Investigation and Implementation of Model Order Reduction Technique for Large Scale Dynamical Systems
- Review article
- Published: 14 January 2022
- Volume 29 , pages 3087–3108, ( 2022 )
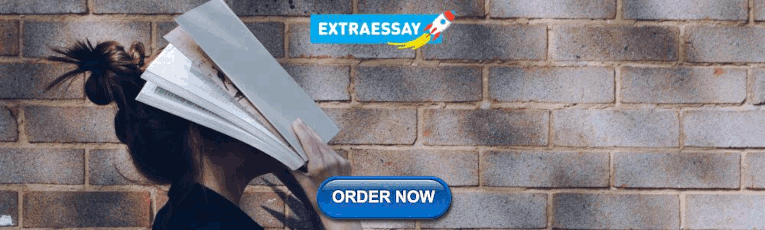
Cite this article
- Santosh Kumar Suman ORCID: orcid.org/0000-0003-1178-3234 1 &
- Awadhesh Kumar 1
976 Accesses
5 Citations
Explore all metrics
Model Order Reduction (MOR) has demonstrated its robustness and wide use in engineering and science for the simulation of large-scale mathematical systems over the last few decades. MOR is currently being intensively optimized for dynamic systems that are becoming increasingly complex. MOR broad applications have been identified not only in the modeling but also for optimization and control engineering applications. In the present article, various methods related to MOR for large-scale linear and nonlinear dynamic systems have been analyzed, mainly pertaining to electrical power systems, control engineering, computational system theory, and design. The paper focuses on a detailed theoretic Perspective for MOR of the large-scale dynamical system to address the key challenges to the approximation along with their application. Firstly, a complete description of the literature search for various approximation techniques has been presented and the various inferences have been mentioned as the outcome. One of the drawbacks that we have found out of the investigation, has been taken as a sample problem. The key demerit of the balanced truncation approach is that the ROM steady-state values do not correspond with the higher-order system (HOS). This drawback has been eliminated in the proposed approach, which leads to the hybridization of balanced truncation and singular perturbation approximation (SPA) into a novel reduction method without the loss of retaining its dynamic behavior. The reduced system has been so designed to preserve the complete parameters of the original system with reasonable accuracy. The approach is based on the retention of the dominant states or modes of the system and is comparatively less important once. The reduced system evolves from the preservation of the dominant states or modes of the original system and thus retains stability intact. The methodology presented has been tested on two typical numerical examples taken from the literature review, to examine the performance, precision, and comparison with other available order reduction methods.
This is a preview of subscription content, log in via an institution to check access.
Access this article
Price includes VAT (Russian Federation)
Instant access to the full article PDF.
Rent this article via DeepDyve
Institutional subscriptions
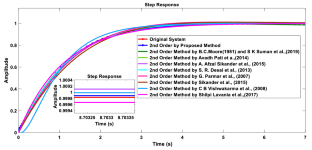
Similar content being viewed by others
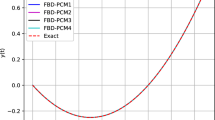
Higher order numerical methods for fractional delay differential equations
Manoj Kumar, Aman Jhinga & Varsha Daftardar-Gejji
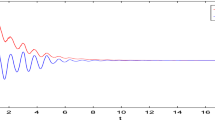
Exponential stability analysis in mean square for a class of stochastic delay differential equations
Yingxin Guo, Shuzhi Sam Ge & Yue Ma
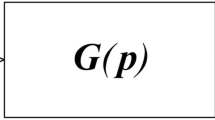
Static and dynamic topology optimization: an innovative unifying approach
Paolo Venini & Marco Pingaro
Data Availability
My manuscript has no associated data.
Li S, Xiang Z (2020) Sampled-data decentralized output feedback control for a class of switched large-scale stochastic nonlinear systems. IEEE Syst J 14(2):1602–1610. https://doi.org/10.1109/JSYST.2019.2934512
Article Google Scholar
Patalano S, Mango Furnari A, Vitolo F, Dion JL, Plateaux R, Renaud F (2021) A critical exposition of model order reduction techniques: application to a slewing flexible beam. Arch Comput Methods Eng. https://doi.org/10.1007/s11831-019-09369-1
Article MathSciNet Google Scholar
He W, Li S, Ahn CK, Guo J, Xiang Z (2020) Global decentralized control of p-normal large-scale nonlinear systems based on sampled-data output feedback. IEEE Syst J. https://doi.org/10.1109/jsyst.2020.2997029
Chan SC, Wu HC, Ho CH, Zhang L (2019) An augmented Lagrangian approach for distributed robust estimation in large-scale systems. IEEE Syst J 13(3):2986–2997. https://doi.org/10.1109/JSYST.2019.2897788
Sikander A, Prasad R (2015) Soft computing approach for model order reduction of linear time invariant systems. Circuits Syst Signal Process 34(11):3471–3487. https://doi.org/10.1007/s00034-015-0018-4
Kumar J, Sikander A, Mehrotra M, Parmar G (2020) A new soft computing approach for order diminution of interval system. Int J Syst Assur Eng Manag 11:366–373. https://doi.org/10.1007/s13198-019-00865-y
Antoulas AC, Benner P, Feng L (2018) Model reduction by iterative error system approximation. Math Comput Model Dyn Syst 42(2):103–118. https://doi.org/10.1080/13873954.2018.1427116
Article MathSciNet MATH Google Scholar
Sikander A, Prasad R (2015) Linear time-invariant system reduction using a mixed methods approach. Appl Math Model 39(16):4848–4858. https://doi.org/10.1016/j.apm.2015.04.014
Sikander A, Prasad R (2019) Reduced order modelling based control of two wheeled mobile robot. J Intell Manuf 30(3):1057–1067. https://doi.org/10.1007/s10845-017-1309-3
Uniyal I, Sikander A (2018) A comparative analysis of PID controller design for AVR based on optimization techniques. In: Advances in intelligent systems and computing, pp 1315–1323. https://doi.org/10.1007/978-981-10-5903-2_138
Prajapati AK, Prasad R (2019) Reduced-order modelling of LTI systems by using Routh approximation and factor division methods. Circuits Syst Signal Process 38(7):3340–3355. https://doi.org/10.1007/s00034-018-1010-6
Baur U, Benner P, Feng L (2014) Model order reduction for linear and nonlinear systems: a system-theoretic perspective. Arch Comput Methods Eng 21(4):331–358. https://doi.org/10.1007/s11831-014-9111-2
Rowley CW (2005) Model reduction for fluids, using balanced proper orthogonal decomposition. Int J Bifurcat Chaos 15(3):997–1013. https://doi.org/10.1142/S0218127405012429
Schilders WHA, Van Der Vorst HA, Rommes J (2008) Model order reduction: theory, research aspects and applications, vol 13. Springer, Berlin
Book MATH Google Scholar
Sundström D (1985) Mathematics in industry. Int J Math Educ Sci Technol. https://doi.org/10.1080/0020739850160226
Mohamed KS (2018) Machine learning for model order reduction. Springer, Berlin
Book Google Scholar
Schilders WHA, van der Vorst HA, Rommes J (2008) Model order reduction: theory, research aspects and applications. Springer, Berlin
Chaturvedi DK (2017) Model order reduction. In: Modeling and simulation of systems using MATLAB ® and Simulink ® . CRC Press, Boca Raton
Pivovarov D et al (2019) Challenges of order reduction techniques for problems involving polymorphic uncertainty. GAMM Mitteilungen. https://doi.org/10.1002/gamm.201900011
Bui-Thanh T, Willcox K, Ghattas O (2008) Parametric reduced-order models for probabilistic analysis of unsteady aerodynamic applications. AIAA J 46(10):2520–2529. https://doi.org/10.2514/1.35850
Mendonça G, Afonso F, Lau F (2019) Model order reduction in aerodynamics: review and applications. Proc Inst Mech Eng Part G J Aerosp Eng 233(15):5816–5836. https://doi.org/10.1177/0954410019853472
Baur U, Benner P, Greiner A, Korvink JG, Lienemann J, Moosmann C (2011) Parameter preserving model order reduction for MEMS applications. Math Comput Model Dyn Syst 17(4):297–317. https://doi.org/10.1080/13873954.2011.547658
Anand S, Fernandes BG (2013) Reduced-order model and stability analysis of low-voltage dc microgrid. IEEE Trans Ind Electron. https://doi.org/10.1109/TIE.2012.2227902
Mariani V, Vasca F, Vásquez JC, Guerrero JM (2015) Model order reductions for stability analysis of islanded microgrids with droop control. IEEE Trans Ind Electron 62(7):4344–4354. https://doi.org/10.1109/TIE.2014.2381151
Bai Z (2002) Krylov subspace techniques for reduced-order modeling of large-scale dynamical systems. Appl Numer Math 43(1–2):9–44. https://doi.org/10.1016/S0168-9274(02)00116-2
Freund RW (2000) Krylov-subspace methods for reduced-order modeling in circuit simulation. J Comput Appl Math 123(1–2):395–421. https://doi.org/10.1016/S0377-0427(00)00396-4
Freund RW (2004) SPRIM: structure-preserving reduced-order interconnect macromodeling. In: IEEE/ACM international conference on computer-aided design, Digest of Technical Papers, ICCAD, pp 80–87. https://doi.org/10.1109/iccad.2004.1382547
Wang X, Yu M, Wang C (2018) Structure-preserving-based model-order reduction of parameterized interconnect systems. Circuits Syst Signal Process 37(1):19–48. https://doi.org/10.1007/s00034-017-0561-2
Freund RW (2011) The SPRIM algorithm for structure-preserving order reduction of general RCL circuits. In: Lecture notes in electrical engineering, pp 25–52. https://doi.org/10.1007/978-94-007-0089-5_2
Beattie C, Gugercin S (2009) Interpolatory projection methods for structure-preserving model reduction. Syst Control Lett 58(2):225–232. https://doi.org/10.1016/j.sysconle.2008.10.016
Odabasioglu A, Celik M, Pileggi LT (1997) PRIMA: Passive reduced-order interconnect macromodeling algorithm. In: IEEE/ACM international conference on computer-aided design, Digest of Technical Papers, pp 433–450. https://doi.org/10.1007/978-1-4615-0292-0_34
Odabasioglu A, Celik M, Pileggi LT (1998) PRIMA: Passive reduced-order interconnect macromodeling algorithm. IEEE Trans Comput Des Integr Circuits Syst 17(8):645–654. https://doi.org/10.1109/43.712097
Ionutiu R, Rommes J, Antoulas AC (2008) Passivity-preserving model reduction using dominant spectral-zero interpolation. IEEE Trans Comput Des Integr Circuits Syst 27(12):2250–2263. https://doi.org/10.1109/TCAD.2008.2006160
Fanizza G, Karlsson J, Lindquist A, Nagamune R (2006) A global analysis approach to passivity preserving model reduction. In: Proceedings of the IEEE conference on decision and control, pp 3399–3404. https://doi.org/10.1109/cdc.2006.376706
Antoulas AC (2005) A new result on passivity preserving model reduction. Syst Control Lett 54(4):361–374. https://doi.org/10.1016/j.sysconle.2004.07.007
Farle O, Burgard S, Dyczij-Edlinger R (2011) Passivity preserving parametric model-order reduction for non-affine parameters. Math Comput Model Dyn Syst 17(3):279–294. https://doi.org/10.1080/13873954.2011.562901
Fuchs A (2013) Nonlinear dynamics in complex systems. Springer, Berlin, p 2008
Burkardt J, Du Q, Gunzburger M, Lee H-C (2003) Reduced order modeling of complex systems. NA03 Dundee
Sargsyan S, Brunton SL, Kutz JN (2015) Nonlinear model reduction for dynamical systems using sparse sensor locations from learned libraries. Phys Rev E Stat Nonlinear Soft Matter Phys. https://doi.org/10.1103/PhysRevE.92.033304
Rafiq D, Bazaz MA (2021) Nonlinear model order reduction via nonlinear moment matching with dynamic mode decomposition. Int J Non Linear Mech. https://doi.org/10.1016/j.ijnonlinmec.2020.103625
Venna J, Kaski S, Aidos H, Nybo K, Peltonen J (2010) Information retrieval perspective to nonlinear dimensionality reduction for data visualization. J Mach Learn Res 11(2):451–490
MathSciNet MATH Google Scholar
Lassila T, Manzoni A, Quarteroni A, Rozza G (2014) Model order reduction in fluid dynamics: challenges and perspectives. In: Reduced order methods for modeling and computational reduction
Preisner T, Mathis W (2010) Scientific computing in electrical engineering SCEE 2008. Springer, Berlin
Google Scholar
Rudnyi E, Korvink J (2006) Modern model order reduction for industrial applications. Report, Germany
Gray PR, Meyer RG (2009) Analysis and design of analog integrated circuits. Wiley, Hoboken
Tan S, He L (2007) Advanced model order reduction techniques in VLSI design. Cambridge University Press, New York
Kerschen G, Golinval JC, Vakakis AF, Bergman LA (2005) The method of proper orthogonal decomposition for dynamical characterization and order reduction of mechanical systems: an overview. Nonlinear Dyn 41(1):147–169. https://doi.org/10.1007/s11071-005-2803-2
Bond B, Daniel L (2005) Parameterized model order reduction of nonlinear dynamical systems. In: IEEE/ACM international conference on computer-aided design, Digest of Technical Papers, ICCAD, pp 487–494. https://doi.org/10.1109/ICCAD.2005.1560117
Qu Z-Q (2004) Model order reduction techniques with applications in finite element analysis. Springer, London
MATH Google Scholar
Brozek T, Iniewski KK (2017) Micro-and nanoelectronics: emerging device challenges and solutions. CRC Press, Boca Raton
Lienemann J, Billger D, Rudnyi EB, Greiner A, Korvink JG (2004) MEMS compact modeling meets model order reduction: examples of the application of Arnoldi methods to microsystem devices
Rudnyi EB, Korvink JG (2006) Model order reduction for large scale engineering models developed in ANSYS. In: International workshop on applied parallel computing. Springer, Berlin, Heidelberg, pp 349–356. https://doi.org/10.1007/11558958_41
Djukic S, Saric A (2012) Dynamic model reduction: an overview of available techniques with application to power systems. Serbian J Electr Eng 9(2):131–169. https://doi.org/10.2298/sjee1202131d
Veroy K, Prud’Homme C, Rovas DV, Patera AT (2003) A posteriori error bounds for reduced-basis approximation of parametrized noncoercive and nonlinear elliptic partial differential equations. In: 16th AIAA computational fluid dynamics conference, p. 3847. https://doi.org/10.2514/6.2003-3847
Rozza G, Huynh DBP, Patera AT (2008) Reduced basis approximation and a posteriori error estimation for affinely parametrized elliptic coercive partial differential equations: application to transport and continuum mechanics. Arch Comput Methods Eng 15(3):229–275. https://doi.org/10.1007/s11831-008-9019-9
Jung N, Patera AT, Haasdonk B, Lohmann B (2011) Model order reduction and error estimation with an application to the parameter-dependent eddy current equation. Math Comput Model Dyn Syst. https://doi.org/10.1080/13873954.2011.582120
Haasdonk B, Ohlberger M (2011) Efficient reduced models and a posteriori error estimation for parametrized dynamical systems by offline/online decomposition. Math Comput Model Dyn Syst. https://doi.org/10.1080/13873954.2010.514703
Lorenzi S, Cammi A, Luzzi L, Rozza G (2016) POD-Galerkin method for finite volume approximation of Navier–Stokes and RANS equations. Comput Methods Appl Mech Eng 311:151–179. https://doi.org/10.1016/j.cma.2016.08.006
Star K, Stabile G, Georgaka S, Belloni F, Rozza G, Degroote J (2019) Pod-Galerkin reduced order model of the Boussinesq approximation for buoyancy-driven enclosed flows. In: International conference on mathematics and computational methods applied to nuclear science and engineering, M and C 2019, pp. 2452–2461
Bui-Thanh T, Damodaran M, Willcox K (2003) Proper orthogonal decomposition extensions for parametric applications in compressible aerodynamics. In: 21st AIAA applied aerodynamics conference, p. 4213. https://doi.org/10.2514/6.2003-4213
Thomas PV, ElSayed MSA, Walch D (2019) Review of model order reduction methods and their applications in aeroelasticity loads analysis for design optimization of complex airframes. J Aerosp Eng. https://doi.org/10.1061/(asce)as.1943-5525.0000972
Binder A, Jadhav O, Mehrmann V (2020) Model order reduction for parametric high dimensional interest rate models in the analysis of financial risk. arXiv
Parmar G, Mukherjee S, Prasad R (2007) System reduction using factor division algorithm and Eigen spectrum analysis. Appl Math Model 31(11):2542–2552. https://doi.org/10.1016/j.apm.2006.10.004
Article MATH Google Scholar
Hwang C, Chen MY (1987) Stable linear system reduction via a multipoint tangent phase continued-fraction expansion. J Chin Inst Eng Trans Chin Inst Eng A/Chung-kuo K Ch’eng Hsuch K’an. https://doi.org/10.1080/02533839.1987.9676990
Antoulas AC (2005) An overview of approximation methods for large-scale dynamical systems. Annu Rev Control. https://doi.org/10.1016/j.arcontrol.2005.08.002
Pal J (1979) Stable reduced-order padé approximants using the Routh–Hurwitz array. Electron Lett 15(8):225–226. https://doi.org/10.1049/el:19790159
Pindor M (2006) Padé approximants. Lect Notes Control Inf Sci. https://doi.org/10.1007/11601609_4
Guillaume P, Huard A (2000) Multivariate Pade approximation. J Comput Appl Math. https://doi.org/10.1016/S0377-0427(00)00337-X
Shamash Y (1975) Linear system reduction using pade approximation to allow retention of dominant modes. Int J Control 21(2):257–272. https://doi.org/10.1080/00207177508921985
Shamash Y (1975) Multivariable system reduction via modal methods and Padé approximation. IEEE Trans Autom Control 20(6):815–817. https://doi.org/10.1109/TAC.1975.1101090
Verma P, Juneja PK, Chaturvedi M (2017) Various mixed approaches of model order reduction. In: Proceedings - 2016 8th international conference on computational intelligence and communication networks, CICN 2016, pp 673–676. https://doi.org/10.1109/CICN.2016.138
Adamou-Mitiche ABH, Mitiche L, Larbi S (2013) Time and frequency approaches in the approximation problem: a comparative study. https://doi.org/10.1109/ICoSC.2013.6750916
Shamash Y (1975) Model reduction using the routh stability criterion and the Pade approximation technique. Int J Control 21(3):475–484. https://doi.org/10.1080/00207177508922004
Chen TC, Chang CY, Han KW (1980) Model reduction using the stability-equation method and the Padé approximation method. J Frankl Inst 309(6):473–490. https://doi.org/10.1016/0016-0032(80)90096-4
Chen TC, Chang CY, Han KW (1979) Reduction of transfer functions by the stability-equation method. J Frankl Inst 308(4):389–404. https://doi.org/10.1016/0016-0032(79)90066-8
Sikander A, Thakur P, Uniyal I (2017) Hybrid method of reduced order modelling for LTI system using evolutionary algorithm. In: Proceedings on 2016 2nd international conference on next generation computing technologies, NGCT 2016, pp 84–88. https://doi.org/10.1109/NGCT.2016.7877394
Lucas TN (1992) A tabular approach to the stability equation method. J Frankl Inst 329(1):171–180. https://doi.org/10.1016/0016-0032(92)90106-Q
Mohammadpour J, Grigoriadis KM (2010) Efficient modeling and control of large-scale systems. Springer, Berlin
Grimme EJ (1997) Krylov projection methods for model reduction. University of Illinois at Urbana-Champaign
Antoulas AC (2005) Approximation of large-scale dynamical systems. Society for Industrial and Applied Mathematics, Philadelphia
Hochbruck M, Lubich C (1997) On Krylov subspace approximations to the matrix exponential operator. SIAM J Numer Anal. https://doi.org/10.1137/S0036142995280572
Bruaset AM (2019) Krylov subspace methods. In: A survey of preconditioned iterative methods. Routledge, Boca Raton
Kumar D, Nagar SK (2014) Model reduction by extended minimal degree optimal Hankel norm approximation. Appl Math Model 38(11–12):2922–2933. https://doi.org/10.1016/j.apm.2013.11.012
Adamjan VM, Arov DZ, Krein MG (1971) Analytic properties of Schmidt pairs for a Hankel operator and the generalized Schur–Takagi problem. Math USSR Sb 15(1):31. https://doi.org/10.1070/SM1971v015n01ABEH001531
Kung SY, Lin DW (1981) Optimal Hankel-norm model reductions: multivariable systems. IEEE Trans Autom Control 26(4):832–852. https://doi.org/10.1109/TAC.1981.1102736
Latham GA, Anderson BDO (1985) Frequency-weighted optimal Hankel-norm approximation of stable transfer functions. Syst Control Lett 5(4):229–236
Hung YS, Glover K (1986) Optimal Hankel-norm approximation of stable systems with first-order stable weighting functions. Syst Control Lett 7(3):165–172. https://doi.org/10.1016/0167-6911(86)90110-6
Antoulas AC, Beattie CA, Gugercin S (2010) Interpolatory model reduction of large-scale dynamical systems. In: Efficient modeling and control of large-scale systems
Korvink JG, Rudnyi EB, Greiner A, Liu Z (2006) MEMS and NEMS simulation. In: MEMS: a practical guide of design, analysis, and applications
Zhou K (1995) Frequency-weighted L∞ norm and optimal Hankel norm model reduction. IEEE Trans Autom Control. https://doi.org/10.1109/9.467681
Benner P, Quintana-Ortí ES, Quintana-Ortí G (2000) Balanced truncation model reduction of large-scale dense systems on parallel computers. Math Comput Model Dyn Syst. https://doi.org/10.1076/mcmd.6.4.383.3658
Antoulas AC, Sorensen DC, Zhou Y (2002) On the decay rate of Hankel singular values and related issues. Syst Control Lett 46(5):323–342. https://doi.org/10.1016/S0167-6911(02)00147-0
Powel ND, Morgansen KA (2015, December) Empirical observability Gramian rank condition for weak observability of nonlinear systems with control. In 2015 54th IEEE conference on decision and control (CDC). IEEE, pp 6342–6348. https://doi.org/10.1109/CDC.2015.7403218
Grippo L, Palagi L, Piccialli V (2011) An unconstrained minimization method for solving low-rank SDP relaxations of the maxcut problem. Math Program. https://doi.org/10.1007/s10107-009-0275-8
Freitas FD, Rommes J, Martins N (2011, March). Low-rank gramian applications in dynamics and control. In 2011 international conference on communications, computing and control applications (CCCA). IEEE, pp 1–6. https://doi.org/10.1109/CCCA.2011.6031400
Markovsky I (2008) Structured low-rank approximation and its applications. Automatica. https://doi.org/10.1016/j.automatica.2007.09.011
Pal J, Prasad R (1986) Stable low order approximants using continued fraction expansions. In MSE international conference on modelling simulation
Baštuğ M, Petreczky M, Wisniewski R, Leth J (2014, June) Model reduction by moment matching for linear switched systems. In 2014 american control conference. IEEE, pp 3942–3947. https://doi.org/10.1109/ACC.2014.6858983
Bultheel A, Van Barel M (1986) Padé techniques for model reduction in linear system theory: a survey. J Comput Appl Math 14(3):401–438. https://doi.org/10.1016/0377-0427(86)90076-2
Parmar G, Mukherjee S, Prasad R (2007) System reduction using Eigen spectrum analysis and Padé approximation technique. Int J Comput Math. https://doi.org/10.1080/00207160701345566
Prajapati AK, Prasad R (2019) Order reduction in linear dynamical systems by using improved balanced realization technique. Circuits Syst Signal Process 38(11):5289–5303. https://doi.org/10.1007/s00034-019-01109-x
Hutton MF, Friedland B (1975) Routh approximations for reducing order of linear, time-invariant systems. IEEE Trans Autom Control 20(3):329–337. https://doi.org/10.1109/TAC.1975.1100953
Pal J (1980) System reduction by a mixed method. IEEE Trans Autom Control 25(5):973–976. https://doi.org/10.1109/TAC.1980.1102485
Rao AS, Lamba SS, Rao SV (1979) Comments on ‘model reduction using the Routh stability criterion.’ IEEE Trans Autom Control 24(3):518–518. https://doi.org/10.1109/TAC.1979.1102069
Mishra RN, Wilson DA (1980) A new algorithm for optimal reduction of multivariable systems. Int J Control. https://doi.org/10.1080/00207178008961054
Hwang C, Wang KY (1984) Optimal Routh approximations for continuous-time systems. Int J Syst Sci 15(3):249–259. https://doi.org/10.1080/00207728408926558
Goyal R, Parmar G, Sikander A (2019) A new approach for simplification and control of linear time invariant systems. Microsyst Technol. https://doi.org/10.1007/s00542-018-4004-1
Gutman P, Mannerfelt CF, Molander P (1982) Contributions to the model reduction problem. IEEE Trans Autom Control 27(2):454–455. https://doi.org/10.1109/TAC.1982.1102930
Gustafson RD (1966) A paper and pencil control system design. J Fluids Eng Trans ASME 88(2):329–336. https://doi.org/10.1115/1.3645858
Shamash Y, Smamash Y (1981) Truncation method of reduction: a viable alternative. Electron Lett 17(2):97–99. https://doi.org/10.1049/el:19810070
Prajapati AK, Prasad R (2019) Model order reduction by using the balanced truncation and factor division methods. IETE J Res 65(6):827–842. https://doi.org/10.1080/03772063.2018.1464971
Davison EJ (1966) A method for simplifying linear dynamic systems. IEEE Trans Autom Control 11(1):93–101. https://doi.org/10.1109/TAC.1966.1098264
Lucas TN (1983) Factor division: a useful algorithm in model reduction. IEE Proc D Control Theory Appl 130(6):362–364. https://doi.org/10.1049/ip-d.1983.0060
Prajapati AK, Prasad R (2019) Reduced order modelling of linear time invariant systems using the factor division method to allow retention of dominant modes. IETE Tech Rev (Inst Electron Telecommun Eng, India) 36(5):449–462. https://doi.org/10.1080/02564602.2018.1503567
Sikander A, Prasad R (2017) A new technique for reduced-order modelling of linear time-invariant system. IETE J Res 63(3):316–324. https://doi.org/10.1080/03772063.2016.1272436
Wan BW (1981) Linear model reduction using Mihailov criterion and Padè approximation technique. Int J Control 33(6):1073–1089. https://doi.org/10.1080/00207178108922977
Rana J (2013) Order reduction using Mihailov criterion and Pade approximations. Int J Innov Eng Technol 2:19–24
Tomar SK, Prasad R (2008) Linear model reduction using Mihailov stability criterion and continued fraction expansions. J Inst Eng India 84:7–10
El-Attar RA, Vidyasagar M (1978) System order reduction using the induced operator norm and its applications to linear regulators. J Frankl Inst 306(6):457–474. https://doi.org/10.1016/0016-0032(78)90053-4
Khademi G, Mohammadi H, Dehghani M (2013) LMI based model order reduction considering the minimum phase characteristic of the system. In: 2013 9th Asian control conference, ASCC 2013, pp 1–6. https://doi.org/10.1109/ASCC.2013.6606180
Pal J, Ray LM (1980) Stable Padé approximants to multivariable systems using a mixed method. Proc IEEE 68(1):176–178. https://doi.org/10.1109/PROC.1980.11603
Chen TC, Chang CY, Han KW (1980) Model reduction using the stability-equation method and the continued-fraction method. Int J Control 32(1):81–94. https://doi.org/10.1080/00207178008922845
Parthasarathy R (1982) System reduction using stability-equation method and modified cauer continued fraction. Proc IEEE 70(10):1234–1236. https://doi.org/10.1109/PROC.1982.12453
Wittmuess P, Tarin C, Sawodny O (2015, December) Parametric modal analysis and model order reduction of systems with second order structure and non-vanishing first order term. In 2015 54th IEEE conference on decision and control (CDC). IEEE, pp 5352–5357. https://doi.org/10.1109/CDC.2015.7403057
Rahrovani S, Vakilzadeh MK, Abrahamsson T (2014) A metric for modal truncation in model reduction problems part 1: performance and error analysis. In: Conference proceedings of the society for experimental mechanics series, pp 781–788. https://doi.org/10.1007/978-1-4614-6585-0_73
Rózsa P, Sinha NK (1975) Minimal realization of a transfer function matrix in canonical forms. Int J Control. https://doi.org/10.1080/00207177508921986
De Schutter B, De Moor B (1995) Minimal realization in the max algebra is an extended linear complementarity problem. Syst Control Lett. https://doi.org/10.1016/0167-6911(94)00062-Z
Senkal D, Efimovskaya A, Shkel AM (2015, March) Minimal realization of dynamically balanced lumped mass WA gyroscope: dual foucault pendulum. In 2015 IEEE international symposium on inertial sensors and systems (ISISS) proceedings. IEEE, pp 1–2. https://doi.org/10.1109/ISISS.2015.7102394
Aoki M (1968) Control of large-scale dynamic systems by aggregation. IEEE Trans Autom Control. https://doi.org/10.1109/TAC.1968.1098900
Hickin J, Sinha NK (1975) Aggregation matrices for a class of low-order models for large-scale systems. Electron Lett. https://doi.org/10.1049/el:19750142
Hwang C (1984) Aggregation matrix for the reduced-order modified Cauer CFE model. Electron Lett 20(4):150–151. https://doi.org/10.1049/el:19840100
Bandler JW, Markettos ND, Sinha NK (1973) Optimum system modelling using recent gradient methods. Int J Syst Sci. https://doi.org/10.1080/00207727308919993
Maurya MK, Kumar A (2017, April) Dimension reduction and controller design for large scale systems using balanced truncation. In 2017 1st international conference on electronics, materials engineering and nano-technology (IEMENTech). IEEE, pp 1–4. https://doi.org/10.1109/IEMENTECH.2017.8076972
Zhou K, Salomon G, Wu E (1999) Balanced realization and model reduction for unstable systems. Int J Robust Nonlinear Control 9(3):183–198
Antoulas AC (2011) 8. Hankel-Norm approximation. In: Approximation of large-scale dynamical systems, society for industrial and applied mathematics (SIAM, 3600 Market Street, Floor 6, Philadelphia, PA 19104)
Cao X, Saltik MB, Weiland S (2019) Optimal Hankel norm model reduction for discrete-time descriptor systems. J Frankl Inst 356(7):4124–4143. https://doi.org/10.1016/j.jfranklin.2018.11.047
Liu Y, Anderson BDO (1989) Singular perturbation approximation of balanced systems. Int J Control 50(4):1379–1405. https://doi.org/10.1109/cdc.1989.70360
Guiver C (2019) The generalised singular perturbation approximation for bounded real and positive real control systems. Math Control Relat Fields 9(2):313–350. https://doi.org/10.3934/MCRF.2019016
Kumar D, Tiwari JP, Nagar SK (2012) Reducing order of large-scale systems by extended balanced singular perturbation approximation. Int J Autom Control 6(1):21–38. https://doi.org/10.1504/IJAAC.2012.045438
Van Der Vorst HA (2000) Krylov subspace iteration. Comput Sci Eng. https://doi.org/10.1109/5992.814655
Sorensen DC (2002) Numerical methods for large eigenvalue problems. Acta Numer. https://doi.org/10.1017/s0962492902000089
Sambariya DK, Sharma O (2016) Routh approximation: an approach of model order reduction in SISO and MIMO systems. Indones J Electr Eng Comput Sci 2(3):486–500. https://doi.org/10.11591/ijeecs.v2.i3.pp486-500
Antoulas AC, Sorensen DC, Gugercin S (2012) A survey of model reduction methods for large-scale systems
Suman SK, Kumar A (2020) Higher-order reduction of linear system and design of controller. Sci J King Faisal Univ 2020(3):1–16
Boley D, Datta BN (1997) Numerical methods for linear control systems. In: Systems and control in the Twenty-First Century, Birkhäuser, Boston, MA, pp 51–74
Suman SK, Kumar A (2020) Model order reduction of transmission line model. WSEAS Trans Circuits Syst 19:62–68. https://doi.org/10.37394/23201.2020.19.7
Willcox KE, Peraire J (2002) Balanced model reduction via the proper introduction. AIAA J. https://doi.org/10.2514/2.1570
Suman SK, Kumar A (2019) Investigation and reduction of large-scale dynamical systems. WSEAS Trans Syst 18:175–180
Dax A (2013) From Eigenvalues to singular values: a review. Adv Pure Math. https://doi.org/10.4236/apm.2013.39a2002
Suman SK (2019) Approximation of large-scale dynamical systems for bench-mark collection. J Mech Continua Math Sci 14(3):196–215
Gugercin S, Antoulas AC (2006) Model reduction of large-scale systems by least squares. Linear Algebra Appl. https://doi.org/10.1016/j.laa.2004.12.022
Gupta AK, Samuel P, Kumar D (2019) A mixed-method for order reduction of linear time invariant systems using big bang-big crunch and Eigen spectrum algorithm. Int J Autom Control 13(2):158–175. https://doi.org/10.1504/ijaac.2019.10018127
Benner P, Gugercin S, Willcox K (2013) A survey of model reduction methods for parametric systems. In: SIAM Review, Max Planck Institute for dynamics of complex technical systems, pp 1–36
Singh J, Vishwakarma CB, Chattterjee K (2016) Biased reduction method by combining improved modified pole clustering and improved Pade approximations. Appl Math Model 40(2):1418–1426. https://doi.org/10.1016/j.apm.2015.07.014
Sandberg H, Rantzer A (2004) Balanced truncation of linear time-varying systems. IEEE Trans Autom Control 49(2):217–229. https://doi.org/10.1109/TAC.2003.822862
Mukherjee S, Mishra RN (1987) Order reduction of linear systems using an error minimization technique. J Frankl Inst. https://doi.org/10.1016/0016-0032(87)90037-8
Mittal AK, Prasad R, Sharma SP (2004) Reduction of linear dynamic systems using an error minimization technique. J Inst Eng Electr Eng Div 84:201–206
Moore BC (1981) Principal component analysis in linear systems: controllability, observability, and model reduction. IEEE Trans Autom Control 26(1):17–32. https://doi.org/10.1109/TAC.1981.1102568
Fernando KV, Nicholson H (1983) On the structure of balanced and other principal representations of SISO systems. IEEE Trans Autom Control 28(2):228–231. https://doi.org/10.1109/TAC.1983.1103195
Al-Saggaf UM, Franklin GF (1988) Model reduction via balanced realizations: an extension and frequency weighting techniques. IEEE Trans Autom Control 37(3):687–692. https://doi.org/10.1109/9.1280
Samar R, Postlethwaite I, Gu DW (1995) Model reduction with balanced realizations. Int J Control 62(1):33–64. https://doi.org/10.1080/00207179508921533
Clapperton B, Crusca F, Aldeen M (1996) Bilinear transformation and generalized singular perturbation model reduction. IEEE Trans Autom Control. https://doi.org/10.1109/9.489281
Glover K (1984) All optimal Hankel-norm approximations of linear multivariable systems and their L,∞-error bounds†. Int J Control 39(6):1115–1193. https://doi.org/10.1080/00207178408933239
Škatarić D, Kovačević NR (2010) The system order reduction via balancing in view of the method of singular perturbation. FME Trans 38(4):181–187
Benner P, Schneider A (2010) Balanced truncation model order reduction for LTI systems with many inputs or outputs. In: Proceedings of the 19th international symposium on mathematical theory of networks and systems–MTNS, pp 1971–1974, [Online]. Available: http://www.tu-chemnitz.de/mathematik/syrene/papers/BenS_2010_MTNS.pdf
Yasuda M (2004) Spectral characterizations for hermitian centrosymmetric K-matrices and hermitian skew-centrosymmetric K-matrices. SIAM J Matrix Anal Appl 25(3):601–605. https://doi.org/10.1137/S0895479802418835
Prajapati AK, Prasad R (2020) Model reduction using the balanced truncation method and the Padé approximation method. IETE Tech Rev (Inst Electron Telecommun Eng, India). https://doi.org/10.1080/02564602.2020.1842257
Ferranti F, Deschrijver D, Knockaert L, Dhaene T (2011) Data-driven parameterized model order reduction using z-domain multivariate orthonormal vector fitting technique. In: Lecture notes in electrical engineering, pp 141–148. https://doi.org/10.1007/978-94-007-0089-5_7
Gugercin S, Antoulas AC (2004) A survey of model reduction by balanced truncation and some new results. Int J Control 77(8):748–766. https://doi.org/10.1080/00207170410001713448
Imran M, Ghafoor A, Sreeram V (2014) A frequency weighted model order reduction technique and error bounds. Automatica 50(12):3304–3309. https://doi.org/10.1016/j.automatica.2014.10.062
Lall S, Marsden JE, Glavaški S (2002) A subspace approach to balanced truncation for model reduction of nonlinear control systems. Int J Robust Nonlinear Control 12(6):519–535. https://doi.org/10.1002/rnc.657
Segalman DJ (2007) Model reduction of systems with localized nonlinearities. J Comput Nonlinear Dyn 2(3):249–266. https://doi.org/10.1115/1.2727495
Pernebo L, Silverman LM (1982) Model reduction via balanced state space representations. IEEE Trans Autom Control 27(2):382–387. https://doi.org/10.1109/TAC.1982.1102945
Gugercin S (2008) An iterative SVD-Krylov based method for model reduction of large-scale dynamical systems. Linear Algebra Appl 428(9):1964–1986. https://doi.org/10.1016/j.laa.2007.10.041
Fernando KV, Nicholson H (1982) Singular perturbational model reduction of balanced systems. IEEE Trans Autom Control 27(2):466–468. https://doi.org/10.1109/TAC.1982.1102932
Fernando KV, Nicholson H (1982) Singular perturbational model reduction in the frequency domain. IEEE Trans Autom Control 27(4):969–970. https://doi.org/10.1109/TAC.1982.1103037
Kokotovic PV, O’Malley RE, Sannuti P (1976) Singular perturbations and order reduction in control theory—an overview. Automatica 12(2):123–132. https://doi.org/10.1016/0005-1098(76)90076-5
Gu DW, Petkov PH, Konstantinov MM (2013) Lower-order controllers. In: Advanced textbooks in control and signal processing, pp 73–91. Springer, London
Safonov MG, Chiang RY (1989) A Schur method for balanced-truncation model reduction. IEEE Trans Autom Control 34(7):729–733. https://doi.org/10.1109/9.29399
Fernando KV, Nicholson H (1983) Singular perturbational approximations for discrete-time balanced systems. IEEE Trans Autom Control 28(2):240–242. https://doi.org/10.1109/TAC.1983.1103202
Gajic Z, Lelic M (2001) Improvement of system order reduction via balancing using the method of singular perturbations. Automatica 37(11):1859–1865. https://doi.org/10.1016/S0005-1098(01)00139-X
Vishwakarma CB, Prasad R (2009) MIMO system reduction using modified pole clustering and genetic algorithm. Model Simul Eng 2009:1–5. https://doi.org/10.1155/2009/540895
Singh V, Chandra D, Kar H (2004) Improved Routh-Padé approximants: a computer-aided approach. IEEE Trans Autom Control 49(2):292–296. https://doi.org/10.1109/TAC.2003.822878
Narwal A, Prasad BR (2016) A novel order reduction approach for LTI systems using cuckoo search optimization and stability equation. IETE J Res 62(2):154–163. https://doi.org/10.1080/03772063.2015.1075915
Sikander A, Thakur P (2018) Reduced order modelling of linear time-invariant system using modified cuckoo search algorithm. Soft Comput 22(10):3449–3459. https://doi.org/10.1007/s00500-017-2589-4
Sikander A, Thakur P, Bansal RC, Rajasekar S (2018) A novel technique to design cuckoo search based FOPID controller for AVR in power systems. Comput Electr Eng 70:261–274
Pal J (1980) Suboptimal control using Pade approximation techniques. IEEE Trans Autom Control 25(5):1007–1008. https://doi.org/10.1109/TAC.1980.1102490
Pati A, Kumar A, Chandra D (2014) Suboptimal control using model order reduction. Chin J Eng 2014(2):1–5. https://doi.org/10.1155/2014/797581
Sikander A, Rajendra Prasad B (2015) A novel order reduction method using cuckoo search algorithm. IETE J Res. https://doi.org/10.1080/03772063.2015.1009396
Desai SR, Prasad R (2013) A novel order diminution of LTI systems using big bang big crunch optimization and Routh approximation. Appl Math Model 37(16–17):8016–8028. https://doi.org/10.1016/j.apm.2013.02.052
Parmar G, Ssi LM, Prasad R, Mukherjee S (2007) Order reduction of linear dynamic systems using stability equation method and GA. Int J Electr Robot Electron Commun Eng 1(1):26–32
Vishwakarma CBCB, Prasad R (2009) Clustering method for reducing order of linear system using Pade approximation. IETE J Res 54(5):326–330. https://doi.org/10.4103/0377-2063.48531
Lavania S (2017) Hybrid techniques for reduction of linear time-invariant systems. J Simul Syst Sci Technol Int. https://doi.org/10.5013/ijssst.a.18.04.03
Komarasamy R, Albhonso N, Gurusamy G (2012) Order reduction of linear systems with an improved pole clustering. J Vib Control 18(12):1876–1885. https://doi.org/10.1177/1077546311426592
Desai SR, Prasad R (2013) A new approach to order reduction using stability equation and big bang big crunch optimization. Syst Sci Control Eng 1(1):20–27. https://doi.org/10.1080/21642583.2013.804463
Abu-Al-Nadi D (2011) Reduced order modeling of linear MIMO systems using particle swarm optimization. In: Seventh international conference on autonomous systems
Vishwakarma CB (2011) Order reduction using modified pole clustering and Pade approximations. World Acad Sci Eng Technol 56:787–791
Mukherjee S, Mittal RC (2005) Model order reduction using response-matching technique. J Frankl Inst. https://doi.org/10.1016/j.jfranklin.2005.01.008
Download references
Author information
Authors and affiliations.
Department of Electrical Engineering, Madan Mohan Malaviya University of Technology, Gorakhpur, Uttar Pradesh, India
Santosh Kumar Suman & Awadhesh Kumar
You can also search for this author in PubMed Google Scholar
Corresponding author
Correspondence to Santosh Kumar Suman .
Additional information
Publisher's note.
Springer Nature remains neutral with regard to jurisdictional claims in published maps and institutional affiliations.
Rights and permissions
Reprints and permissions
About this article
Suman, S.K., Kumar, A. Investigation and Implementation of Model Order Reduction Technique for Large Scale Dynamical Systems. Arch Computat Methods Eng 29 , 3087–3108 (2022). https://doi.org/10.1007/s11831-021-09690-8
Download citation
Received : 08 April 2021
Accepted : 26 November 2021
Published : 14 January 2022
Issue Date : August 2022
DOI : https://doi.org/10.1007/s11831-021-09690-8
Share this article
Anyone you share the following link with will be able to read this content:
Sorry, a shareable link is not currently available for this article.
Provided by the Springer Nature SharedIt content-sharing initiative
- Find a journal
- Publish with us
- Track your research

An official website of the United States government
The .gov means it’s official. Federal government websites often end in .gov or .mil. Before sharing sensitive information, make sure you’re on a federal government site.
The site is secure. The https:// ensures that you are connecting to the official website and that any information you provide is encrypted and transmitted securely.
- Publications
- Account settings
Preview improvements coming to the PMC website in October 2024. Learn More or Try it out now .
- Advanced Search
- Journal List
- Front Robot AI
- PMC10540691

Reduced order modeling and model order reduction for continuum manipulators: an overview
S.m.h. sadati.
1 Robotics and Vision in Medicine (RViM) Lab, School of Biomedical Engineering and Imaging Sciences, King’s College London, London, United kingdom
S. Elnaz Naghibi
2 Department of Aeronautics, Faculty of Engineering, Imperial College London, London, England, United kingdom
Lyndon da Cruz
3 Wellcome/EPSRC Centre for Interventional and Surgical Sciences, University College London, London, England, United kingdom
4 Moorfields Eye Hospital, London, United kingdom
Christos Bergeles
Sarmad Mehrdad , New York University, United States
Phuc Bui , University of Tulsa, United States
Soft robot’s natural dynamics calls for the development of tailored modeling techniques for control. However, the high-dimensional configuration space of the geometrically exact modeling approaches for soft robots, i.e., Cosserat rod and Finite Element Methods (FEM), has been identified as a key obstacle in controller design. To address this challenge, Reduced Order Modeling (ROM), i.e., the approximation of the full-order models, and Model Order Reduction (MOR), i.e., reducing the state space dimension of a high fidelity FEM-based model, are enjoying extensive research. Although both techniques serve a similar purpose and their terms have been used interchangeably in the literature, they are different in their assumptions and implementation. This review paper provides the first in-depth survey of ROM and MOR techniques in the continuum and soft robotics landscape to aid Soft Robotics researchers in selecting computationally efficient models for their specific tasks.
1 Introduction
Robots with continuum structures can mimic highly dexterous and deformable biological bodies, follow curved paths through body lumens (e.g., vessel interiors) in minimally invasive medical interventions, enable safe interactions with the environment ( Rus and Tolley, 2015 ). Considering compliant structures, however, brings forth issues such as uncertain deformations, limited control feedback, reduced control bandwidth and slow response, stability problems, underdamped modes causing persistent vibrations, and lack of precision under external loads ( Cianchetti et al., 2013 ; Blanc et al., 2017 ).
Soft Roboticists have approached such challenges by proposing new theoretical frameworks and tailored software packages ( Sadati et al., 2020a ). On one hand, geometrically exact Cosserat rod Variable Curvature kinematics and Finite Element methods (FEM) have proven to be of high fidelity ( Trivedi et al., 2008 ; Coevoet et al., 2017 ; Grazioso et al., 2019 ), but bring forth computational obstacles when used in real-time applications, such as control, are considered. On the other hand, Reduced Order Modeling (ROM) and Model Order Reduction (MOR) techniques, where low-dimensional continuous or discreterepresentations are used to approximate the full-order system (i.e., variational) kinematics representation or FEM solution for a continuum structure, can both address modeling challenges and improve computational performance ( Book, 1990 ; Della Santina et al., 2110 ; Goury, Duriez ). Such methods have pioneered methods for flexible-link structures ( Book, 1990 ) and highly articulated ( Chirikjian, 1993 ) robots and, more recently, have been permeating the soft robotics community (Goury, Duriez; Sadati et al., 2018 ; Singh et al., 2018 ).
Although Reduced Order Modeling and Model Order Reduction terms are utilized interchangeably in the literature, this paper makes a distinction and highlights their different assumptions, implementation steps, and capabilities, in order to help with deciding the most appropriate method for a given application. To this end, as instances of Reduced Order Modeling, we review discretization-based, modal, and strain techniques, where the continuum kinematics is constructed based on simplifying assumptions. Alternatively, schemes where the high dimensionality of a continuum robot is reduced, e.g., neglecting the less dominant deformation modes in a FEM solution, are considered as instances of Model Order Reduction. In this paper, we don’t consider reduced order integration schemes, e.g., ( Boyer et al., 2020 ; Godage et al., 2016 ; Caasenbrood et al., 1089 ), which may simplify the derivation of the dynamics without necessarily reducing the modeling state space.
Detailed review papers have recently discussed modeling of continuum robots and slender rods, and readers, novice or expert, can find details on modeling assumptions therein ( Della Santina et al., 2110 ; Rao et al., 2020 ; Gilbert, 2020 ; Sadati et al., 2017a ; Armanini et al., 2023 ). Our paper instead provides a classification based on classic continuum mechanics that highlights how to combine assumptions for a soft robot kinematics, governing equations, material mechanics, and solution strategies. More specifically, we reflect on the Reduced Order modeling techniques, as an emerging trend in the Soft Robotics community, and highlight the need for further studies to explore their full potential. Our goal is to provide a “recipe” that supports researchers, with moderate knowledge of the field and an interest in Reduced Order Modeling techniques, in identifying the most suitable modeling assumptions and solution strategy for their application.
The rest of this article is organized as follows. A brief review of the necessary elements of a soft robot model is discussed in Section 2 . A detailed review of Reduced Order Modeling and Model Order Reduction methods and complementary states space coordination and solution strategies is provided in Section 3 . The main features, advantages, and shortcomings of their different implementations are listed and contrasted in Tables 2 – 5 . The manuscript concludes in Section 4 . Table 1 lists the acronyms in literature, although we mostly use their expanded from in the rest of this manuscript.
Acronyms used in the literature for the simplified kinematic representation of a continuum robot.
Reduced Order Models and Model Order Reduction for continuum and soft robots in literature. SF is Shape (Basis) Function.
Summary of the findings from comparative studies of different modeling methods as presented in ( Sadati et al., 2017a ; Sadati et al., 2018 ; Sadati et al., 2019a ; Sadati et al., 2020a ; Rao et al., 2020 ).
2 Soft robot modeling: assumptions and solution strategies
This section categorizes the key elements of a continuum manipulator model from continuum mechanics theory point of view. This is to better understand how Reduced Order Modeling techniques fit in the soft manipulator modeling framework. The two key elements in theoretical modeling of a soft robot (except for deep learning approaches) are:
- A. system kinematics ,
- B. system mechanics ( governing equations or conservational law ),
- C. material mechanics ( constitutional law ); and
- II. the solution strategy (direct or indirect) for the resultant system of differential equations.
In the rest of this paper, we utilized the term “state space” to refer to the time-variant states of a continuum manipulator model. This is to distinguish between the time-variant states of a Reduced Order model (e.g., modal contribution or fitting function coefficients) with the robot configuration (e.g., strains, position, and orientation) and joint (e.g., joint angles and displacements in a Pseudo Rigid Body model) spaces.
2.1 Continuum robot kinematics
The kinematics of a continuum robot can be represented based on:
- a. Variable Curvature (VC), also called Continuum, Differential, Cosserat Rod, Geometrically Exact Kinematics ( Trivedi et al., 2008 ; Rucker and Webster, 2011 ; Till et al., 2019 ),
- 2. Shape (Basis) function-based, also called Geometrical Kinematics ( Armanini et al., 2023 ), solutions that approximate the bending angle ( Gravagne and Walker, 2000 ; Gravagne and Walker, 2002 ; Gravagne et al., 2003 ; Gravagne and Walker, 2012 ), strain field ( Orekhov and Simaan, 2008 ; Della Santina et al., 2020a ; Della Santina and Rus, 2020 ) or shape ( Godage et al., 2011a ; Godage et al., 2011b ; Godage et al., 2011b ; Godage et al., 2015a ; Sadati et al., 2018 ; Singh et al., 2018 ; Sadati et al., 2020a ; Rao et al., 2022 ) of the entire robot backbone via modal or fitting approaches,
- a. Pseudo Rigid Body (PRB) approach that approximates the robot kinematics as a highly-articulated serial rigid-link mechanism with states defined in relative coordinates, i.e., strains as rotation and translation of rigid-robot joints ( Howell and Midha, 1995 ; Howell et al., 1996 ; Yu et al., 2005 ; Sadati et al., 2017a ; Shiva et al., 2019 ; Sadati et al., 2020a ; Rao et al., 2020 ; Schultz et al., 2022 ),
- b. Piecewise Constant Curvature (PCC) that discretizes the backbone as a series of Constant Curvature infinitesimal segments with states defined in relative coordinates ( Sadati et al., 2020a ; Caasenbrood et al., 1089 ; Rao et al., 2020 ; Shiva et al., 2019 ; Renda et al., 2018 ; Renda, Giorgio-Serchi, Boyer, Laschi, Dias, Seneviratne),
- c. Piecewise Variable Curvature (PVC), with basis function-based (e.g., a polynomial) estimation of curvature value along each segment ( Boyer et al., 2020 ), or
- d. Absolute Nodal Coordinate Formulation (ANCF), based on position and orientation of finite number of nodes along the backbone as the system states defined in absolute coordinates ( Shabana, 2018 ; Sadati et al., 2020a ; Huang et al., 2021 ; Shabana and Eldeeb, 2021 ).
Constant Curvature (CC) kinematics can considered as a special case of basis function-based fitting for a continuum segment ( Webster and Jones, 2010 ; Mustaza et al., 2019 ; Della Santina et al., 2020b ). In the case of Piecewise Constant Curvature kinematics, the transformations between the segments can be derived based on rotation matrices ( Sadati et al., 2017a ; Shiva et al., 2019 ), quaternions ( Sadati et al., 2020a ), or screw theory ( Renda et al., 2018 ; Renda, Giorgio-Serchi, Boyer, Laschi, Dias, Seneviratne). The underlying transformation can be based on Constant Curvature assumptions ( Sadati et al., 2017a ; Sadati et al., 2017b ; Della Santina et al., 2020b ; Rao et al., 2020 ), discretization of Cosserat Rod (i.e., Variable Curvature) kinematics ( Renda et al., 2018 ; Shiva et al., 2019 ; Sadati et al., 2020a ), or deformation map of an Euler-Bernoulli beam ( Sadati et al., 2020a ). In the case of Absolute Nodal Coordinate Formulation, such transformations can be based on a basis function such as a polynomial ( Huang et al., 2021 ) or deformation map of an Euler-Bernoulli beam ( Sadati et al., 2020a ). Furthermore, fitting solutions are proposed for approximating the kinematics of a soft robot cross section geometry deformation, showing 1%–3% increase in the accuracy of a pneumatic soft appendage known as STIFF-FLOP ( Sadati et al., 2017b ).
Variable Curvature (also referred to as Continuum representation ( Armanini et al., 2023 ) provides a geometrically exact kinematic representation at the cost of a system with infinite number of states not suitable for controller design. Basis function-based methods present a low-dimensional continuous formulation suited for controller design with high modeling accuracy, however, are prone to simulation errors. The Pseudo Rigid Body method enables the usage of rigid-body robotic modeling and control techniques for flexible and continuum robots but is usually applicable for a small system (with small number, less than five, number of discretized segments) and usually suffers from singularity issues. This is due to the exponential growth of the derivations for the distal segments in a series rigid link mechanism. The Piecewise Constant Curvature techniques improve the modeling accuracy of a Pseudo Rigid Body representation by considering the structure deformation as a circular arc with limited applicability to large systems (with large number of discretized segments) and with external or body loadings. The Piecewise Variable Curvature technique provides a better estimate of the beam deformation with a polynomial curvature representation but is still not applicable to large systems due to the complex derivations for distal segments, similar to the case of the Pseudo Rigid Body method. Absolute Nodal Coordinate Formulation is the most accurate method for modeling a large system, via optimal sparse matrix formulation of the system dynamic equations, and for incorporating different material mechanics models and actuation forces ( Shabana, 2018 ), at the cost of a large state space dimension and complex models for internal forces and actuation inputs. Figure 1 presents the different kinematic representations for a continuum manipulator in the Soft Robotics literature.

Different kinematics representations for a continuum manipulator in Soft Robotics literature. Here R and p denote the local frame rotation matrix and position vector, m and I are the mass and inertia, v and u are the local translational (shear and elongation) and rotational (bending and torsion) strains, ϵ and α are the translation and Euler angular rotation of an element in the local frame, s is the unit length along the backbone, C and S are the coefficient and basis function for the basis function-based kinematics, i is a free index, δ and ′ are operators denoting infinitesimal change and partial differentiation along and w.r.t. s .
2.2 System mechanics (governing equations) derivation
The system mechanics can be derived for:
- 1. Euler-Bernoulli Beam Theory (static), i.e., based on Euler-Bernoulli beam formulation ( Wegiriya et al., 2019 ; Yu et al., 2021 ),
- 2. Cosserat rod method (static and dynamic) ( Trivedi et al., 2008 ; Rucker and Webster, 2011 ; Burgner-Kahrs et al., 2015 ; Sadati et al., 2017a ; Sadati et al., 2017b ; Gazzola et al., 2018 ; Till et al., 2019 ; Rao et al., 2020 ),
- 3. Principle of Virtual Work (PVW, static and dynamic) ( Sadati et al., 2017a ; Sadati et al., 2017b ),
- 4. Lagrangian Formulation (dynamic) based on shape function-based kinematics ( Godage et al., 2016 ; Mustaza et al., 2019 ; Della Santina et al., 2020a ; Della Santina and Rus, 2020 ), or discretized kinematics ( Godage et al., 2015b ; Sadati et al., 2020a ; Della Santina et al., 2020b ; Habibi et al., 2020 ; Schultz et al., 2022 ),
- 5. TMT Dynamics (dynamic) ( Sadati et al., 2018 ; Sadati et al., 2019a ; Sadati et al., 2020a ; Sadati et al., 2022 ), which is the vector format for the Principle of Virtual Work that derives the system dynamics using a vector formalism with fewer derivation steps compared to the Lagrangian approach, and
- 6. Recursive Computation Scheme (dynamic) ( Godage et al., 2016 ) (also called Reduced Order Integration scheme ( Caasenbrood et al., 1089 ) or Inverse Dynamic Model ( Boyer et al., 2020 ), for forming the equation of motion in a vector formalism by recursively evaluating the nonlinear equations of motion based on Lagrangian or Cosserat rod dynamics.
Euler-Bernoulli Beam theory provides the simplest mechanics formulation for a continuum robot but is only applicable to small deformation cases. Cosserat rod mechanics provides an exact solution in the form of a boundary value problem, known to be computationally expensive to solve via numerical methods. The Principle of Virtual Work is a powerful method of deriving governing equations to incorporate a robot structural complexity and inhomogeneities but challenging to derive for dynamic cases. Lagrangian dynamics (i.e., derivation of the equations of motion using Lagrangian methods) is the commonly utilized method for dynamic cases but results in complex nonlinear relations that are hard to rearrange in a vector formalism, i.e., linear w.r.t. the acceleration terms, when using a standard symbolic mathematical software, e.g., Matlab. Identifying repetitive structures in the Lagrangian formulation can simplify this problem (35).
TMT and Recursive Computation Scheme are proposed to derive such a form. TMT method, which is named after the formulation of the generalized mass matrix in a dynamic system ( Sadati et al., 2020a ), is an easy-to-interpret analytical derivation for the system dynamic equation of motion via direct evaluation of the terms in a vector format. However, it can be analytically challenging to derive the vector format of the virtual work associated with some of the terms in a continuum system dynamics. It is worth noting that the Principle of Virtual Work, Lagrangian, and TMT Dynamic formulations result in the same set of final derivations, but with different derivation steps. The Recursive Computation Scheme simplifies the vector-format formulation of a complex dynamic model based on the numerical evaluation of the equation of motion terms by selectively setting the inertial, damping, gravitational, and elastic terms to zero. As a result, it is more computationally expensive due to multiple recursive numerical evaluations of the governing equations.
2.3 Material mechanics (constitutional law)
The material mechanics for a continuum robot is usually derived based on:
- 1. Hooke’s law ( Trivedi et al., 2008 ; Rucker and Webster, 2011 ; Godage et al., 2016 ; Sadati et al., 2017a ; Sadati et al., 2017b ; Till et al., 2019 ) (i.e., linear elasticity, infinitesimal strain theory) for elastic materials (e.g., Nitinol),
- a. Neo-Hookean ( Trivedi et al., 2008 ; Sadati et al., 2017a ; Sadati et al., 2017b ; Shiva et al., 2019 ),
- b. Mooney-Rivlin ( Gopesh and Friend, 2020 ),
- c. Gent ( Shiva et al., 2019 ), or
- a. Non-Newtonian fluid via viscosity power law ( Sadati et al., 2018 ; Mustaza et al., 2019 ; Sadati et al., 2020a ) and
- b. Kelvin-Voigt method ( Mustaza et al., 2019 ).
Hooke’s law is the commonly used material model with acceptable accuracy for slender systems with small strains. Finite strain theory is used for cases experiencing large strains but cannot capture the material hysteresis and creep effects. The neo-Hookean method is the standard technique for hyper-elastic materials but with limited accuracy in practical cases. Mooney-Rivlin method offers a more accurate model with a standard identification procedure but is still limited to strains less than 100%. The Gent method is the standard technique for larger strain cases and is commonly used for modeling rubber structures. Modeling the material hysteresis is possible by considering a hyper-viscoelastic model. The viscosity power law is a simple method but is more suitable for fluid mediums. The Kelvin-Voigt method can provide a good estimate of the material behavior with a standard process to identify the material properties. The nonlinear formulation of hyper-elastic and hyper-viscoelastic models makes their implementation challenging in a continuum robot model. The Principle of Virtual Work is the most powerful method of deriving the system constitutional law to incorporate different material mechanics representations in a continuum robot model.
2.4 Model formation and solution
A variety of combinations of the above choices for the system kinematics, mechanics, and material mechanics are possible to model a continuum robot. As a result, a system of Ordinary (ODE, mostly for dynamic models with discretized or reduced order kinematics) or Partial Differential Equations (PDE, mostly for quasi-static models, most forms of FEM, and Cosserat rod dynamics) is formed to be solved numerically considering the system’s initial and/or boundary conditions. An ODE formulation is possible for a model with variable curvature kinematics and Cosserat rod mechanics with general loading if load Boundary Conditions (e.g., moment, shear, and tension) are known ( Xu et al., 2013 ; Sadati et al., 2020b ) or for quasi-static models based on discretized kinematics in the absence of distributed, body, or external loads ( Shiva et al., 2019 ).
The common solution strategies, as for any dynamic system, are:
- a. Temporal (time-domain) discretization & integration of a dynamic model (most common) ( Godage et al., 2015b ; Godage et al., 2016 ; Sadati et al., 2020a ), or
- b. Spatial (spatial-domain, e.g., along the backbone curve) discretization and integration of a dynamic model ( Till, 2019 ; Till et al., 2019 ; Till et al., 2019 ; Till et al., 2020 ) or a static model (special cases as explained above) ( Xu et al., 2013 ; Shiva et al., 2019 ; Sadati et al., 2020b );
- a. Shooting (optimization-based) methods for static or Cosserat rod dynamic models which relies on guessing the solution vector and Jacobian-based numerical optimization techniques ( Godage et al., 2011a ; Rucker and Webster, 2011 ; Sadati et al., 2017a ; Sadati et al., 2017b ; Sadati et al., 2018 ; Aloi and Rucker, 2019 ; Till et al., 2019 ; Sadati et al., 2020a ),
- b. Ritz-Galerkin ( Tunay, 2013 ; Sadati et al., 2018 ) method for basis function-based solutions where the system of equations is weighted (i.e., multiplied) by the basis functions vector to achieve higher estimation accuracy.
Direct methods are numerically more efficient because they do not rely on an initial guess, but they are limited to system models in the form of ODEs. Temporal domain integration is the standard technique used in the community but may suffer from time-stepping issues for systems with highly oscillatory states. Spatial domain integration techniques can better handle a system with highly oscillatory states but are not as developed as the temporal domain integration schemes. Indirect schemes are suitable for geometrically exact models that are usually in the form of a BVP. Shooting methods are the standard methods to solve such systems at the cost of computational performance, resulting in slow simulations. The Ritz-Galerkin method can turn a BVP into an ODE to lower the simulation computational cost at the cost of a more complex derivation, challenges with selecting suitable basis functions, and convergence guarantee studies.
2.5 Data-driven and learning-based models
Although data-driven techniques do not necessarily fit in our presented categories above, a short survey of their advantages and limitations can help better justify the usage of Reduced Order Modeling techniques for soft manipulators. Analytical models for continuum robots present a robust solution in the presence of unknown conditions. However, it is not easy to capture commonly observed phenomena in soft robot real behavior such as fabrication inconsistencies, visco-hyper-elastic material properties, material creep, and hysteresis. Learnt solutions (when trained) are on the other hand real-time, accurate (within the training dataset domain), capable of handling complex structural geometries and nonlinearities, and easy to implement by not requiring significant knowledge of continuum robot theory ( Thuruthel et al., 2017 ; Thuruthel et al., 2018a ; Jolaei et al., 2020 ; Truby et al., 2020 ; Kim et al., 2021 ; Wang et al., 2021 ; Wang et al., 2022 ). However, the validity of a deep learning-based solution without a conservational law is limited to the richness and generality of the dataset used for learning, hence lacking robustness in presence of new conditions, contacts, and external loads which are not tested before (i.e., available in the dataset). Lack of generality and robustness guarantee are the main drawbacks of data-driven methods.
The learning-based solution drawbacks limit the usability of such methods to the case of improving a soft robot modeling accuracy for repetitive tasks but challenging to apply in highly varying and dynamic environments such as medical surgery. Koopman operator techniques ( Bruder et al., 2019 ; Bruder et al., 2021a ; Bruder et al., 2021b ), learning the residual (unmodelled) system dynamics, and a combination of learning-based forward models with analytical controller designs can address some of these issues ( Della Santina et al., 2110 ). Alternatively, training such models based on high-fidelity analytical or FEM-based models may solve the robustness and lack of generality issues of data-driven approaches by providing them with rich enough datasets that are hard to gather with a real robot ( Iyengar et al., 2020 ; Iyengar and Stoyanov, 2021 ). A more detailed review of the topic is presented in ( Della Santina et al., 2110 ; Thuruthel et al., 2018b ). The rest of this paper provides a review of Reduced Order Modeling and Model Order Reduction techniques for modeling soft manipulators.
3 Reduced order modeling and model order reduction
Any effort to approximate the differential evolution of states, i.e., differential kinematics of a Cosserat rod, in terms of a representation with finite number of states, e.g., Pseudo Rigid Body kinematics, is often called Model Order Reduction approach in classic continuum mechanics. Although commonly employed for FEM ( Tunay, 2013 ), Computational Fluid Dynamics ( Naghibi et al., 2019 ), and in control theory ( Moore, 1981 ; Antoulas, 2005 ; Van Dooren et al., 2008 ), the application of lightweight slender rigid manipulators for space explorations, undergoing infinitesimal deformations, has motivated the usage of Model Order Reduction techniques in robotics research since 1980s ( Book, 1990 ). More recently, Model Order Reduction (also referred to as Reduction or, interchangeably, as Reduced Order Modeling) techniques are utilized for Soft Robotics research to capture the complex dynamics of active highly deformable structures. In this section, we follow the naming conventions used by ( Thieffry et al., 2018a ; Sadati et al., 2018 ) in which Reduced Order Modeling refers to the methods that construct a reduced order kinematics for a soft robot, while Model Order Reduction is used when compression techniques are utilized the reduce the state space dimension of a FEM model.
The main features, advantages, and shortcomings of the different implementations of Reduced Order Modeling and Model Order Reduction are presented and compared with each other in Table 2 . A brief review of these methods in Soft Robotics research is presented in this section. Relevant coordinate formulations and solution strategy are discussed at the end of this section and summarized in Tables 3 , ,4, 4 , respectively.
State Space Coordination in continuum and Soft Robot modeling.
Solution strategies for reduced order modeling of soft robots.
3.1 Reduced Order Modeling (ROM) for kinematic formulation
Cosserat rod formulation of continuum robots results in a set of Partial Differential Equations (PDE) that form a Boundary Value Problem (BVP) in continuous domain. Traditionally, the Cosserat rod BVP formulation is turned into a discretized BVP with finite number of states, e.g., via Pseudo Rigid Body or Reduced Order kinematics, to be solved by an optimization-based (e.g., shooting) numerical method. Alternatively, weak-form solutions can be sought for a BVP, a common practice in applied mathematics and FEM analysis. To this end, the separation of variables method is used to decouple the temporal (time-dependent, i.e., states evolution over time) and spatial (space-dependent, i.e., states evolution along the robot backbone arc length) characteristics. As a result, the system of PDEs is replaced by two decoupled Ordinary Differential Equations (ODE), each with respect to either the spatial (arc length) or temporal (time) variables. Hence, a solution can be provided using an ODE solver (either analytical or numerical). The former method results in a kinematic formulation similar to rigid body robots, while the former approach requires fewer modeling states, hence is more computationally efficient. Seeking a solution via forward integration and minimal state space dimension of a Reduced Order Model weak solution are desirable for Soft Robotics controller design ( Della Santina et al., 2110 ). We have categorized the different weak-form solutions to approximate a soft robot kinematics in Table 2 .
A basis function-based (modal and fitting) approach can simply the complexity and high-dimensionality of the state space of a soft robot model via:
- 1. Modal approaches where the system kinematics is approximated by a weighted sum of the dominant deformation modes, or
- 2. fitting where the system kinematics is interpolated based on a basis function with control parameters.
As a result, the time-variant weights (modal contributions) in the modal approach or the control parameters of the fitting approach are the new system modeling states, and the mode shapes or fitting basis function are the spatial (i.e., w.r.t. curve length) basis functions. A basis function-based approach separates the variables of the original PDE system and enables seeking an ODE solution. Similar to the application of this method in flexible-arm robotics ( Book, 1990 ), mode shapes (shape or basis functions) can be found based on:
- a. spectral (e.g., Fourier) ( Chirikjian, 1992 ; Chirikjian and Burdick, 1994 ) or power (e.g., Taylor, i.e., simple polynomials or splines) ( Sadati et al., 2018 ; Della Santina et al., 2020a ; Sadati et al., 2022 ) series expansions for a modal approach, and
- b. configuration fitting via Lagrange, Hermite Bezier, Pythagorean Hodograph (PH), B-Spline, NURBS polynomial) ( Singh et al., 2018 ) for a fitting approach;
- 2. eigenfunctions based on an eigenvalue problem (via Singular Value Decomposition) after linearizing the system;
- 3. experimental-based mode shape (via a modal test) or basis shape function selection based on the actual system tests ( Rao et al., 2021 ; Rao et al., 2022 ).
Although investigated for flexible-arm robots in the 1980s ( Book, 1990 ), cases (2) and (3) are relatively unexplored for soft robots. The case of the Eigenvector solution to a finite element problem is discussed as a part of Model Order Reduction techniques in the following sections ( Book, 1990 ).
Modal approach results in a truncated series solution (with a finite number of terms that are adequate to achieve the desired simulation accuracy) with a finite number of terms (time-variant coefficient and space-variant basis functions). The fitting approach does not necessarily result in a series solution format as it employs a predefined basis function. In both cases, the solution for the system kinematics should satisfy the system initial (i.e., initial stationary state) and boundary (e.g., right angle at the robot base) conditions. As a result, the approximate solution complies with the overall system kinematics (e.g., configuration ( Sadati et al., 2020a ) or curvature ( Della Santina and Rus, 2020 ) along a continuum arm backbone), and overall system velocity field. The basis function-based kinematics approximation improves the simulation numerical performance and results in a system of acceptable dimensions for controller design. This way, rather than attacking the general modeling problem directly, a low-dimensional approximate model is introduced and studied in detail ( Della Santina, 2020 ).
Reduced Order Modeling in the form of assumed continuous modes is a common approach in computer graphics for reducing the rendering computational cost of large scenes where modeling accuracy is not a priority ( Barbic et al., 2009 ; Badías et al., 2018 ). In the robotic community, a similar approach has been taken since the 1980s for modeling and control of flexible-arm ( Book and Majette, 1983 ; Tsujisawa and Book, 1989 ; Book, 1990 ; Moberg, 2010 ; De Luca and Book, 2016 ) and hyper-redundant under-actuated ( Chirikjian, 1992 ; Chirikjian, 1993 ; Chirikjian and Burdick, 1994 ; Chirikjian, 1995 ; Chirikjian and Burdick, 1995 ; Chirikjian, 1997 ) robots. All the reported instances of Reduced Order Modeling in this survey have utilized an elastic material mechanics based on Hooke’s law. In the rest of this section, we summarized the main different Reduced Order formulations presented for a soft robot kinematics.
3.1.1 Modal approach
Modal approach is equivalent to interpolating a precomputed basis (the dominant deformations) to model the run-time pose of an object ( Xu and Barbič, 2016 ). In a FEM-based framework, the complex deformation of a continuum object can be reduced to a weighted summation over a finite-dimensional subset of all possible deformations of an object, which is referred to as the dominant deformation subset ( Wang et al., 2015 ) or assumed modes. Such a method achieves good computational performance but at the cost of accuracy, which can be improved by carefully selecting the dominant deformation subset and increasing the number of terms in the summation series. Unlike mode shapes that are stand-alone analytical solutions from the modal analysis of a system, assumed modes (basis or shape functions), despite satisfying the system BCs, may not represent a modal solution for the system and only provide a basis for approximating the system solution, to be verified numerically. For example, although assuming a polynomial solution for the deformation of a continuum manipulator can provide adequate simulation accuracy and computational efficiency, it is not a solution for the modal analysis of the same system. A modal representation of a soft robot kinematics simplifies the infinite-state-space-dimension of the system variable curvature kinematics and transform it to a finite dimensional representation, i.e., a fitting problem with a few weighting coefficients as the system states, and hence results in less theoretical complexity.
The modal approach is a common method for dynamic modeling of flexible-link robots and structures ( Book, 1990 ; Sayahkarajy, 2018 ). For example, Wu et al. have investigated nonlinear model order reduction based on the system modal derivatives ( Wu and Tiso, 2016 ) limited to simple geometries. Alternatively, Weeger et al. utilized pre-defined shapes in the form of 4D NURBS curves to express the configuration and quaternion orientation of a Cosserat rod centerline in large deformation ( Weeger et al., 2017 ). Liu et al. utilized a polynomial collocation spectral method (in which the series index is the basis function wave numbers, i.e., frequency in the spatial domain) for a Kirchhoff’s rod discretized on a set of Chebyshev or Legendre Gauss-Lobatto control points ( Liu et al., 2018 ). They showed the spectral method precision exponentially increases as the number of the nodes increases.
Modal approaches have been preliminarily investigated for Soft Robots in the form of run-time fitting techniques based on a precomputed basis (i.e., assumed deformation modes) as presented by Wang et al. ( Wang et al., 2015 ) and Xu et al. ( Xu and Barbič, 2016 ). Della Santina et al. (1806) utilized a robot with compliant joints stabilized via sub-manifolds on the system state space (i.e., system nonlinear continuation of linear eigenspaces, called nonlinear normal modes) on which the system would naturally evolve for motion generation. Alternatively, one could bypass the constitutional and conservational laws (system and material mechanics) by matching the basis functions from the experimental observations as the ground truth as presented by Chirikjian et al. for modeling volume preserving 3D deformations of soft simple geometries ( Chirikjian, 1995 ). A similar method has been presented for characterization of worm locomotion kinematics ( Digumarti et al., 2019 ). SOFA (Software Open Framework Architecture), as a computationally efficient simulation tool for evaluating random deformations of soft objects, can serve as an ideal platform in such method to identify an object’s dominant deformation basis.
3.1.2 Polynomial shape (PS) fitting for configuration space characterization
Amongst the early works on Reduced Order Modeling in Soft Robotics, the shape fitting concept was utilized by Godage et al. since 2011 as a singularity free representation of a continuum manipulator kinematics in which the backbone Cartesian configuration is expressed in terms of a power series with basis functions based on a manipulator actuation chambers length ( Godage et al., 2011a ; Godage et al., 2016 ). However, their representation could not capture the backbone twist and the choice of a hard-to-interpret basis function resulted in a series solution with many coefficients to identify (e.g., 165 ( Godage et al., 2011a ) and a complicated system mechanics derivations ( Godage et al., 2011a ; Godage et al., 2011b ; Godage et al., 2016 ). Sadati et al. showed the advantageous numerical performance and accuracy of using a simpler alternative based on parametrization of the configuration space, i.e., the robot backbone shape ( Sadati et al., 2018 ). A truncated Lagrange polynomial passing through a number of equally spaced control points was used to describe a continuum manipulator shape (configuration or kinematics) with the Cartesian position of the control points as the system modeling states. This method was referred to as Polynomial Shape fitting for soft robot kinematics.
Although it could successfully handle hyper-elastic deformation of the robot cross section, considerations for braided pneumatic actuators, and general loading cases for planar motions, the use of the Frenet-Serret tangent frame formulation invalidates the 3D solutions in the case of a twisted backbone due to external loading. The issue lies with a non-physical twist predicted based on the mathematical definition of a Frenet–Serret curvilinear frame. The same issue presents itself in the work by Singh et al. ( Singh, 2018 ; Singh et al., 2018 ) and utilized by Wiese et al. ( Wiese et al., 2019 ). Higher modeling and control accuracy could perhaps be achieved by considering this effect, noting that neglecting to do so may result in severe errors in the case of out-of-plane bending. Furthermore, the convergence of a Lagrange polynomial series solution (i.e., solution accuracy increase by the addition of control points) is not guaranteed with a set of equally-spaced points ( Sadati et al., 2018 ). This is due to Runge’s phenomenon (oscillation problem of the fitted curve at the ends of an interval) that can be addressed by using Chebyshev or Legendre Guass-Lobatto techniques ( Liu et al., 2018 ).
To address these issues, a general 3D method is presented in ( Sadati et al., 2019a ; Sadati et al., 2020a ) in which a 7D series solution (i.e., with seven independent series, three for the cartesian coordinates vector and four for the orientation quaternion vector) represents a continuum rod backbone’s 3D Cartesian position and quaternion orientation. This approach is similar to that of (98) but employs simple polynomials instead of NURBS curves. Instead of using Cartesian locations as system states as in (12), the new method uses the polynomial coefficients themselves, thereby improving convergence otherwise affected by Runge’s phenomenon. This also simplifies the derivation complexity caused by the nonlinear form of Lagrange polynomials.
However, in the 7D solution, the conformity of the local frame to the robot backbone tangent vector is governed by the system conservational law (i.e., compliance potential field). These constraints may not perfectly hold for a coarse spatial integration step. The exact formulation of the deformed frame twist (or local curvilinear frame in general) is needed for modeling instability-induced rapid snapping motions that are observed in some continuum robots such as Concentric Tube Robots ( Mitros et al., 2021 ). This is addressed based on a Bishop’s frame formulation for the tangent frame in (55) by introducing a correction twist on an arbitrary tangent frame to the robot backbone. As a result, the system kinematics can be described by a 4D series solution (three for the cartesian coordinates and one for the correction twist) at the cost of slightly more complex derivation of the governing equations. A spline may be used instead of a polynomial when certain degree of continuity is required along the robot overall backbone, e.g., due to the change in the robot structure as in a Concentric Tube Robot. The transition between the spline segments can be continuously implemented based on logistic functions. This representation is used to present the first dynamic model of an everting growing (vine) robot in 3D motion ( Berthet-Rayne et al., 2021 ). The dynamic modeling and controller design of hybrid rigid-soft robots based on the Polynomial Shape kinematic are implemented in an open-source Matlab software package, called “TMTDyn” ( Sadati et al., 2020a ).
Although being the most common choice, polynomials aren’t the only options to describe the shape of a soft robot. Singh et al. compared Hermite, Bezier, Pythagorean Hodograph (PH), BSplines, NURBS curves for this purpose and showcased, in simulation and experiments, the advantages of a PH curve representation for the controller design of a continuum arm ( Singh, 2018 ; Singh et al., 2018 ). This representation is later adopted for potential field-based obstacle avoidance of continuum arm on a mobile platform while navigating an unconstructed environment ( Mbakop et al., 2021 ).
3.1.3 Polynomial curvature (PC) fitting for curvilinear space characterization
Amongst the early works, shape approximation techniques were more common to model flexible arm robots ( Book, 1990 ). On the other hand, polynomial approximation of the strain (curvature and torsion) along a continuum backbone (Polynomial Curvature kinematics) was investigated to model planar motion of a hyper-redundant robot and later a DNA strand by Chirikjian et al. ( Chirikjian, 1992 ; Chirikjian, 1993 ; Chirikjian and Burdick, 1994 ; Chirikjian and Burdick, 1995 ; Chirikjian, 1997 ) who referred to it as “Modal Approach.” It is introduced to constrain a hyper redundant arm curvature to a representation with a few DOFs based on trigonometric (sine and cosine) functions as the assumed mode shapes for the curvature values, and time-variant coefficients as the modal participation factors.
In soft robotics research, Polynomial Curvature kinematics and Lagrange dynamics of a planar Kirchhoff rod (i.e., infinite shear) were studied by Della Santina et al. (who, for the first time, referred to it as Polynomial Curvature kinematics) highlighting their advantages and challenges in controller design ( Della Santina et al., 2110 ; Della Santina and Rus, 2020 ; Della Santina et al., 2020a ; Della Santina, 2020 ). A 1D series solution was used for the curvature of a planar beam backbone to overcome the robot vibration and reduced performance associated with a Constant Curvature-based controller. A similar method with Cosserat rod mechanics was studied for quasi-static 3D modeling of continuum rod in (27) focusing on the compatibility of the curvilinear constraints such as those seen in a Concentric Tube Robots ( Sears and Dupont, 2006 ; Webster et al., 2006 ; Mitros et al., 2021 ). More recently, 3D implementation of the Polynomial Curvature kinematics is showcased and verified in standard simulation scenarios by Boyer et al. ( Boyer et al., 2020 ), who referred to their presented kinematics as “Piecewise Variable Curvature”. There, the problem of deriving the complex governing equations for the system dynamics is addressed based on a recursive computational scheme which they referred to as “Inverse Dynamic Model.” Later, this method is showcased for modeling closed-chain (parallel) soft robots ( Armanini et al., 2021 ) and made available in an open-source Matlab software package, called “SoRoSim” ( Teejo Mathew et al., 2021 ).
Alternatively, the right basis function candidate can be identified based on the fitting performance of different shape functions to capture the shape of a real soft robot. As an instant of Polynomial Curvature method, Rao et al. have demonstrated that an Euler curve (a curve whose curvature varies linearly along the arc length) is a viable candidate to capture the planar deformation of a continuum appendage under external tip load ( Rao et al., 2021 ; Rao et al., 2022 ). Similarly, modal test results on the actual soft robots can be used to construct the basis functions for the robot’s reduced order kinematics, as practiced for flexible arm robotics in 1980s ( Book, 1990 ).
The curvilinear space, i.e., strains (shear, elongation, curvature, and torsion) requires one fewer state compared to the rectilinear space (i.e., absolute Cartesian position and orientation) to describe continuum backbone kinematics, since the curvilinear space is derived through spatial differentiation from the rectilinear parameters. However, deriving the rectilinear parameters is still required for calculating the local effect of external loading and the system inertial dynamics. This requires spatial integration of a set of non-integrable trigonometric functions. These integrations are handled via analytical approximation (e.g., Taylor series expansion ( Della Santina, 2020 ; Della Santina and Rus, 2020 )) of the integrands ( Della Santina et al., 2020a ; Della Santina, 2020 ; Della Santina and Rus, 2020 ) or numerical integration methods ( Orekhov and Simaan, 2008 ). The former method is complex and inaccurate for large deformations (outside the Taylor series convergence radius) but necessary for integration in Lagrangian dynamics. The latter method is only compatible with optimization-based solutions (e.g., shooting method). More accurate solutions for these integrals are proposed based on the formulation of the closed-form integrals with 0th-order Bessel functions for the 2D case ( Chirikjian, 1992 ) or, more generally, the use of integrable basis functions such as helical functions ( Grazioso et al., 2019 ). The Polynomial Shap approach overcomes these limitations (i.e., integration complexity, inaccuracy, and lack of generality) by relying on a differentiation step to obtain the curvilinear space parameters (required to calculate the strain actions) instead of the integration step required by the polynomial Curvature method to obtain the rectilinear space parameters.
However, unlike the Polynomial Shape method, the definition of curvilinear frame is not problematic in the case of Polynomial Curvature method due to the direct parametrization of curvature and torsion. The only exception is the method presented by Grazioso et al. ( Grazioso et al., 2019 ) in which the deformation map derivation relies on a curve in the Frenet-Serret frame that is constructed by analytical integration of helical basis functions for the curvilinear (curvature) space. There. similar to the works by Singh et al. ( Singh, 2018 ; Singh et al., 2018 ), the problem with an deformed local frame twist isn’t addressed.
Most of the recent Polynomial Curvature related studies are limited to theoretical investigations ( Orekhov and Simaan, 2008 ; Grazioso et al., 2019 ; Della Santina et al., 2020a ; Della Santina, 2020 ; Della Santina and Rus, 2020 ). Future experimental studies on soft robot hardware and a comparison with Polynomial Shape methods can help to achieve a fair comparison and conclusion on the most appropriate method for a given Soft Robotics application.
3.2 Model Order Reduction (MOR) for finite element models
In this paper, we used the Reduced Order Modeling term for the techniques that provide an approximate solution (usually in a basis function-based from) for the system kinematics. The system kinematics and material mechanics are then fed into the system mechanics to derive the system’s equation of motions. Alternatively, we employed the term Model Order Reduction (MOR) to refer to the techniques that reduce the state space dimension of an already developed model (usually based on FEM) for a soft system. Although Reduced Order Modeling and Model Order Reduction are employed interchangeably in some literature, the reason for the use of different terms in this paper is that Model Order Reduction, unlike Reduced Order Models for a robot kinematics, is not a fundamental block (i.e., the system kinematics, system mechanics, and material mechanics) of a continuum robot model. Model Order Reduction is also referred to as System Compression.
The Model Order Reduction utilizes Principal Orthogonal Decomposition or Principal Component Analysis techniques which are widely used in computational mechanics. There, Singular Value Decomposition is performed on the state variables of several sample deformations of a soft robot (minimum 2 within each actuation range). The results are truncated up to a tolerance to define the system reduced basis and to neglect insignificant system states. The insignificant states are hard to reach (require large excitation energy) or hard to observe (produce small observable energy) ( Antoulas, 2005 ). Such basis need not necessarily have any physical meaning. Measures are considered to achieve desired tolerance while preserving the system properties such as stability, passivity, and contractility. The compressed (reduced) system can be used for nonlinear controller and observer design with stability guarantee ( Chenevier et al., 2018 ).
Principle Orthogonal Decomposition, although being the only technique suitable for a nonlinear system ( Thieffry et al., 2018a ), is computationally expensive and therefore not real-time. To achieve real-time performance, Thriftey et al. ( Thieffry et al., 2018a ; Thieffry et al., 2018b ; Thieffry et al., 2019 ; Thieffry et al., 2020 ) performed the same analysis on a linearized FEM model around an equilibrium point, and utilized their reduced system to formulate a stable observer and controller. As a result, their reduced model was 3–100 times faster in simulations than the original FEM model. Alternatively, Goury et al. (Goury, Duriez) computed a reduced integration domain (and weights associated with it) by computing the reduced basis on the sample deformation space and simultaneously storing the elements contributions based on an assumed tolerance. The Galerkin method was then used to solve the resulted system. Elastic material mechanics based on co-rotational linear model ( Chenevier et al., 2018 ) and hype-elastic material mechanics based on neo-Hookean (Goury, Duriez; Chenevier et al., 2018 ) and Mooney-Rivlin (Goury, Duriez) models have been utilized in different Model Order Reduction implementations in the literature.
Model Order Reduction method is implemented in the “Soft Robotics Toolkit,” an open-source simulation package based on SOFA-framework. This implementation benefits from the real-time performance, open-source (hence expandable), accurate, and configurable numerical methods of SOFA and is experimentally verified for nonlinear controller design of soft robots of general shape (Goury, Duriez; Thieffry et al., 2018a ; Bieze et al., 2018 ; Thieffry et al., 2018b ; Thieffry et al., 2019 ; Thieffry et al., 2020 ; Katzschmann et al., 2019 ). However, the underlying FEM model results in high computational cost, lack of theoretical clarity (i.e., non-interpretability of the modes and complex nonlinear formulation), and complexity in implementing new assumptions (e.g., constraint) and elements (e.g., braided pneumatic actuators). Furthermore, the current implementation of SOFA-framework cannot handle Coriolis terms in dynamics of rigid bodies and hence poses difficulty in modeling hybrid rigid-continuum body structures. The plugin enables implementing a variety of material laws that are already available in SOFA.
3.3 State space coordination and dimensionality
A continuum system can be modeled based on states defined in relative (i.e., the continuum manipulator local tangent frame) and absolute (i.e., ground fixed reference/inertial frame) coordinates, which are called “relative states” and “absolute states,” respectively, in the rest of this paper. Relative states are usually referred to local strain or free parameters of a fitting method that describes a single segment w.r.t. the previous segment (local frame) in a series chain (e.g., in the case of Constant Curvature case). An absolute state refers to the robot shape itself (e.g., node position and quaternion orientation), participation coefficients of the robot shape modal representation, or free parameters of an fitting method that describes a robot segment w.r.t. the global frame (e.g., Hermite, Bezier, PH, or NURBS fitting of a robot backbone shape). Recently, a hybrid-state model is presented that combines the absolute (e.g., position) and relative (e.g., local correction twist angle to represent a Bishop’s frame) states (e.g., in the case of Concentric Tube Robots) ( Sadati et al., 2022 ).
Except for FEM research, relative state representations are dominant for modeling continuum robots due to simplicity in dealing with system elastic energy, internal forces, and actuation inputs. Modal representations with relative states require lower order basis functions too, e.g., a polynomial curvatures representation relies on 2 nd -order polynomials while polynomial shape representations require a 3 rd -order polynomial to capture the backbone basic motion and satisfy the boundary conditions (e.g., backbone right angle at the robot base). However, implementation of external force fields, contacts, and constraints are challenging. Furthermore, the system dynamic derivation involves an integration stage to account for the inertial effects that is usually dealt with based on approximate numerical solutions that may reduce the overall accuracy and increase the numerical cost of the system simulation. The complexity of the equations of motion increases as the number of elements, states, and segments in a system increases, making them unsuitable for large-scale systems. The similarity of such representations with the models for a rigid-link serial mechanism makes it easier to utilize well-developed techniques from rigid-body robotics. As a result, relative states are favorable for control system design in continuum robotics research.
On the other hand, absolute states result in an easier derivation of inertial terms, external forces, contact, and constraint (e.g., for parallel mechanisms). The system dynamic derivation involves a differentiation stage that can be accurately handled analytically, and the equation of motion derivation complexity does not significantly increase as the number of elements in the system increases, making it suitable for large-scale systems. As a result, absolute states are favored for FEM modeling in the Soft Robotics community. Furthermore, considering the robot shape in task space (configuration in global frame), as the system absolute states, makes such methods more suitable for trajectory tracking, shape and/or tip motion control.
Absolute representation of position and orientation does not kinematically constrain the local frame to the backbone curve tangent and this should be enforced by the governing equations, i.e., due to balance of shear and bending virtual energies. A hybrid state space method is recently proposed that benefits from absolute states for the robot position while defines a relative correction twist angle to define a local Bishop’s frame based on an arbitrary tangent frame to the resultant curve. As a result, for the first time, a seamless framework is derived that can handle instability instances in the dynamics of a continuum system, in this case a Concentric Tube Robot ( Sadati et al., 2022 ). Such a hybrid presentation results in a more complex derivation of the Coriolis terms due to the need for derivation of the backbone curve tangent frame. However, it benefits from a smaller state space since the orientation is defined based on a single correction twist angle instead of three states for Euler angles or for states for a quaternion representation.
In the case of geometrically exact modeling, variable Curvature kinematics result in an infinite-dimensional states space based on strains (relative states) along the robot backbone. Alternatively, FEM and Absolute Nodal Coordinate Formulations, which result in a large-dimensional state space based on only position (FEA ( Coevoet et al., 2017 ) or both position and orientation (Absolute Nodal Coordinate Formulations ( Shabana, 2018 ; Huang et al., 2021 ; Shabana and Eldeeb, 2021 ) of a finite number of nodes along the robot backbone, are instances of cases with absolute states. On the reduced order modeling front, Constant Curvature assumptions, modal, and fitting-based representation of a continuum backbone strain domain deal with relative states. On the other hand, modal, and fitting-base approximation of the robot shape present a reduced order representation of a system with absolute states. Recent formulation of a Concentric Tube Robot kinematics based on polynomial shape approximation in reference coordinates for the backbone position and Bishop’s frame representation for orientation in relative coordinates is an example of hybrid state formulation. The main features, advantages, and shortcomings of different state space coordination employed in continuum and Soft Robotics research are summarized in Table 3 .
3.4 Solution strategies
The resulted approximate solution for the system kinematic or mechanics based on reduced order modeling or model order reduction is called a weak solution in continuum mechanics and applied mathematics. Such solution is then substituted in the system governing equations. Afterwards, the Ritz or Ritz-Galerkin method can be used to solve the resulting system ( Hesthaven et al., 2007 ).
Substituting an approximate (e.g., basis function-based) solution for the system deformation map. i.e., kinematics, in the system governing equations is called the Ritz approach to solve a system of complex PDEs. Although it is not referenced in most Soft Robotics research works, most of the proposed models for soft robots based on a basis function based approximation utilize the Ritz method. Later, the spatial domain of the resulted decoupled system can be numerically integrated while the time-varying coefficients are optimized to minimize the governing equation residual error.
Alternatively, the coefficients can be considered as the system’s EOM states for which the time series can be found from the numerical integration in time ( Sadati et al., 2018 ). The Galerkin method of weighted residuals, known as Ritz-Galerkin method, may provide a better approximation where the weighted residual of the system is minimized instead of the residual function itself ( Tunay, 2013 ). In simple words, both sides of the governing equations resulted from the Ritz method is multiplied by a vector, called weighting vector, which usually consists of the shape (basis) functions. Tunay et al. ( Tunay, 2013 ), Sadati et al. ( Sadati et al., 2018 ), and Goury et al. (Goury, Duriez) have investigated Ritz and Ritz-Galerkin solutions for continuum robots in the soft robotics community. Table 4 summarizes the main features of these solution strategies.
3.5 Controller design implications
Standard control architectures (e.g., Jacobian-based inverse dynamics ( Coevoet et al., 2017 ; Goury, Duriez; Thieffry et al., 2018a ; Thieffry et al., 2018b ; Katzschmann et al., 2019 ; Thieffry et al., 2019 ; Thieffry et al., 2020 ), feedback linearization ( Della Santina et al., 2020a ; Sadati et al., 2020a ; Della Santina and Rus, 2020 ), potential field planning ( Mbakop et al., 2021 ) for finite-state space systems, such as rigid body robots, have been integrated with Reduced Order models of soft manipulators (see Table 2 ). From the formulation perspective, the use of absolute states with simpler mapping between the control system states and the robot workspace (e.g., shape position and orientation) may simplify the controller design for tracking and planning scenarios in the robot task space. On the other hand, the use of relative states, with a simpler mapping between the system states and the actuation inputs, may simplify the configuration space controller design. This is useful for tasks involving vibration attenuation or under-actuated system control design. A detailed discussion on model-based control of soft robots is presented in (9). A detailed comparative study of different control strategies for Reduced Order Model of soft manipulators is needed to better highlight the performance of different combinations of the Reduced Order modeling and control technique.
3.6 Comparative studies and software packages
To reach a fair comparison between the different methods in the literature, it is important to minimize the effect of experimental hardware and procedures on the results between different studies. To this end, comparative studies of different methods based on experimental results with a unique setup can be helpful. We have conducted a series of such comparative studies based on experiments with a soft appendage with pneumatic braided actuators, known as STIFF-FLOP (STIFFness controllable Flexible and Learn-able Manipulator for surgical OPerations) in ( Sadati et al., 2017a ; Sadati et al., 2018 ; Sadati et al., 2019a ; Sadati et al., 2020a ). A more recent study by Rao et al. (2020) compared the accuracy of different modeling techniques (Constant Curvature, Pseudo Rigid Body, Piecewise Constant Curvature, and Variable Curvature) for a tendon-driven continuum robot. To help with choosing the right elements for modeling a soft manipulator, Table 5 provides a summary of findings based on these comparative studies.
There has been an increasing interest in the application and contribution of reduced order basis function-based methods handling singularity issues ( Godage et al., 2011a ; Godage et al., 2011b ; Godage et al., 2015a ; Godage et al., 2016 ), general loading cases ( Sadati et al., 2018 ; Sadati et al., 2019a ; Sadati et al., 2020a ; Rao et al., 2021 ; Rao et al., 2022 ; Sadati et al., 2022 ), kinematic constraints ( Orekhov and Simaan, 2008 ), force observation ( Aloi and Rucker, 2019 ; Aloi et al., 2022 ), controller design ( Singh et al., 2018 ; Della Santina et al., 2020a ; Della Santina, 2020 ; Della Santina and Rus, 2020 ), and path planning ( Mbakop et al., 2021 ) emphasizing the ever-increasing importance of Reduced Order Modeling methods in the Soft Robotics community.
Tailored software packages are developed mostly for FEM-based ( Naughton et al., 2009 ; Coevoet et al., 2017 ; Gazzola et al., 2018 ; Sadati et al., 2019b ; Grazioso et al., 2019 ; Hu et al., 2019 ; Mathew, Hmida, Renda; Huang et al., 2020 ; Li et al., 2020 ; Munawar et al., 2020 ; Bern and Rus, 2021 ; Graule et al., 2021 ) implementations targeting fast simulation of complex scenarios for realistic graphical representations and learning-based research. The exceptions are TMTDyn (based on the TMT method) ( Sadati et al., 2019b ; Sadati et al., 2020a ) and SoRoSim (based on a Recursive Computational Scheme) (Mathew, Hmida, Renda), which are Matlab packages for the theoretical derivation of a continuum robot Lagrangian dynamics suited for theoretical dynamical system analysis and nonlinear controller design. Although, similar functionalities have been or can be achieved by further developments around FEM-based packages such as SOFA ( Coevoet et al., 2017 ), PyElastica ( Naughton et al., 2009 ), and DiffPD ( Ma et al., 2021 ). Ease of use, integration with existing tools, and suitability to research objectives alongside excellent accuracy, robustness, and real-time computational performance are key to ensuring wide acceptability.
4 Conclusion and future directions
Reduced Order Modeling and Model Order Reduction techniques have been extensively investigated to obtain models of acceptable dimension for Soft Robotics control. In this paper, we reviewed and compared the techniques identified in the literature, summarizing our findings in Tables 2 , Table 3 , Table 4 , Table 5 . Although, Reduced Order Modeling and Model Order Reduction techniques are promising paths towards Soft Robotics control, they require further theoretical development, experimental validation, and comparative study.
The quest for finding a better shape fitting technique for soft manipulators is still ongoing with recent studies on Euler curves and Magnus expansion technique. Modeling complex soft manipulators with parallel structure, braided, growing, and concentric elements are the most recent trends in relevant modeling research. On the control research front, application of shape fitting techniques for shape control, path planning, and obstacle avoidance have been among the most recent studies.
Amongst the Reduced Order Modeling techniques, the Polynomial Shape method has been primarily investigated for modeling complex continuum systems, such as Concentric Tube and Eversion Growing Robots. On the other hand, the Polynomial Curvature method has been mostly studied for the control problem of simple continuum manipulators. Both the Polynomial Shape and Polynomial Curvature methods for soft manipulators are now supported by open-source software, dynamical implementation of general deformation and loading cases, experimental studies, and controller designs, but require further theoretical developments. Such developments need to focus on integrating nonlinear material mechanics laws, simulation stability analysis for modeling hyper-elastic material models, and further experimental investigations. On the other hand, Model Order Reduction implementations for soft robots, which relies on a preliminary FEM model, will benefit from more collaborative work, dissemination, and support for implementation by experimentalists.
Immediate next research steps are simulation numerical stability analysis for high bandwidth and unstable rapid motions of soft manipulators, high-bandwidth motion and vibration attenuation control, force and stiffness regulation, external force and impact observation, multi-arm coordination, robot planning for self-collision and obstacle avoidance, and path following based on techniques such as follow-the-leader. The Polynomial Shape and Curvature methods are yet to be explored for parallel structure and path planning problems respectively. Finally, the potential of run-time fitting techniques based on modal test results on the actual system, or a precomputed basis (normal nonlinear modes) are untapped.
In the long run, it is interesting to investigate the Reduced Order Modeling techniques for the emerging research directions in the Soft Robotics such as multi-physics simulations (e.g., soft swimming or flying robots), machine learning and data-driven techniques, and task automation. Finally, developing software toolkits and experimental comparative studies are essential to broaden the reach and impact of the Reduced order Modeling techniques and to help identify each method’s potential from an application-oriented and control perspective.
Acknowledgments
The authors would like to thank Ian D. Walker from Clemson University, United States, Cosimo Della Santina from Delft University, Netherland, and Gregory Chirikjian from National University of Singapore for helpful discussions and feedback on the manuscript.
Funding Statement
This research was supported by an ERC Starting Grant [714562], by core funding from the Wellcome/EPSRC Centre for Medical Engineering [WT203148/Z/16/Z]. The authors acknowledge support from the Department of Health and Social Care (DHSC) through the National Institute for Health and Care Research (NIHR) MedTech Co-operative award for Cardiovascular Diseases to Guy’s and St Thomas NHS Foundation Trust in partnership with King’s College London. For the purpose of Open Access, the Author has applied a CC BY public copyright license to any Author Accepted Manuscript version arising from this submission.
Author contributions
SS have authored the manuscript. SN has contributed in the discussions lead to effective categorization of the theoretical techniques presented in this review article. LC and CB supervised the efforts lead to the preparation of this draft and contributed to the discussions in improving the quality of the review article. All authors contributed to the article and approved the submitted version.
Conflict of interest
The authors declare that the research was conducted in the absence of any commercial or financial relationships that could be construed as a potential conflict of interest.
Publisher’s note
All claims expressed in this article are solely those of the authors and do not necessarily represent those of their affiliated organizations, or those of the publisher, the editors and the reviewers. Any product that may be evaluated in this article, or claim that may be made by its manufacturer, is not guaranteed or endorsed by the publisher.
- Albu-Schaeffer A., Della Santina C. (2020). A review on nonlinear modes in conservative mechanical systems . Available from: http://www.sciencedirect.com/science/article/pii/S1367578820300687 .
- Aloi V., Dang K. T., Barth E. J., Rucker C. (2022). Estimating forces along continuum robots . IEEE Robot. Autom. Lett. 1–8 , 8877–8884. 10.1109/lra.2022.3188905 [ CrossRef ] [ Google Scholar ]
- Aloi V., Rucker C. (2019). “ Estimating loads along elastic rods ,” in Proceedings of the IEEE International Conference on Robotics and Automation (ICRA), Montreal, Canada, May 2019 (IEEE; ), 7. [ Google Scholar ]
- Antoulas A. C. (2005). Approximation of large-scale dynamical systems . Philadelphia: SIAM, 490. [ Google Scholar ]
- Armanini C., Boyer F., Mathew A., Duriez C., Renda F. (2023). Soft robots modeling: A structured overview . IEEE Trans. Robot. 39 ( 3 ), 1728–1748. 10.1109/tro.2022.3231360 [ CrossRef ] [ Google Scholar ]
- Armanini C., Hussain I., Iqbal M. Z., Gan D., Prattichizzo D., Renda F. (2021). Discrete Cosserat approach for closed-chain soft robots: Application to the fin-ray finger . IEEE Trans. Robot. 1–10 , 2083–2098. 10.1109/tro.2021.3075643 [ CrossRef ] [ Google Scholar ]
- Badías A., Alfaro I., González D., Chinesta F., Cueto E. (2018). Reduced order modeling for physically-based augmented reality . Comput. Methods Appl. Mech. Eng. 341 , 53–70. 10.1016/j.cma.2018.06.011 [ CrossRef ] [ Google Scholar ]
- Barbic J., da Silva M., Popović J. (2009). Deformable object animation using reduced optimal control . ACM Trans. Graph 28 ( 3 ), 1–9. 10.1145/1531326.1531359 [ CrossRef ] [ Google Scholar ]
- Bern J. M., Rus D. (2021). “ Soft Ik with stiffness control ,” in Proceedings of the 2021 IEEE 4th International Conference on Soft Robotics (RoboSoft), New Haven, CT, USA, April 2021, 465–471. [ Google Scholar ]
- Berthet-Rayne P., Sadati S. M. H., Patel N., Giannarou S., Leff D. R., Bergeles C. (2021). MAMMOBOT: A miniature steerable soft growing robot for early breast cancer detection . IEEE Robot. Autom. Lett. , 8. 10.1109/LRA.2021.3068676 [ CrossRef ] [ Google Scholar ]
- Bieze T. M., Largilliere F., Kruszewski A., Zhang Z., Merzouki R., Duriez C. (2018). Finite element method-based kinematics and closed-loop control of soft, continuum manipulators . Soft Robot. 5 ( 3 ). 10.1089/soro.2017.0079 [ PubMed ] [ CrossRef ] [ Google Scholar ]
- Blanc L., Delchambre A., Lambert P. (2017). Flexible medical devices: Review of controllable stiffness solutions . Actuators 6 ( 3 ), 23. 10.3390/act6030023 [ CrossRef ] [ Google Scholar ]
- Book W. J., Majette M. (1983). Controller design for flexible, distributed parameter mechanical arms via combined state space and frequency domain techniques . J. Dyn. Syst. Meas. Control 105 ( 4 ), 245–254. 10.1115/1.3140666 [ CrossRef ] [ Google Scholar ]
- Book W. J. (1990). Modeling, design, and control of flexible manipulator arms: A tutorial review . 29th IEEE Conf. Decis. Control 2 , 500–506. [ Google Scholar ]
- Boyer F., Lebastard V., Candelier F., Renda F. (2020). Dynamics of continuum and soft robots: A strain parametrization based approach . IEEE Trans. Robot. , 19. 10.1109/TRO.2020.3036618 [ CrossRef ] [ Google Scholar ]
- Bruder D., Fu X., Gillespie R. B., Remy C. D., Vasudevan R. (2021). Data-driven control of soft robots using koopman operator theory . IEEE Trans. Robot. 37 ( 3 ), 948–961. 10.1109/tro.2020.3038693 [ CrossRef ] [ Google Scholar ]
- Bruder D., Fu X., Gillespie R. B., Remy C. D., Vasudevan R. (2021). Koopman-based control of a soft continuum manipulator under variable loading conditions . IEEE Robot. Autom. Lett. 6 ( 4 ), 6852–6859. 10.1109/lra.2021.3095268 [ CrossRef ] [ Google Scholar ]
- Bruder D., Remy C. D., Vasudevan R. (2019). “ Nonlinear system identification of soft robot dynamics using koopman operator theory ,” in Proceedings of the 2019 International Conference on Robotics and Automation (ICRA), Montreal, QC, Canada, May 2019, 6244–6250. [ Google Scholar ]
- Burgner-Kahrs J., Rucker D. C., Choset H. (2015). Continuum robots for medical applications: A survey . IEEE Trans. Robot. 31 ( 6 ), 1261–1280. 10.1109/tro.2015.2489500 [ CrossRef ] [ Google Scholar ]
- Caasenbrood B., Pogromsky A., Nijmeijer H., Control-oriented models for hyperelastic soft robots through differential geometry of curves . Soft Robot. , 10.1089/soro.2021.0035 [ PubMed ] [ CrossRef ]
- Chenevier J., González D., Aguado J. V., Chinesta F., Cueto E. (2018). Reduced-order modeling of soft robots . PLOS ONE 13 ( 2 ), e0192052. 10.1371/journal.pone.0192052 [ PMC free article ] [ PubMed ] [ CrossRef ] [ Google Scholar ]
- Chirikjian G. S. (1993). “ A continuum approach to hyper-redundant manipulator dynamics ,” in Proceedings of the IEEE/RSJ International Conference on Intelligent Robots and Systems (IROS), Yokohama, Japan, July 1993, 1059–1066. 2 [ Google Scholar ]
- Chirikjian G. S., Burdick J. W. (1994). A modal approach to hyper-redundant manipulator kinematics . IEEE Trans. Robot. Autom. 10 ( 3 ), 343–354. 10.1109/70.294209 [ CrossRef ] [ Google Scholar ]
- Chirikjian G. S., Burdick J. W. (1995). The kinematics of hyper-redundant robot locomotion . IEEE Trans. Robot. Autom. 11 ( 6 ), 781–793. 10.1109/70.478426 [ CrossRef ] [ Google Scholar ]
- Chirikjian G. S. (1995). Closed-form primitives for generating locally volume preserving deformations . J. Mech. Des. 117 ( 3 ), 347–354. 10.1115/1.2826687 [ CrossRef ] [ Google Scholar ]
- Chirikjian G. S. (1997). Inverse kinematics of binary manipulators using a continuum model . J. Intell. Robot. Syst. , 19 , 5–22. 10.1023/A:1007942530293 [ CrossRef ] [ Google Scholar ]
- Chirikjian G. S. (1992). Theory and applications of hyper-redundant robotic manipulators . Pasadena, California: California Institute of Technology. [ Google Scholar ]
- Cianchetti M., Ranzani T., Gerboni G., De Falco I., Laschi C., Menciassi A. (2013). “ STIFF-FLOP surgical manipulator: Mechanical design and experimental characterization of the single module ,” in Proceedings of the IEEE International Conference on Intelligent Robots and Systems (IROS), Tokyo, Japan, November 2013 (IEEE; ), 3576–3581. [ Google Scholar ]
- Coevoet E., Morales-Bieze T., Largilliere F., Zhang Z., Thieffry M., Sanz-Lopez M., et al. (2017). Software toolkit for modeling, simulation, and control of soft robots . Adv. Robot. 31 ( 22 ), 1208–1224. 10.1080/01691864.2017.1395362 [ CrossRef ] [ Google Scholar ]
- De Luca A., Book W. J. (2016). “ Robots with flexible elements ,” in Springer handbook of robotics (Germany: Springer; ), 243–282. [ Google Scholar ]
- Della Santina C., Bicchi A., Rus D. (2020). On an improved state parametrization for soft robots with piecewise constant curvature and its use in model based control . IEEE Robot. Autom. Lett. 1–1 , 1001–1008. 10.1109/lra.2020.2967269 [ CrossRef ] [ Google Scholar ]
- Della Santina C., Duriez C., Rus D. Model based control of soft robots: A survey of the state of the art and open challenges . Available from: http://arxiv.org/abs/2110.01358 .
- Della Santina C., Katzschmann R. K., Bicchi A., Rus D. (2020). Model-based dynamic feedback control of a planar soft robot: Trajectory tracking and interaction with the environment . Int. J. Robot. Res. 39 , 490–513. 10.1177/0278364919897292 [ CrossRef ] [ Google Scholar ]
- Della Santina C., Lakatos D., Bicchi A., Albu-Schäffer A. Using nonlinear normal modes for execution of efficient cyclic motions in soft robots . Available from: http://arxiv.org/abs/1806.08389 .
- Della Santina C., Rus D. (2020). Control oriented modeling of soft robots: The polynomial curvature case . IEEE Robot. Autom. Lett. 5 ( 2 ), 290–298. 10.1109/lra.2019.2955936 [ CrossRef ] [ Google Scholar ]
- Della Santina C. (2020). “ The soft inverted pendulum with affine curvature ,” in Proceedings of the 2020 59th IEEE Conference on Decision and Control (CDC), Jeju, Korea (South), December 2020, 8. [ Google Scholar ]
- Digumarti K. M., Trimmer B., Conn A. T., Rossiter J. (2019). Quantifying dynamic shapes in soft morphologies . Soft Robot. 6 , 733–744. 10.1089/soro.2018.0105 [ PubMed ] [ CrossRef ] [ Google Scholar ]
- Gazzola M., Dudte L. H., McCormick A. G., Mahadevan L. (2018). Forward and inverse problems in the mechanics of soft filaments . R. Soc. Open Sci. 5 ( 6 ), 171628. 10.1098/rsos.171628 [ PMC free article ] [ PubMed ] [ CrossRef ] [ Google Scholar ]
- Gilbert H. B., 2020, On the mathematical modeling of slender biomedical continuum robots . Front. Robot. AI ; 8 :732643, 10.3389/frobt.2021.732643 [ PMC free article ] [ PubMed ] [ CrossRef ] [ Google Scholar ]
- Gopesh T., Friend J. (2020). Facile analytical extraction of the hyperelastic constants for the two-parameter mooney–rivlin model from experiments on soft polymers . Soft Robot. 8 ( 4 ). 10.1089/soro.2019.0123 [ PMC free article ] [ PubMed ] [ CrossRef ] [ Google Scholar ]
- Godage I. S., Branson D. T., Guglielmino E., Medrano-Cerda G. A., Caldwell D. G. (2011). “ Shape function-based kinematics and dynamics for variable length continuum robotic arms ,” in Proceedings of the 2011 IEEE International Conference on Robotics and Automation (ICRA), Shanghai, China, May 2011 (IEEE; ), 452–457. [ Google Scholar ]
- Godage I. S., Guglielmino E., Branson D. T., Medrano-Cerda G. A., Caldwell D. G. (2011). “ Novel modal approach for kinematics of multisection continuum arms ,” in Proceedings of the IEEE International Conference on Intelligent Robots and Systems (IROS), San Francisco, California, USA, September 2011 (IEEE; ), 1093–1098. [ Google Scholar ]
- Godage I. S., Medrano-Cerda G. A., Branson D. T., Guglielmino E., Caldwell D. G. (2016). Dynamics for variable length multisection continuum arms . Int. J. Robot. Res. 35 ( 6 ), 695–722. 10.1177/0278364915596450 [ CrossRef ] [ Google Scholar ]
- Godage I. S., Medrano-Cerda G. A., Branson D. T., Guglielmino E., Caldwell D. G. (2015). Modal kinematics for multisection continuum arms . Bioinspiration Ampmathsemicolon Biomim. 10 ( 3 ), 035002. 10.1088/1748-3190/10/3/035002 [ PubMed ] [ CrossRef ] [ Google Scholar ]
- Godage I. S., Wirz R., Walker I. D., Webster R. J. (2015). Accurate and efficient dynamics for variable-length continuum arms: A center of gravity approach . Soft Robot. 2 ( 3 ), 96–106. 10.1089/soro.2015.0006 [ CrossRef ] [ Google Scholar ]
- Goury O., Duriez C. (2018). Fast, generic, and reliable control and simulation of soft robots using model order reduction . IEEE Trans. Robot. 34 ( 6 ), 1565–1576. 10.1109/tro.2018.2861900 [ CrossRef ] [ Google Scholar ]
- Graule M. A., Teeple C. B., McCarthy T. P., Kim G. R., Louis St.R. C., Wood R. J. (2021). “ SoMo: Fast and accurate simulations of continuum robots in complex environments ,” in Proceedings of the 2021 IEEE/RSJ International Conference on Intelligent Robots and Systems (IROS), Prague, Czech Republic, May 2021, 3934–3941. [ Google Scholar ]
- Gravagne I., Walker I. D. (2012). Kinematics for constrained continuum robots using wavelet decomposition . Clemson, SC: Clemson University, 292–298. [ Google Scholar ]
- Gravagne I. A., Rahn C. D., Walker I. D. (2003). Large deflection dynamics and control for planar continuum robots . IEEEASME Trans. Mechatron. 8 ( 2 ), 299–307. 10.1109/tmech.2003.812829 [ CrossRef ] [ Google Scholar ]
- Gravagne I. A., Walker I. D. (2000). “ Kinematic transformations for remotely-actuated planar continuum robots ,” in Proceedings of the 2000 ICRA Millennium Conference IEEE International Conference on Robotics and Automation Symposia Proceedings (Cat No00CH37065), San Francisco, CA, USA, April 2000, 19–26. 1 [ Google Scholar ]
- Gravagne I. A., Walker I. D. (2002). Manipulability, force, and compliance analysis for planar continuum manipulators . IEEE Trans. Robot. Autom. 18 ( 3 ), 263–273. 10.1109/tra.2002.1019457 [ PubMed ] [ CrossRef ] [ Google Scholar ]
- Grazioso S., Di Gironimo G., Siciliano B. (2019). A geometrically exact model for soft continuum robots: The finite element deformation space formulation . Soft Robot. 6 ( 6 ), 790–811. 10.1089/soro.2018.0047 [ PubMed ] [ CrossRef ] [ Google Scholar ]
- Habibi H., Yang C., Godage I. S., Kang R., Walker I. D., Branson D. T. (2020). A lumped-mass model for large deformation continuum surfaces actuated by continuum robotic arms . J. Mech. Robot. 12 ( 1 ), 011014. 10.1115/1.4045037 [ CrossRef ] [ Google Scholar ]
- Hesthaven J. S., Gottlieb S., Gottlieb D. (2007). Spectral methods for time-dependent problems , 21 . Cambridge: Cambridge University Press. [ Google Scholar ]
- Howell L. L., Midha A., Norton T. W. (1996). Evaluation of equivalent spring stiffness for use in a pseudo-rigid-body model of large-deflection compliant mechanisms . J. Mech. Des. 118 ( 1 ), 126–131. 10.1115/1.2826843 [ CrossRef ] [ Google Scholar ]
- Howell L. L., Midha A. (1995). Parametric deflection approximations for end-loaded, large-deflection beams in compliant mechanisms . J. Mech. Des. 117 ( 1 ), 156–165. 10.1115/1.2826101 [ CrossRef ] [ Google Scholar ]
- Hu Y., Liu J., Spielberg A., Tenenbaum J. B., Freeman W. T., Wu J., et al. (2019). “ ChainQueen: A real-time differentiable physical simulator for soft robotics ,” in Proceedings of the 2019 International Conference on Robotics and Automation (ICRA), Montreal, Canada, May 2019, 6265–6271. [ Google Scholar ]
- Huang W., Huang X., Majidi C., Jawed M. K. (2020). Dynamic simulation of articulated soft robots . Nat. Commun. 11 ( 1 ), 2233. 10.1038/s41467-020-15651-9 [ PMC free article ] [ PubMed ] [ CrossRef ] [ Google Scholar ]
- Huang X., Zou J., Gu G. (2021). Kinematic modeling and control of variable curvature soft continuum robots . IEEEASME Trans. Mechatron. 26 , 3175–3185. 10.1109/tmech.2021.3055339 [ CrossRef ] [ Google Scholar ]
- Iyengar K., Dwyer G., Stoyanov D. (2020). Investigating exploration for deep reinforcement learning of concentric tube robot control . Int. J. Comput. Assist. Radiol. Surg. 15 ( 3 ). 10.1007/s11548-020-02194-z [ PMC free article ] [ PubMed ] [ CrossRef ] [ Google Scholar ]
- Iyengar K., Stoyanov D. (2021). “ Deep reinforcement learning for concentric tube robot control with a goal-based curriculum ,” in Proceedings of the IEEE International Conference on Robotics and Automation (ICRA), Xi'an, China, May 2021, 7. [ Google Scholar ]
- Jolaei M., Hooshiar A., Dargahi J., Packirisamy M. (2020). Toward task autonomy in robotic cardiac ablation: Learning-based kinematic control of soft tendon-driven catheters . Soft Robot. 8 ( 3 ). 10.1089/soro.2020.0006 [ PubMed ] [ CrossRef ] [ Google Scholar ]
- Katzschmann R. K., Thieffry M., Goury O., Kruszewski A., Guerra T. M., Duriez C., et al. (2019). “ Dynamically closed-loop controlled soft robotic arm using a reduced order finite element model with state observer ,” in Proceedings of the IEEE 2019 International Conference on Soft Robotics, Séoul, South Korea, April 2019. [ Google Scholar ]
- Kim D., Kim S. H., Kim T., Kang B., Lee M., Park W., et al. (2021). Review of machine learning methods in soft robotics . PLOS ONE 16 , e0246102. 10.1371/journal.pone.0246102 [ PMC free article ] [ PubMed ] [ CrossRef ] [ Google Scholar ]
- Li M., Ferguson Z., Schneider T., Langlois T., Zorin D., Panozzo D., et al. (2020). Incremental potential contact: Intersection-and inversion-free, large-deformation dynamics . ACM Trans. Graph 39 ( 4 ). 10.1145/3386569.3392425 [ CrossRef ] [ Google Scholar ]
- Liu Y., Wang M., Meng L. (2018). Spectral collocation method in the large deformation analysis of flexible beam . Int. J. Appl. Math. 48 ( 4 ), 8. [ Google Scholar ]
- Ma P., Du T., Zhang J. Z., Wu K., Spielberg A., Katzschmann R. K., et al. (2021). DiffAqua: A differentiable computational design pipeline for soft underwater swimmers with shape interpolation . ACM Trans. Graph 40 ( 4 ), 1–14. 10.1145/3476576.3476704 [ CrossRef ] [ Google Scholar ]
- Mathew A. T., Hmida I. B., Renda F. (2022). SoRoSim: A MATLAB toolbox for soft robotics based on the geometric variable-strain approach . [ Google Scholar ]
- Mbakop S., Tagne G., Drakunov S., Merzouki R. (2021). Parametric PH curves-model based kinematic control of the shape of mobile soft-manipulators in unstructured environment . IEEE Trans. Ind. Electron 69 ( 10 ), 1. 10.1109/TIE.2021.3123635: [ CrossRef ] [ Google Scholar ]
- Mitros Z., Henry R., Sadati S. M. H., Cruz L. D., Bergeles C. (2021). From theoretical work to clinical translation: Progress in concentric tube robots . Annu. Rev. Control 5 , 335–359. 10.1146/annurev-control-042920-014147 [ CrossRef ] [ Google Scholar ]
- Moberg S. (2010). Modeling and control of flexible manipulators . Available from: http://urn.kb.se/resolve?urn=urn:nbn:se:liu:diva-60831 .
- Moore B. (1981). Principal component analysis in linear systems: Controllability, observability, and model reduction . IEEE Trans. Autom. Control 26 ( 1 ), 17–32. 10.1109/tac.1981.1102568 [ CrossRef ] [ Google Scholar ]
- Munawar A., Srishankar N., Fischer G. S. (2020). “ An open-source framework for rapid development of interactive soft-body simulations for real-time training ,” in Proceedings of the IEEE International Conference on Robotics and Automation (ICRA), Paris, France, May 2020 (IEEE; ), 7. [ Google Scholar ]
- Mustaza S. M., Elsayed Y., Lekakou C., Saaj C., Fras J. (2019). Dynamic modeling of fiber-reinforced soft manipulator: A visco-hyperelastic material-based continuum mechanics approach . Soft Robot. 6 ( 3 ). 10.1089/soro.2018.0032 [ PubMed ] [ CrossRef ] [ Google Scholar ]
- Naghibi S. E., Karabasov S. A., Jalali M. A., Sadati S. M. H. (2019). Fast spectral solutions of the double-gyre problem in a turbulent flow regime . Appl. Math. Model 66 , 745–767. 10.1016/j.apm.2018.09.026 [ CrossRef ] [ Google Scholar ]
- Naughton N., Sun J., Tekinalp A., Chowdhary G., Gazzola M. Elastica: A compliant mechanics environment for soft robotic control . Available from: http://arxiv.org/abs/2009.08422 .
- Orekhov A. L., Simaan N. Solving Cosserat rod models via collocation and the Magnus expansion . Available from: http://arxiv.org/abs/2008.01054 .
- Rao P., Peyron Q., Burgner-Kahrs J. (2022). Shape representation and modeling of tendon-driven continuum robots using euler arc splines . IEEE Robot. Autom. Lett. 7 ( 3 ), 8114–8121. 10.1109/lra.2022.3185377 [ CrossRef ] [ Google Scholar ]
- Rao P., Peyron Q., Burgner-Kahrs J. (2021). “ Using euler curves to model continuum robots ,” in Proceedings of the 2021 IEEE International Conference on Robotics and Automation (ICRA), Xi'an, China, May 2021, 1402–1408. [ Google Scholar ]
- Rao P., Peyron Q., Lilge S., Burgner-Kahrs J. How to model tendon-driven continuum robots and benchmark modelling performance . Front. Robot. AI 7 . 10.3389/frobt.2020.630245/full [ PMC free article ] [ PubMed ] [ CrossRef ] [ Google Scholar ]
- Renda F., Boyer F., Dias J., Seneviratne L. (2018). Discrete Cosserat approach for multisection soft manipulator dynamics . IEEE Trans. Robot. 34 , 1518–1533. 10.1109/tro.2018.2868815 [ CrossRef ] [ Google Scholar ]
- Renda F., Giorgio-Serchi F., Boyer F., Laschi C., Dias J., Seneviratne L. (2018). A unified multi-soft-body dynamic model for underwater soft robots . Int. J. Robot. Res. 37 ( 6 ), 648–666. 10.1177/0278364918769992 [ CrossRef ] [ Google Scholar ]
- Rucker D. C., Webster R. J. (2011). Statics and dynamics of continuum robots with general tendon routing and external loading . IEEE Trans. Robot. 27 ( 6 ), 1033–1044. 10.1109/tro.2011.2160469 [ CrossRef ] [ Google Scholar ]
- Rus D., Tolley M. T. (2015). Design, fabrication and control of soft robots . Nature 521 ( 7553 ), 467–475. 10.1038/nature14543 [ PubMed ] [ CrossRef ] [ Google Scholar ]
- Sadati S., Shiva A., Renson L., Rucker C., Althoefer K., Nanayakkara T., et al. (2019). “ Reduced order vs. Discretized lumped system models with absolute and relative states for continuum manipulators ,” in Robotics: Science and systems (Germany: Freiburg; ), 10. [ Google Scholar ]
- Sadati S. M. H., Mitros Z., Henry R., Zeng L., Cruz L. da, Bergeles C. (2022). Real-time dynamics of concentric tube robots with reduced-order kinematics based on shape interpolation . IEEE Robot. Autom. Lett. 7 ( 2 ), 5671–5678. 10.1109/lra.2022.3151399 [ CrossRef ] [ Google Scholar ]
- Sadati S. M. H., Naghibi S. E., Shiva A., Michael B., Renson L., Howard M., et al. (2020). TMTDyn: A Matlab package for modeling and control of hybrid rigid–continuum robots based on discretized lumped systems and reduced-order models . Int. J. Robot. Res. 40 ( 1 ), 296–347. 10.1177/0278364919881685 [ CrossRef ] [ Google Scholar ]
- Sadati S. M. H., Naghibi S. E., Shiva A., Noh Y., Gupta A., Walker I. D., et al. (2017). A geometry deformation model for braided continuum manipulators . Front. Robot. AI 4 . 10.3389/frobt.2017.00022 [ CrossRef ] [ Google Scholar ]
- Sadati S. M. H., Naghibi S. E., Shiva A., Walker I. D., Althoefer K., Nanayakkara T. (2017). “ Mechanics of continuum manipulators, a comparative study of five methods with experiments ,” in Towards autonomous robotic systems [internet] (Surrey, UK: Springer International Publishing; ), 686–702. 10.1007/978-3-319-64107-2_56 [ CrossRef ] [ Google Scholar ]
- Sadati S. M. H., Naghibi S. E., Walker I. D., Althoefer K., Nanayakkara T. (2018). Control space reduction and real-time accurate modeling of continuum manipulators using Ritz and ritz–galerkin methods . IEEE Robot. Autom. Lett. 3 ( 1 ), 328–335. 10.1109/lra.2017.2743100 [ CrossRef ] [ Google Scholar ]
- Sadati S. M. H., Shiva A., Herzig N., Rucker C. D., Hauser H., Walker I. D., et al. (2020). Stiffness imaging with a continuum appendage: Real-time shape and tip force estimation from base load readings . IEEE Robot. Autom. Lett. 5 ( 2 ), 2824–2831. 10.1109/lra.2020.2972790 [ CrossRef ] [ Google Scholar ]
- Sadati S. M. H., Zschaler S., Bergeles C. (2019). “ A matlab-internal DSL for modelling hybrid rigid-continuum robots with TMTDyn ,” in Proceedings of the 2019 ACM/IEEE 22nd International Conference on Model Driven Engineering Languages and Systems Companion (MODELS-C), Munich, Germany, September 2019, 559–567. [ Google Scholar ]
- Sayahkarajy M. (2018). Mode shape analysis, modal linearization, and control of an elastic two-link manipulator based on the normal modes . Appl. Math. Model 59 . 10.1016/j.apm.2018.02.003 [ CrossRef ] [ Google Scholar ]
- Schultz J. A., Sanders H., Bui P. D. H., Layer B., Killpack M. (2022). “ Modeling the dynamics of soft robots by discs and threads ,” in Proceedings of the 2022 International Conference on Robotics and Automation (ICRA), Philadelphia, PA, USA, May 2022, 3223–3229. [ Google Scholar ]
- Sears P., Dupont P. (2006). “ A steerable needle Technology using curved concentric tubes ,” in Proceedings of the 2006 IEEE/RSJ International Conference on Intelligent Robots and Systems, Beijing, China, October 2006, 2850–2856. [ Google Scholar ]
- Shabana A. A. (2018). Continuum-based geometry/analysis approach for flexible and soft robotic systems . Soft Robot. 5 ( 5 ). 10.1089/soro.2018.0007 [ PubMed ] [ CrossRef ] [ Google Scholar ]
- Shabana A. A., Eldeeb A. E. (2021). Motion and shape control of soft robots and materials . Nonlinear Dyn. 104 ( 1 ), 165–189. 10.1007/s11071-021-06272-y [ CrossRef ] [ Google Scholar ]
- Shiva A., Sadati S. M. H., Noh Y., Fraś J., Ataka A., Würdemann H., et al. (2019). Elasticity versus hyperelasticity considerations in quasistatic modeling of a soft finger-like robotic appendage for real-time position and force estimation . Soft Robot. 6 ( 2 ), 228–249. 10.1089/soro.2018.0060 [ PubMed ] [ CrossRef ] [ Google Scholar ]
- Singh I., Amara Y., Melingui A., Mani Pathak P., Merzouki R. (2018). Modeling of continuum manipulators using pythagorean Hodograph curves . Soft Robot. 5 ( 4 ), 425–442. 10.1089/soro.2017.0111 [ PubMed ] [ CrossRef ] [ Google Scholar ]
- Singh I. (2018). Curve based approach for shape reconstruction of continuum manipulators . Lille, France: Universite de Lille. [ Google Scholar ]
- Teejo Mathew A., Ben Hmida I., Armanini C., Boyer F., Renda F. SoRoSim: A MATLAB toolbox for soft robotics based on the geometric . Available from: https://ui.adsabs.harvard.edu/abs/2021arXiv210705494T .
- Thieffry M., Kruszewski A., Duriez C., Guerra T. marie. (2018). Control design for soft robots based on reduced order model . IEEE Robot. Autom. Lett. 1–1 , 1. 10.1109/lra.2018.2876734 [ CrossRef ] [ Google Scholar ]
- Thieffry M., Kruszewski A., Guerra T. M., Duriez C. (2020). “ LPV framework for non-linear dynamic control of soft robots using finite element model ,” in 21st IFAC world congress (Berlin, France. Available from: https://hal.archives-ouvertes.fr/hal-02567575 . [ Google Scholar ]
- Thieffry M., Kruszewski A., Guerra T. M., Duriez C. (2018). “ Reduced order control of soft robots with guaranteed stability ,” in Proceedings of the 2018 European Control Conference (ECC), Limassol, Cyprus, March 2018 (IEEE; ), 635–640. [ Google Scholar ]
- Thieffry M., Kruszewski A., Guerra T.-M., Duriez C. (2019). Trajectory tracking control design for large-scale linear dynamical systems with applications to soft robotics . IEEE Trans. Control Syst. Technol. 29 ( 2 ), 556–566. 10.1109/TCST.2019.2953624 [ CrossRef ] [ Google Scholar ]
- Thuruthel T. G., Ansari Y., Falotico E., Laschi C. (2018). Control strategies for soft robotic manipulators: A survey . Soft Robot. 5 ( 2 ), 149–163. 10.1089/soro.2017.0007 [ PubMed ] [ CrossRef ] [ Google Scholar ]
- Thuruthel T. G., Falotico E., Renda F., Laschi C. (2017). Learning dynamic models for open loop predictive control of soft robotic manipulators . Bioinspir Biomim. 12 ( 6 ), 066003. 10.1088/1748-3190/aa839f [ PubMed ] [ CrossRef ] [ Google Scholar ]
- Thuruthel T. G., Falotico E., Renda F., Laschi C. (2018). Model-based reinforcement learning for closed-loop dynamic control of soft robotic manipulators . IEEE Trans. Robot. 35 ( 1 ), 124–134. 10.1109/TRO.2018.2878318 [ CrossRef ] [ Google Scholar ]
- Till J., Aloi V., Riojas K E., Anderson P. L., Webster R. J., Rucker C. (2020). A dynamic model for concentric tube robots . IEEE Trans. Robot. 36 ( 6 ), 1–15. 10.1109/TRO.2020.3000290 [ PMC free article ] [ PubMed ] [ CrossRef ] [ Google Scholar ]
- Till J., Aloi V., Rucker C. (2019). Real-time dynamics of soft and continuum robots based on Cosserat rod models . Int. J. Robot. Res. 38 ( 6 ), 723–746. 10.1177/0278364919842269 [ CrossRef ] [ Google Scholar ]
- Till J. D. (2019). On the statics, dynamics, and stability of continuum robots: Model formulations and efficient computational schemes . Knoxville, TN, US]: University of Tennessee. [ Google Scholar ]
- Trivedi D., Lotfi A., Rahn C. D. (2008). Geometrically exact models for soft robotic manipulators . IEEE Trans. Robot. 24 ( 4 ), 773–780. 10.1109/tro.2008.924923 [ CrossRef ] [ Google Scholar ]
- Truby R. L., Santina C. D., Rus D. (2020). Distributed proprioception of 3D configuration in soft, sensorized robots via deep learning . IEEE Robot. Autom. Lett. 5 ( 2 ), 3299–3306. 10.1109/lra.2020.2976320 [ CrossRef ] [ Google Scholar ]
- Tsujisawa T., Book W. J. (1989). “ A reduced order model derivation for lightweight arms with a parallel mechanism ,” in Proceedings of the 1989 International Conference on Robotics and Automation, Scottsdale, AZ, USA, May 1989. [ Google Scholar ]
- Tunay I. (2013). Spatial continuum models of rods undergoing large deformation and inflation . IEEE Trans. Robot. 29 ( 2 ), 297–307. 10.1109/tro.2012.2232532 [ CrossRef ] [ Google Scholar ]
- Van Dooren P., Gallivan K. A., Absil P. A. (2008). H2-optimal model reduction of MIMO systems . Appl. Math. Lett. 21 ( 12 ), 1267–1273. 10.1016/j.aml.2007.09.015 [ CrossRef ] [ Google Scholar ]
- Wang H., Thuruthel T. G., Gilday K., Abdulali A., Iida F. (2022). “ Machine learning for soft robot sensing and control: A tutorial study ,” in Proceedings of the 2022 IEEE 5th International Conference on Industrial Cyber-Physical Systems (ICPS), Coventry, UK, May 2022, 01–06. [ Google Scholar ]
- Wang X., Li Y., Kwok K. W. (2021). A survey for machine learning-based control of continuum robots . Front. Robot. AI 8 , 730330. 10.3389/frobt.2021.730330 [ PMC free article ] [ PubMed ] [ CrossRef ] [ Google Scholar ]
- Wang Y., Jacobson A., Barbi J., Kavan L. ( 2015). Linear subspace design for real-time shape deformation . ACM Trans. Graph 34 ( 4 ), 57:1-57:11. 10.1145/2766952 [ CrossRef ] [ Google Scholar ]
- Webster R. J., Jones B. A. (2010). Design and kinematic modeling of constant curvature continuum robots: A review . Int. J. Robot. Res. 29 ( 13 ), 1661–1683. 10.1177/0278364910368147 [ CrossRef ] [ Google Scholar ]
- Webster R. J., Okamura A. M., Cowan N. J. (2006). “ Toward active cannulas: Miniature snake-like surgical robots ,” in Proceedings of the 2006 IEEE/RSJ International Conference on Intelligent Robots and Systems, Beijing, China, October 2006, 2857–2863. [ Google Scholar ]
- Weeger O., Yeung S. K., Dunn M. L. (2017). Isogeometric collocation methods for Cosserat rods and rod structures . Comput. Methods Appl. Mech. Eng. 316 , 100–122. 10.1016/j.cma.2016.05.009 [ CrossRef ] [ Google Scholar ]
- Wegiriya H., Herzig N., Abad S. A., Sadati S. M. H., Nanayakkara T. (2019). A stiffness controllable multimodal whisker sensor follicle for texture comparison . IEEE Sens. J. 10 . 10.1109/JSEN.2019.2951755 [ CrossRef ] [ Google Scholar ]
- Wiese M., Rüstmann K., Raatzl A. (2019). “ Kinematic modeling of a soft pneumatic actuator using cubic hermite splines ,” in Proceedings of the 2019 IEEE/RSJ International Conference on Intelligent Robots and Systems (IROS), Macau, China, November 2019, 7176–7182. [ Google Scholar ]
- Wu L., Tiso P. (2016). Nonlinear model order reduction for flexible multibody dynamics: A modal derivatives approach . Multibody Syst. Dyn. 36 ( 4 ), 405–425. 10.1007/s11044-015-9476-5 [ CrossRef ] [ Google Scholar ]
- Xu H., Barbič J. (2016). Pose-space subspace dynamics . ACM Trans. Graph 35 ( 4 ), 1–14. 10.1145/2897824.2925916 [ CrossRef ] [ Google Scholar ]
- Xu R., Asadian A., Naidu A. S., Patel R. V. (2013). “ Position control of concentric-tube continuum robots using a modified Jacobian-based approach ,” in Proceedings of the 2013 IEEE International Conference on Robotics and Automation, Karlsruhe, Germany, May 2013, 5813–5818. [ Google Scholar ]
- Yu Y. Q., Howell L. L., Lusk C., Yue Y., He M. G. (2005). Dynamic modeling of compliant mechanisms based on the pseudo-rigid-body model . J. Mech. Des. 127 ( 4 ), 760–765. 10.1115/1.1900750 [ CrossRef ] [ Google Scholar ]
- Yu Z., Sadati S. M. H., Wegiriya H., Childs P., Nanayakkara T. (2021). A method to use nonlinear dynamics in a whisker sensor for terrain identification by mobile robots , in Proceedings of the IEEE International Conference on Intelligent Robots and Systems (IROS), Prague, Czech Republic, August 2021. [ Google Scholar ]
Academia.edu no longer supports Internet Explorer.
To browse Academia.edu and the wider internet faster and more securely, please take a few seconds to upgrade your browser .
Enter the email address you signed up with and we'll email you a reset link.
- We're Hiring!
- Help Center
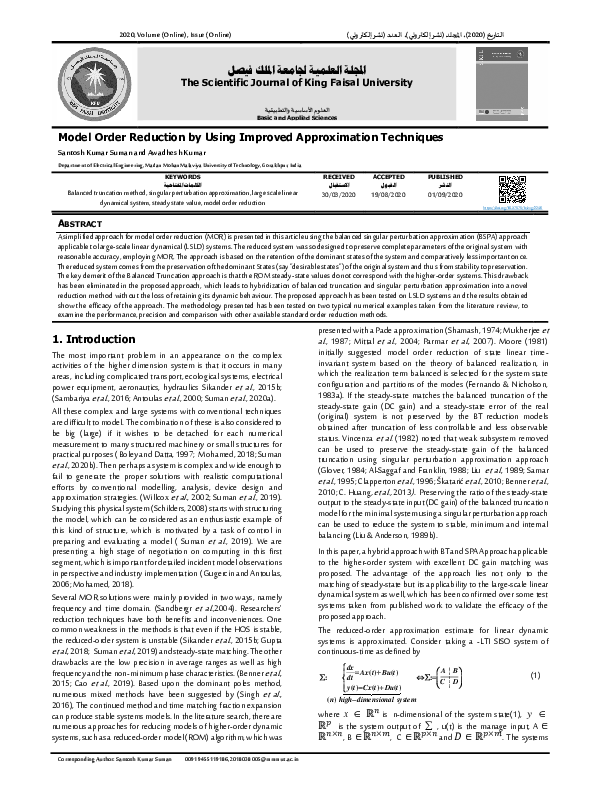
Model Order Reduction by Using Improved Approximation Techniques

Basic and Applied Sciences - Scientific Journal of King Faisal University
Related Papers
International Journal of Mathematical, Engineering and Management Sciences
Santosh Kumar Suman
A simplified approach for model order reduction (MOR) idea is planned for better understanding and explanation of large- scale linear dynamical (LSLD) system. Such approaches are designed to well understand the description of the LSLD system based upon the Balanced Singular Perturbation Approximation (BSPA) approach. BSPA is tested for minimum / non-minimal and continuous/discrete-time systems valid for linear time-invariant (LTI) systems. The reduced-order model (ROM) is designed to preserved complete parameters with reasonable accuracy employing MOR. The Proposed approach is based upon retaining the dominant modes (may desirable states) of the system and eliminating comparatively the less significant eigenvalues. As the ROM has been derived from retaining the dominant modes of the large- scale linear dynamical stable system, which preserves stability. The strong aspect of the balanced truncation (BT) method is that the steady-state values of the ROM do not match with the original ...
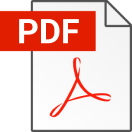
Sanchit Gupta , Shivam Singhal
In this paper, to find a lower order model equivalent to a given higher order system, a simple method based on T.C Hsia's approximation method is proposed. To examine the accuracy of the proposed method, it is compared with the Hutton and Fried-land's approach which uses α and β parameters. To demonstrate the correctness and efficacy of the proposed method, a fourth order system is reduced to first and second order using both the methods. The various performance indices of the reduced systems, obtained from the graphs plotted in Matlab platform are compared and analysed.
Circuits, Systems, and Signal Processing
Arvind kumar Prajapati
WSEAS TRANSACTIONS on SYSTEMS archive
We present an efficient implementation of the balance truncation approximation method for largescale dynamical system, which a benchmark collection Inclusive of some needful real-world examples. In this paper we proposed a new procedure the reduction method based balance truncation is explored for getting structure preserving reduced order model of a large-scale dynamical system, we have considered model order reduction of higher order LTI systems. That aims at finding Error estimation and H∞ and H2 norm using Approximation of original and reduced system. Hence necessary to effortlessness the analysis of the system using approximation Algorithms. The response evaluation is considered in terms of response constraints and graphical assessments. It is reported that the different states of reduced order model compare using a numerical methods is almost alike in performance to that of with original systems. all simulation results have been obtained via MATLAB based novel software (sssMOR...
IETE Journal of Research
Advances in Intelligent Systems and Computing
Othman Maganga , Keith Burnham , Toheed Aizad
Michael Striebel
[Proceedings] IECON '90: 16th Annual Conference of IEEE Industrial Electronics Society
Ahmad Tawayha
Page 1. Model Order Reduction of Nonlinear Dynamical Systems by Chenjie Gu A dissertation submitted in partial satisfaction of the requirements for the degree of Doctor of Philosophy in Engineering – Electrical Engineering and Computer Science in the Graduate Division of the University of California, Berkeley Committee in charge: Professor Jaijeet Roychowdhury, Chair Professor Robert Brayton Professor Jon Wilkening Fall 2011 Page 2. Model Order Reduction of Nonlinear Dynamical Systems Copyright 2011 by Chenjie Gu Page 3.
Younes Chahlaoui
RELATED PAPERS
Sebastian Paredes Guerra
Rafael Bruno
Quaternary International
Jesse Vermaire
Revista de Salud Pública
Carlos Tadeu Siepierski
Syamimi Azizan
Martin Stabel Garrote
Mark Van Der Laan
IET Conference Publications
Tran quoc duyet
Éthique publique
Philippe De Villé
Biophysical Journal
eilon sherman
sonia de gregorio
Indo Nordic Authors' Collective
Dr. Uday Dokras
Proceedings of the tenth international conference on Information Systems - ICIS '89
John Sviokla
Proceedings of the International Conference on Web Intelligence
Diego Pinheiro
Sorin Musuroi
Journal of Arrhythmia
Cassandra Hoorn
JAI (Jurnal Anestesiologi Indonesia)
hasanul arifin
International Urogynecology Journal
JUAN FRANCISCO MACIAS BERROCAL
Open Journal of Air Pollution
maria sol gomez
Cambridge Prisms: Global Mental Health
Marguerite Marlow
Physical Chemistry Chemical Physics
Administrative Theory and Praxis
Maria Lungu
Human Brain Mapping
Proceedings of the Nutrition Society
Maria Pufulete
RELATED TOPICS
- We're Hiring!
- Help Center
- Find new research papers in:
- Health Sciences
- Earth Sciences
- Cognitive Science
- Mathematics
- Computer Science
- Academia ©2024
Thank you for visiting nature.com. You are using a browser version with limited support for CSS. To obtain the best experience, we recommend you use a more up to date browser (or turn off compatibility mode in Internet Explorer). In the meantime, to ensure continued support, we are displaying the site without styles and JavaScript.
- View all journals
- My Account Login
- Explore content
- About the journal
- Publish with us
- Sign up for alerts
- Open access
- Published: 26 November 2023
Impact of industrial robots on environmental pollution: evidence from China
- Yanfang Liu 1
Scientific Reports volume 13 , Article number: 20769 ( 2023 ) Cite this article
3622 Accesses
1 Citations
2 Altmetric
Metrics details
- Environmental sciences
- Environmental social sciences
The application of industrial robots is considered a significant factor affecting environmental pollution. Selecting industrial wastewater discharge, industrial SO 2 emissions and industrial soot emissions as the evaluation indicators of environmental pollution, this paper uses the panel data model and mediation effect model to empirically examine the impact of industrial robots on environmental pollution and its mechanisms. The conclusions are as follows: (1) Industrial robots can significantly reduce environmental pollution. (2) Industrial robots can reduce environmental pollution by improving the level of green technology innovation and optimizing the structure of employment skills. (3) With the increase in emissions of industrial wastewater, industrial SO 2 , and industrial dust, the impacts generated by industrial robots are exhibiting trends of a “W” shape, gradual intensification, and progressive weakening. (4) Regarding regional heterogeneity, industrial robots in the eastern region have the greatest negative impact on environmental pollution, followed by the central region, and the western region has the least negative impact on environmental pollution. Regarding time heterogeneity, the emission reduction effect of industrial robots after 2013 is greater than that before 2013. Based on the above conclusions, this paper suggests that the Chinese government and enterprises should increase investment in the robot industry. Using industrial robots to drive innovation in green technology and optimize employment skill structures, reducing environmental pollution.
Similar content being viewed by others
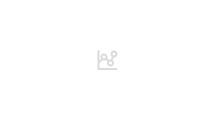
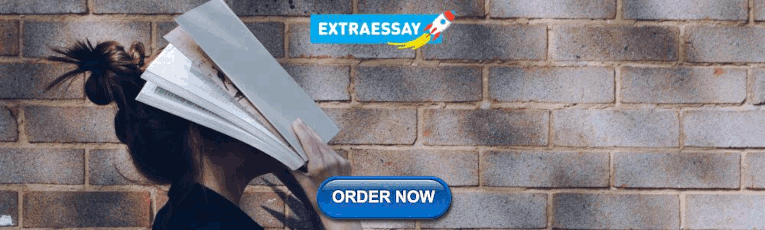
The impact of industrial robot adoption on corporate green innovation in China
Lin Liang, Liujie Lu & Ling Su
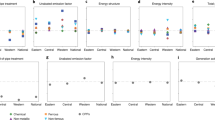
Air pollution reduction and climate co-benefits in China’s industries
Haoqi Qian, Shaodan Xu, … Libo Wu
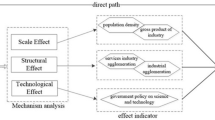
The impact of smart city construction (SCC) on pollution emissions (PE): evidence from China
GuoWei Zhang, XianMin Sun & Shen Zhong
Introduction
Since the reform and opening up, China’s rapid economic growth has created a world-renowned “economic growth miracle” 1 . With the rapid economic growth, China’s environmental pollution problem is becoming more and more serious 2 . According to the “ Global Environmental Performance Index Report ” released by Yale University in the United States in 2022, China’s environmental performance index scores 28.4 points, ranking 160th out of 180 participating countries. The aggravation of environmental pollution not only affects residents’ health 3 , but also affects the efficiency of economic operation 4 . According to calculation of the General Administration of Environmental Protection, the World Bank and the Chinese Academy of Sciences, China’s annual losses caused by environmental pollution account for about 10% of GDP. Exploring the factors that affect environmental pollution and seeking ways to reduce environmental pollution are conducive to the development of economy within the scope of environment.
Industrial robots are machines that can be automatically controlled, repeatedly programmed, and multi-purpose 5 . They replace the low-skilled labor force engaged in procedural work 6 , reducing the raw materials required for manual operation. Industrial robots improve the clean technology level and energy efficiency of coal combustion, reducing pollutant emissions in front-end production. Industrial robots also monitor the energy consumption and sewage discharge in the production process in real time. The excessive discharge behavior of enterprises in the production process is regulated, reducing the emission of pollutants in the end treatment. Based on the selection and coding of literature (Appendix A ), this paper uses the meta-analysis method to compare the impacts of multiple factors such as economics, population, technology, and policy on environmental pollution. As shown in Table 1 , compared to other factors, industrial robots demonstrate greater advantages in reducing environmental pollution. There is a lack of research on the relationship between industrial robots and environmental pollution in China. With the advent of artificial intelligence era, China’s industrial robot industry has developed rapidly. According to data released by the International Federation of Robotics (IFR), from 1999 to 2019, China’s industrial robot ownership and installation shows an increasing trend year by year (Fig. 1 ). In 2013 and 2016, China’s industrial robot installation (36,560) and ownership (349,470) exceeds Japan for the first time, becoming the world’s largest country in terms of installation and ownership of industrial robots. Whether the application of industrial robots in China contributes to the reduction of environmental pollution? What is the mechanism of the impact of China’s industrial robots on environmental pollution? Researching this issue is crucial for filling the gaps in existing research and providing a reference for other countries to achieve emission reduction driven by robots.
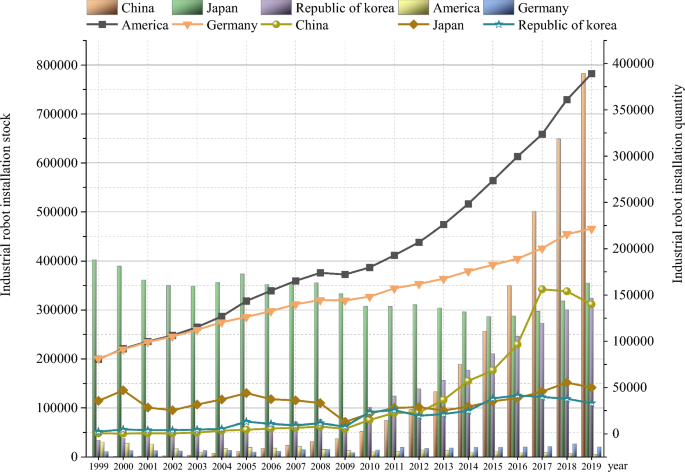
Industrial robot installations in the world’s top five industrial robot markets from 1999 to 2019.
Based on the above analysis, this paper innovatively incorporates industrial robots and environmental pollution into a unified framework. Based on the panel data of 30 provinces in China from 2006 to 2019, this paper uses the ordinary panel model and mediating effect model to empirically test the impact of industrial robots on China’s environmental pollution and its transmission channels. The panel quantile model is used to empirically analyze the heterogeneous impact of industrial robots on environmental pollution under different environmental pollution levels.
Literature review
A large number of scholars have begun to study the problem of environmental pollution. Its research content mainly includes two aspects: The measurement of environmental pollution and its influencing factors. Regarding the measurement, some scholars have used SO 2 emissions 7 , industrial soot emissions 8 and PM2.5 concentration 9 and other single indicators to measure the degree of environmental pollution. The single indicator cannot fully and scientifically reflect the degree of environmental pollution. To make up for this defect, some scholars have included industrial SO 2 emissions, industrial wastewater discharge and industrial soot emissions into the environmental pollution evaluation system, and used the entropy method to measure environmental pollution level 10 . This method ignores the different characteristics and temporal and spatial trends of different pollutants, which makes the analysis one-sided. Regarding the influencing factors, economic factors such as economic development level 11 , foreign direct investment 12 and income 13 , population factors such as population size 14 and urbanization level 15 , energy consumption 16 all have an impact on environmental pollution. Specifically, economic development and technological innovation can effectively reduce environmental pollution 17 . The expansion of population size can aggravate environmental pollution. Income inequality can reduce environmental pollution, but higher income inequality may aggravate environmental pollution 18 . There are “pollution heaven hypothesis” and “pollution halo hypothesis” between foreign direct investment and environmental pollution 19 . Technological factors also have a non-negligible impact on environmental pollution 20 .
With continuous deepening of research, scholars have begun to focus on the impact of automation technology, especially industrial robot technology, on the environment. Ghobakhloo et al. 21 theoretically analyzed the impact of industrial robots on energy sustainability, contending that the application of industrial robots could foster sustainable development of energy. Using data from multiple countries, a few scholars have empirically analyzed the effect of industrial robots on environmental pollution (Table 2 ). Luan et al. 22 used panel data from 73 countries between 1993 and 2019 to empirically analyze the impact of industrial robots on air pollution, finding that the use of industrial robots intensifies environmental pollution. Using panel data from 66 countries from 1993 to 2018, Wang et al. 23 analyzed the impact of industrial robots on carbon intensity and found that industrial robots can reduce carbon intensity. On the basis of analyzing the overall impact of industrial robots on environmental pollution, some scholars conducted in-depth exploration of its mechanism. Based on data from 72 countries between 1993 and 2019, Chen et al. 5 explored the impact of industrial robots on the ecological footprint, discovering that industrial robots can reduce the ecological footprint through time saving effect, green employment effect and energy upgrading effect. Using panel data from 35 countries between 1993 and 2017, Li et al. 24 empirically examined the carbon emission reduction effect of industrial robots, finding that industrial robots can effectively reduce carbon emissions by increasing green total factor productivity and reducing energy intensity. Although the above studies have successfully estimated the overall impact of industrial robots on environmental pollution and its mechanisms, they have not fully considered the role of technological progress, labor structure and other factors in the relationship between the two. These studies all chose data from multiple countries as research samples and lack research on the relationship between industrial robots and environmental pollution in China, an emerging country.
The above literature provides inspiration for this study, but there are still shortcomings in the following aspects: Firstly, there is a lack of research on the relationship between industrial robots and environmental pollution in emerging countries. There are significant differences between emerging and developed countries in terms of institutional background and the degree of environmental pollution. As a representative emerging country, research on the relationship between industrial robots and environmental pollution in China can provide reliable references for other emerging countries. Secondly, theoretically, the study of the impact of industrial robots on environmental pollution is still in its initial stage. There are few studies that deeply explore its impact mechanism, and there is a lack of analysis of the role of technological progress and labor structure in the relationship between the two.
The innovations of this paper are as follows: (1) In terms of sample selection, this paper selects panel data from 30 provinces in China from 2006 to 2019 as research samples to explore the relationship between industrial robots and environmental pollution in China, providing references for other emerging countries to improve environmental quality using industrial robots. (2) In terms of theory, this paper is not limited to revealing the superficial relationship between industrial robots and environmental pollution. it starts from a new perspective and provides an in-depth analysis of how industrial robots affect environmental pollution through employment skill structure and green technology innovation. This not only enriches research in the fields of industrial robots and the environment, but is also of great significance in guiding the direction of industrial policy and technology research and development.
Theoretical analysis and hypothesis
Industrial robots and environmental pollution.
As shown in Fig. 2 , the impact of industrial robots on environmental pollution is mainly reflected in two aspects: Front-end production and end treatment. In front-end production, industrial robots enable artificial substitution effects 25 . Manual operation is replaced by machine operation, reducing the raw materials needed for manual operation. Through the specific program setting of industrial robots, clean energy is applied to industrial production 26 . The use of traditional fuels such as coal and oil is reduced. In terms of end treatment, the traditional pollutant concentration tester only measures a single type of pollutant. Its data cannot be obtained in time. It is easy to cause pollution incidents. Industrial robots can measure a variety of pollutants, and have the function of remote unmanned operation and warning. It reflects the pollution situation in time, reducing the probability of pollution incidents. The use of robots can upgrade sewage treatment equipment and improve the accuracy of pollution treatment, reducing pollutant emissions. Based on the above analysis, this paper proposes hypothesis 1.
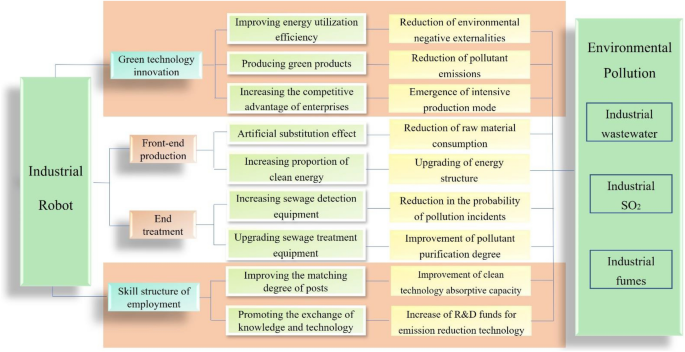
The impact of industrial robots on environmental pollution.
Hypothesis 1
The use of industrial robots can reduce environmental pollution.
Mediating effect of green technology innovation
Industrial robots can affect environmental pollution by promoting green technology innovation. The transmission path of “industrial robots-green technology innovation-environmental pollution” is formed. Industrial robots are the materialization of technological progress in the field of enterprise R&D. Its impact on green technology innovation is mainly manifested in the following two aspects: Firstly, industrial robots classify known knowledge, which helps enterprises to integrate internal and external knowledge 27 . The development of green technology innovation activities of enterprises is promoted. Secondly, enterprises can simulate existing green technologies through industrial robots. The shortcomings of green technology in each link are found. Based on this, enterprises can improve and perfect green technology in a targeted manner. Industrial robots can collect and organize data, which enables enterprises to predict production costs and raw material consumption. Excessive procurement by enterprises can occupy working capital. Inventory backlog leads to warehousing, logistics and other expenses, increasing storage costs 28 . Forecasting the consumption of raw materials allows enterprises to purchase precisely, preventing over-procurement and inventory backlog, thereby reducing the use of working capital and storage costs 29 . The production cost of enterprises is reduced. Enterprises have more funds for green technology research and development.
The continuous innovation of green technology is helpful to solve the problem of environmental pollution. Firstly, green technology innovation helps use resources better 30 , lowers dependence on old energy, and reduces environmental damage. Secondly, green technology innovation promotes the greening of enterprises in manufacturing, sales and after-sales 31 . The emission of pollutants in production process is reduced. Finally, green technology innovation improves the advantages of enterprises in market competition 32 . The production possibility curve expands outward, which encourages enterprises to carry out intensive production. Based on the above analysis, this paper proposes hypothesis 2.
Hypothesis 2
Industrial robots can reduce environmental pollution through green technology innovation.
Mediating effect of employment skill structure
Industrial robots can affect environmental pollution through employment skill structure. The transmission path of “industrial robots-employment skill structure-environmental pollution” is formed. Industrial robots have substitution effect and creation effect on the labor force, improving the employment skill structure. Regarding the substitution effect, enterprises use industrial robots to complete simple and repetitive tasks to improve production efficiency, which crowds out low-skilled labor 6 . Regarding the creation effect, industrial robots create a demand for new job roles that matches automation, such as robot engineers, data analysts, machine repairers, which increases the number of highly skilled labor 33 . The reduction of low-skilled labor and increase of high-skilled labor improve employment skill structure.
High-skilled labor is reflected in the level of education 34 . Its essence is to have a higher level of skills and environmental awareness, which is the key to reducing environmental pollution. Compared with low-skilled labor, high-skilled labor has stronger ability to acquire knowledge and understand skills, which improves the efficiency of cleaning equipment and promotes emission reduction. The interaction and communication between highly skilled labor is also crucial for emission reduction. The excessive wage gap between employees brings high communication costs, which hinders the exchange of knowledge and technology between different employees. The increase in the proportion of high-skilled labor can solve this problem and improve the production efficiency of enterprises 35 . The improvement of production efficiency enables more investment in emission reduction research, decreasing pollutant emissions. Based on the above analysis, this paper proposes hypothesis 3.
Hypothesis 3
Industrial robots can reduce environmental pollution by optimizing employment skills structure.
Model construction and variable selection
Model construction, panel data model.
The panel data model is a significant statistical method, first introduced by Mundlak 36 . Subsequently, numerous scholars have used this model to examine the baseline relationships between core explanatory variables and explained variables 37 . To test the impact of industrial robots on environmental pollution, this paper sets the following panel data model:
In formula ( 1 ), Y it is the explained variable, indicating the degree of environmental pollution in region i in year t . IR it is the core explanatory variable, indicating the installation density of industrial robots in region i in year t . X it is a series of control variables, including economic development level (GDP), urbanization level (URB), industrial structure (EC), government intervention (GOV) and environmental regulation (ER). \(\lambda i\) is the regional factor. \(\varphi t\) is the time factor. \(\varepsilon it\) is the disturbance term.
Mediating effect model
To test the transmission mechanism of industrial robots affecting environmental pollution, this paper sets the following mediating effect model:
In formula ( 2 ), M is the mediating variable, which mainly includes green technology innovation and employment skill structure. Formula ( 2 ) measures the impact of industrial robots on mediating variables. Formula ( 3 ) measures the impact of intermediary variables on environmental pollution. According to the principle of mediating effect 38 , the direct effect \(\theta 1\) , mediating effect \(\beta 1 \times \theta 2\) and total effect \(\alpha 1\) satisfy \(\alpha 1 = \theta 1 + \beta 1 \times \theta 2\) .
Panel quantile model
The panel quantile model was first proposed by Koenke and Bassett 39 . It is mainly used to analyze the impact of core explanatory variables on the explained variables under different quantiles 40 . To empirically test the heterogeneous impact of industrial robots on environmental pollution under different levels of environmental pollution, this paper sets the following panel quantile model:
In formula ( 4 ), \(\tau\) represents the quantile value. \(\gamma 1\) reflects the difference in the impact of industrial robots on environmental pollution at different quantiles. \(\gamma 2\) indicates the different effects of control variables at different quantiles.
Variable selection
Explained variable.
The explained variable is environmental pollution. Considering the timeliness and availability of data, this paper selects industrial wastewater discharge, industrial SO 2 emissions and industrial soot emissions as indicators of environmental pollution.
Explanatory variable
According to production theory, industrial robots can enhance production efficiency 41 . Efficient production implies reduced energy wastage, which in turn decreases the emission of pollutants. Industrial robots can upgrade pollution control equipment, heightening the precision in pollution treatment and reducing pollutant discharge. Referring to Acemoglu and Restrepo 25 , this paper selects the installation density of industrial robots as a measure. The specific formula is as follows:
In formula ( 5 ), Labor ji is the number of labor force in industry j in region i . IR jt is the stock of industrial robot use in industry j in the year t .
Mediating variable
Green technology innovation. Industrial robots can increase the demand for highly-skilled labor 42 , subsequently influencing green technology innovation. Compared to ordinary labor, highly-skilled labor possesses a richer knowledge base and technological learning capability, improving the level of green technology innovation. Green technology innovation can improve energy efficiency 43 , reducing pollution generated by energy consumption. The measurement methods of green technology innovation mainly include three kinds: The first method is to use simple technology invention patents as measurement indicators. Some of technical invention patents are not applied to the production process of enterprise, they cannot fully reflect the level of technological innovation. The second method is to use green product innovation and green process innovation as measurement indicators. The third method is to use the number of green patent applications or authorizations as a measure 44 . This paper selects the number of green patent applications as a measure of green technology innovation.
Employment skill structure. The use of industrial robots reduces the demand for labor performing simple repetitive tasks and increases the need for engineers, technicians, and other specialized skilled personnel, improving the employment skill structure 45 . Compared to ordinary workers, highly-skilled laborers typically have a stronger environmental awareness 46 . Such environmental consciousness may influence corporate decisions, prompting companies to adopt eco-friendly production methods, thus reducing environmental pollution. There are two main methods to measure the structure of employment skills: One is to use the proportion of employees with college degree or above in the total number of employees as a measure. The other is to use the proportion of researchers as a measure. The educational level can better reflect the skill differences of workers. This paper uses the first method to measure the employment skill structure.
Control variable
Economic development level. According to the EKC hypothesis 47 , in the initial stage of economic development, economic development mainly depends on input of production factors, which aggravates environmental pollution. With the continuous development of economy, people begin to put forward higher requirements for environmental quality. The government also begins to adopt more stringent policies to control environmental pollution, which can reduce the level of environmental pollution. According to Liu and Lin 48 , This paper uses per capita GDP to measure economic development level.
Urbanization level. The improvement of urbanization level has both positive and negative effects on pollution. Urbanization can improve the agglomeration effect of cities. The improvement of agglomeration effect can not only promote the sharing of public resources such as infrastructure, health care, but also facilitate the centralized treatment of pollution. The efficiency of environmental governance is improved 49 . The acceleration of urbanization can increase the demand for housing, home appliances and private cars, which increases pollutant emissions 50 . This paper uses the proportion of urban population to total population to measure the level of urbanization.
Industrial structure. Industrial structure is one of the key factors that determine the quality of a country’s environmental conditions 51 . The increase in the proportion of capital and technology-intensive industries can effectively improve resource utilization efficiency and improve resource waste 52 . This paper selects the ratio of the added value of the tertiary industry to the secondary industry to measure industrial structure.
Government intervention. Government intervention mainly affects environmental pollution from the following two aspects: Firstly, the government can give high-tech, energy-saving and consumption-reducing enterprises relevant preferential policies, which promotes the development of emission reduction technologies for these enterprises 53 . Secondly, the government strengthens environmental regulation by increasing investment in environmental law enforcement funds, thus forcing enterprises to save energy and reduce emissions 54 . This paper selects the proportion of government expenditure in GDP to measure government intervention.
Environmental regulation. The investment in environmental pollution control is conducive to the development of clean and environmental protection technology, optimizing the process flow and improving the green production efficiency of enterprises 55 . Pollutant emissions are reduced. This paper selects the proportion of investment in pollution control to GDP to measure environmental regulation.
Data sources and descriptive statistics
This paper selects the panel data of 30 provinces in China from 2006 to 2019 as the research sample. Among them, the installation data of industrial robots are derived from International Federation of Robotics (IFR). The data of labor force and employees with college degree or above are from China Labor Statistics Yearbook . Other data are from the China Statistical Yearbook . The descriptive statistics of variables are shown in Table 3 . Considering the breadth of application and the reliability of analysis capabilities, this paper uses Stata 16 for regression analysis.
Results analysis
Spatial and temporal characteristics of environmental pollution and industrial robots in china, environmental pollution.
Figure 3 a shows the overall trend of average industrial wastewater discharge in China from 2006 to 2019. From 2006 to 2019, the discharge of industrial wastewater shows a fluctuating downward trend, mainly due to the improvement of wastewater treatment facilities and the improvement of treatment capacity. Figure 3 b shows the changing trend of average industrial wastewater discharge in 30 provinces of China from 2006 to 2019. Industrial wastewater discharge in most provinces has declined. There are also some provinces such as Fujian, Guizhou and Qinghai, which have increased industrial wastewater discharge. Their emission reduction task is very arduous.
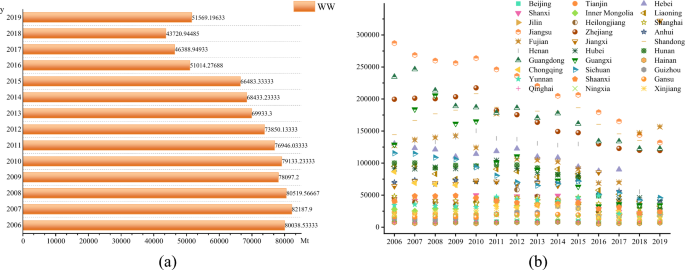
Industrial wastewater discharge from 2006 to 2019.
Figure 4 a shows the overall trend of average industrial SO 2 emissions in China from 2006 to 2019. From 2006 to 2019, industrial SO 2 emissions shows a fluctuating downward trend, indicating that air pollution control and supervision are effective. Figure 4 b shows the trend of average industrial SO 2 emissions in 30 provinces of China from 2006 to 2019. Similar to industrial wastewater, industrial SO 2 emissions decrease in most provinces.
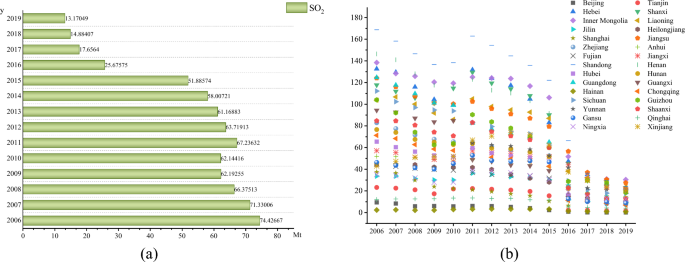
Industrial SO 2 emissions from 2006 to 2019.
Figure 5 a shows the overall trend of average industrial soot emissions in China from 2006 to 2019. Different from industrial wastewater and industrial SO 2 , the emission of industrial soot is increasing year by year. From the perspective of governance investment structure, compared with industrial wastewater and industrial SO 2 , the investment proportion of industrial soot is low. From the perspective of source, industrial soot mainly comes from urban operation, industrial manufacturing and so on. The acceleration of urbanization and the expansion of manufacturing scale have led to an increase in industrial soot emissions. Figure 5 b shows the trend of industrial soot emissions in 30 provinces in China from 2006 to 2019. The industrial soot emissions in most provinces have increased.
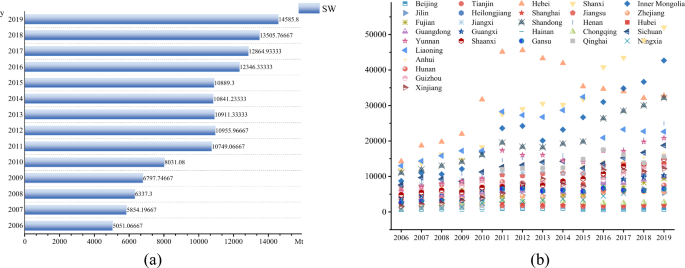
Industrial soot emissions from 2006 to 2019.
Figure 6 shows the spatial distribution characteristics of industrial wastewater, industrial SO 2 and industrial soot emissions. The three types of pollutant emissions in the central region are the largest, followed by the eastern region, and the three types of pollutant emissions in the western region are the smallest. Due to resource conditions and geographical location, the central region is mainly dominated by heavy industry. The extensive development model of high input and consumption makes its pollutant emissions higher than the eastern and western regions. The eastern region is mainly capital-intensive and technology-intensive industries, which makes its pollutant emissions lower than the central region. Although the leading industry in the western region is heavy industry, its factory production and transportation scale are not large, which produces less pollutants.
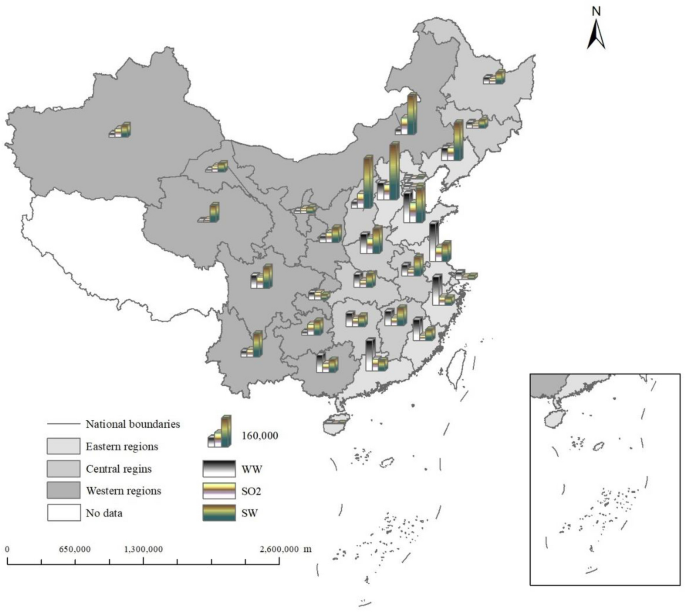
Spatial distribution characteristics of industrial wastewater, industrial SO 2 and industrial soot.
Industrial robots
Figure 7 a shows the overall trend of installation density of industrial robots in China from 2006 to 2019. From 2006 to 2019, the installation density of industrial robots in China shows an increasing trend year by year. The increase of labor cost and the decrease of industrial robot cost make enterprises use more industrial robots, which has a substitution effect on labor force. The installation density of industrial robots is increased. Figure 7 b shows the trend of installation density of industrial robots in 30 provinces of China from 2006 to 2019. The installation density of industrial robots in most provinces has increased. Among them, the installation density of industrial robots in Guangdong Province has the largest growth rate. The installation density of industrial robots in Heilongjiang Province has the smallest growth rate.
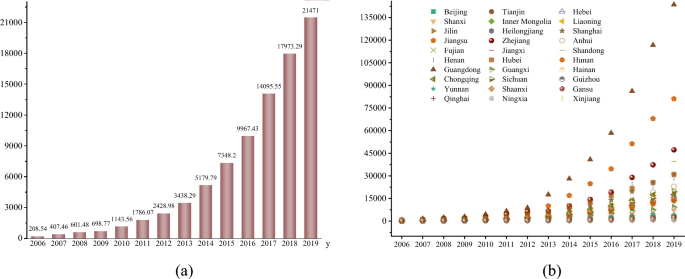
Installation density of industrial robots from 2006 to 2019.
Figure 8 shows the spatial distribution characteristics of installation density of industrial robots. The installation density of industrial robots in the eastern region is the largest, followed by the central region, and the installation density of industrial robots in the western region is the smallest. The eastern region is economically developed and attracts lots of talents to gather here, which provides talent support for the development of industrial robots. Advanced technology also leads to the rapid development of industrial robots in the eastern region. The economy of western region is backward, which inhibits the development of industrial robots.
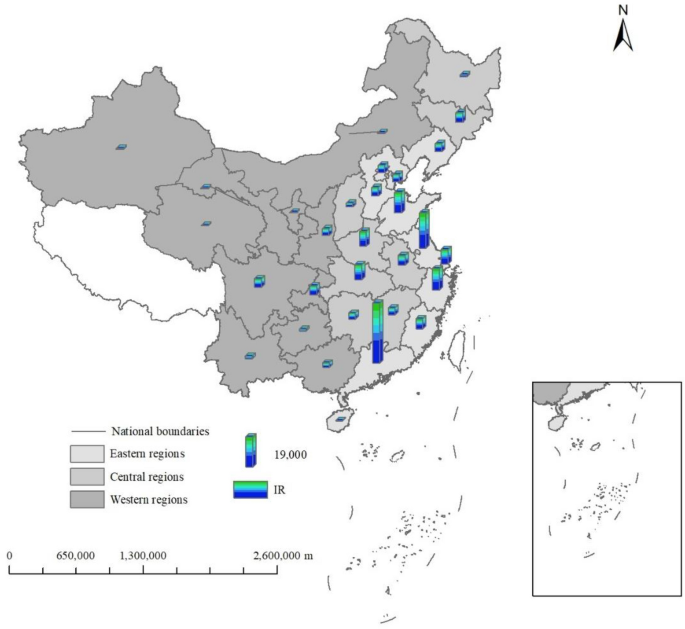
Spatial distribution characteristics of industrial robots.
Benchmark regression results
Table 4 reports the estimation results of the ordinary panel model. Among them, the F test and LM test show that the mixed OLS model should not be used. The Hausman test shows that the fixed effect model should be selected in the fixed effect model and random effect model. This paper selects the estimation results of the fixed effect model to explain.
Regarding the core explanatory variable, industrial robots have a significant negative impact on the emissions of industrial wastewater, industrial SO 2 and industrial soot. Specifically, industrial robots have the greatest negative impact on industrial soot emissions, with a coefficient of -0.277 and passing the 1% significance level. The negative impact of industrial robots on industrial wastewater discharge is second, with an estimated coefficient of -0.242, which also passes the 1% significance level. The negative impact of industrial robots on industrial SO 2 emissions is the smallest, with an estimated coefficient of -0.0875 and passing the 10% significant level. Compared with industrial wastewater and SO 2 , industrial robots have some unique advantages in reducing industrial soot emissions. Firstly, in terms of emission sources, industrial soot emissions mainly come from physical processes such as cutting. These processes can be significantly improved through precise control of industrial robots. Industrial SO 2 comes from the combustion process. Industrial wastewater originates from various industrial processes. It is difficult for industrial robots to directly control these processes. Secondly, in terms of source control and terminal treatment, industrial robots can reduce excessive processing and waste of raw materials, thereby controlling industrial soot emissions at the source. For industrial SO 2 and industrial wastewater, industrial robots mainly play a role in terminal treatment. Since the terminal treatment of industrial SO 2 and industrial wastewater often involves complex chemical treatment processes, it is difficult for industrial robot technology to fully participate in these processes. This makes the impact of industrial robots in the field of industrial SO 2 and industrial wastewater more limited than that in the field of industrial soot.
Regarding the control variables, the level of economic development has a significant inhibitory effect on industrial SO 2 emissions. The higher the level of economic development, the stronger the residents’ awareness of environmental protection, which constrains the pollution behavior of enterprises. The government also adopts strict policies to control pollutant emissions. The impact of urbanization level on the discharge of industrial wastewater, industrial SO 2 and industrial soot is significantly negative. The improvement of urbanization level can improve the efficiency of resource sharing and the centralized treatment of pollutants, reducing environmental pollution. The industrial structure significantly reduces industrial SO 2 and industrial soot emissions. The upgrading of industrial structure not only reduces the demand for energy, but also improves the efficiency of resource utilization. The degree of government intervention only significantly reduces the discharge of industrial wastewater. The possible reason is that to promote economic development, the government invests more money in high-yield areas, which crowds out investment in the environmental field. Similar to the degree of government intervention, environmental regulation has a negative impact on industrial wastewater discharge. The government’s environmental governance investment has not given some support to the enterprise’s clean technology research, which makes the pollution control investment not produce good emission reduction effect.
Mediation effect regression results
Green technology innovation.
Table 5 reports the results of intermediary effect model when green technology innovation is used as an intermediary variable. Industrial robots can have a positive impact on green technology innovation. For every 1% increase in the installation density of industrial robots, the level of green technology innovation increases by 0.722%. After adding the green technology innovation, the estimated coefficient of industrial robots has decreased, which shows that the intermediary variable is effective.
In the impact of industrial robots on industrial wastewater discharge, the mediating effect of green technology innovation accounts for 8.17% of the total effect. In the impact of industrial robots on industrial SO 2 emissions, the mediating effect of green technology innovation accounts for 11.8% of the total effect. In the impact of industrial robots on industrial soot emissions, the mediating effect of green technology innovation accounts for 3.72% of the total effect.
Employment skill structure
Table 6 reports the results of intermediary effect model when the employment skill structure is used as an intermediary variable. Industrial robots have a positive impact on the employment skill structure. For every 1% increase in the installation density of industrial robots, the employment skill structure is improved by 0.0837%. Similar to green technology innovation, the intermediary variable of employment skill structure is also effective.
In the impact of industrial robots on industrial wastewater discharge, the mediating effect of employment skill structure accounts for 6.67% of the total effect. In the impact of industrial robots on industrial SO 2 emissions, the mediating effect of employment skill structure accounts for 20.66% of the total effect. In the impact of industrial robots on industrial soot emissions, the mediating effect of employment skill structure accounts for 15.53% of the total effect.
Robustness test and endogeneity problem
Robustness test.
To ensure the robustness of the regression results, this paper tests the robustness by replacing core explanatory variables, shrinking tail and replacing sample. Regarding the replacement of core explanatory variables, in the benchmark regression, the installation density of industrial robots is measured by the stock of industrial robots. Replacing the industrial robot stock with the industrial robot installation quantity, this paper re-measures the industrial robot installation density. Regarding the tail reduction processing, this paper reduces the extreme outliers of all variables in the upper and lower 1% to eliminate the influence of extreme outliers. Regarding the replacement of samples, this paper removes the four municipalities from the sample. The estimation results are shown in Table 7 . Industrial robots still have a significant negative impact on environmental pollution, which confirms the robustness of benchmark regression results.
Endogeneity problem
Logically speaking, although the use of industrial robots can reduce environmental pollution, there may be reverse causality. Enterprises may increase the use of industrial robots to meet emission reduction standards, which increases the use of industrial robots in a region. Due to the existence of reverse causality, there is an endogenous problem that cannot be ignored between industrial robots and environmental pollution.
To solve the impact of endogenous problems on the estimation results, this paper uses the instrumental variable method to estimate. According to the selection criteria of instrumental variables, this paper selects the installation density of industrial robots in the United States as the instrumental variable. The trend of the installation density of industrial robots in the United States during the sample period is similar to that of China, which is consistent with the correlation characteristics of instrumental variables. The application of industrial robots in the United States is rarely affected by China’s economic and social factors, and cannot affect China’s environmental pollution, which is in line with the exogenous characteristics of instrumental variables.
Table 8 reports the estimation results of instrumental variable method. Among them, the column (1) is listed as the first stage regression result. The estimated coefficient of instrumental variable is significantly positive, which is consistent with the correlation. Column (2), column (3) and column (4) of Table 8 are the second stage regression results of industrial wastewater, industrial SO 2 and industrial soot emissions as explanatory variables. The estimated coefficients of industrial robots are significantly negative, which again verifies the hypothesis that industrial robots can reduce environmental pollution. Compared with Table 4 , the absolute value of estimated coefficient of industrial robots is reduced, which indicates that the endogenous problems caused by industrial robots overestimate the emission reduction effect of industrial robots. The test results prove the validity of the instrumental variables.
Panel quantile regression results
Traditional panel data models might obscure the differential impacts of industrial robots at specific pollution levels. To address this issue, this paper uses a panel quantile regression model to empirically analyze the effects of industrial robots across different environmental pollution levels.
Table 9 shows that industrial robots have a negative impact on industrial wastewater discharge. With the increase of the quantile of industrial wastewater discharge, the regression coefficient of industrial robots shows a W-shaped change. Specifically, when the industrial wastewater discharge is in the 0.1 quantile, the regression coefficient of industrial robot is − 0.229, and it passes the 1% significant level. When the industrial wastewater discharge is in the 0.25 quantile, the impact of industrial robots on industrial wastewater discharge is gradually enhanced. Its regression coefficient decreases from − 0.229 to − 0.256. When the industrial wastewater discharge is in the 0.5 quantile, the regression coefficient of industrial robot increases from − 0.256 to − 0.152. When the industrial wastewater discharge is at the 0.75 quantile, the regression coefficient of industrial robot decreases from − 0.152 to − 0.211. When the industrial wastewater discharge is in the 0.9 quantile, the regression coefficient of industrial robot increases from − 0.211 to − 0.188. For every 1% increase in the installation density of industrial robots, the discharge of industrial wastewater is reduced by 0.188%.
When industrial wastewater discharge is at a low percentile, the use of industrial robots can replace traditional production methods, reducing energy waste and wastewater discharge. As industrial wastewater discharge increases, the production process becomes more complex. Industrial robots may be involved in high-pollution, high-emission productions, diminishing the robots’ emission-reducing effects. When industrial wastewater discharge reaches high levels, pressured enterprises seek environmentally friendly production methods and use eco-friendly industrial robots to reduce wastewater discharge. As wastewater discharge continues to rise, enterprises tend to prioritize production efficiency over emission control, weakening the negative impact of industrial robots on wastewater discharge. When wastewater discharge is at a high percentile, enterprises should balance production efficiency and environmental protection needs, by introducing eco-friendly industrial robots to reduce wastewater discharge.
Table 10 shows that with the increase of industrial SO 2 emission quantile level, the negative impact of industrial robots on industrial SO 2 emissions gradually increases. Specifically, when industrial SO 2 emissions are below 0.5 quantile, the impact of industrial robots on industrial SO 2 emissions is not significant. When the industrial SO 2 emissions are above 0.5 quantile, the negative impact of industrial robots on industrial SO 2 emissions gradually appears.
When industrial SO 2 emissions are at a low percentile, the application of industrial robots primarily aims to enhance production efficiency, not to reduce SO 2 emissions. Enterprises should invest in the development of eco-friendly industrial robots, ensuring they are readily available for deployment when a reduction in industrial SO 2 emissions is necessary. As industrial SO 2 emissions continue to rise, both the government and the public pay increasing attention to the issue of SO 2 emissions. To meet stringent environmental standards, enterprises begin to use industrial robots to optimize the production process, reduce reliance on sulfur fuels, and consequently decrease SO 2 emissions. Enterprises should regularly evaluate the emission reduction effectiveness of industrial robots, using the assessment data to upgrade and modify the robots’ emission reduction technologies.
Table 11 shows that with the increase of industrial soot emissions quantile level, the negative impact of industrial robots on industrial soot emissions gradually weakens. Specifically, when industrial soot emissions are below 0.75 quantile, industrial robots have a significant negative impact on industrial soot emissions. This negative effect decreases with the increase of industrial soot emissions. When the industrial soot emissions are above 0.75 quantile, the negative impact of industrial robots on industrial soot emissions gradually disappears.
When industrial soot emissions are at a low percentile, they come from a few sources easily managed by industrial robots. As industrial soot emissions increase, the sources become more diverse and complex, making it harder for industrial robots to control. Even with growing environmental awareness, it may take time to effectively use robots in high-emission production processes and control industrial soot emissions. Enterprises should focus on researching how to better integrate industrial robot technology with production processes that have high soot emission levels. The government should provide financial and technical support to enterprises, assisting them in using industrial robots more effectively for emission reduction.
Figure 9 intuitively reflects the trend of the regression coefficient of industrial robots with the changes of industrial wastewater, industrial SO 2 and industrial soot emissions. Figure 9 a shows that with the increase of industrial wastewater discharge, the regression coefficient of industrial robots shows a W-shaped trend. Figure 9 b shows that with the increase of industrial SO 2 emissions, the regression coefficient of industrial robots gradually decreases. The negative impact of industrial robots on industrial SO 2 emissions is gradually increasing. Figure 9 c shows that with the increase of industrial soot emissions, the regression coefficient of industrial robots shows a gradual increasing trend. The negative impact of industrial robots on industrial soot emissions has gradually weakened. Figure 9 a, b and c confirm the estimation results of Tables 9 , 10 and 11 .
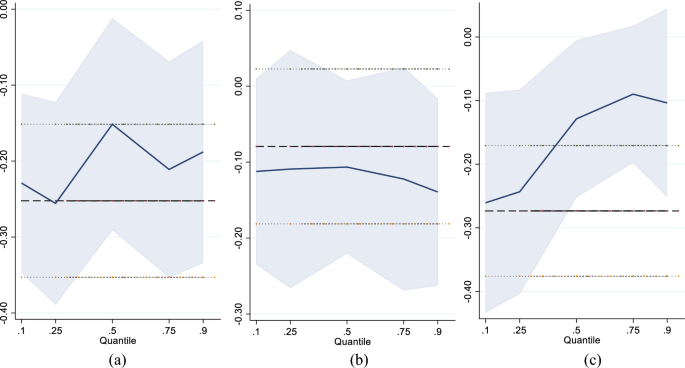
Change of quantile regression coefficient.
Heterogeneity analysis
Regional heterogeneity.
This paper divides China into three regions: Eastern, central and western regions according to geographical location. The estimated results are shown in Table 12 . The industrial robots in eastern region have the greatest negative impact on three pollutants, followed by central region, and the industrial robots in western region have the least negative impact on three pollutants. The use of industrial robots in eastern region far exceeds that in central and western regions. The eastern region is far more than central and western regions in terms of human capital, technological innovation and financial support. Compared with central and western regions, the artificial substitution effect, upgrading of sewage treatment equipment and improvement of energy utilization efficiency brought by industrial robots in eastern region are more obvious.
Time heterogeneity
The development of industrial robots is closely related to policy support 56 . In 2013, the Ministry of Industry and Information Technology issued the “ Guiding Opinions on Promoting the Development of Industrial Robot Industry ”. This document proposes: By 2020, 3 to 5 internationally competitive leading enterprises and 8 to 10 supporting industrial clusters are cultivated. In terms of high-end robots, domestic robots account for about 45% of the market share, which provides policy support for the development of industrial robots. Based on this, this paper divides the total sample into two periods: 2006–2012 and 2013–2019, and analyzes the heterogeneous impact of industrial robots on environmental pollution in different periods. The estimation results are shown in Table 13 . Compared with 2006–2012, the emission reduction effect of industrial robots during 2013–2019 is greater.
The use of industrial robots can effectively reduce environmental pollution, which is consistent with hypothesis 1. This is contrary to the findings of Luan et al. 22 , who believed that the use of industrial robots would exacerbate air pollution. The inconsistency in research conclusions may be due to differences in research focus, sample size, and maturity of industrial robot technology. In terms of research focus, this paper mainly focuses on the role of industrial robots in reducing pollutant emissions during industrial production processes. Their research focuses more on the energy consumption caused by the production and use of industrial robots, which could aggravate environmental pollution. In terms of sample size, the sample size of this paper is 30 provinces in China from 2006 to 2019. These regions share consistency in economic development, industrial policies and environmental regulations. Their sample size is 74 countries from 1993 to 2019. These countries cover different geographical, economic and industrial development stages, affecting the combined effect of robots on environmental pollution. In terms of the maturity of industrial robots, the maturity of industrial robot technology has undergone tremendous changes from 1993 to 2019. In the early stages, industrial robot technology was immature, which might cause environmental pollution. In recent years, industrial robot technology has gradually matured, and its operating characteristics have become environmentally friendly. Their impact on environmental pollution has gradually improved. This paper mainly conducts research on the mature stage of industrial robot technology. Their research covers the transition period from immature to mature industrial robot technology. The primary reason that the use of industrial robots can reduce environmental pollution is: The use of industrial robots has a substitution effect on labor force, which reduces the raw materials needed for manual operation. For example, in the industrial spraying of manufacturing industry, the spraying robot can improve the spraying quality and material utilization rate, thereby reducing the waste of raw materials by manual operation. Zhang et al. 57 argued that energy consumption has been the primary source of environmental pollution. Coal is the main energy in China, and the proportion of clean energy is low 58 . In 2022, clean energy such as natural gas, hydropower, wind power and solar power in China accounts for only 25.9% of the total energy consumption, which can cause serious environmental pollution problems. Industrial robots can promote the use of clean energy in industrial production and the upgrading of energy structure 24 . The reduction of raw materials and the upgrading of energy structure can control pollutant emissions in front-end production. On September 1, 2021, the World Economic Forum (WEF) released the report “ Using Artificial Intelligence to Accelerate Energy Transformation ”. The report points out that industrial robots can upgrade pollution monitoring equipment and sewage equipment, which reduces pollutant emissions in end-of-pipe treatment. Ye et al. 59 also share the same viewpoint.
The use of industrial robots can reduce environmental pollution through green technology innovation, which is consistent with hypothesis 2. Industrial robots promote the integration of knowledge, which helps enterprises to carry out green technology innovation activities. Meanwhile, Jung et al. 60 suggested that industrial robots can lower production costs for companies, allowing them to invest in green technology research. The level of green technology innovation is improved. Green technology innovation reduces environmental pollution through the following three aspects: Firstly, the improvement of energy utilization efficiency. China’s utilization efficiency of traditional energy sources such as coal is not high. The report of “ 2013-Global Energy Industry Efficiency Research ” points out that China’s energy utilization rate is only ranked 74th in the world in 2013. Low energy efficiency brings serious environmental pollution problems 61 . Du et al. 62 found that the innovation of green technologies, such as clean coal, can enhance energy efficiency and decrease environmental pollution. Secondly, the production of green products. Green technology innovation accelerates the green and recyclable process of production, thereby reducing the pollutants generated in production process. Thirdly, the improvement of enterprise competitive advantage. Green technology innovation can enable enterprises to gain greater competitive advantage in green development 63 . The supply of environmentally friendly products increases, which not only meets the green consumption needs of consumers, but also reduces the emission of pollutants.
Industrial robots can reduce environmental pollution by optimizing the structure of employment skills, which is consistent with hypothesis 3. Autor et al. 64 contended that industrial robots would replace conventional manual labor positions, reducing the demand for low-skilled labor. Industrial robots represent the development of numerical intelligence. With the continuous development of digital intelligence, the demand for high-skilled labor in enterprises has increased. Koch et al. 65 demonstrated that the use of industrial robots in Spanish manufacturing firms leads to an increase in the number of skilled workers. In February 2020, the Ministry of Human Resources and Social Security, the State Administration of Market Supervision and the National Bureau of Statistics jointly issues 16 new professions such as intelligent manufacturing engineering and technical personnel, industrial Internet engineering and technical personnel, and virtual reality engineering and technical personnel to the society. These new occupations increase the demand for highly skilled labor. The reduction of low-skilled labor and increase of high-skilled labor optimize the structure of employment skills. The optimization of employment skill structure narrows the wage gap between employees, reducing the communication cost of employees. Employees learn and exchange technology with each other, which not only improves the absorption capacity of clean technology. It also improves the production efficiency of enterprises and increases corporate profits, so that enterprises can use more funds for clean technology research and development, thereby reducing environmental pollution.
Conclusions and policy recommendations
Based on the panel data of 30 provinces in China from 2006 to 2019, this paper uses the panel data model and mediating effect model to empirically test the impact of industrial robots on environmental pollution and its transmission mechanism. This paper uses panel quantile model, regional samples and time samples to further analyze the heterogeneous impact of industrial robots on environmental pollution. The conclusions are as follows: (1) Industrial robots can significantly reduce environmental pollution. For every 1% increase in industrial robots, the emissions of industrial wastewater, industrial SO 2 , and industrial dust and smoke decrease by − 0.242%, − 0.0875%, and − 0.277%. This finding is contrary to that of Luan et al. 22 , who argued that the use of industrial robots exacerbates air pollution. The results of this paper provide a contrasting perspective, highlighting the potential value of industrial robots in mitigating environmental pollution. (2) Industrial robots can reduce environmental pollution by improving green technology innovation level and optimizing employment skills structure. In the impact of industrial robots on industrial wastewater discharge, the mediating effect of green technology innovation accounts for 8.17% of total effect. The mediating effect of employment skill structure accounts for 6.67% of total effect. In the impact of industrial robots on industrial SO 2 emissions, the mediating effect of green technology innovation accounts for 11.8% of total effect. The mediating effect of employment skill structure accounts for 20.66% of total effect. In the impact of industrial robots on industrial soot emissions, the mediating effect of green technology innovation accounts for 3.72% of total effect. The mediating effect of employment skill structure accounts for 15.53% of total effect. While Obobisa et al. 66 and Zhang et al. 67 highlighted the role of green technological innovation in addressing environmental pollution. Chiacchio et al. 68 and Dekle 69 focused on the effects of industrial robots on employment. The mediating impact of technology and employment in the context of robots affecting pollution hasn’t been addressed. Our research provides the first in-depth exploration of this crucial intersection. (3) Under different environmental pollution levels, the impact of industrial robots on environmental pollution is different. Among them, with the increase of industrial wastewater discharge, the impact of industrial robots on industrial wastewater discharge shows a “W-shaped” change. With the increase of industrial SO 2 emissions, the negative impact of industrial robots on industrial SO 2 emissions is gradually increasing. On the contrary, with the increase of industrial soot emissions, the negative impact of industrial robots on industrial soot emissions gradually weakens. (4) Industrial robots in different regions and different periods have heterogeneous effects on environmental pollution. Regarding regional heterogeneity, industrial robots in eastern region have the greatest negative impact on environmental pollution, followed by central region, and western region has the least negative impact on environmental pollution. Regarding time heterogeneity, the negative impact of industrial robots on environmental pollution in 2013–2019 is greater than that in 2006–2012. Chen et al. 5 and Li et al. 24 both examined the overarching impact of industrial robots on environmental pollution. They did not consider the varying effects of robots on pollution across different regions and time periods. Breaking away from the limitations of previous holistic approaches, our study offers scholars a deeper understanding of the diverse environmental effects of industrial robots.
According to the above research conclusions, this paper believes that the government and enterprises can promote emission reduction through industrial robots from the following aspects.
Increase the scale of investment in robot industry and promote the development of robot industry. China’s industrial robot ownership ranks first in the world. Its industrial robot installation density is lower than that of developed countries such as the United States, Japan and South Korea. The Chinese government should give some financial support to robot industry and promote the development of robot industry, so as to effectively reduce environmental pollution. The R&D investment of industrial robots should be increased so that they can play a full role in reducing raw material consumption, improving energy efficiency and sewage treatment capacity.
Give full play to the role of industrial robots in promoting green technology innovation. Industrial robots can reduce environmental pollution through green technology innovation. The role of industrial robots in innovation should be highly valued. The advantages of knowledge integration and data processing of industrial robots should be fully utilized. Meanwhile, the government should support high-polluting enterprises that do not have industrial robots from the aspects of capital, talents and technology, so as to open up the channels for these enterprises to develop and improve clean technology by using industrial robots.
Give full play to the role of industrial robots in optimizing employment skills structure. The use of industrial robots can create jobs with higher skill requirements and increase the demand for highly skilled talents. China is relatively short of talents in the field of emerging technologies. The education department should actively build disciplines related to industrial robots to provide talent support for high-skilled positions. Enterprises can also improve the skill level of the existing labor force through on-the-job training and job competition.
Data availability
The datasets used or analyzed during the current study are available from Yanfang Liu on reasonable request.
Liu, Y. & Dong, F. How technological innovation impacts urban green economy efficiency in emerging economies: A case study of 278 Chinese cities. Resour. Conserv. Recycl. 169 , 105534 (2021).
Article Google Scholar
Wang, Y. & Chen, X. Natural resource endowment and ecological efficiency in China: Revisiting resource curse in the context of ecological efficiency. Resour. Policy 66 , 101610 (2020).
Kampa, M. & Castanas, E. Human health effects of air pollution. Environ. Pollut. 151 , 362–367 (2008).
Article CAS PubMed Google Scholar
Feng, Y., Chen, H., Chen, Z., Wang, Y. & Wei, W. Has environmental information disclosure eased the economic inhibition of air pollution?. J. Clean. Prod. 284 , 125412 (2021).
Article CAS Google Scholar
Chen, Y., Cheng, L. & Lee, C.-C. How does the use of industrial robots affect the ecological footprint? International evidence. Ecol. Econ. 198 , 107483 (2022).
Krenz, A., Prettner, K. & Strulik, H. Robots, reshoring, and the lot of low-skilled workers. Eur. Econ. Rev. 136 , 103744 (2021).
Xu, C., Zhao, W., Zhang, M. & Cheng, B. Pollution haven or halo? The role of the energy transition in the impact of FDI on SO 2 emissions. Sci. Total Environ. 763 , 143002 (2021).
Article ADS CAS PubMed Google Scholar
Yuan, H. et al. Influences and transmission mechanisms of financial agglomeration on environmental pollution. J. Environ. Manag. 303 , 114136 (2022).
Liu, G., Dong, X., Kong, Z. & Dong, K. Does national air quality monitoring reduce local air pollution? The case of PM 2.5 for China. J. Environ. Manag. 296 , 113232 (2021).
Ren, S., Hao, Y. & Wu, H. Digitalization and environment governance: Does internet development reduce environmental pollution?. J. Environ. Plan. Manag. 66 , 1533–1562 (2023).
Zhao, J., Zhao, Z. & Zhang, H. The impact of growth, energy and financial development on environmental pollution in China: New evidence from a spatial econometric analysis. Energy Econ. 93 , 104506 (2021).
Wang, H. & Liu, H. Foreign direct investment, environmental regulation, and environmental pollution: An empirical study based on threshold effects for different Chinese regions. Environ. Sci. Pollut. Res. 26 , 5394–5409 (2019).
Albulescu, C. T., Tiwari, A. K., Yoon, S.-M. & Kang, S. H. FDI, income, and environmental pollution in Latin America: Replication and extension using panel quantiles regression analysis. Energy Economics 84 , 104504 (2019).
Li, K., Fang, L. & He, L. How population and energy price affect China’s environmental pollution?. Energy Policy 129 , 386–396 (2019).
Liang, L., Wang, Z. & Li, J. The effect of urbanization on environmental pollution in rapidly developing urban agglomerations. J. Clean. Prod. 237 , 117649 (2019).
Sharma, R., Shahbaz, M., Kautish, P. & Vo, X. V. Does energy consumption reinforce environmental pollution? Evidence from emerging Asian economies. J. Environ. Manag. 297 , 113272 (2021).
Chen, F., Wang, M. & Pu, Z. The impact of technological innovation on air pollution: Firm-level evidence from China. Technol. Forecast. Soc. Change 177 , 121521 (2022).
Hao, Y., Chen, H. & Zhang, Q. Will income inequality affect environmental quality? Analysis based on China’s provincial panel data. Ecol. Ind. 67 , 533–542 (2016).
Liu, Q., Wang, S., Zhang, W., Zhan, D. & Li, J. Does foreign direct investment affect environmental pollution in China’s cities? A spatial econometric perspective. Sci. Total Environ. 613 , 521–529 (2018).
Article ADS PubMed Google Scholar
Mughal, N. et al. The role of technological innovation in environmental pollution, energy consumption and sustainable economic growth: Evidence from South Asian economies. Energy Strat. Rev. 39 , 100745 (2022).
Ghobakhloo, M. & Fathi, M. Industry 4.0 and opportunities for energy sustainability. J. Clean. Prod. 295 , 126427 (2021).
Luan, F., Yang, X., Chen, Y. & Regis, P. J. Industrial robots and air environment: A moderated mediation model of population density and energy consumption. Sustain. Prod. Consump. 30 , 870–888 (2022).
Wang, Q., Li, Y. & Li, R. Do industrial robots reduce carbon intensity? The role of natural resource rents and corruption control. Environ. Sci. Pollut. Res. https://doi.org/10.1007/s11356-023-29760-7 (2023).
Li, Y., Zhang, Y., Pan, A., Han, M. & Veglianti, E. Carbon emission reduction effects of industrial robot applications: Heterogeneity characteristics and influencing mechanisms. Technol. Soc. 70 , 102034 (2022).
Acemoglu, D. & Restrepo, P. Robots and jobs: Evidence from US labor markets. J. Polit. Econ. 128 , 2188–2244 (2020).
Liu, J., Liu, L., Qian, Y. & Song, S. The effect of artificial intelligence on carbon intensity: Evidence from China’s industrial sector. Socio Econ. Plan. Sci. 83 , 101002 (2022).
Lee, C.-C., Qin, S. & Li, Y. Does industrial robot application promote green technology innovation in the manufacturing industry?. Technol. Forecast. Soc. Change 183 , 121893 (2022).
Riza, M., Purba, H. H. & Mukhlisin,. The implementation of economic order quantity for reducing inventory cost. Res. Logist. Prod. 8 , 207–216 (2018).
Google Scholar
Tang, Z. & Ge, Y. CNN model optimization and intelligent balance model for material demand forecast. Int. J. Syst. Assur. Eng. Manag. 13 , 978–986 (2022).
Wang, Q. & Ren, S. Evaluation of green technology innovation efficiency in a regional context: A dynamic network slacks-based measuring approach. Technol. Forecast. Soc. Change 182 , 121836 (2022).
Chang, K., Liu, L., Luo, D. & Xing, K. The impact of green technology innovation on carbon dioxide emissions: The role of local environmental regulations. J. Environ. Manag. 340 , 117990 (2023).
Tu, Y. & Wu, W. How does green innovation improve enterprises’ competitive advantage? The role of organizational learning. Sustain. Prod. Consum. 26 , 504–516 (2021).
Dauth, W., Findeisen, S., Südekum, J. & Woessner, N. German robots-the impact of industrial robots on workers (2017).
Berger, N. & Fisher, P. A well-educated workforce is key to state prosperity. Economic Policy Institute 22 , 1–14 (2013).
Bourke, J. & Roper, S. AMT adoption and innovation: An investigation of dynamic and complementary effects. Technovation 55 , 42–55 (2016).
Mundlak, Y. On the pooling of time series and cross section data. Econometrica J. Econom. Soc. 46 , 69–85 (1978).
Article MathSciNet MATH Google Scholar
Sun, B., Li, J., Zhong, S. & Liang, T. Impact of digital finance on energy-based carbon intensity: Evidence from mediating effects perspective. J. Environ. Manag. 327 , 116832 (2023).
MacKinnon, D. P., Warsi, G. & Dwyer, J. H. A simulation study of mediated effect measures. Multivar. Behav. Res. 30 , 41–62 (1995).
Koenker, R. & Bassett, G. Jr. Regression quantiles. Econometrica J. Econom. Soc. 23 , 33–50 (1978).
Akram, R., Chen, F., Khalid, F., Ye, Z. & Majeed, M. T. Heterogeneous effects of energy efficiency and renewable energy on carbon emissions: Evidence from developing countries. J. Clean. Prod. 247 , 119122 (2020).
Pham, A.-D. & Ahn, H.-J. Rigid precision reducers for machining industrial robots. Int. J. Precis. Eng. Manuf. 22 , 1469–1486 (2021).
Du, L. & Lin, W. Does the application of industrial robots overcome the Solow paradox? Evidence from China. Technol. Soc. 68 , 101932 (2022).
Sun, H., Edziah, B. K., Sun, C. & Kporsu, A. K. Institutional quality, green innovation and energy efficiency. Energy Policy 135 , 111002 (2019).
Wang, X., Su, Z. & Mao, J. How does haze pollution affect green technology innovation? A tale of the government economic and environmental target constraints. J. Environ. Manag. 334 , 117473 (2023).
Tang, C., Huang, K. & Liu, Q. Robots and skill-biased development in employment structure: Evidence from China. Econ. Lett. 205 , 109960 (2021).
Cicatiello, L., Ercolano, S., Gaeta, G. L. & Pinto, M. Willingness to pay for environmental protection and the importance of pollutant industries in the regional economy. Evidence from Italy. Ecol. Econ. 177 , 106774 (2020).
Xie, Q., Xu, X. & Liu, X. Is there an EKC between economic growth and smog pollution in China? New evidence from semiparametric spatial autoregressive models. J. Clean. Prod. 220 , 873–883 (2019).
Liu, K. & Lin, B. Research on influencing factors of environmental pollution in China: A spatial econometric analysis. J. Clean. Prod. 206 , 356–364 (2019).
Wang, Y. & Wang, J. Does industrial agglomeration facilitate environmental performance: New evidence from urban China?. J. Environ. Manag. 248 , 109244 (2019).
Cheng, Z. & Hu, X. The effects of urbanization and urban sprawl on CO 2 emissions in China. Environ. Dev. Sustain. 25 , 1792–1808 (2023).
Hu, W., Tian, J. & Chen, L. An industrial structure adjustment model to facilitate high-quality development of an eco-industrial park. Sci. Total Environ. 766 , 142502 (2021).
Hao, Y. et al. Reexamining the relationships among urbanization, industrial structure, and environmental pollution in China—New evidence using the dynamic threshold panel model. Energy Rep. 6 , 28–39 (2020).
Guo, Y., Xia, X., Zhang, S. & Zhang, D. Environmental regulation, government R&D funding and green technology innovation: Evidence from China provincial data. Sustainability 10 , 940 (2018).
Ouyang, X., Li, Q. & Du, K. How does environmental regulation promote technological innovations in the industrial sector? Evidence from Chinese provincial panel data. Energy Policy 139 , 111310 (2020).
Zhang, W. & Li, G. Environmental decentralization, environmental protection investment, and green technology innovation. Environ. Sci. Pollut. Res. https://doi.org/10.1007/s11356-020-09849-z (2020).
Cheng, H., Jia, R., Li, D. & Li, H. The rise of robots in China. J. Econ. Perspect. 33 , 71–88 (2019).
Zhang, X. et al. Evaluating the relationships among economic growth, energy consumption, air emissions and air environmental protection investment in China. Renew. Sustain. Energy Rev. 18 , 259–270 (2013).
Jia, Z. & Lin, B. How to achieve the first step of the carbon-neutrality 2060 target in China: The coal substitution perspective. Energy 233 , 121179 (2021).
Ye, Z. et al. Tackling environmental challenges in pollution controls using artificial intelligence: A review. Sci. Total Environ. 699 , 134279 (2020).
Jung, J. H. & Lim, D.-G. Industrial robots, employment growth, and labor cost: A simultaneous equation analysis. Technol. Forecas. Soc. Change 159 , 120202 (2020).
Liu, H., Zhang, Z., Zhang, T. & Wang, L. Revisiting China’s provincial energy efficiency and its influencing factors. Energy 208 , 118361 (2020).
Article PubMed Google Scholar
Du, K. & Li, J. Towards a green world: How do green technology innovations affect total-factor carbon productivity. Energy Policy 131 , 240–250 (2019).
Li, G., Wang, X., Su, S. & Su, Y. How green technological innovation ability influences enterprise competitiveness. Technol. Soc. 59 , 101136 (2019).
Autor, D. H., Levy, F. & Murnane, R. J. The skill content of recent technological change: An empirical exploration. Q. J. Econ. 118 , 1279–1333 (2003).
Article MATH Google Scholar
Koch, M., Manuylov, I. & Smolka, M. Robots and firms. Econ. J. 131 , 2553–2584 (2021).
Obobisa, E. S., Chen, H. & Mensah, I. A. The impact of green technological innovation and institutional quality on CO 2 emissions in African countries. Technol. Forecast. Soc. Change 180 , 121670 (2022).
Zhang, M. & Liu, Y. Influence of digital finance and green technology innovation on China’s carbon emission efficiency: Empirical analysis based on spatial metrology. Sci. Total Environ. 838 , 156463 (2022).
Chiacchio, F., Petropoulos, G. & Pichler, D. The impact of industrial robots on EU employment and wages: A local labour market approach (Bruegel working paper, 2018).
Dekle, R. Robots and industrial labor: Evidence from Japan. J. Jpn. Int. Econ. 58 , 101108 (2020).
Download references
Author information
Authors and affiliations.
Harbin Vocational College of Science and Technology, Harbin, 150300, Heilongjiang, People’s Republic of China
Yanfang Liu
You can also search for this author in PubMed Google Scholar
Contributions
Y.L.: Conceptualization, Resources, Supervision, Methodology, Software. I have read and agreed to the published version of the manuscript.
Corresponding author
Correspondence to Yanfang Liu .
Ethics declarations
Competing interests.
The author declares no competing interests.
Additional information
Publisher's note.
Springer Nature remains neutral with regard to jurisdictional claims in published maps and institutional affiliations.
Supplementary Information
Supplementary information., rights and permissions.
Open Access This article is licensed under a Creative Commons Attribution 4.0 International License, which permits use, sharing, adaptation, distribution and reproduction in any medium or format, as long as you give appropriate credit to the original author(s) and the source, provide a link to the Creative Commons licence, and indicate if changes were made. The images or other third party material in this article are included in the article's Creative Commons licence, unless indicated otherwise in a credit line to the material. If material is not included in the article's Creative Commons licence and your intended use is not permitted by statutory regulation or exceeds the permitted use, you will need to obtain permission directly from the copyright holder. To view a copy of this licence, visit http://creativecommons.org/licenses/by/4.0/ .
Reprints and permissions
About this article
Cite this article.
Liu, Y. Impact of industrial robots on environmental pollution: evidence from China. Sci Rep 13 , 20769 (2023). https://doi.org/10.1038/s41598-023-47380-6
Download citation
Received : 24 July 2023
Accepted : 13 November 2023
Published : 26 November 2023
DOI : https://doi.org/10.1038/s41598-023-47380-6
Share this article
Anyone you share the following link with will be able to read this content:
Sorry, a shareable link is not currently available for this article.
Provided by the Springer Nature SharedIt content-sharing initiative
By submitting a comment you agree to abide by our Terms and Community Guidelines . If you find something abusive or that does not comply with our terms or guidelines please flag it as inappropriate.
Quick links
- Explore articles by subject
- Guide to authors
- Editorial policies
Sign up for the Nature Briefing: Anthropocene newsletter — what matters in anthropocene research, free to your inbox weekly.

ORIGINAL RESEARCH article
This article is part of the research topic.
Low-Carbon Economy and Sustainable Development: Driving Force, Synergistic Mechanism, and Implementation Path
resources on carbon emissions reduction in China Provisionally Accepted
- 1 University of South China, China
The final, formatted version of the article will be published soon.
The Accountability Audit of Natural Resources (AANR) is a major institutional arrangement for advancing the construction of an ecological civilization in China. Based on the panel data of 271 cities in China from 2005 to 2017, this paper investigates the relationship between the AANR and carbon dioxide (CO2) emissions using a multiperiod difference-in-differences (DID) model. The results show that AANR significantly increases the CO2 emissions reduction rate by 0.009 units at the 5% significance level. The results still hold after a series of robustness tests. Given all else being equal, this significant effect is 0.001. Further analyses show that AANR improves pilot cities' CO2 emissions reduction rate mainly by enhancing their green innovation capability. The mediating effect of cities' green technology innovation capability plays a role of 96.00%, while the AANR's direct effect only accounts for 4.00%. The AANR has significantly positive effects of 0.017 and 0.029 for western cities and cities with high fiscal pressure at the 5% and 1% significance levels, respectively. Therefore, strengthening AANR implementation through enhancing the mediating efficiency of cities' green technology innovations, and implementing dynamically differentiated AANR policies in Chinese meso-cities will contribute to the achievement of China's carbon peaking and carbon neutrality targets.
Keywords: AANR, CO2 emissions reduction rate, government concern for the environment, Green technology innovation capability, multiperiod DID model
Received: 21 Nov 2023; Accepted: 18 Apr 2024.
Copyright: © 2024 Tang, Shu and Li. This is an open-access article distributed under the terms of the Creative Commons Attribution License (CC BY) . The use, distribution or reproduction in other forums is permitted, provided the original author(s) or licensor are credited and that the original publication in this journal is cited, in accordance with accepted academic practice. No use, distribution or reproduction is permitted which does not comply with these terms.
* Correspondence: Mx. Xuefeng Li, University of South China, Hengyang, 421001, Hunan Province, China
People also looked at
- What's My Car Worth?
- Buyer's Guide
2024 Ford F-150 Lightning Price Drops as Much as $5500
With order books reopened, the most drastic price reduction is for the extended-range Lightning Flash, which drops from $75,590 to $70,090. The base Pro model's price is unchanged.
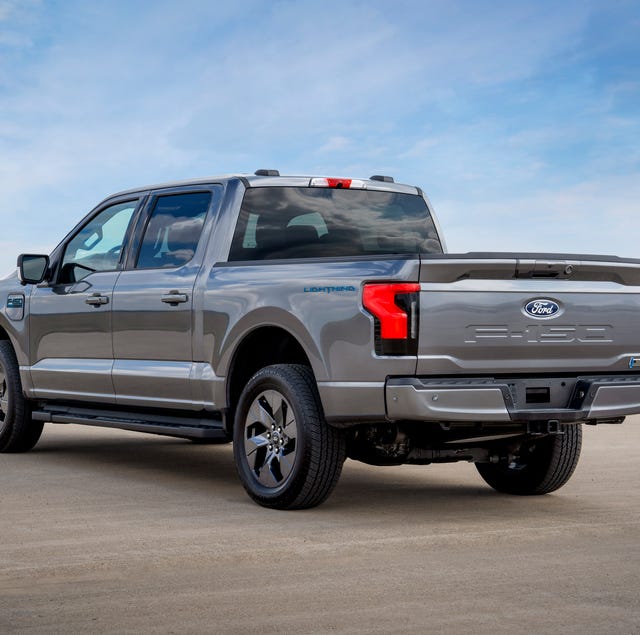
- The price for the new Flash trim drops the most, cutting $5500 from the price at the start of 2024 from $75,590 to $70,090.
- The price changes are concurrent with a handful of midyear changes to the Lightning such as a new heat pump system and an updated charging display for the dash.
Ford reopened the order books for the 2024 F-150 Lightning, allowing customers to buy the electric pickup once more—this time with lower prices for three trims. Pricing for three of the Lightning's five trims has been reduced, while the base Pro and top-trim Platinum hold their original prices.
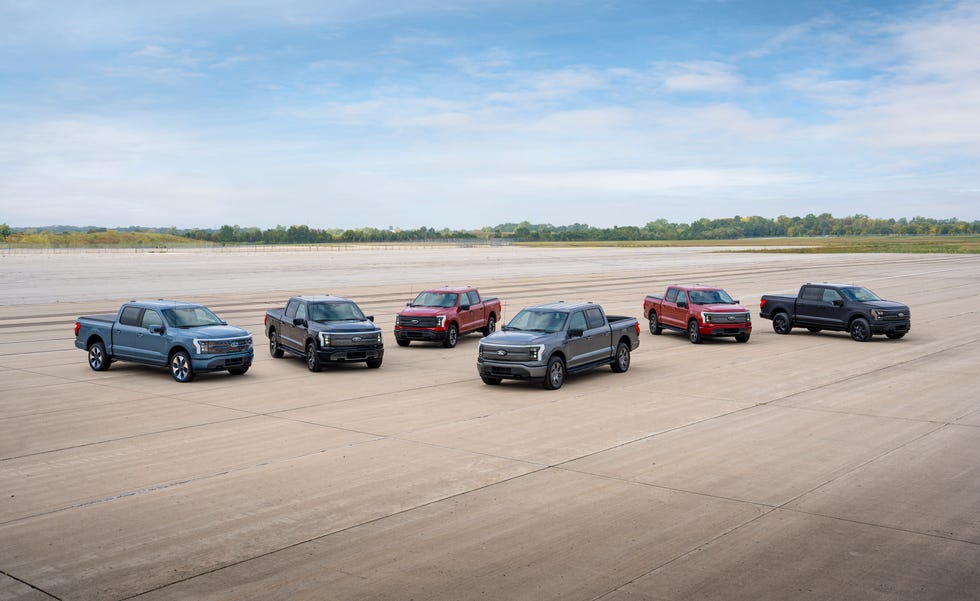
The Flash model, which is new for 2024, earns the most significant price reduction of $5500 and drops from $75,590 to $70,090. Pricing changes for the XLT and Lariat models are smaller, but not insignificant. The XLT now starts at $65,090, and the extended-range battery-equipped Lariat now starts at $79,090. Those changes mark reductions of $2000 and $2500 respectively. Pricing for the Pro and Platinum trims remains unchanged compared to the start of the model year at $57,090 and $87,090 respectively.
Additional Updates
The price changes are concurrent with multiple separate alterations to the Lightning's equipment offerings. A new vapor injection heat pump system has been added to the Lightning to moderate battery temperatures. Charging information for the instrument cluster has been updated, adding current charge status, charging speeds, and an estimated finish time to the display.
Ford's towing technology package now comes standard on all but the base Pro model, while the manufacturer's Blue Cruise hands-free driving joins the upper trims. Keyless entry, a walk-away locking function, and a change that powers up the ProPower onboard generator as soon as you unlock the truck round out the changes.
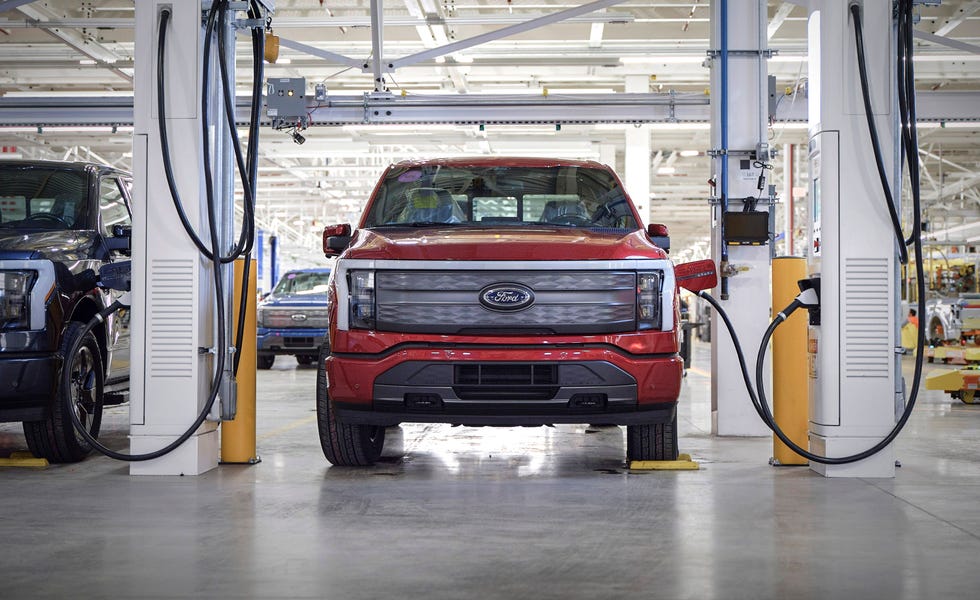
Price adjustments for the F-150 Lightning are nothing new. Whether it's Ford raising prices three times in five months (a thing that occurred in 2022 ), or it's Ford dropping prices for the electric pickup by $10,000 in one go (something that happened in 2023 ), the Lightning's pricing has ping-ponged with no real sign of settling.
This most recent fluctuation comes months after Ford announced plans to scale back F-150 Lightning production, effective April 1. The production changes come as Ford attempts to "match production to customer demand." A statement released by Ford at the time explained that the company expects continued EV sales growth this year, with the caveat that the growth will be smaller than Ford's initial estimates.
Jack Fitzgerald’s love for cars stems from his as yet unshakable addiction to Formula 1. After a brief stint as a detailer for a local dealership group in college, he knew he needed a more permanent way to drive all the new cars he couldn’t afford and decided to pursue a career in auto writing. By hounding his college professors at the University of Wisconsin-Milwaukee, he was able to travel Wisconsin seeking out stories in the auto world before landing his dream job at Car and Driver . His new goal is to delay the inevitable demise of his 2010 Volkswagen Golf.
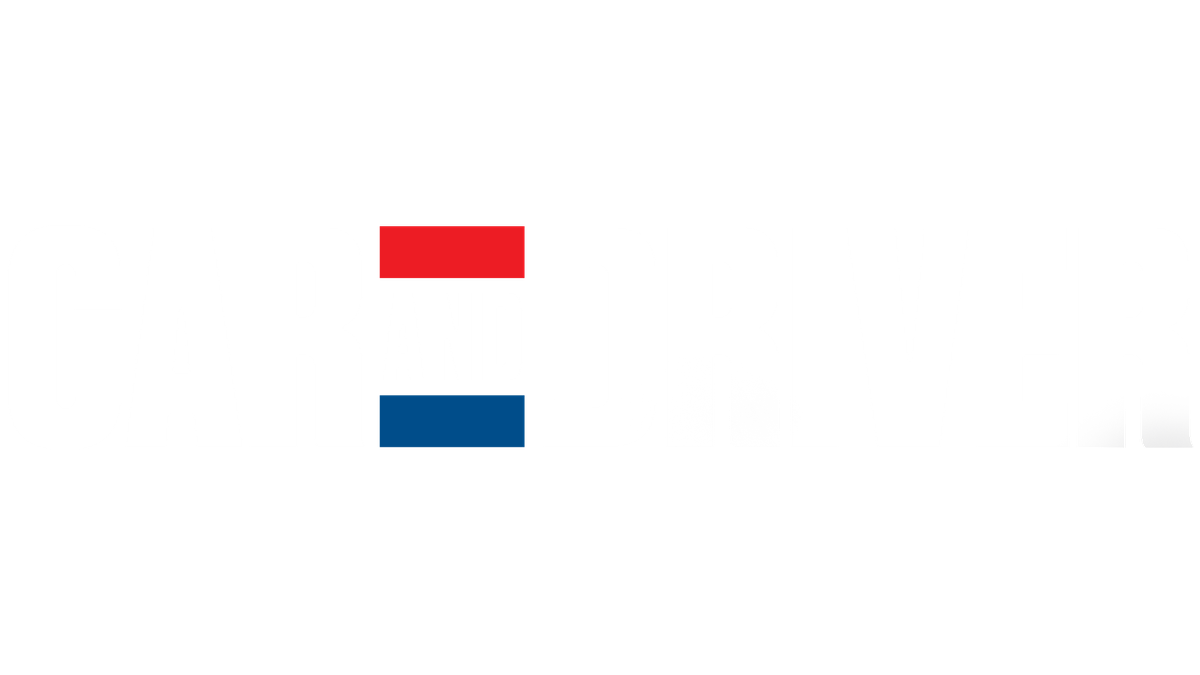
.css-190qir1:before{background-color:#000000;color:#fff;left:0;width:50%;border:0 solid transparent;bottom:48%;height:0.125rem;content:'';position:absolute;z-index:-10;} News .css-188buow:after{background-color:#000000;color:#fff;right:0;width:50%;border:0 solid transparent;bottom:48%;height:0.125rem;content:'';position:absolute;z-index:-10;}
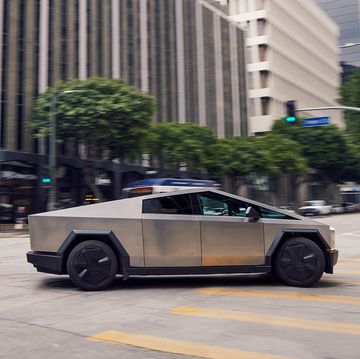
Test: Rivian R1T Shows How Cold Affects EV Range
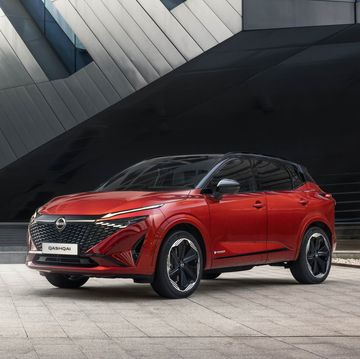
Nissan Reveals Striking New Qashqai SUV for Europe
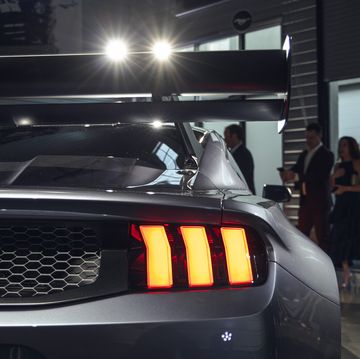
2025 Mustang GTD Order Applications Are Now Open
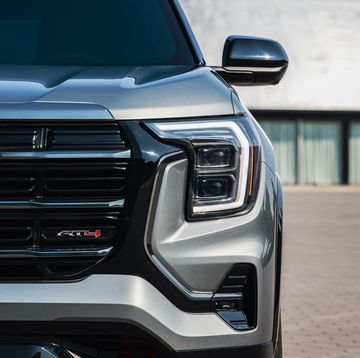
2025 GMC Terrain Teases Assertive New Front End
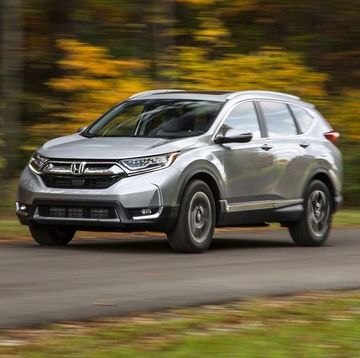
NHTSA Upgrades Probe Into 3 Million Hondas
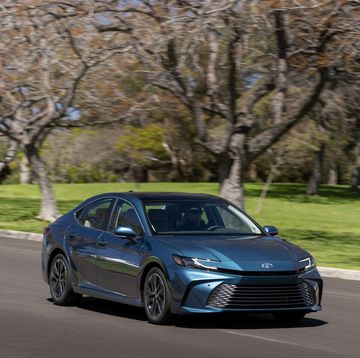
Hybrid-Only 2025 Toyota Camry Starts At $29,495
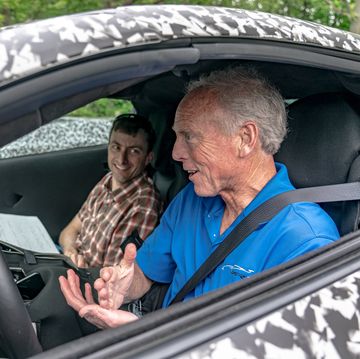
Corvette Engineering Icon Tadge Juechter to Retire
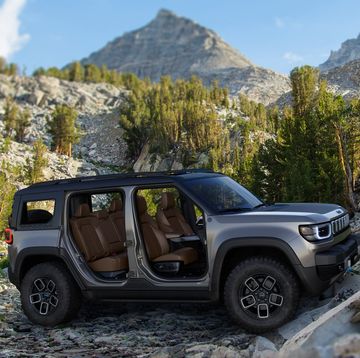
Jeep Recon, Wagoneer S EVs Could Get Gas Versions
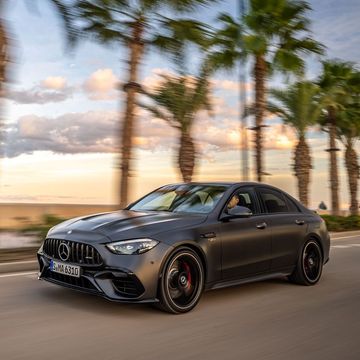
2024 AMG C63 Is a 671-HP PHEV That Starts at $85K
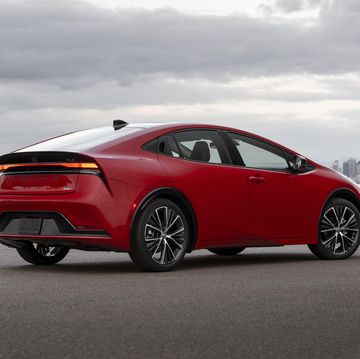
Toyota Prius Recalled: Rear Doors Can Fly Open
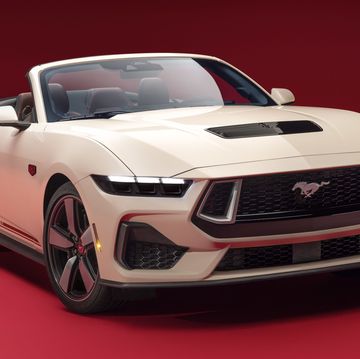
Ford Reveals Mustang 60th Anniversary Edition
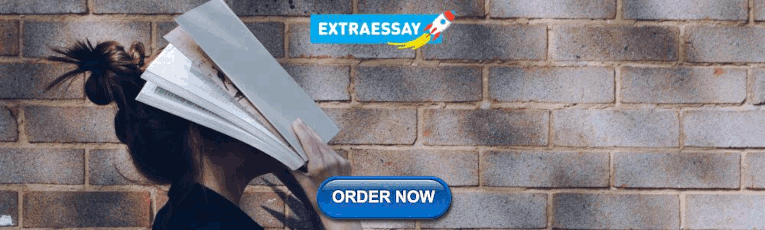
IMAGES
VIDEO
COMMENTS
Complex practical system designs pose significant challenges from a research perspective, often resulting in high computational requirements for analysis. Model order reduction (MOR) is a valuable technique to address this issue. This study provides a comprehensive review of the literature on MOR, focusing specifically on high-dimensional complex systems. It examines the fundamental theories ...
The large-scale structure systems in engineering are complex, high dimensional, and variety of physical mechanism couplings; it will be difficult to analyze the dynamic behaviors of complex systems quickly and optimize system parameters. Model order reduction (MOR) is an efficient way to address those problems and widely applied in the engineering areas. This paper focuses on the model order ...
This paper focuses on the model order reduction of high-dimensional complex systems and reviews basic theories, well-posedness, and limitations of common methods of the model order reduction using ...
Jan 2008. Model Order Reduction: Theory, Research Aspects and Applications. pp.33-45. The development of Model Order Reduction techniques for various problems was triggered by the success of ...
This work will be featured as a chapter in the upcoming Handbook on Model Order Reduction, (P. Benner, S. Grivet-Talocia, A. Quarteroni, G. Rozza, W. H. A. Schilders, L. M. Silveira, eds, to appear on DE GRUYTER) and reviews the numerical treatment of the most important matrix manifolds that arise in the context of model reduction.
The race for the most efficient, accurate, and universal algorithm in scientific computing drives innovation. At the same time, this healthy competition is only beneficial if the research output is actually comparable to prior results. Fairly comparing algorithms can be a complex endeavor, as the implementation, configuration, compute environment, and test problems need to be well-defined. Due ...
In this paper, singular perturbation theory is exploited to obtain a reduced-order model of a slow-fast piecewise linear 2-DOF oscillator subjected to harmonic excitation. The nonsmooth ...
Research on model reduction methods for parametric dynamic systems has become a central issue of concern with regard to the order reduction of dynamic systems. The desired approach is to generate a parametric reduced model that approximates the original full-order dynamic system with high fidelity over a given parameter range.
The POD is a powerful and effective model order reduction method which aims at obtaining the most important components of a high-dimensional complex system by using a few proper orthogonal modes, and it is widely studied and applied by a large number of researchers in the past few decades. The large-scale structure systems in engineering are complex, high dimensional, and variety of physical ...
Cover design: deblik, Berlin Printed on acid-free paper 987654321 springer.com Preface The idea for this book originated during the workshop "Model order reduction, coupled problems and optimization" held at the Lorentz Center in Leiden from September 19-23, 2005.
Model order reduction : methods, concepts and properties. A framework for model reduction and synthesis is presented, which greatly enlarges the options for the re-use of reduced order models in circuit simulation by simulators of choice and allows for synthesis without controlled sources. Expand.
Model Order Reduction (MOR) has demonstrated its robustness and wide use in engineering and science for the simulation of large-scale mathematical systems over the last few decades. MOR is currently being intensively optimized for dynamic systems that are becoming increasingly complex. MOR broad applications have been identified not only in the modeling but also for optimization and control ...
In contrast, this paper focuses on a different research problem that reduces the order of water quality dynamics model, which has not been studied in the literature, with the end-goal of performing post-MOR model driven control of water quality dynamics. B. Paper contributions The major objective of the paper is to investigate the
TLDR. This work explores the development and the analysis of an efficient reduced order model for the study of a bifurcating phenomenon, known as the Coandă effect, in a multi-physics setting involving fluid and solid media, and provides several insights on how the introduction of an elastic structure influences the bifURcating behaviour. Expand.
sophisticated solution methods that make use of properties of the underlying systems. For example, whereas in the 1960s and 1970s one often had to construct special basis. functions to solve ...
A detailed review of Reduced Order Modeling and Model Order Reduction methods and complementary states space coordination and solution strategies is provided in Section 3. The main features, advantages, and shortcomings of their different implementations are listed and contrasted in Tables 2 - 5. The manuscript concludes in Section 4.
Model (Order) Reduction • ~1 000 000 hits in Google … • www.modelreduction.com , web.mit.edu/mor/ , … • Many different research communities use different forms of model reduction: Fluid dynamics Mechanics Computational biology Circuit design Control theory … • Many heuristics available. More or less well-motivated.
In this paper, to find a lower order model equivalent to a given higher order system, a simple method based on T.C Hsia's approximation method is proposed. ... Model Order Reduction: Theory, Research Aspects and Applications. NY, NY: Springer. Segalman, D.J. (2007). Model reduction of systems with localized nonlinearities. Journal of ...
The innovations of this paper are as follows: (1) In terms of sample selection, this paper selects panel data from 30 provinces in China from 2006 to 2019 as research samples to explore the ...
Building performance prediction is a significant area of research, due to its potential to enhance the efficiency of building energy management systems. Its importance is particularly evident when such predictions are validated against field data. This paper presents an intelligent computational model combining Monte Carlo analysis, Energy Plus, and an artificial neural network (ANN) to refine ...
The accurate prediction of highway passenger volume is very important for China's transportation planning and economic development. Based on a neural network, this paper establishes a prediction model by using historical road passenger traffic and related influencing factor data, aiming to provide an accurate road passenger traffic prediction. Firstly, the historical highway passenger volume ...
This paper addresses these complexities by developing a mathematical model to construct a sustainable suppliers-to-consumers pricing model, incorporating the BTS, in the perishable goods market. Our research reveals that the decision to adopt the BTS hinges on factors like the spoilage reduction effect, premium effect, production cost, and tag ...
The Accountability Audit of Natural Resources (AANR) is a major institutional arrangement for advancing the construction of an ecological civilization in China. Based on the panel data of 271 cities in China from 2005 to 2017, this paper investigates the relationship between the AANR and carbon dioxide (CO2) emissions using a multiperiod difference-in-differences (DID) model. The results show ...
With order books reopened, the most drastic price reduction is for the extended-range Lightning Flash, which drops from $75,590 to $70,090. The base Pro model's price is unchanged.