queuing theory Recently Published Documents
Total documents.
- Latest Documents
- Most Cited Documents
- Contributed Authors
- Related Sources
- Related Keywords
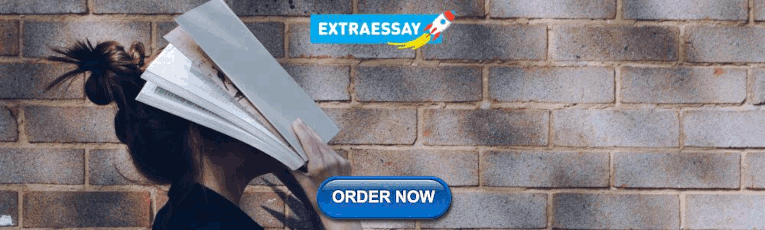
Queuing Theory of Improved Practical Byzantine Fault Tolerant Consensus
In recent years, the use of consensus mechanism to maintain the security of blockchain system has become a considerable concern of the community. Delegated proof of stake (DPoS) and practical Byzantine fault tolerant (PBFT) consensus mechanisms are key technologies in maintaining the security of blockchain system. First, this study proposes a consensus mechanism combining DPoS and PBFT, which can rapidly deal with malicious witness nodes and shorten the time of block verification. Second, the M/PH/1 queuing model is used to analyze the performance of the proposed consensus mechanism, and the performance of the improved practical Byzantine fault tolerant consensus mechanism is evaluated from steady-state conditions and key performance measure of the system. Third, the current study uses the theoretical method of open (Jackson) queuing network, combined with the blockchain consensus process, and provides theoretical analysis with special cases. Lastly, this research utilizes numerical examples to verify the computability of the theoretical results. The analytic method is expected to open a series of potentially promising research in queueing theory of blockchain systems.
Brownian non-Gaussian polymer diffusion and queing theory in the mean-field limit
Abstract We link the Brownian non-Gaussian diffusion of a polymer center of mass to a microscopic cause: the polymerization/depolymerization phenomenon occurring when the polymer is in contact with a monomer chemostat. The anomalous behavior is triggered by the polymer critical point, separating the dilute and the dense phase in the grand canonical ensemble. In the mean-field limit we establish contact with queuing theory and show that the kurtosis of the polymer center of mass diverges alike a response function when the system becomes critical, a result which holds for general polymer dynamics (Zimm, Rouse, reptation). Both the equilibrium and nonequilibrium behaviors are solved exactly as a reference study for novel stochastic modeling and experimental setup.
Analysis of the Queuing System for the Indonesian Islamic Bank (BSI) Bengkulu Branch
The purpose of this study is to determine the average level of customer arrivals and the average service time of customers in the queue. The analytical model used in this study is a multi-channel single-phase queuing theory analysis with a mathematical formula. The queuing process is a process related to the arrival of the customer to a queuing system, then waiting in the queue until the waiter selects the customer according to the service discipline, and finally the customer leaves the queuing system after the service is finished. At Bank Syariah Indonesia (BSI) KC Bengkulu S. Parman 1 there are 5 tellers provided to serve customers who will make deposits, withdrawals and cash transfers. Queues that occur at the optimal service level can be obtained by the performance of the queuing system with the calculation results, namely, the average number of customers in the queue (nq) 31.88 customers, customers in the total system 33.08 people, the average time in the queue 0, 000767 and the total system time is 0.034097 or 2 minutes. Thus, customers do not take too long to make transactions. With the number of tellers as many as five people, there is a long waiting time for customers (Wq) in the queue, which is 0.02777 hours or 2 minutes and the average number of customers in the queue (Ls) is 2 people..
Application of Queuing Theory to Analyse an ATM Queuing System
Performance analysis of peer-to-peer networks based on two-phase service queuing theory, queuing theory in traffic management system.
Abstract: In cities where the number of vehicles has consistently expanded faster than the transportation infrastructure available to serve them. More on queuing theory and its crucial application has been discussed in the current study. In Thudiyalur, Gandhipuram, and Periyanaickenpalayam, all in Coimbatore, this research examines the usefulness of queuing theory in the field of traffic management. The concept of traffic intensity isapplied to a set of areas in queuing theory in this study. Keywords: Traffic intensity, Queuing theory, Single server Poisson model
5G Infrastructure Network Slicing: E2E Mean Delay Model and Effectiveness Assessment to Reduce Downtimes in Industry 4.0
Fifth Generation (5G) is expected to meet stringent performance network requisites of the Industry 4.0. Moreover, its built-in network slicing capabilities allow for the support of the traffic heterogeneity in Industry 4.0 over the same physical network infrastructure. However, 5G network slicing capabilities might not be enough in terms of degree of isolation for many private 5G networks use cases, such as multi-tenancy in Industry 4.0. In this vein, infrastructure network slicing, which refers to the use of dedicated and well isolated resources for each network slice at every network domain, fits the necessities of those use cases. In this article, we evaluate the effectiveness of infrastructure slicing to provide isolation among PLs in an industrial private 5G network. To that end, we develop a queuing theory-based model to estimate the E2E mean packet delay of the infrastructure slices. Then, we use this model to compare the E2E mean delay for two configurations, i.e., dedicated infrastructure slices with segregated resources for each PL against the use of a single shared infrastructure slice to serve the performance-sensitive traffic from PLs. Also we evaluate the use of TSN against bare Ethernet to provide layer 2 connectivity among the 5G system components. We use a complete and realistic setup based on experimental and simulation data of the scenario considered. Our results support the effectiveness of infrastructure slicing to provide isolation in performance among the different slices. Then, using dedicated slices with segregated resources for each PL might reduce the number of the production downtimes and associated costs as the malfunctioning of a PL will not affect the network performance perceived by the performance-sensitive traffic from other PLs. Last, our results show that, besides the improvement in performance, TSN technology truly provides full isolation in the transport network compared to standard Ethernet thanks to traffic prioritization, traffic regulation, and bandwidth reservation capabilities.
The impact of seasonality on efficient airport capacity utilization
The major cause of under-capacity or overcapacity at smaller airports is seasonality. Such airports are finding it difficult to determine the capacity to meet the demand and adequately handle passengers in both high and low season. If the capacity is not optimally defined, excessive congestions and waiting times occur, resulting in lower service quality. Airports greatly benefit from capacity utilization analysis in terms of more accurate planning, designing, and adjusting capacity to the current demand in order to encourage further development as well as to reduce additional costs. Using queuing theory, this paper aims to answer the following question: is the passenger capacity at Rijeka International Airport (Croatia) optimally determined to meet the demand promptly, both in high and low season, without causing excessive congestions and waiting times. The results obtained indicate the occurrence of overcapacity since high season demand can be well served, even with reduced capacity used in the low season when demand is significantly lower.
Workload Control and Order Dispatching Rules
The purpose of this study is to choose an order dispatching rule and measure the work-in-process and lead-time in the production process of a conveyor chain manufacturer. The main strategic issue for the manufacturer is dependability, which requires meeting deadlines and managing internal lead-times. The study integrates two techniques, workload control (WLC) and an analytical hierarchy process (AHP), respectively systems for production planning and control, and multi-criteria decision support, both widely used in handling manufacturing strategic issues. The research method is a field experiment. Supported by the AHP and according to strategic criteria, practitioners selected the early due date rule (the order with the closest due date comes first) to release 231 orders. Then, employing a methodology designed to support WLC applications, the study measured key parameters that provide information regarding the overall performance of the manufacturer, the input rate, work-in-process, lead-time, throughput performance, and the level of safety stock. Using the model and a graphical tool derived from queuing theory, the throughput diagram, the study provides evidence that, although the manufacturing process is satisfactorily balanced and achieves acceptable performance, the level of safety stock is small and should be increased to prevent starvation on the shop floor.
Mathematical Model of the Delay in Communication Networks Based on QS with a Time Lag
In the mathematical modeling of modern computer networks, telecommunication networks, traffic flows, logistics and many others, the methods of queuing theory are widely used. In turn, in studies of queuing systems (QS) G/G/1 with arbitrary distribution laws of intervals between adjacent requirements of the incoming flow and their service time, the spectral decomposition method (MSD) of solving the Lindley integral equation is often used. This method is based on the search for zeros and poles of the constructed spectral decomposition in the form of some fractional-rational function using numerical methods to determine the roots of polynomials. In this case, the coefficients of the polynomial in the numerator of the expansion are expressed through the unknown parameters of the distribution laws used to describe the QS. In the case of teletraffic research, usually these unknown parameters of the distribution laws can be determined through the numerical characteristics of the intervals between traffic packets by the method of moments. The purpose of this article is to present a fundamentally new mathematical model of a system formed by two flows with distribution laws shifted to the right. This is possible only for those probability distribution laws whose density functions are Laplace transformable. The main advantages of such systems, let us call them time lag systems, are that they provide less queue latency compared to conventional systems, and that they extend the range of traffic parameters. The article presents the results obtained on the average delay of requests in the queue for a system with exponential and hyper-Erlang distributions, an algorithm for calculating the average delay and the results of computational experiments in the Mathcad package.
Export Citation Format
Share document.

An official website of the United States government
The .gov means it’s official. Federal government websites often end in .gov or .mil. Before sharing sensitive information, make sure you’re on a federal government site.
The site is secure. The https:// ensures that you are connecting to the official website and that any information you provide is encrypted and transmitted securely.
- Publications
- Account settings
Preview improvements coming to the PMC website in October 2024. Learn More or Try it out now .
- Advanced Search
- Journal List
- IEEE J Transl Eng Health Med
An Integrated Model of Patient and Staff Satisfaction Using Queuing Theory
Alexander komashie.
Wycliffe Hall, Oxford University, OxfordOX2 6PWU.K.
Engineering Design Centre, Department of Engineering, University of Cambridge, CambridgeCB2 1PZU.K.
Ali Mousavi
Systems Engineering Research Group, Brunel University, UxbridgeUB8 3PHU.K.
P. John Clarkson
Terry young.
Department of Computer Science and Mathematics, Brunel University, UxbridgeUB8 3PHU.K.
This paper investigates the connection between patient satisfaction, waiting time, staff satisfaction, and service time. It uses a variety of models to enable improvement against experiential and operational health service goals. Patient satisfaction levels are estimated using a model based on waiting (waiting times). Staff satisfaction levels are estimated using a model based on the time spent with patients (service time). An integrated model of patient and staff satisfaction, the effective satisfaction level model, is then proposed (using queuing theory). This links patient satisfaction, waiting time, staff satisfaction, and service time, connecting two important concepts, namely, experience and efficiency in care delivery and leading to a more holistic approach in designing and managing health services. The proposed model will enable healthcare systems analysts to objectively and directly relate elements of service quality to capacity planning. Moreover, as an instrument used jointly by healthcare commissioners and providers, it affords the prospect of better resource allocation.
1. Healthcare provision may be considered as involving the patient side of care and the staff side of care. 2. Analytical models exist for modelling patient or customer satisfaction. 3. We develop an analytical model for understanding staff satisfaction. 4. We propose an integrated model of patient and staff satisfaction by superimposing the staff satisfaction on top of patient satisfaction to determine the Effective Satisfaction Level (ESL) using Queuing Theory.

I. Introduction
Patients’ satisfaction is generally accepted as a key indicator of the quality of care [1] – , [3] . Patients’ waiting time is also considered as one measure of access to healthcare [4] , [5] . In Great Britain and other developed countries, the goal in recent years has been to focus on improvements in patient experience often through setting challenging targets for healthcare providers [6] , [7] . This practice of setting targets for healthcare providers especially in the UK has led to some improvement in care but also some unexpected practices on the part of providers that result in bad experiences for the patient [8] , [9] . For instance, several inappropriate practices by National Health Service (NHS) Trusts in England as a result of performance targets have been found. These include, staff making changes to the records of thousands of patients in other to meet the target, ambulance Trusts were found to have corrected response times in order to meet Government target of 8 minutes and in some cases patients are held in ambulances outside Accident and Emergency departments until staff are confident of meeting the four hour target [8] – , [12] . This evidence provided the main motivation for the current work. The starting question was this – “why will healthcare professionals trained to provide the best care for patients engage in practices that are harmful to the patients?” From the perspective of queuing theory, and by the consideration of Little’s Law [13] , the authors identified three key variables of the problem that are very closely linked - Waiting time, Service time (or service rate) and Number in system. If these are so closely linked, then it may be expected that setting targets for waiting time - the patient side of care, may have indirect consequences for service rate – on the staff side of care - if nothing is done about the number in system. The authors, therefore, reasoned that the ideal of shaping healthcare provision around patients’ needs and preferences [14] must recognize the demand this places on staff resources [15] and the risk to quality when staff are stressed [16] – , [18] . This led to the particular interest in service time (or the time staff spend with patients) in the current paper. A recent study has shown that in some situations much needed care is left undone due to staff not having enough time for tasks [15] . This recognition creates a triplet of challenges to those in service provision, namely to optimize patient satisfaction, staff satisfaction and service efficiency. The need to understand the interaction between patients’ needs, staff needs and service efficiency (or service quality) has been recognized for many decades. For instance, Donabedian [19] in his 1966 seminal paper on healthcare quality underscored this need by stating that “…before one can make judgments about quality, one needs to understand how patients and physicians interact and how physicians function in the process of providing care.” More recently, Oliva & Sterman [20] in a study in the service sector found that employees will reduce the time spent with customers and/or work longer hours to meet throughput targets. Furthermore, they noticed that in the absence of an understanding of the interaction between customers, staff and quality, management often interprets this reduction in time spent with customers as a productivity gain, leading to even tighter performance targets and eventually an unintended “erosion of service quality” . The challenge of understanding this interaction between patients’ needs, staff needs and service efficiency is the motivation for this paper.
To address this challenge, the paper reports on an approach that adds new methods to established ways of modelling healthcare processes. The contribution of the paper lies both in the integrated solution (Effective Satisfaction Level (ESL) model) proposed and the staff satisfaction model developed. This paper attempts to introduce the concept of Queuing Theory into the challenge presented above. This focuses the problem on patients’ satisfaction with waiting time and staff satisfaction with service time.
The paper first presents previous research involving traditional applications of Queuing Theory in Healthcare and customer satisfaction research with its application to patient satisfaction with waiting time. Secondly, the paper argues that despite significant work on staff satisfaction, no model exists that explains staff satisfaction behavior with service time and reports an empirical work that fills this gap. Thirdly, the proposed Effective Satisfaction Level (ESL) model, believed to be the best trade-off between patients and staff needs, is presented before finally finishing with some discussion and conclusions.
Some terms are used in the paper which are important for understanding the concepts presented. These terms are defined as follows:
- • Satisfaction Level: The estimated value of the satisfaction of patients or staff based on their expected waiting times or ideal service times.
- • Total Satisfaction Curve (TSC): The graph of the weighted sum of patient satisfaction and staff satisfaction levels at a specific value of expected waiting time and ideal service time.
- • Total Satisfaction Level (TSL): A value of satisfaction on the TSC.
- • Effective Satisfaction Level (ESL): The maximum TSL on the optimum TSC.
- • Effective Operating Point (EOP): The operating point that corresponds to the ESL.
II. Previous Research
A. queuing theory: relationship between service time and waiting time.
The field of queuing theory originated in the early 1900s and is well established with applications in diverse areas including manufacturing, computing, telecommunication and healthcare [21] . Within healthcare, bed occupancy has received significant attention with two examples being cooper & Corcoran [22] and Gorunescu et al. [23] . Several researchers, however, have undertaken to survey the breadth of the application of queuing theory in healthcare [24] – , [26] . Of interest to the current study is the finding of a more recent survey by Fomundam & Herrmann [27] . The researchers found a diversity of application of queuing theory in healthcare including waiting time and utilization analysis, system design, appointment systems and system sizing. They, however, concluded that performance targets imposed on healthcare services are only likely to lead to congestions and poor quality of service and are unlikely to be a successful approach to containing and reducing healthcare costs. This finding and those of Ball et al. [15] of valuable care being left undone by nurses on wards make the need to better understand the symbiotic relationship between the patient side of care and the staff side of care urgent. This paper seeks to provide one way of looking at this problem.
Figure 1 is an illustration of a simple healthcare delivery process with a single server (a doctor) and a single queue with patients. This and figures 2 , ,3, 3 , ,6 6 and and7 7 were developed by the authors to help present the concepts in this paper in a clear and logical manner. The discussions in the rest of this paper will be developed around this basic illustration of the healthcare process.

An illustration of a basic healthcare process showing a single doctor serving a single queue of patients.

Behavior of patient satisfaction with waiting time.

Behavior of staff satisfaction with service time.

Conceptual representation of the ESL concept.

Hypothetical illustration of the effective satisfaction Level (ESL).
The figure distinguishes the patient side of care from the staff side of care and indicates that Queuing Theory provides well established methods for understanding these two sides in terms of the relationship between waiting time and service time [28] . A summary of this relationship for a basic queuing discipline employed in this paper is presented in appendix A .
Focusing on the patient side of care, the paper will next provide a summary of previous work into measuring patient satisfaction and its application to waiting time.
B. Measuring Patients’ Satisfaction Levels
Satisfaction research in healthcare has focused predominantly on patients [29] – , [33] , although there is background in customer and employee satisfaction [34] – , [36] . The most popular method has been through surveys of patients. Analytically, the expectancy disconfirmation model [37] , first proposed by Anderson [38] and confirmed by Oliver [39] is well attested and, critically, provides a useful connection to waiting and service times. Anderson notes that the level of satisfaction or dissatisfaction depends upon the users’ expectations of what they will receive and their perception of what they have received. For a review, see [40] .
Meanwhile, Kahneman & Tversky [41] with their Prospect Theory, argue that people generally determine value by the changes in wealth or wellbeing, rather than the final states. This idea, combined with the expectancy disconfirmation model [39] , has led to the development of analytical models for calculating satisfaction [42] and a better understanding of the behavior of patient satisfaction with waiting time as shown in figure 2 . The curve shown in figure 2 is often approximated to a hyperbolic tangent function with a number of modified parameters for modelling purposes as shown in Mousavi et al. [42] .
It is important to note that this paper focuses on patient satisfaction with waiting because waiting time is one of the key factors in care delivery but not the only one. It is also known that waiting time is one of the measures of access to healthcare [4] , [5] . It is hoped that further research on the subject will lead to models that incorporate multiple factors.
III. Measuring Healthcare Staff Satisfaction Levels
Unlike the patient side of care, the authors could not find any analytical model in the literature for calculating the satisfaction of staff with service time. It is not claimed here that service time, considered the time staff spend with patients, is the only determinant of their satisfaction. It is known that factors such as supervisory support, pay, development opportunities, work environment and many more also affect staff job satisfaction in healthcare [43] , [44] . The choice of service time, was influenced by the observations of the effects of waiting time targets that motivated the current research. Several inappropriate practices have been found in the English health service that included leaving patient waiting in ambulances until staff were confident of meeting waiting time targets [8] – , [12] . The empirical research was therefore designed around investigating and modelling the nature of staff satisfaction with service time. The authors acknowledge that a simpler model could have been developed in terms of the utilization factor, which is the ratio of arrival rate to service rate for a single server system [28] , but admit that the current form of the model is constrained by the initial design of the research. Furthermore, the authors have learnt from experience working in healthcare that most healthcare practitioners will not be familiar with the concept of the utilization factor but are more likely to understand service time as the time they spend with patients. This is also supported by our data collection process in which we interviewed 68 doctors and nurses in two Accident and Emergency departments and found they were pleased to communicate in terms of service time. As a starting point, a hypothetical behavior was considered as shown in figure 3 . The goal here is to connect staff satisfaction with service time through an empirical study and thus to fill a gap in the literature.
The study began by selecting a double hyperbolic tangent function, similar to that already proposed for customer satisfaction [42] and fitted empirical staff satisfaction findings to it. The double hyperbolic tangent function was employed rather than a single hyperbolic tangent function because it was hypothesized, as in figure 3 , that staff satisfaction would decline if staff were rushed, but also if they were impeded in the timely discharge of their duties. The data collection methods and model development are discussed in the following section.
A. Data Collection and Model Development
At the time of this research, a major evaluation project around Accident and Emergency involving a satisfaction survey was already underway, using a questionnaire with a five point Likert scale. A subset of these questions were initially used but this only showed how satisfaction rose or fell with longer or shorter service times. Figure 4 summarizes the empirical data from the semi-structured interview with 68 doctors and nurses in two Accident and Emergency departments in London. It can be seen that the majority of staff are either dissatisfied or very dissatisfied when they spend less time with patients than what they thought was ideal. A significant number also were neutral, dissatisfied or very dissatisfied if they spent more than the ideal time.

Summary of data from staff satisfaction interviews showing satisfaction with service time less or more than ideal service time.
Staff were further interviewed in order to probe their satisfaction with service time in more detail. In each interview a service time norm was established for three stages of the Accident and Emergency (A&E) pathway (triage, first assessment, treatment) and staff members were then asked how their sense of satisfaction changed at five, fifteen and twenty minutes away from that norm on either side. This data is the basis for the staff satisfaction behavior model shown in figure 5 . In all, 68 doctors and nurses in two accident and emergency departments were interviewed.

Behavior of staff satisfaction with service time based on empirical data.
The best-fit analysis on the empirical data was conducted using the MATLAB curve fitting toolbox and the result is as shown in figure 5 and the most representative model for staff satisfaction is given by equation 1
With this model it became possible to connect staff satisfaction with service time with patient satisfaction with waiting time and develop the concept of the Effective Satisfaction Level (ESL).
IV. Determining the Effective Satisfaction Level (ESL)
Finally, the Queuing Theory relationship between waiting time and service time, patient satisfaction with waiting time and staff satisfaction with service time are integrated to develop the concept of the Effective Satisfaction Level (ESL). Figure 6 shows the various aspects put together.
Operating at point “OP 1 ”, in the direction of arrow “A” in Figure 7 , (to the left of the EOP) means staff are taking longer than they expect for their processes and hence queues will increase and result in lower values of patient and staff satisfaction and therefore, a lower value of Total Satisfaction Level (TSL 1 ). Similarly, operating at point “OP 2 ”, in the direction of arrow “B” (to the right of the EOP) means staff are working faster than they would normally like to do. This will potentially reduce patient waiting time in the queue and therefore increase their satisfaction, but the decrease in staff satisfaction will result in a decrease in total satisfaction level to TSL 2 . It may be necessary at times to maximize patient satisfaction in this way but this may require a shared understanding in a staff team to be effective. In this case we may assume that the encounter is effective and leads to patient satisfaction, but this has not been included in our modelled.
A. Experimental Results
The MATLAB software was used to implement the queuing models presented in equations 2 through 4 of appendix A together with the patient satisfaction model discussed in section IIB and the staff satisfaction model developed in section III . This allowed us to test the hypothetical behavior illustrated in figure 7 above and also the relationship between patient satisfaction with waiting time and staff satisfaction with service time. Figures 8 through 10 demonstrate how the Effective Satisfaction Level (ESL) may be found.

Variations in Patient Satisfaction with Staff Satisfaction.
Figure 8 shows one result from the implementation which reveals how patient satisfaction with waiting time varies with staff satisfaction with service time. The figure shows three curves for which the expected patients’ waiting times are 0.5hrs, 2hrs and 3hrs respectively. In each case the ideal service time is 2hrs. As expected, if the patient expectation is 0.5hrs, there is no way to satisfy both patients and staff. However, if patients expect 2hrs, then there is a solution where both patients and staff are close to maximum. The reason the curve does not quite go through the point (1, 1) is because the model rewards shorter-than-expected delays with slightly higher levels of patient satisfaction than that corresponding to the expected delay. A patient would be happy to leave after two hours, but a little happier to leave before that. This becomes clear if patients expect to wait 3hrs and get through in 2.
Plot of patient and staff satisfaction against waiting time ratio.
Similarly, S 2 and P 2 are the staff and patient satisfaction curves at an expected waiting time of 2hrs. It is observed that in this case, maximum staff satisfaction occurs at point B which is much closer to the maximum patient satisfaction than point A. Also the difference between staff satisfaction and patient satisfaction levels is almost negligible. Note that ideal service time is fixed at 2hrs. The ESL (see figure 7 ) may, therefore, be expected to occur in the vicinity of point B, where staff satisfaction and patient satisfaction are at or near their maximum. This shows that the model has the potential to accurately predict what may be expected from doctors and nurses for a desired level of patient experience.
It is suggested that the ESL must be the goal of most healthcare systems. Even when capacity constraints make it difficult for a system to operate at the ESL, it may still be desirable to know how far the system is from its ESL. This concept uniquely provides a meaningful method for assessing the capability of a healthcare system to examine the validity of any arbitrary target.
The introduction of the ESL provides numerous opportunities for exploring and better understanding the queuing problem in a healthcare system. Examples of questions that may be explored are: 1. What is the optimum level of resources required for a system to operate at the ESL? 2. What level of demand can a system accept without moving off the ESL? Or, 3. What is an acceptable number of patients waiting in a queue at the ESL?
Figure 10 further shows the Total Satisfaction Curve (TSC) by which the ESL is identified. TSC 1 is the resulting total satisfaction curve when the expected waiting time is 0.5hrs whilst TSC 2 is the total satisfaction curve when the expected waiting time is 2.0hrs. Other TSCs are shown for various values of expected waiting time. The curves show that the maximum value of the total satisfaction of staff and patient is higher when the expected waiting time is 2.0hrs than when it is 0.5 hrs. This point of maximum total satisfaction, therefore, corresponds to high values of both staff satisfaction and patient satisfaction and is what we call the Effective Satisfaction Level (ESL).

Variations in total satisfaction curves (TSC).
V. Discussion and Conclusions
It has been found that the approach to managing queues in a healthcare system through arbitrary targets and performance ratings is problematic [8] , [10] . The authors argue that in the management of queues in the British National Health Service, significant emphasis is placed on satisfying patients without understanding the implications on the staff that are the key resources in the system. This paper has focused on showing the link that exists between the service time of staff and the waiting time of patients and hence between the satisfaction of staff with service time and patient satisfaction with waiting time.
Based on empirical data, a model for estimating the satisfaction of staff with service time was developed. The development of this model made it possible to directly relate the satisfaction of staff to that of patients. The Effective Satisfaction Level (ESL) was developed by analytically relating the satisfaction of staff to that of patients. Through the concept of the ESL we present an argument that a synergy between patient satisfaction and staff satisfaction is the key to sustainable improvement in healthcare quality because it is a more transparent approach. This paper, therefore, suggests that most healthcare systems must ideally operate at the ESL and where this is not possible due to resource constraints, it is still important to know how far a system is from its ESL. The results have shown that the ESL occurs when the ideal service time is close to or equal to the actual service time with the corresponding actual waiting time also close to or equal to the expected waiting time. This means that there is the potential to be able to accurately predict what may be expected from doctors and nurses for a desired level of patient experience.
A. Assumptions, Limitations and Further Work
A number of assumptions were made in the application of queuing theory in this paper:
- 1. The entire A&E system has been considered as a single server and a single queue (M/G/1) system [28] . As a future work, a more complex (M/G/n) will be studied.
- 2. It was also assumed that the time between patient inter-arrival times into the A&E system is random and exponentially distributed.
- 3. The service times were also assumed to be random and follow any general distribution.
- 4. Patients in queue were also assumed to be served on a First Come First Served (FCFS) basis.
- 5. For simplicity, other factors such as communication, cleanliness, dignity and access to care which may influence satisfaction, were not included at present.
A number of limitations may be identified with regard to this study which also provide opportunities for further work. Firstly, one may argue that the satisfaction of patients is not just about waiting time. Whilst we admit the truth of this argument, we believe that most of the factors that affect patient experience and eventually the quality of care such as communication, confidence in staff, dignity, access to care and cleanliness may also be time related [15] . Hence a study of the relationships of such factors with staff service times planned as the future direction of this research may facilitate the inclusion of multiple factors and eventually the development of a more unifying satisfaction model.
Thirdly, the assumptions listed above show that the mathematical formulation is not a perfect representation of a real A&E system. However, our goal at this stage is to provide a quantitative explanation to a seemingly obvious phenomenon. The importance of this approach is that it enables us to more easily control the parameters that influence the phenomenon or the interaction between staff and patients. This concept, as with every scientific theory, requires further research and enhancement. We consider that an exploration of the extension of this concept to a service system as a network of queues is a logical next step.
B. Implications
The understanding of the relationship between the satisfaction of staff and that of patients will lead to more realistic expectations of healthcare systems and their performance or non-performance. It should be possible to know the extent to which increases in the satisfaction of patients by the reduction of waiting times may be pursued in a system of limited resources.
There should also be no more need for healthcare managers to employ “coping” methods [8] , [10] , [47] , since any unrealistic expectations should be detected by the proposed model.
It is finally emphasized that a healthcare system may ideally operate at the ESL and where this is not possible due to resource constraints, it is still important to know how far a system is from its ESL and how much resource is required to move it there.
Acknowledgment
This research was also supported by the National Institute for Health Research (NIHR) Collaboration for Leadership in Applied Health Research and Care East of England (CLAHRC EoE) at Cambridge and Peterborough NHS Foundation Trust. We also give special thanks to the following persons for their various contributions to this work: Justin Gore for being a co-supervisor of the project; Professors Lorraine De Souza of Brunel University and Janet Smart of Oxford University for their many constructive critiques of the initial work. The views expressed are those of the authors and not necessarily those of the NHS, the NIHR or the Department of Health.
Biographies
Alexander Komashie received the B.Sc. degree in mechanical engineering from the Kwame Nkrumah University of Science and Technology, Kumasi, Ghana, and the M.Sc. degree in advanced manufacturing systems and the Ph.D. degree in systems engineering from Brunel University, London, U.K. He is currently with Wycliffe Hall College, Oxford University, and affiliated to the Engineering Design Centre, Cambridge University, where he was a Post-Doctoral Researcher. His research interests are in the application of queuing theory, discrete event simulation, design process, complexity, and systems thinking for improving healthcare delivery.
Ali Mousavi received the Ph.D. degree. He is currently a Senior Lecturer and the Head of the Systems Engineering Research Group with Brunel University. His research interest is in mathematical modeling and simulation, applied control, and computing.
P. John Clarkson received the Ph.D. degree. He returned to the Engineering Department, Cambridge University, U.K., in 1995, following a seven-year spell with PA Consulting Group’s Technology Division, where he was the Manager of the Advanced Process Group. He was appointed as the Director of the Engineering Design Centre in 1997 and a University Professor in 2004. He is currently a Professor of Engineering Design and the Director of the Engineering Design Centre with the Engineering Department, Cambridge University. He is directly involved in the teaching of design at all levels of the undergraduate course. His research interests are in the general area of engineering design, in particular, the development of design methodologies to address specific design issues, for example, process management, change management, healthcare design, and inclusive design.
Appendix A. Queuing Theory Relationship Between Waiting Time and Service Time
Queuing theory is a well-established field of research that has been applied to many systems including healthcare. Several queuing models exist, from single stations to complex networks of queues implementing several queuing disciplines. In this paper we employ one of the most basic queuing models – M/G/1 in order to demonstrate how patient satisfaction with waiting time may be connected to staff satisfaction with service time. These models often involve a set of assumptions, known parameters, unknown parameters and analytical solutions to some of the standard models as briefly discussed below.
A. The Initial System Assumptions
We assume a healthcare system with only one doctor/nurse serving a single queue of patients. The queuing discipline is First Come First Serviced (FCFS) - this may be extended to cover a priority based queues. The arrival process is a Poisson distribution therefore inter-arrival times are exponentially distributed [48] . Service time is any general distribution (triangular in this case).
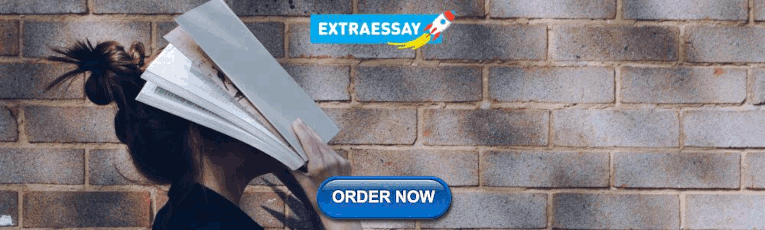
B. Known Parameters
The following parameters are known for the above system;
Number of servers = 1
C. Unknown Parameters
According to Little’s Law [13] , which is the basis of the following analysis, there exists a definite relationship between work-in-process, production rate and throughput time. The statement of the law is as follows:
D. M/G/1, FCFS (Exponential Inter-Arrival, any General Service Distribution, 1 Server, With a First Come First Served Queuexs)
The M/G/1 model is a standard model in the field of queuing theory for which a standard set of solutions exist [28] , [45] . The following standard solutions were employed in this study:
Estimated total time of patients,
Also, estimated total number of patients in system,
therefore, the expect value of the actual waiting time may be simply determined as:
Appendix B. Staff Satisfactioin Model
In order to be able to connect patient satisfaction with waiting time with staff satisfaction with service time, it was necessary to develop an analytical model of the behavior of staff satisfaction with service time since there was nothing of the kind in the literature.
Mathematically, we started by formulating the staff satisfaction model as a double hyperbolic function following the concept of customer satisfaction in Mousavi et al (2001) as follows;
The empirical data collected by interviewing 68 doctors and nurses in two Accident and Emergency departments in London was fitted to this model in order to determine the value of the constants. The result has been discussed in the paper in section III .
Funding Statement
This work was supported by the North West London Hospitals National Health Service Trust through a Ph.D. Fellowship.
Research on Optimization of Queuing System Based on Computer Simulation
Ieee account.
- Change Username/Password
- Update Address
Purchase Details
- Payment Options
- Order History
- View Purchased Documents
Profile Information
- Communications Preferences
- Profession and Education
- Technical Interests
- US & Canada: +1 800 678 4333
- Worldwide: +1 732 981 0060
- Contact & Support
- About IEEE Xplore
- Accessibility
- Terms of Use
- Nondiscrimination Policy
- Privacy & Opting Out of Cookies
A not-for-profit organization, IEEE is the world's largest technical professional organization dedicated to advancing technology for the benefit of humanity. © Copyright 2024 IEEE - All rights reserved. Use of this web site signifies your agreement to the terms and conditions.
Application of queuing theory in production-inventory optimization
- Original Research
- Open access
- Published: 04 July 2015
- Volume 11 , pages 485–494, ( 2015 )
Cite this article
You have full access to this open access article
- Reza Rashid 1 ,
- Seyed Farzad Hoseini 1 ,
- M. R. Gholamian 1 &
- Mohammad Feizabadi 2
16k Accesses
10 Citations
Explore all metrics
This paper presents a mathematical model for an inventory control system in which customers’ demands and suppliers’ service time are considered as stochastic parameters. The proposed problem is solved through queuing theory for a single item. In this case, transitional probabilities are calculated in steady state. Afterward, the model is extended to the case of multi-item inventory systems. Then, to deal with the complexity of this problem, a new heuristic algorithm is developed. Finally, the presented bi-level inventory-queuing model is implemented as a case study in Electroestil Company.
Similar content being viewed by others
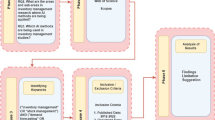
Applications of Artificial Intelligence in Inventory Management: A Systematic Review of the Literature
Özge Albayrak Ünal, Burak Erkayman & Bilal Usanmaz
Optimization of Inventory Management: A Literature Review
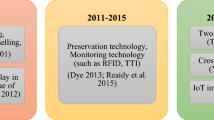
Internet of things for perishable inventory management systems: an application and managerial insights for micro, small and medium enterprises
Pratik Maheshwari, Sachin Kamble, … Sunil Tiwari
Avoid common mistakes on your manuscript.
Introduction
Nowadays, supply chains play an important role to meet diverse needs of customers. A supply chain can be defined as a network of organizations that collaborate to control and manage materials and information flow from suppliers to customers (Aitken 1998 ). One of the challenging issues in supply chain management is to find optimal policies for inventory systems. The main objective of inventory management is to balance conflicting goals such as stock costs and shortage costs (Arda and Hennet 2006 ).
Day by day, the number of researchers, attracted to production-inventory systems in supply chain, is increasing. In inventory management point of view, a manufacturer with a limited production capacity needs to hold finished goods inventory as safety stocks. For this inventory system, if demand is less than service capacity, the manufacturer needs to use different policies for different inventory levels.
For instance, it should use two points of inventory level: one for starting and the other for finishing the production line. For better description of the model, Fig. 1 illustrates the inventory level for the system when production rate μ and demand rate D are considered deterministic. Actually, in a real-world system, different stochastic parameters may affect the model and increase the complexity of the system.
Inventory level of the production model
In summary, it is clear that in spite of many contributions to the stock control, there is little consideration regarding production-inventory models in stochastic environments. For this reason, we represent a mathematical model in which the main contributions of this paper can be summarized as follows:
A production inventory system is developed in an uncertain environment.
Queuing theory is used to provide a stochastic model.
The proposed model is extended for multi-item inventory systems.
A new heuristic algorithm is proposed to solve the model.
All the steady-state equations are solved in one state.
This model can be probably applied to inventory systems where demand and production time are uncertain. Company has setup costs and it also holds finish goods inventory.
The remainder of this paper is organized as follows. In Sect. 2 , a brief literature review is presented. In Sect. 3 , an inventory model is presented and then a branch and bound algorithm is proposed. Afterward, the model is validated in Sect. 4 . Then, conclusions are made in Sect. 5 .
Literature review
In the literature, there are some researchers who have dealt with production-inventory systems. For example, Yu and Dong ( 2014 ) considered a two-stage production lot sizing problem which used an inventory system with random demand arrivals. To solve the problem, they proposed a numerical approach for the problem. Baek and Moon ( 2014 ) considered a lost sales production-inventory system in an uncertain environment. They also used queuing theory to present a stochastic model for the system. Also, some production-inventory papers in the literature assumed that the lead time is negligible or it can be ignored in practice when it is short in contrast to other time factors (Karimi-Nasab and Seyedhoseini 2013 ). Most of such research papers did not consider lost sales. Many of them, however, considered back orders as a rational managerial policy.
Chang and Lu ( 2011 ) considered a serial production system controlled by the base-stock policy. They presented a phase-type approximation for a controlled base-stock serial production system. They also proposed a cost model to determine the optimal base-stock level.
Jewkes and Alfa ( 2009 ) considered a production system in which a supplier produces semi-finished items on a make-to-stock basis for a manufacturer that customizes the items on a make-to-order basis. The manufacturer attempts to determine the optimal point of differentiation and its optimal semi-finished goods buffer size. They used matrix geometric methods to evaluate performance through various measures for this system.
Some researchers worked on multi-item inventory systems. For instance, Shavandi et al. ( 2012 ) proposed a new constrained multi-item pricing and inventory model. They covered three categories of perishable products in their model. Taleizadeh et al. ( 2012 ) dealt with a multi-product inventory control problem in which periods between two replenishments were assumed to be independent random variables.
Generally, in a stock control system, most of the parameters are not deterministic. Thus, some researchers used queuing theory to construct their models. In this area, there are some researchers who provided a model for stochastic demand. Some researchers presented a model for stochastic lead time. Parlar ( 1997 ) presented an inventory model which was combined with queuing theory to consider demand and lead time as stochastic parameters. Ha ( 1997 ) considered Poisson distribution for demands and exponential for production times in a single item make-to-stock production system. He proposed an M / M /1/ S queuing system for modeling the system. Arda and Hennet ( 2006 ) addressed inventory control of a multi-supplier strategy in a two-level supply chain. They considered random arrivals for customers and random delivery time for suppliers and represented the system as a queuing network.
Sapna Isotupa ( 2006 ) considered lost sales of ( s , Q ) inventory system with two customer groups and illustrated the Markov processes. Boute et al. ( 2007 ), in a two-echelon supply chain, show that by including the impact of the order decision on lead times, the order pattern can be smoothed to a considerable extent without increasing stock levels. Karimi-Nasab and Konstantaras ( 2013 ) considered special sales offer for a single item from the supplier under stochastic replenishment intervals.
Jain and Raghavan ( 2009 ) studied batch ordering in multi-echelon supply chains and used queuing theory to capture the behavior of the manufacturing supply chain network. Babai et al. ( 2011 ) investigated stochastic demand and lead time and analyzed a single item inventory system through queuing theory. Bahri and Tarokh ( 2012 ) assumed that the delivery lead time is stochastic and follows an exponential distribution. Also, the shortage during the lead time is permitted and completely back ordered for the buyer.
Seyedhoseini et al. ( 2014 ) considered Poisson demand for customer in a cross docking problem. They employed queuing theory to provide a stochastic model. Salameh et al. ( 2014 ) combined the separate works on substitution and joint replenishment and introduced a solution procedure for solving the joint replenishment model with substitution for two products within the framework of the classical economic order quantity model. Karimi-Nasab and Sabri-Laghaie ( 2014 ) extended classical economic production quantity model to the case of stochastically generated poor-quality items, while an imperfect screening scheme was devised to recognize such items from healthy ones.
Krommyda et al. ( 2015 ) studied an inventory control problem in which demand was satisfied using two mutually substitutable products. Their aim was to determine the order quantity for each product that maximizes the joint profit function.
Seyedhoseini et al. ( 2015 ) applied queuing theory to propose a mathematical model for inventory systems with substitute flexibility. (Rashid et al. 2015 ) also considered a location-inventory model. To prepare a stochastic inventory model, they used bi-level Markov process. Considering the effectiveness of queuing theory in inventory problems, we have also used queuing theory to develop a stochastic stock control model.
As demonstrated in Table 1 , there are some researchers who considered ( R , Q ) policy in stochastic programming. However, some of them did not consider multi-item inventory, and some of them did not consider production inventory. Also, few researchers have analyzed their model with queuing theory. Hence, we propose a mathematical model for the production-inventory problem in the stochastic environment.
Model development
In this section, first, the proposed model is developed. Then, it is extended for the multi-item production-inventory system. Afterward, a new algorithm is proposed to solve the model. As for notifications, parameters are described as follows:
Model description
In this paper, a historical production-inventory model is developed. Moreover, a new stochastic multi-item production-inventory model in which shortages have been considered lost sales is proposed. In this strategy, the production cycle starts when the manufacturer’s inventory falls to or below the reorder point r and stops when its on-hand inventory reaches its optimal level R .
Inspired by Sapna Isotupa ( 2006 ) who developed a stochastic inventory model for ( R , Q ) systems, we also developed a new stochastic model for the historical production-inventory model. Thus, the customers’ arrival is assumed to be Poisson distribution with rate of D , and production time is negative exponentially distribution with rate of μ . Also, it has been assumed that if one type of products is not under production, its production capacity would equally divide to the other products. To achieve a comprehensive model, queuing theory is employed to describe the inventory system. The transition diagram for this model is illustrated in Fig. 2 .
Transition diagram for a product in the proposed model
For this diagram, states of the system have been decomposed into two sets: A and B . In set A , the system is in production mode and in set B system in demand mode; in demand mode, no production would occur. Also, states of the system are represented by ( I , J ), where I ∊ {0, 1, …, R } demonstrates the level of inventory and J ∊ { P , D } demonstrates the mode of the system. Henceforth, let π i , j denote the steady-state probability of state ( I , J ). For this queue, three lemmas are proposed to set steady-state probabilities.
Steady-state probabilities in terms of state \(\pi_{R - 1,P}\) for the system can be calculated as follows:
For a Markov process, Eq. 5 is true:
where N is the number of all steady states, and λ jx is the probability rate from state j to state x .
Regarding Fig. 1 and Eq. 5 , Eq. 1 is true. Using Eq. 1 , for L between 3 and R − r , Eq. 6 could emerge, which proves Eq. 2 ,
When I is smaller than r , then Eq. 7 is true:
Using Eqs. 2 and 7 could result in Eq. 3 . As for set B, it is clear that the values of steady-state probabilities are equal and also Eq. 8 is true:
So, Eq. 4 is true.Considering Lemma 1, π R −1, P is equal to \(\frac{1}{F}\) , where F can be calculated through Eq. 9 :
The expected value for shortage can be computed through the following equation:
The shortage occurs when the producer has no inventory and also a demand is announced to the retailer. In this section, g is used for representing a state of shortage. For computing the expected value of shortage, we decompose state (0, P ) as in Fig. 3 .
For this queue, if \(\pi_{g}^{s}\) represents a steady-state probability of g shortages, then it would be clear that:
Considering Eq. 11 , \(\pi_{g}^{s}\) could be calculated as follows:
By considering Eq. 12 , Eq. 13 could be obtained as
Also, the average shortage is equal to:
where z is equal to \(\frac{D}{D + \mu }\) . In addition, it is known that:
Considering Eqs. 14 and 15 could result in Eq. 10 .
Transition diagram for state (0, p)
For this inventory system, the expected value of the inventory level can be calculated by A.π R-1,P , where A can be calculated by Eq. 16 .
For this inventory system, Eq. 17 is true:
Also, for this queue the next three equations are true:
Using Eqs. 17 , 18 , 19 , and 20 , Eq. 16 can emerge.
Multi-product inventory model
In this section, we considered multi-item inventory to determine near optimal R i , r i and μ i for each product. This objective is denoted through minimizing Eq. 21 :
For the inventory system, M types of products are considered. To present our heuristic algorithm, it is assumed that on demand mood the product has a capacity (production rate) that can be equally divided into the other products. So, Eq. 20 could be used to approximate the service rate for the type k product:
where n represents the number of products and p l is equal to \(\sum_{i = 0}^{R - 1} \pi_{i,P,l}\) , which represents the probability of being in production mood for product l . It can be calculated from Eq. 23 . In Eq. 22 , \(\sum _{l \ne k} \left( {1 - p_{l} } \right)\) approximates the number of products in the demand mood and \(\sum _{l \ne k} \left( {p_{l} } \right)\) approximates the number of products in the production mood.
Figure 4 illustrates the inventory level, rate of production and rate of demand for the two products.
Illustration of the inventory level for two products
In this study, μ i is considered to be bigger than D i . For fixed values of different variables, it is clear that increase in R i causes an increase in \(\bar{I}_{i}\) . Furthermore, it causes \(\pi_{r + 1,D,i}\) and \(\bar{S}_{i}\) to be decreased. So, for fixed values of different variables, the long-run expected cost is pseudo-convex in R .
On the other hand, for fixed values of different variables, increase in r i would increase the probabilities of states which have more than \(r_{i}\) inventory level, and it causes \(\pi_{r + 1,D,i}\) and \(\bar{I}_{i}\) to be increased. Moreover, it causes a decrease in \(\bar{S}_{i}\) . Consequently, for a fixed R , the long-run expected cost is pseudo-convex in r i .
- Heuristic algorithm
Regarding the complexity of our model, simple models would take big computational times. For this reason, we proposed a two-phased algorithm.
In continuous space, z is convex due to r i and R i . We suppose r i and R i to be continuous and optimal and these were calculated by the steepest ascent method. For better description, it is assumed that there is only one product and if r li and R li denote the solution of the steepest ascent method, then Fig. 5 demonstrates the optimal solution in discrete space for just one product.
Solution of the discrete problem
Considering Fig. 5 , for the discrete problem, the nearest points in any direction to the optimal solution could be found in a space in which r i is | r li | or | r li | + 1, and R i is | R li | or | R li | + 1. Consequently, a search among these nodes can provide a perfect local solution. Assuming a number of product equal to M , there would be 2 2 M nodes in the space that needs to be searched. So, for big M , phase two is proposed.
Considering the optimal solution resulting from the steepest deepest algorithm, we proceed with the following steps:
Step 1 Calculate μ i for each of the products for the continuous solution.
Step 2 For each product, search the nodes where | r li | or | r li | + 1, and R i is | R li | or | R li | + 1, and then calculate Eq. 24 , independently,
Step 3 Choose the nodes which have the minimum z i for each product.
Computational results
In this research, the proposed algorithm is coded in C++ software. For evaluation of the algorithm, we produced five examples for M equal to 10, where all parameters are selected randomly while creating the data set. The parameters are set as follows: \(C_{i,I} \in \left[ {1,10} \right]\) , C i , S ∊ [1, 10], D i ∊ [1, 10], μ = 10 M and SE i ∊ [30, 60]. In this case, instead of letting R go to infinity, the maximum value of R was set to be 10,000. Therefore, the maximum value of s was 9999. We also limited the computational time to 5 h and mean solving time for our algorithm was 223.097 s, while none of the examples could be solved through searching all feasible solutions.
In this model, an example is generated to analyze the parameters of the model in which C I , C S , D , SE and \(\mu\) are equal to 5, 20, 3, 200 and 4, respectively. Also, the behavior of inventory costs has been studied for different values of R and r in Fig. 6 .
Behavior of the model regarding r and R \(\left( {\varvec{r} < \varvec{R}} \right)\)
It is proposed to use this model for companies using workshop system and reorder point for replenishing their finished goods inventory. To provide a better description of the model, an example is presented in Table 2 .
A near optimal solution for this example is 164 where ( \(r_{1} , r_{2} ,r_{3} , R_{1} , R_{2} , R_{3}\) ) is equal to (1, 1, 1, 4, 3, 3), respectively. If each product is to be planned separately, the optimal cost would be equal to 211, 28 % more than our model’s costs. We considered Product 3 and analyzed costs related to D in Fig. 7 . To analyze the performance of our heuristic, R and Q are limited to be less than 100, and the optimal solution for different D s are prepared.
Sensitivity of different costs due to the demand rate
As in Fig. 7 , the same pattern has been demonstrated for shortage and holding costs. On the other hand, the pattern of setup cost is totally different. Furthermore, there is not a great difference among heuristic and optimal costs.
This model could be implemented properly for workshop systems. The Electroestil Company was established in 1980 and now it is one of the most popular brands of refrigerator production in Iran. Electroestil produces different kinds of refrigerators for houses and shops. It has 20 types of refrigerators for houses and 15 types for shops.
In this company, there are four workshops and 1500 employees. This company has a production capacity of 800 products per day for house refrigerators and 120 products per day for shop refrigerators. Experts in Electroestil approximate that demands of house refrigerators for different product types are equal and they are equal to 30 per day, and demands of shop refrigerators for different product types are equal and they are equal to 7 per day. They have defined C I to be equal to 100,000 tomans for each product, C S to 2 million tomans—Iran’s currency—for each product, and SE to 300,000 tomans for each product. For this company, Table 3 illustrates the performance of the proposed model. Significant changes in inventory costs have been determined if the proposed model is used.
As shown in this table, there is a big difference between the optimal solution and the current situation of the company. In the current situation, shortage costs and setup costs are small, but holding costs are big. For this company, the rate of production is much more than the rate of demand. This could be a reason for the low quantity of r .
In this model, the demand rate has significant effects on r and R . Therefore, we only considered ESR320 which is a shop refrigerator. For this product, R and r were analyzed according to the demand rate in Fig. 7 . The same pattern was displayed for them. Also, it has been discovered that as the demand rate becomes bigger, when it is bigger than the service rate, the optimal costs decrease. For example, the costs for D = 64 is equal to 30 which is significantly less than for D = 7. However, for small demand rates, its increase makes optimal costs bigger. For example, in D = 13, the optimal cost is equal to 302.
Conclusion and future directions
In this paper, a new production-inventory model is developed (Fig. 8 ). To prepare a cohesive model, demand and production time are considered as stochastic parameters and queuing theory is used to calculate long-run inventory costs. The proposed model also has been extended for multi-item inventory systems. The inventory model has been analyzed concerning r and R . These parameter behaviors are investigated. We also proposed a heuristic algorithm to solve the problem. The results demonstrate the efficiency of the proposed algorithm. To prepare a better description of the model, a real-world example is studied and analyzed. Respecting the collected data, it has been observed that there is a significant relation between the demand rate and production rate. If demand is smaller than service rate, increase of demand causes an increase in optimal costs. On the other hand, when it is bigger than the service rate, a small increase may reduce the optimal costs.
Relation between R , r and D for ESR320
Lost sales shortage may be considered as one of the weaknesses in our model. So, in future, the model can be extended through considering back-order shortage. This may increase the complexity of the problem while the model would become more realistic. Another extension of this research is possible by covering corruption rates for perishable inventories.
Aitken JM (1998) Supply chain integration within the context of a supplier association: case studies of four supplier associations. Cranfield University, UK
Google Scholar
Alimardani M, Jolai F, Rafiei H (2013) Bi-product inventory planning in a three-echelon supply chain with backordering, Poisson demand, and limited warehouse space. J Ind Eng Int 9:22
Article Google Scholar
Arda Y, Hennet J-C (2006) Inventory control in a multi-supplier system. Int J Prod Econ 104:249–259
Babai MZ, Jemai Z, Dallery Y (2011) Analysis of order-up-to-level inventory systems with compound Poisson demand. Eur J Oper Res 210:552–558
Article MATH MathSciNet Google Scholar
Baek JW, Moon SK (2014) The M/M/1 queue with a production-inventory system and lost sales. Appl Math Comput 233:534–544
Article MathSciNet Google Scholar
Bahri M, Tarokh MJ (2012) A seller-buyer supply chain model with exponential distribution lead time. J Ind Eng Int 8:1–7
Boute RN, Disney SM, Lambrecht MR, Van Houdt B (2007) An integrated production and inventory model to dampen upstream demand variability in the supply chain. Eur J Oper Res 178:121–142
Article MATH Google Scholar
Chang K-H, Lu Y-S (2011) Inventory management in a base-stock controlled serial production system with finite storage space. Math Comput Model 54:2750–2759
Guerrero W, Yeung T, Guéret C (2013) Joint-optimization of inventory policies on a multi-product multi-echelon pharmaceutical system with batching and ordering constraints. Eur J Oper Res 231:98–108
Ha AY (1997) Stock-rationing policy for a make-to-stock production system with two priority classes and backordering. Naval Res Logist 44:457–472
Hennet J-C, Arda Y (2008) Supply chain coordination: a game-theory approach. Eng Appl Artif Intell 21:399–405
Hill RM, Seifbarghy M, Smith DK (2007) A two-echelon inventory model with lost sales. Eur J Oper Res 69:753–766
Jain S, Raghavan NS (2009) A queuing approach for inventory planning with batch ordering in multi-echelon supply chains. Central Eur J Oper Res 17:95–110
Jewkes EM, Alfa AS (2009) A queueing model of delayed product differentiation. Eur J Oper Res 199:734–743
Karimi-Nasab M, Konstantaras I (2013) An inventory control model with stochastic review interval and special sale offer. Eur J Oper Res 227:81–87
Karimi-Nasab M, Sabri-Laghaie K (2014) Developing approximate algorithms for EPQ problem with process compressibility and random error in production/inspection. Int J Prod Res 52:2388–2421
Karimi-Nasab M, Seyedhoseini S (2013) Multi-level lot sizing and job shop scheduling with compressible process times: a cutting plane approach. Eur J Oper Res 231:598–616
Krommyda I, Skouri K, Konstantaras I (2015) Optimal ordering quantities for substitutable products with stock-dependent demand. Appl Math Model 39:147–164
Parlar M (1997) Continuous-review inventory problem with random supply interruptions. Eur J Oper Res 99:366–385
Rashid R, Bozorgi-Amiri A, Seyedhoseini S (2015) Developing a new stochastic competitive model regarding inventory and price. J Ind Eng Int, pp 1–10
Salameh MK, Yassine AA, Maddah B, Ghaddar L (2014) Joint replenishment model with substitution. Appl Math Model 38:3662–3671
Sapna Isotupa K (2006) An (s, Q) Markovian inventory system with lost sales and two demand classes. Math Comput Model 43:687–694
Seyedhoseini S, Rashid R, Teimoury E (2014) Developing a cross-docking network design model under uncertain environment. J Ind Eng Int, pp 1–12
Seyedhoseini S, Rashid R, Kamalpour I, Zangeneh E (2015) Application of queuing theory in inventory systems with substitution flexibility. J Ind Eng Int, pp 1–8
Shavandi H, Mahlooji H, Nosratian NE (2012) A constrained multi-product pricing and inventory control problem. Appl Soft Comput 12:2454–2461
Taleizadeh AA, Niaki STA, Seyedjavadi SMH (2012) Multi-product multi-chance-constraint stochastic inventory control problem with dynamic demand and partial back-ordering: a harmony search algorithm. J Manuf Syst 31:204–213
Tlili M, Moalla M, Campagne J-P (2012) The trans-shipment problem in a two-echelon, multi-location inventory system with lost sales. Int J Prod Res 50:3547–3559
Yu AJ, Dong Y (2014) A numerical solution for a two-stage production and inventory system with random demand arrivals. Comput Oper Res 44:13–21
Download references
Author information
Authors and affiliations.
Department of Industrial Engineering, Iran University of Science and Technology, Narmak, 16844, Tehran, Iran
Reza Rashid, Seyed Farzad Hoseini & M. R. Gholamian
Department of Industrial Engineering, Sharif University, Azady, Tehran, Iran
Mohammad Feizabadi
You can also search for this author in PubMed Google Scholar
Corresponding author
Correspondence to Seyed Farzad Hoseini .
Rights and permissions
Open Access This article is distributed under the terms of the Creative Commons Attribution 4.0 International License (http://creativecommons.org/licenses/by/4.0/), which permits unrestricted use, distribution, and reproduction in any medium, provided you give appropriate credit to the original author(s) and the source, provide a link to the Creative Commons license, and indicate if changes were made.
Reprints and permissions
About this article
Rashid, R., Hoseini, S.F., Gholamian, M.R. et al. Application of queuing theory in production-inventory optimization. J Ind Eng Int 11 , 485–494 (2015). https://doi.org/10.1007/s40092-015-0115-9
Download citation
Received : 04 December 2014
Accepted : 29 May 2015
Published : 04 July 2015
Issue Date : December 2015
DOI : https://doi.org/10.1007/s40092-015-0115-9
Share this article
Anyone you share the following link with will be able to read this content:
Sorry, a shareable link is not currently available for this article.
Provided by the Springer Nature SharedIt content-sharing initiative
- Production inventory
- Queuing theory
- Multi-item inventory
- Find a journal
- Publish with us
- Track your research
Academia.edu no longer supports Internet Explorer.
To browse Academia.edu and the wider internet faster and more securely, please take a few seconds to upgrade your browser .
- We're Hiring!
- Help Center
Queuing Model
- Most Cited Papers
- Most Downloaded Papers
- Newest Papers
- Save to Library
- Last »
Enter the email address you signed up with and we'll email you a reset link.
- Academia.edu Publishing
- We're Hiring!
- Help Center
- Find new research papers in:
- Health Sciences
- Earth Sciences
- Cognitive Science
- Mathematics
- Computer Science
- Academia ©2024
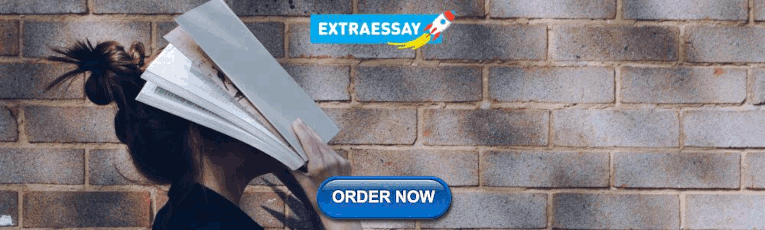
IMAGES
VIDEO
COMMENTS
Queueing theory, broadly speaking, concerns the design and analysis of resource-constrained systems in which customers need to potentially wait for access to a resource. This paradigm arises in many applied settings, ranging from service and health operations to communications and computer systems to ride-sharing and job-matching platforms to ...
Explore the latest full-text research PDFs, articles, conference papers, preprints and more on QUEUEING THEORY. ... The paper presents a proposed queuing model based on Kendall's notation for ...
In Thudiyalur, Gandhipuram, and Periyanaickenpalayam, all in Coimbatore, this research examines the usefulness of queuing theory in the field of traffic management. The concept of traffic intensity isapplied to a set of areas in queuing theory in this study. Keywords: Traffic intensity, Queuing theory, Single server Poisson model.
Dear Colleagues, The purpose of this special issue is to contribute to the queueing theory and stochastic models with novel papers. Queueing models are one of the most well-known theories of stochastic models, and their progress and development are increasing exponentially since A.K. Erlang (1917) and T.O. Engset (1918) who studied ...
Queueing theory, broadly speaking, concerns the design and analysis of resource-constrained systems in which customers need to potentially wait for access to a resource. This paradigm arises in many applied settings, ranging from service and health operations to communications and computer systems to ride-sharing and job-matching platforms to ...
In 2002, Gorunescu et al. [53, 54] published two of the best-known papers addressing queueing theory and bed management combined. ... Building on previous research in queueing theory, they developed and solved a mathematical programming model that used empirical data from EDs and took into account patient preferences. ... They concluded that ...
Research papers show that the Queuing Theory can be used efficiently in health care. The Queuing Theory was first analyzed by Agner Krarup Erlang, a Danish engineer, mathematician, researcher, in 1913 in the context of telephone traffic. ... (providers) needed. Queue models generally deal with customer arrivals at a service facility. We will ...
Queueing models. Customers requiring service are generated over time by an input source. These customers enter the queueing system and join a queue. At certain times, a member of the queue is selected for service by some rule known as the queue discipline. The required service is then performed for the customer by the service mechanism, after ...
Abstract. This paper reviews the contributions and applications of queueing theory in the field of health care management problems. This review proposes a system of classification of health care areas which are examined with the assistance of queueing models. The categories described in the literature are expanded and a detailed taxonomy for ...
This research suggests that analytical queueing models may offer the most cost-effective technique for computer system performance modeling. Benchmarking is older. Simulation is more popular. But analytical queueing models may offer the most cost-effective technique for computer system performance modeling.
Problems related to patient scheduling and queueing in emergency departments are gaining increasing attention in theory, in the fields of operations research and emergency and healthcare services, and in practice. This paper aims to provide an extensive review of studies addressing queueing-related problems explicitly related to emergency departments. We have reviewed 229 articles and books ...
This paper contains some fundamental queuing theory formulas, some of which are specifically derived for the M/M/1 queuing model. This study then presents a simulation of the M/M/1 queuing model ...
The paper first presents previous research involving traditional applications of Queuing Theory in Healthcare and customer satisfaction research with its application to patient satisfaction with waiting time. Secondly, the paper argues that despite significant work on staff satisfaction, no model exists that explains staff satisfaction behavior ...
In 2018, Vijay Prasad et. al., in their research article found the required number of servers and expected number of customers in the system of multi server queuing model by using LPP- graphical method. T Srinivasa Rao et.al., in 2018, in their research paper examined a two- stage queuing system
Abstract. This article provides a review on application of queueing models in Hospital sector. In this article, the methodology for the review is ba sed on a modification of an existing litera ...
This paper firstly introduces the queuing theory and discrete event system simulation of the basic knowledge, including queuing theory basic concept, basic composition, research contents, performance metrics, several classical queuing system models and discrete event system simulation steps. Then the characteristics, modeling principles and basic steps of several commonly used are studied ...
This paper presents a mathematical model for an inventory control system in which customers' demands and suppliers' service time are considered as stochastic parameters. The proposed problem is solved through queuing theory for a single item. In this case, transitional probabilities are calculated in steady state. Afterward, the model is extended to the case of multi-item inventory systems ...
The proposed model in Section 3.2 has been solved without considering the M/M/1 queuing model, and its result is compared with the situation that the M/M/1 queuing model has been considered. The result is shown in Table 9 , which states that considering waiting time at terminal stops by having the M/M/1 queuing model resulted in investing more ...
1 Queueing Theory Basics (see Hillier and Lieberman 17.2,7) Learning Objectives 1.Know the goals of queueing theory. 2.Be able to identify the de ning characteristics of a queue system from the standard 5-character identi ers. 3.Be able to calculate the arrival-service ratio and the utilization factor ˆfrom a given
The most common combination of models in determining the patient's flow are patients's inflow model and queuing m. odel or Markov Chain Model. According to . Boyce, Kraft, Svenonius, & Borko, (1991) queuing theory is a branch of operation research because the findings can be used in decision-making and to determine the resources
This paper discusses analytic solution methods for queueing models with multiple waiting lines. The methods are briefly illustrated, using key models like the 22 switch, the shortest queue and the ...
In this paper, we examine a priority-based queuing model and perform the mathematical analysis of different media calls processing in converged network environment. We use for this purpose a queuing system model M3/G3/1/NPRP in order to process effectively input jobs/requests (or packets).
This paper aims to show that queuing theory satisfies the model when tested with a. real-case scenario. Authors obtained the data from a restaurant "Bapu Ki Kutiya" in Bhopal, M.P. in. order ...