When you choose to publish with PLOS, your research makes an impact. Make your work accessible to all, without restrictions, and accelerate scientific discovery with options like preprints and published peer review that make your work more Open.
- PLOS Biology
- PLOS Climate
- PLOS Complex Systems
- PLOS Computational Biology
- PLOS Digital Health
- PLOS Genetics
- PLOS Global Public Health
- PLOS Medicine
- PLOS Mental Health
- PLOS Neglected Tropical Diseases
- PLOS Pathogens
- PLOS Sustainability and Transformation
- PLOS Collections
- How to Write Your Methods

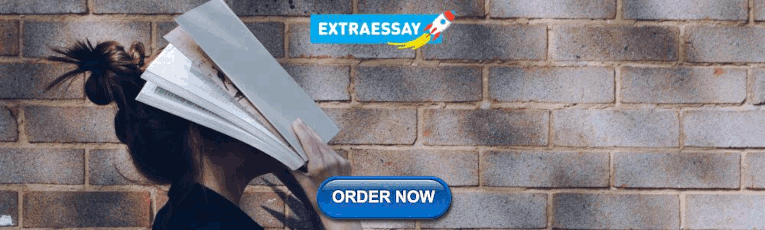
Ensure understanding, reproducibility and replicability
What should you include in your methods section, and how much detail is appropriate?
Why Methods Matter
The methods section was once the most likely part of a paper to be unfairly abbreviated, overly summarized, or even relegated to hard-to-find sections of a publisher’s website. While some journals may responsibly include more detailed elements of methods in supplementary sections, the movement for increased reproducibility and rigor in science has reinstated the importance of the methods section. Methods are now viewed as a key element in establishing the credibility of the research being reported, alongside the open availability of data and results.
A clear methods section impacts editorial evaluation and readers’ understanding, and is also the backbone of transparency and replicability.
For example, the Reproducibility Project: Cancer Biology project set out in 2013 to replicate experiments from 50 high profile cancer papers, but revised their target to 18 papers once they understood how much methodological detail was not contained in the original papers.

What to include in your methods section
What you include in your methods sections depends on what field you are in and what experiments you are performing. However, the general principle in place at the majority of journals is summarized well by the guidelines at PLOS ONE : “The Materials and Methods section should provide enough detail to allow suitably skilled investigators to fully replicate your study. ” The emphases here are deliberate: the methods should enable readers to understand your paper, and replicate your study. However, there is no need to go into the level of detail that a lay-person would require—the focus is on the reader who is also trained in your field, with the suitable skills and knowledge to attempt a replication.
A constant principle of rigorous science
A methods section that enables other researchers to understand and replicate your results is a constant principle of rigorous, transparent, and Open Science. Aim to be thorough, even if a particular journal doesn’t require the same level of detail . Reproducibility is all of our responsibility. You cannot create any problems by exceeding a minimum standard of information. If a journal still has word-limits—either for the overall article or specific sections—and requires some methodological details to be in a supplemental section, that is OK as long as the extra details are searchable and findable .
Imagine replicating your own work, years in the future
As part of PLOS’ presentation on Reproducibility and Open Publishing (part of UCSF’s Reproducibility Series ) we recommend planning the level of detail in your methods section by imagining you are writing for your future self, replicating your own work. When you consider that you might be at a different institution, with different account logins, applications, resources, and access levels—you can help yourself imagine the level of specificity that you yourself would require to redo the exact experiment. Consider:
- Which details would you need to be reminded of?
- Which cell line, or antibody, or software, or reagent did you use, and does it have a Research Resource ID (RRID) that you can cite?
- Which version of a questionnaire did you use in your survey?
- Exactly which visual stimulus did you show participants, and is it publicly available?
- What participants did you decide to exclude?
- What process did you adjust, during your work?
Tip: Be sure to capture any changes to your protocols
You yourself would want to know about any adjustments, if you ever replicate the work, so you can surmise that anyone else would want to as well. Even if a necessary adjustment you made was not ideal, transparency is the key to ensuring this is not regarded as an issue in the future. It is far better to transparently convey any non-optimal methods, or methodological constraints, than to conceal them, which could result in reproducibility or ethical issues downstream.
Visual aids for methods help when reading the whole paper
Consider whether a visual representation of your methods could be appropriate or aid understanding your process. A visual reference readers can easily return to, like a flow-diagram, decision-tree, or checklist, can help readers to better understand the complete article, not just the methods section.
Ethical Considerations
In addition to describing what you did, it is just as important to assure readers that you also followed all relevant ethical guidelines when conducting your research. While ethical standards and reporting guidelines are often presented in a separate section of a paper, ensure that your methods and protocols actually follow these guidelines. Read more about ethics .
Existing standards, checklists, guidelines, partners
While the level of detail contained in a methods section should be guided by the universal principles of rigorous science outlined above, various disciplines, fields, and projects have worked hard to design and develop consistent standards, guidelines, and tools to help with reporting all types of experiment. Below, you’ll find some of the key initiatives. Ensure you read the submission guidelines for the specific journal you are submitting to, in order to discover any further journal- or field-specific policies to follow, or initiatives/tools to utilize.
Tip: Keep your paper moving forward by providing the proper paperwork up front
Be sure to check the journal guidelines and provide the necessary documents with your manuscript submission. Collecting the necessary documentation can greatly slow the first round of peer review, or cause delays when you submit your revision.
Randomized Controlled Trials – CONSORT The Consolidated Standards of Reporting Trials (CONSORT) project covers various initiatives intended to prevent the problems of inadequate reporting of randomized controlled trials. The primary initiative is an evidence-based minimum set of recommendations for reporting randomized trials known as the CONSORT Statement .
Systematic Reviews and Meta-Analyses – PRISMA The Preferred Reporting Items for Systematic Reviews and Meta-Analyses ( PRISMA ) is an evidence-based minimum set of items focusing on the reporting of reviews evaluating randomized trials and other types of research.
Research using Animals – ARRIVE The Animal Research: Reporting of In Vivo Experiments ( ARRIVE ) guidelines encourage maximizing the information reported in research using animals thereby minimizing unnecessary studies. (Original study and proposal , and updated guidelines , in PLOS Biology .)
Laboratory Protocols Protocols.io has developed a platform specifically for the sharing and updating of laboratory protocols , which are assigned their own DOI and can be linked from methods sections of papers to enhance reproducibility. Contextualize your protocol and improve discovery with an accompanying Lab Protocol article in PLOS ONE .
Consistent reporting of Materials, Design, and Analysis – the MDAR checklist A cross-publisher group of editors and experts have developed, tested, and rolled out a checklist to help establish and harmonize reporting standards in the Life Sciences . The checklist , which is available for use by authors to compile their methods, and editors/reviewers to check methods, establishes a minimum set of requirements in transparent reporting and is adaptable to any discipline within the Life Sciences, by covering a breadth of potentially relevant methodological items and considerations. If you are in the Life Sciences and writing up your methods section, try working through the MDAR checklist and see whether it helps you include all relevant details into your methods, and whether it reminded you of anything you might have missed otherwise.
Summary Writing tips
The main challenge you may find when writing your methods is keeping it readable AND covering all the details needed for reproducibility and replicability. While this is difficult, do not compromise on rigorous standards for credibility!
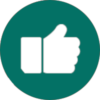
- Keep in mind future replicability, alongside understanding and readability.
- Follow checklists, and field- and journal-specific guidelines.
- Consider a commitment to rigorous and transparent science a personal responsibility, and not just adhering to journal guidelines.
- Establish whether there are persistent identifiers for any research resources you use that can be specifically cited in your methods section.
- Deposit your laboratory protocols in Protocols.io, establishing a permanent link to them. You can update your protocols later if you improve on them, as can future scientists who follow your protocols.
- Consider visual aids like flow-diagrams, lists, to help with reading other sections of the paper.
- Be specific about all decisions made during the experiments that someone reproducing your work would need to know.

Don’t
- Summarize or abbreviate methods without giving full details in a discoverable supplemental section.
- Presume you will always be able to remember how you performed the experiments, or have access to private or institutional notebooks and resources.
- Attempt to hide constraints or non-optimal decisions you had to make–transparency is the key to ensuring the credibility of your research.
- How to Write a Great Title
- How to Write an Abstract
- How to Report Statistics
- How to Write Discussions and Conclusions
- How to Edit Your Work
The contents of the Peer Review Center are also available as a live, interactive training session, complete with slides, talking points, and activities. …
The contents of the Writing Center are also available as a live, interactive training session, complete with slides, talking points, and activities. …
There’s a lot to consider when deciding where to submit your work. Learn how to choose a journal that will help your study reach its audience, while reflecting your values as a researcher…
- USC Libraries
- Research Guides
Organizing Your Social Sciences Research Paper
- 6. The Methodology
- Purpose of Guide
- Design Flaws to Avoid
- Independent and Dependent Variables
- Glossary of Research Terms
- Reading Research Effectively
- Narrowing a Topic Idea
- Broadening a Topic Idea
- Extending the Timeliness of a Topic Idea
- Academic Writing Style
- Choosing a Title
- Making an Outline
- Paragraph Development
- Research Process Video Series
- Executive Summary
- The C.A.R.S. Model
- Background Information
- The Research Problem/Question
- Theoretical Framework
- Citation Tracking
- Content Alert Services
- Evaluating Sources
- Primary Sources
- Secondary Sources
- Tiertiary Sources
- Scholarly vs. Popular Publications
- Qualitative Methods
- Quantitative Methods
- Insiderness
- Using Non-Textual Elements
- Limitations of the Study
- Common Grammar Mistakes
- Writing Concisely
- Avoiding Plagiarism
- Footnotes or Endnotes?
- Further Readings
- Generative AI and Writing
- USC Libraries Tutorials and Other Guides
- Bibliography
The methods section describes actions taken to investigate a research problem and the rationale for the application of specific procedures or techniques used to identify, select, process, and analyze information applied to understanding the problem, thereby, allowing the reader to critically evaluate a study’s overall validity and reliability. The methodology section of a research paper answers two main questions: How was the data collected or generated? And, how was it analyzed? The writing should be direct and precise and always written in the past tense.
Kallet, Richard H. "How to Write the Methods Section of a Research Paper." Respiratory Care 49 (October 2004): 1229-1232.
Importance of a Good Methodology Section
You must explain how you obtained and analyzed your results for the following reasons:
- Readers need to know how the data was obtained because the method you chose affects the results and, by extension, how you interpreted their significance in the discussion section of your paper.
- Methodology is crucial for any branch of scholarship because an unreliable method produces unreliable results and, as a consequence, undermines the value of your analysis of the findings.
- In most cases, there are a variety of different methods you can choose to investigate a research problem. The methodology section of your paper should clearly articulate the reasons why you have chosen a particular procedure or technique.
- The reader wants to know that the data was collected or generated in a way that is consistent with accepted practice in the field of study. For example, if you are using a multiple choice questionnaire, readers need to know that it offered your respondents a reasonable range of answers to choose from.
- The method must be appropriate to fulfilling the overall aims of the study. For example, you need to ensure that you have a large enough sample size to be able to generalize and make recommendations based upon the findings.
- The methodology should discuss the problems that were anticipated and the steps you took to prevent them from occurring. For any problems that do arise, you must describe the ways in which they were minimized or why these problems do not impact in any meaningful way your interpretation of the findings.
- In the social and behavioral sciences, it is important to always provide sufficient information to allow other researchers to adopt or replicate your methodology. This information is particularly important when a new method has been developed or an innovative use of an existing method is utilized.
Bem, Daryl J. Writing the Empirical Journal Article. Psychology Writing Center. University of Washington; Denscombe, Martyn. The Good Research Guide: For Small-Scale Social Research Projects . 5th edition. Buckingham, UK: Open University Press, 2014; Lunenburg, Frederick C. Writing a Successful Thesis or Dissertation: Tips and Strategies for Students in the Social and Behavioral Sciences . Thousand Oaks, CA: Corwin Press, 2008.
Structure and Writing Style
I. Groups of Research Methods
There are two main groups of research methods in the social sciences:
- The e mpirical-analytical group approaches the study of social sciences in a similar manner that researchers study the natural sciences . This type of research focuses on objective knowledge, research questions that can be answered yes or no, and operational definitions of variables to be measured. The empirical-analytical group employs deductive reasoning that uses existing theory as a foundation for formulating hypotheses that need to be tested. This approach is focused on explanation.
- The i nterpretative group of methods is focused on understanding phenomenon in a comprehensive, holistic way . Interpretive methods focus on analytically disclosing the meaning-making practices of human subjects [the why, how, or by what means people do what they do], while showing how those practices arrange so that it can be used to generate observable outcomes. Interpretive methods allow you to recognize your connection to the phenomena under investigation. However, the interpretative group requires careful examination of variables because it focuses more on subjective knowledge.
II. Content
The introduction to your methodology section should begin by restating the research problem and underlying assumptions underpinning your study. This is followed by situating the methods you used to gather, analyze, and process information within the overall “tradition” of your field of study and within the particular research design you have chosen to study the problem. If the method you choose lies outside of the tradition of your field [i.e., your review of the literature demonstrates that the method is not commonly used], provide a justification for how your choice of methods specifically addresses the research problem in ways that have not been utilized in prior studies.
The remainder of your methodology section should describe the following:
- Decisions made in selecting the data you have analyzed or, in the case of qualitative research, the subjects and research setting you have examined,
- Tools and methods used to identify and collect information, and how you identified relevant variables,
- The ways in which you processed the data and the procedures you used to analyze that data, and
- The specific research tools or strategies that you utilized to study the underlying hypothesis and research questions.
In addition, an effectively written methodology section should:
- Introduce the overall methodological approach for investigating your research problem . Is your study qualitative or quantitative or a combination of both (mixed method)? Are you going to take a special approach, such as action research, or a more neutral stance?
- Indicate how the approach fits the overall research design . Your methods for gathering data should have a clear connection to your research problem. In other words, make sure that your methods will actually address the problem. One of the most common deficiencies found in research papers is that the proposed methodology is not suitable to achieving the stated objective of your paper.
- Describe the specific methods of data collection you are going to use , such as, surveys, interviews, questionnaires, observation, archival research. If you are analyzing existing data, such as a data set or archival documents, describe how it was originally created or gathered and by whom. Also be sure to explain how older data is still relevant to investigating the current research problem.
- Explain how you intend to analyze your results . Will you use statistical analysis? Will you use specific theoretical perspectives to help you analyze a text or explain observed behaviors? Describe how you plan to obtain an accurate assessment of relationships, patterns, trends, distributions, and possible contradictions found in the data.
- Provide background and a rationale for methodologies that are unfamiliar for your readers . Very often in the social sciences, research problems and the methods for investigating them require more explanation/rationale than widely accepted rules governing the natural and physical sciences. Be clear and concise in your explanation.
- Provide a justification for subject selection and sampling procedure . For instance, if you propose to conduct interviews, how do you intend to select the sample population? If you are analyzing texts, which texts have you chosen, and why? If you are using statistics, why is this set of data being used? If other data sources exist, explain why the data you chose is most appropriate to addressing the research problem.
- Provide a justification for case study selection . A common method of analyzing research problems in the social sciences is to analyze specific cases. These can be a person, place, event, phenomenon, or other type of subject of analysis that are either examined as a singular topic of in-depth investigation or multiple topics of investigation studied for the purpose of comparing or contrasting findings. In either method, you should explain why a case or cases were chosen and how they specifically relate to the research problem.
- Describe potential limitations . Are there any practical limitations that could affect your data collection? How will you attempt to control for potential confounding variables and errors? If your methodology may lead to problems you can anticipate, state this openly and show why pursuing this methodology outweighs the risk of these problems cropping up.
NOTE : Once you have written all of the elements of the methods section, subsequent revisions should focus on how to present those elements as clearly and as logically as possibly. The description of how you prepared to study the research problem, how you gathered the data, and the protocol for analyzing the data should be organized chronologically. For clarity, when a large amount of detail must be presented, information should be presented in sub-sections according to topic. If necessary, consider using appendices for raw data.
ANOTHER NOTE : If you are conducting a qualitative analysis of a research problem , the methodology section generally requires a more elaborate description of the methods used as well as an explanation of the processes applied to gathering and analyzing of data than is generally required for studies using quantitative methods. Because you are the primary instrument for generating the data [e.g., through interviews or observations], the process for collecting that data has a significantly greater impact on producing the findings. Therefore, qualitative research requires a more detailed description of the methods used.
YET ANOTHER NOTE : If your study involves interviews, observations, or other qualitative techniques involving human subjects , you may be required to obtain approval from the university's Office for the Protection of Research Subjects before beginning your research. This is not a common procedure for most undergraduate level student research assignments. However, i f your professor states you need approval, you must include a statement in your methods section that you received official endorsement and adequate informed consent from the office and that there was a clear assessment and minimization of risks to participants and to the university. This statement informs the reader that your study was conducted in an ethical and responsible manner. In some cases, the approval notice is included as an appendix to your paper.
III. Problems to Avoid
Irrelevant Detail The methodology section of your paper should be thorough but concise. Do not provide any background information that does not directly help the reader understand why a particular method was chosen, how the data was gathered or obtained, and how the data was analyzed in relation to the research problem [note: analyzed, not interpreted! Save how you interpreted the findings for the discussion section]. With this in mind, the page length of your methods section will generally be less than any other section of your paper except the conclusion.
Unnecessary Explanation of Basic Procedures Remember that you are not writing a how-to guide about a particular method. You should make the assumption that readers possess a basic understanding of how to investigate the research problem on their own and, therefore, you do not have to go into great detail about specific methodological procedures. The focus should be on how you applied a method , not on the mechanics of doing a method. An exception to this rule is if you select an unconventional methodological approach; if this is the case, be sure to explain why this approach was chosen and how it enhances the overall process of discovery.
Problem Blindness It is almost a given that you will encounter problems when collecting or generating your data, or, gaps will exist in existing data or archival materials. Do not ignore these problems or pretend they did not occur. Often, documenting how you overcame obstacles can form an interesting part of the methodology. It demonstrates to the reader that you can provide a cogent rationale for the decisions you made to minimize the impact of any problems that arose.
Literature Review Just as the literature review section of your paper provides an overview of sources you have examined while researching a particular topic, the methodology section should cite any sources that informed your choice and application of a particular method [i.e., the choice of a survey should include any citations to the works you used to help construct the survey].
It’s More than Sources of Information! A description of a research study's method should not be confused with a description of the sources of information. Such a list of sources is useful in and of itself, especially if it is accompanied by an explanation about the selection and use of the sources. The description of the project's methodology complements a list of sources in that it sets forth the organization and interpretation of information emanating from those sources.
Azevedo, L.F. et al. "How to Write a Scientific Paper: Writing the Methods Section." Revista Portuguesa de Pneumologia 17 (2011): 232-238; Blair Lorrie. “Choosing a Methodology.” In Writing a Graduate Thesis or Dissertation , Teaching Writing Series. (Rotterdam: Sense Publishers 2016), pp. 49-72; Butin, Dan W. The Education Dissertation A Guide for Practitioner Scholars . Thousand Oaks, CA: Corwin, 2010; Carter, Susan. Structuring Your Research Thesis . New York: Palgrave Macmillan, 2012; Kallet, Richard H. “How to Write the Methods Section of a Research Paper.” Respiratory Care 49 (October 2004):1229-1232; Lunenburg, Frederick C. Writing a Successful Thesis or Dissertation: Tips and Strategies for Students in the Social and Behavioral Sciences . Thousand Oaks, CA: Corwin Press, 2008. Methods Section. The Writer’s Handbook. Writing Center. University of Wisconsin, Madison; Rudestam, Kjell Erik and Rae R. Newton. “The Method Chapter: Describing Your Research Plan.” In Surviving Your Dissertation: A Comprehensive Guide to Content and Process . (Thousand Oaks, Sage Publications, 2015), pp. 87-115; What is Interpretive Research. Institute of Public and International Affairs, University of Utah; Writing the Experimental Report: Methods, Results, and Discussion. The Writing Lab and The OWL. Purdue University; Methods and Materials. The Structure, Format, Content, and Style of a Journal-Style Scientific Paper. Department of Biology. Bates College.
Writing Tip
Statistical Designs and Tests? Do Not Fear Them!
Don't avoid using a quantitative approach to analyzing your research problem just because you fear the idea of applying statistical designs and tests. A qualitative approach, such as conducting interviews or content analysis of archival texts, can yield exciting new insights about a research problem, but it should not be undertaken simply because you have a disdain for running a simple regression. A well designed quantitative research study can often be accomplished in very clear and direct ways, whereas, a similar study of a qualitative nature usually requires considerable time to analyze large volumes of data and a tremendous burden to create new paths for analysis where previously no path associated with your research problem had existed.
To locate data and statistics, GO HERE .
Another Writing Tip
Knowing the Relationship Between Theories and Methods
There can be multiple meaning associated with the term "theories" and the term "methods" in social sciences research. A helpful way to delineate between them is to understand "theories" as representing different ways of characterizing the social world when you research it and "methods" as representing different ways of generating and analyzing data about that social world. Framed in this way, all empirical social sciences research involves theories and methods, whether they are stated explicitly or not. However, while theories and methods are often related, it is important that, as a researcher, you deliberately separate them in order to avoid your theories playing a disproportionate role in shaping what outcomes your chosen methods produce.
Introspectively engage in an ongoing dialectic between the application of theories and methods to help enable you to use the outcomes from your methods to interrogate and develop new theories, or ways of framing conceptually the research problem. This is how scholarship grows and branches out into new intellectual territory.
Reynolds, R. Larry. Ways of Knowing. Alternative Microeconomics . Part 1, Chapter 3. Boise State University; The Theory-Method Relationship. S-Cool Revision. United Kingdom.
Yet Another Writing Tip
Methods and the Methodology
Do not confuse the terms "methods" and "methodology." As Schneider notes, a method refers to the technical steps taken to do research . Descriptions of methods usually include defining and stating why you have chosen specific techniques to investigate a research problem, followed by an outline of the procedures you used to systematically select, gather, and process the data [remember to always save the interpretation of data for the discussion section of your paper].
The methodology refers to a discussion of the underlying reasoning why particular methods were used . This discussion includes describing the theoretical concepts that inform the choice of methods to be applied, placing the choice of methods within the more general nature of academic work, and reviewing its relevance to examining the research problem. The methodology section also includes a thorough review of the methods other scholars have used to study the topic.
Bryman, Alan. "Of Methods and Methodology." Qualitative Research in Organizations and Management: An International Journal 3 (2008): 159-168; Schneider, Florian. “What's in a Methodology: The Difference between Method, Methodology, and Theory…and How to Get the Balance Right?” PoliticsEastAsia.com. Chinese Department, University of Leiden, Netherlands.
- << Previous: Scholarly vs. Popular Publications
- Next: Qualitative Methods >>
- Last Updated: Mar 21, 2024 9:59 AM
- URL: https://libguides.usc.edu/writingguide
Have a language expert improve your writing
Run a free plagiarism check in 10 minutes, automatically generate references for free.
- Knowledge Base
- Dissertation
- What Is a Research Methodology? | Steps & Tips
What Is a Research Methodology? | Steps & Tips
Published on 25 February 2019 by Shona McCombes . Revised on 10 October 2022.
Your research methodology discusses and explains the data collection and analysis methods you used in your research. A key part of your thesis, dissertation, or research paper, the methodology chapter explains what you did and how you did it, allowing readers to evaluate the reliability and validity of your research.
It should include:
- The type of research you conducted
- How you collected and analysed your data
- Any tools or materials you used in the research
- Why you chose these methods
- Your methodology section should generally be written in the past tense .
- Academic style guides in your field may provide detailed guidelines on what to include for different types of studies.
- Your citation style might provide guidelines for your methodology section (e.g., an APA Style methods section ).
Instantly correct all language mistakes in your text
Be assured that you'll submit flawless writing. Upload your document to correct all your mistakes.
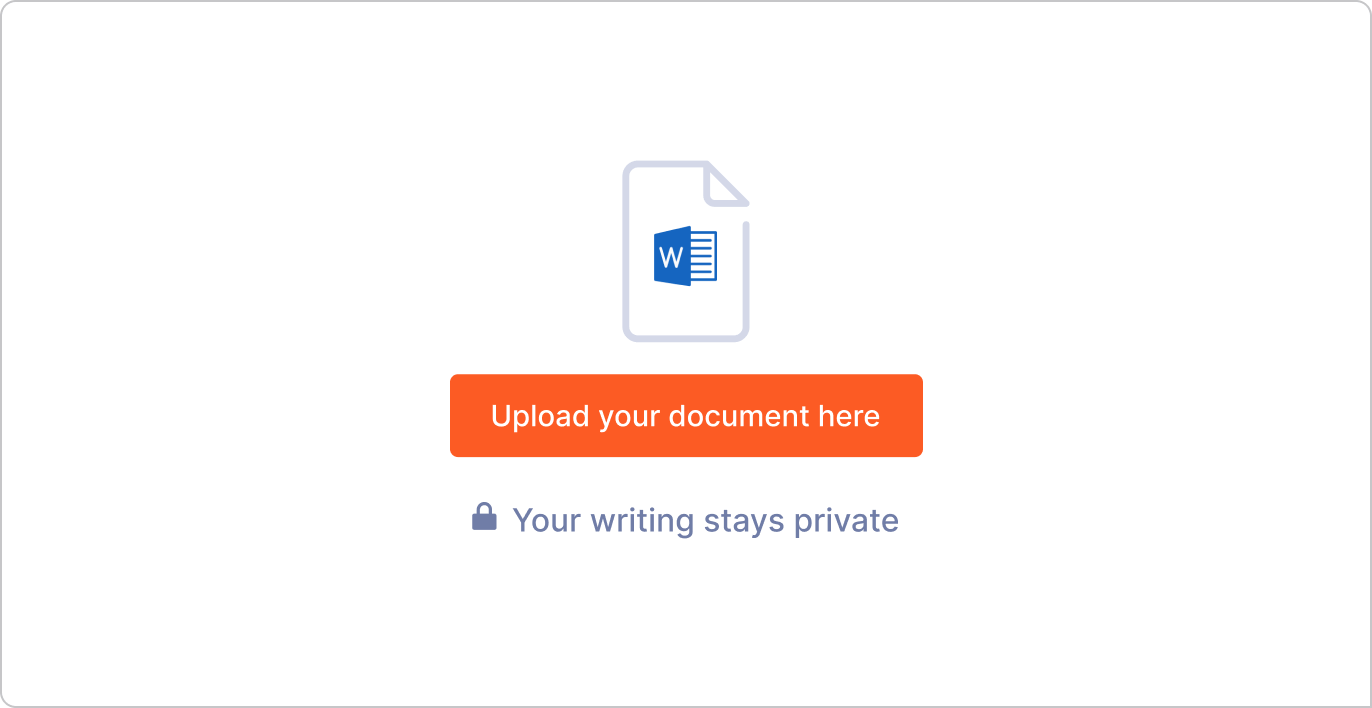
Table of contents
How to write a research methodology, why is a methods section important, step 1: explain your methodological approach, step 2: describe your data collection methods, step 3: describe your analysis method, step 4: evaluate and justify the methodological choices you made, tips for writing a strong methodology chapter, frequently asked questions about methodology.
The only proofreading tool specialized in correcting academic writing
The academic proofreading tool has been trained on 1000s of academic texts and by native English editors. Making it the most accurate and reliable proofreading tool for students.
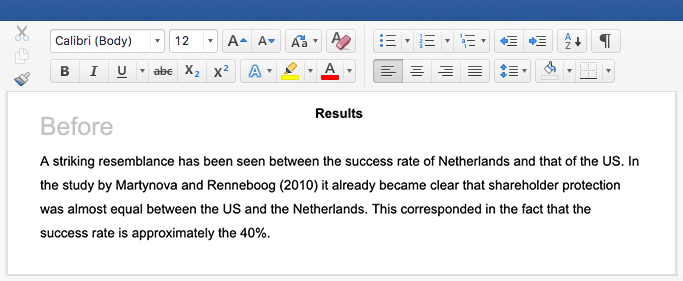
Correct my document today
Your methods section is your opportunity to share how you conducted your research and why you chose the methods you chose. It’s also the place to show that your research was rigorously conducted and can be replicated .
It gives your research legitimacy and situates it within your field, and also gives your readers a place to refer to if they have any questions or critiques in other sections.
You can start by introducing your overall approach to your research. You have two options here.
Option 1: Start with your “what”
What research problem or question did you investigate?
- Aim to describe the characteristics of something?
- Explore an under-researched topic?
- Establish a causal relationship?
And what type of data did you need to achieve this aim?
- Quantitative data , qualitative data , or a mix of both?
- Primary data collected yourself, or secondary data collected by someone else?
- Experimental data gathered by controlling and manipulating variables, or descriptive data gathered via observations?
Option 2: Start with your “why”
Depending on your discipline, you can also start with a discussion of the rationale and assumptions underpinning your methodology. In other words, why did you choose these methods for your study?
- Why is this the best way to answer your research question?
- Is this a standard methodology in your field, or does it require justification?
- Were there any ethical considerations involved in your choices?
- What are the criteria for validity and reliability in this type of research ?
Once you have introduced your reader to your methodological approach, you should share full details about your data collection methods .
Quantitative methods
In order to be considered generalisable, you should describe quantitative research methods in enough detail for another researcher to replicate your study.
Here, explain how you operationalised your concepts and measured your variables. Discuss your sampling method or inclusion/exclusion criteria, as well as any tools, procedures, and materials you used to gather your data.
Surveys Describe where, when, and how the survey was conducted.
- How did you design the questionnaire?
- What form did your questions take (e.g., multiple choice, Likert scale )?
- Were your surveys conducted in-person or virtually?
- What sampling method did you use to select participants?
- What was your sample size and response rate?
Experiments Share full details of the tools, techniques, and procedures you used to conduct your experiment.
- How did you design the experiment ?
- How did you recruit participants?
- How did you manipulate and measure the variables ?
- What tools did you use?
Existing data Explain how you gathered and selected the material (such as datasets or archival data) that you used in your analysis.
- Where did you source the material?
- How was the data originally produced?
- What criteria did you use to select material (e.g., date range)?
The survey consisted of 5 multiple-choice questions and 10 questions measured on a 7-point Likert scale.
The goal was to collect survey responses from 350 customers visiting the fitness apparel company’s brick-and-mortar location in Boston on 4–8 July 2022, between 11:00 and 15:00.
Here, a customer was defined as a person who had purchased a product from the company on the day they took the survey. Participants were given 5 minutes to fill in the survey anonymously. In total, 408 customers responded, but not all surveys were fully completed. Due to this, 371 survey results were included in the analysis.
Qualitative methods
In qualitative research , methods are often more flexible and subjective. For this reason, it’s crucial to robustly explain the methodology choices you made.
Be sure to discuss the criteria you used to select your data, the context in which your research was conducted, and the role you played in collecting your data (e.g., were you an active participant, or a passive observer?)
Interviews or focus groups Describe where, when, and how the interviews were conducted.
- How did you find and select participants?
- How many participants took part?
- What form did the interviews take ( structured , semi-structured , or unstructured )?
- How long were the interviews?
- How were they recorded?
Participant observation Describe where, when, and how you conducted the observation or ethnography .
- What group or community did you observe? How long did you spend there?
- How did you gain access to this group? What role did you play in the community?
- How long did you spend conducting the research? Where was it located?
- How did you record your data (e.g., audiovisual recordings, note-taking)?
Existing data Explain how you selected case study materials for your analysis.
- What type of materials did you analyse?
- How did you select them?
In order to gain better insight into possibilities for future improvement of the fitness shop’s product range, semi-structured interviews were conducted with 8 returning customers.
Here, a returning customer was defined as someone who usually bought products at least twice a week from the store.
Surveys were used to select participants. Interviews were conducted in a small office next to the cash register and lasted approximately 20 minutes each. Answers were recorded by note-taking, and seven interviews were also filmed with consent. One interviewee preferred not to be filmed.
Mixed methods
Mixed methods research combines quantitative and qualitative approaches. If a standalone quantitative or qualitative study is insufficient to answer your research question, mixed methods may be a good fit for you.
Mixed methods are less common than standalone analyses, largely because they require a great deal of effort to pull off successfully. If you choose to pursue mixed methods, it’s especially important to robustly justify your methods here.
Prevent plagiarism, run a free check.
Next, you should indicate how you processed and analysed your data. Avoid going into too much detail: you should not start introducing or discussing any of your results at this stage.
In quantitative research , your analysis will be based on numbers. In your methods section, you can include:
- How you prepared the data before analysing it (e.g., checking for missing data , removing outliers , transforming variables)
- Which software you used (e.g., SPSS, Stata or R)
- Which statistical tests you used (e.g., two-tailed t test , simple linear regression )
In qualitative research, your analysis will be based on language, images, and observations (often involving some form of textual analysis ).
Specific methods might include:
- Content analysis : Categorising and discussing the meaning of words, phrases and sentences
- Thematic analysis : Coding and closely examining the data to identify broad themes and patterns
- Discourse analysis : Studying communication and meaning in relation to their social context
Mixed methods combine the above two research methods, integrating both qualitative and quantitative approaches into one coherent analytical process.
Above all, your methodology section should clearly make the case for why you chose the methods you did. This is especially true if you did not take the most standard approach to your topic. In this case, discuss why other methods were not suitable for your objectives, and show how this approach contributes new knowledge or understanding.
In any case, it should be overwhelmingly clear to your reader that you set yourself up for success in terms of your methodology’s design. Show how your methods should lead to results that are valid and reliable, while leaving the analysis of the meaning, importance, and relevance of your results for your discussion section .
- Quantitative: Lab-based experiments cannot always accurately simulate real-life situations and behaviours, but they are effective for testing causal relationships between variables .
- Qualitative: Unstructured interviews usually produce results that cannot be generalised beyond the sample group , but they provide a more in-depth understanding of participants’ perceptions, motivations, and emotions.
- Mixed methods: Despite issues systematically comparing differing types of data, a solely quantitative study would not sufficiently incorporate the lived experience of each participant, while a solely qualitative study would be insufficiently generalisable.
Remember that your aim is not just to describe your methods, but to show how and why you applied them. Again, it’s critical to demonstrate that your research was rigorously conducted and can be replicated.
1. Focus on your objectives and research questions
The methodology section should clearly show why your methods suit your objectives and convince the reader that you chose the best possible approach to answering your problem statement and research questions .
2. Cite relevant sources
Your methodology can be strengthened by referencing existing research in your field. This can help you to:
- Show that you followed established practice for your type of research
- Discuss how you decided on your approach by evaluating existing research
- Present a novel methodological approach to address a gap in the literature
3. Write for your audience
Consider how much information you need to give, and avoid getting too lengthy. If you are using methods that are standard for your discipline, you probably don’t need to give a lot of background or justification.
Regardless, your methodology should be a clear, well-structured text that makes an argument for your approach, not just a list of technical details and procedures.
Methodology refers to the overarching strategy and rationale of your research. Developing your methodology involves studying the research methods used in your field and the theories or principles that underpin them, in order to choose the approach that best matches your objectives.
Methods are the specific tools and procedures you use to collect and analyse data (e.g. interviews, experiments , surveys , statistical tests ).
In a dissertation or scientific paper, the methodology chapter or methods section comes after the introduction and before the results , discussion and conclusion .
Depending on the length and type of document, you might also include a literature review or theoretical framework before the methodology.
Quantitative research deals with numbers and statistics, while qualitative research deals with words and meanings.
Quantitative methods allow you to test a hypothesis by systematically collecting and analysing data, while qualitative methods allow you to explore ideas and experiences in depth.
A sample is a subset of individuals from a larger population. Sampling means selecting the group that you will actually collect data from in your research.
For example, if you are researching the opinions of students in your university, you could survey a sample of 100 students.
Statistical sampling allows you to test a hypothesis about the characteristics of a population. There are various sampling methods you can use to ensure that your sample is representative of the population as a whole.
Cite this Scribbr article
If you want to cite this source, you can copy and paste the citation or click the ‘Cite this Scribbr article’ button to automatically add the citation to our free Reference Generator.
McCombes, S. (2022, October 10). What Is a Research Methodology? | Steps & Tips. Scribbr. Retrieved 20 March 2024, from https://www.scribbr.co.uk/thesis-dissertation/methodology/
Is this article helpful?
Shona McCombes
Other students also liked, how to write a dissertation proposal | a step-by-step guide, what is a literature review | guide, template, & examples, what is a theoretical framework | a step-by-step guide.
- Locations and Hours
- UCLA Library
- Research Guides
- Research Tips and Tools
Advanced Research Methods
Writing the research paper.
- What Is Research?
- Library Research
- Writing a Research Proposal
Before Writing the Paper
Methods, thesis, and hypothesis, clarity, precision, and academic expression, format your paper, typical problems, a few suggestions, avoid plagiarism.
- Presenting the Research Paper
- Try to find a subject that really interests you.
- While you explore the topic, narrow or broaden your target and focus on something that gives the most promising results.
- Don't choose a huge subject if you have to write a 3 page long paper, and broaden your topic sufficiently if you have to submit at least 25 pages.
- Consult your class instructor (and your classmates) about the topic.
- Find primary and secondary sources in the library.
- Read and critically analyse them.
- Take notes.
- Compile surveys, collect data, gather materials for quantitative analysis (if these are good methods to investigate the topic more deeply).
- Come up with new ideas about the topic. Try to formulate your ideas in a few sentences.
- Review your notes and other materials and enrich the outline.
- Try to estimate how long the individual parts will be.
- Do others understand what you want to say?
- Do they accept it as new knowledge or relevant and important for a paper?
- Do they agree that your thoughts will result in a successful paper?
- Qualitative: gives answers on questions (how, why, when, who, what, etc.) by investigating an issue
- Quantitative:requires data and the analysis of data as well
- the essence, the point of the research paper in one or two sentences.
- a statement that can be proved or disproved.
- Be specific.
- Avoid ambiguity.
- Use predominantly the active voice, not the passive.
- Deal with one issue in one paragraph.
- Be accurate.
- Double-check your data, references, citations and statements.
Academic Expression
- Don't use familiar style or colloquial/slang expressions.
- Write in full sentences.
- Check the meaning of the words if you don't know exactly what they mean.
- Avoid metaphors.
- Almost the rough content of every paragraph.
- The order of the various topics in your paper.
- On the basis of the outline, start writing a part by planning the content, and then write it down.
- Put a visible mark (which you will later delete) where you need to quote a source, and write in the citation when you finish writing that part or a bigger part.
- Does the text make sense?
- Could you explain what you wanted?
- Did you write good sentences?
- Is there something missing?
- Check the spelling.
- Complete the citations, bring them in standard format.
Use the guidelines that your instructor requires (MLA, Chicago, APA, Turabian, etc.).
- Adjust margins, spacing, paragraph indentation, place of page numbers, etc.
- Standardize the bibliography or footnotes according to the guidelines.
- EndNote and EndNote Basic by UCLA Library Last Updated Mar 18, 2024 667 views this year
- Zotero by UCLA Library Last Updated Jan 18, 2023 507 views this year
(Based on English Composition 2 from Illinois Valley Community College):
- Weak organization
- Poor support and development of ideas
- Weak use of secondary sources
- Excessive errors
- Stylistic weakness
When collecting materials, selecting research topic, and writing the paper:
- Be systematic and organized (e.g. keep your bibliography neat and organized; write your notes in a neat way, so that you can find them later on.
- Use your critical thinking ability when you read.
- Write down your thoughts (so that you can reconstruct them later).
- Stop when you have a really good idea and think about whether you could enlarge it to a whole research paper. If yes, take much longer notes.
- When you write down a quotation or summarize somebody else's thoughts in your notes or in the paper, cite the source (i.e. write down the author, title, publication place, year, page number).
- If you quote or summarize a thought from the internet, cite the internet source.
- Write an outline that is detailed enough to remind you about the content.
- Read your paper for yourself or, preferably, somebody else.
- When you finish writing, check the spelling;
- Use the citation form (MLA, Chicago, or other) that your instructor requires and use it everywhere.
Plagiarism : somebody else's words or ideas presented without citation by an author
- Cite your source every time when you quote a part of somebody's work.
- Cite your source every time when you summarize a thought from somebody's work.
- Cite your source every time when you use a source (quote or summarize) from the Internet.
Consult the Citing Sources research guide for further details.
- << Previous: Writing a Research Proposal
- Next: Presenting the Research Paper >>
- Last Updated: Jan 4, 2024 12:24 PM
- URL: https://guides.library.ucla.edu/research-methods
- Bipolar Disorder
- Therapy Center
- When To See a Therapist
- Types of Therapy
- Best Online Therapy
- Best Couples Therapy
- Best Family Therapy
- Managing Stress
- Sleep and Dreaming
- Understanding Emotions
- Self-Improvement
- Healthy Relationships
- Student Resources
- Personality Types
- Verywell Mind Insights
- 2023 Verywell Mind 25
- Mental Health in the Classroom
- Editorial Process
- Meet Our Review Board
- Crisis Support
How to Write a Methods Section for a Psychology Paper
Tips and Examples of an APA Methods Section
Kendra Cherry, MS, is a psychosocial rehabilitation specialist, psychology educator, and author of the "Everything Psychology Book."
:max_bytes(150000):strip_icc():format(webp)/IMG_9791-89504ab694d54b66bbd72cb84ffb860e.jpg)
Emily is a board-certified science editor who has worked with top digital publishing brands like Voices for Biodiversity, Study.com, GoodTherapy, Vox, and Verywell.
:max_bytes(150000):strip_icc():format(webp)/Emily-Swaim-1000-0f3197de18f74329aeffb690a177160c.jpg)
Verywell / Brianna Gilmartin
The methods section of an APA format psychology paper provides the methods and procedures used in a research study or experiment . This part of an APA paper is critical because it allows other researchers to see exactly how you conducted your research.
Method refers to the procedure that was used in a research study. It included a precise description of how the experiments were performed and why particular procedures were selected. While the APA technically refers to this section as the 'method section,' it is also often known as a 'methods section.'
The methods section ensures the experiment's reproducibility and the assessment of alternative methods that might produce different results. It also allows researchers to replicate the experiment and judge the study's validity.
This article discusses how to write a methods section for a psychology paper, including important elements to include and tips that can help.
What to Include in a Method Section
So what exactly do you need to include when writing your method section? You should provide detailed information on the following:
- Research design
- Participants
- Participant behavior
The method section should provide enough information to allow other researchers to replicate your experiment or study.
Components of a Method Section
The method section should utilize subheadings to divide up different subsections. These subsections typically include participants, materials, design, and procedure.
Participants
In this part of the method section, you should describe the participants in your experiment, including who they were (and any unique features that set them apart from the general population), how many there were, and how they were selected. If you utilized random selection to choose your participants, it should be noted here.
For example: "We randomly selected 100 children from elementary schools near the University of Arizona."
At the very minimum, this part of your method section must convey:
- Basic demographic characteristics of your participants (such as sex, age, ethnicity, or religion)
- The population from which your participants were drawn
- Any restrictions on your pool of participants
- How many participants were assigned to each condition and how they were assigned to each group (i.e., randomly assignment , another selection method, etc.)
- Why participants took part in your research (i.e., the study was advertised at a college or hospital, they received some type of incentive, etc.)
Information about participants helps other researchers understand how your study was performed, how generalizable the result might be, and allows other researchers to replicate the experiment with other populations to see if they might obtain the same results.
In this part of the method section, you should describe the materials, measures, equipment, or stimuli used in the experiment. This may include:
- Testing instruments
- Technical equipment
- Any psychological assessments that were used
- Any special equipment that was used
For example: "Two stories from Sullivan et al.'s (1994) second-order false belief attribution tasks were used to assess children's understanding of second-order beliefs."
For standard equipment such as computers, televisions, and videos, you can simply name the device and not provide further explanation.
Specialized equipment should be given greater detail, especially if it is complex or created for a niche purpose. In some instances, such as if you created a special material or apparatus for your study, you might need to include an illustration of the item in the appendix of your paper.
In this part of your method section, describe the type of design used in the experiment. Specify the variables as well as the levels of these variables. Identify:
- The independent variables
- Dependent variables
- Control variables
- Any extraneous variables that might influence your results.
Also, explain whether your experiment uses a within-groups or between-groups design.
For example: "The experiment used a 3x2 between-subjects design. The independent variables were age and understanding of second-order beliefs."
The next part of your method section should detail the procedures used in your experiment. Your procedures should explain:
- What the participants did
- How data was collected
- The order in which steps occurred
For example: "An examiner interviewed children individually at their school in one session that lasted 20 minutes on average. The examiner explained to each child that he or she would be told two short stories and that some questions would be asked after each story. All sessions were videotaped so the data could later be coded."
Keep this subsection concise yet detailed. Explain what you did and how you did it, but do not overwhelm your readers with too much information.
Tips for How to Write a Methods Section
In addition to following the basic structure of an APA method section, there are also certain things you should remember when writing this section of your paper. Consider the following tips when writing this section:
- Use the past tense : Always write the method section in the past tense.
- Be descriptive : Provide enough detail that another researcher could replicate your experiment, but focus on brevity. Avoid unnecessary detail that is not relevant to the outcome of the experiment.
- Use an academic tone : Use formal language and avoid slang or colloquial expressions. Word choice is also important. Refer to the people in your experiment or study as "participants" rather than "subjects."
- Use APA format : Keep a style guide on hand as you write your method section. The Publication Manual of the American Psychological Association is the official source for APA style.
- Make connections : Read through each section of your paper for agreement with other sections. If you mention procedures in the method section, these elements should be discussed in the results and discussion sections.
- Proofread : Check your paper for grammar, spelling, and punctuation errors.. typos, grammar problems, and spelling errors. Although a spell checker is a handy tool, there are some errors only you can catch.
After writing a draft of your method section, be sure to get a second opinion. You can often become too close to your work to see errors or lack of clarity. Take a rough draft of your method section to your university's writing lab for additional assistance.
A Word From Verywell
The method section is one of the most important components of your APA format paper. The goal of your paper should be to clearly detail what you did in your experiment. Provide enough detail that another researcher could replicate your study if they wanted.
Finally, if you are writing your paper for a class or for a specific publication, be sure to keep in mind any specific instructions provided by your instructor or by the journal editor. Your instructor may have certain requirements that you need to follow while writing your method section.
Frequently Asked Questions
While the subsections can vary, the three components that should be included are sections on the participants, the materials, and the procedures.
- Describe who the participants were in the study and how they were selected.
- Define and describe the materials that were used including any equipment, tests, or assessments
- Describe how the data was collected
To write your methods section in APA format, describe your participants, materials, study design, and procedures. Keep this section succinct, and always write in the past tense. The main heading of this section should be labeled "Method" and it should be centered, bolded, and capitalized. Each subheading within this section should be bolded, left-aligned and in title case.
The purpose of the methods section is to describe what you did in your experiment. It should be brief, but include enough detail that someone could replicate your experiment based on this information. Your methods section should detail what you did to answer your research question. Describe how the study was conducted, the study design that was used and why it was chosen, and how you collected the data and analyzed the results.
Erdemir F. How to write a materials and methods section of a scientific article ? Turk J Urol . 2013;39(Suppl 1):10-5. doi:10.5152/tud.2013.047
Kallet RH. How to write the methods section of a research paper . Respir Care . 2004;49(10):1229-32. PMID: 15447808.
American Psychological Association. Publication Manual of the American Psychological Association (7th ed.). Washington DC: The American Psychological Association; 2019.
American Psychological Association. APA Style Journal Article Reporting Standards . Published 2020.
By Kendra Cherry, MSEd Kendra Cherry, MS, is a psychosocial rehabilitation specialist, psychology educator, and author of the "Everything Psychology Book."
What is Research Methodology? Definition, Types, and Examples
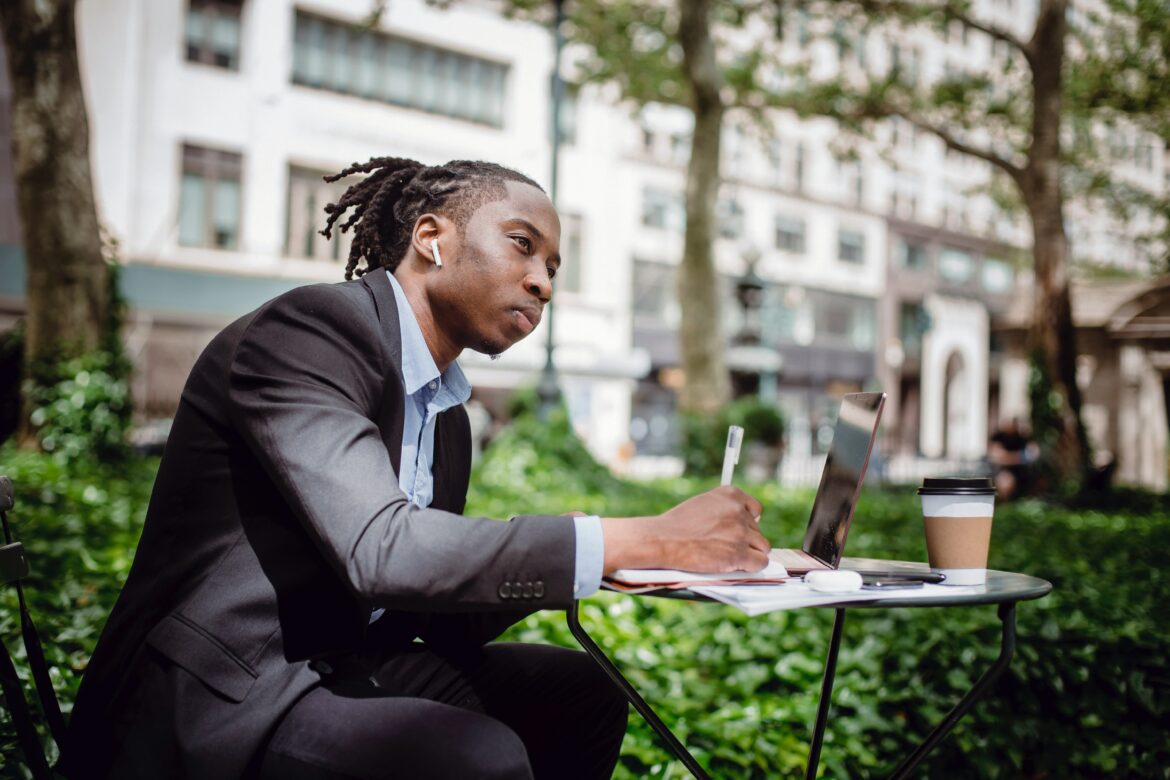
Research methodology 1,2 is a structured and scientific approach used to collect, analyze, and interpret quantitative or qualitative data to answer research questions or test hypotheses. A research methodology is like a plan for carrying out research and helps keep researchers on track by limiting the scope of the research. Several aspects must be considered before selecting an appropriate research methodology, such as research limitations and ethical concerns that may affect your research.
The research methodology section in a scientific paper describes the different methodological choices made, such as the data collection and analysis methods, and why these choices were selected. The reasons should explain why the methods chosen are the most appropriate to answer the research question. A good research methodology also helps ensure the reliability and validity of the research findings. There are three types of research methodology—quantitative, qualitative, and mixed-method, which can be chosen based on the research objectives.
What is research methodology ?
A research methodology describes the techniques and procedures used to identify and analyze information regarding a specific research topic. It is a process by which researchers design their study so that they can achieve their objectives using the selected research instruments. It includes all the important aspects of research, including research design, data collection methods, data analysis methods, and the overall framework within which the research is conducted. While these points can help you understand what is research methodology, you also need to know why it is important to pick the right methodology.
Why is research methodology important?
Having a good research methodology in place has the following advantages: 3
- Helps other researchers who may want to replicate your research; the explanations will be of benefit to them.
- You can easily answer any questions about your research if they arise at a later stage.
- A research methodology provides a framework and guidelines for researchers to clearly define research questions, hypotheses, and objectives.
- It helps researchers identify the most appropriate research design, sampling technique, and data collection and analysis methods.
- A sound research methodology helps researchers ensure that their findings are valid and reliable and free from biases and errors.
- It also helps ensure that ethical guidelines are followed while conducting research.
- A good research methodology helps researchers in planning their research efficiently, by ensuring optimum usage of their time and resources.
Writing the methods section of a research paper? Let Paperpal help you achieve perfection
Types of research methodology.
There are three types of research methodology based on the type of research and the data required. 1
- Quantitative research methodology focuses on measuring and testing numerical data. This approach is good for reaching a large number of people in a short amount of time. This type of research helps in testing the causal relationships between variables, making predictions, and generalizing results to wider populations.
- Qualitative research methodology examines the opinions, behaviors, and experiences of people. It collects and analyzes words and textual data. This research methodology requires fewer participants but is still more time consuming because the time spent per participant is quite large. This method is used in exploratory research where the research problem being investigated is not clearly defined.
- Mixed-method research methodology uses the characteristics of both quantitative and qualitative research methodologies in the same study. This method allows researchers to validate their findings, verify if the results observed using both methods are complementary, and explain any unexpected results obtained from one method by using the other method.
What are the types of sampling designs in research methodology?
Sampling 4 is an important part of a research methodology and involves selecting a representative sample of the population to conduct the study, making statistical inferences about them, and estimating the characteristics of the whole population based on these inferences. There are two types of sampling designs in research methodology—probability and nonprobability.
- Probability sampling
In this type of sampling design, a sample is chosen from a larger population using some form of random selection, that is, every member of the population has an equal chance of being selected. The different types of probability sampling are:
- Systematic —sample members are chosen at regular intervals. It requires selecting a starting point for the sample and sample size determination that can be repeated at regular intervals. This type of sampling method has a predefined range; hence, it is the least time consuming.
- Stratified —researchers divide the population into smaller groups that don’t overlap but represent the entire population. While sampling, these groups can be organized, and then a sample can be drawn from each group separately.
- Cluster —the population is divided into clusters based on demographic parameters like age, sex, location, etc.
- Convenience —selects participants who are most easily accessible to researchers due to geographical proximity, availability at a particular time, etc.
- Purposive —participants are selected at the researcher’s discretion. Researchers consider the purpose of the study and the understanding of the target audience.
- Snowball —already selected participants use their social networks to refer the researcher to other potential participants.
- Quota —while designing the study, the researchers decide how many people with which characteristics to include as participants. The characteristics help in choosing people most likely to provide insights into the subject.
What are data collection methods?
During research, data are collected using various methods depending on the research methodology being followed and the research methods being undertaken. Both qualitative and quantitative research have different data collection methods, as listed below.
Qualitative research 5
- One-on-one interviews: Helps the interviewers understand a respondent’s subjective opinion and experience pertaining to a specific topic or event
- Document study/literature review/record keeping: Researchers’ review of already existing written materials such as archives, annual reports, research articles, guidelines, policy documents, etc.
- Focus groups: Constructive discussions that usually include a small sample of about 6-10 people and a moderator, to understand the participants’ opinion on a given topic.
- Qualitative observation : Researchers collect data using their five senses (sight, smell, touch, taste, and hearing).
Quantitative research 6
- Sampling: The most common type is probability sampling.
- Interviews: Commonly telephonic or done in-person.
- Observations: Structured observations are most commonly used in quantitative research. In this method, researchers make observations about specific behaviors of individuals in a structured setting.
- Document review: Reviewing existing research or documents to collect evidence for supporting the research.
- Surveys and questionnaires. Surveys can be administered both online and offline depending on the requirement and sample size.
Let Paperpal help you write the perfect research methods section. Start now!
What are data analysis methods.
The data collected using the various methods for qualitative and quantitative research need to be analyzed to generate meaningful conclusions. These data analysis methods 7 also differ between quantitative and qualitative research.
Quantitative research involves a deductive method for data analysis where hypotheses are developed at the beginning of the research and precise measurement is required. The methods include statistical analysis applications to analyze numerical data and are grouped into two categories—descriptive and inferential.
Descriptive analysis is used to describe the basic features of different types of data to present it in a way that ensures the patterns become meaningful. The different types of descriptive analysis methods are:
- Measures of frequency (count, percent, frequency)
- Measures of central tendency (mean, median, mode)
- Measures of dispersion or variation (range, variance, standard deviation)
- Measure of position (percentile ranks, quartile ranks)
Inferential analysis is used to make predictions about a larger population based on the analysis of the data collected from a smaller population. This analysis is used to study the relationships between different variables. Some commonly used inferential data analysis methods are:
- Correlation: To understand the relationship between two or more variables.
- Cross-tabulation: Analyze the relationship between multiple variables.
- Regression analysis: Study the impact of independent variables on the dependent variable.
- Frequency tables: To understand the frequency of data.
- Analysis of variance: To test the degree to which two or more variables differ in an experiment.
Qualitative research involves an inductive method for data analysis where hypotheses are developed after data collection. The methods include:
- Content analysis: For analyzing documented information from text and images by determining the presence of certain words or concepts in texts.
- Narrative analysis: For analyzing content obtained from sources such as interviews, field observations, and surveys. The stories and opinions shared by people are used to answer research questions.
- Discourse analysis: For analyzing interactions with people considering the social context, that is, the lifestyle and environment, under which the interaction occurs.
- Grounded theory: Involves hypothesis creation by data collection and analysis to explain why a phenomenon occurred.
- Thematic analysis: To identify important themes or patterns in data and use these to address an issue.
How to choose a research methodology?
Here are some important factors to consider when choosing a research methodology: 8
- Research objectives, aims, and questions —these would help structure the research design.
- Review existing literature to identify any gaps in knowledge.
- Check the statistical requirements —if data-driven or statistical results are needed then quantitative research is the best. If the research questions can be answered based on people’s opinions and perceptions, then qualitative research is most suitable.
- Sample size —sample size can often determine the feasibility of a research methodology. For a large sample, less effort- and time-intensive methods are appropriate.
- Constraints —constraints of time, geography, and resources can help define the appropriate methodology.
Got writer’s block? Kickstart your research paper writing with Paperpal now!
How to write a research methodology .
A research methodology should include the following components: 3,9
- Research design —should be selected based on the research question and the data required. Common research designs include experimental, quasi-experimental, correlational, descriptive, and exploratory.
- Research method —this can be quantitative, qualitative, or mixed-method.
- Reason for selecting a specific methodology —explain why this methodology is the most suitable to answer your research problem.
- Research instruments —explain the research instruments you plan to use, mainly referring to the data collection methods such as interviews, surveys, etc. Here as well, a reason should be mentioned for selecting the particular instrument.
- Sampling —this involves selecting a representative subset of the population being studied.
- Data collection —involves gathering data using several data collection methods, such as surveys, interviews, etc.
- Data analysis —describe the data analysis methods you will use once you’ve collected the data.
- Research limitations —mention any limitations you foresee while conducting your research.
- Validity and reliability —validity helps identify the accuracy and truthfulness of the findings; reliability refers to the consistency and stability of the results over time and across different conditions.
- Ethical considerations —research should be conducted ethically. The considerations include obtaining consent from participants, maintaining confidentiality, and addressing conflicts of interest.
Streamline Your Research Paper Writing Process with Paperpal
The methods section is a critical part of the research papers, allowing researchers to use this to understand your findings and replicate your work when pursuing their own research. However, it is usually also the most difficult section to write. This is where Paperpal can help you overcome the writer’s block and create the first draft in minutes with Paperpal Copilot, its secure generative AI feature suite.
With Paperpal you can get research advice, write and refine your work, rephrase and verify the writing, and ensure submission readiness, all in one place. Here’s how you can use Paperpal to develop the first draft of your methods section.
- Generate an outline: Input some details about your research to instantly generate an outline for your methods section
- Develop the section: Use the outline and suggested sentence templates to expand your ideas and develop the first draft.
- P araph ras e and trim : Get clear, concise academic text with paraphrasing that conveys your work effectively and word reduction to fix redundancies.
- Choose the right words: Enhance text by choosing contextual synonyms based on how the words have been used in previously published work.
- Check and verify text : Make sure the generated text showcases your methods correctly, has all the right citations, and is original and authentic. .
You can repeat this process to develop each section of your research manuscript, including the title, abstract and keywords. Ready to write your research papers faster, better, and without the stress? Sign up for Paperpal and start writing today!
Frequently Asked Questions
Q1. What are the key components of research methodology?
A1. A good research methodology has the following key components:
- Research design
- Data collection procedures
- Data analysis methods
- Ethical considerations
Q2. Why is ethical consideration important in research methodology?
A2. Ethical consideration is important in research methodology to ensure the readers of the reliability and validity of the study. Researchers must clearly mention the ethical norms and standards followed during the conduct of the research and also mention if the research has been cleared by any institutional board. The following 10 points are the important principles related to ethical considerations: 10
- Participants should not be subjected to harm.
- Respect for the dignity of participants should be prioritized.
- Full consent should be obtained from participants before the study.
- Participants’ privacy should be ensured.
- Confidentiality of the research data should be ensured.
- Anonymity of individuals and organizations participating in the research should be maintained.
- The aims and objectives of the research should not be exaggerated.
- Affiliations, sources of funding, and any possible conflicts of interest should be declared.
- Communication in relation to the research should be honest and transparent.
- Misleading information and biased representation of primary data findings should be avoided.
Q3. What is the difference between methodology and method?
A3. Research methodology is different from a research method, although both terms are often confused. Research methods are the tools used to gather data, while the research methodology provides a framework for how research is planned, conducted, and analyzed. The latter guides researchers in making decisions about the most appropriate methods for their research. Research methods refer to the specific techniques, procedures, and tools used by researchers to collect, analyze, and interpret data, for instance surveys, questionnaires, interviews, etc.
Research methodology is, thus, an integral part of a research study. It helps ensure that you stay on track to meet your research objectives and answer your research questions using the most appropriate data collection and analysis tools based on your research design.
Accelerate your research paper writing with Paperpal. Try for free now!
- Research methodologies. Pfeiffer Library website. Accessed August 15, 2023. https://library.tiffin.edu/researchmethodologies/whatareresearchmethodologies
- Types of research methodology. Eduvoice website. Accessed August 16, 2023. https://eduvoice.in/types-research-methodology/
- The basics of research methodology: A key to quality research. Voxco. Accessed August 16, 2023. https://www.voxco.com/blog/what-is-research-methodology/
- Sampling methods: Types with examples. QuestionPro website. Accessed August 16, 2023. https://www.questionpro.com/blog/types-of-sampling-for-social-research/
- What is qualitative research? Methods, types, approaches, examples. Researcher.Life blog. Accessed August 15, 2023. https://researcher.life/blog/article/what-is-qualitative-research-methods-types-examples/
- What is quantitative research? Definition, methods, types, and examples. Researcher.Life blog. Accessed August 15, 2023. https://researcher.life/blog/article/what-is-quantitative-research-types-and-examples/
- Data analysis in research: Types & methods. QuestionPro website. Accessed August 16, 2023. https://www.questionpro.com/blog/data-analysis-in-research/#Data_analysis_in_qualitative_research
- Factors to consider while choosing the right research methodology. PhD Monster website. Accessed August 17, 2023. https://www.phdmonster.com/factors-to-consider-while-choosing-the-right-research-methodology/
- What is research methodology? Research and writing guides. Accessed August 14, 2023. https://paperpile.com/g/what-is-research-methodology/
- Ethical considerations. Business research methodology website. Accessed August 17, 2023. https://research-methodology.net/research-methodology/ethical-considerations/
Paperpal is a comprehensive AI writing toolkit that helps students and researchers achieve 2x the writing in half the time. It leverages 21+ years of STM experience and insights from millions of research articles to provide in-depth academic writing, language editing, and submission readiness support to help you write better, faster.
Get accurate academic translations, rewriting support, grammar checks, vocabulary suggestions, and generative AI assistance that delivers human precision at machine speed. Try for free or upgrade to Paperpal Prime starting at US$19 a month to access premium features, including consistency, plagiarism, and 30+ submission readiness checks to help you succeed.
Experience the future of academic writing – Sign up to Paperpal and start writing for free!
Related Reads:
- Dangling Modifiers and How to Avoid Them in Your Writing
- Webinar: How to Use Generative AI Tools Ethically in Your Academic Writing
- Research Outlines: How to Write An Introduction Section in Minutes with Paperpal Copilot
- How to Paraphrase Research Papers Effectively
Language and Grammar Rules for Academic Writing
Climatic vs. climactic: difference and examples, you may also like, how to write an essay introduction (with examples)..., similarity checks: the author’s guide to plagiarism and..., what is a master’s thesis: a guide for..., should you use ai tools like chatgpt for..., what are the benefits of generative ai for..., how to avoid plagiarism tips and advice for..., plagiarism checkers vs. ai content detection: navigating the..., plagiarism prevention: why you need a plagiarism check..., how long should a chapter be, how to cite social media sources in academic writing .

- How to Do Research for an Excellent Essay: The Complete Guide
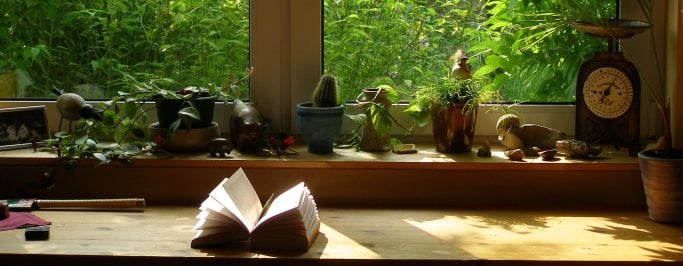
One of the biggest secrets to writing a good essay is the Boy Scouts’ motto: ‘be prepared’. Preparing for an essay – by conducting effective research – lays the foundations for a brilliant piece of writing, and it’s every bit as important as the actual writing part. Many students skimp on this crucial stage, or sit in the library not really sure where to start; and it shows in the quality of their essays. This just makes it easier for you to get ahead of your peers, and we’re going to show you how. In this article, we take you through what you need to do in order to conduct effective research and use your research time to best effect.
Allow enough time
First and foremost, it’s vital to allow enough time for your research. For this reason, don’t leave your essay until the last minute . If you start writing without having done adequate research, it will almost certainly show in your essay’s lack of quality. The amount of research time needed will vary according to whether you’re at Sixth Form or university, and according to how well you know the topic and what teaching you’ve had on it, but make sure you factor in more time than you think you’ll need. You may come across a concept that takes you longer to understand than you’d expected, so it’s better to allow too much time than too little.
Read the essay question and thoroughly understand it
If you don’t have a thorough understanding of what the essay question is asking you to do, you put yourself at risk of going in the wrong direction with your research. So take the question, read it several times and pull out the key things it’s asking you to do. The instructions in the question are likely to have some bearing on the nature of your research. If the question says “Compare”, for example, this will set you up for a particular kind of research, during which you’ll be looking specifically for points of comparison; if the question asks you to “Discuss”, your research focus may be more on finding different points of view and formulating your own.
Begin with a brainstorm
Start your research time by brainstorming what you already know. Doing this means that you can be clear about exactly what you’re already aware of, and you can identify the gaps in your knowledge so that you don’t end up wasting time by reading books that will tell you what you already know. This gives your research more of a direction and allows you to be more specific in your efforts to find out certain things. It’s also a gentle way of introducing yourself to the task and putting yourself in the right frame of mind for learning about the topic at hand.
Achieve a basic understanding before delving deeper
If the topic is new to you and your brainstorm has yielded few ideas, you’ll need to acquire a basic understanding of the topic before you begin delving deeper into your research. If you don’t, and you start by your research by jumping straight in at the deep end, as it were, you’ll struggle to grasp the topic. This also means that you may end up being too swayed by a certain source, as you haven’t the knowledge to question it properly. You need sufficient background knowledge to be able to take a critical approach to each of the sources you read. So, start from the very beginning. It’s ok to use Wikipedia or other online resources to give you an introduction to a topic, though bear in mind that these can’t be wholly relied upon. If you’ve covered the topic in class already, re-read the notes you made so that you can refresh your mind before you start further investigation.
Working through your reading list
If you’ve been given a reading list to work from, be organised in how you work through each of the items on it. Try to get hold of as many of the books on it as you can before you start, so that you have them all easily to hand, and can refer back to things you’ve read and compare them with other perspectives. Plan the order in which you’re going to work through them and try to allocate a specific amount of time to each of them; this ensures that you allow enough time to do each of them justice and that focus yourself on making the most of your time with each one. It’s a good idea to go for the more general resources before honing in on the finer points mentioned in more specialised literature. Think of an upside-down pyramid and how it starts off wide at the top and becomes gradually narrower; this is the sort of framework you should apply to your research.
Ask a librarian
Library computer databases can be confusing things, and can add an extra layer of stress and complexity to your research if you’re not used to using them. The librarian is there for a reason, so don’t be afraid to go and ask if you’re not sure where to find a particular book on your reading list. If you’re in need of somewhere to start, they should be able to point you in the direction of the relevant section of the library so that you can also browse for books that may yield useful information.
Use the index
If you haven’t been given specific pages to read in the books on your reading list, make use of the index (and/or table of contents) of each book to help you find relevant material. It sounds obvious, but some students don’t think to do this and battle their way through heaps of irrelevant chapters before finding something that will be useful for their essay.
Taking notes
As you work through your reading, take notes as you go along rather than hoping you’ll remember everything you’ve read. Don’t indiscriminately write down everything – only the bits that will be useful in answering the essay question you’ve been set. If you write down too much, you risk writing an essay that’s full of irrelevant material and getting lower grades as a result. Be concise, and summarise arguments in your own words when you make notes (this helps you learn it better, too, because you actually have to think about how best to summarise it). You may want to make use of small index cards to force you to be brief with what you write about each point or topic. We’ve covered effective note-taking extensively in another article, which you can read here . Note-taking is a major part of the research process, so don’t neglect it. Your notes don’t just come in useful in the short-term, for completing your essay, but they should also be helpful when it comes to revision time, so try to keep them organised.
Research every side of the argument
Never rely too heavily on one resource without referring to other possible opinions; it’s bad academic practice. You need to be able to give a balanced argument in an essay, and that means researching a range of perspectives on whatever problem you’re tackling. Keep a note of the different arguments, along with the evidence in support of or against each one, ready to be deployed into an essay structure that works logically through each one. If you see a scholar’s name cropping up again and again in what you read, it’s worth investigating more about them even if you haven’t specifically been told to do so. Context is vital in academia at any level, so influential figures are always worth knowing about.
Keep a dictionary by your side
You could completely misunderstand a point you read if you don’t know what one important word in the sentence means. For that reason, it’s a good idea to keep a dictionary by your side at all times as you conduct your research. Not only does this help you fully understand what you’re reading, but you also learn new words that you might be able to use in your forthcoming essay or a future one . Growing your vocabulary is never a waste of time!
Start formulating your own opinion
As you work through reading these different points of view, think carefully about what you’ve read and note your own response to different opinions. Get into the habit of questioning sources and make sure you’re not just repeating someone else’s opinion without challenging it. Does an opinion make sense? Does it have plenty of evidence to back it up? What are the counter-arguments, and on balance, which sways you more? Demonstrating your own intelligent thinking will set your essay apart from those of your peers, so think about these things as you conduct your research.
Be careful with web-based research
Although, as we’ve said already, it’s fine to use Wikipedia and other online resources to give you a bit of an introduction to a topic you haven’t covered before, be very careful when using the internet for researching an essay. Don’t take Wikipedia as gospel; don’t forget, anybody can edit it! We wouldn’t advise using the internet as the basis of your essay research – it’s simply not academically rigorous enough, and you don’t know how out of date a particular resource might be. Even if your Sixth Form teachers may not question where you picked up an idea you’ve discussed in your essays, it’s still not a good habit to get into and you’re unlikely to get away with it at a good university. That said, there are still reliable academic resources available via the internet; these can be found in dedicated sites that are essentially online libraries, such as JSTOR. These are likely to be a little too advanced if you’re still in Sixth Form, but you’ll almost certainly come across them once you get to university.
Look out for footnotes
In an academic publication, whether that’s a book or a journal article, footnotes are a great place to look for further ideas for publications that might yield useful information. Plenty can be hidden away in footnotes, and if a writer is disparaging or supporting the ideas of another academic, you could look up the text in question so that you can include their opinion too, and whether or not you agree with them, for extra brownie points.
Don’t save doing all your own references until last
If you’re still in Sixth Form, you might not yet be required to include academic references in your essays, but for the sake of a thorough guide to essay research that will be useful to you in the future, we’re going to include this point anyway (it will definitely come in useful when you get to university, so you may as well start thinking about it now!). As you read through various books and find points you think you’re going to want to make in your essays, make sure you note down where you found these points as you go along (author’s first and last name, the publication title, publisher, publication date and page number). When you get to university you will be expected to identify your sources very precisely, so it’s a good habit to get into. Unfortunately, many students forget to do this and then have a difficult time of going back through their essay adding footnotes and trying to remember where they found a particular point. You’ll save yourself a great deal of time and effort if you simply note down your academic references as you go along. If you are including footnotes, don’t forget to add each publication to a main bibliography, to be included at the end of your essay, at the same time.
Putting in the background work required to write a good essay can seem an arduous task at times, but it’s a fundamental step that can’t simply be skipped. The more effort you put in at this stage, the better your essay will be and the easier it will be to write. Use the tips in this article and you’ll be well on your way to an essay that impresses!
To get even more prepared for essay writing you might also want to consider attending an Oxford Summer School .
Image credits: banner
- PRO Courses Guides New Tech Help Pro Expert Videos About wikiHow Pro Upgrade Sign In
- EDIT Edit this Article
- EXPLORE Tech Help Pro About Us Random Article Quizzes Request a New Article Community Dashboard This Or That Game Popular Categories Arts and Entertainment Artwork Books Movies Computers and Electronics Computers Phone Skills Technology Hacks Health Men's Health Mental Health Women's Health Relationships Dating Love Relationship Issues Hobbies and Crafts Crafts Drawing Games Education & Communication Communication Skills Personal Development Studying Personal Care and Style Fashion Hair Care Personal Hygiene Youth Personal Care School Stuff Dating All Categories Arts and Entertainment Finance and Business Home and Garden Relationship Quizzes Cars & Other Vehicles Food and Entertaining Personal Care and Style Sports and Fitness Computers and Electronics Health Pets and Animals Travel Education & Communication Hobbies and Crafts Philosophy and Religion Work World Family Life Holidays and Traditions Relationships Youth
- Browse Articles
- Learn Something New
- Quizzes Hot
- This Or That Game New
- Train Your Brain
- Explore More
- Support wikiHow
- About wikiHow
- Log in / Sign up
- Education and Communications
- College University and Postgraduate
- Academic Writing
How to Write a Research Essay
Last Updated: January 12, 2023 Fact Checked
This article was co-authored by Michelle Golden, PhD . Michelle Golden is an English teacher in Athens, Georgia. She received her MA in Language Arts Teacher Education in 2008 and received her PhD in English from Georgia State University in 2015. There are 11 references cited in this article, which can be found at the bottom of the page. This article has been fact-checked, ensuring the accuracy of any cited facts and confirming the authority of its sources. This article has been viewed 383,369 times.
Research essays are extremely common assignments in high school, college, and graduate school, and are not unheard of in middle school. If you are a student, chances are you will sooner or later be faced with the task of researching a topic and writing a paper about it. Knowing how to efficiently and successfully do simple research, synthesize information, and clearly present it in essay form will save you many hours and a lot of frustration.
Researching a Topic

- Be sure to stay within the guidelines you are given by your teacher or professor. For example, if you are free to choose a topic but the general theme must fall under human biology, do not write your essay on plant photosynthesis.
- Stick with topics that are not overly complicated, especially if the subject is not something you plan to continue studying. There's no need to make things harder on yourself!

- Specialty books; these can be found at your local public or school library. A book published on your topic is a great resource and will likely be one of your most reliable options for finding quality information. They also contain lists of references where you can look for more information.
- Academic journals; these are periodicals devoted to scholarly research on a specific field of study. Articles in academic journals are written by experts in that field and scrutinized by other professionals to ensure their accuracy. These are great options if you need to find detailed, sophisticated information on your topic; avoid these if you are only writing a general overview.
- Online encyclopedias; the most reliable information on the internet can be found in online encyclopedias like Encyclopedia.com and Britannica.com. While online wikis can be very helpful, they sometimes contain unverified information that you should probably not rely upon as your primary resources.
- Expert interviews; if possible, interview an expert in the subject of your research. Experts can be professionals working in the field you are studying, professors with advanced degrees in the subject of interest, etc.

- Organize your notes by sub-topic to keep them orderly and so you can easily find references when you are writing.
- If you are using books or physical copies of magazines or journals, use sticky tabs to mark pages or paragraphs where you found useful information. You might even want to number these tabs to correspond with numbers on your note sheet for easy reference.
- By keeping your notes brief and simple, you can make them easier to understand and reference while writing. Don't make your notes so long and detailed that they essentially copy what's already written in your sources, as this won't be helpful to you.

- Sometimes the objective of your research will be obvious to you before you even begin researching the topic; other times, you may have to do a bit of reading before you can determine the direction you want your essay to take.
- If you have an objective in mind from the start, you can incorporate this into online searches about your topic in order to find the most relevant resources. For example, if your objective is to outline the environmental hazards of hydraulic fracturing practices, search for that exact phrase rather than just "hydraulic fracturing."

- Avoid asking your teacher to give you a topic. Unless your topic was assigned to you in the first place, part of the assignment is for you to choose a topic relevant to the broader theme of the class or unit. By asking your teacher to do this for you, you risk admitting laziness or incompetence.
- If you have a few topics in mind but are not sure how to develop objectives for some of them, your teacher can help with this. Plan to discuss your options with your teacher and come to a decision yourself rather than having him or her choose the topic for you from several options.
Organizing your Essay

- Consider what background information is necessary to contextualize your research topic. What questions might the reader have right out of the gate? How do you want the reader to think about the topic? Answering these kinds of questions can help you figure out how to set up your argument.
- Match your paper sections to the objective(s) of your writing. For example, if you are trying to present two sides of a debate, create a section for each and then divide them up according to the aspects of each argument you want to address.

- An outline can be as detailed or general as you want, so long as it helps you figure out how to construct the essay. Some people like to include a few sentences under each heading in their outline to create a sort of "mini-essay" before they begin writing. Others find that a simple ordered list of topics is sufficient. Do whatever works best for you.
- If you have time, write your outline a day or two before you start writing and come back to it several times. This will give you an opportunity to think about how the pieces of your essay will best fit together. Rearrange things in your outline as many times as you want until you have a structure you are happy with.

- Style guides tell you exactly how to quote passages, cite references, construct works cited sections, etc. If you are assigned a specific format, you must take care to adhere to guidelines for text formatting and citations.
- Some computer programs (such as EndNote) allow you to construct a library of resources which you can then set to a specific format type; then you can automatically insert in-text citations from your library and populate a references section at the end of the document. This is an easy way to make sure your citations match your assigned style format.

- You may wish to start by simply assigning yourself a certain number of pages per day. Divide the number of pages you are required to write by the number of days you have to finish the essay; this is the number of pages (minimum) that you must complete each day in order to pace yourself evenly.
- If possible, leave a buffer of at least one day between finishing your paper and the due date. This will allow you to review your finished product and edit it for errors. This will also help in case something comes up that slows your writing progress.
Writing your Essay

- Keep your introduction relatively short. For most papers, one or two paragraphs will suffice. For really long essays, you may need to expand this.
- Don't assume your reader already knows the basics of the topic unless it truly is a matter of common knowledge. For example, you probably don't need to explain in your introduction what biology is, but you should define less general terms such as "eukaryote" or "polypeptide chain."

- You may need to include a special section at the beginning of the essay body for background information on your topic. Alternatively, you can consider moving this to the introductory section, but only if your essay is short and only minimal background discussion is needed.
- This is the part of your paper where organization and structure are most important. Arrange sections within the body so that they flow logically and the reader is introduced to ideas and sub-topics before they are discussed further.
- Depending upon the length and detail of your paper, the end of the body might contain a discussion of findings. This kind of section serves to wrap up your main findings but does not explicitly state your conclusions (which should come in the final section of the essay).
- Avoid repetition in the essay body. Keep your writing concise, yet with sufficient detail to address your objective(s) or research question(s).

- Always use quotation marks when using exact quotes from another source. If someone already said or wrote the words you are using, you must quote them this way! Place your in-text citation at the end of the quote.
- To include someone else's ideas in your essay without directly quoting them, you can restate the information in your own words; this is called paraphrasing. Although this does not require quotation marks, it should still be accompanied by an in-text citation.

- Except for very long essays, keep your conclusion short and to the point. You should aim for one or two paragraphs, if possible.
- Conclusions should directly correspond to research discussed in the essay body. In other words, make sure your conclusions logically connect to the rest of your essay and provide explanations when necessary.
- If your topic is complex and involves lots of details, you should consider including a brief summary of the main points of your research in your conclusion.

- Making changes to the discussion and conclusion sections instead of the introduction often requires a less extensive rewrite. Doing this also prevents you from removing anything from the beginning of your essay that could accidentally make subsequent portions of your writing seem out of place.
- It is okay to revise your thesis once you've finished the first draft of your essay! People's views often change once they've done research on a topic. Just make sure you don't end up straying too far from your assigned topic if you do this.
- You don't necessarily need to wait until you've finished your entire draft to do this step. In fact, it is a good idea to revisit your thesis regularly as you write. This can save you a lot of time in the end by helping you keep your essay content on track.

- Computer software such as EndNote is available for making citation organization as easy and quick as possible. You can create a reference library and link it to your document, adding in-text citations as you write; the program creates a formatted works cited section at the end of your document.
- Be aware of the formatting requirements of your chosen style guide for works cited sections and in-text citations. Reference library programs like EndNote have hundreds of pre-loaded formats to choose from.

- Create a catchy title. Waiting until you have finished your essay before choosing a title ensures that it will closely match the content of your essay. Research papers don't always take on the shape we expect them to, and it's easier to match your title to your essay than vice-versa.
- Read through your paper to identify and rework sentences or paragraphs that are confusing or unclear. Each section of your paper should have a clear focus and purpose; if any of yours seem not to meet these expectations, either rewrite or discard them.
- Review your works cited section (at the end of your essay) to ensure that it conforms to the standards of your chosen or assigned style format. You should at least make sure that the style is consistent throughout this section.
- Run a spell checker on your entire document to catch any spelling or grammar mistakes you may not have noticed during your read-through. All modern word processing programs include this function.

- Note that revising your draft is not the same as proofreading it. Revisions are done to make sure the content and substantive ideas are solid; editing is done to check for spelling and grammar errors. Revisions are arguably a more important part of writing a good paper.
- You may want to have a friend, classmate, or family member read your first draft and give you feedback. This can be immensely helpful when trying to decide how to improve upon your first version of the essay.
- Except in extreme cases, avoid a complete rewrite of your first draft. This will most likely be counterproductive and will waste a lot of time. Your first draft is probably already pretty good -- it likely just needs some tweaking before it is ready to submit.
Community Q&A

- Avoid use of the word "I" in research essay writing, even when conveying your personal opinion about a subject. This makes your writing sound biased and narrow in scope. Thanks Helpful 0 Not Helpful 0
- Even if there is a minimum number of paragraphs, always do 3 or 4 more paragraphs more than needed, so you can always get a good grade. Thanks Helpful 0 Not Helpful 0

- Never plagiarize the work of others! Passing off others' writing as your own can land you in a lot of trouble and is usually grounds for failing an assignment or class. Thanks Helpful 12 Not Helpful 1
You Might Also Like

- ↑ https://owl.purdue.edu/owl/general_writing/common_writing_assignments/research_papers/choosing_a_topic.html
- ↑ https://libguides.mit.edu/select-topic
- ↑ https://www.indeed.com/career-advice/career-development/research-objectives
- ↑ https://www.hunter.cuny.edu/rwc/handouts/the-writing-process-1/organization/Organizing-an-Essay
- ↑ https://www.lynchburg.edu/academics/writing-center/wilmer-writing-center-online-writing-lab/the-writing-process/organizing-your-paper/
- ↑ https://www.mla.org/MLA-Style
- ↑ http://www.apastyle.org/
- ↑ https://writing.wisc.edu/Handbook/PlanResearchPaper.html
- ↑ https://owl.purdue.edu/owl/research_and_citation/apa6_style/apa_formatting_and_style_guide/in_text_citations_the_basics.html
- ↑ https://opentextbc.ca/writingforsuccess/chapter/chapter-12-peer-review-and-final-revisions/
- ↑ https://openoregon.pressbooks.pub/wrd/back-matter/creating-a-works-cited-page/
About This Article

The best way to write a research essay is to find sources, like specialty books, academic journals, and online encyclopedias, about your topic. Take notes as you research, and make sure you note which page and book you got your notes from. Create an outline for the paper that details your argument, various sections, and primary points for each section. Then, write an introduction, build the body of the essay, and state your conclusion. Cite your sources along the way, and follow the assigned format, like APA or MLA, if applicable. To learn more from our co-author with an English Ph.D. about how to choose a thesis statement for your research paper, keep reading! Did this summary help you? Yes No
- Send fan mail to authors
Reader Success Stories

Nov 18, 2018
Did this article help you?

Jun 11, 2017

Christina Wonodi
Oct 12, 2016

Caroline Scott
Jan 28, 2017

Fhatuwani Musinyali
Mar 14, 2017

Featured Articles

Trending Articles

Watch Articles

- Terms of Use
- Privacy Policy
- Do Not Sell or Share My Info
- Not Selling Info
wikiHow Tech Help Pro:
Develop the tech skills you need for work and life
- Privacy Policy
Buy Me a Coffee

Home » Research Methods – Types, Examples and Guide
Research Methods – Types, Examples and Guide
Table of Contents

Research Methods
Definition:
Research Methods refer to the techniques, procedures, and processes used by researchers to collect , analyze, and interpret data in order to answer research questions or test hypotheses. The methods used in research can vary depending on the research questions, the type of data that is being collected, and the research design.
Types of Research Methods
Types of Research Methods are as follows:
Qualitative research Method
Qualitative research methods are used to collect and analyze non-numerical data. This type of research is useful when the objective is to explore the meaning of phenomena, understand the experiences of individuals, or gain insights into complex social processes. Qualitative research methods include interviews, focus groups, ethnography, and content analysis.
Quantitative Research Method
Quantitative research methods are used to collect and analyze numerical data. This type of research is useful when the objective is to test a hypothesis, determine cause-and-effect relationships, and measure the prevalence of certain phenomena. Quantitative research methods include surveys, experiments, and secondary data analysis.
Mixed Method Research
Mixed Method Research refers to the combination of both qualitative and quantitative research methods in a single study. This approach aims to overcome the limitations of each individual method and to provide a more comprehensive understanding of the research topic. This approach allows researchers to gather both quantitative data, which is often used to test hypotheses and make generalizations about a population, and qualitative data, which provides a more in-depth understanding of the experiences and perspectives of individuals.
Key Differences Between Research Methods
The following Table shows the key differences between Quantitative, Qualitative and Mixed Research Methods
Examples of Research Methods
Examples of Research Methods are as follows:
Qualitative Research Example:
A researcher wants to study the experience of cancer patients during their treatment. They conduct in-depth interviews with patients to gather data on their emotional state, coping mechanisms, and support systems.
Quantitative Research Example:
A company wants to determine the effectiveness of a new advertisement campaign. They survey a large group of people, asking them to rate their awareness of the product and their likelihood of purchasing it.
Mixed Research Example:
A university wants to evaluate the effectiveness of a new teaching method in improving student performance. They collect both quantitative data (such as test scores) and qualitative data (such as feedback from students and teachers) to get a complete picture of the impact of the new method.
Applications of Research Methods
Research methods are used in various fields to investigate, analyze, and answer research questions. Here are some examples of how research methods are applied in different fields:
- Psychology : Research methods are widely used in psychology to study human behavior, emotions, and mental processes. For example, researchers may use experiments, surveys, and observational studies to understand how people behave in different situations, how they respond to different stimuli, and how their brains process information.
- Sociology : Sociologists use research methods to study social phenomena, such as social inequality, social change, and social relationships. Researchers may use surveys, interviews, and observational studies to collect data on social attitudes, beliefs, and behaviors.
- Medicine : Research methods are essential in medical research to study diseases, test new treatments, and evaluate their effectiveness. Researchers may use clinical trials, case studies, and laboratory experiments to collect data on the efficacy and safety of different medical treatments.
- Education : Research methods are used in education to understand how students learn, how teachers teach, and how educational policies affect student outcomes. Researchers may use surveys, experiments, and observational studies to collect data on student performance, teacher effectiveness, and educational programs.
- Business : Research methods are used in business to understand consumer behavior, market trends, and business strategies. Researchers may use surveys, focus groups, and observational studies to collect data on consumer preferences, market trends, and industry competition.
- Environmental science : Research methods are used in environmental science to study the natural world and its ecosystems. Researchers may use field studies, laboratory experiments, and observational studies to collect data on environmental factors, such as air and water quality, and the impact of human activities on the environment.
- Political science : Research methods are used in political science to study political systems, institutions, and behavior. Researchers may use surveys, experiments, and observational studies to collect data on political attitudes, voting behavior, and the impact of policies on society.
Purpose of Research Methods
Research methods serve several purposes, including:
- Identify research problems: Research methods are used to identify research problems or questions that need to be addressed through empirical investigation.
- Develop hypotheses: Research methods help researchers develop hypotheses, which are tentative explanations for the observed phenomenon or relationship.
- Collect data: Research methods enable researchers to collect data in a systematic and objective way, which is necessary to test hypotheses and draw meaningful conclusions.
- Analyze data: Research methods provide tools and techniques for analyzing data, such as statistical analysis, content analysis, and discourse analysis.
- Test hypotheses: Research methods allow researchers to test hypotheses by examining the relationships between variables in a systematic and controlled manner.
- Draw conclusions : Research methods facilitate the drawing of conclusions based on empirical evidence and help researchers make generalizations about a population based on their sample data.
- Enhance understanding: Research methods contribute to the development of knowledge and enhance our understanding of various phenomena and relationships, which can inform policy, practice, and theory.
When to Use Research Methods
Research methods are used when you need to gather information or data to answer a question or to gain insights into a particular phenomenon.
Here are some situations when research methods may be appropriate:
- To investigate a problem : Research methods can be used to investigate a problem or a research question in a particular field. This can help in identifying the root cause of the problem and developing solutions.
- To gather data: Research methods can be used to collect data on a particular subject. This can be done through surveys, interviews, observations, experiments, and more.
- To evaluate programs : Research methods can be used to evaluate the effectiveness of a program, intervention, or policy. This can help in determining whether the program is meeting its goals and objectives.
- To explore new areas : Research methods can be used to explore new areas of inquiry or to test new hypotheses. This can help in advancing knowledge in a particular field.
- To make informed decisions : Research methods can be used to gather information and data to support informed decision-making. This can be useful in various fields such as healthcare, business, and education.
Advantages of Research Methods
Research methods provide several advantages, including:
- Objectivity : Research methods enable researchers to gather data in a systematic and objective manner, minimizing personal biases and subjectivity. This leads to more reliable and valid results.
- Replicability : A key advantage of research methods is that they allow for replication of studies by other researchers. This helps to confirm the validity of the findings and ensures that the results are not specific to the particular research team.
- Generalizability : Research methods enable researchers to gather data from a representative sample of the population, allowing for generalizability of the findings to a larger population. This increases the external validity of the research.
- Precision : Research methods enable researchers to gather data using standardized procedures, ensuring that the data is accurate and precise. This allows researchers to make accurate predictions and draw meaningful conclusions.
- Efficiency : Research methods enable researchers to gather data efficiently, saving time and resources. This is especially important when studying large populations or complex phenomena.
- Innovation : Research methods enable researchers to develop new techniques and tools for data collection and analysis, leading to innovation and advancement in the field.
About the author
Muhammad Hassan
Researcher, Academic Writer, Web developer
You may also like

Case Study – Methods, Examples and Guide

Research Paper Conclusion – Writing Guide and...

Appendices – Writing Guide, Types and Examples

Research Report – Example, Writing Guide and...

Delimitations in Research – Types, Examples and...

Scope of the Research – Writing Guide and...

An official website of the United States government
The .gov means it’s official. Federal government websites often end in .gov or .mil. Before sharing sensitive information, make sure you’re on a federal government site.
The site is secure. The https:// ensures that you are connecting to the official website and that any information you provide is encrypted and transmitted securely.
- Publications
- Account settings
Preview improvements coming to the PMC website in October 2024. Learn More or Try it out now .
- Advanced Search
- Journal List
- Neurol Res Pract

How to use and assess qualitative research methods
Loraine busetto.
1 Department of Neurology, Heidelberg University Hospital, Im Neuenheimer Feld 400, 69120 Heidelberg, Germany
Wolfgang Wick
2 Clinical Cooperation Unit Neuro-Oncology, German Cancer Research Center, Heidelberg, Germany
Christoph Gumbinger
Associated data.
Not applicable.
This paper aims to provide an overview of the use and assessment of qualitative research methods in the health sciences. Qualitative research can be defined as the study of the nature of phenomena and is especially appropriate for answering questions of why something is (not) observed, assessing complex multi-component interventions, and focussing on intervention improvement. The most common methods of data collection are document study, (non-) participant observations, semi-structured interviews and focus groups. For data analysis, field-notes and audio-recordings are transcribed into protocols and transcripts, and coded using qualitative data management software. Criteria such as checklists, reflexivity, sampling strategies, piloting, co-coding, member-checking and stakeholder involvement can be used to enhance and assess the quality of the research conducted. Using qualitative in addition to quantitative designs will equip us with better tools to address a greater range of research problems, and to fill in blind spots in current neurological research and practice.
The aim of this paper is to provide an overview of qualitative research methods, including hands-on information on how they can be used, reported and assessed. This article is intended for beginning qualitative researchers in the health sciences as well as experienced quantitative researchers who wish to broaden their understanding of qualitative research.
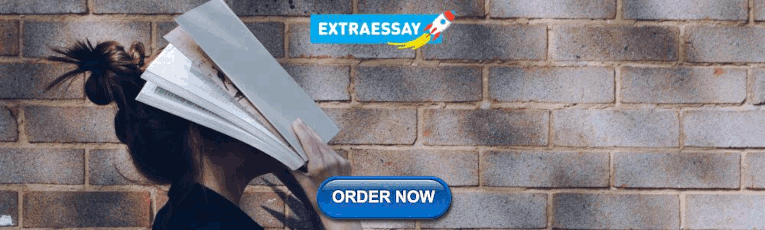
What is qualitative research?
Qualitative research is defined as “the study of the nature of phenomena”, including “their quality, different manifestations, the context in which they appear or the perspectives from which they can be perceived” , but excluding “their range, frequency and place in an objectively determined chain of cause and effect” [ 1 ]. This formal definition can be complemented with a more pragmatic rule of thumb: qualitative research generally includes data in form of words rather than numbers [ 2 ].
Why conduct qualitative research?
Because some research questions cannot be answered using (only) quantitative methods. For example, one Australian study addressed the issue of why patients from Aboriginal communities often present late or not at all to specialist services offered by tertiary care hospitals. Using qualitative interviews with patients and staff, it found one of the most significant access barriers to be transportation problems, including some towns and communities simply not having a bus service to the hospital [ 3 ]. A quantitative study could have measured the number of patients over time or even looked at possible explanatory factors – but only those previously known or suspected to be of relevance. To discover reasons for observed patterns, especially the invisible or surprising ones, qualitative designs are needed.
While qualitative research is common in other fields, it is still relatively underrepresented in health services research. The latter field is more traditionally rooted in the evidence-based-medicine paradigm, as seen in " research that involves testing the effectiveness of various strategies to achieve changes in clinical practice, preferably applying randomised controlled trial study designs (...) " [ 4 ]. This focus on quantitative research and specifically randomised controlled trials (RCT) is visible in the idea of a hierarchy of research evidence which assumes that some research designs are objectively better than others, and that choosing a "lesser" design is only acceptable when the better ones are not practically or ethically feasible [ 5 , 6 ]. Others, however, argue that an objective hierarchy does not exist, and that, instead, the research design and methods should be chosen to fit the specific research question at hand – "questions before methods" [ 2 , 7 – 9 ]. This means that even when an RCT is possible, some research problems require a different design that is better suited to addressing them. Arguing in JAMA, Berwick uses the example of rapid response teams in hospitals, which he describes as " a complex, multicomponent intervention – essentially a process of social change" susceptible to a range of different context factors including leadership or organisation history. According to him, "[in] such complex terrain, the RCT is an impoverished way to learn. Critics who use it as a truth standard in this context are incorrect" [ 8 ] . Instead of limiting oneself to RCTs, Berwick recommends embracing a wider range of methods , including qualitative ones, which for "these specific applications, (...) are not compromises in learning how to improve; they are superior" [ 8 ].
Research problems that can be approached particularly well using qualitative methods include assessing complex multi-component interventions or systems (of change), addressing questions beyond “what works”, towards “what works for whom when, how and why”, and focussing on intervention improvement rather than accreditation [ 7 , 9 – 12 ]. Using qualitative methods can also help shed light on the “softer” side of medical treatment. For example, while quantitative trials can measure the costs and benefits of neuro-oncological treatment in terms of survival rates or adverse effects, qualitative research can help provide a better understanding of patient or caregiver stress, visibility of illness or out-of-pocket expenses.
How to conduct qualitative research?
Given that qualitative research is characterised by flexibility, openness and responsivity to context, the steps of data collection and analysis are not as separate and consecutive as they tend to be in quantitative research [ 13 , 14 ]. As Fossey puts it : “sampling, data collection, analysis and interpretation are related to each other in a cyclical (iterative) manner, rather than following one after another in a stepwise approach” [ 15 ]. The researcher can make educated decisions with regard to the choice of method, how they are implemented, and to which and how many units they are applied [ 13 ]. As shown in Fig. 1 , this can involve several back-and-forth steps between data collection and analysis where new insights and experiences can lead to adaption and expansion of the original plan. Some insights may also necessitate a revision of the research question and/or the research design as a whole. The process ends when saturation is achieved, i.e. when no relevant new information can be found (see also below: sampling and saturation). For reasons of transparency, it is essential for all decisions as well as the underlying reasoning to be well-documented.

Iterative research process
While it is not always explicitly addressed, qualitative methods reflect a different underlying research paradigm than quantitative research (e.g. constructivism or interpretivism as opposed to positivism). The choice of methods can be based on the respective underlying substantive theory or theoretical framework used by the researcher [ 2 ].
Data collection
The methods of qualitative data collection most commonly used in health research are document study, observations, semi-structured interviews and focus groups [ 1 , 14 , 16 , 17 ].
Document study
Document study (also called document analysis) refers to the review by the researcher of written materials [ 14 ]. These can include personal and non-personal documents such as archives, annual reports, guidelines, policy documents, diaries or letters.
Observations
Observations are particularly useful to gain insights into a certain setting and actual behaviour – as opposed to reported behaviour or opinions [ 13 ]. Qualitative observations can be either participant or non-participant in nature. In participant observations, the observer is part of the observed setting, for example a nurse working in an intensive care unit [ 18 ]. In non-participant observations, the observer is “on the outside looking in”, i.e. present in but not part of the situation, trying not to influence the setting by their presence. Observations can be planned (e.g. for 3 h during the day or night shift) or ad hoc (e.g. as soon as a stroke patient arrives at the emergency room). During the observation, the observer takes notes on everything or certain pre-determined parts of what is happening around them, for example focusing on physician-patient interactions or communication between different professional groups. Written notes can be taken during or after the observations, depending on feasibility (which is usually lower during participant observations) and acceptability (e.g. when the observer is perceived to be judging the observed). Afterwards, these field notes are transcribed into observation protocols. If more than one observer was involved, field notes are taken independently, but notes can be consolidated into one protocol after discussions. Advantages of conducting observations include minimising the distance between the researcher and the researched, the potential discovery of topics that the researcher did not realise were relevant and gaining deeper insights into the real-world dimensions of the research problem at hand [ 18 ].
Semi-structured interviews
Hijmans & Kuyper describe qualitative interviews as “an exchange with an informal character, a conversation with a goal” [ 19 ]. Interviews are used to gain insights into a person’s subjective experiences, opinions and motivations – as opposed to facts or behaviours [ 13 ]. Interviews can be distinguished by the degree to which they are structured (i.e. a questionnaire), open (e.g. free conversation or autobiographical interviews) or semi-structured [ 2 , 13 ]. Semi-structured interviews are characterized by open-ended questions and the use of an interview guide (or topic guide/list) in which the broad areas of interest, sometimes including sub-questions, are defined [ 19 ]. The pre-defined topics in the interview guide can be derived from the literature, previous research or a preliminary method of data collection, e.g. document study or observations. The topic list is usually adapted and improved at the start of the data collection process as the interviewer learns more about the field [ 20 ]. Across interviews the focus on the different (blocks of) questions may differ and some questions may be skipped altogether (e.g. if the interviewee is not able or willing to answer the questions or for concerns about the total length of the interview) [ 20 ]. Qualitative interviews are usually not conducted in written format as it impedes on the interactive component of the method [ 20 ]. In comparison to written surveys, qualitative interviews have the advantage of being interactive and allowing for unexpected topics to emerge and to be taken up by the researcher. This can also help overcome a provider or researcher-centred bias often found in written surveys, which by nature, can only measure what is already known or expected to be of relevance to the researcher. Interviews can be audio- or video-taped; but sometimes it is only feasible or acceptable for the interviewer to take written notes [ 14 , 16 , 20 ].
Focus groups
Focus groups are group interviews to explore participants’ expertise and experiences, including explorations of how and why people behave in certain ways [ 1 ]. Focus groups usually consist of 6–8 people and are led by an experienced moderator following a topic guide or “script” [ 21 ]. They can involve an observer who takes note of the non-verbal aspects of the situation, possibly using an observation guide [ 21 ]. Depending on researchers’ and participants’ preferences, the discussions can be audio- or video-taped and transcribed afterwards [ 21 ]. Focus groups are useful for bringing together homogeneous (to a lesser extent heterogeneous) groups of participants with relevant expertise and experience on a given topic on which they can share detailed information [ 21 ]. Focus groups are a relatively easy, fast and inexpensive method to gain access to information on interactions in a given group, i.e. “the sharing and comparing” among participants [ 21 ]. Disadvantages include less control over the process and a lesser extent to which each individual may participate. Moreover, focus group moderators need experience, as do those tasked with the analysis of the resulting data. Focus groups can be less appropriate for discussing sensitive topics that participants might be reluctant to disclose in a group setting [ 13 ]. Moreover, attention must be paid to the emergence of “groupthink” as well as possible power dynamics within the group, e.g. when patients are awed or intimidated by health professionals.
Choosing the “right” method
As explained above, the school of thought underlying qualitative research assumes no objective hierarchy of evidence and methods. This means that each choice of single or combined methods has to be based on the research question that needs to be answered and a critical assessment with regard to whether or to what extent the chosen method can accomplish this – i.e. the “fit” between question and method [ 14 ]. It is necessary for these decisions to be documented when they are being made, and to be critically discussed when reporting methods and results.
Let us assume that our research aim is to examine the (clinical) processes around acute endovascular treatment (EVT), from the patient’s arrival at the emergency room to recanalization, with the aim to identify possible causes for delay and/or other causes for sub-optimal treatment outcome. As a first step, we could conduct a document study of the relevant standard operating procedures (SOPs) for this phase of care – are they up-to-date and in line with current guidelines? Do they contain any mistakes, irregularities or uncertainties that could cause delays or other problems? Regardless of the answers to these questions, the results have to be interpreted based on what they are: a written outline of what care processes in this hospital should look like. If we want to know what they actually look like in practice, we can conduct observations of the processes described in the SOPs. These results can (and should) be analysed in themselves, but also in comparison to the results of the document analysis, especially as regards relevant discrepancies. Do the SOPs outline specific tests for which no equipment can be observed or tasks to be performed by specialized nurses who are not present during the observation? It might also be possible that the written SOP is outdated, but the actual care provided is in line with current best practice. In order to find out why these discrepancies exist, it can be useful to conduct interviews. Are the physicians simply not aware of the SOPs (because their existence is limited to the hospital’s intranet) or do they actively disagree with them or does the infrastructure make it impossible to provide the care as described? Another rationale for adding interviews is that some situations (or all of their possible variations for different patient groups or the day, night or weekend shift) cannot practically or ethically be observed. In this case, it is possible to ask those involved to report on their actions – being aware that this is not the same as the actual observation. A senior physician’s or hospital manager’s description of certain situations might differ from a nurse’s or junior physician’s one, maybe because they intentionally misrepresent facts or maybe because different aspects of the process are visible or important to them. In some cases, it can also be relevant to consider to whom the interviewee is disclosing this information – someone they trust, someone they are otherwise not connected to, or someone they suspect or are aware of being in a potentially “dangerous” power relationship to them. Lastly, a focus group could be conducted with representatives of the relevant professional groups to explore how and why exactly they provide care around EVT. The discussion might reveal discrepancies (between SOPs and actual care or between different physicians) and motivations to the researchers as well as to the focus group members that they might not have been aware of themselves. For the focus group to deliver relevant information, attention has to be paid to its composition and conduct, for example, to make sure that all participants feel safe to disclose sensitive or potentially problematic information or that the discussion is not dominated by (senior) physicians only. The resulting combination of data collection methods is shown in Fig. 2 .

Possible combination of data collection methods
Attributions for icons: “Book” by Serhii Smirnov, “Interview” by Adrien Coquet, FR, “Magnifying Glass” by anggun, ID, “Business communication” by Vectors Market; all from the Noun Project
The combination of multiple data source as described for this example can be referred to as “triangulation”, in which multiple measurements are carried out from different angles to achieve a more comprehensive understanding of the phenomenon under study [ 22 , 23 ].
Data analysis
To analyse the data collected through observations, interviews and focus groups these need to be transcribed into protocols and transcripts (see Fig. 3 ). Interviews and focus groups can be transcribed verbatim , with or without annotations for behaviour (e.g. laughing, crying, pausing) and with or without phonetic transcription of dialects and filler words, depending on what is expected or known to be relevant for the analysis. In the next step, the protocols and transcripts are coded , that is, marked (or tagged, labelled) with one or more short descriptors of the content of a sentence or paragraph [ 2 , 15 , 23 ]. Jansen describes coding as “connecting the raw data with “theoretical” terms” [ 20 ]. In a more practical sense, coding makes raw data sortable. This makes it possible to extract and examine all segments describing, say, a tele-neurology consultation from multiple data sources (e.g. SOPs, emergency room observations, staff and patient interview). In a process of synthesis and abstraction, the codes are then grouped, summarised and/or categorised [ 15 , 20 ]. The end product of the coding or analysis process is a descriptive theory of the behavioural pattern under investigation [ 20 ]. The coding process is performed using qualitative data management software, the most common ones being InVivo, MaxQDA and Atlas.ti. It should be noted that these are data management tools which support the analysis performed by the researcher(s) [ 14 ].

From data collection to data analysis
Attributions for icons: see Fig. Fig.2, 2 , also “Speech to text” by Trevor Dsouza, “Field Notes” by Mike O’Brien, US, “Voice Record” by ProSymbols, US, “Inspection” by Made, AU, and “Cloud” by Graphic Tigers; all from the Noun Project
How to report qualitative research?
Protocols of qualitative research can be published separately and in advance of the study results. However, the aim is not the same as in RCT protocols, i.e. to pre-define and set in stone the research questions and primary or secondary endpoints. Rather, it is a way to describe the research methods in detail, which might not be possible in the results paper given journals’ word limits. Qualitative research papers are usually longer than their quantitative counterparts to allow for deep understanding and so-called “thick description”. In the methods section, the focus is on transparency of the methods used, including why, how and by whom they were implemented in the specific study setting, so as to enable a discussion of whether and how this may have influenced data collection, analysis and interpretation. The results section usually starts with a paragraph outlining the main findings, followed by more detailed descriptions of, for example, the commonalities, discrepancies or exceptions per category [ 20 ]. Here it is important to support main findings by relevant quotations, which may add information, context, emphasis or real-life examples [ 20 , 23 ]. It is subject to debate in the field whether it is relevant to state the exact number or percentage of respondents supporting a certain statement (e.g. “Five interviewees expressed negative feelings towards XYZ”) [ 21 ].
How to combine qualitative with quantitative research?
Qualitative methods can be combined with other methods in multi- or mixed methods designs, which “[employ] two or more different methods [ …] within the same study or research program rather than confining the research to one single method” [ 24 ]. Reasons for combining methods can be diverse, including triangulation for corroboration of findings, complementarity for illustration and clarification of results, expansion to extend the breadth and range of the study, explanation of (unexpected) results generated with one method with the help of another, or offsetting the weakness of one method with the strength of another [ 1 , 17 , 24 – 26 ]. The resulting designs can be classified according to when, why and how the different quantitative and/or qualitative data strands are combined. The three most common types of mixed method designs are the convergent parallel design , the explanatory sequential design and the exploratory sequential design. The designs with examples are shown in Fig. 4 .

Three common mixed methods designs
In the convergent parallel design, a qualitative study is conducted in parallel to and independently of a quantitative study, and the results of both studies are compared and combined at the stage of interpretation of results. Using the above example of EVT provision, this could entail setting up a quantitative EVT registry to measure process times and patient outcomes in parallel to conducting the qualitative research outlined above, and then comparing results. Amongst other things, this would make it possible to assess whether interview respondents’ subjective impressions of patients receiving good care match modified Rankin Scores at follow-up, or whether observed delays in care provision are exceptions or the rule when compared to door-to-needle times as documented in the registry. In the explanatory sequential design, a quantitative study is carried out first, followed by a qualitative study to help explain the results from the quantitative study. This would be an appropriate design if the registry alone had revealed relevant delays in door-to-needle times and the qualitative study would be used to understand where and why these occurred, and how they could be improved. In the exploratory design, the qualitative study is carried out first and its results help informing and building the quantitative study in the next step [ 26 ]. If the qualitative study around EVT provision had shown a high level of dissatisfaction among the staff members involved, a quantitative questionnaire investigating staff satisfaction could be set up in the next step, informed by the qualitative study on which topics dissatisfaction had been expressed. Amongst other things, the questionnaire design would make it possible to widen the reach of the research to more respondents from different (types of) hospitals, regions, countries or settings, and to conduct sub-group analyses for different professional groups.
How to assess qualitative research?
A variety of assessment criteria and lists have been developed for qualitative research, ranging in their focus and comprehensiveness [ 14 , 17 , 27 ]. However, none of these has been elevated to the “gold standard” in the field. In the following, we therefore focus on a set of commonly used assessment criteria that, from a practical standpoint, a researcher can look for when assessing a qualitative research report or paper.
Assessors should check the authors’ use of and adherence to the relevant reporting checklists (e.g. Standards for Reporting Qualitative Research (SRQR)) to make sure all items that are relevant for this type of research are addressed [ 23 , 28 ]. Discussions of quantitative measures in addition to or instead of these qualitative measures can be a sign of lower quality of the research (paper). Providing and adhering to a checklist for qualitative research contributes to an important quality criterion for qualitative research, namely transparency [ 15 , 17 , 23 ].
Reflexivity
While methodological transparency and complete reporting is relevant for all types of research, some additional criteria must be taken into account for qualitative research. This includes what is called reflexivity, i.e. sensitivity to the relationship between the researcher and the researched, including how contact was established and maintained, or the background and experience of the researcher(s) involved in data collection and analysis. Depending on the research question and population to be researched this can be limited to professional experience, but it may also include gender, age or ethnicity [ 17 , 27 ]. These details are relevant because in qualitative research, as opposed to quantitative research, the researcher as a person cannot be isolated from the research process [ 23 ]. It may influence the conversation when an interviewed patient speaks to an interviewer who is a physician, or when an interviewee is asked to discuss a gynaecological procedure with a male interviewer, and therefore the reader must be made aware of these details [ 19 ].
Sampling and saturation
The aim of qualitative sampling is for all variants of the objects of observation that are deemed relevant for the study to be present in the sample “ to see the issue and its meanings from as many angles as possible” [ 1 , 16 , 19 , 20 , 27 ] , and to ensure “information-richness [ 15 ]. An iterative sampling approach is advised, in which data collection (e.g. five interviews) is followed by data analysis, followed by more data collection to find variants that are lacking in the current sample. This process continues until no new (relevant) information can be found and further sampling becomes redundant – which is called saturation [ 1 , 15 ] . In other words: qualitative data collection finds its end point not a priori , but when the research team determines that saturation has been reached [ 29 , 30 ].
This is also the reason why most qualitative studies use deliberate instead of random sampling strategies. This is generally referred to as “ purposive sampling” , in which researchers pre-define which types of participants or cases they need to include so as to cover all variations that are expected to be of relevance, based on the literature, previous experience or theory (i.e. theoretical sampling) [ 14 , 20 ]. Other types of purposive sampling include (but are not limited to) maximum variation sampling, critical case sampling or extreme or deviant case sampling [ 2 ]. In the above EVT example, a purposive sample could include all relevant professional groups and/or all relevant stakeholders (patients, relatives) and/or all relevant times of observation (day, night and weekend shift).
Assessors of qualitative research should check whether the considerations underlying the sampling strategy were sound and whether or how researchers tried to adapt and improve their strategies in stepwise or cyclical approaches between data collection and analysis to achieve saturation [ 14 ].
Good qualitative research is iterative in nature, i.e. it goes back and forth between data collection and analysis, revising and improving the approach where necessary. One example of this are pilot interviews, where different aspects of the interview (especially the interview guide, but also, for example, the site of the interview or whether the interview can be audio-recorded) are tested with a small number of respondents, evaluated and revised [ 19 ]. In doing so, the interviewer learns which wording or types of questions work best, or which is the best length of an interview with patients who have trouble concentrating for an extended time. Of course, the same reasoning applies to observations or focus groups which can also be piloted.
Ideally, coding should be performed by at least two researchers, especially at the beginning of the coding process when a common approach must be defined, including the establishment of a useful coding list (or tree), and when a common meaning of individual codes must be established [ 23 ]. An initial sub-set or all transcripts can be coded independently by the coders and then compared and consolidated after regular discussions in the research team. This is to make sure that codes are applied consistently to the research data.
Member checking
Member checking, also called respondent validation , refers to the practice of checking back with study respondents to see if the research is in line with their views [ 14 , 27 ]. This can happen after data collection or analysis or when first results are available [ 23 ]. For example, interviewees can be provided with (summaries of) their transcripts and asked whether they believe this to be a complete representation of their views or whether they would like to clarify or elaborate on their responses [ 17 ]. Respondents’ feedback on these issues then becomes part of the data collection and analysis [ 27 ].
Stakeholder involvement
In those niches where qualitative approaches have been able to evolve and grow, a new trend has seen the inclusion of patients and their representatives not only as study participants (i.e. “members”, see above) but as consultants to and active participants in the broader research process [ 31 – 33 ]. The underlying assumption is that patients and other stakeholders hold unique perspectives and experiences that add value beyond their own single story, making the research more relevant and beneficial to researchers, study participants and (future) patients alike [ 34 , 35 ]. Using the example of patients on or nearing dialysis, a recent scoping review found that 80% of clinical research did not address the top 10 research priorities identified by patients and caregivers [ 32 , 36 ]. In this sense, the involvement of the relevant stakeholders, especially patients and relatives, is increasingly being seen as a quality indicator in and of itself.
How not to assess qualitative research
The above overview does not include certain items that are routine in assessments of quantitative research. What follows is a non-exhaustive, non-representative, experience-based list of the quantitative criteria often applied to the assessment of qualitative research, as well as an explanation of the limited usefulness of these endeavours.
Protocol adherence
Given the openness and flexibility of qualitative research, it should not be assessed by how well it adheres to pre-determined and fixed strategies – in other words: its rigidity. Instead, the assessor should look for signs of adaptation and refinement based on lessons learned from earlier steps in the research process.
Sample size
For the reasons explained above, qualitative research does not require specific sample sizes, nor does it require that the sample size be determined a priori [ 1 , 14 , 27 , 37 – 39 ]. Sample size can only be a useful quality indicator when related to the research purpose, the chosen methodology and the composition of the sample, i.e. who was included and why.
Randomisation
While some authors argue that randomisation can be used in qualitative research, this is not commonly the case, as neither its feasibility nor its necessity or usefulness has been convincingly established for qualitative research [ 13 , 27 ]. Relevant disadvantages include the negative impact of a too large sample size as well as the possibility (or probability) of selecting “ quiet, uncooperative or inarticulate individuals ” [ 17 ]. Qualitative studies do not use control groups, either.
Interrater reliability, variability and other “objectivity checks”
The concept of “interrater reliability” is sometimes used in qualitative research to assess to which extent the coding approach overlaps between the two co-coders. However, it is not clear what this measure tells us about the quality of the analysis [ 23 ]. This means that these scores can be included in qualitative research reports, preferably with some additional information on what the score means for the analysis, but it is not a requirement. Relatedly, it is not relevant for the quality or “objectivity” of qualitative research to separate those who recruited the study participants and collected and analysed the data. Experiences even show that it might be better to have the same person or team perform all of these tasks [ 20 ]. First, when researchers introduce themselves during recruitment this can enhance trust when the interview takes place days or weeks later with the same researcher. Second, when the audio-recording is transcribed for analysis, the researcher conducting the interviews will usually remember the interviewee and the specific interview situation during data analysis. This might be helpful in providing additional context information for interpretation of data, e.g. on whether something might have been meant as a joke [ 18 ].
Not being quantitative research
Being qualitative research instead of quantitative research should not be used as an assessment criterion if it is used irrespectively of the research problem at hand. Similarly, qualitative research should not be required to be combined with quantitative research per se – unless mixed methods research is judged as inherently better than single-method research. In this case, the same criterion should be applied for quantitative studies without a qualitative component.
The main take-away points of this paper are summarised in Table Table1. 1 . We aimed to show that, if conducted well, qualitative research can answer specific research questions that cannot to be adequately answered using (only) quantitative designs. Seeing qualitative and quantitative methods as equal will help us become more aware and critical of the “fit” between the research problem and our chosen methods: I can conduct an RCT to determine the reasons for transportation delays of acute stroke patients – but should I? It also provides us with a greater range of tools to tackle a greater range of research problems more appropriately and successfully, filling in the blind spots on one half of the methodological spectrum to better address the whole complexity of neurological research and practice.
Take-away-points
Acknowledgements
Abbreviations, authors’ contributions.
LB drafted the manuscript; WW and CG revised the manuscript; all authors approved the final versions.
no external funding.
Availability of data and materials
Ethics approval and consent to participate, consent for publication, competing interests.
The authors declare no competing interests.
Publisher’s Note
Springer Nature remains neutral with regard to jurisdictional claims in published maps and institutional affiliations.
How To Write A Research Paper
Research Paper Methods Section

How To Write The Methods Section of a Research Paper Step-by-Step
13 min read
Published on: Mar 6, 2024
Last updated on: Mar 5, 2024
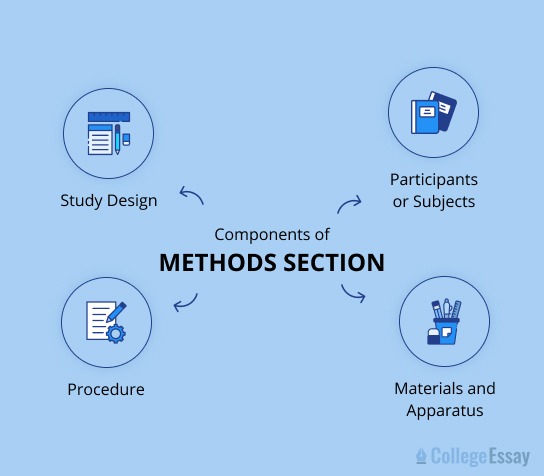
People also read
How to Write a Research Paper Step by Step
How to Write a Proposal For a Research Paper in 10 Steps
A Comprehensive Guide to Creating a Research Paper Outline
Types of Research - Methodologies and Characteristics
300+ Engaging Research Paper Topics to Get You Started
Interesting Psychology Research Topics & Ideas
Qualitative Research - Types, Methods & Examples
Understanding Quantitative Research - Definition, Types, Examples, And More
Research Paper Example - Examples for Different Formats
How To Start A Research Paper - Steps With Examples
How to Write an Abstract That Captivates Your Readers
How To Write a Literature Review for a Research Paper | Steps & Examples
Types of Qualitative Research Methods - An Overview
Understanding Qualitative vs. Quantitative Research - A Complete Guide
How to Cite a Research Paper in Different Citation Styles
Easy Sociology Research Topics for Your Next Project
200+ Outstanding History Research Paper Topics With Expert Tips
How To Write a Hypothesis in a Research Paper | Steps & Examples
How to Write an Introduction for a Research Paper - A Step-by-Step Guide
How to Write a Good Research Paper Title
How to Write a Conclusion for a Research Paper in 3 Simple Steps
How to Write an Abstract For a Research Paper with Examples
How To Write a Thesis For a Research Paper Step by Step
How to Write a Discussion For a Research Paper | Objectives, Steps & Examples
How to Write the Results Section of a Research Paper - Structure and Tips
How to Write a Problem Statement for a Research Paper in 6 Steps
Share this article
The method and material section stands as the cornerstone of any research paper. Crafting this section with precision is important, especially when aiming for a target journal.
If you're navigating the intricacies of research paper writing and pondering on how to ace the methodology, fear not – we've got you covered. Our guide will walk you through the essentials, ensuring your methodology shines in the eyes of your target journal.
Let's jump into the basics of the method section!
On This Page On This Page -->
What is the Methods Section of a Research Paper?
The methods section of a research paper provides a detailed description of the procedures, techniques, and methods employed to conduct the study ( American Psychological Association, 2020 ). It outlines the steps taken to collect, analyze, and interpret data, allowing other researchers to replicate the study and assess the validity of the results.
This section includes information on the study design, participants, materials or apparatus used, data collection procedures, and statistical analyses. Typically, the methodology section is placed after the introduction and before the results section in a research paper.
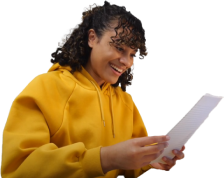
Tough Essay Due? Hire Tough Writers!
Importance of Methods Section
The methods section of a research paper holds significant importance. Here is why:
- Replicability: The methods section ensures the replicability of the study by providing a clear and comprehensive account of the procedures used.
- Transparency: It enhances transparency, allowing other researchers to understand and evaluate the validity of the study's findings.
- Credibility: A well-documented methods section enhances the credibility of the research, instilling confidence in the study's design and execution.
- Guidance for Future Research: It serves as a guide for future research, offering insights into methodologies that can be applied or modified in similar studies.
- Ethical Considerations: The section highlights ethical considerations, promoting responsible and accountable research practices.
Structure of Methods Section of a Research Paper
There are some important parts of the method section of a research paper that you will need to include, whether you have done an experimental study or a descriptive study.
Provided structured approach below ensures clarity and replicability of the research methodology:
Formatting of the Methods Section
Make the main " Methods " heading centered, bold, and capitalized. For subtopics under "Methods," like participant details or data collection, use left-aligned, bold, and title cases.
Feel free to include even sub-headings for more specifics. This formatting helps readers easily follow your study steps.
Next, we will address the most common query, i.e., how to write the methodology section of a research paper. Letâs explain the steps for writing the methodology section of a research paper:
Step 1: Start with Study Design
The initial step in the method section of a research paper is to provide a clear description of the study type. This involves outlining the overall plan and structure of the research.
Different types of studies, such as cohort, case-control, and cross-sectional, may be employed based on the research objectives.
For instance:
Starting with the study design sets the stage for understanding the methodology. It provides readers with a foundation for subsequent sections in the methods portion of the research paper.
Step 2: Describe Participants
In the methods section, the second step involves providing a detailed account of the participants involved in the study. Start by describing the characteristics of both human and non-human subjects, using clear and descriptive language.
Address specific demographic characteristics relevant to your study, such as age, sex, ethnic or racial group, gender identity, education level, and socioeconomic status. Clearly outlining these essential details ensures transparency, replicability, and a comprehensive understanding of the study's sample.
Sampling Procedures:
- Clearly outline how participants were selected, specifying any inclusion and exclusion criteria applied.
- Appropriately identify the sampling procedure used, such as random sampling, convenience sampling, or stratified sampling.
- If applicable, note the percentage of invited participants who actually participated.
- Specify if participants were self-selected or chosen by their institutions (e.g., schools submitting student data).
Sample Size and Power:
- Detail the intended sample size estimation per condition and the statistical power aimed for in the study.
- Provide information on any analyses conducted to determine the sample size and power.
- Emphasize the importance of statistical power for detecting effects if present.
- State whether the final sample size differed from the originally intended sample.
- Base your interpretations of study outcomes solely on the final sample, reinforcing the importance of transparency in reporting.
Step 3: State Materials or Apparatus
In the third step, thoroughly describe the materials or apparatus used in your research. In addition, gives detailed information on the tools and techniques employed to measure relevant outcome variables.
Primary and Secondary Measures:
- Clearly define both primary and secondary outcome measures aligned with research questions.
- Specify all instruments used, citing hardware models, software versions, or references to manuals/articles.
- Report settings of specialized apparatus, such as screen resolution.
Reliability and Validity:
- For each instrument, detail measures of reliability and validity.
- Include an explanation of how consistently (reliability) and precisely (validity) the method measures the targeted variables.
- Provide examples or reference materials to illustrate the reliability and validity of tests, questionnaires, or interviews.
Covariates and Quality Assurance:
- Describe any covariates considered and their relevance to explaining or predicting outcomes.
- Review methods used to assure measurement quality, such as researcher training, multiple assessors, translation procedures, and pilot studies.
- For subjectively coded data, report interrater reliability scores to gauge consistency among raters.
Step 4 Write the Procedure
Next is the procedure section of the research paper, which thoroughly details the procedures applied for administering the study, processing data, and planning data analyses.
Data Collection Methods and Research Design
- Summarize data collection methods (e.g., surveys, tests) and the overall research design.
- Provide detailed procedures for administering surveys, tests, or any other data collection instruments.
- Clarify the research design framework, specifying whether it's experimental, quasi-experimental, descriptive, correlational, and/or longitudinal.
- For multi-group studies, report assignment methods, group instructions, interventions, and session details.
Data Analysis
- Clearly state the planned data analysis methods for each research question or hypothesis.
- Specify descriptive statistics, inferential statistical tests, and any other analysis techniques.
- Include software or tools used for data analysis (e.g., SPSS, R).
- Provide a brief rationale for choosing each analysis method.
Step 5: Mention Ethical Approvals
In the fifth step of the methods section, explicitly address the ethical considerations of your research, ensuring transparency and adherence to ethical standards. Here are some key ethical considerations:
- IRB Approval:
Clearly state that the research received approval from the Institutional Review Board (IRB) or an equivalent ethical review body.
- Informed Consent:
Specify the process of obtaining informed consent, including the provision of information sheets to participants.
- Confidentiality:
Describe measures taken to maintain confidentiality, such as assigning unique identification numbers and securing data.
- Participant Rights:
Emphasize participants' right to withdraw from the study at any point without consequences.
- Debriefing:
Mention if debriefing procedures were implemented to address any participant concerns post-study.
Methods Section of Research Paper Examples
Exploring sample methodology sections is crucial when composing your first research paper, as it enhances your understanding of the structure. We provide PDF examples of methodology sections that you can review to gain inspiration for your own research paper.
Methods Section of A Qualitative Research Paper
Methods Section of Research Paper Template
Methods Section of Research Proposal Example
Methods Section of Research Paper APA
How To Write A Method For An Experiment
Journal Guidelines to Consider
When writing the methods section, be mindful of the specific guidelines set by your target journal. These guidelines can vary, impacting the structure, word limitations, and even the presentation of your methodology.
Here's a detailed explanation, along with an example:
Structure & Word Limitations
If a journal follows APA guidelines, it might allow flexibility in structuring the method section. However, some journals may impose strict limitations on the manuscript's length and the number of subsections.
For instance, a journal might specify a maximum of 3000 words for the entire paper and limit the method section to 500 words. In such cases, ensure you adhere to these constraints, potentially submitting supplemental files for additional details.
Standardized Checklists
Journals often request authors to use standardized checklists for various study types to ensure completeness.
For a randomized clinical trial, the CONSORT(Consolidated Standards of Reporting Trials) checklist might be required. If your research involves observational studies, the STROBE (Strengthening the Reporting of Observational Studies in Epidemiology) checklist may be applicable.
For diagnostic accuracy studies, adherence to the STARD (Standards for the Reporting of Diagnostic Accuracy Studies) checklist is common. These checklists serve as a systematic way to include essential details in your manuscript, aligning with the journal's preferred reporting standards.
Blind Review Procedures
Some journals implement single- or double-blind review procedures. If a double-blind review is in place, authors need to remove any information that might reveal their identity or institutional affiliations.
For instance, the method section cannot explicitly mention the institution's name, researchers' identities, or the institutional ethics committee. This ensures an unbiased evaluation of the research without reviewers being influenced by the authors' affiliations.
The Dos And Donâts Of Writing The Methods Section
While it's important to be thorough, certain elements are better suited for other sections of the paper. Here are some Doâs and Donâts of writing the methods section:
Dos of Writing the Methods Section
Here are what to include in the methods section:
- Clarity and Precision: Clearly and concisely describe the procedures used in your study. Ensure that another researcher can replicate your work based on your explanation.
- Chronological Order: Present the methods in a logical and chronological sequence. This helps readers follow the flow of your research.
- Detail and Specificity: Provide sufficient detail to allow for replication. Specify equipment, materials, and procedures used, including any modifications.
- Consistency with Study Design: Align your methods with the overall design of your study. Clearly state whether it's experimental, observational, or another design.
- Inclusion of Participants: Detail participant characteristics, including demographics and any inclusion/exclusion criteria. Clearly state the sample size.
- Operational Definitions: Define and operationalize key variables. Clearly explain how each variable was measured or manipulated.
- Transparency in Data Collection: Describe the data collection process, including the timing, location, and any relevant protocols followed during the study.
- Statistical Information: Outline the statistical methods used for analysis. Specify the software, tests employed and significance levels.
- Ethical Considerations: Discuss ethical approvals obtained, informed consent procedures, and measures taken to ensure participant confidentiality. Address any potential conflicts of interest.
Don'ts of Writing the Methods Section
- Extraneous Details: Unlike the discussion section avoid including unnecessary details or information that does not contribute directly to understanding the research methods.
- Results Discussion: Refrain from discussing or interpreting the results in the methods section. Focus solely on describing the methods employed.
- Ambiguity and Vagueness: Steer clear of vague or ambiguous language. Be precise and specific in your descriptions.
- Overemphasis on Background: While some background information is relevant, avoid turning the methods section into an extensive literature review . Keep the focus on the research methods.
- Personal Opinions: Do not include personal opinions or anecdotes. Stick to factual and objective descriptions.
- Excessive Jargon: Minimize the use of technical jargon that may be confusing to readers who are not experts in your field. If necessary, provide clear explanations.
- Inadequate Explanation of Modifications: If you deviate from standard procedures, clearly explain the modifications and justify why they were made.
- Inconsistency with Design: Ensure that your methods align with the study design. Avoid inconsistencies that could create confusion for readers.
In conclusion , learning the art of writing the methods section is pivotal for any research paper. Following a step-by-step approach, from defining the study design to detailed data collection and analysis, ensures clarity and replicability.
Remember, precision matters. If you find yourself grappling with the intricacies of your methodology, don't hesitate to reach out to CollegeEssay.org.
Our professional writing service is ready to assist you in crafting a robust and well-structured methods section.
Connect with our research paper writing service for expert guidance and conquer the challenges of research paper writing.
Nova A. (Literature, Marketing)
As a Digital Content Strategist, Nova Allison has eight years of experience in writing both technical and scientific content. With a focus on developing online content plans that engage audiences, Nova strives to write pieces that are not only informative but captivating as well.
Paper Due? Why Suffer? That’s our Job!
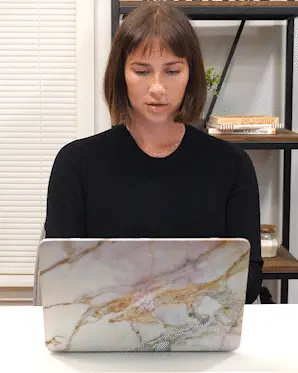
Keep reading
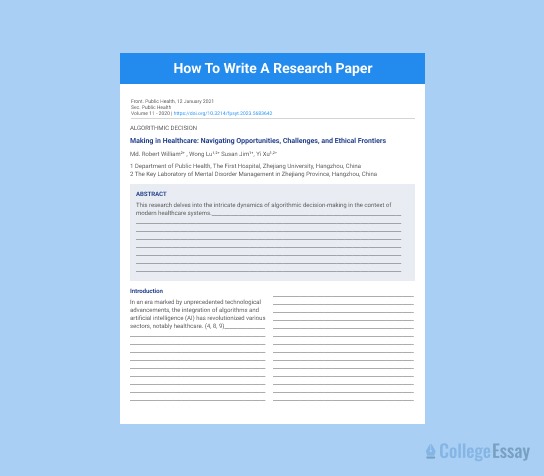
- Privacy Policy
- Cookies Policy
- Terms of Use
- Refunds & Cancellations
- Our Writers
- Success Stories
- Our Guarantees
- Affiliate Program
- Referral Program
- AI Essay Writer
Disclaimer: All client orders are completed by our team of highly qualified human writers. The essays and papers provided by us are not to be used for submission but rather as learning models only.
Have a language expert improve your writing
Run a free plagiarism check in 10 minutes, generate accurate citations for free.
- Knowledge Base
- Research paper
Writing a Research Paper Introduction | Step-by-Step Guide
Published on September 24, 2022 by Jack Caulfield . Revised on March 27, 2023.
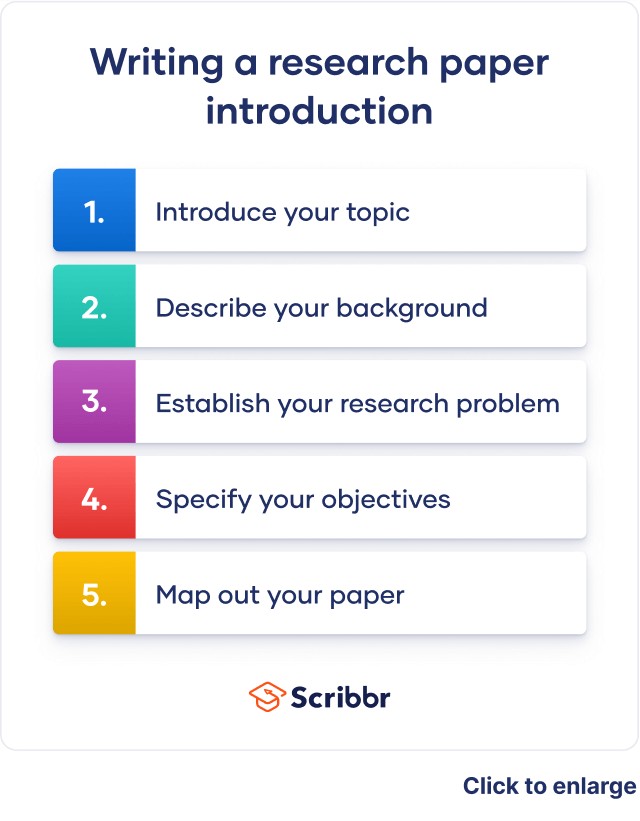
The introduction to a research paper is where you set up your topic and approach for the reader. It has several key goals:
- Present your topic and get the reader interested
- Provide background or summarize existing research
- Position your own approach
- Detail your specific research problem and problem statement
- Give an overview of the paper’s structure
The introduction looks slightly different depending on whether your paper presents the results of original empirical research or constructs an argument by engaging with a variety of sources.
Instantly correct all language mistakes in your text
Upload your document to correct all your mistakes in minutes
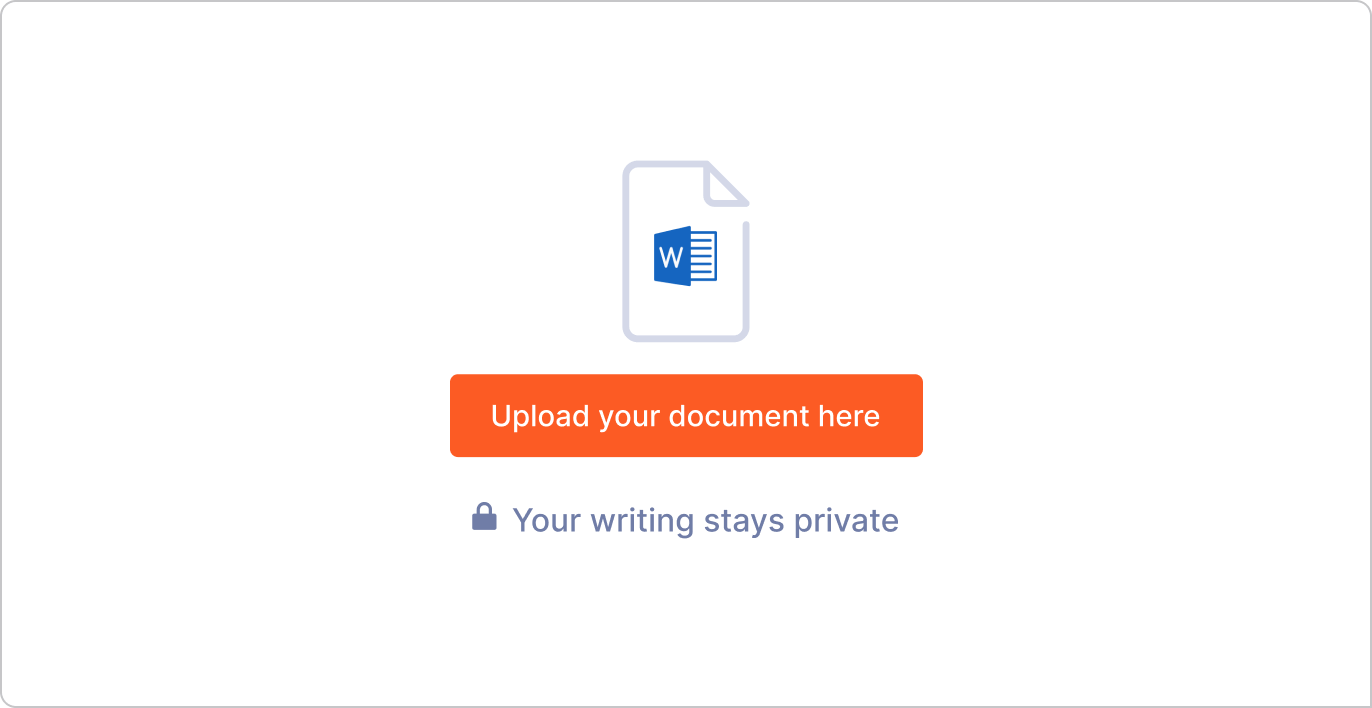
Table of contents
Step 1: introduce your topic, step 2: describe the background, step 3: establish your research problem, step 4: specify your objective(s), step 5: map out your paper, research paper introduction examples, frequently asked questions about the research paper introduction.
The first job of the introduction is to tell the reader what your topic is and why it’s interesting or important. This is generally accomplished with a strong opening hook.
The hook is a striking opening sentence that clearly conveys the relevance of your topic. Think of an interesting fact or statistic, a strong statement, a question, or a brief anecdote that will get the reader wondering about your topic.
For example, the following could be an effective hook for an argumentative paper about the environmental impact of cattle farming:
A more empirical paper investigating the relationship of Instagram use with body image issues in adolescent girls might use the following hook:
Don’t feel that your hook necessarily has to be deeply impressive or creative. Clarity and relevance are still more important than catchiness. The key thing is to guide the reader into your topic and situate your ideas.
Receive feedback on language, structure, and formatting
Professional editors proofread and edit your paper by focusing on:
- Academic style
- Vague sentences
- Style consistency
See an example
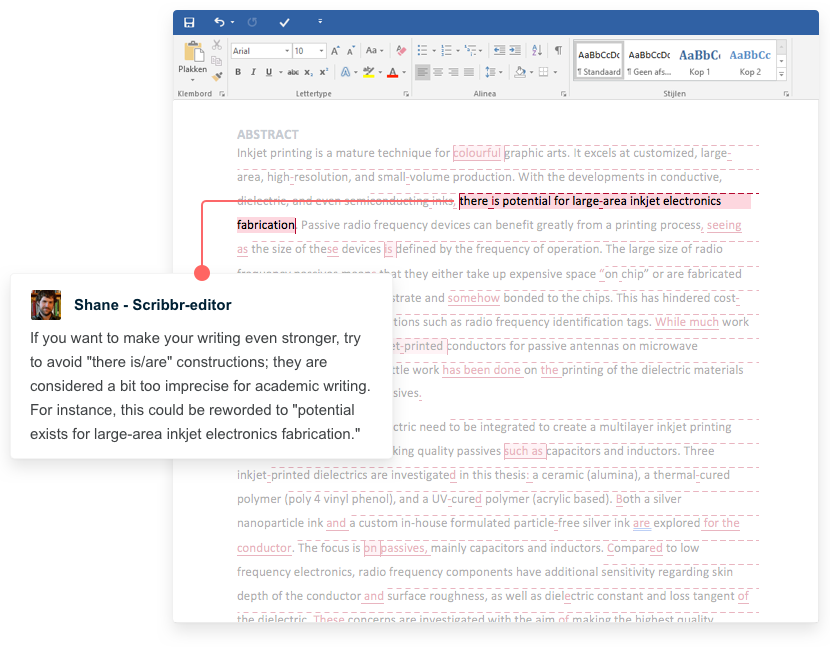
This part of the introduction differs depending on what approach your paper is taking.
In a more argumentative paper, you’ll explore some general background here. In a more empirical paper, this is the place to review previous research and establish how yours fits in.
Argumentative paper: Background information
After you’ve caught your reader’s attention, specify a bit more, providing context and narrowing down your topic.
Provide only the most relevant background information. The introduction isn’t the place to get too in-depth; if more background is essential to your paper, it can appear in the body .
Empirical paper: Describing previous research
For a paper describing original research, you’ll instead provide an overview of the most relevant research that has already been conducted. This is a sort of miniature literature review —a sketch of the current state of research into your topic, boiled down to a few sentences.
This should be informed by genuine engagement with the literature. Your search can be less extensive than in a full literature review, but a clear sense of the relevant research is crucial to inform your own work.
Begin by establishing the kinds of research that have been done, and end with limitations or gaps in the research that you intend to respond to.
The next step is to clarify how your own research fits in and what problem it addresses.
Argumentative paper: Emphasize importance
In an argumentative research paper, you can simply state the problem you intend to discuss, and what is original or important about your argument.
Empirical paper: Relate to the literature
In an empirical research paper, try to lead into the problem on the basis of your discussion of the literature. Think in terms of these questions:
- What research gap is your work intended to fill?
- What limitations in previous work does it address?
- What contribution to knowledge does it make?
You can make the connection between your problem and the existing research using phrases like the following.
Now you’ll get into the specifics of what you intend to find out or express in your research paper.
The way you frame your research objectives varies. An argumentative paper presents a thesis statement, while an empirical paper generally poses a research question (sometimes with a hypothesis as to the answer).
Argumentative paper: Thesis statement
The thesis statement expresses the position that the rest of the paper will present evidence and arguments for. It can be presented in one or two sentences, and should state your position clearly and directly, without providing specific arguments for it at this point.
Empirical paper: Research question and hypothesis
The research question is the question you want to answer in an empirical research paper.
Present your research question clearly and directly, with a minimum of discussion at this point. The rest of the paper will be taken up with discussing and investigating this question; here you just need to express it.
A research question can be framed either directly or indirectly.
- This study set out to answer the following question: What effects does daily use of Instagram have on the prevalence of body image issues among adolescent girls?
- We investigated the effects of daily Instagram use on the prevalence of body image issues among adolescent girls.
If your research involved testing hypotheses , these should be stated along with your research question. They are usually presented in the past tense, since the hypothesis will already have been tested by the time you are writing up your paper.
For example, the following hypothesis might respond to the research question above:
The only proofreading tool specialized in correcting academic writing - try for free!
The academic proofreading tool has been trained on 1000s of academic texts and by native English editors. Making it the most accurate and reliable proofreading tool for students.
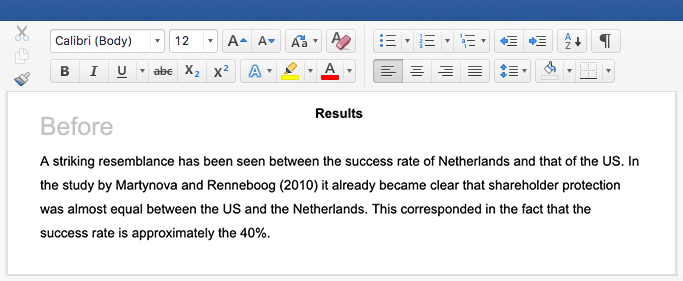
Try for free
The final part of the introduction is often dedicated to a brief overview of the rest of the paper.
In a paper structured using the standard scientific “introduction, methods, results, discussion” format, this isn’t always necessary. But if your paper is structured in a less predictable way, it’s important to describe the shape of it for the reader.
If included, the overview should be concise, direct, and written in the present tense.
- This paper will first discuss several examples of survey-based research into adolescent social media use, then will go on to …
- This paper first discusses several examples of survey-based research into adolescent social media use, then goes on to …
Full examples of research paper introductions are shown in the tabs below: one for an argumentative paper, the other for an empirical paper.
- Argumentative paper
- Empirical paper
Are cows responsible for climate change? A recent study (RIVM, 2019) shows that cattle farmers account for two thirds of agricultural nitrogen emissions in the Netherlands. These emissions result from nitrogen in manure, which can degrade into ammonia and enter the atmosphere. The study’s calculations show that agriculture is the main source of nitrogen pollution, accounting for 46% of the country’s total emissions. By comparison, road traffic and households are responsible for 6.1% each, the industrial sector for 1%. While efforts are being made to mitigate these emissions, policymakers are reluctant to reckon with the scale of the problem. The approach presented here is a radical one, but commensurate with the issue. This paper argues that the Dutch government must stimulate and subsidize livestock farmers, especially cattle farmers, to transition to sustainable vegetable farming. It first establishes the inadequacy of current mitigation measures, then discusses the various advantages of the results proposed, and finally addresses potential objections to the plan on economic grounds.
The rise of social media has been accompanied by a sharp increase in the prevalence of body image issues among women and girls. This correlation has received significant academic attention: Various empirical studies have been conducted into Facebook usage among adolescent girls (Tiggermann & Slater, 2013; Meier & Gray, 2014). These studies have consistently found that the visual and interactive aspects of the platform have the greatest influence on body image issues. Despite this, highly visual social media (HVSM) such as Instagram have yet to be robustly researched. This paper sets out to address this research gap. We investigated the effects of daily Instagram use on the prevalence of body image issues among adolescent girls. It was hypothesized that daily Instagram use would be associated with an increase in body image concerns and a decrease in self-esteem ratings.
The introduction of a research paper includes several key elements:
- A hook to catch the reader’s interest
- Relevant background on the topic
- Details of your research problem
and your problem statement
- A thesis statement or research question
- Sometimes an overview of the paper
Don’t feel that you have to write the introduction first. The introduction is often one of the last parts of the research paper you’ll write, along with the conclusion.
This is because it can be easier to introduce your paper once you’ve already written the body ; you may not have the clearest idea of your arguments until you’ve written them, and things can change during the writing process .
The way you present your research problem in your introduction varies depending on the nature of your research paper . A research paper that presents a sustained argument will usually encapsulate this argument in a thesis statement .
A research paper designed to present the results of empirical research tends to present a research question that it seeks to answer. It may also include a hypothesis —a prediction that will be confirmed or disproved by your research.
Cite this Scribbr article
If you want to cite this source, you can copy and paste the citation or click the “Cite this Scribbr article” button to automatically add the citation to our free Citation Generator.
Caulfield, J. (2023, March 27). Writing a Research Paper Introduction | Step-by-Step Guide. Scribbr. Retrieved March 22, 2024, from https://www.scribbr.com/research-paper/research-paper-introduction/
Is this article helpful?
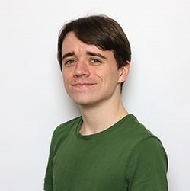
Jack Caulfield
Other students also liked, writing strong research questions | criteria & examples, writing a research paper conclusion | step-by-step guide, research paper format | apa, mla, & chicago templates, unlimited academic ai-proofreading.
✔ Document error-free in 5minutes ✔ Unlimited document corrections ✔ Specialized in correcting academic texts
Featured Clinical Reviews
- Screening for Atrial Fibrillation: US Preventive Services Task Force Recommendation Statement JAMA Recommendation Statement January 25, 2022
- Evaluating the Patient With a Pulmonary Nodule: A Review JAMA Review January 18, 2022
Select Your Interests
Customize your JAMA Network experience by selecting one or more topics from the list below.
- Academic Medicine
- Acid Base, Electrolytes, Fluids
- Allergy and Clinical Immunology
- American Indian or Alaska Natives
- Anesthesiology
- Anticoagulation
- Art and Images in Psychiatry
- Artificial Intelligence
- Assisted Reproduction
- Bleeding and Transfusion
- Caring for the Critically Ill Patient
- Challenges in Clinical Electrocardiography
- Climate and Health
- Climate Change
- Clinical Challenge
- Clinical Decision Support
- Clinical Implications of Basic Neuroscience
- Clinical Pharmacy and Pharmacology
- Complementary and Alternative Medicine
- Consensus Statements
- Coronavirus (COVID-19)
- Critical Care Medicine
- Cultural Competency
- Dental Medicine
- Dermatology
- Diabetes and Endocrinology
- Diagnostic Test Interpretation
- Drug Development
- Electronic Health Records
- Emergency Medicine
- End of Life, Hospice, Palliative Care
- Environmental Health
- Equity, Diversity, and Inclusion
- Facial Plastic Surgery
- Gastroenterology and Hepatology
- Genetics and Genomics
- Genomics and Precision Health
- Global Health
- Guide to Statistics and Methods
- Hair Disorders
- Health Care Delivery Models
- Health Care Economics, Insurance, Payment
- Health Care Quality
- Health Care Reform
- Health Care Safety
- Health Care Workforce
- Health Disparities
- Health Inequities
- Health Policy
- Health Systems Science
- History of Medicine
- Hypertension
- Images in Neurology
- Implementation Science
- Infectious Diseases
- Innovations in Health Care Delivery
- JAMA Infographic
- Law and Medicine
- Leading Change
- Less is More
- LGBTQIA Medicine
- Lifestyle Behaviors
- Medical Coding
- Medical Devices and Equipment
- Medical Education
- Medical Education and Training
- Medical Journals and Publishing
- Mobile Health and Telemedicine
- Narrative Medicine
- Neuroscience and Psychiatry
- Notable Notes
- Nutrition, Obesity, Exercise
- Obstetrics and Gynecology
- Occupational Health
- Ophthalmology
- Orthopedics
- Otolaryngology
- Pain Medicine
- Palliative Care
- Pathology and Laboratory Medicine
- Patient Care
- Patient Information
- Performance Improvement
- Performance Measures
- Perioperative Care and Consultation
- Pharmacoeconomics
- Pharmacoepidemiology
- Pharmacogenetics
- Pharmacy and Clinical Pharmacology
- Physical Medicine and Rehabilitation
- Physical Therapy
- Physician Leadership
- Population Health
- Primary Care
- Professional Well-being
- Professionalism
- Psychiatry and Behavioral Health
- Public Health
- Pulmonary Medicine
- Regulatory Agencies
- Reproductive Health
- Research, Methods, Statistics
- Resuscitation
- Rheumatology
- Risk Management
- Scientific Discovery and the Future of Medicine
- Shared Decision Making and Communication
- Sleep Medicine
- Sports Medicine
- Stem Cell Transplantation
- Substance Use and Addiction Medicine
- Surgical Innovation
- Surgical Pearls
- Teachable Moment
- Technology and Finance
- The Art of JAMA
- The Arts and Medicine
- The Rational Clinical Examination
- Tobacco and e-Cigarettes
- Translational Medicine
- Trauma and Injury
- Treatment Adherence
- Ultrasonography
- Users' Guide to the Medical Literature
- Vaccination
- Venous Thromboembolism
- Veterans Health
- Women's Health
- Workflow and Process
- Wound Care, Infection, Healing
- Download PDF
- Share X Facebook Email LinkedIn
- Permissions
Reporting Use of AI in Research and Scholarly Publication—JAMA Network Guidance
- 1 Executive Managing Editor, JAMA and JAMA Network
- 2 Associate Editor, JAMA
- 3 University of California, San Francisco
- 4 Yale School of Medicine, New Haven, Connecticut
- 5 JAMA and JAMA Network
- 6 Editor in Chief, JAMA and JAMA Network
- Editorial Guidance for Authors, Peer Reviewers, and Editors on Use of AI Annette Flanagin, RN, MA; Jacob Kendall-Taylor, BA; Kirsten Bibbins-Domingo, PhD, MD, MAS JAMA
- Editorial JAMA Call for Papers for AI in Medicine Rohan Khera, MD, MS; Atul J. Butte, MD, PhD; Michael Berkwits, MD, MSCE; Yulin Hswen, ScD, MPH; Annette Flanagin, RN, MA; Hannah Park; Gregory Curfman, MD; Kirsten Bibbins-Domingo, PhD, MD, MAS JAMA
Reports on the use of artificial intelligence (AI) and machine learning, including large language models (LLM), in medical research have intensified in the last year. Although machine learning research began 70 years ago with the conceptual development of artificial neural network algorithms, 1 AI research and use in clinical practice and health care are relatively recent advances. Throughout these developments, JAMA has sought to define the broad scope of discovery and innovation in medical applications of AI and to address potential challenges in its implementation. The journal’s 2016 publication of a study of deep learning algorithms for the detection of diabetic retinopathy from fundal photographs is a useful example. 2 The study represented a novel tool that could enable large-scale screening for a key vision-threatening disorder across the world. However, the accompanying Editorial 3 highlighted important challenges, spanning the need for broader patient representativeness, the investment necessary in validating the model in the context of its deployment and subsequent implementation, and whether clinicians would entrust decision-making to AI tools. The Editorial also called attention to concerns regarding AI eventually replacing humans in clinical systems. Although published before the recent AI boom, 4 this study and the comments about it augured many of today’s promises and concerns regarding AI in clinical research and practice. 5 - 11
Over the past decade, advances in AI have enabled many innovations that facilitate rapid research and resulted in tools for disease prediction, diagnosis, and prognostication. During the last year, JAMA provided guidance to authors and peer reviewers on the transparent, appropriate, and accountable use of AI. 12 , 13 Herein, we provide more detailed recommendations for authors and researchers. Editors and readers rely on authors to report their use of AI and to be fully accountable for such use. Transparency is crucial in employing AI technologies given its rapid development and yet-unknown functionalities. The guidance below is general and based on some common problems we have seen in submitted manuscripts; reporting guidelines will provide more specific guidance. This information has been added to the Instructions for Authors for JAMA and the JAMA Network journals. 14
AI Used in Manuscript Preparation
When traditional and generative AI technologies are used to create, review, revise, or edit any of the content in a manuscript, authors should report in the Acknowledgment section the following:
Name of the AI software platform, program, or tool
Version and extension numbers
Manufacturer
Date(s) of use
A brief description of how the AI was used and on what portions of the manuscript or content
Confirmation that the author(s) takes responsibility for the integrity of the content generated
Note that this guidance does not apply to basic tools for checking grammar, spelling, references, and similar.
AI Used in Research
When AI (eg, LLM or natural language processing [NLP], supervised or unsupervised machine learning for predictive/prescriptive or clustering tasks, chatbots, or similar technologies) is used as part of a scientific study, authors should
Follow relevant reporting guidelines for specific study designs when they exist (see examples in the Box ) and report each recommended guideline element with sufficient detail to enable reproducibility. 15 - 25
Avoid inclusion of identifiable patient information in text, tables, and figures.
Be aware of copyright and intellectual property concerns if including content (text, images) generated by AI, and indicate rights or permissions to publish that content as determined by the AI service or owner.
Also address the following:
Examples of AI-Related Reporting Guidelines
CONSORT-AI for clinical trial reports evaluating interventions with an AI component 15
SPIRIT-AI for clinical trial protocols evaluating interventions with an AI component 16
MI-CLAIM for studies including clinical AI modeling 17
CLAIM for studies describing applications of AI in medical imaging 18
MINIMAR (MINimumInformation for Medical AIReporting) for studies of AI in health care 19
DECIDE-AI for studies describing the early-stage live clinical evaluation of AI-based decision support systems 20
Recommendations for Reporting Machine Learning Analyses in Clinical Research for studies of machine learning analyses 21
Other AI reporting extensions and guidelines (under development):
STARD-AI for AI-centered diagnostic test accuracy studies 22
TRIPOD-AI for prediction model studies based on machine learning techniques 23
PROBAST-AI for risk of bias assessment of machine learning–based prediction model studies 23
CANGARU for ethical use, disclosure, and reporting of AI in scholarly publication 24
CHART for studies assessing use of chatbots and LLMs for health information 25
Methods Section
Include the study design and, if a relevant reporting guideline exists ( Box ), indicate how it was followed, with sufficient detail to enable reproducibility.
Describe how AI was used for specific aspects of the study (eg, to generate or refine study hypotheses, assist in the generation of a list of adjustment variables, create graphs to show visual relationships).
For studies using LLMs, provide the name of the platform or program, tool, version, and manufacturer; specify dates and prompt(s) used and their sequence and any revisions to prompts in response to initial outputs.
For studies reporting machine learning and algorithm development, include details about datasets used for development, training, and validation. Clearly state if algorithms were trained and tested only on previously collected or existing data sets or if the study includes prospective deployment. Include the machine learning model and describe the variables and outcome(s) and selection of the fine-tuning parameters. Describe any assumptions involved (eg, log linearity, proportionality) and how these assumptions were tested.
Indicate the metric used to evaluate the performance of the algorithms, including bias, discrimination, calibration, reclassification, and others as appropriate.
Indicate the methods used to address missing data.
Indicate institutional review board/ethics review, approval, waiver, or exemption.
Describe methods or analyses included to address and manage AI-related methodologic bias and inaccuracy of AI-generated content.
Indicate, when appropriate, if sensitivity analyses were performed to explore the performance of the AI model in vulnerable or underrepresented subgroups.
Provide a data sharing statement, including if code will be shared. 26
Results Section
When reporting comparisons, provide performance assessments (eg, against standard of care), include effect sizes and measures of uncertainty (eg, 95% CIs) and other measurements such as likelihood ratios, and include information about performance errors, inaccurate or missing data, and sufficient detail for others to reproduce the findings.
Report the results of analyses to address methodologic bias and population representation.
If examples of generated text or content are included in tables or figures, be sure to indicate the source and licensing information, as noted above.
Discussion Section
Discuss the potential for AI-related bias and what was done to identify and mitigate such bias.
Discuss the potential for inaccuracy of AI-generated content and what was done to identify and manage this.
Discuss generalizability of findings across populations and results of analyses performed to explore the performance of the AI model in vulnerable or underrepresented subgroups.
Conclusions
We welcome feedback on these recommendations. We will continue to monitor reporting guideline development and update these recommendations and our Instructions for Authors with the aim of providing authors, researchers, reviewers, and editors with information to help guide the transparent and accountable reporting of use of AI in research and publication.
Corresponding Author: Annette Flanagin, RN, MA, JAMA and JAMA Network ( [email protected] ).
Published Online: March 7, 2024. doi:10.1001/jama.2024.3471
Conflict of Interest Disclosures: Dr Khera reported receiving grants from NHLBI, Doris Duke Charitable Foundation, Bristol Myers Squibb, and Novo Nordisk, and serving as cofounder of Evidence2Health and Ensight-AI, outside the submitted work. Dr Pirracchio reported receiving personal fees from Philips and AOP outside the submitted work. No other disclosures were reported.
See More About
Flanagin A , Pirracchio R , Khera R , Berkwits M , Hswen Y , Bibbins-Domingo K. Reporting Use of AI in Research and Scholarly Publication—JAMA Network Guidance. JAMA. Published online March 07, 2024. doi:10.1001/jama.2024.3471
Manage citations:
© 2024
Artificial Intelligence Resource Center
Cardiology in JAMA : Read the Latest
Browse and subscribe to JAMA Network podcasts!
Others Also Liked
- Register for email alerts with links to free full-text articles
- Access PDFs of free articles
- Manage your interests
- Save searches and receive search alerts
Help | Advanced Search
Computer Science > Computer Vision and Pattern Recognition
Title: mm1: methods, analysis & insights from multimodal llm pre-training.
Abstract: In this work, we discuss building performant Multimodal Large Language Models (MLLMs). In particular, we study the importance of various architecture components and data choices. Through careful and comprehensive ablations of the image encoder, the vision language connector, and various pre-training data choices, we identified several crucial design lessons. For example, we demonstrate that for large-scale multimodal pre-training using a careful mix of image-caption, interleaved image-text, and text-only data is crucial for achieving state-of-the-art (SOTA) few-shot results across multiple benchmarks, compared to other published pre-training results. Further, we show that the image encoder together with image resolution and the image token count has substantial impact, while the vision-language connector design is of comparatively negligible importance. By scaling up the presented recipe, we build MM1, a family of multimodal models up to 30B parameters, including both dense models and mixture-of-experts (MoE) variants, that are SOTA in pre-training metrics and achieve competitive performance after supervised fine-tuning on a range of established multimodal benchmarks. Thanks to large-scale pre-training, MM1 enjoys appealing properties such as enhanced in-context learning, and multi-image reasoning, enabling few-shot chain-of-thought prompting.
Submission history
Access paper:.
- Download PDF
- Other Formats
References & Citations
- Google Scholar
- Semantic Scholar
BibTeX formatted citation

Bibliographic and Citation Tools
Code, data and media associated with this article, recommenders and search tools.
- Institution
arXivLabs: experimental projects with community collaborators
arXivLabs is a framework that allows collaborators to develop and share new arXiv features directly on our website.
Both individuals and organizations that work with arXivLabs have embraced and accepted our values of openness, community, excellence, and user data privacy. arXiv is committed to these values and only works with partners that adhere to them.
Have an idea for a project that will add value for arXiv's community? Learn more about arXivLabs .
Thank you for visiting nature.com. You are using a browser version with limited support for CSS. To obtain the best experience, we recommend you use a more up to date browser (or turn off compatibility mode in Internet Explorer). In the meantime, to ensure continued support, we are displaying the site without styles and JavaScript.
- View all journals
- My Account Login
- Explore content
- About the journal
- Publish with us
- Sign up for alerts
- Open access
- Published: 19 March 2024
TacticAI: an AI assistant for football tactics
- Zhe Wang ORCID: orcid.org/0000-0002-0748-5376 1 na1 ,
- Petar Veličković ORCID: orcid.org/0000-0002-2820-4692 1 na1 ,
- Daniel Hennes ORCID: orcid.org/0000-0002-3646-5286 1 na1 ,
- Nenad Tomašev ORCID: orcid.org/0000-0003-1624-0220 1 ,
- Laurel Prince 1 ,
- Michael Kaisers 1 ,
- Yoram Bachrach 1 ,
- Romuald Elie 1 ,
- Li Kevin Wenliang 1 ,
- Federico Piccinini 1 ,
- William Spearman 2 ,
- Ian Graham 3 ,
- Jerome Connor 1 ,
- Yi Yang 1 ,
- Adrià Recasens 1 ,
- Mina Khan 1 ,
- Nathalie Beauguerlange 1 ,
- Pablo Sprechmann 1 ,
- Pol Moreno 1 ,
- Nicolas Heess ORCID: orcid.org/0000-0001-7876-9256 1 ,
- Michael Bowling ORCID: orcid.org/0000-0003-2960-8418 4 ,
- Demis Hassabis 1 &
- Karl Tuyls ORCID: orcid.org/0000-0001-7929-1944 5
Nature Communications volume 15 , Article number: 1906 ( 2024 ) Cite this article
20k Accesses
241 Altmetric
Metrics details
- Computational science
- Information technology
Identifying key patterns of tactics implemented by rival teams, and developing effective responses, lies at the heart of modern football. However, doing so algorithmically remains an open research challenge. To address this unmet need, we propose TacticAI, an AI football tactics assistant developed and evaluated in close collaboration with domain experts from Liverpool FC. We focus on analysing corner kicks, as they offer coaches the most direct opportunities for interventions and improvements. TacticAI incorporates both a predictive and a generative component, allowing the coaches to effectively sample and explore alternative player setups for each corner kick routine and to select those with the highest predicted likelihood of success. We validate TacticAI on a number of relevant benchmark tasks: predicting receivers and shot attempts and recommending player position adjustments. The utility of TacticAI is validated by a qualitative study conducted with football domain experts at Liverpool FC. We show that TacticAI’s model suggestions are not only indistinguishable from real tactics, but also favoured over existing tactics 90% of the time, and that TacticAI offers an effective corner kick retrieval system. TacticAI achieves these results despite the limited availability of gold-standard data, achieving data efficiency through geometric deep learning.
Similar content being viewed by others
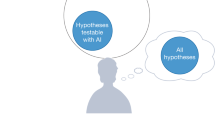
Artificial intelligence and illusions of understanding in scientific research
Lisa Messeri & M. J. Crockett
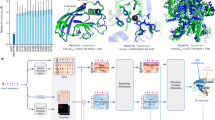
Highly accurate protein structure prediction with AlphaFold
John Jumper, Richard Evans, … Demis Hassabis
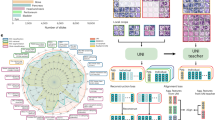
Towards a general-purpose foundation model for computational pathology
Richard J. Chen, Tong Ding, … Faisal Mahmood
Introduction
Association football, or simply football or soccer, is a widely popular and highly professionalised sport, in which two teams compete to score goals against each other. As each football team comprises up to 11 active players at all times and takes place on a very large pitch (also known as a soccer field), scoring goals tends to require a significant degree of strategic team-play. Under the rules codified in the Laws of the Game 1 , this competition has nurtured an evolution of nuanced strategies and tactics, culminating in modern professional football leagues. In today’s play, data-driven insights are a key driver in determining the optimal player setups for each game and developing counter-tactics to maximise the chances of success 2 .
When competing at the highest level the margins are incredibly tight, and it is increasingly important to be able to capitalise on any opportunity for creating an advantage on the pitch. To that end, top-tier clubs employ diverse teams of coaches, analysts and experts, tasked with studying and devising (counter-)tactics before each game. Several recent methods attempt to improve tactical coaching and player decision-making through artificial intelligence (AI) tools, using a wide variety of data types from videos to tracking sensors and applying diverse algorithms ranging from simple logistic regression to elaborate neural network architectures. Such methods have been employed to help predict shot events from videos 3 , forecast off-screen movement from spatio-temporal data 4 , determine whether a match is in-play or interrupted 5 , or identify player actions 6 .
The execution of agreed-upon plans by players on the pitch is highly dynamic and imperfect, depending on numerous factors including player fitness and fatigue, variations in player movement and positioning, weather, the state of the pitch, and the reaction of the opposing team. In contrast, set pieces provide an opportunity to exert more control on the outcome, as the brief interruption in play allows the players to reposition according to one of the practiced and pre-agreed patterns, and make a deliberate attempt towards the goal. Examples of such set pieces include free kicks, corner kicks, goal kicks, throw-ins, and penalties 2 .
Among set pieces, corner kicks are of particular importance, as an improvement in corner kick execution may substantially modify game outcomes, and they lend themselves to principled, tactical and detailed analysis. This is because corner kicks tend to occur frequently in football matches (with ~10 corners on average taking place in each match 7 ), they are taken from a fixed, rigid position, and they offer an immediate opportunity for scoring a goal—no other set piece simultaneously satisfies all of the above. In practice, corner kick routines are determined well ahead of each match, taking into account the strengths and weaknesses of the opposing team and their typical tactical deployment. It is for this reason that we focus on corner kick analysis in particular, and propose TacticAI, an AI football assistant for supporting the human expert with set piece analysis, and the development and improvement of corner kick routines.
TacticAI is rooted in learning efficient representations of corner kick tactics from raw, spatio-temporal player tracking data. It makes efficient use of this data by representing each corner kick situation as a graph—a natural representation for modelling relationships between players (Fig. 1 A, Table 2 ), and these player relationships may be of higher importance than the absolute distances between them on the pitch 8 . Such a graph input is a natural candidate for graph machine learning models 9 , which we employ within TacticAI to obtain high-dimensional latent player representations. In the Supplementary Discussion section, we carefully contrast TacticAI against prior art in the area.
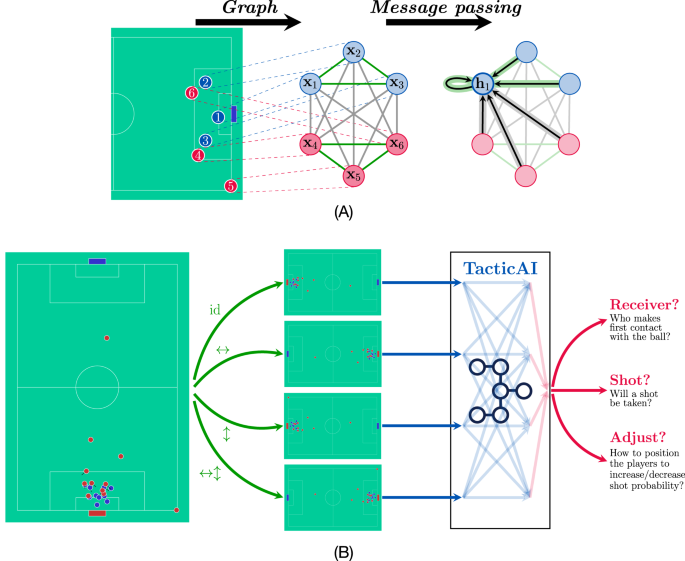
A How corner kick situations are converted to a graph representation. Each player is treated as a node in a graph, with node, edge and graph features extracted as detailed in the main text. Then, a graph neural network operates over this graph by performing message passing; each node’s representation is updated using the messages sent to it from its neighbouring nodes. B How TacticAI processes a given corner kick. To ensure that TacticAI’s answers are robust in the face of horizontal or vertical reflections, all possible combinations of reflections are applied to the input corner, and these four views are then fed to the core TacticAI model, where they are able to interact with each other to compute the final player representations—each internal blue arrow corresponds to a single message passing layer from ( A ). Once player representations are computed, they can be used to predict the corner’s receiver, whether a shot has been taken, as well as assistive adjustments to player positions and velocities, which increase or decrease the probability of a shot being taken.
Uniquely, TacticAI takes advantage of geometric deep learning 10 to explicitly produce player representations that respect several symmetries of the football pitch (Fig. 1 B). As an illustrative example, we can usually safely assume that under a horizontal or vertical reflection of the pitch state, the game situation is equivalent. Geometric deep learning ensures that TacticAI’s player representations will be identically computed under such reflections, such that this symmetry does not have to be learnt from data. This proves to be a valuable addition, as high-quality tracking data is often limited—with only a few hundred matches played each year in every league. We provide an in-depth overview of how we employ geometric deep learning in TacticAI in the “Methods” section.
From these representations, TacticAI is then able to answer various predictive questions about the outcomes of a corner—for example, which player is most likely to make first contact with the ball, or whether a shot will take place. TacticAI can also be used as a retrieval system—for mining similar corner kick situations based on the similarity of player representations—and a generative recommendation system, suggesting adjustments to player positions and velocities to maximise or minimise the estimated shot probability. Through several experiments within a case study with domain expert coaches and analysts from Liverpool FC, the results of which we present in the next section, we obtain clear statistical evidence that TacticAI readily provides useful, realistic and accurate tactical suggestions.
To demonstrate the diverse qualities of our approach, we design TacticAI with three distinct predictive and generative components: receiver prediction, shot prediction, and tactic recommendation through guided generation, which also correspond to the benchmark tasks for quantitatively evaluating TacticAI. In addition to providing accurate quantitative insights for corner kick analysis with its predictive components, the interplay between TacticAI’s predictive and generative components allows coaches to sample alternative player setups for each routine of interest, and directly evaluate the possible outcomes of such alternatives.
We will first describe our quantitative analysis, which demonstrates that TacticAI’s predictive components are accurate at predicting corner kick receivers and shot situations on held-out test corners and that the proposed player adjustments do not strongly deviate from ground-truth situations. However, such an analysis only gives an indirect insight into how useful TacticAI would be once deployed. We tackle this question of utility head-on and conduct a comprehensive case study in collaboration with our partners at Liverpool FC—where we directly ask human expert raters to judge the utility of TacticAI’s predictions and player adjustments. The following sections expand on the specific results and analysis we have performed.
In what follows, we will describe TacticAI’s components at a minimal level necessary to understand our evaluation. We defer detailed descriptions of TacticAI’s components to the “Methods” section. Note that, all our error bars reported in this research are standard deviations.
Benchmarking TacticAI
We evaluate the three components of TacticAI on a relevant benchmark dataset of corner kicks. Our dataset consists of 7176 corner kicks from the 2020 to 2021 Premier League seasons, which we randomly shuffle and split into a training (80%) and a test set (20%). As previously mentioned, TacticAI operates on graphs. Accordingly, we represent each corner kick situation as a graph, where each node corresponds to a player. The features associated with each node encode the movements (velocities and positions) and simple profiles (heights and weights) of on-pitch players at the timestamp when the corresponding corner kick was being taken by the attacking kicker (see the “Methods” section), and no information of ball movement was encoded. The graphs are fully connected; that is, for every pair of players, we will include the edge connecting them in the graph. Each of these edges encodes a binary feature, indicating whether the two players are on opposing teams or not. For each task, we generated the relevant dataset of node/edge/graph features and corresponding labels (Tables 1 and 2 , see the “Methods” section). The components were then trained separately with their corresponding corner kick graphs. In particular, we only employ a minimal set of features to construct the corner kick graphs, without encoding the movements of the ball nor explicitly encoding the distances between players into the graphs. We used a consistent training-test split for all benchmark tasks, as this made it possible to benchmark not only the individual components but also their interactions.
Accurate receiver and shot prediction through geometric deep learning
One of TacticAI’s key predictive models forecasts the receiver out of the 22 on-pitch players. The receiver is defined as the first player touching the ball after the corner is taken. In our evaluation, all methods used the same set of features (see the “Receiver prediction” entry in Table 1 and the “Methods” section). We leveraged the receiver prediction task to benchmark several different TacticAI base models. Our best-performing model—achieving 0.782 ± 0.039 in top-3 test accuracy after 50,000 training steps—was a deep graph attention network 11 , 12 , leveraging geometric deep learning 10 through the use of D 2 group convolutions 13 . We supplement this result with a detailed ablation study, verifying that both our choice of base architecture and group convolution yielded significant improvements in the receiver prediction task (Supplementary Table 2 , see the subsection “Ablation study” in the “Methods” section). Considering that corner kick receiver prediction is a highly challenging task with many factors that are unseen by our model—including fatigue and fitness levels, and actual ball trajectory—we consider TacticAI’s top-3 accuracy to reflect a high level of predictive power, and keep the base TacticAI architecture fixed for subsequent studies. In addition to this quantitative evaluation with the evaluation dataset, we also evaluate the performance of TacticAI’s receiver prediction component in a case study with human raters. Please see the “Case study with expert raters” section for more details.
For shot prediction, we observe that reusing the base TacticAI architecture to directly predict shot events—i.e., directly modelling the probability \({\mathbb{P}}(\,{{\mbox{shot}}}| {{\mbox{corner}}}\,)\) —proved challenging, only yielding a test F 1 score of 0.52 ± 0.03, for a GATv2 base model. Note that here we use the F 1 score—the harmonic mean of precision and recall—as it is commonly used in binary classification problems over imbalanced datasets, such as shot prediction. However, given that we already have a potent receiver predictor, we decided to use its output to give us additional insight into whether or not a shot had been taken. Hence, we opted to decompose the probability of taking a shot as
where \({\mathbb{P}}(\,{{\mbox{receiver}}}| {{\mbox{corner}}}\,)\) are the probabilities computed by TacticAI’s receiver prediction system, and \({\mathbb{P}}(\,{{\mbox{shot}}}| {{\mbox{receiver}}},{{\mbox{corner}}}\,)\) models the conditional shot probability after a specific player makes first contact with the ball. This was implemented through providing an additional global feature to indicate the receiver in the corresponding corner kick (Table 1 ) while the architecture otherwise remained the same as that of receiver prediction (Supplementary Fig. 2 , see the “Methods” section). At training time, we feed the ground-truth receiver as input to the model—at inference time, we attempt every possible receiver, weighing their contributions using the probabilities given by TacticAI’s receiver predictor, as per Eq. ( 1 ). This two-phased approach yielded a final test F 1 score of 0.68 ± 0.04 for shot prediction, which encodes significantly more signal than the unconditional shot predictor, especially considering the many unobservables associated with predicting shot events. Just as for receiver prediction, this performance can be further improved using geometric deep learning; a conditional GATv2 shot predictor with D 2 group convolutions achieves an F 1 score of 0.71 ± 0.01.
Moreover, we also observe that, even just through predicting the receivers, without explicitly classifying any other salient features of corners, TacticAI learned generalisable representations of the data. Specifically, team setups with similar tactical patterns tend to cluster together in TacticAI’s latent space (Fig. 2 ). However, no clear clusters are observed in the raw input space (Supplementary Fig. 1 ). This indicates that TacticAI can be leveraged as a useful corner kick retrieval system, and we will present our evaluation of this hypothesis in the “Case study with expert raters” section.
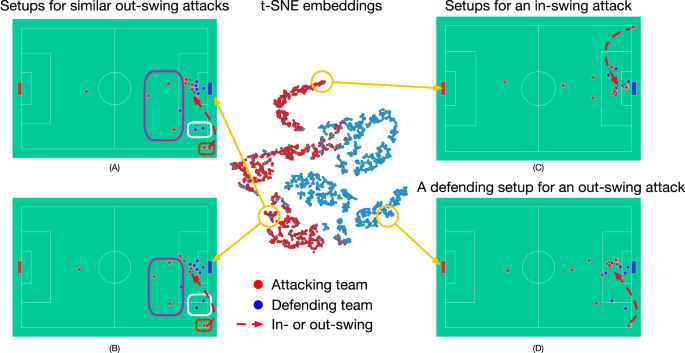
We visualise the latent representations of attacking and defending teams in 1024 corner kicks using t -SNE. A latent team embedding in one corner kick sample is the mean of the latent player representations on the same attacking ( A – C ) or defending ( D ) team. Given the reference corner kick sample ( A ), we retrieve another corner kick sample ( B ) with respect to the closest distance of their representations in the latent space. We observe that ( A ) and ( B ) are both out-swing corner kicks and share similar patterns of their attacking tactics, which are highlighted with rectangles having the same colours, although they bear differences with respect to the absolute positions and velocities of the players. All the while, the latent representation of an in-swing attack ( C ) is distant from both ( A ) and ( B ) in the latent space. The red arrows are only used to demonstrate the difference between in- and out-swing corner kicks, not the actual ball trajectories.
Lastly, it is worth emphasising that the utility of the shot predictor likely does not come from forecasting whether a shot event will occur—a challenging problem with many imponderables—but from analysing the difference in predicted shot probability across multiple corners. Indeed, in the following section, we will show how TacticAI’s generative tactic refinements can directly influence the predicted shot probabilities, which will then corresponds to highly favourable evaluation by our expert raters in the “Case study with expert raters” section.
Controlled tactic refinement using class-conditional generative models
Equipped with components that are able to potently relate corner kicks with their various outcomes (e.g. receivers and shot events), we can explore the use of TacticAI to suggest adjustments of tactics, in order to amplify or reduce the likelihood of certain outcomes.
Specifically, we aim to produce adjustments to the movements of players on one of the two teams, including their positions and velocities, which would maximise or minimise the probability of a shot event, conditioned on the initial corner setup, consisting of the movements of players on both teams and their heights and weights. In particular, although in real-world scenarios both teams may react simultaneously to the movements of each other, in our study, we focus on moderate adjustments to player movements, which help to detect players that are not responding to a tactic properly. Due to this reason, we simplify the process of tactic refinement through generating the adjustments for only one team while keeping the other fixed. The way we train a model for this task is through an auto-encoding objective: we feed the ground-truth shot outcome (a binary indicator) as an additional graph-level feature to TacticAI’s model (Table 1 ), and then have it learn to reconstruct a probability distribution of the input player coordinates (Fig. 1 B, also see the “Methods” section). As a consequence, our tactic adjustment system does not depend on the previously discussed shot predictor—although we can use the shot predictor to evaluate whether the adjustments make a measurable difference in shot probability.
This autoencoder-based generative model is an individual component that separates from TacticAI’s predictive systems. All three systems share the encoder architecture (without sharing parameters), but use different decoders (see the “Methods” section). At inference time, we can instead feed in a desired shot outcome for the given corner setup, and then sample new positions and velocities for players on one team using this probability distribution. This setup, in principle, allows for flexible downstream use, as human coaches can optimise corner kick setups through generating adjustments conditioned on the specific outcomes of their interest—e.g., increasing shot probability for the attacking team, decreasing it for the defending team (Fig. 3 ) or amplifying the chance that a particular striker receives the ball.
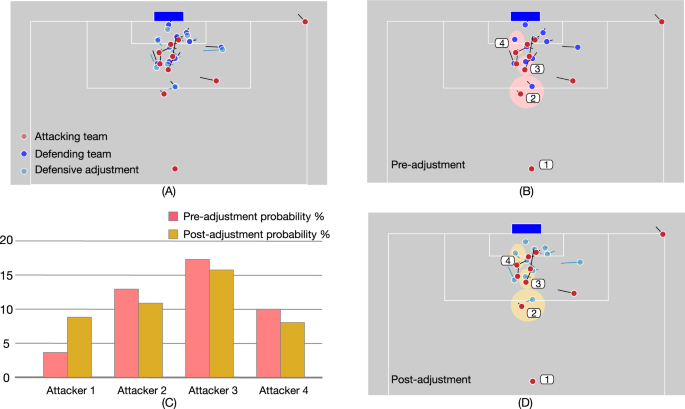
TacticAI makes it possible for human coaches to redesign corner kick tactics in ways that help maximise the probability of a positive outcome for either the attacking or the defending team by identifying key players, as well as by providing temporally coordinated tactic recommendations that take all players into consideration. As demonstrated in the present example ( A ), for a corner kick in which there was a shot attempt in reality ( B ), TacticAI can generate a tactically-adjusted setting in which the shot probability has been reduced, by adjusting the positioning of the defenders ( D ). The suggested defender positions result in reduced receiver probability for attacking players 2–5 (see bottom row), while the receiver probability of Attacker 1, who is distant from the goalpost, has been increased ( C ). The model is capable of generating multiple such scenarios. Coaches can inspect the different options visually and additionally consult TacticAI’s quantitative analysis of the presented tactics.
We first evaluate the generated adjustments quantitatively, by verifying that they are indistinguishable from the original corner kick distribution using a classifier. To do this, we synthesised a dataset consisting of 200 corner kick samples and their corresponding conditionally generated adjustments. Specifically, for corners without a shot event, we generated adjustments for the attacking team by setting the shot event feature to 1, and vice-versa for the defending team when a shot event did happen. We found that the real and generated samples were not distinguishable by an MLP classifier, with an F 1 score of 0.53 ± 0.05, indicating random chance level accuracy. This result indicates that the adjustments produced by TacticAI are likely similar enough to real corner kicks that the MLP is unable to tell them apart. Note that, in spite of this similarity, TacticAI recommends player-level adjustments that are not negligible—in the following section we will illustrate several salient examples of this. To more realistically validate the practical indistinguishability of TacticAI’s adjustments from realistic corners, we also evaluated the realism of the adjustments in a case study with human experts, which we will present in the following section.
In addition, we leveraged our TacticAI shot predictor to estimate whether the proposed adjustments were effective. We did this by analysing 100 corner kick samples in which threatening shots occurred, and then, for each sample, generated one defensive refinement through setting the shot event feature to 0. We observed that the average shot probability significantly decreased, from 0.75 ± 0.14 for ground-truth corners to 0.69 ± 0.16 for adjustments ( z = 2.62, p < 0.001). This observation was consistent when testing for attacking team refinements (shot probability increased from 0.18 ± 0.16 to 0.31 ± 0.26 ( z = −4.46, p < 0.001)). Moving beyond this result, we also asked human raters to assess the utility of TacticAI’s proposed adjustments within our case study, which we detail next.
Case study with expert raters
Although quantitative evaluation with well-defined benchmark datasets was critical for the technical development of TacticAI, the ultimate test of TacticAI as a football tactic assistant is its practical downstream utility being recognised by professionals in the industry. To this end, we evaluated TacticAI through a case study with our partners at Liverpool FC (LFC). Specifically, we invited a group of five football experts: three data scientists, one video analyst, and one coaching assistant. Each of them completed four tasks in the case study, which evaluated the utility of TacticAI’s components from several perspectives; these include (1) the realism of TacticAI’s generated adjustments, (2) the plausibility of TacticAI’s receiver predictions, (3) effectiveness of TacticAI’s embeddings for retrieving similar corners, and (4) usefulness of TacticAI’s recommended adjustments. We provide an overview of our study’s results here and refer the interested reader to Supplementary Figs. 3 – 5 and the Supplementary Methods for additional details.
We first simultaneously evaluated the realism of the adjusted corner kicks generated by TacticAI, and the plausibility of its receiver predictions. Going through a collection of 50 corner kick samples, we first asked the raters to classify whether a given sample was real or generated by TacticAI, and then they were asked to identify the most likely receivers in the corner kick sample (Supplementary Fig. 3 ).
On the task of classifying real and generated samples, first, we found that the raters’ average F 1 score of classifying the real vs. generated samples was only 0.60 ± 0.04, with individual F 1 scores ( \({F}_{1}^{A}=0.54,{F}_{1}^{B}=0.64,{F}_{1}^{C}=0.65,{F}_{1}^{D}=0.62,{F}_{1}^{E}=0.56\) ), indicating that the raters were, in many situations, unable to distinguish TacticAI’s adjustments from real corners.
The previous evaluation focused on analysing realism detection performance across raters. We also conduct a study that analyses realism detection across samples. Specifically, we assigned ratings for each sample—assigning +1 to a sample if it was identified as real by a human rater, and 0 otherwise—and computed the average rating for each sample across the five raters. Importantly, by studying the distribution of ratings, we found that there was no significant difference between the average ratings assigned to real and generated corners ( z = −0.34, p > 0.05) (Fig. 4 A). Hence, the real and generated samples were assigned statistically indistinguishable average ratings by human raters.
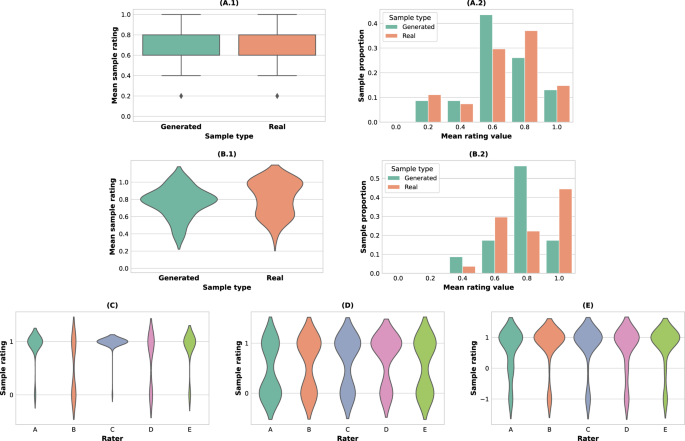
In task 1, we tested the statistical difference between the real corner kick samples and the synthetic ones generated by TacticAI from two aspects: ( A.1 ) the distributions of their assigned ratings, and ( A.2 ) the corresponding histograms of the rating values. Analogously, in task 2 (receiver prediction), ( B.1 ) we track the distributions of the top-3 accuracy of receiver prediction using those samples, and ( B.2 ) the corresponding histogram of the mean rating per sample. No statistical difference in the mean was observed in either cases (( A.1 ) ( z = −0.34, p > 0.05), and ( B.1 ) ( z = 0.97, p > 0.05)). Additionally, we observed a statistically significant difference between the ratings of different raters on receiver prediction, with three clear clusters emerging ( C ). Specifically, Raters A and E had similar ratings ( z = 0.66, p > 0.05), and Raters B and D also rated in similar ways ( z = −1.84, p > 0.05), while Rater C responded differently from all other raters. This suggests a good level of variety of the human raters with respect to their perceptions of corner kicks. In task 3—identifying similar corners retrieved in terms of salient strategic setups—there were no significant differences among the distributions of the ratings by different raters ( D ), suggesting a high level of agreement on the usefulness of TacticAI’s capability of retrieving similar corners ( F 1,4 = 1.01, p > 0.1). Finally, in task 4, we compared the ratings of TacticAI’s strategic refinements across the human raters ( E ) and found that the raters also agreed on the general effectiveness of the refinements recommended by TacticAI ( F 1,4 = 0.45, p > 0.05). Note that the violin plots used in B.1 and C – E model a continuous probability distribution and hence assign nonzero probabilities to values outside of the allowed ranges. We only label y -axis ticks for the possible set of ratings.
For the task of identifying receivers, we rated TacticAI’s predictions with respect to a rater as +1 if at least one of the receivers identified by the rater appeared in TacticAI’s top-3 predictions, and 0 otherwise. The average top-3 accuracy among the human raters was 0.79 ± 0.18; specifically, 0.81 ± 0.17 for the real samples, and 0.77 ± 0.21 for the generated ones. These scores closely line up with the accuracy of TacticAI in predicting receivers for held-out test corners, validating our quantitative study. Further, after averaging the ratings for receiver prediction sample-wise, we found no statistically significant difference between the average ratings of predicting receivers over the real and generated samples ( z = 0.97, p > 0.05) (Fig. 4 B). This indicates that TacticAI was equally performant in predicting the receivers of real corners and TacticAI-generated adjustments, and hence may be leveraged for this purpose even in simulated scenarios.
There is a notably high variance in the average receiver prediction rating of TacticAI. We hypothesise that this is due to the fact that different raters may choose to focus on different salient features when evaluating the likely receivers (or even the amount of likely receivers). We set out to validate this hypothesis by testing the pair-wise similarity of the predictions by the human raters through running a one-away analysis of variance (ANOVA), followed by a Tukey test. We found that the distributions of the five raters’ predictions were significantly different ( F 1,4 = 14.46, p < 0.001) forming three clusters (Fig. 4 C). This result indicates that different human raters—as suggested by their various titles at LFC—may often use very different leads when suggesting plausible receivers. The fact that TacticAI manages to retain a high top-3 accuracy in such a setting suggests that it was able to capture the salient patterns of corner kick strategies, which broadly align with human raters’ preferences. We will further test this hypothesis in the third task—identifying similar corners.
For the third task, we asked the human raters to judge 50 pairs of corners for their similarity. Each pair consisted of a reference corner and a retrieved corner, where the retrieved corner was chosen either as the nearest-neighbour of the reference in terms of their TacticAI latent space representations, or—as a feature-level heuristic—the cosine similarities of their raw features (Supplementary Fig. 4 ) in our corner kick dataset. We score the raters’ judgement of a pair as +1 if they considered the corners presented in the case to be usefully similar, otherwise, the pair is scored with 0. We first computed, for each rater, the recall with which they have judged a baseline- or TacticAI-retrieved pair as usefully similar—see description of Task 3 in the Supplementary Methods . For TacticAI retrievals, the average recall across all raters was 0.59 ± 0.09, and for the baseline system, the recall was 0.36 ± 0.10. Secondly, we assess the statistical difference between the results of the two methods by averaging the ratings for each reference–retrieval pair, finding that the average rating of TacticAI retrievals is significantly higher than the average rating of baseline method retrievals ( z = 2.34, p < 0.05). These two results suggest that TacticAI significantly outperforms the feature-space baseline as a method for mining similar corners. This indicates that TacticAI is able to extract salient features from corners that are not trivial to extract from the input data alone, reinforcing it as a potent tool for discovering opposing team tactics from available data. Finally, we observed that this task exhibited a high level of inter-rater agreement for TacticAI-retrieved pairs ( F 1,4 = 1.01, p > 0.1) (Fig. 4 D), suggesting that human raters were largely in agreement with respect to their assessment of TacticAI’s performance.
Finally, we evaluated TacticAI’s player adjustment recommendations for their practical utility. Specifically, each rater was given 50 tactical refinements together with the corresponding real corner kick setups—see Supplementary Fig. 5 , and the “Case study design” section in the Supplementary Methods . The raters were then asked to rate each refinement as saliently improving the tactics (+1), saliently making them worse (−1), or offering no salient differences (0). We calculated the average rating assigned by each of the raters (giving us a value in the range [− 1, 1] for each rater). The average of these values across all five raters was 0.7 ± 0.1. Further, for 45 of the 50 situations (90%), the human raters found TacticAI’s suggestion to be favourable on average (by majority voting). Both of these results indicate that TacticAI’s recommendations are salient and useful to a downstream football club practitioner, and we set out to validate this with statistical tests.
We performed statistical significance testing of the observed positive ratings. First, for each of the 50 situations, we averaged its ratings across all five raters and then ran a t -test to assess whether the mean rating was significantly larger than zero. Indeed, the statistical test indicated that the tactical adjustments recommended by TacticAI were constructive overall ( \({t}_{49}^{{{{{{{{\rm{avg}}}}}}}}}=9.20,\, p \, < \, 0.001\) ). Secondly, we verified that each of the five raters individually found TacticAI’s recommendations to be constructive, running a t -test on each of their ratings individually. For all of the five raters, their average ratings were found to be above zero with statistical significance ( \({t}_{49}^{A}=5.84,\, {p}^{A} \, < \, 0.001;{t}_{49}^{B}=7.88,\; {p}^{B} \, < \, 0.001;{t}_{49}^{C}=7.00,\; {p}^{C} \, < \, 0.001;{t}_{49}^{D}=6.04,\; {p}^{D} \, < \, 0.001;{t}_{49}^{E}=7.30,\, {p}^{E} \, < \, 0.001\) ). In addition, their ratings also shared a high level of inter-agreement ( F 1,4 = 0.45, p > 0.05) (Fig. 4 E), suggesting a level of practical usefulness that is generally recognised by human experts, even though they represent different backgrounds.
Taking all of these results together, we find TacticAI to possess strong components for prediction, retrieval, and tactical adjustments on corner kicks. To illustrate the kinds of salient recommendations by TacticAI, in Fig. 5 we present four examples with a high degree of inter-rater agreement.
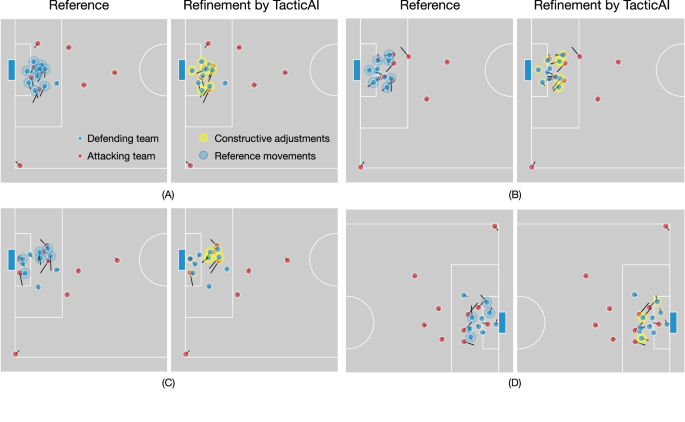
These examples are selected from our case study with human experts, to illustrate the breadth of tactical adjustments that TacticAI suggests to teams defending a corner. The density of the yellow circles coincides with the number of times that the corresponding change is recognised as constructive by human experts. Instead of optimising the movement of one specific player, TacticAI can recommend improvements for multiple players in one generation step through suggesting better positions to block the opposing players, or better orientations to track them more efficiently. Some specific comments from expert raters follow. In A , according to raters, TacticAI suggests more favourable positions for several defenders, and improved tracking runs for several others—further, the goalkeeper is positioned more deeply, which is also beneficial. In B , TacticAI suggests that the defenders furthest away from the corner make improved covering runs, which was unanimously deemed useful, with several other defenders also positioned more favourably. In C , TacticAI recommends improved covering runs for a central group of defenders in the penalty box, which was unanimously considered salient by our raters. And in D , TacticAI suggests substantially better tracking runs for two central defenders, along with a better positioning for two other defenders in the goal area.
We have demonstrated an AI assistant for football tactics and provided statistical evidence of its efficacy through a comprehensive case study with expert human raters from Liverpool FC. First, TacticAI is able to accurately predict the first receiver after a corner kick is taken as well as the probability of a shot as the direct result of the corner. Second, TacticAI has been shown to produce plausible tactical variations that improve outcomes in a salient way, while being indistinguishable from real scenarios by domain experts. And finally, the system’s latent player representations are a powerful means to retrieve similar set-piece tactics, allowing coaches to analyse relevant tactics and counter-tactics that have been successful in the past.
The broader scope of strategy modelling in football has previously been addressed from various individual angles, such as pass prediction 14 , 15 , 16 , shot prediction 3 or corner kick tactical classification 7 . However, to the best of our knowledge, our work stands out by combining and evaluating predictive and generative modelling of corner kicks for tactic development. It also stands out in its method of applying geometric deep learning, allowing for efficiently incorporating various symmetries of the football pitch for improved data efficiency. Our method incorporates minimal domain knowledge and does not rely on intricate feature engineering—though its factorised design naturally allows for more intricate feature engineering approaches when such features are available.
Our methodology requires the position and velocity estimates of all players at the time of execution of the corner and subsequent events. Here, we derive these from high-quality tracking and event data, with data availability from tracking providers limited to top leagues. Player tracking based on broadcast video would increase the reach and training data substantially, but would also likely result in noisier model inputs. While the attention mechanism of GATs would allow us to perform introspection of the most salient factors contributing to the model outcome, our method does not explicitly model exogenous (aleatoric) uncertainty, which would be valuable context for the football analyst.
While the empirical study of our method’s efficacy has been focused on corner kicks in association football, it readily generalises to other set pieces (such as throw-ins, which similarly benefit from similarity retrieval, pass and/or shot prediction) and other team sports with suspended play situations. The learned representations and overall framing of TacticAI also lay the ground for future research to integrate a natural language interface that enables domain-grounded conversations with the assistant, with the aim to retrieve particular situations of interest, make predictions for a given tactical variant, compare and contrast, and guide through an interactive process to derive tactical suggestions. It is thus our belief that TacticAI lays the groundwork for the next-generation AI assistant for football.
We devised TacticAI as a geometric deep learning pipeline, further expanded in this section. We process labelled spatio-temporal football data into graph representations, and train and evaluate on benchmarking tasks cast as classification or regression. These steps are presented in sequence, followed by details on the employed computational architecture.
Raw corner kick data
The raw dataset consisted of 9693 corner kicks collected from the 2020–21, 2021–22, and 2022–23 (up to January 2023) Premier League seasons. The dataset was provided by Liverpool FC and comprises four separate data sources, described below.
Our primary data source is spatio-temporal trajectory frames (tracking data), which tracked all on-pitch players and the ball, for each match, at 25 frames per second. In addition to player positions, their velocities are derived from position data through filtering. For each corner kick, we only used the frame in which the kick is being taken as input information.
Secondly, we also leverage event stream data, which annotated the events or actions (e.g., passes, shots and goals) that have occurred in the corresponding tracking frames.
Thirdly, the line-up data for the corresponding games, which recorded the players’ profiles, including their heights, weights and roles, is also used.
Lastly, we have access to miscellaneous game data, which contains the game days, stadium information, and pitch length and width in meters.
Graph representation and construction
We assumed that we were provided with an input graph \({{{{{{{\mathcal{G}}}}}}}}=({{{{{{{\mathcal{V}}}}}}}},\,{{{{{{{\mathcal{E}}}}}}}})\) with a set of nodes \({{{{{{{\mathcal{V}}}}}}}}\) and edges \({{{{{{{\mathcal{E}}}}}}}}\subseteq {{{{{{{\mathcal{V}}}}}}}}\times {{{{{{{\mathcal{V}}}}}}}}\) . Within the context of football games, we took \({{{{{{{\mathcal{V}}}}}}}}\) to be the set of 22 players currently on the pitch for both teams, and we set \({{{{{{{\mathcal{E}}}}}}}}={{{{{{{\mathcal{V}}}}}}}}\times {{{{{{{\mathcal{V}}}}}}}}\) ; that is, we assumed all pairs of players have the potential to interact. Further analyses, leveraging more specific choices of \({{{{{{{\mathcal{E}}}}}}}}\) , would be an interesting avenue for future work.
Additionally, we assume that the graph is appropriately featurised. Specifically, we provide a node feature matrix, \({{{{{{{\bf{X}}}}}}}}\in {{\mathbb{R}}}^{| {{{{{{{\mathcal{V}}}}}}}}| \times k}\) , an edge feature tensor, \({{{{{{{\bf{E}}}}}}}}\in {{\mathbb{R}}}^{| {{{{{{{\mathcal{V}}}}}}}}| \times | {{{{{{{\mathcal{V}}}}}}}}| \times l}\) , and a graph feature vector, \({{{{{{{\bf{g}}}}}}}}\in {{\mathbb{R}}}^{m}\) . The appropriate entries of these objects provide us with the input features for each node, edge, and graph. For example, \({{{{{{{{\bf{x}}}}}}}}}_{u}\in {{\mathbb{R}}}^{k}\) would provide attributes of an individual player \(u\in {{{{{{{\mathcal{V}}}}}}}}\) , such as position, height and weight, and \({{{{{{{{\bf{e}}}}}}}}}_{uv}\in {{\mathbb{R}}}^{l}\) would provide the attributes of a particular pair of players \((u,\, v)\in {{{{{{{\mathcal{E}}}}}}}}\) , such as their distance, and whether they belong to the same team. The graph feature vector, g , can be used to store global attributes of interest to the corner kick, such as the game time, current score, or ball position. For a simplified visualisation of how a graph neural network would process such an input, refer to Fig. 1 A.
To construct the input graphs, we first aligned the four data sources with respect to their game IDs and timestamps and filtered out 2517 invalid corner kicks, for which the alignment failed due to missing data, e.g., missing tracking frames or event labels. This filtering yielded 7176 valid corner kicks for training and evaluation. We summarised the exact information that was used to construct the input graphs in Table 2 . In particular, other than player heights (measured in centimeters (cm)) and weights (measured in kilograms (kg)), the players were anonymous in the model. For the cases in which the player profiles were missing, we set their heights and weights to 180 cm and 75 kg, respectively, as defaults. In total, we had 385 such occurrences out of a total of 213,246( = 22 × 9693) during data preprocessing. We downscaled the heights and weights by a factor of 100. Moreover, for each corner kick, we zero-centred the positions of on-pitch players and normalised them onto a 10 m × 10 m pitch, and their velocities were re-scaled accordingly. For the cases in which the pitch dimensions were missing, we used a standard pitch dimension of 110 m × 63 m as default.
We summarised the grouping of the features in Table 1 . The actual features used in different benchmark tasks may differ, and we will describe this in more detail in the next section. To focus on modelling the high-level tactics played by the attacking and defending teams, other than a binary indicator for ball possession—which is 1 for the corner kick taker and 0 for all other players—no information of ball movement, neither positions nor velocities, was used to construct the input graphs. Additionally, we do not have access to the player’s vertical movement, therefore only information on the two-dimensional movements of each player is provided in the data. We do however acknowledge that such information, when available, would be interesting to consider in a corner kick outcome predictor, considering the prevalence of aerial battles in corners.
Benchmark tasks construction
TacticAI consists of three predictive and generative models, which also correspond to three benchmark tasks implemented in this study. Specifically, (1) Receiver prediction, (2) Threatening shot prediction, and (3) Guided generation of team positions and velocities (Table 1 ). The graphs of all the benchmark tasks used the same feature space of nodes and edges, differing only in the global features.
For all three tasks, our models first transform the node features to a latent node feature matrix, \({{{{{{{\bf{H}}}}}}}}={f}_{{{{{{{{\mathcal{G}}}}}}}}}({{{{{{{\bf{X}}}}}}}},\, {{{{{{{\bf{E}}}}}}}},\, {{{{{{{\bf{g}}}}}}}})\) , from which we could answer queries: either about individual players—in which case we learned a relevant classifier or regressor over the h u vectors (the rows of H )—or about the occurrence of a global event (e.g. shot taken)—in which case we classified or regressed over the aggregated player vectors, ∑ u h u . In both cases, the classifiers were trained using stochastic gradient descent over an appropriately chosen loss function, such as categorical cross-entropy for classifiers, and mean squared error for regressors.
For different tasks, we extracted the corresponding ground-truth labels from either the event stream data or the tracking data. Specifically, (1) We modelled receiver prediction as a node classification task and labelled the first player to touch the ball after the corner was taken as the target node. This player could be either an attacking or defensive player. (2) Shot prediction was modelled as graph classification. In particular, we considered a next-ball-touch action by the attacking team as a shot if it was a direct corner, a goal, an aerial, hit on the goalposts, a shot attempt saved by the goalkeeper, or missing target. This yielded 1736 corners labelled as a shot being taken, and 5440 corners labelled as a shot not being taken. (3) For guided generation of player position and velocities, no additional label was needed, as this model relied on a self-supervised reconstruction objective.
The entire dataset was split into training and evaluation sets with an 80:20 ratio through random sampling, and the same splits were used for all tasks.
Graph neural networks
The central model of TacticAI is the graph neural network (GNN) 9 , which computes latent representations on a graph by repeatedly combining them within each node’s neighbourhood. Here we define a node’s neighbourhood, \({{{{{{{{\mathcal{N}}}}}}}}}_{u}\) , as the set of all first-order neighbours of node u , that is, \({{{{{{{{\mathcal{N}}}}}}}}}_{u}=\{v\,| \,(v,\, u)\in {{{{{{{\mathcal{E}}}}}}}}\}\) . A single GNN layer then transforms the node features by passing messages between neighbouring nodes 17 , following the notation of related work 10 , and the implementation of the CLRS-30 benchmark baselines 18 :
where \(\psi :{{\mathbb{R}}}^{k}\times {{\mathbb{R}}}^{k}\times {{\mathbb{R}}}^{l}\times {{\mathbb{R}}}^{m}\to {{\mathbb{R}}}^{{k}^{{\prime} }}\) and \(\phi :{{\mathbb{R}}}^{k}\times {{\mathbb{R}}}^{{k}^{{\prime} }}\to {{\mathbb{R}}}^{{k}^{{\prime} }}\) are two learnable functions (e.g. multilayer perceptrons), \({{{{{{{{\bf{h}}}}}}}}}_{u}^{(t)}\) are the features of node u after t GNN layers, and ⨁ is any permutation-invariant aggregator, such as sum, max, or average. By definition, we set \({{{{{{{{\bf{h}}}}}}}}}_{u}^{(0)}={{{{{{{{\bf{x}}}}}}}}}_{u}\) , and iterate Eq. ( 2 ) for T steps, where T is a hyperparameter. Then, we let \({{{{{{{\bf{H}}}}}}}}={f}_{{{{{{{{\mathcal{G}}}}}}}}}({{{{{{{\bf{X}}}}}}}},\, {{{{{{{\bf{E}}}}}}}},\, {{{{{{{\bf{g}}}}}}}})={{{{{{{{\bf{H}}}}}}}}}^{(T)}\) be the final node embeddings coming out of the GNN.
It is well known that Eq. ( 2 ) is remarkably general; it can be used to express popular models such as Transformers 19 as a special case, and it has been argued that all discrete deep learning models can be expressed in this form 20 , 21 . This makes GNNs a perfect framework for benchmarking various approaches to modelling player–player interactions in the context of football.
Different choices of ψ , ϕ and ⨁ yield different architectures. In our case, we utilise a message function that factorises into an attentional mechanism, \(a:{{\mathbb{R}}}^{k}\times {{\mathbb{R}}}^{k}\times {{\mathbb{R}}}^{l}\times {{\mathbb{R}}}^{m}\to {\mathbb{R}}\) :
yielding the graph attention network (GAT) architecture 12 . In our work, specifically, we use a two-layer multilayer perceptron for the attentional mechanism, as proposed by GATv2 11 :
where \({{{{{{{{\bf{W}}}}}}}}}_{1},\, {{{{{{{{\bf{W}}}}}}}}}_{2}\in {{\mathbb{R}}}^{k\times h}\) , \({{{{{{{{\bf{W}}}}}}}}}_{e}\in {{\mathbb{R}}}^{l\times h}\) , \({{{{{{{{\bf{W}}}}}}}}}_{g}\in {{\mathbb{R}}}^{m\times h}\) and \({{{{{{{\bf{a}}}}}}}}\in {{\mathbb{R}}}^{h}\) are the learnable parameters of the attentional mechanism, and LeakyReLU is the leaky rectified linear activation function. This mechanism computes coefficients of interaction (a single scalar value) for each pair of connected nodes ( u , v ), which are then normalised across all neighbours of u using the \({{{{{{{\rm{softmax}}}}}}}}\) function.
Through early-stage experimentation, we have ascertained that GATs are capable of matching the performance of more generic choices of ψ (such as the MPNN 17 ) while being more scalable. Hence, we focus our study on the GAT model in this work. More details can be found in the subsection “Ablation study” section.
Geometric deep learning
In spite of the power of Eq. ( 2 ), using it in its full generality is often prone to overfitting, given the large number of parameters contained in ψ and ϕ . This problem is exacerbated in the football analytics domain, where gold-standard data is generally very scarce—for example, in the English Premier League, only a few hundred games are played every season.
In order to tackle this issue, we can exploit the immense regularity of data arising from football games. Strategically equivalent game states are also called transpositions, and symmetries such as arriving at the same chess position through different move sequences have been exploited computationally since the 1960s 22 . Similarly, game rotations and reflections may yield equivalent strategic situations 23 . Using the blueprint of geometric deep learning (GDL) 10 , we can design specialised GNN architectures that exploit this regularity.
That is, geometric deep learning is a generic methodology for deriving mathematical constraints on neural networks, such that they will behave predictably when inputs are transformed in certain ways. In several important cases, these constraints can be directly resolved, directly informing neural network architecture design. For a comprehensive example of point clouds under 3D rotational symmetry, see Fuchs et al. 24 .
To elucidate several aspects of the GDL framework on a high level, let us assume that there exists a group of input data transformations (symmetries), \({\mathfrak{G}}\) under which the ground-truth label remains unchanged. Specifically, if we let y ( X , E , g ) be the label given to the graph featurised with X , E , g , then for every transformation \({\mathfrak{g}}\in {\mathfrak{G}}\) , the following property holds:
This condition is also referred to as \({\mathfrak{G}}\) -invariance. Here, by \({\mathfrak{g}}({{{{{{{\bf{X}}}}}}}})\) we denote the result of transforming X by \({\mathfrak{g}}\) —a concept also known as a group action. More generally, it is a function of the form \({\mathfrak{G}}\times {{{{{{{\mathcal{S}}}}}}}}\to {{{{{{{\mathcal{S}}}}}}}}\) for some state set \({{{{{{{\mathcal{S}}}}}}}}\) . Note that a single group element, \({\mathfrak{g}}\in {\mathfrak{G}}\) can easily produce different actions on different \({{{{{{{\mathcal{S}}}}}}}}\) —in this case, \({{{{{{{\mathcal{S}}}}}}}}\) could be \({{\mathbb{R}}}^{| {{{{{{{\mathcal{V}}}}}}}}| \times k}\) ( X ), \({{\mathbb{R}}}^{| {{{{{{{\mathcal{V}}}}}}}}| \times | {{{{{{{\mathcal{V}}}}}}}}| \times l}\) ( E ) and \({{\mathbb{R}}}^{m}\) ( g ).
It is worth noting that GNNs may also be derived using a GDL perspective if we set the symmetry group \({\mathfrak{G}}\) to \({S}_{| {{{{{{{\mathcal{V}}}}}}}}}|\) , the permutation group of \(| {{{{{{{\mathcal{V}}}}}}}}|\) objects. Owing to the design of Eq. ( 2 ), its outputs will not be dependent on the exact permutation of nodes in the input graph.
Frame averaging
A simple mechanism to enforce \({\mathfrak{G}}\) -invariance, given any predictor \({f}_{{{{{{{{\mathcal{G}}}}}}}}}({{{{{{{\bf{X}}}}}}}},\, {{{{{{{\bf{E}}}}}}}},\, {{{{{{{\bf{g}}}}}}}})\) , performs frame averaging across all \({\mathfrak{G}}\) -transformed inputs:
This ensures that all \({\mathfrak{G}}\) -transformed versions of a particular input (also known as that input’s orbit) will have exactly the same output, satisfying Eq. ( 5 ). A variant of this approach has also been applied in the AlphaGo architecture 25 to encode symmetries of a Go board.
In our specific implementation, we set \({\mathfrak{G}}={D}_{2}=\{{{{{{{{\rm{id}}}}}}}},\leftrightarrow,\updownarrow,\leftrightarrow \updownarrow \}\) , the dihedral group. Exploiting D 2 -invariance allows us to encode quadrant symmetries. Each element of the D 2 group encodes the presence of vertical or horizontal reflections of the input football pitch. Under these transformations, the pitch is assumed completely symmetric, and hence many predictions, such as which player receives the corner kick, or takes a shot from it, can be safely assumed unchanged. As an example of how to compute transformed features in Eq. ( 6 ), ↔( X ) horizontally reflects all positional features of players in X (e.g. the coordinates of the player), and negates the x -axis component of their velocity.
Group convolutions
While the frame averaging approach of Eq. ( 6 ) is a powerful way to restrict GNNs to respect input symmetries, it arguably misses an opportunity for the different \({\mathfrak{G}}\) -transformed views to interact while their computations are being performed. For small groups such as D 2 , a more fine-grained approach can be assumed, operating over a single GNN layer in Eq. ( 2 ), which we will write shortly as \({{{{{{{{\bf{H}}}}}}}}}^{(t)}={g}_{{{{{{{{\mathcal{G}}}}}}}}}({{{{{{{{\bf{H}}}}}}}}}^{(t-1)},\, {{{{{{{\bf{E}}}}}}}},\, {{{{{{{\bf{g}}}}}}}})\) . The condition that we need a symmetry-respecting GNN layer to satisfy is as follows, for all transformations \({\mathfrak{g}}\in {\mathfrak{G}}\) :
that is, it does not matter if we apply \({\mathfrak{g}}\) it to the input or the output of the function \({g}_{{{{{{{{\mathcal{G}}}}}}}}}\) —the final answer is the same. This condition is also referred to as \({\mathfrak{G}}\) -equivariance, and it has recently proved to be a potent paradigm for developing powerful GNNs over biochemical data 24 , 26 .
To satisfy D 2 -equivariance, we apply the group convolution approach 13 . Therein, views of the input are allowed to directly interact with their \({\mathfrak{G}}\) -transformed variants, in a manner very similar to grid convolutions (which is, indeed, a special case of group convolutions, setting \({\mathfrak{G}}\) to be the translation group). We use \({{{{{{{{\bf{H}}}}}}}}}_{{\mathfrak{g}}}^{(t)}\) to denote the \({\mathfrak{g}}\) -transformed view of the latent node features at layer t . Omitting E and g inputs for brevity, and using our previously designed layer \({g}_{{{{{{{{\mathcal{G}}}}}}}}}\) as a building block, we can perform a group convolution as follows:
Here, ∥ is the concatenation operation, joining the two node feature matrices column-wise; \({{\mathfrak{g}}}^{-1}\) is the inverse transformation to \({\mathfrak{g}}\) (which must exist as \({\mathfrak{G}}\) is a group); and \({{\mathfrak{g}}}^{-1}{\mathfrak{h}}\) is the composition of the two transformations.
Effectively, Eq. ( 8 ) implies our D 2 -equivariant GNN needs to maintain a node feature matrix \({{{{{{{{\bf{H}}}}}}}}}_{{\mathfrak{g}}}^{(t)}\) for every \({\mathfrak{G}}\) -transformation of the current input, and these views are recombined by invoking \({g}_{{{{{{{{\mathcal{G}}}}}}}}}\) on all pairs related together by applying a transformation \({\mathfrak{h}}\) . Note that all reflections are self-inverses, hence, in D 2 , \({\mathfrak{g}}={{\mathfrak{g}}}^{-1}\) .
It is worth noting that both the frame averaging in Eq. ( 6 ) and group convolution in Eq. ( 8 ) are similar in spirit to data augmentation. However, whereas standard data augmentation would only show one view at a time to the model, a frame averaging/group convolution architecture exhaustively generates all views and feeds them to the model all at once. Further, group convolutions allow these views to explicitly interact in a way that does not break symmetries. Here lies the key difference between the two approaches: frame averaging and group convolutions rigorously enforce the symmetries in \({\mathfrak{G}}\) , whereas data augmentation only provides implicit hints to the model about satisfying them. As a consequence of the exhaustive generation, Eqs. ( 6 ) and ( 8 ) are only feasible for small groups like D 2 . For larger groups, approaches like Steerable CNNs 27 may be employed.
Network architectures
While the three benchmark tasks we are performing have minor differences in the global features available to the model, the neural network models designed for them all have the same encoder–decoder architecture. The encoder has the same structure in all tasks, while the decoder model is tailored to produce appropriately shaped outputs for each benchmark task.
Given an input graph, TacticAI’s model first generates all relevant D 2 -transformed versions of it, by appropriately reflecting the player coordinates and velocities. We refer to the original input graph as the identity view, and the remaining three D 2 -transformed graphs as reflected views.
Once the views are prepared, we apply four group convolutional layers (Eq. ( 8 )) with a GATv2 base model (Eqs. ( 3 ) and ( 4 )) as the \({g}_{{{{{{{{\mathcal{G}}}}}}}}}\) function. Specifically, this means that, in Eqs. ( 3 ) and ( 4 ), every instance of \({{{{{{{{\bf{h}}}}}}}}}_{u}^{(t-1)}\) is replaced by the concatenation of \({({{{{{{{{\bf{h}}}}}}}}}_{{\mathfrak{h}}}^{(t-1)})}_{u}\parallel {({{{{{{{{\bf{h}}}}}}}}}_{{{\mathfrak{g}}}^{-1}{\mathfrak{h}}}^{(t-1)})}_{u}\) . Each GATv2 layer has eight attention heads and computes four latent features overall per player. Accordingly, once the four group convolutions are performed, we have a representation of \({{{{{{{\bf{H}}}}}}}}\in {{\mathbb{R}}}^{4\times 22\times 4}\) , where the first dimension corresponds to the four views ( \({{{{{{{{\bf{H}}}}}}}}}_{{{{{{{{\rm{id}}}}}}}}},\, {{{{{{{{\bf{H}}}}}}}}}_{\leftrightarrow },\, {{{{{{{{\bf{H}}}}}}}}}_{\updownarrow },\, {{{{{{{{\bf{H}}}}}}}}}_{\leftrightarrow \updownarrow }\in {{\mathbb{R}}}^{22\times 4}\) ), the second dimension corresponds to the players (eleven on each team), and the third corresponds to the 4-dimensional latent vector for each player node in this particular view. How this representation is used by the decoder depends on the specific downstream task, as we detail below.
For receiver prediction, which is a fully invariant function (i.e. reflections do not change the receiver), we perform simple frame averaging across all views, arriving at
and then learn a node-wise classifier over the rows of \({{{{{{{{\bf{H}}}}}}}}}^{{{{{{{{\rm{node}}}}}}}}}\in {{\mathbb{R}}}^{22\times 4}\) . We further decode H node into a logit vector \({{{{{{{\bf{O}}}}}}}}\in {{\mathbb{R}}}^{22}\) with a linear layer before computing the corresponding softmax cross entropy loss.
For shot prediction, which is once again fully invariant (i.e. reflections do not change the probability of a shot), we can further average the frame-averaged features across all players to get a global graph representation:
and then learn a binary classifier over \({{{{{{{{\bf{h}}}}}}}}}^{{{{{{{{\rm{graph}}}}}}}}}\in {{\mathbb{R}}}^{4}\) . Specifically, we decode the hidden vector into a single logit with a linear layer and compute the sigmoid binary cross-entropy loss with the corresponding label.
For guided generation (position/velocity adjustments), we generate the player positions and velocities with respect to a particular outcome of interest for the human coaches, predicted over the rows of the hidden feature matrix. For example, the model may adjust the defensive setup to decrease the shot probability by the attacking team. The model output is now equivariant rather than invariant—reflecting the pitch appropriately reflects the predicted positions and velocity vectors. As such, we cannot perform frame averaging, and take only the identity view’s features, \({{{{{{{{\bf{H}}}}}}}}}_{{{{{{{{\rm{id}}}}}}}}}\in {{\mathbb{R}}}^{22\times 4}\) . From this latent feature matrix, we can then learn a conditional distribution from each row, which models the positions or velocities of the corresponding player. To do this, we extend the backbone encoder with conditional variational autoencoder (CVAE 28 , 29 ). Specifically, for the u -th row of H id , h u , we first map its latent embedding to the parameters of a two-dimensional Gaussian distribution \({{{{{{{\mathcal{N}}}}}}}}({\mu }_{u}| {\sigma }_{u})\) , and then sample the coordinates and velocities from this distribution. At training time, we can efficiently propagate gradients through this sampling operation using the reparameterisation trick 28 : sample a random value \({\epsilon }_{u} \sim {{{{{{{\mathcal{N}}}}}}}}(0,1)\) for each player from the unit Gaussian distribution, and then treat μ u + σ u ϵ u as the sample for this player. In what follows, we omit edge features for brevity. For each corner kick sample X with the corresponding outcome o (e.g. a binary value indicating a shot event), we extend the standard VAE loss 28 , 29 to our case of outcome-conditional guided generation as
where h u is the player embedding corresponding to the u th row of H id , and \({\mathbb{KL}}\) is Kullback–Leibler (KL) divergence. Specifically, the first term is the generation loss between the real player input x u and the reconstructed sample decoded from h u with the decoder p ϕ . Using the KL term, the distribution of the latent embedding h u is regularised towards p ( h u ∣ o ), which is a multivariate Gaussian in our case.
A complete high-level summary of the generic encoder–decoder equivariant architecture employed by TacticAI can be summarised in Supplementary Fig. 2 . In the following section, we will provide empirical evidence for justifying these architectural decisions. This will be done through targeted ablation studies on our predictive benchmarks (receiver prediction and shot prediction).
Ablation study
We leveraged the receiver prediction task as a way to evaluate various base model architectures, and directly quantitatively assess the contributions of geometric deep learning in this context. We already see that the raw corner kick data can be better represented through geometric deep learning, yielding separable clusters in the latent space that could correspond to different attacking or defending tactics (Fig. 2 ). In addition, we hypothesise that these representations can also yield better performance on the task of receiver prediction. Accordingly, we ablate several design choices using deep learning on this task, as illustrated by the following four questions:
Does a factorised graph representation help? To assess this, we compare it against a convolutional neural network (CNN 30 ) baseline, which does not leverage a graph representation.
Does a graph structure help? To assess this, we compare against a Deep Sets 31 baseline, which only models each node in isolation without considering adjacency information—equivalently, setting each neighbourhood \({{{{{{{{\mathcal{N}}}}}}}}}_{u}\) to a singleton set { u }.
Are attentional GNNs a good strategy? To assess this, we compare against a message passing neural network 32 , MPNN baseline, which uses the fully potent GNN layer from Eq. ( 2 ) instead of the GATv2.
Does accounting for symmetries help? To assess this, we compare our geometric GATv2 baseline against one which does not utilise D 2 group convolutions but utilises D 2 frame averaging, and one which does not explicitly utilise any aspect of D 2 symmetries at all.
Each of these models has been trained for a fixed budget of 50,000 training steps. The test top- k receiver prediction accuracies of the trained models are provided in Supplementary Table 2 . As already discussed in the section “Results”, there is a clear advantage to using a full graph structure, as well as directly accounting for reflection symmetry. Further, the usage of the MPNN layer leads to slight overfitting compared to the GATv2, illustrating how attentional GNNs strike a good balance of expressivity and data efficiency for this task. Our analysis highlights the quantitative benefits of both graph representation learning and geometric deep learning for football analytics from tracking data. We also provide a brief ablation study for the shot prediction task in Supplementary Table 3 .
Training details
We train each of TacticAI’s models in isolation, using NVIDIA Tesla P100 GPUs. To minimise overfitting, each model’s learning objective is regularised with an L 2 norm penalty with respect to the network parameters. During training, we use the Adam stochastic gradient descent optimiser 33 over the regularised loss.
All models, including baselines, have been given an equal hyperparameter tuning budget, spanning the number of message passing steps ({1, 2, 4}), initial learning rate ({0.0001, 0.00005}), batch size ({128, 256}) and L 2 regularisation coefficient ({0.01, 0.005, 0.001, 0.0001, 0}). We summarise the chosen hyperparameters of each TacticAI model in Supplementary Table 1 .
Data availability
The data collected in the human experiments in this study have been deposited in the Zenodo database under accession code https://zenodo.org/records/10557063 , and the processed data which is used in the statistical analysis and to generate the relevant figures in the main text are available under the same accession code. The input and output data generated and/or analysed during the current study are protected and are not available due to data privacy laws and licensing restrictions. However, contact details of the input data providers are available from the corresponding authors on reasonable request.
Code availability
All the core models described in this research were built with the Graph Neural Network processors provided by the CLRS Algorithmic Reasoning Benchmark 18 , and their source code is available at https://github.com/google-deepmind/clrs . We are unable to release our code for this work as it was developed in a proprietary context; however, the corresponding authors are open to answer specific questions concerning re-implementations on request. For general data analysis, we used the following freely available packages: numpy v1.25.2 , pandas v1.5.3 , matplotlib v3.6.1 , seaborn v0.12.2 and scipy v1.9.3 . Specifically, the code of the statistical analysis conducted in this study is available at https://zenodo.org/records/10557063 .
The International Football Association Board (IFAB). Laws of the Game (The International Football Association Board, 2023).
Tuyls, K. et al. Game plan: what AI can do for football, and what football can do for AI. J. Artif. Intell. Res. 71 , 41–88 (2021).
Article Google Scholar
Goka, R., Moroto, Y., Maeda, K., Ogawa, T. & Haseyama, M. Prediction of shooting events in soccer videos using complete bipartite graphs and players’ spatial–temporal relations. Sensors 23 , 4506 (2023).
Article ADS PubMed PubMed Central Google Scholar
Omidshafiei, S. et al. Multiagent off-screen behavior prediction in football. Sci. Rep. 12 , 8638 (2022).
Article ADS CAS PubMed PubMed Central Google Scholar
Lang, S., Wild, R., Isenko, A. & Link, D. Predicting the in-game status in soccer with machine learning using spatiotemporal player tracking data. Sci. Rep. 12 , 16291 (2022).
Baccouche, M., Mamalet, F., Wolf, C., Garcia, C. & Baskurt, A. Action classification in soccer videos with long short-term memory recurrent neural networks. In International Conference on Artificial Neural Networks (eds Diamantaras, K., Duch, W. & Iliadis, L. S.) pages 154–159 (Springer, 2010).
Shaw, L. & Gopaladesikan, S. Routine inspection: a playbook for corner kicks. In Machine Learning and Data Mining for Sports Analytics: 7th International Workshop, MLSA 2020, Co-located with ECML/PKDD 2020 , Proceedings, Ghent, Belgium, September 14–18, 2020, Vol . 7 , 3–16 (Springer, 2020).
Araújo, D. & Davids, K. Team synergies in sport: theory and measures. Front. Psychol. 7 , 1449 (2016).
Article PubMed PubMed Central Google Scholar
Veličković, P. Everything is connected: graph neural networks. Curr. Opin. Struct. Biol. 79 , 102538 (2023).
Article PubMed Google Scholar
Bronstein, M. M., Bruna, J., Cohen, T. & Veličković, P. Geometric deep learning: grids, groups, graphs, geodesics, and gauges. arXiv preprint arXiv:2104.13478 (2021).
Brody, S., Alon, U. & Yahav, E. How attentive are graph attention networks? In International Conference on Learning Representations (ICLR, 2022). https://openreview.net/forum?id=F72ximsx7C1 .
Veličković, P. et al. Graph attention networks. In International Conference on Learning Representations (ICLR, 2018). https://openreview.net/forum?id=rJXMpikCZ .
Cohen, T. & Welling, M. Group equivariant convolutional networks. In International Conference on Machine Learning (eds Balcan, M. F. & Weinberger, K. Q.) 2990–2999 (PMLR, 2016).
Honda, Y., Kawakami, R., Yoshihashi, R., Kato, K. & Naemura, T. Pass receiver prediction in soccer using video and players’ trajectories. In 2022 IEEE/CVF Conference on Computer Vision and Pattern Recognition Workshops (CVPRW) 3502–3511 (2022). https://ieeexplore.ieee.org/document/9857310 .
Hubáček, O., Sourek, G. & Železný, F. Deep learning from spatial relations for soccer pass prediction. In MLSA@PKDD/ECML (eds Brefeld, U., Davis, J., Van Haaren, J. & Zimmermann, A.) Vol. 11330, (Lecture Notes in Computer Science, Springer, Cham, 2018).
Sanyal, S. Who will receive the ball? Predicting pass recipient in soccer videos. J Visual Commun. Image Represent. 78 , 103190 (2021).
Gilmer, J., Schoenholz, S. S., Riley, P. F., Vinyals, O. & Dahl, G. E. Neural message passing for quantum chemistry. In International Conference on Machine Learning (Precup, D. & Teh, Y. W.) 1263–1272 (PMLR, 2017).
Veličković, P. et al. The CLRS algorithmic reasoning benchmark. In International Conference on Machine Learning (eds Chaudhuri, K. et al.) 22084–22102 (PMLR, 2022).
Vaswani, A. et al. Attention is all you need. In Advances in Neural Information Processing Systems (eds Guyon, I. et al.) Vol. 30 (Curran Associates, Inc., 2017).
Veličković, P. Message passing all the way up. In ICLR 2022 Workshop on Geometrical and Topological Representation Learning (GTRL, 2022). https://openreview.net/forum?id=Bc8GiEZkTe5 .
Baranwal, A., Kimon, F. & Aukosh, J. Optimality of message-passing architectures for sparse graphs. In Thirty-seventh Conference on Neural Information Processing Systems (2023). https://papers.nips.cc/paper_files/paper/2023/hash/7e991aa4cd2fdf0014fba2f000f542d0-Abstract-Conference.html .
Greenblatt, R. D., Eastlake III, D. E. & Crocker, S. D. The Greenblatt chess program. In Proc. Fall Joint Computer Conference , 14–16 , 801–810 (Association for Computing Machinery, 1967). https://dl.acm.org/doi/10.1145/1465611.1465715 .
Schijf, M., Allis, L. V. & Uiterwijk, J. W. Proof-number search and transpositions. ICGA J. 17 , 63–74 (1994).
Fuchs, F., Worrall, D., Fischer, V. & Welling, M. SE(3)-transformers: 3D roto-translation equivariant attention networks. Adv. Neural Inf. Process. Syst. 33 , 1970–1981 (2020).
Google Scholar
Silver, D. et al. Mastering the game of Go with deep neural networks and tree search. Nature 529 , 484–489 (2016).
Article ADS CAS PubMed Google Scholar
Satorras, V. G., Hoogeboom, E. & Welling, M. E ( n ) equivariant graph neural networks. In International Conference on Machine Learning (eds Meila, M. & Zhang, T.) 9323–9332 (PMLR, 2021).
Cohen, T. S. & Welling, M. Steerable CNNs. In International Conference on Learning Representations (ICLR, 2017). https://openreview.net/forum?id=rJQKYt5ll .
Kingma, D. P. & Welling, M. Auto-encoding variational Bayes. In 2nd International Conference on Learning Representations, ICLR 2014, Banff, AB, Canada, April 14–16, 2014, Conference Track Proceedings (ICLR, 2014). https://openreview.net/forum?id=33X9fd2-9FyZd .
Sohn, K., Lee, H. & Yan, X. Learning structured output representation using deep conditional generative models. In Advances in Neural Information Processing Systems (eds Cortes, C, Lawrence, N., Lee, D., Sugiyama, M. & Garnett, R.) Vol. 28 (Curran Associates, Inc., 2015).
Fernández, J. & Bornn, L. Soccermap: a deep learning architecture for visually-interpretable analysis in soccer. In Machine Learning and Knowledge Discovery in Databases. Applied Data Science and Demo Track: European Conference, ECML PKDD 2020, Ghent, Belgium, September 14–18, 2020, Proceedings, Part V (eds Dong, Y., Ifrim, G., Mladenić, D., Saunders, C. & Van Hoecke, S.) 491–506 (Springer, 2021).
Zaheer, M. et al. Deep sets. In Advances in Neural Information Processing Systems Vol. 30 (eds Guyon, I., et al.) (Curran Associates, Inc., 2017).
Gilmer, J., Schoenholz, S. S., Riley, P. F., Vinyals, O. & Dahl, G. E. Neural message passing for quantum chemistry. In Proc. 34th International Conference on Machine Learning , Vol. 70 of Proceedings of Machine Learning Research, 6–11 Aug 2017 (eds Precup, D. & Whye Teh, Y) 1263–1272 (PMLR, 2017).
Kingma, G. E. & Ba, J. Adam: a method for stochastic optimization. In ICLR (Poster) , (eds Bengio, Y. & LeCun, Y.) (International Conference of Learning Representations (ICLR), 2015). https://openreview.net/forum?id=8gmWwjFyLj .
Download references
Acknowledgements
We gratefully acknowledge the support of James French, Timothy Waskett, Hans Leitert and Benjamin Hervey for their extensive efforts in analysing TacticAI’s outputs. Further, we are thankful to Kevin McKee, Sherjil Ozair and Beatrice Bevilacqua for useful technical discussions, and Marc Lanctôt and Satinder Singh for reviewing the paper prior to submission.
Author information
These authors contributed equally: Zhe Wang, Petar Veličković, Daniel Hennes.
Authors and Affiliations
Google DeepMind, 6-8 Handyside Street, London, N1C 4UZ, UK
Zhe Wang, Petar Veličković, Daniel Hennes, Nenad Tomašev, Laurel Prince, Michael Kaisers, Yoram Bachrach, Romuald Elie, Li Kevin Wenliang, Federico Piccinini, Jerome Connor, Yi Yang, Adrià Recasens, Mina Khan, Nathalie Beauguerlange, Pablo Sprechmann, Pol Moreno, Nicolas Heess & Demis Hassabis
Liverpool FC, AXA Training Centre, Simonswood Lane, Kirkby, Liverpool, L33 5XB, UK
William Spearman
Liverpool FC, Kirkby, UK
University of Alberta, Amii, Edmonton, AB, T6G 2E8, Canada
Michael Bowling
Google DeepMind, London, UK
You can also search for this author in PubMed Google Scholar
Contributions
Z.W., D. Hennes, L.P. and K.T. coordinated and organised the research effort leading to this paper. P.V. and Z.W. developed the core TacticAI models. Z.W., W.S. and I.G. prepared the Premier League corner kick dataset used for training and evaluating these models. P.V., Z.W., D. Hennes and N.T. designed the case study with human experts and Z.W. and P.V. performed the qualitative evaluation and statistical analysis of its outcomes. Z.W., P.V., D. Hennes, N.T., L.P., M. Kaisers, Y.B., R.E., L.K.W., F.P., W.S., I.G., N.H., M.B., D. Hassabis and K.T. contributed to writing the paper and providing feedback on the final manuscript. J.C., Y.Y., A.R., M. Khan, N.B., P.S. and P.M. contributed valuable technical and implementation discussions throughout the work’s development.
Corresponding authors
Correspondence to Zhe Wang , Petar Veličković or Karl Tuyls .
Ethics declarations
Competing interests.
The authors declare no competing interests but the following competing interests: TacticAI was developed during the course of the Authors’ employment at Google DeepMind and Liverpool Football Club, as applicable to each Author.
Peer review
Peer review information.
Nature Communications thanks Rui Luo and the other anonymous reviewer(s) for their contribution to the peer review of this work. A peer review file is available.
Additional information
Publisher’s note Springer Nature remains neutral with regard to jurisdictional claims in published maps and institutional affiliations.
Supplementary information
Supplementary information, peer review file, rights and permissions.
Open Access This article is licensed under a Creative Commons Attribution 4.0 International License, which permits use, sharing, adaptation, distribution and reproduction in any medium or format, as long as you give appropriate credit to the original author(s) and the source, provide a link to the Creative Commons licence, and indicate if changes were made. The images or other third party material in this article are included in the article’s Creative Commons licence, unless indicated otherwise in a credit line to the material. If material is not included in the article’s Creative Commons licence and your intended use is not permitted by statutory regulation or exceeds the permitted use, you will need to obtain permission directly from the copyright holder. To view a copy of this licence, visit http://creativecommons.org/licenses/by/4.0/ .
Reprints and permissions
About this article
Cite this article.
Wang, Z., Veličković, P., Hennes, D. et al. TacticAI: an AI assistant for football tactics. Nat Commun 15 , 1906 (2024). https://doi.org/10.1038/s41467-024-45965-x
Download citation
Received : 13 October 2023
Accepted : 08 February 2024
Published : 19 March 2024
DOI : https://doi.org/10.1038/s41467-024-45965-x
Share this article
Anyone you share the following link with will be able to read this content:
Sorry, a shareable link is not currently available for this article.
Provided by the Springer Nature SharedIt content-sharing initiative
By submitting a comment you agree to abide by our Terms and Community Guidelines . If you find something abusive or that does not comply with our terms or guidelines please flag it as inappropriate.
Quick links
- Explore articles by subject
- Guide to authors
- Editorial policies
Sign up for the Nature Briefing newsletter — what matters in science, free to your inbox daily.

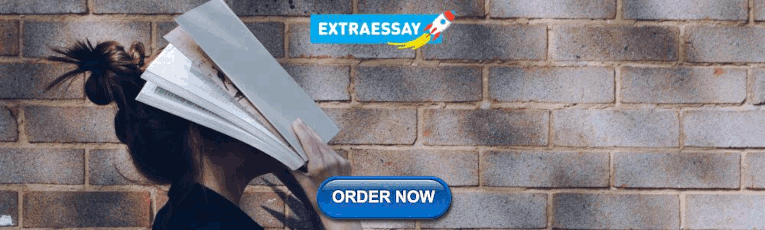
IMAGES
VIDEO
COMMENTS
Research methods are specific procedures for collecting and analyzing data. Developing your research methods is an integral part of your research design. When planning your methods, there are two key decisions you will make. First, decide how you will collect data. Your methods depend on what type of data you need to answer your research question:
Your research methodology discusses and explains the data collection and analysis methods you used in your research. A key part of your thesis, dissertation, or research paper, the methodology chapter explains what you did and how you did it, allowing readers to evaluate the reliability and validity of your research and your dissertation topic.
Research papers in the social and natural sciences often follow APA style. This article focuses on reporting quantitative research methods. In your APA methods section, you should report enough information to understand and replicate your study, including detailed information on the sample, measures, and procedures used.
4. Use subheadings: Dividing the Methods section in terms of the experiments helps the reader to follow the section better. You may write the specific objective of each experiment as a subheading. Alternatively, if applicable, the name of each experiment can also be used as subheading. 5.
Your Methods Section contextualizes the results of your study, giving editors, reviewers and readers alike the information they need to understand and interpret your work. Your methods are key to establishing the credibility of your study, along with your data and the results themselves. A complete methods section should provide enough detail ...
I. Groups of Research Methods. There are two main groups of research methods in the social sciences: The empirical-analytical group approaches the study of social sciences in a similar manner that researchers study the natural sciences.This type of research focuses on objective knowledge, research questions that can be answered yes or no, and operational definitions of variables to be measured.
The methodology section of your paper describes how your research was conducted. This information allows readers to check whether your approach is accurate and dependable. A good methodology can help increase the reader's trust in your findings. First, we will define and differentiate quantitative and qualitative research.
Revised on 10 October 2022. Your research methodology discusses and explains the data collection and analysis methods you used in your research. A key part of your thesis, dissertation, or research paper, the methodology chapter explains what you did and how you did it, allowing readers to evaluate the reliability and validity of your research.
Writing the Research Paper. Write a detailed outline. Almost the rough content of every paragraph. The order of the various topics in your paper. On the basis of the outline, start writing a part by planning the content, and then write it down. Put a visible mark (which you will later delete) where you need to quote a source, and write in the ...
INTRODUCTION. Scientific research is usually initiated by posing evidenced-based research questions which are then explicitly restated as hypotheses.1,2 The hypotheses provide directions to guide the study, solutions, explanations, and expected results.3,4 Both research questions and hypotheses are essentially formulated based on conventional theories and real-world processes, which allow the ...
To write your methods section in APA format, describe your participants, materials, study design, and procedures. Keep this section succinct, and always write in the past tense. The main heading of this section should be labeled "Method" and it should be centered, bolded, and capitalized. Each subheading within this section should be bolded ...
Definition, Types, and Examples. Research methodology 1,2 is a structured and scientific approach used to collect, analyze, and interpret quantitative or qualitative data to answer research questions or test hypotheses. A research methodology is like a plan for carrying out research and helps keep researchers on track by limiting the scope of ...
Allow enough time. First and foremost, it's vital to allow enough time for your research. For this reason, don't leave your essay until the last minute. If you start writing without having done adequate research, it will almost certainly show in your essay's lack of quality. The amount of research time needed will vary according to ...
Download Article. 1. Break up your essay into sub-topics. You will probably need to address several distinct aspects of your research topic in your essay. This is an important tactic for producing a well-organized research essay because it avoids 'stream of consciousness' writing, which typically lacks order.
The research methodology is an important section of any research paper or thesis, as it describes the methods and procedures that will be used to conduct the research. It should include details about the research design, data collection methods, data analysis techniques, and any ethical considerations.
Quantitative research methods are used to collect and analyze numerical data. This type of research is useful when the objective is to test a hypothesis, determine cause-and-effect relationships, and measure the prevalence of certain phenomena. Quantitative research methods include surveys, experiments, and secondary data analysis.
Conduct preliminary research. Develop a thesis statement. Create a research paper outline. Write a first draft of the research paper. Write the introduction. Write a compelling body of text. Write the conclusion. The second draft. The revision process.
3. Mixed-Methods research methodology. Mixed-methods research combines both qualitative and quantitative research methodologies to provide a more comprehensive understanding of the research problem. This approach leverages the strengths of both methodologies to provide a deeper insight into the research question of a research paper.
Abstract. This paper aims to provide an overview of the use and assessment of qualitative research methods in the health sciences. Qualitative research can be defined as the study of the nature of phenomena and is especially appropriate for answering questions of why something is (not) observed, assessing complex multi-component interventions ...
Step 1: Start with Study Design. The initial step in the method section of a research paper is to provide a clear description of the study type. This involves outlining the overall plan and structure of the research. Different types of studies, such as cohort, case-control, and cross-sectional, may be employed based on the research objectives.
Here are some tips for writing strong examples of research methodology. 1. Concentrate on your purposes and research questions. You should clearly show why your methods match your objectives and persuade the readers that you've selected the best possible method to answer your research questions and problem statement.
INTRODUCTION. This chapter presents the research methodologies adopted for the research. A combination of both qualitative and quantitative methodological approaches was adopted by the researcher in order to attain a realistic result from the research. Specifically, the chapter discusses the range of methods used by the researcher for the ...
Research Methods: Application And Evaluation. Introduction This research paper examines the usability and demand of the thread mill bicycle in the UK market. The concept of the thread mill bicycle is a new concept and it's quite useful for the users. This process combines different mechanical parts and improves its usefulness for the users.
Table of contents. Step 1: Introduce your topic. Step 2: Describe the background. Step 3: Establish your research problem. Step 4: Specify your objective (s) Step 5: Map out your paper. Research paper introduction examples. Frequently asked questions about the research paper introduction.
Indicate the methods used to address missing data. Indicate institutional review board/ethics review, approval, waiver, or exemption. Describe methods or analyses included to address and manage AI-related methodologic bias and inaccuracy of AI-generated content.
In this work, we discuss building performant Multimodal Large Language Models (MLLMs). In particular, we study the importance of various architecture components and data choices. Through careful and comprehensive ablations of the image encoder, the vision language connector, and various pre-training data choices, we identified several crucial design lessons. For example, we demonstrate that ...
Identifying key patterns of tactics implemented by rival teams, and developing effective responses, lies at the heart of modern football. However, doing so algorithmically remains an open research ...