 4.88 | 3.50 | 5.50 |
2002:Q3 | 50 | 5.10 | 3.80 | 5.50 |
2003:Q3 | 41 | 5.00 | 4.31 | 5.40 |
2004:Q3 | 46 | 5.00 | 4.00 | 5.50 |
2005:Q3 | 50 | 5.00 | 4.25 | 5.50 |
2006:Q3 | 53 | 4.95 | 4.00 | 5.50 |
2007:Q3 | 52 | 4.65 | 4.20 | 5.50 |
2008:Q3 | 48 | 5.00 | 4.00 | 5.50 |
2009:Q3 | 45 | 5.00 | 4.00 | 6.00 |
2010:Q3 | 50 | 5.78 | 4.50 | 6.80 |
2011:Q3 | 42 | 6.00 | 4.75 | 7.00 |
2012:Q3 | 49 | 6.00 | 4.75 | 7.00 |
2013:Q3 | 63 | 6.00 | 4.75 | 7.00 |
2014:Q3 | 65 | 5.50 | 4.50 | 6.70 |
2015:Q3 | 62 | 5.00 | 4.25 | 5.80 |
2016:Q3 | 56 | 4.80 | 4.50 | 5.50 |
2017:Q3 | 44 | 4.50 | 3.50 | 5.00 |
2018:Q3 | 34 | 4.30 | 3.80 | 4.60 |
2019:Q3 | 33 | 4.10 | 3.88 | 4.60 |
2020:Q3 | 48 | 4.10 | 3.50 | 6.00 |
2021:Q3 | 37 | 3.78 | 3.00 | 4.25 |
2022:Q3 | 30 | 4.10 | 3.50 | 4.50 |
2023:Q3 | 42 | 4.00 | 3.75 | 4.55 |
2024:Q3 | 44 | 4.40 | 3.50 | 5.16 |
Technical Note Moody's Aaa and Baa Historical Rates The historical values of Moody's Aaa and Baa rates are proprietary and, therefore, not available in the data files on the Bank’s website or on the tables that accompany the survey’s complete write-up in the PDF.
The Federal Reserve Bank of Philadelphia thanks the following forecasters for their participation in recent surveys:
William Adams , Comerica Bank; Ed Al-Hussainy and Alexander Spitz , Columbia Threadneedle Investments; Scott Anderson and Doug Porter, BMO Capital Markets; Robert J. Barbera , Johns Hopkins University Center for Financial Economics; Peter Bernstein , RCF Economic and Financial Consulting, Inc.; Wayne Best and Michael Brown , Visa, Inc.; Jay Bryson , Wells Fargo; Seth Carpenter , Morgan Stanley; Christine Chmura , Ph.D. , and Xiaobing Shuai , Ph.D. , Chmura Economics & Analytics; Gary Ciminero , CFA , GLC Financial Economics; Grant Collins , AIM Research, LLC; Rajeev Dhawan , Georgia State University; Bill Diviney , ABN AMRO Bank NV; Gabriel Ehrlich , Daniil Manaenkov , and Yinuo Zhang , RSQE, University of Michigan; Michael R. Englund , Action Economics, LLC; Michael Feroli , J.P. Morgan; Tani Fukui and Shan Ahmed , MetLife Investment Management; Sacha Gelfer , Bentley University; James Glassman , Independent Economist; Jan Hatzius , Goldman Sachs; Steve Kihm , Citizens Utility Board of Wisconsin; Yaniv Konchitchki , University of California, Berkeley; Thomas Lam , Independent Economist (Singapore); Brian Martin , Australia New Zealand Bank (ANZ); Robert McNab , Old Dominion University; R. Anthony Metz , Pareto Optimal Economics, LLC; R. M. Monaco , TitanRM; Joel L. Naroff , Naroff Economics, LLC; Nomura Securities International ; Brendon Ogmundson , BC Real Estate Association; Perc Pineda, Ph.D. , Plastics Industry Association; Joel Prakken and Chris Varvares , S&P Global Market Intelligence; Jason Prole , Capital Risk Management; Michael Roberts , Dan Roberts , and Jeffrey Baldwin , Roberts Capital Advisors, LLC; Parker Ross , Arch Capital Group; Philip Rothman , East Carolina University; Allen Sinai and Lu Yu , Decision Economics, Inc.; Sean Snaith , University of Central Florida; Stephen Stanley , Santander US Capital Markets; Charles Steindel , Editor, NABE Business Economics ; Susan M. Sterne , Economic Analysis Associates, Inc.; Edward Sullivan , Portland Cement Association; Ryan Sweet , Oxford Economics USA, Inc.; Jordan Vickers and Maira Trimble , Eaton Corporation; Lawrence Werther , Daiwa Capital Markets America; Mark Zandi , Moody’s Analytics.
This is a partial list of participants. We also thank those who wish to remain anonymous.
Return to the main page for the Survey of Professional Forecasters .
Youth Unemployment High in South Asia
Youth unemployment is being cited as one of the core drivers of the unrest in Bangladesh , which led to weeks of protests and Prime Minister Sheikh Hasina stepping down from office.
The following chart, based on ILO data , shows how labor force unemployment for people aged 15-24 years in Bangladesh stood at 15.7 percent in 2023, above the world average for youth unemployment of 13.8 percent and the low and middle income average of 14.1 percent. Youth unemployment is a regional issue, with India having hit a similar level in 2023, while Nepal and Sri Lanka’s rates last year were worse, both surpassing the 20-percent mark. Of this selection of countries, Pakistan fared better in 2023 at around 9.7 percent.
According to a report by the Japan Times, the latest figures indicate that in 2024, roughly 40 percent of Bangladeshi youth are not in education, employment or training, including those no longer looking for work or registered unemployed. The authors write that stagnant job growth in the private sector as well as a cooling economy has made public sector jobs more attractive. Protests started weeks ago over a quota for such civil service jobs which reserved 30 percent of government roles to relatives of veterans of the 1971 war of independence from Pakistan.
While all of the countries' unemployment rates have fallen from a pandemic-induced peak, they have in all five cases risen in the past decade.
Description
This chart shows the share of youth labor force who are unemployed in a selection of South Asian countries, by year.
Can I integrate infographics into my blog or website?
Yes, Statista allows the easy integration of many infographics on other websites. Simply copy the HTML code that is shown for the relevant statistic in order to integrate it. Our standard is 660 pixels, but you can customize how the statistic is displayed to suit your site by setting the width and the display size. Please note that the code must be integrated into the HTML code (not only the text) for WordPress pages and other CMS sites.
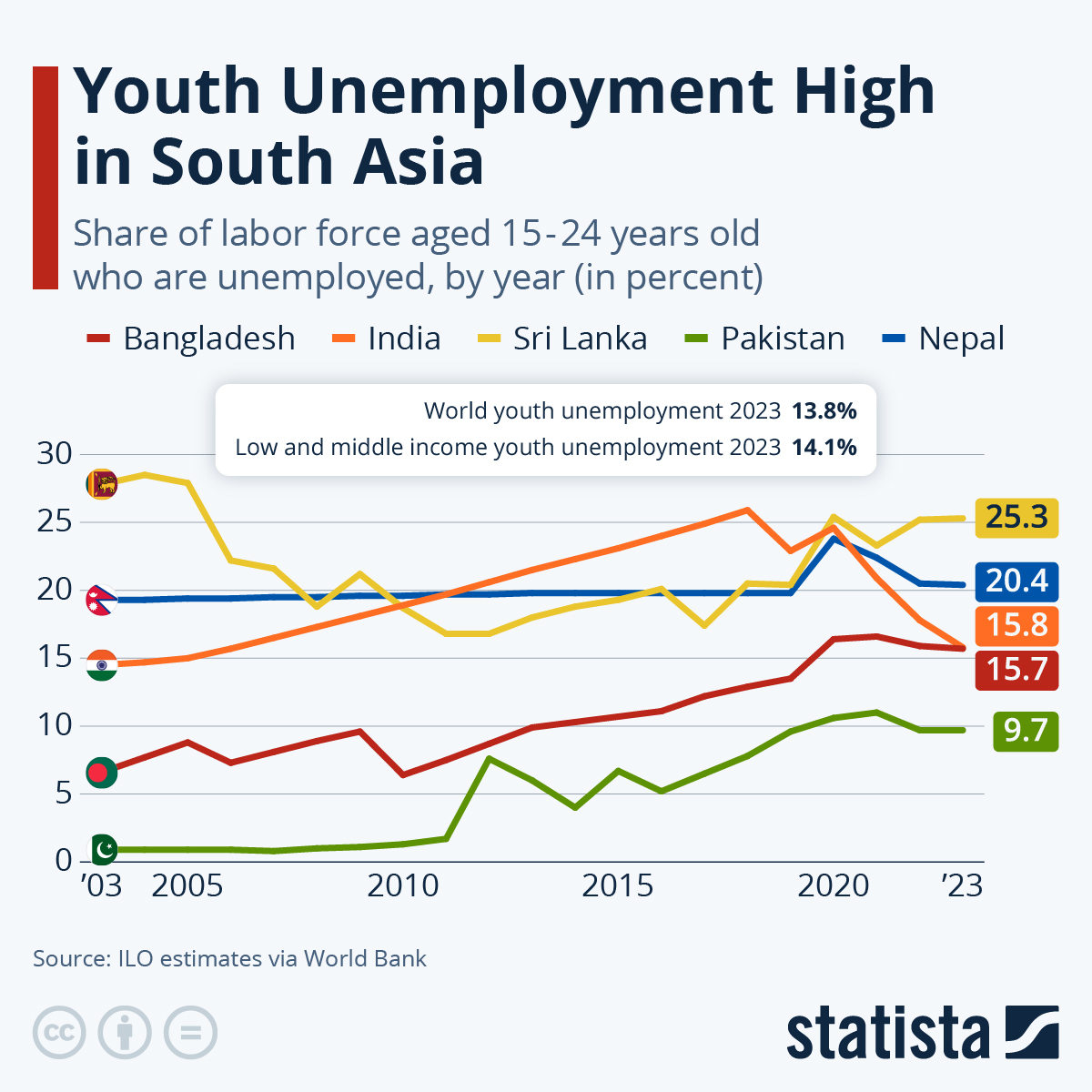
Infographic Newsletter
Statista offers daily infographics about trending topics, covering: Economy & Finance , Politics & Society , Tech & Media , Health & Environment , Consumer , Sports and many more.
Related Infographics
Indian budget, how big is india's new jobs plan, international politics, u.s. image deteriorates in parts of asia, heavy monsoon rains hit india one month into season, forced labor & human trafficking, which region has the highest number of child laborers, indian worker satisfaction tops regional average, what the u.s. is most worried about, child poverty, 1 in 7 children live in poverty in the u.s., where most people were internally displaced in 2023, world’s most pessimistic nation, gloomy greeks remain the world’s most pessimistic nation, inflation in the global economy, stubborn inflation fuels fears of 'no landing' scenario, the employment gap for people with disabilities, citizenship amendment act, india sees relatively few refugees from caa countries.
- Who may use the "Chart of the Day"? The Statista "Chart of the Day", made available under the Creative Commons License CC BY-ND 3.0, may be used and displayed without charge by all commercial and non-commercial websites. Use is, however, only permitted with proper attribution to Statista. When publishing one of these graphics, please include a backlink to the respective infographic URL. More Information
- Which topics are covered by the "Chart of the Day"? The Statista "Chart of the Day" currently focuses on two sectors: "Media and Technology", updated daily and featuring the latest statistics from the media, internet, telecommunications and consumer electronics industries; and "Economy and Society", which current data from the United States and around the world relating to economic and political issues as well as sports and entertainment.
- Does Statista also create infographics in a customized design? For individual content and infographics in your Corporate Design, please visit our agency website www.statista.design
Any more questions?
Get in touch with us quickly and easily. we are happy to help.
Feel free to contact us anytime using our contact form or visit our FAQ page .
Statista Content & Design
Need infographics, animated videos, presentations, data research or social media charts?
More Information
The Statista Infographic Newsletter
Receive a new up-to-date issue every day for free.
- Our infographics team prepares current information in a clear and understandable format
- Relevant facts covering media, economy, e-commerce, and FMCG topics
- Use our newsletter overview to manage the topics that you have subscribed to
Skip to content

Labour Market Report August 2024
Date published: 13 August 2024
The latest labour market statistics were published today (13th August 2024) by the Northern Ireland Statistics & Research Agency.
Payrolled employees increased over the month and median earnings decreased
- The number of employees receiving pay through HMRC PAYE in NI in July 2024 was 807,700, an increase of 0.1% over the month and 2.3% over the year.
- Earnings data from HMRC PAYE indicated that NI employees had a median monthly pay of £2,249 in July 2024, a decrease of £82 (3.5%) over the month and an increase of £164 (7.9%) over the year.
- When considering the annual change in median monthly pay by industry sector, the largest percentage increases over the year were recorded in the ‘Education’ (11.9%), ‘Other service activities’ (10.9%), ‘Agriculture, forestry and fishing’ (9.6%) and ‘Arts, entertainment and recreation’ (9.2%) sectors. These sectors all had median monthly earnings below the NI average.
- The estimates from HMRC PAYE for the latest period, are based on early data and, therefore, are more likely to be subject to larger revisions.
Increase in the seasonally adjusted claimant count rate over the month
- In July 2024, the seasonally adjusted number of people on the claimant count was 41,000 (4.2% of the workforce), an increase of 6.1% from the previous month’s revised figure. The July 2024 claimant count remains 37.4% higher than the pre-pandemic count in March 2020. These increases are largely due to the increase in the administrative earnings threshold for Universal Credit in May 2024.
Proposed redundancies lower than previous year while Confirmed redundancies almost double
- NISRA, acting on behalf of the Department for the Economy, received confirmation that 40 redundancies occurred in July 2024. Over the year August 2023 to July 2024, 2,550 redundancies were confirmed, which was almost double the figure for the previous year (1,340).
- There were 2,820 redundancies proposed in the twelve months to July 2024, which was around three quarters of the figure for the previous year (3,940).
Labour Force Survey headline measures
- The latest NI seasonally adjusted unemployment rate (the proportion of economically active people aged 16 and over who were unemployed) for the period April-June 2024 was estimated from the Labour Force Survey at 1.9%. This was a decrease of 0.2 percentage points (pps) over the quarter and a decrease of 0.7pps over the year.
- The proportion of people aged 16 to 64 in work (the employment rate) decreased by 0.3pps over the quarter and increased by 1.2pps over the year to 71.6%.
- The total number of weekly hours worked in NI was estimated at 29.0 million hours, an increase of 2.2% on the previous quarter and an increase of 2.7% on the equivalent period last year.
- The economic inactivity rate (the proportion of people aged 16 to 64 who were not working and not seeking or available to work) increased by 0.5pps over the quarter and decreased by 0.7pps over the year to 27.1%.
The statistical bulletin and associated tables are available on the Labour Market Report - August 2024 page.
Latest news
- Labour Market Report August 2024 13 August 2024
- Weekly deaths tables – week ending 2 August 2024 09 August 2024
- Census 2021 update 08 August 2024
- Weekly deaths tables – week ending 26 July 2024 02 August 2024
More news …
Skip to content

Northern Ireland Labour Market Statistics
Date published: 13 August 2024
The labour market statistics were published today by the Northern Ireland Statistics & Research Agency.

Payrolled employees increased over the month and median earnings decreased
- The number of employees receiving pay through HMRC PAYE in NI in July 2024 was 807,700, an increase of 0.1% over the month and 2.3% over the year.
- Earnings data from HMRC PAYE indicated that NI employees had a median monthly pay of £2,249 in July 2024, a decrease of £82 (3.5%) over the month and an increase of £164 (7.9%) over the year.
- When considering the annual change in median monthly pay by industry sector, the largest percentage increases over the year were recorded in the ‘Education’ (11.9%), ‘Other service activities’ (10.9%), ‘Agriculture, forestry and fishing’ (9.6%) and ‘Arts, entertainment and recreation’ (9.2%) sectors. These sectors all had median monthly earnings below the NI average.
- The estimates from HMRC PAYE for the latest period, are based on early data and, therefore, are more likely to be subject to larger revisions.
Increase in the seasonally adjusted claimant count rate over the month
- In July 2024, the seasonally adjusted number of people on the claimant count was 41,000 (4.2% of the workforce), an increase of 6.1% from the previous month’s revised figure. The July 2024 claimant count remains 37.4% higher than the pre-pandemic count in March 2020. These increases are largely due to the increase in the administrative earnings threshold for Universal Credit in May 2024.
Proposed redundancies lower than previous year while Confirmed redundancies almost double
- NISRA, acting on behalf of the Department for the Economy, received confirmation that 40 redundancies occurred in July 2024. Over the year August 2023 to July 2024, 2,550 redundancies were confirmed, which was almost double the figure for the previous year (1,340).
- There were 2,820 redundancies proposed in the twelve months to July 2024, which was around three quarters of the figure for the previous year (3,940).
Labour Force Survey headline measures
- The latest NI seasonally adjusted unemployment rate (the proportion of economically active people aged 16 and over who were unemployed) for the period April-June 2024 was estimated from the Labour Force Survey at 1.9%. This was a decrease of 0.2 percentage points (pps) over the quarter and a decrease of 0.7pps over the year.
- The proportion of people aged 16 to 64 in work (the employment rate) decreased by 0.3pps over the quarter and increased by 1.2pps over the year to 71.6%.
- The total number of weekly hours worked in NI was estimated at 29.0 million hours, an increase of 2.2% on the previous quarter and an increase of 2.7% on the equivalent period last year.
- The economic inactivity rate (the proportion of people aged 16 to 64 who were not working and not seeking or available to work) increased by 0.5pps over the quarter and decreased by 0.7pps over the year to 27.1%.
- The latest Labour Market release shows that over the year both payrolled employee numbers and earnings have increased. In addition, all the Labour Force Survey headline measures have improved over the year, with the unemployment and economic inactivity rates both decreasing and the employment rate increasing.
- The latest HMRC payroll data shows that payrolled employees increased by 0.1% over the month and by 2.3% over the year. Payrolled earnings decreased by 3.5% over the month and were 7.9% higher than July 2023.
- Households reported, via the Labour Force Survey (LFS), over the year to April-June 2024, a 1.2pps increase in the employment rate (to 71.6%) and decreases of 0.7pps in both the economic inactivity rate (to 27.1%) and the unemployment rate (to 1.9%). None of these annual changes were statistically significant.
- The total number of hours worked in April-June 2024 increased by 2.7% over the year, to 29.0 million hours per week. This is 0.6% below the pre-pandemic position recorded in October-December 2019.
- There was an increase of 6.1% in the claimant count estimate over the month to July 2024, from the revised figure for June 2024. The claimant count rate for July 2024 was 4.2%, an increase from the revised rate for June 2024 (3.9%). These increases are largely due to the increase in the administrative earnings threshold for Universal Credit in May 2024.
- Finally, in July 2024, the Department was notified of 40 confirmed redundancies, bringing the rolling twelve-month total of confirmed redundancies to 2,550, almost double the figure for the previous year (1,340). Although the rolling twelve-month total of confirmed redundancies is substantially higher than that of the previous year, it is similar to the levels seen in the decade preceding the pandemic. Over the year, there were 2,820 proposed redundancies reported to the Department, around three quarters of the figure for the previous year (3,940), and below the trend seen immediately before the pandemic.
Notes to editors:
- The statistical report and associated tables are available at:
https://www.nisra.gov.uk/publications/labour-market-report-august-2024
- The Northern Ireland Statistics and Research Agency wishes to thank the participating households and businesses for their co-operation in agreeing to take part in the surveys and for facilitating the collection of the relevant data.
- ‘Over the quarter’ refer to comparisons between the latest quarterly estimates for the period April-June 2024 and the quarter preceding that (i.e. January-March 2024). ‘Over the year’ refer to comparisons between the latest quarterly estimates for the period April-June 2024 and those of the corresponding quarter one year previously (i.e. April-June 2023). Changes that are significant in a statistical sense (i.e. where the estimated change exceeded the variability expected from a sample survey of this size and was likely to reflect real change) are specifically highlighted.
- Estimates relating to April-June 2024 should be compared with the estimates for January-March 2024. This provides a more robust estimate than comparing with the estimates for March-May 2024, as the April and May data are included within both estimates.
- The official measure of unemployment is from the Labour Force Survey. This measure of unemployment relates to people without a job who were available for work and had either looked for work in the last four weeks or were waiting to start a job. This is the International Labour Organisation definition. Labour Force Survey estimates are subject to sampling error. This means that the exact figure is likely to be contained in a range surrounding the estimate quoted. For example, the unemployment rate is likely to fall within 0.6pps of the quoted estimate (i.e. between 1.3% and 2.5%).
- The claimant count is an administrative data source derived from Jobs and Benefits Offices systems, which records the number of people claiming unemployment-related benefits. In March 2018, the NI claimant count measure changed from one based solely on Jobseekers Allowance (JSA) to an experimental measure based on JSA claimants and out-of-work Universal Credit (UC) claimants who were claiming principally for the reason of being unemployed. Those claiming unemployment-related benefits (either UC or JSA) may be wholly unemployed and seeking work or may be employed but with low income and/or low hours, that make them eligible for unemployment-related benefit support. Under UC a broader span of claimants became eligible for unemployment-related benefit than under the previous benefit regime.
- Redundancies are provided by companies under the Employment Rights (Northern Ireland) Order 1996 (Amended 8 October 2006) whereby they are legally required to notify the Department of impending redundancies of 20 or more employees. Companies who propose fewer than 20 redundancies are not required to notify the Department, therefore the figures provided are likely to be an underestimate of total job losses, however, it is not possible to quantify the extent of the shortfall. All other things being equal we would expect more redundancies in sectors dominated by large businesses as they are the businesses that meet the 20 or more collective redundancy criteria.
- To prevent the potential identification of individual businesses, redundancy totals relating to fewer than three businesses are not disclosed. The Statistical Disclosure Control Policy is available here: https://www.nisra.gov.uk/publications/redundancies-background-information . Where the number of businesses does not meet the threshold for release (as detailed in the Statistical Disclosure Control Policy), individual monthly totals are not published.
- HMRC’s Pay As You Earn (PAYE) Real Time Information (RTI) system is an administrative data source. The PAYE RTI system is the system employers use to take Income Tax and National Insurance contributions before they pay wages to employees. These data relate to employees paid by employers only, and do not include self-employment income.
- Estimates of the number of paid employees and employee earnings from PAYE are classed as official statistics in development as they are still in their development phase. As a result, the data are subject to revisions. Early estimates (flash estimates) for July 2024 are based on around 85% of information and will be subject to revision in the next month’s release when between 98% and 99% of data will be available (main estimates). The size of revisions to main and flash estimates are similar for employees, while revisions to earnings flash estimates are typically larger than main estimate revisions. The HMRC PAYE covers the whole population rather than a sample of employees or companies. Data are based on where employees live and not the location of their place of work within the UK. Data are seasonally adjusted but not adjusted for inflation.
- The Labour Market Report will be of interest to policy makers, public bodies, the business community, banks, economic commentators, academics, and the general public with an interest in the local economy.
- The next scheduled release of the Labour Market Report will be published on the NISRA website on Tuesday 10th September 2024.
- Feedback is welcomed and should be addressed to:
Responsible statistician:
Mark McFetridge,
Economic & Labour Market Statistics (ELMS),
[email protected] or Tel: 028 902 55172.
Latest news
- Northern Ireland Labour Market Statistics 13 August 2024
- Murphy announces Assured Skills Welding Academy at South West College 12 August 2024
- Murphy announces Assured Skills Academy with EY 05 August 2024
- Department for the Economy opens consultation on Postgraduate Award Scheme 01 August 2024
More news …
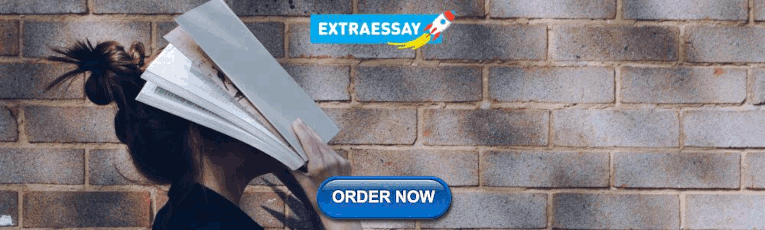
IMAGES
COMMENTS
During the pandemic, teen summer employment hit its lowest point since the Great Recession. Fewer than a third (30.8%) of U.S. teens had a paying job last summer. In 2019, 35.8% of teens worked over the summer. short readsMay 14, 2021.
Here are five facts about how the COVID-19 downturn is affecting unemployment among American workers. The unemployment rate for women in May (14.3%) was higher than the unemployment rate for men (11.9%). This stands in contrast to the Great Recession, when the unemployment rate for women had peaked at 9.4% in July 2010 compared with a peak of ...
[author-bio] The unemployment rate soared from a 50-year low of 3.5 percent to 14.8 percent in April 2020 at the beginning of the COVID-19 pandemic, and then fell faster than many forecasters ...
The unemployment rate in the United States falls slowly in expansions, and it may not reach its previous low point before the next recession begins. Based on this feature, I document that the frequent recessions prior to 1983 are associated with an upward trend in the unemployment rate. In contrast, the long expansions beginning in 1983 are associated with a downward trend.
April 2022, No. 22-12. Following early 2020 responses to the pandemic, labor force participation declined dramatically and has remained below its 2019 level, whereas the unemployment rate recovered briskly. We estimate the trend of labor force participation and unemployment and find a substantial impact of the pandemic on estimates of trend.
This paper presents a simple methodology for real-time unemployment rate projections. at this approach pe. formed considerably better in 2020 at the onset of theCOVID-19 rece. sion. We then provide unemployment projections and an alternative scenari. analysis for 2021 based on the methodology we build using real-time data.
In April 2020, the unemployment rate reached 14.8%—the highest rate observed since data collection began in 1948. In July 2021, unemployment remained higher (5.4%) than it had been in
Answer. 6 points about that: 1) You can use discrete variables, for example, dummys with a value of 1 in certain years and zero in others. 2) Sometimes, the use of the log reduces the problems ...
unemployment rate is about 4.4%. Unemployment and the Broader Economy The unemployment rate is most often used as a measure of labor market strength, but it is also a useful indicator and predictor of the broader state of the economy. Unemployment and Economic Activity Gross domestic product (GDP) and the unemployment rate -run relationship.
Frequency: Monthly. The unemployment rate represents the number of unemployed as a percentage of the labor force. Labor force data are restricted to people 16 years of age and older, who currently reside in 1 of the 50 states or the District of Columbia, who do not reside in institutions (e.g., penal and mental facilities, homes for the aged ...
Thus, our first research question is whether young adults are more vulnerable to economic shocks compared to their older counterparts. ... Our assessment of unemployment rates in 34 OECD countries reveals that the average rate of youth unemployment in 2007 was 13.4%, compared to 18.9% in 2011, so the delta of youth unemployment before and after ...
After record-high unemployment due to the COVID-19 pandemic, the unemployment rate in the U.S. reached record-lows in 2022 and 2023 as the economy rebounded. Unemployment trends across groups
The seasonally adjusted U-6 rate stood at 9.4% in January; since 1994 it has ranged from 6.8% (in October 2000) to 17.1% (most recently in April 2010). While the U-6 typically runs anywhere from 3 to 7 percentage points higher than the regular unemployment rate, with the gap wider during recessions and narrower in good economic times, it tends ...
rising rates of joblessness—particularly when evaluating policies that would encourage those outcomes—and formulate more effective strategies to curb rising unemployment such that mobility for low-wage workers can be protected. Workers, Wages, and Workplaces Fundamentally, the question of unemployment is a question of work. It follows that the
Macro 2.3 - Unemployment and Labor Force Statistics. The formula for the unemployment rate is: Unemployed/Labor Force x 100 = Unemployment Rate. 2. Who is not counted in the unemployment rate? The official unemployment rate (U-3) does not count people who are not actively looking for work.
Units: Percent, Seasonally Adjusted Frequency: Monthly Notes: The unemployment rate represents the number of unemployed as a percentage of the labor force. Labor force data are restricted to people 16 years of age and older, who currently reside in 1 of the 50 states or the District of Columbia, who do not reside in institutions (e.g., penal and mental facilities, homes for the aged), and who ...
In 2021, the unemployment rate for persons with a disability was 10.1%, approximately twice as high as those without a disability 4 . With attention to psychiatric disabilities, more than 11 million adults in the United States are diagnosed with a serious mental illness (i.e., schizophrenia, anxiety, bipolar disorder), and 90% of this ...
Units: Percent, Seasonally Adjusted Frequency: Monthly Notes: The unemployment rate represents the number of unemployed as a percentage of the labor force. Labor force data are restricted to people 16 years of age and older, who currently reside in 1 of the 50 states or the District of Columbia, who do not reside in institutions (e.g., penal and mental facilities, homes for the aged), and who ...
However, for 2025 and 2026, the forecasters have shifted their highest unemployment rate expectations from a range of 3.7 percent to 4.2 percent in the previous survey to 4.3 percent to 4.8 percent in the current survey. Mean Probabilities for Unemployment Rate in 2024 (chart) Mean Probabilities for Unemployment Rate in 2025 (chart)
The following chart, based on ILO data, shows how labor force unemployment for people aged 15-24 years in Bangladesh stood at 15.7 percent in 2023, above the world average for youth unemployment ...
The latest NI seasonally adjusted unemployment rate (the proportion of economically active people aged 16 and over who were unemployed) for the period April-June 2024 was estimated from the Labour Force Survey at 1.9%. This was a decrease of 0.2 percentage points (pps) over the quarter and a decrease of 0.7pps over the year.
The latest NI seasonally adjusted unemployment rate (the proportion of economically active people aged 16 and over who were unemployed) for the period April-June 2024 was estimated from the Labour Force Survey at 1.9%. This was a decrease of 0.2 percentage points (pps) over the quarter and a decrease of 0.7pps over the year.