SYSTEMATIC REVIEW article
A study of the impact of project-based learning on student learning effects: a meta-analysis study.
- 1 Institute of Computer and Information Science, Chongqing Normal University, Chongqing, China
- 2 Institute of Smart Education, Chongqing Normal University, Chongqing, China
Introduction: With the educational reform for skills in the 21st century, a large number of scholars have explored project-based learning. However, whether project-based learning can effectively improve the learning effect of students has not yet reached a unified conclusion.
Method: This study uses a meta-analysis method to transform 66 experimental or quasi-experimental research papers based on project-based learning over the past 20 years into 190 effect values from the sample size, mean, and standard deviation of experimental data during their experiments, and to conduct in-depth quantitative analysis.
Results: The results of the study showed that compared with the traditional teaching model, project-based learning significantly improved students’ learning outcomes and positively contributed to academic achievement, affective attitudes, and thinking skills, especially academic achievement.
Discussion: The results of the moderating effects test indicated that the effectiveness of project-based learning and teaching was influenced by different moderating variables, including country region, subject area, type of course, academic period, group size, class size, and experimental period : (1) from the perspective of country geography, the effects of project-based learning in Asia, especially in Southeast Asia, were significantly better than those in Western Europe and North America; (2) in terms of curriculum, project-based learning promotes student learning effects more significantly in engineering and technology subjects, and is better applied in laboratory classes than in theory classes; (3) from a pedagogical point of view, project-based learning is more suitable for small group teaching, in which the group size is 4-5 people teaching the best results; (4) in view of the experimental period, 9-18 weeks is more appropriate and has more obvious advantages for application at the high school level.
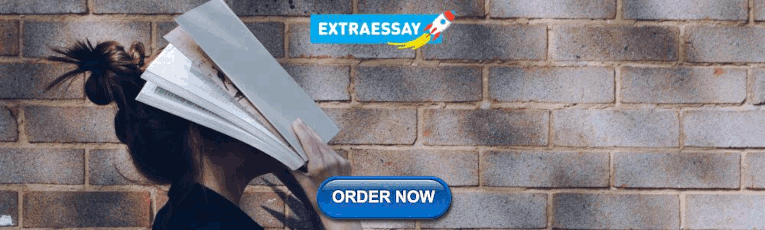
1. Introduction
Project-based learning (PBL) is a new model of inquiry-based learning that is centered on the concepts and principles of a subject, with the help of multiple resources and continuous inquiry-based learning activities in the real world, with the aim of producing a complete project work and solving multiple interrelated problems within a certain period of time ( Jingfu and Zhixian, 2002 ). s a new student-centered teaching approach, project-based learning directly points to the goal of cultivating 21st-century skills, especially higher-order thinking skills, and higher-order thinking occurs based on problem-solving, a challenging problem that emphasizes real-world situations and open environments, and project-based learning motivates students to continuously explore in the process of problem-solving, thus promoting the development of higher-order thinking.
In the era of digital transformation of education, the new generation of information technologies such as artificial intelligence, big data, and metaverse are bringing great changes to education at an unimaginable speed, and at the same time posing unprecedented challenges to talent training. Cultivating students with higher-order thinking skills that can adapt to the future development of society and reasonably cope with the complex real world has become an important mission in the current education reform and development around the world ( Ma and Yang, 2021 ). Different types of problems produce different teaching methods and also guide the development of students’ different thinking skills. Project-based learning, as a new type of teaching and learning method in the context of curriculum and teaching reform, takes real life as the background, is driven by practical problems, breaks the disciplinary boundaries, integrates multiple disciplines into one project, and develops students’ future-oriented abilities——creative thinking, problem raising, problem solving, critical thinking, communication and collaboration, etc. The advantages of this approach over traditional teaching and learning models are being recognized and explored. A large number of studies on the effects of project-based learning have been done, but there is not complete agreement on the effects on the development of students’ thinking skills, academic performance, and affective attitudes.
Over the past few decades, project-based learning has received a lot of attention in the field of education. Many studies have shown that project-based learning can improve students’ learning motivation, problem-solving skills, teamwork, and communication skills. However, due to the complexity and diversity of project-based learning, as well as differences in research methods, research findings on its effectiveness and influencing factors vary. A key research question in project-based learning meta-analytic studies is to assess the impact of project-based learning on student learning outcomes, including student performance in the areas of academic achievement, thinking skills, and affective attitudes. By combining the results of multiple independent studies, more accurate and reliable conclusions can be obtained to further understand the effects of project-based learning. In addition, project-based learning meta-analysis studies can help reveal the factors and mechanisms influencing project-based learning. By comparing the learning effects under different project-based learning conditions, researchers can analyze the impact of factors such as project characteristics, instructional design, and learning environment on student learning. This can help guide the design and implementation of project-based learning and promote effective student learning. Based on this, this study compensates for the limitations of individual studies by integrating and synthesizing multiple independent studies in order to systematically assess the effects of project-based learning, provide more accurate and reliable evidence, and reduce the chance of research findings. At the same time, project-based learning meta-analysis can provide a broader perspective to help researchers and educational policy makers gain a comprehensive understanding of the effects and influencing factors of project-based learning, so that they can develop more effective teaching strategies and policies to promote the improvement and development of project-based learning.
2. Literature review and theoretical framework
One view is that project-based learning can significantly improve student learning outcomes, including academic achievement, motivation, and higher-order thinking skills. Karpudewan et al. (2016) explored the feasibility of improving energy literacy among secondary school students using a project-based instructional approach. The quantitative results of the study showed that students exposed to a PBL curriculum had better performance on energy-related knowledge, attitudes, behaviors, and beliefs. The quantitative results of the study showed that students exposed to the PBL curriculum outperformed students taught using the traditional curriculum. The quantitative results of the study showed that students exposed to the PBL course outperformed students taught with traditional courses in terms of energy-related knowledge, attitudes, behaviors, and beliefs. The results of Zhang Ying’s intrinsic motivation scale, which was administered to 21 private university students before and after they received project-based learning, showed that there were significant differences in students’ interest, autonomy, and competence before and after, which positively influenced students’ intrinsic motivation to learn ( Zhang, 2022 ). Yun (2022) used the fifth-grade project “Searching for Roots. Xu Hui Yuan” project-based learning as an example to discuss that project-based in-depth ritual education can develop students’ core literacy. Biazus and Mahtari (2022) conducted a quasi-experiment using project-based learning and direct instructional learning models and found that the PBL model had a significant impact on the enhancement of creative thinking skills of secondary school students. Parrado-Martínez and Sánchez-Andújar (2020) explored the effects of project-based learning on ninth-grade students’ writing skills and found that cooperative work in project-based learning potentially promoted students’ critical thinking, communication, and collaboration skills, significantly improving middle school students’ English writing skills. Hernández-Ramos and De La Paz (2009) found that students in project-based learning conditions showed significant improvements in content knowledge measures and growth in their historical thinking skills compared to students in control schools. Most researchers agree that STEM as a form of project-based learning and STEM integration will have a positive impact on education, with the advantages outweighing the disadvantages ( Hamad et al., 2022 ; Wardat et al., 2022 ).
Another view is that project-based learning has the same effect or even some negative effects compared to traditional instruction. García-Rodríguez et al. (2021) conducted an intervention experiment in undergraduate education to test the effectiveness of a student-centered project-based learning approach in promoting student skill acquisition. The study found that students’ problem-solving and information management skills, two instrumental general competencies were not improved. The results of ÇAKICI’s project-based learning activities on fifth-grade children’s science achievement showed that although project-based activities significantly improved children’s science achievement, attitudes toward science did not change. Gratchev and Jeng (2018) explored whether the combination of traditional teaching methods and project-based learning activities improved students’ learning experiences, and data collected over 3 years showed that the two groups’ achievements were very similar, and the findings indicated that students were less motivated to accept new learning methods such as PBL. Parrado-Martínez and Sánchez-Andújar (2020) found that the implementation of PBL did not significantly change students’ perceived utility of teamwork, communication, and creativity. Kızkapan and Bektaş (2017) examined the effects of project-based learning and traditional learning methods on the academic performance of seventh graders, and the results showed no significant differences between the experimental and control groups on post-test “achievement test” scores. Sivia et al. (2019) used a mixed triangulation-convergence approach to examine the difference in student engagement between project-based and non-project-based learning units and found that project-based learning did not significantly increase student engagement. Karaçalli and Korur (2014) used a quasi-experimental design to teach the experimental group using a project-based learning approach, and the results showed no statistically significant effect on students’ attitudes toward learning across groups.
In summary, a review of the literature reveals that the research findings and teaching effectiveness of project-based learning have not yet been uniformly determined, and few studies have systematically analyzed and evaluated the optimal group size, class size, curriculum type, and subject area of project-based learning. Therefore, based on 66 empirical research papers that conducted experimental or quasi-experimental studies on project-based learning and traditional teaching, this study quantifies the true magnitude of the impact of the project-based learning approach on students’ learning outcomes and seeks to summarize the experience of applying project-based learning in schools in order to provide a reference for developing project-based teaching. And an attempt is made to answer the following research questions:
1. Does project-based learning significantly improve students’ thinking skills, academic performance, and affective attitudes compared to traditional teaching methods?
2. How do different moderating variables (type of course, learning section, group size, class size, subject category, experiment period, country region.) affect students’ learning effects?
Since the purpose of this study was to explore the effect of project-based learning on learning effectiveness and to explore other factors that may moderate this effect. Therefore, based on relevant research findings on the effect of project-based integrated learning on learning effectiveness and the results of literature coding, the meta-analytic theoretical framework for this study, as shown in Figure 1 .
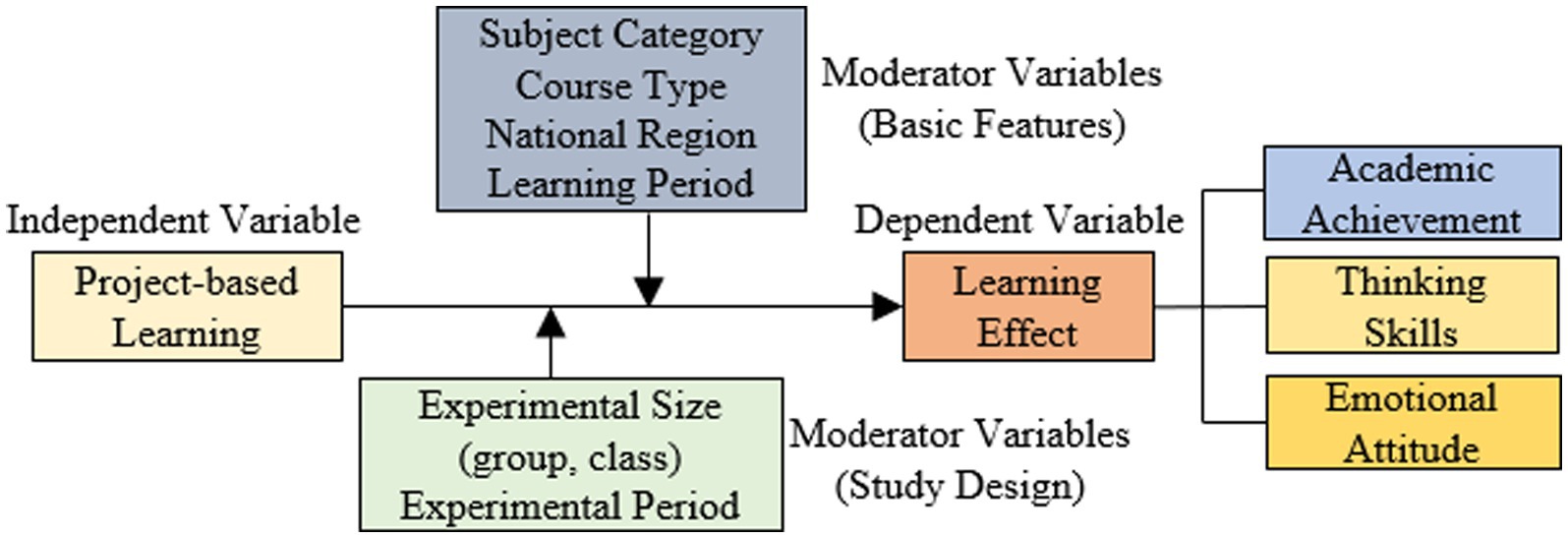
Figure 1 . Research framework diagram.
3. Study design
3.1. methods.
Meta-Analysis is a quantitative analysis method that extracts and organizes multiple results of experimental or quasi-experimental studies on the same research question and then produces an average effect value by weighting the sample size, standard mean deviation, and other data from the existing research results and analyzes the effect value to obtain the results. The meta-analysis method has been widely used in education. This study compares and combines literature on the same research topic but with different research results by extracting data such as pre and post-test means, sample sizes, and standardized mean differences from relevant literature, while using the standard deviation (SMD), which can correct for small sample bias, as the effective value to indicate the degree of influence of project-based instruction on student learning outcomes. The study entered the relevant data into CMA meta-analysis software (Comprehensive Meta Analysis 3.0) for data analysis.
3.2. Research process
To ensure the quality of the study, this study strictly followed the meta-analysis criteria proposed by Glass (1976) , which was mainly divided into four assessment procedures: literature collection, literature coding, effect size calculation, and moderating variable analysis, and finally a comprehensive effect size exploration and study results.
3.2.1. Literature search
To ensure the timeliness of the study, this study mainly searched the relevant research on the topic of project-based learning since 2003 to 2023, mainly in CNKI, Springer Link, Web of Science, Semantic Scholar and other databases, and searched the literature by “AND” or “OR” logical word collocation of project-based learning and learning effectiveness keywords. The keywords of project-based learning include: project-based learning, PBL, project teaching; the keywords of learning effect include: learning effect, learning performance, learning achievement, learning*, learning outcome, learning result, etc. And the selected articles are all from SSCI or SCI authoritative journals, Chinese core journals of article literature type and part of the master’s degree thesis. To avoid omissions, this study also supplemented the search with the references of relevant articles.
3.2.2. Literature selection and inclusion criteria
To find articles that meet the subject matter requirements, this study used the ( Page, 2021 ) process for literature processing ( Vrabel, 2009 ), the literature search, screening, and inclusion process is shown in Figure 2 . Combining the needs of the meta-analysis method itself and ensuring the accuracy and rigor of the research results, the following selection and inclusion criteria were used: (1) duplicate literature had to be removed; (2) it had to be a study of the effects of project-based learning versus traditional teaching models on learning effectiveness; (3) it had to be an empirical research type article; (4) complete data that could calculate the effect values had to be available. A total of 91 articles were screened by two researchers in the inclusion phase, and those with inconsistent screening were discussed, and the final decision was made to include 66 articles in the meta-analysis, which met the inclusion criteria for the number of articles in the meta-analysis method.
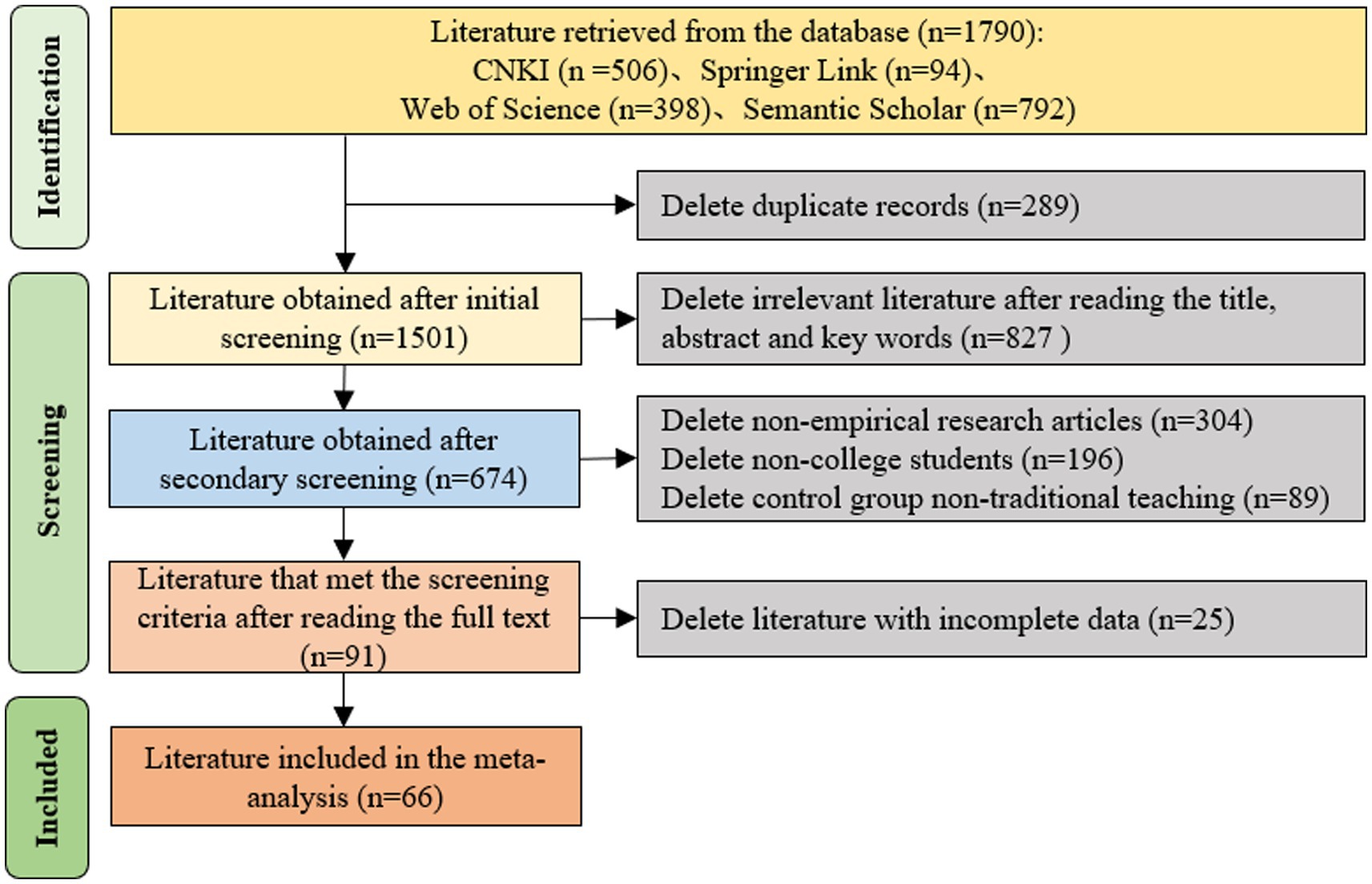
Figure 2 . Flow chart of literature screening.
3.2.3. Literature code
The concept of project-based learning was first introduced by American educator William Heard Kilpatrick proposed ( Kilpatrick, 1918 ). In the 1920s and 1930s, project-based learning was widely used in the lower grades of elementary and secondary schools in the United States; in 1969, McMaster University in Canada officially launched the PBL teaching model within the school. To compare the variability of the effects of project-based learning in countries around the world, the regions of the countries where the study was conducted were coded and divided into North America, Oceania, Southeast Asia, and other regions. As project-based learning is used more frequently in the classroom, whether there is an ideal group size to facilitate student learning outcomes ( Wei et al., 2020 ), and the impact of group size on academic achievement ( Al Mulhim and Eldokhny, 2020 ), which academic section, subject, and course type is better taught, are questions that should be addressed. Therefore, the coding of this study included the following seven main items: subject category, course type, country region, academic section, class size, group size, and experimental period, and categorized learning outcomes into three main categories: academic achievement, thinking skills, and emotional attitudes. Because this study included 66 documents with 190 effect sizes, only part of the feature coding content is displayed, as shown in Table 1 ( Kelly and Mayer, 2004 ; Mioduser and Betzer, 2007 ; Hernández-Ramos and De La Paz, 2009 ; Domínguez and Elizondo, 2010 ; Keleşoğlu, 2011 ; Çakici and Türkmen, 2013 ; Karaçalli and Korur, 2014 ; Bilgin et al., 2015 ; Astawa et al., 2017 ; Kızkapan and Bektaş, 2017 ; ShiXuan, 2017 ; Yuan, 2017 ; Praba et al., 2018 ; Yexin, 2019 ; Faqing, 2020 ; Gao, 2020 ; Lei, 2020 ; Ling, 2020 ; Linxiao, 2020 ; Lu, 2020 ; Luo, 2020 ; Mingquan, 2020 ; Rui, 2020 ; Yanan, 2020 ; Yang, 2020 ; Akharraz, 2021 ; Cong, 2021 ; Migdad et al., 2021 ; Xiaolei, 2021 ; Wang, 2021a , b , 2022 ; Jina, 2022 ; Ma, 2022 ; Xu, 2022 ; Xuezhi, 2022 ; Yating, 2022 ; Ying, 2022 ; Yuting, 2022 ; Zhang, 2022 ). To ensure the objectivity of the coding process, this study was completed independently by two researchers for the 66 empirical research articles included in the meta-analysis, and the coding results were tested for consistency using SPSS 24.0, and the Kappa value was 0.864, which was greater than 0.7, indicating that the coding effect was valid and the results were credible.
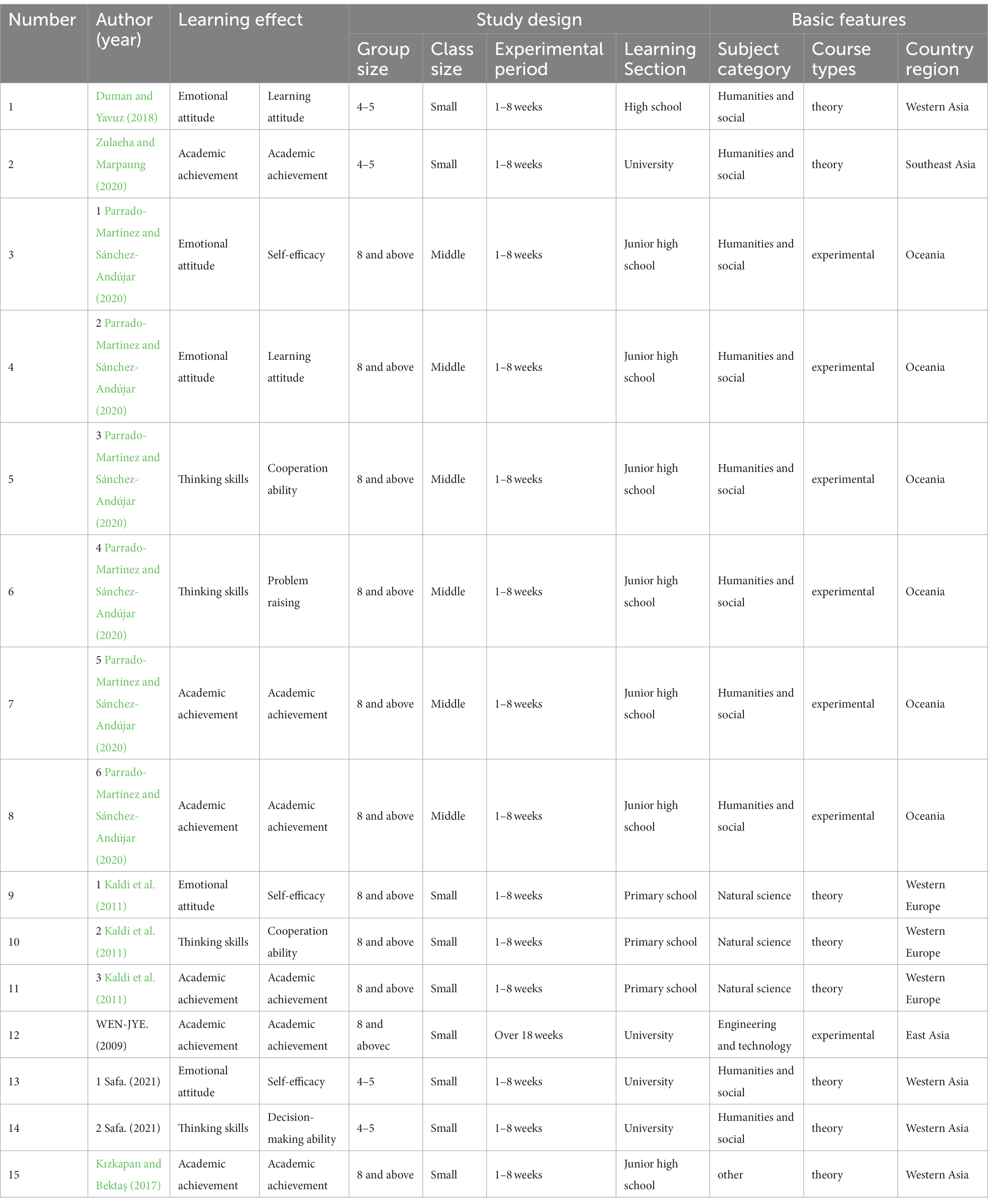
Table 1 . Code list (due to space limitation, only part of the coding content is shown).
3.2.4. Data analysis
Based on the completion of the literature coding, the calculation of the effect size (Standardized difference in means), including sample size, standard deviation, and mean value, was performed by finding the relevant experimental data in the literature. The effect size values were calculated as follows:
Starting with Mean, SD, N in each group.
Raw difference in means.
RawDiff = Mean1-Mean2.
SDP = Sqr (((N1–1) * SD1^2 + (N2-1) * SD2^2)/(N1 + N2–2))).
Standardized difference in means.
StdDiff = RawDiff/SDP.
The next stage was data analysis by (1) publication bias test. A funnel plot was used for qualitative analysis, while a combination of Begg’s rank test and loss of safety coefficient was used for quantitative analysis; (2) Heterogeneity test. The aim was to determine whether there was heterogeneity among the samples in this study; (3) Calculation of effect size values. To quantify the degree of influence of project chemistry learning on learning outcomes; (4) the moderating variables were tested. All data analyses in this study were conducted using Comprehensive Meta Analysis 3.0.
4.1. General effect size results
4.1.1. publication bias test.
In this study, the std. diff in means (SMD) value was selected as the unbiased effect value, and also to ensure the possibility that the results reported in the literature do not deviate from the true results, the publication bias was analyzed qualitatively using funnel plots, and the publication bias was analyzed qualitatively using Begg’s rank test, Trim and Fill and Fail-safe N to quantitatively analyze publication bias. Publication bias is critical to the results of meta-analysis, and if the research literature is not systematically representative of all existing research in the field in general, it indicates that publication bias may exist ( Higgins and Thompson, 2002 ). As shown in Figure 3 , the majority of study effect values were clustered within the funnel plot, and a small number of effect values were relative to the right, with Begg’s rank test Z = 5.082 > 1.960 ( p < 0.05), indicating a possible publication bias. Therefore, the severity of publication bias was further identified using the loss of safety factor, which showed N = 2,546, much larger than “5K + 10” ( K = 190), suggesting that an additional 2,546 unpublished studies would be required to reverse the results ( Rothstein et al., 2006 ), and it can be concluded that there is no significant publication bias in this study.
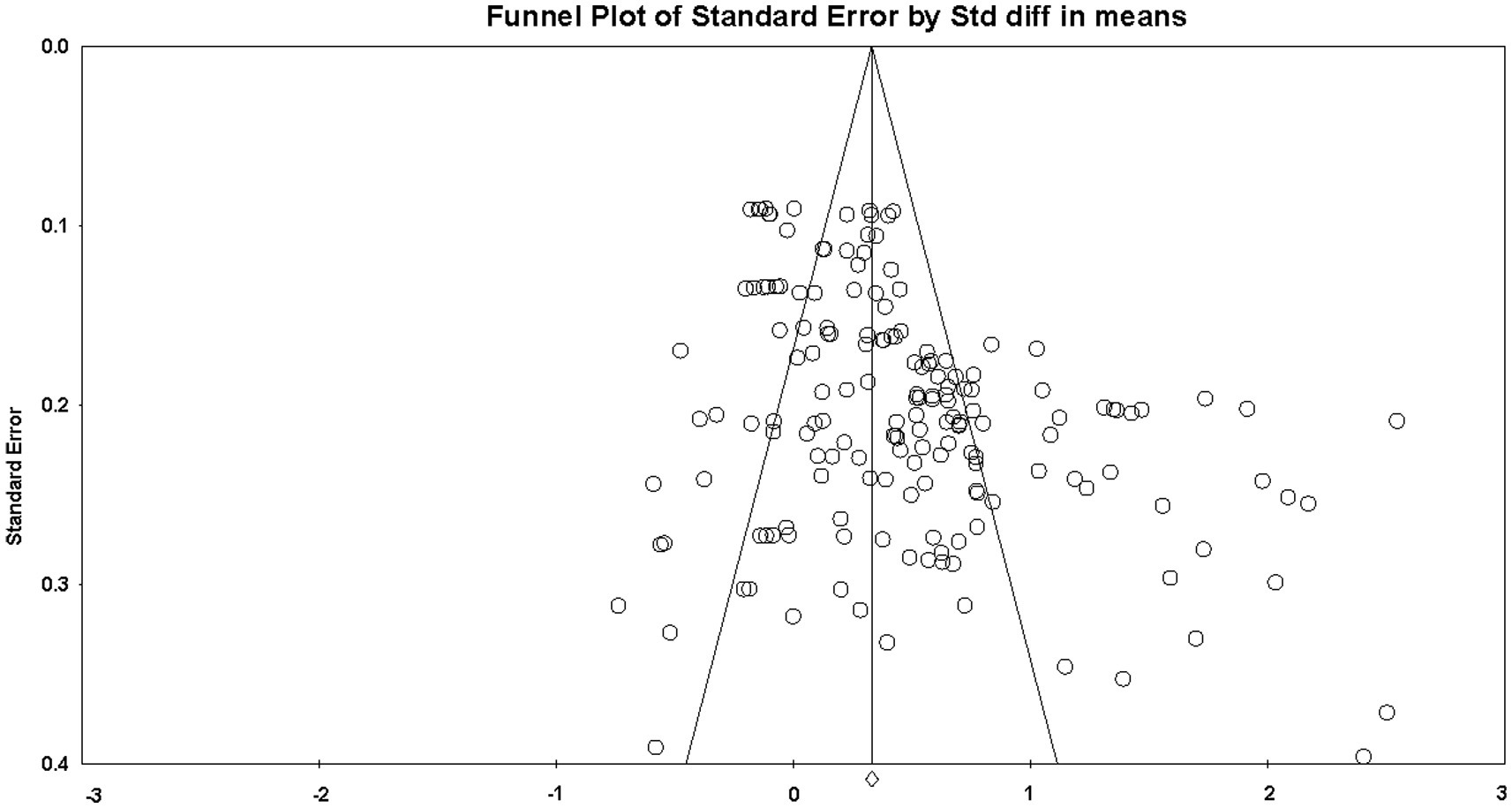
Figure 3 . Publication bias funnel plot.
4.1.2. Heterogeneity test
To ensure that the effect values of the independent samples in this study are combinable, Q and I2 values were used to define heterogeneity. Higgins et al. classified heterogeneity as low, medium, or high, as measured by the magnitude of the I2 statistic, which was 25, 50, and 75%, respectively. In addition, if the Q statistic is significant then the hypothesis that there is no heterogeneity among the sample data should be rejected. Based on the forest plot of I2 = 87.4% > 50% and Q = 1496.2 ( p < 0.001), the results indicate that there is a high degree of heterogeneity between the samples, therefore, this study used a random effects model for correlation analysis to eliminate some of the effects of heterogeneity, and also further indicates that it is necessary to conduct a moderated effects test to examine the effect of project-based learning on learning effects.
4.2. Results about problem of studies’ fields
4.2.1. the overall impact of project-based learning on student learning outcomes.
Cohen (1988) proposed the effect value analysis theory in 1988, he believed that the effect standard measure effect is determined by the effect value (ES), when the ES is less than 0.2, it means that there is a small effect impact, when the ES is between 0.2–0.8 means that there is a moderate effect, when the ES > 0.8 means that there is a significant effect impact. This study included 190 experimental data from 66 empirical research papers, and as shown in Table 2 , the combined effect value of the impact of project-based learning on student learning outcomes was 0.441, close to 0.5 and p < 0.001, indicating that project-based learning has a large degree of impact on learning outcomes and is an effective teaching approach.

Table 2 . Main effects test.
In this study, the literature included in the meta-analysis was divided into three subcategories of academic achievement, thinking skills, and emotional attitudes according to the “three-dimensional goals” for analysis. Moderately positive impact (SMD = 0.650), and the total effect values for affective attitudes and thinking skills were 0.389 and 0.386, respectively.
Based on the deeper connotation of “three-dimensional goals,” this study classifies affective attitudes into learning motivation, learning attitude, learning interest, and self-efficacy; thinking skills into creative thinking ability, computational thinking ability, decision-making ability, critical thinking ability, problem-solving ability, problem raising ability, collaboration ability, and comprehensive application ability. As shown in Table 3 . In terms of affective attitudes, project-based learning influenced more on students’ interest in learning (SMD = 0.713), and also had moderate positive effects on learning motivation (SMD = 0.401) and learning attitudes (SMD = 0.536), with lower effects on self-efficacy; in terms of thinking skills, project-based learning had the most significant effects on students’ creative thinking skills (SMD = 0.626) and computational thinking skills (SMD = 0.719) had the most significant effect, followed by problem solving, collaboration, and general application skills, but the effects on decision making, critical thinking, and problem raising skills did not reach a statistically significant level.
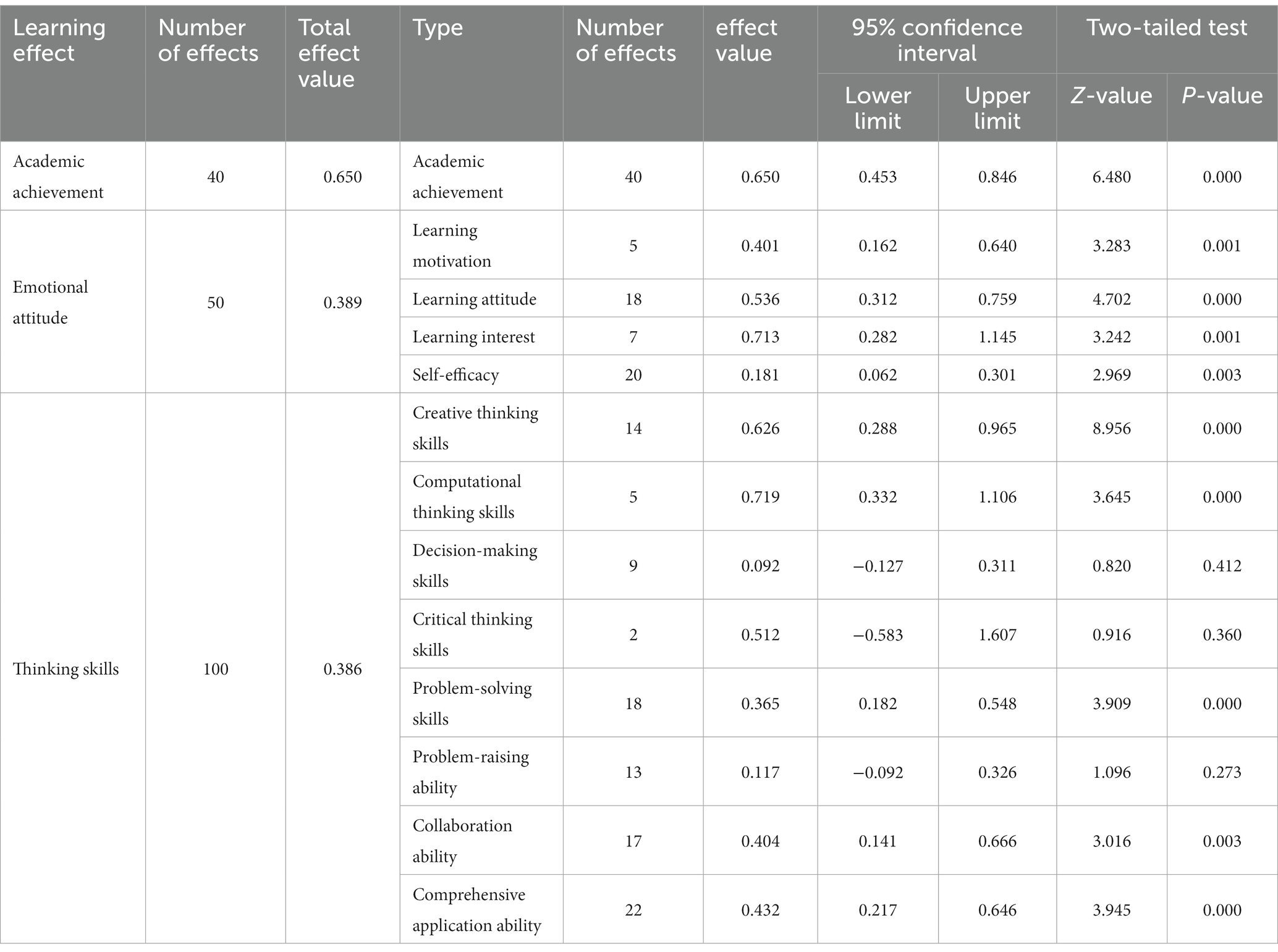
Table 3 . Effects of project-based learning on different learning outcomes.
4.2.2. Examining the effects of different moderating variables on student learning
First, in terms of country region as a moderating variable, the overall effect value of its moderating effect on learning effectiveness was 0.358 and p < 0.001, indicating a moderate effect and the effects varied across countries. In terms of effect values between groups, although project-based learning originated in the United States and was first applied in American countries such as Canada, its effect on student learning outcomes was not significant (SMD = 0.061, p = 0.429 > 0.05), and there was no significant difference in whether or not project-based learning was used; instead, the application of project-based learning produced better learning outcomes in Asian countries, especially in Southeast Asian countries (SMD = 0.684), followed by West Asia (SMD = 0.594).
Second, looking at the school level as the moderating variable, the overall effect value SMD = 0.355, in order of effect value from smallest to largest, is university (SMD = 0.116) < junior high school (SMD = 0.520) < primary school (SMD = 0.527) < high school (SMD = 0.720), which indicates that there are differences in the effects of project-based learning on the learning outcomes of students in different school levels, with the effects on high school, primary school, and junior high school, while the effect on college was relatively small.
Third, using group size as the moderating variable, the combined effect value of group size on learning effectiveness is 0.592 ( p < 0.001), which is close to 0.6, indicating that the effect of group size on students’ learning effectiveness is more significant and has a moderate to a high degree of facilitating effect. In terms of the effect values of different sizes, the effect values are all positive, indicating that the group learning style is effective and has different degrees of facilitating effects on learning effects, with the most significant facilitating effect of a group size of 4–5 students on learning effects (SMD = 0.909).
Fourth, to test the applicability of project-based learning on different class sizes, the class sizes were divided into three sizes according to the sample size: small (1 ~ 100 students), medium (100 ~ 200 students), and large (200 ~ 300 students), and the data in Table 4 show that the overall effect value of the moderating effect of class size on the learning effect is 0.378, p < 0.001, indicating that project-based learning on different class size. Looking specifically at each size, the degree of impact was higher for small class sizes (SMD = 0.483), followed by medium size (SMD = 0.466), but lower and not significant for large class sizes (SMD = 0.106, p = 0.101 < 0.05).
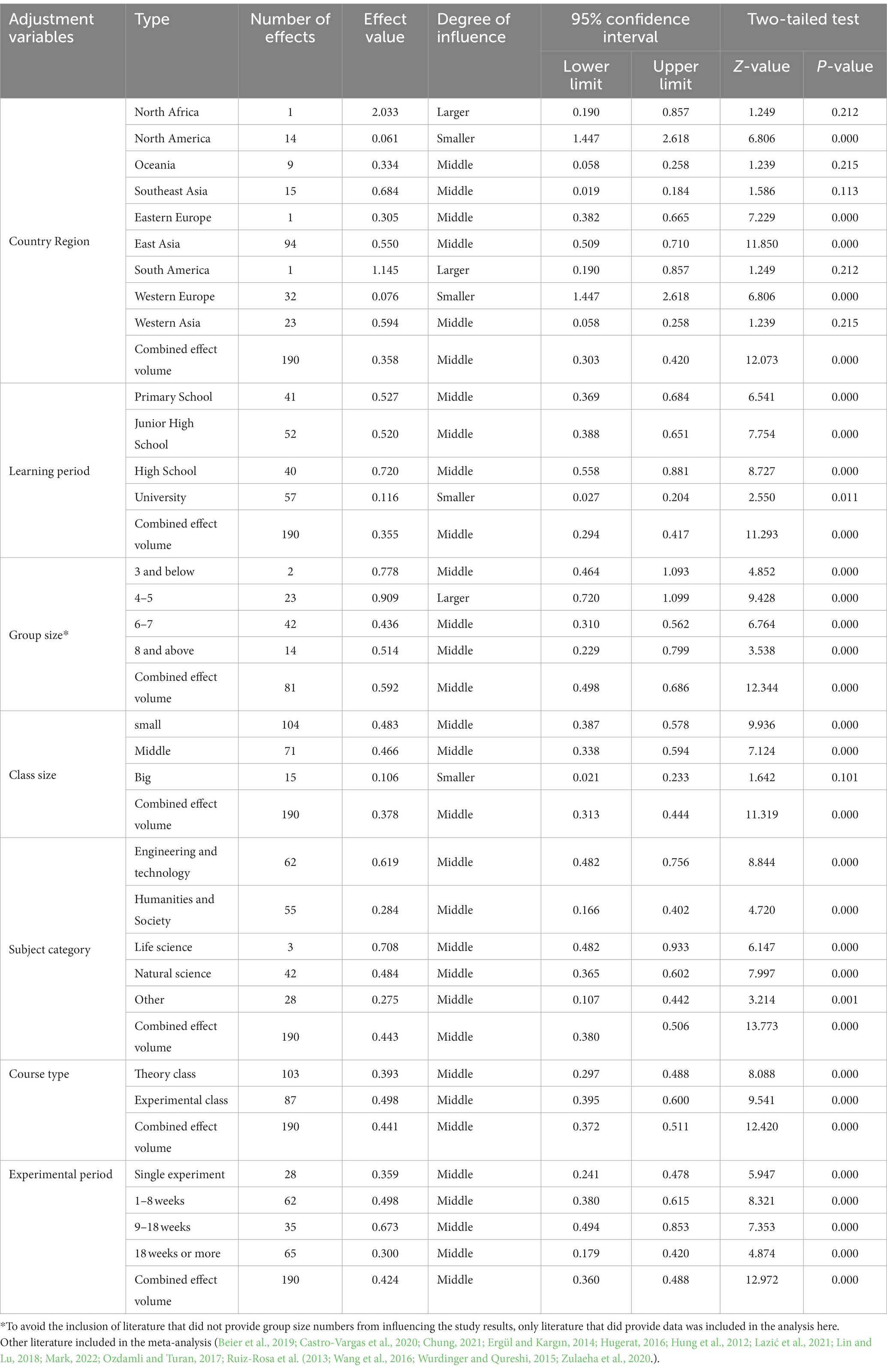
Table 4 . Results of moderating effects of different moderating variables.
Fifth, when subject categories were viewed as moderating variables, all subject effect values were larger than 0, with a combined effect value of SMD = 0.443 ( p < 0.001), suggesting that project-based learning had a positive degree of enhancement on learning effectiveness across subjects, reaching a statistically significant difference. Due to the relatively small amount of literature in other categories and life sciences, this study focuses on the effects of project-based learning on learning outcomes in engineering and technology, humanities and social, and natural sciences. In each of the subjects, Engineering and Technology (SMD = 0.619) > Natural Sciences (SMD = 0.484) > Humanities and Society (SMD = 0.284), the results indicate that project-based learning has the most significant impact on learning effectiveness in Engineering and Technology and relatively less in Humanities and Society.
Sixth, the overall effect value SMD = 0.441 when looking at the type of course as a moderating variable, while the between-group effect test between experimental and theoretical classes reached a statistically significant level ( p < 0.001). The effect of project-based learning on student learning outcomes was more pronounced in experimental classes (SMD = 0.498), which was greater than the overall combined effect value, consistent with the finding that project-based learning is more suitable and effective teaching strategy for engineering and technology disciplines, while the use of project-based teaching in theory classes (SMD = 0.393) was below the average effect value.
Seventh, in terms of the experimental period as a moderating variable, there were significant differences in project-based learning across experimental periods ( p < 0.001), with a moderating overall effect value of SMD = 0.424. The best effect of instructional facilitation was observed for the duration of 9–18 weeks (SMD = 0.673), which was better than single experiments (SMD = 0.359) and 1–8 weeks (SMD = 0.498), with a relatively weak effect on learning outcomes beyond 18 weeks (SMD = 0.3000).
5. Discussion
This study used meta-analysis to systematically review and quantitatively analyze 66 experimental or quasi-experimental research papers published between 2003 and 2023 on the effects of project-based instruction on student learning, and to dissect the differences brought about by different moderating variables. The results show that: ① project-based learning can significantly improve students’ learning outcomes compared with traditional teaching models; ② the effects of project-based teaching and learning are influenced by different moderating variables, including subject area, course type, academic period, group size, class size, and experiment period. The results derived from the meta-analysis are further discussed and analyzed below.
5.1. Project-based learning has a positive effect on student learning outcomes
First, the combined effect value of SMD = 0.441 ( p < 0.001) for the effect of project-based learning on learning outcomes indicates that compared to the traditional teaching model, project-based teaching has a moderately positive contribution to students’ academic achievement, thinking skills, and affective attitudes, which is consistent with the results of previous studies ( Wenlan and Jiao, 2019 ). This is consistent with previous studies. Compared with the traditional “teacher teach-student receive-evaluate and feedback” model, project-based learning is closer to a “complete learning process” ( Changming, 2020 ). It is a student-centered learning activity in which students show richer affective attitudes such as interest in learning and attitudes toward learning, which can positively guide students’ motivation to learn and influence their academic performance, and is naturally more effective in developing students’ emotional attitudes and values, and thinking skills.
Second, project-based learning has a significant positive effect on students’ thinking skills (SMD = 0.387, p < 0.001) and affective attitudes (SMD = 0.379, p < 0.001), indicating that the effect of project-based learning on students’ learning outcomes is not only the effect of academic performance, but also the effect of self-emotional attitudes and values, creative thinking skills, computational thinking skills, and other higher-order The impact of project-based learning on students’ learning is not only on their academic performance, but also on their self-emotional attitudes and values, creative thinking skills, computational thinking skills and other higher-order thinking skills. Project-based learning is a classroom activity that effectively develops students’ core literacies ( Hongxing, 2017 ) and promotes the development of higher-order thinking ( Weihong and Yinglong, 2019 ). The real value of project-based learning lies in its ability to enhance students’ higher-order thinking skills, such as creative thinking skills, problem-solving skills, and integrated application skills, by exploring real problems in small groups as a way to acquire the core concepts and principles of subject knowledge, and by posing driving questions around a topic based on real situations and students’ deep involvement in the investigation. Education for the future requires project-based learning to develop students’ 21st century skills and core literacies for their future careers and lives.
5.2. Moderating effects of different variables on student learning outcomes
To better analyze the impact brought by different moderating variables, this study categorized the moderating variables into four major categories: first, country region; second, curriculum, including subject categories and course types; third, teaching, including experimental period and learning periods; and fourth, experimental scale, including class size and group size. The results of the meta-analysis show as follows: (1) the application effect of project-based learning in Asia is better than that in countries in Oceania and Western Europe; (2) project-based learning has different degrees of influence on different disciplines and is better applied in the type of laboratory course; (3) in terms of the experimental period, the experimental period of 9–18 weeks is more appropriate and the application advantage of project-based learning at the high school level is more obvious; (4) project-based learning is more suitable for small-class teaching, in which the best effect is achieved when the group size is 4–5 students.
In terms of country region, the combined effect value of project-based learning is 0.358, and the application effect varies in different countries. In the Asian region, especially Southeast Asia, the effect of project-based learning is significantly better than that of Western Europe and North America. This study suggests the following reasons: First, Southeast Asian countries are relatively lagging in economic development, and industrialization and modernization are slower, so students and teachers pay more attention to practical learning methods, and project-based learning is a practice-based, problem-solving-oriented learning method that can better help them adapt and master skills and knowledge in actual work. Secondly, because the level of basic education in some Southeast Asian countries is relatively low due to various factors such as history, culture, and society, the project-based learning method can help students understand practical problems more deeply, comprehend knowledge, and enhance their hands-on and problem-solving abilities. Third, in Western European countries, students and teachers focus more on theoretical knowledge and logical thinking, individual student performance, and competition, and in countries such as Oceania, students and teachers focus more on practicality and teamwork. In Asia, however, the educational culture emphasizes a focus on discipline, order, and respect for teachers, making project-based learning more acceptable to students and parents. Students’ attitudes toward learning are also generally more serious, hard-working, and diligent, focusing on academic performance and opportunities for advancement, so students are more willing to engage in project-based learning in the hope of achieving better learning outcomes. Fourthly, in Asia, especially in East Asia, there is a strong demand for high-quality human resources, and project-based learning can cultivate students’ practical skills and innovative spirit, making them more competitive and capable of adapting to the future society.
In terms of curriculum, the combined effects of project-based learning on different subject areas and different course types were approximately equal, at 0.443 and 0.441, respectively, and the effect on student learning in engineering and technology disciplines was more significant (SMD = 0.619) and larger than the average effect, which is consistent with previous research findings that PBL is more appropriate for teaching in engineering ( Kolmos and De Graaff, 2014 ). Facing the rapidly developing society, the traditional teaching methods seem to be unable to better develop students’ skills to meet the market demand, and the research results also show that the application effect of PBL in experimental classes (SMD = 0.498) is better than that in theoretical classes (SMD = 0.393), because PBL can give students a complete understanding of the process of a project from problem raising to problem-solving, which provides them with valuable practical experience.
From the instructional aspect, the experimental period of 9–18 weeks (SMD = 0.673) had the greatest impact on student learning effects, and the impact of project-based learning for more than 18 weeks (SMD = 0.359) was relatively low, while the results of the study showed that project-based learning had a greater impact at the high school level (SMD = 0.720), followed by elementary school, middle school, and university, a finding that supports the results of Mehmet’s study ( Ayaz and Soeylemez, 2015 ). The moderating effect of the experimental period showed that the longer the experiment, the better the effect of about half a semester, and the project-based learning did not have a lasting and stable effect on students’ learning outcomes. Currently project-based learning is carried out more often at the primary and secondary school levels, and the teaching effect is more significant, but the application effect in universities is relatively low (SMD = 0.116), and the results of the study also indicate that the application promotion effect is most obvious in engineering and technology disciplines, so in the follow-up study, the application of project-based learning at the higher education level should be actively explored.
In terms of experimental scale, the effect of project-based learning on small class teaching (SMD = 0.483) is greater than that of medium class (SMD = 0.466) and large class (SMD = 0.106), and the teaching effect is better for group size of 4–5 people (SMD = 0.909), 8 people and above (SMD = 0.514), and 6–7 people (SMD = 0.436) in decreasing order. Therefore, project-based learning is more suitable for small-class teaching, and the number of people in the group collaborative learning is more conducive to the learning effect of around 4–5 people, which is almost consistent with the results of Wei et al. (2020) study on the effect of cooperative learning on learning effect. The relationship between class size and educational output has been discussed by a number of economists from the perspective of the economics of education, and is referred to as the “class size effect.” In small classes, teachers can spend more time on teaching and learning, each student can receive more attention from the teacher, and teachers and students can have more time to interact, thus having more opportunities to demonstrate and participate in collaborative group learning. In terms of group size, although there is no uniform standard, in general, too few or too many group members are not conducive to a higher degree of impact on the learning effect. From the research results, the best learning effect is produced by 4–5 students, with more reasonable task distribution among group members, all with a clear division of labor and sufficient interaction, which is more conducive to the formation of the group effect, thus better promoting the learning effect.
5.3. How does the impact of project-based learning on learning outcomes occur?
The results of the study show that project-based learning has a moderate positive contribution to learning effectiveness under different measurement measures dimensions, and how its effect occurs. The theoretical framework of the impact of project-based learning on learning effectiveness is drawn in conjunction with the specific processes and key features of project-based learning, as shown in Figure 4 , and will be analyzed in the following in conjunction with the theoretical framework.
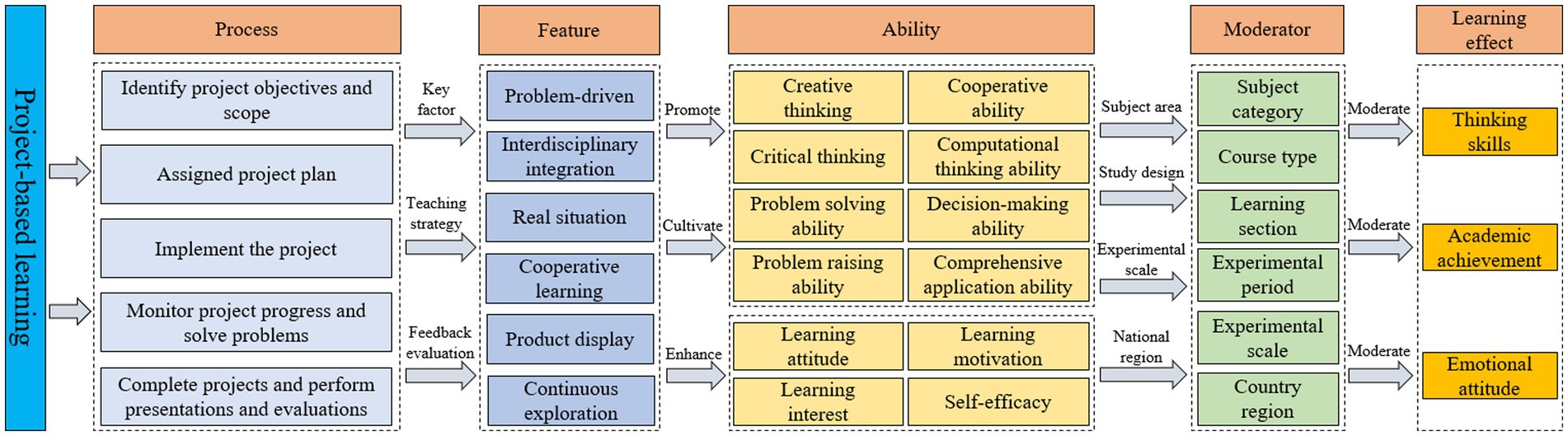
Figure 4 . Theoretical framework for the impact of project-based learning on learning effects.
In terms of the specific process of project-based learning, it includes five steps: identifying project goals and scope, developing a project plan, implementing the project, monitoring project progress and solving problems, completing the project and presenting and evaluating it, and these steps include key activities that affect learning outcomes such as problem orientation, cooperative learning, and authenticity, which together affect students’ learning outcomes.
Specifically, project-based learning is usually oriented to real-life problems, requiring students to apply their knowledge and skills to solve problems, and the driving questions stimulate students’ interest in learning; it integrates the knowledge and skills of multiple disciplines, blending theoretical knowledge with practice and cultivating students’ creative thinking skills and comprehensive application skills; in the process of implementing projects, group members divide the work and cooperate to identify problems and After the project is completed and presented, the teacher gives timely feedback and evaluation to influence students’ attitude in project-based learning and improve the learning effect. In conclusion, the specific process and characteristics of project-based learning are the key factors to enhance students’ learning effect. Reasonable design of project characteristics and the application of different variables in project-based learning can effectively enhance students’ learning effect.
5.4. When is it more effective to use project-based learning?
The findings suggest that learning effects are influenced by different moderating variables, and this study suggests combining the effects of different variables for project-based learning in order to achieve the optimal effect size. For high school students in the field of engineering and technology subject areas of laboratory courses to 9–18 weeks as the experimental period, based on small class teaching, and group size of 4–5 people using the PBL method of teaching, to promote the improvement of student learning outcomes more effective. In experimental courses, the use of project-based learning can enable students to gain a deeper understanding of the principles and practical operations of experiments, increase their interest and motivation, and promote the development of their active learning and innovative thinking skills, thus improving learning outcomes. Small class teaching and group work can better meet students’ individual needs, enhance their sense of participation and belonging, and increase their interest and motivation in learning. Finally, the 9–18 weeks experimental cycle allows students to make the most of their time and explore the subject matter in depth, enabling them to gain deeper understanding and experience in their learning. It is hoped that the results of this study will provide a reference for front-line educators to carry out project-based teaching and explore more effective ways to promote learning outcomes.
6. Conclusion
This study conducted a meta-analysis of 66 empirical research papers on the use of project-based learning interventions for learning, and the findings provide evidence for the use of project-based learning in education to develop students’ core literacy and higher-order thinking skills, and 21st-century skills. The results show that: (1) project-based learning can significantly improve students’ learning outcomes compared with traditional teaching models; (2) the effects of project-based teaching are influenced by different moderating variables, including subject area, course type, academic period, group size, class size, and experiment period. From the perspective of countries and regions, the effect of project-based learning in Asia, especially in Southeast Asia, is significantly better than that in Western Europe and North America; from the perspective of courses, project-based learning has a more obvious effect on promoting students’ learning in engineering and technology disciplines, and the application effect in experimental classes is better than that in theory classes; from the perspective of teaching, project-based learning is more suitable for small-class teaching, in which the best effect is achieved with a group size of 4–5 students From the perspective of teaching, project-based learning is more suitable for small class teaching, and the best effect is achieved in group size of 4–5 students.
7. Limitation
Although our findings have important implications for educators, they still have some limitations. For example, some studies using project-based learning for teaching and learning lacked sufficient statistical information for inclusion in the analysis, and most of the studies did not provide a specific classification of learning effectiveness, limiting our ability to analyze learning effectiveness enhancement in more detail. Subsequent research can be carried out in depth in two aspects: (1) the current empirical studies on project-based learning focus on primary and secondary schools, with less research on the impact on universities and young children; with the popularity of higher education, future research can be conducted on the above research subjects; (2) taking the digital transformation of education as an opportunity to explore the integration of technology and project-based learning to better develop students’ core literacy and 21st century skills.
Data availability statement
The original contributions presented in the study are included in the article/supplementary material, further inquiries can be directed to the corresponding author.
Author contributions
YM: critically review the work, provide commentary, supervise and direct the writing of the draft. LZ: conceptualization, methodology, validation, quantitative data analysis, writing, review and editing. All authors contributed to the article and approved the submitted version.
This work was supported by the Chongqing graduate education teaching reform research project (No. yjg201009), the Postgraduate Research Innovation Project of Chongqing in 2023 (No. CYS23419, No. CYS23416), and the Special Project of Chongqing Normal University Institute of Smart Education in 2023 (No. YZH23013).
Acknowledgments
We would like to sincerely thank all the teachers and students of Computer and Information Science, Chongqing Normal University, for their support and contributions to us, especially for the support from the Smart Education Research Institute.
Conflict of interest
The authors declare that the research was conducted in the absence of any commercial or financial relationships that could be construed as a potential conflict of interest.
Publisher’s note
All claims expressed in this article are solely those of the authors and do not necessarily represent those of their affiliated organizations, or those of the publisher, the editors and the reviewers. Any product that may be evaluated in this article, or claim that may be made by its manufacturer, is not guaranteed or endorsed by the publisher.
Akharraz, M. (2021). The impact of project-based learning on students’ cultural awareness .
Google Scholar
Al Mulhim, E., and Eldokhny, A. (2020). The impact of collaborative group size on students’ achievement and product quality in project-based learning environments. Int. J. Emerg. Technol. Learn. 15, 157–174. doi: 10.3991/ijet.v15i10.12913
CrossRef Full Text | Google Scholar
Astawa, N. L., Artini, L. P., and Nitiasih, P. K. (2017). Project-based learning activities and EFL students’ productive skills in English. J. Lang. Teach. Res. 8, 1147–1155. doi: 10.17507/jltr.0806.16
Ayaz, M., and Soeylemez, M. (2015). The effect of the project-based learning approach on the academic achievements of the students in science classes in Turkey: a meta-analysis study. EB 40, 255–283. doi: 10.15390/EB.2015.4000
Beier, M. E., Kim, M. H., Saterbak, A., Leautaud, V., Bishnoi, S., and Gilberto, J. M. (2019). The effect of authentic project-based learning on attitudes and career aspirations in STEM. J. Res. Sci. Teach. 56, 3–23. doi: 10.1002/tea.21465
Biazus, M., and Mahtari, S. (2022). The impact of project-based learning (PjBL) model on secondary students’ creative thinking skills. Int. J. Essential Competencies Educ. 1, 38–48. doi: 10.36312/ijece.v1i1.752
Bilgin, I., Karakuyu, Y., and Ay, Y. (2015). The effects of project based learning on undergraduate Students' achievement and self-efficacy beliefs towards science teaching. Eurasia J Math Sci. Technol. Educ. 11, 469–477. doi: 10.12973/eurasia.2014.1015a
Çakici, Y., and Türkmen, N. (2013). An investigation of the effect of project-based learning approach on Children's achievement and attitude in science. Online J. Sci. Technol. 3, 9–17.
Castro-Vargas, C., Cabana-Caceres, M., and Andrade-Arenas, L. (2020). Impact of project-based learning on networking and communications competences. Int. J. Adv. Comput. Sci. Appl. 11:2020. doi: 10.14569/IJACSA.2020.0110957
PubMed Abstract | CrossRef Full Text | Google Scholar
Changming, L. (2020). Why do we need project-based learning? Primary Secondary School Manage 08, 5–6.
Chung, S.J. (2021). Students’ perception of self-regulated learning in a project-based learning .
Cohen, J. (1988). Statistical power analysis for behavioral science. Technometrics 31, 499–500.
Cong, Li. (2021). Research on the design and implementation of project-based teaching of high school chemistry based on STSE education . [Master’s thesis]. China: Hunan Institute of Technology Available at: https://kns.cnki.net/KCMS/detail/detail.aspx?dbname=CMFD202201&filename=1021880452.nh
Domínguez, C., and Elizondo, A. J. (2010). Database design learning: A project-based approach organized through a course management system. Comput. Educ. 55, 1312–1320. doi: 10.1016/j.compedu.2010.06.001
Duman, B., and Yavuz, Ö. K. (2018). The effect of project-based learning on students’ attitude towards English classes. J. Educ. Train. Stud. 6:186. doi: 10.11114/jets.v6i11a.3816
Ergül, N. R., and Kargın, E. K. (2014). The effect of project based learning on students’ science success. Procedia. Soc. Behav. Sci. 136, 537–541. doi: 10.1016/j.sbspro.2014.05.371
Faqing. (2020). Research on the impact of project-based learning STEM curriculum on elementary school students’ problem solving ability [Master’s thesis]. China: Huazhong Normal University. Available at: https://kns.cnki.net/kcms2/article/abstract?v=s1YNj1Y_QLPkngH9X91x7Fs23_bcTKtQ_HV8_ZRG-u1wLLGRrl6pB21f7OyV3756xBZmpbJQSOsehywyktxXqDM37fhvBhTkVIdtKvLX5mrirj4EiSiDZyCFW4nENRtZYbN0hR_pNI0=\u0026amp;uniplatform=NZKPT\u0026amp;language=CHS
Gao, Yan-jun. (2020). Research on the teaching model of high school biology unit based on project-based learning (Master’s thesis, Southwest University. Availabe at: https://kns.cnki.net/KCMS/detail/detail.aspx?dbname=CMFD202101&filename=1021533870.nh
García-Rodríguez, F. J., Ruiz-Rosa, I., and Gutiérrez-Tao, D. (2021). Project-based learning as a tool to foster entrepreneurial competences (el aprendizaje basado en proyectos como herramienta para potenciar la competencia emprendedora). Cult. Educ. 33, 316–344. doi: 10.1080/11356405.2021.1904657
Glass, G. (1976). Primary, secondary, and metaanalysis of research. Educ. Res. 5, 3–5. doi: 10.3102/0013189X005010003
Gratchev, I., and Jeng, D. S. (2018). Introducing a project-based assignment in a traditionally taught engineering course. Eur. J. Eng. Educ. 43, 788–799. doi: 10.1080/03043797.2018.1441264
Hamad, S., Tairab, H., Wardat, Y., Rabbani, L., AlArabi, K., Yousif, M., et al. (2022). Understanding science teachers’ implementations of integrated STEM: teacher perceptions and practice. Sustainability 14:3594. doi: 10.3390/su14063594
Hernández-Ramos, P. F., and De La Paz, S. (2009). Learning history in middle school by designing multimedia in a project-based learning experience. J. Res. Technol. Educ. 42, 151–173. doi: 10.1080/15391523.2009.10782545
Higgins, J. P., and Thompson, S. G. (2002). Quantifying heterogeneity in a meta-analysis. Stat. Med. 21, 1539–1558. doi: 10.1002/sim.1186
Hongxing, H. (2017). Project-based learning: classroom teaching activities to cultivate students’ core literacy. J. Lanzhou Univ. 06, 165–172. doi: 10.13885/j.issn.1000-2804.2017.06.021
Hugerat, M. (2016). How teaching science using project-based learning strategies affects the classroom learning environment. Learn. Environ. Res. 19, 383–395. doi: 10.1007/s10984-016-9212-y
Hung, C., Hwang, G., and Huang, I. (2012). A project-based digital storytelling approach for improving Students' learning motivation, problem-solving competence and learning achievement. J. Educ. Technol. Soc. 15, 368–379.
Jina, Du. (2022). The application of project-based learning based on problem awareness in primary school mathematics classroom . [Master’s thesis]. China: Jimei University Available at: https://kns.cnki.net/KCMS/detail/detail.aspx?dbname=CMFD202301&filename=1022632607.nh
Jingfu, L., and Zhixian, Z. (2002). Project-based learning model. Foreign Educ. Res. 11, 18–22.
Kaldi, S., Filippatou, D., and Govaris, C. (2011). Project-based learning in primary schools: effects on pupils' learning and attitudes. Education 39, 35–47. doi: 10.1080/03004270903179538
Karaçalli, S., and Korur, F. (2014). The effects of project-based learning on Students' academic achievement, attitude, and retention of knowledge: the subject of “Electricity in our Lives”. Sch. Sci. Math. 114, 224–235. doi: 10.1111/ssm.12071
Karpudewan, M., Ponniah, J., and Zain, A. N. M. (2016). Project-based learning: an approach to promote energy literacy among secondary school students. Asia Pac. Educ. Res. 25, 229–237. doi: 10.1007/s40299-015-0256-z
Keleşoğlu, A. (2011). I nvestigating the effects of project-based learning on students’ Academic Achievement and Attitudes Towards English Lesson. vol.1 The Online Journal Of New Horizons In Education.
Kelly, G.J., and Mayer, R.E. (2004). Enhancing undergraduate students’ chemistry understanding through project-based learning in an IT environment .
Kilpatrick, W. H. (1918). The Project Method: The Use of the Purposeful Act in the Education Process. Teach. Coll. Rec . 19, 319–335.
Kızkapan, O., and Bektaş, O. (2017). The effect of project based learning on seventh grade students’ academic achievement. Int. J. Instr. 10, 37–54. doi: 10.12973/iji.2017.1013a
Kolmos,, and Graaff, D. (2014). Problem-based and project-based learning in engineering education Merging models . doi: 10.1017/CBO9781139013451.012.
Lazić, B. D., Knežević, J. B., and Maričić, S. M. (2021). The influence of project-based learning on student achievement in elementary mathematics education. S. Afr. J. Educ. 41, 1–10. doi: 10.15700/saje.v41n3a1909
Lei, Zhou. (2020). The design and implementation of project-based learning for high school chemistry teaching . [Master’s thesis]. China: Yunnan Normal University Availabe at: https://kns.cnki.net/KCMS/detail/detail.aspx?dbname=CMFD202101&filename=1020760511.nh
Lin, K., and Lu, S. (2018). Effects of project-based activities in developing high school students’ energy literacy. J. Balt. Sci. Educ. 17, 867–877. doi: 10.33225/jbse/18.17.867
Ling, C. (2020). Design and practice of teaching activities based on project-based learning to cultivate primary school students’ computational thinking . [Master’s thesis]. China: Chongqing Normal University Available at: https://kns.cnki.net/KCMS/detail/detail.aspx?dbname=CMFD202201&filename=1020648502.nh
Linxiao, P. (2020) The application of project learning in senior high school biology classroom study .
Lu, P. (2020). Research on teaching design of junior high school information technology curriculum based on project-based learning . [Master’s thesis]. China: Beijing University of Technology. Available at: https://kns.cnki.net/KCMS/detail/detail.aspx?dbname=CMFD202101&filename=1021563235.nh
Luo, J. (2020). Research on the development of computational thinking skills based on project-based learning . [Master’s thesis]. China: Huazhong Normal University. Available at: https://kns.cnki.net/KCMS/detail/detail.aspx?dbname=CMFD202101&filename=1020119469.nh
Ma, Q. (2022) Project learning self-efficacy in elementary programming impact study .
Ma, S., and Yang, X. (2021). Cooperative reasoning learning to promote the development of higher-order thinking. Educ. Dev. Res. 24, 64–73. doi: 10.14121/j.cnki.1008-3855.2021.24.011
Mark, Y. (2022). The effectiveness of PBL classes using multimedia tools: a case study of a university liberal arts English class. Multimedia Lang. Teach. 25, 237–257.
Migdad, S. I., Joma, A., and Arvisais, O. (2021). The impact of the project-based learning strategy on leadership skills acquisition among Palestinian refugees students in Gaza. Didactique 2, 4–39. doi: 10.37571/2021.01012
Mingquan, L. (2020) Project learning in senior high school geography teaching research and practice .
Mioduser, D., and Betzer, N. (2007). The contribution of project-based-learning to high-achievers’ acquisition of technological knowledge and skills. Int. J. Technol. Des. Educ. 18, 59–77. doi: 10.1007/s10798-006-9010-4
Ozdamli, F., and Turan, B.Y. (2017). Effects of a technology supported project based learning (TS-PBL) approach on the success of a mobile application development course and the students’ opinions .
Page, M. J. (2021). PRISMA 2020 explanation and elaboration: updated guidance and exemplars for reporting systematic reviews. BMJ (Clinical research ed.) , 372:n160. doi: 10.1136/bmj.n160
Parrado-Martínez, P., and Sánchez-Andújar, S. (2020). Development of competences in postgraduate studies of finance: A project-based learning (PBL) case study. Int. Rev. Econ. Educ. 35:100192. doi: 10.1016/j.iree.2020.100192
Praba, L.T., Artini, L.P., and Ramendra, D.P. (2018). Project-based learning and writing skill in EFL: Are they related?
Rothstein, H.R, Sutton, A. J., and Borenstein, M. Publication bias in meta-analysis: Prevention, assessment, and adjustments[M] (2006). Hoboken: John Wiley & Sons: 350.
Rui, Jiao. (2020). An empirical study on the development of computational thinking skills of high school students based on project-based learning . [Master’s thesis]. China: Shaanxi Normal University. Available at: https://kns.cnki.net/KCMS/detail/detail.aspx?dbname=CMFD202201&filename=1020131664.nh
Ruiz-Rosa, I., Taño, D., and García-Rodríguez, F. J. (2021). Project-Based Learning as a tool to foster entrepreneurial competences (El Aprendizaje Basado en Proyectos como herramienta para potenciar la competencia emprendedora). Cult. Educ. 33, 1–29. doi: 10.1080/11356405.2021.1904657
Saleh, S., Muhammad, A., and Abdullah, S.M. (2020). Stem project-based approach in enhancing conceptual understanding and inventive thinking skills among secondary school students .
Santyasa, I. W., Rapi, N., and Sara, I. W. (2020). Project based learning and academic procrastination of students in learning physics. Int. J. Instr. 13, 489–508. doi: 10.29333/iji.2020.13132a
Shin, M. (2018). Effects of project-based learning on students’ motivation and self-efficacy . English Teaching . 73, 95–114.
ShiXuan, M. (2017). Research on the design of project-based learning activities based on Moodle . [Master’s thesis]. China: Nanjing Normal University. Available at: https://kns.cnki.net/KCMS/detail/detail.aspx?dbname=CMFD201901\u0026amp;filename=1018254455.nh
Shyr, W. (2012). Teaching mechatronics: an innovative group project-based approach. Comput. Appl. Eng. Educ. 20, 93–102. doi: 10.1002/cae.20377
Sivia, A., MacMath, S., Novakowski, C., and Britton, V. (2019). Examining student engagement during a project-based unit in secondary science. Can. J. Sci. Math. Technol. Educ. 19, 254–269. doi: 10.1007/s42330-019-00053-x
Tseng, K., Chang, C., Lou, S., and Chen, W. (2013). Attitudes towards science, technology, engineering and mathematics (STEM) in a project-based learning (PjBL) environment. Int. J. Technol. Des. Educ. 23, 87–102. doi: 10.1007/s10798-011-9160-x
Vrabel, M. (2009). Preferred reporting items for systematic reviews and meta-analyses: the PRISMA statement. Rev. Esp. Nutr. Hum. Diet. 18:e123. doi: 10.1371/journal.pmed.1000097
Wang, J. (2021a) Project learning perspective of organic chemistry teaching design and implementation of high school .
Wang, Y. (2021b). Design and practice of open source hardware project-based learning for primary schools with problem-solving skills development . [Master’s thesis]. China: Northeast Normal University. Available at: https://kns.cnki.net/KCMS/detail/detail.aspx?dbname=CMFD202201&filename=1021631051.nh
Wang, H. (2022). Research on the design and application of a comprehensive practical activity curriculum for primary schools based on project-based learning . [Master’s thesis]. China: Mudanjiang Normal University. Available at: https://kns.cnki.net/KCMS/detail/detail.aspx?dbname=CMFD202301&filename=1022609345.nh
Wang, H., Huang, I., and Hwang, G. (2016). Comparison of the effects of project-based computer programming activities between mathematics-gifted students and average students. J. Comput. Educ. 3, 33–45. doi: 10.1007/s40692-015-0047-9
Wardat, Y., Belbase, S., and Tairab, H. (2022). Mathematics teachers’ perceptions of trends in international mathematics and science study (TIMSS)-related practices in Abu Dhabi emirate schools. Sustainability 14:5436. doi: 10.3390/su14095436
Wei, W., Yongquan, D., and Miao, Y. (2020). The impact of cooperative learning on students' learning outcomes: A meta-analysis based on 48 experimental or quasi-experimental studies. Shanghai J. Educ. Res. 07, 34–40+59. doi: 10.16194/j.cnki.31-1059/g4.2020.07.008
Weihong, L., and Yinglong, X. (2019). Promoting Students' high-level thinking development through project-based learning -- design and implementation of "baby market" exploration project. Basic Educ Curric 06, 20–23.
Wenlan, Z., and Jiao, H. (2019). Does project-based learning play a role in learning? -- meta-analysis based on 46 experimental and quasi-experimental studies. Res. Visual Educ. 02, 95–104. doi: 10.13811/j.cnki.eer.2019.02.012
Wurdinger, S., and Qureshi, M. (2015). Enhancing college students’ life skills through project based learning. Innov. High. Educ. 40, 279–286. doi: 10.1007/s10755-014-9314-3
Xiaolei, H. (2021). Research on the design and practice of high school information technology curriculum based on project-based learning . [Master’s thesis]. China: Southwest University Available at: https://kns.cnki.net/KCMS/detail/detail.aspx?dbname=CMFD202201&filename=1021768096.nh
Xu, C. (2022). A practical study of project-based learning for developing core literacy in subjects . Master’s thesis]. Southwest University. Available at: https://kns.cnki.net/KCMS/detail/detail.aspx?dbname=CMFD202201&filename=1021767951.nh
Xuezhi, Li. (2022). Research on the design and practice of project-based learning in junior high school mathematics curriculum . [Master’s thesis]. China: Ningxia University https://kns.cnki.net/KCMS/detail/detail.aspx?dbname=CMFD202301&filename=1022059895.nh
Yanan, H. (2020). An experimental study of Jigsaw-based project-based learning in elementary school IT class . [Master’s thesis]. China: Loudoun University. Available at: https://kns.cnki.net/KCMS/detail/detail.aspx?dbname=CMFD202101\u0026amp;filename=1020380160.nh
Yang, X. (2020), Practical research on teaching reform of information technology curriculum in Baochang No. 1 Middle School . [Master’s thesis]. China: Inner Mongolia Normal University. Available at: https://kns.cnki.net/KCMS/detail/detail.aspx?dbname=CMFD201801&filename=1017093188.nh
Yating, B. (2022). Research on the design and practice of teaching elemental compounds in high school based on project-based learning . Master’s thesis]. China: Fuyang Normal University. Available at: https://kns.cnki.net/KCMS/detail/detail.aspx?dbname=CMFD202301&filename=1022640350.nh
Yexin, Li. (2019) The application of robot project learning in middle school teaching research .
Ying, Z. (2022). A study on the influence of project-based teaching on the intrinsic motivation of private university students’ English learning. J. Sci. Educ . 13, 29–31. doi: 10.16400/j.cnki.kjdk.2022.13.010
Yuan, X.-F. (2017). A Study on the Teaching Reform of Information Technology Course in Baochang No.1 Middle School - Taking “Project-based Learning” as an Example 2017. Available at: https://kns.cnki.net/KCMS/detail/detail.aspx?dbname=CMFD201801&filename=1017093188.nh
Yun, X. (2022). Practical exploration of project-oriented deep ritual education -- take the project-oriented learning of searching for roots Xu Huiyuan in grade 5 as an example. Shanghai J. Educ. Res. 9, 64–68. doi: 10.16194/j.cnki.31-1059/g4.2022.09.006
Yuting, Tang. (2022). Practical research on project-based learning based on the development of scientific inquiry ability in secondary school biology teaching . [Master’s thesis]. China: Guizhou Normal University. Available at: https://kns.cnki.net/KCMS/detail/detail.aspx?dbname=CMFD202202\u0026amp;filename=1022573120.nh
Zhang, Y. (2022). A study on the effect of project-based teaching on the intrinsic motivation of private university students’ English learning. J. Sci. Educ. 13, 29SPi_ENDASH31. doi: 10.16400/j.cnki.kjdk.2022.13.010
Zhang, Ji-hong. (2022) Based on the junior high school students information technology subject core. Mesh type teaching mode design and application research .
Zulaeha, D., and Marpaung, D. (2020). Project-based learning approach to improve students’ writing skill. PROJECT 3:120. doi: 10.22460/project.v3i1.p120-126
Keywords: project-based learning, learning effects, 21st century skills, higher-order thinking, meta-analysis
Citation: Zhang L and Ma Y (2023) A study of the impact of project-based learning on student learning effects: a meta-analysis study. Front. Psychol . 14:1202728. doi: 10.3389/fpsyg.2023.1202728
Received: 09 April 2023; Accepted: 13 June 2023; Published: 17 July 2023.
Reviewed by:
Copyright © 2023 Zhang and Ma. This is an open-access article distributed under the terms of the Creative Commons Attribution License (CC BY) . The use, distribution or reproduction in other forums is permitted, provided the original author(s) and the copyright owner(s) are credited and that the original publication in this journal is cited, in accordance with accepted academic practice. No use, distribution or reproduction is permitted which does not comply with these terms.
*Correspondence: Yan Ma, [email protected]
Disclaimer: All claims expressed in this article are solely those of the authors and do not necessarily represent those of their affiliated organizations, or those of the publisher, the editors and the reviewers. Any product that may be evaluated in this article or claim that may be made by its manufacturer is not guaranteed or endorsed by the publisher.

An official website of the United States government
The .gov means it’s official. Federal government websites often end in .gov or .mil. Before sharing sensitive information, make sure you’re on a federal government site.
The site is secure. The https:// ensures that you are connecting to the official website and that any information you provide is encrypted and transmitted securely.
- Publications
- Account settings
Preview improvements coming to the PMC website in October 2024. Learn More or Try it out now .
- Advanced Search
- Journal List
- Med Sci Educ
- v.31(3); 2021 Jun

Effective Learning Behavior in Problem-Based Learning: a Scoping Review
Azril shahreez abdul ghani.
1 Department of Basic Medical Sciences, Kulliyah of Medicine, Bandar Indera Mahkota Campus, International Islamic University Malaysia, Kuantan, 25200 Pahang Malaysia
2 Department of Medical Education, School of Medical Sciences, Health Campus, Universiti Sains Malaysia, Kubang Kerian, Kota Bharu, 16150 Kelantan Malaysia
Ahmad Fuad Abdul Rahim
Muhamad saiful bahri yusoff, siti nurma hanim hadie.
3 Department of Anatomy, School of Medical Sciences, Health Campus, Universiti Sains Malaysia, Kubang Kerian, 16150 Kota Bharu, Kelantan Malaysia
Problem-based learning (PBL) emphasizes learning behavior that leads to critical thinking, problem-solving, communication, and collaborative skills in preparing students for a professional medical career. However, learning behavior that develops these skills has not been systematically described. This review aimed to unearth the elements of effective learning behavior in a PBL context, using the protocol by Arksey and O’Malley. The protocol identified the research question, selected relevant studies, charted and collected data, and collated, summarized, and reported results. We discovered three categories of elements—intrinsic empowerment, entrustment, and functional skills—proven effective in the achievement of learning outcomes in PBL.
Introduction
Problem-based learning (PBL) is an educational approach that utilizes the principles of collaborative learning in small groups, first introduced by McMaster Medical University [ 1 ]. The shift of the higher education curriculum from traditional, lecture-based approaches to an integrated, student-centered approach was triggered by concern over the content-driven nature of medical knowledge with minimal clinical application [ 2 ]. The PBL pedagogy uses a systematic approach, starting with an authentic, real-life problem scenario as a context in which learning is not separated from practice as students collaborate and learn [ 3 ]. The tutor acts as a facilitator who guides the students’ learning, while students are required to solve the problems by discussing them with group members [ 4 ]. The essential aspect of the PBL process is the ability of the students to recognize their current knowledge, determine the gaps in their knowledge and experience, and acquire new knowledge to bridge the gaps [ 5 ]. PBL is a holistic approach that gives students an active role in their learning.
Since its inception, PBL has been used in many undergraduate and postgraduate degree programs, such as medicine [ 6 , 7 ], nursing [ 8 ], social work education [ 9 ], law [ 10 ], architecture [ 11 ], economics [ 12 ], business [ 13 ], science [ 14 ], and engineering [ 15 ]. It has also been applied in elementary and secondary education [ 16 – 18 ]. Despite its many applications, its implementation is based on a single universal workflow framework that contains three elements: problem as the initiator for learning, tutor as a facilitator in the group versions, and group work as a stimulus for collaborative interaction [ 19 ]. However, there are various versions of PBL workflow, such as the seven-step technique based on the Maastricht “seven jumps” process. The tutor’s role is to ensure the achievement of learning objectives and to assess students’ performance [ 20 , 21 ].
The PBL process revolves around four types of learning principles: constructive, self-directed, collaborative, and contextual [ 19 ]. Through the constructive learning process, the students are encouraged to think about what is already known and integrate their prior knowledge with their new understanding. This process helps the student understand the content, form a new opinion, and acquire new knowledge [ 22 ]. The PBL process encourages students to become self-directed learners who plan, monitor, and evaluate their own learning, enabling them to become lifelong learners [ 23 ]. The contextualized collaborative learning process also promotes interaction among students, who share similar responsibilities to achieve common goals relevant to the learning context [ 24 ]. By exchanging ideas and providing feedback during the learning session, the students can attain a greater understanding of the subject matter [ 25 ].
Dolmans et al. [ 19 ] pointed out two issues related to the implementation of PBL: dominant facilitators and dysfunctional PBL groups. These problems inhibit students’ self-directed learning and reduce their satisfaction level with the PBL session. A case study by Eryilmaz [ 26 ] that evaluated engineering students’ and tutors’ experience of PBL discovered that PBL increased the students’ self-confidence and improved essential skills such as problem-solving, communications, critical thinking, and collaboration. Although most of the participants in the study found PBL satisfactory, many complained about the tutor’s poor guidance and lack of preparation. Additionally, it was noted that 64% of the first-year students were unable to adapt to the PBL system because they had been accustomed to conventional learning settings and that 43% of students were not adequately prepared for the sessions and thus were minimally involved in the discussion.
In a case study by Cónsul-giribet [ 27 ], newly graduated nursing professionals reported a lack of perceived theoretical basic science knowledge at the end of their program, despite learning through PBL. The nurses perceived that this lack of knowledge might affect their expertise, identity, and professional image.
Likewise, a study by McKendree [ 28 ] reported the outcomes of a workshop that explored the strengths and weaknesses of PBL in an allied health sciences curriculum in the UK. The workshop found that problems related to PBL were mainly caused by students, the majority of whom came from conventional educational backgrounds either during high school or their first degree. They felt anxious when they were involved in PBL, concerned about “not knowing when to stop” in exploring the learning needs. Apart from a lack of basic science knowledge, the knowledge acquired during PBL sessions remains unorganized [ 29 ]. Hence, tutors must guide students in overcoming this situation by instilling appropriate insights and essential skills for the achievement of the learning outcomes [ 30 ]. It was also evident that the combination of intention and motivation to learn and desirable learning behavior determined the quality of learning outcomes [ 31 , 32 ]. However, effective learning behaviors that help develop these skills have not been systematically described. Thus, this scoping review aimed to unearth the elements of effective learning behavior in the PBL context.
Scoping Review Protocol
This scoping review was performed using a protocol by Arksey and O’Malley [ 33 ]. The protocol comprises five phases: (i) identification of research questions, (ii) identification of relevant articles, (iii) selection of relevant studies, (iv) data collection and charting, and (v) collating, summarizing, and reporting the results.
Identification of Research Questions
This scoping review was designed to unearth the elements of effective learning behavior that can be generated from learning through PBL instruction. The review aimed to answer one research question: “What are the effective learning behavior elements related to PBL?” For the purpose of the review, an operational definition of effective learning behavior was constructed, whereby it was defined as any learning behavior that is related to PBL instruction and has been shown to successfully attain the desired learning outcomes (i.e., cognitive, skill, or affective)—either quantitatively or qualitatively—in any intervention conducted in higher education institutions.
The positive outcome variables include student viewpoint or perception, student learning experience and performance, lecturer viewpoint and expert judgment, and other indirect variables that may be important indicators of successful PBL learning (i.e., attendance to PBL session, participation in PBL activity, number of interactions in PBL activity, and improvement in communication skills in PBL).
Identification of Relevant Articles
An extensive literature search was conducted on articles published in English between 2015 and 2019. Three databases—Google Scholar, Scopus, and PubMed—were used for the literature search. Seven search terms with the Boolean combination were used, whereby the keywords were identified from the Medical Subject Headings (MeSH) and Education Resources Information Center (ERIC) databases. The search terms were tested and refined with multiple test searches. The final search terms with the Boolean operation were as follows: “problem-based learning” AND (“learning behavior” OR “learning behaviour”) AND (student OR “medical students” OR undergraduate OR “medical education”).
Selection of Relevant Articles
The articles from the three databases were exported manually into Microsoft Excel. The duplicates were removed, and the remaining articles were reviewed based on the inclusion and exclusion criteria. These criteria were tested on titles and abstracts to ensure their robustness in capturing the articles related to learning behavior in PBL. The shortlisted articles were reviewed by two independent researchers, and a consensus was reached either to accept or reject each article based on the set criteria. When a disagreement occurred between the two reviewers, the particular article was re-evaluated independently by the third and fourth researchers (M.S.B.Y and A.F.A.R), who have vast experience in conducting qualitative research. The sets of criteria for selecting abstracts and final articles were developed. The inclusion and exclusion criteria are listed in Table Table1 1 .
Inclusion and exclusion criteria
Criteria | Inclusion criteria | Exclusion criteria |
---|---|---|
Criteria for abstract selection | 1. Describe at least one effective learning behaviour in PBL setting in higher education setting 2. Provides evidence of a robust study design that is not limited to randomized controlled trials 3. Provides evidence of evaluation of a PBL 4. Outcomes of the study that are measurable either quantitatively or qualitatively | 1. Primary and secondary students’ populations 2. Primary and secondary education context |
Criteria for full article selection | 1. Elaboration on the elements of effective learning behaviour are provided 2. Clear methodology on the measurement of the outcome 3. PBL context 4. Functional element that has been proven to promote learning 5. Well design research intervention | 1. Review articles, published theses, books, research report, editorial and letters will be excluded from the searching process |
Data Charting
The selected final articles were reviewed, and several important data were extracted to provide an objective summary of the review. The extracted data were charted in a table, including the (i) title of the article, (ii) author(s), (iii) year of publication, (iv) aim or purpose of the study, (v) study design and method, (iv) intervention performed, and (v) study population and sample size.
Collating, Summarizing, and Reporting the Results
A content analysis was performed to identify the elements of effective learning behaviors in the literature by A.S.A.G and S.N.H.H, who have experience in conducting qualitative studies. The initial step of content analysis was to read the selected articles thoroughly to gain a general understanding of the articles and extract the elements of learning behavior which are available in the articles. Next, the elements of learning behavior that fulfil the inclusion criteria were extracted. The selected elements that were related to each other through their content or context were grouped into subtheme categories. Subsequently, the combinations of several subthemes expressing similar underlying meanings were grouped into themes. Each of the themes and subthemes was given a name, which was operationally defined based on the underlying elements. The selected themes and subthemes were presented to the independent researchers in the team (M.S.B.Y and A.F.A.R), and a consensus was reached either to accept or reformulate each of the themes and subthemes. The flow of the scoping review methods for this study is illustrated in Fig. 1 .

The flow of literature search and article selection
Literature Search
Based on the keyword search, 1750 articles were obtained. Duplicate articles that were not original articles found in different databases and resources were removed. Based on the inclusion and exclusion criteria of title selection, the eligibility of 1750 abstracts was evaluated. The articles that did not fulfil the criteria were removed, leaving 328 articles for abstract screening. A total of 284 articles were screened according to the eligibility criteria for abstract selection. Based on these criteria, 284 articles were selected and screened according to the eligibility criteria for full article selection. Fourteen articles were selected for the final review. The information about these articles is summarized in Table Table2 2 .
Studies characteristics
Author (year) | Location | Study design/method | Subjects | Intervention | Outcome |
---|---|---|---|---|---|
Arana-Arexolaleiba et al. [ ] | Spain | Quasi-experimental design (one group pretest–posttest design) Questionnaire only | 97 undergraduate engineering students and 20 tutors | Assessing PBL learning environment and supervision on student learning approach | Environments with higher constructive variables and supervisor formative assessment stimulate deeper learning approach in students |
Khoiriyah et al. [ ] | Indonesia | Quasi-experimental design (one group posttest-only design) and semi-structured interview Questionnaire & Interview protocol | 310 undergraduate students, 10 tutors and 15 content experts | Evaluating self-assessment scale for active learning and critical thinking (SSACT) in PBL | SSACT improves students critical thinking and self-directed learning |
Khumsikiew et al. [ ] | Thailand | Quasi-experimental design (one group pretest–posttest design) Questionnaire only | 36 undergraduate pharmacy students | Assessing the effect of student competence in PBL with clinical environment | Student clinical skills performance and satisfaction was significantly increase in the PBL with clinical environment |
Rakhudu [ ] | South Africa | Sequential explanatory mixed method design and focus group discussion Questionnaire | 135 undergraduate nursing students (2011–2013 academic year) 21 participate in FGD 114 participate in questionnaire | Evaluating the effect of PBL scenario in quality improvement in health care unit on nursing student | PBL scenario effective in promoting interdisciplinary and interinstitutional collaboration |
Tarhan et al. [ ] | Turkey | Quasi-experimental design (one group pretest–posttest design) and semi-structured interview Questionnaire and Interviews protocol | 36 undergraduate biochemistry course students | Evaluating the effect of PBL on student interest in biochemistry course | PBL Improve students investigating process, associate information’s, collaborative skills, responsibility and idea expressions |
Chou et al. [ ] | China | Sequential explanatory mixed method design Observation checklist and post-PBL homework reflections | 45 undergraduate medical students and 44 undergraduate nursing students All students participate All students participate but only the IP groups were analyzed | Assessing the effect interprofessional PBL in learning clinical ethics | The IPE learning through PBL improve respect towards each other and avoid the development of stereotyped behavior |
Chung et al. [ ] | China | Quasi-experimental design (one group pretest–posttest design) and action research Observation, instructional journal, interviews protocol and questionnaire | 51 undergraduate business students | Evaluating the effect of PBL on students learning outcome s of industrial-oriented competences | Significantly enhanced students’ learning motivation, learning outcomes and development of instructional knowledge and capability |
Geitz et al. [ ] | Netherlands | Semi-structured interview Interview protocol | 62 undergraduate students and 4 tutors in business administration 8 students (selected randomly) and all 4 tutors were selected for the qualitative study | Evaluating the effect of sustainable feedback on self-efficacy and goal orientation given during the PBL sessions | PBL participants positively valued the feedback, their personal characteristics, previous experience with feedback and concomitant perceptions appeared to have greatly influenced both tutors’ and students’ specific, individual behavior, and responses |
Dawilai et al. [ ] | Thailand | Quasi-experimental design (one group posttest-only design) and interview Questionnaire and interview protocol | 29 English foreign language students All participate in the questionnaire 10 students with improvement in writing course were selected for the interview | Evaluating self-regulated learning in problem-based blended learning (PBBL) | PBBL students reported to apply cognitive strategy and effectively used their time and study environment |
Gutman [ ] | Israel | Quasi-experimental design (non-equivalent control group posttest-only design) Questionnaire only | 62 pre-service teachers | Evaluating achievement goal motivation (AGM) and research literacy skills (RL) between PBL process scaffolding with moderator-based learning (OLC + M) and social based learning (OLC + S) | The PBL participants reported to show significant improvement in AGM Only OLC + S showed significant improvement in RL |
Li [ ] | China | Semi-structured interview Interview protocol | 14 students | Evaluating student learning outcome and attitude between single disciplinary course PBL and lecture | The PBL participants reported to have better outcome in interdisciplinary learning, self-directed learning, problem solving, creative thinking, communication and knowledge retentions. They also showed positive attitude of PBL is they recognize its effectiveness in skill development rather than exam oriented |
Asad et al. [ ] | Saudi Arabia | Cross-sectional study (period cross sectional) Questionnaire only | 120 undergraduate medical students | Evaluating student opinion on effectiveness of PBL and interactive lectures | The PBL participants reported to have better outcome in modes of learning facilitation, professional development, learning behavior, and environment |
Hursen [ ] | Cyprus | Quasi-experimental design (one group pretest–posttest design) and interview Questionnaire and interview protocol | 25 students | Evaluating the effect of using Facebook in PBL on adults’ self-efficacy perception for research inquiry | The PBL participants reported to have positive increase in perception of self-efficacy for sustaining research |
William et al. [ ] | Singapore | Quasi-experimental design (non-equivalent control group posttest-only design) Questionnaire only | 149 students | Evaluating the effect of supply chain game in PBL environment | The game based PBL reported to increase score on metacognition function and motivation function. The game based PBL also showed significant correlation between motivation and positive game experience with the students’ perceived learning |
Study Characteristics
The final 14 articles were published between 2015 and 2019. The majority of the studies were conducted in Western Asian countries ( n = 4), followed by China ( n = 3), European countries ( n = 2), Thailand ( n = 2), Indonesia ( n = 1), Singapore ( n = 1), and South Africa ( n = 1). Apart from traditional PBL, some studies incorporated other pedagogic modalities into their PBL sessions, such as online learning, blended learning, and gamification. The majority of the studies targeted a single-profession learner group, and one study was performed on mixed interprofessional health education learners.
Results of Thematic Analysis
The thematic analysis yielded three main themes of effective learning behavior: intrinsic empowerment, entrustment, and functional skills. Intrinsic empowerment overlies four proposed subthemes: proactivity, organization, diligence, and resourcefulness. For entrustment, there were four underlying subthemes: students as assessors, students as teachers, feedback-giving, and feedback-receiving. The functional skills theme contains four subthemes: time management, digital proficiency, data management, and collaboration.
Theme 1: Intrinsic Empowerment
Intrinsic empowerment enforces student learning behavior that can facilitate the achievement of learning outcomes. By empowering the development of these behaviors, students can become lifelong learners [ 34 ]. The first element of intrinsic empowerment is proactive behavior. In PBL, the students must be proactive in analyzing problems [ 35 , 36 ] and their learning needs [ 35 , 37 ], and this can be done by integrating prior knowledge and previous experience through a brainstorming session [ 35 , 38 ]. The students must be proactive in seeking guidance to ensure they stay focused and confident [ 39 , 40 ]. Finding ways to integrate content from different disciplines [ 35 , 41 ], formulate new explanations based on known facts [ 34 , 35 , 41 ], and incorporate hands-on activity [ 35 , 39 , 42 ] during a PBL session are also proactive behaviors.
The second element identified is “being organized” which reflects the ability of students to systematically manage their roles [ 43 ], ideas, and learning needs [ 34 ]. The students also need to understand the task for each learning role in PBL, such as chairperson or leader, scribe, recorder, and reflector. This role needs to be assigned appropriately to ensure that all members take part in the discussion [ 43 ]. Similarly, when discussing ideas or learning needs, the students need to follow the steps in the PBL process and organize and prioritize the information to ensure that the issues are discussed systematically and all aspects of the problems are covered accordingly [ 34 , 37 ]. This team organization and systematic thought process is an effective way for students to focus, plan, and finalize their learning tasks.
The third element of intrinsic empowerment is “being diligent.” Students must consistently conduct self-revision [ 40 ] and keep track of their learning plan to ensure the achievement of their learning goal [ 4 , 40 ]. The students must also be responsible for completing any given task and ensuring good understanding prior to their presentation [ 40 ]. Appropriate actions need to be undertaken to find solutions to unsolved problems [ 40 , 44 ]. This effort will help them think critically and apply their knowledge for problem-solving.
The fourth element identified is “being resourceful.” Students should be able to acquire knowledge from different resources, which include external resources (i.e., lecture notes, textbooks, journal articles, audiovisual instructions, the Internet) [ 38 , 40 , 45 ] and internal resources (i.e., students’ prior knowledge or experience) [ 35 , 39 ]. The resources must be evidence-based, and thus should be carefully selected by evaluating their cross-references and appraising them critically [ 37 ]. Students should also be able to understand and summarize the learned materials and explain them using their own words [ 4 , 34 ]. The subthemes of the intrinsic empowerment theme are summarized in Table Table3 3 .
Intrinsic empowerment subtheme with the learning behavior elements
Intrinsic empowerment | |||
---|---|---|---|
Proactive | Being organized | Being diligent | Resourceful |
• Analyze problems and learning needs • Seek guidance • Integrate subjects from different disciplines • Incorporate hands on activities | • Organize PBL team by assigning roles • Organize discussed ideas or learning needs • Prioritize ideas or learning needs | • Consistent in self-study • Keep track with plans • Responsible in completing the task • Responsible in understanding the learning materials | • Use various resources • Appraise the resources • Use evidence-based resources • Paraphrase the resources |
Theme 2: Entrustment
Entrustment emphasizes the various roles of students in PBL that can promote effective learning. The first entrusted role identified is “student as an assessor.” This means that students evaluate their own performance in PBL [ 46 ]. The evaluation of their own performance must be based on the achievement of the learning outcomes and reflect actual understanding of the content as well as the ability to apply the learned information in problem-solving [ 46 ].
The second element identified in this review is “student as a teacher.” To ensure successful peer teaching in PBL, students need to comprehensively understand the content of the learning materials and summarize the content in an organized manner. The students should be able to explain the gist of the discussed information using their own words [ 4 , 34 ] and utilize teaching methods to cater to differences in learning styles (i.e., visual, auditory, and kinesthetic) [ 41 ]. These strategies help capture their group members’ attention and evoke interactive discussions among them.
The third element of entrustment is to “give feedback.” Students should try giving constructive feedback on individual and group performance in PBL. Feedback on individual performance must reflect the quality of the content and task presented in the PBL. Feedback on group performance should reflect the ways in which the group members communicate and complete the group task [ 47 ]. To ensure continuous constructive feedback, students should be able to generate feedback questions beforehand and immediately deliver them during the PBL sessions [ 44 , 47 ]. In addition, the feedback must include specific measures for improvement to help their peers to take appropriate action for the future [ 47 ].
The fourth element of entrustment is “receive feedback.” Students should listen carefully to the feedback given and ask questions to clarify the feedback [ 47 ]. They need to be attentive and learn to deal with negative feedback [ 47 ]. Also, if the student does not receive feedback, they should request it either from peers or teachers and ask specific questions, such as what aspects to improve and how to improve [ 47 ]. The data on the subthemes of the entrustment theme are summarized in Table Table4 4 .
Entrustment subtheme with the learning behavior elements
Entrustment | |||
---|---|---|---|
Student as assessor | Student as teacher | Give feedback | Receive feedback |
• Evaluate individual performance • Evaluate group performance | • Prepare teaching materials • Use various learning styles | • Give feedback on individual task • Give feedback on group learning process • Prepare feedback questions beforehand • Suggest measures for future improvement | • Clarify feedback • Request feedback from peers and teachers |
Theme 3: Functional Skills
Functional skills refer to essential skills that can help students learn independently and competently. The first element identified is time management skills. In PBL, students must know how to prioritize learning tasks according to the needs and urgency of the tasks [ 40 ]. To ensure that students can self-pace their learning, a deadline should be set for each learning task within a manageable and achievable learning schedule [ 40 ].
Furthermore, students should have digital proficiency, the ability to utilize digital devices to support learning [ 38 , 40 , 44 ]. The student needs to know how to operate basic software (e.g., Words and PowerPoints) and the basic digital tools (i.e., social media, cloud storage, simulation, and online community learning platforms) to support their learning [ 39 , 40 ]. These skills are important for peer learning activities, which may require information sharing, information retrieval, online peer discussion, and online peer feedback [ 38 , 44 ].
The third functional skill identified is data management, the ability to collect key information in the PBL trigger and analyze that information to support the solution in a problem-solving activity [ 39 ]. Students need to work either individually or in a group to collect the key information from a different trigger or case format such as text lines, an interview, an investigation, or statistical results [ 39 ]. Subsequently, students also need to analyze the information and draw conclusions based on their analysis [ 39 ].
The fourth element of functional skill is collaboration. Students need to participate equally in the PBL discussion [ 41 , 46 ]. Through discussion, confusion and queries can be addressed and resolved by listening, respecting others’ viewpoints, and responding professionally [ 35 , 39 , 43 , 44 ]. In addition, the students need to learn from each other and reflect on their performance [ 48 ]. Table Table5 5 summarizes the data on the subthemes of the functional skills theme.
Functional skills subtheme with the learning behavior elements
Functional skills | |||
---|---|---|---|
Time management | Digital proficiency | Data management | Collaborative skill |
• Create learning schedule • Set up deadline for each task • Prioritize work for each task | • Use digital devices • Use digital tools | • Collect data • Analyze data | • Discuss professionally • Learn from each other |
This scoping review outlines three themes of effective learning behavior elements in the PBL context: intrinsic empowerment, entrustment, and functional skills. Hence, it is evident from this review that successful PBL instruction demands students’ commitment to empower themselves with value-driven behaviors, skills, and roles.
In this review, intrinsic empowerment is viewed as enforcement of students’ internal strength in performing positive learning behaviors related to PBL. This theme requires the student to proactively engage in the learning process, organize their learning activities systematically, persevere in learning, and be intelligently resourceful. One of the elements of intrinsic empowerment is the identification and analysis of problems related to complex scenarios. This element is aligned with a study by Meyer [ 49 ], who observed students’ engagement in problem identification and clarification prior to problem-solving activities in a PBL session related to multiple engineering design. Rubenstein and colleagues [ 50 ] discovered in a semi-structured interview the importance of undergoing a problem identification process before proposing a solution during learning. It was reported that the problem identification process in PBL may enhance the attainment of learning outcomes, specifically in the domain of concept understanding [ 51 ].
The ability of the students to acquire and manage learning resources is essential for building their understanding of the learned materials and enriching discussion among team members during PBL. This is aligned with a study by Jeong and Hmelo-Silver [ 52 ], who studied the use of learning resources by students in PBL. The study concluded that in a resource-rich environment, the students need to learn how to access and understand the resources to ensure effective learning. Secondly, they need to process the content of the resources, integrate various resources, and apply them in problem-solving activities. Finally, they need to use the resources in collaborative learning activities, such as sharing and relating to peer resources.
Wong [ 53 ] documented that excellent students spent considerably more time managing academic resources than low achievers. The ability of the student to identify and utilize their internal learning resources, such as prior knowledge and experience, is also important. A study by Lee et al. [ 54 ] has shown that participants with high domain-specific prior knowledge displayed a more systematic approach and high accuracy in visual and motor reactions in solving problems compared to novice learners.
During the discussion phase in PBL, organizing ideas—e.g., arranging relevant information gathered from the learning resources into relevant categories—is essential for communicating the idea clearly [ 34 ]. This finding is in line with a typology study conducted by Larue [ 55 ] on second-year nursing students’ learning strategies during a group discussion. The study discovered that although the content presented by the student is adequate, they unable to make further progress in the group discussion until they are instructed by the tutor on how to organize the information given into a category [ 55 ].
Hence, the empowerment of student intrinsic behavior may enhance students’ learning in PBL by allowing them to make a decision in their learning objectives and instilling confidence in them to achieve goals. A study conducted by Kirk et al. [ 56 ] proved that highly empowered students obtain better grades, increase learning participation, and target higher educational aspirations.
Entrustment is the learning role given to students to be engaging and identify gaps in their learning. This theme requires the student to engage in self-assessment, prepare to teach others, give constructive feedback, and value the feedback received. One of the elements of entrustment is the ability to self-assess. In a study conducted by Mohd et al. [ 57 ] looking at the factors in PBL that can strengthen the capability of IT students, they discovered that one of the critical factors that contribute to these skills is the ability of the student to perform self-assessment in PBL. As mentioned by Daud, Kassim, and Daud [ 58 ], the self-assessment may be more reliable if the assessment is performed based on the objectives set beforehand and if the criteria of the assessment are understood by the learner. This is important to avoid the fact that the result of the self-assessment is influenced by the students’ perception of themselves rather than reflecting their true performance. However, having an assessment based on the learning objective only focuses on the immediate learning requirements in the PBL. To foster lifelong learning skills, it should also be balanced with the long-term focus of assessment, such as utilizing the assessment to foster the application of knowledge in solving real-life situations. This is aligned with the review by Boud and Falchikov [ 59 ] suggesting that students need to become assessors within the concept of participation in practice, that is, the kind that is within the context of real life and work.
The second subtheme of entrustment is “students as a teacher” in PBL. In our review, the student needs to be well prepared with the teaching materials. A cross-sectional study conducted by Charoensakulchai and colleagues discovered that student preparation is considered among the important factors in PBL success, alongside other factors such as “objective and contents,” “student assessment,” and “attitude towards group work” [ 60 ]. This is also aligned with a study conducted by Sukrajh [ 61 ] using focus group discussion on fifth-year medical students to explore their perception of preparedness before conducting peer teaching activity. In this study, the student in the focus group expressed that the preparation made them more confident in teaching others because preparing stimulated them to activate and revise prior knowledge, discover their knowledge gaps, construct new knowledge, reflect on their learning, improve their memory, inspire them to search several resources, and motivate them to learn the topics.
The next element of “student as a teacher” is using various learning styles to teach other members in the group. A study conducted by Almomani [ 62 ] showed that the most preferred learning pattern by the high school student is the visual pattern, followed by auditory pattern and then kinesthetic. However, in the university setting, Hamdani [ 63 ] discovered that students prefer a combination of the three learning styles. Anbarasi [ 64 ] also explained that incorporating teaching methods based on the student’s preferred learning style further promotes active learning among the students and significantly improved the long-term retrieval of knowledge. However, among the three learning styles group, he discovered that the kinesthetic group with the kinesthetic teaching method showed a significantly higher post-test score compared to the traditional group with the didactic teaching method, and he concluded that this is because of the involvement of more active learning activity in the kinesthetic group.
The ability of students to give constructive feedback on individual tasks is an important element in promoting student contribution in PBL because feedback from peers or teachers is needed to reassure themselves that they are on the right track in the learning process. Kamp et al. [ 65 ] performed a study on the effectiveness of midterm peer feedback on student individual cognitive, collaborative, and motivational contributions in PBL. The experimental group that received midterm peer feedback combined with goal-setting with face-to-face discussion showed an increased amount of individual contributions in PBL. Another element of effective feedback is that the feedback is given immediately after the observed behavior. Parikh and colleagues survey student feedback in PBL environments among 103 final-year medical students in five Ontario schools, including the University of Toronto, McMaster University, Queens University, the University of Ottawa, and the University of Western Ontario. They discovered that there was a dramatic difference between McMaster University and other universities in the immediacy of feedback they practiced. Seventy percent of students at McMaster reported receiving immediate feedback in PBL, compared to less than 40 percent of students from the other universities, in which most of them received feedback within one week or several weeks after the PBL had been conducted [ 66 ]. Another study, conducted among students of the International Medical University of Kuala Lumpur examining the student expectation on feedback, discovered that immediate feedback is effective if the feedback is in written form, simple but focused on the area of improvement, and delivered by a content expert. If the feedback is delivered by a content non-expert and using a model answer, it must be supplemented with teacher dialogue sessions to clarify the feedback received [ 67 ].
Requesting feedback from peers and teachers is an important element of the PBL learning environment, enabling students to discover their learning gaps and ways to fill them. This is aligned with a study conducted by de Jong and colleagues [ 68 ], who discovered that high-performing students are more motivated to seek feedback than low-performing students. The main reason for this is because high-performing students seek feedback as a tool to learn from, whereas low-performing students do so as an academic requirement. This resulted in high-performing students collecting more feedback. A study by Bose and Gijselaers [ 69 ] examined the factors that promote feedback-seeking behavior in medical residency. They discovered that feedback-seeking behavior can be promoted by providing residents with high-quality feedback to motivate them to ask for feedback for improvement.
By assigning an active role to students as teachers, assessors, and feedback providers, teachers give them the ownership and responsibility to craft their learning. The learner will then learn the skills to monitor and reflect on their learning to achieve academic success. Furthermore, an active role encourages students to be evaluative experts in their own learning, and promoting deep learning [ 70 ].
Functional skills refer to essential abilities for competently performing a task in PBL. This theme requires the student to organize and plan time for specific learning tasks, be digitally literate, use data effectively to support problem-solving, and work together efficiently to achieve agreed objectives. One of the elements in this theme is to have a schedule of learning tasks with deadlines. In a study conducted by Tadjer and colleagues [ 71 ], they discovered that setting deadlines with a restricted time period in a group activity improved students’ cognitive abilities and soft skills. Although the deadline may initially cause anxiety, coping with it encourages students to become more creative and energetic in performing various learning strategies [ 72 , 73 ]. Ballard et al. [ 74 ] reported that students tend to work harder to complete learning tasks if they face multiple deadlines.
The students also need to be digitally literate—i.e., able to demonstrate the use of technological devices and tools in PBL. Taradi et al. [ 75 ] discovered that incorporating technology in learning—blending web technology with PBL—removes time and place barriers in the creation of a collaborative environment. It was found that students who participated in web discussions achieved a significantly higher mean grade on a physiology final examination than those who used traditional methods. Also, the incorporation of an online platform in PBL can facilitate students to develop investigation and inquiry skills with high-level cognitive thought processes, which is crucial to successful problem-solving [ 76 ].
In PBL, students need to work collaboratively with their peers to solve problems. A study by Hidayati et al. [ 77 ] demonstrated that effective collaborative skills improve cognitive learning outcomes and problem-solving ability among students who undergo PBL integrated with digital mind maps. To ensure successful collaborative learning in PBL, professional communication among students is pertinent. Research by Zheng and Huang [ 78 ] has proven that co-regulation (i.e., warm and responsive communication that provides support to peers) improved collaborative effort and group performance among undergraduate and master’s students majoring in education and psychology. This is also in line with a study by Maraj and colleagues [ 79 ], which showed the strong team interaction within the PBL group leads to a high level of team efficacy and academic self-efficacy. Moreover, strengthening communication competence, such as by developing negotiation skills among partners during discussion sessions, improves student scores [ 80 ].
PBL also includes opportunities for students to learn from each other (i.e., peer learning). A study by Maraj et al. [ 79 ] discovered that the majority of the students in their study perceived improvement in their understanding of the learned subject when they learned from each other. Another study by Lyonga [ 81 ] documented the successful formation of cohesive group learning, where students could express and share their ideas with their friends and help each other. It was suggested that each student should be paired with a more knowledgeable student who has mastered certain learning components to promote purposeful structured learning within the group.
From this scoping review, it is clear that functional skills equip the students with abilities and knowledge needed for successful PBL. Studies have shown that strong time management skills, digital literacy, data management, and collaborative skills lead to positive academic achievement [ 77 , 82 , 83 ].
Limitation of the Study
This scoping review is aimed to capture the recent effective learning behavior in problem-based learning; therefore, the literature before 2015 was not included. Without denying the importance of publication before 2015, we are relying on Okoli and Schabram [ 84 ] who highlighted the impossibility of retrieving all the published articles when conducting a literature search. Based on this ground, we decided to focus on the time frame between 2015 and 2019, which is aligned with the concepts of study maturity (i.e., the more mature the field, the higher the published articles and therefore more topics were investigated) by Kraus et al. [ 85 ]. In fact, it was noted that within this time frame, a significant number of articles have been found as relevant to PBL with the recent discovery of effective learning behavior. Nevertheless, our time frame did not include the timing of the coronavirus disease 19 (COVID-19) pandemic outbreak, which began at the end of 2019. Hence, we might miss some important elements of learning behavior that are required for the successful implementation of PBL during the COVID-19 pandemic.
Surprisingly, the results obtained from this study are also applicable for the PBL sessions administration during the COVID-19 pandemic situation as one of the functional skills identified is digital proficiency. This skill is indeed important for the successful implementation of online PBL session.
This review identified the essential learning behaviors required for effective PBL in higher education and clustered them into three main themes: (i) intrinsic empowerment, (ii) entrustment, and (iii) functional skills. These learning behaviors must coexist to ensure the achievement of desired learning outcomes. In fact, the findings of this study indicated two important implications for future practice. Firstly, the identified learning behaviors can be incorporated as functional elements in the PBL framework and implementation. Secondly, the learning behaviors change and adaption can be considered to be a new domain of formative assessment related to PBL. It is noteworthy to highlight that these learning behaviors could help in fostering the development of lifelong skills for future workplace challenges. Nevertheless, considerably more work should be carried out to design a solid guideline on how to systematically adopt the learning behaviors in PBL sessions, especially during this COVID-19 pandemic situation.
This study was supported by Postgraduate Incentive Grant-PhD (GIPS-PhD, grant number: 311/PPSP/4404803).
Declarations
The study has received an ethical approval from the Human Research Ethics Committee of Universiti Sains Malaysia.
No informed consent required for the scoping review.
The authors declare no competing interests.
Publisher's Note
Springer Nature remains neutral with regard to jurisdictional claims in published maps and institutional affiliations.
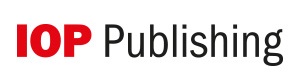
We apologize for the inconvenience...
To ensure we keep this website safe, please can you confirm you are a human by ticking the box below.
If you are unable to complete the above request please contact us using the below link, providing a screenshot of your experience.
https://ioppublishing.org/contacts/
- Our Mission
Project-Based Learning and the Research Paper
Students take responsibility for their learning and develop solutions for complex problems when their research paper becomes a PBL unit.
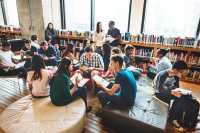
In 11th grade, students in my county are expected to generate a research paper or product. In the past, I stuck to the traditional paper, mostly because doing so was comfortable for me as an English teacher. I can do papers. I can do essays. I can provide feedback and teach revision.
However, last year I took a risk—instead of the traditional paper, I told my students we would be embarking on a project-based learning (PBL) journey. They seemed excited, mostly because they thought they wouldn’t have to write a paper. In the end, they did so much more than that.
Project-Based Learning in High School English
I had my students form groups and then gave each group the task of choosing an issue they were interested in. The project would involve coming up with a viable solution. As a class we brainstormed all kinds of big issues, including school shootings, poverty, LGBT rights, bullying, and homelessness, as well as local issues like the need for more options in school lunches, such as vegan and gluten- and diary-free options.
Next they had to brainstorm possible resources and questions they needed to answer. Therein lies the beauty of PBL: Since I couldn’t anticipate their every need, they had to take responsibility for their own learning, and solve problems as they encountered them.
Mini-Lessons and Formative Assessments
Their first formative assessment developed organically as they realized they would need to email professionals who could answer questions to guide their research. These adults included our school principal and our security team, as well as local government officials. Students reached out to their local delegates and other elected representatives, including the congresswoman for our district.
I gave a mini-lesson on how to write a formal email, and then the students composed their emails. Before they sent the messages, I previewed them, offering feedback and constructive criticism (formative assessment), and students made revisions.
By the next class period, my students had one of two versions of the same problem: Their recipients had responded or they hadn’t, but either way my students didn’t know what to do.
I gave a mini-lesson on how to send a follow-up email when someone doesn’t respond and on how to move forward when they do. They wrote follow-up emails, and again I previewed these. As we awaited responses, several groups asked if they could poll the staff or their peers about school lunch choices, improving school security, and expanding the school parking lot. This exercise could benefit all groups, so we decided to make it the next task.
I showed them how to create an online survey using Office 365. Their surveys had to contain at least two graphics and 10 good questions (open ended, multiple choice, or order of importance).
Meanwhile, students continued getting responses from their email recipients and needed to set up interviews with those people. How to write good interview questions became the next mini-lesson. My students were largely unaware of how to interview someone and didn’t realize that the questions they prepared were critical in gaining the evidence they needed to support their proposals.
For example, a group who wanted to expand the school parking lot went from asking broad questions like, “Do you think we need a larger parking lot?” to very specific ones like, “How many accidents have occurred in the parking lot since the school opened?” Most of the interviews were conducted over the phone, but some were in person—one group, with permission from their parents, interviewed members of a local homeless camp.
Students also had to find at least three solid sources and take notes to be embedded in their final product, with correct citations. They found statistics and data to support their proposals, and made sure to address counterarguments.
The Final Products
I gave my students options for their final products. All of them had to contain their survey results, research, and emails and interviews in one form or another. They came up with ideas like a public service announcement, a formal proposal, a bill, a documentary, a photo essay, or a piece of music or art.
I created rubrics and exemplars so students would know what I was expecting. I didn’t want to be too controlling, but I wanted high-quality products. They shared their final products with a variety of authentic audiences, including their congresswoman, our principal, a county supervisor, and our security team.
I gave each group one summative grade, but in the future I plan to split the grade: 70 percent of each student’s grade will be for their group’s work, and 30 percent will be an individual grade based on my observations, students’ self-reflections, and peer reflections.
As students shared their projects and we reflected on the process together, a few things became clear to me. First, I’ll never teach the research paper any other way because the PBL model we used helped develop real-world problem solvers, thinkers, and doers instead of rule followers. I learned that to encourage students to step out of their comfort zones, I too had to step out of mine, but beautiful, authentic learning happens when we create the right conditions for it.
Classroom Q&A
With larry ferlazzo.
In this EdWeek blog, an experiment in knowledge-gathering, Ferlazzo will address readers’ questions on classroom management, ELL instruction, lesson planning, and other issues facing teachers. Send your questions to [email protected]. Read more from this blog.
Project-Based Learning Helps Connect Lessons to Students’ Lives

- Share article
Today’s post wraps up a multipart series offering suggestions about how teachers can help students see that lessons are relevant to their lives outside of school.
Project-Based Learning
Janet B. Walton is Senior Research Scholar at North Carolina State University’s College of Education. She is a co-editor of the STEM Road Map curriculum series, a series of books that provides project-based curriculum for students in K-12:
Both educational research and teachers’ firsthand experiences tell us that students are more engaged in learning when they can draw connections between learning content and their own experiences. We also know that this engagement can lead to student learning that is deeper, more personally meaningful, and more effectively retained than learning that lacks such connections. These kinds of connections give students the sense that learning is relevant—or relatable and meaningful—to them.
Relevance in terms of lesson content and delivery can be thought about in a few different ways. Relevance can be personal, where academic content is related to students’ individual interests and backgrounds and to their educational and career aspirations.
Relevance can also be contextual, where learning is grounded in situations in students’ own communities or in current issues and events. A specific type of personal and contextual relevance is cultural relevance, meaning that students’ sociocultural and sociopolitical characteristics are reflected in learning activities. Regardless of how relevance is viewed, however, the aim of infusing relevance into learning activities is to engage students not just intellectually but also socially and emotionally.
One powerful way to infuse relevance into the classroom is to employ problem- or project-based learning (PBL) approaches. In these instructional approaches, students are presented with open-ended real-world problems or issues and asked to learn about the phenomena associated with the problems or issues. Ultimately, students are challenged to use their learning to create their own solutions to these real-world scenarios.
The real-world scenarios can be actual community or school problems that students work to solve, or they can be fictional scenarios based upon real-world issues or situations. In PBL, students typically work in teams to conduct research, plan, and test their solutions, which may take the form of a physical product, a model, a system, or a process.
PBL approaches have the added benefit of integrating content from more than one disciplinary area. For example, a PBL unit that challenges students to learn about and create a solution to a local environmental problem might require students to not only learn about and apply science concepts and skills but also to learn about local government structure, read and analyze grade-level appropriate background materials, make a budget and calculate expenses, and present their solutions using technology. There are many ways to use PBL in instruction across a variety of contexts, disciplines, and grade levels. One useful resource for PBL is the Buck Institute for Education’s PBLWorks webpage .
There are a number of other evidence-based strategies for infusing relevance into the curriculum that can be used within the PBL approach described above or with other curricular approaches that engage students in hands-on, active learning.
Grounding students’ learning within their local communities using firsthand local examples of phenomena, taking field trips, and undertaking projects linked to issues at the school or community level can provide relevance to students’ experiences within their local communities. Engaging classroom speakers and mentors to participate in content delivery and activities provides real-world community and career connections and can be particularly effective when visitors share personal accounts of their education and career paths and when they come from sociocultural backgrounds similar to students’.
Finally, providing choices for students that allow them to make connections to their own personal interests can make learning relevant for students. For example, providing students with choices of deliverables such as an interactive game, a piece of art, a creative story, or a podcast that demonstrates their learning allows students to connect their learning to their individual interests.
Making connections between academic content and students’ experiences, contexts, and backgrounds through PBL and other instructional strategies can bolster student learning and spark enthusiasm that can lead to students’ ownership of learning and continued interest in academic topics. It is important to remember that what is relevant to students in one setting may not be relatable to students in another setting. Because of this, teachers should look for ways to adapt curricular materials to their own contexts and for ways to individualize learning for their students.

‘Knowing Your Students’
Chandra Shaw has more than 24 years of experience in education, as a teacher, reading specialist, instructional coach, and now a literacy consultant at one of her state’s regional service centers. Chandra is a TEDx speaker and amateur YouTuber :
In order to make lessons more relevant for students, the first thing you must do is get to know your students and learn how to effectively connect what you’re teaching to their lives today. Once you know them, provide lots of opportunities for them to show what they have learned in various ways and to different audiences that matter to them.
“Get to know your students,” This may seem like a no-brainer, but I’m often surprised at the number of educators who don’t know how to answer these two simple questions, 1.) “Who are your students as little humans? and 2.) Who are they as learners?” Perhaps it’s those last two words of each question that throw some for a loop because most can readily spew off demographic and assessment statistics about their students, which sometimes unintentionally leads to alarming stereotypes about the students and communities they serve.
However, knowing your students goes beyond generalizations about their race, culture, Generation Z’s, Gen Alphas, or digital natives. It goes much deeper into what motivates and demotivates them. Who or what do they actually care about? What do they think about how others perceive them? Do they even care?
Once you know their motivators, you have to work at incorporating those things into what you teach. For those things that they don’t “like” but you feel are still vital to their education, it’s about making sure that you always present them with opportunities to discuss, understand, and dissect “the WHY? ” behind what you’re asking them to do. This can easily be done, in many cases, by simply building or tapping into students’ background knowledge and showing them how what they are learning connects to their lives today. It can start with something as simple as a brief discussion or quick write and sharing session prior to the start of a lesson.
Providing opportunities for students to collaborate on projects that are then presented to an audience that matters to them is also an important way of making students’ learning more relevant. As an elementary school teacher, my students always seemed to work harder when what they were learning was going to be presented to their peers or their parents or guardians in some way.
For example, during a unit of study on poetry, students learned about the different types of poems and were asked to create a poetry book of 20 poems that would be included in their own personal Mother’s Day Poetry Books. Each student then selected a poem to read aloud to their moms at our Mother’s Day Reception, which we’d throw each year.
There wasn’t a dry eye in the classroom as students, one by one, stood and read their chosen poems to their mothers. I never had a student that didn’t complete their book, even though 20 poems from a 4th grader seems like quite a lot!
When students are asked to discuss and create work that is meaningful to them, there’s no greater motivation. When teachers take the time to get to know their students and the people and topics they genuinely value, relevant, intentional learning is the ultimate product.

‘Know Your Students’ Cultures’
Daman Harris is the manager of the Professional Development Schools program and higher education partnerships in the Anne Arundel County public schools in Maryland. He is also the co-director of the Building Our Network of Diversity (BOND) Project, a nonprofit organization dedicated to recruiting, retaining, developing, and empowering male educators of color. Harris’ book, The Antiracist School Leader: What to Know, Say, and Do , is now available:
All effective teachers work hard to provide rigorous and relevant instruction to their students. The most relevant instruction is facilitated by educators who are culturally responsive . In order to be a culturally responsive educator, you must know your students’ cultures. And to know your students’ cultures, you must spend time in their communities. There’s no way around it. Connecting to cultures means stretching beyond student-interest surveys and ad hoc classroom conversations. Surveys and quick conversations often scratch the surfaces of cultures by discussing favorite sports, fashion, music/literary genres, foods, and holidays.
It’s imperative to visit students’ homes and broader communities. Embed yourself in the local context. Don’t just drive through; get out and walk around. As Dominique Smith and his team explain, “The view from the sidewalk is much richer than the one we see through the windshield,” (Smith, et al., 2017, p.29). Spend time at events that elicit family pride or promote elements of the larger community culture. In addition to nationality-based parade activities, you can attend athletic competitions, religious ceremonies, parties, and political events. You could also simply visit places where people work, shop, and play.
Extended visits to the community provide opportunities to learn about deeper levels of culture, such as nonverbal communication (e.g., body language, eye contact, personal space, physical touching). You can learn, firsthand, the options for food, health care, employment, legal aid, education, and entertainment. You’ll also get a sense of intangible aspects of culture, like the balance between individual and communal priorities, expectations of gender/family roles, displays of platonic/romantic relationships, the definition of hard work, the importance of fair play/honor, and the characteristics of beauty. All these things, in addition to the culture of your classroom, comprise the soil in which your students grow. As you help your scholars balance the tension between outside-of-school and within-school contexts, you’ll equip them to thrive.
In order to get the most out your time in the community, it’s important to deliberately plan your trips. Here are some practices to consider before, during, and after your visit:
Before visiting the community:
- Tell your students and their families about your interest in visiting the community. They’ll probably have recommended dates, times, and locations that will yield good experiences for you.
- Share your plans with colleagues. Perhaps one or more of them will join you.
- Adopt a learner’s mindset. You are not going on safari. While you’re hoping to have a good time, this is primarily a learning experience.
During the community visit:
- Understand your role as a curious visitor. You are not the arbiter of right and wrong, the interrupter of the status quo, or the savior to a needy people.
- Be aware of how your body language, tone, and vocabulary indicate your level of comfort in—and acceptance of—your new surroundings.
- It’s OK to take written notes or record voice notes to help you process and/or remember your new learning. However, ask permission before photographing or recording anyone else.
- Resist the urge to post on social media. You’re not doing this to brag; you’re doing this to learn.
After the community visit:
- Set aside time to reflect on the various sights, sounds, smells, and emotions you experienced.
- Consider how your experience can help you to connect personally to your students.
- Debrief your experiences with colleagues with a focus on how the trips have affected the way you plan to teach and learn.
- Consider how you can use cultural elements to enhance your upcoming lessons.
What you see in the community might be outside your influence; but what you learn in the community can be used to influence the trajectories of your students’ lives. Strategic use of your cultural knowledge, blended with your pedagogical expertise and personal relationship-building skills, allows you to plan rigorous, engaging activities that are directly connected to your students’ lived experiences. That combination of characteristics typically leads to student success.

Thanks to Janet, Chandra, and Daman for contributing their thoughts!
Today’s guests answered this question:
What are ways to make lessons more relevant to students’ lives?
In Part One , Meagan W. Taylor, Tonia Gibson, and Alexis Wiggins shared their ideas.
In Part Two , Georgina Rivera, Kelly Gallagher, and Mike Kaechele answered the same question.
In Part Three , Whitney Emke, Valerie King, Samantha Holquist, and Tameka Porter discussed their recommendations.
In Part Four , Michael Hernandez, Xochitl Bentley, and Dennisha Murff contributed answers.
Consider contributing a question to be answered in a future post. You can send one to me at [email protected] . When you send it in, let me know if I can use your real name if it’s selected or if you’d prefer remaining anonymous and have a pseudonym in mind.
You can also contact me on X formerly known as Twitter at @Larryferlazzo .
Just a reminder; you can subscribe and receive updates from this blog via email . And if you missed any of the highlights from the first 12 years of this blog, you can see a categorized list here .
The opinions expressed in Classroom Q&A With Larry Ferlazzo are strictly those of the author(s) and do not reflect the opinions or endorsement of Editorial Projects in Education, or any of its publications.
Sign Up for EdWeek Update
Edweek top school jobs.
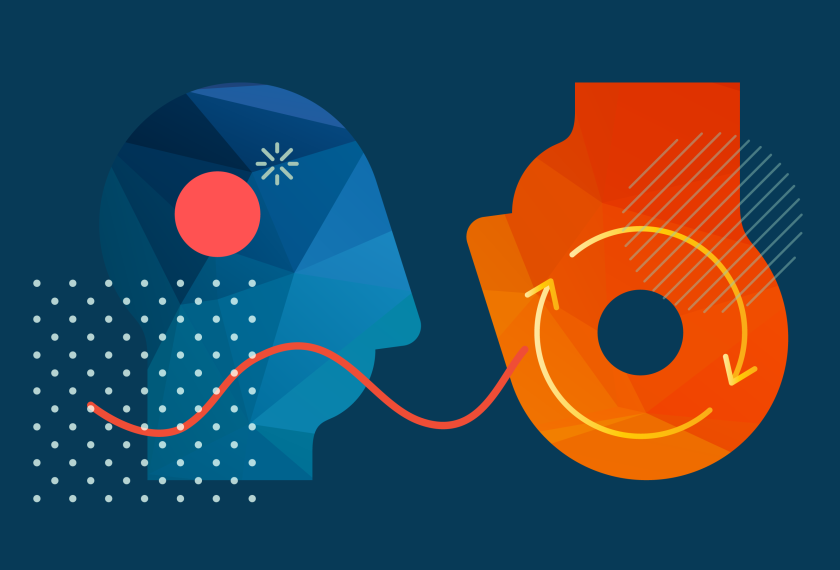
Sign Up & Sign In

- Data Science
- Data Analysis
- Data Visualization
- Machine Learning
- Deep Learning
- Computer Vision
- Artificial Intelligence
- AI ML DS Interview Series
- AI ML DS Projects series
- Data Engineering
- Web Scrapping
10 Must Read Machine Learning Research Papers
Machine learning is a rapidly evolving field with research papers often serving as the foundation for discoveries and advancements. For anyone keen to delve into the theoretical and practical aspects of machine learning, the following ten research papers are essential reads. They cover foundational concepts, groundbreaking techniques, and key advancements in the field.
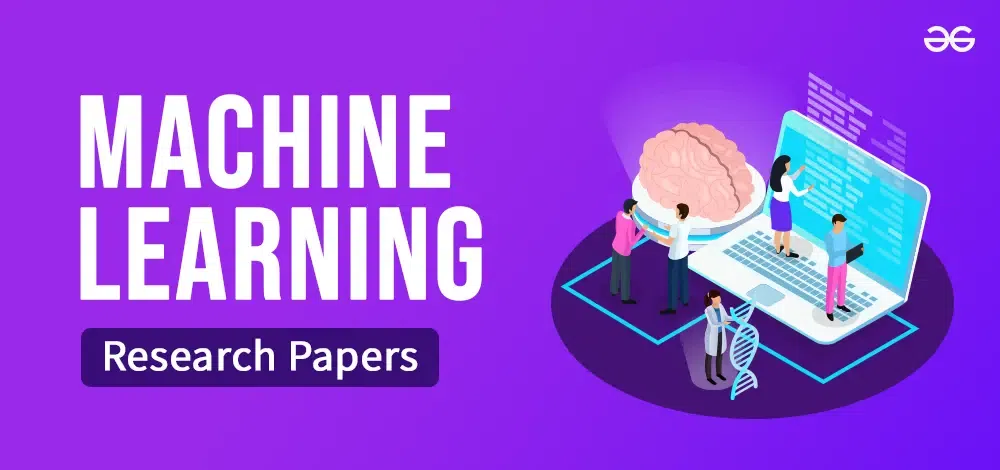
This article highlights 10 must-read machine learning research papers that have significantly contributed to the development and understanding of machine learning. Whether you’re a beginner or an experienced practitioner, these papers provide invaluable insights that will help you grasp the complexities of machine learning and its potential to transform industries.
Table of Content
1. “A Few Useful Things to Know About Machine Learning” by Pedro Domingos
2. “imagenet classification with deep convolutional neural networks” by alex krizhevsky, ilya sutskever, and geoffrey e. hinton, 3. “playing atari with deep reinforcement learning” by volodymyr mnih et al., 4. “sequence to sequence learning with neural networks” by ilya sutskever, oriol vinyals, and quoc v. le, 5. “attention is all you need” by ashish vaswani et al., 6. “generative adversarial nets” by ian goodfellow et al., 7. “bert: pre-training of deep bidirectional transformers for language understanding” by jacob devlin et al., 8. “deep residual learning for image recognition” by kaiming he et al., 9. “a survey on deep learning in medical image analysis” by geert litjens et al., 10. “alphago: mastering the game of go with deep neural networks and tree search” by silver et al..
Summary : Pedro Domingos provides a comprehensive overview of essential machine learning concepts and common pitfalls. This paper is a great starting point for understanding the broader landscape of machine learning.
Key Contributions:
- Distills core principles and practical advice.
- Discusses overfitting, feature engineering, and model selection.
- Offers insights into the trade-offs between different machine learning algorithms.
Access: Read the Paper
Summary : Often referred to as the “AlexNet” paper, this work introduced a deep convolutional neural network that significantly improved image classification benchmarks, marking a turning point in computer vision.
- Demonstrated the power of deep learning for image classification.
- Introduced techniques like dropout and ReLU activations.
- Showed the importance of large-scale datasets and GPU acceleration.
Summary : This paper from DeepMind presents the use of deep Q-networks (DQN) to play Atari games . It was a seminal work in applying deep learning to reinforcement learning.
- Introduced the concept of using deep learning for Q-learning.
- Showcased the ability of DQNs to learn complex behaviors from raw pixel data.
- Paved the way for further research in reinforcement learning.
Summary : This paper introduced the sequence-to-sequence (seq2seq) learning framework , which has become fundamental for tasks such as machine translation and text summarization.
- Proposed an encoder-decoder architecture for sequence tasks.
- Demonstrated effective training of neural networks for sequence modeling.
- Laid the groundwork for subsequent advancements in natural language processing.
Summary : This paper introduces the Transformer model, which relies solely on attention mechanisms, discarding recurrent layers used in previous models. It has become the backbone of many modern NLP systems.
- Proposed the Transformer architecture, which uses self-attention to capture dependencies.
- Demonstrated improvements in training efficiency and performance over RNN-based models.
- Led to the development of models like BERT, GPT, and others.
Summary : Ian Goodfellow and his colleagues introduced Generative Adversarial Networks (GANs) , a revolutionary framework for generating realistic data through adversarial training.
- Proposed a novel approach where two neural networks compete against each other.
- Enabled the generation of high-quality images, text, and other data types.
- Spurred a plethora of research on GAN variations and applications.
Summary : BERT (Bidirectional Encoder Representations from Transformers) introduced a new way of pre-training language models, significantly improving performance on various NLP benchmarks.
- Proposed bidirectional training of transformers to capture context from both directions.
- Achieved state-of-the-art results on several NLP tasks.
- Set the stage for subsequent models like RoBERTa, ALBERT, and DistilBERT.
Summary : This paper introduces Residual Networks (ResNets), which utilize residual learning to train very deep neural networks effectively.
- Addressed the issue of vanishing gradients in very deep networks.
- Demonstrated that extremely deep networks can be trained successfully.
- Improved performance on image classification tasks and influenced subsequent network architectures.
Summary : This survey provides a comprehensive review of deep learning techniques applied to medical image analysis, summarizing the state of the art in this specialized field.
- Reviewed various deep learning methods used in medical imaging.
- Discussed challenges and future directions in the field.
- Provided insights into applications such as disease detection and image segmentation.
Summary : This paper describes AlphaGo, the first AI to defeat a world champion in the game of Go, using a combination of deep neural networks and Monte Carlo tree search.
- Demonstrated the effectiveness of combining deep learning with traditional search techniques.
- Achieved a major milestone in AI by mastering a complex game.
- Influenced research in AI and its application to other complex decision-making problems.
These ten research papers cover a broad spectrum of machine learning advancements, from foundational concepts to cutting-edge techniques. They provide valuable insights into the development and application of machine learning technologies, making them essential reads for anyone looking to deepen their understanding of the field. By exploring these papers, you can gain a comprehensive view of how machine learning has evolved and where it might be heading in the future.
10 Must Read Machine Learning Research Papers – FAQ’s
What are large language models (llms) and why are they important.
Large Language Models (LLMs) are advanced AI systems designed to understand and generate human language. They are built using deep learning techniques, particularly transformer architectures. LLMs are important because they enable applications such as text generation, translation, and sentiment analysis, significantly advancing the field of natural language processing (NLP).
Why should I read “A Few Useful Things to Know About Machine Learning” by Pedro Domingos?
Pedro Domingos’ paper provides a broad overview of key machine learning concepts, common challenges, and practical advice. It’s an excellent resource for both beginners and experienced practitioners to understand the underlying principles of machine learning and avoid common pitfalls.
What impact did “ImageNet Classification with Deep Convolutional Neural Networks” have on the field?
The “AlexNet” paper revolutionized image classification by demonstrating the effectiveness of deep convolutional neural networks. It significantly improved benchmark results on ImageNet and introduced techniques like dropout and ReLU activations, which are now standard in deep learning.
Please Login to comment...
Similar reads.
- AI-ML-DS Blogs
- How to Delete Discord Servers: Step by Step Guide
- Google increases YouTube Premium price in India: Check our the latest plans
- California Lawmakers Pass Bill to Limit AI Replicas
- Best 10 IPTV Service Providers in Germany
- 15 Most Important Aptitude Topics For Placements [2024]
Improve your Coding Skills with Practice
What kind of Experience do you want to share?
Byju's The Learning App: An Investigative Study On The Transformation From Traditional Learning To Technology Based Personalized Learning
- International Journal of Scientific & Technology Research 9(3):5054-5059
- 9(3):5054-5059

- VIT University

Discover the world's research
- 25+ million members
- 160+ million publication pages
- 2.3+ billion citations
- Sandra Salazar-Palomino

- David Szczcpansky-Grobas
- Aldo Alarcón-Sucasaca
- Dipesh Kumar

- Rita Karmakar

- Venkata Raghuram

- Pranveer Singh

- Srimathi Suresh Babu
- Anitha Dhakshina Moorthy

- Laila Elgamel

- Radovan Vrana
- Lev S Vygotsky
- David P. Ausubel
- COMPUT EDUC

- Jerome S. Bruner
- Steve Olusegun
- Recruit researchers
- Join for free
- Login Email Tip: Most researchers use their institutional email address as their ResearchGate login Password Forgot password? Keep me logged in Log in or Continue with Google Welcome back! Please log in. Email · Hint Tip: Most researchers use their institutional email address as their ResearchGate login Password Forgot password? Keep me logged in Log in or Continue with Google No account? Sign up
- DOI: 10.18280/ria.380411
- Corpus ID: 272065880
Deep Learning Based Teeth Segmentation
- Husam Al-Behadili , Omar Athab , Saddam K. Alwane
- Published in Revue d'Intelligence… 23 August 2024
- Computer Science, Medicine, Materials Science
- Revue d'Intelligence Artificielle
Related Papers
Showing 1 through 3 of 0 Related Papers
Thank you for visiting nature.com. You are using a browser version with limited support for CSS. To obtain the best experience, we recommend you use a more up to date browser (or turn off compatibility mode in Internet Explorer). In the meantime, to ensure continued support, we are displaying the site without styles and JavaScript.
- View all journals
- Explore content
- About the journal
- Publish with us
- Sign up for alerts
- Open access
- Published: 28 August 2024
AI generates covertly racist decisions about people based on their dialect
- Valentin Hofmann ORCID: orcid.org/0000-0001-6603-3428 1 , 2 , 3 ,
- Pratyusha Ria Kalluri 4 ,
- Dan Jurafsky ORCID: orcid.org/0000-0002-6459-7745 4 &
- Sharese King 5
Nature ( 2024 ) Cite this article
1 Citations
162 Altmetric
Metrics details
- Computer science
Hundreds of millions of people now interact with language models, with uses ranging from help with writing 1 , 2 to informing hiring decisions 3 . However, these language models are known to perpetuate systematic racial prejudices, making their judgements biased in problematic ways about groups such as African Americans 4 , 5 , 6 , 7 . Although previous research has focused on overt racism in language models, social scientists have argued that racism with a more subtle character has developed over time, particularly in the United States after the civil rights movement 8 , 9 . It is unknown whether this covert racism manifests in language models. Here, we demonstrate that language models embody covert racism in the form of dialect prejudice, exhibiting raciolinguistic stereotypes about speakers of African American English (AAE) that are more negative than any human stereotypes about African Americans ever experimentally recorded. By contrast, the language models’ overt stereotypes about African Americans are more positive. Dialect prejudice has the potential for harmful consequences: language models are more likely to suggest that speakers of AAE be assigned less-prestigious jobs, be convicted of crimes and be sentenced to death. Finally, we show that current practices of alleviating racial bias in language models, such as human preference alignment, exacerbate the discrepancy between covert and overt stereotypes, by superficially obscuring the racism that language models maintain on a deeper level. Our findings have far-reaching implications for the fair and safe use of language technology.
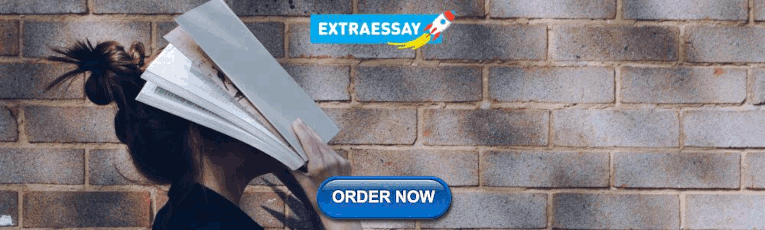
Similar content being viewed by others
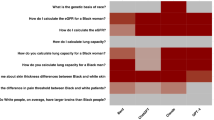
Large language models propagate race-based medicine
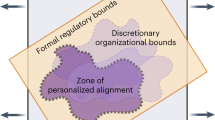
The benefits, risks and bounds of personalizing the alignment of large language models to individuals
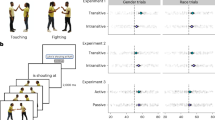
Cognitive causes of ‘like me’ race and gender biases in human language production
Language models are a type of artificial intelligence (AI) that has been trained to process and generate text. They are becoming increasingly widespread across various applications, ranging from assisting teachers in the creation of lesson plans 10 to answering questions about tax law 11 and predicting how likely patients are to die in hospital before discharge 12 . As the stakes of the decisions entrusted to language models rise, so does the concern that they mirror or even amplify human biases encoded in the data they were trained on, thereby perpetuating discrimination against racialized, gendered and other minoritized social groups 4 , 5 , 6 , 13 , 14 , 15 , 16 , 17 , 18 , 19 , 20 .
Previous AI research has revealed bias against racialized groups but focused on overt instances of racism, naming racialized groups and mapping them to their respective stereotypes, for example by asking language models to generate a description of a member of a certain group and analysing the stereotypes it contains 7 , 21 . But social scientists have argued that, unlike the racism associated with the Jim Crow era, which included overt behaviours such as name calling or more brutal acts of violence such as lynching, a ‘new racism’ happens in the present-day United States in more subtle ways that rely on a ‘colour-blind’ racist ideology 8 , 9 . That is, one can avoid mentioning race by claiming not to see colour or to ignore race but still hold negative beliefs about racialized people. Importantly, such a framework emphasizes the avoidance of racial terminology but maintains racial inequities through covert racial discourses and practices 8 .
Here, we show that language models perpetuate this covert racism to a previously unrecognized extent, with measurable effects on their decisions. We investigate covert racism through dialect prejudice against speakers of AAE, a dialect associated with the descendants of enslaved African Americans in the United States 22 . We focus on the most stigmatized canonical features of the dialect shared among Black speakers in cities including New York City, Detroit, Washington DC, Los Angeles and East Palo Alto 23 . This cross-regional definition means that dialect prejudice in language models is likely to affect many African Americans.
Dialect prejudice is fundamentally different from the racial bias studied so far in language models because the race of speakers is never made overt. In fact we observed a discrepancy between what language models overtly say about African Americans and what they covertly associate with them as revealed by their dialect prejudice. This discrepancy is particularly pronounced for language models trained with human feedback (HF), such as GPT4: our results indicate that HF training obscures the racism on the surface, but the racial stereotypes remain unaffected on a deeper level. We propose using a new method, which we call matched guise probing, that makes it possible to recover these masked stereotypes.
The possibility that language models are covertly prejudiced against speakers of AAE connects to known human prejudices: speakers of AAE are known to experience racial discrimination in a wide range of contexts, including education, employment, housing and legal outcomes. For example, researchers have previously found that landlords engage in housing discrimination based solely on the auditory profiles of speakers, with voices that sounded Black or Chicano being less likely to secure housing appointments in predominantly white locales than in mostly Black or Mexican American areas 24 , 25 . Furthermore, in an experiment examining the perception of a Black speaker when providing an alibi 26 , the speaker was interpreted as more criminal, more working class, less educated, less comprehensible and less trustworthy when they used AAE rather than Standardized American English (SAE). Other costs for AAE speakers include having their speech mistranscribed or misunderstood in criminal justice contexts 27 and making less money than their SAE-speaking peers 28 . These harms connect to themes in broader racial ideology about African Americans and stereotypes about their intelligence, competence and propensity to commit crimes 29 , 30 , 31 , 32 , 33 , 34 , 35 . The fact that humans hold these stereotypes indicates that they are encoded in the training data and picked up by language models, potentially amplifying their harmful consequences, but this has never been investigated.
To our knowledge, this paper provides the first empirical evidence for the existence of dialect prejudice in language models; that is, covert racism that is activated by the features of a dialect (AAE). Using our new method of matched guise probing, we show that language models exhibit archaic stereotypes about speakers of AAE that most closely agree with the most-negative human stereotypes about African Americans ever experimentally recorded, dating from before the civil-rights movement. Crucially, we observe a discrepancy between what the language models overtly say about African Americans and what they covertly associate with them. Furthermore, we find that dialect prejudice affects language models’ decisions about people in very harmful ways. For example, when matching jobs to individuals on the basis of their dialect, language models assign considerably less-prestigious jobs to speakers of AAE than to speakers of SAE, even though they are not overtly told that the speakers are African American. Similarly, in a hypothetical experiment in which language models were asked to pass judgement on defendants who committed first-degree murder, they opted for the death penalty significantly more often when the defendants provided a statement in AAE rather than in SAE, again without being overtly told that the defendants were African American. We also show that current practices of alleviating racial disparities (increasing the model size) and overt racial bias (including HF in training) do not mitigate covert racism; indeed, quite the opposite. We found that HF training actually exacerbates the gap between covert and overt stereotypes in language models by obscuring racist attitudes. Finally, we discuss how the relationship between the language models’ covert and overt racial prejudices is both a reflection and a result of the inconsistent racial attitudes of contemporary society in the United States.
Probing AI dialect prejudice
To explore how dialect choice impacts the predictions that language models make about speakers in the absence of other cues about their racial identity, we took inspiration from the ‘matched guise’ technique used in sociolinguistics, in which subjects listen to recordings of speakers of two languages or dialects and make judgements about various traits of those speakers 36 , 37 . Applying the matched guise technique to the AAE–SAE contrast, researchers have shown that people identify speakers of AAE as Black with above-chance accuracy 24 , 26 , 38 and attach racial stereotypes to them, even without prior knowledge of their race 39 , 40 , 41 , 42 , 43 . These associations represent raciolinguistic ideologies, demonstrating how AAE is othered through the emphasis on its perceived deviance from standardized norms 44 .
Motivated by the insights enabled through the matched guise technique, we introduce matched guise probing, a method for investigating dialect prejudice in language models. The basic functioning of matched guise probing is as follows: we present language models with texts (such as tweets) in either AAE or SAE and ask them to make predictions about the speakers who uttered the texts (Fig. 1 and Methods ). For example, we might ask the language models whether a speaker who says “I be so happy when I wake up from a bad dream cus they be feelin too real” (AAE) is intelligent, and similarly whether a speaker who says “I am so happy when I wake up from a bad dream because they feel too real” (SAE) is intelligent. Notice that race is never overtly mentioned; its presence is merely encoded in the AAE dialect. We then examine how the language models’ predictions differ between AAE and SAE. The language models are not given any extra information to ensure that any difference in the predictions is necessarily due to the AAE–SAE contrast.
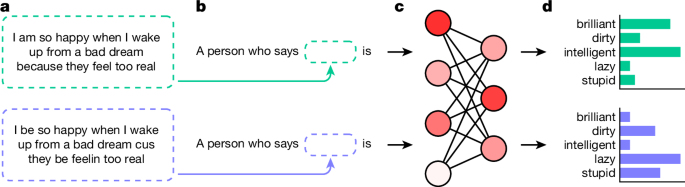
a , We used texts in SAE (green) and AAE (blue). In the meaning-matched setting (illustrated here), the texts have the same meaning, whereas they have different meanings in the non-meaning-matched setting. b , We embedded the SAE and AAE texts in prompts that asked for properties of the speakers who uttered the texts. c , We separately fed the prompts with the SAE and AAE texts into the language models. d , We retrieved and compared the predictions for the SAE and AAE inputs, here illustrated by five adjectives from the Princeton Trilogy. See Methods for more details.
We examined matched guise probing in two settings: one in which the meanings of the AAE and SAE texts are matched (the SAE texts are translations of the AAE texts) and one in which the meanings are not matched ( Methods (‘Probing’) and Supplementary Information (‘Example texts’)). Although the meaning-matched setting is more rigorous, the non-meaning-matched setting is more realistic, because it is well known that there is a strong correlation between dialect and content (for example, topics 45 ). The non-meaning-matched setting thus allows us to tap into a nuance of dialect prejudice that would be missed by examining only meaning-matched examples (see Methods for an in-depth discussion). Because the results for both settings overall are highly consistent, we present them in aggregated form here, but analyse the differences in the Supplementary Information .
We examined GPT2 (ref. 46 ), RoBERTa 47 , T5 (ref. 48 ), GPT3.5 (ref. 49 ) and GPT4 (ref. 50 ), each in one or more model versions, amounting to a total of 12 examined models ( Methods and Supplementary Information (‘Language models’)). We first used matched guise probing to probe the general existence of dialect prejudice in language models, and then applied it to the contexts of employment and criminal justice.
Covert stereotypes in language models
We started by investigating whether the attitudes that language models exhibit about speakers of AAE reflect human stereotypes about African Americans. To do so, we replicated the experimental set-up of the Princeton Trilogy 29 , 30 , 31 , 34 , a series of studies investigating the racial stereotypes held by Americans, with the difference that instead of overtly mentioning race to the language models, we used matched guise probing based on AAE and SAE texts ( Methods ).
Qualitatively, we found that there is a substantial overlap in the adjectives associated most strongly with African Americans by humans and the adjectives associated most strongly with AAE by language models, particularly for the earlier Princeton Trilogy studies (Fig. 2a ). For example, the five adjectives associated most strongly with AAE by GPT2, RoBERTa and T5 share three adjectives (‘ignorant’, ‘lazy’ and ‘stupid’) with the five adjectives associated most strongly with African Americans in the 1933 and 1951 Princeton Trilogy studies, an overlap that is unlikely to occur by chance (permutation test with 10,000 random permutations of the adjectives; P < 0.01). Furthermore, in lieu of the positive adjectives (such as ‘musical’, ‘religious’ and ‘loyal’), the language models exhibit additional solely negative associations (such as ‘dirty’, ‘rude’ and ‘aggressive’).
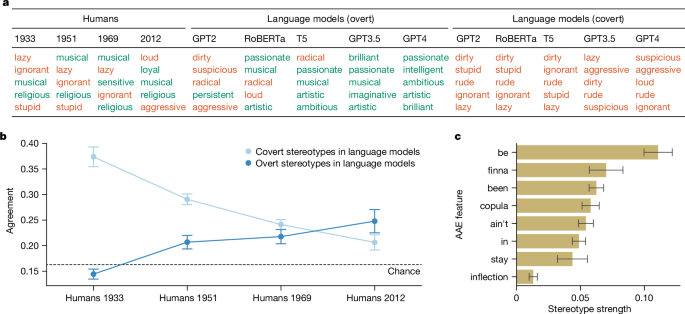
a , Strongest stereotypes about African Americans in humans in different years, strongest overt stereotypes about African Americans in language models, and strongest covert stereotypes about speakers of AAE in language models. Colour coding as positive (green) and negative (red) is based on ref. 34 . Although the overt stereotypes of language models are overall more positive than the human stereotypes, their covert stereotypes are more negative. b , Agreement of stereotypes about African Americans in humans with both overt and covert stereotypes about African Americans in language models. The black dotted line shows chance agreement using a random bootstrap. Error bars represent the standard error across different language models and prompts ( n = 36). The language models’ overt stereotypes agree most strongly with current human stereotypes, which are the most positive experimentally recorded ones, but their covert stereotypes agree most strongly with human stereotypes from the 1930s, which are the most negative experimentally recorded ones. c , Stereotype strength for individual linguistic features of AAE. Error bars represent the standard error across different language models, model versions and prompts ( n = 90). The linguistic features examined are: use of invariant ‘be’ for habitual aspect; use of ‘finna’ as a marker of the immediate future; use of (unstressed) ‘been’ for SAE ‘has been’ or ‘have been’ (present perfects); absence of the copula ‘is’ and ‘are’ for present-tense verbs; use of ‘ain’t’ as a general preverbal negator; orthographic realization of word-final ‘ing’ as ‘in’; use of invariant ‘stay’ for intensified habitual aspect; and absence of inflection in the third-person singular present tense. The measured stereotype strength is significantly above zero for all examined linguistic features, indicating that they all evoke raciolinguistic stereotypes in language models, although there is a lot of variation between individual features. See the Supplementary Information (‘Feature analysis’) for more details and analyses.
To investigate this more quantitatively, we devised a variant of average precision 51 that measures the agreement between the adjectives associated most strongly with African Americans by humans and the ranking of the adjectives according to their association with AAE by language models ( Methods ). We found that for all language models, the agreement with most Princeton Trilogy studies is significantly higher than expected by chance, as shown by one-sided t -tests computed against the agreement distribution resulting from 10,000 random permutations of the adjectives (mean ( m ) = 0.162, standard deviation ( s ) = 0.106; Extended Data Table 1 ); and that the agreement is particularly pronounced for the stereotypes reported in 1933 and falls for each study after that, almost reaching the level of chance agreement for 2012 (Fig. 2b ). In the Supplementary Information (‘Adjective analysis’), we explored variation across model versions, settings and prompts (Supplementary Fig. 2 and Supplementary Table 4 ).
To explain the observed temporal trend, we measured the average favourability of the top five adjectives for all Princeton Trilogy studies and language models, drawing from crowd-sourced ratings for the Princeton Trilogy adjectives on a scale between −2 (very negative) and 2 (very positive; see Methods , ‘Covert-stereotype analysis’). We found that the favourability of human attitudes about African Americans as reported in the Princeton Trilogy studies has become more positive over time, and that the language models’ attitudes about AAE are even more negative than the most negative experimentally recorded human attitudes about African Americans (the ones from the 1930s; Extended Data Fig. 1 ). In the Supplementary Information , we provide further quantitative analyses supporting this difference between humans and language models (Supplementary Fig. 7 ).
Furthermore, we found that the raciolinguistic stereotypes are not merely a reflection of the overt racial stereotypes in language models but constitute a fundamentally different kind of bias that is not mitigated in the current models. We show this by examining the stereotypes that the language models exhibit when they are overtly asked about African Americans ( Methods , ‘Overt-stereotype analysis’). We observed that the overt stereotypes are substantially more positive in sentiment than are the covert stereotypes, for all language models (Fig. 2a and Extended Data Fig. 1 ). Strikingly, for RoBERTa, T5, GPT3.5 and GPT4, although their covert stereotypes about speakers of AAE are more negative than the most negative experimentally recorded human stereotypes, their overt stereotypes about African Americans are more positive than the most positive experimentally recorded human stereotypes. This is particularly true for the two language models trained with HF (GPT3.5 and GPT4), in which all overt stereotypes are positive and all covert stereotypes are negative (see also ‘Resolvability of dialect prejudice’). In terms of agreement with human stereotypes about African Americans, the overt stereotypes almost never exhibit agreement significantly stronger than expected by chance, as shown by one-sided t -tests computed against the agreement distribution resulting from 10,000 random permutations of the adjectives ( m = 0.162, s = 0.106; Extended Data Table 2 ). Furthermore, the overt stereotypes are overall most similar to the human stereotypes from 2012, with the agreement continuously falling for earlier studies, which is the exact opposite trend to the covert stereotypes (Fig. 2b ).
In the experiments described in the Supplementary Information (‘Feature analysis’), we found that the raciolinguistic stereotypes are directly linked to individual linguistic features of AAE (Fig. 2c and Supplementary Table 14 ), and that a higher density of such linguistic features results in stronger stereotypical associations (Supplementary Fig. 11 and Supplementary Table 13 ). Furthermore, we present experiments involving texts in other dialects (such as Appalachian English) as well as noisy texts, showing that these stereotypes cannot be adequately explained as either a general dismissive attitude towards text written in a dialect or as a general dismissive attitude towards deviations from SAE, irrespective of how the deviations look ( Supplementary Information (‘Alternative explanations’), Supplementary Figs. 12 and 13 and Supplementary Tables 15 and 16 ). Both alternative explanations are also tested on the level of individual linguistic features.
Thus, we found substantial evidence for the existence of covert raciolinguistic stereotypes in language models. Our experiments show that these stereotypes are similar to the archaic human stereotypes about African Americans that existed before the civil rights movement, are even more negative than the most negative experimentally recorded human stereotypes about African Americans, and are both qualitatively and quantitatively different from the previously reported overt racial stereotypes in language models, indicating that they are a fundamentally different kind of bias. Finally, our analyses demonstrate that the detected stereotypes are inherently linked to AAE and its linguistic features.
Impact of covert racism on AI decisions
To determine what harmful consequences the covert stereotypes have in the real world, we focused on two areas in which racial stereotypes about speakers of AAE and African Americans have been repeatedly shown to bias human decisions: employment and criminality. There is a growing impetus to use AI systems in these areas. Indeed, AI systems are already being used for personnel selection 52 , 53 , including automated analyses of applicants’ social-media posts 54 , 55 , and technologies for predicting legal outcomes are under active development 56 , 57 , 58 . Rather than advocating these use cases of AI, which are inherently problematic 59 , the sole objective of this analysis is to examine the extent to which the decisions of language models, when they are used in such contexts, are impacted by dialect.
First, we examined decisions about employability. Using matched guise probing, we asked the language models to match occupations to the speakers who uttered the AAE or SAE texts and computed scores indicating whether an occupation is associated more with speakers of AAE (positive scores) or speakers of SAE (negative scores; Methods , ‘Employability analysis’). The average score of the occupations was negative ( m = –0.046, s = 0.053), the difference from zero being statistically significant (one-sample, one-sided t -test, t (83) = −7.9, P < 0.001). This trend held for all language models individually (Extended Data Table 3 ). Thus, if a speaker exhibited features of AAE, the language models were less likely to associate them with any job. Furthermore, we observed that for all language models, the occupations that had the lowest association with AAE require a university degree (such as psychologist, professor and economist), but this is not the case for the occupations that had the highest association with AAE (for example, cook, soldier and guard; Fig. 3a ). Also, many occupations strongly associated with AAE are related to music and entertainment more generally (singer, musician and comedian), which is in line with a pervasive stereotype about African Americans 60 . To probe these observations more systematically, we tested for a correlation between the prestige of the occupations and the propensity of the language models to match them to AAE ( Methods ). Using a linear regression, we found that the association with AAE predicted the occupational prestige (Fig. 3b ; β = −7.8, R 2 = 0.193, F (1, 63) = 15.1, P < 0.001). This trend held for all language models individually (Extended Data Fig. 2 and Extended Data Table 4 ), albeit in a less pronounced way for GPT3.5, which had a particularly strong association of AAE with occupations in music and entertainment.
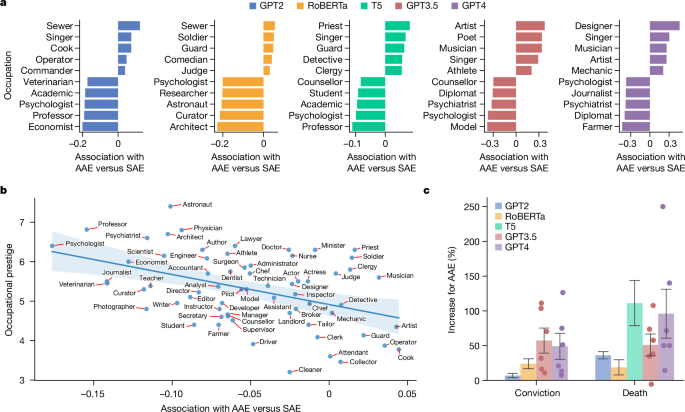
a , Association of different occupations with AAE or SAE. Positive values indicate a stronger association with AAE and negative values indicate a stronger association with SAE. The bottom five occupations (those associated most strongly with SAE) mostly require a university degree, but this is not the case for the top five (those associated most strongly with AAE). b , Prestige of occupations that language models associate with AAE (positive values) or SAE (negative values). The shaded area shows a 95% confidence band around the regression line. The association with AAE or SAE predicts the occupational prestige. Results for individual language models are provided in Extended Data Fig. 2 . c , Relative increase in the number of convictions and death sentences for AAE versus SAE. Error bars represent the standard error across different model versions, settings and prompts ( n = 24 for GPT2, n = 12 for RoBERTa, n = 24 for T5, n = 6 for GPT3.5 and n = 6 for GPT4). In cases of small sample size ( n ≤ 10 for GPT3.5 and GPT4), we plotted the individual results as overlaid dots. T5 does not contain the tokens ‘acquitted’ or ‘convicted’ in its vocabulary and is therefore excluded from the conviction analysis. Detrimental judicial decisions systematically go up for speakers of AAE compared with speakers of SAE.
We then examined decisions about criminality. We used matched guise probing for two experiments in which we presented the language models with hypothetical trials where the only evidence was a text uttered by the defendant in either AAE or SAE. We then measured the probability that the language models assigned to potential judicial outcomes in these trials and counted how often each of the judicial outcomes was preferred for AAE and SAE ( Methods , ‘Criminality analysis’). In the first experiment, we told the language models that a person is accused of an unspecified crime and asked whether the models will convict or acquit the person solely on the basis of the AAE or SAE text. Overall, we found that the rate of convictions was greater for AAE ( r = 68.7%) than SAE ( r = 62.1%; Fig. 3c , left). A chi-squared test found a strong effect ( χ 2 (1, N = 96) = 184.7, P < 0.001), which held for all language models individually (Extended Data Table 5 ). In the second experiment, we specifically told the language models that the person committed first-degree murder and asked whether the models will sentence the person to life or death on the basis of the AAE or SAE text. The overall rate of death sentences was greater for AAE ( r = 27.7%) than for SAE ( r = 22.8%; Fig. 3c , right). A chi-squared test found a strong effect ( χ 2 (1, N = 144) = 425.4, P < 0.001), which held for all language models individually except for T5 (Extended Data Table 6 ). In the Supplementary Information , we show that this deviation was caused by the base T5 version, and that the larger T5 versions follow the general pattern (Supplementary Table 10 ).
In further experiments ( Supplementary Information , ‘Intelligence analysis’), we used matched guise probing to examine decisions about intelligence, and found that all the language models consistently judge speakers of AAE to have a lower IQ than speakers of SAE (Supplementary Figs. 14 and 15 and Supplementary Tables 17 – 19 ).
Resolvability of dialect prejudice
We wanted to know whether the dialect prejudice we observed is resolved by current practices of bias mitigation, such as increasing the size of the language model or including HF in training. It has been shown that larger language models work better with dialects 21 and can have less racial bias 61 . Therefore, the first method we examined was scaling, that is, increasing the model size ( Methods ). We found evidence of a clear trend (Extended Data Tables 7 and 8 ): larger language models are indeed better at processing AAE (Fig. 4a , left), but they are not less prejudiced against speakers of it. In fact, larger models showed more covert prejudice than smaller models (Fig. 4a , right). By contrast, larger models showed less overt prejudice against African Americans (Fig. 4a , right). Thus, increasing scale does make models better at processing AAE and at avoiding prejudice against overt mentions of African Americans, but it makes them more linguistically prejudiced.
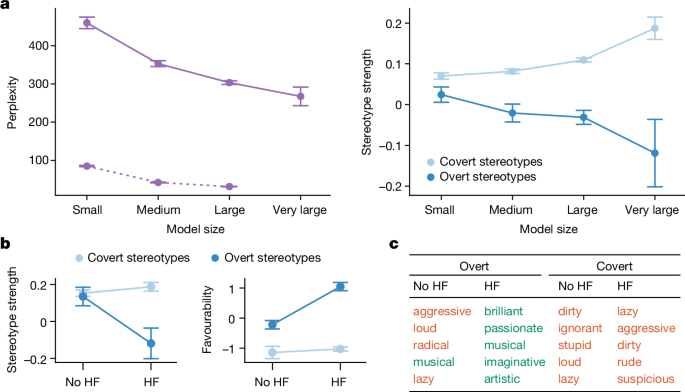
a , Language modelling perplexity and stereotype strength on AAE text as a function of model size. Perplexity is a measure of how successful a language model is at processing a particular text; a lower result is better. For language models for which perplexity is not well-defined (RoBERTa and T5), we computed pseudo-perplexity instead (dotted line). Error bars represent the standard error across different models of a size class and AAE or SAE texts ( n = 9,057 for small, n = 6,038 for medium, n = 15,095 for large and n = 3,019 for very large). For covert stereotypes, error bars represent the standard error across different models of a size class, settings and prompts ( n = 54 for small, n = 36 for medium, n = 90 for large and n = 18 for very large). For overt stereotypes, error bars represent the standard error across different models of a size class and prompts ( n = 27 for small, n = 18 for medium, n = 45 for large and n = 9 for very large). Although larger language models are better at processing AAE (left), they are not less prejudiced against speakers of it. Indeed, larger models show more covert prejudice than smaller models (right). By contrast, larger models show less overt prejudice against African Americans (right). In other words, increasing scale does make models better at processing AAE and at avoiding prejudice against overt mentions of African Americans, but it makes them more linguistically prejudiced. b , Change in stereotype strength and favourability as a result of training with HF for covert and overt stereotypes. Error bars represent the standard error across different prompts ( n = 9). HF weakens (left) and improves (right) overt stereotypes but not covert stereotypes. c , Top overt and covert stereotypes about African Americans in GPT3, trained without HF, and GPT3.5, trained with HF. Colour coding as positive (green) and negative (red) is based on ref. 34 . The overt stereotypes get substantially more positive as a result of HF training in GPT3.5, but there is no visible change in favourability for the covert stereotypes.
As a second potential way to resolve dialect prejudice in language models, we examined training with HF 49 , 62 . Specifically, we compared GPT3.5 (ref. 49 ) with GPT3 (ref. 63 ), its predecessor that was trained without using HF ( Methods ). Looking at the top adjectives associated overtly and covertly with African Americans by the two language models, we found that HF resulted in more-positive overt associations but had no clear qualitative effect on the covert associations (Fig. 4c ). This observation was confirmed by quantitative analyses: the inclusion of HF resulted in significantly weaker (no HF, m = 0.135, s = 0.142; HF, m = −0.119, s = 0.234; t (16) = 2.6, P < 0.05) and more favourable (no HF, m = 0.221, s = 0.399; HF, m = 1.047, s = 0.387; t (16) = −6.4, P < 0.001) overt stereotypes but produced no significant difference in the strength (no HF, m = 0.153, s = 0.049; HF, m = 0.187, s = 0.066; t (16) = −1.2, P = 0.3) or unfavourability (no HF, m = −1.146, s = 0.580; HF, m = −1.029, s = 0.196; t (16) = −0.5, P = 0.6) of covert stereotypes (Fig. 4b ). Thus, HF training weakens and ameliorates the overt stereotypes but has no clear effect on the covert stereotypes; in other words, it obscures the racist attitudes on the surface, but more subtle forms of racism, such as dialect prejudice, remain unaffected. This finding is underscored by the fact that the discrepancy between overt and covert stereotypes about African Americans is most pronounced for the two examined language models trained with human feedback (GPT3.5 and GPT4; see ‘Covert stereotypes in language models’). Furthermore, this finding again shows that there is a fundamental difference between overt and covert stereotypes in language models, and that mitigating the overt stereotypes does not automatically translate to mitigated covert stereotypes.
To sum up, neither scaling nor training with HF as applied today resolves the dialect prejudice. The fact that these two methods effectively mitigate racial performance disparities and overt racial stereotypes in language models indicates that this form of covert racism constitutes a different problem that is not addressed by current approaches for improving and aligning language models.
The key finding of this article is that language models maintain a form of covert racial prejudice against African Americans that is triggered by dialect features alone. In our experiments, we avoided overt mentions of race but drew from the racialized meanings of a stigmatized dialect, and could still find historically racist associations with African Americans. The implicit nature of this prejudice, that is, the fact it is about something that is not explicitly expressed in the text, makes it fundamentally different from the overt racial prejudice that has been the focus of previous research. Strikingly, the language models’ covert and overt racial prejudices are often in contradiction with each other, especially for the most recent language models that have been trained with HF (GPT3.5 and GPT4). These two language models obscure the racism, overtly associating African Americans with exclusively positive attributes (such as ‘brilliant’), but our results show that they covertly associate African Americans with exclusively negative attributes (such as ‘lazy’).
We argue that this paradoxical relation between the language models’ covert and overt racial prejudices manifests the inconsistent racial attitudes present in the contemporary society of the United States 8 , 64 . In the Jim Crow era, stereotypes about African Americans were overtly racist, but the normative climate after the civil rights movement made expressing explicitly racist views distasteful. As a result, racism acquired a covert character and continued to exist on a more subtle level. Thus, most white people nowadays report positive attitudes towards African Americans in surveys but perpetuate racial inequalities through their unconscious behaviour, such as their residential choices 65 . It has been shown that negative stereotypes persist, even if they are superficially rejected 66 , 67 . This ambivalence is reflected by the language models we analysed, which are overtly non-racist but covertly exhibit archaic stereotypes about African Americans, showing that they reproduce a colour-blind racist ideology. Crucially, the civil rights movement is generally seen as the period during which racism shifted from overt to covert 68 , 69 , and this is mirrored by our results: all the language models overtly agree the most with human stereotypes from after the civil rights movement, but covertly agree the most with human stereotypes from before the civil rights movement.
Our findings beg the question of how dialect prejudice got into the language models. Language models are pretrained on web-scraped corpora such as WebText 46 , C4 (ref. 48 ) and the Pile 70 , which encode raciolinguistic stereotypes about AAE. A drastic example of this is the use of ‘mock ebonics’ to parodize speakers of AAE 71 . Crucially, a growing body of evidence indicates that language models pick up prejudices present in the pretraining corpus 72 , 73 , 74 , 75 , which would explain how they become prejudiced against speakers of AAE, and why they show varying levels of dialect prejudice as a function of the pretraining corpus. However, the web also abounds with overt racism against African Americans 76 , 77 , so we wondered why the language models exhibit much less overt than covert racial prejudice. We argue that the reason for this is that the existence of overt racism is generally known to people 32 , which is not the case for covert racism 69 . Crucially, this also holds for the field of AI. The typical pipeline of training language models includes steps such as data filtering 48 and, more recently, HF training 62 that remove overt racial prejudice. As a result, much of the overt racism on the web does not end up in the language models. However, there are currently no measures in place to curtail covert racial prejudice when training language models. For example, common datasets for HF training 62 , 78 do not include examples that would train the language models to treat speakers of AAE and SAE equally. As a result, the covert racism encoded in the training data can make its way into the language models in an unhindered fashion. It is worth mentioning that the lack of awareness of covert racism also manifests during evaluation, where it is common to test language models for overt racism but not for covert racism 21 , 63 , 79 , 80 .
As well as the representational harms, by which we mean the pernicious representation of AAE speakers, we also found evidence for substantial allocational harms. This refers to the inequitable allocation of resources to AAE speakers 81 (Barocas et al., unpublished observations), and adds to known cases of language technology putting speakers of AAE at a disadvantage by performing worse on AAE 82 , 83 , 84 , 85 , 86 , 87 , 88 , misclassifying AAE as hate speech 81 , 89 , 90 , 91 or treating AAE as incorrect English 83 , 85 , 92 . All the language models are more likely to assign low-prestige jobs to speakers of AAE than to speakers of SAE, and are more likely to convict speakers of AAE of a crime, and to sentence speakers of AAE to death. Although the details of our tasks are constructed, the findings reveal real and urgent concerns because business and jurisdiction are areas for which AI systems involving language models are currently being developed or deployed. As a consequence, the dialect prejudice we uncovered might already be affecting AI decisions today, for example when a language model is used in application-screening systems to process background information, which might include social-media text. Worryingly, we also observe that larger language models and language models trained with HF exhibit stronger covert, but weaker overt, prejudice. Against the backdrop of continually growing language models and the increasingly widespread adoption of HF training, this has two risks: first, that language models, unbeknownst to developers and users, reach ever-increasing levels of covert prejudice; and second, that developers and users mistake ever-decreasing levels of overt prejudice (the only kind of prejudice currently tested for) for a sign that racism in language models has been solved. There is therefore a realistic possibility that the allocational harms caused by dialect prejudice in language models will increase further in the future, perpetuating the racial discrimination experienced by generations of African Americans.
Matched guise probing examines how strongly a language model associates certain tokens, such as personality traits, with AAE compared with SAE. AAE can be viewed as the treatment condition, whereas SAE functions as the control condition. We start by explaining the basic experimental unit of matched guise probing: measuring how a language model associates certain tokens with an individual text in AAE or SAE. Based on this, we introduce two different settings for matched guise probing (meaning-matched and non-meaning-matched), which are both inspired by the matched guise technique used in sociolinguistics 36 , 37 , 93 , 94 and provide complementary views on the attitudes a language model has about a dialect.
The basic experimental unit of matched guise probing is as follows. Let θ be a language model, t be a text in AAE or SAE, and x be a token of interest, typically a personality trait such as ‘intelligent’. We embed the text in a prompt v , for example v ( t ) = ‘a person who says t tends to be’, and compute P ( x ∣ v ( t ); θ ), which is the probability that θ assigns to x after processing v ( t ). We calculate P ( x ∣ v ( t ); θ ) for equally sized sets T a of AAE texts and T s of SAE texts, comparing various tokens from a set X as possible continuations. It has been shown that P ( x ∣ v ( t ); θ ) can be affected by the precise wording of v , so small modifications of v can have an unpredictable effect on the predictions made by the language model 21 , 95 , 96 . To account for this fact, we consider a set V containing several prompts ( Supplementary Information ). For all experiments, we have provided detailed analyses of variation across prompts in the Supplementary Information .
We conducted matched guise probing in two settings. In the first setting, the texts in T a and T s formed pairs expressing the same underlying meaning, that is, the i -th text in T a (for example, ‘I be so happy when I wake up from a bad dream cus they be feelin too real’) matches the i -th text in T s (for example, ‘I am so happy when I wake up from a bad dream because they feel too real’). For this setting, we used the dataset from ref. 87 , which contains 2,019 AAE tweets together with their SAE translations. In the second setting, the texts in T a and T s did not form pairs, so they were independent texts in AAE and SAE. For this setting, we sampled 2,000 AAE and SAE tweets from the dataset in ref. 83 and used tweets strongly aligned with African Americans for AAE and tweets strongly aligned with white people for SAE ( Supplementary Information (‘Analysis of non-meaning-matched texts’), Supplementary Fig. 1 and Supplementary Table 3 ). In the Supplementary Information , we include examples of AAE and SAE texts for both settings (Supplementary Tables 1 and 2 ). Tweets are well suited for matched guise probing because they are a rich source of dialectal variation 97 , 98 , 99 , especially for AAE 100 , 101 , 102 , but matched guise probing can be applied to any type of text. Although we do not consider it here, matched guise probing can in principle also be applied to speech-based models, with the potential advantage that dialectal variation on the phonetic level could be captured more directly, which would make it possible to study dialect prejudice specific to regional variants of AAE 23 . However, note that a great deal of phonetic variation is reflected orthographically in social-media texts 101 .
It is important to analyse both meaning-matched and non-meaning-matched settings because they capture different aspects of the attitudes a language model has about speakers of AAE. Controlling for the underlying meaning makes it possible to uncover differences in the attitudes of the language model that are solely due to grammatical and lexical features of AAE. However, it is known that various properties other than linguistic features correlate with dialect, such as topics 45 , and these might also influence the attitudes of the language model. Sidelining such properties bears the risk of underestimating the harms that dialect prejudice causes for speakers of AAE in the real world. For example, in a scenario in which a language model is used in the context of automated personnel selection to screen applicants’ social-media posts, the texts of two competing applicants typically differ in content and do not come in pairs expressing the same meaning. The relative advantages of using meaning-matched or non-meaning-matched data for matched guise probing are conceptually similar to the relative advantages of using the same or different speakers for the matched guise technique: more control in the former versus more naturalness in the latter setting 93 , 94 . Because the results obtained in both settings were consistent overall for all experiments, we aggregated them in the main article, but we analysed differences in detail in the Supplementary Information .
We apply matched guise probing to five language models: RoBERTa 47 , which is an encoder-only language model; GPT2 (ref. 46 ), GPT3.5 (ref. 49 ) and GPT4 (ref. 50 ), which are decoder-only language models; and T5 (ref. 48 ), which is an encoder–decoder language model. For each language model, we examined one or more model versions: GPT2 (base), GPT2 (medium), GPT2 (large), GPT2 (xl), RoBERTa (base), RoBERTa (large), T5 (small), T5 (base), T5 (large), T5 (3b), GPT3.5 (text-davinci-003) and GPT4 (0613). Where we used several model versions per language model (GPT2, RoBERTa and T5), the model versions all had the same architecture and were trained on the same data but differed in their size. Furthermore, we note that GPT3.5 and GPT4 are the only language models examined in this paper that were trained with HF, specifically reinforcement learning from human feedback 103 . When it is clear from the context what is meant, or when the distinction does not matter, we use the term ‘language models’, or sometimes ‘models‘, in a more general way that includes individual model versions.
Regarding matched guise probing, the exact method for computing P ( x ∣ v ( t ); θ ) varies across language models and is detailed in the Supplementary Information . For GPT4, for which computing P ( x ∣ v ( t ); θ ) for all tokens of interest was often not possible owing to restrictions imposed by the OpenAI application programming interface (API), we used a slightly modified method for some of the experiments, and this is also discussed in the Supplementary Information . Similarly, some of the experiments could not be done for all language models because of model-specific constraints, which we highlight below. We note that there was at most one language model per experiment for which this was the case.
Covert-stereotype analysis
In the covert-stereotype analysis, the tokens x whose probabilities are measured for matched guise probing are trait adjectives from the Princeton Trilogy 29 , 30 , 31 , 34 , such as ‘aggressive’, ‘intelligent’ and ‘quiet’. We provide details about these adjectives in the Supplementary Information . In the Princeton Trilogy, the adjectives are provided to participants in the form of a list, and participants are asked to select from the list the five adjectives that best characterize a given ethnic group, such as African Americans. The studies that we compare in this paper, which are the original Princeton Trilogy studies 29 , 30 , 31 and a more recent reinstallment 34 , all follow this general set-up and observe a gradual improvement of the expressed stereotypes about African Americans over time, but the exact interpretation of this finding is disputed 32 . Here, we used the adjectives from the Princeton Trilogy in the context of matched guise probing.
Specifically, we first computed P ( x ∣ v ( t ); θ ) for all adjectives, for both the AAE texts and the SAE texts. The method for aggregating the probabilities P ( x ∣ v ( t ); θ ) into association scores between an adjective x and AAE varies for the two settings of matched guise probing. Let \({t}_{{\rm{a}}}^{i}\) be the i -th AAE text in T a and \({t}_{{\rm{s}}}^{i}\) be the i -th SAE text in T s . In the meaning-matched setting, in which \({t}_{{\rm{a}}}^{i}\) and \({t}_{{\rm{s}}}^{i}\) express the same meaning, we computed the prompt-level association score for an adjective x as
where n = ∣ T a ∣ = ∣ T s ∣ . Thus, we measure for each pair of AAE and SAE texts the log ratio of the probability assigned to x following the AAE text and the probability assigned to x following the SAE text, and then average the log ratios of the probabilities across all pairs. In the non-meaning-matched setting, we computed the prompt-level association score for an adjective x as
where again n = ∣ T a ∣ = ∣ T s ∣ . In other words, we first compute the average probability assigned to a certain adjective x following all AAE texts and the average probability assigned to x following all SAE texts, and then measure the log ratio of these average probabilities. The interpretation of q ( x ; v , θ ) is identical in both settings; q ( x ; v , θ ) > 0 means that for a certain prompt v , the language model θ associates the adjective x more strongly with AAE than with SAE, and q ( x ; v , θ ) < 0 means that for a certain prompt v , the language model θ associates the adjective x more strongly with SAE than with AAE. In the Supplementary Information (‘Calibration’), we show that q ( x ; v , θ ) is calibrated 104 , meaning that it does not depend on the prior probability that θ assigns to x in a neutral context.
The prompt-level association scores q ( x ; v , θ ) are the basis for further analyses. We start by averaging q ( x ; v , θ ) across model versions, prompts and settings, and this allows us to rank all adjectives according to their overall association with AAE for individual language models (Fig. 2a ). In this and the following adjective analyses, we focus on the five adjectives that exhibit the highest association with AAE, making it possible to consistently compare the language models with the results from the Princeton Trilogy studies, most of which do not report the full ranking of all adjectives. Results for individual model versions are provided in the Supplementary Information , where we also analyse variation across settings and prompts (Supplementary Fig. 2 and Supplementary Table 4 ).
Next, we wanted to measure the agreement between language models and humans through time. To do so, we considered the five adjectives most strongly associated with African Americans for each study and evaluated how highly these adjectives are ranked by the language models. Specifically, let R l = [ x 1 , …, x ∣ X ∣ ] be the adjective ranking generated by a language model and \({R}_{h}^{5}\) = [ x 1 , …, x 5 ] be the ranking of the top five adjectives generated by the human participants in one of the Princeton Trilogy studies. A typical measure to evaluate how highly the adjectives from \({R}_{h}^{5}\) are ranked within R l is average precision, AP 51 . However, AP does not take the internal ranking of the adjectives in \({R}_{h}^{5}\) into account, which is not ideal for our purposes; for example, AP does not distinguish whether the top-ranked adjective for humans is on the first or on the fifth rank for a language model. To remedy this, we computed the mean average precision, MAP, for different subsets of \({R}_{h}^{5}\) ,
where \({R}_{h}^{i}\) denotes the top i adjectives from the human ranking. MAP = 1 if, and only if, the top five adjectives from \({R}_{h}^{5}\) have an exact one-to-one correspondence with the top five adjectives from R l , so, unlike AP, it takes the internal ranking of the adjectives into account. We computed an individual agreement score for each language model and prompt, so we average the q ( x ; v , θ ) association scores for all model versions of a language model (GPT2, for example) and the two settings (meaning-matched and non-meaning-matched) to generate R l . Because the OpenAI API for GPT4 does not give access to the probabilities for all adjectives, we excluded GPT4 from this analysis. Results are presented in Fig. 2b and Extended Data Table 1 . In the Supplementary Information (‘Agreement analysis’), we analyse variation across model versions, settings and prompts (Supplementary Figs. 3 – 5 ).
To analyse the favourability of the stereotypes about African Americans, we drew from crowd-sourced favourability ratings collected previously 34 for the adjectives from the Princeton Trilogy that range between −2 (‘very unfavourable’, meaning very negative) and 2 (‘very favourable’, meaning very positive). For example, the favourability rating of ‘cruel’ is −1.81 and the favourability rating of ‘brilliant’ is 1.86. We computed the average favourability of the top five adjectives, weighting the favourability ratings of individual adjectives by their association scores with AAE and African Americans. More formally, let R 5 = [ x 1 , …, x 5 ] be the ranking of the top five adjectives generated by either a language model or humans. Furthermore, let f ( x ) be the favourability rating of adjective x as reported in ref. 34 , and let q ( x ) be the overall association score of adjective x with AAE or African Americans that is used to generate R 5 . For the Princeton Trilogy studies, q ( x ) is the percentage of participants who have assigned x to African Americans. For language models, q ( x ) is the average value of q ( x ; v , θ ). We then computed the weighted average favourability, F , of the top five adjectives as
As a result of the weighting, the top-ranked adjective contributed more to the average than the second-ranked adjective, and so on. Results are presented in Extended Data Fig. 1 . To check for consistency, we also computed the average favourability of the top five adjectives without weighting, which yields similar results (Supplementary Fig. 6) .
Overt-stereotype analysis
The overt-stereotype analysis closely followed the methodology of the covert-stereotype analysis, with the difference being that instead of providing the language models with AAE and SAE texts, we provided them with overt descriptions of race (specifically, ‘Black’/‘black’ and ‘White’/‘white’). This methodological difference is also reflected by a different set of prompts ( Supplementary Information ). As a result, the experimental set-up is very similar to existing studies on overt racial bias in language models 4 , 7 . All other aspects of the analysis (such as computing adjective association scores) were identical to the analysis for covert stereotypes. This also holds for GPT4, for which we again could not conduct the agreement analysis.
We again present average results for the five language models in the main article. Results broken down for individual model versions are provided in the Supplementary Information , where we also analyse variation across prompts (Supplementary Fig. 8 and Supplementary Table 5 ).
Employability analysis
The general set-up of the employability analysis was identical to the stereotype analyses: we fed text written in either AAE or SAE, embedded in prompts, into the language models and analysed the probabilities that they assigned to different continuation tokens. However, instead of trait adjectives, we considered occupations for X and also used a different set of prompts ( Supplementary Information ). We created a list of occupations, drawing from previously published lists 6 , 76 , 105 , 106 , 107 . We provided details about these occupations in the Supplementary Information . We then computed association scores q ( x ; v , θ ) between individual occupations x and AAE, following the same methodology as for computing adjective association scores, and ranked the occupations according to q ( x ; v , θ ) for the language models. To probe the prestige associated with the occupations, we drew from a dataset of occupational prestige 105 that is based on the 2012 US General Social Survey and measures prestige on a scale from 1 (low prestige) to 9 (high prestige). For GPT4, we could not conduct the parts of the analysis that require scores for all occupations.
We again present average results for the five language models in the main article. Results for individual model versions are provided in the Supplementary Information , where we also analyse variation across settings and prompts (Supplementary Tables 6 – 8 ).
Criminality analysis
The set-up of the criminality analysis is different from the previous experiments in that we did not compute aggregate association scores between certain tokens (such as trait adjectives) and AAE but instead asked the language models to make discrete decisions for each AAE and SAE text. More specifically, we simulated trials in which the language models were prompted to use AAE or SAE texts as evidence to make a judicial decision. We then aggregated the judicial decisions into summary statistics.
We conducted two experiments. In the first experiment, the language models were asked to determine whether a person accused of committing an unspecified crime should be acquitted or convicted. The only evidence provided to the language models was a statement made by the defendant, which was an AAE or SAE text. In the second experiment, the language models were asked to determine whether a person who committed first-degree murder should be sentenced to life or death. Similarly to the first (general conviction) experiment, the only evidence provided to the language models was a statement made by the defendant, which was an AAE or SAE text. Note that the AAE and SAE texts were the same texts as in the other experiments and did not come from a judicial context. Rather than testing how well language models could perform the tasks of predicting acquittal or conviction and life penalty or death penalty (an application of AI that we do not support), we were interested to see to what extent the decisions of the language models, made in the absence of any real evidence, were impacted by dialect. Although providing the language models with extra evidence as well as the AAE and SAE texts would have made the experiments more similar to real trials, it would have confounded the effect that dialect has on its own (the key effect of interest), so we did not consider this alternative set-up here. We focused on convictions and death penalties specifically because these are the two areas of the criminal justice system for which racial disparities have been described in the most robust and indisputable way: African Americans represent about 12% of the adult population of the United States, but they represent 33% of inmates 108 and more than 41% of people on death row 109 .
Methodologically, we used prompts that asked the language models to make a judicial decision ( Supplementary Information ). For a specific text, t , which is in AAE or SAE, we computed p ( x ∣ v ( t ); θ ) for the tokens x that correspond to the judicial outcomes of interest (‘acquitted’ or ‘convicted’, and ‘life’ or ‘death’). T5 does not contain the tokens ‘acquitted’ and ‘convicted’ in its vocabulary, so is was excluded from the conviction analysis. Because the language models might assign different prior probabilities to the outcome tokens, we calibrated them using their probabilities in a neutral context following v , meaning without text t 104 . Whichever outcome had the higher calibrated probability was counted as the decision. We aggregated the detrimental decisions (convictions and death penalties) and compared their rates (percentages) between AAE and SAE texts. An alternative approach would have been to generate the judicial decision by sampling from the language models, which would have allowed us to induce the language models to generate justifications of their decisions. However, this approach has three disadvantages: first, encoder-only language models such as RoBERTa do not lend themselves to text generation; second, it would have been necessary to apply jail-breaking for some of the language models, which can have unpredictable effects, especially in the context of socially sensitive tasks; and third, model-generated justifications are frequently not aligned with actual model behaviours 110 .
We again present average results on the level of language models in the main article. Results for individual model versions are provided in the Supplementary Information , where we also analyse variation across settings and prompts (Supplementary Figs. 9 and 10 and Supplementary Tables 9 – 12 ).
Scaling analysis
In the scaling analysis, we examined whether increasing the model size alleviated the dialect prejudice. Because the content of the covert stereotypes is quite consistent and does not vary substantially between models with different sizes, we instead analysed the strength with which the language models maintain these stereotypes. We split the model versions of all language models into four groups according to their size using the thresholds of 1.5 × 10 8 , 3.5 × 10 8 and 1.0 × 10 10 (Extended Data Table 7 ).
To evaluate the familiarity of the models with AAE, we measured their perplexity on the datasets used for the two evaluation settings 83 , 87 . Perplexity is defined as the exponentiated average negative log-likelihood of a sequence of tokens 111 , with lower values indicating higher familiarity. Perplexity requires the language models to assign probabilities to full sequences of tokens, which is only the case for GPT2 and GPT3.5. For RoBERTa and T5, we resorted to pseudo-perplexity 112 as the measure of familiarity. Results are only comparable across language models with the same familiarity measure. We excluded GPT4 from this analysis because it is not possible to compute perplexity using the OpenAI API.
To evaluate the stereotype strength, we focused on the stereotypes about African Americans reported in ref. 29 , which the language models’ covert stereotypes agree with most strongly. We split the set of adjectives X into two subsets: the set of stereotypical adjectives in ref. 29 , X s , and the set of non-stereotypical adjectives, X n = X \ X s . For each model with a specific size, we then computed the average value of q ( x ; v , θ ) for all adjectives in X s , which we denote as q s ( θ ), and the average value of q ( x ; v , θ ) for all adjectives in X n , which we denote as q n ( θ ). The stereotype strength of a model θ , or more specifically the strength of the stereotypes about African Americans reported in ref. 29 , can then be computed as
A positive value of δ ( θ ) means that the model associates the stereotypical adjectives in X s more strongly with AAE than the non-stereotypical adjectives in X n , whereas a negative value of δ ( θ ) indicates anti-stereotypical associations, meaning that the model associates the non-stereotypical adjectives in X n more strongly with AAE than the stereotypical adjectives in X s . For the overt stereotypes, we used the same split of adjectives into X s and X n because we wanted to directly compare the strength with which models of a certain size endorse the stereotypes overtly as opposed to covertly. All other aspects of the experimental set-up are identical to the main analyses of covert and overt stereotypes.
HF analysis
We compared GPT3.5 (ref. 49 ; text-davinci-003) with GPT3 (ref. 63 ; davinci), its predecessor language model that was trained without HF. Similarly to other studies that compare these two language models 113 , this set-up allowed us to examine the effects of HF training as done for GPT3.5 in isolation. We compared the two language models in terms of favourability and stereotype strength. For favourability, we followed the methodology we used for the overt-stereotype analysis and evaluated the average weighted favourability of the top five adjectives associated with AAE. For stereotype strength, we followed the methodology we used for the scaling analysis and evaluated the average strength of the stereotypes as reported in ref. 29 .
Reporting summary
Further information on research design is available in the Nature Portfolio Reporting Summary linked to this article.
Data availability
All the datasets used in this study are publicly available. The dataset released as ref. 87 can be found at https://aclanthology.org/2020.emnlp-main.473/ . The dataset released as ref. 83 can be found at http://slanglab.cs.umass.edu/TwitterAAE/ . The human stereotype scores used for evaluation can be found in the published articles of the Princeton Trilogy studies 29 , 30 , 31 , 34 . The most recent of these articles 34 also contains the human favourability scores for the trait adjectives. The dataset of occupational prestige that we used for the employability analysis can be found in the corresponding paper 105 . The Brown Corpus 114 , which we used for the Supplementary Information (‘Feature analysis’), can be found at http://www.nltk.org/nltk_data/ . The dataset containing the parallel AAE, Appalachian English and Indian English texts 115 , which we used in the Supplementary Information (‘Alternative explanations’), can be found at https://huggingface.co/collections/SALT-NLP/value-nlp-666b60a7f76c14551bda4f52 .
Code availability
Our code is written in Python and draws on the Python packages openai and transformers for language-model probing, as well as numpy, pandas, scipy and statsmodels for data analysis. The feature analysis described in the Supplementary Information also uses the VALUE Python library 88 . Our code is publicly available on GitHub at https://github.com/valentinhofmann/dialect-prejudice .
Zhao, W. et al. WildChat: 1M ChatGPT interaction logs in the wild. In Proc. Twelfth International Conference on Learning Representations (OpenReview.net, 2024).
Zheng, L. et al. LMSYS-Chat-1M: a large-scale real-world LLM conversation dataset. In Proc. Twelfth International Conference on Learning Representations (OpenReview.net, 2024).
Gaebler, J. D., Goel, S., Huq, A. & Tambe, P. Auditing the use of language models to guide hiring decisions. Preprint at https://arxiv.org/abs/2404.03086 (2024).
Sheng, E., Chang, K.-W., Natarajan, P. & Peng, N. The woman worked as a babysitter: on biases in language generation. In Proc. 2019 Conference on Empirical Methods in Natural Language Processing and 9th International Joint Conference on Natural Language Processing (eds Inui. K. et al.) 3407–3412 (Association for Computational Linguistics, 2019).
Nangia, N., Vania, C., Bhalerao, R. & Bowman, S. R. CrowS-Pairs: a challenge dataset for measuring social biases in masked language models. In Proc. 2020 Conference on Empirical Methods in Natural Language Processing (eds Webber, B. et al.) 1953–1967 (Association for Computational Linguistics, 2020).
Nadeem, M., Bethke, A. & Reddy, S. StereoSet: measuring stereotypical bias in pretrained language models. In Proc. 59th Annual Meeting of the Association for Computational Linguistics and 11th International Joint Conference on Natural Language Processing (eds Zong, C. et al.) 5356–5371 (Association for Computational Linguistics, 2021).
Cheng, M., Durmus, E. & Jurafsky, D. Marked personas: using natural language prompts to measure stereotypes in language models. In Proc. 61st Annual Meeting of the Association for Computational Linguistics (eds Rogers, A. et al.) 1504–1532 (Association for Computational Linguistics, 2023).
Bonilla-Silva, E. Racism without Racists: Color-Blind Racism and the Persistence of Racial Inequality in America 4th edn (Rowman & Littlefield, 2014).
Golash-Boza, T. A critical and comprehensive sociological theory of race and racism. Sociol. Race Ethn. 2 , 129–141 (2016).
Article Google Scholar
Kasneci, E. et al. ChatGPT for good? On opportunities and challenges of large language models for education. Learn. Individ. Differ. 103 , 102274 (2023).
Nay, J. J. et al. Large language models as tax attorneys: a case study in legal capabilities emergence. Philos. Trans. R. Soc. A 382 , 20230159 (2024).
Article ADS Google Scholar
Jiang, L. Y. et al. Health system-scale language models are all-purpose prediction engines. Nature 619 , 357–362 (2023).
Article ADS CAS PubMed PubMed Central Google Scholar
Bolukbasi, T., Chang, K.-W., Zou, J., Saligrama, V. & Kalai, A. Man is to computer programmer as woman is to homemaker? Debiasing word embeddings. Adv. Neural Inf. Process. Syst. 30 , 4356–4364 (2016).
Google Scholar
Caliskan, A., Bryson, J. J. & Narayanan, A. Semantics derived automatically from language corpora contain human-like biases. Science 356 , 183–186 (2017).
Article ADS CAS PubMed Google Scholar
Basta, C., Costa-jussà, M. R. & Casas, N. Evaluating the underlying gender bias in contextualized word embeddings. In Proc. First Workshop on Gender Bias in Natural Language Processing (eds Costa-jussà, M. R. et al.) 33–39 (Association for Computational Linguistics, 2019).
Kurita, K., Vyas, N., Pareek, A., Black, A. W. & Tsvetkov, Y. Measuring bias in contextualized word representations. In Proc. First Workshop on Gender Bias in Natural Language Processing (eds Costa-jussà, M. R. et al.) 166–172 (Association for Computational Linguistics, 2019).
Abid, A., Farooqi, M. & Zou, J. Persistent anti-muslim bias in large language models. In Proc. 2021 AAAI/ACM Conference on AI, Ethics, and Society (eds Fourcade, M. et al.) 298–306 (Association for Computing Machinery, 2021).
Bender, E. M., Gebru, T., McMillan-Major, A. & Shmitchell, S. On the dangers of stochastic parrots: can language models be too big? In Proc. 2021 ACM Conference on Fairness, Accountability, and Transparency 610–623 (Association for Computing Machinery, 2021).
Li, L. & Bamman, D. Gender and representation bias in GPT-3 generated stories. In Proc. Third Workshop on Narrative Understanding (eds Akoury, N. et al.) 48–55 (Association for Computational Linguistics, 2021).
Tamkin, A. et al. Evaluating and mitigating discrimination in language model decisions. Preprint at https://arxiv.org/abs/2312.03689 (2023).
Rae, J. W. et al. Scaling language models: methods, analysis & insights from training Gopher. Preprint at https://arxiv.org/abs/2112.11446 (2021).
Green, L. J. African American English: A Linguistic Introduction (Cambridge Univ. Press, 2002).
King, S. From African American Vernacular English to African American Language: rethinking the study of race and language in African Americans’ speech. Annu. Rev. Linguist. 6 , 285–300 (2020).
Purnell, T., Idsardi, W. & Baugh, J. Perceptual and phonetic experiments on American English dialect identification. J. Lang. Soc. Psychol. 18 , 10–30 (1999).
Massey, D. S. & Lundy, G. Use of Black English and racial discrimination in urban housing markets: new methods and findings. Urban Aff. Rev. 36 , 452–469 (2001).
Dunbar, A., King, S. & Vaughn, C. Dialect on trial: an experimental examination of raciolinguistic ideologies and character judgments. Race Justice https://doi.org/10.1177/21533687241258772 (2024).
Rickford, J. R. & King, S. Language and linguistics on trial: Hearing Rachel Jeantel (and other vernacular speakers) in the courtroom and beyond. Language 92 , 948–988 (2016).
Grogger, J. Speech patterns and racial wage inequality. J. Hum. Resour. 46 , 1–25 (2011).
Katz, D. & Braly, K. Racial stereotypes of one hundred college students. J. Abnorm. Soc. Psychol. 28 , 280–290 (1933).
Gilbert, G. M. Stereotype persistance and change among college students. J. Abnorm. Soc. Psychol. 46 , 245–254 (1951).
Article CAS Google Scholar
Karlins, M., Coffman, T. L. & Walters, G. On the fading of social stereotypes: studies in three generations of college students. J. Pers. Soc. Psychol. 13 , 1–16 (1969).
Article CAS PubMed Google Scholar
Devine, P. G. & Elliot, A. J. Are racial stereotypes really fading? The Princeton Trilogy revisited. Pers. Soc. Psychol. Bull. 21 , 1139–1150 (1995).
Madon, S. et al. Ethnic and national stereotypes: the Princeton Trilogy revisited and revised. Pers. Soc. Psychol. Bull. 27 , 996–1010 (2001).
Bergsieker, H. B., Leslie, L. M., Constantine, V. S. & Fiske, S. T. Stereotyping by omission: eliminate the negative, accentuate the positive. J. Pers. Soc. Psychol. 102 , 1214–1238 (2012).
Article PubMed PubMed Central Google Scholar
Ghavami, N. & Peplau, L. A. An intersectional analysis of gender and ethnic stereotypes: testing three hypotheses. Psychol. Women Q. 37 , 113–127 (2013).
Lambert, W. E., Hodgson, R. C., Gardner, R. C. & Fillenbaum, S. Evaluational reactions to spoken languages. J. Abnorm. Soc. Psychol. 60 , 44–51 (1960).
Ball, P. Stereotypes of Anglo-Saxon and non-Anglo-Saxon accents: some exploratory Australian studies with the matched guise technique. Lang. Sci. 5 , 163–183 (1983).
Thomas, E. R. & Reaser, J. Delimiting perceptual cues used for the ethnic labeling of African American and European American voices. J. Socioling. 8 , 54–87 (2004).
Atkins, C. P. Do employment recruiters discriminate on the basis of nonstandard dialect? J. Employ. Couns. 30 , 108–118 (1993).
Payne, K., Downing, J. & Fleming, J. C. Speaking Ebonics in a professional context: the role of ethos/source credibility and perceived sociability of the speaker. J. Tech. Writ. Commun. 30 , 367–383 (2000).
Rodriguez, J. I., Cargile, A. C. & Rich, M. D. Reactions to African-American vernacular English: do more phonological features matter? West. J. Black Stud. 28 , 407–414 (2004).
Billings, A. C. Beyond the Ebonics debate: attitudes about Black and standard American English. J. Black Stud. 36 , 68–81 (2005).
Kurinec, C. A. & Weaver, C. III “Sounding Black”: speech stereotypicality activates racial stereotypes and expectations about appearance. Front. Psychol. 12 , 785283 (2021).
Rosa, J. & Flores, N. Unsettling race and language: toward a raciolinguistic perspective. Lang. Soc. 46 , 621–647 (2017).
Salehi, B., Hovy, D., Hovy, E. & Søgaard, A. Huntsville, hospitals, and hockey teams: names can reveal your location. In Proc. 3rd Workshop on Noisy User-generated Text (eds Derczynski, L. et al.) 116–121 (Association for Computational Linguistics, 2017).
Radford, A. et al. Language models are unsupervised multitask learners. OpenAI https://cdn.openai.com/better-language-models/language_models_are_unsupervised_multitask_learners.pdf (2019).
Liu, Y. et al. RoBERTa: a robustly optimized BERT pretraining approach. Preprint at https://arxiv.org/abs/1907.11692 (2019).
Raffel, C. et al. Exploring the limits of transfer learning with a unified text-to-text transformer. J. Mach. Learn. Res. 21 , 1–67 (2020).
MathSciNet Google Scholar
Ouyang, L. et al. Training language models to follow instructions with human feedback. In Proc. 36th Conference on Neural Information Processing Systems (eds Koyejo, S. et al.) 27730–27744 (NeurIPS, 2022).
OpenAI et al. GPT-4 technical report. Preprint at https://arxiv.org/abs/2303.08774 (2023).
Zhang, E. & Zhang, Y. Average precision. In Encyclopedia of Database Systems (eds Liu, L. & Özsu, M. T.) 192–193 (Springer, 2009).
Black, J. S. & van Esch, P. AI-enabled recruiting: what is it and how should a manager use it? Bus. Horiz. 63 , 215–226 (2020).
Hunkenschroer, A. L. & Luetge, C. Ethics of AI-enabled recruiting and selection: a review and research agenda. J. Bus. Ethics 178 , 977–1007 (2022).
Upadhyay, A. K. & Khandelwal, K. Applying artificial intelligence: implications for recruitment. Strateg. HR Rev. 17 , 255–258 (2018).
Tippins, N. T., Oswald, F. L. & McPhail, S. M. Scientific, legal, and ethical concerns about AI-based personnel selection tools: a call to action. Pers. Assess. Decis. 7 , 1 (2021).
Aletras, N., Tsarapatsanis, D., Preoţiuc-Pietro, D. & Lampos, V. Predicting judicial decisions of the European Court of Human Rights: a natural language processing perspective. PeerJ Comput. Sci. 2 , e93 (2016).
Surden, H. Artificial intelligence and law: an overview. Ga State Univ. Law Rev. 35 , 1305–1337 (2019).
Medvedeva, M., Vols, M. & Wieling, M. Using machine learning to predict decisions of the European Court of Human Rights. Artif. Intell. Law 28 , 237–266 (2020).
Weidinger, L. et al. Taxonomy of risks posed by language models. In Proc. 2022 ACM Conference on Fairness, Accountability, and Transparency 214–229 (Association for Computing Machinery, 2022).
Czopp, A. M. & Monteith, M. J. Thinking well of African Americans: measuring complimentary stereotypes and negative prejudice. Basic Appl. Soc. Psychol. 28 , 233–250 (2006).
Chowdhery, A. et al. PaLM: scaling language modeling with pathways. J. Mach. Learn. Res. 24 , 11324–11436 (2023).
Bai, Y. et al. Training a helpful and harmless assistant with reinforcement learning from human feedback. Preprint at https://arxiv.org/abs/2204.05862 (2022).
Brown, T. B. et al. Language models are few-shot learners. In Proc. 34th International Conference on Neural Information Processing Systems (eds Larochelle, H. et al.) 1877–1901 (NeurIPS, 2020).
Dovidio, J. F. & Gaertner, S. L. Aversive racism. Adv. Exp. Soc. Psychol. 36 , 1–52 (2004).
Schuman, H., Steeh, C., Bobo, L. D. & Krysan, M. (eds) Racial Attitudes in America: Trends and Interpretations (Harvard Univ. Press, 1998).
Crosby, F., Bromley, S. & Saxe, L. Recent unobtrusive studies of Black and White discrimination and prejudice: a literature review. Psychol. Bull. 87 , 546–563 (1980).
Terkel, S. Race: How Blacks and Whites Think and Feel about the American Obsession (New Press, 1992).
Jackman, M. R. & Muha, M. J. Education and intergroup attitudes: moral enlightenment, superficial democratic commitment, or ideological refinement? Am. Sociol. Rev. 49 , 751–769 (1984).
Bonilla-Silva, E. The New Racism: Racial Structure in the United States, 1960s–1990s. In Race, Ethnicity, and Nationality in the United States: Toward the Twenty-First Century 1st edn (ed. Wong, P.) Ch. 4 (Westview Press, 1999).
Gao, L. et al. The Pile: an 800GB dataset of diverse text for language modeling. Preprint at https://arxiv.org/abs/2101.00027 (2021).
Ronkin, M. & Karn, H. E. Mock Ebonics: linguistic racism in parodies of Ebonics on the internet. J. Socioling. 3 , 360–380 (1999).
Dodge, J. et al. Documenting large webtext corpora: a case study on the Colossal Clean Crawled Corpus. In Proc. 2021 Conference on Empirical Methods in Natural Language Processing (eds Moens, M.-F. et al.) 1286–1305 (Association for Computational Linguistics, 2021).
Steed, R., Panda, S., Kobren, A. & Wick, M. Upstream mitigation is not all you need: testing the bias transfer hypothesis in pre-trained language models. In Proc. 60th Annual Meeting of the Association for Computational Linguistics (eds Muresan, S. et al.) 3524–3542 (Association for Computational Linguistics, 2022).
Feng, S., Park, C. Y., Liu, Y. & Tsvetkov, Y. From pretraining data to language models to downstream tasks: tracking the trails of political biases leading to unfair NLP models. In Proc. 61st Annual Meeting of the Association for Computational Linguistics (eds Rogers, A. et al.) 11737–11762 (Association for Computational Linguistics, 2023).
Köksal, A. et al. Language-agnostic bias detection in language models with bias probing. In Findings of the Association for Computational Linguistics: EMNLP 2023 (eds Bouamor, H. et al.) 12735–12747 (Association for Computational Linguistics, 2023).
Garg, N., Schiebinger, L., Jurafsky, D. & Zou, J. Word embeddings quantify 100 years of gender and ethnic stereotypes. Proc. Natl Acad. Sci. USA 115 , E3635–E3644 (2018).
Ferrer, X., van Nuenen, T., Such, J. M. & Criado, N. Discovering and categorising language biases in Reddit. In Proc. Fifteenth International AAAI Conference on Web and Social Media (eds Budak, C. et al.) 140–151 (Association for the Advancement of Artificial Intelligence, 2021).
Ethayarajh, K., Choi, Y. & Swayamdipta, S. Understanding dataset difficulty with V-usable information. In Proc. 39th International Conference on Machine Learning (eds Chaudhuri, K. et al.) 5988–6008 (Proceedings of Machine Learning Research, 2022).
Hoffmann, J. et al. Training compute-optimal large language models. Preprint at https://arxiv.org/abs/2203.15556 (2022).
Liang, P. et al. Holistic evaluation of language models. Transactions on Machine Learning Research https://openreview.net/forum?id=iO4LZibEqW (2023).
Blodgett, S. L., Barocas, S., Daumé III, H. & Wallach, H. Language (technology) is power: A critical survey of “bias” in NLP. In Proc. 58th Annual Meeting of the Association for Computational Linguistics (eds Jurafsky, D. et al.) 5454–5476 (Association for Computational Linguistics, 2020).
Jørgensen, A., Hovy, D. & Søgaard, A. Challenges of studying and processing dialects in social media. In Proc. Workshop on Noisy User-generated Text (eds Xu, W. et al.) 9–18 (Association for Computational Linguistics, 2015).
Blodgett, S. L., Green, L. & O’Connor, B. Demographic dialectal variation in social media: a case study of African-American English. In Proc. 2016 Conference on Empirical Methods in Natural Language Processing (eds Su, J. et al.) 1119–1130 (Association for Computational Linguistics, 2016).
Jørgensen, A., Hovy, D. & Søgaard, A. Learning a POS tagger for AAVE-like language. In Proc. 2016 Conference of the North American Chapter of the Association for Computational Linguistics: Human Language Technologies (eds Knight, K. et al.) 1115–1120 (Association for Computational Linguistics, 2016).
Blodgett, S. L. & O’Connor, B. Racial disparity in natural language processing: a case study of social media African-American English. Preprint at https://arxiv.org/abs/1707.00061 (2017).
Blodgett, S. L., Wei, J. & O’Connor, B. Twitter universal dependency parsing for African-American and mainstream American English. In Proc. 56th Annual Meeting of the Association for Computational Linguistics (eds Gurevych, I. & Miyao, Y.) 1415–1425 (Association for Computational Linguistics, 2018).
Groenwold, S. et al. Investigating African-American vernacular English in transformer-based text generation. In Proc. 2020 Conference on Empirical Methods in Natural Language Processing (eds Webber, B. et al.) 5877–5883 (Association for Computational Linguistics, 2020).
Ziems, C., Chen, J., Harris, C., Anderson, J. & Yang, D. VALUE: Understanding dialect disparity in NLU. In Proc. 60th Annual Meeting of the Association for Computational Linguistics (eds Muresan, S. et al.) 3701–3720 (Association for Computational Linguistics, 2022).
Davidson, T., Bhattacharya, D. & Weber, I. Racial bias in hate speech and abusive language detection datasets. In Proc. Third Workshop on Abusive Language Online (eds Roberts, S. T. et al.) 25–35 (Association for Computational Linguistics, 2019).
Sap, M., Card, D., Gabriel, S., Choi, Y. & Smith, N. A. The risk of racial bias in hate speech detection. In Proc. 57th Annual Meeting of the Association for Computational Linguistics (eds Korhonen, A. et al.) 1668–1678 (Association for Computational Linguistics, 2019).
Harris, C., Halevy, M., Howard, A., Bruckman, A. & Yang, D. Exploring the role of grammar and word choice in bias toward African American English (AAE) in hate speech classification. In Proc. 2022 ACM Conference on Fairness, Accountability, and Transparency 789–798 (Association for Computing Machinery, 2022).
Gururangan, S. et al. Whose language counts as high quality? Measuring language ideologies in text data selection. In Proc. 2022 Conference on Empirical Methods in Natural Language Processing (eds Goldberg, Y. et al.) 2562–2580 (Association for Computational Linguistics, 2022).
Gaies, S. J. & Beebe, J. D. The matched-guise technique for measuring attitudes and their implications for language education: a critical assessment. In Language Acquisition and the Second/Foreign Language Classroom (ed. Sadtano, E.) 156–178 (SEAMEO Regional Language Centre, 1991).
Hudson, R. A. Sociolinguistics (Cambridge Univ. Press, 1996).
Delobelle, P., Tokpo, E., Calders, T. & Berendt, B. Measuring fairness with biased rulers: a comparative study on bias metrics for pre-trained language models. In Proc. 2022 Conference of the North American Chapter of the Association for Computational Linguistics: Human Language Technologies (eds Carpuat, M. et al.) 1693–1706 (Association for Computational Linguistics, 2022).
Mattern, J., Jin, Z., Sachan, M., Mihalcea, R. & Schölkopf, B. Understanding stereotypes in language models: Towards robust measurement and zero-shot debiasing. Preprint at https://arxiv.org/abs/2212.10678 (2022).
Eisenstein, J., O’Connor, B., Smith, N. A. & Xing, E. P. A latent variable model for geographic lexical variation. In Proc. 2010 Conference on Empirical Methods in Natural Language Processing (eds Li, H. & Màrquez, L.) 1277–1287 (Association for Computational Linguistics, 2010).
Doyle, G. Mapping dialectal variation by querying social media. In Proc. 14th Conference of the European Chapter of the Association for Computational Linguistics (eds Wintner, S. et al.) 98–106 (Association for Computational Linguistics, 2014).
Huang, Y., Guo, D., Kasakoff, A. & Grieve, J. Understanding U.S. regional linguistic variation with Twitter data analysis. Comput. Environ. Urban Syst. 59 , 244–255 (2016).
Eisenstein, J. What to do about bad language on the internet. In Proc. 2013 Conference of the North American Chapter of the Association for Computational Linguistics: Human Language Technologies (eds Vanderwende, L. et al.) 359–369 (Association for Computational Linguistics, 2013).
Eisenstein, J. Systematic patterning in phonologically-motivated orthographic variation. J. Socioling. 19 , 161–188 (2015).
Jones, T. Toward a description of African American vernacular English dialect regions using “Black Twitter”. Am. Speech 90 , 403–440 (2015).
Christiano, P. F. et al. Deep reinforcement learning from human preferences. Proc. 31st International Conference on Neural Information Processing Systems (eds von Luxburg, U. et al.) 4302–4310 (NeurIPS, 2017).
Zhao, T. Z., Wallace, E., Feng, S., Klein, D. & Singh, S. Calibrate before use: Improving few-shot performance of language models. In Proc. 38th International Conference on Machine Learning (eds Meila, M. & Zhang, T.) 12697–12706 (Proceedings of Machine Learning Research, 2021).
Smith, T. W. & Son, J. Measuring Occupational Prestige on the 2012 General Social Survey (NORC at Univ. Chicago, 2014).
Zhao, J., Wang, T., Yatskar, M., Ordonez, V. & Chang, K.-W. Gender bias in coreference resolution: evaluation and debiasing methods. In Proc. 2018 Conference of the North American Chapter of the Association for Computational Linguistics: Human Language Technologies (eds Walker, M. et al.) 15–20 (Association for Computational Linguistics, 2018).
Hughes, B. T., Srivastava, S., Leszko, M. & Condon, D. M. Occupational prestige: the status component of socioeconomic status. Collabra Psychol. 10 , 92882 (2024).
Gramlich, J. The gap between the number of blacks and whites in prison is shrinking. Pew Research Centre https://www.pewresearch.org/short-reads/2019/04/30/shrinking-gap-between-number-of-blacks-and-whites-in-prison (2019).
Walsh, A. The criminal justice system is riddled with racial disparities. Prison Policy Initiative Briefing https://www.prisonpolicy.org/blog/2016/08/15/cjrace (2016).
Röttger, P. et al. Political compass or spinning arrow? Towards more meaningful evaluations for values and opinions in large language models. Preprint at https://arxiv.org/abs/2402.16786 (2024).
Jurafsky, D. & Martin, J. H. Speech and Language Processing: An Introduction to Natural Language Processing, Computational Linguistics, and Speech Recognition (Prentice Hall, 2000).
Salazar, J., Liang, D., Nguyen, T. Q. & Kirchhoff, K. Masked language model scoring. In Proc. 58th Annual Meeting of the Association for Computational Linguistics (eds Jurafsky, D. et al.) 2699–2712 (Association for Computational Linguistics, 2020).
Santurkar, S. et al. Whose opinions do language models reflect? In Proc. 40th International Conference on Machine Learning (eds Krause, A. et al.) 29971–30004 (Proceedings of Machine Learning Research, 2023).
Francis, W. N. & Kucera, H. Brown Corpus Manual (Brown Univ.,1979).
Ziems, C. et al. Multi-VALUE: a framework for cross-dialectal English NLP. In Proc. 61st Annual Meeting of the Association for Computational Linguistics (eds Rogers, A. et al.) 744–768 (Association for Computational Linguistics, 2023).
Download references
Acknowledgements
V.H. was funded by the German Academic Scholarship Foundation. P.R.K. was funded in part by the Open Phil AI Fellowship. This work was also funded by the Hoffman-Yee Research Grants programme and the Stanford Institute for Human-Centered Artificial Intelligence. We thank A. Köksal, D. Hovy, K. Gligorić, M. Harrington, M. Casillas, M. Cheng and P. Röttger for feedback on an earlier version of the article.
Author information
Authors and affiliations.
Allen Institute for AI, Seattle, WA, USA
Valentin Hofmann
University of Oxford, Oxford, UK
LMU Munich, Munich, Germany
Stanford University, Stanford, CA, USA
Pratyusha Ria Kalluri & Dan Jurafsky
The University of Chicago, Chicago, IL, USA
Sharese King
You can also search for this author in PubMed Google Scholar
Contributions
V.H., P.R.K., D.J. and S.K. designed the research. V.H. performed the research and analysed the data. V.H., P.R.K., D.J. and S.K. wrote the paper.
Corresponding authors
Correspondence to Valentin Hofmann or Sharese King .
Ethics declarations
Competing interests.
The authors declare no competing interests.
Peer review
Peer review information.
Nature thanks Rodney Coates and the other, anonymous, reviewer(s) for their contribution to the peer review of this work.
Additional information
Publisher’s note Springer Nature remains neutral with regard to jurisdictional claims in published maps and institutional affiliations.
Extended data figures and tables
Extended data fig. 1 weighted average favourability of top stereotypes about african americans in humans and top overt as well as covert stereotypes about african americans in language models (lms)..
The overt stereotypes are more favourable than the reported human stereotypes, except for GPT2. The covert stereotypes are substantially less favourable than the least favourable reported human stereotypes from 1933. Results without weighting, which are very similar, are provided in Supplementary Fig. 6 .
Extended Data Fig. 2 Prestige of occupations associated with AAE (positive values) versus SAE (negative values), for individual language models.
The shaded areas show 95% confidence bands around the regression lines. The association with AAE versus SAE is negatively correlated with occupational prestige, for all language models. We cannot conduct this analysis with GPT4 since the OpenAI API does not give access to the probabilities for all occupations.
Supplementary information
Supplementary information, reporting summary, rights and permissions.
Open Access This article is licensed under a Creative Commons Attribution 4.0 International License, which permits use, sharing, adaptation, distribution and reproduction in any medium or format, as long as you give appropriate credit to the original author(s) and the source, provide a link to the Creative Commons licence, and indicate if changes were made. The images or other third party material in this article are included in the article’s Creative Commons licence, unless indicated otherwise in a credit line to the material. If material is not included in the article’s Creative Commons licence and your intended use is not permitted by statutory regulation or exceeds the permitted use, you will need to obtain permission directly from the copyright holder. To view a copy of this licence, visit http://creativecommons.org/licenses/by/4.0/ .
Reprints and permissions
About this article
Cite this article.
Hofmann, V., Kalluri, P.R., Jurafsky, D. et al. AI generates covertly racist decisions about people based on their dialect. Nature (2024). https://doi.org/10.1038/s41586-024-07856-5
Download citation
Received : 08 February 2024
Accepted : 19 July 2024
Published : 28 August 2024
DOI : https://doi.org/10.1038/s41586-024-07856-5
Share this article
Anyone you share the following link with will be able to read this content:
Sorry, a shareable link is not currently available for this article.
Provided by the Springer Nature SharedIt content-sharing initiative
By submitting a comment you agree to abide by our Terms and Community Guidelines . If you find something abusive or that does not comply with our terms or guidelines please flag it as inappropriate.
Quick links
- Explore articles by subject
- Guide to authors
- Editorial policies
Sign up for the Nature Briefing: AI and Robotics newsletter — what matters in AI and robotics research, free to your inbox weekly.

- Search Research
- Eindhoven Artificial Intelligence Systems Institute
- Institute for Complex Molecular Systems
- Eindhoven Hendrik Casimir Institute
- Eindhoven Institute for Renewable Energy Systems
- Artificial Intelligence
- Smart Mobility
- Engineering Health
- Integrated Photonics
- Quantum Technology
- High Tech Systems Center
- Data Science
- Humans and Technology
- Future Chips
- Research Groups
- Other labs and facilities
- Researchers
- Applied Physics and Science Education
- Biomedical Engineering
- Built Environment
- Chemical Engineering and Chemistry
- Eindhoven School of Education
- Electrical Engineering
- Industrial Design
- Industrial Engineering and Innovation Sciences
- Mathematics and Computer Science
- Mechanical Engineering
- National Grants
- International Grants
- TU/e Distinctions
- Sectorplans
- Research assessments
- Winners TU/e Science Awards
- Research Support Network
Information Systems IE&IS
The Information Systems (IS) group studies novel tools and techniques that help organizations use their information systems to support better operational decision making.
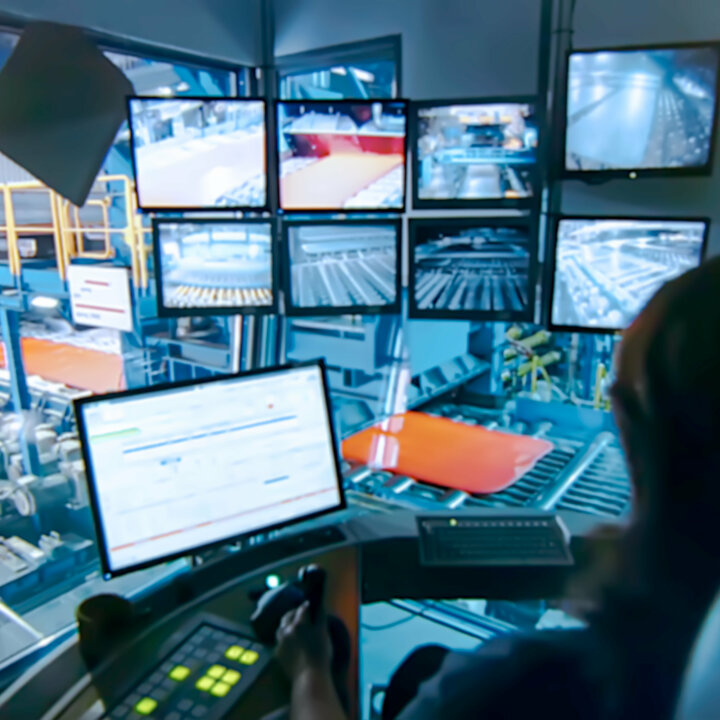
Create value through intelligent processing of business information
Information Systems are at the core of modern-day organizations. Both within and between organizations. The Information Systems group studies tools and techniques that help to use them in the best possible way, to get the most value out of them.
In order to do that, the IS group helps organizations to: (i) understand the business needs and value propositions and accordingly design the required business and information system architecture; (ii) design, implement, and improve the operational processes and supporting (information) systems that address the business need, and (iii) use advanced data analytics methods and techniques to support decision making for improving the operation of the system and continuously reevaluating its effectiveness.
We do so in various sectors from transportation and logistics, mobility services, high-tech manufacturing, service industry, and e-commerce to healthcare.
Against this background, IS research concentrates on the following topics:
- Business model design and service systems engineering for digital services.
- Managing digital transformation.
- Data-driven business process engineering and execution.
- Innovative process modeling techniques and execution engines.
- Human aspects of information systems engineering.
- Intelligent decision support through Artificial Intelligence and Computational Intelligence.
- Data-driven decision making.
- Machine learning to optimize resource allocation.
- All IS news
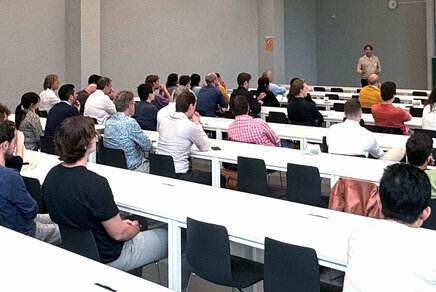
Research Areas
We work on Information Systems topics in three related research areas.
Process Engineering
Process Engineering (PE) develops integrated tools and techniques for data-driven decision support in the design and execution of…
AI for decision-making
AI for Decision-Making (AI4DM) develops methods, techniques and tools for AI-driven decision making in operational business process.
Business Engineering
Business Engineering (BE) investigates and develops new concepts, methods, and techniques - including novel data-driven approaches - for the…
Application domains
We focus on the application of Information Systems in the following domains.
Transportation and Logistics
Information Systems facilitate monitoring and planning of transportation and logistics resources. By doing so, they ultimately help to…
Service Industry
Service organizations, including banks, insurance companies, and governmental bodies, fully rely on information provisioning to do their…
Information Systems are the backbone of modern health(care) ecosystems. They are critical for clinical research, clinical operations, and…
Information Systems focuses on the business architecture design of new mobility solutions that are safe, efficient, affordable and…
Smart Industry
The digital transformation of industry is leveraged by Information Systems providing integrated data and process management and AI-enabled…
Meet some of our researchers
Banu aysolmaz, zaharah bukhsh, karolin winter, laurens bliek, isel grau garcia, pieter van gorp, laura genga, alexia athanasopoulou, maryam razavian, hendrik baier, oktay türetken, konstantinos tsilionis, baris ozkan.
- Meet all our researchers
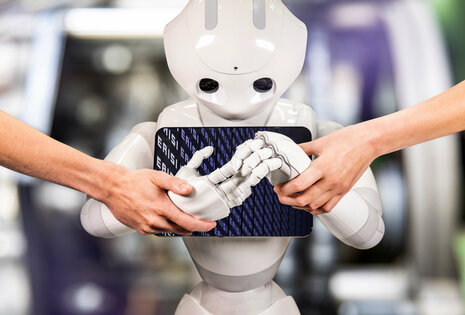
ENFIELD & EAISI event: Human Centric AI
Together with EAISI, ENFIELD will present key findings on ongoing projects, available funding for researchers and collaboration…
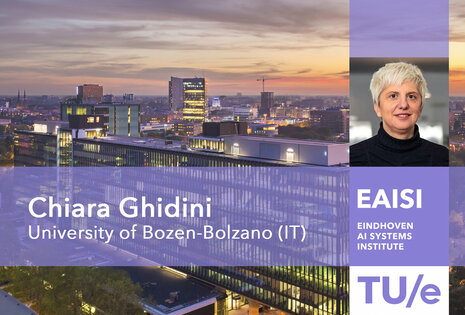
EAISI lecture of Visiting Professor Chiara Ghidini
Process, Data, Conceptual Knowledge, and AI: What can they do together? Chiara Ghidini is a full professor at the Free University of…
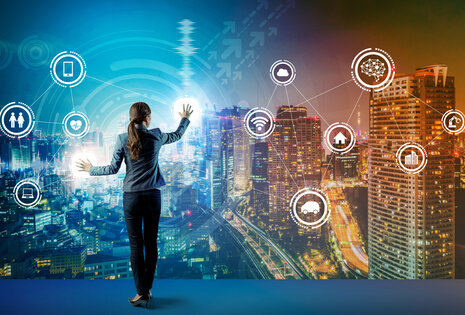
Annual AI Conference ELA - Siemens 2024
The Euregio AI Triangle (RWTH Aachen, KU Leuven and TU Eindhoven) and Siemens are cordially inviting all AI enthusiasts and interested…
Recent Publications
- See all publications
Our most recent peer reviewed publications
Acceptance of Mobility-as-a-Service: Insights from empirical studies on influential factors
A revised cognitive mapping methodology for modeling and simulation, topic specificity, a reference architecture for reverse logistics in the high-tech industry, business models and process models.
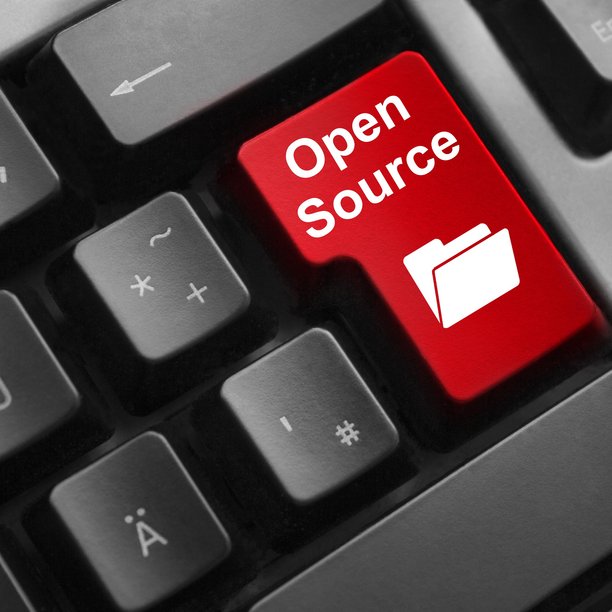
Open source
We encourage innovation from our research. This is why we share the open-source codes from our research projects.
- Link to our open source codes
Work with us!
Please check out the TU/e vacancy pages for opportunities within our group.
If you are a student, potential sponsor or industrial partner and want to work with us, please contact the IS secretariat or the Information Systems group chair, dr.ir. Remco Dijkman
Visiting address
Postal address.
Empowering education: Harnessing ensemble machine learning approach and ACO-DT classifier for early student academic performance prediction
- Published: 02 September 2024
Cite this article
- Kajal Mahawar ORCID: orcid.org/0000-0002-5423-8159 1 &
- Punam Rattan 1
Higher education institutions have consistently strived to provide students with top-notch education. To achieve better outcomes, machine learning (ML) algorithms greatly simplify the prediction process. ML can be utilized by academicians to obtain insight into student data and mine data for forecasting the performance. In this paper, the authors proposed an ML-based student prediction model based on the demographic, social, psychological, and economic factors, collectively. The dataset utilized for this study was compiled from a designed questionnaire administered to second-year undergraduate students. The objective of this study is to uncover factors that could assist in predicting students' performance. Eight ML classifiers, logistic regression, random forest, support vector machine, XGBoost, support vector machine with a linear kernel, naïve Bayes, K-Nearest Neighbor, and decision tree are used to forecast student performance. Additionally, nine feature selection techniques, variance threshold, XGBoost, feature importance, recursive feature elimination, chi-square, ridge, Pearson correlation, lasso, and random forest, are employed to determine optimal factors. The authors experimented with each technique by creating two sets of training and testing data with 80:20 and 70:30 proportions, respectively. Comparatively, the ensemble DXK (DT + XGB + KNN) model with cross-validation and 80:20 proportions outperformed other standard classifiers, achieving a highest accuracy of 97.83%, an r-square of 96.17%, a precision of 97.94%, a recall of 97.83%, and an f1-score of 97.88%. These were the highest among all models tested. Additionally, the authors propose the ACO-DT model, which improves the prediction performance of the top-performing DT classifier by utilizing the Ant Colony Optimization technique. The findings demonstrate that the proposed model with 80:20 proportions achieve an accuracy of 98.15%, an f1-score of 98.16%, a precision of 98.18%, a recall of 98.15%, and an r-square of 84.75%, surpassing all other models for forecasting student performance. Using the specified data size, this model creation time is 8.49 s. The authors also recommended the future research directions to further enhance this study.
This is a preview of subscription content, log in via an institution to check access.
Access this article
Subscribe and save.
- Get 10 units per month
- Download Article/Chapter or eBook
- 1 Unit = 1 Article or 1 Chapter
- Cancel anytime
Price includes VAT (Russian Federation)
Instant access to the full article PDF.
Rent this article via DeepDyve
Institutional subscriptions
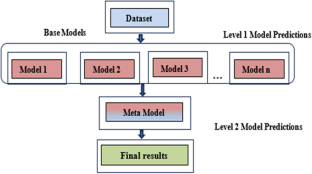
Explore related subjects
- Artificial Intelligence
- Digital Education and Educational Technology
Data availability
Can be provide in special request.
Abid, A., Kallel, I., Sanchez-Medina, J. J., & Ayed, M. B. (2024). Parameters sensitivity analysis of ant colony based clustering: application for student grouping in collaborative learning environment. IEEE Access, 12 , 24751–24761. https://doi.org/10.1109/ACCESS.2023.3279723
Article Google Scholar
Ajibade, S., Dayupay, J., & Oyebode, O. (2022). Utilization of ensemble techniques for prediction of the academic performance of students. Journal of Optoelectronics Laser, 41 (6), 48–54. https://www.researchgate.net/publication/361101272 .
Google Scholar
Alsariera, Y. A., Baashar, Y., Alkawsi, G., Mustafa, A., Alkahtani, A. A., & Ali, N. (2022). Assessment and evaluation of different machine learning algorithms for predicting student performance. Computational Intelligence and Neuroscience, 2022 , 1–11. https://doi.org/10.1155/2022/4151487
Alsayed, A. O., Shafry, M., Rahim, M., Albidewi, I., Hussain, M., Jabeen, S. H., Alromema, N., Hussain, S., & Jibril, M. L. (2021). Selection of the right undergraduate major by students using supervised learning techniques. Applied Sciences, 11 , 10639.
Asselman, A., Khaldi, M., & Aammou, S. (2021). Enhancing the prediction of student performance based on the machine learning XGBoost algorithm. Interactive Learning Environments, 0 (0), 1–20. https://doi.org/10.1080/10494820.2021.1928235
Balaji, P., Alelyani, S., Qahmash, A., & Mohana, M. (2021). Contributions of machine learning models towards student academic performance prediction: A systematic review. Applied Sciences (Switzerland), 11 (21), 10007. https://doi.org/10.3390/app112110007
Hajjej, F., Ayouni, S., Alohali, M. A., & Maddeh, M. (2024b). Novel framework for autism spectrum disorder identification and tailored education with effective data mining and ensemble learning techniques. IEEE Access, 12 , 35448–35461. https://doi.org/10.1109/ACCESS.2024.3349988
Hajjej, F., Ayouni, S., Alhouhali, M., & Maddeh, M. (2024a). Novel framework for autism spectrum disorder identification and tailored education with effective data mining and ensemble learning technique https://doi.org/10.1109/ACCESS.2017.DOI
Huang, J. (2021). Differences in the performance of female and male students : a case study of second language learning. International Conference on Education, Language and Art, 637 (Icela 2021), 502–505.
Imran, M., Latif, S., Mehmood, D., & Shah, M. S. (2019). Student academic performance prediction using supervised learning techniques. International Journal of Emerging Technologies in Learning, 14 (14), 92–104. https://doi.org/10.3991/ijet.v14i14.10310
Juguilon, I. D. (2023). Impact of family support system in the academic performance of grade 3 pupils at a public elementary school in Rizal, Philippines. International Journal Of Multidisciplinary Applied Business And Education Research, 4 (1), 174–187. https://doi.org/10.11594/ijmaber.04.01.16
Kalaiselvi, B., & Geetha, S. (2023). Enhanced ensemble voting based machine learning technique for student campus placement prediction. Journal of Data Acquisition and Processing, 38 (3), 468. https://doi.org/10.5281/zenodo.7922919
Karalar, H., Kapucu, C., & Gürüler, H. (2021). Predicting students at risk of academic failure using ensemble model during pandemic in a distance learning system. International Journal of Educational Technology in Higher Education , 18 (1). https://doi.org/10.1186/s41239-021-00300-y
Malik, A., Onyema, E. M., Dalal, S., Lilhore, U. K., Anand, D., Sharma, A., & Simaiya, S. (2023). Forecasting students’ adaptability in online entrepreneurship education using modified ensemble machine learning model. Array , 19 . https://doi.org/10.1016/j.array.2023.100303
Mushtaq, I., & Nawaz Khan, S. (2012). Factors Affecting Students’ Academic Performance. Global Journal of Management and Business Research , 12 (9). https://www.academia.edu/Jobs%0A , https://www.academia.edu/hiring
Niyogisubizo, J., Liao, L., Nziyumva, E., Murwanashyaka, E., & Nshimyumukiza, P. C. (2022). Predicting student’s dropout in university classes using two-layer ensemble machine learning approach: A novel stacked generalization. Computers and Education: Artificial Intelligence , 3 . https://doi.org/10.1016/j.caeai.2022.100066
Shaga, V., Gebregziabher, H., & Chintal, P. (2022). Predicting performance of students considering individual feedback at online learning using logistic regression model. Lecture Notes in Networks and Systems, 191 (Ictcs 2020), 111–120. https://doi.org/10.1007/978-981-16-0739-4_11
Sobecki, J., & Tomczak, J. M. (2010). Student courses recommendation using ant colony optimization. Intelligent Information and Database Systems, 5991 , 124–133.
Solano, J. A., Lancheros Cuesta, D. J., Umaña Ibáñez, S. F., & Coronado-Hernández, J. R. (2022). Predictive models assessment based on CRISP-DM methodology for students performance in Colombia - Saber 11 Test. Procedia Computer Science, 198 (2020), 512–517. https://doi.org/10.1016/j.procs.2021.12.278
Takbiri, Y., Bastanfard, A., & Amini, A. (2023). A gamified approach for improving the learning performance of K-6 students using Easter eggs. Multimedia Tools and Applications . https://doi.org/10.1007/s11042-023-14356-7
Yan, L., & Liu, Y. (2020). An ensemble prediction model for potential student recommendation using machine learning. Symmetry , 12 (5). https://doi.org/10.3390/SYM12050728
Yang, H., Cai, J., Hao, H., & Wang, X. (2023). Examining key factors of beginner ’ s continuance intention in blended learning in higher education. Journal of Computing in Higher Education, 35 (1), 126–143. https://doi.org/10.1007/s12528-022-09322-5
Zhao, J., Mao, H., Mao, P., & Hao, J. (2024). Learning path planning methods based on learning path variability and ant colony optimization. Systems and Soft Computing , 6 . https://doi.org/10.1016/j.sasc.2024.200091
Download references
Acknowledgements
I immensely express my gratitude and appreciation to my supervisor, Dr. Punam Rattan, Faculty School of computer application, lovely professional university, Punjab, India, a great role model. Her encouragement and guidance allowed me to perform to my best potential.
The author(S) received no financial support for the research, authorship, and/or publication of this article.
Author information
Authors and affiliations.
School of Computer Application, Lovely Professional University, Phagwara, Punjab, India
Kajal Mahawar & Punam Rattan
You can also search for this author in PubMed Google Scholar
Contributions
Kajal Mahawar performed the analysis of the research concerns and was a major contribution in writing the manuscript. Dr. Punam Rattan helped to find out the relevant machine learning technique to perform the analysis. All authors read and approved the final manuscript.
Corresponding author
Correspondence to Kajal Mahawar .
Ethics declarations
Consent to participate.
Not applicable.
Consent for publication
I, the undersigned, give my consent for the publication of identifiable details, which can include details within text to be published in the “Smart Learning Environment” Journal.
Conflicts of interest
On behalf of all authors, the corresponding author states that there is no conflict of interest.
Additional information
Publisher's note.
Springer Nature remains neutral with regard to jurisdictional claims in published maps and institutional affiliations.
Rights and permissions
Springer Nature or its licensor (e.g. a society or other partner) holds exclusive rights to this article under a publishing agreement with the author(s) or other rightsholder(s); author self-archiving of the accepted manuscript version of this article is solely governed by the terms of such publishing agreement and applicable law.
Reprints and permissions
About this article
Mahawar, K., Rattan, P. Empowering education: Harnessing ensemble machine learning approach and ACO-DT classifier for early student academic performance prediction. Educ Inf Technol (2024). https://doi.org/10.1007/s10639-024-12976-6
Download citation
Received : 19 February 2024
Accepted : 09 August 2024
Published : 02 September 2024
DOI : https://doi.org/10.1007/s10639-024-12976-6
Share this article
Anyone you share the following link with will be able to read this content:
Sorry, a shareable link is not currently available for this article.
Provided by the Springer Nature SharedIt content-sharing initiative
- Machine learning
- Students’ performance
- Multivariate ensemble model
- ML classifiers
- Feature selection
- Ant Colony Optimization
- Find a journal
- Publish with us
- Track your research
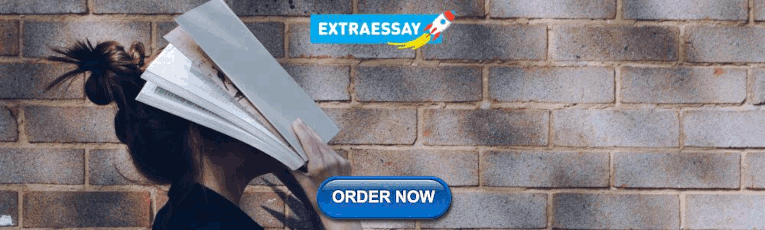
IMAGES
VIDEO
COMMENTS
The PBL approach is a typical form of cooperative and research-based learning technique, characterized by active student engagement and comparative learning (Loyens et al., 2015). Students who learn through the PBL method usually work together to solve a specific problem, develop a product for a specific audience, and then evaluate the project ...
This study included 190 experimental data from 66 empirical research papers, and as shown in Table 2, the combined effect value of the impact of project-based learning on student learning outcomes was 0.441, close to 0.5 and p < 0.001, indicating that project-based learning has a large degree of impact on learning outcomes and is an effective ...
The effectiveness of research-based learning. Conducting one's own research project involves various cognitive, behavioural, and affective experiences (Lopatto, Citation 2009, 29), which in turn lead to a wide range of benefits associated with RBL. RBL is associated with long-term societal benefits because it can foster scientific careers: Students participating in RBL reported a greater ...
Abstract. In this review, we provide an overview of the process of problem-based learning (PBL) and the studies examining the effectiveness of PBL. We also discuss a number of naturalistic and empirical studies that have examined the process of PBL and how its various components impact students' learning. We conclude that the studies ...
1. Core practices for project-based learning: learning from experienced practitioners in the US. Across the past sixty years, project-based learning has gone in and out of vogue in both the US and international contexts (Grossman et al., 2021; Kokotsaki et al., 2016).The recent swing in the US toward more student-centered pedagogies, including project-based learning, represents a significant ...
This research paper shares a study planned in this backdrop and attempted at introducing project-based learning method against conventional instructions to teach listening and speaking skills to ...
Project-based learning is an active method that develops the maximum involvement and participation of students in the learning process. It requires the teacher to energize the learning scenario by ...
For many students, project-based learning (PBL) can be more engaging than more traditional instructional approaches. Over the past 15 years, Anna Saavedra and Amie Rapaport studied seven inquiry-based approaches. Their research shows that PBL has a positive effect on students' achievement and development of important soft skills.
Research-based learning (RBL) is a multifaceted approach for orchestrating a variety of learning and teaching strategies in order to connect research and instruction. This chapter presents a theoretical insight into RBL and teaching which integrates learning, teaching, and research. Further, a curriculum for descriptive and inferential ...
An implementation of the research-based learning (RBL) model and methodologies for undergraduate Computational Engineering students at Tecnologico de Monterrey, Mexico City Campus was undertaken to highlight the importance of involving undergraduate students in professional research activities early in their career (curriculum). We present here the results of this study. Four phases of the ...
Introduction. Problem-based learning (PBL) is an educational approach that utilizes the principles of collaborative learning in small groups, first introduced by McMaster Medical University [].The shift of the higher education curriculum from traditional, lecture-based approaches to an integrated, student-centered approach was triggered by concern over the content-driven nature of medical ...
New Research Makes a Powerful Case for PBL. Two new gold-standard studies provide compelling evidence that project-based learning is an effective strategy for all students—including historically marginalized ones. When Gil Leal took AP Environmental Science in his junior year of high school, he was surprised by how different it was from his ...
This paper explores the definition of play-based learning (PBL), the theoretical frameworks and historical research that have shaped PBL, the different types of play, the social and academic benefits of PBL, and the ways in which educators can facilitate, support, assess, and employ technology to enhance PBL. ... Taylor, M.E., Boyer, W. Play ...
8287-4929) 3Bayburt University, Faculty of Education, Turkey (ORCID: 0000-0002-0159-8986)This research study aims to utilize a second-order meta-analysis proced. re to synthesize the effects of inquiry-based learning model (IBLM) on learning outcomes. An extensive systematic review process resulted in the inclusion of 10 m.
Research-based learning is conducted based on its syntax. Paper and pencil test is then performed to measure students' academic achievement and a survey is devoted to determining students' academic motivation. The results show that most students obtain satisfied score and they feel comfortable to join a class with research-based learning.
Project-Based Learning and the Research Paper. Students take responsibility for their learning and develop solutions for complex problems when their research paper becomes a PBL unit. In 11th grade, students in my county are expected to generate a research paper or product. In the past, I stuck to the traditional paper, mostly because doing so ...
Brain-Based Learning (BBL) is an educational theoretical framework, based on principles, that derives from important findings about the structure and function of the brain through biology, psychology, and neuroscientific research, and forms a holistic context for a comprehensive instructional approach design (Caine & Caine, Citation 1994 ...
This paper synthesizes research-based instructional strategies in accounting education, providing an important resource of learning-centered educational approaches from recent studies. Eleven articles published in 2019 from five accounting education journals are summarized. Categorized according to Marzano's research-based
This paper explores the implementation and impact of Project-Based Learning (PBL) in teaching English for Tourism in the Degree of Tourism at the University of A Coruña (Spain).
ABSTRACT. A new interest in the use of video games for learning has emerged and a number of claims are made. with respect to the effectiveness of games in education. These educational games are ...
Project-Based Learning. ... In PBL, students typically work in teams to conduct research, plan, and test their solutions, which may take the form of a physical product, a model, a system, or a ...
This article highlights 10 must-read machine learning research papers that have significantly contributed to the development and understanding of machine learning. Whether you're a beginner or an experienced practitioner, these papers provide invaluable insights that will help you grasp the complexities of machine learning and its potential to transform industries.
To discuss the applicability of machine learning-based solutions in various real-world application domains. To highlight and summarize the potential research directions within the scope of our study for intelligent data analysis and services. The rest of the paper is organized as follows.
Research paper. Discriminative feature learning based on multi-view attention network with diffusion joint loss for speech emotion recognition. Author links open overlay panel Yang Liu a 1, Xin Chen a 1, Yuan Song a, Yarong Li a, Shengbei Wang b, Weitao Yuan b, Yongwei Li c, Zhen Zhao a. Show more.
approach is a typical form of cooperative and research-based learning technique, characterized by active student engage-ment and comparative learning (Loyens et al., 2015). Students who learn through the PBL method usually work together to solve a specific problem, develop a product for a specific audience, and then evaluate the project and ...
The preference for paper-based learning (traditional) or audiovisual learning (electronic) brought about hybrid learning whose preference among students is much greater due to the interaction with ...
Semantic Scholar extracted view of "Deep Learning Based Teeth Segmentation" by Husam Al-Behadili et al. ... Semantic Scholar's Logo. Search 220,731,149 papers from all fields of science. Search. Sign In Create Free Account. DOI: 10.18280/ria.380411; ... AI-powered research tool for scientific literature, based at Ai2. Learn More. About
Furthermore, we note that GPT3.5 and GPT4 are the only language models examined in this paper that were trained with HF, specifically reinforcement learning from human feedback 103. When it is ...
In order to do that, the IS group helps organizations to: (i) understand the business needs and value propositions and accordingly design the required business and information system architecture; (ii) design, implement, and improve the operational processes and supporting (information) systems that address the business need, and (iii) use advanced data analytics methods and techniques to ...
Higher education institutions have consistently strived to provide students with top-notch education. To achieve better outcomes, machine learning (ML) algorithms greatly simplify the prediction process. ML can be utilized by academicians to obtain insight into student data and mine data for forecasting the performance. In this paper, the authors proposed an ML-based student prediction model ...