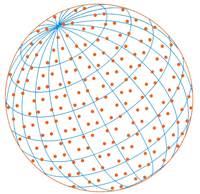
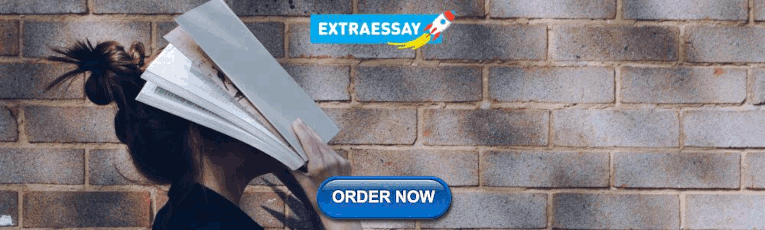
Analysis of Air Pollution Data in India between 2015 and 2019
1 Center for Policy Research on Energy and Environment, School of Public and International Affairs, Princeton University, Princeton, NJ 08544, USA 2 Department of Civil and Environmental Engineering, Princeton University, Princeton, NJ 08544, USA
Copyright The Author(s). This is an open access article distributed under the terms of the Creative Commons Attribution License (CC BY 4.0) , which permits unrestricted use, distribution, and reproduction in any medium, provided the original author and source are cited.
- Download: PDF | Supplemental Material
Sharma, D., Mauzerall, D. (2022). Analysis of Air Pollution Data in India between 2015 and 2019. Aerosol Air Qual. Res. 22, 210204. https://doi.org/10.4209/aaqr.210204
- Analysis of PM 10 , PM 2.5 , SO 2 , NO 2 and O 3 measurements across India from 2015–2019.
- First comprehensive analysis of Indian government and US Air-Now data.
- More national ambient air quality standard exceedances in north than south India.
- Provides baseline for evaluation of mitigation measures and atmospheric models.
India suffers from among the worst air pollution in the world. In response, a large government effort to increase air quality monitoring is underway. We present the first comprehensive analysis of government air quality observations from 2015–2019 for PM 10 , PM 2.5 , SO 2 , NO 2 and O 3 from the Central Pollution Control Board (CPCB) Continuous Ambient Air Quality Monitoring (CAAQM) network and the manual National Air Quality Monitoring Program (NAMP), as well as PM 2.5 from the US Air-Now network. We address inconsistencies and data gaps in datasets using a rigorous procedure to ensure data representativeness. We find particulate pollution dominates the pollution mix across India with virtually all sites in northern India (divided at 23.5°N) exceeding the annual average PM 10 and PM 2.5 residential national ambient air quality standards (NAAQS) by 150% and 100% respectively, and in southern India exceeding the PM 10 standard by 50% and the PM 2.5 standard by 40%. Annual average SO 2 , NO 2 and MDA8 O 3 generally meet the residential NAAQS across India. Northern India has (~10%–130%) higher concentrations of all pollutants than southern India, with only SO 2 having similar concentrations. Although inter-annual variability exists, we found no significant trend of these pollutants over the five-year period. In the five cities with Air-Now PM 2.5 measurements - Delhi, Kolkata, Mumbai, Hyderabad and Chennai, there is reasonable agreement with CPCB data. The PM 2.5 CPCB CAAQM data compares well with satellite derived annual surface PM 2.5 concentrations (Hammer et al. , 2020), with the exception of the western desert region prior to 2018 when surface measurements exceeded satellite retrievals. Our reanalyzed dataset is useful for evaluation of Indian air quality from satellite data, atmospheric models, and low-cost sensors. Our dataset also provides a baseline to evaluate the future success of National Clean Air Programme as well as aids in assessment of existing and future air pollution mitigation policies.
Keywords: Air pollution, India, surface observations, CPCB, continuous and manual data, US AirNow
1 INTRODUCTION
Concerns over poor air quality in India have increased over the past few years with increasing evidence of the adverse impacts on health (Balakrishnan et al. , 2014; Chowdhury and Dey, 2016; Balakrishnan et al. , 2019), agricultural yields (Avnery et al. , 2011, 2013; Ghude et al. , 2014; Gao et al. , 2020) and the economy (Pandey et al. , 2021). Rapid growth and industrialization in India have resulted in some of the most polluted air in the world. Projections forecast further decreases in air quality and a 24% increase in PM 2.5 associated premature mortalities by 2050 relative to 2015 (GBD MAPS Working Group, 2018; Brauer et al. , 2019). According to recent estimates based on the Global Exposure Mortality Model (GEMM), total premature mortality due to ambient PM 2.5 exposure in India increased approximately 47% between 2000 and 2015 (Chowdhury et al. , 2020). Surface O 3 concentrations are also likely to increase with growing industrial emissions and increasing temperatures due to climate change resulting in additional stress on agricultural yields and public health (Avnery et al. , 2011; Silva et al. , 2017).
India has a national ambient surface monitoring network that started in 1987 and has become more extensive over time with a substantial increase in the number and spatial extent of continuous and manual monitoring stations between 2015 and 2019. At present, the Central Pollution Control Board (CPCB), along with the State Pollution Control Boards (SPCBs), run the most extensive monitoring network in the country under the National Air Quality Monitoring Program (NAMP). As of 2019, NAMP cooperatively operated (with CPCB and SPCBs) over 750 manual monitoring stations (compared with 20 in 1987 when monitoring first began and 450 in 2015 when our analysis starts) which publicly archive annual average concentrations of PM 10 , PM 2.5 , SO 2 and NO 2 ( https://cpcb.nic.in/namp-data/ ). As of 2019, over 220 Continuous Ambient Air Quality Monitoring (CAAQM) stations operated (compared with less than 50 stations in 2015 when our analysis starts). CPCB archives publicly available, real time data, every 15 minutes, from over 220 stations across India of an extensive list of criteria and non-criteria air pollutants and meteorological variables ( https://app.cpcbccr.com/ccr/ ). Stations vary in the air pollutant species and meteorological data they collect. The manual monitors provide better spatial coverage than the continuous monitors but provide data on fewer air pollutants at much lower temporal resolution (annual average values versus every 15 minutes). However, both sets of monitoring stations sample exclusively urban areas despite the fact that rural areas have significant emissions from households and agricultural waste burning (Balakrishnan et al. , 2014; Venkatraman et al. , 2018). Pant et al. (2019) and the Supplementary Information (SI) (Section 1) describe other Indian monitoring networks which are less extensive and are not publicly available. India has fewer monitoring stations than most south and east Asian countries, with ~1 monitor/6.8 million persons (Apte and Pant 2019; Brauer et al. , 2019; Martin et al. , 2019). Despite recent increases in urban monitoring stations across India, vast regions do not have monitors and except for satellite data for a few species, little information is available on surface concentrations of air pollutants in non-urban locations in India.
Recently, extreme levels of fine particulate air pollution in India, combined with a growing appreciation of the adverse impacts of elevated air pollution on health, led the Indian government to launch the National Clean Air Program (NCAP) in 2019 (Ministry of Environment, Forests and Climate Change NCAP, 2019). NCAP targets a reduction of 20–30% in PM 10 and PM 2.5 concentrations by 2024 relative to 2017 levels. One focus of NCAP is augmentation of the national monitoring network for which substantial financial support was announced in the 2020 Union Budget.
Despite a growing monitoring network and the need for analysis, prior to our work, no study holistically analyzed existing government surface air pollutant monitoring data across India. Most research studies analyzing ground monitoring data have focused on Delhi and the surrounding National Capital Region (NCR) (Guttikunda and Gurjar, 2012; Sahu and Kota, 2017; Sharma et al. , 2018; Chowdhury et al. , 2019; Guttikunda et al. , 2019; Wang and Chen, 2019; Hama et al. , 2020), and other major cities (Gurjar et al. , 2016; Sreekanth et al. , 2018, Yang et al. , 2018; Chen et al. , 2020). In addition, some studies also used ground observations to bias correct satellite measurements for India (Pande et al. , 2018; Chowdhury et al. , 2019; Navinya et al. , 2020). However, a need remains for a comprehensive analysis of all surface data collected by manual NAMP and continuous CAAQM monitoring networks between 2015–2019 over which period monitoring increased substantially.
Here we provide the first national analysis of all available surface measurements of key criteria pollutants (PM 10 , PM 2.5 , SO 2 , NO 2 and O 3 ) across India between 2015–2019. We use publicly available data from the NAMP manual and CAAQM real-time stations which have different spatial distributions and temporal resolutions. Collating spatio-temporal distributions of pollutant concentrations on inter-annual, annual, seasonal and monthly timescales, we present an overview of the variability in air pollution levels across the country and separately analyze pollution levels in northern (north of 23°N) and southern India. We conduct case studies of five cities in India in which U.S. State Department PM 2.5 monitors (Air-Now network) are present and, using additional data collected by CAAQM monitors, compare pollution status between these cities. We also compare analyzed annual average PM 2.5 from the CAAQM network with the satellite derived surface PM 2.5 (Hammer et al. , 2020) and find good agreement between the two datasets. Our analysis will provide a valuable baseline to evaluate the future success of the NCAP in meeting its air pollution mitigation targets.
2 METHODOLOGY
2.1 criteria pollutant data.
We analyze all open-source data available from the manual (NAMP) and continuous (CAAQM) networks, as well as from the US Embassy and consulates Air-Now network from 2015–2019 for five criteria pollutants—PM 10 , PM 2.5 , SO 2 , NO 2 and O 3 .
Datasets from 2015-2018 were acquired for NAMP and were acquired from 2015–2019 for CPCB-CAAQM and Air-Now networks directly from the following sources:
- NAMP manual monitoring network ( https://cpcb.nic.in/namp-data/ ): Annual average and annual maximum and minimum concentrations were obtained from a total of 730 manual stations. Higher resolution temporal measurements are not publicly reported by NAMP. We analyze data from 2015–2018 as datasets for 2019 were unavailable when our analysis was completed in December 2020.
- CAAQM continuous monitoring network from the Central Control Room for Air Quality Management website ( https://app.cpcbccr.com/ccr/ ): One-hour averages were calculated from reported 15 minute average concentrations. Neither the continuous nor manual monitoring stations include geolocations. To obtain the latitude/longitude coordinates of each station, we used the monitoring station name and geolocated them using Google maps.
- S. State Department Air-Now network ( https://www.airnow.gov/ ): One-hour average PM 2.5 concentrations were obtained for monitors located in Delhi, Mumbai, Hyderabad, Kolkata and Chennai.
2.2 Data Quality Control
We directly utilize the data available from the NAMP and Air-Now networks, but process the data we use from the CAAQM network to ensure representative monthly, seasonal, and annual average air pollutant concentrations using the following method:
- Missing data is removed. Values in excess of the reported range (see Table S1) are assumed to be errors and are removed. Values of 999.99 for PM 10 and PM 5 are retained as they may represent concentrations above the upper detection limit of the instrument. The U.S. Air-Now network data in New Delhi report 1-hour average PM 2.5 concentrations between 1300 and 1486 µg m – 3 during Diwali for each year. As CAAQM does not report values in excess of 999.99 µg m – 3 for PM 2.5 our annual means based on CAAQM will likely be biased low in some locations. In sequences of 24 or more consecutive identical hourly values, only the first value out of the sequence is retained. Data were processed following the QA/QC procedure described below. The percentage of data removed due to this processing is provided in Tables S2(a) and S2(b).
- Diurnal mean values are calculated for criteria pollutants PM 10 , PM 5 , SO 2 , NO 2 and O 3 for each 12-hour day-night interval (between 6 am–6 pm and 6 pm–6 am (next day)), using a minimum of one hourly observation for each 12-hour period. Daily means are calculated only for days that have a daytime or nighttime mean value. For O 3 , daily mean (MDA8) values are calculated as the maximum of 8-hour moving averages over a 24-hour period using at least 6 hourly observations. For all pollutants, monthly mean values are calculated for months that have at least 8 daily mean values (at least 25% of observations). To obtain annual average concentrations, we calculate quarterly means and require at least one monthly mean value as input to each quarterly mean concentration. At least two quarterly mean values are used for calculating annual average concentrations. This procedure is followed to ensure representativeness of data in diurnal, daily, monthly, seasonal, annual and interannual timeseries. Fig. 1 shows a flow chart describing the methodology for generating each step of the time-series.

3 RESULTS
3.1 strengths and weaknesses of available air quality datasets.
Until the start of 2018 the Indian monitoring network had limited extent. Very few stations have operated continuously from 2015 to the present. The number of stations in the continuous monitoring network has increased dramatically since 2017 ( Fig. 2 ) making it far more feasible now to evaluate air quality across India than in the past. However, spatial coverage is still limited with unequal distribution of monitors. All monitors are in cities, with a concentration in the largest cities, and none are in rural areas. Fig. 3 shows the percentage of valid hourly observations, compared with total hours annually, from each CAAQM station between 2015 and 2019. Although the current data is sufficient to provide an overview of air quality across much of India, it is currently challenging to use air quality datasets to conduct long term trend analysis due to their limited spatial and temporal coverage.
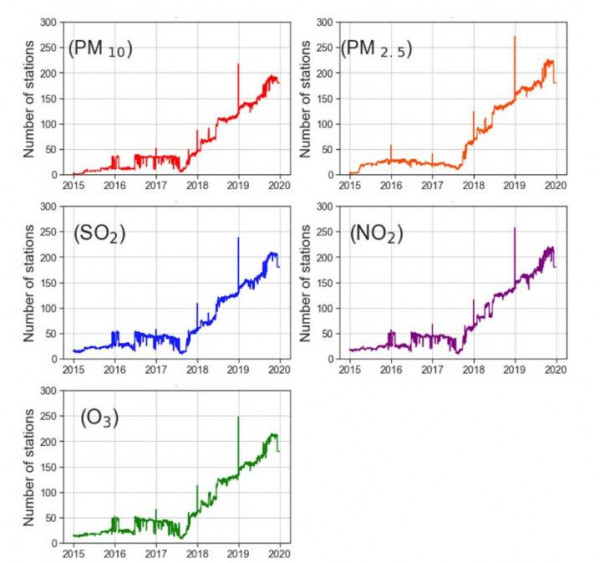
3.2 Spatial Distribution of Air Pollutants from 2015–2019
Figs. 4 and 5 show annual average concentrations of five criteria pollutants (PM 10 , PM 2.5 , SO 2 , NO 2 and O 3 ) at continuous and manual monitoring stations across India, from 2015 to 2019. The general distribution pattern of air pollution, showing higher pollution levels in northern than southern India, is captured in both the manual and continuous monitoring station data.

The number of continuous and manual monitoring stations have both increased substantially between 2015 and 2019 with 15 (147) CAAQM stations meeting our criteria for PM 10 , 33 (181) for PM 2.5 , 31 (163) for SO 2 , 34 (175) for NO 2 and 32 (168) for O 3 and in 2015 (2019) (see Figs. 4 and 5 for details of other years and manual stations). Of the total, nearly 60% of the CAAQM continuous monitoring stations are in northern India with 20% of the total stations in Delhi in 2019. Despite being a high pollution zone with nearly 15% of the Indian population ( http://up.gov.in/upstateglance.aspx ), the Indo Gangetic Plain has only 13% (9%) of total continuous (manual) monitoring stations. NAMP manual monitoring stations are more widely distributed than continuous monitors across India, with more monitors in the south and thus provide more representative spatial distributions of pollutants. However, they only provide annual average pollutant concentrations and thus cannot be used to analyze seasonal variations.
Elevated concentrations of PM 10 and PM 2.5 were recorded by both CAAQM and NAMP manual monitors across northern Indian states in all years, with particularly high concentrations across the Indo-Gangetic Plain (IGP). Ground observations of SO 2 are generally low across the country with high concentrations found at a few urban and industrial locations. This has been corroborated by previous studies (Guttikunda and Calori, 2013). The role of alkaline dust in scavenging SO 2 in India likely reduces ambient concentrations (Kulshrestha et al. , 2003). In contrast, annual average NO 2 and MDA8 O 3 concentrations are highly variable depending on location with higher O 3 concentrations often seen in the IGP region.
3.3 Annual Variation in Pollutant Concentrations in Northern and Southern India
The spatial distribution of pollutants is affected by meteorology, geography, topography, population density, location specific emission sources including industries, vehicular density, resuspended dust from poor land use management etc. In northern India (north of 23.5°N), higher population density and higher associated activities in industry, transport, power generation, seasonal crop residue burning, and more frequent dust storms contribute to higher particulate loads than in southern India (Sharma and Dixit, 2016; Cusworth et al. , 2018). We observed significant differences between northern and southern India in the spatio-temporal patterns of PM 10 , PM 2.5 , SO 2 , NO 2 and MDA8 O 3 .
Fig. 6 shows annual average concentrations (µg m – 3 ) of PM 10 , PM 2.5 , SO 2 , NO 2 and MDA8 O 3 respectively, for northern and southern India (divided at 23.5°N) from CAAQM stations. The number of stations used to calculate annual average values is shown in Fig. 4 for each species. Annual average concentrations of PM 10 , PM 2.5 , and NO 2 are higher in northern India, whereas SO 2 and MDA8O 3 are similar in the north and the south. Annual average concentrations from CAAQM continuous and NAMP manual monitoring stations, combined (S1 a), and only manual monitoring Stations (S1 b) are plotted separately in Fig. S1. We found inter-annual variability but no significant annual trend in the timeseries of these pollutants. Annual average concentrations over the five year period in northern (and southern) India were: 197 ± 84 µg m – 3 (93 ± 30 µg m – 3 ) for PM 10 , 109 ± 29 µg m – 3 (47 ± 16 µg m – 3 ) for PM 2.5 , 12 ± 7 µg m – 3 (12 ± 10 µg m – 3 ) SO 2 , 35 ± 21 µg m – 3 (27 ± 16 µg m – 3 ) for NO 2 and 73 ± 29 µg m – 3 (66 ± 31 µg m – 3 ) for MDA8 O 3 . In the five-year period, annual NAAQS were met at approximately 3% of all CAAQM stations measuring PM 10 , 13% of PM 2.5 , 70% of NO 2 and 98% of SO 2 (Table S3). MDA8 O 3 standard of 100 µg m – 3 (to be met 98% of the time within a year) was met at 77% of all CAAQM stations between 2015–2019, inclusive. Particulate matter dominates the pollution mix with national average annual mean concentrations exceeding the NAAQ standard for all analyzed years and in northern India more than double the allowed concentration. Fig. 7 shows annual average concentrations of these pollutants from CAAQM stations that meet our analysis criteria and are available each year from 2015 through 2019. The change in annual concentrations relative to the annual average concentrations in 2015–2017 at the stations operational throughout this period is shown in Fig. S2 in order to provide a comparison useful for evaluating the success of the NCAP.

3.5 Seasonal and Monthly Patterns of Air Pollutants
Seasonal concentrations of air pollutants in India are heavily influenced by meteorology and location. Influence of meteorology on spatio-temporal distributions of pollutants across India is described in Section S3. Fig. S3 shows the mean seasonal distribution of boundary layer height, surface pressure, precipitation, and omega/vertical and horizontal wind velocity. We calculate seasonal and monthly concentrations of PM 10 , PM 2.5 , SO 2 , NO 2 and MDA8 O 3 between 2015–2019 for northern and southern India in each season ( Fig. 8 ) and month ( Fig. 9 ) and show seasonal spatial distributions of these pollutants across India (Fig. S4). We analyze seasonal composites computed as averages for the spring or pre-monsoon period, March–April–May (MAM), the monsoon period, June–July–August (JJA), the autumn or post monsoon period, September–October–November (SON) and winter, December–January–February (DJF). In all seasons, substantially higher concentrations are observed for PM 10 and PM 2.5 , in northern India with concentrations of NO 2 , SO 2 and MDA8 O 3 only slightly more elevated in northern than southern India. The DJF average concentrations are highest for PM 10 , PM 2.5 and NO 2 in northern (southern) India: 270 ± 51 (137 ± 11) µg m –3 , 170 ± 26 (69 ± 2) µg m –3 , 47 ± 2 (35 ± 7) µg m –3 , respectively. Seasonal average concentrations of SO 2 peak in MAM in northern India (15 ± 3 µg m –3 ) and in DJF in southern India (16 ± 4 µg m –3 ), with highest concentrations in winter across the country. For DA8 O 3 , highest seasonal concentrations occur in MAM (DJF) in the north 71.8 ± 28 µg m –3 and south (84 ± 8 µg m –3 ).
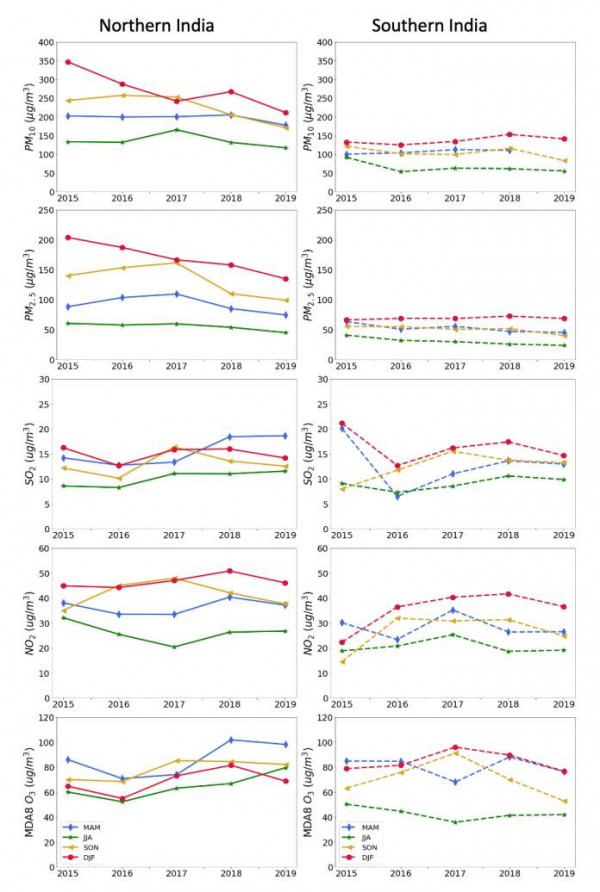
Monthly variations in pollution are also a function of regional circulation patterns. The summer monsoon facilitates dilution of pollution via strong south-westerly winds from the Arabian Sea and wet scavenging of anthropogenic pollution (Zhu et al. , 2012). Wet deposition removes PM 10 , PM 2.5 and water soluble SO 2 (Chin, 2012) leading to substantially lower ambient concentrations of these pollutants in JJA across India. Minimum concentrations of all pollutants occur in August.
Outside the monsoon, weak regional circulation and large scale high pressure systems result in accumulation of pollutants near the surface which is most pronounced in winter. Highest monthly concentrations are seen in November–January, inclusive, for PM 10 , PM 2.5 , SO 2 and NO 2 . For, MDA8O 3 , highest monthly concentrations are recorded in May (January) for northern (southern) India. Precursor emissions, surface temperature and solar insolation modulate a complex chemistry that drives the ozone cycle (Lu et al. , 2018).
3.6 Case studies of Delhi, Kolkata, Mumbai, Hyderabad and Chennai
Delhi, Kolkata, Mumbai, Hyderabad and Chennai are the five cities in India in which the U.S. State Department Air-Now network real time monitoring stations record PM 2.5 concentrations at the US embassy and consulates. In these five cities, we compare daily and monthly mean PM 2.5 measurements from the Air-Now and CAAQM networks. Fig. 10 shows scatterplots between daily mean PM 2.5 from the Air-Now monitor located in each of the five cities with all CPCB CAAQM monitors in those cities for 2015–2019, inclusive. We find a good correlation between the daily average PM 2.5 concentrations from the two networks at all the cities (r > 0.8), except Chennai (r~0.47) where CPCB concentrations are biased higher than the Air-Now concentrations. On highly polluted days in Delhi, the Air-Now monitors report higher PM 2.5 concentrations than the CPCB monitors in part because Air-Now monitors are able to report hourly concentrations above 1000 µg m –3 while the CPCB monitors cannot.

We examine how concentrations of PM 10 , PM 2.5 , SO 2 , NO 2 and O 3 vary between cities in which Air-Now monitors exist from 2015–2019 (see Fig. 11). Fig. 11 compares the monthly average concentrations of PM 2.5 between the two networks, examines the variation in concentrations over time for other species measured only by CPCB, and compares observed concentrations with the annual NAAQS for residential areas. Annual average concentrations from the stations combined in each city that meet our criteria is shown in Fig. S5 and a timeseries for each pollutant at each station is shown in Fig. S6. From CAAQM and Air-Now networks, we find Delhi has the highest daily, monthly mean and annual average concentrations of PM 10 and PM 2.5 , followed by Kolkata and Mumbai (Figs. 10, 11; Fig. S5).

For Delhi, between 2015 and 2019, annual average concentrations of PM 2.5 from the CAAQM station closest to the U.S. embassy (RK Puram, Delhi) greatly exceeded the residential NAAQS for PM 2.5 of 40 µg m –3 and ranged from 101 to 119 µg m –3 with the Air-Now station ranging from 95 to 124 µg m –3 . Chennai has the lowest monthly and annual average concentrations of PM 2.5 . The US state department annual average PM 2.5 values overall are consistent with the CAAQM stations and show a similar trend across cities. All five cities failed to meet the annual average CPCB PM 10 standard of 60 µg m –3 in all years.
Monthly and annual average SO 2 concentrations are far below the annual standard of 50 µg m –3 at all locations throughout the year in these five cities with Delhi reporting the highest annual average concentrations among the five cities followed by Mumbai. Starting in 2018 both Delhi and Mumbai had SO 2 concentrations lower than prior years.
Monthly average NO 2 concentrations are highest in Delhi in all years and starting in 2017, decrease from a peak over 100 µg m –3 in 2017 to a peak of 52 µg m –3 in 2019. Kolkata and Hyderabad also have relatively high concentrations of NO 2 with annual average concentrations exceeding the residential NAAQS of 40 µg m –3 starting in 2018.
Monthly MDA8 O 3 concentrations across all five cities are similar, particularly after 2018 and are generally falling below the residential 8-hour average NAAQS of 100 µg m 3 . Similar monthly tropospheric ozone concentrations in these cities, despite different levels of particulate matter, NO 2 and meteorology, make it a topic for further investigation.
4 DISCUSSION
4.1 growing dataset and existing gaps.
Prior to 2015 surface air quality monitoring data was available from only a few stations in India. Over the period we analyzed, 2015–2019, the number of monitoring stations across India increased dramatically. Our compilation and rigorous quality control of these data provide, for the first time, a comprehensive dataset of criteria pollutants that can be used to evaluate air pollutant concentrations simulated by atmospheric chemical transport models, satellite retrievals and reanalysis. Our dataset also provides a baseline for the NCAP. Previous studies have used ground observations from selected locations without transparently addressing existing data gaps and are not clear in their evaluation and quality assurance of surface observations. Here, we have carefully evaluated the archived data for completeness and accuracy, discarding values in excess of instrumental range, and requiring representative temporal coverage for each averaging period at each monitor. For example, for inclusion in our analysis a monitor measuring a species we analyze must report daily averages at least one hour per 12-hour daytime or night-time period, eight days for each monthly average, and one month per quarter and atleast two quarters for each annual average (see Tables S2(a), S2(b) and S3). However, spatial coverage remains spotty with monitoring stations predominantly located in large cities; smaller cities and rural locations lack coverage. Further expansion of the monitoring networks to facilitate an improved understanding of spatial distributions of pollutants across urban/rural India and to evaluate future trends in pollutant concentrations is needed. Very few stations provide valid observations continuously from 2015 onwards limiting our ability to analyze past trends in air quality. However, trend analyses starting in 2018 will be valuable and possible in the future.
4.2 Differences in Air Quality Observations
We compare monthly, seasonal and annual mean concentrations of air pollutants we analyze with other studies that have analyzed surface measurements of the same pollutants, cities and time periods across India (Table S5). We find that the range of concentrations of criteria pollutants reported in our analysis of CPCB data are similar to the values presented in research studies using ground observations during the same period (Kota et al. , 2018; Sreekanth et al. , 2018; Guttikunda et al. , 2019; Mahesh et al. , 2019; Ravinder et al. , 2019; Jain et al. , 2020; Tyagi et al. , 2020; Jat et al. , 2021). However, as shown in Table S5, in case studies covering extreme events and studies in bigger cities and more polluted regions, like Delhi and the IGP, differences exist between the CPCB concentrations we calculate and those reported in the literature from surface monitoring stations, models and satellite data (Kota et al. , 2018; Tyagi et al. , 2019; Jat et al. , 2021).
In Fig. 12 , we compare the spatial patterns of annual average surface PM 2.5 concentrations derived from satellite data with measurements from the CPCB continuous network. The surface satellite concentrations were obtained by combining data from Aerosol Optical Depth (AOD) from MODIS (Moderate Resolution Imaging Spectroradiometer), MISR (Multi-angle Imaging Spectroradiometer), MAIAC (Multi Angle Implementation of Satellite Correction) and SeaWiFS (Sea Viewing Wide Field of View Sensor) satellite products and using the GEOS-Chem model to obtain gridded surface PM 2.5 concentrations at 0.05° × 0.05° (Hammer et al. , 2020). The product we use is V4.GL.03 available at https://sites.wustl.edu/acag/datasets/surface-pm2-5/#V4.GL.03 . Reasonable agreement is seen between the annual mean surface concentrations of PM 2.5 derived from the satellite data and from the CPCB CAAQM observations from 2015-2019. Agreement is particularly good over the IGP and in central and southern India. However, along the western desert region (near Thar desert in Rajasthan), satellite concentrations of surface PM 2.5 (~40–50 µg m –3 ) were substantially lower than concentrations obtained from the CPCB CAAQM monitors (~80–100 µg m –3 ) for 2015–2017. In 2018 and 2019 the correspondence between the two datasets improved with most annual mean PM 2.5 concentrations in the western desert region generally between ~40 and 60 µg m –3 .
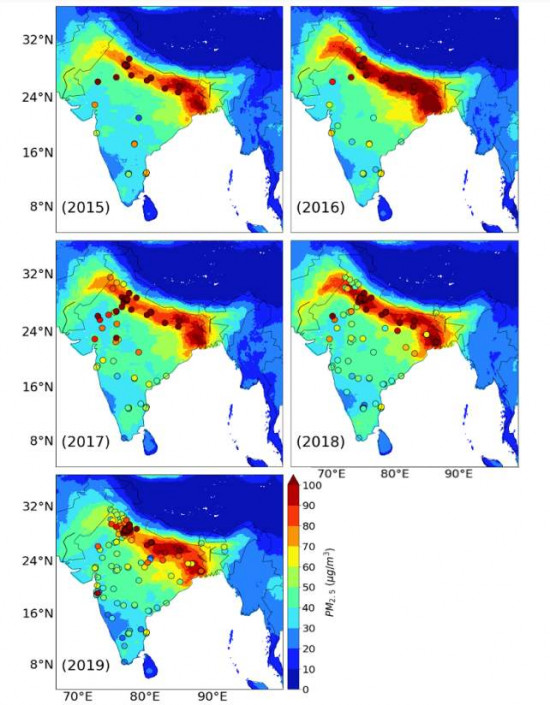
5 CONCLUSIONS
This study provides the first comprehensive analysis of all existing government monitoring data available for PM 10 , PM 2.5 , SO 2 , NO 2 and MDA8 O 3 using the continuous (CAAQM) and manual (NAMP) monitoring networks in India as well as the data from the US State Department Air-Now network, between 2015 and 2019 (2018 for NAMP). Our analysis shows that the Indian data record, in terms of number of monitoring stations, observations and quality of data, has improved significantly over this period. Despite the effort to augment surface monitoring infrastructure, gaps remain in spatial and temporal coverage and additional monitoring stations in small cities and rural areas are needed. Monitoring stations located in bigger cities (e.g., five Air-Now cities) have better data quality, from more widely distributed stations within the city, than is available for smaller cities. Pollution hotspots are occasionally found in smaller cities where monitoring stations are sparse. No stations have yet been placed in rural areas and are needed there in order to better characterize air quality and pollution sources across India (e.g., the effect of agricultural waste burning on air quality).
We find that fine particulate pollution dominates the pollution mix across India with virtually all sites in northern India (north of 23.5°N) exceeding the annual average PM 10 and PM 2.5 national residential ambient air quality standards (NAAQS) by 150% and 100% respectively, and in southern India (south of 23.5°N) exceeding the PM 10 standard by 50% and PM 2.5 standard by 40%. Comparison of PM 2.5 surface observations from the CPCB continuous monitoring network with surface satellite concentrations finds good agreement across India, particularly for 2017 and 2018. Prior to 2017 CAAQM concentrations were substantially higher than indicated by the satellite data over the western desert region. Annual average SO 2 , NO 2 and MDA8 O 3 generally meet the residential NAAQS across India. We find that northern India has (~10%–130%) higher average concentrations of all pollutants than southern India, except for SO 2 where the concentrations are similar. Although inter-annual variability exists, no significant trend of these pollutants was observed over the five-year period except for a small decrease over time in PM 10 and PM 2.5 in winter, which is more pronounced in the stations in northern and central India.
Our analysis of surface measurements is valuable for evaluating air pollutant concentrations simulated in atmospheric chemistry models. We found good agreement between the annual average CAAQM PM 2.5 we analyzed and satellite derived surface PM 2.5 from Hammer et al. (2020). Our data set can also be used to evaluate satellite retrievals of NO 2 and O 3 as well as seasonal variability in PM 2.5 concentrations. Finally, India is targeting a reduction of 20–30% in particulate pollution under NCAP by 2024 relative to 2017. Our analysis from 2015–2019 at different spatial and temporal scales of surface pollution provides a baseline to evaluate the future success of the programme as well as aids in the assessment of existing and future air pollution mitigation policies.
ADDITIONAL INFORMATION
data access.
The raw data from the continuous CPCB monitors used in our analyses along with the code for data quality control and the calculation of various temporal averages is available at https://doi.org/10.34770/60j3-yp02
ACKNOWLEDGEMENTS
We thank Mi Zhou for early assistance in data processing and two anonymous reviewers for helpful suggestions to improve our manuscript. Funding for D.S. was provided by a Science, Technology and Environmental Policy fellowship at the Center for Policy Research on Energy and Environment at Princeton University.
- Apte, J.S., Pant, P. (2019). Toward cleaner air for a billion Indians. PNAS 116, 10614–10616. https://doi.org/10.1073/pnas.1905458116
- Avnery, S., Mauzerall, D.L., Fiore, A.M. (2013). Increasing global agricultural production by reducing ozone damages via methane emission controls and ozone‐resistant cultivar selection. Global Change Biol. 19, 1285–1299. https://doi.org/10.1111/gcb.12118
- Avnery, S., Mauzerall, D.L., Liu, J., Horowitz, L.W. (2011). Global crop yield reductions due to surface ozone exposure: 2. Year 2030 potential crop production losses and economic damage under two scenarios of O 3 pollution. Atmos. Environ. 45, 2297–2309. https://doi.org/10.1016/j.atmosenv.2011.01.002
- Balakrishnan, K., Cohen, A., Smith, K.R. (2014). Addressing the burden of disease attributable to air pollution in India: The need to integrate across household and ambient air pollution exposures. Environ. Health Perspect. 122. https://doi.org/10.1289/ehp.1307822
- Balakrishnan, K., Dey, S., Gupta, T., Dhaliwal, R.S., Brauer, M., Cohen, A.J., Stanaway, J.D., Beig, G., Joshi, T.K., Aggarwal, A.N., Sabde, Y., Sadhu, H., Frostad, J., Causey, K., Godwin, W., Shukla, D.K., Kumar, G.A., Varghese, C.M., Muraleedharan, P., Agrawal, A., et al. (2019). The impact of air pollution on deaths, disease burden, and life expectancy across the states of India: The Global Burden of Disease Study 2017. Lancet Planet. Heath 3, e26–e39. https://doi.org/10.1016/S2542-5196(18)30261-4
- Brauer, M., Guttikunda, S.K., Nishad, K.A., Dey, S., Tripathi, S.N., Weagle, C., Martin, R.V. (2019). Examination of monitoring approaches for ambient air pollution: A case study for India. Atmos. Environ. 216, 116940. https://doi.org/10.1016/j.atmosenv.2019.116940
- Chen, Y., Wild, O., Conibear, L., Ran, L., He, J., Wang, L., Wang, Y. (2020). Local characteristics of and exposure to fine particulate matter (PM 2.5 ) in four Indian megacities. Atmos. Environ. 5, 100052. https://doi.org/10.1016/j.aeaoa.2019.100052
- Chin, M. (2012). Dirtier air from a weaker monsoon. Nat. Geosci. 5, 449–450. https://doi.org/10.1038/ngeo1513
- Chowdhury, S., Dey, S. (2016). Cause-specific premature death from ambient PM 2.5 exposure in India: Estimate adjusted for baseline mortality. Environ. Int. 91, 283–290. https://doi.org/10.1016/j.envint.2016.03.004
- Chowdhury, S., Dey, S., Di Girolamo, L., Smith, K.R., Pillarisetti, A., Lyapustin, A. (2019). Tracking ambient PM 2.5 build-up in Delhi national capital region during the dry season over 15 years using a high-resolution (1 km) satellite aerosol dataset. Atmos. Environ. 204, 142–150. https://doi.org/10.1016/j.atmosenv.2019.02.029
- Chowdhury, S., Pozzer, A., Dey, S., Klingmueller, K., Lelieveld, J. (2020). Changing risk factors that contribute to premature mortality from ambient air pollution between 2000 and 2015. Environ. Res. Lett. 15, 074010. https://doi.org/10.1088/1748 9326/ab8334
- Cusworth, D.H., Mickley, L.J., Sulprizio, M.P., Liu, T., Marlier, M.E., DeFries, R.S., Guttikunda, S.K., Gupta, P. (2018). Quantifying the influence of agricultural fires in northwest India on urban air pollution in Delhi, India. Environ. Res. Lett. 13, 044018. https://doi.org/10.1088/1748-9326/aab303
- Gao, M., Gao, J., Zhu, B., Kumar, R., Lu, X., Song, S., Zhang, Y., Jia, B., Wang, P., Beig, G., Hu, J., Ying, Q., Zhang, H., Sherman, P., McElroy, M.B. (2020). Ozone pollution over China and India: Seasonality and sources. Atmos. Chem. Phys. 20, 4399–4414. https://doi.org/10.5194/acp-20-4399-2020
- GDB Maps Working Group (2018). Burden of disease attributable to major air pollution sources in India.
- Ghude, S.D., Jena, C., Chate, D.M., Beig, G., Pfister, G.G., Kumar, R., Ramanathan, V. (2014). Reductions in India's crop yield due to ozone. Geophys. Res. Lett. 41, 5685–5691. https://doi.org/10.1002/2014GL060930
- Gurjar, B.R., Ravindra, K., Nagpure, A.S. (2016). Air pollution trends over Indian megacities and their local-to-global implications. Atmos. Environ. 142, 475–495. https://doi.org/10.1016/j.atmosenv.2016.06.030
- Guttikunda, S.K., Gurjar, B.R. (2012). Role of meteorology in seasonality of air pollution in megacity Delhi, India. Environ. Monit. Assess. 184, 3199–3211. https://doi.org/10.1007/s10661-011-2182-8
- Guttikunda, S.K., Calori, G. (2013). A GIS based emissions inventory at 1 km× 1 km spatial resolution for air pollution analysis in Delhi, India. Atmos. Environ. 67, 101–111. https://doi.org/10.1016/j.atmosenv.2012.10.040
- Guttikunda, S.K., Nishadh, K.A., Jawahar, P. (2019). Air pollution knowledge assessments (APnA) for 20 Indian cities. Urban Clim. 27, 124–141. https://doi.org/10.1016/j.uclim.2018.11.005
- Hama, S.M.L., Kumar, P., Harrison, R.M., Bloss, W.J., Khare, M., Mishra, S., Namdeo, A., Sokhi, R., Goodman, P., Sharma, C. (2020). Four-year assessment of ambient particulate matter and trace gases in the Delhi-NCR region of India. Sustainable Cities Soc. 54, 102003. https://doi.org/10.1016/j.scs.2019.102003
- Hammer, M.S., van Donkelaar, A., Li, C., Lyapustin, A., Sayer, A.M., Hsu, N.C., Levy, R.C., Garay, M.J., Kalashnikova, O.V., Kahn, R.A., Brauer, M., Apte, J.S., Henze, D.K., Zhang, L., Zhang, Q., Ford, B., Pierce, J.R., Martin, R.V. (2020). Global estimates and long-term trends of fine particulate matter concentrations (1998–2018). Environ. Sci. Technol. 54, 7879–7890. https://doi.org/10.1021/acs.est.0c01764
- Jain, S., Sharma, S.K., Vijayan, N., Mandal, T.K. (2020). Seasonal characteristics of aerosols (PM 2.5 and PM 10 ) and their source apportionment using PMF: A four year study over Delhi, India. Environ. Pollut. 262, 114337. https://doi.org/10.1016/j.envpol.2020.114337
- Jat, R., Gurjar, B.R., Lowe, D. (2021). Regional pollution loading in winter months over India using high resolution WRF-Chem simulation. Atmos. Res. 249, 105326. https://doi.org/10.1016/j.atmosres.2020.105326
- Kota, S.H., Guo, H., Myllyvirta, L., Hu, J., Sahu, S.K., Garaga, R., Ying, Q., Gao, A., Dahiya, S., Wang, Y., Zhang, H. (2018). Year-long simulation of gaseous and particulate air pollutants in India. Atmos. Environ. 180, 244–255. https://doi.org/10.1016/j.atmosenv.2018.03.003
- Kulshrestha, M.J., Kulshrestha, U.C., Parashar, D.C., Vairamani, M. (2003). Estimation of SO 4 contribution by dry deposition of SO 2 onto the dust particles in India. Atmos. Environ. 37, 3057–3063. https://doi.org/10.1016/S1352-2310(03)00290-5
- Lu, X., Zhang, L., Liu, X., Gao, M., Zhao, Y., Shao, J. (2018). Lower tropospheric ozone over India and its linkage to the South Asian monsoon. Atmos. Chem. Phys. 18, 3101–3118. https://doi.org/10.5194/acp-18-3101-2018
- Mahesh, B., Rama, B.V., Spandana, B., Sarma, M.S.S.R.K.N., Niranjan, K., Sreekanth, V. (2019). Evaluation of MERRAero PM 2.5 over Indian cities. Adv. Space Res. 64, 328–334. https://doi.org/10.1016/j.asr.2019.04.026
- Martin, R.V., Brauer, M., van Donkelaar, A., Shaddick, G., Narain, U., Dey, S. (2019). No one knows which city has the highest concentration of fine particulate matter. Atmos. Environ. 3, 100040. https://doi.org/10.1016/j.aeaoa.2019.100040
- Ministry of Environment, Forests and Climate Change (MoEF) (2019). National Clean Air Program (NCAP). Government of India. http://moef.gov.in/wp-content/uploads/2019/05/NCAP_Report.pdf
- National Ambient Air Quality Monitoring Program (NAMP) (2020). Data Year wise. https://cpcb.nic.in/namp-data/ (accessed 15 December 2020).
- Navinya, C.D., Vinoj, V., Pandey, S.K. (2020). Evaluation of PM 2.5 surface concentrations simulated by NASA’s MERRA Version 2 aerosol reanalysis over India and its relation to the air quality index. Aerosol Air Qual. Res. 20, 1329–1339. https://doi.org/10.4209/aaqr.2019.12.0615
- Pande, P., Dey, S., Chowdhury, S., Choudhary, P., Ghosh, S., Srivastava, P., Sengupta, B. (2018). Seasonal transition in PM 10 exposure and associated all-cause mortality risks in India. Environ. Sci. Technol. 52, 8756–8763. https://doi.org/10.1021/acs.est.8b00318
- Pandey, A., Brauer, M., Cropper, M.L., Balakrishnan, K., Mathur, P., Dey, S., Turkgulu, B., Kumar, G.A., Khare, M., Beig, G., Gupta, T., Krishnankutty, R.P., Causey, K., Cohen, A.J., Bhargava, S., Aggarwal, A.N., Agrawal, A., Awasthi, S., Bennitt, F., Bhagwat, S., et al. (2021). Health and economic impact of air pollution in the states of India: The Global Burden of Disease Study 2019. Lancet Planet. Heath 5, e25–e38. https://doi.org/10.1016/S2542-5196(20)30298-9
- Pant, P., Lal, R.M., Guttikunda, S.K., Russell, A.G., Nagpure, A.S., Ramaswami, A., Peltier, R.E. (2019). Monitoring particulate matter in India: recent trends and future outlook. Air Qual. Atmos. Health 12, 45–58. https://doi.org/10.1007/s11869-018-0629-6
- Ravindra, K., Singh, T., Mor, Sahil, Singh, V., Mandal, T.K., Bhatti, M.S., Gahlawat, S.K., Dhankhar, R., Mor, Suman, Beig, G. (2019). Real-time monitoring of air pollutants in seven cities of North India during crop residue burning and their relationship with meteorology and transboundary movement of air. Sci. Total Environ. 690, 717–729. https://doi.org/10.1016/j.scitotenv.2019.06.216
- Sahu, S.K., Kota, S.H. (2017). Significance of PM 2.5 air quality at the Indian capital. Aerosol Air Qual. Res. 17, 588–597. https://doi.org/10.4209/aaqr.2016.06.0262
- Sharma, M., Dikshit, O. (2016). Comprehensive study on air pollution and green house gases (GHGs) in Delhi. A report submitted to Government of NCT Delhi and DPCC Delhi, 1–334. (accessed 20 December 2020).
- Sharma, S.K., Mandal, T.K., Sharma, A., Jain, S. (2018). Carbonaceous species of PM 2.5 in megacity Delhi, India during 2012–2016. Bull. Environ. Contam. Toxicol. 100, 6952.5701. https://doi.org/10.1007/s00128-018-2313-9
- Silva, R.A., West, J.J., Lamarque, J.-F., Shindell, D.T., Collins, W.J., Faluvegi, G., Folberth, G.A., Horowitz, L.W., Nagashima, T., Naik, V., Rumbold, S.T., Sudo, K., Takemura, T., Bergmann, D., Cameron-Smith, P., Doherty, R.M., Josse, B., MacKenzie, I.A., Stevenson, D.S., Zeng, G. (2017). Future global mortality from changes in air pollution attributable to climate change. Nature Clim. Change 7, 647–651. https://doi.org/10.1038/nclimate3354
- Sreekanth, V., Mahesh, B., Niranjan, K. (2018). Gradients in PM 2.5 over India: Five city study. Urban Clim. 25, 992.5108. https://doi.org/10.1016/j.uclim.2018.06.001
- Tyagi, B., Singh, J., Beig, G. (2020). Seasonal progression of surface ozone and NO x concentrations over three tropical stations in North-East India. Environ. Pollut. 258, 113662. https://doi.org/10.1016/j.envpol.2019.113662
- Venkataraman, C., Brauer, M., Tibrewal, K., Sadavarte, P., Ma, Q., Cohen, A., Chaliyakunnel, S., Frostad, J., Klimont, Z., Martin, R.V., Millet, D.B., Philip, S., Walker, K., Wang, S. (2018). Source influence on emission pathways and ambient PM 2.5 pollution over India (2015–2050). Atmos. Chem. Phys. 18, 8017–8039. https://doi.org/10.5194/acp-18-8017-2018
- Wang, Y., Chen, Y. (2019). Significant climate impact of highly hygroscopic atmospheric aerosols in Delhi, India. Geophys. Res. Lett. 46, 55352.55545. https://doi.org/10.1029/2019GL082339
- Yang, X., Jiang, L., Zhao, W., Xiong, Q., Zhao, W., Yan, X. (2018). Comparison of ground-based PM 2.5 and PM 10 concentrations in China, India, and the US. Int. J. Environ. Res. Public Health 15, 1382. https://doi.org/10.3390/ijerph15071382
- Zhu, J., Liao, H., Li, J. (2012). Increases in aerosol concentrations over eastern China due to the decadal‐scale weakening of the East Asian summer monsoon. Geophy. Res. Lett. 39, L09809. https://doi.org/10.1029/2012GL051428
Share this article with your colleagues
Other readers also read ...
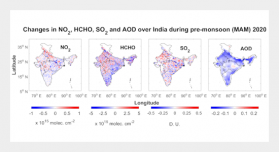
Read more: ...
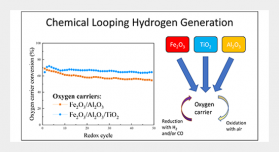
Subscribe to our Newsletter
Aerosol and Air Quality Research has published over 2,000 peer-reviewed articles. Enter your email address to receive latest updates and research articles to your inbox every second week.
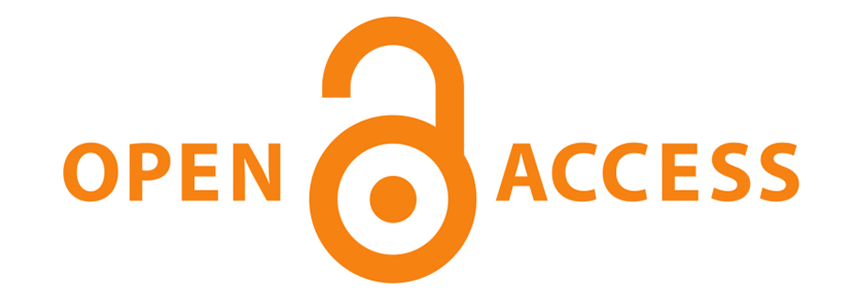
2022 Impact Factor: 4.0 5-Year Impact Factor: 3.4

- Source Impact Analysis Using Char-EC/Soot-EC Ratios in the Central Indo-Gangetic Plain (IGP) of India ( Volume 21, Issue 9, September 2021 )
- What to Wear: The Filtration Performance of Alternative Materials Used to Construct Do-It-Yourself Masks ( Volume 21, Issue 8, August 2021 )
- Fabrication of Iron-based Oxygen Carriers on Various Supports for Chemical Looping Hydrogen Generation ( Volume 21, Issue 4, April 2021 )
- Assessing Mitigation Strategies to Reduce Potential Exposures to Indoor Particle Release Events ( Volume 22, Issue 9, September 2022 )
- Insights into Reaction Conditions for Selective Catalytic Reduction of NOx with a MnAl Oxide Catalyst at Low Temperatures ( Volume 21, Issue 11, November 2021 )
- Investigating Two-dimensional Horizontal Mesh Grid Effects on the Eulerian Atmospheric Transport Model Using Artificial Neural Network ( Articles In Press )
- 15 Years of Moss Biomonitoring for Air Quality Assessment in Albania ( Articles In Press )
- Influence of Relative Humidity and Aging on Morphology and Chemical Composition on Biomass Burning Particle ( Articles In Press )
- Identification of Saharan-Dust Intrusions over Sofia, Bulgaria, Using Near-Ground PM10 and PM2.5 Mass Concentration Measurements ( Articles In Press )
- Assessment and Quantification of Methane Emission from Indian Livestock and Manure Management ( Articles In Press )
You may also like
- Indoor and Ambient Air Pollution in Chennai, India during COVID-19 Lockdown: An Affordable Sensors Study
- COVID-19 and Air Pollution in Indian Cities: World’s Most Polluted Cities
- Identification and Quantification of Emission Hotspots of Air Pollutants over Bhubaneswar: A Smart City in Eastern India
- Patterns and Sources of PM10 in the Ecologically Sensitive Himalayan Region in Himachal Pradesh, India
- Validation of In-field Calibration for Low-Cost Sensors Measuring Ambient Particulate Matter in Kolkata, India
- Air Quality, Atmospheric Variables and Spread of COVID-19 in Delhi (India): An Analysis
Research Categories
- Aerosol Physics and Instrumentation
- Indoor Air Quality
- Aerosol and Atmospheric Chemistry
- Bioaerosols
- Urban Air Quality
- CO2 Capture, Storage and Emission
- Nanoparticles
- Control Techniques and Strategy
- Low-Cost Sensors
- Physical and Chemical Characteristics of Aerosols
Research Regions
- Middle East
- South America
- South East Asia
Air Quality Index Analysis of Indian Cities During COVID-19 Using Machine Learning Models: A Comparative Study
Ieee account.
- Change Username/Password
- Update Address
Purchase Details
- Payment Options
- Order History
- View Purchased Documents
Profile Information
- Communications Preferences
- Profession and Education
- Technical Interests
- US & Canada: +1 800 678 4333
- Worldwide: +1 732 981 0060
- Contact & Support
- About IEEE Xplore
- Accessibility
- Terms of Use
- Nondiscrimination Policy
- Privacy & Opting Out of Cookies
A not-for-profit organization, IEEE is the world's largest technical professional organization dedicated to advancing technology for the benefit of humanity. © Copyright 2024 IEEE - All rights reserved. Use of this web site signifies your agreement to the terms and conditions.
A Critical Review on Air Quality Index
- Conference paper
- First Online: 30 December 2017
- Cite this conference paper
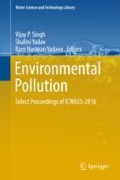
- Shweta Kumari 5 &
- Manish Kumar Jain 5
Part of the book series: Water Science and Technology Library ((WSTL,volume 77))
1867 Accesses
23 Citations
Air quality index (AQI) is used worldwide to inform the public about levels of air pollution (degradation or improvement) and associated to different biological effects. Different types of anthropogenic activity mainly transportation have an enormous impact on the ambient air quality in several ways. The transportation dependence continues to grow; it is adversely affecting the quality of human life. Due to pollution, the ambient air quality in major cities (Delhi, Agra, Kanpur, Lucknow, Varanasi, Faridabad, Ahmedabad, Chennai, Bangalore and Hyderabad) in India is very poor. According to WHO surveys, India is one of the most polluted countries in the world. Concentrations of air pollutants affect Air Quality Index. Air Quality scenario in most of the Indian cities presents a harsh picture, the majority of national monitoring stations have recorded particulate concentrations exceeding the WHO recommended guideline. The higher the AQI value, the greater the level of air pollution and greater the health concern. This review paper is helpful to understand the development of Air quality Index in India with the experience of the world.
This is a preview of subscription content, log in via an institution to check access.
Access this chapter
Institutional subscriptions
Bishoi B, Prakash A, Jain VK (2009) A comparative study of air quality index based on factor analysis and US-EPA methods for an urban environment. Aerosol Air Quality Res 9(1):1–17
Google Scholar
Beig G, Ghude SD, Deshpande A (2010) Scientific evaluation of air quality standards and defining air quality index for India. RR-127
Chen W, Tang H, Zhao H (2016) Urban air quality evaluation under two versions of the national ambient air quality of China. Atmos Pollut Res 7(1):49–57
CPCB (2014) National air quality index report, Central Pollution Control Board, New Delhi, India
EPA (1999) Air quality index reporting; final rule. Federal Register, Part III, CFR Part 58
Index Air Quality (2003) A guide to air quality and your health. Washington, USEPA Air and Radiation, Environmental Protection Agency, EPA-454/K-03-002, 19, 11-01
Inhaber H (1976) Environmental indices. Wiley, New York
Lohani BN (1984) Environmental quality management. South Asian Publishers, New Delhi
Kumar A, Goyal P (2011) Forecasting of daily air quality index in Delhi. Sci Total Environ 409(24):5517–55223
Article Google Scholar
Kyrkilis G, Chaloulakou A, Kassomenos PA (2007) Development of an aggregate air quality index for an urban mediterranean agglomeration: relation to potential health effects. Environ Int 33(5):670–676
Ott WR, Thorn GC (1976) Air pollution index system in the United States and Canada. J Air Pollut Control Assoc 26(5):460–470
Pandey M, Singh V, Vaishya RC (2014) Geomatics approach for assessment of respiratory disease mapping. Int Arch Photogram Remote Sens Spat Inform Sci 40(8):205
Wang LK, Pereira NC, Hung YT (2005) Advanced air and noise pollution control. Humana Press, Totowa, N.J., USA, pp 3–33
Book Google Scholar
Wang L, Zhang P, Tan S, Zhao X, Cheng D, Wei W, Su J, Pan X (2013) Assessment of urban air quality in China using air pollution indices (APIs). J Air Waste Manag Assoc 63(2):170–178
Download references
Author information
Authors and affiliations.
Department of Environmental Science & Engineering, Indian Institute of Technology (Indian School of Mines), Dhanbad, 826004, Jharkhand, India
Shweta Kumari & Manish Kumar Jain
You can also search for this author in PubMed Google Scholar
Corresponding author
Correspondence to Manish Kumar Jain .
Editor information
Editors and affiliations.
Department of Biological and Agricultural Engineering, and Zachry Department of Civil Engineering, Texas A&M University, College Station, Texas, USA
Vijay P Singh
Department of Civil Engineering, AISECT University, Bhopal, Madhya Pradesh, India
Shalini Yadav
AISECT University, Hazaribag, Jharkhand, India
Ram Narayan Yadava
Rights and permissions
Reprints and permissions
Copyright information
© 2018 Springer Nature Singapore Pte Ltd.
About this paper
Cite this paper.
Kumari, S., Jain, M.K. (2018). A Critical Review on Air Quality Index. In: Singh, V., Yadav, S., Yadava, R. (eds) Environmental Pollution. Water Science and Technology Library, vol 77. Springer, Singapore. https://doi.org/10.1007/978-981-10-5792-2_8
Download citation
DOI : https://doi.org/10.1007/978-981-10-5792-2_8
Published : 30 December 2017
Publisher Name : Springer, Singapore
Print ISBN : 978-981-10-5791-5
Online ISBN : 978-981-10-5792-2
eBook Packages : Earth and Environmental Science Earth and Environmental Science (R0)
Share this paper
Anyone you share the following link with will be able to read this content:
Sorry, a shareable link is not currently available for this article.
Provided by the Springer Nature SharedIt content-sharing initiative
- Publish with us
Policies and ethics
- Find a journal
- Track your research

An official website of the United States government
The .gov means it’s official. Federal government websites often end in .gov or .mil. Before sharing sensitive information, make sure you’re on a federal government site.
The site is secure. The https:// ensures that you are connecting to the official website and that any information you provide is encrypted and transmitted securely.
- Publications
- Account settings
Preview improvements coming to the PMC website in October 2024. Learn More or Try it out now .
- Advanced Search
- Journal List

Air pollution in Delhi, India: It’s status and association with respiratory diseases
Abhishek dutta.
Department of Environmental Science, Faculty of Science, Chulalongkorn University, Pathumwan, Bangkok, Thailand
Wanida Jinsart
Associated data.
Data Availability: Air quality data of Delhi that support the findings of this study are owned by the Central Pollution Control Board (CPCB). For further information about the air quality data please visit https://cpcb.nic.in/real-time-air-qulity-data/ or https://app.cpcbccr.com/ccr/#/dashboard-emergency-stats . Meteorological data of Delhi can be obtained from the Regional Meteorological Centre, India Meteorological Department ( https://rmcnewdelhi.imd.gov.in/ ). Both for data and permission to use the data, please contact the Deputy Director General of Meteorology (DDGM), Regional Meteorological Centre, Lodi Road, New Delhi – 110003 via E-mail: moc.liamg@ihledwencfwr . Daily hospital visit data between the years 2016 and 2018 for respiratory diseases (ICD-10) J00-J99, used in this study, were collected from Vardhman Mahavir Medical College Safdarjung hospital, Ansari Nagar East, New Delhi. For data and permission to use data please contact the Medical Superintendent M.S. Office, New OPD Building, Safdarjung Hospital, New Delhi-110 029.Tel (011-26190763), e mail: ni.cin.hjs-cmmv@eciffosm .
The policymakers need research studies indicating the role of different pollutants with morbidity for polluted cities to install a strategic air quality management system. This study critically assessed the air pollution of Delhi for 2016–18 to found out the role of air pollutants in respiratory morbidity under the ICD-10, J00-J99. The critical assessment of Delhi air pollution was done using various approaches. The mean PM 2.5 and PM 10 concentrations during the measurement period exceeded both national and international standards by a wide margin. Time series charts indicated the interdependence of PM 2.5 and PM 10 and connection with hospital visits due to respiratory diseases. Violin plots showed that daily respiratory disease hospital visits increased during the winter and autumn seasons. The winter season was the worst from the city’s air pollution point of view, as revealed by frequency analyses. The single and multi-pollutant GAM models indicated that short-term exposure to PM 10 and SO 2 led to increased hospital visits due to respiratory diseases. Per 10 units increase in concentrations of PM 10 brought the highest increase in hospital visits of 0.21% (RR: 1.00, 95% CI: 1.001, 1.002) at lag0-6 days. This study found the robust effect of SO 2 persisted in Delhi from lag0 to lag4 days and lag01 to lag06 days for single and cumulative lag day effects, respectively. While every 10 μg m -3 increase of SO 2 concentrations on the same day (lag0) led to 32.59% (RR: 1.33, 95% CI: 1.09, 1.61) rise of hospital visits, the cumulative concentration of lag0-1 led to 37.21% (RR: 1.37, 95% CI:1.11, 1.70) rise in hospital visits which further increased to even 83.33% (RR: 1.83, 95% CI:1.35, 2.49) rise at a lag0-6 cumulative concentration in Delhi. The role of SO 2 in inducing respiratory diseases is worrying as India is now the largest anthropogenic SO 2 emitter in the world.
1. Introduction
Time and again, the policymakers felt the requirements of understanding the status of air pollution in growing cities and association of short-term air pollution exposures spanning one or a few days on morbidity. This is particularly more relevant for the world’s fast-growing cities to accrue benefits of sustainable development. Epidemiological studies conducted in the past in cities held air pollution responsible for inducing different health hazards. The quasi-poison regression model within over-dispersed Generalized Additive Model (GAM) has been very handy for many researchers for exploring the association of air pollution with different morbidity and mortality [ 1 – 6 ]. In a time series where the respondent variable depends on the nonlinear relationship of independent variables, GAM model finds its best applicability. In GAM, the nonlinear confounders can be controlled using smooth functions to correctly estimate the best connection between dependent and independent variables [ 7 – 12 ]. Accordingly, researchers used the GAM model extensively to indicate the role of air pollution in causing health effects for US and European cities [ 13 , 14 ].
Chinese and Indian cities frequently grabbed the world’s attention because of increasing air pollution and reported health effects on city dwellers. Indian cities were in the limelight because of the uncontrollable nature of air pollution in already declared polluted cities. Different Chinese cities have been put under strict scanners by the researchers who continuously reported or updated the policymakers on air pollution and health hazards so that policy-level initiatives may defuse the situation. Recently Lu et al. [ 15 ] reported that research ably supported the polluted Chinese cities to progress in air pollution control and place the much-needed strategic air quality management system. Another recent article indicated that out of 31 research papers published during 2010–2020 investigating the role of different air pollutants on the health of city dwellers using the GAM model, the majority, i.e., 17 were in the backdrop of Chinese cities and 3 for Indian cities [ 16 ]. GAM successfully explored the role of different pollutants in establishing their relationships with different types of respiratory morbidity/mortality for 21 cities of China, India, Iran, Brazil. Denmark and Kuwait ( S1 Table ). Zhao et al. [ 17 ], using GAM, reported that Dongguan city dwellers in China faced the threat of enhanced respiratory diseases due to short term exposure to CO. Song et al. [ 18 ] found respiratory diseases amongst the children of Shijiazhuang city of China due to PM 10 , SO 2 , NO 2 presence in the air. Cai et al. [ 19 ], studied the total respiratory diseases mortality of Shenzhen, China, and linked them with PM 2.5 presence in ambient air through GAM modelling. Liang et al. [ 20 ] used GAM model to indicate a direct relationship between pulmonary disease in Beijing with air pollution. Very recently Wang et al. [ 21 ] confirmed the role of particulate matter (PM) with pneumonia hospitalizations of children in Hefei, China.
Delhi, the capital city of India, is the second most populated and one of the most polluted cities in the world and should be the obvious choice for pollution and health hazard research. The recent air quality report of IQ Air has ranked Delhi first out of the air-polluted capital cities of 106 countries based on PM 2.5 concentration [ 22 ]. According to WHO, Delhi is the sixth-worst polluted city amongst 13 notable other Indian cities. Indeed, the city-dwellers had terrible times when PM 2.5 of Delhi stood at 440 μg m -3 during October 2019, i.e., 12 times the US recommended level. Past studied blamed the huge transport sector with the largest vehicle stock of the country as the critical emission source [ 23 – 27 ]. Chen et al. [ 28 ] demonstrated that local transport emissions and neighboring states contributed dominantly to PM 2.5 and O 3 concentration strengthening in Delhi. Sreekanth et al. [ 29 ] found high PM 2.5 pollution persists across all the seasons in Delhi despite pollution control efforts in vogue. In the pan-Indian context, air pollution significantly contributed to morbidity and premature mortality in India for a long time [ 30 ]. Sharma et al. [ 31 ] reviewed 234 journal papers and noted the knowledge gaps in connecting hospital admissions of patients with air pollution of Delhi. Balyan et al. [ 32 ] also noted that a deeper understanding of ambient pollutants at the city level and their effect on morbidity was lacking.
Against the background above, the primary objective of this paper to explore the environmental data of Delhi for confirming the poor air quality status of the city and, after that, assess the role of air pollutants with morbidity (respiratory diseases) through the application of the GAM model. A more profound grasp of the city air quality and influences of ambient air pollution on respiratory diseases is much needed. Such studies may provide all critical information for initiating actions to curb air pollution, health risk, developing public health policies, and above all, a strategic environmental management system for Delhi.
2. Study location
As a highly populated and polluted city, Delhi provides an opportunity to apply the GAM model for ascertaining how much the prevailing air pollution is responsible for respiratory diseases of the city dwellers. Delhi has spread over 1,483 km 2 and a population size of about 11 million per the 2011 census study. With time Delhi emerged as a significant city of the country so far as commerce, industry, medical service, and education are concerned. As per Köppen’s climate classification, Delhi’s climate is extreme with five seasons. The summer is scorching (April–June), while winter is freezing (December-January). The average temperature range during the summer is between 25°C to 45°C, while the winter temperature range is between 22°C to 5°C [ 33 ]. The comfortable season spring prevails from February to March, and autumn runs from mid-September to late November. The rainy monsoon season spans almost three months, starting from July. Air pollution varies across seasons due to the influence of climatic conditions [ 34 ].
3. Materials and methods
3.1. air pollution data.
Daily average data for three years, January 2016 to December 2018, (1096 data points) of key air pollutants were collected from the State Pollution Control Board (SPCB), Delhi. The pollutants were sulfur dioxide (SO 2 ), nitrogen dioxide (NO 2 ), carbon monoxide (CO), particulate meter 10 micrometers or less (PM 10 ), and particulate meter 2.5 micrometers or less (PM 2.5 ) as recorded by 11 NAMP (National Air Quality Monitoring Programme) stations of the city as shown in Fig 1 and S2 Table .

3.2. Meteorological data
Time series meteorological data for 1 January 2016–31 December 2018 were collected from Regional Meteorological Department located in Delhi. The data were of a total of 1096 days and included daily average temperature (T), daily average relative humidity (RH), daily average wind speed (WS), and daily rainfall (RF). The collected meteorological and air monitoring data will be adequate to estimate the confounding effect of meteorological conditions on morbidity related to respiratory diseases using GAM model.
3.3. Hospital visit data
We considered respiratory diseases covered by J00-J99 under the ICD-10 classification system. Data related to daily hospital outpatient visits of patients for respiratory diseases under International Classification of Diseases-10 (ICD-10), J00-J99 for 2016–2018 (1096 days) were collected from Safdarjung Medical College and Hospital (SMCH) of Delhi. The SMCH had its existence from pre-independence days of India and now functioning under the Ministry of Health and Family Welfare, Government of India. SMCH has many different specialties and super specialty departments, and Respiratory Medicine (RM) is one. Fig 1 shows that all the 11 air pollution monitoring stations considered in this study are located within a road distance of 12 km from SMCH. The hospital records contained information related visit date of patients, age, gender, and final medical diagnosis for each patient. The patient data were grouped age-wise under three categories (i) elderly people (more than or equal to 65 years), (ii) middle-aged (45–64 years), and (iii) young (less than or equal to 44 years). For hospital data collection formal request letter was submitted to the hospital authority. As the data were old data without identifiers and not having any possibility of ascertaining the identities of the individuals to whom the data belong, the hospital waived IRB approval.
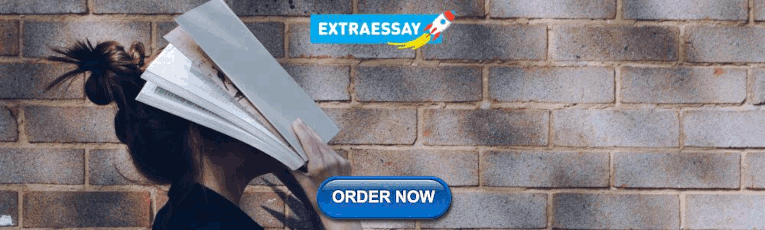
3.4. Methods of analysis
3.4.1 summary statistics and analysis of time series.
Summary statistics of climatic variables, air pollutants, and hospital visits of the patients such as mean, standard deviation, maximum, minimum, and different quartiles were computed using the SPSS 25 version of the software. Daily hospital visit counts for three years (2016–2018) in SMCH were structured based on the patient’s age, sex, and visit dates. Violin plots were developed for three air pollutants (PM 10 , PM 2.5 , and CO), two climatic variables (T, RH), and hospital visits of patients regarding five seasons of Delhi, indicating the distribution of data prevailing in the city during different seasons. Violin plots have been drawn with XLSTAT statistical software. Time series plots were developed using the SPSS 25 version of the software with time dimensions on the horizontal axis and hospital visits, pollutants and, meteorological variables on the vertical coordinate axes to shed light on the data distribution for three years.
3.4.2 Frequency analysis
The seasonal distribution of PM 2.5 and PM 10 concentrations in Delhi during 2016–18 has been done by frequency analysis [ 35 ]. Under frequency analysis, first, the city level average concentrations of PM per day were calculated by averaging the concentration of 11 monitoring stations. Then, PM concentrations (both for PM 10 and PM 2.5 ), i.e., number of per day observations for the period 2016–18 falling under six categories like 0–25, 25–50, 50–100, 100–200, 200–300, and more than 300 μg m -3 worked out. So, the three-year period (2016–18) data or 1096 observations were segregated session-wise for each of the six categories, and the frequency of their appearances was then expressed in percentage terms. The calculations were done with the help of data analysis ’ToolPak’ of excel. As per the air quality index (AQI) Of India, the range 0–100 is considered a good category, 100–200 as moderate, 200–300 as poor, and above 300 as very poor or severe.
3.4.3 Correlation analysis
To understand the interrelationship between climatic variables and air pollutants data for Delhi (2016–2018), we executed Pearson correlation analysis using SPSS version 25.0 (SPSS Inc., Chicago, IL, USA) software. The coefficients of correlations were established between daily meteorological variables and air pollutants for Delhi. The correlation coefficients at p < .01 were accepted as statistically significant [ 36 ]. For better visualization, correlation matrix plots have been drawn with R software’s ’corrplot’ package.
3.4.4 Generalized Additive Models (GAM)
The nonlinear associations of various independent variables (climatic variables and criteria pollutants) and the outcome variable (hospital visits due to respiratory diseases) of Delhi can be better explained by (GAM) model. GAM explicitly allows the relationship between outcome variables independent variables to be developed based on the smooth functions fitted to some independent variables, thereby bringing the flavor of parametric relationships of the covariates in a regression model [ 37 , 38 ]. Accordingly, in this study, the potential confounding effects of few independent variables that entered the regression model were controlled with non-parametric smoothening splines. Smoothening splines of 7 degrees of freedom (df) per year were fitted to calendar time (time since 1 January 1970) to control long-term trends and possible calendar effects [ 39 , 40 ]. In line with Wei [ 41 ] smoothening splines with 7 df were also applied to mean RH and mean temperature (T) to control their respective confounding effects on the regression model. A linear term of mean wind speed (WS) was allowed to prevail. A dummy variable as the day of the week (DOW) was additionally introduced in the categorical form to control for week effects. As per Peng et al. and Zheng et al. [ 39 , 42 ], the dfs for smoothing splines were allowed to be determined by the generalized cross-validation (GCV) scores. Finally, based on the description of the regression model formation above, we formed the following GAM model ( Eq 1 ) in our present study with usual notations and applied.
where i denotes the day of observation; E ( Y i ) denotes the daily hospital visits expected due to respiratory diseases; β denotes regression coefficient; X i denotes the daily mean concentration of pollutants; s stands for the smoothing spline applied, and α is the intercept. Once the basic GAM model is set with the smoothing splines for RH, T, and time variables, the independent variables like PM 2.5 , PM 10 , NO 2 , SO 2 , and CO (per day concentrations) were added to the basic model to make it the multi-pollutant GAM model. We also constructed two single pollutant models for PM 2.5 and PM 10 , respectively, to understand their respective sole effects on respiratory diseases related to hospital visits in the city under study. In the single-pollutant model, PM 2.5 and PM 10 concentrations, in turn, were entered as independent variables in the base model. Generally, single pollutant models do not reflect the synergistic effect of pollutants on morbidity, but in consideration with the multi-pollutant models, they provide crucial complementary understanding.
The respective coefficients of pollutants of the multi-pollutant and single-pollutant GAM models, found out as regression model output, were the inputs in deriving the relative risk (RR) of hospital visits due to one unit rise of each modelled air pollutants in the ambient air.
Past studies have shown that the air pollutants remain in the ambient air and create lingering effects on morbidity. Accordingly, we have considered pollutant concentrations for a single day and multiple days in the study. We tested the lingering effects of air pollution for single-day lags and cumulative lag days. Single-day lag (lag0) means air pollutant concentrations on the same day of the hospital visit, while lag6 indicates air pollutant concentrations of 6 days before the hospital visit. Similarly, for cumulative concentrations of pollutants lag0-1indicate the mean of pollutant concentration of the current day and previous day of the hospital visit (i.e., 2 days mean). Similarly, lag 0–2 indicates the mean of current day pollutant concentration, 1 day before and 2 days before the visit (i.e. 3 days mean). In the same way, lag0-3, lag0-4, lag0-5, and lag0-6 means 4 days, 5 days, 6 days, and 7 days mean pollutant concentrations, respectively. We used single lags of 0, 1, 2, 3, 5, and 5 days (lag0–lag 5) and cumulative lags of 0–1, 0–2, 0–3, 0–4, 0–5, and 0–6 days (lag 0–1 to lag0-6) to explore the lag pattern of health effects in the multi pollutants and single pollutant models. The R software with "mgcv" package (version 4.0.2) was applied to construct the GAM models. For visualizations of GAM models developed in this study, we have used visual tools of the mgcViz R package.
3.5. Relative Risk (RR)
Relative risk (RR), often used in epidemiological studies, helps understand the risk of the outcome of an intervened event with non-intervened events. Thus, RR compares one group with another group. In this study, the exposure-response coefficient β of pollutants obtained from the GAM models under different lag conditions have been used to estimate RR and their 95% confidence intervals (95% CIs). RR for the i th predictor variable and its confidence intervals were calculated using the following Eqs 2 , 3 and 4 .
where Δ C i is the rise of the i th pollutant concentration in air and S.E i is the standard error of i th pollutants. Here, Δ C will be 1 unit increase in CO and 10 units increase in all other pollutants. RR provides information on the rise of hospital visits due to each unit increase of a pollutant’s concentration level. To make the RR estimates of daily hospital visits due to air pollution more expressive, we also calculated the percentage change (PC, %,) at 95% CI in the following way ( Eq 5 ).
PC = Percentage change of daily hospital visits due to air pollution
In all analyses p-value < 0.05 considered significant.
4. Results and discussion
4.1 data distribution and time-series analyses.
The distribution of criteria pollutants, climatic variables (T and RH), and daily counts of hospital visits in Delhi are placed in Table 1 for 2016–18. Table 1 indicates that the mean value of PM 2.5 and PM 10 concentrations exceeded the guidelines of NAAQS and WHO both by a wide margin. They shoot to as high as 693.08 μg m - ³ for PM 10 and 478.25 μg m - ³ for PM 2.5 during 2016–2018. The mean RH value of 58.5% (range, 98.3% to 12.5%) in Delhi indicates the city’s humid condition higher than the ideal level relative humidity for health and comfort of 30–50%. The three years mean temperature of 25.63 ± 7.65 °C with a maximum as high as 45°C and a minimum of 0.5°C along with a higher level of RH indicates the extreme climate of Delhi. Daily mean hospital visits of patients for respiratory diseases during 2016–18 was 20±23.52.
Table 2 reveals that a total of 22,253 patients visited SMCH, Delhi, either for outpatient consultation or admission for respiratory diseases during 2016–2018, as retrieved from hospital records. The maximum number of people who visited the hospital for respiratory ailments for a day was 176, and the minimum 0 patients. Out of the total patients, 63.5% were female, and 30% had ≥65 years of age. Similarly, out of male patients, 52% were aged ≥65 years, as shown in Table 2 .
Time series charts in ( Fig 2A–2F ) depict behaviors of meteorological variables (RH, temperature), air pollutants (PM 2.5 , PM 10 , and CO), hospital visits, and their interrelationship during 2016–2018 for Delhi. PM 2.5 and PM 10 were positively correlated in Delhi during 2016–18, indicating the interdependency ( Fig 2A ) while maintaining a positive correlation with hospital visits due to respiratory diseases ( Fig 2B and 2C ). Fig 2D–2E shows that hospital visits tended to negatively correlate with RH and temperature. Fig 2(F) shows a positive correlation of hospital visits with CO concentration too in the city’s environment.

The time series of Delhi from 2016–2018 (A) PM 2.5 Vs Hospital visit, (B) PM 10 Vs Hospital visit, (C) RH Vs Hospital visit, (D) T Vs Hospital visit, (E) CO Vs Hospital visit, (F) PM 2.5 Vs PM 10 .
Violin plots of three air pollutants (PM 10 , PM 2.5 , and CO), two meteorological variables (T, RH), and hospital visits of patients were drawn for the five distinct seasons of Delhi have been provided in ( Fig 3A–3F ) below. Fig 3A indicates that PM 2.5 dominates the city environment during winter and autumn. Fig 3B indicates that PM 10 dominates the city air during the winter and summer seasons, but the median value of PM 10 concentrations was higher during winter. The concentration of CO in the air remains high during winter and low during the monsoon season ( Fig 3C ). Fig 3D clearly shows that the city experiences comparatively higher RH during summer and monsoon, with the highest median value during monsoon. Fig 3E indicates that the city experiences the hottest season during summer and autumn. From Fig 3F , it can be observed that during the winter and autumn season’s daily hospital visits due to respiratory diseases increased. The rectangles within the violin plots indicate finishing points of the first and third quartile of data distribution with central dots as medians. The upper and lower whiskers show data spread.

(A) PM 2.5 , (B) PM 10 , (C) CO, (D) RH, (E) Temperature, (F) Hospital visit.
4.2 Seasonal distribution of PM 2.5 and PM 10 in Delhi
The frequency distribution of PM 2.5 and PM 10 concentrations for five Delhi seasons are shown in Fig 4 . Fig 4 indicates that the winter season was terrible from the air pollution point of view as almost 95.2% of the time, the ambient PM 2.5 concentrations recorded to be more than 100 μg m -3 . Alarmingly, 100% of the time, the ambient PM 10 concentrations crossed the 100 μg m -3 benchmark during winter, indicating very harsh wintertime for the city dwellers. The spring season brought some relief for the city dwellers when 42.2% of the time PM 2.5 concentrations crossed 100 μg m -3 benchmark, but PM 10 remained very strong with 99.4% of the time crossing the 100 μg m -3 benchmark. During summer, about 76.9% of the time PM 2.5 concentrations were under the ’good’ category, and 15.8% of the time PM 2.5 concentrations were more than the 100 μg m -3 benchmark. During summer PM 2.5 concentrations improved considerably with only 15.8% of the time, its concentrations were more than the 100 μg m -3 benchmark, but PM 10 remained razing with 97.8% time crossing 100 μg m -3 benchmark. However, two and half months of monsoon (July, August, and mid-September) brought relief from PM 2.5 pollution. Almost 100% of the time, PM 2.5 concentrations remained under the ’good’ category, but PM 10 remained 51.1% crossing the 100 μg m -3 benchmark during monsoon. From autumn (mid-September to late November), PM pollution built up with 97.8% of the time PM 2.5 concentrations crossing 100 μg m -3 benchmark, as shown in Fig 4 . In summary, the frequency distribution of PM 2.5 and PM 10 concentrations indicates that except winter, the PM concentrations remained very high, which could be a possible cause of health hazards for the city dwellers.

4.3 Correlation between pollutants and meteorological variables
Positive correlation existed between two important gaseous pollutants SO 2 and NO 2 (r = 0.341), while PM 10 maintained a mild positive correlation with SO 2 (r = 0.281). PM 10 almost had linear positive correlation both with NO 2 (r = 0.783) and CO (r = 0.733) as shown in Table 3 and Fig 5 . PM 2.5 also had positive correlation with SO 2 (r = 0.137), and positive linear correlation with NO 2 (r = 0.673) and CO (r = 0.757). Also, PM 10 and PM 2.5 maintained positive linear correlation.

Blue, red, and while indicate positive, negative, and no correlation respectively.
**. Correlation is significant at the 0.01 level (2-tailed).
*. Correlation is significant at the 0.05 level (2-tailed).
4.4 Association of criteria pollutants with respiratory diseases, Delhi
Multi-pollutant and single pollutant GAM models were formed for Delhi to understand the impact of air pollutants on hospital visits due to respiratory diseases. Multi pollutant models indicate combined effects of the involved pollutants on the hospital visits, whereas single pollutant GAM models cast light on the sole effect of pollutants. The models were tested with different lag concentrations to comprehensively understand the impact of short-term exposure of pollutants on hospital visit counts due to respiratory diseases.
4.4.1. Association of criteria pollutants with respiratory diseases in Delhi (multi-pollutant models)
In the multi-pollutant model, criteria pollutants for 2016–18 were included in the base GAM model. Table 4 and Fig 6 indicate the relative risks (RR) of hospital visits due to a rise of 1 unit increase in CO and 10 units for all other pollutant concentrations for different single lag days. The RR patterns in Table 4 indicate synergistic effects of criteria pollutants on respiratory diseases related hospital visits in the city. Table 4 reveals that both PM 2.5 and PM 10 concentrations of all the 6 single lag days had no significant effect on respiratory disease-related hospital visits. The effect of NO 2 on hospital visits was there during lag1 day concentrations only but without any positive acceleration. The effect of SO 2 on respiratory diseases-related hospital visits was found to be robust instantaneously, i.e., the increase of every 10 ppb SO 2 on the same day (lag 0) resulted in a 32.6% (RR: 1.326, 95% CI: 1.089, 1.614) rise in hospital visits. The effect of SO 2 on hospital visits persisted throughout the lag days from lag0 up lag4. The increase in CO on hospital visits throughout the different lag days (lag0 to lag6) was found to be non-significant for respiratory diseases.

* Figs. in the brackets indicates PC (% change in hospital visits)
Note: p < 0.05, p < 0.01, and p < 0.001 considered significant
Table 5 and Fig 6 below indicate the relative risks (RR) pattern of change in hospital visits due to a rise of 1 unit increase in CO and 10 units for all other pollutant concentrations for different cumulative concentrations of pollutants. Both for PM 2.5 and PM 10 , in terms of cumulative days effect of air pollution, no significant effect could be found. NO 2 and CO were also not significantly responsible for enhancing respiratory diseases in the city. However, per 10 ppb rise in cumulative lag days, concentrations of SO 2 led to a comparatively more robust effect on respiratory diseases than single-day lag effects. At lag0-1 per 10 ppb, rise in concentrations of SO 2 was associated with the percentage change in hospital visits of 37.21% (RR: 1.372, 95% CI: 1.107, 1.701), which increased to 83.34% (RR: 1.833, 95% CI: 1.351, 2.489) during the lag0-6 day. The result indicates the robust effect of pollutants SO 2 on respiratory disease-related hospital visits in Delhi.
Note: p < 0.05, p < 0.01, and p < 0.001 considered significant.
Figs Figs7 7 and and8 8 below, drawn with the "mgcViz" R software package (Fasiolo et al., [ 43 ], provide the visual representation of the smoothing applied to the non-parametric terms and performance of the GAM model at lag0 respectively.

4.4.2. Association of criteria pollutants with respiratory diseases in Delhi (Single-pollutant models)
Two single-pollutant models were developed with pollutants PM 2.5 and PM 10, respectively, to understand the sole effect of PM pollution on respiratory diseases. We fitted different single lag days and cumulative lag days to express the association of daily hospital visits for respiratory diseases with a 10μg m -3 increase in PM 10 or PM 2.5 in Delhi. Both PM 2.5 and PM 10 did not show any significant association with the number of respiratory disease-related hospital visits in Delhi for all the single lag days considered here, as revealed by the p values ( Table 6 and Fig 9 ). In other words, the association of PM 2.5 and PM 10 with the respiratory disease was negligible as RR was found to be less than the baseline (RR<1).

*Note: p < 0.05, p < 0.01, and p < 0.001 considered significant
However, in cumulative exposure single-pollutant models, PM 10 was found to have persistently enhanced hospital visits of patients with the respiratory disease excepting lag 0–2 days, as shown in Table 6 . Table 6 shows that per 10 units increase in concentrations of PM 10 brought the highest increase in hospital visits of 0.21% (RR: 1.002, 95% CI: 1.001, 1.002) at lag0-6 days. PM 2.5 association with respiratory disease-related hospital visits found to be non-significant during all the cumulative lag days considered.
5. Conclusion and discussion
The study investigated first the level of air pollution in Delhi and then assessed the impact of air pollution on respiratory diseases. The result suggests that Delhi has been struggling to cope up with the increasing nature of criteria pollutants in the first place. A total of 22,253 patients visited the Delhi hospital either for outpatient consultation or admission for respiratory diseases for 2016–2018. The study found that the mean value of PM 2.5 and PM 10 concentrations for the period 2016–2018 were 107.32±71.06 μg m -3 and 210.61±95.90 μg m -3 for Delhi, respectively, which were substantially higher than the NAAQS and WHO standards. Out of the five seasons in Delhi, the winter season is hugely dominated by PM 2.5 and PM 10 pollution, as revealed by frequency analyses. Initial time series analysis revealed that PM 2.5 maintained a positive correlation with PM 10 have while PM 2.5 , PM 10 , and CO maintained a positive correlation with hospital visits during 2016–18 in Delhi. Pearson correlation analysis confirmed that PM 10 in Delhi had almost positive linear correlations with NO 2 and CO while PM 10 maintained a strong positive correlation with PM 2.5 . Interestingly, SO 2 too maintained a significant positive correlation with PM 2.5 , PM 10 , NO 2 , and CO. Previous studies in the Indian city of Mumbai highlighted the strong positive correlation of PM 2.5 with NO 2 and referred to them as a dummy indicator of air pollution due to transport-related emissions in the city [ 44 ]. In the same line, significant positive correlations between PM concentrations and gaseous pollutants, shown by air pollution data, point towards transport-related pollution, solvent evaporation, and waste disposal as sources [ 45 , 46 ].
This study shows PM 10 to have persistent enhancing effects on the number of hospital visits with the respiratory disease during all the cumulative lag days excepting lag 0–2 days. Luong et al. [ 47 ] reported PM 10 and respiratory disease-related hospital admission in polluted Hanoi city of Vietnam. Past studies confirmed the role of PM in inducing oxidative stress in the human respiratory system [ 48 ]. PM 10 impact on respiratory diseases in Delhi may be aggravated due to the road dust fraction of PM 10 that has significant oxidative potential [ 49 ]. It was interesting to note that in multi-pollutant models, the role of PM 10 causing respiratory diseases got subdued due to the combined presence of other pollutants in Delhi city.
This study found that short-term exposure to SO 2 and PM 10 led to increased hospital visits of the city dwellers due to respiratory diseases under (ICD-10) J00-J99. The present study reports the mean SO 2 in ambient air for three years (2016–18) as 14.65 ppb or 38.25 μg m -3 . SO 2 is a very critical gaseous pollutant connected with public health [ 50 ]. Past studies reported that an ordinary person could withstand only 2.62 μg m -3 of SO 2 in the ambient air without any respiratory problem [ 51 ]. However, short but higher concentration exposure to SO 2 gas can cause persistent pulmonary problems [ 52 ]. Orellano et al. [ 53 ], in a more recent and extensive review and metadata analysis, confirmed that short-term exposure to SO 2 , varying from few hours to days, can lead to an increased risk of respiratory morbidity/mortality. Our findings agree with that and found a robust effect of SO 2 on respiratory diseases hospital visits in Delhi. This study shows the robust effect of SO 2 persisted in Delhi throughout the single lag days (from lag0 up lag4) and had an instantaneous (same day, lag 0) increase of 32.6% (RR: 1.326, 95% CI: 1.089, 1.614) of hospital visits. The cumulative concentrations of SO 2 were more robust than the single lag day concentration in Delhi. While every 10 μg m -3 SO 2 concentrations on the same day (lag0) showing 32.59% (RR: 1.326, 95% CI: 1.089, 1.614) rise of hospital visits, the cumulative concentration on the day and its previous day (lag0-1) showing 37.21% (RR: 1.372, 95% CI: 1.107, 1.701) rise in hospital visits which further increased to even 83.33% (RR: 1.833, 95% CI: 1.351, 2.489) rise at a lag0-6 cumulative concentration of the pollutant in Delhi. Ren et al. [ 54 ], using the GAM model, confirmed the SO 2 effect on respiratory diseases in the fast-industrializing Chinese city of Wuhan and found that a 10 μg m -3 rise in SO 2 concentrations led to a rise of RR for respiratory disease mortality by 1.9% at lag0 day or same day. More recently, another two highly industrializing cities of Zhoushan and Hangzhou of China with the comparatively lesser presence of average SO 2 of 6.12 μg m -3 and 17.25 μg m -3 in ambient air, respectively, confirmed the active role of SO 2 in enhancing hospital visits of the patient for respiratory diseases [ 55 ]. Phosri et al. [ 56 ] also reported the effect of SO 2 for hospital admissions for respiratory diseases in industrializing Bangkok city of Thailand.
Recent COVID-19 and air pollution studies in Delhi indicated that even during the rigorous ’lockdown’ period, there was only a marginal decrease of mean SO 2 in the ambient air than in the regular times [ 33 , 57 ]. Therefore, it proves that a significant portion of ambient SO 2 in Delhi is likely to be from non-local origins like distant transfer, fossil fuel-fired thermal power plants in the bordering areas of Delhi, and biomass burning in the neighboring states. India’s recognition as the largest anthropogenic SO 2 emitter replacing China in recent times will be much more worrisome in the context of this study’s findings [ 58 , 59 ].
Suneja et al. [ 60 ], through an experimental study in Delhi, reported the seven-year (2011–2018) mean value of SO 2 level was 2.26 ppb, while this study found a much higher three-year average (2016–18) of 14.65 ppb, indicating the rise of SO concentrations in Delhi in the more recent years. The association of respiratory diseases with PM 10 and SO 2 was found stable in different lag days analyses, indicating the problem’s depth for the city dwellers. The robust and instantaneous nature of the relationship between SO 2 and respiratory morbidity indicated in this study and evidence of similar relationships found in the previous studies highlight the necessity of taking policy-level measures to reduce SO 2 in the ambient air. Limited GAM model application in Indian cities to link air pollution and health effects is not a limitation of the present study findings but rather a call for more sponsored research in the area.
Supporting information
Acknowledgments.
The authors thank the Central Pollution Control Board and the Indian Meteorological Department of Delhi city for providing air pollution and meteorological information, respectively.
Funding Statement
This study was supported by the Graduate School Thesis Grant GCUGR1225632064D, Chulalongkorn University, Bangkok, Thailand. The funders had no role in study design, data collection and analysis, decision to publish, or preparation of the manuscript.
Data Availability
- Open access
- Published: 05 December 2021
Air quality index variation before and after the onset of COVID-19 pandemic: a comprehensive study on 87 capital, industrial and polluted cities of the world
- Mohammad Sarmadi ORCID: orcid.org/0000-0003-0708-8749 1 , 2 ,
- Sajjad Rahimi 1 , 2 ,
- Mina Rezaei 2 ,
- Daryoush Sanaei 3 &
- Mostafa Dianatinasab ORCID: orcid.org/0000-0002-0185-5807 4
Environmental Sciences Europe volume 33 , Article number: 134 ( 2021 ) Cite this article
19k Accesses
30 Citations
23 Altmetric
Metrics details
Coronavirus disease 2019 (COVID-19) pandemic provided an opportunity for the environment to reduce ambient pollution despite the economic, social and health disruption to the world. The purpose of this study was to investigate the changes in the air quality indexes (AQI) in industrial, densely populated and capital cities in different countries of the world before and after 2020. In this ecological study, we used AQI obtained from the free available databases such as the World Air Quality Index (WAQI). Bivariate correlation analysis was used to explore the correlations between meteorological and AQI variables. Mean differences (standard deviation: SD) of AQI parameters of different years were tested using paired-sample t -test or Wilcoxon signed-rank test as appropriate. Multivariable linear regression analysis was conducted to recognize meteorological variables affecting the AQI parameters.
AQI-PM 2.5 , AQI-PM 10 and AQI-NO 2 changes were significantly higher before and after 2020, simultaneously with COVID-19 restrictions in different cities of the world. The overall changes of AQI-PM 2.5 , AQI-PM 10 and AQI-NO 2 in 2020 were – 7.36%, – 17.52% and – 20.54% compared to 2019. On the other hand, these results became reversed in 2021 (+ 4.25%, + 9.08% and + 7.48%). In general, the temperature and relative humidity were inversely correlated with AQI-PM 2.5 , AQI-PM 10 and AQI-NO 2 . Also, after adjusting for other meteorological factors, the relative humidity was inversely associated with AQI-PM 2.5 , AQI-PM 10 and AQI-NO 2 (β = − 1.55, β = − 0.88 and β = − 0.10, P < 0.01, respectively).
Conclusions
The results indicated that air quality generally improved for all pollutants except carbon monoxide and ozone in 2020; however, changes in 2021 have been reversed, which may be due to the reduction of some countries’ restrictions. Although this quality improvement was temporary, it is an important result for planning to control environmental pollutants.
Introduction
The coronavirus disease 2019 (COVID-19) pandemic caused by the SARS-CoV-2 virus emerged from Wuhan, China [ 1 ] and caused more than 250 million infected people and 5 million death up to 10 November 2020. COVID-19 is associated with multiple affecting factors such as air pollution, sociodemographic indices, which have a two-way relationship [ 2 , 3 ]. After about 2 years, many countries especially developed countries with mass vaccination, have not yet been able to fully restore their social and economic activities to pre-2020 levels [ 4 , 5 ]. The economic and social impacts have been significant and coinciding with the pandemic declaration of the disease by the World Health Organization (WHO) on 11 March 2020 [ 6 ], many countries put temporary closures of their industries and jobs on the agenda and on the other hand, restrictions within cities were seriously pursued in most countries [ 7 ]. These actions include restrictions on inter-city and intra-city traffic, closing businesses, closure of schools and small communities, suspension of tourist visas and so on. Most countries imposed national or local restrictions and closures until early May, after which the restrictions became easy and less severe [ 8 , 9 ].
Considering the closure of industries and restrictions on community activities, including inter-city transportation and mobility, it is expected that these measures will have significant effects on the amount of air pollutants from industries and vehicles, especially in large cities [ 10 , 11 ]. Important environmental pollutants include particulate matters (different combinations of solid, liquid and vapor particles), which are mainly in two types of particles with an aerodynamic diameter < 2.5 µm (PM 2.5 ) and < 10 µm (PM 10 ), tropospheric ozone [O 3 ], nitrogen dioxide [NO 2 ], sulfur dioxide [SO 2 ], carbon monoxide (CO) [ 12 ]. The UK Department for Transport reported that traffic was significantly reduced (69%) during the holidays, which also had a significant impact on the concentration of environmental pollutants [ 13 ]. Nitrogen oxides are caused by the combustion of fossil fuels, which is a good indicator of vehicle-related air pollution. It has been shown that more than 50% and 23% of the total nitrogen oxides (NO X ) in developed and developing countries are related to transportation [ 14 ]. This pollutant can also react with volatile organic compounds and the sun's ultraviolet rays to produce tropospheric ozone, which poses a serious health threat such as inducing airways inflammation and increasing airway hyperreactivity [ 15 , 16 , 17 ]. In previous studies, it was shown that particulate pollutants, e.g., PM 2.5 , PM 10 , NO 2 and ozone were the most important pollutants contributing to COVID-19 mortality and incidence [ 18 , 19 , 20 ].
A report by the WHO states that about 7 million deaths worldwide every year are due to air pollution [ 21 ]. Also, according to the European Environmental Agency reports, 251,000–495,000, 31,000–76,000 and 9400–28,900 premature deaths are attributable to PM 2.5 , NO 2 and O 3 reported for the EU-28, respectively [ 22 ]. As such, according to the previous studies in India, about 1.24 million deaths are related to air pollution, of which 0.67 million are due to particulate pollutants [ 23 , 24 ]. A study in China also estimated that reduction in NO 2 leads to a reduction of about 8911 deaths [ 25 ]. In terms of total economic benefits of the protection of human life, nitrogen dioxide has had the greatest impact, with about $ 10 billion in about 20 major cities surveyed in the world [ 26 ]. Previous studies have shown that the highest incidence of air pollution-related diseases is in the Western Pacific and South-East Asia regions, which is associated with heavy industry and air pollution hotspots in these areas [ 27 ]. The effects of air pollution on different types of diseases have shown that these exposures play a significant role in the development and progression of a vast variety of diseases, especially in urban areas [ 24 , 25 , 28 ]. As a result of COVID-19, the widespread restrictions and closures in different countries were applied and, as a consequence, the results of studies in the United States [ 29 ], Italy [ 30 ], the United Kingdom [ 31 ], Spain [ 32 ], China [ 33 ] and India [ 34 ] have shown that the concentrations of indicator pollutants are significantly reduced [ 35 ], especially for nitrogen dioxide and particulate matter. This reduction in the concentration of pollutants improves the air quality index (AQI) of cities and has positive effects on community health. However, despite the widespread lockdown caused by COVID-19 in some areas, the concentrations of many pollutants, including sulfur dioxide and ozone, have increased [ 36 , 37 , 38 , 39 , 40 ].
So far, in most studies, only a limited number of cities in one or a few countries have been limitedly addressed, while the present study focuses on changes in the AQI of pollutants in important industrial and capital cities of the world before and after 2020 (and before and after mass vaccination programs). Inspecting changes in air pollutants can help to revise air quality guidelines in different countries and enable policymakers and researchers to examine the effects of reducing or increasing air pollution on the prevalence, incidence and burden of several diseases and then, if applicable, to apply preventive measures. On the other hand, the study of changes in AQI relative to their concentration can be interpreted in a more understandable way for the whole community, which is the focus of this study. Finally, the purpose of this study was to investigate the changes in the AQI related to standard pollutants in 87 industrial, densely populated and capital cities in different countries of the world before, during and after 2020.
Study area and data source
This is an ecological and city-level study based on the “Strengthening the Reporting of Observational Studies in Epidemiology” (STROBE) guideline for observational studies [ 41 ], which was designed to examine changes in air quality index (AQI) of various pollutants during the first 121 days of 2020 (due to the implementation of the most COVID-19 restrictions) and comparison with past and next year [ 40 ]. The inclusion criteria for the cities study is the World Air Quality Index (WAQI) project report from the website https://aqicn.org/ ; this report has been published online for employment of air pollution data on COVID-19 by researchers, during the first 6 months of 2015 to 2018 (January 1 to June 30) and full data for 2019 to 2020 has in more than 380 cities [ 42 ]. The distribution map of cities has already been published in a study by researchers [ 40 ].
Also, information about different cities from 2021 until April 30 has been published on the organization's website. According to the study objectives, cities that met the main inclusion criteria were included in the study. These criteria for selecting the cities in order of importance are as follows: (1) the AQI of different pollutants and meteorological variables for it should be fully published; (2) it should be the capital of the country and (3) It should be one of the top 100 polluted cities in the world (in terms of particles) according to the report of the World Health Organization https://www.who.int/ . If in one country, the information for its capital was not complete, other cities of that country that had the third criterion were used. The choice of a country’s capital and major cities can be important because of the significance of maximizing the lockdown executive restrictions of countries. Latitude and longitude of cities were extracted from Google and the distribution map of cities was drawn in ArcGIS 10.3 software (Fig. 1 ). Due to the distribution of air pollution monitoring stations and additional information, most of the selected cities are from Asia and Europe. Further information is available on the WAQI website.
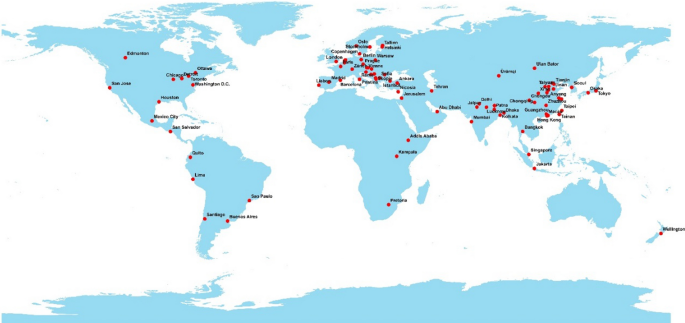
Map of world showing location in 87 different cities included in the study
Outcome and quantitative variables
To analyze various air pollution indicators, we extracted the AQI values related to PM 2.5 , PM 10 , NO 2 and SO 2 , CO and tropospheric O 3 in the first 4 months of 2018 to 2021 and entered the Graph Pad Prism software. The pollutant concentrations were measured as ground-based measurements. The AQI calculation method for each pollutant was shown on the website https://aqicn.org/calculator . The values of the air quality index with respect to different pollutants along with their related color and description of the index are shown in Fig. 2 .
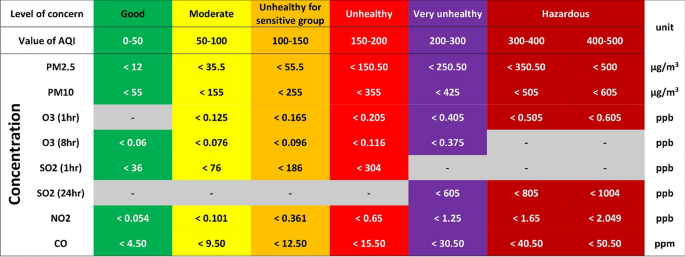
Air quality index and cut-off concentrations and health messages. Good air quality is considered satisfactory and air pollution poses little or no risk, moderate air quality is acceptable; however, for some pollutants there may be a moderate health concern, unhealthy for sensitive groups members of sensitive groups may experience health effects, unhealthy everyone may begin to experience health effects, very unhealthy health warnings of emergency conditions and hazardous health alert: everyone may experience more serious health effects
The median (IQR = interquartile range), minimum and maximum daily AQI values were reported at different stations for the cities. The average values of the median for 1 month were considered as the monthly average. Then, the 4-month average was analyzed to compare the mean of 2018 to 2021 with the 2020 baseline as a lockdown scale and the severe restriction of countries. To control the quality of the data, we removed values equal to zero and abnormal numbers such as more than 1000 from the data. Also, each city for which pollutant information was reported for < 25 days (< 80%) was excluded from the study. If the calculated final outcome values were abnormal, the quality of the data in that city was rechecked to ensure the accuracy of the information. The outcomes examined include the percentage of changes in AQI pollutants compared to 2020 and the average AQI pollutants for each city.
In this study, we tried to use the average values of each year as a scale of comparison with next years, which shows the impact of lockdown in 2020 (severe restrictions) and 2021 (easier restrictions or coinciding with mass vaccination) with previous years. To investigate the effect of the restrictions in 2020 and 2021, the percentage difference of AQI values between different years was calculated by the following formulas [ 43 ]:
%ΔAQI 2020 x indicates the percentage of AQI changes compared to 2018 and 2019. AQI 2020x represents the average AQI of pollutant x in 2020 and AQI 2019x or AQI 2018x is the average AQI of pollutant x in 2019 or 2018. For the percentage difference between 2020 and 2021, the same variables are defined. In this case, if the percentage of positive difference is reported, it indicates an increase in the values of the AQI in 2020 compared to other years and if it is negative, it means a decrease in AQI. In order to reduce the bias of changes in air pollutants, climate variables that have a direct and indirect effect on the concentration of pollutants were extracted for each city.
Statistical analysis
Descriptive data of the study were presented using the median (IQR), maximum, minimum and first and third quartile reports using box and whiskers diagrams. AQI of ambient pollutants variables (PM 2.5 , PM 10 , NO 2 , SO 2 , CO and tropospheric O 3 ) was stratified by Human Development Index (HDI) and Gross National Income (GNI). The statistical significance of mean differences in AQI variables among countries (developed or developing) and (L&LM: low and lower-middle income: < 4045$, upper-middle income: 4046–12,535$ and high income: > 12,535) categories was assessed using independent-sample t-tests. The United Nations considers countries with HDI ≥ 0.788 as “developed” and any score below that as “developing” [ 44 ]. Median and IQR for AQIs were also calculated stratified by HDI and GNI categories. The average of AQI for each city was also mapped using GraphPad Prism software. The data have been analyzed with GraphPad Prism and SPSS software. We assessed the correlation between AQI variables and meteorological parameters using Pearson and Spearman rank correlation coefficients based on normality of data. In addition, the statistical significance of differences in AQI variables among years (2020–2018, 2020–2019, 2021–2020) and GDP (high and low) categories was assessed using paired-sample t -tests [ 10 ]. We used multivariable linear regression to analyze AQIs in combination with meteorological parameters to each other.
Description analysis of AQI variation
A total of 87 industrial, polluted and crowded megacities from 57 different countries were included in the study. Of these, 58 cities are the main capitals of the countries and the rest are among the 100 most polluted and industrial cities in the world. Figure 3 shows the average AQI of various pollutants in cities with complete data in the first 4 months of 2018 to 2021 (January 1 to April 30). The results showed that Zurich (2.31), Tallinn (8.65), Washington (0.18), Tallinn (3.98) and Edmonton (0.10) had the lowest AQIs for PM 2.5 , PM 10 , SO 2 , NO 2 and O 3 , respectively. During 2020, the cities of Dhaka (182.18), Delhi (106.36), Ulaanbaatar (11.19), Seoul (26.86) and Jerusalem (36.62) also had the highest values of AQI, respectively. In terms of AQI-CO, many cities had average values of 0.10 in 2020 and Istanbul with 19.69 had the highest AQI-CO.
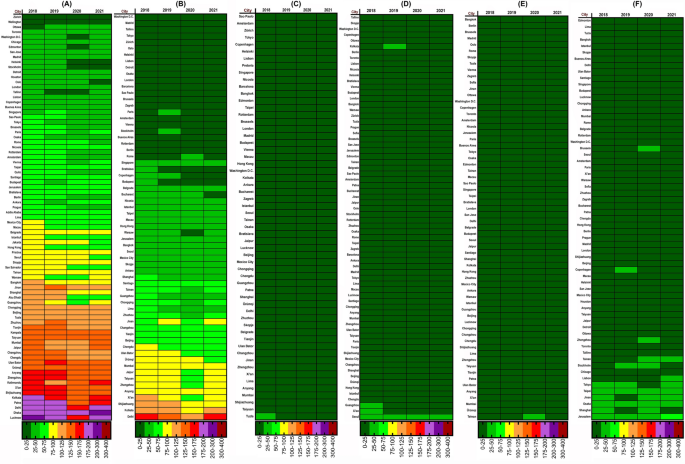
Heat Map created with GraphPad Prism showing average medians of the four first months the AQIs following 2020 lockdown and comparisons with 2018, 2019 and 2021. A AQI-PM 2.5 variation, B AQI-PM 10 variation, C AQI-SO 2 variation, D AQI-NO 2 variation, E AQI-CO variation and F AQI-O 3 variation
According to the findings in Fig. 3 , most of the problems caused by air pollution are related to particles, which are shown in Fig. 3 A, B. AQI values in these pollutants have been reported for them up to 200–300 (Very Unhealthy). All cities surveyed had AQI < 100 for SO 2 , NO 2 , CO and O 3 . The results are shown in Figs. 4 and 5 to determine the percentage difference between the values of 2020 and 2019 and 2021 (before, during and after of lockdown).
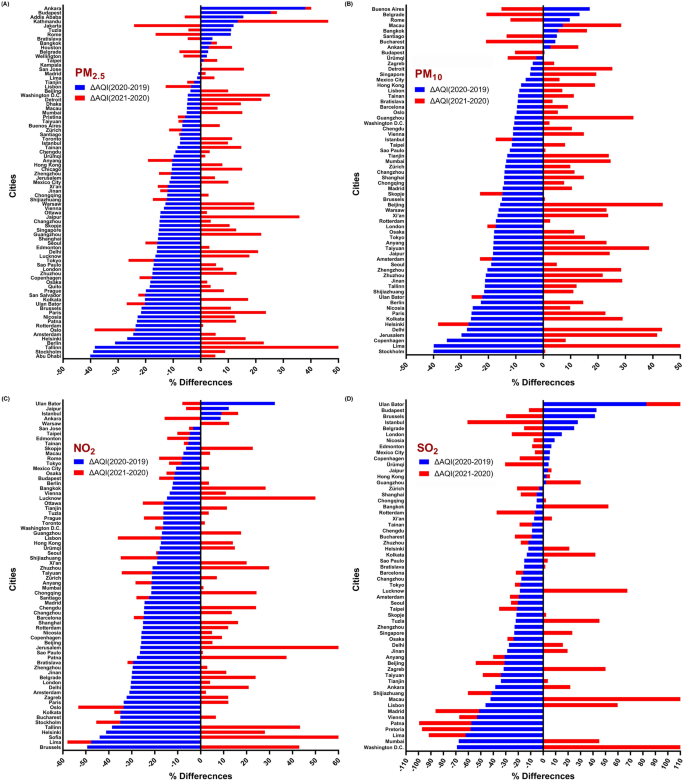
Percentage change of AQI PM 2.5 , PM 10 , NO 2 and SO 2 between 2020 during the lockdown period and 2019 and 2021 in different cities in the world. A AQI-PM 2.5 variation, B AQI-PM 10 variation, C AQI-NO 2 variation and D AQI-SO 2 variation
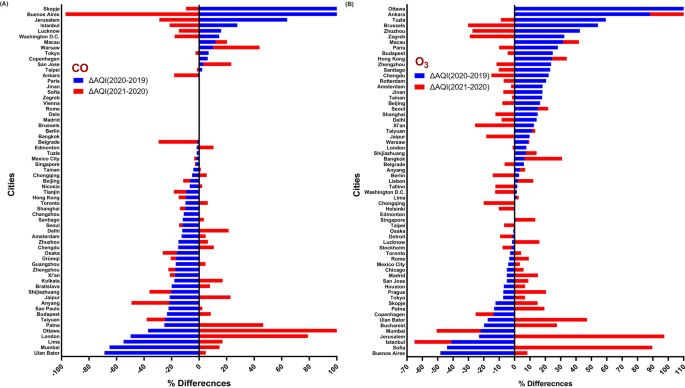
Percentage change of AQI-CO and O 3 between 2020 during the lockdown period and 2019 and 2021 in different cities in the world. A AQI-CO variation and B AQI-O 3 variation
According to Fig. 3 , cities in which the quality index has decreased or increased, compared to 2019, could be distinguished. Based on Fig. 4 , the cities of Abu Dhabi (− 40.13%) and Stockholm (− 40.05%) have the highest percentage decrease of PM 2.5 and PM 10 and the cities of Ankara (+ 37.97%) and Buenos Aires (+ 16.95%) have the highest percentage increase in 2020 compared to 2019.
Actually, in cities with a positive difference percentage, the AQI has not only decreased, but also increased. The results show that only 25% (17/67) and 13% (7/55) of the cities that had lower AQI-PM 2.5 and AQI-PM 10 in 2020 than in 2019 had also a decreasing trend in 2021, but in other cases, AQI values have increased during 2021.
The cities of Washington (-69.15%) and Ulaanbaatar (+ 82.78%) had the most negative and positive differences in terms of AQI-SO 2 in 2020 compared to 2019, respectively, although air quality in Washington has declined in 2021 and has had the highest increase (+ 206.34%) among all cities.
Also, in terms of NO 2 pollution, Brussels (− 49.41%) and Ulaanbaatar (+ 32.33%) reported the most negative and positive differences in 2020 compared to 2019, respectively.
The result of AQI-CO illustrated that the cities of Ulaanbaatar (− 68.81%) and Skopje (+ 6089.22%) had the highest negative and positive differences compared to 2019, although in 2021 AQI-CO has significantly increased in the Ottawa (+ 124.64%). Another major pollutant in air quality is tropospheric O 3 , which has the highest percentage of negative and positive differences in 2020 compared to 2019 for the cities of Brussels (− 48.29%) and Ottawa (+ 9515.12%). The percentage difference of AQI for pollutants in other cities between 2020 with 2019 and 2021 can be seen in Figs. 4 and 5 . The results showed that in general, AQI had a significant relationship between different pollutants in cities with complete data between 2020, 2018 and 2019 ( P < 0.01), except for AQI-O3, which there was no statistically significant report ( P > 0.05) between 2020 and 2018. Figure 6 (box and whiskers chart) shows the median values, along with the minimum, maximum and first and third quarters of the data for all cities. Also, the results of the Wilcoxon test analysis with star and NA symbols are shown in Fig. 5 . It also reports the percentage difference between the median values of 2020 and 2018 as well as 2019 and 2021.
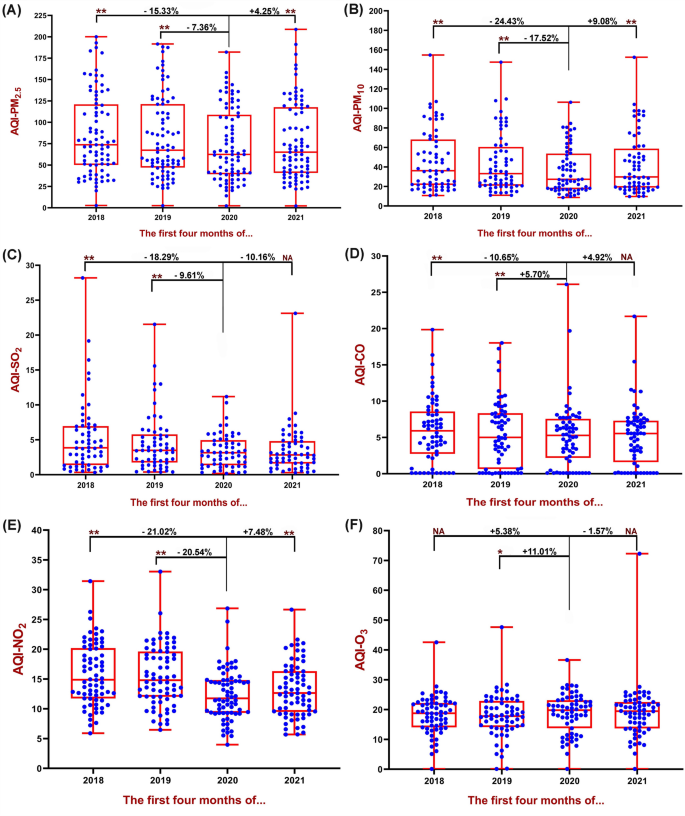
Box and whiskers plot depicting AQI variations for 87 cities in the World. The data show average of the first 4 months of 2018–2021 (January 1st–April 30th). A AQI-PM 2.5 variation, B AQI-PM 10 variation, C AQI-SO 2 variation, D AQI-CO variation, C AQI-NO 2 variation and C AQI-O 3 variation. ** P < 0.01, * P < 0.05, NA > 0.05
The results showed that the values of the AQI-PMs have decreased during rising the COVID-19 in 2020 (7.36% for PM 2.5 and 17.52% for PM 10 ), but during 2021, these values increased significantly (4.25% for PM 2.5 and 9.08% for PM 10 ). Also, AQI-SO2 has decreased compared to 2019 (9.61%), but for CO (5.7%) and O 3 (11.01%), these values have increased. Also, the difference between the average AQI-NO 2 has significantly decreased in 2020 compared to 2019 (20.54%) and 2018 (21.02%); however, these values have increased significantly in 2021 (7.48%).
Figure 7 is drawn to compare the percentage change of different cities for meteorological variables in 2020 compared to 2019 and 2021. Calcutta (− 28.59%), Tallinn (− 20,750.64%) and Mexico City ( − 26.77%) had the highest percentage of decrease in wind speed, temperature and relative humidity, while Pretoria (+ 1734.55%), Helsinki (+ 913.18%) and Tokyo (+ 85.37%) had the highest percentage increase in 2020 compared to 2019.
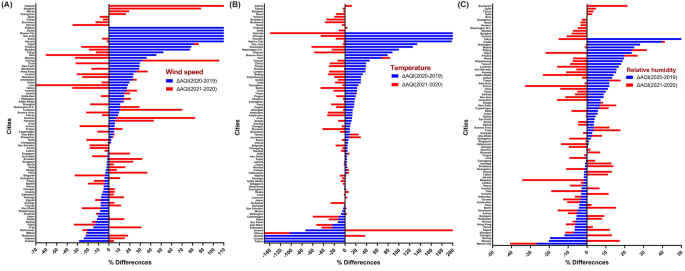
Percentage change of meteorological variables between 2020, during the lockdown period and 2019 and 2021 in different cities in the world. A Wind speed variation, B temperature variation and C relative humidity variation
Figure 8 shows the box and whiskers diagrams for meteorological variables in the 3 years from 2019 to 2021. According to the findings of the study, the average temperature, wind speed and relative humidity in 2020 compared to 2019 have increased to values of + 37.60%, + 7.00% and + 1.01%, respectively ( P < 0.05)
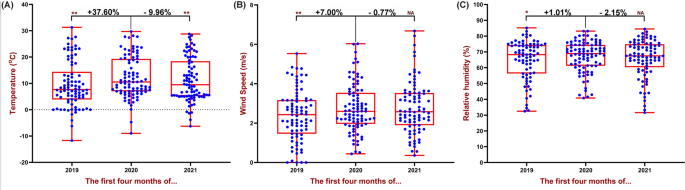
Box and whiskers plot depicting meteorological factors variations for 87 cities in the World. The data show average of the first 4 months of 2018–2021 (January 1st–April 30th). A temperature variation, B wind speed variation and C relative humidity variation. ** P < 0.01, * P < 0.05, NA: P > 0.05
Correlation and regression analysis of meteorological variables and AQI
The association between the AQI of different pollutants and meteorological variables for 2019, 2020 and 2021 is reported in Fig. 9 .
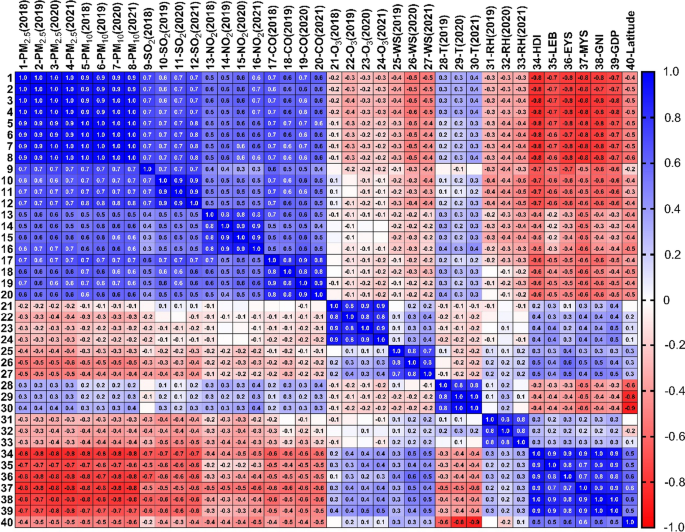
Correlations between AQI’s air pollutants and meteorological and socio-economic factors during 2020, lockdown periods and 2018, 2019 and 2021
The results of correlation analysis showed that there was a significant negative correlation between AQI-PMs and wind speed in all years ( P < 0.05). However, there was a negative and significant correlation between wind speed and AQI-SO 2 and AQI-NO 2 only in 2020 ( P < 0.05).
Also, a positive and significant correlation was observed between wind speed and AQI-O 3 in 2020 and 2021 ( r = 0.27 in 2020 and r = 0.39 in 2021; P < 0.05). AQI-PM 2.5 and temperature also showed a positive and significant correlation in all 3 years ( P < 0.01). Furthermore, a negative and significant difference was observed between relative humidity and AQI-PMs, as well as SO 2 and NO 2 in all years ( P < 0.05).
The results of multiple regression analyses for the AQI of each pollutant by adjusting the meteorological variables are reported in Table 1 . The results showed a correlation among AQI-PMs and AQI-SO 2 with relative humidity (β = − 1.55 for PM 2.5 , β = − 0.88 for PM 10 and β = − 0.10 for SO 2 , P < 0.001).
Socioeconomic and dependent variables
Other analyses were accomplished for country categories (based on HDI and GNI) to investigate the average AQI variables in different categories. There were significant differences between AQI parameters in developed and developing, as well as high-income and < UMI countries ( P < 0.01) (Fig. 10 ). Among developing countries, the median (IQR) of AQI-PM 2.5 , AQI-PM 10 , AQI-SO 2 and AQI-NO 2 were 117.00 (IQR: 86.00–136.00), 60.20 (IQR: 42.60–76.50), 4.41 (IQR: 3.20–5.89), 14.60 (IQR: 11.10–16.80), which increased with decreasing national HDI ( P < 0.01). Also, the mentioned AQI variables in high-income countries were lower than upper-middle income countries and increased with the decrease in national HDI (Figs. 10 , 11 ). Furthermore, a negative and significant correlation was observed between HDI, GDP and GNI with AQI-PMs and AQI-CO ( r > 0.65 for PMs and r > 0.40 for CO; P < 0.01). On the other hand, there is a positive association between HDI, GDP and GNI and AQI-SO 2, AQI-NO 2 and AQI-O 3 in all 3 years ( P < 0.01). Furthermore, a negative and significant difference was observed between HDI, GDP and GNI, socio-economic variables and temperature, as well as a positive correlation for relative humidity and wind speed ( P < 0.05). There is a positive association between wind speed and latitude, but a negative association for temperature and latitude ( P < 0.01).
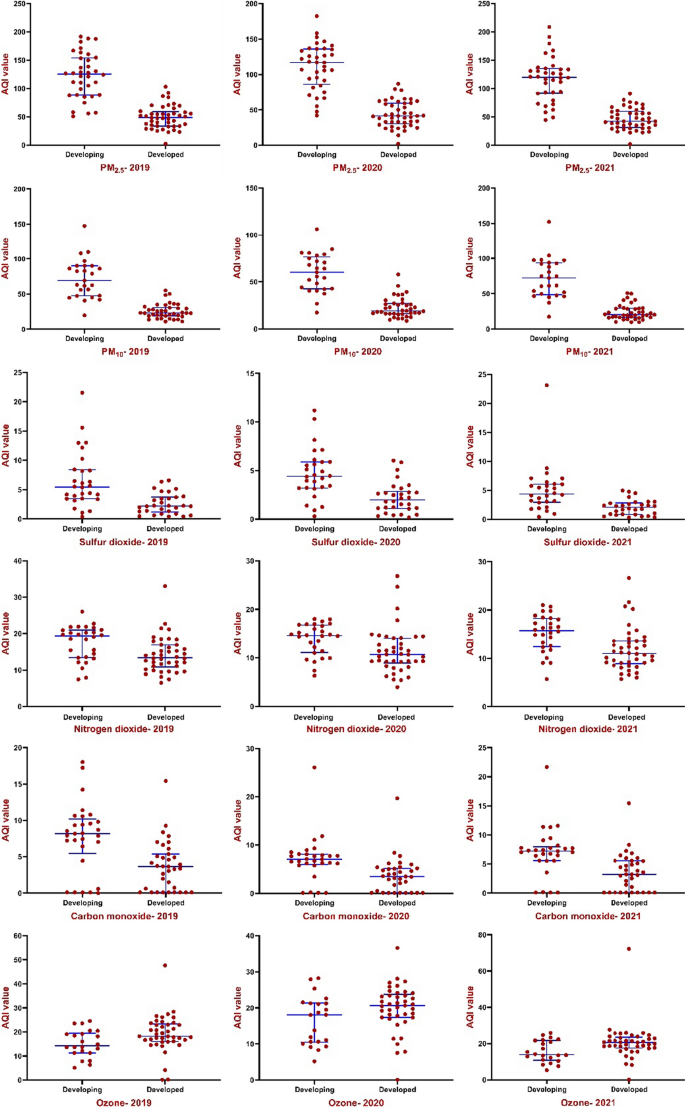
Differences of AQI parameters in developed and developing countries by independent-sample t-test. AQI values in developing countries were significantly higher than those in developed countries except for ozone. Horizontal blue lines represent group median with interquartile range
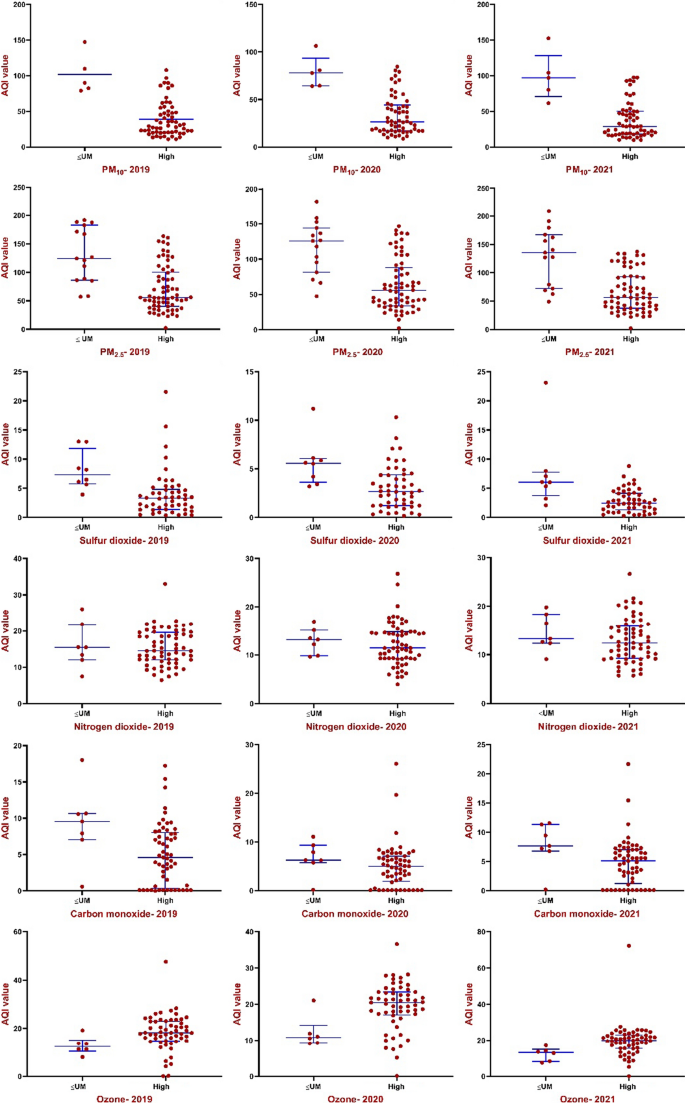
Differences of AQI parameters in high-income and upper-middle and lower (< UM) income countries by independent-sample t -test. AQI values in high-income countries were significantly lower than those in < UM countries except ozone. Horizontal blue lines represent group median with interquartile range
The results of the present study showed that the AQI of the first 4 months of the year in most cities in 2020, compared to the period before the COVID-19 pandemic (2019), has significantly improved; however, this has been reversed for many cities in 2021 (Figs. 4 , 5 ). What is certain is that most large and industrial cities are exposed to particulate pollutants and nitrogen oxides [ 45 ], which according to the findings of the present study, the AQI-PM 2.5 and NO 2 during the lockdown period in 2020 have increased significantly (Fig. 6 ). These results belong to the outdoor environment, but indoor environments may be different [ 46 , 47 , 48 ]. Our findings are in line with several published studies that were indicative of reducing these pollutants due to lockdown and widespread restrictions in countries from the beginning of January to the end of May [ 11 , 49 , 50 , 51 , 52 ]. Studies show that the Community Mobility Index, which represents the activities of educational sectors, transport, industries and social places, has significantly decreased during the first half of 2020 and has been effective in distributing concentrations of environmental pollutants [ 40 ]. Moreover, Fig. 3 A clearly shows that in most of the cities surveyed, which were in poor condition in terms of AQI-PM 2.5 in the same period before the pandemic, mentioned index was improved during the first 4 months of 2020 and with the establishment of COVID-19 restrictions and its related factors. Studies have shown that the use of e-Learning during the COVID-19 era might significantly reduce traffic and driving and thus reduce environmental pollution [ 53 , 54 ].
The reduction of pollutants is also seen in Fig. 3 B for PM 10 . Although PM 10 concentrations in the environment are usually higher than PM 2.5 but associated with PM 2.5 pollution are greater in urban areas. Given the health problems caused by PM 2.5 , this improvement in the AQI will be noteworthy for health policymakers. The results showed that the highest levels of AQI-PM 2.5 were observed in the cities of Patna, Delhi and Dhaka in 2019 and in the cities of Lucknow, Delhi, Dhaka, in 2020, respectively. Of the four cities mentioned, 3 are related to India and the other is related to Bangladesh, which are countries with low air quality and income. One of the reasons for the high number of PMs in these cities, in addition to the large transport fleet [ 55 ], is the use of household fuels such as wood and dry waste in these cities [ 10 , 34 , 56 ]. Also, in low-income or developing countries, due to the weak economy and lack of full implementation of environmental laws, the AQI in their cities is usually unfavorable [ 57 , 58 ], which is in line with the results of our study.
India has been one of the countries with a sharp decline in the index of retail and recreation activities, transit stations and workplaces [ 40 ] and as a result, the sharp decline in AQI values in Indian cities may be due to this. It should be noted that to interpret the results of PM reduction, it is important to know the source of their production. PMs in an urban environment can be caused by the combustion of fossil fuels in vehicles as well as by emissions from various industries and natural events [ 59 , 60 , 61 , 62 ]. On the other hand, phenomena such as inversion in densely populated and industrial cities can act as a cover and prevent the spread of particles and pollutants in wider environments, thereby increasing the concentration of pollutants in the city. This phenomenon is more observed in areas with cold weather and cloudless skies, especially in winters [ 10 , 63 ]. Overall, the findings showed that the average AQI-PM 2.5 in 2020 compared to 2019 and 2018 was decreased by about 7% and 15% and AQI-PM 10 declined by 18% and 24%, which indicates an overall improvement in PMs quality (Fig. 6 A, B). It should be noted that many air quality monitoring stations may be located in areas close to major roads and airports, which will show high particulate matter levels.
Also, the average AQI-NO 2 in 2020, compared to 2019 and 2018, has decreased by about 21% (Fig. 6 E). The results of studies have shown that as the restrictions caused by COVID-19 in countries increased, the intensity reduction of PMs pollutants and NO 2 have also increased [ 40 , 64 ]. It has also shown that Gross Domestic Product (GDP) in most countries has declined during the second quarter of 2020, reflecting the lockdown impact on the economy and industrial closures [ 40 ]. Higher concentrations of pollutants are higher in developing cities except for ozone concentrations (Figs. 10 , 11 ). Several studies showed that developing countries are in a poor situation in terms of air pollution than developed countries, which is due to strict environmental requirements and high-income status in developed countries [ 57 , 65 ]. On the other hand, during the global economic recession from 2003 to 2007, it was shown that the concentration of NO 2 emissions has decreased by about 20% [ 66 ].
Reports from European organizations revealed that the most important source of NO 2 emissions in European countries is related to road transport [ 39 , 67 , 68 ]. It has also been reported that during the years from 2017 to 2018, the concentrations of NO 2 , SO 2 and CO were decreased by 4.1, 6.7 and 4.3%, respectively [ 67 ]. In a study of 20 major cities around the world, Sannigrahi et al. reported that restrictions in countries between February 1 and May 11 have reduced the amount of NO 2 by about 28 tons and CO by about 184 tons in these 20 cities [ 26 ]. On the other hand, it has reduced about 1310, 401 and 430 premature deaths associated with NO 2 , PM 2.5 and PM 10 . However, previous studies have shown a significant association between air pollution and COVID-19 disease [ 69 , 70 , 71 ].
In general, AQI-SO 2 has decreased by 11% in 2020 compared to 2019, which can be referred to as the closure of medium and large industries and travel restrictions in the transport fleet, which are the most important sources of sulfur production [ 72 ]. Also, the production of acid rain is of effects associated with high concentrations of SO 2 and NO 2 ; it has detrimental effects on the environment and aquatic ecosystems. In addition, acid rain on fertile lands washes away minerals and devalues soil [ 10 , 73 ]. A study in Delhi, India, showed that lockdown significantly reduced the concentrations of SO 2 and nitrogen oxide [ 74 ]. Also, the results of a study in New York showed that AQI-SO 2 was decreased from 1.29 to 0.52 (59.68%) during the lockdown period and was decreased from 30.45 to 20.20 (33.66%) for NO 2 [ 75 ].
Ozone levels compared to 2019 have generally increased by about 11% (− 48 to + 88%), which is consistent with various studies [ 36 , 37 , 38 , 40 ]. Two studies in Barcelona, Spain [ 76 ], and Rajasthan, India [ 77 ], showed that, during the lockdown period, ozone concentrations were increased by about 50% and 45%, respectively. In addition, Wang et al. estimated that urban areas have significantly higher ozone levels than rural areas (145% vs. 46%) [ 76 ]. Studies have shown that NO 2 is formed from the reaction of NO with ozone [ 78 , 79 ] and if NO 2 levels decrease, ozone levels are likely to increase, which an increase in AQI-O 3 levels was observed in 60% of the cities of the present study, compared to 2019. Actually, due to the fact that ozone is a secondary pollutant and is dependent on precursors such as nitrogen oxides and volatile organic compounds (VOC), an increase in ozone concentration in the atmosphere can be expected if the emission of its precursors decreases [ 80 ].
Finally, it should be noted that meteorological variables can also, directly and indirectly, affect the concentration of pollutants in an area, which should be considered in interpreting the results of COVID-19 disease [ 3 , 81 ]. Among the effects of meteorological variables are emission, transport, deformation and deposition [ 82 ]. The results of regression analyses showed that there is a significant inverse association between relative humidity and AQI-PM 2.5 , AQI-PM 10 and AQI-SO 2 in the presence of climatic variables of temperature and wind speed, while this association was reported to be positive and insignificant for ozone (Table 1 ). A study in three major cities in China has shown that the association between temperature and ozone is directly and the correlation between wind speed and air pollutants is the inverse [ 82 ], which is consistent with the results of our study. Another study in Turkey showed that low ambient temperature and low wind speed were associated with high concentrations of PMs and SO 2 [ 83 ]. It is also commonly mentioned that ozone production can be limited in low temperature and high rainfall conditions [ 84 ].
Strengths and limitation
To our best knowledge, this is among the first studies to examine the changes in AQI before, during and after 2020. In this study, considering the selection of large, industrial and capital cities as a sample size, the effects of lockdown and the executive constraints of countries can be further examined, compared and measured. On the other hand, according to the conducted literature review, most restrictions have been implemented in the first 4 months of 2020 and countries have reduced the severity of restrictions from May onwards; thus, in this study, the first 4 months in different years were intended as the basis of studies. In addition, the advantage of this study compared to other studies is the investigation of all indicators of standard pollution, which in the presence of each other, gives us a better view of the air quality of a city. However, the COVID-19 and environmental studies have several limitations that must be considered in each ecological study [ 85 ]. The first limitation is the location of air quality monitoring stations, which was not considered in this study due to lack of access. Also, the exact history of lockdowns is different for each city, which cannot be separated, but in general, considering the mean median of the data for several months and in different years, the impact of lockdowns with these differences is evident. This work did not take into account the specific situation of the epidemic situation of COVID-19 in various countries, the strict degree of epidemic prevention measures taken by various countries and the differences in the level of industrialization, urbanization and modernization of the cities. The reported result of higher AQI in high-income countries might confound by having good record stations in precise estimates on AQI. On the other hand, the elimination of some pollutants and especially meteorological variables (not adjusted in regression analysis) due to the lack of complete reporting of their values in all stations is another limitation of this study. Furthermore, the lack of access to long-term information on pollutants in cities was led to the consideration of fewer cities and comparison only with 2018 and 2019. Other factors such as improvement in AQI criteria, access to technologies services and increased awareness of the disease may occur also in countries with upper-level income that do not consider in this study. Failure to consider factors such as the transfer of particulate matters from one area to another and the factors affecting them in studies of environmental pollutants, especially particulate matters, are considered design limitations.
Although the COVID-19 pandemic had irreparable destructive effects on human societies, it has been able to improve the air quality of most areas with executive restrictions in different countries. Findings illustrated that about 83, 86, 91, 76, 79 and 41% of cities revealed a decrease in AQI values of PM 2.5 , PM 10 , NO 2 , SO 2 , CO and O 3 in 2020 compared to 2019. Also, the results showed that AQI generally improved for all pollutants except carbon monoxide and ozone in 2020, compared to 2019, in the 87 cities (8–25%); however, changes in 2021 compared to 2020 have been reversed and AQI values for PM 2.5 , PM 10 , CO and NO 2 pollutants have generally increased (4–7%) due to the reduction of countries restrictions. In general, the implementation of strict laws related to COVID-19 restrictions can show the executive power of countries in reducing pollutants in non-crisis situations. Although this quality improvement was temporary, it is an important result that health policymakers can use to improve environmental conditions and maintain human health.
Availability of data and materials
All data generated or analyzed during this study are included in this published article (“ Methods ” section) and publicly available dataset. Data related to the AQI variables available in https://aqicn.org/ website. Also, the datasets used and/or analyzed during the current study are available from the corresponding author on reasonable request.
Abbreviations
Air quality index
Carbon monoxide
Coronavirus disease 2019
Gross domestic product
Interquartile range
- Nitrogen dioxide
- Particulate matter
Sulfur dioxide
Strengthening the reporting of observational studies in epidemiology
Standard deviation
World Air Quality Index
Severe acute respiratory syndrome coronavirus 2
United Kingdom
United States
US Environmental Protection Agency
World Health Organization
Guan W-J et al (2020) Clinical characteristics of coronavirus disease 2019 in China. N Engl J Med 382(18):1708–1720
Article CAS Google Scholar
Sarmadi M et al (2021) COVID-19, body mass index and cholesterol: an ecological study using global data. BMC Public Health 21(1):1712
Sarmadi M, Marufi N, Kazemi Moghaddam V (2020) Association of COVID-19 global distribution and environmental and demographic factors: an updated three-month study. Environ Res 188:109748
Hughes JW, Hughes CO, Seneca JJ (2020) Coronavirus economic recuperation continues: New Jersey and the nation—not yet back to the future. Rutgers University. p. 38
Martin R (2021) Rebuilding the economy from the COVID crisis: time to rethink regional studies? Reg Stud Reg Sci 8(1):143–161
Google Scholar
World Health Organization. WHO Director-General’s opening remarks at the media briefing on COVID-19. 11 March 2020. 2020 [cited 2021 15 June]; Available from: https://www.who.int/director-general/speeches/detail/who-director-general-s-opening-remarks-at-the-media-briefing-on-covid-19---11-march-2020
Tison GH et al (2020) Worldwide effect of COVID-19 on physical activity: a descriptive study. Ann Intern Med 173(9):767–770
Article Google Scholar
Zhang H et al (2021) Global association between satellite-derived nitrogen dioxide (NO 2 ) and lockdown policies under the COVID-19 pandemic. Sci Total Environ 761:144148
Health, A.G.D.o. Easing the COVID-19 restrictions—no time for complacency, An opinion piece by the Australian Government’s Deputy Chief Medical Officer, Professor Michael Kidd . 2020 [cited 2021 20 June]; Available from: https://www.health.gov.au/news/easing-the-covid-19-restrictions-no-time-for-complacency
Vadrevu KP et al (2020) Spatial and temporal variations of air pollution over 41 cities of India during the COVID-19 lockdown period. Sci Rep 10(1):16574
Zhang Z et al (2020) Unprecedented temporary reduction in global air pollution associated with COVID-19 forced confinement: a continental and city scale analysis. Remote Sens 12(15):2420
Johannson KA, Balmes JR, Collard HR (2015) Air pollution exposure: a novel environmental risk factor for interstitial lung disease? Chest 147(4):1161–1167
GOV.UK. Department for Transport publishes official statistics relating to the transport system in Great Britain. 2021 [cited 2021 20 April]; Available from: https://www.gov.uk/government/organisations/department-for-transport/about/statistics
Crippa M et al (2018) Gridded emissions of air pollutants for the period 1970–2012 within EDGAR v4.3.2. Earth Syst Sci Data 10(4):1987–2013
Alexis NE et al (2010) Low-level ozone exposure induces airways inflammation and modifies cell surface phenotypes in healthy humans. Inhal Toxicol 22(7):593–600
Larsen ST et al (2010) Ozone increases airway hyperreactivity and mucus hyperproduction in mice previously exposed to allergen. J Toxicol Environ Health A 73(11):738–747
Scannell C et al (1996) Greater ozone-induced inflammatory responses in subjects with asthma. Am J Respir Crit Care Med 154(1):24–29
Katoto PDMC et al (2021) Acute and chronic exposure to air pollution in relation with incidence, prevalence, severity and mortality of COVID-19: a rapid systematic review. Environ Health 20(1):41
Páez-Osuna F, Valencia-Castañeda G, Rebolledo UA (2022) The link between COVID-19 mortality and PM2.5 emissions in rural and medium-size municipalities considering population density, dust events, and wind speed. Chemosphere 286:131634
Linares C et al (2021) Short-term associations of air pollution and meteorological variables on the incidence and severity of COVID-19 in Madrid (Spain): a time series study. Environ Sci Eur 33(1):107
World Health Organization. Air pollution. 2021 [cited 2021 July 9]; Available from: https://www.who.int/health-topics/air-pollution#tab=tab_1
European Environment Agency. Air quality in Europe: 2020 report. 2021 Sep 2020; 164]. Available from: https://www.eea.europa.eu//publications/air-quality-in-europe-2020-report
Balakrishnan K et al (2019) The impact of air pollution on deaths, disease burden, and life expectancy across the states of India: the Global Burden of Disease Study 2017. The Lancet Planetary Health 3(1):e26–e39
Chakraborty P et al (2020) Exposure to nitrogen dioxide (NO 2 ) from vehicular emission could increase the COVID-19 pandemic fatality in India: a perspective. Bull Environ Contam Toxicol 105(2):198–204
Chen K et al (2020) Air pollution reduction and mortality benefit during the COVID-19 outbreak in China. The Lancet Planetary Health 4(6):e210–e212
Sannigrahi S et al (2021) Examining the status of improved air quality in world cities due to COVID-19 led temporary reduction in anthropogenic emissions. Environ Res 196:110927
Babatola SS (2018) Global burden of diseases attributable to air pollution. J Public Health Afr 9(3):813–813
Achakulwisut P et al (2019) Global, national, and urban burdens of paediatric asthma incidence attributable to ambient NO 2 pollution: estimates from global datasets. The Lancet Planetary Health 3(4):e166–e178
Liu Q et al (2021) Spatiotemporal impacts of COVID-19 on air pollution in California, USA. Sci Total Environ 750:141592
Briz-Redón Á, Belenguer-Sapiña C, Serrano-Aroca Á (2021) Changes in air pollution during COVID-19 lockdown in Spain: a multi-city study. J Environ Sci 101:16–26
Wyche KP et al (2021) Changes in ambient air quality and atmospheric composition and reactivity in the South East of the UK as a result of the COVID-19 lockdown. Sci Total Environ 755:142526
Baldasano JM (2020) COVID-19 lockdown effects on air quality by NO 2 in the cities of Barcelona and Madrid (Spain). Sci Total Environ 741:140353
Bao R, Zhang A (2020) Does lockdown reduce air pollution? Evidence from 44 cities in northern China. Sci Total Environ 731:139052
Gautam AS et al (2021) Temporary reduction in air pollution due to anthropogenic activity switch-off during COVID-19 lockdown in northern parts of India. Environ Dev Sustain 23(6):8774–8797
Gössling S, Humpe A (2020) The global scale, distribution and growth of aviation: implications for climate change. Global Environ Change 65:102194
Wang Y et al (2021) Enhanced atmospheric oxidation capacity and associated ozone increases during COVID-19 lockdown in the Yangtze River Delta. Sci Total Environ 768:144796
Siciliano B et al (2020) Increased ozone levels during the COVID-19 lockdown: analysis for the city of Rio de Janeiro, Brazil. Sci Total Environ 737:139765
Brancher M (2021) Increased ozone pollution alongside reduced nitrogen dioxide concentrations during Vienna’s first COVID-19 lockdown: significance for air quality management. Environ Pollut 284:117153
Higham JE et al (2021) UK COVID-19 lockdown: 100 days of air pollution reduction? Air Qual Atmos Health 14(3):325–332
He C et al (2021) Global, continental, and national variation in PM2.5, O 3 , and NO 2 concentrations during the early 2020 COVID-19 lockdown. Atmos Pollut Res 12(3):136–145
Elm EV et al (2007) Strengthening the reporting of observational studies in epidemiology (STROBE) statement: guidelines for reporting observational studies. BMJ 335(7624):806–808
World Air Quality Index. Air Quality Historical Data Platform. 2021 [cited 2021 25 April]; Available from: https://aqicn.org/contact/ .
Singh V et al (2020) Diurnal and temporal changes in air pollution during COVID-19 strict lockdown over different regions of India. Environ Pollut 266:115368
Xu Z et al (2017) Socioeconomic status is associated with global diabetes prevalence. Oncotarget 8(27):44434
Zhao X et al (2019) Spatiotemporal variation in PM2.5 concentrations and their relationship with socioeconomic factors in China’s major cities. Environ Int 133:105145
Du W et al (2021) Influence of COVID-19 lockdown overlapping Chinese Spring Festival on household PM2.5 in rural Chinese homes. Chemosphere 278:130406
Noorimotlagh Z et al (2021) A systematic review of possible airborne transmission of the COVID-19 virus (SARS-CoV-2) in the indoor air environment. Environ Res 193:110612
Liu H et al (2021) Investigating SARS-CoV-2 persistent contamination in different indoor environments. Environ Res 202:111763
Bauwens M et al (2020) Impact of coronavirus outbreak on NO 2 pollution assessed using TROPOMI and OMI observations. Geophys Res Lett 47(11):e2020GL087978
Kazakos V, Taylor J, Luo Z (2021) Impact of COVID-19 lockdown on NO 2 and PM2.5 exposure inequalities in London, UK. Environ Res 198:111236
Ravindra K et al (2021) Impact of COVID-19 lockdown on ambient air quality in megacities of India and implication for air pollution control strategies. Environ Sci Pollut Res 28(17):21621–21632
Venter ZS et al (2020) COVID-19 lockdowns cause global air pollution declines. Proc Natl Acad Sci 117(32):18984–18990
Favale T et al (2020) Campus traffic and e-Learning during COVID-19 pandemic. Comput Netw 176:107290
Agarwal A et al (2021) Effect of E-learning on public health and environment during COVID-19 lockdown. Big Data Mining Anal 4(2):104–115
Li L et al (2020) Air quality changes during the COVID-19 lockdown over the Yangtze River Delta Region: an insight into the impact of human activity pattern changes on air pollution variation. Sci Total Environ 732:139282
Kumar P et al (2020) Temporary reduction in fine particulate matter due to ‘anthropogenic emissions switch-off’ during COVID-19 lockdown in Indian cities. Sustain Cities Soc 62:102382
Krzyzanowsk M, Schwela D (1999) 7 - Patterns of air pollution in developing countries. In: Holgate ST et al (eds) Air pollution and health. Academic Press, London, pp 105–113
Chapter Google Scholar
Bruce N, Perez-Padilla R, Albalak R (2000) Indoor air pollution in developing countries: a major environmental and public health challenge. Bull World Health Organ 78:1078–1092
CAS Google Scholar
Andrade MDF et al (2017) Air quality in the megacity of São Paulo: evolution over the last 30 years and future perspectives. Atmos Environ 159:66–82
Castanho ADA, Artaxo P (2001) Wintertime and summertime São Paulo aerosol source apportionment study. Atmos Environ 35(29):4889–4902
Guo H et al (2017) Source apportionment of PM2.5 in North India using source-oriented air quality models. Environ Pollut 231:426–436
Pereira GM et al (2017) Particulate pollutants in the Brazilian city of São Paulo: 1-year investigation for the chemical composition and source apportionment. Atmos Chem Phys 17(19):11943–11969
Freitas ED et al (2007) Interactions of an urban heat island and sea-breeze circulations during winter over the metropolitan area of São Paulo, Brazil. Bound-Layer Meteorol 122(1):43–65
Nigam R et al (2021) Positive effects of COVID-19 lockdown on air quality of industrial cities (Ankleshwar and Vapi) of Western India. Sci Rep 11(1):4285
West S et al. (2021) Urban air pollution and children in low- and middle- income countries
Jin-Tai L, Da P, Rui-Xiong Z (2013) Trend and interannual variability of Chinese air pollution since 2000 in association with socioeconomic development: a brief overview. Atmos Ocean Sci Lett 6(2):84–89
European Environment Agency (2020) European Union emission inventory report 1990–2018, under the UNECE Convention on Long-range Transboundary Air Pollution (LRTAP). Luxembourg: Publications Office of the European Union, 2020. p. 160
Sicard P et al (2020) Amplified ozone pollution in cities during the COVID-19 lockdown. Sci Total Environ 735:139542
Konstantinoudis G et al (2021) Long-term exposure to air-pollution and COVID-19 mortality in England: a hierarchical spatial analysis. Environ Int 146:106316
Travaglio M et al (2021) Links between air pollution and COVID-19 in England. Environ Pollut 268:115859
Sarmadi M et al (2021) Association of COVID-19 distribution with air quality, sociodemographic factors, and comorbidities: an ecological study of US states. Air Qual Atmos Health 14(4):455–465
Roy, S., et al., Geospatial analysis of COVID-19 lockdown effects on air quality in the South and Southeast Asian region. Science of The Total Environment, 2021. 756 : p. 144009.
Manisalidis I et al (2020) Environmental and health impacts of air pollution: a review. Front Public Health 8(14):13
Mahato S, Pal S, Ghosh KG (2020) Effect of lockdown amid COVID-19 pandemic on air quality of the megacity Delhi, India. Sci Total Environ 730:139086
Shehzad K et al. (2021) COVID-19 outbreak, lockdown, and air quality: fresh insights from New York City. Environ Sci Pollut Res
Wang L et al (2020) Unexpected rise of ozone in urban and rural areas, and sulfur dioxide in rural areas during the coronavirus city lockdown in Hangzhou, China: implications for air quality. Environ Chem Lett 18(5):1713–1723
Sharma S et al (2020) Effect of restricted emissions during COVID-19 on air quality in India. Sci Total Environ 728:138878
Bechle MJ, Millet DB, Marshall JD (2013) Remote sensing of exposure to NO 2 : satellite versus ground-based measurement in a large urban area. Atmos Environ 69:345–353
Munir S, Chen H, Ropkins K (2012) Modelling the impact of road traffic on ground level ozone concentration using a quantile regression approach. Atmos Environ 60:283–291
Liu H et al (2019) Emissions and health impacts from global shipping embodied in US–China bilateral trade. Nature Sustain 2(11):1027–1033
Sarmadi M et al. (2021) Interaction between meteorological parameters and COVID-19: an ecological study on 406 authorities of the UK . Environ Sci Pollut Res
Zhang H et al (2015) Relationships between meteorological parameters and criteria air pollutants in three megacities in China. Environ Res 140:242–254
Ilten N, Selici AT (2008) Investigating the impacts of some meteorological parameters on air pollution in Balikesir, Turkey. Environ Monit Assess 140(1):267–277
Kwak H-Y et al (2017) Identifying the correlation between rainfall, traffic flow performance and air pollution concentration in Seoul using a path analysis. Transport Res Procedia 25:3552–3563
Barceló MA, Saez M (2021) Methodological limitations in studies assessing the effects of environmental and socioeconomic variables on the spread of COVID-19: a systematic review. Environ Sci Eur 33(1):108
Download references
Acknowledgements
The authors would like to thank the Torbat Heydariyeh University of Medical Sciences for their financial support.
This work was supported by the Torbat Heydariyeh University of Medical Sciences (Grant Number: 99000217).
Author information
Authors and affiliations.
Department of Environmental Health Engineering, School of Health, Torbat Heydariyeh University of Medical Sciences, Torbat Heydariyeh, Iran
Mohammad Sarmadi & Sajjad Rahimi
Health Sciences Research Center, Torbat Heydariyeh University of Medical Sciences, Torbat Heydariyeh, Iran
Mohammad Sarmadi, Sajjad Rahimi & Mina Rezaei
Department of Environmental Health Engineering, Faculty of Public Health and Safety, Shahid Beheshti University of Medical Science, Tehran, Iran
Daryoush Sanaei
Department of Complex Genetics and Epidemiology, School of Nutrition and Translational Research in Metabolism, Maastricht University, Maastricht, The Netherlands
Mostafa Dianatinasab
You can also search for this author in PubMed Google Scholar
Contributions
MS: conceptualization, methodology, data curation, formal analysis, writing—original draft preparation, writing—review and editing, supervision, funding acquisition. SR: conceptualization, data curation, formal analysis, writing—original draft preparation, writing—review an editing. MR: data curation, writing—original draft, writing—review and editing. DS: data curation, writing—original draft, writing—review and editing. MD: methodology, formal analysis, writing—original draft preparation. All authors read and approved the final manuscript.
Corresponding authors
Correspondence to Mohammad Sarmadi or Sajjad Rahimi .
Ethics declarations
Ethics approval and consent to participate.
The ethics code (IR.THUMS.REC.1400.008) was obtained from the Ethics Committee of Torbat Heydariyeh University of Medical Sciences. This study works with aggregate data, therefore there are no individual data, and therefore the consent to participate is not applicable.
Consent for publication
Not applicable.
Competing interests
The authors declare that they have no known competing financial interests or personal relationships that could have appeared to influence the work reported in this paper.
Additional information
Publisher's note.
Springer Nature remains neutral with regard to jurisdictional claims in published maps and institutional affiliations.
Rights and permissions
Open Access This article is licensed under a Creative Commons Attribution 4.0 International License, which permits use, sharing, adaptation, distribution and reproduction in any medium or format, as long as you give appropriate credit to the original author(s) and the source, provide a link to the Creative Commons licence, and indicate if changes were made. The images or other third party material in this article are included in the article's Creative Commons licence, unless indicated otherwise in a credit line to the material. If material is not included in the article's Creative Commons licence and your intended use is not permitted by statutory regulation or exceeds the permitted use, you will need to obtain permission directly from the copyright holder. To view a copy of this licence, visit http://creativecommons.org/licenses/by/4.0/ .
Reprints and permissions
About this article
Cite this article.
Sarmadi, M., Rahimi, S., Rezaei, M. et al. Air quality index variation before and after the onset of COVID-19 pandemic: a comprehensive study on 87 capital, industrial and polluted cities of the world. Environ Sci Eur 33 , 134 (2021). https://doi.org/10.1186/s12302-021-00575-y
Download citation
Received : 30 September 2021
Accepted : 20 November 2021
Published : 05 December 2021
DOI : https://doi.org/10.1186/s12302-021-00575-y
Share this article
Anyone you share the following link with will be able to read this content:
Sorry, a shareable link is not currently available for this article.
Provided by the Springer Nature SharedIt content-sharing initiative
- Air quality index
- Restriction
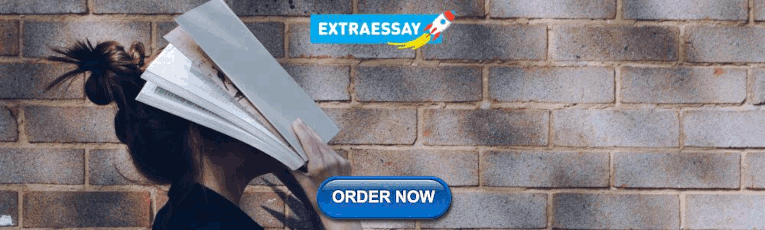
COMMENTS
The Air Quality Index (AQI) demonstrates improvement during the lockdown as its value dramatically dropped (45 - 68 %). ... method like mean, median, or mode of each feature in which the lost values are found. 3.2 Training of Models In this research paper, the training model is developed on the Python platform with the help of sklearn and ...
This paper aims to analyze the air quality in India and the effects of seasons and COVID-19 on the concentration of pollutants in the air and thereby their effect on the air quality index (AQI). The analysis is performed on a full scale, taking into consideration different levels of granularities such as daily, weekly and monthly data. This study performs extensive preprocessing of the time ...
The Air Quality Index (AQI) in India is steadily deteriorating, leading to a rise in the mortality rate due to Lung Cancer. This decline in air quality can be attributed to various factors such as ...
ABSTRACT India suffers from among the worst air pollution in the world. In response, a large government effort to increase air quality monitoring is underway. We present the first comprehensive analysis of government air quality observations from 2015-2019 for PM10, PM2.5, SO2, NO2 and O3 from the Central Pollution Control Board (CPCB) Continuous Ambient Air Quality Monitoring (CAAQM ...
2.1. Data assimilation for the assessment of air pollution parameters. Remote sensing data were analyzed on Google Earth Engine, which allows geospatial analysis (Gorelick et al., 2017).NO 2 and O 3 data were collected from the Copernicus Sentinel-5 Precursor Tropospheric Monitoring Instrument, which is widely used for air quality-based applications (Veefkind et al., 2012) to monitor the daily ...
We forecast the air quality of India by using machine learning to predict the air quality index of a given area. Air quality index of India is a standard measure used to indicate the pollutant (so2, no2, rspm, spm. etc.) levels over a period. We developed a model to predict the air quality index based on historical data of
The air quality index is the measuring number of total air pollution in the air which includes PM2.5, PM10, CO2, CO, NOx, and HC. ... Find a journal Publish with us Track your research Search. Cart. Home ... Scenario of Air Quality Index in India and Its Effect on Human Health and Policies for Green and Clean India. Conference paper; First ...
Abstract: Rapid urbanisation has led to degradation in air quality index in past decades caused by pollutants generated by factories, industries and transportation. Designing an automated system for air quality tracking and monitoring is essential for generating awareness. Restrictions imposed by COVID 19 lockdown has resulted in the degradation of pollutants in air and having a great impact ...
Based on the air pollutant concentration, Air Quality Index (AQI) is deliberated. ... In this paper, several scenarios have been taken for checking the impacts of lockdown on the air quality throughout the world. ... on ambient aerosols (PM2.5): a study on Indo-Gangetic Plain (IGP) Cities, India. Stochastic Environmental 660 Research and Risk ...
AQI—An air quality index is a digit used by government workplaces to show how the environment is actually sullied or how bad it is to get [].SO 2 —Sulfur dioxide (SO 2) adds to respiratory side effects in both sound patients and those with basic pneumonic illness.Controlled human introduction considers have shown that test SO 2 presentation causes changes in aviation route physiology ...
Six different methods of estimating Air quality Index (AQI) based on four pollutants synergistic effect viz., PM10, PM2.5, SO2 and NO2 were used to compare the prevailing ambient air quality in ...
In this paper, we have studied the different research papers and made a comparative study to find the research gaps. The proposed model is a step up in the standard of living in regard to human health. The proposed model is consisting of three main components a) Air quality index b) health impact and c) safest path.
on air quality in near rea l time and to forecast 1-3 days in advance for the first time in I ndia, which is combined wi th the early warning system on weather parameter.
[email protected]. 2 Department of Environment Engineering, Lovely Professional University, Phagwara - 144411, Punjab, India; [email protected], [email protected]. Abstract ...
publisher of research papers in 2022, but it was ranked only 153rd for the number of ... The University of Chicago's Air Quality Life Index ranks India as the second-most-polluted
A national ambient air quality standard of China was released in 1996 (NAAQS-1996) to define API calculation. The API (Air Pollution Index) is an index that indicates the pollution level of the atmosphere, ranging from 0 to 500. The higher the API value, the heavier the atmospheric pollution.
Abstract. The increasing level of pollutants in ambient air in (2015-2020) has deteriorated the air quality of India at an. alarming rate. In the present study, the changes of the concentration of ...
Another recent article indicated that out of 31 research papers published during 2010-2020 investigating the role of different air pollutants on the health of city dwellers using the ... As per the air quality index (AQI) Of India, the range 0-100 is considered a good category, 100-200 as moderate, 200-300 as poor, and above 300 as very ...
Background Coronavirus disease 2019 (COVID-19) pandemic provided an opportunity for the environment to reduce ambient pollution despite the economic, social and health disruption to the world. The purpose of this study was to investigate the changes in the air quality indexes (AQI) in industrial, densely populated and capital cities in different countries of the world before and after 2020. In ...
Quality In dex values. This is done as, if the A ir Quality. Index value is b etween 0-50 then it is classified as goo d, 51 -100 as moderate, 101-150 as unhealthy for sensitive. groups, 151-200 ...