Unemployment during the pandemic: How to avoid going for broke
Key takeaways.
- Without significant policy changes, employers will be hit with hefty tax increases to pay for mounting unemployment insurance (UI) claims.
- Thinning tax bases make financing UI more challenging.
- Having state UI trust funds in the red may make it much harder for job markets to recover.
Since the onset of the COVID-19 pandemic in late February, tens of millions of Americans have lost their jobs. Anxiety among many employers and consumers is still high — suggesting little hope of a rapid recovery.
This leaves state and local governments with gaping budget shortfalls amid falling income and sales tax revenues while demand for public services rises. A particularly fast-growing area of state expenditure is the payment of unemployment insurance (UI) benefits.
There has been extensive discussion among policymakers and the media regarding the trade-offs of more generous or longer-lasting UI benefits, such as the federal government’s provision of an additional $600 per week that expired July 31. But there has been very little talk about the tax hikes they will incur.
Many states have depleted their UI trust funds in the current crisis and have started to borrow from the federal government to pay their residents’ UI benefits. In the absence of additional policy changes, employers will be hit with significant UI tax increases over the next few years. And that will likely prevent some of the jobs that were lost from coming back.
In this policy brief, we explain how state unemployment insurance programs are financed and the threats to their solvency. We also discuss two reforms: one to relieve employers faced with crippling payroll tax increases in the coming years, and another to ensure that state UI trusts have enough money for future payouts.
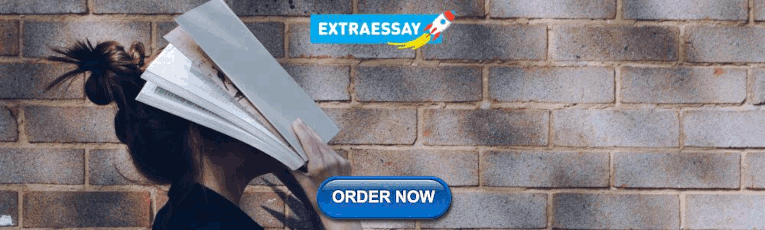
Understanding unemployment insurance
Unemployment insurance is one of the largest social insurance programs in the United States, with each state running its own UI program to pay benefits to people laid off from their jobs. In most states, UI replaces about half of a worker’s earnings up to a weekly benefit maximum ($443 in the median state) for a maximum of 26 weeks (6 months).
While providing a needed cushion to workers, UI leaves policymakers with a difficult balancing act. As benefits become more generous, many recipients reduce their efforts to find and maintain jobs, reducing total income and burdening other workers (Johnston and Mas 2018). But if benefits become stingier, the cushion provides less support leaving some unemployed vulnerable to fall behind on their bills or lose their housing (Ganong and Noel 2019). [1]
Benefits are generally paid to people with relatively low saving rates, so the money that is distributed is quickly spent, providing short-term stimulus for consumer goods. This leads economists to refer to UI as an “automatic stabilizer.” Without the need for additional legislation, states automatically spend more money on unemployment benefits when economic conditions deteriorate, and spending naturally retracts as the economy recovers.
During the strong labor market leading up to the pandemic, just 220,000 workers filed new UI claims in the typical week. In late February, the unemployment rate was at 3.5 percent — a 60-year low — and about 1.7 million Americans were receiving UI benefits.
But two months later, the pandemic’s sudden and massive shock to the economy vaulted the U.S. unemployment rate to 14.7 percent — an 80-year-high. This April, rates varied substantially across states, from a high of 28.2 percent in Nevada to a low of 8.3 percent in Nebraska.
During the last week of March, 6.9 million Americans filed new claims for UI benefits. As demonstrated in Figure 1, this was 10 times higher than the corresponding peak in new UI claims during the depths of the Great Recession more than a decade ago. By early May of this year, more than 25 million Americans were receiving UI payments and in every week since early March, new UI claims have exceeded the Great Recession peak of 660,000.
Figure 1: Weekly Initial Unemployment Insurance Claims (Thousands)
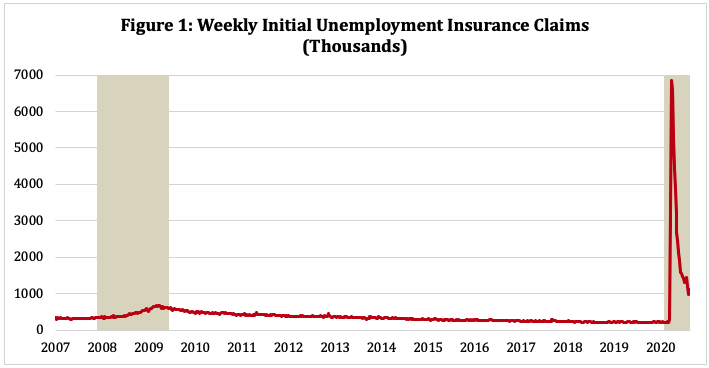
From March through the end of July, the federal CARES (Coronavirus Aid, Relief, and Economic Security) Act increased unemployment benefits for each recipient by $600 per week. That meant the average UI recipient was paid one-third more in unemployment than she earned while working (Ganong et al. 2020).
This raised concerns that workers had little incentive to return to work or find a new job, a condition necessary for labor market restructuring and recovery. [2] This additional UI funding expired at the end of July after lawmakers were unable to agree on another round of federal spending. President Trump attempted to provide a $300-dollar weekly “top-up” by executive order (with states given the option to provide an additional $100). Whether and when that happens is unclear given that states have to apply for the funding. [3]
UI benefits are financed by a payroll tax on employers. Unlike other taxes, UI tax rates are “experience-rated,” which means that an employer’s future tax rate rises if its employees claim UI benefits, and its tax rate falls when the firm avoids layoffs. This gives employers a strong incentive to balance the demand for layoffs with the cost that they impose on the UI system.
One consequence of experience-rating UI taxes is that tax rates increase as the economy begins to recover from recession. This significantly raises the cost of hiring new workers or retaining old ones, likely weighing down recovery of the labor market.
As shown in Figure 2, the average UI tax rate increased by more than 50 percent from 2009 to 2012 as the recovery was haltingly underway. This increase was especially high in middle-class industries — like construction and manufacturing — that were hit hardest during the Great Recession. As this same figure shows, average tax rates were more than 2.5 times as high among employers in construction as among all employers in the years following the three most recent recessions.
Figure 2: Average UI Tax Rate on Total Wages (1990-2018)
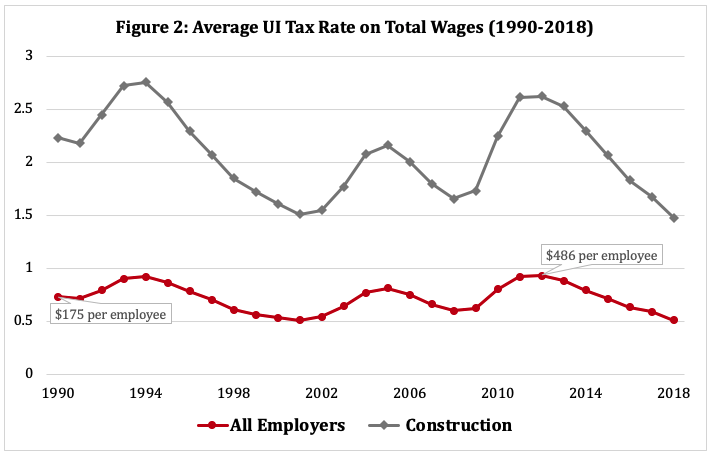
Surviving firms have to cover the UI costs generated by the employers that went out of business — causing them to be doubly burdened. Given the much larger increase in UI claims during the current recession relative to previous ones and the likely greater rate of firm exit, the increase in UI taxes could be substantially higher over the next few years than in the years following the Great Recession. This will encourage outsourcing and automation, induce some firms to shut down, and impede employment.
Softening the blow to businesses
Unless employment recovers with impressive speed, each claim will draw an average of $7,000 in payments from state UI trust funds. Those payments will transform into an estimated $270 billion dollars in payroll tax increases on firms over the next few years, reducing the ability of firms to resume normal hiring and employment and further stalling a labor market comeback. [4]
In March and April of this year, 20 states suspended experience rating to shield their employers from an avalanche of additional UI taxes in the upcoming years. These states span the political spectrum as well as geography, including Arizona, Georgia, Idaho, Maine, Maryland, Ohio, Texas, and Washington. [5]
While this policy change will — all else equal — hasten the labor market recovery in these states, it may also lead to a substantial increase in layoffs since it removes firms’ financial incentives to retain workers. Consistent with this, a comparison of five states that suspended experience rating with five neighboring states that did not reveals that layoff rates (defined as new UI claims divided by the workforce) were 30 percent higher in the five that shut down experience rating. [6]
States are therefore in a bind. By maintaining experience rating, a wave of future tax increases may hamper the economic recovery and prolong unemployment. But suspending experience rating may induce additional layoffs today, when things are most dire.
To soften the blow over the next few years while maintaining the incentives for employers to retain their workforce, states could adjust each company’s UI costs so that they are temporarily evaluated based on conditions in their industry — reducing the scope for tax increases that were out of the firm’s control.
For the next few years, employers would essentially be graded on a curve, comparing their layoff history with industry peers rather than a non-existent perfect firm. For example, since restaurants have been hit especially hard during the pandemic while the average technology firm has thrived, a restaurant that laid off 10 percent of its workers would face a smaller tax increase than a computer software company that did the same. Employers would have essentially equal incentives to maintain their workforce, but would not face crushing tax increases if they happen to be in an industry that was differentially hit by the COVID pandemic and the resulting lockdowns.
The benefits of such a policy could be substantial. Research suggests that employment is highly sensitive to UI tax increases in part because they hit firms that are already on the proverbial ropes. Anderson and Meyer (1997) find that a 1 percent increase in costs from UI taxes reduces employment by 2 percent. More recent research by Johnston (2020) finds even larger effects.
Shoring up the trust funds
The pandemic has shed light on the vulnerability of UI financing. Better maintenance of UI trust funds is vital to prepare states for the next economic downturn and improve prospects for future recoveries.
There is a large and growing gap in UI tax costs across jurisdictions. States like California and Florida have a low maximum tax rate and an annual tax base of around $7,000 — the lowest allowed by federal law — resulting in maximum potential UI taxes of about $400 per worker. In contrast, states like Washington and Oregon maintain large tax bases ($52,700 and $42,100, respectively) resulting in potential UI taxes of more than $2,000 per worker. [7]
In good times, states store revenues from UI taxes in a trust fund and that fund is drawn down in the depth of recessions. In recent years, however, state trust funds have been low even in good times — a function of benefits that are more generous than their financing (von Wachter 2016). The Department of Labor’s 2020 Solvency Report shows that despite a 10-year economic expansion, 21 state UI trust funds were below the minimum recommended reserve, just prior to the pandemic (U.S. Department of Labor 2020). [8] As of August 2020, 11 states have already depleted their UI trust funds and have started to receive loans from the federal government to pay UI benefits. [9]
These deficits may contribute to lethargic recoveries. When trust funds are low, states must steeply raise rates to recover their costs and pay benefits. The timing of these increases could not be worse. Weak trust funds also undermine experience rating. When a state trust fund is in debt to the federal government, federal UI taxes rise on all firms in that state until the federal loan is repaid, regardless of the firm’s layoffs.
In California, for instance, the large loan balance accrued during the 2008 recession was not repaid in full until 2018, hiking payroll taxes for employers across the board. This weakens the intended incentives of experience rating to encourage employment stability and curb abuse of the UI system. According to the same Labor Department Solvency Report cited above, California’s UI trust fund was in the worst position of all 50 states just prior to the pandemic (Appendix Figure 1). [10]
The thinning tax base is a leading cause of low UI reserves. States choose how much of a worker’s earnings are exposed to UI taxation, but the federal government can “update” the minimum requirement to keep pace with inflation and the rise in average earnings. The current federal requirement of $7,000 has —remarkably — not been updated since 1982, eroding the tax base unless states have legislated increases or proactively linked their taxable UI earnings base to inflation or wage growth.
Another important consequence of a small tax base is that UI taxes become much more regressive. This can reduce the employment opportunities for part-time workers or those with low earnings since firms essentially pay an equal tax for each worker (Guo and Johnston 2020). In a state like California, an employer would pay the same UI tax for a worker who earned $8,000 annually as for one who earned $40,000.
But the latter worker is eligible for a weekly UI benefit that is five times larger ($400 per week versus just $80 per week for the lower-paid worker). Expanding the UI program’s taxable wage base in states like California would reduce the implicit penalty on hiring low-wage earners (principally seasonal and part-time workers as well as students).
To restore the health of UI trust funds, governments should expand their tax bases to be proportional to the level of benefits in their state. A basic reform to shore up trust funds could be to require states to have taxable wage bases at least half as large as their annual insurable earnings.
Figure 3 plots the ratio of insured wages to taxable wages across the country, with larger values indicating greater insurance than funding.
Figure 3: Ratio of Annual Insured Wages to Taxable Wages (2015)
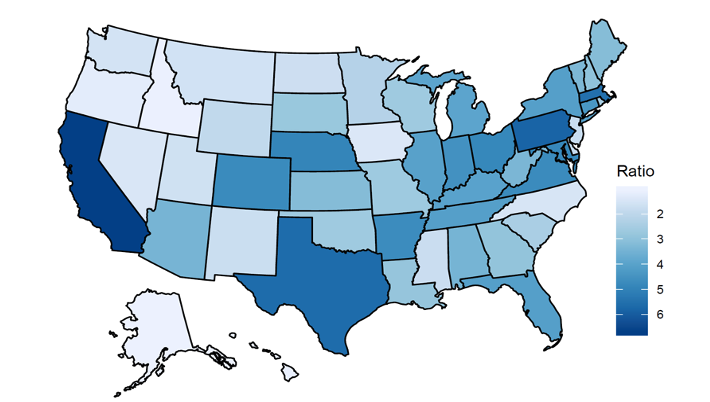
In California the UI-insurable income is $47,000, more than six times greater than the tax base of only $7,000. This reform would naturally link revenues to the generosity of the state’s UI system, allow states to lower tax rates, and bring in sufficient revenues to cushion workers the next time there is an economic shock. Harmonizing tax bases across states would also reduce the incentive for multi-state firms to reallocate jobs and operations based on state UI tax differences (Guo 2020).
Time for action
The COVID-19 crisis has put unemployment insurance at center stage of American politics and economic policy. It has provided a lifeline for tens of millions of workers who have lost their jobs since the pandemic’s onset six months ago, while at the same time exposing the system’s vulnerabilities. Given the complexity of UI financing and the scarcity of empirical evidence on which to rely, this is an important area for additional work and exploration.
Unless policymakers take steps to reform how the states’ unemployment insurance trust funds are financed, tax hikes will hurt labor market recoveries across the country — and with them, the American worker.
Mark Duggan is the Trione Director of SIEPR and the Wayne and Jodi Cooperman Professor of Economics at Stanford. Audrey Guo is an assistant professor of economics at Santa Clara University’s Leavey School of Business. Andrew C. Johnston is an assistant professor of economics, as well as applied econometrics at the University of California at Merced.
The authors are grateful to Isaac Sorkin for his helpful feedback.
1 States differ in where they choose to fall on that trade-off. The maximum weekly benefit varies substantially across states, from a low of $235 in Mississippi to a high of $790 in Washington. Some states also have a maximum duration of less than 26 weeks.
2 Recent research suggests that, at least in the short term, the disincentive effects of the increases in UI benefits (caused by the CARES Act) were minimal (Altonji et al. 2020).
3 More than half of states had applied or signaled their intention to apply as of August 21. Only South Dakota announced that it would not be applying (Iacurci 2020). States that are approved are guaranteed just three weeks of federal funding for the enhanced UI benefits, though more federal funding may be available.
4 For this calculation, we extrapolate weekly UI claims through the end of the year and assume that half of those claims become benefit spells. We use data on average weekly benefit amounts and average UI spell durations to calculate the typical cost of a UI benefit spell at a little over $7,000. The product of these two values is an estimate of the UI benefit costs that will factor into UI taxes over the coming years. The actual average value could be substantially higher if the recovery is slow, as this would lead to longer and more costly average UI benefit periods.
5 These 20 states are Alabama, Arizona, Georgia, Idaho, Iowa, Louisiana, Maine, Maryland, Minnesota, Missouri, Montana, Nebraska, North Carolina, North Dakota, Ohio, Pennsylvania, South Carolina, Texas, Utah, Washington, and the District of Columbia.
6 The matched pairs are — with the states that suspended experience rating listed first — Alabama and Mississippi, Ohio and Indiana, North Dakota and South Dakota, Arizona and New Mexico, and Idaho and Oregon.
7 Appendix Table 1 lists the UI tax base in each state in 2020 along with each state’s maximum per-worker tax and maximum weekly UI benefit.
8 The Department of Labor recommends that states have reserves in their trust funds that are at least as large as the highest recent years of UI benefit payout.
9 As of August 25, 2020, 11 states have borrowed $24.4 billion from the federal unemployment account. California, New York, and Texas account for 82% of that borrowing .
10 As shown in Appendix Figure 1, California’s solvency ratio of 0.21 was lower than the other 49 states, the District of Columbia, and Puerto Rico.
Altonji, Joseph, Zara Contractor, Lucas Finamor, Ryan Haygood, Ilse Lindenlaub, Costas Meghir, Cormac O’Dea, Dana Scott, Liana Wang, and Ebonya Washington. “Employment Effects of Unemployment Insurance Generosity during the Pandemic.” Working Paper (2020).
Anderson, Patricia M., and Bruce D. Meyer. "The effects of firm specific taxes and government mandates with an application to the U.S. unemployment insurance program." Journal of Public Economics 65, no. 2 (1997): 119-145.
Ganong, Peter, and Pascal Noel. "Consumer spending during unemployment: Positive and normative implications." American Economic Review 109, no. 7 (2019): 2383-2424.
Ganong, Peter, Pascal Noel, and Joseph S. Vavra. U.S. Unemployment Insurance Replacement Rates During the Pandemic , no. w27216. National Bureau of Economic Research (2020).
Guo, Audrey. "The effects of unemployment insurance taxation on multi-establishment firms." Working Paper (2020).
Guo, Audrey, and Andrew C. Johnston. "The Finance of Unemployment Compensation and its Consequence for the Labor Market." Working Paper (2020).
Iacurci, Greg. “ This Map Shows Where States Stand on the Extra $300 Weekly Unemployment Benefits. ” CNBC, August 21, 2020.
Johnston, Andrew C. “Unemployment Insurance Taxes and Labor Demand: Quasi-experimental Evidence from Administrative Data.” Forthcoming at American Economic Journal: Economic Policy (2020).
Johnston, Andrew C., and Alexandre Mas. "Potential unemployment insurance duration and labor supply: The individual and market-level response to a benefit cut." Journal of Political Economy 126, no. 6 (2018): 2480-2522.
U.S. Department of Labor. State Unemployment Insurance Trust Fund Solvency Report 2020. February 2020.
Von Wachter, Till. “ Unemployment Insurance Reform: A Primer. ” Washington Center for Equitable Growth. October 2016.
Appendix Table A
Source: US Dept of Labor Significant Provisions of State Unemployment Insurance Laws 2019
*For single workers. Some states offer additional dependent allowances
Appendix Figure 1 - State UI Trust Fund Solvency (as of 1/1/2020)
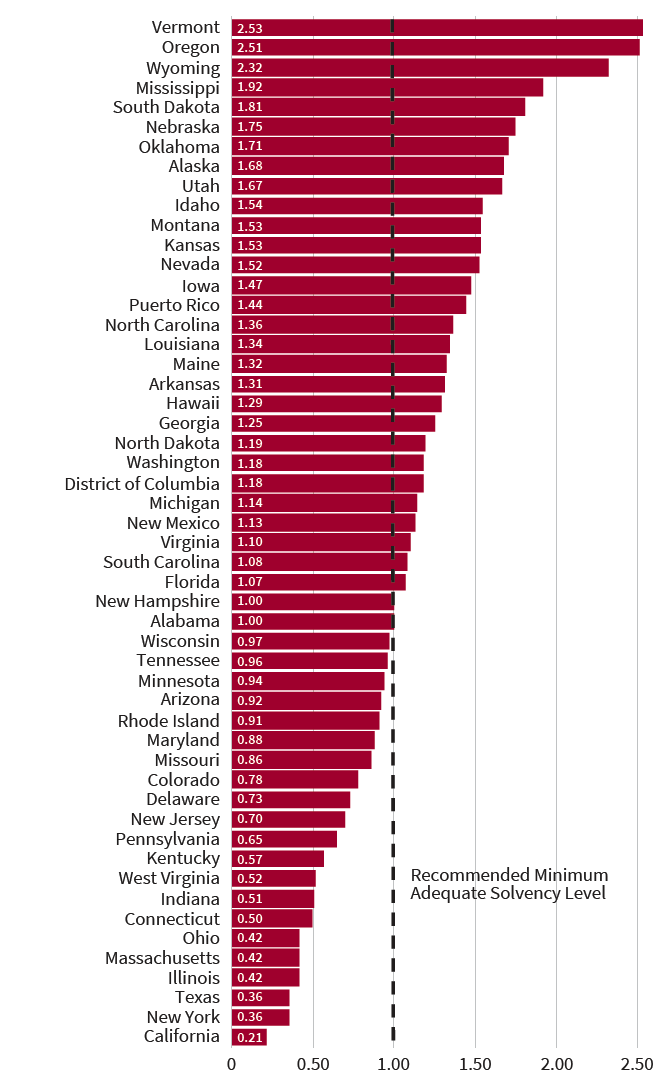
Source: U.S. Department of Labor Trust Fund Solvency Report 2020
Related Topics
- Policy Brief
More Publications
Increasing wireless value: technology, spectrum, and incentives, careful what you wish for: the shale gas revolution and natural gas exports, estimating equilibrium in health insurance exchanges: analysis of the californian market under the aca.
- - Google Chrome
Intended for healthcare professionals
- Access provided by Google Indexer
- My email alerts
- BMA member login
- Username * Password * Forgot your log in details? Need to activate BMA Member Log In Log in via OpenAthens Log in via your institution

Search form
- Advanced search
- Search responses
- Search blogs
- News & Views
- Covid-19, unemployment...
Covid-19, unemployment, and health: time for deeper solutions?
Read our latest coverage of the coronavirus outbreak.
- Related content
- Peer review
- Martin Hensher , associate professor of health systems financing and organisation 1 2
- 1 Deakin Health Economics, Deakin University, 221 Burwood Highway, Burwood, VIC 3125, Australia
- 2 Menzies Institute for Medical Research, University of Tasmania
- Correspondence to: martin.hensher{at}deakin.edu.au
As covid-19 drives unemployment rates around the world to levels unseen in generations, once radical economic policy proposals are rapidly gaining a hearing. Martin Hensher examines how job guarantee or universal basic income schemes might support better health and better economics
Covid-19 has been a dramatic global health and economic shock. As SARS-CoV-2 spread across nations, economic activity plummeted, first as individuals changed their behaviour and then as government “lockdowns” took effect. 1 Macroeconomic forecasters foresee a major recession continuing through 2020 and into 2021. 2 Although the governments of many nations have taken novel steps to protect workers, unemployment has risen dramatically in many countries ( box 1 , fig 1 ); poverty and hunger are on the rise in low and middle income countries. 5 Covid-19 has directly caused illness and death at a large scale, and further threatens health through disruption of access to health services for other conditions.
Covid-19 and unemployment
Although unemployment soared in response to covid-19 in some nations, the policy measures undertaken by others have prevented many workers from becoming technically unemployed. In the United Kingdom, the headline rate of unemployment for April-June 2020 was 3.9%—only slightly higher than the 3.89% rate in April-June 2019. Yet in June 2020 9.3 million people were in the coronavirus job retention scheme (“furlough”) and another 2.7 million had claimed a self-employment income support scheme grant; there had been the largest ever decrease in weekly hours worked; 650 000 fewer workers were reported on payrolls in June than in March; and the benefit claimant count had more than doubled from 1.24 million to 2.63 million people. 3 The Australian Bureau of Statistics has produced an adjusted estimate of Australian unemployment that includes all those temporarily stood down or laid off, to allow a closer comparison with US and Canadian statistics ( fig 1 ). As emergency support measures are wound back, concern is growing that the downwards trend from the April peak might not be maintained in coming months.

Unemployment rates in Australia, Canada, and the United States from March to July 2020. 4
- Download figure
- Open in new tab
- Download powerpoint
The pandemic continues to spread, and hopes for a rapid “return to normal” look increasingly unfounded. The economic consequences of covid-19 have the potential to further damage human health if not managed effectively—even after the pandemic has faded. Even with the most rose tinted views of recovery, the effects of covid-19 on unemployment are likely to be substantial and long lived. Ambitious responses to the imminent scourge of mass unemployment are being discussed. Two such proposals—a job guarantee and universal basic income—might protect and promote health as well as prosperity. Governments around the world should consider radical plans to safeguard their citizens’ livelihoods and wellbeing.
Unemployment and health in the time of covid-19
Decades of accumulated evidence show a strong and consistent association between unemployment and a range of adverse health outcomes, including all cause mortality, death from cardiovascular disease and suicide, and higher rates of mental distress, substance abuse, depression, and anxiety. 6 7 8 Job insecurity is similarly associated with poorer self-assessed health status, mental distress, depression, and anxiety. 9 Unemployment and economic adversity are intimately related with despair and lack of hope, which have increasingly been linked with mortality and the rise and severity of the US opioid epidemic. 10 11 Whether recessions and mass unemployment increase aggregate mortality is less clear; historical studies indicated improvements in mortality during the Great Depression in the 1930s, 7 but more recent US research found that older workers (aged 45-66) who lose their jobs in a recession have higher mortality than those who lose their jobs in boom times. 12 Insecurity, precariousness, and austerity harmed both unemployed and employed people during the protracted economic crisis in Greece after 2008-09. 13 Meanwhile, differing welfare state institutions and unemployment insurance arrangements directly limit or amplify health inequalities in a society. 7 14
These factors could adversely affect the health of growing numbers of unemployed workers after covid-19. 15 16 Governments, business lobbyists, and civil society advocates around the world are debating how economies might best recover from the covid recession. Although governments currently acknowledge the need to spend freely during the crisis, experience suggests that pressure to pursue misguided austerity policies might grow, threatening subsequent recovery. Options on the table range from “green new deal” programmes to build a post-carbon economy and national industrial strategies to bring globalised manufacturing back onshore through to calls for reducing wages and labour protections to “free up” labour markets. Yet these are all indirect approaches to the effects of unemployment. Proposals for a job guarantee or a universal basic income seek to act more directly to support individual citizens.
The job guarantee
The idea of a right to employment can be traced back to the US New Deal in the 1930s, and to Article 23 of the 1948 United Nations Universal Declaration of Human Rights. More recently, in the contest for the Democratic Party’s 2020 candidate for US president, senators Bernie Sanders, Kirsten Gillibrand, and Cory Booker all included a job guarantee in their platforms, as did Alexandria Ocasio-Cortez’s green new deal resolution. More than one detailed proposal for a Federal Job Guarantee has been published in the US 17 18 and in Australia. 19 In one US proposal, 18 a federally funded public service employment programme would provide a standing offer of work at a living wage ($15 (£12; €13) an hour), along with key benefits including healthcare coverage. Employees of this programme would be deployed on a wide range of public works and community development activities, delivered through federal, state, local, and non-profit agencies. The proposal argues that this would effectively eliminate unwanted joblessness and underemployment and would rapidly force the private sector to increase wages to match this “living wage” alternative, lifting millions out of poverty and greatly improving the incomes of working poor people. 18 Proponents argue that the job guarantee is the most efficient “automatic stabiliser” for the economy throughout the business cycle, able to adjust up and down to reflect the changing economic health of the private sector. In economic downturns, it would provide guaranteed employment to stop people falling into poverty and losing “employability,” while also supporting aggregate demand to lift the economy out of recession. In boom times, workers will simply exit the programme for the private sector, as firms offer higher wages to secure the additional labour they need.
In the US, the job guarantee has been proposed as not only a key tool for recovery from covid-19, 20 but also a mechanism to ensure that this recovery breaks down historically entrenched racial inequalities in wealth. 21 Similarly, an emerging job guarantee proposal for Australia could rectify decades of welfare policy failures that have disproportionately affected indigenous Australians. 22 Proponents point to successful past or present international experiences with full or targeted employment guarantee programmes, including Argentina’s Plan Jefes, South Africa’s Expanded Public Works Programme, India’s National Rural Employment Guarantee Act, Belgium’s Youth Job Guarantee, the US Youth Incentive Entitlement Pilot Projects, and the UK’s Future Jobs Fund. 20
Universal basic income
Over the past few years, there has been a global explosion of interest in the concept of universal basic income. 23 24 25 Andrew Yang, another former contender for the 2020 Democrat presidential nomination, made universal basic income a central plank of his platform. Such proposals share key characteristics: they are a transfer of income (from the state to individuals) that is provided universally (to everyone, with no targeting), unconditionally (with no requirements, for example to work), and in cash (with no controls on what the money can be spent on). 25 Proposals also typically specify an income that is sufficiently generous that it can fully cover a basic level of living expenses. 23 Universal basic income is a direct means of reducing poverty, by ensuring that all in society receive enough to live with dignity; it could reduce income inequality; it could radically simplify current social welfare systems and remove poverty traps and disincentives to move from welfare into work; it could improve the ability of workers to refuse poorly paid, insecure, exploitative or unsafe jobs, through a reduced fear of loss of income; and it could be a buffer against technological unemployment, as automation and artificial intelligence replace human labour. 23 25 Universality is the key difference from today’s welfare systems; everyone should receive universal basic income as a right of citizenship, and its receipt by all should build the solidarity and legitimacy that will sustain this right. Universal basic income could improve health and reduce health inequities through direct action on various social determinants of health. 26 27 This variety of aims leads to the concept being simultaneously supported by those on the left as a radical, anti-capitalist policy, often viewed as an essential component of the ecological degrowth agenda, and by libertarian, tech capitalists as an efficient solution to the risk that ever expanding digital automation will destroy more jobs than it creates, and as a vital measure to help capitalism survive mass technological unemployment in the future. 28
In the wake of the covid-19 economic shock, universal basic income has been discussed as a potentially powerful policy solution to unprecedented economic dislocation. It has specifically been suggested as a tool for limiting the economic, social, and psychological trauma of covid-19. 29 The Spanish government has just introduced a nationwide, means tested minimum income programme (not universal) as a direct response to covid related unemployment. 30 The US government has made unconditional, one-off economic impact payments to most (but not all) American households. Near universal and unconditional universal basic income programmes have only operated at nationwide scale in two countries, Mongolia and Iran. The Mongolian programme has since ceased, and the Iranian programme is no longer strictly universal (the richest people are no longer eligible). Partial schemes and regional pilots, however, have been run successfully in a wide range of nations. 25 A recent trial that provided universal basic income to 2000 recipients in Finland found that employment outcomes, health, and wellbeing measures were better in the universal basic income group than in the comparison group, 31 and the Scottish government has been contemplating a three year trial of universal basic income in an experimental group of recipients. 32
Potential health benefits
Given the substantial evidence linking unemployment to poor health, proponents of both job guarantee and universal basic income schemes point to their potential health benefits as major arguments in their favour ( table 1 ). 20 26 These measures could be expected to positively affect health through four main pathways: direct effects for individual beneficiaries; knock-on effects improving labour market conditions for all workers; the macroeconomic and distributive benefits of more widespread prosperity; and more localised community effects unlocked by these programmes.
Health effects of job guarantee (JG) and universal basic income (UBI) programmes
- View inline
Multiple mechanisms would work through these four pathways to deliver potential health benefits, including reduced mortality and improved physical and mental health status. Key mechanisms include reducing poverty, improving economic security, improving the quality of jobs and work, and rebuilding stronger local communities. Unsurprisingly, pathways that link unemployment with poorer health will be more reliably affected by job guarantee programmes than by universal basic income. But universal basic income offers alternative pathways for better health through informal caring and non-market activities. Both types of programme could help resolve one of the problems that the covid-19 pandemic has brought into sharp focus—that low paid, insecure, and casualised workforces cannot afford to self-isolate or stay at home when sick or potentially infected because they lack access to paid sick leave. This problem has proved especially disastrous for those who care for elderly people.
Controversies and choices
Supporters of job guarantee or universal basic income programmes typically have different priorities and view them as two alternative options, not as complementary programmes that could co-exist. Most job guarantee proposals see it as not only a means to fight unemployment, but also an explicit instrument of macroeconomic policy 38 ; universal basic income would not function as an “automatic stabiliser” in the same way. Critics of job guarantee and universal basic income schemes primarily question their affordability and potential macroeconomic consequences ( box 2 ).
Economic controversies
Implementing a job guarantee or universal basic income programme would be a major economic reform in any nation and a decisive break with the economic orthodoxy that has prevailed since the Thatcher-Reagan revolution of the 1980s. It would undoubtedly be controversial. Most obviously, some would question them on cost and affordability grounds. A job guarantee programme would incur a substantial net cost to governments—modelling of proposed programmes indicates a net cost to the federal budget equivalent to 1.5% of annual general domestic product (GDP) in the US 18 and 2.6% in Australia (based on a net budgetary cost of A$51.7bn). 19 By comparison, the Australian government is spending A$70bn, or 3.6% of its GDP, on its emergency JobKeeper employment protection programme this year—budget costs of these magnitudes are not unheard of. The gross costs of a universal basic income programme would be substantially larger: income of $12 000 (close to the 2017 US poverty line) for every US adult would cost the federal budget about $3tn, or nearly 14% of GDP. 23 Yet this gross cost estimate is arguably misleading, 39 not only because universal basic income would be partially offset by large savings from current welfare programmes, but because so many recipients would return much or all of it in the form of tax payments. One estimate of the net cost of such a programme indicates that it could be as low as 2.95% of US GDP. 39 These proposals emerge as a growing number of economists are saying that the governments of countries in possession of their own sovereign currency can never “run out of money” and can always purchase whatever goods and services are for sale in the currency they issue. 38 40 They also suggest that inflation—the other risk often pointed to by critics of job guarantee or universal basic income—is currently highly unlikely, with a general fear that the covid-19 recession will prove to be deflationary rather than inflationary.
For those concerned with health, however, philosophical differences might be of more interest. Social determinants and socioeconomic inequalities are well understood to be powerful forces driving health outcomes at both individual and population levels. Universal basic income seeks to reduce poverty and inequality by putting in place an absolute floor—a minimum income provided to everyone in society. A job guarantee seeks to affect poverty by ensuring that anyone who wants to work can work, for a living wage in a decent job. But in so doing, a job guarantee also explicitly increases the relative power of workers, ensuring that a larger share of national income flows to labour, rather than to the owners of capital—potentially reducing some of the extreme inequalities in income and wealth distribution that have arisen over the past four decades. One criticism of universal basic income is that it might (whether inadvertently or by design) become a “plutocratic, philanthropic” programme 28 —scraps from the table of the ultra wealthy, which might cement dependence and powerlessness in a future of technological unemployment. Equally, a job guarantee might be criticised as being a mid 20th century solution to a 21st century problem, which will reinforce social hierarchies by insisting on participation in paid employment as the solution to poverty.
The unemployment triggered by covid-19 in so many countries is a clear and present danger to individual and population health. Tinkering around the margins of current welfare systems, exhortations for yet more labour market “flexibility,” or an unwillingness to maintain public spending through a potentially long and drawn out downturn all offer a fast track to poor outcomes. The scale of the covid economic shock demands more radical action. The substantial health harms of unemployment might be mitigated by a universal basic income programme, but if unemployment is the problem, then employment seems likely to deliver more effective mitigation along the many and complex pathways by which these harms are transmitted. If so, implementing national job guarantee programmes should be a more urgent priority for governments in the immediate aftermath of covid-19. A successful job guarantee scheme would avert the harms of unemployment, strengthen the position of ordinary working people, and deliver a more broadly distributed prosperity in the short to medium term. This would be a much better position from which to then debate and trial universal basic income, allowing it to be correctly framed as a strategic, long term solution to the changing future of work, rather than simply as a response to the current economic crisis.
Key messages
Covid-19 has triggered economic recession and unprecedented rapid rises in unemployment in many countries
Mass unemployment has the potential to cause grave harm to individual and population health if not effectively mitigated
The scale of the crisis means that radical solutions might need to be considered, such as a job guarantee or universal basic income programmes
These policies have the potential to protect human health and dignity, but would mark a significant break with economic orthodoxy
Acknowledgments
I acknowledge the Wurundjeri people of the Kulin Nation as the traditional owners of the land on which this work was undertaken.
Contributors and sources: MH has worked on health financing, planning, and economics as a senior policy maker and researcher in the UK, South Africa, and Australia and as a consultant for the World Bank, World Health Organization and the European Commission. His research on the ecological and economic sustainability of healthcare systems has included examining a number of emerging heterodox economic approaches, two of which are gaining in significance: ecological economics and modern monetary theory. Members of these schools have promoted universal basic income and a job guarantee, respectively, over many years. This article builds on the existing academic literature to consider very recent policy proposals that are emerging in response to the threat of mass unemployment in the wake of covid-19.
Patient involvement: No patients were involved.
Competing interests: I have read and understood BMJ policy on declaration of interests and have the following interests to declare: this research was supported by an Australian Government Research Training Scholarship.
- ↵ Goolsbee A, Syverson C. Fear, lockdown, and diversion: comparing drivers of pandemic economic decline 2020. National Bureau of Economic Research, June 2020. https://www.nber.org/papers/w27432
- ↵ International Monetary Fund. World economic outlook update: a crisis like no other, an uncertain recovery. June 2020. https://www.imf.org/en/Publications/WEO/Issues/2020/06/24/WEOUpdateJune2020
- ↵ Office for National Statistics. Labour market overview, UK: August 2020. 2020. https://www.ons.gov.uk/employmentandlabourmarket/peopleinwork/employmentandemployeetypes/bulletins/uklabourmarket/august2020
- ↵ Australian Bureau Statistics. Understanding unemployment and the loss of work during the covid-19 period: an Australian and international perspective. 13 August 2020. https://www.abs.gov.au/articles/understanding-unemployment-and-loss-work-during-covid-19-period-australian-and-international-perspective
- ↵ World Bank. World Bank Group. 100 countries get support in response to covid-19. 19 May 2020. https://www.worldbank.org/en/news/press-release/2020/05/19/world-bank-group-100-countries-get-support-in-response-to-covid-19-coronavirus
- Stuckler D ,
- von dem Knesebeck O
- Beckfield J
- Beckfield J ,
- Eikemo TA ,
- McNamara C ,
- Kugenthiran N ,
- Collins D ,
- Christensen H ,
- Fullwiler S ,
- Tcherneva P ,
- ↵ Mitchell WF, Watts M. Investing in a job guarantee for Australia. Centre of Full Employment and Equity. July 2020. http://www.fullemployment.net/publications/reports/2020/CofFEE_Research_Report_2000-02.pdf
- Tcherneva P
- Hamilton D ,
- Asanthe-Muhammad D ,
- Collins C ,
- ↵ Pearson N. The case for a government jobs guarantee. The Australian. 4 July 2020. https://www.theaustralian.com.au/commentary/the-case-for-a-government-jobs-guarantee/news-story/dee6e9545cd5af967c853e2f0481b02d
- Rothstein J
- Banerjee A ,
- Niehaus P ,
- Gentiline U ,
- Rigolini J ,
- Ruckert A ,
- Fouksman E ,
- Johnson MT ,
- Johnson EA ,
- ↵ Dombey D, Sandbu M. Spain to push through minimum income guarantee to fight poverty. Financial Times, London. 28 May 2020. https://www.ft.com/content/0b6a5d25-e078-4dfc-b314-f659b566317e
- ↵ Kangas O, Jauhiainen S, Simanainen M, Ylikännö M. Evaluation of the Finnish Basic Income Experiment/Suomen perustulokokeilun arviointi Helsinki: Ministry of Social Affairs and Health/Sosiaali- ja terveysministeriö; 2020. https://julkaisut.valtioneuvosto.fi/bitstream/handle/10024/162219/STM_2020_15_rap.pdf
- ↵ Paton C. Coronavirus in Scotland: Nicola Sturgeon eyes plans for universal basic income. The Times, London. 5 May 2020. https://www.thetimes.co.uk/article/nicola-sturgeon-eyes-plans-for-universal-basic-income-c9rhfwbx7
- Nagelhout GE ,
- de Goeij MCM ,
- de Vries H ,
- Kleinman R ,
- Fischer B ,
- Gardiner C ,
- Geldenhuys G ,
- Olanrewaju O ,
- Lafortune L
- Mitchell W ,
- Widerquist K
- Skidelsky R
An Unemployment Crisis after the Onset of COVID-19
Nicolas Petrosky-Nadeau and Robert G. Valletta
Download PDF (115 KB)
FRBSF Economic Letter 2020-12 | May 18, 2020
The COVID-19 pandemic has upended the U.S. labor market, with massive job losses and a spike in unemployment to its highest level since the Great Depression. How long unemployment will remain at crisis levels is highly uncertain and will depend on the speed and success of coronavirus containment measures. Historical patterns of monthly flows in and out of unemployment, adjusted for unique aspects of the coronavirus economy, can help in assessing potential paths of unemployment. Unless hiring rises to unprecedented levels, unemployment could remain severely elevated well into next year.
The wave of initial job losses during the coronavirus disease 2019 (COVID-19) pandemic has been massive, with more than 20 million jobs swept away between March and April. This is much larger than losses recorded during similar time frames in any other postwar recession. As a result, the April unemployment rate spiked to the highest level recorded since the Great Depression of the 1930s.
In this Economic Letter , we assess possible paths for unemployment through 2021. Although the initial scale of the crisis is clear, substantial uncertainty surrounds the future path of unemployment. This uncertainty primarily revolves around the success of virus containment measures and how quickly economic activity can recover. Fundamental measurement challenges are also likely to affect the official unemployment rate: some laid-off workers cannot actively search for new jobs because of shelter-in-place restrictions and hence may be counted as out of the labor force, rather than unemployed.
To assess the possible path of the measured unemployment rate through next year, we focus on the underlying monthly flows in and out of unemployment, accounting for historical patterns and unique aspects of the coronavirus economy; our approach and results are described in detail in Petrosky-Nadeau and Valletta (2020). Our analysis suggests that returning to pre-outbreak unemployment levels by sometime in 2021 would require a significantly more rapid pace of hiring than during any past economic recovery.
Initial wave of job losses and unemployment
Even before the Bureau of Labor Statistics (BLS) released April employment and unemployment numbers on May 8, the unprecedented scale of job losses due to coronavirus containment measures was clear. About 25 million new unemployment insurance (UI) claims were filed between mid-March, when U.S. containment measures started to spread widely and the BLS monthly survey was conducted, and mid-April when the next month’s BLS survey was conducted. During periods of intensive job loss, weekly reports on new UI claims provide a good measure of job losses because most laid-off workers are eligible for UI benefits. However, the current massive scale of new claims has swamped state UI agencies and likely delayed processing of many claims. As such, the recent surge should be interpreted as a loose lower-bound estimate of initial job losses.
A comparison with the Great Recession of 2007-09 starkly illustrates the severity of the current situation (Figure 1). Initial UI claims during the first month of the COVID-19 crisis were about 10 times larger than claims during the worst periods of the Great Recession.
Figure 1 Monthly initial unemployment insurance claims
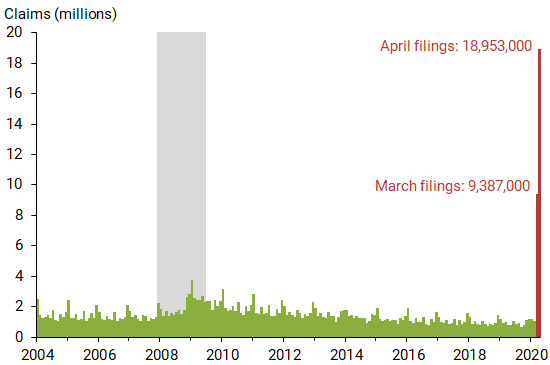
Note: Data from the U.S. Department of Labor, not seasonally adjusted (last two data points rounded to nearest thousand; April data through May 2). Gray bar indicates NBER recession dates.
These initial job losses, combined with a likely pronounced reduction in hiring activity, imply a sharp increase in the unemployment rate. Before the April BLS report was released, we projected that the unemployment rate was likely to rise nearly 15 percentage points, from 4.4% in March to 19.0% in April.
Other recent projections of the April unemployment rate span a very wide range (Faria-e-Castro 2020, Wolfers 2020, Coibion, Gorodnichenko, and Weber 2020, and Bick and Blandin 2020). The wide range partly reflects the challenge of measuring unemployment when shelter-in-place restrictions prevent active job search in much of the country. This is evident in the estimates by Coibion et al. (2020) and Bick and Blandin (2020), which differed substantially despite their reliance on careful surveys designed to approximate the official BLS approach.
The official April employment report released on May 8 showed that unemployment rose to 14.7%, a huge increase but below our projection. However, the report also noted a large increase in the number of workers on unpaid absences, likely reflecting virus-related business closures. Counting these workers as unemployed would push the unemployment rate much closer to our 19% projection. We therefore have not modified our prior projections.
Unemployment projections based on labor market flows
Our approach to projecting the unemployment rate relies on the monthly flows between unemployment, employment, and out of the labor force (nonparticipation), similar to Şahin and Patterson (2012). In particular, the monthly change in the unemployment rate reflects the difference between the number who enter unemployment (inflows) and the number who exit unemployment (outflows), with employment and nonparticipation as possible initial or subsequent status. This framework accounts for the key determinants of pandemic-related unemployment, with initial UI claims (inflows through job loss) and depressed hiring (outflows) determining the initial spike in unemployment. Using this approach, we explore different scenarios for unemployment through the end of 2021. For all scenarios, we assume that job losses are most severe in April (about 25 million), then ease substantially in May (7.8 million) and June (2.6 million), before returning to their historical trend in July (1.4 million).
The path of the unemployment rate afterward depends on unemployment outflows, primarily reflected in the pace of hiring among the pool of unemployed individuals. Tremendous uncertainty surrounds the timing and strength of the hiring surge as the economy recovers. If the virus is contained quickly and the economic recovery is vigorous, hiring could rapidly resume, particularly if many businesses and workers have maintained their connections. However, hiring could be slow if virus outbreaks or continued containment measures make employers hesitant based on low demand for their products. We therefore explore a range of hiring scenarios over the coming months.
The first scenario, “historical outflow dynamics,” assumes that the pace of hiring corresponds statistically to the typical recovery from past recessions. Because hiring tends to bounce back slowly following recessions, and given the severity of the current downturn, this scenario is relatively adverse.
Our second scenario, “hiring bounce,” incorporates very strong hiring activity following an assumed end of COVID-19 restrictions in July 2020. This scenario provides a baseline for assessing the pace of hiring required to reverse the initial labor market shock. It assumes a return to pre-outbreak hiring rates by the end of the third quarter of 2020. However, the pace of hiring implied by this scenario is extremely high by historical standards given the vast pool of unemployed individuals. In particular, this scenario requires around 9 million hires from unemployment per month during the third quarter, nearly four times faster than the most robust hiring rate during the recovery from the Great Recession.
Our third scenario, “GDP/hiring forecast,” bases hiring projections on the historical relationship between GDP growth and overall exit rates from unemployment to employment or nonparticipation. This requires a GDP forecast. We rely on a recent San Francisco Fed forecast of GDP growth for 2020-21, specifically the more favorable of two alternatives discussed in qualitative terms in Leduc (2020). It assumes that growth bounces back in the second half of this year and continues at a strong pace next year.
Figure 2 shows the unemployment paths for these scenarios. In the historical outflow dynamics scenario (dark blue line), unemployment quickly peaks around 20% and then stays in double digits through early 2021. By contrast, the hiring bounce scenario (light blue line) reflects a stronger recovery in hiring activity, so the unemployment rate drops much more rapidly. At the end of 2020 most of the job losses have been reversed, and unemployment approaches pre-outbreak levels. For the GDP/hiring forecast scenario (yellow line), unemployment peaks above 18% in the second quarter of 2020, followed by a rapid decline in the third quarter due to underlying limited changes in the hiring rate implied by its historical relationship with GDP growth.
Figure 2 Unemployment rate paths under different scenarios
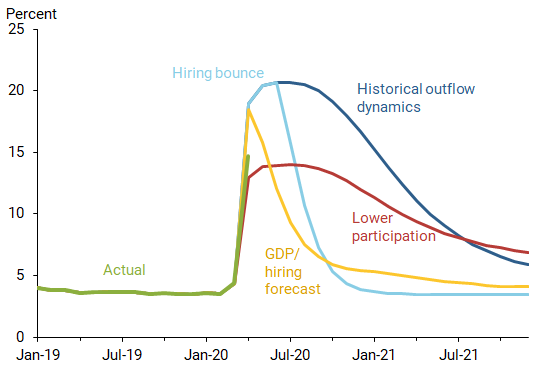
Incorporating unemployment and nonparticipation ambiguities
As noted earlier, widespread shelter-in-place restrictions may preclude active job searches among laid-off workers, causing them to report themselves as out of the labor force rather than unemployed. Consistent with this, the official labor force participation rate fell 2.5 percentage points to 60.2% in April. We explore the potential impact of these measurement challenges through alternative assumptions about flow rates between different labor market states.
In particular, historical patterns of worker flows from employment to nonparticipation then back into employment during recoveries suggest that nearly half of those workers laid off during the pandemic could leave the labor force upon suffering a job loss. This moderates the initial rise in unemployment, shown as the lower participation scenario (red line) in Figure 2. As individuals return to the labor market during the recovery, lifting the labor force participation rate back toward its previous trend, the pace of return to a pre-outbreak unemployment rate is also muted. In fact, the historical outflow dynamics and lower participation scenarios converge at 8% unemployment in mid-2021. However, these two scenarios imply vastly different trajectories for the labor force participation rate. Figure 3 shows the paths for these scenarios over an extended time frame relative to the trend projected by the Congressional Budget Office (2020).
Figure 3 Labor force participation rate under different scenarios
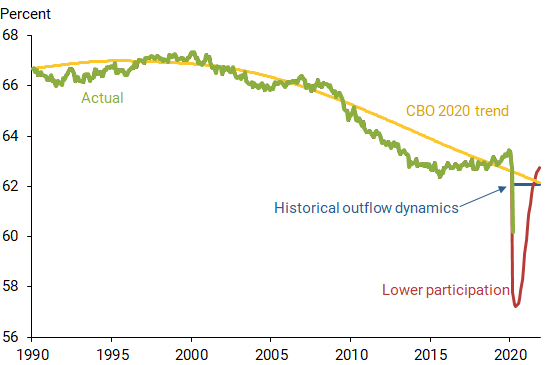
Conclusions: An uncertain road to recovery
The COVID-19 pandemic has created tremendous labor market disruptions and profound hardship throughout the United States and the world. This is partly reflected in the sudden unprecedented increase in the U.S. unemployment rate in April, the first month for which the full effects of coronavirus containment measures are evident. To get a handle on the severity of the labor market disruption, we assess possible paths for unemployment through the end of 2021. Tremendous uncertainty surrounds unemployment projections over the next few years, so we do not claim that any specific scenario qualifies as “likely.” On the pessimistic side, absent a historically unprecedented burst of hiring, the unemployment rate could remain in double digits through 2021. From a more optimistic perspective, if shutdowns are lifted quickly and employers capitalize on the large pool of available workers by ramping up hiring, the unemployment rate could be back down near its pre-outbreak level by mid-2021.
Uncertainty about the path of the unemployment rate also reflects measurement challenges arising from the ambiguous labor force status of laid-off workers whose active job search is limited by shelter-in-place measures. This may temper the official unemployment rate, but at the expense of a lower labor force participation rate, which is an alternative indicator of labor market dislocation and hardship. Given the implied uncertainty about the measurement of future labor market conditions, it is imperative to closely monitor a wide range of indicators to assess how the U.S. labor market is evolving in response to the COVID-19 shock.
Nicolas Petrosky-Nadeau is a vice president in the Economic Research Department of the Federal Reserve Bank of San Francisco.
Robert G. Valletta is a senior vice president in the Economic Research Department of the Federal Reserve Bank of San Francisco.
Bick, Alexander, and Adam Blandin. 2020. “Real Time Labor Market Estimates during the 2020 Coronavirus Outbreak.” Manuscript, Arizona State University, April 15.
Coibion, Olivier, Yuriy Gorodnichenko, and Michael Weber. 2020. “Labor Markets During the COVID-19 Crisis: A Preliminary View.” BFI Working Paper, Becker Friedman Institute for Economics, University of Chicago, April 13.
Congressional Budget Office. 2020. “The Budget and Economic Outlook: 2020 to 2030.” Report 56020, January 28.
Faria-e-Castro, Miguel. 2020. “Back-of-the-Envelope Estimates of Next Quarter’s Unemployment Rate.” On the Economy, FRB St. Louis blog, March 24.
Leduc, Sylvain. 2020. “FedViews.” FRB San Francisco, April 6.
Petrosky-Nadeau, Nicolas, and Robert G. Valletta. 2020. “Unemployment Paths in a Pandemic Economy.” FRB San Francisco Working Paper 2020-18, May.
Şahin, Ayşegül, and Christina Patterson. 2012. “The Bathtub Model of Unemployment: The Importance of Labor Market Flow Dynamics.” Liberty Street Economics, FRB New York blog, March 28.
Wolfers, Justin. 2020. “The Unemployment Rate Is Probably Around 13%.” New York Times (The Upshot), April 16.
Opinions expressed in FRBSF Economic Letter do not necessarily reflect the views of the management of the Federal Reserve Bank of San Francisco or of the Board of Governors of the Federal Reserve System. This publication is edited by Anita Todd and Karen Barnes. Permission to reprint portions of articles or whole articles must be obtained in writing. Please send editorial comments and requests for reprint permission to [email protected]
- Share full article
Advertisement
Supported by
What’s Going On in This Graph? | Unemployment During the Pandemic
Who wants a job, but doesn’t have one? How has the composition of the unemployed in the United States changed during the pandemic?

By The Learning Network
This graph shows the composition of the unemployed in the United States from January – September 2020. The graph appeared elsewhere on NYTimes.com.
By Friday morning, Nov. 6, we will reveal the graph’s free online link, additional background and questions, shout-outs for great student headlines, and Stat Nuggets.
After looking closely at the graph above (or at this full-size image ), answer these four questions:
What do you notice?
What do you wonder?
What impact does this have on you and your community?
What’s going on in this graph? Write a catchy headline that captures the graph’s main idea.
The questions are intended to build on one another, so try to answer them in order.
2. Next, join the conversation online by clicking on the comment button and posting in the box. (Teachers of students younger than 13 are welcome to post their students’ responses.)
3. Below the response box, there is an option for students to click on “Email me when my comment is published.” This sends the link to their response which they can share with their teacher.
4. After you have posted, read what others have said, then respond to someone else by posting a comment. Use the “Reply” button to address that student directly.
On Wednesday, Nov. 4, teachers from our collaborator, the American Statistical Association , will facilitate this discussion from 9 a.m. to 2 p.m. Eastern time.
5. By Friday morning, Nov. 6, we will reveal more information about the graph, including a free link to the article that included this graph, at the bottom of this post. We encourage you to post additional comments based on the article, possibly using statistical terms defined in the Stat Nuggets.
• See all graphs in this series or a slide show of 60 of our favorite graphs .
• View our archives that link to all past releases, organized by topic , graph type and Stat Nugget .
• Learn more about the “ Notice and Wonder ” teaching strategy and how and why other teachers are using this feature from our on-demand webinar .
• Sign up for our free weekly Learning Network newsletter so you never miss a graph. Graphs are always released by the Friday before the Wednesday live-moderation to give teachers time to plan ahead.
• Go to the American Statistical Association K-12 website , which includes teacher statistics resources, professional development opportunities, and more.
Students 13 and older in the United States and the United Kingdom, and 16 and older elsewhere, are invited to comment. All comments are moderated by the Learning Network staff, but please keep in mind that once your comment is accepted, it will be made public.
UPDATED: Nov. 5, 2020
“Covid-19 tore a hole through the U.S. economy,” says The New York Times’ October 3, 2020 article “Job Gains Lose Momentum As Promising Recovery Stalls.” As of October, the national economy had 11 million fewer jobs than before the pandemic. The total number of unemployed workers both in and out of the workforce, which is calculated from different sources than the jobs data, went from 12 million in January 2020, then exploded to 32 million in April 2020 (167 percent increase) before subsiding to 19 million by September 2020. (This was an 58 percent increase over January 2020). It is not surprising that in an October 5-11, 2020 online survey conducted by SurveyMonkey for The New York Times, 30 percent of respondents said that they were financially worse off.
This “vase” graph appeared in the October 12, 2020 New York Times article “ The Shape(s) of a Crisis .” It shows the composition of the “total unemployed”, those who have been temporarily laid off, have been out of work for less than four weeks, or are in the “other” category. The “other” category of unemployed workers includes those who had temporary jobs or who chose to leave their jobs because of transportation issues, needing to care for their family, susceptibility to the virus, and other issues. The graph also shows the number of workers who are not in the labor force (including those who have been unemployed for more than four weeks), but want a job.
How many workers have returned to work? Based on the shapes of the subgroups in the vase graph, which workers may have shifted between from one subgroup to another?
Here are some of the student headlines that capture the stories of these graphs: “Unemployment: The Silent Killer” by Sadie of Dallas; “As Covid-19 Spreads, So Does Unemployment” by Katie of Andover, Massachusetts; “Increasing Pandemic, Lowering Paychecks” by Aboubakary and “A Job Pandemic” by Victor, both of the Bronx, New York; and “Is Corona Firing People?” by Zamir and “Unemployment: A Covid Conundrum” by Aidan, both of New York.
You may want to think critically about these questions:
The number of temporarily laid off workers has diminished since April. Based on evidence in the graph, what employment status could these people now have? Some options may be within the graph; others may be outside the graph.
Some workers are unemployed because of family or transportation issues. What are some of the specifics of these issues? Where would these people be shown in the graph in April? In August?
Workers have been affected differently based on the business sector in which they worked. After studying the graphs in the October 3, 2020 New York Times article “ Jobs Gains Lose Momentum as Promising Recovery Stalls ,” answer these questions:
Which business sectors have seen an increase in jobs?
Which business sectors are seeing the greatest adverse effect due to the pandemic and have reduced their workforce?
Overall, what has been the net effect (increases in the number of employees minus the decreases in the number of employees)? What surprises do you see in job gains and losses?
This graph could be called a vase graph because of its shape. Why do you think the graphic designers used a vase graph rather than the typical time series graph with stacked areas for each of the subgroups with Jan. 2020 – Sept. 2020 on the x -axis and number of unemployed on the y -axis?
Because of the Veteran’s Day holiday on Wednesday, November 11, there will be no graph release for next week. The next release will be by Friday, Nov. 13 with live-moderation on Wednesday, Nov. 18. The topic: Thanksgiving favorite foods. So you don’t miss any releases, you can receive the 2020-2021 “What’s Going On In This Graph?” schedule by subscribing here to the Learning Network Friday newsletter. Keep noticing and wondering.
Stat Nuggets for “ The Shape(s) of a Crisis ”
To see the archives of all Stat Nuggets with links to their graphs, go to this index .
STACKED AREA GRAPH
Stacked area graphs show trends over time and compare the relative sizes of subgroups of a whole. The comparison may be in terms of absolute numbers or percentages.
In the pandemic unemployment graph, the time period is January 2020 to September 2020. The whole is the total number of unemployed plus people who are not in the labor force but want a job. The category of unemployed is divided into the subgroups of temporarily laid off, permanently laid off, and other. The other category includes workers who chose to leave their jobs or who had temporary jobs. What is usual about this graph is that time is on the vertical axis and the number of workers is on the horizontal axis. The variable, number of workers, is represented by the total distance from both sides of the center vertical line.
The graphs for “What’s Going On in This Graph?” are selected in partnership with Sharon Hessney. Ms. Hessney wrote the “reveal” and Stat Nuggets with Erica Chauvet, mathematics professor at Waynesburg University in Pennsylvania, and moderates online with Dashiell Young-Saver, a high school statistics teacher in San Antonio and data science graduate student at Harvard. He is the founder of Skew The Script, which provides relevant math and statistics curriculum to teachers.
How Has the COVID-19 Pandemic Affected the U.S. Labor Market?
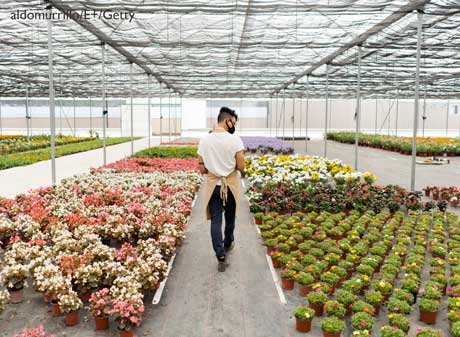
Social distancing and the partial economic shutdown in response to the COVID-19 pandemic have had a profound impact on the U.S. economy, including on people’s jobs and livelihoods.
The overall immediate effects on the labor market have been easy to see: The unemployment rate shot up in the early months of the COVID-19 crisis in the U.S., and payroll employment numbers show that more than 20 million jobs were lost in April—a record amount for one month. (Employment has increased every month since then, and unemployment declined to 7.9% in September after a 14.7% April peak.)
But these aggregate numbers don’t tell the whole story. There are many ways to dissect data to get a more complete sense of how the pandemic has affected the U.S. labor market, including which workers have felt the most impact.
This post provides a roundup of some recent St. Louis Fed analyses that examined different aspects of unemployment and employment during the pandemic. Some takeaways:
- When other measures of unemployment started declining, the share of those unemployed for at least 15 weeks continued to rise.
- The youngest workers saw the biggest decline in employment.
- The leisure and hospitality sector lost the most jobs in the early months of the pandemic.
- The lowest-earning occupations were hit the hardest by the pandemic.
What are various measures of unemployment showing us?
An Oct. 5 FRED Blog post discussed how six measures of labor underutilization from the U.S. Bureau of Labor Statistics (BLS) changed with the pandemic.
Among the measures included in the above graph from the blog post are the official unemployment rate; a measure of those unemployed 15 weeks or longer; and measures that take into account discouraged workers and others who are marginally attached to the labor force, and those who want full-time work but can only get part-time work.
As noted in the post and seen in the graph, all of the measures increased dramatically, but they didn’t all move in parallel—in other words, the distance between the lines didn’t stay constant.
“The lines fanned out, showing that it wasn’t one particular type of unemployment that was responsible for the overall surge,” said the post, which was suggested by Christian Zimmermann , assistant vice president of research information services.
Of particular note, the share of workers unemployed for at least 15 weeks was still increasing in August while the other unemployment measures were decreasing, the post pointed out. These workers made up 5.1% of the labor force in August, although the share ticked down to 4.6% in September.
(For more information, check out this June 2018 Open Vault blog post on the different measures of unemployment and this August 2020 post on labor force participation.)
What do we learn from looking at employment levels by age group?
An Oct. 8 FRED Blog post , also suggested by Zimmermann, breaks down employment levels by age group using data from the BLS.
As shown in the graph above, the youngest groups were hit the hardest during the COVID-19 related recession. The blog post noted that, from February to April:
- 35% of workers 16-19 years old lost their jobs.
- 30% of those 20-24 years old lost their jobs.
- Job losses for the other age groups ranged from 11% to 16%.
The declines in employment for the older age groups were still sizeable, but much smaller than those of the two youngest groups, the post noted.
“Are the young taking one for the team just for this recession?” the post asked. “Or have they always been first to be let go?”
The youngest group saw the largest decline in employment in each of the three previous recessions, as discussed in the post. In some previous cases, those 55 years and older and/or those 45 to 54 years old even saw employment gains. (See graphs for the 2007-09 recession , the 2001 recession and the 1990-91 recession .)
“All in all, it appears ‘normal’ that the 16- to 19-year-old age group is hit hardest by recessions and that the oldest workers are largely unaffected, at least in terms of employment,” the post said. “The current recession is a little different in that the older groups have also been affected, just not as much as the younger groups.”
Which industries have been most affected?
In a Regional Economist article published in August, Senior Economist Maximiliano Dvorkin noted that safety measures have impacted businesses that involve direct contact with customers or clients in particular. He examined which industries were the most affected by labor market disruptions during the early months of the pandemic. (He also analyzed which occupations were most affected, but this post focuses on industries.)
Dvorkin looked at several goods-producing industries and service-providing industries. The industries Dvorkin looked at are mining and logging; construction; durable goods manufacturing; nondurable goods manufacturing; trade, transportation and utilities; information; financial activities; professional and business services; education and health services; leisure and hospitality; other services; and government. He found that the leisure and hospitality services sector saw the largest decline from February to April, with nearly half of these jobs being lost. This was followed by the “other services” sector—which includes businesses such as repair and maintenance and beauty shops—where about one in five jobs was lost.
In contrast, the financial activities sector and the government sector saw relatively small declines in employment over this period, he found.
February to April Payroll Employment Declines by Industry
Leisure and Hospitality: -48.3%
Other Services: -22.0%
Financial Activities: -3.0%
Government: -4.4%
SOURCES: U.S. Bureau of Labor Statistics and Maximiliano Dvorkin’s calculations. Adapted from Table 1 in “ Which Jobs Have Been Hit Hardest by COVID-19? ,” a Regional Economist article by Dvorkin published Aug. 17, 2020.
Which wage earners have been hit the hardest?
In an Economic Synopses essay published in July , Economist Serdar Birinci and Research Associate Aaron Amburgey looked at how the pandemic has impacted various occupations across the earnings distribution.
Overall, they found that workers in occupations with lower average earnings were disproportionally displaced by the pandemic, whereas workers in occupations with higher average earnings were impacted to a lesser extent.
For their analysis, Birinci and Amburgey broke occupations into five equal groups (quintiles) based on the average earnings of someone in that particular line of work. The table below, which is recreated from their essay, shows some examples that are included in each group.
The authors noted that the majority of the jobs that were furloughed or lost between January and April were in the lower-earnings occupations. In particular, they found that occupations in the lowest and second-lowest earnings groups accounted for 34% and 25%, respectively, of the increase in unemployment over that period.
They also found that the unemployment rates for the lower-earnings groups increased by much more than for the higher-earnings groups. For example, workers in occupations in the lowest-earnings group saw their unemployment rate increase by 20.4 percentage points from January to April. In comparison, workers in occupations in the highest-earnings group experienced only a 3.2 percentage point increase in their unemployment rate over that period.
“These results provide further evidence that the COVID-19 crisis has had an unbalanced effect on different earnings groups in the labor market,” they wrote.
1 The industries Dvorkin looked at are mining and logging; construction; durable goods manufacturing; nondurable goods manufacturing; trade, transportation and utilities; information; financial activities; professional and business services; education and health services; leisure and hospitality; other services; and government.
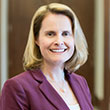
Kristie Engemann is a senior coordinator in the St. Louis Fed External Engagement and Corporate Communications Division.
Related Topics
This blog explains everyday economics, consumer topics and the Fed. It also spotlights the people and programs that make the St. Louis Fed central to America’s economy. Views expressed are not necessarily those of the St. Louis Fed or Federal Reserve System.
Media questions
All other blog-related questions
COVID-19 job and income loss leading to more hunger and financial hardship
Subscribe to global connection, mathieu despard , mathieu despard faculty director - social policy institute at washington university in st. louis michal grinstein-weiss , michal grinstein-weiss nonresident senior fellow - economic studies @michalgw yung chun , and yung chun data analyst iii - social policy institute at washington university in st. louis stephen roll stephen roll research assistant professor, social policy institute, brown school - washington university in st. louis.
July 13, 2020
A startling indicator of the economic impact of COVID-19 is that unemployment rates reached the highest level since the Great Depression in April. As a result, claims for unemployment benefits have risen dramatically, though millions of people who have lost their jobs have been unable to apply or have had trouble applying for this benefit. Yet these figures do not reveal the extent to which households are struggling financially as a result of a COVID-19 related job loss.
To report findings about COVID-19 job and income losses and financial hardships, the Social Policy Institute at Washington University in St. Louis administered a unique nationally representative survey to 5,500 respondents from April 27 to May 12 . A job loss is one of the worst financial shocks most families will face, making it extremely difficult to make ends meet and avoid devastating downstream effects like foreclosures or evictions. During the COVID-19 pandemic, unemployment reached historic heights with more than 40 million unemployment benefit claims filed —even exceeding the unemployment levels during the Great Depression
Impacts of COVID-19 on job loss
The survey found that 24 percent of respondents lost a job or income due to COVID-19. Most of these job or income losses were due to being furloughed or experiencing reduced work hours.
However, these job and income losses were not experienced equally. Hispanic, low-income, and young individuals (between the ages of 18 and 24) had the highest rates of job and income loss compared to other racial/ethnic, income, and age groups, as reflected in the charts below.

While job and income loss rates were very similar among moderate-, middle-, and high-income respondents, low-income and Hispanic respondents—those least able to cope with economic shocks—had a distinctly higher rate of job and income loss. That job and income losses were highest among Hispanic respondents is likely related to their disproportionate representation in industries hard hit by COVID-19 related layoffs such as hospitality and construction. Conversely, black workers are disproportionately represented in industries such as health care and transportation that have been less affected.
Differences in COVID-19 related job and income losses were most pronounced by age. Young adults between the ages of 18 to 24 experienced job or income losses nearly twice as much as older age groups.
Yet are COVID-19 related job and income losses affecting the ability of U.S. households to make ends meet? One way to know is to look at different types of economic hardship, such as difficulty paying for housing and other bills, putting off medical care and filling prescriptions, and experiencing food insecurity. As the graph below indicates, COVID-19 related job and income losses are clearly related to increased hardship such as difficulty making housing payments—even after controlling for income, age, gender, and household size (Figure 4).

Households that experienced a COVID-19-related job or income loss were two to three times more likely to experience economic hardship than those who did not experience a loss, with all of these differences being statistically significant ( p < .001). These hardships reflect important aspects of family well-being related to housing, nutrition, and health.
Other factors, such as race and ethnicity or income, increased the chances of respondents reporting hardships. Compared to white households, black households had significantly higher chances of difficulties making housing and other bill payments ( p < .001) and putting off filing prescriptions ( p < .05). Also, Hispanic households had a much greater chance of experiencing food insecurity ( p < .001) compared to white households. As can be expected, the chances of experiencing hardship were much higher for low-income compared to moderate-, middle-, and high-income households for all types of hardship.
Survey respondents were also asked whether COVID-19 has made it hard to afford food for the adults and children in their households. Again, COVID-19 job and income loss is a significant factor. Households experiencing a job or income loss were more than twice as likely to have a hard time paying for food for adults, after controlling for income, age, gender, and household size (Figure 5).

Consequences of economic hardship
These survey findings illustrate that the economic pain of families who have lost jobs or income due to COVID-19 is very real. These findings look beyond the official unemployment and job loss figures to see just how affected families are struggling. These findings are particularly notable given that the survey administered by Social Policy Institute was conducted early in the pandemic (April 2020) and is the first of several waves administered every three months in 2020.
The hardships presented here have many negative consequences for households. Difficulty paying rent puts households and families at risk for eviction, especially when eviction moratoria some municipalities implemented expire and households struggle to pay back-due rent. The negative downstream effects of eviction are varied and far-reaching: loss of possessions, disruptive school changes, loss of friends for children, and mental health distress just to name a few.
The health consequences of food insecurity are far-reaching. Among children, consequences include birth defects, anemia, asthma, and cognitive, behavioral, and mental health problems. Compounding these issues, individuals may put off medical care or avoid filling prescriptions as a result of the high cost and loss of income. These individuals jeopardize their health, especially if they have serious or chronic illnesses.
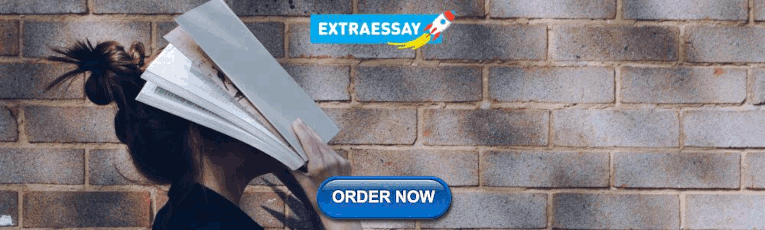
Helping families cope with job and income loss during the COVID-19 pandemic
The federal Coronavirus Aid, Relief, & Economic Security (CARES) Act signed into law in late March 2020 includes various provisions that ought to help many struggling families, including extended unemployment benefits and economic impact payments . However, CARES Act limitations calling for additional policy responses remain:
- Additional unemployment benefit payments of $600 a week are set to expire in July 2020 . Laid-off workers in hard-hit industries like hospitality that will take longer to recover will struggle if they lose these extra payments.
- No provision for expanded health coverage or help to transition to Affordable Care Act plans for workers who lost health insurance when they were laid off or for furloughed workers who could not afford their insurance premiums.
- No provision for expanding and extending direct rental assistance. While many municipalities and states have implemented eviction moratoriums, many are set to expire in July, or when disaster or public health emergency declarations expire. A looming eviction crisis is on the horizon among tenants who won’t be able to pay back-due rent. However, Congress can pass additional legislation regarding emergency housing assistance and use the COVID-19 crisis as an opportunity to tackle the country’s affordable housing crisis.
Related Content
Alan Berube
May 13, 2020
Annelies Goger, Tracy Hadden Loh, Caroline George
April 6, 2020
Ingrid Ellen, Erin Graves, Katherine O’Regan, Jenny Schuetz
June 8, 2020
Labor & Unemployment
Global Economy and Development
March 29, 2024
William A. Galston
March 28, 2024
Joseph W. Kane, Fred Dews
ORIGINAL RESEARCH article
Unemployment, employability and covid19: how the global socioeconomic shock challenged negative perceptions toward the less fortunate in the australian context.
- 1 Research School of Population Health, The Australian National University, Canberra, ACT, Australia
- 2 Institute of Child Protection Studies, The Australian Catholic University, Melbourne, VIC, Australia
- 3 Melbourne Institute of Applied Economic and Social Research, The University of Melbourne, Melbourne, VIC, Australia
Unemployed benefit recipients are stigmatized and generally perceived negatively in terms of their personality characteristics and employability. The COVID19 economic shock led to rapid public policy responses across the globe to lessen the impact of mass unemployment, potentially shifting community perceptions of individuals who are out of work and rely on government income support. We used a repeated cross-sections design to study change in stigma tied to unemployment and benefit receipt in a pre-existing pre-COVID19 sample ( n = 260) and a sample collected during COVID19 pandemic ( n = 670) by using a vignette-based experiment. Participants rated attributes of characters who were described as being employed, working poor, unemployed or receiving unemployment benefits. The results show that compared to employed characters, unemployed characters were rated substantially less favorably at both time points on their employability and personality traits. The difference in perceptions of the employed and unemployed was, however, attenuated during COVID19 with benefit recipients perceived as more employable and more Conscientious than pre-pandemic. These results add to knowledge about the determinants of welfare stigma highlighting the impact of the global economic and health crisis on perception of others.
Introduction
The onset of COVID19 pandemic saw unemployment climb to the highest rate since the Great Depression in many regions globally 1 . Over just one month, from March to April 2020 unemployment rate in the United States increased from 4.4% to over 14.7% and in Australia the effective rate of unemployment increased from 5.4 to 11.7% ( Australian Bureau of Statistics, 2020 ) 2 . In Australia, a number of economic responses were rapidly introduced including a wage subsidy scheme (Jobkeeper) to enable employees to keep their employees connected to the workforce, one-off payments to many welfare recipients, and a doubling of the usual rate of the unemployment benefits (Jobseeker payment) through a new Coronavirus supplement payment. At the time of writing in July 2020, many countries, including Australia remain in the depths of a health and economic crisis.
A rich research literature from a range of disciplines has documented the pervasive negative community views toward those who are unemployed and receiving unemployment benefits, with the extent of this “welfare stigma” being particularly pronounced in countries with highly targeted benefit systems such as the United States and Australia ( Fiske et al., 2002 ; Baumberg, 2012 ; Contini and Richiardi, 2012 ; Schofield and Butterworth, 2015 ). The stigma and potential discrimination associated with unemployment and benefit receipt are known to have negative impacts on health, employability and equality (for meta-analyses, see Shahidi et al., 2016 ). In addition, the receipt of unemployment benefits co-occurs with other stigmatized characteristics such as poverty and unemployment ( Schofield and Butterworth, 2018a ). The changing context related to the COVID19 crisis provides a novel opportunity to better understand the determinants of stigmatizing perceptions of unemployment and benefit receipt.
Negative community attitudes and perceptions of benefit recipients are commonly explained by the concept of “deservingness” ( van Oorschot and Roosma, 2017 ). The unemployed are typically seen as less deserving of government support than other groups because they are more likely to be seen as responsible for their own plight, ungrateful for support, not in genuine need ( Petersen et al., 2011 ; van Oorschot and Roosma, 2017 ), and lacking reciprocity (i.e., seen as taking more than they have given – or will give – back to society; van Oorschot, 2000 ; Larsen, 2008 ; Petersen et al., 2011 ; Aarøe and Petersen, 2014 ). Given the economic shock associated with COVID19, unemployment and reliance on income support are less likely to seen as an outcome within the individuals control and may therefore amplify perceptions of deservingness. Prior work has shown that experimentally manipulating perceived control over circumstances does indeed change negative stereotypes ( Aarøe and Petersen, 2014 ).
A number of experimental paradigms have been used to investigate perceptions of “welfare recipients” and the “unemployed.” The stereotype content model (SCM; Fiske et al., 2002 ), for example, represents the stereotypes of social groups on two dimensions: warmth, relating to being friendly and well–intentioned (rather than ill–intentioned); and competence, relating to one’s capacity to pursue intentions ( Fiske et al., 2002 ). Using this model, the “unemployed” have been evaluated as low in warmth and competence across a variety of welfare regime types ( Fiske et al., 2002 ; Bye et al., 2014 ). The structure of stereotypes has also been studied using the Big Five personality dimensions ( Schofield and Butterworth, 2018b ; Schofield et al., 2019 ): Openness, Conscientiousness, Extraversion, Agreeableness, and Emotional Stability (for background on the Big Five see: Goldberg, 1993 ; Hogan et al., 1996 ; Saucier and Goldberg, 1996 ; McCrae and Terracciano, 2005 ; Srivastava, 2010 ; Chan et al., 2012 ; Löckenhoff et al., 2014 ). There are parallels between the Big Five and the SCM: warmth relating to the dimension of Agreeableness, and competence relating to Conscientiousness ( Digman, 1997 ; Ward et al., 2006 ; Cuddy et al., 2008 ; Abele et al., 2016 ) and these constructs have been found to predict employability and career success ( Barrick et al., 2001 ; Cuesta and Budría, 2017 ). Warmth and agreeableness have also been linked to the welfare-specific characteristics of deservingness ( Aarøe and Petersen, 2014 ).
The term “employability” has been previously defined as a set of achievements, skills and personal attributes that make a person more likely to gain employment and leading to success in their chosen career pathway ( Pegg et al., 2012 ; O’Leary, 2017 , 2019 ). While there are few studies examining perceptions of others, perceptions of one’s own employability have been recently studied in university students, jobseekers ( Atitsogbe et al., 2019 ) and currently employed workers ( Plomp et al., 2019 ; Yeves et al., 2019 ), consistently showing higher levels of perceived employability being linked to personal and job-related wellbeing as well as career success. Examining other’s perceptions of employability may be more relevant to understand factors impacting on actual employment outcomes. A majority of studies examining other’s perceptions of employability have focused on job specific skills study ( Lowden et al., 2011 ; Dhiman, 2012 ; Saad and Majid, 2014 ).
Building on this previous work, our own research has focused on the effects of unemployment by drawing on frameworks of Big Five, SCM and employability in pre-COVID19 samples ( Schofield and Butterworth, 2018b ; Schofield et al., 2019 ). Our studies consistently show that unemployed individuals receiving government payments are perceived as less employable (poorer “quality” workers and less desirable for employment) and less Conscientious. We found similar but weaker pattern related to Agreeableness, Emotional Stability, and the extent that a person is perceived as “uniquely human” ( Schofield et al., 2019 ). Further, we found that vignette characters described as currently employed but with a history of welfare receipt were indistinguishable from those described as employed and with no reference to benefit receipt ( Schofield et al., 2019 ). Findings such as this provide experimental evidence that welfare stigma is malleable and can be challenged by information inconsistent with negative stereotype ( Schofield and Butterworth, 2018b ; Schofield et al., 2019 ; see also Petersen et al., 2011 ).
The broad aim of the current study was to extend this previous work by examining the impact of COVID19 on person perceptions tied to employment and benefit recipient status. It repeats a pre-COVID19 study of an Australian general population sample in the COVID19 context, drawing on the same sampling frame, materials and study design to maximize comparability. The study design recognizes that the negative perceptions of benefit recipients may reflect a combination of difference sources of stigma: poverty, lack of work, and benefit receipt. Therefore, the original study used four different conditions to seek to differentiate these different sources: (1) Employed ; (2) Working poor ; (3) Unemployed ; and (4) Unemployed benefit recipient . Finally, for the COVID19 sample we added a novel fifth condition: (5) Unemployment benefit recipient also receiving the “Coronavirus” supplement . We except that the reference to a payment specifically applicable to the COVID19 context may lead to more favorable perceptions (more deserving) than the other unemployed and benefit receipt characters.
The study capitalizes on a major exogenous event, the COVID19 crisis, which we hypothesize will alter perceptions of deservingness by fundamentally challenging social identities and perceptions of one’s own vulnerability to unemployment. The study tests three hypotheses, and in doing so makes an important empirical and theoretical contribution to understanding how deservingness influences person perception, and understanding of the potential “real world” barriers experienced by people seeking employment in the COVID19 context.
Hypothesis 1
The pre-COVID19 assessment uses a subset of data from a pre-registered study, but this reuse of the data was not preregistered 3 . We hypothesize that, at Time 1 (pre-COVID19 assessment) we will find that employed characters will be rated more favorably than characters described as unemployed and receiving unemployment benefits, particularly on dimensions of Conscientiousness, Worker and Boss suitability. Moreover, we expect a gradient in perceptions across the four experimental conditions, from employed to working poor, to unemployed to unemployed receiving benefits and to show a similar trend for the other outcome measures included in the study.
Hypothesis 2
We hypothesize that the character in the unemployed condition(s) would be rated less negatively relative to the employed condition(s) at Time 2, compared to Time 1. We predict a two-way interaction between time and condition for the key measures (Conscientiousness, Worker and Boss suitability) and a similar trend on other outcomes.
Hypothesis 3
We expect that explicit reference to the unemployed benefit character receiving the “Coronavirus supplement” payment will increase the salience of the COVID19 context and lead to more positive ratings of this character relative to the standard unemployed benefit condition in the pre-COVID19 and COVID19 occasions.
Materials and Methods
Participants.
Two general population samples (pre-COVID19 and COVID19) were recruited from the same source: The Australian Online Research Unit (ORU) panel. The ORU is an online survey platform that provides access to a cohort of members of the general public who are interested in contributing to research. The ORU randomly selects potential participants who meet study eligibility criteria, and provides the participant with an incentive for their participation. The sample for the Time 1 (pre-COVID19) occasion was part of a larger study (768 participants) collected in November 2018. From this initial dataset, we were able to use data from 260 (50.1% female, M Age = 42.1 [16.7] years, range: 18–82) participants who were presented with the one vignette scenario that we could replicate at the time of the social restrictions applicable in the COVID19 context (i.e., the vignette character was not described as going out and visiting friends, as these behaviors were illegal at Time 2). The sample for Time 2 (COVID19) was collected in May–June 2020, at the height of the lock down measures in Australia and included 670 participants (40.5% female, M Age = 51.0 [15.8] years, range: 18–85). The two samples were broadly similar (see below), though the proportion of male participants at Time 2 was greater than at Time 1.
The pre-COVID assessment at Time 1 was restricted to those participants who completed the social-distancing consistent vignette in the first place to avoid potential order/context effects. This provided, on average, 65 respondents in each of the four experimental conditions. Using the results from our previous published studies as indicators of effect size ( Schofield and Butterworth, 2018b ; Schofield et al., 2019 ). Monte Carlo simulation was used to identify the Time 2 sample size that would provide 90% power to detect an interaction effect that represented a 50% decline in the difference between the two employment and two unemployment conditions on the three-key measures at the COVID occasion relative to the pre-COVID difference. This sample size of 135 per condition also provided between 60 and 90% power to detect a difference of a similar magnitude between the employed and unemployment benefit conditions across the two measurement occasions. Given previous evidence that the differences between employed and unemployed/welfare conditions is robust and large for Conscientiousness and Worker suitability ( Schofield and Butterworth, 2018b ), the current study is also adequately powered to detect the most replicable effects of unemployment and welfare on perceptions of a person’s character (even in the absence of the hypothesized interaction effect).
Materials and Procedure
The procedures were identical on both study occasions. Participants read a brief vignette that described a fictional character, and then rated the character on measures reflecting personality dimensions, their suitability as a worker or boss, morality, warmth, and competence, and the participant’s beliefs the character should feel guilt and shame, or feel angry and disgusted. At Time 1 (pre-COVID19 context) participants then repeated this process with a second vignette, but we do not consider data from the second vignette.
Manipulation
The key experimental conditions were operationalized by a single sentence embedded within the vignette that was randomly allocated to different participants (employed: “S/he is currently working as a sales assistant in a large department store”; working poor: “S/he is currently working as a sales assistant, on a minimum-wage, in a large department store”; unemployed: “S/he is currently unemployed”; and receipt of unemployment benefits: “S/he is currently unemployed, and is receiving government benefits due to his/her unemployment”). The four experimental conditions were identical at both time points. At Time 2, an additional COVID19-specific condition was included (to maximize the salience of the COVID19 context): “S/he is currently unemployed and is receiving government benefits, including the Coronavirus supplement, due to his/her unemployment.”
All three study conditions will imply poverty/low income. In Australia, few minimum-wage jobs are supplemented by tips, and so a minimum-wage job indicates a level of relative poverty. A full-time worker in a minimum wage job is in the bottom quartile of income earners ( Australian Bureau of Statistics, 2017 ). Prior to the COVID19 crisis and the increase in payment level, a single person with no dependents receiving unemployment benefits received approximately 75% of the minimum-wage in cash assistance. During COVID19 and at the time of the data collection, the rate of pay exceeds the minimum-wage.
Several characteristics of the vignette character, including age and relationship status, were balanced across study participants. Age was specified as either 27 or 35 years, relationship status was either “single” or “lives with his/her partner.” The character’s gender was also varied and names were stereotypically White.
For Time 1, manipulated characteristics yielded 32 unique vignettes, comprised of four key experimental conditions (employed, working poor, unemployed, and unemployment benefits) × 2 ages × 2 genders × 2 relationship statuses. For Time 2, manipulated characteristics yielded 40 unique vignettes, comprised of five key experimental conditions (employed, working poor, unemployed, unemployment benefits, and unemployed + coronavirus supplement) × 2 ages × 2 genders × 2 relationship statuses. The vignette template construction is presented in Figure 1 including each component of the vignette that was randomly varied.
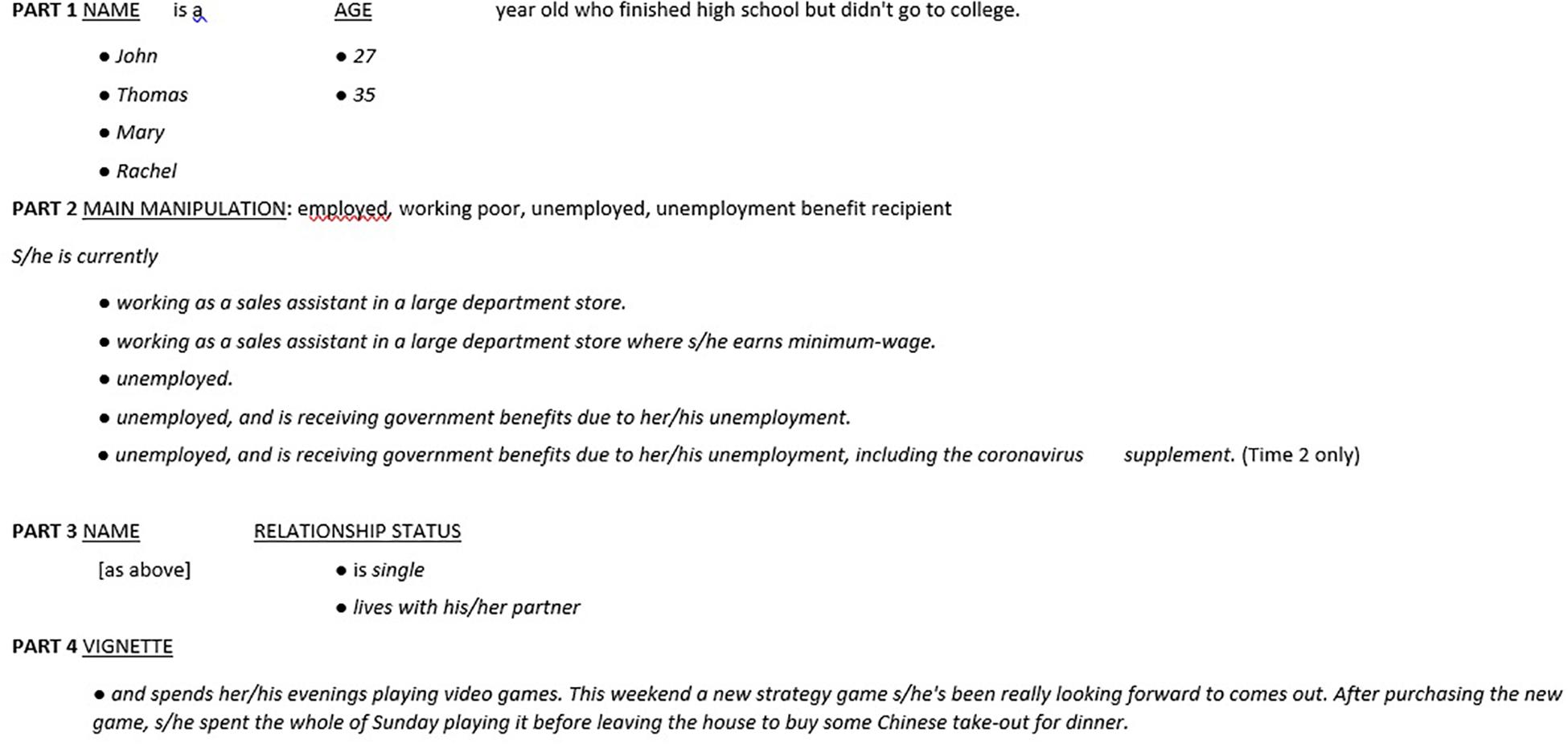
Figure 1. Outline of vignette construction in 4 parts. Bullet pointed options replace the underlined text, with gendered pronouns in each option selected to match character name.
Comprehension Checks
In both studies, participants were required to affirm consent after debriefing or had their data deleted. Participant comprehension of the vignettes was checked via three free-response comprehension questions about the character’s age and weekend activities. Participants who did not answer any questions correctly were not able to continue the study.
Outcome Measures
Personality, employability (suitability as a worker or boss), communion and agency, cognitive and emotional moral judgments, and dehumanization were included as the study outcomes. While not all personality or character dimension measures can be considered as negative or positive, higher scores were used in the study to indicate more “favorable” perceptions by the participants of the characters.
Personality
The Ten Item Personality Inventory was used to measure the Big Five ( Gosling et al., 2003 ) and adapted to other–oriented wording (i.e., “I felt like the person in the story was…”) ( Schofield et al., 2019 ). Two items measured each trait via two paired attributes. One item contained positive attributes and one contained negative attributes. Participants indicated the extent to which “I think [Name] is [attributes]” from 1 (strongly disagree) to 7 (strongly agree). The order of these 10 items was randomized. Agreeableness (α = 0.54) was assessed from “sympathetic, warm” and “critical, quarrelsome” (reversed); Extraversion (α = 0.50) was assessed from “extraverted, enthusiastic” and “reserved, quiet” (reversed); Conscientiousness (α = 0.76) was assessed from “dependable, self-disciplined” and “disorganized, careless” (reversed); Openness to experience (α = 0.36) was assessed from “open to new experiences, complex” and “conventional, uncreative” (reversed); Emotional stability (α = 0.65) was assessed from “calm, emotionally stable.” and “anxious, easily upset” (reversed). The order of these 10 items was randomized.
Employability
Single item measures: “I think [Name] would be a good worker” ( Worker suitability ) and “I think [Name] would be a good boss” ( Boss suitability ) were rated on the same scale as the personality measure. The order of these two items was randomized. Higher scores indicated better employability.
Communion and Agency
Communion and agency was assessed using Bocian et al. (2018) adaptation of Abele et al. (2016) scale that measures the fundamental dimensions of communion and agency using two-subscales for each dimension. The morality and warmth subscales are seen as measures of communion (referred to as warmth in SCM; Fiske, 2018 ); while the competence and assertiveness subscales measure agency (what Fiske refers to as competence in SCM; Fiske, 2018 ). This subscale structure has been identified in multiple samples. Participants indicated the extent to which “I think [Name] [attributes]” from 1 (not at all) to 5 (very much so). Morality (α = 0.92) was measured with six items, e.g., “is just,” “is fair”; Warmth (α = 0.96) with six items, e.g., “is caring,” “is empathetic”; Competence (α = 0.90) with five items, e.g., “is efficient,” “is capable”; and Assertiveness (α = 0.83) with six items, e.g., “is self-confident,” “stands up well under pressure.” These items were presented in a random order.
Dehumanization
Dehumanization was measured with a composite scale of two-items drawn from Bastian et al. (2013) . Based on prior research, we measured dehumanization with two items: “I think [Name] is mechanical and cold, like a robot” and “I think [Name] lacked self-restraint, like an animal” order of these two items was randomized. We reverse coded the two items for the analyses for consistency for the other variables, so that higher scores were indicative of more favorable perceptions.
Moral Emotions
Moral emotions were measured by four items that asked about emotional responses to the character that were framed as self-condemning or other-condemning ( Haidt, 2003 ; Giner-Sorolla and Espinosa, 2011 ). Two other-condemning items asked the participant about their own emotional response to the character in the vignette (Anger: “[Name]’s behavior makes me angry”; Disgust: “I think [Name] is someone who makes me feel disgusted,” α = 0.92). The two self-condemning items asked about the character’s emotional response (Guilt: “[Name] should feel guilty about [his/her] behavior”; Shame: “I think [Name] should feel ashamed of [him/her]self”; α = 0.95). We reverse coded the two scales to ensure consistency with other variables, with higher scores indicative of more favorable perceptions.
Analytical Strategy
With the exception of the Moral emotion (and Communion and Agency) scales that are new to this study and the previously tested Openness to Experience, our previous research has demonstrated differences between the ratings of employed and unemployed characters on the included outcome measures ( Schofield and Butterworth, 2018b ; Schofield et al., 2019 ). We undertake the analysis using a four-step process. We use mixed-effects multi-level models, with the 14 outcome measures nested within participants, and predicted by fixed (between-person) terms representing the experimental “Condition,” “Time” (pre-/COVID19) and their interaction, and controlling for measure differences and allowing for random effects at the participant level: i) We initially assessed the effect of condition in the pre-COVID19 occasion to establish the baseline pattern of results; ii) we then evaluated the interaction term and, specifically, the extent to which the baseline difference observed between employment and unemployment conditions is attenuated at Time 2 (COVID19 occasion); iii) we tested the three-way interaction between condition, occasion and measure to assess whether this two-way interaction varies across the outcome measures; and if significant iv) repeated the modeling approach using separate linear regression models for each outcome measure. Our initial model contrasts the two employed (employed and working poor) and unemployed (unemployed and benefit receipt) conditions. The second model examines the four separate vignette conditions separately, differentiating between unemployed and unemployed benefit conditions. Finally, we contrast the three unemployment benefit conditions: (1) unemployment benefit recipients at Time 1; (2) unemployment benefit recipients at Time 2; and (3) unemployment benefit recipients receiving the Coronavirus payment at Time 2. For all models, we consider unadjusted and adjusted results (controlling for participant demographics). To address a potential bias from gender differences between samples, post-stratification weights were calculated for the COVID19 sample to reflecting the gender by age distribution of the pre-COVID19 sample. All models were weighted.
The two samples from Time 1 (pre-COVID19) and Time 2 (COVID19) were comparable on all demographic variables, except for gender (χ 2 [1, 923] = 7.04, p < 0.001) and employment (χ 2 [1, 910] = 27.66, p < 0.001): The gender distribution was more balanced at Time 1 with 49.8% of males, compared to 59.5% of males at Time 2. There was also a significant increase in unemployment with 20.9% of Time 1 participants out of work compared to 39.3% of the Time 2 participants. This was likely reflective of the employment rate nearly doubling in Australia during COVID19 crisis. Bivariate correlations showed significant positive correlations between all 14 outcomes ( p ’s < 0.001), except for Extraversion that was only positively correlated with Emotional Stability, boss suitability, warmth, assertiveness, and competence ( p’ s < 0.05).
Contrasting Employed and Unemployed Characters
The results, both adjusted and unadjusted, from the initial overall multilevel model using a binary indicator of whether vignette characters were employed (those in the employed or working poor conditions) or unemployed (unemployed or welfare) and testing the interaction between vignette Condition and Time (pre-COVID19 vs COVID19) are presented in the Supplementary Table S1 . The adjusted results (holding participant age, gender, employment, and education constant) indicated that the unemployed characters were rated lower than the employed characters at Time 1 ( b = −0.57). This difference in the ratings of employed and unemployed characters was reduced in the COVID19 assessment at Time 2, declining from 0.57 to 0.26, across all the outcome measures. The addition of the three-way interaction between Condition, Time and outcome measure significantly improved overall model fit, χ 2 (52) = 482.94, p < 0.001, indicating the interaction between Condition and Time varied over measures.
A series of separate regression models considering each outcome separately (see Supplementary Table S2 ) showed a significant effect of Condition (employment rated higher than unemployment) at Time 1 (pre-COVID) for all outcomes except Openness and Extraversion. The lower ratings for unemployed relative to employed characters were significantly moderated at Time 2 on the Competence, Worker and Boss suitability, and Guilt/Shame outcomes ( p’ s < 0.05).
COVID19 and Perceptions of Unemployment Benefit Recipients
The next set of analyses consider the four separate vignette conditions, differentiating between the unemployed and unemployed benefit recipient conditions. The overall mixed-effects multilevel model incorporating the four distinct vignette conditions provided evidence of significant effects for Condition and Condition by Time in both adjusted and unadjusted models. The result for the adjusted model ( Table 1 ), averaged across the various outcomes, replicated the previous finding of a difference in ratings of employed and unemployed characters at Time 1 (pre-COVID19): relative to the employed condition, there was no difference in ratings of the working poor, but the unemployed and the unemployed benefit recipient characters were rated less favorably. There was some evidence of a gradient across the unemployed characters: the average rating of the unemployed condition was higher than the unemployed benefit condition, though this difference was not statistically significant. In the presence of the interaction effect, the non-significant effect of Time shows that, averaged across all the outcome measures, there was no difference in the rating of the characters in the employed condition on the pre-COVID19 and COVID19 occasions. We tested for the effect of sociodemographic characteristics as covariates in the adjusted models (employment and benefit receipt status, education, age, and gender) but found no main effects of any of the covariates except for gender: females tended to rate characters higher ( b = 0.13, 95% CI [0.04, 0.21]) compared to males. Testing the heterogeneity of these patterns across outcomes via the inclusion of a three-way interaction between vignette condition, occasion and measure significantly improved overall model fit, χ 2 (104) = 533.40, p < 0.001, prompting analysis of each outcome separately.
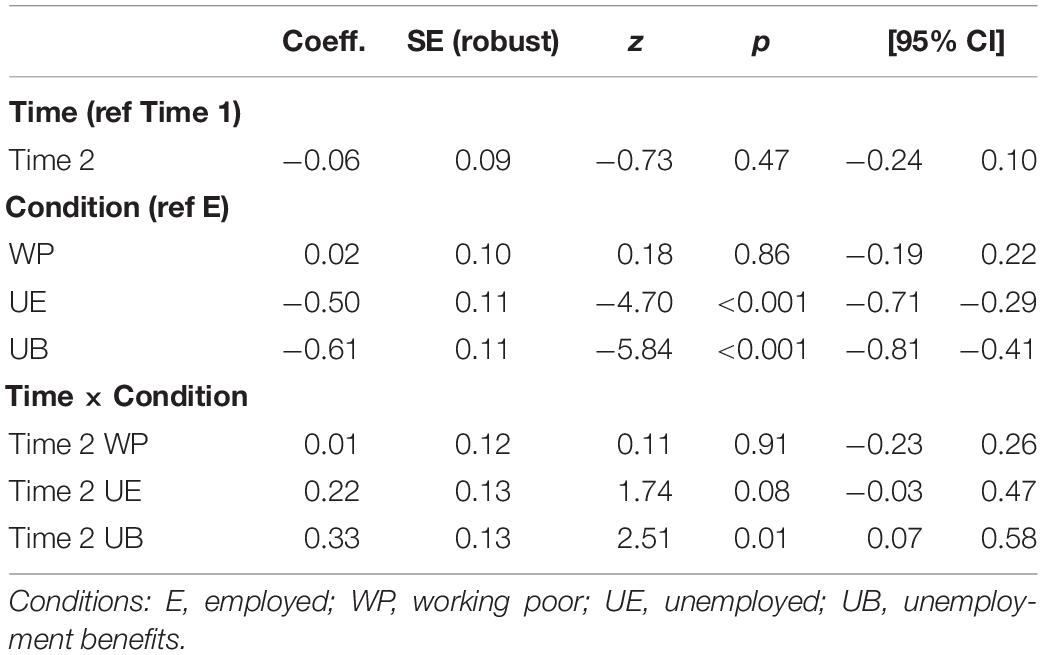
Table 1. Adjusted fixed effects estimates of outcomes as a function of interactions between condition and time.
The separate linear regressions for each outcome measure ( Supplementary Table S3 ) show that ratings of unemployed benefit recipients at the Time 1 (pre-COVID19) were significantly lower than the employed characters for all outcomes except Openness and Extraversion. Statistically significant Condition by Time terms indicated that the unemployed benefit effect was moderated at Time 2 (COVID19) for the three key outcome measures identified in previous research (Conscientiousness, Worker and Boss suitability) and for the measure of Guilt and Shame. Figure 2 depicts this interaction for these four outcomes. These occurred in two profiles. For Conscientiousness, Worker and Boss suitability, COVID19 attenuated the negative perceptions of unemployed relative to employed characters, providing support for Hypothesis 2. By contrast, COVID19 has induced a new difference, such that participants thought employed characters should feel higher levels Guilt and Shame at Time 2, compared to Time 1. While the “working poor” condition was not central to the COVID19 hypotheses, we note that we found no evidence that ratings of these characters on any outcome differed from the standard employed character, or that this difference was changed in assessment at Time 2 (COVID19 occasion).
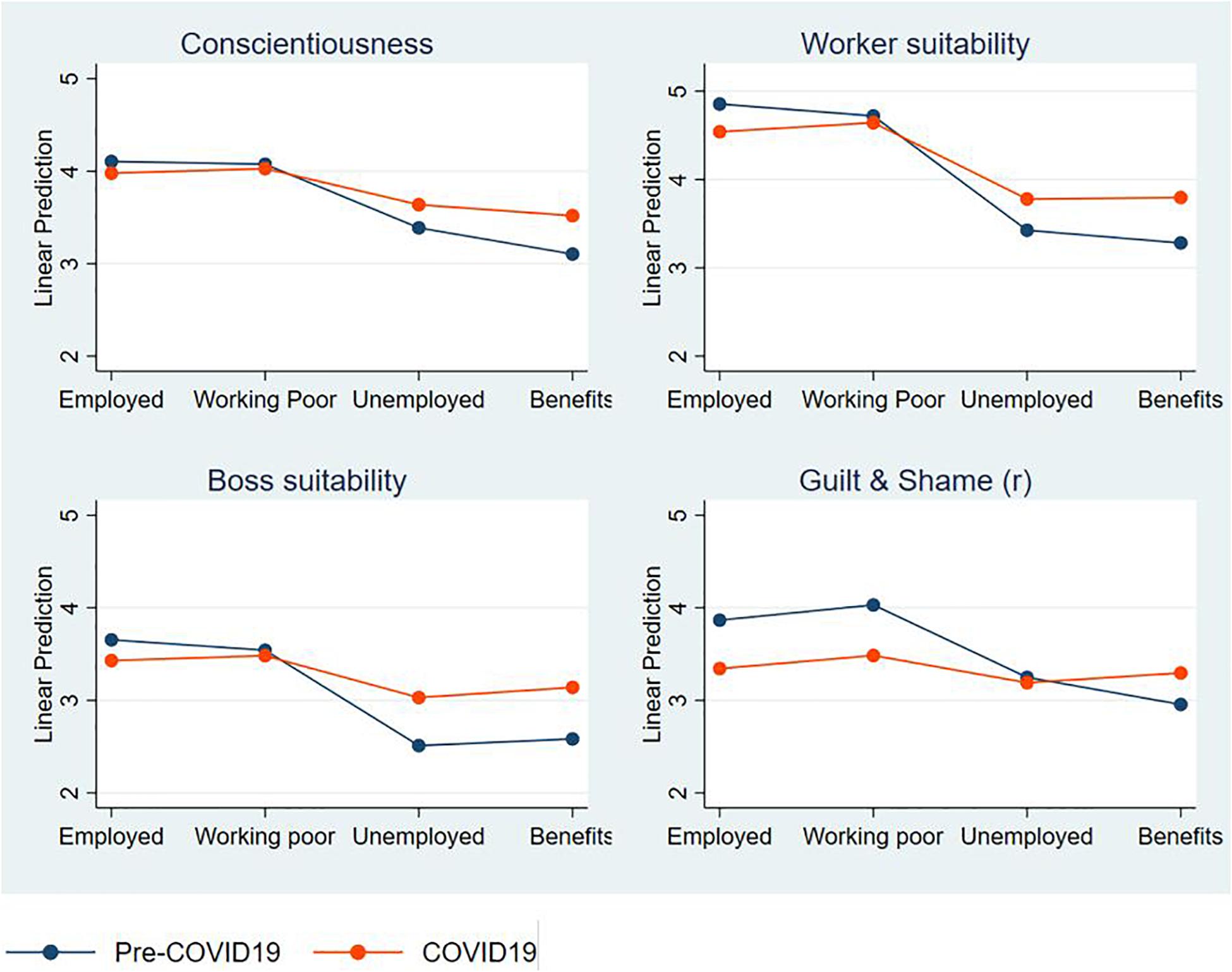
Figure 2. Interaction effect of Time (COVID19) by Condition marginal mean ratings on four outcomes: Conscientiousness, Worker suitability, Boss suitability, and Guilt and Shame (reversed).
The Impact of COVID19 on Perceptions of Unemployment Benefit-Recipients
The inclusion of the fifth COVID19-specific unemployment benefit condition did not generate more positive (or different) ratings than the standard unemployment benefit condition. Overall mixed-effects multilevel models, both adjusted and unadjusted, indicated that participants in the Coronavirus supplement condition (adjusted model: b = 0.26, 95% CI [0.06, 0.45]) and the general unemployed benefit recipient condition at Time 2 (adjusted model: b = 0.28, 95% CI [0.08, 0.48]) were both rated more favorably in comparison to unemployed benefit recipients at Time 1. There was no difference between these two Time 2 benefit recipient groups ( b = 0.03, 95% CI [−0.12, 0.19]). These results did not support hypothesis 3.
Previous research has demonstrated that people who are unemployed, and particularly those receiving unemployment benefits, are perceived more negatively and less employable than those who are employed. However, the economic shock associated with the COVID19 crisis is likely to have challenged people’s sense of their own vulnerability and risk of unemployment, and altered their perceptions of those who are unemployed and receiving government support. The broad aim of the current study was to examine the potential effect of this crisis on person perceptions tied to employment and benefit recipient status. We did this by presenting brief vignettes describing fictional characters, manipulating key experimental conditions related to employment status, and asking study participants to rate the characters’ personality and capability. We contrasted results from two cross-sectional general population samples collected before and during the COVID19 crisis.
The pre-COVID19 assessment replicated our previous findings (e.g., Schofield and Butterworth, 2018b ) showing that employed characters are perceived more favorably than those who were unemployed and receiving government benefits on measures of Conscientiousness and suitability as a worker. These findings supported Hypothesis 1. In comparison, the assessment conducted during the COVID19 crisis showed that unemployed and employed characters were viewed more similarly on these same key measures, with a significant interaction effect providing support for Hypothesis 2. Our third hypothesis, suggesting that n reference to the Coronavirus Supplement (an additional form of income support introduced during the pandemic) would enhance ratings of unemployed benefit recipients at the second assessment occasion, was not supported. We found that benefit recipients at Time 2 were rated more favorably than the benefit group at Time 1, irrespective of whether this COVID19-specific payment was referenced. This suggests the broader context in which the study was conducted was responsible for the change in perceptions.
We sampled participants from the same population, used identical experimental procedures, and found no difference over time in the ratings of employed characters on the key outcome measures of employability (Worker and Boss suitability) and Conscientiousness. The more favorable ratings of unemployed and benefit receiving characters at Time 2 is likely to reflect how the exogenous economic shock brought about by the COVID19 crisis challenged social identities and the stereotypes held of others 4 . The widespread impact and uncontrollable nature of this event are inconsistent with pre-COVID19 views that attribute ill-intent to those receiving to unemployment benefits ( Fiske et al., 2002 ; Baumberg, 2012 ; Contini and Richiardi, 2012 ; Bye et al., 2014 ). We suggest the changing context altered perceptions of the “deservingness” of people who are unemployed as unemployment in the context of COVID19 is less indicative personal failings or a result of one’s “own doing” ( Petersen et al., 2011 ; van Oorschot and Roosma, 2017 ). It is important to recognize, however, that the negative perceptions of unemployed benefit recipients were attenuated in the COVID19 assessment, but they continued to be rated less favorably than those who were employed on the key outcome measures.
In contrast to our findings on the key measures of employability and Conscientiousness, the previous and current research is less conclusive for the other outcome measures. The current study showed a broadly consistent gradient in the perception of employed and unemployed characters for all outcome measures apart from Openness and Extraversion. Findings on these other measures have been weaker and inconsistent across previous studies ( Schofield and Butterworth, 2018b ; Schofield et al., 2019 ), and the current experiment was not designed with sufficient power to demonstrate interaction effects for these measures. There was, however, one measure that showed significant divergence from the expected profile of results. A significant interaction term suggested that study participants at the Time 2 (COVID19) assessment reported that the employed characters should feel greater levels of Guilt and Shame than those who participated in the pre-COVID19 assessment. In contrast, there was consistency in the ratings of unemployed characters on this measure across the two assessment occasions. While not predicted, these results are also interpretable in the context of the pervasive job loss that accompanied the COVID19 crisis. Haller et al. (2020) , for example, argue that the highly distressing, morally difficult, and cumulative nature of COVID19 related stressors presents a perfect storm to result in a guilt and shame responses. The context of mass job losses may leave “surviving” workers feeling increasingly guilty.
The main findings of the current study are consistent with previous experimental studies that show that the stereotypes of unemployed benefit recipients are malleable ( Aarøe, 2011 ; Schofield et al., 2019 ). These previous studies, however, have demonstrated malleability by providing additional information about unemployed individuals that was inconsistent with the unemployed benefit recipient stereotype (e.g., the external causes of their unemployment). In contrast, the current study did not change how the vignette characters were presented or the experimental procedures. Rather, we assessed how the changing context in which study participants were living had altered their perceptions: suggesting the experience of COVID19 altered stereotypical views held by study participants rather than presenting information about the character that would challenge the applicability of the benefit recipient stereotype in this instance.
Perceptions and stereotypes of benefit recipients can be reinforced (and potentially generated) by government actions and policies. Structural stigma can be used as a policy tool to stigmatize benefit receipt as a strategy to reduce dependence on income support and encourage workforce participation ( Moffitt, 1983 ; Stuber and Schlesinger, 2006 ; Baumberg, 2012 ; Contini and Richiardi, 2012 ; Garthwaite, 2013 ). In the current instance, however, the Australian government acted quickly to provide greater support to Australians who lost their jobs (e.g., doubling the rate of payment, removing mandatory reporting to the welfare services) and this may have reduced the stigmatizing structural features of the income support system and contributed to the changed perceptions of benefit recipients identified in this study.
Limitations
The current study took advantage of a natural experimental design and replicated a pre-COVID19 study during the COVID19 crisis. The study is limited by the relatively small sample size at Time 1, which was not designed for current purposes but part of another study. We were not able to include most of the participants from the original Time 1 study as most of the experimental conditions described activities that were illegal/inconsistent with recommend activity at the time of the COVID19 lockdown and social restriction measures. Finally, the data collection for the current study occurred very quickly after the initial and sudden COVID19 lockdowns and economic shock, which is both a strength and a limitation for the generalizability of the results. The pattern of results using the same sampling frame offers compelling support for our hypothesis that the shared economic shock and increase in unemployment attenuates stigmatizing community attitudes toward those who need to receive benefits. Our current conclusions would be further strengthened by a subsequent replication when the public health and economic crises stabilize, to test whether pre-COVID perceptions return.
The current study provides novel information about impact of the COVID19 health and economic crisis, and the impact of the corresponding policy responses on community perceptions. This novel study shows how community perceptions of employment and benefit recipient status have been altered by the COVID19 pandemic. These results add to knowledge about the determinants of welfare stigma, particularly relating to employability, highlighting societal level contextual factors.
Data Availability Statement
The raw data supporting the conclusions of this article will be made available by the authors, without undue reservation.
Ethics Statement
The studies involving human participants were reviewed and approved by Melbourne University Human Research Ethics Committee. The patients/participants provided their written informed consent to participate in this study.
Author Contributions
AS led the review conceptualized by TS and PB. AS and PB conducted the analyses and wrote up the review. TS led the data collection, reviewed and edited the manuscript, and provided data management support. This manuscript is based on previous extensive work by TS and PB on stereotypes toward the unemployed and welfare benefit recipients. All authors contributed to the article and approved the submitted version.
This study was funded by the Australian Research Council (ARC) grant # DP16014178.
Conflict of Interest
The authors declare that the research was conducted in the absence of any commercial or financial relationships that could be construed as a potential conflict of interest.
Supplementary Material
The Supplementary Material for this article can be found online at: https://www.frontiersin.org/articles/10.3389/fpsyg.2020.594837/full#supplementary-material
- ^ https://fred.stlouisfed.org/series/UNRATE
- ^ The Australian figure includes individuals working zero hours who had “no work, not enough work available or were stood down.” The US Bureau of Labor Statistics noted that some people on temporary layoff were not classified as such and the unemployment rate could have been almost 5 percentage points higher.
- ^ https://osf.io/wknb6
- ^ https://pursuit.unimelb.edu.au/articles/our-changing-identities-under-covid-19
Aarøe, L. (2011). Investigating frame strength: the case of episodic and thematic frames. Polit. Commun . 28, 207–226. doi: 10.1080/10584609.2011.568041
CrossRef Full Text | Google Scholar
Aarøe, L., and Petersen, M. B. (2014). Crowding out culture: scandinavians and Americans agree on social welfare in the face of deservingness cues. J. Polit. 76, 684–697. doi: 10.1017/S002238161400019X
Abele, A. E., Hauke, N., Peters, K., Louvet, E., Szymkow, A., and Duan, Y. (2016). Facets of the fundamental content dimensions: agency with competence and assertiveness—Communion with warmth and morality. Front. Psychol. 7:1810. doi: 10.3389/fpsyg.2016.01810
PubMed Abstract | CrossRef Full Text | Google Scholar
Atitsogbe, K. A., Mama, N. P., Sovet, L., Pari, P., and Rossier, J. (2019). Perceived employability and entrepreneurial intentions across university students and job seekers in Togo: the effect of career adaptability and self-efficacy. Front. Psychol. 10:180. doi: 10.3389/fpsyg.2019.00180
Australian Bureau of Statistics (2017). Characteristics of Employment. Australia: Labour Force.
Google Scholar
Australian Bureau of Statistics (2020). Employment and unemployment: International Perspective. Australia: Labour Force.
Barrick, M. R., Mount, M. K., and Judge, T. A. (2001). Personality and performance at the beginning of the new millennium: what do we know and where do we go next? Int. J. Select. Assess. 9, 9–30. doi: 10.1111/1468-2389.00160
Bastian, B., Denson, T. F., and Haslam, N. (2013). The roles of dehumanization and moral outrage in retributive justice. PLoS One 8:e61842. doi: 10.1371/journal.pone.0061842
Baumberg, B. (2012). Three ways to defend welfare in Britain. J. Poverty Soc. Just. 20, 149–161. doi: 10.1332/175982712X652050
Bocian, K., Baryla, W., Kulesza, W. M., Schnall, S., and Wojciszke, B. (2018). The mere liking effect: attitudinal influences on attributions of moral character. J. Exp. Soc. Psychol. 79, 9–20. doi: 10.1016/j.jesp.2018.06.007
Bye, H. H., Herrebrøden, H., Hjetland, G. J., Røyset, G. Ø, and Westby, L. L. (2014). Stereotypes of norwegian social groups. Scand. J. Psychol. 55, 469–476. doi: 10.1111/sjop.12141
Chan, W., McCrae, R. R., De Fruyt, F., Jussim, L., Löckenhoff, C. E., De Bolle, M., et al. (2012). Stereotypes of age differences in personality traits: universal and accurate? J. Personal. Soc. Psychol. 103, 1050–1066. doi: 10.1037/a0029712
Contini, D., and Richiardi, M. G. (2012). Reconsidering the effect of welfare stigma on unemployment. J. Econ. Behav. Organ. 84, 229–244. doi: 10.1016/j.jebo.2012.02.010
Cuddy, A. J., Fiske, S. T., and Glick, P. (2008). Warmth and competence as universal dimensions of social perception: the stereotype content model and the BIAS map. Adv. Exp. Soc. Psychol. 40, 61–149. doi: 10.1016/S0065-2601(07)00002-0
Cuesta, M. B., and Budría, S. (2017). Unemployment persistence: how important are non-cognitive skills? J. Behav. Exp. Econom. 69, 29–37. doi: 10.1016/j.socec.2017.05.006
Dhiman, M. C. (2012). Employers’ perceptions about tourism management employability skills. Anatolia 23, 359–372. doi: 10.1080/13032917.2012.711249
Digman, J. M. (1997). Higher-order factors of the big five. J. Personal. Soc. Psychol. 73:1246.
Fiske, S. T. (2018). Stereotype content: warmth and competence endure. Curr. Direct. Psychol. Sci. 27, 67–73. doi: 10.1177/0963721417738825
Fiske, S. T., Cuddy, A. J., Glick, P., and Xu, J. (2002). A model of (often mixed) stereotype content: competence and warmth respectively follow from perceived status and competition. J. Personal. Soc. Psychol. 82:878. doi: 10.1037/0022-3514.82.6.878
Garthwaite, K. (2013). Fear of the brown envelope: exploring welfare reform with long-term sickness benefits recipients. Soc. Pol. Administration. 48, 782–798. doi: 10.1111/spol.12049
Giner-Sorolla, R., and Espinosa, P. (2011). Social cuing of guilt by anger and of shame by disgust. Psychol. Sci. 22, 49–53. doi: 10.1177/0956797610392925
Goldberg, L. R. (1993). The structure of phenotypic personality traits. Am. Psychol. 48:26. doi: 10.1037/0003-066X.48.1.26
Gosling, S. D., Rentfrow, P. J., and Swann, W. B. Jr. (2003). A very brief measure of the Big-Five personality domains. J. Res. Personal. 37, 504–528. doi: 10.1016/S0092-6566(03)00046-1
Haidt, J. (2003). The moral emotions. Handb. Affect. Sci. 11, 852–870.
Haller, M., Norman, S. B., Davis, B. C., Capone, C., Browne, K., and Allard, C. B. (2020). A model for treating COVID-19–related guilt, shame, and moral injury. Psychol. Trauma 12, S174–S176.
Hogan, R., Hogan, J., and Roberts, B. W. (1996). Personality measurement and employment decisions: questions and answers. Am. Psychol. 51:469. doi: 10.1037/0003-066X.51.5.469
Larsen, K. (2008). Knowledge network hubs and measures of research impact, science structure, and publication output in nanostructured solar cell research. Scientometrics 74, 123–142. doi: 10.1007/s11192-008-0107-2
Löckenhoff, C. E., Chan, W., McCrae, R. R., De Fruyt, F., Jussim, L., De Bolle, M., et al. (2014). Gender stereotypes of personality: universal and accurate? J. Cross-Cult. Psychol. 45, 675–694. doi: 10.1177/0022022113520075
Lowden, K., Hall, S., Elliot, D., and Lewin, J. (2011). Employers’ Perceptions of the Employability Skills of New Graduates. London: Edge Foundation.
McCrae, R. R., and Terracciano, A. (2005). Universal features of personality traits from the observer’s perspective: data from 50 cultures. J. Personal. Soc. Psychol. 88, 547–561. doi: 10.1037/0022-3514.88.3.547
Moffitt, R. (1983). An economic model of welfare stigma. Am. Econom. Rev. 75, 1023–1035.
O’Leary, S. (2017). Graduates’ experiences of, and attitudes towards, the inclusion of employability-related support in undergraduate degree programmes; trends and variations by subject discipline and gender. J. Educ. Work 30, 84–105. doi: 10.1080/13639080.2015.1122181
O’Leary, S. (2019). Gender and management implications from clearer signposting of employability attributes developed across graduate disciplines. Stud. Higher Educ. 1–20. doi: 10.1080/03075079.2019.1640669
Pegg, A., Waldock, J., Hendy-Isaac, S., and Lawton, R. (2012). Pedagogy for Employability. York: Higher Education Academy.
Petersen, M. B., Slothuus, R., Stubager, R., and Togeby, L. (2011). Deservingness versus values in public opinion on welfare: the automaticity of the deservingness heuristic. Eur. J. Political Res. 50, 24–52. doi: 10.1111/j.1475-6765.2010.01923.x
Plomp, J., Tims, M., Khapova, S. N., Jansen, P. G., and Bakker, A. B. (2019). Psychological safety, job crafting, and employability: a comparison between permanent and temporary workers. Front. Psychol. 10:974.
Saad, M. S. M., and Majid, I. A. (2014). Employers’ perceptions of important employability skills required from Malaysian engineering and information and communication technology (ICT) graduates. Global J. Eng. Educ. 16, 110–115.
Saucier, G., and Goldberg, L. R. (1996). “The language of personality: lexical perspectives,” in On the Five-factor Model of Personality: Theoretical Perspectives , ed. J. S. Wiggins (New York, NY: Guilford Press), 21–50.
Schofield, T. P., and Butterworth, P. (2015). Patterns of welfare attitudes in the Australian population. PLoS One 10:0142792. doi: 10.1371/journal.pone.0142792
Schofield, T. P., and Butterworth, P. (2018a). Are negative community attitudes toward welfare recipients associated with unemployment? evidence from an australian cross-sectional sample and longitudinal cohort. Soc. Psychol. Personal. Sci. 9, 503–515. doi: 10.1177/1948550617712031
Schofield, T. P., and Butterworth, P. (2018b). Community attitudes toward people receiving unemployment benefits: does volunteering change perceptions? Basic Appl. Soc. Psychol. 40, 279–292. doi: 10.1080/01973533.2018.1496335
Schofield, T. P., Haslam, N., and Butterworth, P. (2019). The persistence of welfare stigma: does the passing of time and subsequent employment moderate the negative perceptions associated with unemployment benefit receipt? J. Appl. Soc. Psychol. 49, 563–574. doi: 10.1111/jasp.12616
Shahidi, F., Siddiqi, A., and Muntaner, C. (2016). Does social policy moderate the impact of unemployment on health? a multilevel analysis of 23 welfare states. Eur. J. f Public Health 26, 1017–1022. doi: 10.1093/eurpub/ckw050
Srivastava, S. (2010). The five-factor model describes the structure of social perceptions. Psychol. Inquiry 21, 69–75. doi: 10.1080/10478401003648815
Stuber, J., and Schlesinger, M. (2006). Sources of stigma for means-tested government programs. Soc. Sci. Med. 63, 933–945. doi: 10.1016/j.socscimed.2006.01.012
van Oorschot, W. (2000). Who should get what, and why? on deservingness criteria and the conditionality of solidarity among the public. Pol. Pol. 28, 33–48. doi: 10.1332/0305573002500811
van Oorschot, W., and Roosma, F. (2017). The Social Legitimacy of Targeted Welfare. Cheltenham: Edward Elgar Publishing.
Ward, L. C., Thorn, B. E., Clements, K. L., Dixon, K. E., and Sanford, S. D. (2006). Measurement of agency, communion, and emotional vulnerability with the Personal Attributes Questionnaire. J. Pers. Assess . 86, 206–216. doi: 10.1207/s15327752jpa8602_10
Yeves, J., Bargsted, M., Cortes, L., Merino, C., and Cavada, G. (2019). Age and perceived employability as moderators of job insecurity and job satisfaction: a moderated moderation model. Front. Psychol. 10:799. doi: 10.3389/fpsyg.2019.00799
Keywords : COVID19, employability, personality, Big Five, public policy, unemployment
Citation: Suomi A, Schofield TP and Butterworth P (2020) Unemployment, Employability and COVID19: How the Global Socioeconomic Shock Challenged Negative Perceptions Toward the Less Fortunate in the Australian Context. Front. Psychol. 11:594837. doi: 10.3389/fpsyg.2020.594837
Received: 14 August 2020; Accepted: 22 September 2020; Published: 15 October 2020.
Reviewed by:
Copyright © 2020 Suomi, Schofield and Butterworth. This is an open-access article distributed under the terms of the Creative Commons Attribution License (CC BY) . The use, distribution or reproduction in other forums is permitted, provided the original author(s) and the copyright owner(s) are credited and that the original publication in this journal is cited, in accordance with accepted academic practice. No use, distribution or reproduction is permitted which does not comply with these terms.
*Correspondence: Aino Suomi, [email protected]
Disclaimer: All claims expressed in this article are solely those of the authors and do not necessarily represent those of their affiliated organizations, or those of the publisher, the editors and the reviewers. Any product that may be evaluated in this article or claim that may be made by its manufacturer is not guaranteed or endorsed by the publisher.

An official website of the United States government
The .gov means it’s official. Federal government websites often end in .gov or .mil. Before sharing sensitive information, make sure you’re on a federal government site.
The site is secure. The https:// ensures that you are connecting to the official website and that any information you provide is encrypted and transmitted securely.
- Publications
- Account settings
Preview improvements coming to the PMC website in October 2024. Learn More or Try it out now .
- Advanced Search
- Journal List
- Elsevier - PMC COVID-19 Collection

Editor in Chief's Introduction to Essays on the Impact of COVID-19 on Work and Workers
On March 11, 2020, the World Health Organization declared that COVID-19 was a global pandemic, indicating significant global spread of an infectious disease ( World Health Organization, 2020 ). At that point, there were 118,000 confirmed cases of the coronavirus in 110 countries. China had been the first country with a widespread outbreak in January, and South Korea, Iran and Italy following in February with their own outbreaks. Soon, the virus was in all continents and over 177 countries, and as of this writing, the United States has the highest number of confirmed cases and, sadly, the most deaths. The virus was extremely contagious and led to death in the most vulnerable, particularly those older than 60 and those with underlying conditions. The most critical cases led to an overwhelming number being admitted into the intensive care units of hospitals, leading to a concern that the virus would overwhelm local health care systems. Today, in early May 2020, there have been nearly 250,000 deaths worldwide, with over 3,500,000 confirmed cases ( Hopkins, 2020 ). The human toll is staggering, and experts are predicting a second wave in summer or fall.
As the deaths rose from the virus that had no known treatment or vaccine countries shut their borders, banned travel to other countries and began to issue orders for their citizens to stay at home, with no gatherings of more than 10 individuals. Schools and universities closed their physical locations and moved education online. Sporting events were canceled, airlines cut flights, tourism evaporated, restaurants, movie theaters and bars closed, theater productions canceled, manufacturing facilities, services, and retail stores closed. In some businesses and industries, employees have been able to work remotely from home, but in others, workers have been laid off, furloughed, or had their hours cut. The International Labor Organization (ILO) estimates that there was a 4.5% reduction in hours in the first quarter of 2020, and 10.5% reduction is expected in the second quarter ( ILO, 2020a ). The latter is equivalent to 305 million jobs ( ILO, 2020a ).
Globally, over 430 million enterprises are at risk of disruption, with about half of those in the wholesale and retail trades ( ILO, 2020a ). Much focus in the press has been on the impact in Europe and North America, but the effect on developing countries is even more critical. An example of the latter is the Bangladeshi ready-made-garment sector ( Leitheiser et al., 2020 ), a global industry that depends on a supply chain of raw material from a few countries and produces those garments for retail stores throughout North America and Europe. But, in January 2020, raw material from China was delayed by the shutdown in China, creating delays and work stoppages in Bangladesh. By the time Bangladeshi factories had the material to make garments, in March, retailers in Europe and North American began to cancel orders or put them on hold, canceling or delaying payment. Factories shut down and workers were laid off without pay. Nearly a million people lost their jobs. Overall, since February 2020, the factories in Bangladesh have lost nearly 3 billion dollars in revenue. And, the retail stores that would have sold the garments have also closed. This demonstrates the ripple effect of the disruption of one industry that affects multiple countries and sets of workers, because consider that, in turn, there will be less raw material needed from China, and fewer workers needed there. One need only multiply this example by hundreds to consider the global impact of COVID-19 across the world of work.
The ILO (2020b) notes that it is difficult to collect employment statistics from different countries, so a total global unemployment rate is unavailable at this time. However, they predict significant increase in unemployment, and the number of individuals filing for unemployment benefits in the United States may be an indicator of the magnitude of those unemployed. In the United States, over 30 million filed for unemployment between March 11 and April 30 ( Bureau of Labor Statistics, 2020 ), effectively this is an unemployment rate of 18%. By contrast, in February 2020, the US unemployment rate was 3.5% ( Bureau of Labor Statistics, 2020 ).
Clearly, COVID-19 has had an enormous disruption on work and workers, most critically for those who have lost their employment. But, even for those continuing to work, there have been disruptions in where people work, with whom they work, what they do, and how much they earn. And, as of this writing, it is also a time of great uncertainty, as countries are slowly trying to ease restrictions to allow people to go back to work--- in a “new normal”, without the ability to predict if they can prevent further infectious “spikes”. The anxieties about not knowing what is coming, when it will end, or what work will entail led us to develop this set of essays about future research on COVID-19 and its impact on work and workers.
These essays began with an idea by Associate Editor Jos Akkermans, who noted to me that the global pandemic was creating a set of career shocks for workers. He suggested writing an essay for the Journal . The Journal of Vocational Behavior has not traditionally published essays, but these are such unusual times, and COVID-19 is so relevant to our collective research on work that I thought it was a good idea. I issued an invitation to the Associate Editors to submit a brief (3000 word) essay on the implications of COVID-19 on work and/or workers with an emphasis on research in the area. At the same time, a group of international scholars was coming together to consider the effects of COVID-19 on unemployment in several countries, and I invited that group to contribute an essay, as well ( Blustein et al., 2020 ).
The following are a set of nine thoughtful set of papers on how the COVID-19 could (and perhaps will) affect vocational behavior; they all provide suggestions for future research. Akkermans, Richardson, and Kraimer (2020) explore how the pandemic may be a career shock for many, but also how that may not necessarily be a negative experience. Blustein et al. (2020) focus on global unemployment, also acknowledging the privileged status they have as professors studying these phenomena. Cho examines the effect of the pandemic on micro-boundaries (across domains) as well as across national (macro) boundaries ( Cho, 2020 ). Guan, Deng, and Zhou (2020) drawing from cultural psychology, discuss how cultural orientations shape an individual's response to COVID-19, but also how a national cultural perspective influences collective actions. Kantamneni (2020) emphasized the effects on marginalized populations in the United States, as well as the very real effects of racism for Asians and Asian-Americans in the US. Kramer and Kramer (2020) discuss the impact of the pandemic in the perceptions of various occupations, whether perceptions of “good” and “bad” jobs will change and whether working remotely will permanently change where people will want to work. Restubog, Ocampo, and Wang (2020) also focused on individual's responses to the global crisis, concentrating on emotional regulation as a challenge, with suggestions for better managing the stress surrounding the anxiety of uncertainty. Rudolph and Zacher (2020) cautioned against using a generational lens in research, advocating for a lifespan developmental approach. Spurk and Straub (2020) also review issues related to unemployment, but focus on the impact of COVID-19 specifically on “gig” or flexible work arrangements.
I am grateful for the contributions of these groups of scholars, and proud of their ability to write these. They were able to write constructive essays in a short time frame when they were, themselves, dealing with disruptions at work. Some were home-schooling children, some were worried about an absent partner or a vulnerable loved one, some were struggling with the challenges that Restubog et al. (2020) outlined. I hope the thoughts, suggestions, and recommendations in these essays will help to stimulate productive thought on the effect of COVID-19 on work and workers. And, while, I hope this research spurs to better understand the effects of such shocks on work, I really hope we do not have to cope with such a shock again.
- Akkermans J., Richardson J., Kraimer M. The Covid-19 crisis as a career shock: Implications for careers and vocational behavior. Journal of Vocational Behavior. 2020; 119 [ PMC free article ] [ PubMed ] [ Google Scholar ]
- Blustein D.L., Duffy R., Ferreira J.A., Cohen-Scali V., Cinamon R.G., Allan B.A. Unemployment in the time of COVID-19: A research agenda. Journal of Vocational Behavior. 2020; 119 [ PMC free article ] [ PubMed ] [ Google Scholar ]
- Bureau of Labor Statistics (2020). Labor Force Statistics from the Current Population Survey. Retrieved May 6, 2020 from https://data.bls.gov/cgi-bin/surveymost .
- Cho E. Examining boundaries to understand the impact of COVID-19 on vocational behaviors. Journal of Vocational Behavior. 2020; 119 [ PMC free article ] [ PubMed ] [ Google Scholar ]
- Guan Y., Deng H., Zhou X. Understanding the impact of the COVID-19 pandemic on career development: Insights from cultural psychology. Journal of Vocational Behavior. 2020; 119 [ PMC free article ] [ PubMed ] [ Google Scholar ]
- Johns Hopkins (2020) Coronavirus Outbreak Mapped: Retrieved May 5, 2020 from https://coronavirus.jhu.edu/map.html .
- International Labor Organization ILO monitor: COVID-19 and the world of work. Third edition updated estimates and analysis. 2020. https://www.ilo.org/wcmsp5/groups/public/@dgreports/@dcomm/documents/briefingnote/wcms_743146.pdf Retrieved May 5, 2020 from:
- International Labor Organization (2020b) COVID-19 impact on the collection of labour market statistics. Retrieved May 6, 2020 from: https://ilostat.ilo.org .
- Kantamneni, N. (2020). The impact of the COVID-19 pandemic on marginalized populations in the United States: A research agenda. Journal of Vocational Behavior, 119 . [ PMC free article ] [ PubMed ]
- Kramer A., Kramer K.Z. The potential impact of the Covid-19 pandemic on occupational status, work from home, and occupational mobility. Journal of Vocational Behavior. 2020; 119 [ PMC free article ] [ PubMed ] [ Google Scholar ]
- Leitheiser, E., Hossain, S.N., Shuvro, S., Tasnim, G., Moon, J., Knudsen, J.S., & Rahman, S. (2020). Early impacts of coronavirus on Bangladesh apparel supply chains. https://www.cbs.dk/files/cbs.dk/risc_report_-_impacts_of_coronavirus_on_bangladesh_rmg_1.pdf .
- Restubog S.L.D., Ocampo A.C., Wang L. Taking control amidst the Chaos: Emotion regulation during the COVID-19 pandemic. Journal of Vocational Behavior. 2020; 119 [ PMC free article ] [ PubMed ] [ Google Scholar ]
- Rudolph C.W., Zacher H. COVID-19 and careers: On the futility of generational explanations. Journal of Vocational Behavior. 2020; 119 [ PMC free article ] [ PubMed ] [ Google Scholar ]
- Spurk D., Straub C. Flexible employment relationships and careers in times of the COVID-19 pandemic. Journal of Vocational Behavior. 2020; 119 [ PMC free article ] [ PubMed ] [ Google Scholar ]
- World Health Organization (2020). World Health Organization Coronavirus Update. Retrieved May 5, 2020 from: https://www.who.int/emergencies/diseases/novel-coronavirus-2019 .

Generations of Unemployment
From young Americans just beginning their careers to those closer to retirement, millions are stuck in limbo.
By Phil McCausland June 30, 2020

(Cristiana Couceiro / for NBC News)
When Daniel Martinez drives by the car dealership in Houston where he worked as a field service technician, his 3-year-old daughter will point to it and say, “Look, Daddy’s work.”
Martinez, 28, had been earning enough money to support his wife and their daughter and was beginning to look at upgrading the family’s small apartment to a house. But he was laid off in April as coronavirus cases began to soar, and he lost his employer-provided health insurance. The dream of a new home vanished.
“Now I have to say, ‘No, I don’t work there anymore,” he said, recalling his response to his daughter. “I’m just trying to explain to her that, you know, ‘Daddy lost his job but I’m still looking.’ It’s rough.”
Martinez is one of more than 20 million Americans who have become unemployed because of the coronavirus pandemic, which has forced many employers to shut their doors and either furlough or fire employees.
Martinez, after six years of Navy service and three years in his most recent job, had high hopes for the trajectory of his life and his career. He loved the work he did and the community that it came with, but now he’s not sure if he’ll ever get it back.
“It was like a light switch,” he said. “Some days, I feel lost and hopeless because I don’t know what I can do.”
The number of people who have lost their jobs because of the pandemic is unprecedented: The biggest spike in initial unemployment claims in March, at 6.6 million, far surpassed the peak of the Great Recession. With the economic future remaining murky, unemployed workers in the United States, from young people beginning their careers to those closer to retirement, are facing a choice to weather the storm and hope their positions return or give up their chosen career altogether if they aren’t able to find something similar.
Your browser does not support this video
Listen to Martin Peterson's story
Listen to Laurie Rosener's story
Listen to Nicole Anerud's story
Each week, the jobs report published by the Department of Labor earns prominent headlines in newspapers and functions as a key point of discussion on cable news channels and social media. But behind the columns and the panels of pundits opining about how to resolve this labor crisis is a growing number of Americans attempting to read the economic tea leaves and figure out where they belong in the U.S. job market.
Just last week, 1.5 million more people applied for unemployment , the 14th consecutive week in which states processed more than 1 million first-time applications. The unemployment rate was nearly 10 percent higher in May than it was a year prior, according to the Bureau of Labor Statistics.
The U.S. is officially in a recession, as the National Bureau of Economic Research announced in May. The effects are felt deeply by all age groups, but it has deeper implications for older Americans, who even now tend to go longer between jobs, including periods of high employment.
Federal Reserve Chairman Jerome Powell said as much when he testified before Congress in June, suggesting that Congress and the administration need to do more to address the economic fallout of the pandemic.
“I think there are going to be a large number of people who will not be able to immediately go back to work at their old job, or even in their old industry,” Powell told lawmakers. “There will be a significant group that is left over even after we get the employment bounce.”
Until then, many continue to rely on unemployment benefits, especially those that came with the Coronavirus Aid, Relief and Economic Security Act that was enacted in March.
As the labor market continues to shrink, the legislation has become the financial backbone of many Americans’ lives.
Without it, Greg Aplin and his wife, artists and artisan workers in Memphis, Tennessee, would struggle to put food on the table and pay their bills. The couple, both in their 60s, were able to create a lucrative business selling their art after they were both laid off from their jobs a few years ago. But they have had to essentially shut down their business because the festivals and live events they attended to sell their work have been canceled.
“Without the unemployment help, we’d be in a serious world of hurt,” said Aplin, who said he’ll have to start drawing on his Social Security if the CARES Act isn’t extended by lawmakers and instead expires at the end of July. “It’s a little terrifying.”
The share of Americans’ total wage and salary income made up of unemployment benefits has reached historically high levels, according to an analysis by the Economic Policy Institute . Since the 1940s, unemployment assistance has never made up more than 2.5 percent of American income, but that benefit constituted 15 percent of wages and salaries last month.
That’s in part due to the unemployment benefits included in the CARES Act, passed during the early stages of the coronavirus pandemic in the U.S., which provided unemployed Americans an additional $600 a week from the federal government on top of the money provided by individual states.
That softened the blow for Lila Fung who lives in the Queens borough of New York City, but it took a great deal of fighting to get there. With the unemployment system overloaded in New York, as millions applied for the benefit at the same time, it took her more than two months to receive assistance.
“I don’t want to be reliant on it, but it helps,” said Fung, who was laid off the first week of April after starting a new job as a commercial analyst in January. Fung has more than 15 years of professional experience under her belt.
“I’m being proactive every day trying to find work but there’s so much competition for the same job," Fung said. In the past, you probably had 10-15 people applying, but now it’s like 400 or 500 going for the same job, and it’s like, ‘How am I ever going to get noticed? How am I ever going to find a job?’”
It’s also unclear when many can return to work because of the surge in coronavirus cases across the country — just last week, single-day case increases in the U.S. hit a new high, adding nearly 46,000 cases Friday. Josh Bivens, the research director of the Economic Policy Institute, a liberal think tank in Washington affiliated with the labor movement, said this historic level of assistance is necessary to keep the country’s economy alive, but not at the expense of forcing people to choose between their health and a paycheck.
Listen to Daniel Martinez's story
Listen to Lila Fung's story
Listen to Samantha Enterkin's story
“Unlike most recessions where the policy goal is just, as quick as possible, get people back into a job, this was different. We actually wanted to give people the economic space to not work for a while because we wanted to reduce the spread.”
That extra cushion, which has stopped the fall of consumption spending in response to the sudden shock of job losses, has kept many Americans’ lives afloat. But the country is currently poised to leap off a fiscal cliff without an extension of those benefits, Bivens said.
That could be dire economically for the country and for individuals, especially as outbreaks of the virus are growing in Texas, Arizona and across the Southeast.
“If we have another similarly sized shutdown and we don't have the extra $600, I mean, people will literally go hungry,” Bivens said. “We already saw the lines at food banks and things like that in the past three months, it will get exponentially worse if nothing changes.”
But it’s not just the possible loss of the assistance that worries Americans who remain out of work. Many states suspended rent and mortgage payments as the pandemic spread across the country, but those freezes are beginning to expire.
That’s another level of relief lost for the record number of people who are now unemployed, many of whom also lost their job-provided health insurance.
But people like Marty Petersen, 57, don’t have much hope that Congress or the Trump administration will do much to help. Petersen, who worked as a stagehand at a theater in Schenectady, New York, for 31 years until he was laid off because of the pandemic, said that it appears both political parties seem more concerned about political wins than addressing the economic woes of Americans across the country.
“They’re deciding not to work together and we’re all getting caught up and neglected,” Petersen said, noting that he’s had to pull from his retirement savings to help pay for health insurance coverage for his family of four and is considering selling his car to make ends meet if he loses the CARES Act benefits. “We’re the pile of sawdust that they’re creating.”
Democrats have proposed extending the CARES Act unemployment benefits until the end of the year, but the extension has yet to find much bipartisan support.
Many said they feel as if they’re in limbo, already unable to make any plans for their future — financial and otherwise — because of COVID-19, and they worry about losing the one lifeline they feel they have left.
Nevertheless, President Donald Trump has insisted that the economy will quickly bounce back once states come out of lockdowns. But as the number of unemployment claims continues to grow and with a number of states having to reverse reopening plans, it appears as if Trump’s confidence could be misguided.
It’s not convincing to Nicole Anerud, 37, who lost her job in the oil and gas industry three weeks after returning from maternity leave. Anerud, who had dreams of becoming a history professor until the Great Recession forced her to pivot into her most recent role as an oil and gas planner, said that she doesn’t have much hope for finding work again in her current field, especially as Texas faces a growing rate of infection . Now she’s forced to once again rethink her career goals.
“It’s getting harder,” she said from her home in Katy, Texas. “I don’t know what the ramifications are going to be from COVID-19 or how bad it’s going to get, but I’ve got a double-edged worry of trying to find a job while balancing that with my son.”
While no two unemployment stories are the same, the common threads are a sense of dreams deferred and a need to take temporary work in the strange new world the United States has become amid the pandemic.
Emily Nygard, 23, of Tacoma, Washington, thought she had found her dream job at the intersection of law and health when she took a position as a medical reports coordinator right after graduating from college. Seven months after she started, her company laid her off. Her unemployment claim allowed her to pay a mounting number of bills as she applied for dozens of jobs each day.
“I was sitting at home for six weeks just going, ‘you know, the world's been taken. I'm doing nothing, and I just so desperately want to help,’” said Nygard, who said that she cried when she had to apply for unemployment.
She eventually found another position, as a COVID-19 screener at the hospital near her home, but the job is temporary, adding another variable to an already murky future.
“It’s been a little bit of cognitive dissonance of like, ‘what's my work worth? And what's my degree worth? And what's my health worth?’” Nygard said. “Because a lot of the money that I make right now is, ‘Thanks for putting your life on the line.’”
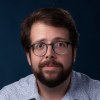
Phil McCausland is an NBC News reporter focused on rural issues and the social safety net.
Design and development: Robin Muccari / NBC News
Art Director: Chelsea Stahl / NBC News
The Pandemic's Impact on Unemployment and Labor Force Participation Trends
Following early 2020 responses to the pandemic, labor force participation declined dramatically and has remained below its 2019 level, whereas the unemployment rate recovered briskly. We estimate the trend of labor force participation and unemployment and find a substantial impact of the pandemic on estimates of trend. It turns out that levels of labor force participation and unemployment in 2021 were approaching their estimated trends. A return to 2019 levels would then represent a tight labor market, especially relative to long-run demographic trends that suggest further declines in the participation rate.
At the end of 2019, the labor market was hotter than it had been in years. Unemployment was at a historic low, and participation in the labor market was finally increasing after a prolonged decline. That tight labor market came to an abrupt halt with the COVID-19 pandemic in the spring of 2020.
Now, two years later, the labor market has mostly recovered from the depths of the pandemic recession. The unemployment rate is close to pre-pandemic lows, and job openings are at record highs. Yet, participation and employment rates have remained persistently below pre-pandemic levels. This suggests the possibility that the pandemic has permanently reduced participation in the economy and that current participation rates reflect a new normal. In this article, we explore how the pandemic has affected labor markets and whether a new normal is emerging.
What Is "Normal"?
One way to define the normal level of a variable is to estimate its trend and compare the observed data with the estimated trend values. Constructing a trend essentially means drawing a smooth line through the variations in the actual data.
But this means that constructing the trend for a point in time typically involves considering what happened both before and after that point in time. Thus, constructing the trend at the end of a sample is especially hard, since we do not yet know how the data will evolve.
We construct trends for three aggregate labor market ratios — the labor force participation (LFP) rate, the unemployment rate and the employment-population ratio (EPOP) — using methods described in our 2019 article " Projecting Unemployment and Demographic Trends ."
First, we estimate statistical models for LFP and unemployment rates of demographic groups defined by age, gender and education. For each gender and education, we decompose its unemployment and LFP into cyclical components common to all age groups and smooth local trends for age and cohort effects.
Second, we aggregate trends from the estimates of the group-specific trends. Specifically, we construct the trend for the aggregate LFP rate as the population-share-weighted sum of the corresponding estimated trends for demographic groups. We construct the aggregate unemployment rate and EPOP trends from the group-specific LFP and unemployment trends and the groups' population shares.
In our previous work, we estimated the trends for the unemployment rate and LFP rate of a gender-education group separately using maximum likelihood methods. The estimates reported in this article are based on the joint estimation of LFP and unemployment rate trends using Bayesian methods.
We separately estimate the trends using data from 1976 to 2019 (pre-pandemic) and from 1976 to 2021 (including the pandemic period). Figures 1, 2 and 3 display annual averages for the three aggregate labor market ratios — the LFP rate, the unemployment rate and EPOP, respectively — from 1976 to 2021.
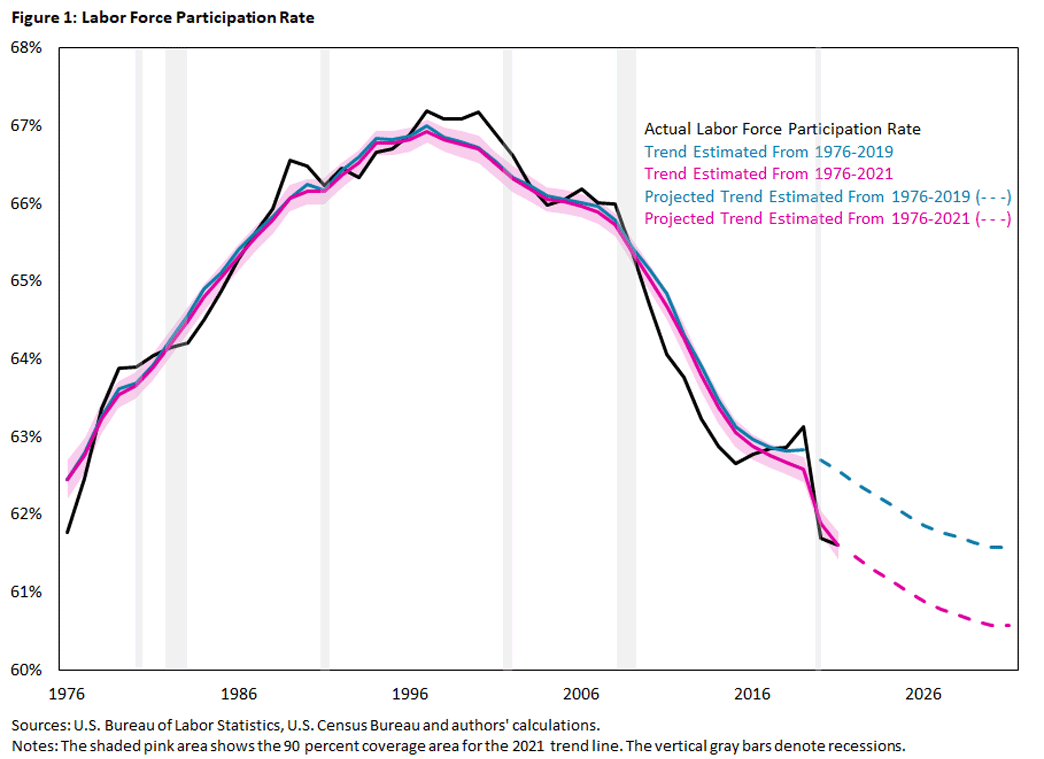
In each figure, the solid black line denotes the observed values, and the blue and pink lines denote the estimated trend using data from 1976 up to and including 2019 and 2021, respectively. The estimated trends are subject to uncertainty, and the plotted trends represent the median estimate of the trend.
For the estimates based on data up to 2021, we also include the 90 percent coverage area shown as the shaded pink area. According to the statistical model, there is a 90 percent probability that the trend is contained in the coverage area. The blue and pink dotted lines represent our projections on how the labor market ratios will evolve until 2031, again based on the estimated trend up to and including 2019 and 2021. The shaded gray vertical areas highlight recessions as defined by the National Bureau of Economic Research (NBER).
Pre-Pandemic Trends: 1976-2019
We start with the pre-pandemic trends for the LFP rate and unemployment rate estimated for data from 1976 through 2019. After a long recovery from the 2007-09 recession, the LFP rate was 63.1 percent in 2019 (slightly above the estimated trend value of 62.8 percent), and the unemployment rate was 3.7 percent (noticeably below its estimated trend value of 4.7 percent).
The LFP rate being above trend and the unemployment rate being below trend reflects the characterization of the 2019 labor market as "hot." But note that even though the LFP rate exceeded its trend value in 2019, it was still lower than during the 2007-09 period. This difference is accounted for by the declining trend in the LFP rate.
As noted in our 2019 article , LFP rates and unemployment rates differ systematically across demographic groups. Participation rates tend to be higher for younger, more-educated workers and for men. Unemployment rates tend to be lower for men and for the older and more-educated population.
Thus, changes in the population composition over time — that is, the relative size of demographic groups — will affect the aggregate LFP and unemployment rates, in addition to changes in the LFP and unemployment rate trends of the demographic groups.
As also noted in our 2019 article, the hump-shaped trend of the aggregate LFP rate reflects a variety of forces:
- Prior to 1990, the aggregate LFP rate was boosted by an upward trend in the LFP rate of women. But after 1990, the LFP rate of women began declining. Combining this with declining trend LFP rates for other demographic groups has reduced the aggregate LFP rate.
- Changes in the age distribution had a limited impact prior to 2005, but the aging population since then has lowered the aggregate LFP rate substantially.
- Increasing educational attainment has contributed positively to aggregate LFP throughout the period.
The steady decline of the unemployment rate trend reflects mostly the contributions from an older and more-educated population and, to some extent, a decline in the trend unemployment rates of demographic groups.
Pre-Pandemic Expectations of Future LFP and Unemployment Trends
Our statistical model of smooth local trends for the LFP and unemployment rates of demographic groups has the property that the best forecast for future trend values of demographic groups is their last estimated trend value. Thus, the model will only predict a change in the trend of aggregate ratios if the population shares of its constituent groups are changing.
We combine the U.S. Census Bureau population forecasts for the gender-age groups with an estimated statistical model of education shares for gender-age groups to forecast population shares of our demographic groups from 2020 to 2031 (the dotted blue lines in Figures 1 and 2).
As we can see, the changing demographics alone imply further reductions of 1 percentage point and 0.2 percentage points in the trend LFP rate and unemployment rate, respectively. This projection is driven by the forecasted aging of the population, which is only partially offset by the forecasted higher educational attainment.
Based on data up to 2019, the same aggregate LFP rates in 2021 as in 2019 would have represented a substantial cyclical deviation upward from the pre-pandemic trends.
It is notable that the unemployment rate is much more volatile relative to its trend than the LFP rate is. In other words, cyclical deviations from trend are much more pronounced for the unemployment rate than for the LFP rate.
In fact, in our estimation, the behavior of the unemployment rate determines the common cyclical component of both the unemployment rate and the LFP rate. Whereas the unemployment rate spikes in recessions, the LFP rate response is more muted and tends to lag recessions. This feature will be important for interpreting how the estimated trend LFP rate changed with the pandemic.
Finally, Figure 3 combines the information from the LFP rate and unemployment rate and plots actual and trend rates for EPOP. On the one hand, given the relatively small trend decline of the unemployment rate, the trend for EPOP mainly reflects the trend for the LFP rate and inherits its hump-shaped path and the projected decline over the next 10 years. On the other hand, EPOP inherits the volatility from the unemployment rate. In 2019, EPOP is notably above trend, by about 1 percentage point.
Unemployment and Labor Force Participation During the Pandemic
The behavior of unemployment resulting from the pandemic-induced recession was different from past recessions:
- The entire increase in unemployment between February and April 2020 was accounted for by the increase in unemployment from temporary layoffs. This differed from previous recessions, when a spike in permanent layoffs led the bulge of unemployment in the trough.
- The recovery started in May 2020, and the speed of recovery was also much faster than in previous recessions. After only seven months, unemployment declined by 8 percentage points.
- The behavior of the unemployment rate is reflected in the 2020 recession being the shortest NBER recession on record: It lasted for two months (March to April 2020).
To summarize, the runup and decline of the unemployment rate during the pandemic were unusually rapid, but the qualitative features were not that different from previous recessions after properly accounting for temporary layoffs, as noted in the 2020 working paper " The Unemployed With Jobs and Without Jobs . "
The decline in the LFP rate was sharp and persistent. The LFP rate dropped from 63.4 percent in February 2020 to 60.2 percent in April 2020, an unprecedented drop during such a short period of time. After a rebound to 61.7 percent in August 2020, the LFP rate essentially moved sideways and remained below 62 percent until the end of 2021.
The large drop in the aggregate LFP rate has been attributed to, among others:
- More people — especially women — leaving the labor force to care for children because of school closings or to care for relatives at increased health risk, as noted in the 2021 work " Assessing Five Statements About the Economic Impact of COVID-19 on Women (PDF) " and the 2021 article " Caregiving for Children and Parental Labor Force Participation During the Pandemic "
- An increase in retirement due to health concerns, as noted in the 2021 working paper " How Has COVID-19 Affected the Labor Force Participation of Older Workers? "
- Generous pandemic income transfers and unemployment insurance programs, as noted in the 2021 article " COVID Transfers Dampening Employment Growth, but Not Necessarily a Bad Thing "
All of these factors might impact the participation trend, but by how much?
The Pandemic's Effect on Trend Estimates for LFP and Unemployment
The aggregate trend assessment for the LFP and unemployment rates has changed considerably as a result of 2020 and 2021. Repeating the estimation of trend and cycle for our demographic groups using data from 1976 up to 2021 yields the pink trend lines in Figures 1 and 2.
The updated trend estimates now put the positive cyclical gap in 2019 for LFP at 0.5 percentage points (rather than 0.3 percentage points) and the negative cyclical gap for the unemployment rate at 1.4 percentage points (rather than 1 percentage point). That is, by this estimate of the trend, the labor market in 2019 was even hotter than by the estimates from the 1976-2019 period.
In 2021, the actual LFP rate is essentially at trend, and the unemployment rate is only slightly above trend. That is, by this estimate of the trend, the labor market is relatively tight.
Notice that even though the new 2021 trend estimates for both the LFP and the unemployment rates differ noticeably from the trend values predicted for 2021 based on data up to 2019, the trend revisions for the LFP rate are limited to more recent years, whereas the trend revisions for the unemployment rate apply to the whole sample.
The difference in revisions is related to how confident we can be about the estimated trends. The 90 percent coverage area is quite narrow for the LFP rate for the entire sample up to the last four years. Thus, there is no need to drastically revise the estimated trend prior to 2017.
On the other hand, the 90 percent coverage area for the trend unemployment rate is quite broad throughout the sample. That is, a wide range of values for trend unemployment is potentially consistent with observed unemployment values. Consequently, the last two observations lead to a wholesale reassessment of the level of the trend unemployment rate.
Another way to frame the 2020-21 trend revisions is as follows. The unemployment rate is very cyclical, deviations from trend are large, and though the sharp increase and decline of the unemployment rate in 2020-21 is unusual, an upward level shift of the trend unemployment rate best reflects the additional pandemic data.
The LFP rate, however, is usually not very cyclical, and it is only weakly related to the unemployment rate. Since the model assumes that the cyclical response does not change over the sample, it then attributes the large 2020-21 drop of the LFP rate to a decline in its trend and ultimately to a decline of the trend LFP rates of most demographic groups.
Finally, the EPOP trend is again mainly determined by the LFP trend, seen in Figure 3. Including the pandemic years noticeably lowers the estimated trend for the years from 2017 onwards. The cyclical gap in 2019 is now estimated to be 1.4 percentage points, and 2021 EPOP is close to its estimated trend.
What Does the Future Hold?
In our framework, current estimates of trend LFP and the unemployment rate for demographic groups are the best forecasts of future rates. Combined with projected demographic changes, this implies a continued noticeable downward trend for the LFP rate and a slight downward trend for the unemployment rate.
The trend unemployment rate is low, independent of how we estimate the trend. But given the highly unusual circumstances of the pandemic, the model may well overstate the decline in the trend LFP rate. Therefore, it is likely that the "true" trend lies somewhere between the trends estimated using data up to 2019 and data up to 2021.
That being a possibility, it remains that labor markets as of now have been unusually tight by most other measures, such as nominal wage growth and posted job openings relative to hires. This suggests that the true trend is closer to the revised 2021 trend than to the 2019 trend. In other words, the LFP rate and unemployment rate at the end of 2021 relative to the 2021 estimate of trend LFP and unemployment rate are consistent with a tight labor market.
Andreas Hornstein is a senior advisor in the Research Department at the Federal Reserve Bank of Richmond. Marianna Kudlyak is a research advisor in the Research Department at the Federal Reserve Bank of San Francisco.
To cite this Economic Brief, please use the following format: Hornstein, Andreas; and Kudlyak, Marianna. (April 2022) "The Pandemic's Impact on Unemployment and Labor Force Participation Trends." Federal Reserve Bank of Richmond Economic Brief , No. 22-12.
This article may be photocopied or reprinted in its entirety. Please credit the authors, source, and the Federal Reserve Bank of Richmond and include the italicized statement below.
V iews expressed in this article are those of the authors and not necessarily those of the Federal Reserve Bank of Richmond or the Federal Reserve System.
Subscribe to Economic Brief
Receive a notification when Economic Brief is posted online.
By submitting this form you agree to the Bank's Terms & Conditions and Privacy Notice.
Thank you for signing up!
As a new subscriber, you will need to confirm your request to receive email notifications from the Richmond Fed. Please click the confirm subscription link in the email to activate your request.
If you do not receive a confirmation email, check your junk or spam folder as the email may have been diverted.
Phone Icon Contact Us
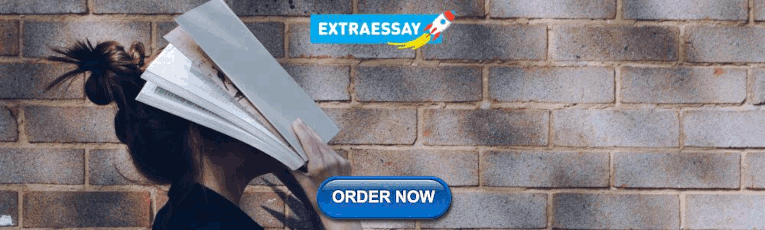
IMAGES
VIDEO
COMMENTS
Nearly 17 million people filed initial claims for unemployment insurance over the past three weeks, suggesting that the unemployment rate is already above 15 percent [1] —well above the rate at ...
Abstract. This essay represents the collective vision of a group of scholars in vocational psychology who have sought to develop a research agenda in response to the massive global unemployment crisis that has been evoked by the COVID-19 pandemic. The research agenda includes exploring how this unemployment crisis may differ from previous ...
The COVID-19 crisis has put unemployment insurance at center stage of American politics and economic policy. It has provided a lifeline for tens of millions of workers who have lost their jobs since the pandemic's onset six months ago, while at the same time exposing the system's vulnerabilities.
As covid-19 drives unemployment rates around the world to levels unseen in generations, once radical economic policy proposals are rapidly gaining a hearing. Martin Hensher examines how job guarantee or universal basic income schemes might support better health and better economics Covid-19 has been a dramatic global health and economic shock. As SARS-CoV-2 spread across nations, economic ...
The COVID-19 pandemic has upended the U.S. labor market, with massive job losses and a spike in unemployment to its highest level since the Great Depression. How long unemployment will remain at crisis levels is highly uncertain and will depend on the speed and success of coronavirus containment measures. Historical patterns of monthly flows in and out of unemployment, adjusted for unique ...
Shown are pre-COVID-19 unemployment rates as of August 2019 (Fig. 2 a), followed by May 2020 (Fig. 2 b) where even the lowest levels of unemployment exceed the highest rates of the pre-pandemic period even in wealthy counties around Nashville (seen in the legend entries), August 2020 (Fig. 2 c), and September 2020 (Fig. 2 d). The overall ...
In the pandemic unemployment graph, the time period is January 2020 to September 2020. The whole is the total number of unemployed plus people who are not in the labor force but want a job. The ...
By Kristie M. Engemann. Social distancing and the partial economic shutdown in response to the COVID-19 pandemic have had a profound impact on the U.S. economy, including on people's jobs and livelihoods. The overall immediate effects on the labor market have been easy to see: The unemployment rate shot up in the early months of the COVID-19 ...
U.S. Economy. COVID-19 is, first and foremost, a public health crisis, but it has had significant economic effects. With tens of millions of Americans told to stay home, the U.S. is at the ...
The survey found that 24 percent of respondents lost a job or income due to COVID-19. Most of these job or income losses were due to being furloughed or experiencing reduced work hours. However ...
Introduction. The onset of COVID19 pandemic saw unemployment climb to the highest rate since the Great Depression in many regions globally 1.Over just one month, from March to April 2020 unemployment rate in the United States increased from 4.4% to over 14.7% and in Australia the effective rate of unemployment increased from 5.4 to 11.7% (Australian Bureau of Statistics, 2020) 2.
at-home orders implemented in response to the COVID-19 pandemic and other pandemic-related factors affecting U.S. demand.5 States and localities implemented these 6orders to mitigate the risks of COVID-19 after it was declared a pandemic disease by the World Health Organization on March 11, 2020.7
I issued an invitation to the Associate Editors to submit a brief (3000 word) essay on the implications of COVID-19 on work and/or workers with an emphasis on research in the area. At the same time, a group of international scholars was coming together to consider the effects of COVID-19 on unemployment in several countries, and I invited that ...
That's in part due to the unemployment benefits included in the CARES Act, passed during the early stages of the coronavirus pandemic in the U.S., which provided unemployed Americans an ...
Write an essay on unemployment due to COVID-19 in 150 words - 49893772. rohitkumar22218 rohitkumar22218 31.01.2022 English Secondary School answered Write an essay on unemployment due to COVID-19 in 150 words See answer Advertisement
The Effect of Lockdown Measures on Unemployment. Unemployment increased sharply in all regions of the United States as a result of the public health response to the COVID-19 pandemic. But in some states the unemployment rate increased by fewer than 5 percentage points between February and June, while in others the rate increased more than 10 ...
April 2022, No. 22-12. Following early 2020 responses to the pandemic, labor force participation declined dramatically and has remained below its 2019 level, whereas the unemployment rate recovered briskly. We estimate the trend of labor force participation and unemployment and find a substantial impact of the pandemic on estimates of trend.