Click through the PLOS taxonomy to find articles in your field.
For more information about PLOS Subject Areas, click here .
Loading metrics
Open Access
Peer-reviewed
Research Article
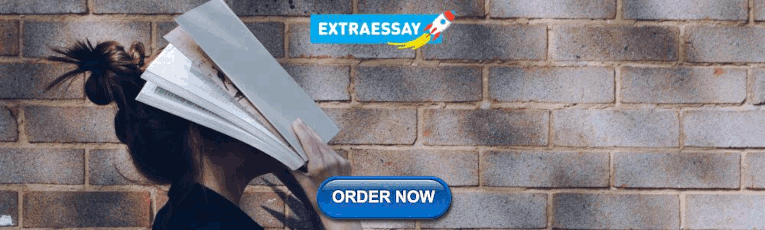
Adoption and income effects of new agricultural technology on family farms in China
Roles Conceptualization, Data curation, Formal analysis, Investigation, Methodology, Software, Validation, Writing – original draft, Writing – review & editing
* E-mail: [email protected]
Affiliation Economics and Management School, Wuhan University, Wuhan, China

- Published: April 26, 2022
- https://doi.org/10.1371/journal.pone.0267101
- Reader Comments
Family farms are the main force that promotes the direct application of new agricultural technology to production. So what are the factors that affect the adoption of new agricultural technology by family farms, and can the adoption of new technology increase the operating income of family farms? Using cross-sectional data from 847 family farms, this study examines the determinants and impacts of multiple new agricultural technologies adoption on family farms’ income in China. To account for selection bias from both observable and unobservable factors, an endogenous switching regression model is employed to evaluate the effects of new agricultural technology on family farms’ income. The empirical results show that the adoption of new agricultural technology is affected by the endowment of farmers and the characteristics of family farms. After controlling for the selection bias, the adoption of new agricultural technologies has a positive and significant impact on family farms’ income. And the impact on the non-adopter family farms is much larger than adopter family farms. Heterogeneity analysis indicates that family farms with a larger area of arable land earn more from the adoption of new technologies than small farms. In all types of technology investigated, the new methods of pest control and the new chemical fertilizer technology have a relatively large impact on family farms’ income, while the new mechanical technology has the least impact on family farms’ income. The adoption of new technologies by family farms is more important for promoting the progress of agricultural science and technology. Therefore, it is necessary to take effective measures to overcome the obstacles to the adoption of new agricultural technologies and pay more attention to the use of new agricultural technologies to improve agricultural production efficiency.
Citation: Wu F (2022) Adoption and income effects of new agricultural technology on family farms in China. PLoS ONE 17(4): e0267101. https://doi.org/10.1371/journal.pone.0267101
Editor: Mario Licata, University of Palermo, ITALY
Received: December 4, 2021; Accepted: April 1, 2022; Published: April 26, 2022
Copyright: © 2022 Fang Wu. This is an open access article distributed under the terms of the Creative Commons Attribution License , which permits unrestricted use, distribution, and reproduction in any medium, provided the original author and source are credited.
Data Availability: All relevant data are within the manuscript and its Supporting Information files.
Funding: Funding: This research was funded by The major project of the key Research Base of Humanities and Social Sciences of the Ministry of Education(No.18JJD790012); The Youth Fund Project of the Humanities and Social Sciences of the Ministry of Education (No.20YJC790197). Author Contributions: Conceptualization, F.W.; data curation, F.W.; formal analysis, F.W.andM.Z.;investigation,F.W.andM.Z.;methodology,F.W.;supervision,M.Z.;validation,F.W.and M.Z.; writing—original draft, F.W; writing—review and editing, F.W.; M.Z. All authors have read and agreed to the published version of the manuscript.
Competing interests: The authors declare no conflict of interest.
Introduction
The Chinese government has been committed to cultivating large-scale agricultural operators, ensuring the safety of grain and agricultural industries and promoting the development of modern agriculture. As the most effective form of the new type of agricultural business, family farms( jiating nongchang ) have pointed out the direction for the construction of Chinese modern agricultural business system [ 1 ]. Family farms are large farms worked and managed by families which have expanded significantly through state-brokered leasing of land. The government encourages the circulation of large tracts of farmland to families who devote themselves to commercial farming [ 2 ]. In recent years, family farms have developed rapidly due to the intensive promulgation of policies for fostering new agricultural business entities. Up to the end of 2018, the number of family farms is over 600,000, which is 4 times the number compared to 2013.
At the same time, many practical problems in production continue to emerge and urgently need to be resolved. The application of agricultural science and technology is a practical problem that needs to be studied and solved urgently. Science and technology is an important engine of agricultural revitalization, and family farms are the main body of a new type of agricultural production, which is at the core of the revitalization of rural industries [ 3 ]. As a consequence, it is necessary to study which factors affect the adoption behavior of agricultural technology in family farms. Farmers’ decisions on technology adoption, including their assessment of the income effect after adoption. However, most of the studies do not further examine the outcome variables of adoption behavior in the current studies on technology adoption, that is, the income effect that farmers consider when adopting. However, for farmers’ adoption decisions, technology adoption incentives and income effects are two inseparable aspects [ 4 ].
To sum up, as a producer, the family farm represents the main direction of China’s current and future advanced agricultural productivity, and is the main force to promote the direct application of new agricultural technologies to production. Family farms have both the level of demand for new technologies and the ability to adopt new technologies. It is much larger than small households. Therefore, family farms are more willing to adopt new agricultural technologies and obtain greater benefits from the adoption of new technologies [ 5 ]. However, whether the theoretical assumptions are consistent with the facts, that is, whether the adoption of new technologies can benefit family farms by bringing higher incomes, empirical tests are needed. To this end, this article uses the survey data of 847 family farms in the planting industry in my country, and uses the endogenous transformation model to explore the influencing factors of the adoption of a variety of new agricultural technologies and their impact on family farm income, and conduct robustness testing and heterogeneity analysis. Finally, it proposes countermeasures and suggestions to promote family farms to adopt new agricultural technologies.
Literature review
With the rise and development of family farms, many literatures have conducted empirical studies on the factors affecting the adoption of new technologies in family farms. For example, relying on a field survey data from 676 family farms in Huang-huai-hai Plain, Gao et al (2017) used bivariate probit and regression linear models to explore the influence factors of the adoption of green control techniques (GCTs) by family farms. The study found that the perceived ease of use and usefulness of technology, the number of family labors, the degree of promotion in agricultural technology sector, and the education and risk preference of farmers have a significant positive impact on the willingness to adopt [ 6 ]. Ni and Liu (2019) studied the influencing factors of family farms’ application behavior of internet agricultural technology by using a survey data of 270 family farms in 9 cities in Jiangsu Province. The results show that the expected benefits, the scale of the network and online learning have a significant positive effect on the application of Internet agricultural technology by farmers [ 7 ]. Based on 2017 survey data of family farms in Heilongjiang, Jiangsu and Sichuan provinces, Xia et al (2019) found that family farmers with longer education and training are more likely to apply green production technology. However, it is not that the more time of training, the better; young, risk-preferred farmers have a higher probability of applying green production technology. Women and farmers who are accustomed to using mobile networks have a higher probability of applying green production technology [ 8 ]. Besides, Cao et al. (2020)used the ordered logistic model to explore the effects of human capital and social capital on soil testing and fertilization behavior The result shows that, overall, human capital and social capital have positive impact on the technology application of family farms [ 9 ].To sum up, although different scholars choose different explanatory variables, the adoption behavior of family farm’s agricultural technology is generally affected by farmers’ individual characteristics, family farm’s resource endowment, external production environment and other factors.
Scholars at home and abroad have also carried out a lot of research on the farmer’s income effect of agricultural technology. For example, Ma and Abdulai (2019) took 481 apple growers in China as an example. The results of the study show that the adoption of IPM technology can significantly increase apple production, net income and agricultural income [ 10 ]. Relying on 822 micro survey data of rice growers in Hubei, Jiangxi and Zhejiang provinces in the Yangtze Basin, Huang and Luo (2020) used the endogenous switching regression (ESR) to explore the cost-saving and income-increasing effect of green control techniques. The results show that: green control techniques have achieved the goal of cost-saving and income-increasing effect for rice growers, but the cost-income improvement is not large [ 11 ]. Using an endogenous switching regression model and a multinomial treatment effects mode, Gao et al (2019) evaluated the farmer’s income effect of agricultural technology, the result show that the adoption of green control techniques has a significant impact on the increase of farmer’s agricultural income [ 12 ]. Based on the micro survey data of farmers in the main apple producing areas of Shaanxi, Gansu and Henan, Hou et al (2019) employed the ESR model to examine the farmer’s agricultural income effect of technology. The results show that the soil testing formula fertilization technology helped farmers increased their average annual agricultural income by 8% [ 13 ]. Moreover, Issahaku and Abdulai (2020) used farm data from three agro-ecological regions in Ghana to examine the impact of crop selection and soil and water conservation on farm performance. The empirical result shows that farmers’ choice of crop selection and soil and water conservation is beneficial to improve crop income [ 14 ]. Tufa et al (2019) assessed the productivity and income impacts of the adoption of ISVAPs (improved soybean varieties and agronomic practices) using plot data collected from 1237 soybean farmers in Malawi. The result of the ESR model shows that the adoption of ISVAPs is related to an average 61 per cent increase in production and 53 per cent increase in income among adopters [ 15 ]. Seen from the above articl e s, most of the studies believe that agricultural technology adoption is beneficial to increase the household income of farmers.
The contribution of this paper to the empirical literature is threefold. Firstly, the research object of the existing literature is mainly limited to small farmers, rarely related to family farms. Small farmers have few plots, small plots, insensitive to the choice and use of technology. They are even unwilling to choose the latest technology. Different from small farmers, family farms specialize in agricultural production, with larger operation scale, stronger economic strength, and stronger willingness and ability to adopt new agricultural technologies. In the past decade, policies have been implemented to encourage the development of family farms in China. Up to the end of 2018, the number of registered family farms is 4 times the number compared to 2013.However, there are not many studies on the benefits of family farms from the adoption of new technologies, which may be due to the lack of available data.
In addition, the existing literature basically only analyzes the adoption behavior and income effects of one or two specific agricultural technologies in agricultural production, such as IPM technology [ 10 ], green control technology [ 11 , 12 ], green production technology [ 13 ], soil and water conservation technology [ 14 ], ISVAPs [ 15 ], water-saving irrigation technology [ 16 ], etc. Li and Kong (2010) believe that, from the perspective of the relationship between technologies, all kinds of technologies are not independent of each other, but influence and interact with each other. Only by adopting a series of related technologies at the same time can we give full play to the potential of various technologies and obtain the benefits of technological changes [ 17 ]. Different from existing research, this article discusses how a variety of new agricultural technologies, such as new varieties, new machinery, new fertilizers, new pesticides, new methods of pest control, new production and new management methods affect the income of family farms.
Secondly, whether a farm adopts new technology is affected by its own endowment, family characteristics and even the external environment, so it is not generated randomly, but a kind of "self-selection" behavior, and the problem of sample self-selection may lead to deviations in the results. Propensity Score Matching(PSM) method was used to eliminate the selectivity bias in the existing literature [ 18 – 20 ]. However, the PSM method solves the problem of selection bias according to observable factors. When there are unobservable factors (such as the innate ability of farmers) that affect the decision-making of new technology adoption on family farms at the same time, the PSM method may still lead to estimation bias [ 10 ]. Hence, this paper will use the endogenous switching regression (ESR) model proposed by Lokshin and sajaia (2004) [ 21 ] to make up for the defects of existing research methods. The ESR model considers the sample selection bias caused by observable and unobservable variables, which makes up for the deficiency of PSM method. In addition, the ESR model fits the income determination equations of new technology adopters and non-adopters respectively, and combines with counterfactual inference analysis, which can break through the unreasonable hypothesis that the treatment effect of two groups of family farms is homogeneous in the treatment effect model (TEM).
Thirdly, the existing literature studies on the income-increasing effect of the use of agricultural technology are either based on the macro data at the national or provincial level, or on the survey data of ordinary peasant households. However, this paper uses the micro-survey data of family farms to investigate the income-increasing effects of the new agricultural operators of family farms. It jointly adopts a variety of new agricultural technologies. The data obtained directly from the interview of family farmers are more reliable, and the sample size is relatively large. As a consequence, the conclusion should be more reliable.
The rest of this paper is organized as follows: Section 2 introduces the research method, data source and main explained and explanatory variables; Section 3 presents and discusses the empirical results; Section 3 provides the results of the robustness test and the expansive study; and the last section draws conclusions and implications for policy.
Research method
Endogenous switching regression model(esr).
In the formula ( 2 ), Y i is the indicator of family farm management performance, such as agricultural total income and per capita income; X i is the vector of individual, family and farm characteristics (such as age, education, family size, farm size, etc.) that may affect farm performance indicators; A i is whether the farmer i adopts new technology variable; ε i is a random disturbance term. In view of the fact that family farmers choose whether to use new agricultural technology or not according to their own conditions, the decision-making ( A i ) of new technology adoption may be affected by some unobservable factors, which are related to the result variable ( Y i ). It leads to the correlation between A i and ε i agriculture in formula ( 1 ). As a consequence, the direct estimation Eq ( 2 ) may lead to estimation errors due to the problem of sample self-selection.
As mentioned before, the ESR model has certain advantages in solving the problem of endogenity and comprehensively considering observable and unobservable factors, and can effectively avoid the "invisible bias" caused by unobservable factors. Therefore, we use the ESR model to examine the impact of new agricultural technology adoption on family farm operating income.
The ESR model is divided into two stages. The first stage is to use the Probit or Logit model to estimate whether the family farm adopts the new agricultural technology selection equation, as shown in Eq ( 1 ); the second stage is to establish the family farm’s operating income determination equation and estimate changes in operating income caused by the adoption of new agricultural technologies by family farms.
Treatment effect estimation based on ESR model
By comparing the expected value of agricultural operating income of family farms that adopt new agricultural technologies and family farms that do not adopt new agricultural technologies under real scenarios and counterfactual scenarios, we can estimate the average processing effect of family farms adopting new agricultural technology decisions.
Data source
The data in this paper come from the three-round Family Farm Survey during 2017~2019 conducted by the Family Farm Research Group of the Economic Development Research Center of Wuhan University. The first round was carried out in 2017 in Wuhan City, Hubei Province and Langxi County, Anhui Province. The second round, fielded in 2018, took place in 20 provinces (districts, municipalities) across China. The third round was carried out in 2019 in Wuxue City and Macheng City, Hubei Province. The subjects of the three-round survey are all local family farmers engaged in agricultural production. The rosters of family farmers were provided in advance by the local agricultural management departments such as the Bureau of Agriculture. Then we conducted a random sampling survey based on the roster. The three-round survey employs the same questionnaire through face-to-face interview. A total sample of 1,373 family farms is obtained. After excluding the missing values and outlier, we obtain the data with 1,324 observations, 847 of which are planting-based. The questionnaire includes questions about the basic information of the farmer’s family, land circulation and utilization, fixed assets and investment, labor and wage, income and expenditure of production and operation, new technology demand and application, natural and market risks, cooperative membership and leading enterprises, finance and insurance, and other related issues. We focus on 847 planting family farms in this paper.
In the survey sample of family farms ( Table 1 ), 69.54% of the family farm operators are aged 36–55; the majority of family farm operators have an education level of junior high school, accounting for 44.98%; the number of family members is mostly 3–4 people, accounting for 58.56%; family farms with an operating scale of more than 50 mu(3.335 hectare), accounted for 83.59%; family farms with operating years of 20 to 30 years accounted for the largest proportion, accounting for 41.32%; family farms with an operating income of less than 500,000 yuan($78,950),accounted for the vast majority, accounting for 61.63%.
- PPT PowerPoint slide
- PNG larger image
- TIFF original image
https://doi.org/10.1371/journal.pone.0267101.t001
Variables and descriptive statistics
Explained variables..
The explained variables are the total operating income and the per capita operating income of family farms. The total operating income of family farms is based on the question in the questionnaire: “How many tens of thousands of yuan was the total operating income of your family farm last year?” In addition, there are great differences in the number of workers involved in agricultural production on family farms(Some farms have only one person engaged in agricultural production, and some even as many as nine people engaged in agricultural production.). As a consequence, it is not enough to take the total income of the family farm as the explanatory variable. We also take the per capita income of the family farm labor force as the explanatory variable. Per capita income is a better reflection of the labor productivity of a family farm. The per capita operating income of the family farm is obtained by dividing the total operating income of the family farm by the number of own labor. In order to reduce the impact of outliers and heteroscedasticity, this paper has carried out a logarithmic treatment on family farms’ operating income.
Core explanatory variable.
The core explanatory variable in the study is a dummy variable that equals one if the family farm adopts any new technology, and equals zero if not. This variable is based on the question in the questionnaire: "Have you used the following new technologies in the past year?" (you can choose more) ", the alternative options are" 1. New varieties(new agricultural varieties such as rice, wheat, corn, rape and soybean); 2. New machinery (agricultural machines such as rice transplanter, planter, harvester, dryer, agricultural tractor); 3. New chemical fertilizers; 4. New pesticides; 5. New pest control methods; 6. New production methods (e.g. new crop cultivation methods, new fertilization formulations, new pesticide spraying methods, new irrigation methods, etc.);7. New management methods (e.g. new field management, new operating methods, new cost control methods, etc.); 8. Did not use any new technology. If the farmer chooses 8, it is considered that the farm does not adopt new technology, with a value of 0. Otherwise, it is considered that new technology is adopted, with a value of 1.
Table 2 shows the adoption of new technology types in family farms.
https://doi.org/10.1371/journal.pone.0267101.t002
As can be seen from Table 3 , the proportion of sample family farms using two new technologies is the largest, accounting for 22.67%, followed by one and three, accounting for 19.01% and 15.70%, respectively. Family farms using five or more new technologies account for relatively few.
https://doi.org/10.1371/journal.pone.0267101.t003
Control variables.
Similar to earlier studies [ 10 – 15 ], we employ three types of control variables, which are personal characteristics of head of family farm, farm operation characteristics and external environment characteristics. The personal characteristics of head of family farm include age, education, years of farming, past career and technology training experience. The characteristics of farm operation include family labors, farm size, contract farming, internet use and whether it is a model family farm. The external environment characteristics include the availability of loans, cooperative membership, and government extensions. In addition, the effects of different years and regions on the adoption of new technologies and operating income of family farms are taken into account in the estimation of the model.
Instrumental variable.
Similar to earlier studies [ 10 , 15 , 19 , 23 ], "Whether the farmer can obtain information about new agricultural technologies from television, radio, newspapers, magazines and other channels" was selected as an instrumental variable. For the validity check of this instrument, we have run simple probit model for selection equation and OLS regression for outcome equations separately and we have checked that this variable is significant when included in the adoption of new technology selection equation but not significant when included in the outcome equations [ 10 ]. Then, following Di Falco et al. (2011) and Kumar et al. (2018), we conduct further falsification test [ 23 , 24 ]. The results show that the selected IV has a significant positive impact on the decision of family farms to adopt new technology but has no impact on the operating income of family farms that do not adopt new technology (see Appendix Table A.I ). The above results together show that this IV is indeed an effective instrumental variable.
The definition and descriptive statistics of variables used in empirical analysis are shown in Table 4 .
https://doi.org/10.1371/journal.pone.0267101.t004
The descriptive statistics provided in Table 5 show that there are systematic differences between adopters and non-adopters of new technologies in terms of observable characteristics at the individual, family and farm levels. In particular, at a given level of significance, the operating income of family farms using new agricultural technologies is also higher. For example, the average annual operating income of family farms using new technologies is 1.06 million yuan ($158,679). The average annual operating income of family farms without new technologies is 0.79 million yuan($117,846), with a difference of 0.27 million yuan($40,844). However, given that the adoption of new technology is the result of "self-choice" of family farms, the differences in agricultural operating income between adopters and non-adopters of new technologies listed in Table 5 are not sufficient to explain the causal relationship between the adoption of new technologies and family farms’ income. As a consequence, this paper uses the ESR model to assess the impact of new technology adoption behavior on family farms’ income. It can correct selection biases and endogenesis from observed and unobserved heterogeneity.
https://doi.org/10.1371/journal.pone.0267101.t005
Empirical results and analysis
The selection and outcome models.
As we can see from Table 6 , it shows the results of the ESR model estimation of the impact of the adoption of new technologies on the operating income indicators of family farms. The lower half of Table 6 shows that the estimated values of the correlation coefficient rho_1/rho_2 in the model are significantly non-zero, indicating that there is a sample selection bias caused by unobservable factors. In particular, the negative correlation coefficient of rho_1/rho_2 indicates the negative selection deviation. This shows that family farms with lower total operating income and per capita operating income are more likely to adopt new agricultural technologies. If this negative selection bias cannot be explained, the impact of the adoption of new technologies on family farms’ income will be underestimated. The LR test values of equation independence reject the original hypothesis that the selection equation and the result equation are independent of each other at the statistical level of 1%. The goodness-of-fit test of the model is significant at 1% level. As a consequence, the ESR model used in this paper is appropriate.
https://doi.org/10.1371/journal.pone.0267101.t006
The results of the first stage estimation of the ESR model show the determinants of the adoption of new technologies in family farms. The results in Table 6 show that the age of the household head is negative and significant at the 5% statistical level, which indicates that the older the farmer, the less likely it is to adopt new agricultural technologies. This is because the older farmers are, the more likely they are to be influenced by traditional habits and tend to be more resistant to new technologies. Family farmers who have training experience are significantly more likely to adopt new technologies. This is because training experience as a way of education, by imparting experience to farmers, let farmers understand and master the use and economic value of new technology, and promote the adoption of new technology by farmers. Family farms invest a lot in the initial stage of construction and often lack their own funds. However, the use of some new technologies requires greater investment, so there is a greater demand for funds. The empirical results show that the status of fund borrowing is significantly positive at the 5% level, indicating that the availability of borrowing has a significant positive impact on the adoption of new technologies by family farms, which is consistent with the research conclusions of Gao et al. (2017,2019) [ 6 , 12 ]. The coefficient of cooperative membership is positive and significant at the 1% level, which indicates that family farms participating in the cooperative are more likely to adopt new agricultural technologies. This is because farmers’ cooperatives can reduce the high-risk and high-cost dilemmas faced by members in using the elements of new technologies and promote the adoption of new technologies [ 10 , 25 ]. Accessing information online has a significant positive impact (at the 5% statistical level) on the adoption of new technologies by family farms. The using of Internet can alleviate the information constraints of family farmers and improve their ability to obtain information. Information flow can improve farmers’ understanding and mastery of technological information and promote farmers to adopt new technologies [ 26 ]. Signing purchase and sales contracts with companies has a significant positive impact(at the 1% statistical level) on farmers’ willingness to adopt new technologies. That is because contract agriculture provides farmers with services such as credit purchase of means of production, credit collateral, technical guidance and training, and information provision. It can help farmers reduce credit constraints and transaction costs for adopting new technologies [ 20 ]. The coefficient of government extension services is positive and significant at the 5% level, indicating that government extension services have a significant role in promoting the adoption of new technologies by family farms The promotion and publicity of new technology by government departments can effectively improve farmers’ awareness of new technology. Besides, technology subsidies provided by the government to farmers who adopt new technologies can also increase their enthusiasm for adopting new technologies [ 27 ]. Finally, the coefficient of the instrumental variable "Access to information" is positive and significant at the 1% level. This indicates that the availability of new technology information has a significant impact on family farms’ decision to adopt new agricultural technologies. Some studies have pointed out that the wider the channels for farmers to obtain technical information, the more adequate the relevant technology information will be, the greater the possibility of using relevant technologies [ 6 , 12 ].
The results of the second stage estimation of the ESR model show the determinants of family farm operating income. As can be seen from the result equation in Table 6 , there are significant differences in coefficient estimates between the two types of family farms with and without new technology in some variables. For example, the effect of education level is significantly positive at the 1% level, which shows that with the improvement of farmers’ human capital, it is helpful to promote the increase of their operating income. The impact of family labors on farm economic benefits is significantly positive at the 1% level. Generally speaking, family farms with more self-owned labor force are more willing to invest more labor force in the process of agricultural production so as to obtain higher land income. However, too many farmers in the family will reduce the per capita operating income of the farm. From the estimated results in Table 6 , it can be seen that regardless of whether new agricultural technologies are adopted, the coefficient of farm scale is positive and significant at the 1% level. This shows that relatively large-scale farms are more likely to drive farms to achieve economic benefits. Loans and contract farming have a significant positive impact (at the statistical levels of 5% and 1%, respectively) on the operating income of family farms that do not adopt new technology. The former is because the difficulty of financing and the shortage of production funds are the outstanding problems faced by family farms. However, farmers can ease the liquidity constraints in the construction of agricultural infrastructure, the purchase of machinery and equipment, and the application of advanced agricultural technology through loans, which can increase production investment and promote income growth. The latter is because when agricultural leading enterprises sign contracts with family farms for the purchase and sale of agricultural products, they usually set a series of quality standards and provide family farms with high-quality inputs and corresponding production technologies, thus stabilizing and improving product quality and output, as well as raising the production technical efficiency and income level of family farms [ 28 ]. Finally, it can be seen from Table 6 that regardless of whether the new agricultural technology is adopted or not, the coefficient of the model family farm is significantly positive at the level of 1%, indicating that obtaining the model certification has a significant and positive impact on the income(both total income and per capita income) of the family farm This is because family farms that have been certified for demonstration have strong standard demonstration significance and economic driving capabilities in terms of basic equipment, production and operation, production efficiency, and demonstration driving, which can significantly promote family farms to quickly improve economic benefits and promote families rapid increase in farm value and efficiency [ 18 ].
Estimating treatment effects
The following further compares the expected income of the family farm (a) with the new technology and the family farm(b) without the new technology. In addition, we intend to compare the family farms with new technology if they do not adopt (c) and the family farms without new technology if they adopt (d), namely the expected income under the actual situation and the counterfactual conditions. The results are shown in Table 7 . We take the average treatment effect of the impact of new technologies on the total income of family farms as an example (per capita income can be similarly explained as a result variable). The expected income of the family farm that actually adopts the new technology is 3.809. The expected income of the family farm that does not adopt the new technology is 3.573 under the counterfactual condition. The difference between the two reflects the average treatment effect of the new technology, that is, the income-increasing effect brought by the use of the new technology. The average treatment effect (ATT) is 0.236, indicating that farms with new technologies will reduce their expected income by 6.20%(The calculation formula is:(3.573–3.809)/3.809*100%). if they are not adopted. Similarly, if farms that do not actually adopt new technologies (b and d) adopt new technologies, the average treatment effect (ATU) is 1.533, indicating that expected income will increase by 44.85%(The calculation formula is:(4.951–3.418)/3.418*100%). As a result, adoption of new farm technologies has a positive effect on the family farms’ income, which is significant at the 1% statistical level. It can also be seen from Table 7 that family farms that do not actually adopt new technologies will have a more significant increase in income if they adopt new technologies (ATU > ATT). As a consequence, a basic policy implication is that family farms that do not adopt new technologies should be encouraged to adopt new technologies at present.
https://doi.org/10.1371/journal.pone.0267101.t007
Robustness test and heterogeneous effects
Robustness test.
In order to solve the endogenous problem between the adoption of new agricultural technology and the income from family farm operations, the article further uses the endogenous treatment effect model (ETEM) for quantitative analysis as a robustness test [ 10 ]. The model can estimate two equations at the same time: the selection Eq ( 1 ) and the outcome Eq ( 2 ). The former examines the factors influencing if the farm chooses to adopt new technologies; the latter analyzes the influence of whether to adopt new technologies and other factors on farm operating income. The ETEM estimation results reflect the marginal impact of never adopting new technologies into adopting new technologies on the income of family farms.
The results estimated by the ETEM model show that the adoption of new technology has a significant positive effect on farm agricultural operating income and per capita operating income (see Table 8 ) after controlling other variables. It indicates that the adoption of new technology can indeed increase family farm operating income. Except that the instrumental variable "Access to information" has a significant positive effect on the adoption of new technology on farms, training experience, internet use, loans, cooperative membership, contract farming, and government extensions have also passed the significance test in the selection equation. Age and education level have significant negative effects on the adoption of new technology on farms. The reasons have been explained earlier, so we will not repeat them. Other variables such as Past career, the number of family farmers, the number of years of farming, the size of the farm, whether it is a demonstration farm or not have no significant impact on the adoption of new technology on the farm. In the result equation, there is an obvious negative relationship between age and farm economic benefits. The number of family farmers, the size of the farm, whether to sign the contract for the purchase and sale of agricultural products, and whether it is a model farm have a significant positive impact on farm income. Similar to the regression results of the ESR model above, from the regression results of these variables, we can make practical policy recommendations for promoting the adoption of new technologies for family farms.
https://doi.org/10.1371/journal.pone.0267101.t008
Heterogeneous effects
Different farm sizes..
Family farm is a new type of management body that the central government focuses on encouraging and supporting development. Family farm will become the main management body of agriculture in our country and the main force in the demand and adoption of new agricultural technology. From the perspective of expected income, compared with traditional small farmers, the operating scale of family farms is ten times or even hundreds of times larger than that of small farmers. As a consequence, the potential income of new technologies is much higher than that of small farmers. However, this paper is more concerned about whether the larger the scale of operation, the greater the potential benefits of the adoption of new technology for family farms that have achieved moderate scale operation. We first calculate the mean value of the cultivated land area variable of family farm management in order to investigate whether there is a difference in the impact of the adoption of new agricultural technology on family farm income under the comparison of larger and smaller business scale. So, the samples are divided into two sample groups: "greater than the average" and "less than the average" for comparative analysis. Table 9 the results of ESR model estimation show that after controlling other variables, the adoption of new agricultural technology has a significant positive effect on the total income of family farm. The impact of the adoption of new agricultural technology on family farms’ income is 2.757 in the "greater than the average" sample group and only 0.227 in the "less than the average" sample group. This shows that the larger the operating area of land, the greater the income-increasing effect brought about by the adoption of new technology on family farms.
https://doi.org/10.1371/journal.pone.0267101.t009
Different types of new technologies.
We make a group analysis according to the type of agricultural technology in order to investigate whether there are differences in the impact of different agricultural technology adoption on family farm income. From the estimated results in Table 10 , compared with other technical types, the income-increasing effect of the new methods of pest control and new chemical fertilizer technology is relatively more obvious. On the one hand, the new method of pest control can reduce the yield loss of agricultural products and bring positive benefits to family farms when the output value spills over the price of pesticides. On the other hand, the demand for high-quality, safe and hygienic edible agricultural products is increasing day by day with the rapid development of economy and the continuous improvement of people’s living standards.
https://doi.org/10.1371/journal.pone.0267101.t010
The adoption of new methods of pest control will weaken the impact on the quality and safety of agricultural products caused by excessive use of pesticides and then affect the price, thus reducing the negative impact of excessive use of pesticides on the income of family farms. In addition, the adoption of new methods of pest control can also save the use of agricultural labor, reduce the labor input cost of family farms, and thus increase the income of family farms. As for the adoption of new chemical fertilizer technologies, such as the use of organic fertilizers can not only provide nutrition for crops, but also increase and renew soil organic matter. In addition, it can promote microbial reproduction, and improve soil properties and biological activities, thereby increasing soil fertility and fertilizer use efficiency. It helps to increase crop yields and improve the quality of agricultural products, thereby increasing the income of family farms.
The adoption of new pesticides and new varieties of technology have a great impact on the income of family farms. The promotion and application of high-efficiency, low-toxicity, safe and high-quality pesticides in a large area has promoted a bumper agricultural harvest. New varieties can enrich the variety of agricultural products. In addition, the diversification of agricultural products makes family farms have more production options, thus increasing new income channels of agricultural production. New production methods, new management methods are significantly positive at 1% statistical level. It indicates that new production methods and new management methods can promote family farms to increase production and income. The new mechanical technology has the least impact on the income of family farms, which may be related to the low degree of mechanization and limited role of family farms in the survey area.
Conclusion and policy implications
China agriculture is still dominated by small-scale farmers, but their demand for new technologies is insufficient. Family farms of scale operators are mainly engaged in agricultural production and are far more willing to adopt new technologies than smallholder farmers. Relying on cross-sectional data from 847 family farms, this study examines the determinants of adoption of multiple new agricultural technologies and its impact on family farms’ income in China. The empirical results show that agricultural technical training, loan availability, internet use, participation in cooperatives, contract farming, government extension services and access to new technology information are main factors that determine farmers’ decision to adopt new agricultural technology, and new agricultural technology adoption has a positive and statistically significant impact on family farms’ income. Under the counterfactual assumption, if the family farms that actually adopt the new technology do not adopt the new technology, the total income of family farms will decrease by 6.20%. If the farms that do not adopt the new technology adopt the new technology, the total agricultural operating income will increase by 44.85%. In addition, the robustness test from the ETEM model also supports the above conclusions. The heterogeneity study found that, the larger the land management area is, the greater the income increase effect brought by the use of new technology is. And the income increase effect of using new methods of pest control and new chemical fertilizer technology is relatively more obvious.
The empirical results of this paper support the view that increasing the adoption of new agricultural technologies will help to improve the economic benefits of family farms. As a consequence, it is necessary to take a variety of effective measures to support family farms to carry out the application of new agricultural technologies and to promote family farms to increase production and income: (1) When researching and developing new agricultural technologies, it is necessary to have a deep understanding of the needs of agricultural producers, so as to match the new technologies developed with their needs and improve the realization of new technologies. (2) By establishing a family farms’ agricultural new technology demand feedback and service evaluation mechanism, forming a farmer’s demand-oriented agricultural new technology service system, rationally providing the agricultural new technology required by farmers, will reduce the supply cost of agricultural new technology services and increase the adoption rate of new technology.(3)Encourage family farms to join agricultural cooperatives, and use cooperatives as a platform and mechanism for regular exchanges between farmers. Through technical guidance and experience exchange, new agricultural technologies can be more smoothly promoted among family farms adjacent to geographical space. (3) The government should expand the service functions of township agricultural technology extension organizations. In addition to teaching new technologies to farmers, the agricultural technology department should also organize farmers to observe and learn from family farms that have adopted new technologies in nearby towns, build farmers’ new technology information exchange platform, and improve the adoption rate of new technologies in a wider geographical range. (4) Carefully select and cultivate new technology promotion demonstration households. Farmers with younger age and higher education level are more willing to adopt new technologies. Agricultural technology extension agencies should select young farmers with strong technology acceptance ability as model households for new technology adoption, in the whole process of new technology application. Give vigorous support and help to improve the success rate of new technology adoption by model households, and promote new agricultural technologies through demonstration effects.
Different from existing research, this article discusses how a variety of new agricultural technologies affect the income of family farms. This is because, in reality, farmers rarely use one agricultural technology, but use a combination of agricultural technologies. We provide supportive evidence to the causal relationship between new agricultural technologies’ adoptoin and family farms’ income. We believe that our research provides an important case study and empirical result to the previous research. Our analysis is based on cross-sectional data collected from 847 family farms in China. Due to data limitations, we are unable to capture the dynamic effects of multiple new agricultural technologies adoption on farm performance. However, we believe this is a promising area to investigate in the future when the required panel data are available.
https://doi.org/10.1371/journal.pone.0267101.t011
Supporting information
https://doi.org/10.1371/journal.pone.0267101.s001
Acknowledgments
Thanks to the Family Farm Research Team at the Economic Development Research Center of Wuhan University. Thanks to all the participants who helped us fill out the questionnaire. Special thanks to the reviewers for their valuable comments.
- View Article
- Google Scholar
To read this content please select one of the options below:
Please note you do not have access to teaching notes, digital technology in agriculture: a review of issues, applications and methodologies.
China Agricultural Economic Review
ISSN : 1756-137X
Article publication date: 27 June 2022
Issue publication date: 23 January 2023
The purpose of this paper is to show the impact of digital agriculture on food supply chain, research trend, emphasis and implications for future research.
Design/methodology/approach
The paper analyzes how the digital technology reshapes the production, assembly, transaction, retail and logistics. Impact from each main technological progress is discussed.
First, digital agriculture develops quickly and changes all parts of the food supply chain. Second, while many technological progresses show their impacts in agriculture and food sector, e-commerce and progress of artificial intelligence show its comprehensive impact on the argi-food sector.
Originality/value
The paper shows the technological trend and progress in food and agriculture sector. Researchers focusing on agricultural economics and agribusiness should pay attention to recent developments in the real world, know the recent developments from other disciplines, get more data for empirical research and show the impact of digital agriculture on consumer's preference and social welfare.
- Remote sensing
- Food delivery
- Internet of Things
Acknowledgements
The authors thank the editor and reviewer for excellent comments and guidance. Both Du and Hatzenbuehler thank the Idaho Agricultural Experiment Station for support.
Du, X. , Wang, X. and Hatzenbuehler, P. (2023), "Digital technology in agriculture: a review of issues, applications and methodologies", China Agricultural Economic Review , Vol. 15 No. 1, pp. 95-108. https://doi.org/10.1108/CAER-01-2022-0009
Emerald Publishing Limited
Copyright © 2022, Emerald Publishing Limited
Related articles
We’re listening — tell us what you think, something didn’t work….
Report bugs here
All feedback is valuable
Please share your general feedback
Join us on our journey
Platform update page.
Visit emeraldpublishing.com/platformupdate to discover the latest news and updates
Questions & More Information
Answers to the most commonly asked questions here
The Future of Digital Agriculture: Technologies and Opportunities
Ieee account.
- Change Username/Password
- Update Address
Purchase Details
- Payment Options
- Order History
- View Purchased Documents
Profile Information
- Communications Preferences
- Profession and Education
- Technical Interests
- US & Canada: +1 800 678 4333
- Worldwide: +1 732 981 0060
- Contact & Support
- About IEEE Xplore
- Accessibility
- Terms of Use
- Nondiscrimination Policy
- Privacy & Opting Out of Cookies
A not-for-profit organization, IEEE is the world's largest technical professional organization dedicated to advancing technology for the benefit of humanity. © Copyright 2024 IEEE - All rights reserved. Use of this web site signifies your agreement to the terms and conditions.
Thank you for visiting nature.com. You are using a browser version with limited support for CSS. To obtain the best experience, we recommend you use a more up to date browser (or turn off compatibility mode in Internet Explorer). In the meantime, to ensure continued support, we are displaying the site without styles and JavaScript.
- View all journals
- Explore content
- About the journal
- Publish with us
- Sign up for alerts
- Published: 27 April 2017
Technology: The Future of Agriculture
- Anthony King
Nature volume 544 , pages S21–S23 ( 2017 ) Cite this article
196k Accesses
213 Citations
224 Altmetric
Metrics details
- Agriculture
A technological revolution in farming led by advances in robotics and sensing technologies looks set to disrupt modern practice.
Over the centuries, as farmers have adopted more technology in their pursuit of greater yields, the belief that 'bigger is better' has come to dominate farming, rendering small-scale operations impractical. But advances in robotics and sensing technologies are threatening to disrupt today's agribusiness model. “There is the potential for intelligent robots to change the economic model of farming so that it becomes feasible to be a small producer again,” says robotics engineer George Kantor at Carnegie Mellon University in Pittsburgh, Pennsylvania.
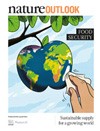
Twenty-first century robotics and sensing technologies have the potential to solve problems as old as farming itself. “I believe, by moving to a robotic agricultural system, we can make crop production significantly more efficient and more sustainable,” says Simon Blackmore, an engineer at Harper Adams University in Newport, UK. In greenhouses devoted to fruit and vegetable production, engineers are exploring automation as a way to reduce costs and boost quality (see ‘ Ripe for the picking ’). Devices to monitor vegetable growth, as well as robotic pickers, are currently being tested. For livestock farmers, sensing technologies can help to manage the health and welfare of their animals (‘ Animal trackers ’). And work is underway to improve monitoring and maintenance of soil quality (‘ Silicon soil saviours ’), and to eliminate pests and disease without resorting to indiscriminate use of agrichemicals (‘ Eliminating enemies ’).
Although some of these technologies are already available, most are at the research stage in labs and spin-off companies. “Big-machinery manufacturers are not putting their money into manufacturing agricultural robots because it goes against their current business models,” says Blackmore. Researchers such as Blackmore and Kantor are part of a growing body of scientists with plans to revolutionize agricultural practice. If they succeed, they'll change how we produce food forever. “We can use technology to double food production,” says Richard Green, agricultural engineer at Harper Adams.
Ripe for the picking
The Netherlands is famed for the efficiency of its fruit- and vegetable-growing greenhouses, but these operations rely on people to pick the produce. “Humans are still better than robots, but there is a lot of effort going into automatic harvesting,” says Eldert van Henten, an agricultural engineer at Wageningen University in the Netherlands, who is working on a sweet-pepper harvester. The challenge is to quickly and precisely identify the pepper and avoid cutting the main stem of the plant. The key lies in fast, precise software. “We are performing deep learning with the machine so it can interpret all the data from a colour camera fast,” says van Henten. “We even feed data from regular street scenes into the neural network to better train it.”
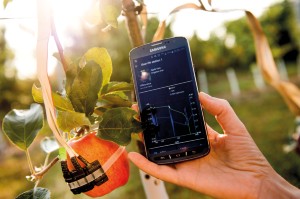
In the United Kingdom, Green has developed a strawberry harvester that he says can pick the fruit faster than humans. It relies on stereoscopic vision with RGB cameras to capture depth, but it is its powerful algorithms that allow it to pick a strawberry every two seconds. People can pick 15 to 20 a minute, Green estimates. “Our partners at the National Physical Laboratory worked on the problem for two years, but had a brainstorm one day and finally cracked it,” says Green, adding that the solution is too commercially sensitive to share. He thinks that supervised groups of robots can step into the shoes of strawberry pickers in around five years. Harper Adams University is considering setting up a spin-off company to commercialize the technology. The big hurdle to commercialization, however, is that food producers demand robots that can pick all kinds of vegetables, says van Henten. The variety of shapes, sizes and colours of tomatoes, for instance, makes picking them a tough challenge, although there is already a robot available to remove unwanted leaves from the plants.
Another key place to look for efficiencies is timing. Picking too early is wasteful because you miss out on growth, but picking too late slashes weeks off the storage time. Precision-farming engineer Manuela Zude-Sasse at the Leibniz Institute for Agricultural Engineering and Bioeconomy in Potsdam, Germany, is attaching sensors to apples to detect their size, and levels of the pigments chlorophyll and anthocyanin. The data are fed into an algorithm to calculate developmental stage, and, when the time is ripe for picking, growers are alerted by smartphone.
So far, Zude-Sasse has put sensors on pears, citrus fruits, peaches, bananas and apples ( pictured ). She is set to start field trials later this year in a commercial tomato greenhouse and an apple orchard. She is also developing a smartphone app for cherry growers. The app will use photographs of cherries taken by growers to calculate growth rate and a quality score.
Growing fresh fruit and vegetables is all about keeping the quality high while minimizing costs. “If you can schedule harvest to optimum fruit development, then you can reap an economic benefit and a quality one,” says Zude-Sasse.
Eliminating enemies
The Food and Agriculture Organization of the United Nations estimates that 20–40% of global crop yields are lost each year to pests and diseases, despite the application of around two-million tonnes of pesticide. Intelligent devices, such as robots and drones, could allow farmers to slash agrichemical use by spotting crop enemies earlier to allow precise chemical application or pest removal, for example. “The market is demanding foods with less herbicide and pesticide, and with greater quality,” says Red Whittaker, a robotics engineer at Carnegie Mellon who designed and patented an automated guidance system for tractors in 1997. “That challenge can be met by robots.”
“We predict drones, mounted with RGB or multispectral cameras, will take off every morning before the farmer gets up, and identify where within the field there is a pest or a problem,” says Green. As well as visible light, these cameras would be able to collect data from the invisible parts of the electromagnetic spectrum that could allow farmers to pinpoint a fungal disease, for example, before it becomes established. Scientists from Carnegie Mellon have begun to test the theory in sorghum ( Sorghum bicolor ), a staple in many parts of Africa and a potential biofuel crop in the United States.
Agribotix, an agriculture data-analysis company in Boulder, Colorado, supplies drones and software that use near-infrared images to map patches of unhealthy vegetation in large fields. Images can also reveal potential causes, such as pests or problems with irrigation. The company processes drone data from crop fields in more than 50 countries. It is now using machine learning to train its systems to differentiate between crops and weeds, and hopes to have this capability ready for the 2017 growing season. “We will be able to ping growers with an alert saying you have weeds growing in your field, here and here,” says crop scientist Jason Barton, an executive at Agribotix.
Modern technology that can autonomously eliminate pests and target agrichemicals better will reduce collateral damage to wildlife, lower resistance and cut costs. “We are working with a pesticide company keen to apply from the air using a drone,” says Green. Rather than spraying a whole field, the pesticide could be delivered to the right spot in the quantity needed, he says. The potential reductions in pesticide use are impressive. According to researchers at the University of Sydney's Australian Centre for Field Robotics, targeted spraying of vegetables used 0.1% of the volume of herbicide used in conventional blanket spraying. Their prototype robot is called RIPPA (Robot for Intelligent Perception and Precision Application) and shoots weeds with a directed micro-dose of liquid. Scientists at Harper Adams are going even further, testing a robot that does away with chemicals altogether by blasting weeds close to crops with a laser. “Cameras identify the growing point of the weed and our laser, which is no more than a concentrated heat source, heats it up to 95 °C, so the weed either dies or goes dormant,” says Blackmore.
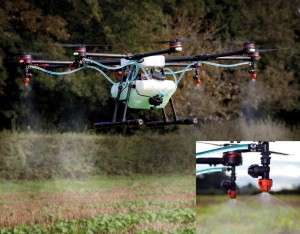
Animal trackers
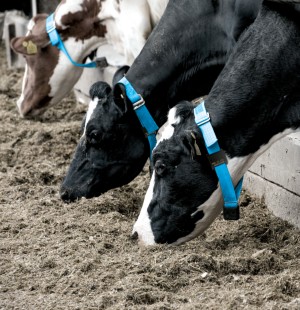
Smart collars — a bit like the wearable devices designed to track human health and fitness — have been used to monitor cows in Scotland since 2010. Developed by Glasgow start-up Silent Herdsman, the collar monitors fertility by tracking activity — cows move around more when they are fertile — and uses this to alert farmers to when a cow is ready to mate, sending a message to his or her laptop or smartphone. The collars ( pictured ), which are now being developed by Israeli dairy-farm-technology company Afimilk after they acquired Silent Herdsman last year, also detect early signs of illness by monitoring the average time each cow spends eating and ruminating, and warning the farmer via a smartphone if either declines.
“We are now looking at more subtle behavioural changes and how they might be related to animal health, such as lameness or acidosis,” says Richard Dewhurst, an animal nutritionist at Scotland's Rural College (SRUC) in Edinburgh, who is involved in research to expand the capabilities of the collar. Scientists are developing algorithms to interrogate data collected by the collars.
In a separate project, Dewhurst is analysing levels of exhaled ketones and sulfides in cow breath to reveal underfeeding and tissue breakdown or excess protein in their diet. “We have used selected-ionflow-tube mass spectrometry, but there are commercial sensors available,” says Dewhurst.
Cameras are also improving the detection of threats to cow health. The inflammatory condition mastitis — often the result of a bacterial infection — is one of the biggest costs to the dairy industry, causing declines in milk production or even death. Thermal-imaging cameras installed in cow sheds can spot hot, inflamed udders, allowing animals to be treated early.
Carol-Anne Duthie, an animal scientist at SRUC, is using 3D cameras to film cattle at water troughs to estimate the carcass grade (an assessment of the quality of a culled cow) and animal weight. These criteria determine the price producers are paid. Knowing the optimum time to sell would maximize profit and provide abattoirs with more-consistent animals. “This has knock on effects in terms of overall efficiency of the entire supply chain, reducing the animals which are out of specification reaching the abattoir,” Duthie explains.
And researchers in Belgium have developed a camera system to monitor broiler chickens in sheds. Three cameras continually track the movements of thousands of individual birds to spot problems quickly. “Analysing the behaviour of broilers can give an early warning for over 90% of problems,” says bioengineer Daniel Berckmans at the University of Leuven. The behaviour-monitoring system is being sold by Fancom, a livestock-husbandry firm in Panningen, the Netherlands. The Leuven researchers have also launched a cough monitor to flag respiratory problems in pigs, through a spin-off company called SoundTalks. This can give a warning 12 days earlier than farmers or vets would normally be able to detect a problem, says Berckmans. The microphone, which is positioned above animals in their pen, identifies sick individuals so that treatment can be targeted. “The idea was to reduce the use of antibiotics,” says Berckmans.
Berckmans is now working on downsizing a stress monitor designed for people so that it will attach to a cow's ear tag. “The more you stress an animal, the less energy is available from food for growth,” he says. The monitor takes 200 physiological measurements a second, alerting farmers through a smartphone when there is a problem.
Silicon soil saviours
The richest resource for arable farmers is soil. But large harvesters damage and compact soil, and overuse of agrichemicals such as nitrogen fertilizer are bad for both the environment and a farmer's bottom line. Robotics and autonomous machines could help.
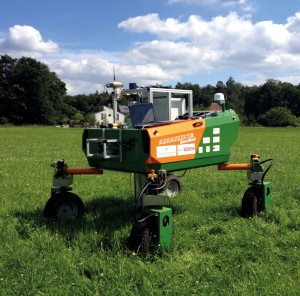
Data from drones are being used for smarter application of nitrogen fertilizer. “Healthy vegetation reflects more near-infrared light than unhealthy vegetation,” explains Barton. The ratio of red to near-infrared bands on a multispectral image can be used to estimate chlorophyll concentration and, therefore, to map biomass and see where interventions such as fertilization are needed after weather or pest damage, for example. When French agricultural technology company Airinov, which offers this type of drone survey, partnered with a French farming cooperative, they found that over a period of 3 years, in 627 fields of oilseed rape ( Brassica napus ), farmers used on average 34 kilograms less nitrogen fertilizer per hectare than they would without the survey data. This saved on average €107 (US$115) per hectare per year.
Bonirob ( pictured ) — a car-sized robot originally developed by a team of scientists including those at Osnabrück University of Applied Sciences in Germany — can measure other indicators of soil quality using various sensors and modules, including a moisture sensor and a penetrometer, which is used to assess soil compaction. According to Arno Ruckelshausen, an agricultural technologist at Osnabrück, Bonirob can take a sample of soil, liquidize it and analyse it to precisely map in real time characteristics such as pH and phosphorous levels. The University of Sydney's smaller RIPPA robot can also detect soil characteristics that affect crop production, by measuring soil conductivity.
Soil mapping opens the door to sowing different crop varieties in one field to better match shifting soil properties such as water availability. “You could differentially seed a field, for example, planting deep-rooting barley or wheat varieties in more sandy parts,” says Maurice Moloney, chief executive of the Global Institute for Food Security in Saskatoon, Canada. Growing multiple crops together could also lead to smarter use of agrichemicals. “Nature is strongly against monoculture, which is one reason we have to use massive amounts of herbicide and pesticides,” says van Henten. “It is about making the best use of resources.”
Mixed sowing would challenge an accepted pillar of agricultural wisdom: that economies of scale and the bulkiness of farm machinery mean vast fields of a single crop is the most-efficient way to farm, and the bigger the machine, the more-efficient the process. Some of the heaviest harvesters weigh 60 tonnes, cost more than a top-end sports car and leave a trail of soil compaction in their wake that can last for years.
But if there is no need for the farmer to drive the machine, then one large vehicle that covers as much area as possible is no longer needed. “As soon as you remove the human component, size is irrelevant,” says van Henten. Small, autonomous robots make mixed planting feasible and would not crush the soil.
In April, researchers at Harpers Adams began a proof-of-concept experiment with a hectare of barley. “We plan to grow and harvest the entire crop from start to finish with no humans entering the field,” says Green. The experiment will use existing machinery, such as tractors, that have been made autonomous, rather than new robots, but their goal is to use the software developed during this trial as the brains of purpose-built robots in the future. “Robots can facilitate a new way of doing agriculture,” says van Henten. Many of these disruptive technologies may not be ready for the prime time just yet, but the revolution is coming.
You can also search for this author in PubMed Google Scholar
Related links
Related links in nature research.
Bioengineering: Solar upgrade
Agrobiodiversity: The living library
Outlook on agriculture and drought
Related external links
Hands free hectare 2
Rights and permissions
Reprints and permissions
About this article
Cite this article.
King, A. Technology: The Future of Agriculture. Nature 544 , S21–S23 (2017). https://doi.org/10.1038/544S21a
Download citation
Published : 27 April 2017
Issue Date : 27 April 2017
DOI : https://doi.org/10.1038/544S21a
Share this article
Anyone you share the following link with will be able to read this content:
Sorry, a shareable link is not currently available for this article.
Provided by the Springer Nature SharedIt content-sharing initiative
This article is cited by
Enhancing hill farming efficiency using unmanned agricultural vehicles: a comprehensive review.
- Mrutyunjay Padhiary
- Laxmi Narayan Sethi
- Avinash Kumar
Transactions of the Indian National Academy of Engineering (2024)
How to make sense of 3D representations for plant phenotyping: a compendium of processing and analysis techniques
- Negin Harandi
- Breght Vandenberghe
- Arnout Van Messem
Plant Methods (2023)
Precise in-field molecular diagnostics of crop diseases by smartphone-based mutation-resolved pathogenic RNA analysis
- Qingdong Zeng
- Ruijie Deng
Nature Communications (2023)
Nachhaltige Digitalisierung. Gesellschaftliche Transformation, autonome Materialität und der Fall des Digital Farming
- Anna Henkel
Berliner Journal für Soziologie (2023)
Quick links
- Explore articles by subject
- Guide to authors
- Editorial policies
Sign up for the Nature Briefing: Anthropocene newsletter — what matters in anthropocene research, free to your inbox weekly.

- Email Alert
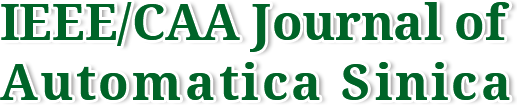
论文 全文 图 表 新闻
- Abstracting/Indexing
- Journal Metrics
- Current Editorial Board
- Early Career Advisory Board
- Previous Editor-in-Chief
- Past Issues
- Current Issue
- Special Issues
- Early Access
- Online Submission
- Information for Authors
- Share facebook twitter google linkedin
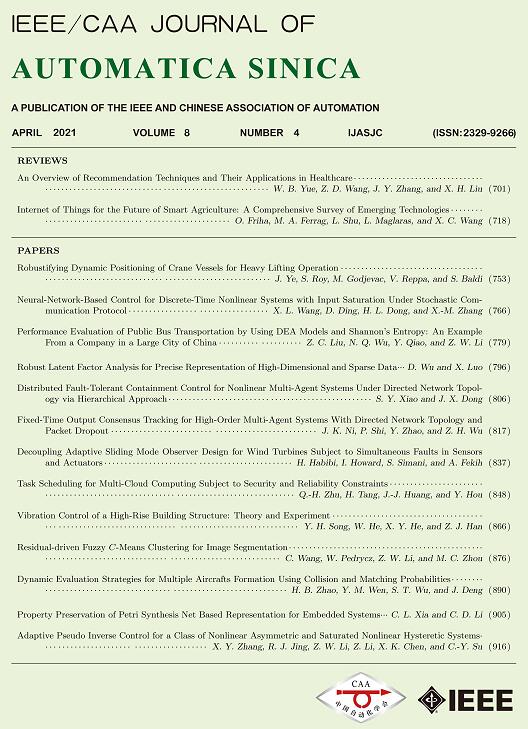
IEEE/CAA Journal of Automatica Sinica
- JCR Impact Factor: 11.8 , Top 4% (SCI Q1) CiteScore: 17.6 , Top 3% (Q1) Google Scholar h5-index: 77, TOP 5
Internet of Things for the Future of Smart Agriculture: A Comprehensive Survey of Emerging Technologies
Doi: 10.1109/jas.2021.1003925.
- Othmane Friha 1 , ,
- Mohamed Amine Ferrag 2 , ,
- Lei Shu 3, 4 , , ,
- Leandros Maglaras 5 , ,
- Xiaochan Wang 6 ,
Networks and Systems Laboratory, University of Badji Mokhtar-Annaba, Annaba 23000, Algeria
Department of Computer Science, Guelma University, Gulema 24000, Algeria
College of Engineering, Nanjing Agricultural University, Nanjing 210095, China
School of Engineering, University of Lincoln, Lincoln LN67TS, UK
School of Computer Science and Informatics, De Montfort University, Leicester LE1 9BH, UK
Department of Electrical Engineering, Nanjing Agricultural University, Nanjing 210095, China
Othmane Friha received the master degree in computer science from Badji Mokhtar-Annaba University, Algeria, in 2018. He is currently working toward the Ph.D. degree in the University of Badji Mokhtar-Annaba, Algeria. His current research interests include network and computer security, internet of things (IoT), and applied cryptography
Mohamed Amine Ferrag received the bachelor degree (June, 2008), master degree (June, 2010), Ph.D. degree (June, 2014), HDR degree (April, 2019) from Badji Mokhtar-Annaba University, Algeria, all in computer science. Since October 2014, he is a Senior Lecturer at the Department of Computer Science, Guelma University, Algeria. Since July 2019, he is a Visiting Senior Researcher, NAULincoln Joint Research Center of Intelligent Engineering, Nanjing Agricultural University. His research interests include wireless network security, network coding security, and applied cryptography. He is featured in Stanford University’s list of the world’s Top 2% Scientists for the year 2019. He has been conducting several research projects with international collaborations on these topics. He has published more than 60 papers in international journals and conferences in the above areas. Some of his research findings are published in top-cited journals, such as the IEEE Communications Surveys and Tutorials , IEEE Internet of Things Journal , IEEE Transactions on Engineering Management , IEEE Access , Journal of Information Security and Applications (Elsevier), Transactions on Emerging Telecommunications Technologies (Wiley), Telecommunication Systems (Springer), International Journal of Communication Systems (Wiley), Sustainable Cities and Society (Elsevier), Security and Communication Networks (Wiley), and Journal of Network and Computer Applications (Elsevier). He has participated in many international conferences worldwide, and has been granted short-term research visitor internships to many renowned universities including, De Montfort University, UK, and Istanbul Technical University, Turkey. He is currently serving on various editorial positions such as Editorial Board Member in Journals (Indexed SCI and Scopus) such as, IET Networks and International Journal of Internet Technology and Secured Transactions (Inderscience Publishers)
Lei Shu (M’07–SM’15) received the B.S. degree in computer science from South Central University for Nationalities in 2002, and the M.S. degree in computer engineering from Kyung Hee University, South Korea, in 2005, and the Ph.D. degree from the Digital Enterprise Research Institute, National University of Ireland, Ireland, in 2010. Until 2012, he was a Specially Assigned Researcher with the Department of Multimedia Engineering, Graduate School of Information Science and Technology, Osaka University, Japan. He is currently a Distinguished Professor with Nanjing Agricultural University and a Lincoln Professor with the University of Lincoln, U.K. He is also the Director of the NAU-Lincoln Joint Research Center of Intelligent Engineering. He has published over 400 papers in related conferences, journals, and books in the areas of sensor networks and internet of things (IoT). His current H-index is 54 and i10-index is 197 in Google Scholar Citation. His current research interests include wireless sensor networks and IoT. He has also served as a TPC Member for more than 150 conferences, such as ICDCS, DCOSS, MASS, ICC, GLOBECOM, ICCCN, WCNC, and ISCC. He was a Recipient of the 2014 Top Level Talents in Sailing Plan of Guangdong Province, China, the 2015 Outstanding Young Professor of Guangdong Province, and the GLOBECOM 2010, ICC 2013, ComManTel 2014, WICON 2016, SigTelCom 2017 Best Paper Awards, the 2017 and 2018 IEEE Systems Journal Best Paper Awards, the 2017 Journal of Network and Computer Applications Best Research Paper Award, and the Outstanding Associate Editor Award of 2017, and the 2018 IEEE ACCESS. He has also served over 50 various Co-Chair for international conferences/workshops, such as IWCMC, ICC, ISCC, ICNC, Chinacom, especially the Symposium Co-Chair for IWCMC 2012, ICC 2012, the General Co-Chair for Chinacom 2014, Qshine 2015, Collaboratecom 2017, DependSys 2018, and SCI 2019, the TPC Chair for InisCom 2015, NCCA 2015, WICON 2016, NCCA 2016, Chinacom 2017, InisCom 2017, WMNC 2017, and NCCA 2018
Leandros Maglaras (SM’15) received the B.Sc. degree from Aristotle University of Thessaloniki, Greece, in 1998, M.Sc. in industrial production and management from University of Thessaly in 2004, and M.Sc. and Ph.D. degrees in electrical & computer engineering from University of Volos in 2008 and 2014, respectively. He is the Head of the National Cyber Security Authority of Greece and a Visiting Lecturer in the School of Computer Science and Informatics at the De Montfort University, U.K. He serves on the Editorial Board of several International peer-reviewed journals such as IEEE Access , Wiley Journal on Security & Communication Networks , EAI Transactions on e-Learning and EAI Transactions on Industrial Networks and Intelligent Systems . He is an author of more than 80 papers in scientific magazines and conferences and is a Senior Member of IEEE. His research interests include wireless sensor networks and vehicular ad hoc networks
Xiaochan Wang is currently a Professor in the Department of Electrical Engineering at Nanjing Agricultural University. His main research fields include intelligent equipment for horticulture and intelligent measurement and control. He is an ASABE Member, and the Vice Director of CSAM (Chinese Society for Agricultural Machinery), and also the Senior Member of Chinese Society of Agricultural Engineering. He was awarded the Second Prize of Science and Technology Invention by the Ministry of Education (2016) and the Advanced Worker for Chinese Society of Agricultural Engineering (2012), and he also gotten the “Blue Project” in Jiangsu province young and middle-aged academic leaders (2010)
- Corresponding author: Lei Shu, e-mail: [email protected]
- Revised Date: 2020-11-25
- Accepted Date: 2020-12-30
- Agricultural internet of things (IoT) ,
- internet of things (IoT) ,
- smart agriculture ,
- smart farming ,
- sustainable agriculture
Proportional views
通讯作者: 陈斌, [email protected].
沈阳化工大学材料科学与工程学院 沈阳 110142
Figures( 12 ) / Tables( 9 )
Article Metrics
- PDF Downloads( 2290 )
- Abstract views( 12203 )
- HTML views( 1410 )
- We review the emerging technologies used by the Internet of Things for the future of smart agriculture.
- We provide a classification of IoT applications for smart agriculture into seven categories, including, smart monitoring, smart water management, agrochemicals applications, disease management, smart harvesting, supply chain management, and smart agricultural practices.
- We provide a taxonomy and a side-by-side comparison of the state-of-the-art methods toward supply chain management based on the blockchain technology for agricultural IoTs.
- We highlight open research challenges and discuss possible future research directions for agricultural IoTs.
- Copyright © 2022 IEEE/CAA Journal of Automatica Sinica
- 京ICP备14019135号-24
- E-mail: [email protected] Tel: +86-10-82544459, 10-82544746
- Address: 95 Zhongguancun East Road, Handian District, Beijing 100190, China
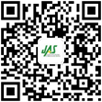
Export File

- Figure 1. The four agricultural revolutions
- Figure 2. Survey structure
- Figure 3. IoT-connected smart agriculture sensors enable the IoT
- Figure 4. The architecture of a typical IoT sensor node
- Figure 5. Fog computing-based agricultural IoT
- Figure 6. SDN/NFV architecture for smart agriculture
- Figure 7. Classification of IoT applications for smart agriculture
- Figure 8. Greenhouse system [ 101 ]
- Figure 9. Aerial-ground robotics system [ 67 ]
- Figure 10. Photovoltaic agri-IoT schematic diagram [ 251 ]
- Figure 11. Smart dairy farming system [ 254 ]
- Figure 12. IoT-based solar insecticidal lamp [ 256 ], [ 257 ]
- Open access
- Published: 08 February 2021
Adoption of improved agricultural technology and its impact on household income: a propensity score matching estimation in eastern Ethiopia
- Muluken G. Wordofa ORCID: orcid.org/0000-0002-5143-180X 1 ,
- Jemal Y. Hassen 1 ,
- Getachew S. Endris 1 ,
- Chanyalew S. Aweke 1 ,
- Dereje K. Moges 1 &
- Debbebe T. Rorisa 2
Agriculture & Food Security volume 10 , Article number: 5 ( 2021 ) Cite this article
22k Accesses
48 Citations
Metrics details
Adoption of improved agricultural technologies remains to be a promising strategy to achieve food security and poverty reduction in many developing countries. However, there are limited rigorous impact evaluations on the contributions of such technologies on household welfare. This paper investigates the impact of improved agricultural technology use on farm household income in eastern Ethiopia.
Primary data for the study was obtained from a random sample of 248 rural households, 119 of which are improved technology users and the rest are non-users. The research employed the Propensity Score Matching (PSM) procedure to establish the causal relationship between adoption of improved crop and livestock technologies and changes in farm income.
Results from the econometric analysis show that households using improved agricultural technologies had, on average, 23,031.28 Birr (Birr is the official currency of Ethiopia. The exchange rate according to the National Bank of Ethiopia (NBE) was 1 USD = 27.6017 Birr on 04 October 2018.) higher annual farm income compared to those households not using such technologies. Our findings highlight the importance of promoting multiple and complementary agricultural technologies among rural smallholders.
Conclusions
We suggest that rural technology generation, dissemination and adoption interventions be strengthened. Moreover, the linkage among research, extension, universities and farmers needs to be enhanced through facilitating a multistakeholders innovation platforms.
Sub-Saharan Africa (SSA) is one of the regions in the world that is mainly characterized by smallholder farm households whose livelihood depends primarily on rainfed agriculture. It is also the region with millions of people living under extreme poverty [ 1 ]. It has been widely acknowledged that smallholder mixed crop-livestock agriculture plays a great role in feeding rural and urban populations in Ethiopia. It is considered as a center-stage of economic development and a platform to win the battle to food security and poverty reduction in the country [ 2 ]. The agricultural sector contributes 43% of Gross Domestic Product (GDP), 90% of export earnings, and 96% of rural employment [ 3 ]. It also provides raw materials for industries in the country.
Realizing the importance of the agricultural sector to the country’s economy, the government of Ethiopia has given a lot of attention to the sector and institutions that support it – research and extension in particular. The government’s emphasis to improve agricultural production and productivity, enhance food security, expedite commercialization and market integration, and improve rural livelihoods of smallholders was deeply reflected in the Agricultural Development Led Industrialization (ADLI) economic growth strategy since 1992, and the 5-year Growth and Transformation Plans (GTP-I&II) since 2010. However, the sector is not well developed especially in terms of technology use and commercialization. Despite its contribution, the performance of the sector has remained largely unsatisfactory [ 4 ].
Agricultural production in the country, and especially in the eastern part, is constrained by numerous factors, including small land size that is often not adequate to be economically viable; climate-change induced unreliable and irregular rainfall that frequently results in periodic drought; poor or declining soil fertility; limited input/output market integration; and very low level of use of improved agricultural technologies [ 5 ]. Although the country’s agricultural extension system is hailed as one of the strongest in Africa, disseminating and popularizing productivity-enhancing improved agricultural technologies and best practices remains to be of paramount importance in order to foster economic growth and alleviate food insecurity and vulnerability to poverty and its correlates.
In order to accelerate diffusion and adoption of agricultural technologies in the country, the Ethiopian Institute of Agricultural Research (EIAR), Regional Agricultural Research Institutes (RARIs), and universities have been experimenting and releasing several improved agricultural technologies in crops, livestock, and natural resource management. In addition, Agricultural Technical and Vocational Education and Training (ATVET) colleges in the country have been training frontline extension professionals who will station at the Farmers’ Training Centers (FTCs) established at the lowest level of administrative units throughout the country. These are some of the efforts made to increase the adoption of improved agricultural technologies by smallholders in the country.
There are recent studies that investigated the contribution of improved crop, livestock and natural resource management technologies on farm household income across Africa. Regarding crop technologies, for instance, using improved and/or drought-/disease-tolerant maize varieties in Kenya [ 6 ], in Zambia [ 7 ], in Zimbabwe [ 8 ], and in Benin [ 9 ] was found to increase farm income. A similar result was also obtained for smallholder maize growers in South Africa who participated in homestead food garden programs [ 10 ]. Adopting improved groundnut varieties in Uganda [ 11 ] and tissue culture banana technology in Kenya [ 12 ] were also found to result in increased household income. In Ethiopia, available literature documents the positive impact of improved wheat technology [ 13 ] and high-yielding sorghum varieties [ 14 ]. Concerning livestock production and management technologies, [ 15 ] in Tanzania and [ 16 ] in Rwanda showed the positive causal effects of dairy and sericulture technologies on household income, respectively. Finally, soil fertility management interventions (i.e., mulching) in Nigeria [ 17 ] and improved fallow techniques in Zambia [ 18 ] were associated with improved farm income of adopters. The abovementioned are some recent examples illustrating the causal relationship between adopting a single agricultural technology and household income.
However, few studies assessed the impact of simultaneous adoption of multiple or a combination of agricultural technologies on household welfare. Such studies can highlight complementarities among technologies and can show how one technology can have a multiplier effect by reinforcing the economic effect of the other technology. An earlier study in Mozambique documented an improved household income as a result of adopting improved seeds and tractors [ 19 ]. A similar impact on farm income was found for farmers adopting fertilizer micro-dosing and tied-ridge technologies in Tanzania [ 20 ]. Likewise, adoption of Sustainable Agricultural Practices [ 21 ] and improved seeds and fertilizer [ 22 ] in Ethiopia resulted in better household income for adopters.
Finally, adoption of multiple and/or complementary agricultural technologies–improved seeds, chemical fertilizers, pesticides and soil and water conservation practices–in the country is shown to enhance consumption expenditure and improve poverty status [ 3 ]. However, there is limited empirical study in eastern Ethiopia regarding the causal effect of multiple agricultural technology adoption on household welfare. Scarcity of such empirical investigations has created a knowledge gap on the performance and impact of such agricultural technologies and best practices in the region. In addition, there is a scanty empirical evidence on the impact and performance of agricultural technologies developed, disseminated and/or scaled-up by agricultural universities and their roles in improving food security and livelihood outcomes for farm households. Therefore, the current study systematically investigated the impact of improved agricultural technologies on farm household income in eastern Ethiopia.
Research design and study area
This study relied on an ex-post data collection from a sample of improved agricultural technology users and non-users. Empirical data for the study comes from households residing in six districts of eastern Ethiopia. These are: Kombolcha, Haramaya, Babile, Meta, Girawa, and Sofi. These districts were selected primarily because they are in the mandate area of the Haramaya University’s agricultural technology dissemination and community service activities. On top of this, representativeness to the major agro-ecological zones to represent diversity of livelihood activities, prevalence of food insecurity, and ease of accessibility were taken into account in the selection process. In Table 1 , we present a brief description of the selected districts.
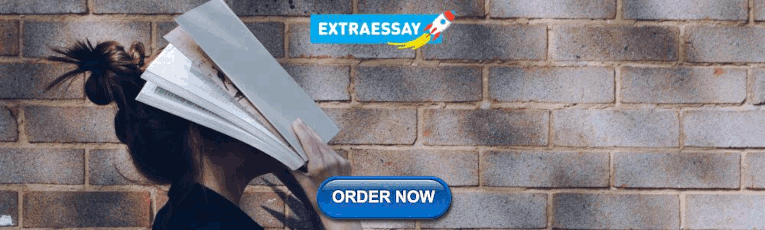
Sampling procedure
A multi-stage sampling procedure was employed in this study. In the first stage, six districts were purposively selected as described above. This study made reference to the following improved crop and livestock technologies developed, disseminated, popularized and/or scaled-up by Haramaya University:
improved crop varieties–Sorghum (Muyira-1, Muyira-2, Melkamash-79, Awash-1050, Dedesa-1050, and Alemaya–70); Maize (Alemaya composite, Rare-1, Bukuri); Wheat (Kulkulu); Groundnut (Oldhale and Roba); Potato (Gudane, Gabisa, Bubu, Chiro, Badhasa, and Harchasa); Sweet Potato; and,
improved livestock technologies – portable poultry houses, poultry birds, cattle breeds, animal feed/forage, and apiculture technologies.
These technologies were chosen following a field scoping survey and a desk review conducted prior to the main survey to map the status and use of improved agricultural technologies that were developed, disseminated, and/or scaled up by Haramaya University.
During the second stage, a list of improved agricultural technology user farmers was generated across the selected districts in consultation with Development Agents (DAs), community/local leaders, administrators of FTCs, and representatives of district Bureau of Agriculture and Natural Resources. The generated list contained 1,785 improved agricultural technology users in the six districts–Girawa (250), Kombolcha (320), Sofi (197), Meta (284), Haramaya (416), and Babile (318). In this study, an improved agricultural technology user is defined as a farm household who has been using one or more of the aforementioned improved agricultural technologies consistently for at least 2 years. From the total households who are currently using improved agricultural technologies, a total of 119 users were randomly selected from the prepared list. Following Probability Proportional to Size (PPS) sampling procedure, this resulted in the random selection of 17 households from Girawa, 21 from Kombolcha, 13 from Sofi, 19 from Meta, 28 from Haramaya, and 21 from Babile districts. Likewise, in order to serve as a comparison group for the purpose of impact evaluation, random samples were drawn from a list of non-users of improved agricultural technologies. This list contained a total of 1,935 households – Girawa (259), Kombolcha (344), Sofi (240), Meta (297), Haramaya (458), and Babile (337). Consequently, a total of 129 households were randomly chosen and included in the study as control groups. Following PPS sampling procedure, this resulted in the random selection of 17 households from Girawa, 23 from Kombolcha, 16 from Sofi, 20 from Meta, 31 from Haramaya, and 22 from Babile. Hence, the overall sample size for this study is 248.
Data collection
This step in the research process started with the selection and training of research assistants (i.e., data collectors/enumerators) as well as translation of the questionnaire to local language (Afan Oromo). Based on information from key-informants, six research assistants who were trained (at agricultural colleges) in crop production, livestock production and management, or natural resource management were selected. Furthermore, three supervisors from Haramaya University were employed in order to closely supervise the process of data collection and provide real-time feedback whenever necessary. Both the enumerators and supervisors were given orientation training on the overall process of data collection.
The questionnaire, developed by the research team, was pre-tested on a randomly sampled 30 non-sample households. Based on the feedback obtained from the pre-testing exercise, additional orientations were given to the enumerators and supervisors. Both qualitative and quantitative data were collected–through face-to-face interviews using paper and pen as well as Focused Group Discussions (FGDs)–from primary and secondary sources. Socio-economic, demographic and institutional data of the study participants (i.e., household heads) were gathered. The participants were also asked about source and time of information on the agricultural technologies/innovations, technology selection criteria, major attributes of the innovations/technologies, crop and livestock technologies adopted and household consumption of food groups, to mention some.
Empirical strategy to data analysis
This study employed descriptive and inferential statistics, and an econometric model to analyze data. Descriptive statistics, such as mean and standard deviation, were used to present summary statistics of quantitative data pertaining to socio-demographic, economic, and institutional characteristics of sample households. Inferential statistics, such as t-test and Chi-Square ( \({\chi }^{2})\) test, were used to assess the existence of statistically significant differences in observations between improved agricultural technology user and non-user groups of respondents. In this study, farm income, the outcome variable, refers to the annual agricultural income (in Birr) obtained from crop and livestock less associated production costs during the last production season preceding the survey.
The theoretical framework used in this study makes a reference to the process of evaluating the impact of a program or an intervention on an outcome indicator. It requires conceptualizing and answering the tough question: ‘what would have happened to participants of a program/an intervention had they not participated in it?’ Referred to as ‘the fundamental problem of causal inference’ or ‘fundamental evaluation problem’ [ 23 ], this is a serious issue since an individual can only be in a state of either participating or not participating in the program at a given time [9; 39]. The ideal way to deal with the problem of counterfactuals is to employ Randomized Control Trials (RCTs) following the potential outcome approach or Roy–Rubin model [ 24 , 25 ]. However, RCTs were not viable in the present study setting due to non-random allocation of farm households to treatment and control groups (i.e., placement/targeting bias) and selection bias.
The alternative to the experimental approach is the use of quasi-experimental approaches, which seek to create, using empirical methods, a comparable control group that can serve as a reasonable counterfactual [ 19 , 26 ]. In the present study, among the available non-experimental approaches, the Propensity Score Matching (PSM) procedure is implemented due to the nature of data available for analysis.
Matching methods in evaluating program/treatment effects
The fundamental notion behind matching is to construct a comparable group of individuals–who are similar to the treatment individuals/groups in all relevant pre-treatment characteristics X–from a sample of untreated ones. In practice, a model (Probit or Logit for binary treatment) is estimated in which participation in a treatment/program is explained by several pre-treatment characteristics and then predictions of this estimation are used to create the propensity score that ranges from 0 to 1.
There are different approaches of implementing PSM, including the Nearest Neighbor (NN) matching, Caliper or Radius matching, Stratification or Interval matching, and Kernel and Local Linear matching [ 27 ]. In the present investigation, the Nearest Neighbor Matching (with 5-Neighbors and One-to-One matching) is implemented.
There are two assumptions surrounding the implementation of the PSM. The first one is referred to as unconfoundedness [ 28 ], selection on observables [ 29 ], or Conditional Independence Assumption (CIA) [ 30 ]. According to this assumption, the treatment needs to fulfill the criterion of being exogenous, implying that any systematic difference in outcomes between the treatment and comparison groups with the same values for characteristics X can be attributed to the treatment. The second assumption, called common support or overlap , ensures that individuals/groups with the same values for characteristics X have a positive probability of being both participants and non-participants of a program/treatment [ 23 ]. The overlap condition enables to compare comparable units. Nevertheless, in order to deal with the ‘curse of dimensionality’ problem, Rosenbaum and Rubin [ 28 ] show that if the potential outcomes of treated ( \({Y}_{1}\) ) and control ( \({Y}_{0}\) ) are independent of treatment allocation conditional on covariates X, then they are also independent of treatment conditional on the propensity score as shown in Eq. 1 .
Generalizing the above issues, assuming that the unconfoundedness assumption holds and there is sufficient overlap between the treatment and comparison groups, the PSM estimator for the Average Treatment Effect on the Treated (ATT) conditional on the propensity score can be written as.
This means, the PSM estimator is simply the mean difference in outcomes over the common support region, appropriately weighted by the propensity score distribution of treated participants [ 31 ].
A number of techniques are available to check covariate balancing during matching process. In terms of mean comparisons, a two-sample t-test (before and after matching) can be used to check the existence or lack of significant differences in covariate means between the treated and comparison groups [ 28 ]. As a rule-of-thumb, there should not be any significant difference in means after matching. Regarding standardized bias, Rosenbaum and Rubin [ 28 ] define the absolute standardized bias (for each covariate X) as the absolute difference in sample means between the matched treatment and comparison samples as a percentage of the square root of the average sample variance in the two groups.
The standardized bias before matching can be written as
The standardized bias after matching can be written as
\(\stackrel{-}{\mathrm{X}}1\) (V1) is the mean (variance) in the treatment group before matching.
\(\stackrel{-}{\mathrm{X}}0\) (V0) the corresponding values for the comparison group.
\(\stackrel{-}{\mathrm{X}}1\) M (V1M) and \(\stackrel{-}{\mathrm{X}}0\) M (V0M) are the mean (variance) values for the matched samples.
Sianesi [ 32 ] suggests the comparison of Pseudo-R 2 before and after matching as a method to check balancing. The Pseudo-R 2 indicates how well the covariates X explain the probability of participating in the treatment. The Pseudo-R 2 has to be very low after matching to indicate success of the matching process. Moreover, the Likelihood Ratio (LR) test on the joint significance of all covariates in the (Logit) model should not be rejected before matching, but should be rejected afterwards [ 31 ].
Results and discussion
Descriptive statistics results.
Results related to socio-demographic characteristics are presented in the top section of Table 2 . From the results, we note that there is a statistically significant difference in age, gender, school years, and farming experiences between users and non-users of improved agricultural technologies, while the mean value for family size of respondents was found to be not significantly different between the two groups. These observations imply that the farm households who used improved agricultural technologies were majorly male farmers and relatively older than those who did not use the technologies. It is widely acknowledged that male farmers are more likely to adopt agricultural technologies than their female counterparts [ 33 , 34 , 35 ]. The reasons could be that female farmers have difficulty accessing inputs [ 36 ] and other norms and beliefs prevailing in the society [ 37 ]. Experience in terms of age is found to positively affect adoption of a System of Rice Intensification (SRI) in India [ 38 ]. However, age of farmers did not significantly affect the adoption of soil-improving practices in Ghana [ 21 ].
Our findings suggest that the farmers who have used technologies from Haramaya University have stayed in schools and on farming activities for more number of years compared to those who did not use the technologies. Our result is consistent with that of Makate et al. [ 28 ] who documented a positive effect of education on adoption of multiple climate smart agricultural innovations in Southern Africa. However, our finding contrasts with that of Nata, Mjelde and Boadu [ 21 ] for Ghana. In general, however, investment in education is essential for development and would encourage farmers to adopt appropriate technologies and practices [ 39 ]. In this study, male household heads appear to have been the preferred targets of improved agricultural dissemination process. Such bias against young, less educated and less experienced, and female household heads is a long-standing bottleneck in adoption and diffusion of agricultural technologies in the country.
The information obtained from several Focused Group Discussions (FGDs) conducted with female headed households specifically revealed that there was a frequent targeting bias against, among others, female farmers and this supports the finding related to gender-based differences among users of the technology [ 33 , 34 , 35 , 40 , 41 , 42 , 43 ]. An FGD participant in Babile revealed that “since most of the extension agents are males, and due to cultural and religious expectation, they do not interact adequately with female headed households as well as female members of the community. This, in my opinion, has created a barrier in obtaining and using relevant information on improved agricultural technologies and practices.” Besides, the qualitative study has also shown that the interventions of Haramaya University were less successful in recognizing gender sensitive preference criteria for the technologies and female targets were found to be more prone to such phenomena compared to the male counterparts.
The second category of explanatory variables are economic factors. Several variables, vis-à-vis livestock ownership, land size, non-farm income, irrigation access, use of conservation practices, and asset value were included in this category. According to the results (depicted in Table 2 ), a statistically significant difference was observed between the users and non-users for three out of six economic variables. Livestock owned, land size, and SWC practices were the significant variables, while non-/off-farm income, access to irrigation, and asset value were found not significantly varying between the two groups of respondents.
These results indicate that farm households who owned more livestock, operated a relatively large plot of land, and participated in SWC practices had a better chance of improved agricultural technology use due to the fact that such households are better-off in taking risks associated with new technologies and practices, or these households received preferential treatment by the promoters of improved agricultural technologies. The result pertaining to the effect of plot area is related to that of Martey, Kuwornu and Adjebeng-Danquah [ 44 ]. Similar findings were documented in Rwanda where asset endowments and participation in farmer organizations, among others, condition adoption of rainwater harvesting technologies to improve agricultural productivity and income [ 45 ]. Availability/endowment of farm assets is also found to positively influence the decision to adopt SRI in India [ 38 ]. Size of land owned by a farmer is found to have positive effect on adoption of multiple CSA innovations in Southern Africa [ 28 ]. Our result contrasts that of Varma [ 38 ], who found that small and marginal farmers are more likely to adopt SRI as compared to large farmers. Based on an inventory of Haramaya University’s technological interventions conducted prior to this study, most of the technologies generated and disseminated by the University are on-farm based and hence required an adequate size of plot for the farmers to participate in and draw benefits from the interventions. The fact that the non-user group possesses lesser farm size would tend to hinder them from utilizing such interventions. The finding pertaining to SWC is consistent with the results obtained from FGDs, which indicated that the target farmers have a long history working with the University on various SWC technologies among which cultivation of leguminous crops and tree species, as well as constructing SWC structures are the major ones.
Among the seven institutional variables considered in this study, only two were found to have significantly different distribution between the users and non-users of improved agricultural technologies. These are: participation in FTCs and access to market information. We find that improved agricultural technology users participated more in FTCs than the non-users. In an earlier study conducted in the study area, it was found that participation in FTC-based agricultural extension services significantly improved household income [ 37 ]. This confirms that FTCs play a great role in fostering economic development and that farm households should be encouraged to interact continuously with such knowledge and technology generation and transfer institutions in rural areas. We also observe that farm households with greater market information access had a significantly higher rate of improved agricultural technology use in the study area. Access to information is found to be positively associated with adoption of multiple CSA innovations in Southern Africa [ 28 ]. Market access has been identified as one of the important elements in enhancing agricultural technology adoption and improved household income. Access to market information is found to significantly influence adoption of improved pigeon peas in semi-arid south-east Kenya [ 46 ].
Regarding the outcome variable, i.e., farm income, we find that, on average, non-users of improved agricultural technology obtained 14,923.26 Birr/year while the users obtained 33,082.35 Birr/year. Users tend to earn more income per annum than the non-users, and the mean difference of it is statistically highly significant as shown in the top part of Table 2 .
Econometric model estimation results
The causal effect of improved agricultural technology use on farm income is estimated using the Propensity Score Matching (PSM) procedure. The analysis employed Nearest Neighbor Matching (with 5-Neighbors and One-to-One matching algorithms) using psmatch2 command implemented on STATA 15.1 platform. In what follows, the results pertaining to estimation of propensity scores, Average Treatment Effect on the Treated (ATT), and post-matching quality analyses are presented.
Estimation of propensity score
The conditional probability of households’ participation in improved agricultural technology use is estimated using a Logistic Regression model. The model considered all observable covariates that affect participation and farm income and for which observational data were available. The results are given in Table 3 . Overall, the model is statistically significant as shown in the lower part of Table 3 . Based on the findings, we note the existence of a statistically significant difference between treated ( n = 119) and control ( n = 129) households regarding the distributions of age, gender, family size, land size, SWC practices, and distance from main market (Column (3), Table 3 ). As depicted in Table 3 , these factors were responsible for households’ differential participation in improved agricultural technology use. Since we are interested in computing the propensity scores, which will be used in the matching process later on, we will not go into the details of why and how each of the covariates affected households’ participation in the intervention. Nevertheless, we indicate that as we proceed with our analysis, these before-matching differences are no longer significant in the aftermath of matching (Column (1), Table 5 ), which is an indication that the PSM was successful to experimentally create a comparable group of control individuals whose outcomes can be compared to that of the treated ones.
Our finding pertaining to the effect of age of on agricultural technology adoption is related to that of Martey, Kuwornu and Adjebeng-Danquah [ 44 ] in Ghana and Varma [ 38 ] in India. However, age of farmers was found not to significantly affect adoption of soil-improving practices in Ghana [ 21 ].Similar to our results, family size and size of cultivated land were found to positively affect agricultural technology use in Ghana [ 44 ].Likewise, availability/endowment of farm assets positively influence the decision to adopt Sustainable Rice Intensification (SRI) in India [ 38 ] and rainwater harvesting technologies in Rwanda [ 45 ]. In a recent study in Southern Africa, size of land owned by a farmer is also found to have a positive effect on adoption of multiple Climate Smart Agricultural (CSA) innovations [ 28 ]. Use of fallow, among SWC practices, is found to enhance technology adoption [ 44 ]. Finally, distance from main market, as an indicator of access to market information, is shown to have a positive effect on adoption in south-east Kenya [ 46 ] and Southern Africa [ 28 ].
Estimation of average treatment effect on the treated (ATT)
The estimation of Average Treatment Effect on the Treated (ATT) is performed using the Nearest Neighbour Matching (with 5-Nearest Neighbours and One-to-One matching algorithms). All the control households (i.e., 129) and 117 of the 119 treated households are used in the matching process, since these were found on the common support region (see Fig. 1 ). The results are presented in Table 4 . In addition to the mean values of the outcome variable, Table 4 contains mean differences between treated and control groups (Column 3) and bootstrap standard errors (with 50 replications) on the mean difference (Column 6). Overall, we found convergence of results between the two matching algorithms (Column 7). However, the discussion in this section is based on the results obtained using the One-to-One matching algorithm as this resulted in a higher level of statistical significance.
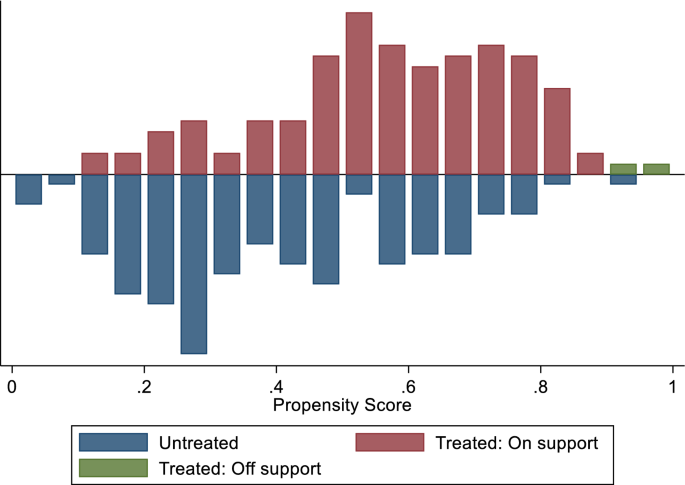
Propensity score graph all (129) untreated and 117 out of 119 treated observations are on common support region
Accordingly, the results show a statistically significant gain in household farm income as a result of using improved agricultural technologies in the study area. More specifically, we found that households using improved agricultural technologies obtained, on average, 23,031.28 Birr higher annual farm income compared to those households not using such technologies. This is a significant result implying that adoption of improved agricultural technologies and practices resulted in improved welfare in the study area. Our result is consistent with previous empirical results of Cunguara and Darnhofer [ 19 ] in Mozambique, who showed an improved household income as a result of adopting improved seeds and tractor; Habtemariam et al. [ 20 ] in Tanzania, who indicated the positive income effect of adopting fertilizer micro-dosing and tied-ridge technologies; and, Teklewold et al. [ 21 ] and Hailu, Abrha and Weldegiorgis [ 22 ] in Ethiopia, who documented a positive income effect of adopting Sustainable Agricultural Practices, and improved seeds and fertilizer, respectively.
Matching quality analyses
The matching quality analyses were performed using t-tests and Standardized Percentage Bias (Table 5 , Columns (1) and (2), respectively) and other measures of covariate imbalance (Table 6 ).
Looking at the t-test results after matching (Column 1, Table 5 ), we found that the statistically significant difference between treated and control groups that were observed for some covariates in the unmatched sample were fully removed. This implies that the matching process was effective in balancing the distributions of the covariates in the matched sample. Likewise, the Standardized Percentage Bias (Column 2, Table 5 ) appears to be in the acceptable range, complementing the post-estimation t-test results and implying further that the PSM performed well in yielding unbiased estimates of ATT.
In addition to the post-estimation t-test and standardized percentage bias results, other measures of covariate imbalance (Table 6 ) also indicate that the matching process is effective in balancing the pre-treatment characteristics.
Finally, the propensity score graph ( psgraph ) in Fig. 1 presents treated and untreated households that are found on the common support region (i.e., 117 and 129, respectively) and the two treated observations that are off the support region.
Conclusion and recommendations
In this study, we evaluated the causal effect of using improved crop and livestock technologies on farm household income using 248 randomly selected households in six districts of eastern Ethiopia. The study employed One-to-One and Nearest Neighbor matching algorithms using the PSM estimation procedure. Through collecting data specifically for the purpose of impact evaluation and implementing rigorous evaluation methods, the key findings of the study showed that smallholder farm households using improved agricultural technologies developed, disseminated and/or scaled-up by Haramaya University had a statistically significant household income compared to those not using these technologies. More specifically, we found that improved agricultural technology use resulted in, on average, 23,031.28 Birr higher annual farm income per household compared to non-use of such technologies. This estimate of farm income is robust as confirmed by the convergence of results obtained using the two matching algorithms and the results of matching quality analyses (i.e., post-matching t-test, standardized percentage bias, and other measures of covariate imbalance).
Based on the results of this study, the following recommendations are suggested to improve agricultural technology dissemination and adoption in the study area. To start with, there should be a reinvigorated awareness creation campaign by the university extension wing, district Bureau of Agriculture and Natural Resources, Non-Governmental Organizations (NGOs) and model farmers regarding the importance of improved crop and livestock technologies that can transform the livelihoods of smallholder farmers. In particular, young farmers need to be encouraged to partake in trying new technologies and best practices. Since the default household heads are males, there should be a provision to target young and women farmers (although they are not household heads). One operational suggestion in this regard requires a general shift of focus from household heads to members of the household. Furthermore, a minimum experience in farming should be sufficient to qualify young farm entrepreneurs to involve in improved agricultural technology adoption. Promoting a multi-stakeholder engagement in the design, dissemination, and scaling-up of proven agricultural technologies should also be the model of the university’s research, extension and community service provision.
Although the evaluation technique employed in this paper was based on a rigorous statistical procedure, it used a cross-sectional data and, hence, there are potentials for improvement through further investigation. The first aspect in this regard requires the collection of longitudinal data from more households across the different agro-ecological zones of the region. Such data can contain baseline information that can enable the use of, for example, randomized control trials (RCTs), which are regarded as “gold” standards in impact evaluation. Moreover, the availability of such a rich dataset can enable combining PSM with Difference-in-Difference (DID), which is superior to using each technique single-handedly. Second, more outcome indicators, such as productivity, food and nutrition security, poverty, consumption expenditure, and asset accumulation can be included to examine if improved agricultural technology adoption results in other added advantages in the study area. Another issue for future consideration can be a move away from individual level evaluations to aggregate levels (such as village, district, etc.) or a combination of both of them. Whereas the former can enable a researcher to better capture spillover effects of a program or an intervention as well as any unobserved heterogeneity between treatment and comparison groups, the latter can help in examining the complementarity of individual and group-based approaches to impact evaluation. Finally, we suggest that future research should evaluate the impact of each improved crop/livestock technology independently in order to provide a more detailed and technology-specific policy implication.
Availability of data and materials
It is possible to access the data on personal request from the principal investigator.
Abbreviations
Agricultural development led industrialization
Average treatment effect on the treated
Agricultural technical and vocational education and training
Conditional independence assumption
Central statistical authority
Development agents
Difference-in-difference
Ethiopian institute of agricultural research
Focused group discussions
Farmers’ training centers
Gross domestic product
Growth and transformation plan
Likelihood ratio
Meters above sea level
Non-Governmental Organizations
Nearest neighbor
Degree celsius
- Propensity score matching
Productive safety net program
Regional agricultural research institutes
Randomized control trials
Sub-saharan africa
Soil and water conservation
Tropical livestock unit
Beegle K, Christiaensen L, Dabalen A, Gaddis I. Poverty in a rising Africa. Washington, DC: The World Bank; 2016.
Book Google Scholar
Agricultural Transformation Agency (ATA). 2017. ATA Annual Report 2017/18. http://www.ata.gov.et/wp-content/uploads/2019/01/ATA_AnnualReport_2010.pdf [Accessed on Nov. 2019]
Biru WD, Zeller M, Loos TK. The impact of agricultural technologies on poverty and vulnerability of smallholders in Ethiopia: a panel data analysis. Social Indicators Res. 2020;147:517–44. https://doi.org/10.1007/s11205-019-02166-0 .
Article Google Scholar
Gregory, PJ. Paul Dorosh and Shahidur Rashid eds. 2013. Food and agriculture in Ethiopia—progress and policy challenges. Food Security 5, 475–476 (2013). https://doi.org/ https://doi.org/10.1007/s12571-013-0259-7
Abebe ZD, Sewnet MA. Adoption of soil conservation practices in North Achefer District, Northwest Ethiopia. Chinese J Population Resources Environ. 2014;12(3):261–8.
Mignouna DB, Manyong VM, Rusike J, Mutabazi KDS, Senkondo EM. Determinants of adopting Imazapyr-resistant maize technologies and its impact on household income in western Kenya. AgBioForum. 2011;14(3):158–63.
Google Scholar
Khonje M, Manda J, Alene AD, Kassie M. Analysis of adoption and impacts of improved maize varieties in eastern Zambia. World Dev. 2015;66(2015):695–706.
Makate C, Wang R, Makate M, Mango N. Impact of drought tolerant maize adoption on maize productivity, sales and consumption in rural Zimbabwe. Agrekon. 2017;56(1):67–81. https://doi.org/10.1080/03031853.2017.1283241 .
Houeninvo GH, Quenum CVC, Nonvide GMA. Impact of improved maize variety adoption on smallholder farmers’ welfare in Benin. Econ Innovation New Technol. 2019. https://doi.org/10.1080/10438599.2019.1669331 .
Bahta YT, Owusu-Sekyere E, Tlalang BE. Assessing participation in homestead food garden programmes, land ownership and their impact on productivity and net returns of smallholder maize producers in South Africa. Agrekon. 2018. https://doi.org/10.1080/03031853.2018.1437051 .
Kassie M, Shiferaw B, Muricho G. Agricultural technology, crop income, and poverty alleviation in Uganda. World Dev. 2011;39(10):1784–95.
Kabunga NS, Dubois T, Qaim M. Impact of tissue culture banana technology on farm household income and food security in Kenya. Food Policy. 2014;45(2014):25–34.
Tesfaye S, Bedada B, Mesay Y. 2016. Impact of improved wheat technology adoption on productivity and income In Ethiopia. African Crop Sci J 24 (Issue Supplementt s1), 127–135. https://doi.org/10.4314/acsj.v24i1.14S
Wake RD, Habteyesus DG. Impact of high yielding wheat varieties adoption on farm income of smallholder farmers in Ethiopia. Int J Agri Extension. 2019;07(01):45–59.
Irene Bayiyana, Aloyce Hepelwa, Elizaphan J.O. Rao & Kenneth Mdadila .2018. Do Dairy Market Hubs improve smallholder farmers’ income? The case of dairy farmers in the Tanga and Morogoro regions of Tanzania. Agrekon https://doi.org/10.1080/03031853.2018.1481758
Habiyaremye A. Estimating the impact of sericulture adoption on farmer income in Rwanda: an application of propensity score matching. Agrekon. 2017;56(3):296–311. https://doi.org/10.1080/03031853.2017.1361853 .
Akinola AA, Sofoluwe NA. Impact of mulching technology adoption on output and net return to yam farmers in Osun State. Nigeria Agrekon. 2012;51(2):75–92. https://doi.org/10.1080/03031853.2012.695144 .
Jama N, Kuntashula E, Samboko PC. Adoption and impact of the improved fallow technique on cotton productivity and income in Zambia. Sustainable Agric Res. 2019;8(2):1–19.
Cunguara B, Darnhofer I. Assessing the impact of improved agricultural technologies on household income in rural Mozambique. Food Policy. 2011;36(2011):378–90.
Habtemariam LT, Mgeni CP, Mutabazi KD, Sieber S. The fam income and food security implications of adopting fertilizer micro-dosing and tied-ridge technologies under semi-arid environments in central Tanzania. J Arid Environ. 2019;166(2019):60–7.
Teklewold H, Kassie M, Shiferaw B, Köhlin G. Cropping system diversification, conservation tillage and modern seed adoption in Ethiopia: Impacts on household income, agrochemical use and demand for labor. Ecol Econ. 2013;93(2013):85–93.
Hailu BK, Abrha BK, Weldegiorgis KA. Adoption and impact of agricultural technologies on farm income: evidence from southern Tigray, Northern Ethiopia. Int J Food Agric Econ. 2014;2(4):91–106.
Heckman JJ, LaLonde RJ, Smith JA. The economics and econometrics of active labour market programs. Handbook of Labour Economics. 1999;3:1865–2097.
Martey E, Kuwornu JK, Adjebeng-Danquah J. Estimating the effect of mineral fertilizer use on land productivity and income: evidence from Ghana. Land Use Policy. 2019;85:463–75.
Matere SJ, Busienei JR, Mbatia OE. Adoption and impact of improved pigeon peas on farming household poverty in semi-arid south east KENYA. J Poverty, Investment Develop. 2020;5(1):36–48.
OjoBaiyegunhi TOLJS. Determinants of climate change adaptation strategies and its impact on the net farm income of rice farmers in south-west Nigeria. Land Use Policy. 2020;95:103946.
Khandker, S. R., Koolwal, G. B., & Samad, H. A. 2010. Handbook on impact evaluation: quantitative methods and practices. World Bank Publications.
Rosenbaum PR, Rubin DB. Constructing a control group using multivariate matched sampling methods that incorporate the propensity score. Am Stat. 1985;39(1):33–8.
Heckman JJ, Robb R. Alternative methods for evaluating the impact of interventions: An overview. J Econometrics. 1985;30(1):239–67.
Lechner M. Earnings and employment effects of continuous Gff-the-Job training in East Germany after Unification. J Business Econ Stat. 1999;17(1):74–90.
Caliendo M, Kopeinig S. Some practical guidance for the implementation of propensity score matching. J Econ Surveys. 2008;22(1):31–72.
Sianesi B. An evaluation of the Swedish system of active labour market programs in the 1990s. Rev Econ Stat. 2004;86(1):133–55.
Belachew A, Mekuria W, Nachimuthu K. Factors influencing adoption of soil and water conservation practices in the northwest Ethiopian highlands. Int Soil Water Conserv Res. 2020;8(2020):80–9. https://doi.org/10.1016/j.iswcr.2020.01.005 .
Mwungu, C.M., Shikuku, K.M., Kinyua, I. and Mwongera, C. 2019. Impact of adopting prioritized climate-smart agricultural technologies on farm income and labor use in rural Tanzania. Invited paper presented at the 6th African Conference of Agricultural Economists, September 23–26, 2019, Abuja, Nigeria.
Tesfay MG. Does fertilizer adoption enhance smallholders’ commercialization? An endogenous switching regression model from northern Ethiopia. Agric Food Secur. 2020;9:3. https://doi.org/10.1186/s40066-020-0256-y .
Gezu D, Gebisa B, Mohammed J, Fikadu T, Habte B. Effects of intra-row spacing and N fertilizer rates on yield and yield components of tomato (Lycopersicon Esculentum L.) at Harawe, Eastern Ethiopia. J Plant Sci. 2019;7(1):8–12.
Wordofa MG, Sassi M. Impact of farmers’ training centers on household income: evidence from propensity score matching in eastern Ethiopia. Social Sci. 2018;7(1):1–12. https://doi.org/10.3390/socsci7010004 .
Varma P. Adoption and the impact of system of rice intensification on rice yields and household income: an analysis for India. Appl Econ. 2019;51(45):4956–72.
Winters P, Maffioli A, Salazar L. Introduction to the special feature: evaluating the impact of agricultural projects in developing countries. J Agric Econ. 2011;62(2):393–402.
Doss CR, Morris ML. How does gender affect the adoption of agricultural innovations? The case of improved maize technology in Ghana. Agric Econ. 2000;25(1):27–39. https://doi.org/10.1111/j.1574-0862.2001.tb00233.x .
Gebre GG, Isoda H, Rahut DB, Amekawa Y, Nomura H. Gender differences in the adoption of agricultural technology: The case of improved maize varieties in southern Ethiopia. Women’s Stud Int. 2019;76:102264. https://doi.org/10.1016/j.wsif.2019.102264 .
Rola-Rubzen MF, Paris T, Hawkins J, Sapkota B. Improving gender participation in agricultural technology adoption in Asia: from rhetoric to practical action. Appl Economic Perspectives Policy. 2020;42(1):113–25. https://doi.org/10.1002/aepp.13011 .
Yishay AB, Jones M, Kondylis F, Mobarak AM. Gender gaps in technology diffusion. J Dev Econ. 2020;143(2020):102380. https://doi.org/10.1016/j.jdeveco.2019.102380 .
Roy AD. Some thoughts on the distribution of earnings. Oxford Economic Papers. 1951;3(2):135–46.
Zingiro A, Okello JJ, Guthiga PM. Assessment of adoption and impact of rainwater harvesting technologies on rural farm household income: the case of rainwater harvesting ponds in Rwanda. Environ Dev Sustain. 2014;16(6):1281–98.
Rubin DB. Estimating causal effects of treatments in randomized and nonrandomized studies. J Edu Psychol. 1974;66(5):688–701.
Central Statistical Agency (CSA). Population Projection of Ethiopia for All Regions at Wereda Level from 2014–2017. Ethiopia: Addis Ababa; 2013.
Mengistu K, Degefu K. Adoption intensity of inorganic fertilizers in maize production: empirical evidence from smallholder farmers in eastern Ethiopia. J Agric Sci. 2017;9(5):124–32.
Nigussie D, Kibebew K, Bosena T, Tesfaye L, Mengistu K and Feyisa H. 2018. Innovations in Technology, Institutional and Extension Approaches towards Sustainable Agriculture and Enhanced Food and Nutrition Security in Africa. Ethiopia.
Cavatassi R, Lipper L, Narloch U. Modern variety adoption and risk management in drought prone areas: insights from the sorghum farmers of eastern Ethiopia. Agric Econ. 2011;42(3):279–92.
Dillon A, K., McGee & G., Oseni. . Agricultural production, dietary diversity and climate variability. J Develop Stud. 2015;51(8):976–95. https://doi.org/10.1080/00220388.2015.1018902 .
Makate C, Makate M, Mango N, Siziba S. Increasing resilience of smallholder farmers to climate change through multiple adoption of proven climate-smart agriculture innovations. Lessons from Southern Africa. J Environ Manage. 2019;231:858–68.
Nata JT, Mjelde JW, Boadu FO. Household adoption of soil-improving practices and food insecurity in Ghana. Agric Food Security. 2014;3(1):17.
Tayib M, Mezene W, Tadesse B, Adem H. Camel Trypanosomiasis in Babile District, Eastern Hararghe Zone, Oromia Regional State, Eastern Ethiopia. Academic J Anim Dis. 2015;4(3):141–5.
Download references
Acknowledgements
We would like to thank the three anonymous reviewers for their invaluable comments and suggestions. We also thank our respondents, enumerators and field supervisors for their dedication and patience throughout the research process. Staff of the Office of Research Affairs and Finance Division of Haramaya University are also appreciated for their smooth facilitation of the research.
Haramaya University provided funding to design the study and carry out data collection, analysis, interpretation of data as well as writing the manuscript.
Author information
Authors and affiliations.
College of Agriculture and Environmental Sciences, Department of Rural Development and Agricultural Extension, Haramaya University, P.O.Box 138, Dire Dawa, Ethiopia
Muluken G. Wordofa, Jemal Y. Hassen, Getachew S. Endris, Chanyalew S. Aweke & Dereje K. Moges
Department of Rural Development and Agricultural Extension, Arsi University, Assela, Ethiopia
Debbebe T. Rorisa
You can also search for this author in PubMed Google Scholar
Contributions
MGW designed the study, analyzed the data and wrote the paper. GSE supervised data collection and analyzed qualitative data. CSA contributed in the design, implementation and analysis of the research. DKM designed the questionnaire and helped in data collection. JYH designed the conceptual ideas of study and its implementation. DTR collected and analyzed data. All authors read and approved the final manuscript.
Corresponding author
Correspondence to Muluken G. Wordofa .
Ethics declarations
Ethics approval and consent to participate.
Not applicable.
Consent for publication
Competing interests.
The authors declare that they have no competing interests.
Additional information
Publisher's note.
Springer Nature remains neutral with regard to jurisdictional claims in published maps and institutional affiliations.
Rights and permissions
Open Access This article is licensed under a Creative Commons Attribution 4.0 International License, which permits use, sharing, adaptation, distribution and reproduction in any medium or format, as long as you give appropriate credit to the original author(s) and the source, provide a link to the Creative Commons licence, and indicate if changes were made. The images or other third party material in this article are included in the article's Creative Commons licence, unless indicated otherwise in a credit line to the material. If material is not included in the article's Creative Commons licence and your intended use is not permitted by statutory regulation or exceeds the permitted use, you will need to obtain permission directly from the copyright holder. To view a copy of this licence, visit http://creativecommons.org/licenses/by/4.0/ . The Creative Commons Public Domain Dedication waiver ( http://creativecommons.org/publicdomain/zero/1.0/ ) applies to the data made available in this article, unless otherwise stated in a credit line to the data.
Reprints and permissions
About this article
Cite this article.
Wordofa, M.G., Hassen, J.Y., Endris, G.S. et al. Adoption of improved agricultural technology and its impact on household income: a propensity score matching estimation in eastern Ethiopia. Agric & Food Secur 10 , 5 (2021). https://doi.org/10.1186/s40066-020-00278-2
Download citation
Received : 23 April 2020
Accepted : 30 October 2020
Published : 08 February 2021
DOI : https://doi.org/10.1186/s40066-020-00278-2
Share this article
Anyone you share the following link with will be able to read this content:
Sorry, a shareable link is not currently available for this article.
Provided by the Springer Nature SharedIt content-sharing initiative
- Agricultural technologies
- Farm income
Agriculture & Food Security
ISSN: 2048-7010
- Submission enquiries: [email protected]
- General enquiries: [email protected]
The impact of environmental regulation and economic expectations on crop-livestock integration among hog farmers: a field study from China
- Research Article
- Published: 01 June 2024
Cite this article
- Jing Cao 1 ,
- Jiapeng Xu 1 ,
- Huimin Cao 1 ,
- Fangfang Wang 1 ,
- Zhenyu Yan ORCID: orcid.org/0000-0002-3154-9763 1 &
- Taimoor Muhammad 1
Decoupling of crop-livestock systems increases the risks of pollution, waste of nutrient resources, and biodiversity loss. Crop-livestock integration (CLI) is an effective solution to these problems, and motivating farmers to adopt CLI is the key. Many countries have implemented environmental regulations (ER) aiming to influence farmers’ CLI adoption decisions. Based on a field study of 316 hog farmers from Shaanxi Province of China, this paper applies the triple-hurdle model to empirically examine the impacts of economic expectations (EE) and ER on CLI adoption decisions. It also verifies the income effect of CLI. The results are as follows: 90.5% of farmers are willing to adopt CLI, but the adoption rate is only 40.8% and the average integration degree is only 0.236; CLI not been widely popularized. EE and ER promote farmers’ CLI adoption significantly, while the impact of interaction between EE and ER on CLI adoption differs. IER weakens the positive impact of EE on farmers’ CLI integration degree, which has a “crowding out effect.” GER negatively moderates the impact of EE on farmers’ adoption willingness of CLI. CER strengthens the positive effect of EE on farmers’ adoption behavior and CLI integration degree. CLI increases the farmers’ income. These results contribute to our understanding of the mechanisms of CLI adoption decisions and sustainable policy optimization for green agricultural development.
This is a preview of subscription content, log in via an institution to check access.
Access this article
Price includes VAT (Russian Federation)
Instant access to the full article PDF.
Rent this article via DeepDyve
Institutional subscriptions
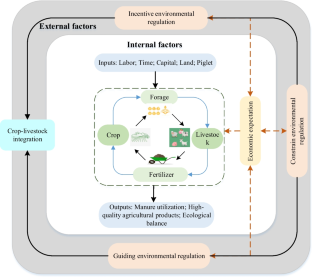
Data availability
All data needed to evaluate the conclusions in the paper are present in the paper.
When testing for sample selection errors, IMR was not significant. Therefore, only the test results of IMR are explained here, and the overall regression results are not reported (Models 1–8).
Asai M, Moraine M, Ryschawy J et al (2018) Critical factors for crop-livestock integration beyond the farm level: a cross-analysis of worldwide case studies. Land Use Policy 73:184–194. https://doi.org/10.1016/j.landusepol.2017.12.010
Article Google Scholar
Bai Z, Ma L, Jin S et al (2016) Nitrogen, phosphorus, and potassium flows through the manure management chain in China. Environ Sci Technol 50:13409–13418. https://doi.org/10.1021/acs.est.6b03348
Article CAS Google Scholar
Bai Z, Ma W, Ma L et al (2018) China’s livestock transition: driving forces, impacts, and consequences. Sci Adv 4:eaar8534. https://doi.org/10.1126/sciadv.aar8534
Bai Z, Fan X, Jin X et al (2022) Relocate 10 billion livestock to reduce harmful nitrogen pollution exposure for 90% of China’s population. Nat Food 3:152–160. https://doi.org/10.1038/s43016-021-00453-z
Bao W, Wu Y, Bao H (2024) Transaction costs, crop-livestock integration participation, and income effects in China. Front Sustain Food Syst 7:1247770. https://doi.org/10.3389/fsufs.2023.1247770
Barnes AP, Willock J, Hall C, Toma L (2009) Farmer perspectives and practices regarding water pollution control programmes in Scotland. Agric Water Manag 96:1715–1722. https://doi.org/10.1016/j.agwat.2009.07.002
Belay DG, Jensen JD (2020) ‘The scarlet letters’: information disclosure and self-regulation: evidence from antibiotic use in Denmark. J Environ Econ Manag 104:102385. https://doi.org/10.1016/j.jeem.2020.102385
Bell LW, Moore AD (2012) Integrated crop–livestock systems in Australian agriculture: trends, drivers and implications. Agric Syst 111:1–12. https://doi.org/10.1016/j.agsy.2012.04.003
Branca G, Perelli C (2020) ‘Clearing the air’: common drivers of climate-smart smallholder food production in Eastern and Southern Africa. J Clean Prod 270:121900. https://doi.org/10.1016/j.jclepro.2020.121900
Burke WJ, Myers RJ, Jayne TS (2015) A triple-hurdle model of production and market participation in Kenya’s dairy market. Am J Agric Econ 97:1227–1246. https://doi.org/10.1093/ajae/aav009
Cao D, Li H, Wang G, Huang T (2017) Identifying and contextualising the motivations for BIM implementation in construction projects: an empirical study in China. Int J Proj Manag 35:658–669. https://doi.org/10.1016/j.ijproman.2016.02.002
Carrer MJ, Maia AG, de Mello BrandãoVinholis M, de Souza Filho HM (2020) Assessing the effectiveness of rural credit policy on the adoption of integrated crop-livestock systems in Brazil. Land Use Policy 92:104468. https://doi.org/10.1016/j.landusepol.2020.104468
Chen Q, Xu Q, Yu X (2023) Triple-hurdle model analysis of aquaculture farmers’ multi-stage willingness to participate in green and healthy aquaculture actions in China: based on ecological cognition and environmental regulation perspectives. Front Sustain Food Syst 7:1211392. https://doi.org/10.3389/fsufs.2023.1211392
Cheng P, Li J, Zhang H, Cheng G (2023) Sustainable management behavior of farmland shelterbelt of farmers in ecologically fragile areas: empirical evidence from Xinjiang, China. Sustainability 15:2011. https://doi.org/10.3390/su15032011
Cortner O, Garrett RD, Valentim JF et al (2019) Perceptions of integrated crop-livestock systems for sustainable intensification in the Brazilian Amazon. Land Use Policy 82:841–853. https://doi.org/10.1016/j.landusepol.2019.01.006
Cui G, Liu Z (2022) The impact of environmental regulations and social norms on farmers’ chemical fertilizer reduction behaviors: an investigation of citrus farmers in southern China. Sustainability 14:8157. https://doi.org/10.3390/su14138157
de Groot JIM, Steg L (2009) Mean or green: which values can promote stable pro-environmental behavior? Conserv Lett 2:61–66. https://doi.org/10.1111/j.1755-263X.2009.00048.x
Dessart FJ, Barreiro-Hurlé J, Van Bavel R (2019) Behavioural factors affecting the adoption of sustainable farming practices: a policy-oriented review. Eur Rev Agric Econ 46:417–471. https://doi.org/10.1093/erae/jbz019
Dos Reis JC, Kamoi MYT, Latorraca D et al (2020) Assessing the economic viability of integrated crop−livestock systems in Mato Grosso, Brazil. Renew Agric Food Syst 35:631–642. https://doi.org/10.1017/S1742170519000280
dos Reis JC, Rodrigues GS, de Barros I et al (2021) Integrated crop-livestock systems: a sustainable land-use alternative for food production in the Brazilian Cerrado and Amazon. J Clean Prod 283:124580. https://doi.org/10.1016/j.jclepro.2020.124580
Du S, Liu J, Fu Z (2021a) The impact of village rules and formal environmental regulations on farmers’ cleaner production behavior: new evidence from China. Int J Environ Res Public Health 18:7311. https://doi.org/10.3390/ijerph18147311
Du S, Luo X, Huang Y et al (2021b) Risk perception, specialized agricultural services and rice farmers’ adoption behavior of biological pesticide technology. Resour Environ Yangtze Basin 30:1768–1779
Google Scholar
Finger R, Lehmann N (2012) Policy reforms to promote efficient and sustainable water use in Swiss agriculture. Water Policy 14:887–901. https://doi.org/10.2166/wp.2012.152
Garrett R, Niles M, Gil J et al (2017) Policies for reintegrating crop and livestock systems: a comparative analysis. Sustainability 9:473. https://doi.org/10.3390/su9030473
Garrett R, Ryschawy J, Bell L et al (2020) Drivers of decoupling and recoupling of crop and livestock systems at farm and territorial scales. Ecol Soc 25. https://doi.org/10.5751/ES-11412-250124
Gil JDB, Garrett R, Berger T (2016) Determinants of crop-livestock integration in Brazil: evidence from the household and regional levels. Land Use Policy 59:557–568. https://doi.org/10.1016/j.landusepol.2016.09.022
Gil JDB, Garrett RD, Rotz A et al (2018) Tradeoffs in the quest for climate smart agricultural intensification in Mato Grosso. Brazil Environ Res Lett 13:064025. https://doi.org/10.1088/1748-9326/aac4d1
Goodhue RE, Mohapatra S, Rausser GC (2010) Interactions between incentive instruments: contracts and quality in processing tomatoes. Am J Agric Econ 92:1283–1293. https://doi.org/10.1093/ajae/aaq061
Gu B (2022) Recoupling livestock and crops. Nat Food 3:102–103. https://doi.org/10.1038/s43016-022-00466-2
Guo Q, Li H, Li S, Nan L (2021) Research on farmers’ pro-environmental behavior from the perspective of paradox existing between behavior and willingness: taking the organic fertilizers application as an example. Resour Environ Yangtze Basin 30:212–224
Guo Z, Chen X, Zhang Y (2022) Impact of environmental regulation perception on farmers’ agricultural green production technology adoption: a new perspective of social capital. Technol Soc 71:102085. https://doi.org/10.1016/j.techsoc.2022.102085
Guo Q (2021) The fading relationship between agriculture and animal husbandry and its reconstruction. Chin Rural Econ 22–35. https://kns.cnki.net/kcms/detail/11.1262.F.20211011.0858.004.html
Hamazakaza P, Kabwe G, Kuntashula E et al (2022) Adoption of sustainable agriculture intensification in maize-based farming systems of Katete District in Zambia. Land 11:880. https://doi.org/10.3390/land11060880
Han Z, Han C, Shi Z et al (2023) Rebuilding the crop-livestock integration system in China ——based on the perspective of circular economy. J Clean Prod 393:136347. https://doi.org/10.1016/j.jclepro.2023.136347
Harper JK, Roth GW, Garalejić B, Škrbić N (2018) Programs to promote adoption of conservation tillage: a Serbian case study. Land Use Policy 78:295–302. https://doi.org/10.1016/j.landusepol.2018.06.028
Ji C, Jin S, Wang H, Ye C (2019) Estimating effects of cooperative membership on farmers’ safe production behaviors: evidence from pig sector in China. Food Policy 83:231–245. https://doi.org/10.1016/j.foodpol.2019.01.007
Jin S, Zhang B, Wu B et al (2020) Decoupling livestock and crop production at the household level in China. Nat Sustain 4:48–55. https://doi.org/10.1038/s41893-020-00596-0
Kaczan DJ, Swallow BM, Adamowicz WL (Vic) (2019) Forest conservation policy and motivational crowding: experimental evidence from Tanzania. Ecol Econ 156:444–453. https://doi.org/10.1016/j.ecolecon.2016.07.002
Kelifa A (2023) Review of Tobit, Heckman and double hurdle econometric models: supported with evidences from the studies conducted in Ethiopia. SN Bus Econ 3:104. https://doi.org/10.1007/s43546-023-00478-5
Khed VD, Krishna VV (2023) Agency and time poverty: linking decision-making powers and leisure time of male and female farmers of Central India. World Dev Perspect 29:100484. https://doi.org/10.1016/j.wdp.2022.100484
Knight J, Deng Q, Li S (2011) The puzzle of migrant labour shortage and rural labour surplus in China, China. Econ Rev 22:585–600. https://doi.org/10.1016/j.chieco.2011.01.006
Li Y, Wang B (2022) Environmental motivation or economic motivation? Explaining individuals’ intention to carry reusable bags for shopping in China. Front Psychol 13:972748. https://doi.org/10.3389/fpsyg.2022.972748
Li F, Ren J, Wimmer S et al (2020) Incentive mechanism for promoting farmers to plant green manure in China. J Clean Prod 267:122197. https://doi.org/10.1016/j.jclepro.2020.122197
Li Y, Sun Z, Accatino F et al (2021) Comparing specialised crop and integrated crop-livestock systems in China with a multi-criteria approach using the emergy method. J Clean Prod 314:127974. https://doi.org/10.1016/j.jclepro.2021.127974
Liu Z, Sun J, Zhu W, Qu Y (2021) Exploring impacts of perceived value and government regulation on farmers’ willingness to adopt wheat straw incorporation in China. Land 10:1051. https://doi.org/10.3390/land10101051
Lu Y, Tan Y, Wang H (2022) Impact of environmental regulation on green technology adoption by farmers microscopic investigation evidence from pig breeding in China. Front Environ Sci 10:885933. https://doi.org/10.3389/fenvs.2022.885933
Ma W, Abdulai A (2016) Does cooperative membership improve household welfare? Evidence from apple farmers in China. Food Policy 58:94–102. https://doi.org/10.1016/j.foodpol.2015.12.002
Ma L, Bai Z, Ma W et al (2019) Exploring future food provision scenarios for China. Environ Sci Technol 53:1385–1393. https://doi.org/10.1021/acs.est.8b04375
Martin G, Moraine M, Ryschawy J et al (2016) Crop–livestock integration beyond the farm level: a review. Agron Sustain Dev 36:53. https://doi.org/10.1007/s13593-016-0390-x
Mather DL, Jayne TS (2018) Fertilizer subsidies and the role of targeting in crowding out: evidence from Kenya. Food Secur 10:397–417. https://doi.org/10.1007/s12571-018-0773-8
Meraner M, Finger R (2019) Risk perceptions, preferences and management strategies: evidence from a case study using German livestock farmers. J Risk Res 22:110–135. https://doi.org/10.1080/13669877.2017.1351476
Midler E, Pascual U, Drucker AG et al (2015) Unraveling the effects of payments for ecosystem services on motivations for collective action. Ecol Econ 120:394–405. https://doi.org/10.1016/j.ecolecon.2015.04.006
Missiame A, Nyikal RA, Irungu P (2021) What is the impact of rural bank credit access on the technical efficiency of smallholder cassava farmers in Ghana? An endogenous switching regression analysis. Heliyon 7:e07102. https://doi.org/10.1016/j.heliyon.2021.e07102
Moraine M, Duru M, Nicholas P et al (2014) Farming system design for innovative crop-livestock integration in Europe. Anim Int J Anim Biosci 8:1204–1217. https://doi.org/10.1017/S1751731114001189
Niles MT, Garrett RD, Walsh D (2017) Ecological and economic benefits of integrating sheep into viticulture production. Agron Sustain Dev 38:1. https://doi.org/10.1007/s13593-017-0478-y
Pelletier LG, Tuson KM, Haddad NK (1997) Client motivation for therapy scale: a measure of intrinsic motivation, extrinsic motivation, and amotivation for therapy. J Pers Assess 68:414–435. https://doi.org/10.1207/s15327752jpa6802_11
Peterson CA, Deiss L, Gaudin ACM (2020) Commercial integrated crop-livestock systems achieve comparable crop yields to specialized production systems: a meta-analysis. PLoS One 15:e0231840. https://doi.org/10.1371/journal.pone.0231840
Poeschl M, Ward S, Owende P (2012) Environmental impacts of biogas deployment – part II: life cycle assessment of multiple production and utilization pathways. J Clean Prod 24:184–201. https://doi.org/10.1016/j.jclepro.2011.10.030
Prokopy LS, Floress K, Arbuckle JG et al (2019) Adoption of agricultural conservation practices in the United States: evidence from 35 years of quantitative literature. J Soil Water Conserv 74:520–534. https://doi.org/10.2489/jswc.74.5.520
Regan JT, Marton S, Barrantes O et al (2017) Does the recoupling of dairy and crop production via cooperation between farms generate environmental benefits? A case-study approach in Europe. Eur J Agron 82:342–356. https://doi.org/10.1016/j.eja.2016.08.005
Ryschawy J, Grillot M, Charmeau A et al (2022) A participatory approach based on the serious game Dynamix to co-design scenarios of crop-livestock integration among farms. Agric Syst 201:103414. https://doi.org/10.1016/j.agsy.2022.103414
Schut AGT, Cooledge EC, Moraine M et al (2021) Reintegration of crop-livestock systems in Europe: an overview. Front Agric Sci Eng 8:111. https://doi.org/10.15302/J-FASE-2020373
Shi H, Sui D, Wu H, Zhao M (2018a) The influence of social capital on farmers’ participation in watershed ecological management behavior: evidence from Heihe Basin. Chin Rural Econ 34–45. https://kns.cnki.net/kcms/detail/11.1262.F.20180201.1801.008.html
Shi Y, Yao L, Zhao M (2018b) The effect of social capital on herds men’s participation willingness in grassland community governance: an analysis based on triple-hurdle model. China Rural Surv 35–50. https://kns.cnki.net/kcms/detail/11.3586.F.20180507.1530.006.html
Shi ZH, Zhang H (2021) Research on social norms, environmental regulations and farmers’ choice of fertilization behavior. Chin J Agric Resour Reg Plan 42:51–61. https://doi.org/10.7621/cjarrp.1005-9121.20211107
Skaalsveen K, Ingram J, Urquhart J (2020) The role of farmers’ social networks in the implementation of no-till farming practices. Agric Syst 181:102824. https://doi.org/10.1016/j.agsy.2020.102824
Sneessens I, Veysset P, Benoit M et al (2016) Direct and indirect impacts of crop–livestock organization on mixed crop–livestock systems sustainability: a model-based study. Animal 10:1911–1922. https://doi.org/10.1017/S1751731116000720
Tan K, Cai G, Du Z et al (2023) Emergy synthesis of decoupling and recoupling crop-livestock systems under unified system boundary and modified indices. Sci Total Environ 877:162880. https://doi.org/10.1016/j.scitotenv.2023.162880
Trujillo-Barrera A, Pennings JME, Hofenk D (2016) Understanding producers’ motives for adopting sustainable practices: the role of expected rewards, risk perception and risk tolerance. Eur Rev Agric Econ 43:359–382. https://doi.org/10.1093/erae/jbv038
Wang X, Tan S (2020) Cost-efficiency analysis of rice-crayfish integrated land operation mode based on non-homogeneous DEA. China Land Sci 34:56–63. https://doi.org/10.11994/zgtdkx.20191225.105326
Wang TX, Teng CG, Zhang ZH (2020) Informal social support, environmental regulation and farmers’ film recycling behavior. J Arid Land Resour Environ 34:109–115. https://doi.org/10.13448/j.cnki.jalre.2020.218
Wang L, Song B, Wu J, Wang L (2022) An empirical study on the influence of environmental regulation and farmers’ cognition on the application behavior of organic fertilizer: based on the survey data of 741 farmers in YE county, PINGDINGSHAN city, Henan province. J Henan Univ Technol Soc Sci 38:57–67. https://doi.org/10.16433/j.cnki.cn41-1379.2022.03.014
Willett W, Rockström J, Loken B et al (2019) Food in the Anthropocene: the EAT–Lancet Commission on healthy diets from sustainable food systems. Lancet 393:447–492. https://doi.org/10.1016/S0140-6736(18)31788-4
Yang C, Liang X, Xue Y et al (2024) Can government regulation weak the gap between green production intention and behavior? Based on the perspective of farmers’ perceptions. J Clean Prod 434:139743. https://doi.org/10.1016/j.jclepro.2023.139743
Yang X, Qi Z (2022) The impact of expected return and technology subsidy on farmers’ adoption of agroecological technology: taking rice–crayfish co–culture technology as an example. J Huazhong Agric Univ Sci Ed 89–100. https://doi.org/10.13300/j.cnki.hnwkxb.2022.05.010
Zhang J, Chen M, Huang C, Lai Z (2022) Labor endowment, cultivated land fragmentation, and ecological farming adoption strategies among farmers in Jiangxi Province, China. Land 11:679. https://doi.org/10.3390/land11050679
Zhao J, Liu L, Qi J, Dong J (2022) Study on the influence of environmental regulation on the environmentally friendly behavior of farmers in China. Front Environ Sci 10:1009151. https://doi.org/10.3389/fenvs.2022.1009151
Zhao Y, Liu L, Zhao J (2023) Perceived benefits, environmental regulation and farmers ’ waste recycling behavior-taking duck farmers as an example. World Agric 98–110. https://doi.org/10.13856/j.cn11-1097/s.2023.04.009
Zhu X, Cai J (2016) The influences of perceived values and capability approach on farmers willingness to exit rural residential land and its intergenerational difference. China Land Sci 30:64–72. https://doi.org/10.11994/zgtdkx.20161024.124548
Download references
This work was supported by Key Think Tank Research Project of Shaanxi Province on “Social Sciences Helping County Economies Develop in High Quality” (2023ZD0662); Humanities and Social Sciences Project of Fundamental Research Funds for the Central Universities in 2023 (452023307); Soft Science Research Program of Shaanxi Province (2022KRM032; 2023-CX-RKX-103); and Social Science Foundation of Shaanxi Province (2021D058; 2022D022). The authors would like to thank the anonymous referees for their helpful suggestions and corrections on the earlier draft of our paper.
Author information
Authors and affiliations.
College of Economics and Management, Northwest A&F University, No. 3 Taicheng Road, Yangling, 712100, Shaanxi, People’s Republic of China
Jing Cao, Jiapeng Xu, Huimin Cao, Fangfang Wang, Zhenyu Yan & Taimoor Muhammad
You can also search for this author in PubMed Google Scholar
Contributions
Jing Cao: methodology; writing—original draft; writing—review and editing.
Jiapeng Xu: conceptualization, supervision, project administration, article modification.
Huimin Cao: data, research methods.
Fangfang Wang: data, research methods.
Zhenyu Yan: conceptualization, supervision, project administration, article modification.
Taimoor Muhammad: data, research methods.
Corresponding author
Correspondence to Zhenyu Yan .
Ethics declarations
Ethics approval.
Not applicable.
Consent to participate
Consent for publication, competing interests.
The authors declare no competing interests.
Additional information
Responsible Editor: Baojing Gu
Publisher's Note
Springer Nature remains neutral with regard to jurisdictional claims in published maps and institutional affiliations.
Rights and permissions
Springer Nature or its licensor (e.g. a society or other partner) holds exclusive rights to this article under a publishing agreement with the author(s) or other rightsholder(s); author self-archiving of the accepted manuscript version of this article is solely governed by the terms of such publishing agreement and applicable law.
Reprints and permissions
About this article
Cao, J., Xu, J., Cao, H. et al. The impact of environmental regulation and economic expectations on crop-livestock integration among hog farmers: a field study from China. Environ Sci Pollut Res (2024). https://doi.org/10.1007/s11356-024-33616-z
Download citation
Received : 03 January 2024
Accepted : 05 May 2024
Published : 01 June 2024
DOI : https://doi.org/10.1007/s11356-024-33616-z
Share this article
Anyone you share the following link with will be able to read this content:
Sorry, a shareable link is not currently available for this article.
Provided by the Springer Nature SharedIt content-sharing initiative
- Crop-livestock integration
- Economic expectations
- Environmental regulation
- Triple-hurdle model
- Pig farmers
- Find a journal
- Publish with us
- Track your research
NTRS - NASA Technical Reports Server
Available downloads, related records.
The state of AI in early 2024: Gen AI adoption spikes and starts to generate value
If 2023 was the year the world discovered generative AI (gen AI) , 2024 is the year organizations truly began using—and deriving business value from—this new technology. In the latest McKinsey Global Survey on AI, 65 percent of respondents report that their organizations are regularly using gen AI, nearly double the percentage from our previous survey just ten months ago. Respondents’ expectations for gen AI’s impact remain as high as they were last year , with three-quarters predicting that gen AI will lead to significant or disruptive change in their industries in the years ahead.
About the authors
This article is a collaborative effort by Alex Singla , Alexander Sukharevsky , Lareina Yee , and Michael Chui , with Bryce Hall , representing views from QuantumBlack, AI by McKinsey, and McKinsey Digital.
Organizations are already seeing material benefits from gen AI use, reporting both cost decreases and revenue jumps in the business units deploying the technology. The survey also provides insights into the kinds of risks presented by gen AI—most notably, inaccuracy—as well as the emerging practices of top performers to mitigate those challenges and capture value.
AI adoption surges
Interest in generative AI has also brightened the spotlight on a broader set of AI capabilities. For the past six years, AI adoption by respondents’ organizations has hovered at about 50 percent. This year, the survey finds that adoption has jumped to 72 percent (Exhibit 1). And the interest is truly global in scope. Our 2023 survey found that AI adoption did not reach 66 percent in any region; however, this year more than two-thirds of respondents in nearly every region say their organizations are using AI. 1 Organizations based in Central and South America are the exception, with 58 percent of respondents working for organizations based in Central and South America reporting AI adoption. Looking by industry, the biggest increase in adoption can be found in professional services. 2 Includes respondents working for organizations focused on human resources, legal services, management consulting, market research, R&D, tax preparation, and training.
Also, responses suggest that companies are now using AI in more parts of the business. Half of respondents say their organizations have adopted AI in two or more business functions, up from less than a third of respondents in 2023 (Exhibit 2).
Gen AI adoption is most common in the functions where it can create the most value
Most respondents now report that their organizations—and they as individuals—are using gen AI. Sixty-five percent of respondents say their organizations are regularly using gen AI in at least one business function, up from one-third last year. The average organization using gen AI is doing so in two functions, most often in marketing and sales and in product and service development—two functions in which previous research determined that gen AI adoption could generate the most value 3 “ The economic potential of generative AI: The next productivity frontier ,” McKinsey, June 14, 2023. —as well as in IT (Exhibit 3). The biggest increase from 2023 is found in marketing and sales, where reported adoption has more than doubled. Yet across functions, only two use cases, both within marketing and sales, are reported by 15 percent or more of respondents.
Gen AI also is weaving its way into respondents’ personal lives. Compared with 2023, respondents are much more likely to be using gen AI at work and even more likely to be using gen AI both at work and in their personal lives (Exhibit 4). The survey finds upticks in gen AI use across all regions, with the largest increases in Asia–Pacific and Greater China. Respondents at the highest seniority levels, meanwhile, show larger jumps in the use of gen Al tools for work and outside of work compared with their midlevel-management peers. Looking at specific industries, respondents working in energy and materials and in professional services report the largest increase in gen AI use.
Investments in gen AI and analytical AI are beginning to create value
The latest survey also shows how different industries are budgeting for gen AI. Responses suggest that, in many industries, organizations are about equally as likely to be investing more than 5 percent of their digital budgets in gen AI as they are in nongenerative, analytical-AI solutions (Exhibit 5). Yet in most industries, larger shares of respondents report that their organizations spend more than 20 percent on analytical AI than on gen AI. Looking ahead, most respondents—67 percent—expect their organizations to invest more in AI over the next three years.
Where are those investments paying off? For the first time, our latest survey explored the value created by gen AI use by business function. The function in which the largest share of respondents report seeing cost decreases is human resources. Respondents most commonly report meaningful revenue increases (of more than 5 percent) in supply chain and inventory management (Exhibit 6). For analytical AI, respondents most often report seeing cost benefits in service operations—in line with what we found last year —as well as meaningful revenue increases from AI use in marketing and sales.
Inaccuracy: The most recognized and experienced risk of gen AI use
As businesses begin to see the benefits of gen AI, they’re also recognizing the diverse risks associated with the technology. These can range from data management risks such as data privacy, bias, or intellectual property (IP) infringement to model management risks, which tend to focus on inaccurate output or lack of explainability. A third big risk category is security and incorrect use.
Respondents to the latest survey are more likely than they were last year to say their organizations consider inaccuracy and IP infringement to be relevant to their use of gen AI, and about half continue to view cybersecurity as a risk (Exhibit 7).
Conversely, respondents are less likely than they were last year to say their organizations consider workforce and labor displacement to be relevant risks and are not increasing efforts to mitigate them.
In fact, inaccuracy— which can affect use cases across the gen AI value chain , ranging from customer journeys and summarization to coding and creative content—is the only risk that respondents are significantly more likely than last year to say their organizations are actively working to mitigate.
Some organizations have already experienced negative consequences from the use of gen AI, with 44 percent of respondents saying their organizations have experienced at least one consequence (Exhibit 8). Respondents most often report inaccuracy as a risk that has affected their organizations, followed by cybersecurity and explainability.
Our previous research has found that there are several elements of governance that can help in scaling gen AI use responsibly, yet few respondents report having these risk-related practices in place. 4 “ Implementing generative AI with speed and safety ,” McKinsey Quarterly , March 13, 2024. For example, just 18 percent say their organizations have an enterprise-wide council or board with the authority to make decisions involving responsible AI governance, and only one-third say gen AI risk awareness and risk mitigation controls are required skill sets for technical talent.
Bringing gen AI capabilities to bear
The latest survey also sought to understand how, and how quickly, organizations are deploying these new gen AI tools. We have found three archetypes for implementing gen AI solutions : takers use off-the-shelf, publicly available solutions; shapers customize those tools with proprietary data and systems; and makers develop their own foundation models from scratch. 5 “ Technology’s generational moment with generative AI: A CIO and CTO guide ,” McKinsey, July 11, 2023. Across most industries, the survey results suggest that organizations are finding off-the-shelf offerings applicable to their business needs—though many are pursuing opportunities to customize models or even develop their own (Exhibit 9). About half of reported gen AI uses within respondents’ business functions are utilizing off-the-shelf, publicly available models or tools, with little or no customization. Respondents in energy and materials, technology, and media and telecommunications are more likely to report significant customization or tuning of publicly available models or developing their own proprietary models to address specific business needs.
Respondents most often report that their organizations required one to four months from the start of a project to put gen AI into production, though the time it takes varies by business function (Exhibit 10). It also depends upon the approach for acquiring those capabilities. Not surprisingly, reported uses of highly customized or proprietary models are 1.5 times more likely than off-the-shelf, publicly available models to take five months or more to implement.
Gen AI high performers are excelling despite facing challenges
Gen AI is a new technology, and organizations are still early in the journey of pursuing its opportunities and scaling it across functions. So it’s little surprise that only a small subset of respondents (46 out of 876) report that a meaningful share of their organizations’ EBIT can be attributed to their deployment of gen AI. Still, these gen AI leaders are worth examining closely. These, after all, are the early movers, who already attribute more than 10 percent of their organizations’ EBIT to their use of gen AI. Forty-two percent of these high performers say more than 20 percent of their EBIT is attributable to their use of nongenerative, analytical AI, and they span industries and regions—though most are at organizations with less than $1 billion in annual revenue. The AI-related practices at these organizations can offer guidance to those looking to create value from gen AI adoption at their own organizations.
To start, gen AI high performers are using gen AI in more business functions—an average of three functions, while others average two. They, like other organizations, are most likely to use gen AI in marketing and sales and product or service development, but they’re much more likely than others to use gen AI solutions in risk, legal, and compliance; in strategy and corporate finance; and in supply chain and inventory management. They’re more than three times as likely as others to be using gen AI in activities ranging from processing of accounting documents and risk assessment to R&D testing and pricing and promotions. While, overall, about half of reported gen AI applications within business functions are utilizing publicly available models or tools, gen AI high performers are less likely to use those off-the-shelf options than to either implement significantly customized versions of those tools or to develop their own proprietary foundation models.
What else are these high performers doing differently? For one thing, they are paying more attention to gen-AI-related risks. Perhaps because they are further along on their journeys, they are more likely than others to say their organizations have experienced every negative consequence from gen AI we asked about, from cybersecurity and personal privacy to explainability and IP infringement. Given that, they are more likely than others to report that their organizations consider those risks, as well as regulatory compliance, environmental impacts, and political stability, to be relevant to their gen AI use, and they say they take steps to mitigate more risks than others do.
Gen AI high performers are also much more likely to say their organizations follow a set of risk-related best practices (Exhibit 11). For example, they are nearly twice as likely as others to involve the legal function and embed risk reviews early on in the development of gen AI solutions—that is, to “ shift left .” They’re also much more likely than others to employ a wide range of other best practices, from strategy-related practices to those related to scaling.
In addition to experiencing the risks of gen AI adoption, high performers have encountered other challenges that can serve as warnings to others (Exhibit 12). Seventy percent say they have experienced difficulties with data, including defining processes for data governance, developing the ability to quickly integrate data into AI models, and an insufficient amount of training data, highlighting the essential role that data play in capturing value. High performers are also more likely than others to report experiencing challenges with their operating models, such as implementing agile ways of working and effective sprint performance management.
About the research
The online survey was in the field from February 22 to March 5, 2024, and garnered responses from 1,363 participants representing the full range of regions, industries, company sizes, functional specialties, and tenures. Of those respondents, 981 said their organizations had adopted AI in at least one business function, and 878 said their organizations were regularly using gen AI in at least one function. To adjust for differences in response rates, the data are weighted by the contribution of each respondent’s nation to global GDP.
Alex Singla and Alexander Sukharevsky are global coleaders of QuantumBlack, AI by McKinsey, and senior partners in McKinsey’s Chicago and London offices, respectively; Lareina Yee is a senior partner in the Bay Area office, where Michael Chui , a McKinsey Global Institute partner, is a partner; and Bryce Hall is an associate partner in the Washington, DC, office.
They wish to thank Kaitlin Noe, Larry Kanter, Mallika Jhamb, and Shinjini Srivastava for their contributions to this work.
This article was edited by Heather Hanselman, a senior editor in McKinsey’s Atlanta office.
Explore a career with us
Related articles.

Moving past gen AI’s honeymoon phase: Seven hard truths for CIOs to get from pilot to scale
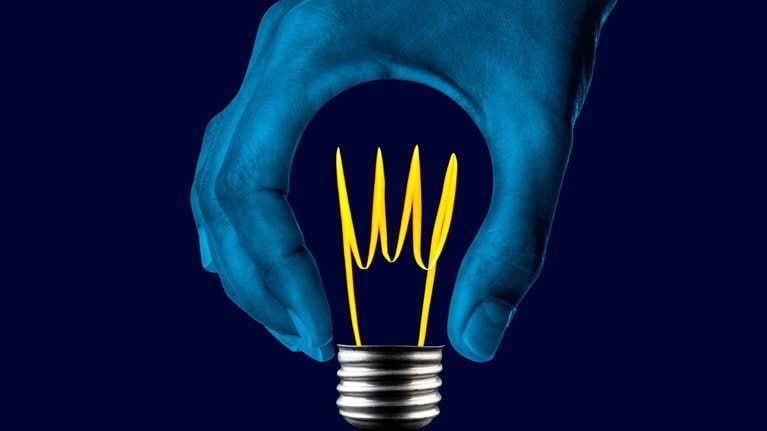
A generative AI reset: Rewiring to turn potential into value in 2024

Implementing generative AI with speed and safety
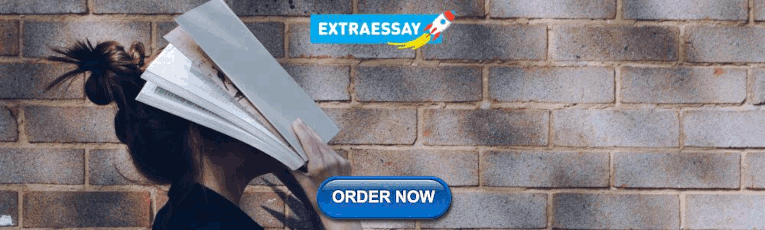
IMAGES
VIDEO
COMMENTS
The contribution of this paper to the empirical literature is threefold. ... we use the ESR model to examine the impact of new agricultural technology adoption on family farm operating income. ... Ni H, Liu ZM. Research on internet agricultural technology adoption behavior and influencing factors in family farm: Take 270 family farms in 9 ...
This paper presents an overview of smart agriculture, a strategy leveraging technology and data analytics, and its potential to advance sustainability socially, economically, and environmentally. Drawing on recent research, we examine the integration of smart technologies in agriculture, their impact on sustainability, and the architectural ...
Second, the environmental effects of agricultural technology adoption have attracted the attention of researchers and policymakers. The negative environmental impacts of technology and agricultural production are confirmed by Khan et al. (2021) for 19 EU (European union) countries in 1995-2019, Rehman et al. (2022) for
1. Introduction. The development and dissemination of novel agricultural technologies is seen as a way of enhancing productivity on the world's 475 million small (< 2 ha) farms, many of which are in low- and middle-income countries (Lowder, Skoet, & Raney, 2016).Development experts generally agree that the responsible development of agriculture is a key element in addressing, at least ...
The intersections in Figure 1 represent AI technology's potential impact on agriculture applications, Q2, ... When studying a research paper's relevance, bibliometric analysis can consider several indicators. ... E.L. Impacts of agricultural practices on subsurface microbial ecology. In Advances in Agronomy; Elsevier: Amsterdam, The ...
Originality/value. The paper shows the technological trend and progress in food and agriculture sector. Researchers focusing on agricultural economics and agribusiness should pay attention to recent developments in the real world, know the recent developments from other disciplines, get more data for empirical research and show the impact of digital agriculture on consumer's preference and ...
It places the digital economy, agricultural technology innovation, and agricultural GTFP in a unified research framework and reveals their intrinsic linkages and impact mechanisms. This will also provide a particular theoretical foundation for other scholars in the field of the digital economy as well as green agriculture areas to study related ...
The impacts of adopting agricultural innovation and technology are widely examined. This paper synthesises 154 studies, which yielded a total of 600 estimates of the impact of adopting agricultural innovation and technology on production, social and economic outcomes. Using meta-regression analysis, the empirical results show that the reported ...
In this paper, we survey the ethical, social, and policy issues that are likely to arise as Artificial Intelligence (AI) begins to impact on agriculture. We highlight possible unintended consequences of the adoption of this technology, which have been neglected in most discussions of the topic to date. A range of current, as well as proposed ...
The impact of 5G on rural areas is a crucial aspect of the paper, as it discusses how 5G technology bridges the digital divide in agriculture. Case studies of 5G deployment in rural farming communities illustrate the economic and social implications of improved connectivity.
This research aims to provide an in-depth analysis of the social and economic impacts of agricultural technologies on farmers' livelihoods. The study was conducted for 32 districts of 3 states (n = 1440) in India during 2020-2021.
This article presents key technological advances in the digital agriculture, which will have significant impact. Artificial intelligence-based techniques, together with big data analytics, address the challenges of agricultural production in terms of productivity and sustainability. Emerging new applications will transform agriculture from the traditional farm practices to a highly automated ...
Agriculture. A technological revolution in farming led by advances in robotics and sensing technologies looks set to disrupt modern practice. Over the centuries, as farmers have adopted more ...
1.1 Digitalisation innovation as a means to increase agricultural sustainability. One of the most prominent fields of agricultural innovation is digitalisation, also considered the fourth revolution in farming (Citation Walter et al., 2017).This process is driven by a rapid increase in the use of big data for further developing existing technologies for farming (e.g. tractor-based tools that ...
Feature papers represent the most advanced research with significant potential for high impact in the field. A Feature Paper should be a substantial original Article that involves several techniques or approaches, provides an outlook for future research directions and describes possible research applications.
This paper presents a comprehensive review of emerging technologies for the internet of things (IoT)-based smart agriculture. We begin by summarizing the existing surveys and describing emergent technologies for the agricultural IoT, such as unmanned aerial vehicles, wireless technologies, open-source IoT platforms, software defined networking (SDN), network function virtualization (NFV ...
The current paper reviews the available rate-of-return (ROR) evidence on the impacts of research on productivity, production and the welfare of individuals in the agricultural sector. The paper then proceeds to draw lessons about the possibilities of using research to stimulate agricultural transformation. The specific objectives are: 1.
Smart agriculture technology based on the Internet of Things ... surveyed 67 research papers that published through 2006 to 2019 on the use of IoT in different agricultural applications; ... Fig. 8 shows the impacts of drones on agricultural development by using real-time data collection and processing.
Adoption of improved agricultural technologies remains to be a promising strategy to achieve food security and poverty reduction in many developing countries. However, there are limited rigorous impact evaluations on the contributions of such technologies on household welfare. This paper investigates the impact of improved agricultural technology use on farm household income in eastern Ethiopia.
of technology in agriculture is evident throughout the world. There are currently 7.2 billion people in the world today. Countries like China and. 25. risen from a total of 4.4billion people in ...
The main purpose of this paper is to introduce the technology impact of agriculture workers in Tirunelveli District. In the last century, the basic agriculture technology like machines has changed ...
Information technology helps to predicts the results related to the agriculture specially plant physiology. Leaf protein study is an important study which helps to solve protein deficiency and ...
It is proposed to build a rural digital infrastructure and promote the construction of digital villages, combining the actual situation of each place, guiding the layout of rural industries in accordance with local conditions, and further giving play to the positive effects om the level of rural economic development. Comprehensively promoting rural revitalisation is an important task for China ...
Decoupling of crop-livestock systems increases the risks of pollution, waste of nutrient resources, and biodiversity loss. Crop-livestock integration (CLI) is an effective solution to these problems, and motivating farmers to adopt CLI is the key. Many countries have implemented environmental regulations (ER) aiming to influence farmers' CLI adoption decisions. Based on a field study of 316 ...
This study explores the impact of market-based environmental regulations on green technological innovation and the differential regulatory effects of corporate social responsibility (CSR) on different levels of green technological innovation. By analyzing data from 746 Chinese A-share listed companies from the period of 2008-2021, this paper examines the effect of market-based environmental ...
Indonesian J Elec Eng & Co mp Sci ISSN: 2502-4752 . Technology in Indian a griculture - a review (R S Upendra) 1075. use the h istorical data for prediction of the occurrence of pests, weeds and ...
NASA's Strategic Plan (2022) outlines specific technology development objectives which direct the Agency to "innovate and advance transformational space technologies." An example of these potentially high-impact space technologies is the low-toxicity or "green" rocket propellant known as ASCENT (or Advanced Spacecraft Energetic Non-Toxic Propellant).
Our analysis finds that demand for occupations such as health professionals and other STEM-related professionals would grow by 17 to 30 percent between 2022 and 2030, (Exhibit 1). Exhibit 1. [email protected]. By contrast, demand for workers in food services, production work, customer services, sales, and office ...
If 2023 was the year the world discovered generative AI (gen AI), 2024 is the year organizations truly began using—and deriving business value from—this new technology.In the latest McKinsey Global Survey on AI, 65 percent of respondents report that their organizations are regularly using gen AI, nearly double the percentage from our previous survey just ten months ago.
These trends suggest that new farming innovations are urgently needed and that these innovations should be integrated into traditional agribusiness. Vertical cultivating and natural cultivating ...