
Want to create or adapt books like this? Learn more about how Pressbooks supports open publishing practices.
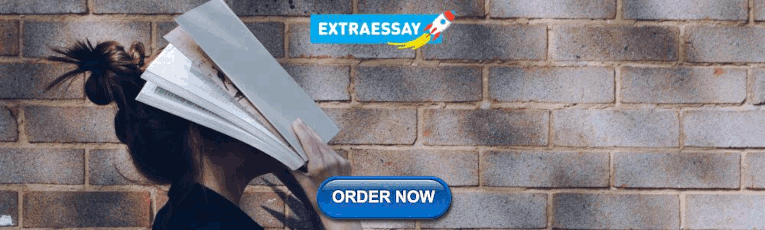
15.7 Definition Essay
Learning objective.
- Read an example of the definition rhetorical mode.
Defining Good Students Means More Than Just Grades
Many people define good students as those who receive the best grades. While it is true that good students often earn high grades, I contend that grades are just one aspect of how we define a good student. In fact, even poor students can earn high grades sometimes, so grades are not the best indicator of a student’s quality. Rather, a good student pursues scholarship, actively participates in class, and maintains a positive, professional relationship with instructors and peers.
Good students have a passion for learning that drives them to fully understand class material rather than just worry about what grades they receive in the course. Good students are actively engaged in scholarship, which means they enjoy reading and learning about their subject matter not just because readings and assignments are required. Of course, good students will complete their homework and all assignments, and they may even continue to perform research and learn more on the subject after the course ends. In some cases, good students will pursue a subject that interests them but might not be one of their strongest academic areas, so they will not earn the highest grades. Pushing oneself to learn and try new things can be difficult, but good students will challenge themselves rather than remain at their educational comfort level for the sake of a high grade. The pursuit of scholarship and education rather than concern over grades is the hallmark of a good student.
Class participation and behavior are another aspect of the definition of a good student. Simply attending class is not enough; good students arrive punctually because they understand that tardiness disrupts the class and disrespects the professors. They might occasionally arrive a few minutes early to ask the professor questions about class materials or mentally prepare for the day’s work. Good students consistently pay attention during class discussions and take notes in lectures rather than engage in off-task behaviors, such as checking their cell phones or daydreaming. Excellent class participation requires a balance between speaking and listening, so good students will share their views when appropriate but also respect their classmates’ views when they differ from their own. It is easy to mistake quantity of class discussion comments with quality, but good students know the difference and do not try to dominate the conversation. Sometimes class participation is counted toward a student’s grade, but even without such clear rewards, good students understand how to perform and excel among their peers in the classroom.
Finally, good students maintain a positive and professional relationship with their professors. They respect their instructor’s authority in the classroom as well as the instructor’s privacy outside of the classroom. Prying into a professor’s personal life is inappropriate, but attending office hours to discuss course material is an appropriate, effective way for students to demonstrate their dedication and interest in learning. Good students go to their professor’s office during posted office hours or make an appointment if necessary. While instructors can be very busy, they are usually happy to offer guidance to students during office hours; after all, availability outside the classroom is a part of their job. Attending office hours can also help good students become memorable and stand out from the rest, particularly in lectures with hundreds enrolled. Maintaining positive, professional relationships with professors is especially important for those students who hope to attend graduate school and will need letters of recommendation in the future.
Although good grades often accompany good students, grades are not the only way to indicate what it means to be a good student. The definition of a good student means demonstrating such traits as engaging with course material, participating in class, and creating a professional relationship with professors. While every professor will have different criteria for earning an A in their course, most would agree on these characteristics for defining good students.
Online Definition Essay Alternatives
Judy Brady provides a humorous look at responsibilities and relationships in I Want a Wife :
- http://www.columbia.edu/~sss31/rainbow/wife.html
Gayle Rosenwald Smith shares her dislike of the name for a sleeveless T-shirt, The Wife-Beater :
- http://faculty.gordonstate.edu/cperkowski/1101/WifeBeater.pdf
Philip Levine defines What Work Is :
- http://www.ibiblio.org/ipa/poems/levine/what_work_is.php
- http://www.poemhunter.com/poem/what-work-is
Writing for Success Copyright © 2015 by University of Minnesota is licensed under a Creative Commons Attribution-NonCommercial-ShareAlike 4.0 International License , except where otherwise noted.
A community blog focused on educational excellence and equity
About the topic
Explore classroom guidance, techniques, and activities to help you meet the needs of ALL students.
most recent articles
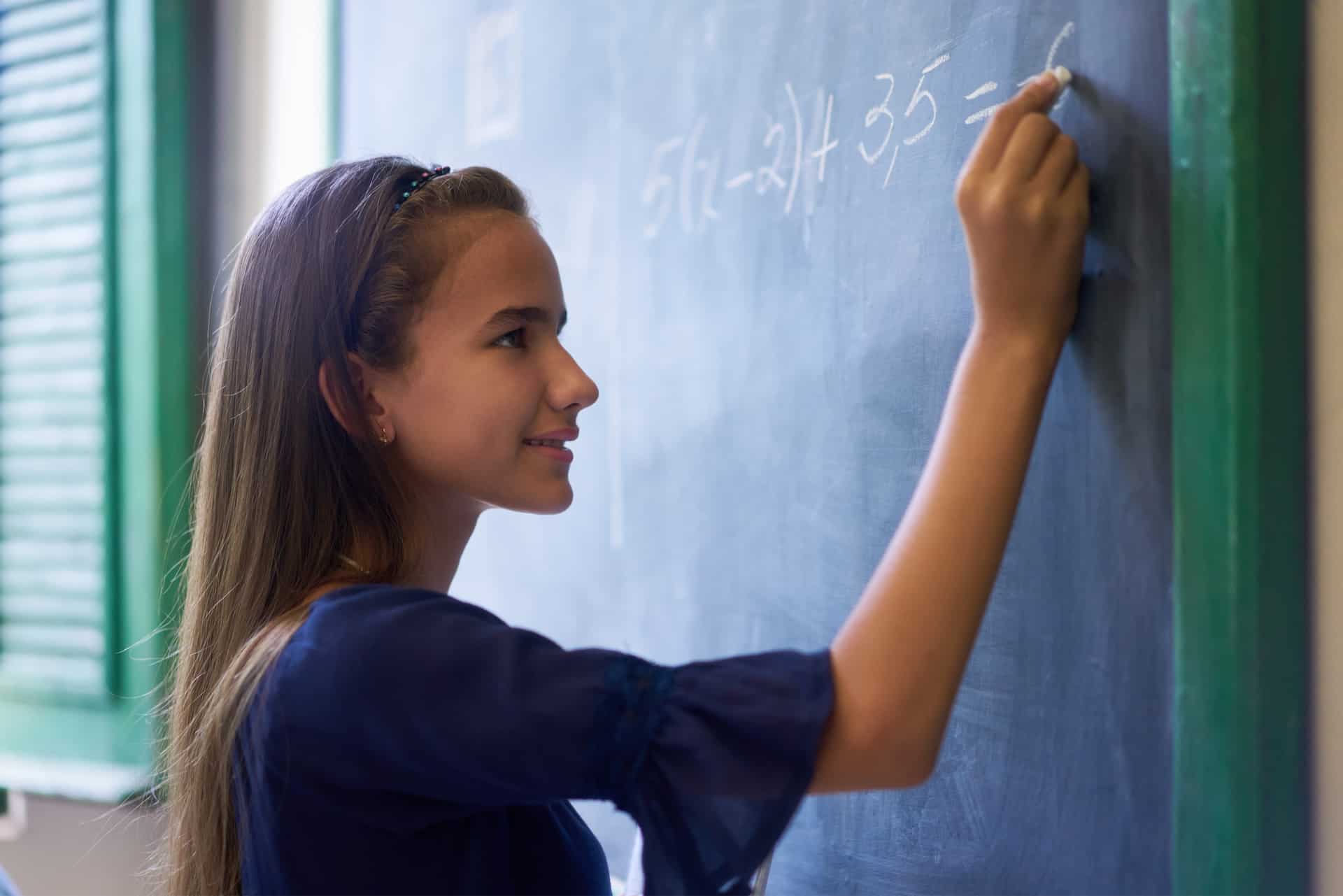
Shaking Up High School Math
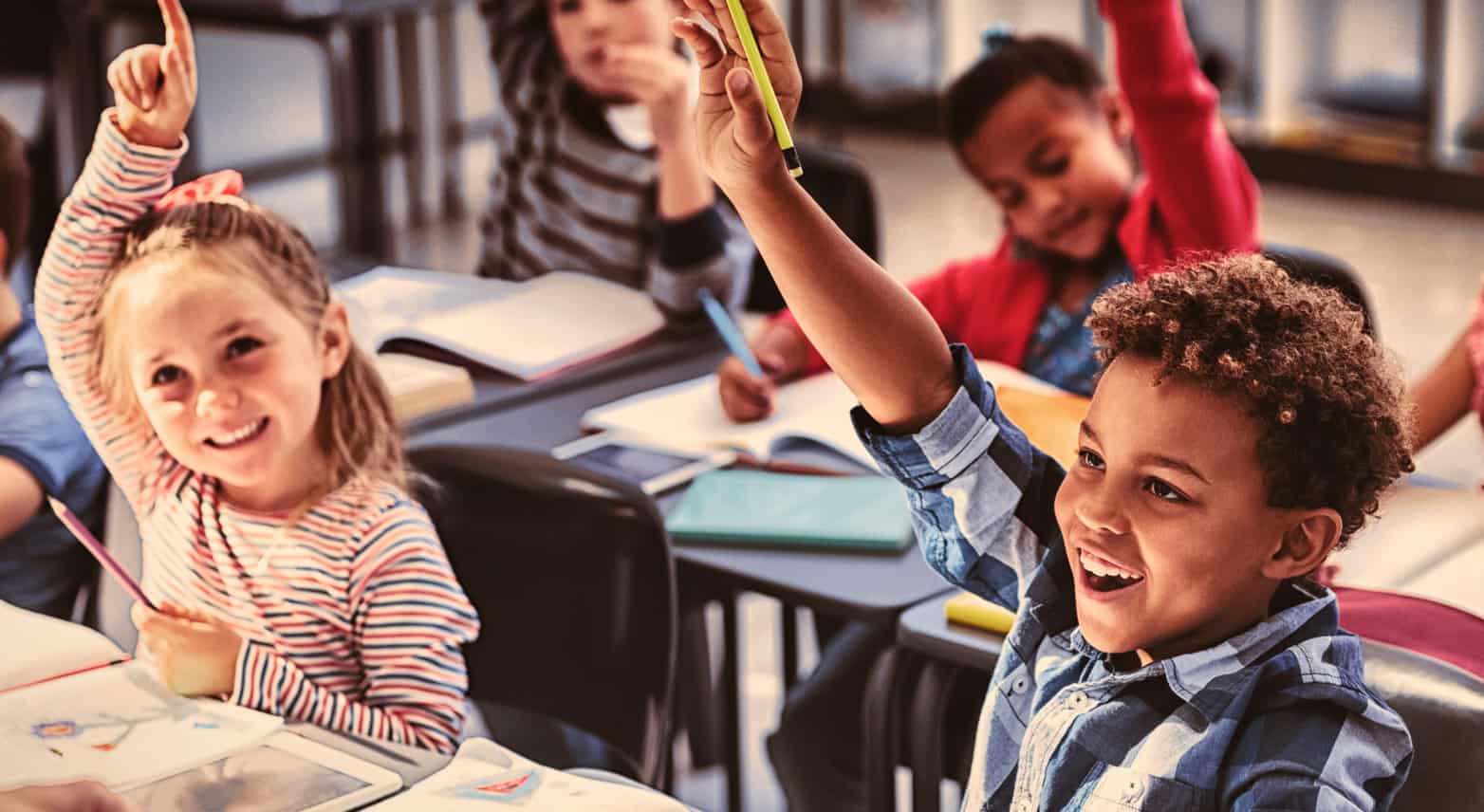
Executive Functions and Literacy Skills in the Classroom
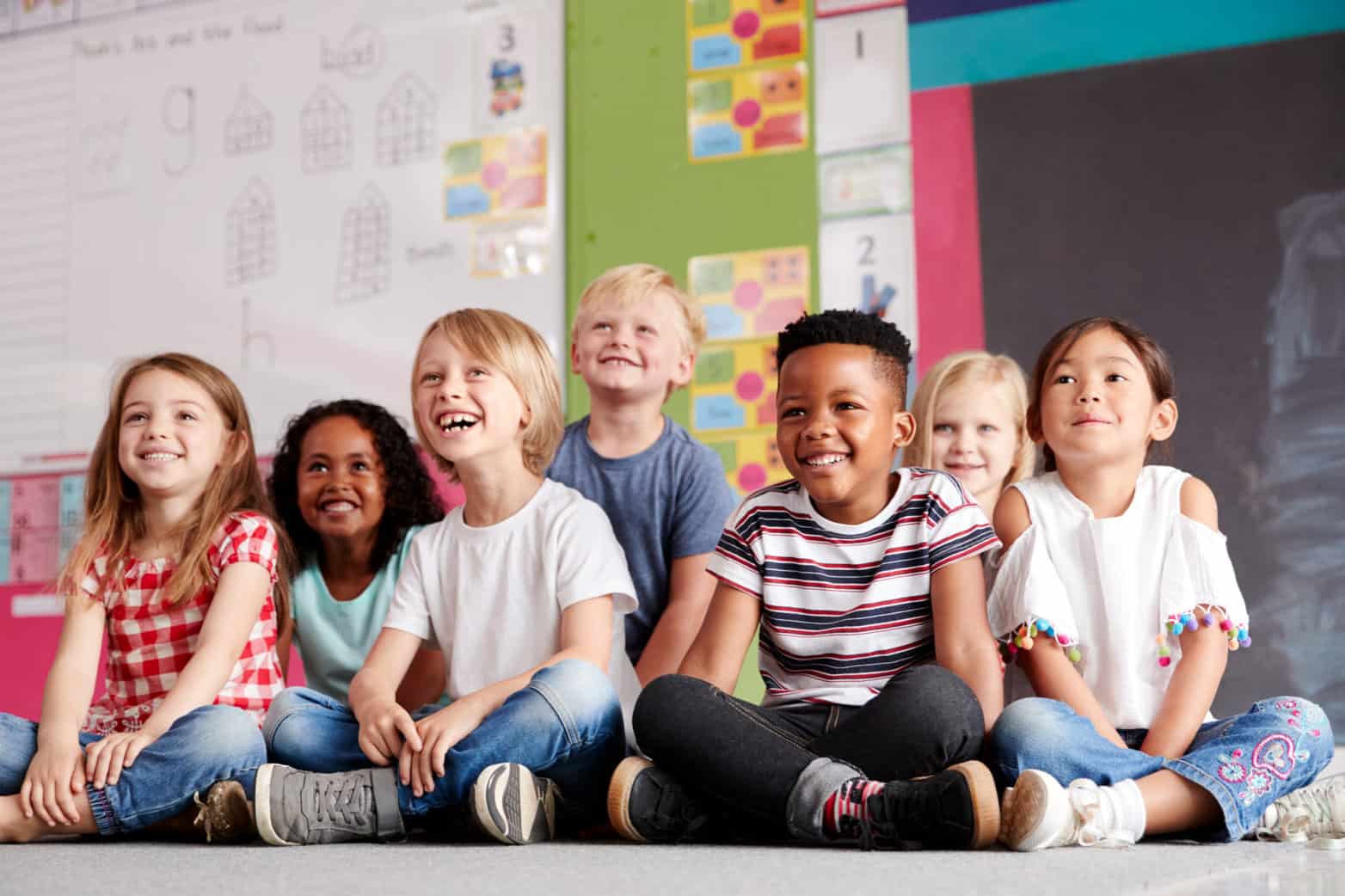
Connecting and Communicating With Families to Help Break Down Barriers to Learning
Discover new tools and materials to integrate into you instruction.
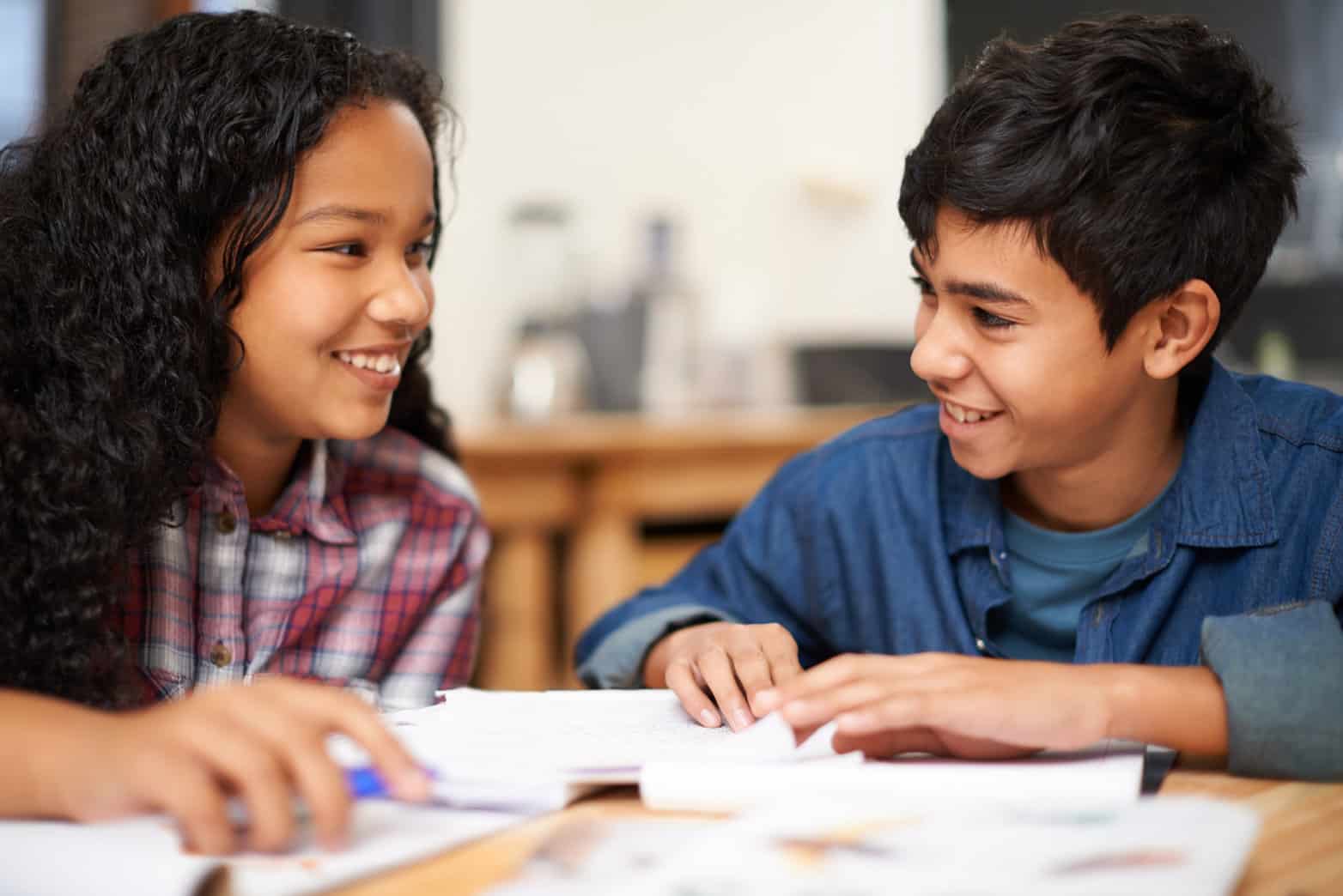
Culture, Community, and Collaboration
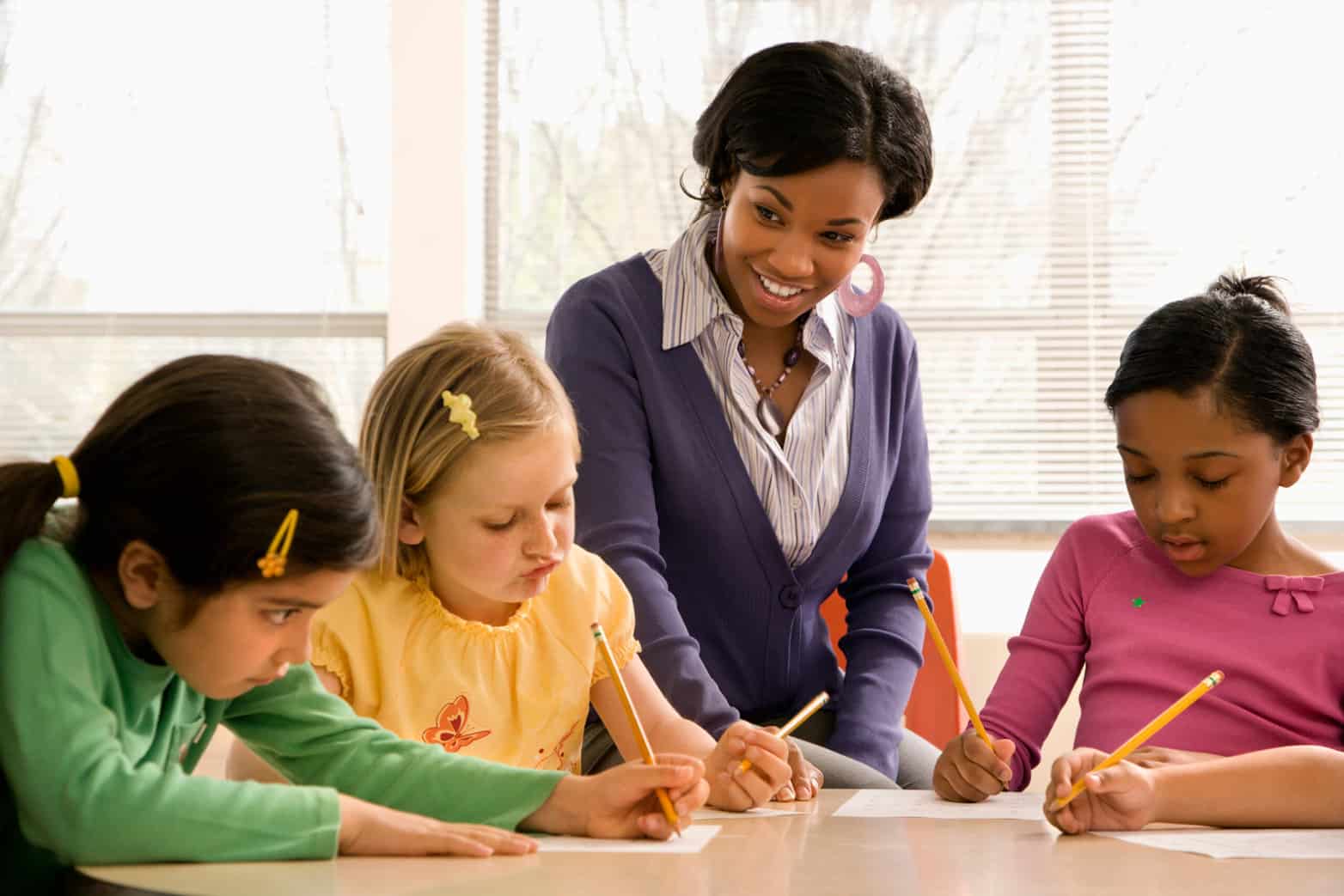
Vertical Progression of Math Strategies – Building Teacher Understanding
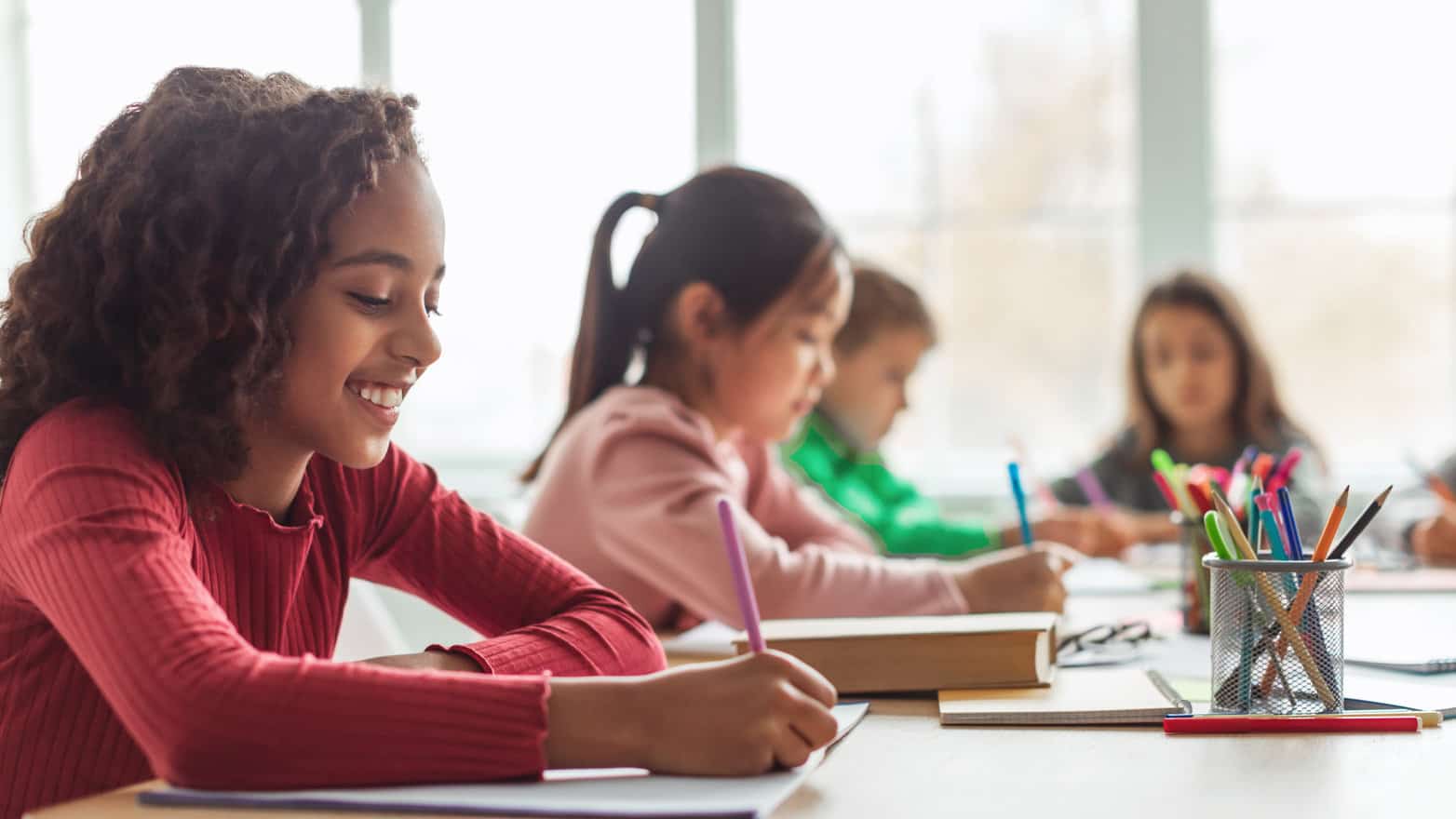
“Can I have this? Can I have that?”
Find instructions and recommendations on how to adapt your existing materials to better align to college- and career-ready standards.
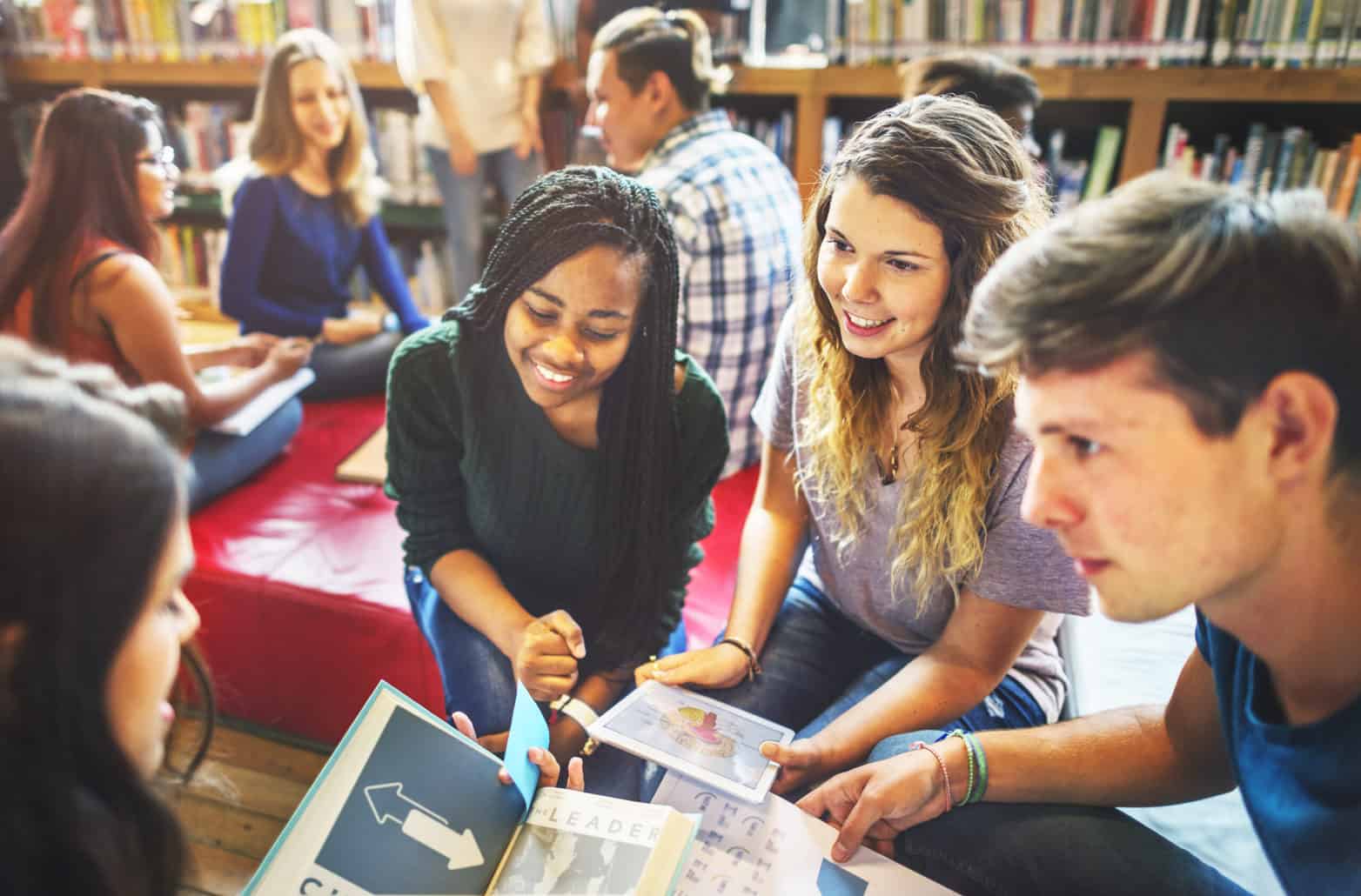
To Teach the Truth
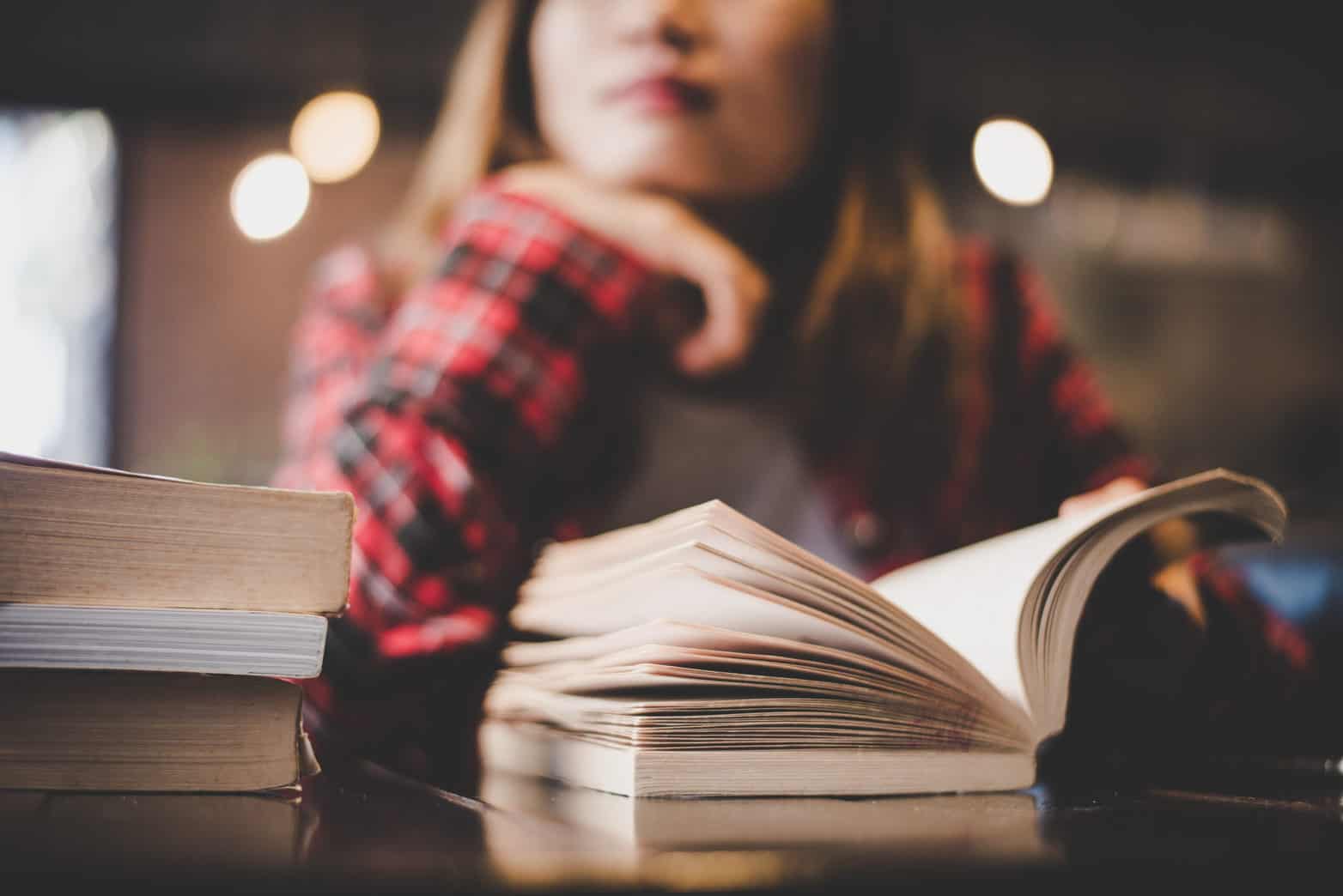
Helping Our Students See Themselves and the World Through the Books They Read in Our Classrooms
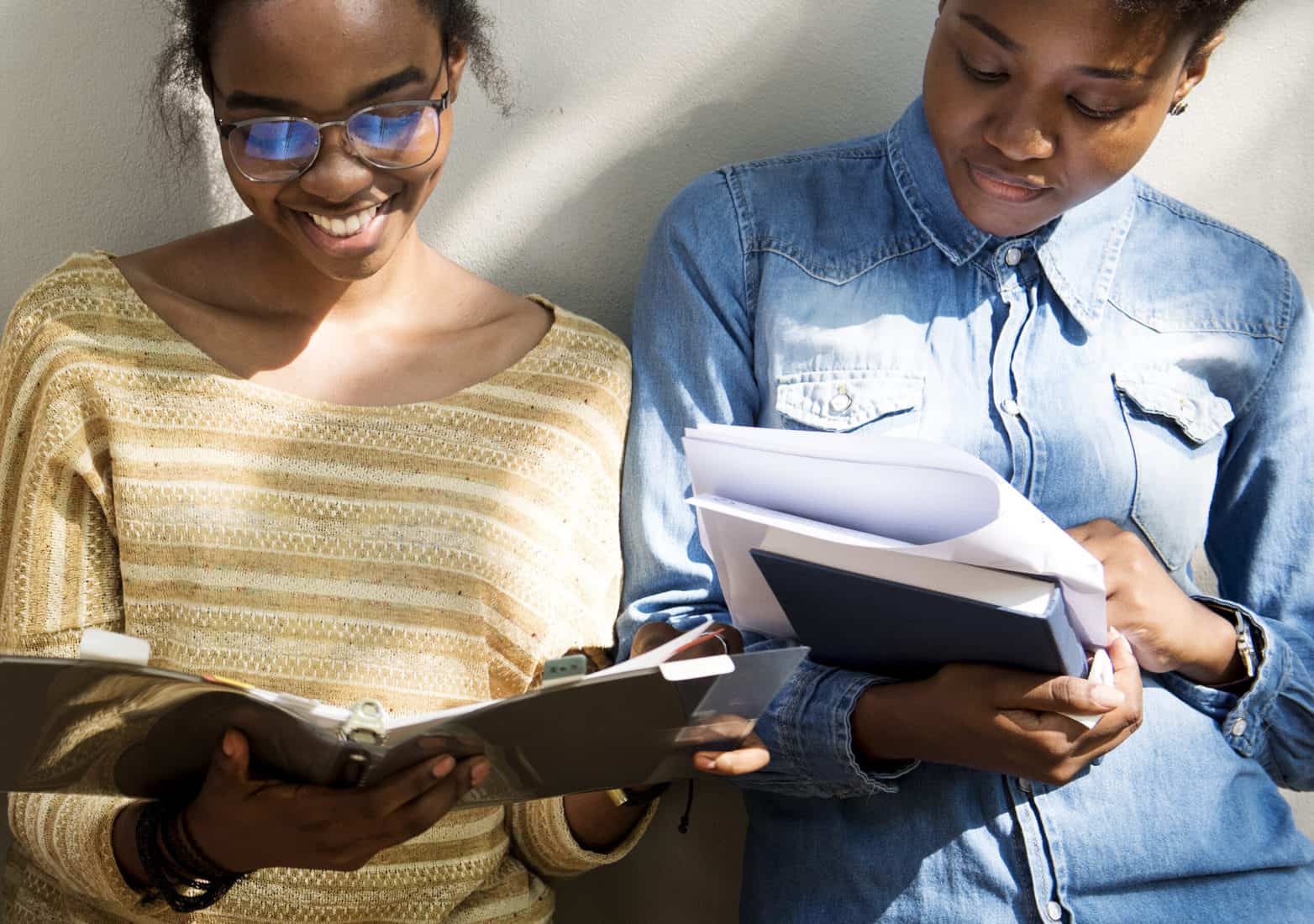
Textbooks: Who Needs Them?
Learn what it means for instructional materials and assessment to be aligned to college- and career-ready standards.
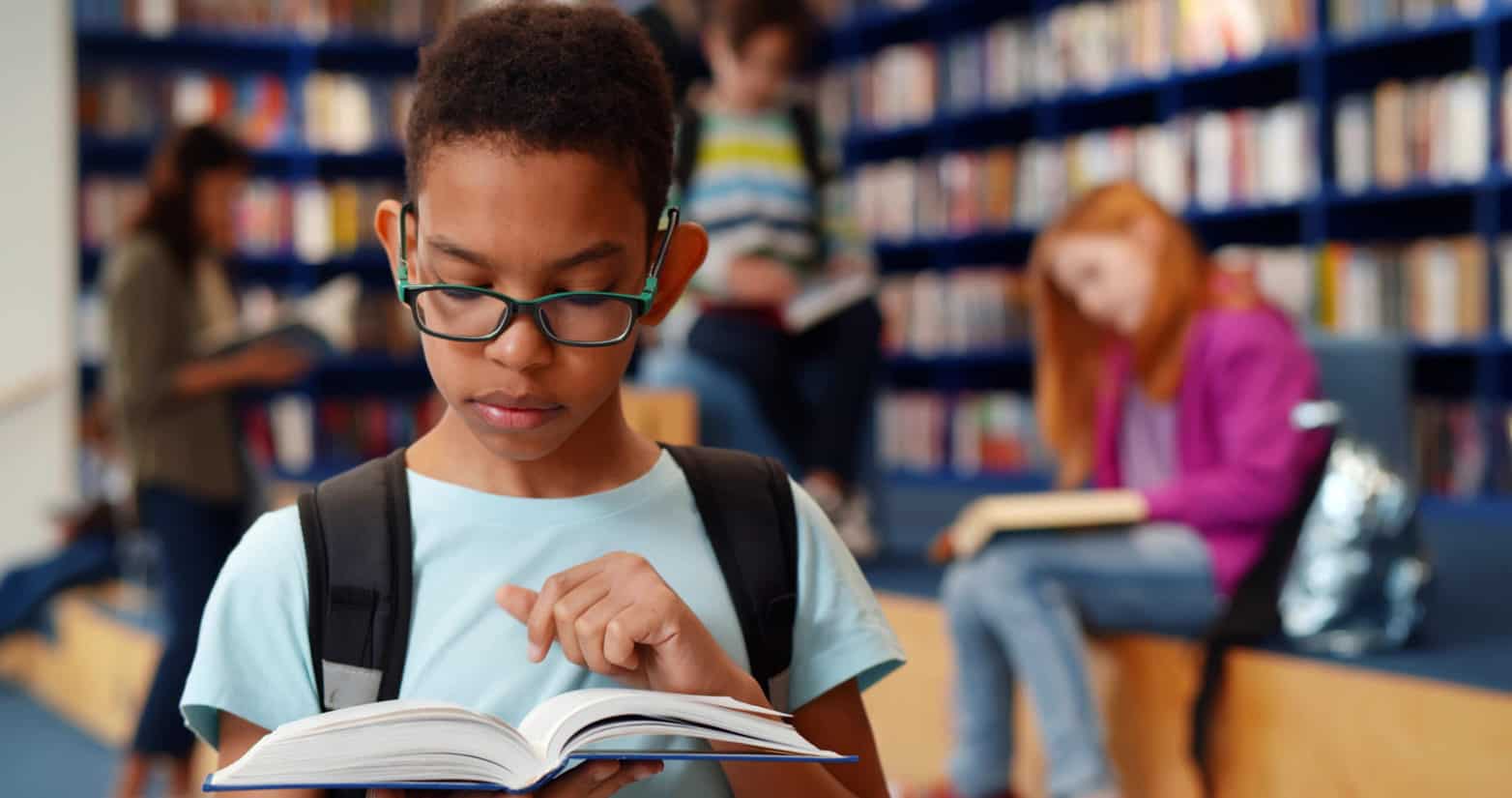
Let’s Not Make Power ELA/Literacy Standards and Talk About Why We Didn’t
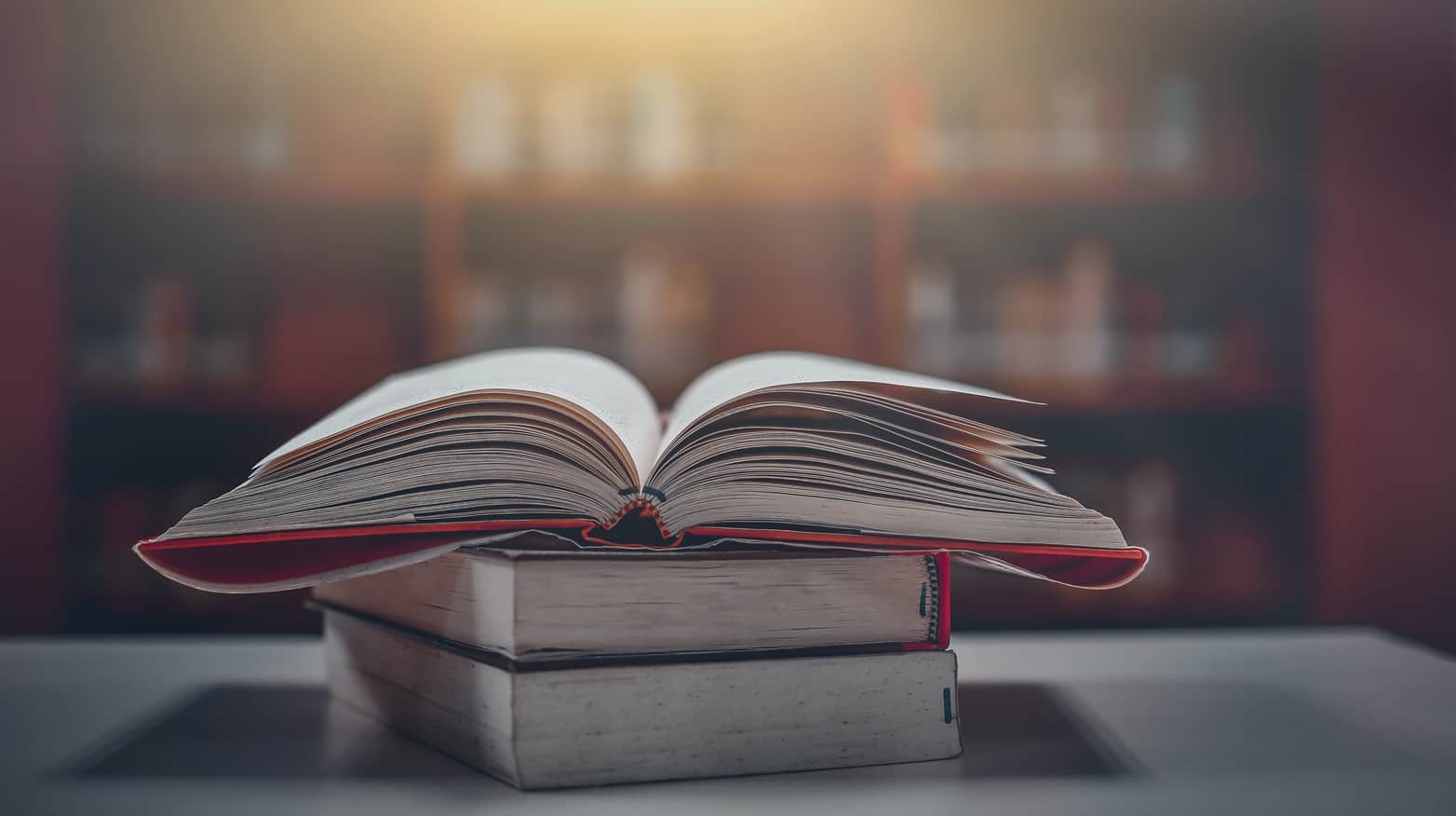
What to Consider if You’re Adopting a New ELA/Literacy Curriculum
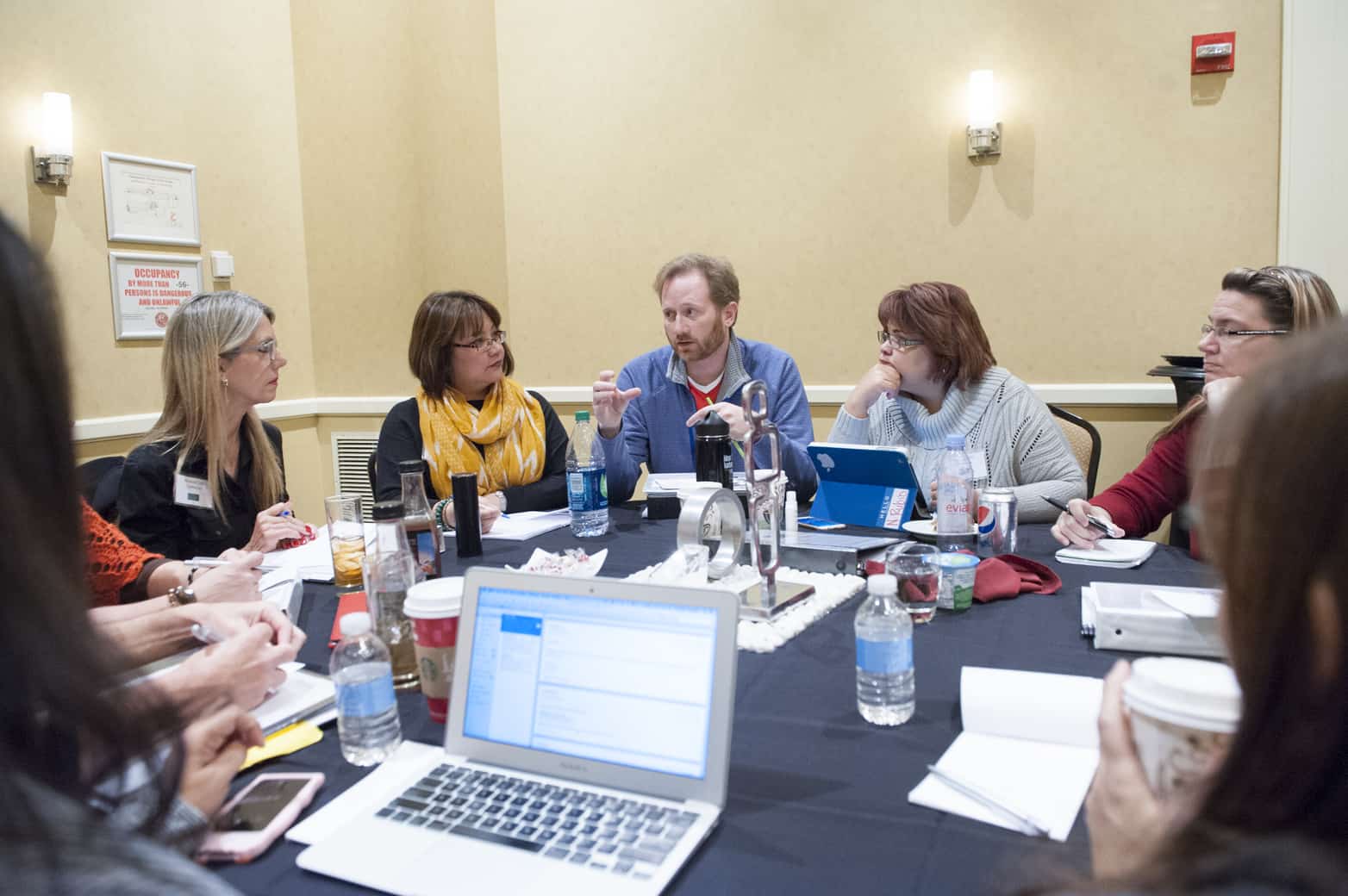
Not Your Mom’s Professional Development
Delve into new research and perspectives on instructional materials and practice.
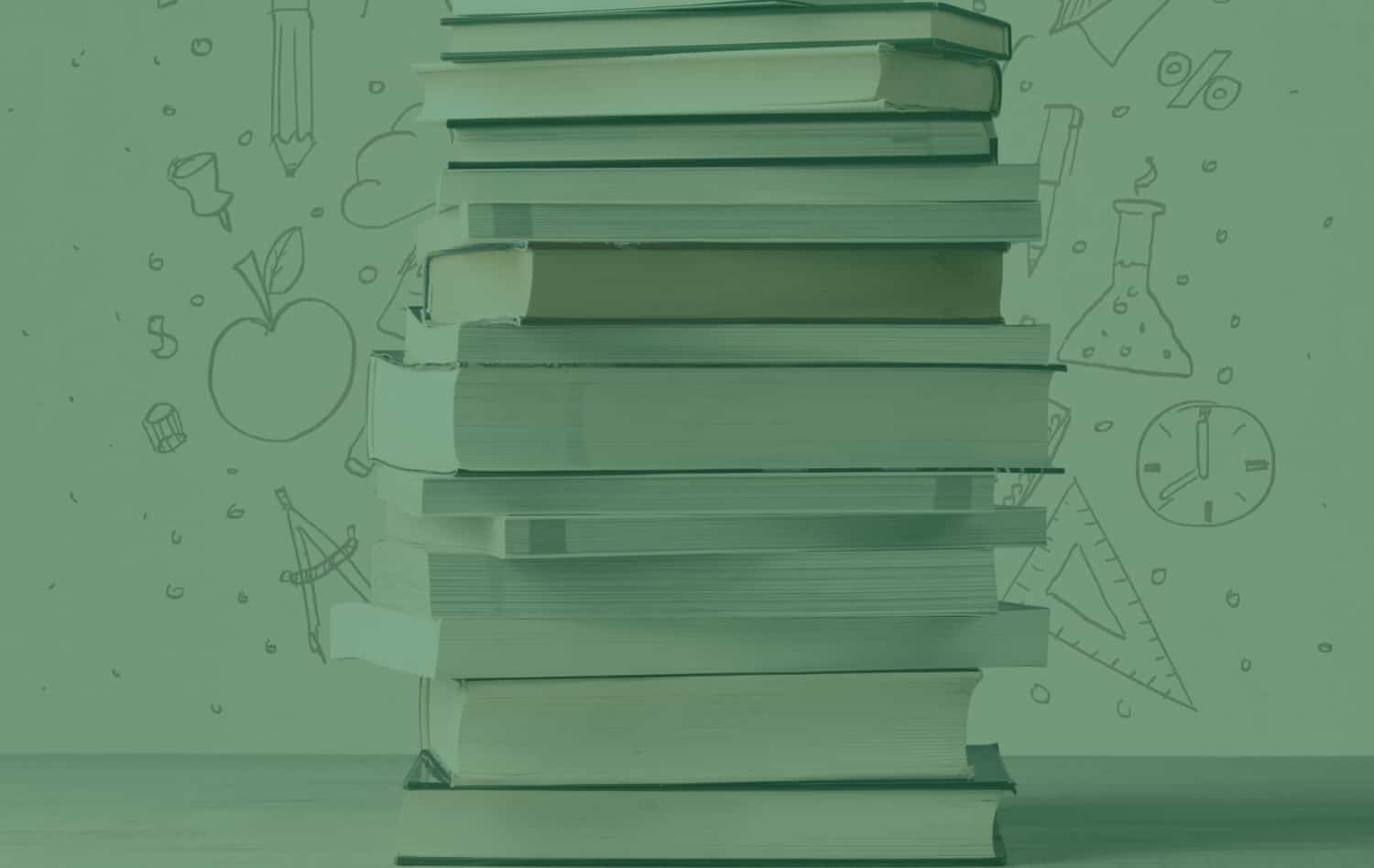
Summer Reading Club 2023
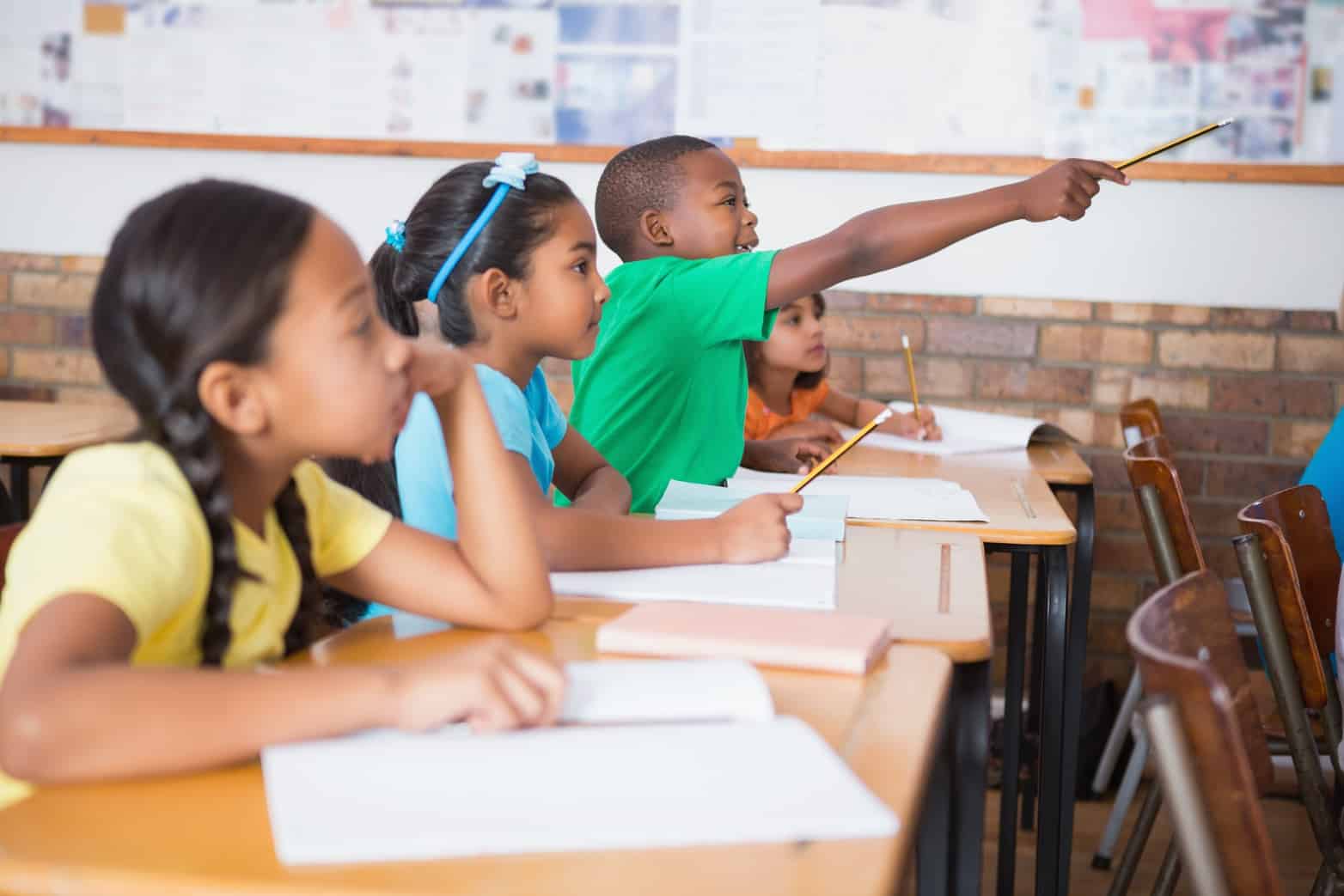
Synergy between College and Career Readiness Standards-Aligned Instruction and Culturally Relevant Pedagogy
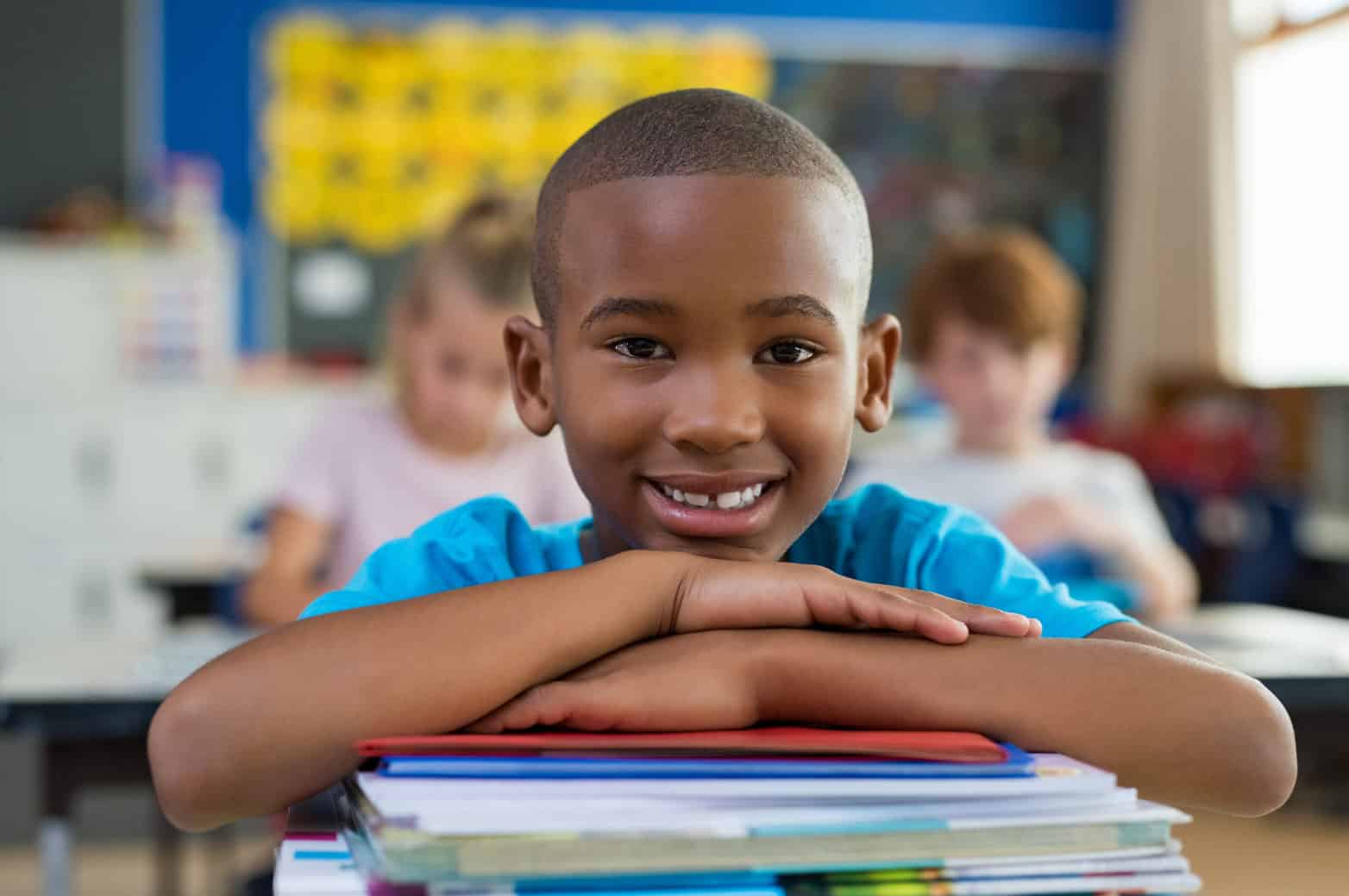
Children Should be Seen AND Heard
- Submissions Guidelines
- About the Blog
What Is Academic Success (Student Learning)?
Reimagining how to foster academic success in the classroom and document students' growth
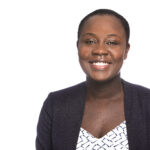
“Teachers genuinely believe in the intellectual potential of these students [of color] and accept, unequivocally, their responsibility to facilitate its realization without ignoring, demeaning, or neglecting their ethnic and cultural identities. They build toward academic success from a basis of cultural validation and strength” (Geneva Gay, 2001).
Within the field of education, “academic success” is a widely used construct that is equated to “student achievement” and often linked to students’ performance on standardized test scores. This narrowed definition of academic success justifies the school failure of Black students and students learning English and shifts the blame to students, their families, and communities.
In her seminal article, “But That’s Just Good Teaching! The Case for Culturally Relevant Pedagogy,” Dr. Ladson-Billings stated that “despite the current social inequities and hostile classroom environment, students must develop their academic skill” (p. 160). In 2014, she clarified that her stance on “academic success” must center “the intellectual growth that students experience as a result of classroom instruction and learning experience” (p. 75).
The measure of academic success should not be solely based on test scores from standardized tests and other traditional definitions of success. Educators and researchers have recognized that White middle-class standards are often set as the benchmarks of success. Schools typically expect all students, regardless of their background or culture, to assimilate to these standards. While Dr. Ladson-Billings and other scholars have affirmed that it is important for all students to have exposure to and know how to navigate the dominant culture, all educators must strive for equity within the traditional definitions of academic success, thus expanding the definition in broader and richer terms to center student learning and academic growth over time.
To move toward equitable educational outcomes for Black students and students learning English, we must reimagine how to foster academic success in the classroom and document students’ growth. To do this, we must shift toward measuring “academic success” through a range of different means, including but not limited to project-based learning, portfolios, and student reflection. Building academic success requires that teachers take responsibility for their students’ learning, create and sustain learning partnerships with students and families, and build students’ agency in their learning while consistently calling out and mitigating the structural racism and injustices that limit historically resilient students’ academic growth.
What are we learning?
Through our work with educators, we have noted several practices that speak to how they foster academic success with their historically resilient students. It is important to note that these educators highlighted the interwoven nature of high-quality instructional materials (HQIM) and teacher practices in fostering student learning and academic growth and success. Below, we provide some examples of how educators have engaged standards-aligned instructional materials in service of culturally relevant instruction and an expanded definition of academic success.
Teachers who embrace cultural relevance believe that historically resilient students have what it takes to succeed, hold high expectations for them, reaffirm their humanity, and create opportunities to experience learning growth. For example, in a conversation with one of the educators, she noted that:
“We need to make sure that our actions as educators are never putting barriers in front of students, and in fact, we are pushing them to shatter glass ceilings, regardless of any label that society may put on them. I think my purpose [as an educator] is to make sure that all of our students succeed beyond our expectations, but especially our students of color who usually are counted out of advanced classes and advanced opportunities.”
Teachers we spoke to know where their students’ strengths and areas of weakness are and use them as a starting point when teaching. This deep knowledge of students is based on a genuine commitment to students’ academic success and requires an investment in every student in the classroom. For example, one teacher described her interactions with students during a math lesson. She noted that:
“I’m able to identify which students really understood the task at hand, and which students really need to be extended a challenge because they kind of had a firm grasp on the content. Then I ask myself, “What students do I need to revisit some previous learning because they may be missing some of those foundational pieces that I need to build on so that they can access the materials on the grade level that I’m presenting?”
Another teacher stressed the importance of checking for students’ understanding of the content before moving on to new materials. This teacher noted that:
“I need that time to come back with them and go over the assignment and talk about those mistakes and help them fix it, and talk about error analysis, things like that.”
Here, we see the teacher taking ownership of and demonstrating a commitment to student learning and academic growth when students do not fully understand the content and dedicating time to reteach and provide scaffolds until the content is understood. This kind of messaging can signal to students that their teacher is invested in them.
As we’ve learned more about the practical applications of Dr. Ladson-Billings’ work, we’ve come to understand how academic success, particularly viewed through the lens of student growth, can’t be defined by a test score or even by successful completion of an assignment. Teachers who embody this understanding move past these limited definitions of academic success to see how their academic learning and growth have evolved over time. This means they scaffold tasks based on what their students bring to the classroom and use assessment as a tool to understand what their students know and can do, not as a way to identify gaps to fill.
Significant time is spent understanding students’ prior knowledge and the grade-level learning targets to craft instruction that is meaningful for all students. Standards-aligned instructional materials have the potential to play an essential role by clearly defining grade-level targets and offering carefully designed activities that may be adapted to meet the needs of all learners. When standard-aligned instructional materials are used in conjunction with culturally responsive pedagogy, we are learning that both student engagement and learning experiences expand across the students’ range of growth and potential. We hope you continue on this journey with us as we explore how teachers work with standards-aligned materials in service of the other aspects of culturally relevant pedagogy.
Ladson‐Billings, G. (1995). But that’s just good teaching! The case for culturally relevant pedagogy. Theory into practice , 34 (3), 159-165.
Ladson-Billings, G. (2014). Culturally relevant pedagogy 2.0: aka the remix. HarvardEducational Review , 84 (1), 74-84.
- Equity in Education
2 thoughts on “ What Is Academic Success (Student Learning)? ”
Equity is a magical place many never see as often as I to intervene and create equity, the reality of what students live and face daily is so politically enforced that I want to scream. The hope that I am challenged with then comes from every individual that is committed to do the work of educating. I find hope in people who are really charged to educate and lift others. My personal dilemma is am I involved in meaningful work. I was at LSU ( Louisiana State University) while I taught in the public schools of Baton Rouge. A woman walked up to me one day on campus and said, ” Why are you teaching with them those people don’t care.” I just looked at her because I was truly one of those people. What do you do when you know the best effort has gone out and Harvard intellectuals say pasting test scores are fleeting. I really believe we must trust that what are engaging in is worth the effort no matter what others think. The saying, “One does better when they know better,” only works when there is a value to do better. Money isn’t the best elevator in education it only helps our commitments and values to challenge the status quo is the best. I want to be the best educator I can be today. Jo Dawkins
Some thoughts on academic success: 1) There is a disconnect between what invested educators consider “academic success” and what others consider this to be. For example, I find that the majority of students and their parents want good grades, but the grades (letter or number) matter more than WHETHER THE STUDENT HAS ACTUALLY LEARNED ANYTHING. This is seen when teachers that give out easy A’s and B’s are lauded as “wonderful” while teachers that “take ownership” and have high expectations for all students (which involves MUCH MORE WORK for the teachers as they must actually read and mark up student work, conference with students and provide extra help, and then grade final versions) are criticized as too demanding when after all that effort, some students get the poor grades they deserve. Another example of this is evident when more than ever before, students are off on VACATION throughout the school year. These incidents were becoming more frequent before the pandemic, and now they have further multiplied. Parents just take their children out of school whenever they want. Well, their message is loud and clear — they must feel that being in school is not connected to academic success and/or school is just not that important. There is a disconnect between academic success and what that means and entails. 2) Academic success needs to be based on more than grades. Not only because of the above-mentioned situations where a letter or number becomes the end-all-be-all rather than the knowledge it represents but because success based solely on a grade is very limiting to students that have different learning styles and abilities. I like to conference with students and have them tell me about the content — I find that this reveals very quickly whether they understand or are having difficulty. Another way to check understanding is by having students demonstrate it in some tangible way — teach another student, teach the class, etc. When a student can do this, he or she has achieved success.
Leave a Reply Cancel reply
Your email address will not be published. Required fields are marked *
About the Author: Abigail Amoako Kayser, Ph.D. is an Assistant Professor at the College of Education at California State University, Fullerton in the Department of Elementary and Bilingual Education. She is a Fulbright Scholar and a former elementary teacher. Through her research and teaching, she aims to advance our understanding of how teachers ensure equitable, just, and anti-racist educational experiences and outcomes for historically resilient students in the U.S. and Ghana.
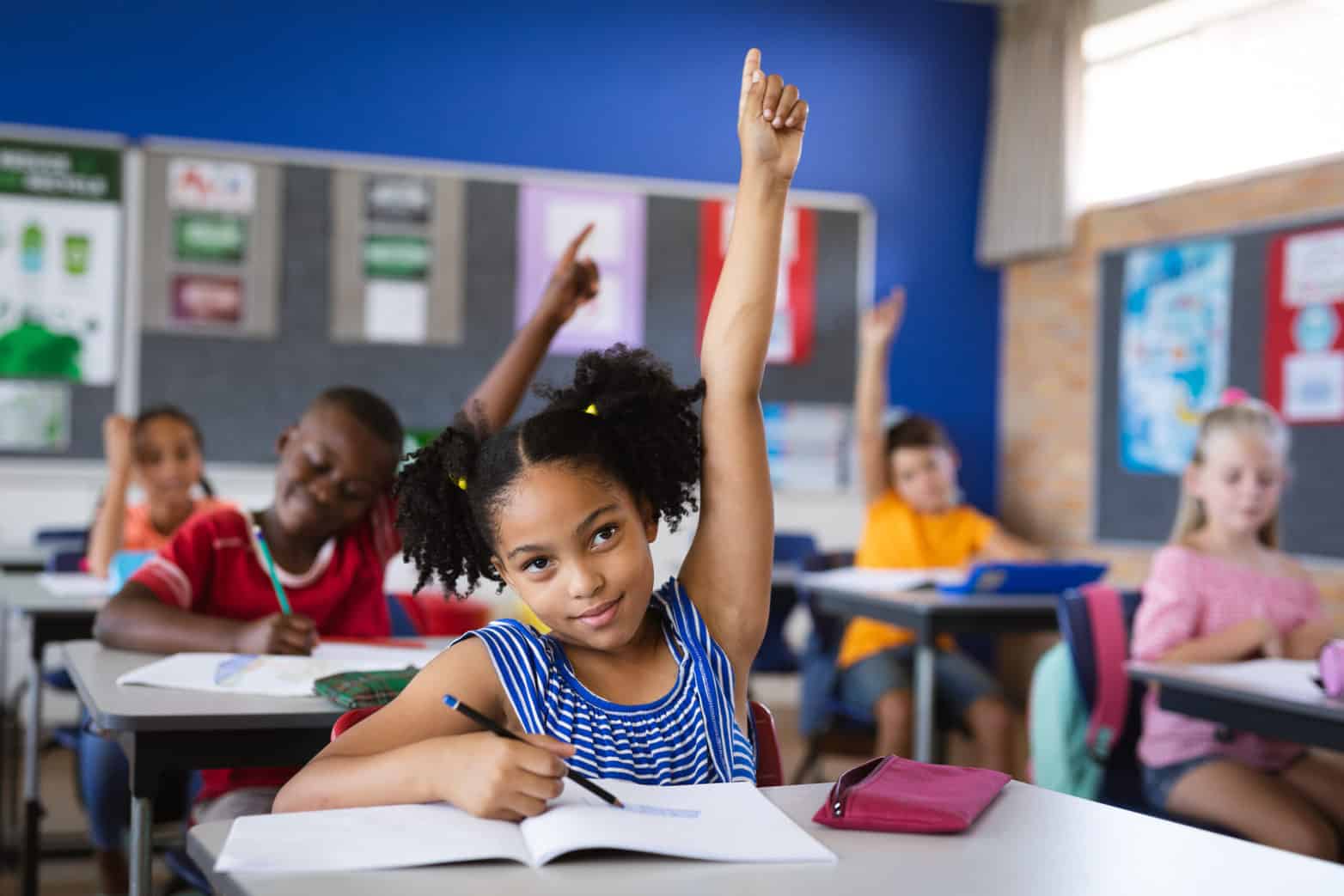
What Is Culturally Relevant Pedagogy?
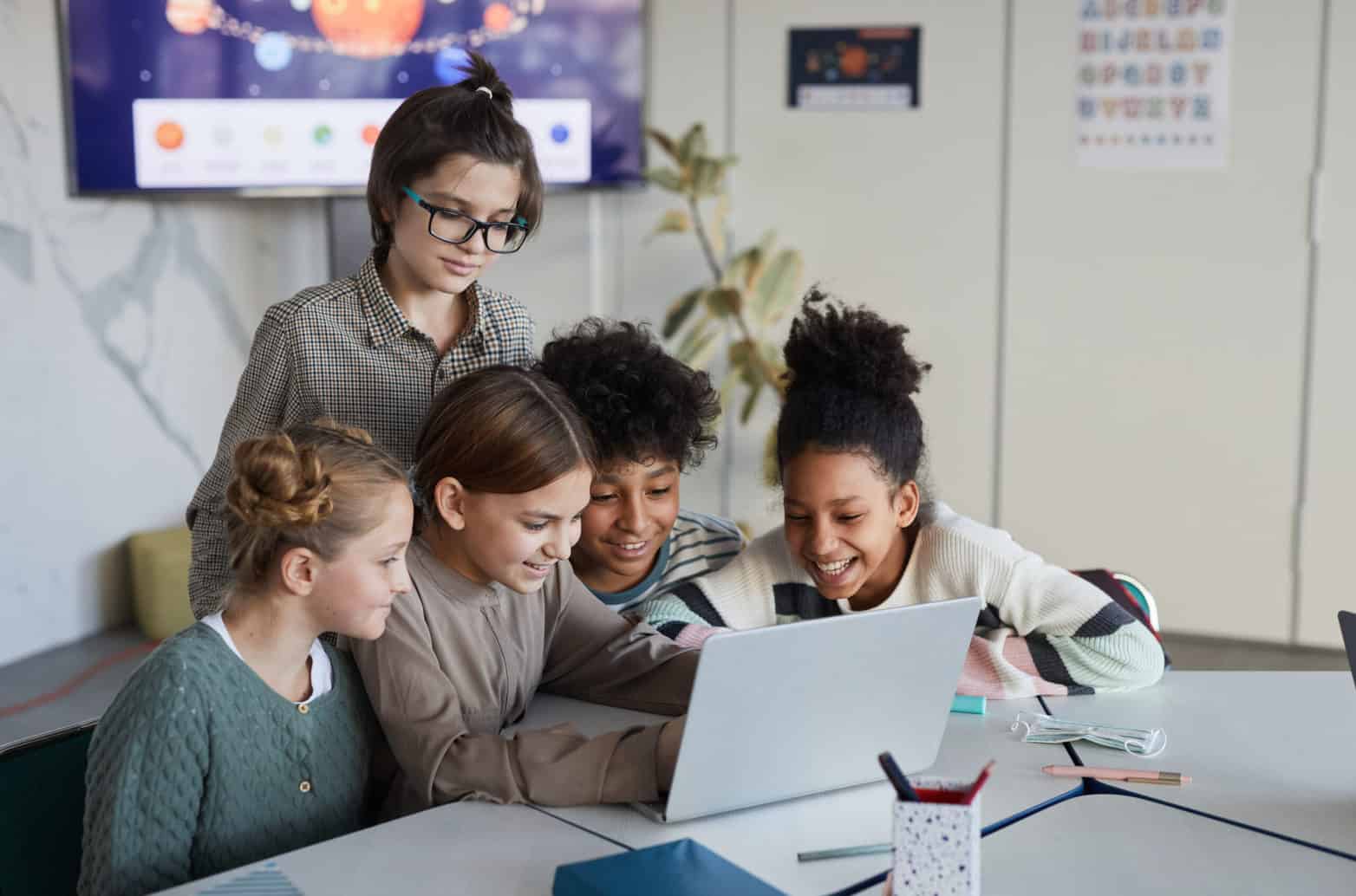
What Is Critical Consciousness?
Stay in touch.
Like what you’re reading? Sign up to receive emails about new posts, free resources, and advice from educators.
Academia.edu no longer supports Internet Explorer.
To browse Academia.edu and the wider internet faster and more securely, please take a few seconds to upgrade your browser .
Enter the email address you signed up with and we'll email you a reset link.
- We're Hiring!
- Help Center
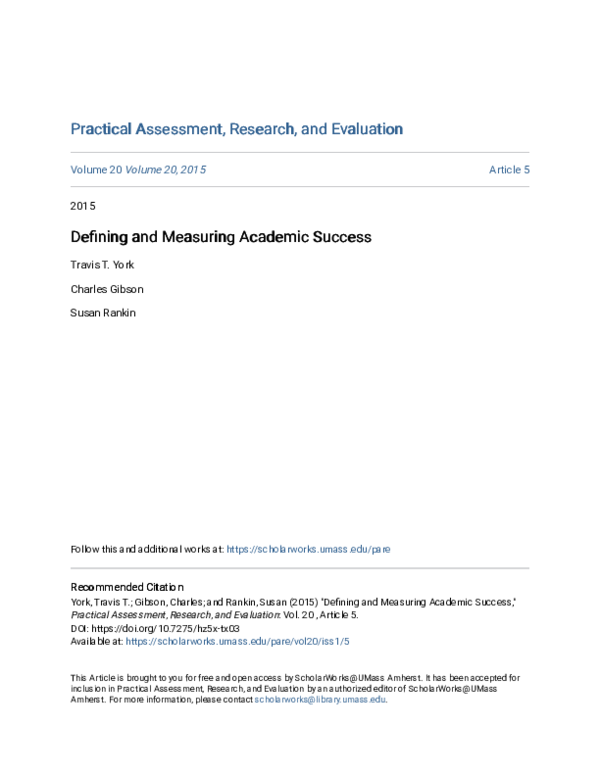
Defining and Measuring Academic Success

2015, Practical Assessment, Research and Evaluation
Despite, and perhaps because of its amorphous nature, the term ‘academic success’ is one of the most widely used constructs in educational research and assessment within higher education. This paper conducts an analytic literature review to examine the use and operationalization of the term in multiple academic fields. Dominant definitions of the term are conceptually evaluated using Astin’s I-E-O model resulting in the proposition of a revised definition and new conceptual model of academic success. Measurements of academic success found throughout the literature are presented in accordance with the presented model of academic success. These measurements are provided with details in a user-friendly table (Appendix B). Results also indicate that grades and GPA are the most commonly used measure of academic success. Finally, recommendations are given for future research and practice to increase effective assessment of academic success.
Related Papers
Zin Eddine Dadach
The final goals of this study is to investigate the impact of using a Programmable Logic Controller (PLC) as a research based learning tool on the performance and motivation of each student in an industrial automation course. For this purpose, ten PLC’s lab experiments are used to visualize the theories covered in the learning outcomes (CLO’S) of the course and investigate their applications in industrial automation. The success of the PLC as research-based tool are demonstrated by the fact the performance of 40 students (73%) is higher in this course than their corresponding average performance in the college. Moreover, using the Dadach Motivation Factor (DMF) as tool to measure motivation of students, our results also indicate that thirty-five students (63.63%) were motivated during the course. In overall, this investigation shows that the utilization of ten PLC lab experiments for the visualization of the opaque theory of industrial automation is successful.
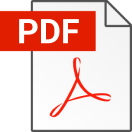
Knowledge and Performance Management
Mercy Ogbeta
Academic resilience and emotional intelligence are considered important personal resources for furthering students’ academic performance. However, many educational organizations seem to trivialize the performance implications of these constructs in teachings and curriculum. Consequently, it can decrease not just their academic performance but also their employability, as they lack the generic competencies to adapt and survive in a stressful context. Even so, empirical evidence on integrating academic resilience, emotional intelligence, and academic performance remains unexplored in the Nigerian university context. Therefore, the study aimed to investigate the linkages between academic resilience, emotional intelligence, and academic performance in Nigeria. The partial least square (PLS) modeling method was utilized for testing the stated hypotheses with data collected from 179 final year undergraduate students in the regular B.Sc. Business Administration and B.Sc. Marketing program ...
International Journal of Higher Education
margie roma
Higher educational institutions (HEIs) play a substantial role in the development of knowledge and skills that can cope with the demands of industries in the fourth industrial revolution (4IR). This study examined the alignment between the current assessment practices used by HEIs and the competencies demanded by the hospitality and tourism industry. It also aimed to develop an assessment strategy typology that could specifically target the competencies required by the industry. In addition, the study was able to determine the three most and the three least preferred assessment methods as perceived by the hotel and restaurant management students in a private university in Mandaluyong City, Philippines. The findings revealed the common assessment methods employed by teachers in Hospitality and Tourism Managemnt (HTM) major courses. The study argues that the use of these identified assessment methods likewise contribute in developing the emerging skills in the 4IR such as sense-maki...
College & Research Libraries
Angela Rockwell
Practical Assessment, Research and Evaluation
Donna H Ziegenfuss
Turkish Journal of Computer and Mathematics Education
Saumya Kumar
The significance accorded to the amorphous concept of Academic Performance of students is immense, owing to the mere reason that better performances academically set the prospect for the better future of any nation. Likewise, understanding the integrities of the concept holds a great connotation. The current paper aims to present a review of the various definitions of "academic performance" along with the factors perceived to have an effect on the academic performance of students belonging to Higher Education Institutions (HEIs). Additionally, an effort has also been made to gain an insight into the reliability of "Grade Point Average" (GPA) as a method for measuring academic performance.
Wanda Robinson
Educating a sufficient nursing workforce to provide high quality, compassionate, and ethical care to an increasingly diverse population is an ongoing challenge and opportunity for nurse educators. Current literature highlights the importance of engaging students in learning to strengthen student achievements. Fostering student engagement within nursing courses is particularly important. Grit (consistency of interest and perseverance of effort) is a factor that may be associated with student course engagement. Demographic characteristics of age, gender, race/ethnicity, prior education, degree program, and self-reported grade point average (GPA) also may be factors associated with student course engagement. Guided by a conceptual model derived from the literature, the purpose of this study was to determine whether grit and demographic characteristics were associated with student course engagement (skills, emotion, participation/interaction, and performance) within a nursing course. Us...
Health and Academic Achievement
Marcela Verešová
Marilee Bresciani Ludvik
The clash of whether higher education should serve the public good or economic stimulation seems more alive than ever to some, and to others, it has come to an end. Not agreeing on the purpose of American higher education certainly makes it difficult to know whether educators are being responsible for delivering what is expected of them. Rather than reviewing the important debate that has already taken place, this chapter seeks to merge the two seemingly juxtaposed disagreements and discuss how bringing the two purposes together may influence how we examine accountability. As such, an inquiry model, including ways to gather and interpret institutional performance indicators for accountability is posited. Practical suggestions for implementation of this methodology are provided.
RELATED PAPERS
Community College Journal of Research and Practice
Juliann McBrayer
Lee O Farrell
Higher education's response to exponential societal shifts
Jillian Kinzie
Higher Education Studies
karma El Hassan
Matthew Gaertner
Monica Burnette
Ann Popkess
The International Review of Research in Open and Distributed Learning
Lynette Bryan
Liz Chinlund
Journal of College Student Development
Fred Newton
WHY SUPERVISORS MATTER FOR COLLEGE STUDENTS: THE RELATIONSHIP BETWEEN TYPE OF EMPLOYMENT AND STUDENT OUTCOMES
Dr. Justin Hultman
Heather Friesen
Meltem Ozmutlu
ANIMA Indonesian Psychological Journal
titik kristiyani
Shafeeka Dockrat
mohamed deen
International Journal of Learning, Teaching and Educational Research
Nahla Moussa
Richard Okundia
The Journal of Campus Activities, Practice, and Scholarship
Christy Moran Craft
MIRDEC-10th, International Academic Conference Global and Contemporary Trends in Social Science (Global Meeting of Social Science Community) 06-08 November 2018, Barcelona, Spain. ISBN: 978-605-81247-2-1. https://www.mirdec.com/barca2018proceedings.
Nazmi Xhomara
Journal of Hispanic Higher Education
Glai Martinez
The problem of college readiness, edited by William G. Tierney and Julia C. Duncheon
Julia Duncheon
Mohamed Tejan Tarawally Deen
Kristopher A Oliveira
David Edens
The Professional Counselor
Jeffrey Warren
Jefferson Chavez
International Journal of Knowledge and learning
David Cheng
Oscar Cervantes
Journal of Multidisciplinary Research
Cristina Lopez Vergara
Azucena Montoya
Journal for the Advancement of Educational Research
Dr. Justin Hultman , Dr. Daniel Eadens
Proceedings of the 2010 …
Hala Bayoumy
putri hazarianti
RELATED TOPICS
- We're Hiring!
- Help Center
- Find new research papers in:
- Health Sciences
- Earth Sciences
- Cognitive Science
- Mathematics
- Computer Science
- Academia ©2024
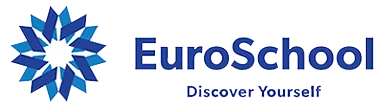
- NEP 2020 Curriculum
- Centre of Wellbeing
- LRPAX Model
- Balanced Schooling
- Safety Certification
- Co-Curricular Learning
- Digital Learning
- Chimney Hills CBSE
- Electronic City CBSE
- Yelahanka CBSE
- Bannerghatta CBSE
- Whitefield CBSE
- Airoli ICSE
- Balkum Thane CBSE
- Ghodbunder Thane ICSE
- Dombivli CBSE
- Upper Thane CBSE
- Kharadi CBSE
- Wakad (CBSE)
- Wakad (ICSE)
- Metro Campus CBSE
- Hitec City CBSE
- Hitech City CBSE
The Meaning, Necessity, and Significance of Academic Success
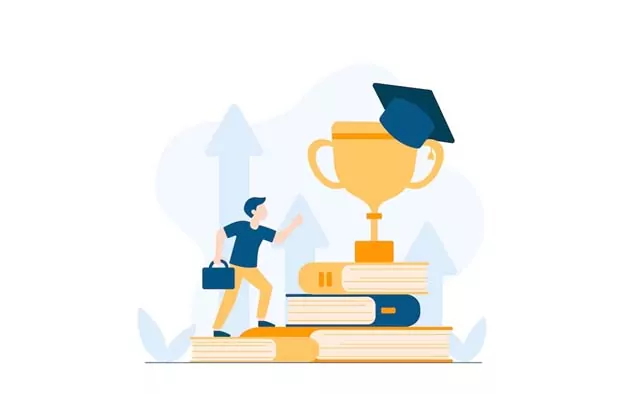
In today’s ever-evolving globalised world, academic success is a term that resonates with students, educators, parents, and policymakers alike. But what exactly does it mean, and why is it so highly sought after? This essay delves into the concept of academic success, presenting illustrative examples and pinpointing essential skills that contribute to achieving it.
Academic Success Meaning
Academic success, a phrase deeply embedded in educational institutions across the globe, refers to the fulfilment of educational goals set within an academic environment. However, it goes beyond just acquiring high marks in examinations; it encompasses a deep understanding of subjects, acquiring pertinent skills, and personal and intellectual growth facilitated through learning. It is pivotal to delineate that the benchmarks for academic success can vary substantially, influenced by individual personal goals, institutional policies, societal norms, and cultural nuances.
Academic success is a nuanced concept, marrying both tangible and intangible aspects of learning and development. It harbours a dual focus on performance and understanding, cultivating not just a repository of knowledge but a mind that is honed to think, analyze, and innovate. It remains rooted in the fostering of a lifelong passion for learning, encouraging individuals to persistently strive for intellectual and personal growth through educational pursuits.
Examples of Academic Success
Here are some examples of academic success:
- Achieving Top Grades:
The most traditional and widely recognised marker of academic success is the attainment of high grades or marks in exams and assessments. For instance, earning A*s in A-Levels or achieving a First-Class Honours degree at a UK university.
- Research and Publications :
For postgraduate students and researchers, academic success can also be gauged by their contribution to their field. This can be in the form of publishing papers in reputed journals or presenting findings at significant conferences.
- Skills Acquisition :
Successfully learning a new language or gaining proficiency in a laboratory technique can also be viewed as academic success, especially if it significantly augments one’s academic or professional arsenal.
- Participation in Academic Competitions:
Representing one’s school or university in academic contests, such as debates, math olympiads, or science fairs, and achieving commendable positions can be a tangible marker of success.
- Graduation and Further Studies:
Completing a course of study, be it at secondary school or university level, is in itself a monumental marker of academic success. Furthermore, securing admission for further studies in reputed institutions can also be seen as an extension of one’s academic accomplishments.
- Skills Vital for Academic Success:
Achieving academic success is not a result of mere luck or inherent genius. More often than not, it’s a culmination of various skills, both inherent and acquired, that a student harnesses over time. Some of the pivotal skills include:
- Time Management:
With multiple assignments, readings, and other commitments, the ability to manage one’s time effectively is paramount. This doesn’t just involve creating schedules, but also prioritising tasks and setting realistic goals.
- Effective Study Techniques:
Successful students often employ a range of study techniques that enhance their understanding and retention. This might include strategies like the Feynman Technique, active recall, or spaced repetition.
- Critical Thinking:
This skill extends beyond rote learning. It involves analysing information, discerning patterns, questioning assumptions, and arriving at informed conclusions. In the British education system, with its emphasis on essay writing and argumentation, critical thinking is especially valued.
- Communication Skills:
Effective communication is two-fold. Firstly, it’s about understanding information (listening or reading comprehension). Secondly, it’s about articulating ideas clearly, whether through essays, presentations, or discussions.
- Self-motivation and Discipline:
Internal motivation often trumps external pressures. A student who is genuinely passionate about a subject or is intrinsically motivated to excel will find the discipline to study consistently, even when external pressures are absent.
- Adaptability:
The academic landscape is not static. New methodologies, technologies, and theories continually emerge. The ability to adapt, evolve, and remain open to new ideas is a crucial asset in the pursuit of academic excellence.
- Seeking Help When Needed :
Academic success is not synonymous with working in isolation. Recognising when one needs assistance, whether in understanding a topic or dealing with stress, and seeking out resources or support, is a sign of a proactive and successful student.
Skills required for Academic Success
Here are some skills that students might need for academic success:
Proficiency in managing one’s time efficiently allows students to handle a multifaceted array of tasks with composure and efficacy. This skill extends beyond mere scheduling, encompassing the aptitude to prioritise tasks, set realistic goals, and avoid procrastination.
- Research Skills:
At various stages of the academic journey, students are required to undertake research, be it for a simple school project or a university dissertation. Developing the ability to find, analyse, and synthesize information from credible sources is pivotal.
Critical thinking underpins a student’s ability to evaluate information deeply and from multiple angles, enabling them to develop well-informed arguments and solutions. It encourages curiosity, investigation, and reflective judgment.
Being able to express one’s ideas lucidly and effectively, both in written and verbal form, is indispensable. It also includes active listening and reading comprehension, understanding and absorbing the information conveyed through various mediums.
- Note-taking and Summarisation :
Learning the art of jotting down pertinent points and summarising large swathes of information into concise notes can aid in better retention and understanding.
- Study Techniques :
Employing effective study techniques, such as the Feynman Technique, active recall, or spaced repetition, can enhance a student’s learning process, making study sessions more productive.
- Digital Literacy :
In a digitally interconnected world, the ability to navigate and utilise digital tools and platforms efficiently has become increasingly important. It includes a basic understanding of digital safety and etiquette.
Academic success, while often quantified by grades and accolades, is a multi-faceted concept that transcends mere numerical achievements. It’s about the journey of learning, the skills honed, and the knowledge acquired. As the world continues to evolve, the skills and attributes that define academic success might shift. However, the core principle remains unchanged: it’s about striving for excellence, pushing one’s boundaries, and cultivating a lifelong passion for learning.
EuroSchool is committed to helping students achieve academic achievement. The school offers a welcoming learning atmosphere, knowledgeable faculty, and a thorough curriculum with a focus on the student. We give kids the tools they need for academic performance by encouraging critical thinking, effective communication, and discipline. Because of the school’s dedication to individualised instruction and holistic development, children succeed academically and develop into well-rounded persons who are prepared to succeed in their academic endeavors and future endeavours.
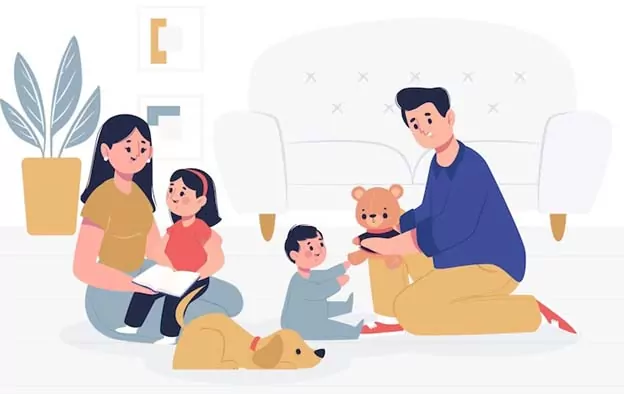
Recent Post
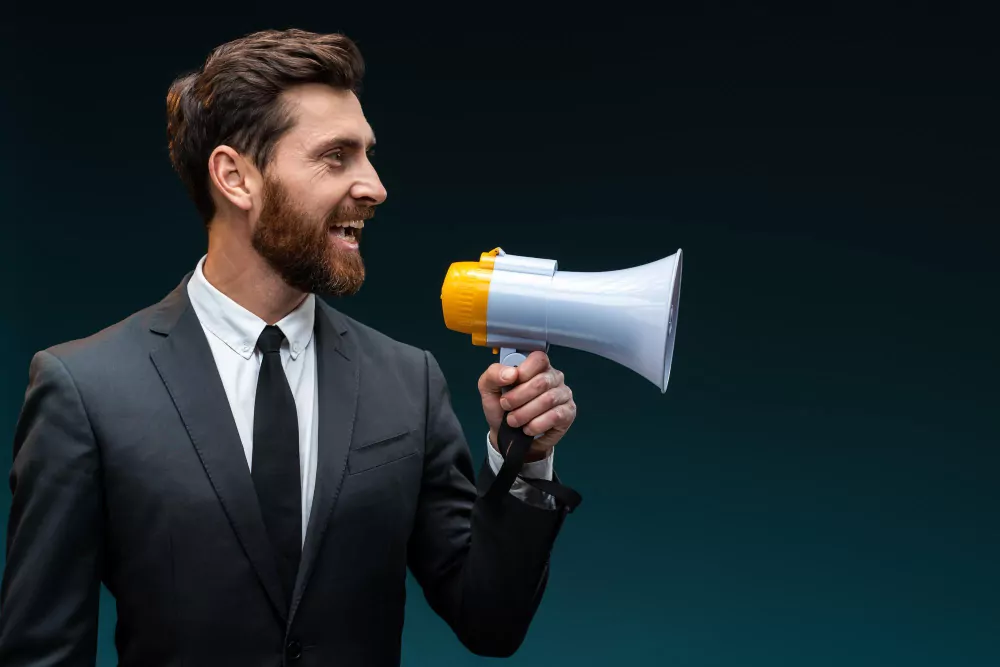
- Behaviour & Discipline
- Child Development
- Important Days
- Parent's Zone
- Play & Activities
WHY EUROSCHOOL
- Testimonials
OUR SCHOOLS
- Undri (ICSE)
To Know More, Email or Call us at :
Digital Marketing Services
© Copyright EuroSchool 2024. All Rights Reserved.
Admission Enquiry
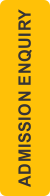

An official website of the United States government
The .gov means it’s official. Federal government websites often end in .gov or .mil. Before sharing sensitive information, make sure you’re on a federal government site.
The site is secure. The https:// ensures that you are connecting to the official website and that any information you provide is encrypted and transmitted securely.
- Publications
- Account settings
Preview improvements coming to the PMC website in October 2024. Learn More or Try it out now .
- Advanced Search
- Journal List
- CBE Life Sci Educ
- v.20(1); Spring 2021
Success for All? A Call to Re-examine How Student Success Is Defined in Higher Education
Maryrose weatherton.
† Department of Ecology and Evolutionary Biology, University of Tennessee, Knoxville, TN 37996
Elisabeth E. Schussler
Associated data.
A central focus in science education is to foster the success of students who identify as Black, Indigenous, and people of color (BIPOC). However, representation and achievement gaps relative to the majority still exist for minoritized students at all levels of science education and beyond. We suggest that majority groups defining the definitions and measures of success may exert “soft power” over minoritized student success. Using a hegemonic and critical race theory lens, we examined five years of research articles in CBE—Life Sciences Education to explore how success was defined and measured and what frameworks guided the definitions of student success. The majority of articles did not explicitly define success, inherently suggesting “everyone knows” its definition. The articles that did define success often used quantitative, academic outcomes like grade point average and exam scores, despite commonly cited frameworks with other metrics. When students defined success, they focused on different aspects, such as gaining leadership skills and building career networks, suggesting a need to integrate student voice into current success definitions. Using these results, we provide suggestions for research, policy, and practice regarding student success. We urge self-reflection and institutional change in our definitions of success, via consideration of a diversity of student voices.
INTRODUCTION
Within the United States, there has been a substantial increase in minority populations over the last 10 years, with the United States projected to be “majority minority” by 2045 ( Vespa et al. , 2018 ). However, this demographic shift has not been mirrored within the scientific disciplines in higher education; while more than 30% of the U.S. population identifies as Black, Indigenous, or other person of color (BIPOC), those groups represent only 21% of science, technology, engineering, and mathematics (STEM) bachelor’s recipients. Furthermore, BIPOC only represent 13% of STEM doctoral recipients, 11% of the STEM workforce, and 4% of R1 faculty ( National Science Foundation [NSF], 2018 ). The trend is similar within the domain of biological sciences, where BIPOC students represent 12% of earned doctorates and 11% of postdoctoral fellows ( Meyers et al. , 2018 ). These differentials in degree acquisition and faculty representation can cascade down to undergraduate and secondary education students. For example, universities lacking diversity in their faculty ranks may see increased stereotype threat to BIPOC students ( Nouwen and Clycq, 2018 ; Park et al. , 2020 ). This can inhibit students’ development of a sense of belonging ( Good et al. , 2012 ; Hurtado et al. , 2015 ), and negatively affect students’ persistence, well-being, and academic achievement.
Differentials in student success have been discussed for at least a quarter century within education research ( Panos and Astin, 1968 ; Boland et al. , 1978 ; Leman, 1999 ), with countless interventions, theoretical models, and diversity initiatives proposed to increase the success of BIPOC students at all levels STEM education. However, the state of BIPOC representation and persistence would suggest that these initiatives have not succeeded within STEM domains broadly or the field of biological sciences specifically. We argue in this Essay that the assumed definition of “success” and its associated metrics may be one factor hindering the very success of the populations we seek to advance.
What Is Success?
To study and facilitate student success, we must first understand what we mean when we say “success.” As with other broad terms like “interest” ( Rowland et al. , 2019 ), the term “success” has a variety of meanings both within and outside the domain of biology education. Student success can be seen in terms of outcomes , like persistence, increase in self-efficacy, and publication rate. However, these concepts can just as easily be seen as components that facilitate success if it is defined as achieving a particular goal. Furthermore, there can be a stark difference in how faculty and students define success ( Dean, 1998 ; Thompson and Jensen-Ryan, 2018 ), as well as how minoritized students (which we use here to include BIPOC, first-generation, low-socioeconomic status, or other underrepresented groups of students) and their majority counterparts define the term ( Tierney, 1992 ; Goyette and Xie, 1999 ; Oh and Kim, 2016 ; O’Shea and Delahunty, 2018 ). For example, in a study at a public California university, Oh and Kim (2016) found that Korean-American undergraduate students most often defined success as reaching the highest levels of academic achievement, while Mexican-American students most often defined success as going to a 4-year university, surpassing the success of their parents, and helping future students find success ( Oh and Kim, 2016 ). These definitions were further aligned with different support needs and levels of student satisfaction. Thus, the meaning of student success depends on the context as well as who you ask, making a singular, unifying definition largely impossible.
Within biology education, we believe that our current definitions of success may lack the requisite diversity to fully capture the contexts of all students. These definitions inform the metrics used in, and conclusions drawn from, empirical research in the field. These, in turn, inform the policies and practices we advocate for, which ultimately affect student outcomes ( Figure 1 ). These definitions are most often created and maintained by those who hold power in the field (e.g., researchers, faculty members, deans, and other university staff), and rarely include meaningful student input, especially from minoritized populations. If these definitions are not created by and for a diverse population, then there will be cascading impacts on outcomes for those left out of the conversation.

How success is defined impacts almost every facet of the research process, as well as policies and practices, and ultimately affects student outcomes. Researchers’ definitions of success will impact how they choose to measure the construct, which in turn will impact how data are interpreted and thus what recommendations are proposed. These recommendations often have real-world impacts on student outcomes in higher education in the form of changes in policy or practices such as pedagogies or departmental requirements.
We argue that our current limited discourse around the meaning of student success is maintained, in part, by social hierarchies that can be examined through the lens of hegemony and critical race theory, which are described in the next section. These forces unwittingly reinforce and reproduce social hierarchies within our education system that may hinder the success of certain student populations who may not define success in the same way the majority does. Thus, we argue that a critical evaluation of success within higher education is imperative. In this Essay , we hope to start a conversation surrounding what success means, who should define it, and how an expansion of our definitions may help to facilitate the success of all students.
Why Social Hierarchies Are Relevant to the Definition of Success
The ideas presented in this Essay assume an institutional reproduction of social hierarchies and are framed by cultural hegemony and critical race theory. Gramscian hegemony is used to explain the power relations between dominant and minority groups. Specifically, it explains the ways in which dominant groups exert “soft power” over nondominant groups to secure and maintain control within society ( Gramsci, 2000 ; Borg et al. , 2002 ). This is accomplished via manipulation of cultural beliefs, language, values, and norms to establish the dominant group’s worldviews as universal, natural, or common sense. These understood rules of society are often invisible, yet powerful. Alternative perspectives, norms, and values are actively discredited by making them seem counterintuitive or unnecessary ( Grimm, 2015 ). However, these counterhegemonic ideas are often the values and perspectives held by nondominant members of society. Hegemony is thus maintained, because one cannot hold dissenting ideologies and also achieve social mobility, reinforcing existing social hierarches and forcing minority groups to conform to these dominant structures ( Dawson, 1982 ; Gramsci, 2000 ).
We can examine the hegemonic forces underlying student success using ideas from critical race theory (CRT). CRT challenges dominant narratives around race and racism in education and identifies how these narratives are often used to subordinate minoritized groups and maintain white supremacy in the United States ( Solorzano 1997 ; Yosso, 2005 ; Ladson-Billings and Tate, 2006 ). Solorzano and Yosso (2001) point out that racial stereotypes form the basis for the dominant “deficit notions” of BIPOC. For example, a common narrative in the United States is the idea of meritocracy, or “pulling yourself up by the bootstraps,” whereby minoritized groups can find success if they work hard enough ( Collins, 2018 ; McGee, 2020 ). This narrative shifts blame for unequal outcomes (e.g., wage gaps, lowered academic persistence and achievement) to minoritized groups who “don’t work hard enough” or are deficit in some other way, instead of recognizing the structures and institutions that center power with the majority. Within academia, these stereotypes establish a cultural norm that is upheld through differences in expectations, school funding, and punishment between BIPOC and white students (i.e., the school-to-prison pipeline; Solorzano, 1997 ; Barnes and Motz, 2018 ). While seemingly inert, these dominant frameworks translate into policies and structures that harm minoritized students ( Solorzano and Yosso, 2001 ). For example, school tracking systems ( Rosenbaum, 1976 ; Southworth and Mickelson, 2007 ), intelligence testing ( Rose, 1976 ; Solorzano and Yosso, 2001 ), and disparate resource availability ( Tate, 2008 ; Green et al. , 2017 ) have historically been used to maintain social hierarchies by advancing the education of white students while justifying modern-day segregation and deficit notions of BIPOC students ( Solorzano and Yosso, 2001 ; Yosso, 2005 ; McGee, 2020 ). Using a CRT lens allows us to explore how our current definitions and metrics of success do not come from neutral, unbiased, or meritocratic ideals, as is often assumed; instead they have been founded on racist principles. We believe that acknowledging this is an important first step to counter these hegemonic forces and begin to redress the harm that they have done to our students.
Linking hegemony and CRT to the concept of student success, we see that majority power can be maintained in higher education by normalizing a restrictive view of student success (e.g., success as having a high grade point average [GPA]). By focusing on outcomes like productivity and employability, these dominant definitions of success ignore large parts of students’ well-being (e.g., social, cultural, or personal outcomes). This can be harmful to students who hold “alternative” definitions of success by making them feel as if they do not belong within academia ( Hurtado and Carter, 1997 ; Hurtado et al. , 2015 ; Goyette and Xie, 1999 ; Tibbetts et al. , 2016 ). For example, in a study of Latinx undergraduates, Hurtado and Carter (1997) found that students’ GPAs were not significantly associated with their sense of belonging. For these students, participation in nonacademic activities like membership in religious or community organizations was significantly related to students’ sense of belonging ( Hurtado and Carter, 1997 ). This example suggests that a focus on academic metrics versus other measures, such as social participation, may impede the retention of some minoritized students. Many CRT scholars have noted that within the United States education system, BIPOC students are forced to “assimilate” into dominant, Eurocentric paradigms, including those surrounding work ethic, educational values, and definitions of achievement ( Carter and Segura, 1979 ; Solorzano and Yosso, 2001 ; McGee, 2020 ). Minoritized students in academia are thus faced with two choices: integrate into an environment that is misaligned with their identities and actively discredits their beliefs or leave the system ( McGee, 2020 ). When they choose to leave, this attrition of minoritized students from academia not only prevents the field of biology from achieving diversity and equity goals, but it also limits minoritized students’ social mobility and therefore reinforces existing social hierarchies.
Certainly, we do not think that institutions or educators who promote individual, quantitative definitions of success do so as an active choice to suppress minoritized students. Because hegemonic power flourishes when cultural norms are taken for granted, ideas of what makes a student successful are often built into our academic systems and assumed to be universally true. Even when educators and researchers may wish to reimagine how success is defined and evaluated, institutional structures like yearly evaluations based on pass/fail rates or GPA requirements for degree progression may leave little room for them to introduce “alternative” definitions of success, such as positive mental health, internal development, or community-based outcomes. Education researchers, as intellectual leaders in the field, have an opportunity to examine and resist dominant social hierarchies by refusing to support hegemonic structures while voicing counter-hegemonic structures and narratives. Foucault (1997) suggests that the “reproductive power” of cultural hegemony can be resisted and fought against through critical discourse and inviting new voices into the conversation. It is indisputable that academic definitions of success are both relevant and useful within higher education; however, we argue that alternative views of success must be normalized to advance institutional and societal goals. Thus, by listening to new voices (e.g., amplifying minoritized students’ views of success) and considering new definitions of student success, education researchers can take the first step toward changing the system that is preventing students from achieving success equally.
How Biology Education Researchers Define Success
One window into the discourse around student success can be found in the empirical literature investigating student success in higher education. We believe that current definitions of student success are limited, and that this is true across STEM domains. However, we have chosen to support our argument by sampling the literature within the domain in which the authors have the most knowledge—biology education. To sample how success is discussed and defined in the literature specific to this domain, we explored education research articles that discussed student success in CBE—Life Sciences Education ( LSE ) over the past 5 years. We examined 1) whether and how success was explicitly defined within research articles and 2) how success was measured. We also noted what theoretical frameworks seemed to be shaping research on student success. This literature examination was restricted to biology education and not intended to be comprehensive. Instead, this examination is used as an example of how probing the term “success” can reveal a need for researchers to re-examine their assumptions as well as consider how different metrics and a diversity of student voices may lead to a more complete definition of what it means for students to be successful.
We used a standard literature review methodology to collect these data ( Creswell and Creswell, 2017 ). We searched the online database of LSE on June 14, 2020, using the term “success,” and limited our search to articles published within the last 5 years (2015–2020). This primary search returned 311 articles, which we further narrowed by selecting only research articles (i.e., not reviews, meeting reports, or editorials). After applying this filter, we were left with 248 results.
As we were specifically interested in how success was defined in higher education, we excluded articles that examined student success in K–12 educational settings. Furthermore, we excluded articles that evaluated the success of specific curricular programs (e.g., research examining the success of an intervention aimed at reducing gender bias) as opposed to research on student success, as well as research papers about instructors and faculty, as they did not explicitly study student success. We did not have any exclusion criteria related to subject domain (e.g., math success, physics success, etc.) though most articles published in LSE are related to life sciences education. We also did not exclude any articles on the basis of study time frame or analysis approaches. This left 52 articles related specifically to research on student success in higher education over the last 5 years (see the Supplemental Material for the list of these articles).
The Majority of Articles Discussing Student Success Did Not Explicitly Define the Term
Of the 52 articles, 21 (40%) gave explicit definitions of student success. The other 31 defined student success implicitly through the variables they measured, often equating student success with quantitative student outcomes, such as exam scores and GPA. Of the papers that explicitly defined success, there were four broad categories for how the concept was defined: academic, persistence, career, and social (see definitions in Table 1 ). Academic definitions (e.g., grades, GPA) were the most prevalent in the literature (80%), followed by persistence (e.g., remaining in major) definitions (47%). Career definitions included obtaining a job in STEM (15%). The least common category was social definitions (4%), with only one paper explicitly defining success as it was related to students becoming leaders in their communities. Each article with an explicit definition could be placed in one or more of these four categories.
Categories of explicit definitions of student success represented in the literature sample ( N = 21 articles that defined success explicitly out of 52 total articles)
There Were Many Different Ways to Measure Success, and Most Were Quantitative
Overall, there were 30 distinct measurements of student success in the articles, 13 of which were mentioned more than once. The majority of papers (88%) measured at least one quantitative outcome related to student success; only six papers captured solely qualitative metrics. Of the papers that measured quantitative outcomes, the most common measure of student success was a suite of persistence measurements, followed by exam scores and course grades ( Table 2 ).
Measurements of student success (outcome variables) represented more than once in the literature sample ( N = 52)
Persistence as a measure of student success came up often and in many different variations in the articles. In total, 20 articles (38%) measured student success as some aspect of persistence, attrition, or retention ( Table 2 ). We separated these into five subcategories based on the authors’ description of the outcome variable. Articles that reported “retention” and “persistence” generally were sorted into the first subcategory: “persistence/retention general.” Most often, papers in this subcategory measured persistence or retention in a degree program. Further, “persistence/retention in major” and “persistence/retention in a STEM field” were two different subcategories, with the former being explicitly about changes in students’ declared major, while the latter included postgraduation outcomes, like attaining a career in a STEM field or continuation to a STEM graduate degree. The outcome “intention to persist/remain” was based on student expectations versus actual retention or persistence from a major or course. Finally, there was only one article within the subcategory of “attrition” ( Wilson et al. , 2018 ); this article measured the percent of graduate students that left their programs without an MS or PhD degree over a period of 8 years.
Theories of Student Success Have Changed over Time
As part of the framing for their student success studies, many of the articles cited one or more theories or theoretical frameworks that guided their work. Across the articles we examined, 23 theories were cited, and five were mentioned within multiple papers: self-efficacy, identity, sense of belonging, social cognitive career theory, and social interdependence theory. Theoretical frameworks can influence almost every aspect of a study, from how the research questions are framed, to how concepts are understood and defined, what data are collected, and how the results are interpreted and situated within the broader field ( Anfara and Metz, 2014 ; Creswell and Creswell, 2017 ; Rowland et al. , 2019 ). In studies of student success, the theoretical frameworks that researchers chose likely influenced how they defined success or the success outcomes they hoped to measure. These theoretical frameworks, then, can be vehicles of hegemonic influence that set the standard for how student success is measured and discussed and are thus integral to consider. We will discuss the evolution of the discourse around student success in order to add context to these frameworks and inform our discussion about how to expand our definitions of success.
Although the term “student success” has been discussed in the education literature for more than a century ( Carmichael, 1913 ; Alexander and Woodruff, 1940 ; Brogden and Taylor, 1950 ), most of the theories or frameworks mentioned in the articles we examined were more recent in origin. The five most commonly cited theories in the articles originated within the biology education literature over the past 30 years. However, this empirical research was built on work done in the past, meaning that even these newer conceptions can have old ideas embedded in them that perpetuate racist stereotypes and ideals.
Popularized in the 1950s and 1960s, universal quantitative measures (e.g., ACT, Scholastic Aptitude Test, Graduate Record Examination scores) were some of the first measures of student success ( Capps and Decosta, 1957 ; Kunhart and Olsen, 1959 ). Early theories of student success proposed success as an outcome based on inherent qualities, like personality ( Robertson and Hall, 1964 ). In the 1970s and 1980s, work on student socialization and integration popularized one of the most commonly used metrics of success, persistence ( Table 2 ). Theories during this time built upon previous work by examining how students’ personal characteristics impacted their interactions with their environments, like Tinto’s (1975) theory of student attrition. As the “positive psychology” movement gained traction in the 1990s and 2000s, more modern theories layered “internal development” factors, like motivation and self-regulation, on top of the interactions among personal characteristics and proximal environmental influences to explain student success. For example, social cognitive career theory claims that increasing students’ feelings of self-efficacy and providing them with relevant learning experiences can mediate background and proximal environmental influences on their career decisions and goal attainment ( Lent et al. , 2002 ). This layering introduces new ideas but retains core older ideas about student success.
While new ideas of success push the field forward, much of the discussion is still framed by antiquated, racist notions that undermine these theories’ ability to reflect the experiences of BIPOC students. For example, Binet’s IQ test has been used to justify “genetic determinism” models of minority education equality ( Rose, 1976 ; Solorzano and Yosso, 2001 ). And although the field’s most influential theories are assumed to be broadly applicable, many of them were developed solely on the basis of majority students. For example, Tinto’s influential institutional departure model ( 1975 ) has been critiqued for its exclusive study of “traditional” students (i.e., white, upper-class students) at “traditional” universities (i.e., primarily white, residential, 4-year institutions; Astin, 1985 ; Attinasi, 1989 ; Tierney, 1992 ; Tinto, 1993 ). These limited populations bounded the results drawn from the theory. This has led, for example, to the problematic conclusion that students must detach from their previous communities in order to find success in higher education. Indeed, the opposite has been found for minority and first-generation students, many of whom draw strengths from their connection to their home communities and cultures ( Muñoz and Maldonado, 2012 ; Yao, 2015 ; Burt et al. , 2019 ).
The use of majority students as the foundation for the theories central to the ideas underlying student success is not only problematic, but harmful to minoritized students. When historically underrepresented students do not meet the standards of success established by the theories (e.g., higher attrition rates, lower course grades, etc.) it is assumed that the deficiencies are on the part of the students, and not the theory. As previously mentioned, this “deficit notion” of BIPOC students prevents equitable outcomes between BIPOC and white students. It also forces minoritized students to choose whether to conform to the majority standards of success or live within a system that does not value what they consider to be successful.
Despite the expansion of our theoretical understanding of student success over the past century, many of our definitions and metrics of success have been stubbornly unchanging. We acknowledge that the practicality and ease of quantitative metrics may be one reason why they are so prominent when measuring student success. It takes much less time to gather the course grades of each student in an introductory biology class than it does to collect interview data about their perspectives on success in the course, for example. Furthermore, latent constructs like student well-being or identity are more difficult to measure compared with academic metrics like GPA, as the former are made up of multiple, diverging indicators, and alternative ways to measure these constructs may not yet have been developed and validated for wider usage. Of course, quantitative metrics can have predictive value and can be excellent tools to answer many research questions. However, we argue that measuring quantitative outcomes is not a panacea for understanding how students achieve success in academia. Thus, we argue that, without addressing the ideas that underlie our notions of success, the field will continue to struggle to address the needs and facilitate the success of all of our students.
Who Gets to Define Student Success?
Of the 52 articles in LSE that discussed student success over the past five years, only one article captured students’ own definitions of success, suggesting a paucity of research in this area. This presents an issue if students have different definitions of success than those who determine the field’s definitions, like researchers and institutional decision makers. Indeed, Thompson and Jensen-Ryan (2018) found that undergraduate students expressed definitions of success that included academic outcomes like graduation as well as emotional outcomes, such as not getting discouraged and increasing their self-efficacy and self-confidence. Many students from historically underrepresented backgrounds (e.g., BIPOC, low-socioeconomic status, first-generation students) reject traditional definitions of success. For example, O’Shea and Delahunty (2018) found three themes of success that emerged from interviews with first-generation undergraduates: success as a form of validation, success as defying the odds, and success as positive feelings about one’s trajectory. Interestingly, many of the students interviewed had very clear ideas of what success was not , including obtaining high grades or passing exams ( O’Shea and Delahunty, 2018 ).
Considering these examples of how students’ and researchers’ perspectives on success can differ, we ask why student voices are so rare in the literature on student success. One explanation goes back to the idea of hegemonic power, wherein members of the majority impose their worldview as cultural “common sense” ( Boggs, 1976 , p. 39). Within academia, hegemonically imposed worldviews can present themselves via the belief that definitions of certain terms are homogeneous and that explicitly defining terms like “success,” “persistence,” and “interest” is not necessary. Indeed, our examination of the literature on student success found that success was only explicitly defined in 40% of the articles we examined. Even when success is defined, researchers and other institutional stakeholders may experience difficulty thinking beyond traditional definitions, as they often realized their positions via traditional success measures (e.g., academic success). Thus, the problem becomes apparent—if most researchers define success in similar ways, and these definitions are validated by achieving intended career outcomes, then these definitions are taken for granted and seen as common sense. Therefore, researchers may not see the need to gather student perceptions of any concept whose definition seem so inherent.
In this way, hegemonic influence is hidden within everyday facets of academia, like the language we use to describe and define concepts. However, a critical examination of the discourse surrounding these concepts can reveal a startling lack of ideological diversity. We argue that the hegemonic framing of embedded discourse prevents the field from moving forward toward more inclusive definitions and metrics. To resist this framing, we must collectively examine and make concrete changes to many of the aspects we have discussed here: how success is defined and measured and whose success we are concerned with. As a community of researchers, we have the collective power to expand our own and our institutions’ discourse in order to validate and facilitate all students’ definitions of success. To that end, we present recommendations in the realms of research, policy, and practice that aim to amplify minoritized student voices, encourage deep self-reflection, and bring about equitable institutional change.
RECOMMENDATIONS
The purpose of this Essay was to examine success through a hegemonic and CRT lens to inform a collective discussion about how our current perspectives on student success may be contributing to unequal attainment of success. Despite a concentrated effort over the past 25 years to increase BIPOC “success” in STEM domains, gaps between BIPOC students and their majority counterparts still exist at all levels of STEM education and beyond ( NSF, 2018 ; Meyers et al. , 2018 ). Although the field’s ideas and theories of student success have evolved and gained more nuance over the past 70 years, our definitions and measures still fall short of being fully inclusive. Our limited examination suggests that current definitions and metrics of student success are mostly academic and quantitative and are most often defined by institutional-level stakeholders, such as researchers. Although these theories were developed via research directed at students, there is a paucity of literature that directly asks students their views on success in higher education. Here, we propose recommendations for individual researchers to more explicitly consider success and center student voice in their empirical work. We then provide recommendations for the community as a whole within the areas of research, policy, and practice.
Recommendations for Individual Researchers
To encourage researchers to more critically examine their potential role in the reproduction of social hierarchies in academia, we recommend that they consider their own definitions of student success and how these definitions influence their empirical work. Furthermore, we encourage researchers to consider what definitions of success are highlighted within their research and how they can amplify diverse perspectives and voices within that research. Explicitly considering what perspectives of success they intend to use before beginning their projects will allow researchers to clearly ground their work and accurately describe what they intend to study, which in turn will lead to clarity in definitions, proposed metrics, and interpretation of results.
To foster these reflections, we model from Rowland et al. (2019) the presentation of guidelines and associated guiding questions for researchers to use as they begin projects investigating student success in higher education:
- Before beginning their research, researchers must self-reflect on the biases, hegemonic frames, and societal norms that they have internalized simply as members of society. Much like the qualitative practice of bracketing, this process will not rid researchers of any biases, but makes them visible, so researchers can reflect on how these biases may shape their interpretations ( Creswell and Miller, 2000 ). For resources to guide self-reflection and examination of internal biases, see Gullo et al. (2018) , Killpack and Melón (2016) , Project Implicit (2011) , and Racial Equity Tools (2020) .
- i. Does my funding source require institutionally relevant data, like student retention or GPA? If so, does my definition align with these metrics? Do I need to add a second definition if I am collecting other metrics?
- ii. How diverse is my intended study population? Are there definitions of success that may more closely align with the views of my intended population?*
- iii. How might my study accurately capture the perspectives of minoritized students, as opposed to invoking “safe multiculturalism” (i.e., an unchallenging, stereotypical, or tokenized view of a culture)? For more information on safe multiculturalism, see May and Sleeter (2010) and Yancy (2016) .
- iv. Do my intended research questions and theoretical frameworks align with the definition(s) of student success I am considering?
- i. Based on my definition, how many measures of success do I need to use?
- ii. Should the definition of success be captured using quantitative or qualitative metrics? If I can use both, is one more appropriate given my intended population,* study context, and time constraints?
- iii. Am I sure that students define success in the way I am intending to measure it?*
- i. Have I chosen this definition of success over others simply because its associated metrics are easy to measure?
- ii. Have I explicitly defined success in any communications about my project?
- iii. Have I sufficiently articulated a rationale for my definition and measures?
- iv. How might the definition of success used in my project be a potential limitation of the study? Have I acknowledged that as part of the study?
*If the answers to some of these questions are unknown, researchers may want to consider a pilot study or a qualitative inquiry into these students’ definitions of success.
We hope the use of these guidelines and associated questions will help researchers appropriately conceptualize success in each study they undertake and encourage researchers to consider how to capture diverse voices and perspectives within their research.
Recommendations for Research, Policy, and Practice
The following section focuses on actions the community can take to disrupt the current thinking about student success in order to reframe it for all students. To reflect the full diversity within STEM, and in consideration of the lack of student voice in the current literature, we suggest a need to truly listen to student perspectives on success at all levels. Lack of student voice is widespread across many domains; indeed, this issue has garnered the attention of the United Nations, which in 2009 proposed “General Comment No. 12—The Right of the Child to be Heard” ( UN, 2009 ). In 2002, the UN Committee on the Rights of the Child (UNCRC) documented its concern that “in education, schoolchildren are not systematically consulted in matters that affect them” ( UN, 2002 ). We suggest that similar issues are present in higher education, even though the majority of our students are considered adults.
In 2007, Lundy proposed a model to conceptualize article 12 of the UNCRC, and we are adapting it here to guide discussions about how to highlight student voice in research on student success and bring meaningful change within the realms of policy and practice ( Figure 2 ). Article 12 delineates two key rights: 1) the right to be heard and 2) the right to have one’s views given due weight ( Lundy, 2007 ). To successfully implement article 12, Lundy proposed that four elements must be considered: space, voice, audience, and influence. The first two elements, space and voice, relate to students’ rights to be heard. The next two elements, audience and influence, relate to students’ rights to have their views given due weight. We propose that the first two elements of Lundy’s model can be used to inform recommendations for research, and the latter two elements can be used to inform recommendations for research, policy, and practice.

This figure highlights four key elements from Lundy’s model, two relating to one’s right to express a view—space and voice—and two relating to one’s right to have their views given due weight—audience and influence. These rights are highly interrelated, and when examined in an academic context, lead to an iterative process for working with students to bring equity to the domains of policy, practice, and research.
Students’ Rights to Be Heard—Recommendations for Research.
A prerequisite for meaningful engagement with students requires the recognition that their opinions are necessary and valid. In regard to discussions of student success, this means we must create space in our discourse where alternative definitions of success are not only allowed but are honored as equally valuable. We believe that the process of making this space can start with this Essay and subsequent conversations. This space will allow the field to begin the process of examining the current hegemonic structures that dominate academia and work to dismantle them.
Student voice is critical to this process. This can be accomplished either informally, through conversations between students and mentors; or formally, through qualitative research regarding how students define success. Involving students in the research process is an empowering way to amplify student voice in our discussion of student success. When applicable, we suggest that researchers employ a community-based participatory research approach, a research strategy that equitably involves community members and researchers in a way that seeks to validate community members’ expertise and empowers non-researchers by sharing the decision-making process (see Minkler and Wallerstein, 2011 ). We also point out that the need to listen to students includes both undergraduate and graduate students. Research on graduate student success is even more limited than for undergraduates, and given the high attrition of graduate students from STEM degree programs ( Chen, 2013 ; Sowell et al. , 2015 ), it is important to also capture their unique perspectives. These conversations can add critical, new perspectives needed by researchers and institutional stakeholders to steer the field in a new direction.
Students’ Rights to Have Their Views Given Due Weight—Recommendations for Research and Policy.
Lundy (2007) recognized that making space and gathering student voice do not necessarily mean that student perspectives will be appropriately heard or acted upon. Indeed, ensuring students maintain their rights is often difficult, especially when their views challenge the dominant thinking, are expensive to enact, or cause controversy ( Lundy, 2007 ). It is important that we are attentive to the latter two elements in Lundy’s model—audience and influence—as merely gathering student perspectives without enacting change (i.e., tokenizing student voice) will not combat systemic issues and may, in fact, be counterproductive ( Alderson, 2000 ).
Students’ perspectives must be presented to the appropriate audiences in order for them to have any influence over research, policy, or practice. Thus, stakeholders at all levels of academic institutions must be exposed to these voices. This can be accomplished broadly through publication and presentation of work that foregrounds student voice and locally through initiatives that present students’ opinions to provosts, deans, department heads, faculty, and other university leadership staff to inform new policies. The UNCRC has warned that “appearing to ‘listen’ to children is relatively unchallenging; giving due weight to their views requires real change” ( UN, 2003 , para. 12).
Individuals (e.g., researchers, students, and staff members) must be engaged in the process of amplifying student voices and expanding our understanding of student success. However, for these recommendations to have lasting effects, the need to expand how success is defined and measured must be understood and enacted by academic institutions and organizations in the field (e.g., NSF, Association of American Universities, American Academy for the Advancement of Science [AAAS]). We discuss this more in the following section. Further, while we present two broad policy suggestions, we urge policymakers to see students not just as respondents or data points, but as partners and cocreators in establishing appropriate policy that serves their unique student populations. For a more in-depth guide to including students in this process, see Lygo-Baker et al. (2019) .
First, we suggest that universities have a policy to incorporate nonacademic measures of success into components of course work or within graduate student evaluations. Many studies have revealed that student mental health and persistence, among other outcomes, are highly impacted by nonacademic factors (i.e., not related to course or grade outcomes) such as creation of a professional network ( Sweitzer, 2009 ), development of metacognitive regulatory strategies ( Green and Macauley, 2007 ; Efklides et al. , 2018 ; O’Shea and Delahunty, 2018 ), and establishment of students’ academic identities ( McAlpine and Amundsen, 2009 ; Oh and Kim, 2016 ; Schnoes et al. , 2018 ). Depending on students’ definitions of success, these factors may be as important, if not more important, than traditionally evaluated outcomes, like GPA, exam scores, or time to degree. This policy would work to honor alternative definitions of success and thus increase sense of belonging for all students in academia. Moreover, incorporating a larger diversity of success metrics will grant advisors, departments, and institutions a more holistic picture of student development and progress.
Second, we suggest that universities add or amplify nonacademic support for students that honors alternative definitions of success. The current hegemonic framing of student success encourages a large amount of institutional support to go toward facilitating academic definitions of success (e.g., tutoring centers, peer coaches, academic advising). While these supports are useful and integral to many students, support structures for nonacademic definitions of success can be equally important to students. Thus, we suggest directing more funding to services such as career centers, which support career-based definitions of success ( Gallup, 2016 ); multicultural student centers and groups, which support community-based definitions of success ( Bell, 2016 ; Tachine et al. , 2017 ); and mental health support, which can work to support definitions of success that include personal growth and development ( Biasi et al. , 2017 ; Vescovelli et al. , 2017 ). By truly listening to, and acting on, the unique voices of students, university leaders can enact changes in institutional policy and structure that may be more likely than current initiatives to facilitate success for all students.
Rethink, Reframe, Start a Conversation—Recommendations for Practice
Our goals to increase representation of minoritized students in STEM, to improve student well-being, and to facilitate student success will only be met once all definitions of success are represented and supported in higher education. However, faculty play a role in everyday interactions with students that can be powerful influencers of success as well. Our perspectives, our biases, our words matter, and we need to carefully reflect on our individual influence. Do we think that graduate students are more successful if they become R1 faculty after graduation than if they become science journalists? Do we have a problem if undergraduates entering our classrooms see success as a “C” as opposed to an “A”? These biases, which come from our own backgrounds as students and researchers, are seemingly innocuous; however, they impact how we interact with students in one-on-one meetings, during office hours, and in the classroom, and therefore impact how supported students feel. Noncontent talk, or the things instructors say that are unrelated to the course material ( Seidel et al. , 2015 ), could be an important way to expose students to multiple, diverse definitions of success or it may reinforce a hegemonic view of student success in university settings. Attention to our own thinking and words and how they impact our discussions with students may be key to breaking down the cycle of hegemonic reproduction in academia. Finally, it is important to acknowledge the difficult work involved in beginning to unpack and examine the facets of internalized racism that underlie the “simple” idea of student success. This work is necessary to move the field forward and build a more equitable environment with diverse conceptions of success.
ENACTING CHANGE
We recognize that raising awareness, encouraging self-reflection, and including student voice may not in and of itself change how success is framed. These steps may be critical to changing the perspectives of individual researchers and adding new definitions and metrics of success but may not be enough to change institutional structures that are deeply embedded in racist social hierarchies. Indeed, a central component of our critical race framework is the notion that racism is not an individual issue, but a deeply ingrained systemic condition ( Ladson-Billings and Tate, 2006; Solorzano and Yosso, 2001 ; Vaught, 2011 ). Thus, in the following section, we highlight some ideas for institutions and organizations to enact lasting structural change as it relates to student success.
First, to properly enact change, we must acknowledge the concept of “empathetic fallacy,” or the incorrect idea that changes will be instituted simply because they are the right thing to do ( Delgado and Stefancic, 2000 ). While making the field’s definitions and metrics of success more diverse and equitable is the right thing to do, this fact is not enough to shift hegemonic structures. Instead, we have provided a table of the benefits of and risks for not expanding our definitions and metrics of success, which we encourage the community to build on ( Table 3 ). These benefits range from positive impacts on student well-being and retention, to more equitable institutional policies and practices and scientific problem solving. Indeed, Freeman and Huang (2015) found that greater author homophily was associated with publication in lower-impact journals and fewer citations, while the opposite was true with more ethnically diverse groups of authors. More diverse teams and institutions solve problems faster ( Reynolds and Lewis, 2017 ), generate more creative solutions ( Dezsö and Ross, 2012 ), and are more resilient in the face of change ( Duchek et al. , 2020 ). Beyond the moral imperative for our field to become more equitable, we argue that combating the current hegemonic structures is essential for future innovation, advancement, and evolution of STEM fields. The risks of not applying these recommendations mirror the current issues with the field that we have discussed previously, including maintenance of social hierarchies, poor student mental health, and continued difficulties meeting diversity and equity goals at academic institutions and within society broadly ( Table 3 ). However, we also must acknowledge that there are costs to these recommendations that also must be taken into account: institutions and individuals must invest time, resources, and emotional energy into enacting these changes.
Illustrative examples of the benefits of applying recommendations and the risks of not applying recommendations related to broadening the field’s metrics and definitions of student success
Changing definitions of success will also require transformative change at the institutional level and beyond. This type of “deep change” or “second-order change” employs multiple change approaches to impact the attitudes, culture, and structure of organizations (Kezar, 2018) and dislodge the current hegemonic cultures, values, and beliefs that currently exist. Although individuals must change as part of this process (first-order change), second-order change involves explicit questioning of the operational frameworks and assumptions under which an organization functions, thus changing not just practices, but ideologies within an institution ( Argyris and Schön, 1997 ; Kezar, 2018).
In the field of STEM education, the efforts to replace lecturing with active learning is one example of second-order change. The benefits of active learning have been known for many years. Early efforts centered on getting individual faculty to change their practices, assuming that diffusion of these innovations would spread active-learning approaches, which is not what happened ( Miller et al. , 2000 ; Bennett and Bennett, 2003 ; Brownell and Tanner, 2012 ). To foster broader change, the Vision and Change initiative was started by individuals who realized that the influence of national organizations such the NSF, AAAS, and disciplinary societies could be used to amplify change ( AAAS, 2011 ). A series of forums and conversations with colleges and universities were organized, exemplifying the second-order change principle of providing opportunities for ongoing collaborative reflection within an institution. National conferences brought together leaders in the field and produced two influential conference reports with data, ideas, and exemplars that individuals and organizations used as levers for change. This highlights another principle of second-order change, using data to illustrate the need for change. NSF actively encouraged research on Vision and Change principles, incentivizing individuals and institutions to prioritize these ideas. While efforts related to Vision and Change have not employed all of the recommendations we suggest in this paper (e.g., including student voice in reforms), we believe it is an illustrative example of institutional change that has made great strides in normalizing active learning as beneficial and lecturing as a potentially harmful to students ( Freeman et al. , 2014 , but see England et al. , 2019 ).
Within our discussion of changing definitions and metrics of success, we believe that similar expansive, enduring initiatives are needed. As seen in the case of Vision and Change , institutional efforts can be amplified by disciplinary societies, foundations, and government agencies. These external influencers can also provide broad policy suggestions and funding initiatives to support systemic change. These efforts are time-consuming, but they represent some of the approaches that would be necessary to change the values, beliefs, and institutional culture surrounding the concept of success.
Student success is a central focus of education, particularly uncovering ways in which minoritized students can achieve representation in STEM at levels equivalent to their representation in U.S. populations. We posit that the lack of diversity in definitions and measures of success is an example of hegemonic influence within academia. Hegemonic power may be unknowingly upheld by researchers, faculty, and other institutional-level stakeholders who consider these dominant ideas of success to be “common sense” or standard. These dominant ideas of success, often stemming from racist structures and theories, reinforce social hierarchies within academia, making it more difficult for minoritized students to achieve social mobility ( Giroux, 1984 ). Movements of counter-hegemony are the only way to subsume dominant cultural norms ( Pratt, 2004 ). Thus, we call on researchers to carefully consider their definitions of success and associated metrics, to fully acknowledge how their conceptualization of success impacts their research, and to make space for and solicit student voice in discussions of student success. We further call on institutions and faculty to consider a more holistic view of student success, and when presented with student voice, to not merely listen, but to give their perspectives “due weight.” And we call on disciplinary societies, government agencies, and other organizations in positions of power and influence to consider how they may play a role in fostering new definitions of success. Finally, we hope that this Essay sparks collective reflection that leads each of us to consider how we participate in hegemonic reproduction, what biases we operate under, and how we can work in our classrooms, with our advisees, and as advocates, to facilitate student success that is as inclusive as we strive to be.
Supplementary Material
Acknowledgments.
We are grateful for the feedback on this article by Miranda Chen Musgrove, Elizabeth Derryberry, Courtney Faber, Nina Fefferman, Joshua Rosenberg, Caroline Wienhold, and Hope Ferguson. We thank the reviewers and editor for their incredibly thoughtful and useful comments on an earlier version of this Essay .
- AAAS. (2011). Vision and Change: A Call to Action . Washington, DC: AAAS. Retrieved October 25, 2020, from https://visionandchange.org/about-vc-a-call-to-action-2011/ [ Google Scholar ]
- Alderson, P. (2000). School students’ views on school councils and daily life at school . Children and Society , 14 , 121–134. 10.1002/(SICI)1099-0860(200004)14:2<121::AID-CHI588>3.3.CO;2-4 [ CrossRef ] [ Google Scholar ]
- Alexander, N., Woodruff, R. J. (1940). Determinants of college success . Journal of Higher Education , 11 ( 9 ), 479–485. 10.2307/1974924 [ CrossRef ] [ Google Scholar ]
- Anfara, V. A. Jr., Mertz, N. T. (Eds.). (2014). Theoretical frameworks in qualitative research . Sage. [ Google Scholar ]
- Argyris, C., Schön, D. A. (1997). Organizational learning: A theory of action perspective . (pp. 345–348), Boston, MA: Addison-Wesley. [ Google Scholar ]
- Astin, A. W. (1985). Involvement: The cornerstone of excellence . Change , 17 ( 4 ), 35–39. 10.1080/00091383.1985.9940532 [ CrossRef ] [ Google Scholar ]
- Attinasi, L. C. Jr., (1989). Getting in: Mexican Americans’ perceptions of university attendance and the implications for freshman year persistence . Journal of Higher Education , 60 ( 3 ), 247-277. 10.1080/00221546.1989.11775035 [ CrossRef ] [ Google Scholar ]
- Barnes, J. C., Motz, R. T. (2018). Reducing racial inequalities in adulthood arrest by reducing inequalities in school discipline: Evidence from the school-to-prison pipeline . Developmental Psychology , 54 ( 12 ), 2328. 10.1037/dev0000613 [ PubMed ] [ CrossRef ] [ Google Scholar ]
- Bell, B. (2016). The impact of the Multicultural Student Services Program on multicultural students first-to-second year persistence at a midwestern private college , Madison, WI: Edgewood College. [ Google Scholar ]
- Bennett, J., Bennett, L. (2003). A review of factors that influence the diffusion of innovation when structuring a faculty training program . Internet and Higher Education , 6 ( 1 ), 53–63. 10.1016/S1096-7516(02)00161-6 [ CrossRef ] [ Google Scholar ]
- Biasi, V., Patrizi, N., Mosca, M., De Vincenzo, C. (2017). The effectiveness of university counselling for improving academic outcomes and well-being . British Journal of Guidance & Counselling , 45 ( 3 ), 248–257. 10.1080/03069885.2016.1263826 [ CrossRef ] [ Google Scholar ]
- Boggs, C. (1976). Gramsci’s Marxism . England: Pluto Press. [ Google Scholar ]
- Boland, J. M., Adelman, S. I., Stevens, J. (1978). Ethnicity as a background factor in college education . Urban Education , 13 ( 1 ), 65–70. 10.1177/0042085978131006 [ CrossRef ] [ Google Scholar ]
- Borg, C., Buttigieg J. A., Mayo P., (Eds.). (2002). Gramsci and education . Lanham, MD: Rowman & Littlefield. [ Google Scholar ]
- Brogden, H. E., Taylor, E. K. (1950). The theory and classification of criterion bias . Educational and Psychological Measurement , 10 ( 2 ), 159–183. 10.1177/001316445001000201 [ CrossRef ] [ Google Scholar ]
- Brownell, S. E., Tanner, K. D. (2012). Barriers to faculty pedagogical change: Lack of training, time, incentives, and… tensions with professional identity? CBE—Life Sciences Education , 11 ( 4 ), 339–346. 10.1187/cbe.12-09-0163 [ PMC free article ] [ PubMed ] [ CrossRef ] [ Google Scholar ]
- Burt, B. A., Williams, K. L., Palmer, G. J. (2019). It takes a village: The role of emic and etic adaptive strengths in the persistence of Black men in engineering graduate programs . American Educational Research Journal , 56 ( 1 ), 39–74. 10.3102/0002831218789595 [ CrossRef ] [ Google Scholar ]
- Capps, M. P., Decosta, F. A. (1957). Contributions of the Graduate Record Examinations and the National Teacher Examinations to the prediction of graduate school success . Journal of Educational Research , 50 ( 5 ), 383–389. 10.1080/00220671.1957.10882393 [ CrossRef ] [ Google Scholar ]
- Carmichael, R. D. (1913). The meaning of graduate study . Science , 37 ( 959 ), 738–743. 10.1126/science.37.959.738 [ PubMed ] [ CrossRef ] [ Google Scholar ]
- Carter, T. P., Segura, R. D. (1979). Mexican Americans in school: A decade of change . Princeton, NJ: College Board Publications. [ Google Scholar ]
- Chen, X. (2013). STEM Attrition: College Students’ Paths into and out of STEM Fields. Statistical Analysis Report. NCES 2014-001 . National Center for Education Statistics. [ Google Scholar ]
- Collins, K. H. (2018). Confronting color-blind STEM talent development: Toward a contextual model for Black student STEM identity . Journal of Advanced Academics , 29 ( 2 ), 143–168. 10.1177/1932202x18757958 [ CrossRef ] [ Google Scholar ]
- Creswell, J. W., Creswell, J. D. (2017). Research design: Qualitative, quantitative, and mixed methods approaches . Thousand Oaks, CA: Sage Publishing. [ Google Scholar ]
- Creswell, J. W., Miller, D. L. (2000). Determining validity in qualitative inquiry . Theory into Practice , 39 ( 3 ), 124–130. 10.1207/s15430421tip3903_2 [ CrossRef ] [ Google Scholar ]
- Dawson, D. (1982). Educational hegemony and the phenomenology of community participation . Journal of Educational Thought (JET)/Revue de la Pensée Educative , 150–160. 10.11575/jet.v16i3 [ CrossRef ] [ Google Scholar ]
- Dean, A. M. (1998). Defining and achieving student success . University Faculty and Student Perspectives, [Master’s thesis, Virginia Polytechnic Institute and State University] VTech works. [ Google Scholar ]
- Delgado, R., Stefancic, J. (2000). Critical race theory: The cutting edge . Philadelphia: Temple University Press. [ Google Scholar ]
- Dezsö, C. L., Ross, D. G. (2012). Does female representation in top management improve firm performance? A panel data investigation . Strategic management journal , 33 ( 9 ), 1072–1089. 10.1002/smj.1955 [ CrossRef ] [ Google Scholar ]
- Duchek, S., Raetze, S., Scheuch, I. (2020). The role of diversity in organizational resilience: a theoretical framework . Business Research , 13 ( 2 ), 387–423. 10.1007/s40685-019-0084-8 [ CrossRef ] [ Google Scholar ]
- Efklides, A., Schwartz, B. L., Brown, V. (2018). Motivation and affect in self-regulated learning: Does metacognition play a role ? In Schunk, D. H., Greene, J. A. (Eds.), Educational psychology handbook series. Handbook of self-regulation of learning and performance (pp. 64–82). England:Routledge: Taylor & Francis Group. [ Google Scholar ]
- England, B. J., Brigati, J. R., Schussler, E. E., Chen, M. M. (2019). Student anxiety and perception of difficulty impact performance and persistence in introductory biology courses . CBE—Life Sciences Education , 18 ( 2 ), ar21. 10.1187/cbe.17-12-0284 [ PMC free article ] [ PubMed ] [ CrossRef ] [ Google Scholar ]
- Foucault, M. (1997). Ethics: Subjectivity and truth (Rabinow, P, Ed.). London: Allen Lane. [ Google Scholar ]
- Freeman, R. B., Huang, W. (2015). Collaborating with people like me: Ethnic coauthorship within the United States . Journal of Labor Economics , 33 ( S1 ), S289–S318. 10.1086/678973 [ CrossRef ] [ Google Scholar ]
- Freeman, S., Eddy, S. L., McDonough, M., Smith, M. K., Okoroafor, N., Jordt, H., Wenderoth, M. P. (2014). Active learning increases student performance in science, engineering, and mathematics . Proceedings of the National Academy of Sciences USA , 111 ( 23 ), 8410–8415. 10.1073/pnas.1319030111 [ PMC free article ] [ PubMed ] [ CrossRef ] [ Google Scholar ]
- Gallup. (2016). Great jobs. Great lives. The Value of career services, inclusive experiences and mentorship for college graduates . Retrieved September 28, 2020, from https://acue.org/wp-content/uploads/2018/11/Gallup-Purdue-Index-Study-Year-3-2016.pdf [ Google Scholar ]
- Giroux, H. A. (1984). Ideology, culture, and the process of schooling . Philadelphia: Temple University Press. [ Google Scholar ]
- Good, C., Rattan, A., Dweck, C. S. (2012). Why do women opt out? Sense of belonging and women’s representation in mathematics . Journal of Personality and Social Psychology , 102 ( 4 ), 700. 10.1037/a0026659 [ PubMed ] [ CrossRef ] [ Google Scholar ]
- Goyette, K., Xie, Y. (1999). Educational expectations of Asian American youths: Determinants and ethnic differences . Sociology of Education , 22–36. 10.2307/2673184 [ CrossRef ] [ Google Scholar ]
- Gramsci, A. (2000). The Gramsci reader: Selected writings, 1916–1935. New York: NYU Press. [ Google Scholar ]
- Green, R., Macauley, P. (2007). Doctoral students’ engagement with information: An American-Australian perspective . Portal: Libraries and the Academy , 7 , 317–332 10.1353/pla.2007.0031 [ CrossRef ] [ Google Scholar ]
- Green, T. L., Sánchez, J., Germain, E. (2017). Communities and school ratings: Examining geography of opportunity in an urban school district located in a resource-rich city . Urban Review , 49 ( 5 ), 777–804. 10.1007/s11256-017-0421-1 [ CrossRef ] [ Google Scholar ]
- Gregg-Jolly, L., Swartz, J., Iverson, E., Stern, J., Brown, N., Lopatto, D. (2016). Situating second-year success: understanding second-year STEM experiences at a liberal arts college . CBE—Life Sciences Education , 15 ( 3 ), ar43. 10.1187/cbe.16-01-0044 [ PMC free article ] [ PubMed ] [ CrossRef ] [ Google Scholar ]
- Grimm, J. (2015). Hegemonic framing of Malcolm X and Martin Luther King, Jr., in northeastern newspapers . Howard Journal of Communications , 26 ( 3 ), 313–332. 10.1080/10646175.2015.1049761 [ CrossRef ] [ Google Scholar ]
- Gullo, G. L., Capatosto, K., Staats, C. (2018). Implicit bias in schools: A practitioner’s guide . England: Routledge. [ Google Scholar ]
- Hurtado, S., Alvarado A. R., Guillermo-Wann C. (2015). Creating inclusive environments: The mediating effect of faculty and staff validation on the relationship of discrimination/bias to students’ sense of belonging . JCSCORE 1 ( 1 ), 59–81. 10.15763/issn.2642-2387.2015.1.1.59-81 [ CrossRef ] [ Google Scholar ]
- Hurtado, S., Carter, D. F. (1997). Effects of college transition and perceptions of the campus racial climate on Latino college students’ sense of belonging . Sociology of Education , 70 ( 4 ), 324–345. 10.2307/2673270 [ CrossRef ] [ Google Scholar ]
- Kezar, A. (2018). How colleges change: Understanding, leading, and enacting change . Routledge, England: Taylor and Francis Group. [ Google Scholar ]
- Killpack, T. L., Melón, L. C. (2016). Toward inclusive STEM classrooms: What personal role do faculty play? CBE—Life Sciences Education , 15 ( 3 ), es3. 10.1187/cbe.16-01-0020 [ PMC free article ] [ PubMed ] [ CrossRef ] [ Google Scholar ]
- Kunhart, W. E., Olsen, L. R. (1959). An analysis of test scores and grades for predicting success of college students in English composition . Journal of Educational Research , 53 ( 2 ), 79–79. 10.1080/00220671.1959.10882616 [ CrossRef ] [ Google Scholar ]
- Ladson-Billings, G., Tate, W. F. (2006). Toward a critical race theory of education . Critical race theory in education: All God’s children got a song , 11 , 30. 10.4324/9781315709796-2 [ CrossRef ] [ Google Scholar ]
- Leman, P. J. (1999). The role of subject area, gender, ethnicity and school background in the degree results of Cambridge University undergraduates . Curriculum Journal , 10 ( 2 ), 231–252. 10.1080/0958517990100205 [ CrossRef ] [ Google Scholar ]
- Lent, R. W., Brown, S. D., Hackett, G. (2002). Social cognitive career theory . Career Choice and Development , 4 , 255–311. [ Google Scholar ]
- Lovitts, B. E. (2002). Leaving the ivory tower: The causes and consequences of departure from doctoral study . Lanham, MD: Rowman & Littlefield. [ Google Scholar ]
- Lundy, L. (2007). “Voice” is not enough: Conceptualising Article 12 of the United Nations Convention on the Rights of the Child . British Educational Research Journal , 33 ( 6 ), 927–942. 10.1080/01411920701657033 [ CrossRef ] [ Google Scholar ]
- Lygo-Baker, S., Kinchin, I. M., Winston, N. E. (Eds.). (2019). Engaging student voices in higher education: Diverse perspectives and expectations in partnership . New York City, NY: Springer. 10.1007/978-3-030-20824-0 [ CrossRef ] [ Google Scholar ]
- Martinez, L. R., Boucaud, D. W., Casadevall, A., August, A. (2018). Factors contributing to the success of NIH-designated underrepresented minorities in academic and nonacademic research positions . CBE—Life Sciences Education , 17 ( 2 ), ar32. 10.1187/cbe.16-09-0287 [ PMC free article ] [ PubMed ] [ CrossRef ] [ Google Scholar ]
- May, S., Sleeter, C. E. (2010). Critical multiculturalism: Theory and praxis . England: Routledge. [ Google Scholar ]
- McAlpine, L., Amundsen, C. (2009). Identity and agency: Pleasures and collegiality among the challenges of the doctoral journey . Studies in Continuing Education , 31 , 109–125. 10.1080/01580370902927378 [ CrossRef ] [ Google Scholar ]
- McGee, E. O. (2020). Interrogating structural racism in STEM higher education . Educational Researcher , 49 ( 9 ), 633–644. 10.3102/0013189x20972718 [ CrossRef ] [ Google Scholar ]
- Meyers, L. C., Brown, A. M., Moneta-Koehler, L., Chalkley, R. (2018). Survey of checkpoints along the pathway to diverse biomedical research faculty . PLoS ONE , 13 ( 1 ), e0190606. 10.1371/journal.pone.0190606 [ PMC free article ] [ PubMed ] [ CrossRef ] [ Google Scholar ]
- Miller, J. W., Martineau, L. P., Clark, R. C. (2000). Technology infusion and higher education: Changing teaching and learning . Innovative Higher Education , 24 ( 3 ), 227–241. 10.1023/b:ihie.0000047412.64840.1c [ CrossRef ] [ Google Scholar ]
- Minkler, M., Wallerstein, N. (2011). Community-based participatory research for health: From process to outcomes . Hoboken, NJ: Wiley. [ Google Scholar ]
- Muñoz, S. M., Maldonado, M. M. (2012). Counterstories of college persistence by undocumented Mexicana students: Navigating race, class, gender, and legal status . International Journal of Qualitative Studies in Education , 25 ( 3 ), 293–315. 10.1080/09518398.2010.529850 [ CrossRef ] [ Google Scholar ]
- National Science Foundation. (2018). Science and engineering indicators . Washington, DC. [ Google Scholar ]
- Nouwen, W., Clycq N. (2018). The role of teacher-pupil relations in stereotype threat effects in Flemish secondary education . Urban Education 54 ( 10 ), 1551–1580. 10.1177/0042085916646627 [ CrossRef ] [ Google Scholar ]
- Oh, C. J., Kim, N. Y. (2016). “success is relative”: Comparative social class and ethnic effects in an academic paradox . Sociological Perspectives , 59 ( 2 ), 270–295. 10.1177/0731121415587115 [ CrossRef ] [ Google Scholar ]
- O’Shea, S., Delahunty, J. (2018). Getting through the day and still having a smile on my face! How do students define success in the university learning environment? Higher Education Research & Development , 37 ( 5 ), 1062–1075. 10.1080/07294360.2018.1463973 [ CrossRef ] [ Google Scholar ]
- Park, J. J., Kim, Y. K., Salazar, C., Eagan, M. K. (2020). Racial discrimination and student-faculty interaction in STEM: Probing the mechanisms influencing inequality . Journal of Diversity in Higher Education . 10.1037/dhe0000224 [ CrossRef ] [ Google Scholar ]
- Panos, R. J., Astin, A. W. (1968). Attrition among college students . American Educational Research Journal , 5 ( 1 ), 57–72. 10.3102/00028312005001057 [ CrossRef ] [ Google Scholar ]
- Pratt, N. (2004). Bringing politics back in: Examining the link between globalization and democratization . Review of International Political Economy , 11 ( 2 ), 311-336. 10.1080/0969229042000249831 [ CrossRef ] [ Google Scholar ]
- Project Implicit. (2011). Home page . Retrieved October 1, 2020, from https://implicit.harvard.edu/implicit/index.jsp
- Racial Equity Tools. (2020). Communicating implicit bias. Retrieved October 1, 2020, from www.racialequitytools.org/act/communicating/implicit-bias
- Reynolds, A., Lewis, D. (2017). Teams solve problems faster when they’re more cognitively diverse . Harvard Business Review , Retrieved October 25, 2020, from https://hbr.org/2017/03/teams-solveproblems-faster-when-theyre-morecognitively-diverse [ Google Scholar ]
- Richardson, K., Clark, Z., Gaines, M., Kingi, H., Miller, S., Pearson, W., Jr., Richardson, L. (2018). Āwhina revolution: A Bayesian analysis of undergraduate and postgraduate completion rates from a program for Māori and Pacific success in STEM disciplines . CBE—Life Sciences Education , 17 ( 1 ), ar15. 10.1187/cbe.17-07-0117 [ PMC free article ] [ PubMed ] [ CrossRef ] [ Google Scholar ]
- Robertson, M., Hall, E. (1964). Predicting success in graduate study . Journal of General Psychology , 71 , 359. [ PubMed ] [ Google Scholar ]
- Rose, S. (1976). Scientific racism and ideology: The IQ racket from Galton to Jensen . In Rose, H., Rose, S. (Eds.), The political economy of science: Ideology of/in the natural sciences (pp. 112–141). London: Macmillan Education UK. 10.1007/978-1-349-15725-9_7 [ CrossRef ] [ Google Scholar ]
- Rosenbaum, J. E. (1976). Making inequality; the hidden curriculum of high school tracking , New York City, NY: Wiley. [ Google Scholar ]
- Rowland, A. A., Knekta, E., Eddy, S., Corwin, L. A. (2019). Defining and measuring students’ interest in biology: An analysis of the biology education literature . CBE—Life Sciences Education , 18 ( 3 ), ar34. 10.1187/cbe.19-02-0037 [ PMC free article ] [ PubMed ] [ CrossRef ] [ Google Scholar ]
- Schnoes, A. M., Caliendo, A., Morand, J., Dillinger, T., Naffziger-Hirsch, M., Moses, B., … & O’Brien, T. C. (2018). Internship experiences contribute to confident career decision making for doctoral students in the life sciences . CBE—Life Sciences Education , 17 ( 1 ), ar16. 10.1187/cbe.17-08-0164 [ PMC free article ] [ PubMed ] [ CrossRef ] [ Google Scholar ]
- Seidel, S. B., Reggi, A. L., Schinske, J. N., Burrus, L. W., Tanner, K. D. (2015). Beyond the biology: A systematic investigation of noncontent instructor talk in an introductory biology course . CBE—Life Sciences Education , 14 ( 4 ), ar43. 10.1187/cbe.15-03-0049 [ PMC free article ] [ PubMed ] [ CrossRef ] [ Google Scholar ]
- Solorzano, D. G. (1997). Images and words that wound: Critical race theory, racial stereotyping, and teacher education . Teacher Education Quarterly , 24 ( 3 ), 5-19. [ Google Scholar ]
- Solorzano, D. G., Yosso, T. J. (2001). From racial stereotyping and deficit discourse toward a critical race theory in teacher education . Multicultural Education , 9 ( 1 ), 2. [ Google Scholar ]
- Southworth, S., Mickelson, R. A. (2007). The interactive effects of race, gender and school composition on college track placement . Social Forces , 86 ( 2 ), 497–523. 10.1093/sf/86.2.497 [ CrossRef ] [ Google Scholar ]
- Sowell, R., Allum, J., Okahana, H. (2015). Doctoral initiative on minority attrition and completion . Washington, DC: Council of Graduate Schools. [ Google Scholar ]
- Spady, W. G. (1970). Dropouts from higher education: An interdisciplinary review and synthesis . Interchange , 1 ( 1 ), 64–85. 10.1007/BF02214313 [ CrossRef ] [ Google Scholar ]
- Sutantoputri, N. W., Sutantoputra, A., Tirtowalujo, I., Murniati, J., Purwanti, M. (2020). Significance of sociocultural factors in attribution of educational outcomes and motivation goals . In Suryani, A., Tirtowalujo, I., Masalam, I, H. (Eds.), Preparing Indonesian youth (pp. 185–202). Netherlands: Brill Sense. [ Google Scholar ]
- Sweitzer, V. B. (2009). Towards a theory of doctoral student professional identity development: A developmental networks approach . Journal of Higher Education , 80 , 1–33. 10.1080/00221546.2009.11772128 [ CrossRef ] [ Google Scholar ]
- Tachine, A. R., Cabrera, N. L., Yellow Bird, E. (2017). Home away from home: Native American students’ sense of belonging during their first year in college . Journal of Higher Education , 88 ( 5 ), 785–807. 10.1080/00221546.2016.1257322 [ CrossRef ] [ Google Scholar ]
- Tate IV, W. F. (2008). “Geography of opportunity”: Poverty, place, and educational outcomes . Educational Researcher , 37 ( 7 ), 397–411. 10.3102/0013189x08326409 [ CrossRef ] [ Google Scholar ]
- Tibbetts, Y., Harackiewicz, J. M., Priniski, S. J., Canning, E. A. (2016). Broadening participation in the life sciences with social-psychological interventions . CBE—Life Sciences Education , 15 ( 3 ), es4. 10.1187/cbe.16-01-0001 [ PMC free article ] [ PubMed ] [ CrossRef ] [ Google Scholar ]
- Tierney, W. G. (1992). Official encouragement, institutional discouragement: Minorities in academe—The Native American experience (Interpretive Perspectives on Education and Policy). New York City, NY: Ablex Publishing. [ Google Scholar ]
- Thompson, J. J., Jensen-Ryan, D. (2018). Becoming a “science person”: Faculty recognition and the development of cultural capital in the context of undergraduate biology research . CBE—Life Sciences Education , 17 ( 4 ), ar62. 10.1187/cbe.17-11-0229 [ PMC free article ] [ PubMed ] [ CrossRef ] [ Google Scholar ]
- Tinto, V. (1975). Dropout from higher education: A theoretical synthesis of recent research . Review of Educational Research , 45 ( 1 ), 89–125. 10.3102/00346543045001089 [ CrossRef ] [ Google Scholar ]
- Tinto, V. (1993). Leaving college: Rethinking the causes and cures of student attrition (2nd ed.). Chicago: University of Chicago Press. 10.7208/chicago/9780226922461.001.0001 [ CrossRef ] [ Google Scholar ]
- United Nations. (2002) Concluding observations of the Committee on the Rights of the Child: United Kingdom of Great Britain and Northern Ireland (UN/CRC/C/15/Add.188) . Geneva. [ Google Scholar ]
- United Nations. (2003) Committee on the Rights of the Child general comment no. 5 (2003): Implementation (UN/ CRC/GC/2003/1) . Geneva. [ Google Scholar ]
- United Nations. (2009). Committee on the Rights of the Child general comment no. 12 (2009): The right of the child to be heard (UN/CRC/C/12) . Geneva. [ Google Scholar ]
- Vaught, S. E. (2011). Racism, public schooling, and the entrenchment of white supremacy: A critical race ethnography . Albany, NY: SUNY Press. [ Google Scholar ]
- Vescovelli, F., Melani, P., Ruini, C., Ricci Bitti, P. E., Monti, F. (2017). University counseling service for improving students’ mental health . Psychological Services , 14 ( 4 ), 470. 10.1037/ser0000166 [ PubMed ] [ CrossRef ] [ Google Scholar ]
- Vespa, J., Armstrong, D. M., Medina, L. (2018). Demographic turning points for the United States: Population projections for 2020 to 2060 . Washington, DC: U.S. Department of Commerce, Economics and Statistics Administration, U.S. Census Bureau. [ Google Scholar ]
- Walck-Shannon, E. M., Cahill, M. J., McDaniel, M. A., Frey, R. F. (2019). Participation in voluntary re-quizzing is predictive of increased performance on cumulative assessments in introductory biology . CBE—Life Sciences Education , 18 ( 2 ), ar15. 10.1187/cbe.18-08-0163 [ PMC free article ] [ PubMed ] [ CrossRef ] [ Google Scholar ]
- Wilson, M. A., DePass, A. L., Bean, A. J. (2018). Institutional interventions that remove barriers to recruit and retain diverse biomedical PhD students . CBE—Life Sciences Education , 17 ( 2 ), ar27. 10.1187/cbe.17-09-0210 [ PMC free article ] [ PubMed ] [ CrossRef ] [ Google Scholar ]
- Yancy, G. (2016). Black bodies, white gazes: The continuing significance of race in America . Lanham, MD: Rowman & Littlefield. [ Google Scholar ]
- Yao, C. W. (2015). Sense of belonging in international students: Making the case against integration to US institutions of higher education . Faculty Publications in Educational Administration , 7 ( 45 ). https://digitalcommons.unl.edu/cehsedadfacpub/45 . [ Google Scholar ]
- Yosso, T. J. (2005). Whose culture has capital? A critical race theory discussion of community cultural wealth . Race Ethnicity and Education , 8 ( 1 ), 69–91. 10.1080/1361332052000341006 [ CrossRef ] [ Google Scholar ]
Home — Essay Samples — Life — Life Experiences — Success

Success Essays
When it comes to writing an essay on success, choosing the right topic is crucial. The topic sets the tone for the entire essay and determines the direction of your writing. A well-chosen topic not only makes your essay more engaging but also allows you to explore different aspects of success and offer valuable insights to your readers.
With the right topic, you can delve into various areas such as personal success, professional success, societal perceptions of success, and the pursuit of happiness. It allows you to critically analyze the concept of success and offers a platform to present your ideas and opinions.
When selecting a topic for your success essay, it's essential to consider your interests, knowledge, and the intended audience. Choose a topic that resonates with you and allows you to explore different dimensions of success. Additionally, consider the relevance of the topic and its potential to engage readers.
It's also important to select a topic that is not only thought-provoking but also offers scope for research and analysis. Look for topics that are current, relevant, and present a unique perspective on success.
Recommended Success Essay Topics
Are you looking for inspiration for your next essay on success? Here's a list of 28 success essay topics to help you get started. Whether you're interested in personal success, business success, or the psychology of success, you're sure to find a topic that interests you.
Personal Success
- Overcoming adversity: A personal journey to success
- The role of perseverance in achieving personal success
- Setting and achieving personal goals: A recipe for success
- Self-discovery and its impact on personal success
- The importance of self-confidence in attaining success
- Lessons learned from failure: The path to personal growth and success
Professional Success
- Work-life balance: The key to professional success
- Leadership qualities and their role in achieving professional success
- Effective communication: A catalyst for professional success
- Entrepreneurship and the journey to business success
- Adapting to change: The secret to sustained professional success
- Building a successful career in the digital age
Societal Perceptions of Success
- Media portrayal of success: Reality vs. illusion
- Cultural influences on the definition of success
- The impact of social media on societal perceptions of success
- Redefining success: Beyond material wealth and fame
- Success and happiness: Exploring the correlation
- Education and its role in shaping societal views on success
The Pursuit of Happiness
- Defining happiness: The ultimate measure of success
- The pursuit of happiness in a materialistic world
- Embracing gratitude: A pathway to happiness and success
- Self-compassion and its impact on overall well-being and success
- Mindfulness and its role in achieving happiness and success
- Community and connection: The foundation of a fulfilling life
These essay topics offer a wide range of options for you to explore and provide ample opportunity to delve into the various aspects of success. Choose a topic that speaks to you and allows you to present your unique insights and perspectives on success.
Strategies for Sustaining Academic Success in College
Being successful in college, made-to-order essay as fast as you need it.
Each essay is customized to cater to your unique preferences
+ experts online
Strategies for Academic Success: Preparation, Organization, and Discipline
What is success and how to define it, how our choices determine our succes: the outliers and into the wild, what the road to success actually looks like, let us write you an essay from scratch.
- 450+ experts on 30 subjects ready to help
- Custom essay delivered in as few as 3 hours
Success and What It Means to Me
Trust - 'you will be successful', conceiving success from the hardships of life, self-improvement and success – hand in hand, get a personalized essay in under 3 hours.
Expert-written essays crafted with your exact needs in mind
Failure is The Key to Success
The definition of success as a lifestyle, the role of risks and plans in achieving success, perseverance and success in maya angelou's graduation, l'oreal - the history of success, malcolm gladwell's "outliers": exploring the definition of success, my personal philosophy of success: the strategies, the role of self-confidence in a person's success, the importance of effective leadership for the success of the organization, a personal opinion on the keys to success: fighting for what you believe in, earning an honest living, and helping others, why happiness is more important than success, william hoy: a deaf baseball player who changed the game, the significance of accomplishment in a person’s life, the importance and role of hard work and efforts in your success, analysis of willy loman’s views on success in contrast to my views, how to find the definition of success, "it is hard to succeed in this dynamic world", success story of oprah winfrey, the pursuit of happyness: a man’s struggle to achieve success, receiving support from others can help you achieve success: my experience, relevant topics.
- Personal Growth and Development
- Overcoming Challenges
- Life Changing Experience
- Expectations
- Why I Want To Be A Nurse
- Volunteering
By clicking “Check Writers’ Offers”, you agree to our terms of service and privacy policy . We’ll occasionally send you promo and account related email
No need to pay just yet!
We use cookies to personalyze your web-site experience. By continuing we’ll assume you board with our cookie policy .
- Instructions Followed To The Letter
- Deadlines Met At Every Stage
- Unique And Plagiarism Free
Advertisement
Academic success from an individual perspective: A proposal for redefinition
- Research note
- Published: 18 November 2020
- Volume 67 , pages 403–413, ( 2021 )
Cite this article
- Oz Guterman ORCID: orcid.org/0000-0001-7994-5848 1
1116 Accesses
7 Citations
3 Altmetric
Explore all metrics
The examination of academic achievements is common in educational research literature, with most studies referring to grades (marks) as measures of success. In addition, outside the realm of research, a student’s grades are usually the main criteria for admission to education programmes, nomination for honours (passing above ordinary level), award of scholarships and so forth. However, scholars have put forward several arguments against the use of grades as the sole or most important measure of academic success. This research note focuses on a specific aspect of this problem, namely the failure to consider learners’ personal perspective regarding their own achievements. Many approaches to evaluating achievements call for their examination in light of previously defined goals. However, each learner defines her or his aspirations and goals differently, while achievements are usually measured on a uniform scale. This research note reviews this problem and considers alternative models (including both their advantages and their shortcomings) for defining academic success in terms of expectations and motivation. In addition, the author proposes a measure to enable the evaluation of academic achievements in terms of an individual student’s goals and aspirations.
La réussite des études d’un point de vue personnel : proposition d’une redéfinition – Il est courant que les ouvrages de recherche éducative se penchent sur les résultats scolaires et universitaires, ces travaux mesurant souvent la réussite des études à l’aune des notes. Cependant, en dehors du domaine de la recherche, les notes sont généralement aussi le principal critère pour être admis à des programmes d’études, se voir proposé pour des distinctions (à un niveau se situant au-delà de l’ordinaire), obtenir des bourses, etc. Toutefois, les chercheurs ont avancé différents arguments contre l’utilisation des notes comme moyen unique ou principal d’évaluation de la réussite des études. Cette note de recherche est axée sur un aspect particulier du problème, à savoir le fait que l’on omet de prendre en compte le point de vue personnel des apprenants sur leurs propres résultats. Nombre d’approches utilisées pour évaluer les résultats nécessitent d’être examinées à la lumière d’objectifs fixés au préalable. Néanmoins, chaque apprenant ne définit pas de la même façon ses aspirations et ses objectifs, alors que pour mesurer les résultats scolaires et universitaires, on applique d’ordinaire un barème uniforme. Dans cette note de recherche, l’auteur étudie ce problème et examine d’autres modèles (y compris leurs avantages et leurs inconvénients) pour définir la réussite des études en termes d’attentes et de motivation. Il propose en outre aussi une mesure pour permettre d’évaluer les résultats scolaires et universitaires à l’aune des objectifs et aspirations individuels des élèves.
This is a preview of subscription content, log in via an institution to check access.
Access this article
Price includes VAT (Russian Federation)
Instant access to the full article PDF.
Rent this article via DeepDyve
Institutional subscriptions
Formal education systems refer to traditional schooling followed by university, college or other adult training, generally recognised with a qualification or certificate.
Aptitude tests measure work-related skills and abilities; knowledge tests assess cognitive ability; and psychometric tests measure an individual’s overall suitability for a job role.
In an academic context, the term honours refers to a degree which has been completed above an ordinary pass mark.
In an educational context, constructivism refers to the experience of finding out how elements of knowledge are “constructed” and how they are related to items of knowledge the learner is already familiar with.
Pedagogy refers to teaching methods and practices.
According to its own website, the National Survey of Student Engagement (NSSE), which is conducted in Canada and the United States, is administered by “a self-supporting auxiliary unit within the Center for Postsecondary Research (CPR) in the Indiana University School of Education” ( https://nsse.indiana.edu/nsse/about-nsse/index.html [accessed 30 October 2020]).
The Freshman Survey (TFS) is administered by the Cooperative Institutional Research Program (CIRP) within the Higher Education Research Institute (HERI) at the University of California, Los Angeles (UCLA), For more information, visit https://heri.ucla.edu/cirp-freshman-survey/ [accessed 30 October 2020].
Astin, A. W. (1991). Assessment for excellence: The philosophy and practice of assessment and evaluation in higher education. American Council on Education Series on Higher Education. New York, NY: Macmillan.
Bandura, A. (1986). The explanatory and predictive scope of self-efficacy theory. Journal of Social and Clinical Psychology, 4 (3), 359–373. https://doi.org/10.1521/jscp.1986.4.3.359 .
Article Google Scholar
Bandura, A. (1993). Perceived self-efficacy in cognitive development and functioning. Educational Psychologist, 28 (2), 117–148. https://doi.org/10.1207/s15326985ep2802_3 .
Bandura, A., Barbaranelli, C., Caprara, G. V., & Pastorelli, C. (1996). Multifaceted impact of self-efficacy beliefs on academic functioning. Child Development, 67 (3), 1206–1222. https://doi.org/10.2307/1131888 .
Bouchey, H. A., & Harter, S. (2005). Reflected appraisals, academic self-perceptions, and math/science performance during early adolescence. Journal of Educational Psychology, 97 (4), 673–686. https://doi.org/10.1037/0022-0663.97.4.673 .
Boud, D., Lawson, R., & Thompson, D. G. (2015). The calibration of student judgement through self-assessment: Disruptive effects of assessment patterns. Higher Education Research & Development, 34 (1), 45–59. https://doi.org/10.1080/07294360.2014.934328 .
Brousselle, A., & Buregeya, J. M. (2018). Theory-based evaluations: Framing the existence of a new theory in evaluation and the rise of the 5th generation. Evaluation, 24 (2), 153–168. https://doi.org/10.1177/1356389018765487 .
Colarelli, S., Dean, R., & Kronstans, C. (1991). Relationship between university characteristics and early job outcomes of accountants. Canadian Journal of Higher Education, 21 (3), 23–46.
DeFreitas, S. C. (2011). Differences between African American and European American first-year college students in the relationship between self-efficacy, outcome expectations, and academic achievement. Social Psychology of Education, 15 (1), 109–123. https://doi.org/10.1007/s11218-011-9172-0 .
Eccles, J. S., Adler, T. F., Futterman, R., Goff, S. B., Kaczala, C. M., Meece, J. L., et al. (1985). Self-perceptions, task perceptions, socializing influences, and the decision to enroll in mathematics. In S. F. Chipman, L. R. Brush, & D. M. Wilson (Eds.), Women and mathematics: Balancing the equation (pp. 95–121). Hillsdale, NJ: Erlbaum.
Google Scholar
Fralick, M. (1993). College success: A study of positive and negative attrition. Community College Review, 20 (5), 29–36. https://doi.org/10.1177/009155219302000505 .
Gstraunthaler, T., & Piber, M. (2012). The performance of museums and other cultural institutions: Numbers or genuine judgments? International Studies of Management & Organization, 42 (2), 29–42. https://doi.org/10.2753/IMO0020-8825420202 .
Guba, E. G., & Lincoln, Y. S. (1989). Fourth generation evaluation . Newbury Park, CA: SAGE.
Guri-Rosenblit, S. (2009). Accessibility to higher education: Social aspects and selection processes . Jerusalem: Van Leer Institute Press.
Guterman, O., & Neuman, A. (2017). Similar goals, different results: Differences in group learning goals and their impact on academic achievements. Journal of Further and Higher Education, 43 (6), 729–741. https://doi.org/10.1080/0309877X.2017.1394990 .
Hepworth, D., Littlepage, B., & Hancock, K. (2018). Factors influencing university student academic success. Educational Research Quarterly, 42 (1), 45–61.
Kautz, T., Heckman, J. J., Diris, R., ter Weel, B., Borghans, L. (2014). Fostering and measuring skills: Improving cognitive and non-cognitive skills to promote lifetime success . IZA Discussion Papers, No. 8696. Bonn: IZA Institute of Labour Economics. Retrieved 25 August 2020 from http://ftp.iza.org/dp8696.pdf .
Khakee, A. (2003). The emerging gap between evaluation research and practice. Evaluation, 9 (3), 340–352. https://doi.org/10.1177/13563890030093007 .
Kuh, G. D., Kinzie, J. L., Buckley, J. A., Bridges, B. K., & Hayek, J. C. (2006). What matters to student success: A review of the literature . Commissioned report for the National Symposium on Postsecondary Student Success: Spearheading a dialog on student success. Washington, DC: National Postsecondary Education Cooperative (NPEC). Retrieved 25 August 2020 from https://nces.ed.gov/npec/pdf/Kuh_Team_Report.pdf .
Mislevy, R. J. (2018). Sociocognitive foundations of educational measurement . New York, NY: Routledge.
Book Google Scholar
Neuman, A., & Guterman, O. (2016). Academic achievement and homeschooling: It all depends on the goals. Studies in Educational Evaluation, 51, 1–6. https://doi.org/10.1016/j.stueduc.2016.08.005 .
Patton, M. Q. (2011). Developmental evaluation: Applying complexity concepts to enhance innovation and use . New York, NY: Guilford Press.
Sarnecky, M. T. (1990). Program evaluation. Part 1: Four generations of theory. Nurse Educator , 15 (5), 25–28. https://doi.org/10.1097/00006223-199009000-00006 .
Shadish, W. R., Cook, T. D., & Leviton, L. C. (1995). Foundations of program evaluation: Theories of practice . Newbury Park, CA: SAGE.
Stufflebeam, D. L., Madaus, J. F., & Kellaghan, T. (2000). Evaluation models: Viewpoints on educational and human services evaluation . Boston, MA: Kluwer Academic.
van Rooij, E. C., Jansen, E. P., & van de Grift, W. J. (2017). First-year university students’ academic success: The importance of academic adjustment. European Journal of Psychology of Education, 33 (4), 749–767. https://doi.org/10.1007/s10212-017-0347-8 .
York, T. T., Gibson, C., & Rankin, S. (2015). Defining and measuring academic success. Practical Assessment, Research & Evaluation , 20 , Art. 5. https://doi.org/10.7275/hz5x-tx03 .
Zajacova, A., Lynch, S. M., & Espenshade, T. J. (2005). Self-efficacy, stress, and academic success in college. Research in Higher Education, 46 (6), 677–706. https://doi.org/10.1007/s11162-004-4139-z .
Download references
Author information
Authors and affiliations.
Department of Human Resources, Western Galilee College, Akko, Israel
Oz Guterman
You can also search for this author in PubMed Google Scholar
Corresponding author
Correspondence to Oz Guterman .
Additional information
Publisher's note.
Springer Nature remains neutral with regard to jurisdictional claims in published maps and institutional affiliations.
Rights and permissions
Reprints and permissions
About this article
Guterman, O. Academic success from an individual perspective: A proposal for redefinition. Int Rev Educ 67 , 403–413 (2021). https://doi.org/10.1007/s11159-020-09874-7
Download citation
Published : 18 November 2020
Issue Date : June 2021
DOI : https://doi.org/10.1007/s11159-020-09874-7
Share this article
Anyone you share the following link with will be able to read this content:
Sorry, a shareable link is not currently available for this article.
Provided by the Springer Nature SharedIt content-sharing initiative
- academic achievements
- personal perspective of learners
- expected goals
- Find a journal
- Publish with us
- Track your research
How to Write a Definition Essay: New Guide with Samples
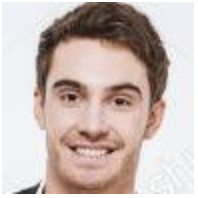
Have you ever found it difficult to explain certain words or ideas? That's because understanding them isn't always easy. To avoid confusion, it's important to really understand the words we use and be able to explain them well.
That's why teachers often assign definition essays in high school and college. But these essays aren't just about repeating dictionary definitions. They dive deep into complex terms, exploring their rich backgrounds and meanings.
In this article, our rewrite essay service will cover different types of these papers, give you practical tips for writing them, and even provide examples to simplify this journey for you!
What is a Definition Essay
A definition essay is a type of writing assignment where you explain the meaning of a specific word or concept. Instead of just giving a simple definition from the dictionary, you dive deeper into what the word really means and explore its different aspects.
For instance, if you're tasked with defining 'success,' you might discuss what success means to different people, how it can vary based on cultural or societal norms, and whether it's purely based on achievements or encompasses personal fulfillment as well.
The purpose of writing definition essays in school is multifaceted. Firstly, it helps you refine your understanding of language by encouraging you to analyze words more critically. It also fosters your ability to think deeply and express complex ideas clearly. Additionally, it cultivates your skills in research, as you may need to gather evidence and examples to support your interpretation of the word or concept. Now that we've cleared the definition essay meaning, let's explain its common types in detail.
Definition Essay Examples
Here's a definition essay example from our custom essay service to help you understand what a good paper looks like. Take a look at how it's structured and formatted if you want to use it as a reference for your own work. And if you're interested, you can always buy essay cheap and get high-quality paper from our platform anytime.
Stuck on Words?
Our writers excel at turning ordinary ideas into extraordinary narratives.
Commonly Used Definition Essay Types
When choosing an intriguing term with a rich historical background for your definition essay, it's essential to carefully consider your options and determine the most effective approach. Here are some common types, as suggested by our dissertation writing help :
.webp)
- Analysis : Break down the topic into its constituent parts and define each part separately.
- Classification : Determine the categories under which the topic can be classified.
- Comparison : Highlight the uniqueness of the topic by comparing and contrasting it with more common subjects.
- Details : Identify the key traits and distinctive qualities that best encapsulate the central idea of your essay.
- Negation : Clarify what your topic is, not to narrow down its definition.
- Origins and Causes : Explore the historical origins and background of the concept, examining where it first appeared and any relevant historical details.
- Results, Effects, and Uses : Discuss the consequences, effects, and practical applications of the subject matter.
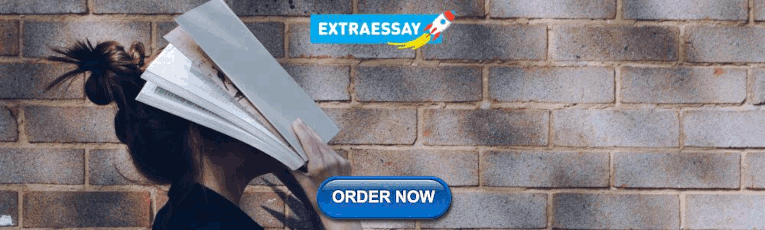
How to Write a Definition Essay
Just like with any writing, a definition essay structure involves an introduction, body, and conclusion. But what makes it interesting is what you explore in the body paragraphs.
For example, you could organize your definition essay outline by discussing the term from various angles. Start with a personal anecdote or story that illustrates the term in action. Then, provide a definition from a reputable source like a textbook or scholarly article. Next, consider interviewing people from different backgrounds to get their perspectives on the term. You could also analyze how the term has evolved over time, looking at historical examples or cultural shifts. Finally, offer your own interpretation of the term, drawing on your own experiences and insights.
For a more in-depth guide on writing a definition essay, let's explore the following sections provided by our experienced research paper writer .
Definition Essay Introduction
In the beginning stages of a definition essay, your reader gets their first taste of what your topic entails. It's crucial that this introduction is both informative and captivating, setting the stage for the rest of your essay. Here's what you need to include:
- Start with something attention-grabbing, like a thought-provoking question or an interesting fact.
- Provide a brief overview of the topic and why it's important to define it.
- Clearly state the term you're defining and your interpretation of it.
Definition Essay Body Paragraphs
In your essay, break down the phrase into its different parts, look at it from various angles, and then provide a relevant explanation. Depending on what your assignment calls for, you might need more than three paragraphs. Feel free to mix up the order or add sections depending on how complex the term is. Here are some ideas for what you can include:
- Start by talking about where the term came from and how it has changed over time. Understanding its origins can give insight into its meaning and significance.
- Look up the official definition of the term and compare it to your own understanding. This can help clarify any differences and give a broader perspective.
- Share your own thoughts and interpretation of the term, using examples or stories to illustrate your point. Your personal experiences can add depth and context to your analysis.
- Find a definition or explanation from an expert or scholar in the field and discuss how it aligns or differs with your own perspective. This can provide credibility and further insight into the term.
- Explore how the term is used in popular culture and what it reveals about societal values and beliefs. This can shed light on how the term is understood and interpreted in different contexts.
Definition Essay Conclusion
In the concluding paragraph, you should tie everything together neatly. Here's how you can structure your conclusion:
- Remind the reader of your main points and why the definition of the term is important.
- Highlight how having a clear understanding of the term can influence our thoughts and actions. This is where you show the broader significance of your analysis.
- Encourage your audience to apply the term accurately in their own discussions and advocate for precision in defining terms within their communities. This empowers readers to take action based on what they've learned.
Tips for Definition Essay Writing Process
Now that we're nearing the end, you might have already grasped how to write a definition essay. However, if you still feel like you're threading a needle while wearing mittens, fear not! Our essay writer has laid out some nifty guidelines to help you ace this challenge:
.webp)
- Choose a term with depth, something that's not ordinary but has a rich backstory and multiple meanings. Think of it like picking a word that's like a Russian nesting doll – there's plenty to explore.
- Use vivid language to paint a picture that engages the senses. For instance, when talking about 'love,' describe the warmth of a hug, the sweetness of Valentine's chocolates, or the sound of laughter with a partner. It helps your readers feel like they're right there with you.
- Explore both the positive and negative associations of your term. Words aren't simple; they come with different meanings. For example, 'power' can mean strength and influence but can also be linked to negative things like abuse and control.
- Use real-life examples to make your points clear in your definition essay. Whether you're talking about successful people from different fields or sharing stories that illustrate 'love,' concrete examples help readers understand.
- Be creative with your approach. Use metaphors, illustrations, or humor to keep things interesting. Remember, it's your essay – make it come alive!
Final Words
As we wrap up, we trust you've grasped the ins and outs of how to write a definition essay and feel inspired to tackle your own. Nobody wants to be left scratching their head over complex topics, right? So why not leverage our academic writing assistance to your advantage? Whether you need help brainstorming extended topics, crafting a sharp analytical piece, or any other form of writing, we've got you covered. Say goodbye to confusion and ignorance – Order essay and let us guide you toward clarity and knowledge.
Want to Get Your Task Done ASAP?
Leave us a notice and get help from professional writers!
Related Articles
.webp)
- Review article
- Open access
- Published: 10 February 2020
Predicting academic success in higher education: literature review and best practices
- Eyman Alyahyan 1 &
- Dilek Düştegör ORCID: orcid.org/0000-0003-2980-1314 2
International Journal of Educational Technology in Higher Education volume 17 , Article number: 3 ( 2020 ) Cite this article
104k Accesses
191 Citations
10 Altmetric
Metrics details
Student success plays a vital role in educational institutions, as it is often used as a metric for the institution’s performance. Early detection of students at risk, along with preventive measures, can drastically improve their success. Lately, machine learning techniques have been extensively used for prediction purpose. While there is a plethora of success stories in the literature, these techniques are mainly accessible to “computer science”, or more precisely, “artificial intelligence” literate educators. Indeed, the effective and efficient application of data mining methods entail many decisions, ranging from how to define student’s success , through which student attributes to focus on , up to which machine learning method is more appropriate to the given problem . This study aims to provide a step-by-step set of guidelines for educators willing to apply data mining techniques to predict student success. For this, the literature has been reviewed, and the state-of-the-art has been compiled into a systematic process, where possible decisions and parameters are comprehensively covered and explained along with arguments. This study will provide to educators an easier access to data mining techniques, enabling all the potential of their application to the field of education.
Introduction
Computers have become ubiquitous, especially in the last three decades, and are significantly widespread. This has led to the collection of vast volumes of heterogeneous data, which can be utilized for discovering unknown patterns and trends (Han et al., 2011 ), as well as hidden relationships (Sumathi & Sivanandam, 2006 ), using data mining techniques and tools (Fayyad & Stolorz, 1997 ). The analysis methods of data mining can be roughly categorized as: 1) classical statistics methods (e.g. regression analysis, discriminant analysis, and cluster analysis) (Hand, 1998 ), 2) artificial intelligence (Zawacki-Richter, Marín, Bond, & Gouverneur, 2019 ) (e.g. genetic algorithms, neural computing, and fuzzy logic), and 3) machine learning (e.g. neural networks, symbolic learning, and swarm optimization) (Kononenko & Kukar, 2007 ). The latter consists of a combination of advanced statistical methods and AI heuristics. These techniques can benefit various fields through different objectives, such as extracting patterns, predicting behavior, or describing trends. A standard data mining process starts by integrating raw data – from different data sources – which is cleaned to remove noise, duplicated or inconsistent data. After that, the cleaned data is transformed into a concise format that can be understood by data mining tools, through filtering and aggregation techniques. Then, the analysis step identifies the existing interesting patterns, which can be displayed for a better visualization (Han et al., 2011 ) (Fig. 1 ).
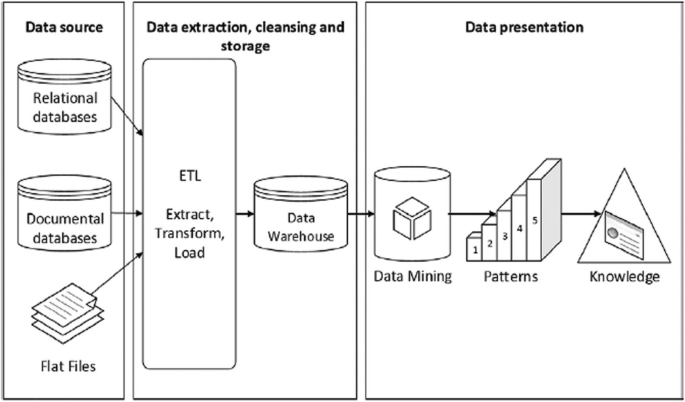
standard data mining process (Han et al. 2011 )
Recently data mining has been applied to various fields like healthcare (Kavakiotis et al., 2017 ), business (Massaro, Maritati, & Galiano, 2018 ), and also education (Adekitan, 2018 ). Indeed, the development of educational database management systems created a large number of educational databases, which enabled the application of data mining to extract useful information from this data. This led to the emergence of Education Data Mining (EDM) (Calvet Liñán & Juan Pérez, 2015 ; Dutt, Ismail, & Herawan, 2017 ) as an independent research field. Nowadays, EDM plays a significant role in discovering patterns of knowledge about educational phenomena and the learning process (Anoopkumar & Rahman, 2016 ), including understanding performance (Baker, 2009 ). Especially, data mining has been used for predicting a variety of crucial educational outcomes, like performance (Xing, 2019 ), retention (Parker, Hogan, Eastabrook, Oke, & Wood, 2006 ), success (Martins, Miguéis, Fonseca, & Alves, 2019 ; Richard-Eaglin, 2017 ), satisfaction (Alqurashi, 2019 ), achievement (Willems, Coertjens, Tambuyzer, & Donche, 2018 ), and dropout rate (Pérez, Castellanos, & Correal, 2018 ).
The process of EDM (see Fig. 2 ) is an iterative knowledge discovery process that consists of hypothesis formulation, testing, and refinement (Moscoso-Zea et al., 2016 ; Sarala & Krishnaiah, 2015 ). Despite many publications, including case studies, on educational data mining, it is still difficult for educators – especially if they are a novice to the field of data mining – to effectively apply these techniques to their specific academic problems. Every step described in Fig. 2 necessitates several decisions and set-up of parameters, which directly affect the quality of the obtained result.
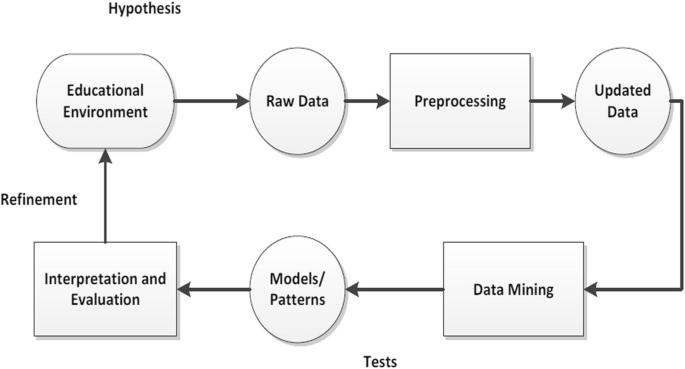
Knowledge discovery process in educational institutions (Moscoso-Zea, Andres-Sampedro, & Lujan-Mora, 2016 )
This study aims to fill the described gap, by providing a complete guideline, providing easier access to data mining techniques and enabling all the potential of their application to the field of education. In this study, we specifically focus on the problem of predicting the academic success of students in higher education. For this, the state-of-the-art has been compiled into a systematic process, where all related decisions and parameters are comprehensively covered and explained along with arguments.
In the following, first, section 2 clarifies what is academic success and how it has been defined and measured in various studies with a focus on the factors that can be used for predicting academic success. Then, section 3 presents the methodology adopted for the literature review. Section 4 reviews data mining techniques used in predicting students’ academic success, and compares their predictive accuracy based on various case studies. Section 5 concludes the review, with a recapitulation of the whole process. Finally, section 6 concludes this paper and outlines the future work.
Academic success definition
Student success is a crucial component of higher education institutions because it is considered as an essential criterion for assessing the quality of educational institutions (National Commission for Academic Accreditation &, 2015 ). There are several definitions of student success in the literature. In (Kuh, Kinzie, Buckley, Bridges, & Hayek, 2006 ), a definition of student success is synthesized from the literature as “Student success is defined as academic achievement, engagement in educationally purposeful activities, satisfaction, acquisition of desired knowledge, skills and competencies, persistence, attainment of educational outcomes, and post-college performance”. While this is a multi-dimensional definition, authors in (York, Gibson, & Rankin, 2015 ) gave an amended definition concentrating on the most important six components, that is to say “Academic achievement, satisfaction, acquisition of skills and competencies, persistence, attainment of learning objectives, and career success” (Fig. 3 ).
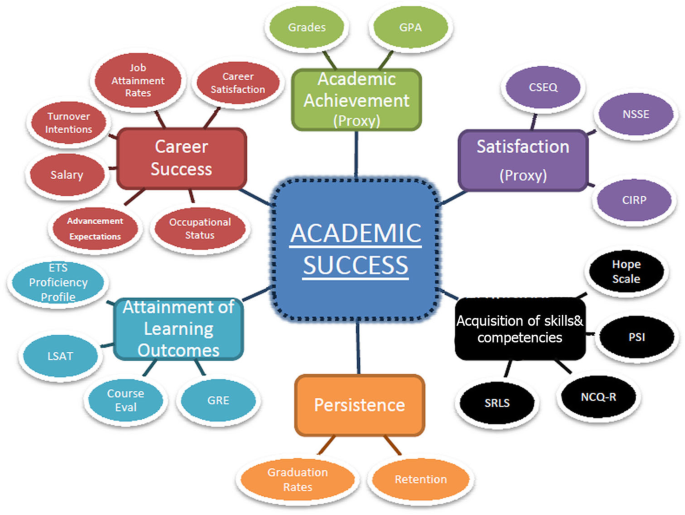
Defining academic success and its measurements (York et al., 2015 )
Despite reports calling for more detailed views of the term, the bulk of published researchers measure academic success narrowly as academic achievement. Academic achievement itself is mainly based on Grade Point Average (GPA), or Cumulative Grade Point Average (CGPA) (Parker, Summerfeldt, Hogan, & Majeski, 2004 ), which are grade systems used in universities to assign an assessment scale for students’ academic performance (Choi, 2005 ), or grades (Bunce & Hutchinson, 2009 ). The academic success has also been defined related to students’ persistence, also called academic resilience (Finn & Rock, 1997 ), which in turn is also mainly measured through the grades and GPA, measures of evaluations by far the most widely available in institutions.
Review methodology
Early prediction of students’ performance can help decision makers to provide the needed actions at the right moment, and to plan the appropriate training in order to improve the student’s success rate. Several studies have been published in using data mining methods to predict students’ academic success. One can observe several levels targeted:
Degree level: predicting students’ success at the time of obtention of the degree.
Year level: predicting students’ success by the end of the year.
Course level: predicting students’ success in a specific course.
Exam level: predicting students’ success in an exam for a specific course.
In this study, the literature related to the exam level is excluded as the outcome of a single exam does not necessarily imply a negative outcome.
In terms of coverage, section 4 and 5 only covers articles published within the last 5 years. This restriction was necessary to scale down the search space, due to the popularity of EDM. The literature was searched from Science Direct, ProQuest, IEEE Xplore, Springer Link, EBSCO, JSTOR, and Google Scholar databases, using academic success , academic achievement , student success , educational data mining , data mining techniques , data mining process and predicting students’ academic performance as keywords. While we acknowledge that there may be articles not included in this review, seventeen key articles about data mining techniques that were reviewed in sections 4 and 5 .
Influential factors in predicting academic success
One important decision related to the prediction of students’ academic success in higher education is to clearly define what is academic success. After that, one can think about the potential influential factors, which are dictating the data that needs to be collected and mined.
While a broad variety of factors have been investigated in the literature with respect to their impact on the prediction of students’ academic success (Fig. 4 ), we focus here on prior-academic achievement , student demographics , e-learning activity , psychological attributes , and environments , as our investigation revealed that they are the most commonly reported factors (summarized in Table 1 ). As a matter of fact, the top 2 factors, namely, prior-academic achievement , and student demographics , were presented in 69% of the research papers. This observation is aligned with the results of The previous literature review which emphasized that the grades of internal assessment and CGPA are the most common factors used to predict student performance in EDM (Shahiri, Husain, & Rashid, 2015 ). With more than 40%, prior academic achievement is the most important factor. This is basically the historical baggage of students. It is commonly identified as grades (or any other academic performance indicators) that students obtained in the past (pre-university data, and university-data). The pre-university data includes high school results that help understand the consistency in students’ performance (Anuradha & Velmurugan, 2015 ; Asif et al., 2015 ; Asif et al., 2017 ; Garg, 2018 ; Mesarić & Šebalj, 2016 ; Mohamed & Waguih, 2017 ; Singh & Kaur, 2016 ). They also provide insight into their interest in different topics (i.e., courses grade (Asif et al., 2015 ; Asif et al., 2017 ; Oshodi et al., 2018 ; Singh & Kaur, 2016 )). Additionally, this can also include pre-admission data which is the university entrance test results (Ahmad et al., 2015 ; Mesarić & Šebalj, 2016 ; Oshodi et al., 2018 ). The university-data consists of grades already obtained by the students since entering the university, including semesters GPA or CGPA (Ahmad et al., 2015 ; Almarabeh, 2017 ; Hamoud et al., 2018 ; Mueen et al., 2016 ; Singh & Kaur, 2016 ), courses marks (Al-barrak & Al-razgan, 2016 ; Almarabeh, 2017 ; Anuradha & Velmurugan, 2015 ; Asif et al., 2015 ; Asif et al., 2017 ; Hamoud et al., 2018 ; Mohamed & Waguih, 2017 ; Mueen et al., 2016 ; Singh & Kaur, 2016 ; Sivasakthi, 2017 ) and course assessment grades (e.g. assignment (Almarabeh, 2017 ; Anuradha & Velmurugan, 2015 ; Mueen et al., 2016 ; Yassein et al., 2017 ); quizzes (Almarabeh, 2017 ; Anuradha & Velmurugan, 2015 ; Mohamed & Waguih, 2017 ; Yassein et al., 2017 ); lab-work (Almarabeh, 2017 ; Mueen et al., 2016 ; Yassein et al., 2017 ); and attendance (Almarabeh, 2017 ; Anuradha & Velmurugan, 2015 ; Garg, 2018 ; Mueen et al., 2016 ; Putpuek et al., 2018 ; Yassein et al., 2017 )).
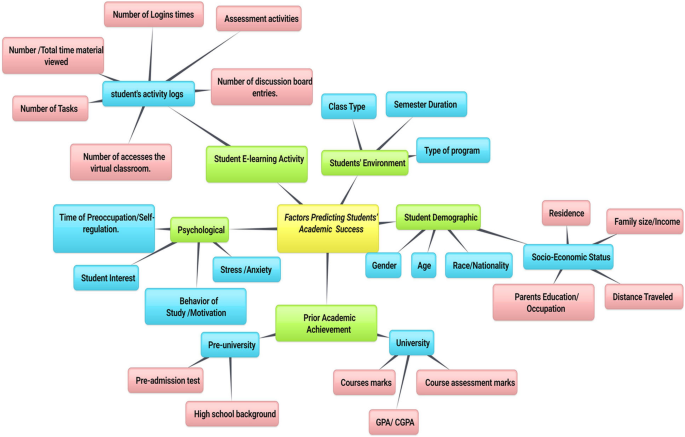
a broad variety of factors potentially impacting the prediction of students’ academic success
Students’ demographic is a topic of divergence in the literature. Several studies indicated its impact on students’ success, for example, gender (Ahmad et al., 2015 ; Almarabeh, 2017 ; Anuradha & Velmurugan, 2015 ; Garg, 2018 ; Hamoud et al., 2018 ; Mohamed & Waguih, 2017 ; Putpuek et al., 2018 ; Sivasakthi, 2017 ), age (Ahmad et al., 2015 ; Hamoud et al., 2018 ; Mueen et al., 2016 ), race/ethnicity (Ahmad et al., 2015 ), socioeconomic status (Ahmad et al., 2015 ; Anuradha & Velmurugan, 2015 ; Garg, 2018 ; Hamoud et al., 2018 ; Mohamed & Waguih, 2017 ; Mueen et al., 2016 ; Putpuek et al., 2018 ), and father’s and mother’s background (Hamoud et al., 2018 ; Mohamed & Waguih, 2017 ; Singh & Kaur, 2016 ) have been shown to be important. Yet, few studies also reported just the opposite, for gender in particular (Almarabeh, 2017 ; Garg, 2018 ).
Some attributes related to the student’s environment were found to be impactful information such as program type (Hamoud et al., 2018 ; Mohamed & Waguih, 2017 ), class type (Mueen et al., 2016 ; Sivasakthi, 2017 ) and semester period (Mesarić & Šebalj, 2016 ).
Among the reviewed papers, also many researchers used Student E-learning Activity information, such as a number of login times, number of discussion board entries, number / total time material viewed (Hamoud et al., 2018 ), as influential attributes and their impact, though minor, were reported.
The psychological attributes are determined as the interests and personal behavior of the student; several studies have shown them to be impactful on students’ academic success. To be more precise, student interest (Hamoud et al., 2018 ), the behavior towards study (Hamoud et al., 2018 ; Mueen et al., 2016 ), stress and anxiety (Hamoud et al., 2018 ; Putpuek et al., 2018 ), self-regulation and time of preoccupation (Garg, 2018 ; Hamoud et al., 2018 ), and motivation (Mueen et al., 2016 ), were found to influence success.
Data mining techniques for prediction of students’ academic success
The design of a prediction model using data mining techniques requires the instantiation of many characteristics, like the type of the model to build, or methods and techniques to apply (Witten, Frank, Hall, & Pal, 2016 ). This section defines these attributes, provide some of their instances, and reveal the statistics of their occurrence among the reviewed papers grouped by the target variable in the student success prediction, that is to say, degree level, year level, and course level.
Degree level
Several case studies have been published, seeking prediction of academic success at the degree level. One can observe two main approaches in term of the model to build: classification where CGPA that is targeted is a category as multi class problem such as (a letter grade (Adekitan & Salau, 2019 ; Asif et al., 2015 ; Asif et al., 2017 ) or overall rating (Al-barrak & Al-razgan, 2016 ; Putpuek et al., 2018 )) or binary class problem such as (pass/fail (Hamoud et al., 2018 ; Oshodi et al., 2018 )). As for the other approach, it is the regression where the numerical value of CGPA is predicted (Asif et al., 2017 ). We can also observe a broad variety in terms of the department students belongs to, from architecture (Oshodi et al., 2018 ), to education (Putpuek et al., 2018 ), with a majority in technical fields (Adekitan & Salau, 2019 ; Al-barrak & Al-razgan, 2016 ; Asif et al., 2015 ; Hamoud et al., 2018 ). An interesting finding is related to predictors: studies that included university-data, especially grades from first 2 years of the program, yielded better performance than studies that included only demographics (Putpuek et al., 2018 ), or only pre-university data (Oshodi et al., 2018 ). Details regarding the algorithm used, the sample size, the best accuracy and corresponding method, as well as the software environment that was used are all in Table 2 .
Less case studies have been reported, seeking prediction of academic success at the year level. Yet, the observations regarding these studies are very similar to the one related to degree level (reported in previous section). Similar to previous sub-section, studies that included only social conditions and pre-university data gave the worse accuracy (Singh & Kaur, 2016 ), while including university-data improved results (Anuradha & Velmurugan, 2015 ). Nevertheless, it is interesting to note that even the best accuracy in (Anuradha & Velmurugan, 2015 ) is inferior to the accuracy in (Adekitan & Salau, 2019 ; Asif et al., 2015 ; Asif et al., 2017 ) reported in previous section. This can be explained by the fact that in (Anuradha & Velmurugan, 2015 ), only 1 year of past university-data is included while in (Asif et al., 2015 ; Asif et al., 2017 ), 2 years of past university-data and in (Adekitan & Salau, 2019 ) 3 years of past university-data is covered. Other details for these methods are in Table 3 .
Course level
Finally, some studies can be reported, seeking the prediction of academic success at the course level. As already mentioned in degree level and year level sections, the comparative work gives accuracies of 62% to 89% while predicting success at a course level can give accuracies more than 89%, which can be seen as a more straightforward task than predicting success at degree level or year level. The best accuracy is obtained in course level with 93%. In (Garg, 2018 ), the target course was an advanced programming course while the influential factor was a previous programming course, also a prerequisite course. This demonstrates how important it is to have a field knowledge and use this knowledge to guide the decisions in the process and target important features. All other details for these methods are in Table 4 .
Data mining process model for student success prediction
This section compiles as a set of guidelines the various steps to take while using educational data mining techniques for student success prediction; all decisions needed to be taken at various stages of the process are explained, along with a shortlist of best practices collected from the literature. The proposed framework (Fig. 5 ) has been derived from well-known processes (Ahmad et al., 2015 ; Huang, 2011 ; Pittman, 2008 ). It consists of six main stages: 1) data collection, 2) data initial preparation, 3) statistical analysis, 4) data preprocessing, 5) data mining implementation, and 6) result evaluation. These stages are detailed in the next subsections.

Stages of the EDM framework
Data collection
In educational data mining, the needed information can be extracted from multiple sources. As indicated in Table 1 , the most influential factor observed in the literature is Prior Academic Achievement. Related data, that is to say, pre-university or university-data, can easily be retrieved from the university Student Information System (SIS) that are so widely used nowadays. SIS can also provide some student demographics (e.g. age, gender, ethnicity), but socio-economic status might not be available explicitly. In that case, this could either be deduced from existing data, or it might be directly acquired from students through surveys. Similarly, students’ environment related information also can be extracted from the SIS, while psychological data would probably need the student to fill a survey. Finally, students’ e-learning activities can be obtained from e-learning system logs (Table 5 ).
Initial preparation of data
In its original form, the data (also called raw data) is usually not ready for analysis and modeling. Data sets that are mostly obtained from merging tables in the various systems cited in Table 5 might contain missing data, inconsistent data, incorrect data, miscoded data, and duplicate data. This is why the raw data needs to go through an initial preparation (Fig. 6 ), consisting of 1) selection, 2) cleaning, and 3) derivation of new variables. This is a vital step, and usually the most time consuming (CrowdFlower, 2016 ).
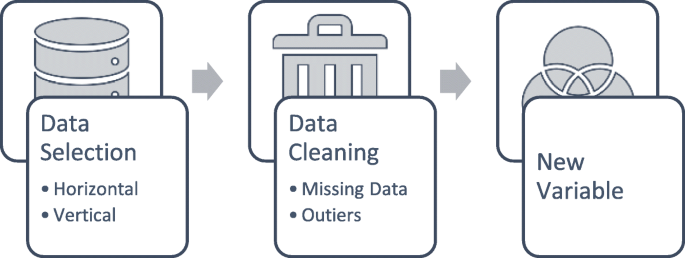
Initial Preparation of Data
Data selection
The dimension of the data gathered can be significant, especially while using prior academic achievements (e.g. if all past courses are included both from high-school and completed undergraduate years). This can negatively impact the computational complexity. Furthermore, including all the gathered data in the analysis can yield below optimal prediction results, especially in case of data redundancy, or data dependency. Thus, it is crucial to determine which attributes are important, or needs to be included in the analysis. This requires a good understanding of the data mining goals as well as the data itself (Pyle, Editor, & Cerra, 1999 ). Data selection, also called “Dimensionality Reduction” (Liu & Motoda, 1998 ), consists in vertical (attributes/variables) selection and horizontal (instance/records) selection (García, Luengo, & Herrera, 2015 ; Nisbet, Elder, & Miner, 2009 ; Pérez et al., 2015 ) (Table 6 ). Also, it is worth noticing that models obtained from a reduced number of features will be easier to understand (Pyle et al., 1999 ).
Data cleaning
Data sources tend to be inconsistent, contain noises, and usually suffer from missing values (Linoff & Berry, 2011 ). When a value is not stored for a variable, it is considered as missing data. When a value is in an abnormal distance from the other values in the dataset, it is called an outlier. Literature reveals that missing values and outliers are very common in the field of EDM. Thus, it is important to know how to handle them without compromising the quality of the prediction. All things considered, dealing with missing values or outliers cannot be done by a general procedure, and several methods need to be considered within the context of the problem. Nevertheless, we try to here to summarize the main approaches observed in the literature and Table 7 provides a succinct summary of them.
If not treated, missing value becomes a problem for some classifiers. For example, Support Vector Machines (SVMs), Neural Networks (NN), Naive Bayes, and Logistic Regression require full observation (Pelckmans, De Brabanter, Suykens, & De Moor, 2005 ; Salman & Vomlel, 2017 ; Schumacker, 2012 ), however, decision trees and random forests can handle missing data (Aleryani, Wang, De, & Iglesia, 2018 ). There are two strategies to deal with missing values. The first one is a listwise deletion, and it consists in deleting either the record (row deletion, when missing values are few) or the attribute/variable (column deletion, when missing values are too many). The second strategy, imputation, that derives the missing value from the remainder of the data (e.g. median, mean, a constant value for numerical value, or randomly selected value from missing values distribution (McCarthy, McCarthy, Ceccucci, & Halawi, 2019 ; Nisbet et al., 2009 )).
Outliers data are also known as anomalies, can easily be identified by visual means, creating a histogram, stem and leaf plots or box plots and looking for very high or very low values. Once identified, outliers can be removed from the modeling data. Another possibility is to converts the numeric variable to a categorical variable (i.e. bin the data) or leaves the outliers in the data (McCarthy et al., 2019 ).
Derivation of new variables
New variables can be derived from existing variables by combining them (Nisbet et al., 2009 ). When done based on domain knowledge, this can improve the data mining system (Feelders, Daniels, & Holsheimer, 2000 ). For example, GPA is a common variable that can be obtained from SIS system. If taken as it is, a student’s GPA reflects his/her average in a given semester. However, this does not explicitly say anything about this student’s trend over several semesters. For the same GPA, one student could be in a steady state, going through an increasing trend, or experiencing a drastic performance drop. Thus, calculating the difference in GPA between consecutive semesters will add an extra information. While there is no systematic method for deriving new variables, Table 8 recapitulates the instances that we observed in the EDM literature dedicated to success prediction.
Statistical analysis
Preliminary statistical analysis, especially through visualization, allows to better understand the data before moving to more sophisticated data mining tasks and algorithms (McCarthy et al., 2019 ). Table 9 summarizes the statistics commonly derived depending on the data type. Data mining tools contain descriptive statistical capabilities. Dedicated tools like STATISTICA (Jascaniene, Nowak, Kostrzewa-Nowak, & Kolbowicz, 2013 ) and SPSS (L. A. D. of S. University of California and F. Foundation for Open Access Statistics, 2004 ) can also provide tremendous insight.
It is important to note that this step can especially help planning further steps in DM process, including data pre-processing to identify the outliers, determining the patterns of missing data, study the distribution of each variable and identify the relationship between independent variables and the target variable (see Table 10 ). Furthermore, statistical analysis is used in the interpreting stage to explain the results of the DM model (Pyle et al., 1999 ).
Data preprocessing
The last step before the analysis of the data and modeling is preprocessing, which consists of 1) data transformation, 2) how to handle imbalanced data sets, and 3) feature selection (Fig. 7 ).
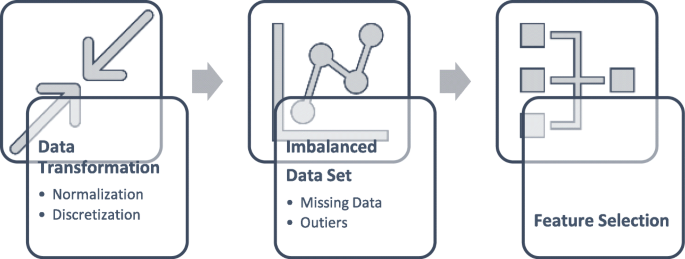
Data Preprocessing
Data transformation
Data transformation is a necessary process to eliminate dissimilarities in the dataset, thus it becomes more appropriate for data mining (Osborne, 2002 ). In EDM for success prediction, we can observe the following operations:
Normalization of numeric attributes: this is a scaling technique used when the data includes varying scales, and the used data mining algorithm cannot provide a clear assumptions of the data distribution (Patro & Sahu, 2015 ). We can cite K-nearest neighbors and artificial neural networks (How to Normalize and Standardize Your Machine Learning Data in Weka, n.d. ) as examples of such algorithms. Normalizing the data may improve the accuracy and the efficiency of the mining algorithms, and provide better results (Shalabi & Al-Kasasbeh, 2006 ). The common normalization techniques are min-max (MM), decimal scaling, Z-score (ZS), median and MAD, double sigmoid (DS), tanh, and bi-weight normalizations (Kabir, Ahmad, & Swamy, 2015 ).
Discretization: The simplest method of discretization binning (García et al., 2015 ), converts a continuous numeric variable into a series of categories by creating a finite number of bins and assigning a specific number of values to each attribute in each bin. Discretization is a necessary step when using DM techniques that allow only for categorical variables (Liu, Hussain, Tan, & Dash, 2002 ; Maimon & Rokach, 2005 ) such as C4.5 (Quinlan, 2014 ), Apriori (Agrawal, 2005 ) and Naïve Bayes (Flores, Gámez, Martínez, & Puerta, 2011 ). Discretization also increases the accuracy of the models by overcoming noisy data, and by identifying outliers’ values. Finally, discrete features are easier to understand, handle, and explain.
Convert to numeric variables: Most DM algorithms offer better results using a numeric variable. Therefore, data needs to be converted into numerical variables, using any of these methods:
Encode labels using a value between [0 and N (class-1)34 ] where N is the number of labels (Why One-Hot Encode Data in Machine Learning, n.d. ).
A dummy variable is a binary variable denoted as (0 or 1) to represent one level of a categorical variable, where (1) reflects the presence of level and (0) reflects the absence of level. One dummy variable will be created for each present level (Mayhew & Simonoff, 2015 ).
Combining levels: this allows reducing the number of levels in categorical variables and improving model performance. This is done by simply combining similar levels into alike groups through domain (Simple Methods to deal with Categorical Variables in Predictive Modeling, n.d. ).
However, note that all these methods do not necessarily lead to improved results. Therefore, it is important to repeat the modeling process by trying different preprocessing scenarios, evaluate the performance of the model, and identify the best results. Table 11 . recapitulates the various EDM application of preprocessing methods.
Imbalanced datasets
It is common in EDM applications that the dataset is imbalanced, meaning that the number of samples from one class is significantly less than the samples from other classes (e.g. number of failing students vs passing students) (El-Sayed, Mahmood, Meguid, & Hefny, 2015 ; Qazi & Raza, 2012 ). This lack of balance may negatively impact the performance of data mining algorithms (Chotmongkol & Jitpimolmard, 1993 ; Khoshgoftaar, Golawala, & Van Hulse, 2007 ; Maheshwari, Jain, & Jadon, 2017 ; Qazi & Raza, 2012 ). Re-sampling (under or over-sampling) is the solution of choice (Chotmongkol & Jitpimolmard, 1993 ; Kaur & Gosain, 2018 ; Maheshwari et al., 2017 ). Under-sampling consists in removing instances from the major class, either randomly or by some techniques to balance the classes. Oversampling consists of increasing the number of instances in the minor class, either by randomly duplicating some samples, or by synthetically generating samples (Chawla, Bowyer, Hall, & Kegelmeyer, 2002 ) (see Table 12 ).
Feature selection
When the data set is prepared and ready for modeling, then the important variables can be chosen and submitted to the modeling algorithm. This step, called feature selection, is an important strategy to be followed to mining the data (Liu & Motoda, 1998 ). Feature selection aims to choose a subset of attributes from the input data with the capability of giving an efficient description for the input data while reducing effects from unrelated variables while preserving sufficient prediction results (Guyon & Elisseeff, 2003 ). Feature selection enables reduced computation time, improved prediction performance while allowing a better understanding of the data (Chandrashekar & Sahin, 2014 ). Feature selection methods are classified into filter and wrapper methods (Kohavi & John, 1997 ). Filter methods work as preprocessing to rank the features, so high-ranking features are identified and applied to the predictor. In wrapper methods, the criterion for selecting the feature is the performance of the forecasting device, meaning that the predictor is wrapped on a search algorithm which will find a subset that gives the highest predictor performance. Moreover, there are embedded methods (Blum & Langley, 1997 ; Guyon & Elisseeff, 2003 ; P. (Institute for the S. of L. and E. Langley, 1994 ) which include variable selection as part of the training process without the need for splitting the data into training and testing sets. However, most data mining tools contains embedded feature selection methods making it easy to try them and chose the best one.
Data mining implementation
Data mining models.
Two types of data mining models are commonly used in EDM applications for success prediction: predictive and descriptive (Kantardzic, 2003 ). Predictive models apply supervised learning functions to provide estimation for expected values of dependent variables according to the features of relevant independent variables (Bramer, 2016 ). Descriptive models are used to produce patterns that describe the fundamental structure, relations, and interconnectedness of the mined data by applying unsupervised learning functions on it (Peng, Kou, Shi, & Chen, 2008 ). Typical examples of predictive models are classification (Umadevi & Marseline, 2017 ) and regression (Bragança, Portela, & Santos, 2018 ), while clustering (Dutt et al., 2017 ) and association (Zhang, Niu, Li, & Zhang, 2018 ), produce descriptive models. As stated in section 4 , classification is the most used method, followed by regression and clustering. The most commonly used classification techniques are Bayesian networks, neural networks, decision trees (Romero & Ventura, 2010 ). Common regression techniques are linear regression and logistic regression analysis (Siguenza-Guzman, Saquicela, Avila-Ordóñez, Vandewalle, & Cattrysse, 2015 ). Clustering uses techniques like neural networks, K-means algorithms, fuzzy clustering and discrimination analysis (Dutt et al., 2017 ). Table 13 shows the recurrence of specific algorithms based on the literature review that we performed.
In the process, first one needs to choose a model, namely predictive or descriptive. Then, the algorithms to build the models are chosen from the 10 techniques considered as the top 10 in DM in terms of performance, always prefer models that are interpretable and understandable such as DT and linear models (Wu et al., 2008 ). Once the algorithms have been chosen, they require to be configured before they are applied. The user must provide suitable values for the parameters in advance in order to obtain good results for the models. There are various strategies to tune parameters for EDM algorithms, used to find the most useful performing parameters. The trial and error approach is one of the simplest and easiest methods for non-expert users (Ruano, Ribes, Sin, Seco, & Ferrer, 2010 ). It consists of performing numerous experiments by modifying the parameters’ values until finding the most beneficial performing parameters.
Data mining tools
Data mining has a stack of open source tools such as machine learning tools which supports the researcher in analyzing the dataset using several algorithms. Such tools are vastly used for predictive analysis, visualization, and statistical modeling. WEKA is the most used tool for predictive modeling (Jayaprakash, 2018 ). This can be explained by its many pre-built tools for data pre-processing, classification, association rules, regression, and visualization, as well as its user-friendliness, and accessibility even to a novice in programming or data mining. But we can also cite RapidMiner and Clementine as stated in Table 4 .
Results evaluation
As several models are usually built, it is important to evaluate them and select the most appropriate. While evaluating the performance of classification algorithms, normally the confusion matrix as shown in Table 14 is used. This table gathers four important metrics related to a given success prediction model:
True Positive (TP): number of successful students classified correctly as “successful”.
False Positive (FP): number of successful students incorrectly classified as “non-successful”.
True Negative (TN): number of did not successful students classified correctly as “non-successful”.
False Negative (FN): number of did not successful students classified incorrectly as “successful”.
Different performance measures are included to evaluate the model of each classifier, almost all measures of performance are based on the confusion matrix and the numbers in it. To produce more accurate results, these measures are evaluated together. In this research, we’ll focus on the measures used in the classification problems. The measures commonly used in the literature are provided in Table 15 .
Early student performance prediction can help universities to provide timely actions, like planning for appropriate training to improve students’ success rate. Exploring educational data can certainly help in achieving the desired educational goals. By applying EDM techniques, it is possible to develop prediction models to improve student success. However, using data mining techniques can be daunting and challenging for non-technical persons. Despite the many dedicated software’s, this is still not a straightforward process, involving many decisions. This study presents a clear set of guidelines to follow for using EDM for success prediction. The study was limited to undergraduate level, however the same principles can be easily adapted to graduate level. It has been prepared for those people who are novice in data mining, machine learning or artificial intelligence.
A variety of factors have been investigated in the literature related to its impact on predicting students ‘academic success which was measured as academic achievement, as our investigation showed that prior-academic achievement, student demographics, e-learning activity, psychological attributes, are the most common factors reported. In terms of prediction techniques, many algorithms have been applied to predict student success under the classification technique.
Moreover, a six stages framework is proposed, and each stage is presented in detail. While technical background is kept to a minimum, as this not the scope of this study, all possible design and implementation decisions are covered, along with best practices compiled from the relevant literature.
It is an important implication of this review that educators and non-proficient users are encouraged to applied EDM techniques for undergraduate students from any discipline (e.g. social sciences). While reported findings are based on the literature (e.g. potential definition of academic success, features to measure it, important factors), any available additional data can easily be included in the analysis, including faculty data (e.g. competence, criteria of recruitment, academic qualifications) may be to discover new determinants.
Availability of data and materials
Not applicable.
Abbreviations
(Probabilistic) neural network
Classification
- Data mining
Decision tree
Educational data mining
K-nearest neighbors
Logistic regression
Naive Bayes
Neural network
Random forest
Rule induction
Random tree
Tree ensemble
Adekitan, A. I. (2018). “Data mining approach to predicting the performance of first year student in a university using the admission requirements,” no. Aina 2002 .
Google Scholar
Adekitan, A. I., & Salau, O. (2019). The impact of engineering students’ performance in the first three years on their graduation result using educational data mining. Heliyon , 5 (2), e01250.
Article Google Scholar
Agrawal, S. (2005). Database Management Systems Fast Algorithms for Mining Association Rules. In In Proc. 20th int. conf. very large data bases, VLDB , (pp. 487–499).
Ahmad, F., Ismail, N. H., & Aziz, A. A. (2015). The Prediction of Students ’ Academic Performance Using Classification Data Mining Techniques, 9 (129), 6415–6426.
Al-barrak, M. A., & Al-razgan, M. (2016). Predicting Students Final GPA Using Decision Trees : A Case Study. International Journal of Information and Education Technology, 6 (7), 528–533.
Aleryani, A., Wang, W., De, B., & Iglesia, L. (2018). Dealing with missing data and uncertainty in the context of data mining. In International Conference on Hybrid Artificial Intelligence Systems .
Almarabeh, H. (2017). Analysis of students’ performance by using different data mining classifiers. International Journal of Modern Education and Computer Science , 9 (8), 9–15.
Alqurashi, E. (2019). Predicting student satisfaction and perceived learning within online learning environments. Distance Education , 40 (1), 133–148.
Anoopkumar, M., & Rahman, A. M. J. M. Z. (2016). A Review on Data Mining techniques and factors used in Educational Data Mining to predict student amelioration. In 2016 International Conference on Data Mining and Advanced Computing (SAPIENCE) , (pp. 122–133).
Chapter Google Scholar
Anuradha, C., & Velmurugan, T. (2015). A Comparative Analysis on the Evaluation of Classification Algorithms in the Prediction of Students Performance. Indian Journal of Science and Technology , 8 (July), 1–12.
Asif, R., Merceron, A., Abbas, S., & Ghani, N. (2017). Analyzing undergraduate students ’ performance using educational data mining. Computers in Education , 113 , 177–194.
Asif, R., Merceron, A., & Pathan, M. K. (2015). Predicting student academic performance at degree level: A case study. International Journal of Intelligent Systems and Applications , 7 (1), 49–61.
Baker, R. Y. A. N. S. J. D. (2009). The State of Educational Data Mining in 2009: A Review and Future Visions. Journal of Educational Data Mining , 5(8), 3–16.
Blum, A. L., & Langley, P. (1997). Selection of relevant features and examples in machine learning. Artificial Intelligence , 97 (1–2), 245–271.
Article MathSciNet MATH Google Scholar
Bragança, R., Portela, F., & Santos, M. (2019). A regression data mining approach in Lean Production. Concurrency and Computation: Practice and Experience, 31(22), e4449.
Bramer, M. (2016). Principles of data mining . London: Springer London.
Book MATH Google Scholar
Bunce, D. M., & Hutchinson, K. D. (2009). The use of the GALT (Group Assessment of Logical Thinking) as a predictor of academic success in college chemistry. Journal of Chemical Education , 70 (3), 183.
Calvet Liñán, L., & Juan Pérez, Á. A. (2015). Educational Data Mining and Learning Analytics: differences, similarities, and time evolution. International Journal of Educational Technology in Higher Education , 12 (3), 98.
Chandrashekar, G., & Sahin, F. (2014). A survey on feature selection methods. Computers & Electrical Engineering , 40 (1), 16–28.
Chawla, N. V., Bowyer, K. W., Hall, L. O., & Kegelmeyer, W. P. (2002). SMOTE: Synthetic minority over-sampling technique. Journal of Artificial Intelligence Research , 16 , 321–357.
Article MATH Google Scholar
Choi, N. (2005). Self-efficacy and self-concept as predictors of college students’ academic performance. Psychology in the Schools , 42 (2), 197–205.
Chotmongkol, V., & Jitpimolmard, S. (1993). Cryptococcal intracerebral mass lesions associated with cryptococcal meningitis. The Southeast Asian Journal of Tropical Medicine and Public Health , 24 (1), 94–98.
CrowdFlower (2016). Data Science Report , (pp. 8–9).
Dutt, A., Ismail, M. A., & Herawan, T. (2017). A systematic review on educational data mining. IEEE Access , 5 , 15991–16005.
El-Sayed, A. A., Mahmood, M. A. M., Meguid, N. A., & Hefny, H. A. (2015). Handling autism imbalanced data using synthetic minority over-sampling technique (SMOTE). In 2015 Third World Conference on Complex Systems (WCCS) , (pp. 1–5).
Fayyad, U., & Stolorz, P. (1997). Data mining and KDD: Promise and challenges. Future Generation Computer Systems , 13 (2–3), 99–115.
Feelders, A., Daniels, H., & Holsheimer, M. (2000). Methodological and practical aspects of data mining. Information Management , 37 (5), 271–281.
Finn, J. D., & Rock, D. A. (1997). Academic success among students at risk for school failure. The Journal of Applied Psychology , 82 (2), 221–234.
Flores, M. J., Gámez, J. A., Martínez, A. M., & Puerta, J. M. (2011). Handling numeric attributes when comparing Bayesian network classifiers: Does the discretization method matter? Applied Intelligence , 34 (3), 372–385.
García, S., Luengo, J., & Herrera, F. (2015). Data preprocessing in data mining , (vol. 72). Cham: Springer International Publishing.
Garg, R. (2018). Predict Sudent performance in different regions of Punjab. International Journal of Advanced Research in Computer Science , 9 (1), 236–241.
Guyon, I., & Elisseeff, A. (2003). An Introduction to Variable and Feature Selection. Journal of Machine Learning Research , 3 (Mar), 1157–1182.
MATH Google Scholar
Hamoud, A. K., Hashim, A. S., & Awadh, W. A. (2018). Predicting Student Performance in Higher Education Institutions Using Decision Tree Analysis. International Journal of Interactive Multimedia and Artificial Intelligence , inPress, 1.
Han, J., Kamber, M., & Pei, J. (2011). Data mining : concepts and techniques. Elsevier Science. Retrieved from https://www.elsevier.com/books/data-mining-concepts-and-techniques/han/978-0-12-381479-1 .
Hand, D. J. (1998). Data mining: Statistics and more? The American Statistician , 52 (2), 112–118.
“How to Normalize and Standardize Your Machine Learning Data in Weka.”n.d. [Online]. Available: https://machinelearningmastery.com/normalize-standardize-machine-learning-data-weka/ . [Accessed: 11 Jun 2019].
S. Huang, “Predictive modeling and analysis of student academic performance in an engineering dynamics course,” All Grad. Theses Diss., 2011.
Jascaniene, N., Nowak, R., Kostrzewa-Nowak, D., & Kolbowicz, M. (2013). Selected aspects of statistical analyses in sport with the use of STATISTICA software. Central European Journal of Sport Sciences and Medicine, 3(3), 3–11.
Jayaprakash, S. (2018). A Survey on Academic Progression of Students in Tertiary Education using Classification Algorithms. International Journal of Engineering Technology Science and Research IJETSR, 5(2), 136–142.
Kabir, W., Ahmad, M. O., & Swamy, M. N. S. (2015). A novel normalization technique for multimodal biometric systems. In 2015 IEEE 58th International Midwest Symposium on Circuits and Systems (MWSCAS) , (pp. 1–4).
Kantardzic, M. (2003). Data mining : concepts, models, methods, and algorithms. Wiley-Interscience. Retrieved from https://ieeexplore-ieee-org.library.iau.edu.sa/book/5265979 .
Kaur, P., & Gosain, A. (2018). Comparing the behavior of oversampling and Undersampling approach of class imbalance learning by combining class imbalance problem with noise , (pp. 23–30). Singapore: Springer.
Kavakiotis, I., Tsave, O., Salifoglou, A., Maglaveras, N., Vlahavas, I., & Chouvarda, I. (2017). Machine learning and data mining methods in diabetes research. Computational and Structural Biotechnology Journal , 15 , 104–116.
Khoshgoftaar, T. M., Golawala, M., & Van Hulse, J. (2007). An Empirical Study of Learning from Imbalanced Data Using Random Forest. In 19th IEEE International Conference on Tools with Artificial Intelligence (ICTAI 2007) , (pp. 310–317).
Kohavi, R., & John, G. H. (1997). Wrappers for feature subset selection. Artificial Intelligence , 97 (1–2), 273–324.
Kononenko, I., & Kukar, M. (2007b). Machine learning and data mining. Machine Learning and Data Mining. Woodhead Publishing Limited. https://doi.org/10.1533/9780857099440 .
Kuh, G. D., Kinzie, J., Buckley, J. A., Bridges, B. K., & Hayek, J. C. (2006). What matters to student success: A review of the literature commissioned report for the National Symposium on postsecondary student success: Spearheading a dialog on student success .
L. A. D. of S. University of California and F. Foundation for Open Access Statistics., F. (2004). A Handbook of Statistical Analyses using SPSS. Journal of Statistical Software (Vol. 11). Foundation for Open Access Statistics. Retrieved from https://doaj.org/article/d7d17defdbea412f9b8c6a74789d735e .
Linoff, G., & Berry, M. J. A. (2011). Data mining techniques : for marketing, sales, and customer relationship management. Wiley. Retrieved from https://www.wiley.com/en-us/Data+Mining+Techniques%3A+For+Marketing%2C+Sales%2C+and+Customer+Relationship+Management%2C+3rd+Edition-p-9781118087459 .
Liu, H., Hussain, F., Tan, C. L., & Dash, M. (2002). Discretization: An enabling technique. Data Mining and Knowledge Discovery , 6 (4), 393–423.
Article MathSciNet Google Scholar
Liu, H., & Motoda, H. (1998). Feature selection for knowledge discovery and data mining . US: Springer.
Maheshwari, S., Jain, R. C., & Jadon, R. S. (2017). A Review on Class Imbalance Problem: Analysis and Potential Solutions. International Journal of Computer Science Issues (IJCSI), 14(6), 43-51.
Maimon, Oded and Rokach, L. (2005). Data mining and knowledge discovery handbook. Journal of Experimental Psychology: General (Vol. 136). Springer. Retrieved from https://www.springer.com/gp/book/9780387254654 .
Martins, M. P. G., Miguéis, V. L., Fonseca, D. S. B., & Alves, A. (2019). A data mining approach for predicting academic success – A case study , (pp. 45–56). Cham: Springer.
Massaro, A., Maritati, V., & Galiano, A. (2018). Data mining model performance of sales predictive algorithms based on Rapidminer workflows. International Journal of Computer Science & Information Technology , 10 (3), 39–56.
Mayhew, M. J., & Simonoff, J. S. (2015). Non-white, no more: Effect coding as an alternative to dummy coding with implications for higher education researchers. Journal of College Student Development , 56 (2), 170–175.
McCarthy, R. V., McCarthy, M. M., Ceccucci, W., & Halawi, L. (2019). Introduction to Predictive Analytics. In Applying Predictive Analytics. Springer International Publishing. https://doi.org/10.1007/978-3-030-14038-0 .
Book Google Scholar
Mesarić, J., & Šebalj, D. (2016). Decision trees for predicting the academic success of students. Croatian Operational Research Review , 7 (2), 367–388.
Mohamed, M. H., & Waguih, H. M. (2017). Early prediction of student success using a data mining classification technique. International Journal of Science and Research , 6 (10), 126–131.
Moscoso-Zea, O., Andres-Sampedro, & Lujan-Mora, S. (2016). Datawarehouse design for educational data mining. In 2016 15th International Conference on Information Technology Based Higher Education and Training (ITHET) , (pp. 1–6).
Mueen, A., Zafar, B., & Manzoor, U. (2016). Modeling and predicting students’ academic performance using data mining techniques. International Journal of Modern Education and Computer Science , 8 (11), 36–42.
“National Commission for Academic Accreditation & Assessment Standards for Quality Assurance and Accreditation of Higher Education Institutions,” 2015.
Nisbet, R., Elder, J. F. (John F., & Miner, G. (2009). Handbook of statistical analysis and data mining applications. Academic Press/Elsevier. Retrieved from https://www.elsevier.com/books/handbook-of-statistical-analysis-and-data-mining-applications/nisbet/978-0-12-416632-5 .
Osborne, J. (2002). Notes on the Use of Data Transformation. Practical Assessment, Research, and Evaluation, 8(6), 1–7.
Oshodi, O. S., Aluko, R. O., Daniel, E. I., Aigbavboa, C. O., & Abisuga, A. O. (2018). Towards reliable prediction of academic performance of architecture students using data mining techniques. Journal of Engineering, Design and Technology , 16(3), 385–397.
P. Institute for the S. of L. and E. Langley (1994). Selection of Relevant Features in Machine Learning. In Proceedings of the AAAI Fall symposium on relevance , (pp. 140–144).
Parker, J. D., Hogan, M. J., Eastabrook, J. M., Oke, A., & Wood, L. M. (2006). Emotional intelligence and student retention: Predicting the successful transition from high school to university. Personality and Individual differences , 41 (7), 1329–1336.
Parker, J. D. A., Summerfeldt, L. J., Hogan, M. J., & Majeski, S. A. (2004). Emotional intelligence and academic success: Examining the transition from high school to university. Personality and individual differences , 36 (1), 163–172.
Patro, S. G. K., & Sahu, K. K. (2015). Normalization: A preprocessing stage. International Advanced Research Journal in Science, Engineering and Technology, 2(3), 20–22.
Pelckmans, K., De Brabanter, J., Suykens, J. A. K., & De Moor, B. (2005). Handling missing values in support vector machine classifiers. Neural Networks , 18 (5–6), 684–692.
Peng, Y., Kou, G., Shi, Y., & Chen, Z. (2008). A descriptive framework for the field of data mining and knowledge discovery. International Journal of Information Technology and Decision Making , 7 (4), 639–682.
Pérez, B., Castellanos, C., & Correal, D. (2018). Predicting student drop-out rates using data mining techniques: A case study , (pp. 111–125). Cham: Springer.
Pérez, J., Iturbide, E., Olivares, V., Hidalgo, M., Almanza, N., & Martínez, A. (2015). A data preparation methodology in data mining applied to mortality population databases. Advances in Intelligent Systems and Computing , 353 , 1173–1182.
Pittman, K. (2008). Comparison of Data Mining Techniques used to Predict Student Retention ,” ProQuest Diss. Publ, (vol. 3297573).
Putpuek, N., Rojanaprasert, N., Atchariyachanvanich, K., & Thamrongthanyawong, T. (2018). Comparative Study of Prediction Models for Final GPA Score : A Case Study of Rajabhat Rajanagarindra University. In 2018 IEEE/ACIS 17th International Conference on Computer and Information Science , (pp. 92–97).
Pyle, D., Editor, S., & Cerra, D. D. (1999). Data preparation for data mining. Applied Artificial Intelligence , 17 (5), 375–381.
Qazi, N., & Raza, K. (2012). Effect of Feature Selection, SMOTE and under Sampling on Class Imbalance Classification. In 2012 UKSim 14th International Conference on Computer Modelling and Simulation , (pp. 145–150).
Quinlan, J. R. (2014). C4. 5: programs for machine learning. Elsevier. Retrieved from https://www.elsevier.com/books/c45/quinlan/978-0-08-050058-4 .
Richard-Eaglin, A. (2017). Predicting student success in nurse practitioner programs. Journal of the American Association of Nurse Practitioners , 29 (10), 600–605.
Romero, C., & Ventura, S. (2010). Educational data mining: A review of the state of the art. IEEE Transactions on Systems, Man, and Cybernetics, Part C (Applications and Reviews) , 40 (6), 601–618.
Ruano, M. V., Ribes, J., Sin, G., Seco, A., & Ferrer, J. (2010). A systematic approach for fine-tuning of fuzzy controllers applied to WWTPs. Environmental Modelling & Software , 25 (5), 670–676.
Salman, I., & Vomlel, J. (2017). A machine learning method for incomplete and imbalanced medical data .
Sarala, V., & Krishnaiah, J. (2015). Empirical study of data mining techniques in education system. International Journal of Advances in Computer Science and Technology (IJACST) , 4(1), 15–21.
Schumacker, R. (2012). Predicting Student Graduation in Higher Education Using Data Mining Models: a Comparison. University of Alabama Libraries. Retrieved from https://ir.ua.edu/bitstream/handle/123456789/1395/file_1.pdf?sequence=1&isAllowed=y .
Shahiri, A. M., Husain, W., & Rashid, N. A. (2015). A review on predicting Student’s performance using data mining techniques. Procedia Computer Science , 72 , 414–422.
Shalabi, L., Shaaban, Z., & Kasasbeh, B. (2006). Data mining: A preprocessing engine. Journal of Computer Science , 2(9), 735–739.
Siguenza-Guzman, L., Saquicela, V., Avila-Ordóñez, E., Vandewalle, J., & Cattrysse, D. (2015). Literature review of data mining applications in academic libraries. Journal of Academic of Librarianship , 41 (4), 499–510.
“Simple Methods to deal with Categorical Variables in Predictive Modeling.” n.d. [Online]. Available: https://www.analyticsvidhya.com/blog/2015/11/easy-methods-deal-categorical-variables-predictive-modeling/ . Accessed 4 July 2019.
Singh, W., & Kaur, P. (2016). Comparative Analysis of Classification Techniques for Predicting Computer Engineering Students’ Academic Performance. International Journal of Advanced Research in Computer Science , 7(6), 31–36.
M. Sivasakthi, “Classification and Prediction based Data Mining Algorithms to Predict Students ’ Introductory programming Performance,” Icici, 0–4, 2017.
Sumathi, S., & Sivanandam, S. N. (2006). Introduction to data mining and its applications. Springer. Retrieved from https://www.springer.com/gp/book/9783540343509 .
Umadevi, S., & Marseline, K. S. J. (2017). A survey on data mining classification algorithms. In 2017 International Conference on Signal Processing and Communication (ICSPC) , (pp. 264–268).
“Why One-Hot Encode Data in Machine Learning?” n.d. [Online]. Available: https://machinelearningmastery.com/why-one-hot-encode-data-in-machine-learning/ . Accessed 4 July 2019.
Willems, J., Coertjens, L., Tambuyzer, B., & Donche, V. (2019). Identifying science students at risk in the first year of higher education: the incremental value of non-cognitive variables in predicting early academic achievement. European Journal of Psychology of Education , 34(4), 847–872.
Witten, I. H., Frank, E., Hall, M. A., & Pal, C. J. (2016). Data Mining: Practical Machine Learning Tools and Techniques. Data Mining: Practical Machine Learning Tools and Techniques (3rd ed.). Elsevier Inc. https://doi.org/10.1016/c2009-0-19715-5 .
Wu, X., et al. (2008). Top 10 algorithms in data mining. Knowledge and Information Systems , 14 (1), 1–37.
Xing, W. (2019). Exploring the influences of MOOC design features on student performance and persistence. Distance Education , 40 (1), 98–113.
Yassein, N. A., Helali, R. G. M., & Mohomad, S. B. (2017). Information Technology & Software Engineering Predicting Student Academic Performance in KSA using Data Mining Techniques. Journal of Information Technology and Software Engineering , 7(5), 1–5.
York, T. T., Gibson, C., & Rankin, S. (2015). Defining and Measuring Academic Success. Practical Assessment, Research & Evaluation , 20 , 5.
Zawacki-Richter, O., Marín, V. I., Bond, M., & Gouverneur, F. (2019). Systematic review of research on artificial intelligence applications in higher education – where are the educators? International Journal of Educational Technology in Higher Education , 16(1), 16–39 Springer Netherlands.
Zhang, L., Niu, D., Li, Y., & Zhang, Z. (2018). A Survey on Privacy Preserving Association Rule Mining. In 2018 5th International Conference on Information Science and Control Engineering (ICISCE) , (pp. 93–97).
Download references
Acknowledgments
Not applicable .
Author information
Authors and affiliations.
Department of Computer Science, College of Sciences and Humanities, Imam Abdulrahman Bin Faisal University, 12020, Jubail, 31961, Saudi Arabia
Eyman Alyahyan
Department of Computer Science, College of Computer Science and Information Technology, Imam Abdulrahman Bin Faisal University, 2435, Dammam, 31441, Saudi Arabia
Dilek Düştegör
You can also search for this author in PubMed Google Scholar
Contributions
This study is part of EA’s MS studies requirements under the supervision of DD. EA carried out the literature review, while DD is responsible of the conceptualization of the paper. EA prepared an initial draft of the manuscript, that DD thoroughly re-organized and corrected. Both authors read and approved the final manuscript.
Corresponding author
Correspondence to Dilek Düştegör .
Ethics declarations
Competing interests.
The authors declare that they have no competing interests.
Additional information
Publisher’s note.
Springer Nature remains neutral with regard to jurisdictional claims in published maps and institutional affiliations.
Rights and permissions
Open Access This article is distributed under the terms of the Creative Commons Attribution 4.0 International License ( http://creativecommons.org/licenses/by/4.0/ ), which permits unrestricted use, distribution, and reproduction in any medium, provided you give appropriate credit to the original author(s) and the source, provide a link to the Creative Commons license, and indicate if changes were made.
Reprints and permissions
About this article
Cite this article.
Alyahyan, E., Düştegör, D. Predicting academic success in higher education: literature review and best practices. Int J Educ Technol High Educ 17 , 3 (2020). https://doi.org/10.1186/s41239-020-0177-7
Download citation
Received : 09 October 2019
Accepted : 21 January 2020
Published : 10 February 2020
DOI : https://doi.org/10.1186/s41239-020-0177-7
Share this article
Anyone you share the following link with will be able to read this content:
Sorry, a shareable link is not currently available for this article.
Provided by the Springer Nature SharedIt content-sharing initiative
- Higher education
- Student success
- Human Editing
- Free AI Essay Writer
- AI Outline Generator
- AI Paragraph Generator
- Paragraph Expander
- Essay Expander
- Literature Review Generator
- Research Paper Generator
- Thesis Generator
- Paraphrasing tool
- AI Rewording Tool
- AI Sentence Rewriter
- AI Rephraser
- AI Paragraph Rewriter
- Summarizing Tool
- AI Content Shortener
- Plagiarism Checker
- AI Detector
- AI Essay Checker
- Citation Generator
- Reference Finder
- Book Citation Generator
- Legal Citation Generator
- Journal Citation Generator
- Reference Citation Generator
- Scientific Citation Generator
- Source Citation Generator
- Website Citation Generator
- URL Citation Generator
- Proofreading Service
- Editing Service
- AI Writing Guides
- AI Detection Guides
- Citation Guides
- Grammar Guides
- Paraphrasing Guides
- Plagiarism Guides
- Summary Writing Guides
- STEM Guides
- Humanities Guides
- Language Learning Guides
- Coding Guides
- Top Lists and Recommendations
- AI Detectors
- AI Writing Services
- Coding Homework Help
- Citation Generators
- Editing Websites
- Essay Writing Websites
- Language Learning Websites
- Math Solvers
- Paraphrasers
- Plagiarism Checkers
- Reference Finders
- Spell Checkers
- Summarizers
- Tutoring Websites
Most Popular
Ai or not ai a student suspects one of their peer reviewer was a bot, how to summarize a research article, loose vs lose.
13 days ago
Typely Review
How to cite a blog, what is success definition essay sample.
freepik.com
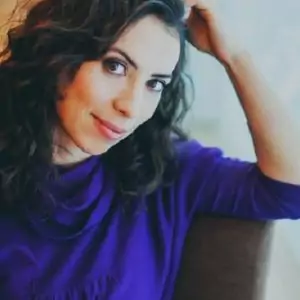
The given prompt: What defines achievement and prosperity in different cultures and individuals?
What is success? A quick look in a dictionary might define it as the attainment of wealth, favor, or eminence. Yet, to different individuals and cultures worldwide, success carries varied meanings, often transcending the typical markers of bank balances, accolades, or societal recognition. Let’s delve into how different cultures and individuals perceive achievement and prosperity.
In many Western societies, success is often tied to professional achievement and material acquisition. A top-notch job, a comfortable house, two cars in the driveway, and perhaps a yearly vacation to an exotic location often signify a successful life. These markers, rooted in capitalism and consumerism, are benchmarks many strive to achieve.
However, travel across the globe to Bhutan, and you’ll find a vastly different metric for success: Gross National Happiness (GNH). Instead of gauging success by economic growth alone, GNH considers factors such as mental well-being, health, and cultural preservation. Here, success isn’t just about individual prosperity but the holistic happiness and well-being of the entire population.
In many African cultures, the idea of Ubuntu emphasizes the interconnectedness of all individuals in a community. Roughly translating to “I am because we are,” success within this framework isn’t just about personal achievements but also how one contributes to and uplifts their community. Here, a successful individual is one who supports and is supported by their community, highlighting collective over individual prosperity.
Even within cultures, personal experiences and backgrounds can shape one’s idea of success. For someone who has grown up in a challenging environment, success might mean achieving stability and safety. For another, it could be about pursuing passions, even if it means not having a conventional nine-to-five job or a linear career path.
Age can also influence how we perceive success. Young adults often link success to recognition, achieving certain milestones by specific ages, or accumulating materialistic assets. As the same individuals age, their perception often shifts, with many placing higher value on health, relationships, contentment, or the legacy they leave behind.
The diverse definitions of success underscore an essential point: success is subjective. While society often promotes specific success markers, it’s vital for individuals to introspect and define what success genuinely means for them. It’s equally crucial to recognize and respect diverse success definitions in others.
In the age of social media, where lives are often showcased as highlight reels, it’s easy to fall into the comparison trap. But, as the age-old saying goes, “Comparison is the thief of joy.” Understanding that success is multifaceted and deeply personal can liberate one from societal pressures and pave the way for a fulfilling life based on one’s values and aspirations.
In conclusion, success, rather than being a one-size-fits-all concept, is a mosaic of beliefs, values, cultural influences, and personal experiences. Whether it’s the Western pursuit of material achievements, Bhutan’s national happiness, or the African emphasis on community, success takes many forms. Recognizing its fluidity enables individuals to carve their paths and find genuine fulfillment. After all, real success might just be about chasing purpose, not just achievements.
Follow us on Reddit for more insights and updates.
Comments (0)
Welcome to A*Help comments!
We’re all about debate and discussion at A*Help.
We value the diverse opinions of users, so you may find points of view that you don’t agree with. And that’s cool. However, there are certain things we’re not OK with: attempts to manipulate our data in any way, for example, or the posting of discriminative, offensive, hateful, or disparaging material.
Cancel reply
Your email address will not be published. Required fields are marked *
Save my name, email, and website in this browser for the next time I comment.
More from Definition Essay Examples and Samples
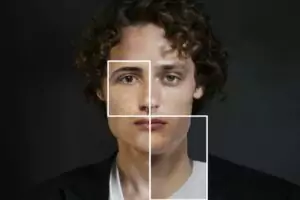
Oct 25 2023
What Is Identity? Definition Essay Sample
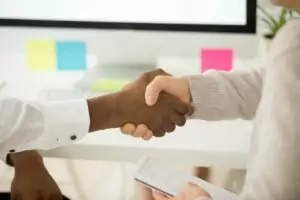
Oct 23 2023
What Is Respect? Definition Essay Example
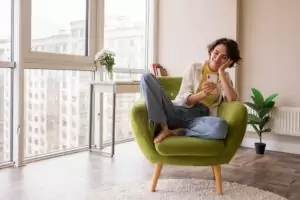
Oct 20 2023
What Is a Home? Essay Definition Examples
Remember Me
What is your profession ? Student Teacher Writer Other
Forgotten Password?
Username or Email

APPLY NOW REQUEST INFO
Apply Today
Ready to apply to Penn LPS Online? Apply Now
Learn more about Penn LPS Online
Request More Information
7 tips to help you achieve academic success
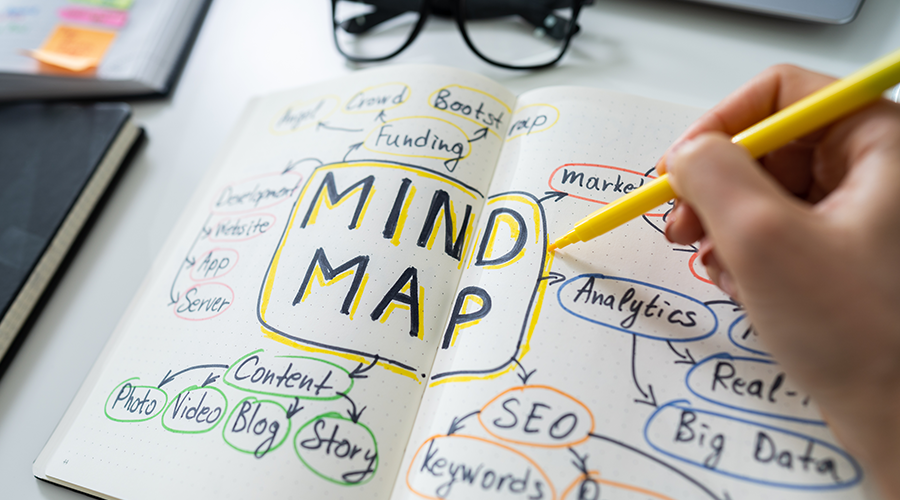
What is academic success?
Academic success means different things to different people. Whether you want to explore a subject area that you’re passionate about or enhance your credentials to advance your career, one of the primary purposes of education is to help you reach your full personal and professional potential. To achieve your vision for success, it’s important to create clear, actionable objectives and develop good habits and skills to guide your learning and keep you on track in your studies.
How do you achieve academic success?
Setting achievable short- and long-term goals is one key to academic success. When you set actionable objectives and incorporate skills and behaviors like time management, good study habits, and resilience, it’s easier to create and execute an effective study plan, track your progress, and reach your milestones. If you’re unsure of where to start when it comes to making a plan, read on to explore seven tips you can implement to align with your unique needs and experiences.
1. Work on your time management skills
One of the most critical skills for academic success is effective management of your study time. Time management skills are essential because they help ensure you stay on top of all your readings, projects, and exams. Managing your time well also requires you to stay organized, prioritize your tasks, and plan out a daily, weekly, and monthly study schedule that you stick to. How you choose to manage your study calendar is up to you, but you should create a system to track your project deadlines, exams, and study sessions in a way that’s easily accessible—whether that be a time management app on your phone, an online daily planner, or a physical calendar. As long as you carve out specific amounts of time each day or week to ensure you accomplish your high-priority items, you will set yourself up for success. It can also be helpful to let the important people in your life know about the times you have reserved for your studies so you can avoid distractions and stay focused on the tasks at hand.
2. Set small goals to help you accomplish large goals
One of the best ways to help you meet your larger, long-term goals is by tackling smaller goals that will help you achieve the results you desire. Create SMART goals that are clear and realistic and use your resources and time productively. SMART is an acronym that stands for Specific, Measurable, Achievable, Relevant, and Time-Bound. By setting SMART goals, you give yourself the opportunity to take a hard look at your objectives, understand what is motivating them, and pinpoint the actions you need to take to meet them. An example of a SMART academic goal is, “I will work to improve my grade from a B to an A by devoting four hours a week to studying my notes, readings, and flashcards for the next three weeks.”
3. Surround yourself with supportive people who motivate you
When you lean on people who support your educational journey, it can invigorate you and help stoke your determination. With family, friends, and coworkers, it’s important to open up about your experience of returning to university to enhance your skills—including both your accomplishments and your challenges. It can also be helpful to share with your instructors and peers at Penn LPS Online. Because your coursework includes group projects, presentations, and online discussions, you’ll have many opportunities to collaborate with your classmates, share your experiences, and help each other to excel in your studies. And you’ll also interact with Ivy League faculty and practitioners who are experts in their fields who will be more than willing to support you by addressing any questions or concerns you may have.
4. Build good study habits
Developing and maintaining good study habits is critical to your academic success. When you make good study habits a part of your daily routine, it can increase your efficiency, confidence, and effectiveness while reducing anxiety about your coursework. The reality is that your days are likely already packed with work, family, and other personal responsibilities, so it’s crucial you carve out time to devote to studying. To help you build strong study habits, it’s beneficial to create a consistent and structured daily plan. Ensure that you have a dedicated environment that is conducive to studying and avoid procrastination by holding yourself accountable to your dedicated study sessions. These tips can help:
- Take effective notes when listening to lectures or reading course materials Taking effective notes is extremely helpful for understanding course material and completing assignments—and there is a definite strategy for doing so. First, be selective and pay attention to the concepts that your instructor indicates are important—and look for section headings or summaries in your readings that highlight essential material. Then, once you’ve identified major concepts, rewrite them in your own words to help make them more understandable and memorable. Finally, go back and recap each idea in one or two sentences to streamline and highlight key takeaways.
- Create mind maps A mind map is a diagram that represents how various ideas relate to the main concept. Using mind maps can be an effective way of organizing and summarizing key concepts, particularly if you’re a visual learner. Start by writing the central concept in the middle of your page and then draw curved lines branching out with related ideas. Then, you can add branches to the related ideas with further points. You can also use different colors, pictures, and line lengths/widths to make your maps more memorable or highlight the most important concepts.
- Break up study sessions While some long study sessions can’t be avoided, if you can break up your study time into shorter increments, you will be doing yourself a favor. That’s because your brain needs these breaks to process and store the information that you learn. And stopping to grab a snack, listen to a podcast, or grab coffee with a friend can help you relax, improve your mood, and allow you to focus better once you return to studying.
5. Develop your patience and resilience
In a world where our attention spans seem to be getting shorter and instant gratification is often celebrated, it can be difficult to cultivate patience. But if you want to be successful in reaching your academic goals, you’re going to have to learn how. Practices that can be helpful to overcome impatience include being more mindful of your reactions to your environment, calming your mind by practicing deep breathing or meditation, and rewarding yourself when you reach set milestones.
Resilience refers to your ability to adapt to and overcome life’s challenges. If you’re interested in learning more about how to become more resilient you should look into APOP 1200: Human Flourishing: Strengths and Resilience at Penn LPS Online. This course explores the science of positive psychology and how we can leverage our strengths to contribute to the greater world and enhance our personal well-being. You will also learn about the physical and psychological protective principles and factors that comprise resilience and how they can be nurtured to help you flourish in your academics, career, and personal life.
6. Take care of yourself physically and mentally
You can’t expect to meet your goals if you experience burnout. That’s why it’s so important that you take care of your physical and mental health. Some effective ways to take care of your body include eating a well-balanced and nutritious diet and exercising regularly. Remember that being active doesn’t have to involve going to the gym—it can also be taking a dance class, going for a bike ride, or walking your dog around the neighborhood. The endorphins that your body produces when you exercise can help improve your disposition and relax your mind. You should also prioritize sleep to help boost your immune system, reduce stress, and increase your ability to focus on your studies. Did you know getting outdoors can improve your mental health? Not only will you enjoy the fresh air, but exposure to sunlight is also believed to increase the release of serotonin, a hormone associated with improved mood and sense of calm and concentration.
Finally, schedule time just for fun! Whether you engage in your favorite hobby, work on a creative project, or go out to dinner with your partner, when you make the time to do activities that you love, it benefits your well-being and ultimately increases your chances of academic success. And it’s important to note that if you do find yourself feeling overwhelmed or struggling mentally during your studies that reaching out to a professional therapist is always a healthy step to take.
7. Practice positive thinking
People who maintain a positive outlook on life may cope better with stress, have stronger immunity, and experience healthier and happier lives. Consequently, cultivating a positive mindset can be invaluable when it comes to learning. Positive psychology is the scientific study of what helps people to thrive in all aspects of their lives. In APOP 1000: Introduction to Positive Psychology at Penn LPS Online, you will explore the underpinnings of positive psychology, address questions surrounding what it means to be happy, and learn the theory and science that supports the conceptual framework of well-being. With hands-on experiments and group activities, you’ll discover how to build well-being in yourself, in organizations, and in communities. So, when you’re registering for courses, do yourself a favor and consider this one.
Are you ready to achieve academic success?
Congratulations on committing to continuing your education and furthering your personal and professional development. In addition to advancing your academic and career goals, lifelong learning can also help you improve your self-confidence and self-worth, renew your creativity and motivation, build soft skills such as critical thinking and adaptability, and enhance your overall quality of life.
The Ivy League courses , certificates , and degree at Penn LPS Online are designed with adult learners like you in mind, offering the flexibility to obtain new career skills, indulge your unique passions, or earn your liberal arts degree on a schedule that works with your busy life. At Penn LPS Online, you can pursue your academic goals while building a foundation grounded in the arts and sciences and broadening your professional prospects.
View our course guide to see what excites you! Enrollment for certificates and courses and applications for the Bachelor of Applied Arts and Sciences program are always open. Visit our online application and get started today!

Definition Essay

Definition Essay - Writing Guide, Examples and Tips
14 min read
Published on: Oct 9, 2020
Last updated on: Jan 31, 2024
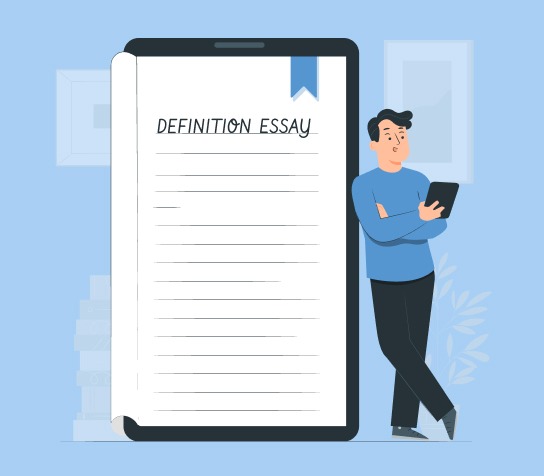
People also read
Interesting Definition Essay Topics for Students
Definition Essay Outline - Format & Guide
Share this article
Many students struggle with writing definition essays due to a lack of clarity and precision in their explanations.
This obstructs them from effectively conveying the essence of the terms or concepts they are tasked with defining. Consequently, the essays may lack coherence, leaving readers confused and preventing them from grasping the intended meaning.
But don’t worry!
In this guide, we will delve into effective techniques and step-by-step approaches to help students craft an engaging definition essay.
Continue reading to learn the correct formation of a definition essay.
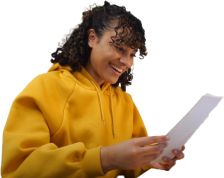
Paper Due? Why Suffer? That's our Job!
On This Page On This Page -->
What is a Definition Essay?
Just as the name suggests, a definition essay defines and explains a term or a concept. Unlike a narrative essay, the purpose of writing this essay is only to inform the readers.
Writing this essay type can be deceivingly tricky. Some terms, concepts, and objects have concrete definitions when explained. In contrast others are solely based on the writerâs understanding and point of view.
A definition essay requires a writer to use different approaches when discussing a term. These approaches are the following:
- Denotation - It is when you provide a literal or academic definition of the term.
- Connotation - It is when the writer provides an implied meaning or definition of the term.
- Enumeration - For this approach, a list is employed to define a term or a concept.
- Analogy - It is a technique in which something is defined by implementing a comparison.
- Negation - It is when you define a term by stating what it is not.
A single or combination of approaches can be used in the essay.
Definition Essay Types
There are several types of definition essays that you may be asked to write, depending on the purpose and scope of the assignment.
In this section, we will discuss some of the most common types of definition essays.
Descriptive Definition Essay
This type of essay provides a detailed description of a term or concept, emphasizing its key features and characteristics.
The goal of a descriptive definition essay is to help readers understand the term or concept in a more profound way.
Stipulative Definition Essay
In a stipulative definition essay, the writer provides a unique definition of a term or concept. This type of essay is often used in academic settings to define a term in a particular field of study.
The goal of a stipulative definition essay is to provide a precise and clear definition that is specific to the context of the essay.
Analytical Definition Essay
This compare and contrast essay type involves analyzing a term or concept in-depth. Breaking it down into its component parts, and examining how they relate to each other.
The goal of an analytical definition essay is to provide a more nuanced and detailed understanding of the term or concept being discussed.
Persuasive Definition Essay
A persuasive definition essay is an argumentative essay that aims to persuade readers to accept a particular definition of a term or concept.
The writer presents their argument for the definition and uses evidence and examples to support their position.
Explanatory Definition Essay
An explanatory definition essay is a type of expository essay . It aims to explain a complex term or concept in a way that is easy to understand for the reader.
The writer breaks down the term or concept into simpler parts and provides examples and analogies to help readers understand it better.
Extended Definition Essay
An extended definition essay goes beyond the definition of a word or concept and provides a more in-depth analysis and explanation.
The goal of an extended definition essay is to provide a comprehensive understanding of a term, concept, or idea. This includes its history, origins, and cultural significance.
How to Write a Definition Essay?
Writing a definition essay is simple if you know the correct procedure. This essay, like all the other formal pieces of documents, requires substantial planning and effective execution.
The following are the steps involved in writing a definition essay effectively:
Instead of choosing a term that has a concrete definition available, choose a word that is complicated . Complex expressions have abstract concepts that require a writer to explore deeper. Moreover, make sure that different people perceive the term selected differently.
Once you have a word to draft your definition essay for, read the dictionary. These academic definitions are important as you can use them to compare your understanding with the official concept.
Drafting a definition essay is about stating the dictionary meaning and your explanation of the concept. So the writer needs to have some information about the term.
In addition to this, when exploring the term, make sure to check the termâs origin. The history of the word can make you discuss it in a better way.
Coming up with an exciting title for your essay is important. The essay topic will be the first thing that your readers will witness, so it should be catchy.
Creatively draft an essay topic that reflects meaning. In addition to this, the usage of the term in the title should be correctly done. The readers should get an idea of what the essay is about and what to expect from the document.
Now that you have a topic in hand, it is time to gather some relevant information. A definition essay is more than a mere explanation of the term. It represents the writerâs perception of the chosen term and the topic.
So having only personal opinions will not be enough to defend your point. Deeply research and gather information by consulting credible sources.
The gathered information needs to be organized to be understandable. The raw data needs to be arranged to give a structure to the content.
Here's a generic outline for a definition essay:
Are you searching for an in-depth guide on crafting a well-structured definition essay?Check out this definition essay outline blog!
6. Write the First Draft
Drafting each section correctly is a daunting task. Understanding what or what not to include in these sections requires a writer to choose wisely.
The start of your essay matters a lot. If it is on point and attractive, the readers will want to read the text. As the first part of the essay is the introduction , it is considered the first impression of your essay.
To write your definition essay introduction effectively, include the following information:
- Start your essay with a catchy hook statement that is related to the topic and the term chosen.
- State the generally known definition of the term. If the word chosen has multiple interpretations, select the most common one.
- Provide background information precisely. Determine the origin of the term and other relevant information.
- Shed light on the other unconventional concepts and definitions related to the term.
- Decide on the side or stance you want to pick in your essay and develop a thesis statement .
After briefly introducing the topic, fully explain the concept in the body section . Provide all the details and evidence that will support the thesis statement. To draft this section professionally, add the following information:
- A detailed explanation of the history of the term.
- Analysis of the dictionary meaning and usage of the term.
- A comparison and reflection of personal understanding and the researched data on the concept.
Once all the details are shared, give closure to your discussion. The last paragraph of the definition essay is the conclusion . The writer provides insight into the topic as a conclusion.
The concluding paragraphs include the following material:
- Summary of the important points.
- Restated thesis statement.
- A final verdict on the topic.
7. Proofread and Edit
Although the writing process ends with the concluding paragraph, there is an additional step. It is important to proofread the essay once you are done writing. Proofread and revise your document a couple of times to make sure everything is perfect.
Before submitting your assignment, make edits, and fix all mistakes and errors.
If you want to learn more about how to write a definition essay, here is a video guide for you!
Definition Essay Structure
The structure of a definition essay is similar to that of any other academic essay. It should consist of an introduction, body paragraphs, and a conclusion.
However, the focus of a definition essay is on defining and explaining a particular term or concept.
In this section, we will discuss the structure of a definition essay in detail.
Introduction
Get the idea of writing an introduction for a definition essay with this example:
Body Paragraphs
Here is an example of how to craft your definition essay body paragraph:
Types of the Term/Concept
If applicable, the writer may want to include a section that discusses the different types or categories of the term or concept being defined.
This section should explain the similarities and differences between the types, using examples and anecdotes to illustrate the points.
Examples of the Term/Concept in Action
The writer should also include real-life examples of the term or concept being defined in action.
This will help the reader better understand the term or concept in context and how it is used in everyday life.
Conclusion
This example will help you writing a conclusion fo you essay:
Definition Essay Examples
It is important to go through some examples and samples before writing an essay. This is to understand the writing process and structure of the assigned task well.
Following are some examples of definition essays to give our students a better idea of the concept.
Understanding the Definition Essay
Definition Essay Example
Definition Essay About Friendship
Definition Essay About Love
Family Definition Essay
Success Definition Essay
Beauty Definition Essay
Definition Essay Topics
Selecting the right topic is challenging for other essay types. However, picking a suitable theme for a definition essay is equally tricky yet important. Pick an interesting subject to ensure maximum readership.
If you are facing writerâs block, here is a list of some great definition essay topics for your help. Choose from the list below and draft a compelling essay.
- Authenticity
- Sustainability
- Mindfulness
Here are some more extended definition essay topics:
- Social media addiction
- Ethical implications of gene editing
- Personalized learning in the digital age
- Ecosystem services
- Cultural assimilation versus cultural preservation
- Sustainable fashion
- Gender equality in the workplace
- Financial literacy and its impact on personal finance
- Ethical considerations in artificial intelligence
- Welfare state and social safety nets
Need more topics? Check out this definition essay topics blog!
Definition Essay Writing Tips
Knowing the correct writing procedure is not enough if you are not aware of the essayâs small technicalities. To help students write a definition essay effortlessly, expert writers of CollegeEssay.org have gathered some simple tips.
These easy tips will make your assignment writing phase easy.
- Choose an exciting yet informative topic for your essay.
- When selecting the word, concept, or term for your essay, make sure you have the knowledge.
- When consulting a dictionary for the definition, provide proper referencing as there are many choices available.
- To make the essay informative and credible, always provide the origin and history of the term.
- Highlight different meanings and interpretations of the term.
- Discuss the transitions and evolution in the meaning of the term in any.
- Provide your perspective and point of view on the chosen term.
Following these tips will guarantee you better grades in your academics.
By following the step-by-step approach explained in this guide, you will acquire the skills to craft an outstanding essay.
Struggling with the thought, " write my college essay for m e"? Look no further.
Our dedicated definition essay writing service is here to craft the perfect essay that meets your academic needs.
For an extra edge, explore our AI essay writer , a tool designed to refine your essays to perfection.
Barbara P (Literature, Marketing)
Barbara is a highly educated and qualified author with a Ph.D. in public health from an Ivy League university. She has spent a significant amount of time working in the medical field, conducting a thorough study on a variety of health issues. Her work has been published in several major publications.
Paper Due? Why Suffer? That’s our Job!
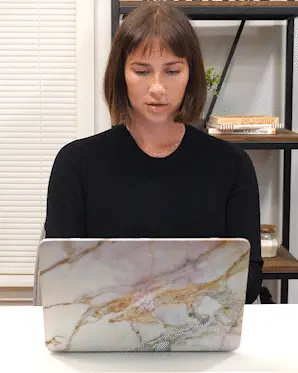
Keep reading
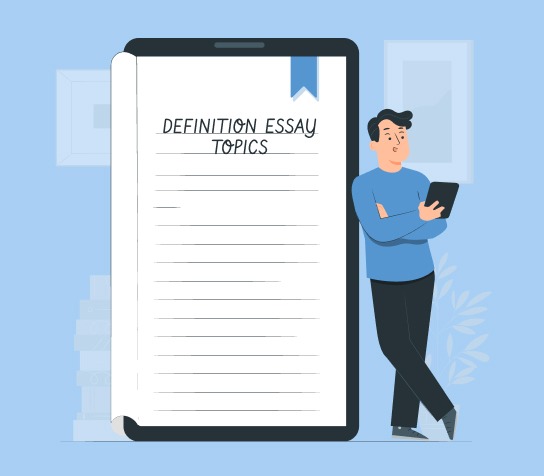
- Privacy Policy
- Cookies Policy
- Terms of Use
- Refunds & Cancellations
- Our Writers
- Success Stories
- Our Guarantees
- Affiliate Program
- Referral Program
- AI Essay Writer
Disclaimer: All client orders are completed by our team of highly qualified human writers. The essays and papers provided by us are not to be used for submission but rather as learning models only.
What Is Success?
- Posted September 5, 2014
- By Mark Russell
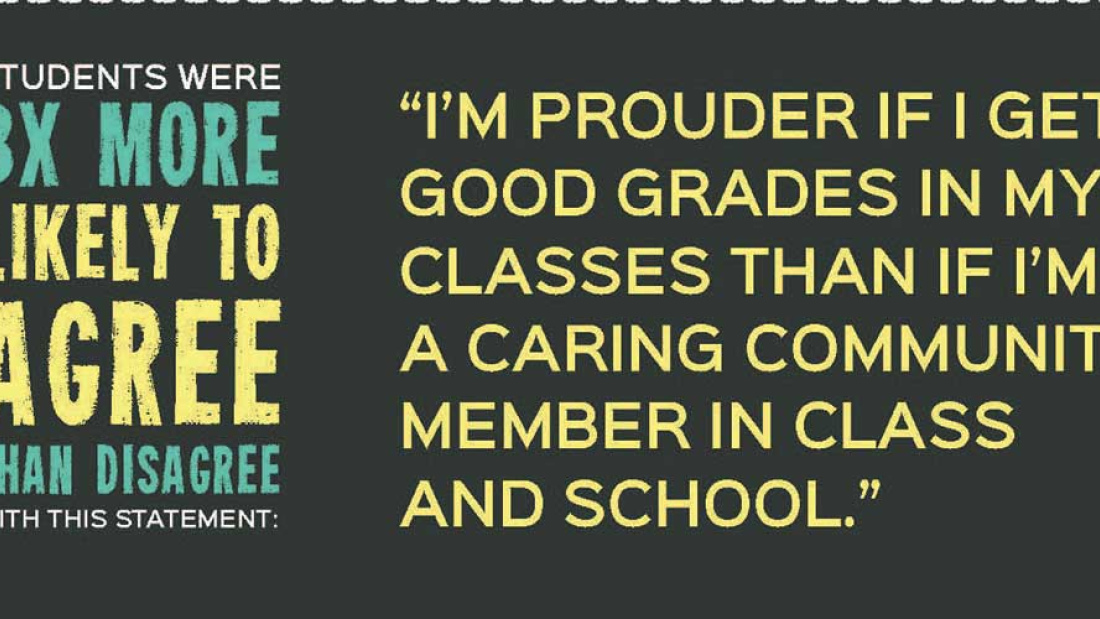
A large majority of youth appear to prioritize aspects of success — achievement and happiness — above caring for others.
This is one highlight of a report released in June by the Ed School’s Making Caring Common Project ( @MCCHarvardEd ) . The report is based chiefly on a survey of nearly 10,000 diverse middle and high school students that the project conducted in 2013. The report also points to a troubling gap between what adults say and what students perceive about the importance of caring, kindness, and respect: Adults claim to prioritize caring, but that’s not the message that teens are hearing.
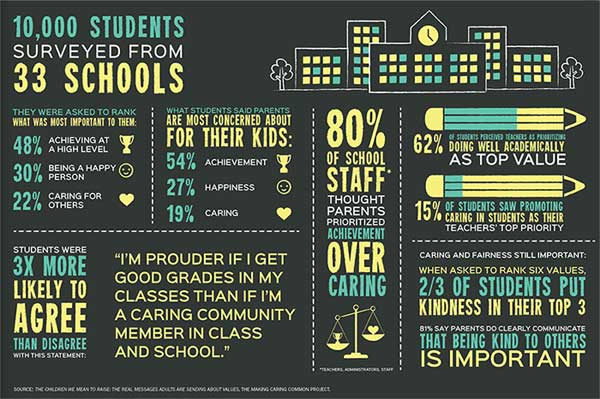
“Our founding fathers envisioned a society that balanced success and happiness with the common good,” says Rick Weissbourd , Ed.D.’87, codirector of the project and a senior lecturer at the Ed School. “We now seem to have lost that balance. And the irony is that the intense focus on happiness doesn’t appear to be making children happier.”
Weissbourd said the good news is that the survey clearly shows that caring is still valued by the students they surveyed.
“Our challenge is to help parents and educators show that they prioritize caring and goodness and give our youth an opportunity to build their moral muscles,” Weissbourd says. “We’ve drawn on our knowledge of moral development and social emotional learning to develop clear strategies designed to do just that.”
Some of the strategies the report suggests include conducting a survey of the school climate, cultivating student leadership to address troubling aspects of the school’s climate, building student’s capacity for empathy and self-regulation and their commitment to the common good, and ensuring that all students have a positive relationship with an adult mentor. — Mark Russell is the project’s media and programming director.

Ed. Magazine
The magazine of the Harvard Graduate School of Education
Related Articles

Cultivating Empathy in the Coronavirus Crisis
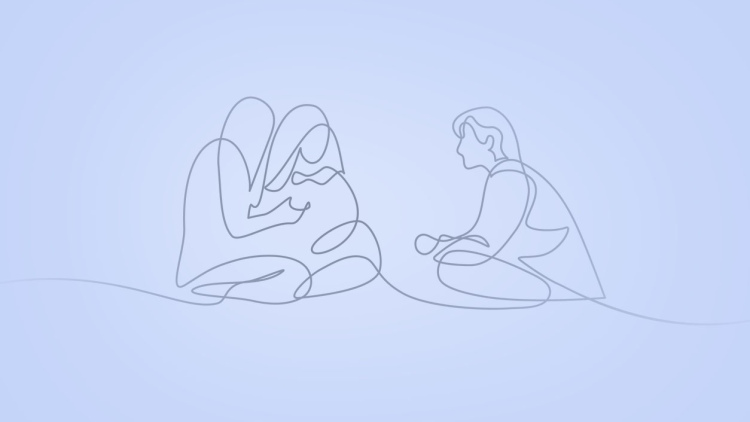
Think Your Students Don't Care About Others?
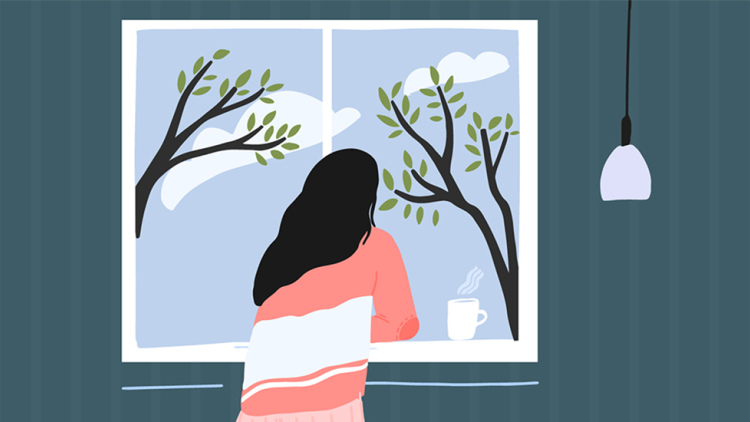
Combatting an Epidemic of Loneliness
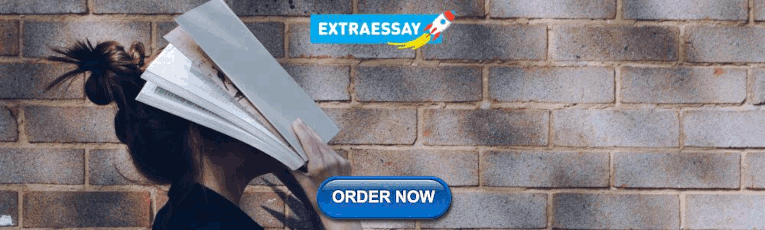
IMAGES
VIDEO
COMMENTS
personal validation. self-efficacy. sense of purpose. active involvement. reflective thinking. social integration, and. self-awareness. What follows is an attempt to clearly define these key principles and to highlight the empirical evidence supporting their positive impact on student success. 1.
Rosemary Stock. Researchers examining academic success often quantify it in terms of assessment grades. This assumption is questioned here by considering the students' understanding of academic success. The presented study aimed to identify students' views of its definition and the factors they perceive as crucial in attaining it.
success based upon the literature as: …student success is defined as academic achievement, engagement in educationally purposeful activities, satisfaction, acquisition of desired knowledge ...
Class participation and behavior are another aspect of the definition of a good student. Simply attending class is not enough; good students arrive punctually because they understand that tardiness disrupts the class and disrespects the professors. They might occasionally arrive a few minutes early to ask the professor questions about class ...
Within the field of education, "academic success" is a widely used construct that is equated to "student achievement" and often linked to students' performance on standardized test scores. This narrowed definition of academic success justifies the school failure of Black students and students learning English and shifts the blame to ...
Volume 20, Number 5, March 2015 ISSN 1531-7714 Defining and Measuring Academic Success Travis T. York, Valdosta State University Charles Gibson & Susan Rankin, The Pennsylvania State University Despite, and perhaps because of its amorphous nature, the term 'academic success' is one of the most widely used constructs in educational research ...
Academic success, while often quantified by grades and accolades, is a multi-faceted concept that transcends mere numerical achievements. It's about the journey of learning, the skills honed, and the knowledge acquired. As the world continues to evolve, the skills and attributes that define academic success might shift.
Practical Assessment, Research & Evaluation, Vol 20, No 5. Page 2 York, Gibson & Rankin, Academic Success. current amorphous construction of academic success and the necessary application of the term within the contexts of departmental goals for students. This broad application of the term limits the ability of educators and administrators to ...
Definition No. of papers using Representative quote; ... academic success). Thus, the problem becomes apparent—if most researchers define success in similar ways, and these definitions are validated by achieving intended career outcomes, then these definitions are taken for granted and seen as common sense. Therefore, researchers may not see ...
Academic success is a goal that all college students strive to achieve throughout their educational journey. ... Success Definition Essay Outline Introduction Defining success in various ways Emphasizing the broader aspects of success beyond materialism The importance of considering life's impact and satisfaction Success is Completeness ...
The examination of academic achievements is common in educational research literature, with most studies referring to grades (marks) as measures of success. In addition, outside the realm of research, a student's grades are usually the main criteria for admission to education programmes, nomination for honours (passing above ordinary level), award of scholarships and so forth. However ...
Academic Success Definition Essay 1147 Words | 5 Pages "Academic success," for me, doesn't mean graduating high school with a 4.0 GPA and attending an Ivy League college with a ton of scholarships. Personally, the term "academic success" means being able to feel proud of my own dedication and the hard work that I put in.
Here's what you need to include: Start with something attention-grabbing, like a thought-provoking question or an interesting fact. Provide a brief overview of the topic and why it's important to define it. Clearly state the term you're defining and your interpretation of it. Definition Essay Body Paragraphs.
Key Elements of a Definition Essay on Success. The structure of a definition essay is similar to that of other academic essays. A good essay always starts with an introduction, followed by body paragraphs, and ends with a conclusion. However, unlike other types of essays, a definition essay focuses on explaining a particular concept or term.
Student success plays a vital role in educational institutions, as it is often used as a metric for the institution's performance. Early detection of students at risk, along with preventive measures, can drastically improve their success. Lately, machine learning techniques have been extensively used for prediction purpose. While there is a plethora of success stories in the literature ...
Phillip J. Moore. Academic achievement was once thought to be the most important outcome of formal educational experiences and while there is little doubt as to the vital role such achievements play in student life and later (Kell, Lubinski, & Benbow, 2013 ), researchers and policy makers are ever increasingly turning to social and emotional ...
What is success? A quick look in a dictionary might define it as the attainment of wealth, favor, or eminence. Yet, to different individuals and cultures worldwide, success carries varied meanings, often transcending the typical markers of bank balances, accolades, or societal recognition. Let's delve into how different cultures and ...
1147 Words5 Pages. "Academic success," for me, doesn't mean graduating high school with a 4.0 GPA and attending an Ivy League college with a ton of scholarships. Personally, the term "academic success" means being able to feel proud of my own dedication and the hard work that I put in. Having the end result ultimately be, walking ...
Academic Success Essay. Academic success could never be fully measured by degrees alone, but it can include a person's well-being as well as their mind and certain characteristics about a person that make them unique. This success can be achieved in different ways by these different people with different strengths, but there is the same ...
1. Work on your time management skills. One of the most critical skills for academic success is effective management of your study time. Time management skills are essential because they help ensure you stay on top of all your readings, projects, and exams. Managing your time well also requires you to stay organized, prioritize your tasks, and ...
It means that writing a definition essay you should use your arguments clearly to prevent your audience from a wrong understanding of a problem. In simple terms, it tells people what your issue is not. Origins & Causes. This is where you tell about the origins of your issue or things that have caused some event or changes.
An explanatory definition essay is a type of expository essay. It aims to explain a complex term or concept in a way that is easy to understand for the reader. The writer breaks down the term or concept into simpler parts and provides examples and analogies to help readers understand it better.
A large majority of youth appear to prioritize aspects of success — achievement and happiness — above caring for others. This is one highlight of a report released in June by the Ed School's Making Caring Common Project (@MCCHarvardEd).The report is based chiefly on a survey of nearly 10,000 diverse middle and high school students that the project conducted in 2013.