
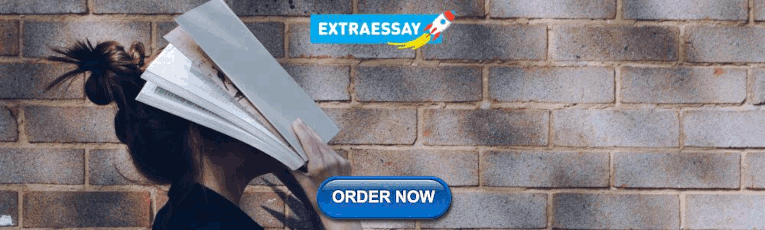
The Process of Problem Solving
- Editor's Choice
- Experimental Psychology
- Problem Solving
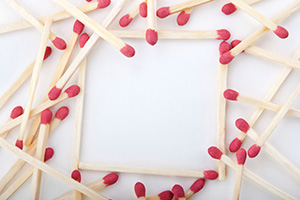
In a 2013 article published in the Journal of Cognitive Psychology , Ngar Yin Louis Lee (Chinese University of Hong Kong) and APS William James Fellow Philip N. Johnson-Laird (Princeton University) examined the ways people develop strategies to solve related problems. In a series of three experiments, the researchers asked participants to solve series of matchstick problems.
In matchstick problems, participants are presented with an array of joined squares. Each square in the array is comprised of separate pieces. Participants are asked to remove a certain number of pieces from the array while still maintaining a specific number of intact squares. Matchstick problems are considered to be fairly sophisticated, as there is generally more than one solution, several different tactics can be used to complete the task, and the types of tactics that are appropriate can change depending on the configuration of the array.
Louis Lee and Johnson-Laird began by examining what influences the tactics people use when they are first confronted with the matchstick problem. They found that initial problem-solving tactics were constrained by perceptual features of the array, with participants solving symmetrical problems and problems with salient solutions faster. Participants frequently used tactics that involved symmetry and salience even when other solutions that did not involve these features existed.
To examine how problem solving develops over time, the researchers had participants solve a series of matchstick problems while verbalizing their problem-solving thought process. The findings from this second experiment showed that people tend to go through two different stages when solving a series of problems.
People begin their problem-solving process in a generative manner during which they explore various tactics — some successful and some not. Then they use their experience to narrow down their choices of tactics, focusing on those that are the most successful. The point at which people begin to rely on this newfound tactical knowledge to create their strategic moves indicates a shift into a more evaluative stage of problem solving.
In the third and last experiment, participants completed a set of matchstick problems that could be solved using similar tactics and then solved several problems that required the use of novel tactics. The researchers found that participants often had trouble leaving their set of successful tactics behind and shifting to new strategies.
From the three studies, the researchers concluded that when people tackle a problem, their initial moves may be constrained by perceptual components of the problem. As they try out different tactics, they hone in and settle on the ones that are most efficient; however, this deduced knowledge can in turn come to constrain players’ generation of moves — something that can make it difficult to switch to new tactics when required.
These findings help expand our understanding of the role of reasoning and deduction in problem solving and of the processes involved in the shift from less to more effective problem-solving strategies.
Reference Louis Lee, N. Y., Johnson-Laird, P. N. (2013). Strategic changes in problem solving. Journal of Cognitive Psychology, 25 , 165–173. doi: 10.1080/20445911.2012.719021
good work for other researcher
APS regularly opens certain online articles for discussion on our website. Effective February 2021, you must be a logged-in APS member to post comments. By posting a comment, you agree to our Community Guidelines and the display of your profile information, including your name and affiliation. Any opinions, findings, conclusions, or recommendations present in article comments are those of the writers and do not necessarily reflect the views of APS or the article’s author. For more information, please see our Community Guidelines .
Please login with your APS account to comment.

Careers Up Close: Joel Anderson on Gender and Sexual Prejudices, the Freedoms of Academic Research, and the Importance of Collaboration
Joel Anderson, a senior research fellow at both Australian Catholic University and La Trobe University, researches group processes, with a specific interest on prejudice, stigma, and stereotypes.

Experimental Methods Are Not Neutral Tools
Ana Sofia Morais and Ralph Hertwig explain how experimental psychologists have painted too negative a picture of human rationality, and how their pessimism is rooted in a seemingly mundane detail: methodological choices.
APS Fellows Elected to SEP
In addition, an APS Rising Star receives the society’s Early Investigator Award.
Privacy Overview
Cookie | Duration | Description |
---|---|---|
__cf_bm | 30 minutes | This cookie, set by Cloudflare, is used to support Cloudflare Bot Management. |
Cookie | Duration | Description |
---|---|---|
AWSELBCORS | 5 minutes | This cookie is used by Elastic Load Balancing from Amazon Web Services to effectively balance load on the servers. |
Cookie | Duration | Description |
---|---|---|
at-rand | never | AddThis sets this cookie to track page visits, sources of traffic and share counts. |
CONSENT | 2 years | YouTube sets this cookie via embedded youtube-videos and registers anonymous statistical data. |
uvc | 1 year 27 days | Set by addthis.com to determine the usage of addthis.com service. |
_ga | 2 years | The _ga cookie, installed by Google Analytics, calculates visitor, session and campaign data and also keeps track of site usage for the site's analytics report. The cookie stores information anonymously and assigns a randomly generated number to recognize unique visitors. |
_gat_gtag_UA_3507334_1 | 1 minute | Set by Google to distinguish users. |
_gid | 1 day | Installed by Google Analytics, _gid cookie stores information on how visitors use a website, while also creating an analytics report of the website's performance. Some of the data that are collected include the number of visitors, their source, and the pages they visit anonymously. |
Cookie | Duration | Description |
---|---|---|
loc | 1 year 27 days | AddThis sets this geolocation cookie to help understand the location of users who share the information. |
VISITOR_INFO1_LIVE | 5 months 27 days | A cookie set by YouTube to measure bandwidth that determines whether the user gets the new or old player interface. |
YSC | session | YSC cookie is set by Youtube and is used to track the views of embedded videos on Youtube pages. |
yt-remote-connected-devices | never | YouTube sets this cookie to store the video preferences of the user using embedded YouTube video. |
yt-remote-device-id | never | YouTube sets this cookie to store the video preferences of the user using embedded YouTube video. |
yt.innertube::nextId | never | This cookie, set by YouTube, registers a unique ID to store data on what videos from YouTube the user has seen. |
yt.innertube::requests | never | This cookie, set by YouTube, registers a unique ID to store data on what videos from YouTube the user has seen. |

An official website of the United States government
The .gov means it’s official. Federal government websites often end in .gov or .mil. Before sharing sensitive information, make sure you’re on a federal government site.
The site is secure. The https:// ensures that you are connecting to the official website and that any information you provide is encrypted and transmitted securely.
- Publications
- Account settings
Preview improvements coming to the PMC website in October 2024. Learn More or Try it out now .
- Advanced Search
- Journal List
- CBE Life Sci Educ
- v.20(3); Fall 2021
A Detailed Characterization of the Expert Problem-Solving Process in Science and Engineering: Guidance for Teaching and Assessment
Argenta m. price.
† Department of Physics, Stanford University, Stanford, CA 94305
Candice J. Kim
‡ Graduate School of Education, Stanford University, Stanford, CA 94305
§ School of Medicine, Stanford University, Stanford, CA 94305
Eric W. Burkholder
Amy v. fritz.
¶ Department of Electrical Engineering, Stanford University, Stanford, CA 94305
Carl E. Wieman
Associated data.
A primary goal of science and engineering (S&E) education is to produce good problem solvers, but how to best teach and measure the quality of problem solving remains unclear. The process is complex, multifaceted, and not fully characterized. Here, we present a detailed characterization of the S&E problem-solving process as a set of specific interlinked decisions. This framework of decisions is empirically grounded and describes the entire process. To develop this, we interviewed 52 successful scientists and engineers (“experts”) spanning different disciplines, including biology and medicine. They described how they solved a typical but important problem in their work, and we analyzed the interviews in terms of decisions made. Surprisingly, we found that across all experts and fields, the solution process was framed around making a set of just 29 specific decisions. We also found that the process of making those discipline-general decisions (selecting between alternative actions) relied heavily on domain-specific predictive models that embodied the relevant disciplinary knowledge. This set of decisions provides a guide for the detailed measurement and teaching of S&E problem solving. This decision framework also provides a more specific, complete, and empirically based description of the “practices” of science.
INTRODUCTION
Many faculty members with new graduate students and many managers with employees who are recent college graduates have had similar experiences. Their advisees/employees have just completed a program of rigorous course work, often with distinction, but they seem unable to solve the real-world problems they encounter. The supervisor struggles to figure out exactly what the problem is and how they can guide the person in overcoming it. This paper is providing a way to answer those questions in the context of science and engineering (S&E). By characterizing the problem-solving process of experts, this paper investigates the “mastery” performance level and specifies an overarching learning goal for S&E students, which can be taught and measured to improve teaching.
The importance of problem solving as an educational outcome has long been recognized, but too often postsecondary S&E graduates have serious difficulties when confronted with real-world problems ( Quacquarelli Symonds, 2018 ). This reflects two long-standing educational problems with regard to problem solving: how to properly measure it, and how to effectively teach it. We theorize that the root of these difficulties is that good “problem solving” is a complex multifaceted process, and the details of that process have not been sufficiently characterized. Better characterization of the problem-solving process is necessary to allow problem solving, and more particularly, the complex set of skills and knowledge it entails, to be measured and taught more effectively. We sought to create an empirically grounded conceptual framework that would characterize the detailed structure of the full problem-solving process used by skilled practitioners when solving problems as part of their work. We also wanted a framework that would allow use and comparison across S&E disciplines. To create such a framework, we examined the operational decisions (choices among alternatives that result in subsequent actions) that these practitioners make when solving problems in their discipline.
Various aspects of problem solving have been studied across multiple domains, using a variety of methods (e.g., Newell and Simon, 1972 ; Dunbar, 2000 ; National Research Council [NRC], 2012b ; Lintern et al. , 2018 ). These ranged from expert self-reflections (e.g., Polya, 1945 ), to studies on knowledge lean tasks to discover general problem-solving heuristics (e.g., Egan and Greeno, 1974 ), to comparisons of expert and novice performances on simplified problems across a variety of disciplines (e.g., Chase and Simon, 1973 ; Chi et al. , 1981 ; Larkin and Reif, 1979 ; Ericsson et al. , 2006 , 2018 ). These studies revealed important novice–expert differences—notably, that experts are better at identifying important features and have knowledge structures that allow them to reduce demands on working memory. Studies that specifically gave the experts unfamiliar problems in their disciplines also found that, relative to novices, they had more deliberate and reflective strategies, including more extensive planning and managing of their own behavior, and they could use their knowledge base to better define the problem ( Schoenfeld, 1985 ; Wineburg, 1998 ; Singh, 2002 ). While these studies focused on discrete cognitive steps of the individual, an alternative framing of problem solving has been in terms of “ecological psychology” of “situativity,” looking at how the problem solver views and interacts with the environment in terms of affordances and constraints ( Greeno, 1994 ). “Naturalistic decision making” is a related framework that specifically examines how experts make decisions in complex, real-world, settings, with an emphasis on the importance of assessing the situation surrounding the problem at hand ( Klein, 2008 ; Mosier et al. , 2018 ).
While this work on expertise has provided important insights into the problem-solving process, its focus has been limited. Most has focused on looking for cognitive differences between experts and novices using limited and targeted tasks, such as remembering the pieces on a chessboard ( Chase and Simon, 1973 ) or identifying the important concepts represented in an introductory physics textbook problem ( Chi et al. , 1981 ). It did not attempt to explore the full process of solving, particularly for solving the type of complex problem that a scientist or engineer encounters as a member of the workforce (“authentic problems”).
There have also been many theoretical proposals as to expert problem-solving practices, but with little empirical evidence as to their completeness or accuracy (e.g., Polya, 1945 ; Heller and Reif, 1984 ; Organisation for Economic Cooperation and Development [OECD], 2019 ). The work of Dunbar (2000) is a notable exception to the lack of empirical work, as his group did examine how biologists solved problems in their work by analyzing lab meetings held by eight molecular biology research groups. His groundbreaking work focused on creativity and discovery in the research process, and he identified the importance of analogical reasoning and distributed reasoning by scientists in answering research questions and gaining new insights. Kozma et al. (2000) studied professional chemists solving problems, but their work focused only on the use of specialized representations.
The “cognitive systems engineering” approach ( Lintern et al. , 2018 ) takes a more empirically based approach looking at experts solving problems in their work, and as such tends to span aspects of both the purely cognitive and the ecological psychological theories. It uses both observations of experts in authentic work settings and retrospective interviews about how experts carried out particular work tasks. This theoretical framing and the experimental methods are similar to what we use, particularly in the “naturalistic decision making” area of research ( Mosier et al. , 2018 ). That work looks at how critical decisions are made in solving specific problems in their real-world setting. The decision process is studied primarily through retrospective interviews about challenging cases faced by experts. As described below, our methods are adapted from that work ( Crandall et al. , 2006 ), though there are some notable differences in focus and field. A particular difference is that we focused on identifying what are decisions to be made, which are more straight-forward to identify from retrospective interviews than how those decisions are made. We all have the same ultimate goal, however, to improve the training/teaching of the respective expertise.
Problem solving is central to the processes of science, engineering, and medicine, so research and educational standards about scientific thinking and the process and practices of science are also relevant to this discussion. Work by Osborne and colleagues describes six styles of scientific reasoning that can be used to explain how scientists and students approach different problems ( Kind and Osborne, 2016 ). There are also numerous educational standards and frameworks that, based on theory, lay out the skills or practices that science and engineering students are expected to master (e.g., American Association for the Advancement of Science [AAAS], 2011 ; Next Generation Science Standards Lead States, 2013 ; OECD, 2019 ; ABET, 2020 ). More specifically related to the training of problem solving, Priemer et al. (2020) synthesizes literature on problem solving and scientific reasoning to create a “STEM [science, technology, engineering, and mathematics] and computer science framework for problem solving” that lays out steps that could be involved in a students’ problem-solving efforts across STEM fields. These frameworks provide a rich groundwork, but they have several limitations: 1) They are based on theoretical ideas of the practice of science, not empirical evidence, so while each framework contains overlapping elements of the problem-solving process, it is unclear whether they capture the complete process. 2) They are focused on school science, rather than the actual problem solving that practitioners carry out and that students will need to carry out in future STEM careers. 3) They are typically underspecified, so that the steps or practices apply generally, but it is difficult to translate them into measurable learning goals for students to practice. Working to address that, Clemmons et al. (2020) recently sought to operationalize the core competencies from the Vision and Change report ( AAAS, 2011 ), establishing a set of skills that biology students should be able to master.
Our work seeks to augment this prior work by building a conceptual framework that is empirically based, grounded in how scientists and engineers solve problems in practice instead of in school. We base our framework on the decisions that need to be made during problem solving, which makes each item clearly defined for practice and assessment. In our analysis of expert problem solving, we empirically identified the entire problem-solving process. We found this includes deciding when and how to use the steps and skills defined in the work described previously but also includes additional elements. There are also questions in the literature about how generalizable across fields a particular set of practices may be. Here, we present the first empirical examination of the entire problem-solving process, and we compare that process across many different S&E disciplines.
A variety of instructional methods have been used to try and teach science and engineering problem solving, but there has been little evidence of their efficacy at improving problem solving (for a review, see NRC, 2012b ). Research explicitly on teaching problem solving has primarily focused on textbook-type exercises and utilized step-by-step strategies or heuristics. These studies have shown limited success, often getting students to follow specific procedural steps but with little gain in actually solving problems and showing some potential drawbacks ( Heller and Reif, 1984 ; Heller et al. , 1992 ; Huffman, 1997 ; Heckler, 2010 ; Kuo et al. , 2017 ). As discussed later, the framework presented here offers guidance for different and potentially more effective approaches to teaching problem solving.
These challenges can be illustrated by considering three different problems taken from courses in mechanical engineering, physics, and biology, respectively ( Figure 1 ). All of these problems are challenging, requiring considerable knowledge and effort by the student to solve correctly. Problems such as these are routinely used to both assess students’ problem-solving skills, and students are expected to learn such skills by practicing doing such problems. However, it is obvious to any expert in the respective fields, that, while these problems might be complicated and difficult to answer, they are vastly different from solving authentic problems in that field. They all have well-defined answers that can be reached by straightforward solution paths. More specifically, they do not involve needing to use judgment to make any decisions based on limited information (e.g., insufficient to specify a correct decision with certainty). The relevant concepts and information and assumptions are all stated or obvious. The failure of problems like these to capture the complexity of authentic problem solving underlies the failure of efforts to measure and teach problem solving. Recognizing this failure motivated our efforts to more completely characterize the problem-solving process of practicing scientists, engineers, and doctors.

Example problems from courses or textbooks in mechanical engineering, physics and biology. Problems from: Mechanical engineering: Wayne State mechanical engineering sample exam problems (Wayne State, n.d.), Physics: A standard physics problem in nearly every advanced quantum mechanics course, Biology: Molecular Biology of the Cell 6th edition, Chapter 7 end of chapter problems ( Alberts et al ., 2014 ).
We are building on the previous work studying expert–novice differences and problem solving but taking a different direction. We sought to create an empirically grounded framework that would characterize the detailed structure of the full problem-solving process by focusing on the operational decisions that skilled practitioners make when successfully solving authentic problems in their scientific, engineering, or medical work. We chose to identify the decisions that S&E practitioners made, because, unlike potentially nebulous skills or general problem-solving steps that might change with the discipline, decisions are sufficiently specified that they can be individually practiced by students and measured by instructors or departments. The authentic problems that we analyzed are typical problems practitioners encounter in “doing” the science or engineering entailed in their jobs. In the language of traditional problem-solving and expertise research, such authentic problems are “ill-structured” ( Simon, 1973 ) and require “adaptive expertise” ( Hatano and Inagaki, 1986 ) to solve. However, our authentic problems are considerably more complex and unstructured than what is normally considered in those literatures, because not only do they lack a clear solution path, but in many cases, it is not clear a priori that they have any solution at all. Determining that, and whether the problem needs to be redefined to be soluble, is part of the successful expert solution process. Another way in which our set of decisions goes beyond the characterization of what is involved in adaptive expertise is the prominent role of making judgments with limited information.
A common reaction of scientists and engineers to seeing the list of decisions we obtain as our primary result is, “Oh, yes, these are things I always do in solving problems. There is nothing new here.” It is comforting that these decisions all look familiar; that supports their validity. However, what is new is not that experts are making such decisions, but rather that there is a relatively small but complete set of decisions that has now been explicitly identified and that applies so generally.
We have used a much larger and broader sample of experts in this work than used in prior expert–novice studies, and we used a more stringent selection criterion. Previous empirical work has typically involved just a few experts, almost always in a single domain, and included graduate students as “experts” in some cases. Our semistructured interview sample was 31 experienced practitioners from 10 different disciplines of science, engineering, and medicine, with demonstrated competence and accomplishments well beyond those of most graduate students. Also, approximately 25 additional experts from across science, engineering, and medicine served as consultants during the planning and execution of this work.
Our research question was: What are the decisions experts make in solving authentic problems, and to what extent is this set of decisions to be made consistent both within and across disciplines?
Our approach was designed to identify the level of consistency and unique differences across disciplines. Our hypothesis was that there would be a manageable number (20–50) of decisions to be made, with a large amount of overlap of decisions made between experts within each discipline and a substantial but smaller overlap across disciplines. We believed that if we had found that every expert and/or discipline used a large and completely unique set of decisions, it would have been an interesting research result but of little further use. If our hypothesis turned out to be correct, we expected that the set of decisions obtained would have useful applications in guiding teaching and assessment, as they would show how experts in the respective disciplines applied their content knowledge to solve problems and hence provide a model for what to teach. We were not expecting to find the nearly complete degree of overlap in the decisions made across all the experts.
We first conducted 22 relatively unstructured interviews with a range of S&E experts, in which we asked about problem-solving expertise in their fields. From these interviews, we developed an initial list of decisions to be made in S&E problem solving. To refine and validate the list, we then carried out a set of 31 semistructured interviews in which S&E experts chose a specific problem from their work and described the solution process in detail. The semistructured interviews were coded for the decisions represented, either explicitly stated or implied by a choice of action. This provided a framework of decisions that characterize the problem-solving process across S&E disciplines. The research was approved by the Stanford Institutional Review Board (IRB no. 48785), and informed consent was obtained from all the participants.
This work involved interviewing many experts across different fields. We defined experts as practicing scientists, engineers, or physicians with considerable experience working as faculty at highly rated universities or having several years of experience working in moderately high-level technical positions at successful companies. We also included a few longtime postdocs and research staff in biosciences to capture more details of experimental decisions from which faculty members in those fields often were more removed. This definition of expert allows us to identify the practices of skilled professionals; we are not studying what makes only the most exceptional experts unique.
Experts were volunteers recruited through direct contact via the research team's personal and professional networks and referrals from experts in our networks. This recruitment method likely biased our sample toward people who experienced relatively similar training (most were trained in STEM disciplines at U.S. universities within the last 15–50 years). Within this limitation, we attempted to get a large range of experts by field and experience. This included people from 10 different fields (including molecular biology/biochemistry, ecology, and medicine), 11 U.S. universities, and nine different companies or government labs, and the sample was 33% female (though our engineering sample only included one female). The medical experts were volunteers from a select group of medical school faculty chosen to serve as clinical reasoning mentors for medical students at a prestigious university. We only contacted people who met our criteria for being an “expert,” and everyone who volunteered was included in the study. Most of the people who were contacted volunteered, and the only reason given for not volunteering was insufficient time. Other than their disciplinary expertise, there was little to distinguish these experts beyond the fact they were acquaintances with members of the team or acquaintances of acquaintances of team or project advisory board members. The precise number from each field was determined largely by availability of suitable experts.
We defined an “authentic problem” to be one that these experts solve in their actual jobs. Generally, this meant research projects for the science and engineering faculty, design problems for the industry engineers, and patient diagnoses for the medical doctors. Such problems are characterized by complexity, with many factors involved and no obvious solution process, and involve substantial time, effort, and resources. Such problems involve far more complexity and many more decisions, particularly decisions with limited information, than the typical problems used in previous problem-solving research or used with students in instructional settings.
Creating an Initial List of Problem-Solving Decisions
We first interviewed 22 experts ( Table 1 ), most of whom were faculty at a prestigious university, in which we asked them to discuss expertise and problem solving in their fields as it related to their own experiences. This usually resulted in their discussing examples of one or more problems they had solved. Based on the first seven interviews, plus reflections on personal experience from the research team and review of the literature on expert problem solving and teaching of scientific practices ( Ericsson et al. , 2006 ; NRC, 2012a ; Wieman, 2015 ), we created a generic list of decisions that were made in S&E problem solving. In the rest of the unstructured interviews (15), we also provided the experts with our list and asked them to comment on any additions or deletions they would suggest. Faculty who had close supervision of graduate students and industry experts who had extensively supervised inexperienced staff were particularly informative. Their observations of the way inexperienced people could fail made them sensitive to the different elements of expertise and where incorrect decisions could be made. Although we initially expected to find substantial differences across disciplines, from early in the process, we noted a high degree of overlap across the interviews in the decisions that were described.
Number of interviews conducted, by field of interviewee
Discipline | Informal interviews (creation of initial list) | Structured interviews (validation/refinement) | Notes |
---|---|---|---|
Biology (5 biochem/molecular bio, 2 cell bio, 1 plant bio, 1 immunology, 1 ecology) | 2 | 8 | Female: 6, URM: 2 5 faculty, 2 industry 3 acad staff/postdoc (year 5+) |
Medicine (6 internal med or pediatrics, 1 oncology, 2 surgery) | 4 | 6 | Female: 4, URM: 1 All medical faculty |
Physics (4 experiment, 3 theory) | 2 | 5 | Female: 1, URM: 1 All faculty |
Electrical Engineering | 4 | 3 | 2 faculty, 4 industry, 1 acad. staff |
Chemical Engineering | 2 | 2 | Female: 1 3 industry, 1 acad. staff |
Mechanical Engineering | 2 | 2 | URM: 1, 2 faculty, 2 industry |
Earth Science | 1 | 2 | Female: 2, 2 faculty, 1 industry |
Chemistry | 1 | 2 | Female: 2, all faculty |
Computer Science | 2 | 1 | Female: 1, 2 faculty, 1 industry |
Biological Engineering | 2 | – | All faculty or acad. staff |
Total | 22 | 31 | Female: 17, URM: 5 |
URM (under-represented minority) included 3 African American and 2 Hispanic/Latinx. One medical faculty member was interviewed twice – in both informal and structure interviews, for a total of 53 interviews with 52 experts.
Refinement and Validation of the List of Decisions
After creating the preliminary list of decisions from the informal interviews, we conducted a separate set of more structured interviews to test and refine the list. Semistructured interviews were conducted with 31 experts from across science, engineering, and medical fields ( Table 1 ). For these interviews, we recruited experts from a range of universities and companies, though the range of institutions is still limited, given the sample size. Interviews were conducted in person or over video chat and were transcribed for analysis. In the semistructured interviews, experts were asked to choose a problem or two from their work that they could recall the details of solving and then describe the process, including all the steps and decisions they made. So that we could get a full picture of the successful problem-solving process, we decided to focus the interviews on problems that they had eventually solved successfully, though their processes inherently involved paths that needed to be revised and reconsidered. Transcripts from interviewees who agreed to have their interview transcript published are available in the supplemental data set.
Our interview protocol (see Supplemental Text) was inspired in part by the critical decision method of cognitive task analysis ( Crandall et al. , 2006 ; Lintern et al. , 2018 ), which was created for research in cognitive systems engineering and naturalistic decision making. There are some notable differences between our work and theirs, both in research goal and method. First, their goal is to improve training in specific fields by focusing on how critical decisions are made in that field during an unusual or important event; the analysis seeks to identify factors involved in making those critical decisions. We are focusing on the overall problem solving and how it compares across many different fields, which quickly led to attention on what decisions are to be made, rather than how a limited set of those decisions are made. We asked experts to describe a specific, but not necessarily unusual, problem in their work, and focused our analysis on identifying all decisions made, not reasons for making them or identifying which were most critical. The specific order of problem-solving steps was also less important to us, in part because it was clear that there was no consistent order that was followed. Second, we are looking at different types of work. Cognitive systems engineering work has primarily focused on performance in professions like firefighters, power plant operators, military technicians, and nurses. These tend to require time-sensitive critical skills that are taught with modest amounts of formal training. We are studying scientists, engineers, and doctors solving problems that require much longer and less time-critical solutions and for which the formal training occupies many years.
Given our different focus, we made several adaptations to eliminate some of the more time-consuming steps from the interview protocol, allowing us to limit the interview time to approximately 1 hour. Both protocols seek to elicit an accurate and complete reporting of the steps taken and decisions made in the process of solving a problem. Our general strategy was: 1) Have the expert explain the problem and talk step by step through the decisions involved in solving it, with relatively few interruptions from the interviewer except to keep the discussion focused on the specific problem and occasionally to ask for clarifications. 2) Ask follow-up questions to probe for more detail about particular steps and aspects of the problem-solving process. 3) Occasionally ask for general thoughts on how a novice's process might differ.
While some have questioned the reliability of information from retrospective interviews ( Nisbett and Wilson, 1977 ), we believe we avoid these concerns, because we are only identifying a decision to be made, which in this case, means identifying a well-defined action that was chosen from alternatives. This is less subjective and much more likely to be accurately recalled than is the rationale behind such a decision. See Ericsson and Simon (1980) . However, the decisions identified may still be somewhat limited—the process of deciding among possible actions might involve additional decisions in the moment, when the solution is still unknown, that we are unable to capture in the retrospective context. For the decisions we can identify, we are able to check their accuracy and completeness by comparing them with the actions taken in the conduct of the research/design. For example, consider this quote from a physician who had to re-evaluate a diagnosis, “And, in my very subjective sense, he seemed like he was being forthcoming and honest. Granted people can fool you, but he seemed like he was being forthcoming. So we had to reevaluate.” The physician then considered alternative diagnoses that could explain a test result that at first had indicated an incorrect diagnosis. While this quote does describe the (retrospective) reasoning behind a decision, we do not need to know whether that reasoning is accurately recalled. We can simply code this as “decision 18, how believable is info?” The physician followed up by considering alternative diagnoses, which in this context was coded as “26, how good is solution?” and “8, potential solutions?” This was followed by the description of the literature and additional tests conducted. These indicated actions taken that confirm the physician made a decision about the reliability of the information given by the patient.
Interview Coding
We coded the semistructured interviews in terms of decisions made, through iterative rounds of coding ( Chi, 1997 ), following a “directed content analysis approach,” which involves coding according to predefined theoretical categories and updating the codes as needed based on the data ( Hsieh and Shannon, 2005 ). Our predefined categories were the list of decisions we had developed during the informal interviews. This approach means that we limited the focus of our qualitative analysis—we were able to test and refine the list of decisions, but we did not seek to identify all possible categories of approach to selecting and solving problems. The goals of each iterative round of coding are described in the next three paragraphs. To code for decisions in general, we matched decisions from the list to statements in each interview, based on the following criteria: 1) there was an explicit statement of a decision or choice made or needing to be made; 2) there was the description of the outcome of a decision, such as listing important features of the problem (that had been decided on) or conclusions arrived at; or 3) there was a statement of actions taken that indicated a decision about the appropriate action had been made, usually from a set of alternatives. Two examples illustrate the types of comments we identified as decisions: A molecular biologist explicitly stated the decisions required to decompose a problem into subproblems (decision 11), “Which cell do we use? The gene. Which gene do we edit? Which part of that gene do we edit? How do we build the enzyme that is going to do the cutting? … And how do we read out that it worked?” An ecologist made a statement that was also coded as a decomposition decision, because it described the action taken: “So I analyze the bird data first on its own, rather than trying to smash all the taxonomic groups together because they seem really apples and oranges. And just did two kinds of analysis, one was just sort of across all of these cases, around the world.” A single statement could be coded as multiple decisions if they were occurring simultaneously in the story being recalled or were intimately interconnected in the context of that interview, as with the ecology quote, in which the last sentence leads into deciding what data analysis is needed. Inherent in nearly every one of these decisions was that there was insufficient information to know the answer with certainty, so judgment was required.
Our primary goal for the first iterative round of coding was to check whether our list was complete by checking for any decisions that were missing, as indicated by either an action taken or a stated decision that was not clearly connected to a decision on our initial list. In this round, we also clarified wording and combined decisions that we were consistently unable to differentiate during the coding. A sample of three interviews (from biology, medicine, and electrical engineering) were first coded independently by four coders (AP, EB, CK, and AF), then discussed. The decision list was modified to add decisions and update wording based on that discussion. Then the interviews were recoded with the new list and rediscussed, leading to more refinements to the list. Two additional interviews (from physics and chemical engineering) were then coded by three coders (AP, EB, and CK) and further similar refinements were made. Throughout the subsequent rounds of coding, we continued to check for missing decisions, but after the additions and adjustments made based on these five interviews, we did not identify any more missing decisions.
In our next round of coding, we focused on condensing overlapping decisions and refining wording to improve the clarity of descriptions as they applied across different disciplinary contexts and to ensure consistent interpretation by different coders. Two or three coders independently coded an additional 11 interviews, iteratively meeting to discuss codes identified in the interviews, refining wording and condensing the list to improve agreement and combine overlapping codes, and then using the updated list to code subsequent interviews. We condensed the list by combining decisions that represented the same cognitive process taking place at different times, that were discipline-specific variations on the same decision, or that were substeps involved in making a larger decision. We noticed that some decisions were frequently co-coded with others, particularly in some disciplines. But if they were identified as distinct a reasonable fraction of the time in any discipline, we listed them as separate. This provided us with a list, condensed from 42 to 29 discrete decisions (plus five additional non-decision themes that were so prevalent that they are important to describe), that gave good consistency between coders.
Finally, we used the resulting codes to tabulate which decisions occurred in each interview, simplifying our coding process to focus on deciding whether or not each decision had occurred, with an example if it did occur to back up the “yes” code, but no longer attempting to capture every time each decision was mentioned. Individual coders identified decisions mentioned in the remaining 15 interviews. Interviews that had been coded with the early versions of the list were also recoded to ensure consistency. Coders flagged any decisions they were unsure about occurring in a particular interview, and two to four coders (AP, EB, CK, and CW) met to discuss those debated codes, with most uncertainties being resolved by explanations from a team member who had more technical expertise in the field of the interview. Minor wording changes were made during this process to ensure that each description of a decision captured all instantiations of the decision across disciplines, but no significant changes to the list were needed or made.
Coding an interview in terms of decisions made and actions taken in the research often required a high level of expertise in the discipline in question. The coder had to be familiar with the conduct of research in the field in order to recognize which actions corresponded to a decision between alternatives, but our team was assembled with this requirement in mind. It included high-level expertise across five different fields of science, engineering, and medicine and substantial familiarity with several other fields.
Supplemental Table S1 shows the final tabulation of decisions identified in each interview. In the tabulation, most decisions were marked as either “yes” or “no” for each interview, though 65 out of 1054 total were marked as “implied,” for one of the following reasons: 1) for 40/65, based on the coder's knowledge of the field, it was clear that a step must have been taken to achieve an outcome or action, even though that decision was not explicitly mentioned (e.g., interviewees describe collecting certain raw data and then coming to a specific conclusion, so they must have decided how to analyze the data, even if they did not mention the analysis explicitly); 2) for 15/65, the interview context was important, in that multiple statements from different parts of the interview taken together were sufficient to conclude that the decision must have happened, though no single statement described that decision explicitly; 3) 10/65 involved a decision that was explicitly discussed as an important step in problem solving, but they did not directly state how it was related to the problem at hand, or it was stated only in response to a direct prompt from the interviewer. The proportion of decisions identified in each interview, broken down by either explicit or explicit + implied, is presented in Supplemental Tables S1 and S2. Table 2 and Figure 2 of the main text show explicit + implied decision numbers.
Problem-solving decisions and percentages of expert interviews in which they occur a
A. Selection and goals (Occur in 100% ) | B. Frame problem (100%) | C. Plan process for solving (100%) | D. Interpret info and choose solutions (100%) | E. Reflect (100%) | F. Implications and communicate results (84%) |
---|---|---|---|---|---|
1. (61%) What is important in field? | 4. (100%) Important features and info? | 10. (100%) Approximations and simplifications to make? | 16. (81%) Which calculations and data analysis? | 23. (77%) Assumptions and simplifications appropriate? | 27. (65%) Broader implications? |
2. (77%) Opportunity fits solver’s expertise? | 5. (100%) What predictive framework? | 11. (68%) How to decompose into sub-problems? | 17. (68%) How to represent and organize information? | 24. (84%) Additional knowledge needed? | 28. (55%) Audience for communication? |
3. (100%) Goals, criteria, constraints? | 6. (97%) How to narrow down problem? | 12. (90%) Most difficult or uncertain areas? | 18. (77%) How believable is information? | 25. (94%) How well is solving approach working? | 29. (68%) Best way to present work? |
7. (97%) Related problems? | 13. (100%) What info needed? | 19. (100%) How does info compare to predictions? | 26. (100%) How good is solution? | ||
8. (100%) Potential Solutions? | 14. (87%) Priorities? | 20. (71%) Any significant anomalies? | |||
9. (74%) Is problem solvable? | 15. (100%) Specific plan for getting information? | 21. (97%) Appropriate conclusions? | |||
22. (97%) What is best solution? |
a See supplementary text and Table S2 for full description and examples of each decision. A set of other non-decision knowledge and skill development themes were also frequently mentioned as important to professional success: Staying up to date in the field (84%), intuition and experience (77%), interpersonal and teamwork (100%), efficiency (32%), and attitude (68%).
b Percentage of interviews in which category or decision was mentioned.
c Numbering is for reference. In practice ordering is fluid – involves extensive iteration with other possible starting points.
d Chosen predictive framework(s) will inform all other decisions.
e Reflection occurs throughout process, and often leads to iteration. Reflection on solution occurs at the end as well.

Proportion of decisions coded in interviews by field. This tabulation includes decisions 1–29, not the additional themes. Error bars represent standard deviations. Number of interviews: total = 31; physical science = 9; biological science = 8; engineering = 8; medicine = 6. Compared with the sciences, slightly fewer decisions overall were identified in the coding of engineering and medicine interviews, largely for discipline-specific reasons. See Supplemental Table S2 and associated discussion.
Two of the interviews that had not been discussed during earlier rounds of coding (one physics [AP and EB], one medicine [AP and CK]) were independently coded by two coders to check interrater reliability using the final list of decisions. The goal of our final coding was to tabulate whether or not each expert described making each decision at any point in the problem-solving process, so the level of detail we chose for coding and interrater reliability was whether or not a decision was present in the entire interview. The decisions identified in each interview were compared for the two coders. For both interviews, the raters disagreed on whether or not only one of the 29 decisions occurred. Codes of “implied” were counted as agreement if the other coder selected either “yes” or “implied.” This equates to a percent agreement of 97% for each interview (28 agree/29 total decisions per interview = 97%). As a side note, there was also one disagreement per interview on the coding of the five other themes, but those themes were not a focus of this work nor the interviews.
We identified a total set of 29 decisions to be made (plus five other themes), all of which were identified in a large fraction of the interviews across all disciplines ( Table 2 and Figure 2 ). There was a surprising degree of overlap across the different fields with all the experts mentioning similar decisions to be made. All 29 were evident by the fifth semistructured interview, and on average, each interview revealed 85% of the 29 decisions. Many decisions occurred multiple times in an interview, with the number of times varying widely, depending on the length and complexity of the problem-solving process discussed.
We focused our analysis on what decisions needed to be made, not on the experts’ processes for making those decisions: noting that a choice happened, not how they selected and chose among different alternatives. This is because, while the decisions to be made were the same across disciplines, how the experts made those decisions varied greatly by discipline and individual. The process of making the decisions relied on specialized disciplinary knowledge and experience and may vary depending on demographics or other factors that our study design (both our sample and nature of retrospective interviews) did not allow us to investigate. However, while that knowledge was distinct and specialized, we could tell that it was consistently organized according to a common structure we call a “predictive framework,” as discussed in the “ Predictive Framework ” section below. Also, while every “decision” reflected a step in the problem solving involved in the work, and the expert being interviewed was involved in making or approving the decision, that does not mean the decision process was carried out only by that individual. In many cases, the experts described the decisions made in terms of ideas and results of their teams, and the importance of interpersonal skills and teamwork was an important non-decision theme raised in all interviews.
We were particularly concerned with the correctness and completeness of the set of decisions. Although the correctness was largely established by the statements in the interviews, we also showed the list of decisions to these experts at the end of the interviews as well as to about a dozen other experts. In all cases, they all agreed that these decisions were ones they and others in their field made when solving problems. The completeness of the list of decisions was confirmed by: 1) looking carefully at all specific actions taken in the described problem-solving process and checking that each action matched a corresponding decision from the list; and 2) the high degree of consistency in the set of decisions across all the interviews and disciplines. This implies that it is unlikely that there are important decisions that we are missing, because that would require any such missing decisions to be consistently unspoken by all 31 interviewees as well as consistently unrecognized by us from the actions that were taken in the problem-solving process.
In focusing on experts’ recollections of their successful solving of problems, our study design may have missed decisions that experts only made during failed problem-solving attempts. However, almost all interviews described solution paths that were not smooth and continuous, but rather involved going down numerous dead ends. There were approaches that were tried and failed, data that turned out to be ambiguous and worthless, and so on. Identifying the failed path involved reflection decisions (23–26). Often decision 9 (is problem solvable?) would be mentioned, because it described a path that was determined to be not solvable. For example, a biologist explained, “And then I ended up just switching to a different strain that did it [crawling off the plate] less. Because it was just … hard to really get them to behave themselves. I suppose if I really needed to rely on that very particular one, I probably would have exhausted the possibilities a bit more.” Thus, we expect unsuccessful problem solving would entail a smaller subset of decisions being made, particularly lack of reflection decisions, or poor choices on the decisions, rather than making a different set of decisions.
The set of decisions represent a remarkably consistent structure underlying S&E problem solving. For the purposes of presentation, we have categorized the decisions as shown in Figure 3 , roughly based on the purposes they achieve. However, the process is far less orderly and sequential than implied by this diagram, or in fact any characterization of an orderly “scientific method.” We were struck by how variable the sequence of decisions was in the descriptions provided. For example, experts who described how they began work on a problem sometimes discussed importance and goals (1–3, what is important in field?; opportunity fits solver’s expertise?; and goals, criteria, constraints?), but others mentioned a curious observation (20, any significant anomalies?), important features of their system that led them to questions (4, important features and info?, 6, how to narrow down problem?), or other starting points. We also saw that there were flexible connections between decisions and repeated iterations—jumping back to the same type of decision multiple times in the solution process, often prompted by reflection as new information and insights were developed. The sequence and number of iterations described varied dramatically by interview, and we cannot determine to what extent this was due to legitimate differences in the problem-solving process or to how the expert recalled and chose to describe the process. This lack of a consistent starting point, with jumping and iterating between decisions, has also been identified in the naturalistic decision-making literature ( Mosier et al. , 2018 ). Finally, the experts also often described considering multiple decisions simultaneously. In some interviews, a few decisions were always described together, while in others, they were clearly separate decisions. In summary, while the specific decisions themselves are fully grounded in expert practice, the categories and order shown here are artificial simplifications for presentation purposes.

Representation of problem-solving decisions by categories. The black arrows represent a hypothetical but unrealistic order of operations, the blue arrows represent more realistic iteration paths. The decisions are grouped into categories for presentation purposes; numbers indicate the number of decisions in each category. Knowledge and skill development were commonly mentioned themes but are not decisions.
The decisions contained in the seven categories are summarized here. See Supplemental Table S2 for specific examples of each decision across multiple disciplines.
Category A. Selection and Goals of the Problem
This category involves deciding on the importance of the problem, what criteria a solution must meet, and how well it matches the capabilities, resources, and priorities of the expert. As an example, an earth scientist described the goal of her project (decision 3, goals, criteria, constraints?) to map and date the earliest volcanic rocks associated with what is now Yellowstone and explained why the project was a good fit for her group (2, opportunity fits solver’s expertise?) and her decision to pursue the project in light of the significance of this type of eruption in major extinction events (1, what is important in field?). In many cases, decisions related to framing (see category B) were mentioned before decisions in this category or were an integral part of the process for developing goals.
Decisions in this category are:
- 1. What is important in the field?
- What are important questions or problems? Where is the field heading? Are there advances in the field that open new possibilities?
- 2. Opportunity fits solver's expertise?
- If and where are there gaps/opportunities to solve in field? Given experts’ unique perspectives and capabilities, are there opportunities particularly accessible to them? (This could involve challenging the status quo, questioning assumptions in the field.)
- 3. Goals, criteria, constraints?
- a. What are the goals, design criteria, or requirements of the problem or its solution?
- b. What is the scope of the problem?
- c. What constraints are there on the solution?
- d. What will be the criteria on which the solution is evaluated?
Category B. Frame Problem
These decisions lead to a more concrete formulation of the solution process and potential solutions. This involves identifying the key features of the problem and deciding on predictive frameworks to use (see “ Predictive Framework ” section below), as well as narrowing down the problem, often forming specific questions or hypotheses. Many of these decisions are guided by past problem solutions with which the expert is familiar and sees as relevant. The framing decisions of a physician can be seen in his discussion of a patient with liver failure who had previously been diagnosed with HIV but had features (4, important features and info?; 5, what predictive framework?) that made the physician question the HIV diagnosis (5, what predictive framework?; 26, how good is solution?). His team then searched for possible diagnoses that could explain liver failure and lead to a false-positive HIV test (7, related problems?; 8, potential solutions?), which led to their hypothesis the patient might have Q fever (6, how to narrow down problem?; 13, what info needed?; 15, specific plan for getting info?). While each individual decision is strongly supported by the data, the categories are groupings for presentation purposes. In particular, framing (category B) and planning (see category C) decisions often blended together in interviews.
- a. Which available information is relevant to problem solving and why?
- b. (When appropriate) Create/find a suitable abstract representation of core ideas and information Examples: physics, equation representing process involved; chemistry, bond diagrams/potential energy surfaces; biology, diagram of pathway steps.
- 5. What predictive framework?
- Which potential predictive frameworks to use? (Decide among possible predictive frameworks or create framework.) This includes deciding on the appropriate level of mechanism and structure that the framework needs to embody to be most useful for the problem at hand.
- 6. How to narrow down the problem?
- How to narrow down the problem? Often involves formulating specific questions and hypotheses.
- 7. Related problems?
- What are related problems or work seen before, and what aspects of their problem-solving process and solutions might be useful in the present context? (This may involve reviewing literature and/or reflecting on experience.)
- 8. Potential solutions?
- What are potential solutions? (This is based on experience and fitting some criteria for solution they have for a problem having general key features identified.)
- 9. Is problem solvable?
- Is the problem plausibly solvable and is the solution worth pursuing given the difficulties, constraints, risks, and uncertainties?
Category C. Plan the Process for Solving
These decisions establish the specifics needed to solve the problem and include: how to simplify the problem and decompose it into pieces, what specific information is needed, how to obtain that information, and what are the resources needed and priorities? Planning by an ecologist can be seen in her extensive discussion of her process of simplifying (10, approximations/simplifications to make?) a meta-analysis project about changes in migration behavior, which included deciding what types of data she needed (13, what info needed?), planning how to conduct her literature search (15, specific plan for getting info?), difficulties in analyzing the data (12, most difficult/uncertain areas?; 16, which calculations and data analysis?), and deciding to analyze different taxonomic groups separately (11, how to decompose into subproblems?). In general, decomposition often resulted in multiple iterations through the problem-solving decisions, as subsets of decisions need to be made about each decomposed aspect of a problem. Framing (category B) and planning (category C) decisions occupied much of the interviews, indicating their importance.
- 10. Approximations and simplifications to make?
- What approximations or simplifications are appropriate? How to simplify the problem to make it easier to solve? Test possible simplifications/approximations against established criteria.
- 11. How to decompose into subproblems?
- How to decompose the problem into more tractable subproblems? (Subproblems are independently solvable pieces with their own subgoals.)
- 12. Most difficult or uncertain areas?
- a. What are acceptable levels of uncertainty with which to proceed at various stages?
- 13. What info needed?
- a. What will be sufficient to test and distinguish between potential solutions?
- 14. Priorities?
- What to prioritize among many competing considerations? What to do first and how to obtain necessary resources?
- Considerations could include: What's most important? Most difficult? Addressing uncertainties? Easiest? Constraints (time, materials, etc.)? Cost? Optimization and trade-offs? Availability of resources? (facilities/materials, funding sources, personnel)
- 15. Specific plan for getting information?
- a. What are the general requirements of a problem-solving approach, and what general approach will they pursue? (These decisions are often made early in the problem-solving process as part of framing.)
- b. How to obtain needed information? Then carry out those plans. (This could involve many discipline- and problem-specific investigation possibilities such as: designing and conducting experiments, making observations, talking to experts, consulting the literature, doing calculations, building models, or using simulations.)
- c. What are achievable milestones, and what are metrics for evaluating progress?
- d. What are possible alternative outcomes and paths that may arise during the problem-solving process, both consistent with predictive framework and not, and what would be paths to follow for the different outcomes?
Category D. Interpret Information and Choose Solution(s)
This category includes deciding how to analyze, organize, and draw conclusions from available information, reacting to unexpected information, and deciding upon a solution. A biologist studying aging in worms described how she analyzed results from her experiments, which included representing her results in survival curves and conducting statistical analyses (16, which calculations and data analysis?; 17, how to represent and organize info?), as well as setting up blind experiments (15, specific plan for getting info?) so that she could make unbiased interpretations (18, how believable is info?) of whether a worm was alive or dead. She also described comparing results with predictions to justify the conclusion that worm aging was related to fertility (19, how does info compare to predictions?; 21, appropriate conclusions?; 22, what is best solution?). Deciding how results compared with expectations based on a predictive framework was a key decision that often preceded several other decisions.
- 16. Which calculations and data analysis?
- What calculations and data analysis are needed? Once determined, these must then be carried out.
- 17. How to represent and organize information?
- What is the best way to represent and organize available information to provide clarity and insights? (Usually this will involve specialized and technical representations related to key features of predictive framework.)
- 18. How believable is the information?
- Is information valid, reliable, and believable (includes recognizing potential biases)?
- 19. How does information compare to predictions?
- As new information comes in, particularly from experiments or calculations, how does it compare with expected results (based on the predictive framework)?
- 20. Any significant anomalies?
- a. Does potential anomaly fit within acceptable range of predictive framework(s) (given limitations of predictive framework and underlying assumptions and approximations)?
- b. Is potential anomaly an unusual statistical variation or relevant data? Is it within acceptable levels of uncertainty?
- 21. Appropriate conclusions?
- What are appropriate conclusions based on the data? (This involves making conclusions and deciding if they are justified.)
- 22. What is the best solution?
- a. Which of multiple candidate solutions are consistent with all available information and which can be rejected? (This could be based on comparing data with predicted results.)
- b. What refinements need to be made to candidate solutions?
Category E. Reflect
Reflection decisions occur throughout the process and include deciding whether assumptions are justified, whether additional knowledge or information is needed, how well the solution approach is working, and whether potential and then final solutions are adequate. These decisions match the categories of reflection identified by Salehi (2018) . A mechanical engineer described developing a model (to inform surgical decisions) of which muscles allow the thumb to function in the most useful manner (22, what is best solution?), including reflecting on how well engineering approximations applied in the biological context (23, assumptions and simplifications appropriate?). He also described reflecting on his approach, that is, why he chose to use cadaveric models instead of mathematical models (25, how well is solving approach working?), and the limitations of his findings in that the “best” muscle identified was difficult to access surgically (26, how good is solution?; 27, broader implications?). Reflection decisions are made throughout the problem-solving process, often lead to reconsidering other decisions, and are critical for success.
- 23. Assumptions and simplifications appropriate?
- a. Do the assumptions and simplifications made previously still look appropriate considering new information?
- b Does predictive framework need to be modified?
- 24. Additional knowledge needed?
- a. Is solver's relevant knowledge sufficient?
- b. Is more information needed and, if so, what?
- c. Does some information need to be checked? (Is there a need to repeat experiment or check a different source?)
- 25. How well is the problem-solving approach working?
- How well is the problem-solving approach working, and does it need to be modified? This includes possibly modifying the goals. (One needs to reflect on one's strategy by evaluating progress toward the solution.) and reflecting on one’s strategy by evaluating progress toward the solution.
- 26. How good is the solution?
- a. Decide by exploring possible failure modes and limitations—“try to break” solution.
- b. Does it “make sense” and pass discipline-specific tests for solutions of this type of problem?
- c. Does it completely meet the goals/criteria?
Category F. Implications and Communication of Results
These are decisions about the broader implications of the work, and how to communicate results most effectively. For example, a theoretical physicist developing a method to calculate the magnetic moment of the muon decided on who would be interested in his work (28, audience for communication?) and what would be the best way to present it (29, best way to present work?). He also discussed the implications of preliminary work on a simplified aspect of the problem (10, approximations and simplifications to make?) in terms of evaluating its impact on the scientific community and deciding on next steps (27, broader implications?; 29, best way to present work?). Many interviewees described that making decisions in this category affected their decisions in other categories.
- 27. Broader implications?
- What are the broader implications of the results, including over what range of contexts does the solution apply? What outstanding problems in the field might it solve? What novel predictions can it enable? How and why might this be seen as interesting to a broader community?
- 28. Audience for communication?
- What is the audience for communication of work, and what are their important characteristics?
- 29. Best way to present work?
- What is the best way to present the work to have it understood, and its correctness and importance appreciated? How to make a compelling story of the work?
Category G. Ongoing Skill and Knowledge Development
Although we focused on decisions in the problem-solving process, the experts volunteered general skills and knowledge they saw as important elements of problem-solving expertise in their fields. These included teamwork and interpersonal skills (strongly emphasized), acquiring experience and intuition, and keeping abreast of new developments in their fields.
Non-decision themes in this category are:
- 30. Stay up to date in field
- a. Reviewing literature, which does involve making decisions as to which is important.
- b. Learning relevant new knowledge (ideas and technology from literature, conferences, colleagues, etc.)
- 31. Intuition and experience
- Acquiring experience and associated intuition to improve problem solving.
- 32. Interpersonal, teamwork
- Includes navigating collaborations, team management, patient interactions, communication skills, etc., particularly as how these apply in the context of the various types of problem-solving processes.
- 33. Efficiency
- Time management including learning to complete certain common tasks efficiently and accurately.
- 34. Attitude
- Motivation and attitude toward the task. Factors such as interest, perseverance, dealing with stress, and confidence in decisions.
Predictive Framework
How the decisions were made was highly dependent on the discipline and problem. However, there was one element that was fundamental and common across all interviews: the early adoption of a “predictive framework” that the experts used throughout the problem-solving process. We define this framework as “a mental model of key features of the problem and the relationships between the features.” All the predictive frameworks involved some degree of simplification and approximation and an underlying level of mechanism that established the relationships between key features. The frameworks provided a structure of knowledge and facilitated the application of that knowledge to the problem at hand, allowing experts to repeatedly run “mental simulations” to make predictions for dependencies and observables and to interpret new information.
As an example, an ecologist described her predictive framework for migration, which incorporated important features such as environmental conditions and genetic differences between species and the mechanisms by which these interacted to impact the migration patterns for a species. She used this framework to guide her meta-analysis of changes in migration patterns, affecting everything from her choice of data sets to include to her interpretation of why migration patterns changed for different species. In many interviews, the frameworks used evolved as additional information was obtained, with additional features being added or underlying assumptions modified. For some problems, the relevant framework was well established and used with confidence, while for other problems, there was considerable uncertainty as to a suitable framework, so developing and testing the framework was a substantial part of the solution process.
A predictive framework contains the expert knowledge organization that has been observed in previous studies of expertise ( Egan and Greeno, 1974 ) but goes further, as here it serves as an explicit tool that guides most decisions and actions during the solving of complex problems. Mental models and mental simulations that are described in the naturalistic decision-making literature are similar, in that they are used to understand the problem and guide decisions ( Klein, 2008 ; Mosier et al. , 2018 ), but they do not necessarily contain the same level of mechanistic understanding of relationships that underlies the predictive frameworks used in science and engineering problem solving. While the use of predictive frameworks was universal, the individual frameworks themselves explicitly reflected the relevant specialized knowledge, structure, and standards of the discipline, and arguably largely define a discipline ( Wieman, 2019 ).
Discipline-Specific Variation
While the set of decisions to be made was highly consistent across disciplines, there were extensive differences within and across disciplines and work contexts, which reflected the differences in perspectives and experiences. These differences were usually evident in how experts made each of the specific decisions, but not in the choice of which decisions needed to be made. In other words, the solution methods, which included following standard accepted procedures in each field, were very different. For example, planning in some experimental sciences may involve formulating a multiyear construction and data-collection effort, while in medicine it may be deciding on a simple blood test. Some decisions, notably in categories A, D, and F, were less likely to be mentioned in particular disciplines, because of the nature of the problems. Specifically, decisions 1 (what is important in field?), 2 (opportunity fits solver’s expertise?), 27 (broader implications?), 28 (audience for communication?), and 29 (best way to present work?) were dependent on the scope of the problem being described and the expert's specific role in it. These were mentioned less frequently in interviews where the problem was assigned to the expert (most often engineering or industry) or where the importance or audience was implicit (most often in medicine). Decisions 16 (which calculations and data analysis?) and 17 (how to represent and organize info?) were particularly unlikely to be mentioned in medicine, because test results are typically provided to doctors not in the form or raw data, but rather already analyzed by a lab or other medical technology professional, so the doctors we interviewed did not need to make decisions themselves about how to analyze or represent the data. Qualitatively, we also noticed some differences between disciplines in the patterns of connections between decisions. When the problem involved development of a tool or product, most commonly the case in engineering, the interview indicated relatively rapid cycles between goals (3), framing problem/potential solutions (8), and reflection on the potential solution (26), before going through the other decisions. Biology, the experimental science most represented in our interviews, had strong links between planning (15), deciding on appropriate conclusions (21), and reflection on the solution (26). This is likely because the respective problems involved complex systems with many unknowns, so careful planning was unusually important for achieving definitive conclusions. See Supplemental Text and Supplemental Table S2 for additional notes on decisions that were mentioned at lower frequency and decisions that were likely to be interconnected, regardless of field.
This work has created a framework of decisions to characterize problem solving in science and engineering. This framework is empirically based and captures the successful problem-solving process of all experts interviewed. We see that several dozen experts across many different fields all make a common set of decisions when solving authentic problems. There are flexible linkages between decisions that are guided by reflection in a continually evolving process. We have also identified the nature of the “predictive frameworks” that S&E experts consistently use in problem solving. These predictive frameworks reveal how these experts organize their disciplinary knowledge to facilitate making decisions. Many of the decisions we identified are reflected in previous work on expertise and scientific problem solving. This is particularly true for those listed in the planning and interpreting information categories ( Egan and Greeno, 1974 ). The priority experts give to framing and planning decisions over execution compared with novices has been noted repeatedly (e.g., Chi et al. , 1988 ). Expert reflection has been discussed, but less extensively ( Chase and Simon, 1973 ), and elements of the selection and implications and communication categories have been included in policy and standards reports (e.g., AAAS, 2011 ). Thus, our framework of decisions is consistent with previous work on scientific practices and expertise, but it is more complete, specific, empirically based, and generalizable across S&E disciplines.
A limitation of this study is the small number of experts we have in total, from each discipline, and from underrepresented groups (especially lack of female representation in engineering). The lack of randomized selection of participants may also bias the sample toward experts who experienced similar academic training (STEM disciplines at U.S. universities). This means we cannot prove that there are not some experts who follow other paths in problem solving. As with any scientific model, the framework described here should be subjected to further tests and modifications as necessary. However, to our knowledge, this is a far larger sample than used in any previous study of expert problem solving. Although we see a large amount of variation both within and across disciplines in the problem-solving process, this is reflected in how experts make decisions, not in what decisions they make. The very high degree of consistency in the decisions made across the entire sample strongly suggests that we are capturing elements that are common to all experts across science and engineering. A second limitation is that decisions often overlap and co-occur in an interview, so the division between decision items is often somewhat ambiguous and could be defined somewhat differently. As noted, a number of these decisions can be interconnected, and in some fields are nearly always interconnected.
The set of decisions we have observed provides a general framework for characterizing, analyzing, and teaching S&E problem solving. These decisions likely define much of the set of cognitive skills a student needs to practice and master to perform as a skilled practitioner in S&E. This framework of decisions provides a detailed and structured way to approach the teaching and measurement of problem solving at the undergraduate, graduate, and professional training levels. For teaching, we propose using the process of “deliberate practice” ( Ericsson, 2018 ) to help students learn problem solving. Deliberate practice of problem solving would involve effective scaffolding and concentrated practice, with feedback, at making the specific decisions identified here in relevant contexts. In a course, this would likely involve only an appropriately selected set of the decisions, but a good research mentor would ensure that trainees have opportunities to practice and receive feedback on their performance on each of these 29 decisions. Future work is needed to determine whether there are additional decisions that were not identified in experts but are productive components of student problem solving and should also be practiced. Measurements of individual problem-solving expertise based on our decision list and the associated discipline-specific predictive frameworks will allow a detailed measure of an individual's discipline-specific problem-solving strengths and weaknesses relative to an established expert. This can be used to provide targeted feedback to the learner, and when aggregated across students in a program, feedback on the educational quality of the program. We are currently working on the implementation of these ideas in a variety of instructional settings and will report on that work in future publications.
As discussed in the Introduction , typical science and engineering problems fail to engage students in the complete problem-solving process. By considering which of the 29 decisions are required to answer the problem, we can more clearly articulate why. The biology problem, for example, requires students to decide on a predictive framework and access the necessary content knowledge, and they need to decide which information they need to answer the problem. However, other decisions are not required or are already made for them, such as deciding on important features and identifying anomalies. We propose that different problems, designed specifically to require students to make sets of the problem-solving decisions from our framework, will provide more effective tools for measuring, practicing, and ultimately mastering the full S&E problem-solving process.
Our preliminary work with the use of such decision-based problems for assessing problem-solving expertise is showing great promise. For several different disciplines, we have given test subjects a relevant context, requiring content knowledge covered in courses they have taken, and asked them to make decisions from the list presented here. Skilled practitioners in the relevant discipline respond in very consistent ways, while students respond very differently and show large differences that typically correlate with their different educational experiences. What apparently matters is not what content they have seen, but rather what decisions they have had practice making. Our approach was to identify the decisions made by experts, this being the task that educators want students to master. Our data do not exclude the possibility that students engage in and/or should learn other decisions as a productive part of the problem-solving process while they are learning. Future work would seek to identify decisions made at intermediate levels during the development of expertise, to identify potential learning progressions that could be used to teach problem solving more efficiently. What we have seen is consistent with previous work identifying expert–novice differences but provides a much more extensive and detailed picture of a student's strengths and weaknesses and the impacts of particular educational experiences. We have also carried out preliminary development of courses that explicitly involve students making and justifying many of these decisions in relevant contexts, followed by feedback on their decisions. Preliminary results from these courses are also encouraging. Future work will involve the more extensive development and application of decision-based measurement and teaching of problem solving.
Supplementary Material
Acknowledgments.
We acknowledge the many experts who agreed to be interviewed for this work, M. Flynn for contributions on expertise in mechanical engineering, and Shima Salehi for useful discussions. This work was funded by the Howard Hughes Medical Institute through an HHMI Professor grant to C.E.W.
- ABET. (2020). Criteria for accrediting engineering programs, 2020–2021 . Retrieved November 23, 2020, from www.abet.org/accreditation/accreditation-criteria/criteria-for-accrediting-engineering-programs-2020-2021
- Alberts, B., Johnson, A., Lewis, J., Morgan, D., Raff, M., Roberts, K., Walter, P. (2014). Control of gene expression . In Molecular Biology of the Cell (6th ed., pp. 436–437). New York: Garland Science. Retrieved November 12, 2020, from https://books.google.com/books?id=2xIwDwAAQBAJ [ Google Scholar ]
- American Association for the Advancement of Science. (2011). Vision and change in undergraduate biology education: A call to action . Washington, DC. Retrieved February 12, 2021, from https://visionandchange.org/finalreport [ Google Scholar ]
- Chase, W. G., Simon, H. A. (1973). Perception in chess . Cognitive Psychology , 4 ( 1 ), 55–81. 10.1016/0010-0285(73)90004-2 [ CrossRef ] [ Google Scholar ]
- Chi, M. T. H. (1997). Quantifying qualitative analyses of verbal data: A practical guide . Journal of the Learning Sciences , 6 ( 3 ), 271–315. 10.1207/s15327809jls0603_1 [ CrossRef ] [ Google Scholar ]
- Chi, M. T. H., Feltovich, P. J., Glaser, R. (1981). Categorization and representation of physics problems by experts and novices . Cognitive Science , 5 ( 2 ), 121–152. 10.1207/s15516709cog0502_2 [ CrossRef ] [ Google Scholar ]
- Chi, M. T. H., Glaser, R., Farr, M. J.( (1988). The nature of expertise . Hillsdale, NJ: Erlbaum. [ Google Scholar ]
- Clemmons, A. W., Timbrook, J., Herron, J. C., Crowe, A. J. (2020). BioSkills Guide: Development and national validation of a tool for interpreting the Vision and Change core competencies . CBE—Life Sciences Education , 19 ( 4 ), ar53. 10.1187/cbe.19-11-0259 [ PMC free article ] [ PubMed ] [ CrossRef ] [ Google Scholar ]
- Crandall, B., Klein, G. A., Hoffman, R. R. (2006). Working minds: A practitioner's guide to cognitive task analysis . Cambridge, MA: MIT Press. [ Google Scholar ]
- Dunbar, K. (2000). How scientists think in the real world: Implications for science education . Journal of Applied Developmental Psychology , 21 ( 1 ), 49–58. 10.1016/S0193-3973(99)00050-7 [ CrossRef ] [ Google Scholar ]
- Egan, D. E., Greeno, J. G. (1974). Theory of rule induction: Knowledge acquired in concept learning, serial pattern learning, and problem solving in L . In Gregg, W. (Ed.), Knowledge and cognition . Potomac, MD: Erlbaum. [ Google Scholar ]
- Ericsson, K. A. (2018). The differential influence of experience, practice, and deliberate practice on the development of superior individual performance of experts . In Ericcson, K. A., Hoffman, R. R., Kozbelt, A., Williams, A. M. (Eds.), The Cambridge handbook of expertise and expert performance (2nd ed., pp. 745–769). Cambridge, United Kingdom: Cambridge University Press. 10.1017/9781316480748.038 [ CrossRef ] [ Google Scholar ]
- Ericsson, K. A., Charness, N., Feltovich, P. J., Hoffman, R. R., (Eds.) (2006). The Cambridge handbook of expertise and expert performance . Cambridge, United Kingdom: Cambridge University Press. [ Google Scholar ]
- Ericsson, K. A., Hoffman, R. R., Kozbelt, A., Williams, A. A., (Eds.) (2018). The Cambridge handbook of expertise and expert performance (2nd ed.). Cambridge, United Kingdom: Cambridge University Press. [ Google Scholar ]
- Ericsson, K. A., Simon, H. A. (1980). Verbal reports as data . Psychological Review , 87 ( 3 ), 215–251. 10.1037/0033-295X.87.3.215 [ CrossRef ] [ Google Scholar ]
- Greeno, J. G. (1994). Gibson's affordances . Psychological Review , 101 ( 2 ), 336–342. doi: 10.1037/0033-295X.101.2.336 [ PubMed ] [ CrossRef ] [ Google Scholar ]
- Hatano, G., Inagaki, K. (1986). Two courses of expertise . In Stevenson, H. W., Azuma, H., Hakuta, K. (Eds.), A series of books in psychology. Child development and education in Japan (pp. 262–272). New York: Freeman/Times Books/Henry Holt. [ Google Scholar ]
- Heckler, A. F. (2010). Some consequences of prompting novice physics students to construct force diagrams . International Journal of Science Education , 32 ( 14 ), 1829–1851. 10.1080/09500690903199556 [ CrossRef ] [ Google Scholar ]
- Heller, J. I., Reif, F. (1984). Prescribing effective human problem-solving processes: Problem description in physics . Cognition and Instruction , 1 ( 2 ), 177–216. 10.1207/s1532690xci0102_2 [ CrossRef ] [ Google Scholar ]
- Heller, P., Keith, R., Anderson, S. (1992). Teaching problem solving through cooperative grouping. Part 1: Group versus individual problem solving . American Journal of Physics , 60 , 627–636. 10.1119/1.17117 [ CrossRef ] [ Google Scholar ]
- Hsieh, H-F., Shannon, S. E. (2005). Three approaches to qualitative content analysis . Qualitative Health Research , 15 ( 9 ), 1277–1288. 10.1177/1049732305276687 [ PubMed ] [ CrossRef ] [ Google Scholar ]
- Huffman, D. (1997). Effect of explicit problem-solving instruction on high school students’ problem-solving performance and conceptual understanding of physics . Journal of Research in Science Teaching , 34 ( 6 ), 551–570. 10.1002/(SICI)1098-2736(199708)34:6<551::AID-TEA2>3.0.CO;2-M [ CrossRef ] [ Google Scholar ]
- Kind, P., Osborne, J. (2016). Styles of scientific reasoning: A cultural rationale for science education? Science Education , 10 ( 1 ), 8–31. 10.1002/sce.21251 [ CrossRef ] [ Google Scholar ]
- Klein, G. (2008). Naturalistic decision making . Human Factors , 50 ( 3 ), 456–460. [ PubMed ] [ Google Scholar ]
- Kozma, R., Chin, E., Russell, J., Marx, N. (2000). The roles of representations and tools in the chemistry laboratory and their implications for chemistry learning . Journal of the Learning Sciences , 9 ( 2 ), 105–143. [ Google Scholar ]
- Kuo, E., Hallinen, N. R., Conlin, L. D. (2017). When procedures discourage insight: Epistemological consequences of prompting novice physics students to construct force diagrams . International Journal of Science Education , 39 ( 7 ), 814–839. 10.1080/09500693.2017.1308037 [ CrossRef ] [ Google Scholar ]
- Larkin, J., Reif, F. (1979). Understanding and teaching problem-solving in physics . European Journal of Science Education , 1 ( 2 ), 191–203. doi: 10.1080/0140528790010208 [ CrossRef ] [ Google Scholar ]
- Lintern, G., Moon, B., Klein, G., Hoffman, R. (2018). Eliciting and representing the knowledge of experts . In Ericcson, K. A., Hoffman, R. R., Kozbelt, A., Williams, A. M. (Eds.), The Cambridge handbook of expertise and expert performance (2nd ed). (pp. 165–191). Cambridge, United Kingdom: Cambridge University Press. [ Google Scholar ]
- Mosier, K., Fischer, U., Hoffman, R. R., Klein, G. (2018). Expert professional judgments and “naturalistic decision making.” In Ericcson, K. A., Hoffman, R. R., Kozbelt, A., Williams, A. M. (Eds.), The Cambridge handbook of expertise and expert performance (2nd ed). (pp. 453–475). Cambridge, United Kingdom: Cambridge University Press. [ Google Scholar ]
- National Research Council (NRC). (2012a). A framework for K–12 science education: Practices, crosscutting concepts, and core ideas . Washington, DC: National Academies Press. [ Google Scholar ]
- NRC. (2012b). Problem solving, spatial thinking, and the use of representations in science and engineering . In Discipline-based education research: Understanding and improving learning in undergraduate science and engineering (pp. 75–118). Washington, DC: National Academies Press. 10.17226/13362 [ CrossRef ] [ Google Scholar ]
- Newell, A., Simon, H. A. (1972). Human problem solving . Prentice-Hall. [ Google Scholar ]
- Next Generation Science Standards Lead States. (2013). Next Generation Science Standards: For states, by states . Washington, DC: National Academies Press. [ Google Scholar ]
- Nisbett, R. E., Wilson, T. D. (1977). Telling more than we can know: Verbal reports on mental processes . Psychological Review , 84 ( 3 ), 231–259. 10.1037/0033-295X.84.3.231 [ CrossRef ] [ Google Scholar ]
- Organisation for Economic Cooperation and Development. (2019). PISA 2018 science framework . In PISA 2018 assessment and analytical framework (pp. 97–117). Paris: OECD Publishing. 10.1787/f30da688-en [ CrossRef ] [ Google Scholar ]
- Polya, G. (1945). How to solve it: A new aspect of mathematical method . Princeton, NJ: Princeton University Press. [ Google Scholar ]
- Priemer, B., Eilerts, K., Filler, A., Pinkwart, N., Rösken-Winter, B., Tiemann, R., Upmeier Zu Belzen, A. (2020). A framework to foster problem-solving in STEM and computing education . Research in Science & Technological Education , 38 ( 1 ), 105–130. 10.1080/02635143.2019.1600490 [ CrossRef ] [ Google Scholar ]
- Quacquarelli Symonds. (2018). The global skills gap in the 21st century . Retrieved July 20, 2021, from www.qs.com/portfolio-items/the-global-skills-gap-in-the-21st-century/
- Salehi, S. (2018 ). Improving problem-solving through reflection (Doctoral dissertation) . Stanford Digital Repository, Stanford University. Retrieved February 18, 2021, from https://purl.stanford.edu/gc847wj5876 [ Google Scholar ]
- Schoenfeld, A. H. (1985). Mathematical problem solving . Orlando, FL: Academic Press. [ Google Scholar ]
- Simon, H. (1973). The structure of ill structured problems . Artificial Intelligence , 4 ( 3–4 ), 181–201. 10.1016/0004-3702(73)90011-8 [ CrossRef ] [ Google Scholar ]
- Singh, C. (2002). When physical intuition fails . American Journal of Physics , 70 , 1103–1109. 10.1119/1.1512659 [ CrossRef ] [ Google Scholar ]
- Wayne State University. (n.d). Mechanical engineering practice qualifying exams. Wayne State University Mechanical Engineering department . Retrieved February 23, 2021, from https://engineering.wayne.edu/me/exams/mechanics_of_materials_-_sample_pqe_problems_.pdf
- Wieman, C. E. (2015). Comparative cognitive task analyses of experimental science and instructional laboratory courses . Physics Teacher , 53 , 349–351. 10.1119/1.4928349 [ CrossRef ] [ Google Scholar ]
- Wieman, C. E. (2019). Expertise in university teaching & the implications for teaching effectiveness, evaluation & training . Daedalus , 148 ( 4 ), 47–78. 10.1162/daed_a_01760 [ CrossRef ] [ Google Scholar ]
- Wineburg, S. (1998). Reading Abraham Lincoln: An expert/expert study in the interpretation of historical texts . Cognitive Science , 22 ( 3 ), 319–346. 10.1016/S0364-0213(99)80043-3 [ CrossRef ] [ Google Scholar ]
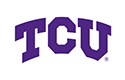
Research Process: Research Problem
- Getting Started
- Research Problem
- Research Question(s)
- Literature Review
- Research Methods
- Quantifying Results
- Writing & Presenting
- Search Tips
Quick Overview
Basically, the research problem defines and guides your entire research process.
1. Pick something you are actually interested in.
2. Your problem statement should be clear and concise.
3. The problem should be important.
4. The problem should increase or advance knowledge in the field (or for the company). It should demonstrate a gap between what is known and what is unknown, or a gap between what is wanted and what is observed. The problem statement should indicate an area of conflict, concern, or controversy.
5. The problem should be something that is feasible (time, money, resources) for you to tackle.
6. You should be able to gather information and/or data to help solve the problem. (i.e., you can research the problem)
Full Overview of the Research Problem
Constructing the Research Problem: A book chapter
Above is a link to a short book chapter that covers what a research problem is and how to create it. The chapter discusses different types of research problems and different approaches to a research problem. It also provides some examples.
Video on Research Problem
The video below is geared towards a specific type of research in health sciences. Focus on the discussions of the research problem.
Examples of Problem Statements
Problem Statement #1:
Overfill has been a serious problem facing our city waste facilities for the last decade. By some estimations, our city dumps are, on average, 30% above capacity—an unsanitary, unsafe, and unwise position for our city to be in.
Several methods have been proposed in order to combat this. Perhaps the most popular of these is the simplest: building two new landfills on the county outskirts. Others have proposed stronger recycling campaigns and larger per-bag waste disposal costs as a way to lessen the potential damage of our trash situation.
Bluffington is close to drowning in trash. Action is needed if our city is to remain the clean, safe place to live it has always been.
Problem Statement #2:
We want all of our software releases to go to production seamlessly, without defects, where everyone is aware and informed of the outcomes and status.
Today we have too many release failures that result in too many rollback failures. If we ignore this problem; resources will need to increase to handle the cascading problems, and we may miss critical customer deadlines which could result in lost revenue, SLA penalties, lost business, and further damage to our quality reputation.
We will use our Kaizen Blitz methodology in evaluating the last release to help us improve our processes.
- << Previous: Getting Started
- Next: Research Question(s) >>
- Last Updated: Oct 26, 2023 8:00 AM
- URL: https://libguides.tcu.edu/ResearchProcess

The Art of Effective Problem Solving: A Step-by-Step Guide
Author: Daniel Croft
Daniel Croft is an experienced continuous improvement manager with a Lean Six Sigma Black Belt and a Bachelor's degree in Business Management. With more than ten years of experience applying his skills across various industries, Daniel specializes in optimizing processes and improving efficiency. His approach combines practical experience with a deep understanding of business fundamentals to drive meaningful change.
Whether we realise it or not, problem solving skills are an important part of our daily lives. From resolving a minor annoyance at home to tackling complex business challenges at work, our ability to solve problems has a significant impact on our success and happiness. However, not everyone is naturally gifted at problem-solving, and even those who are can always improve their skills. In this blog post, we will go over the art of effective problem-solving step by step.
You will learn how to define a problem, gather information, assess alternatives, and implement a solution, all while honing your critical thinking and creative problem-solving skills. Whether you’re a seasoned problem solver or just getting started, this guide will arm you with the knowledge and tools you need to face any challenge with confidence. So let’s get started!
Problem Solving Methodologies
Individuals and organisations can use a variety of problem-solving methodologies to address complex challenges. 8D and A3 problem solving techniques are two popular methodologies in the Lean Six Sigma framework.
Methodology of 8D (Eight Discipline) Problem Solving:
The 8D problem solving methodology is a systematic, team-based approach to problem solving. It is a method that guides a team through eight distinct steps to solve a problem in a systematic and comprehensive manner.
The 8D process consists of the following steps:

- Form a team: Assemble a group of people who have the necessary expertise to work on the problem.
- Define the issue: Clearly identify and define the problem, including the root cause and the customer impact.
- Create a temporary containment plan: Put in place a plan to lessen the impact of the problem until a permanent solution can be found.
- Identify the root cause: To identify the underlying causes of the problem, use root cause analysis techniques such as Fishbone diagrams and Pareto charts.
- Create and test long-term corrective actions: Create and test a long-term solution to eliminate the root cause of the problem.
- Implement and validate the permanent solution: Implement and validate the permanent solution’s effectiveness.
- Prevent recurrence: Put in place measures to keep the problem from recurring.
- Recognize and reward the team: Recognize and reward the team for its efforts.
Download the 8D Problem Solving Template
A3 Problem Solving Method:
The A3 problem solving technique is a visual, team-based problem-solving approach that is frequently used in Lean Six Sigma projects. The A3 report is a one-page document that clearly and concisely outlines the problem, root cause analysis, and proposed solution.
The A3 problem-solving procedure consists of the following steps:
- Determine the issue: Define the issue clearly, including its impact on the customer.
- Perform root cause analysis: Identify the underlying causes of the problem using root cause analysis techniques.
- Create and implement a solution: Create and implement a solution that addresses the problem’s root cause.
- Monitor and improve the solution: Keep an eye on the solution’s effectiveness and make any necessary changes.
Subsequently, in the Lean Six Sigma framework, the 8D and A3 problem solving methodologies are two popular approaches to problem solving. Both methodologies provide a structured, team-based problem-solving approach that guides individuals through a comprehensive and systematic process of identifying, analysing, and resolving problems in an effective and efficient manner.
Step 1 – Define the Problem
The definition of the problem is the first step in effective problem solving. This may appear to be a simple task, but it is actually quite difficult. This is because problems are frequently complex and multi-layered, making it easy to confuse symptoms with the underlying cause. To avoid this pitfall, it is critical to thoroughly understand the problem.
To begin, ask yourself some clarifying questions:
- What exactly is the issue?
- What are the problem’s symptoms or consequences?
- Who or what is impacted by the issue?
- When and where does the issue arise?
Answering these questions will assist you in determining the scope of the problem. However, simply describing the problem is not always sufficient; you must also identify the root cause. The root cause is the underlying cause of the problem and is usually the key to resolving it permanently.
Try asking “why” questions to find the root cause:
- What causes the problem?
- Why does it continue?
- Why does it have the effects that it does?
By repeatedly asking “ why ,” you’ll eventually get to the bottom of the problem. This is an important step in the problem-solving process because it ensures that you’re dealing with the root cause rather than just the symptoms.
Once you have a firm grasp on the issue, it is time to divide it into smaller, more manageable chunks. This makes tackling the problem easier and reduces the risk of becoming overwhelmed. For example, if you’re attempting to solve a complex business problem, you might divide it into smaller components like market research, product development, and sales strategies.
To summarise step 1, defining the problem is an important first step in effective problem-solving. You will be able to identify the root cause and break it down into manageable parts if you take the time to thoroughly understand the problem. This will prepare you for the next step in the problem-solving process, which is gathering information and brainstorming ideas.
Step 2 – Gather Information and Brainstorm Ideas

Gathering information and brainstorming ideas is the next step in effective problem solving. This entails researching the problem and relevant information, collaborating with others, and coming up with a variety of potential solutions. This increases your chances of finding the best solution to the problem.
Begin by researching the problem and relevant information. This could include reading articles, conducting surveys, or consulting with experts. The goal is to collect as much information as possible in order to better understand the problem and possible solutions.
Next, work with others to gather a variety of perspectives. Brainstorming with others can be an excellent way to come up with new and creative ideas. Encourage everyone to share their thoughts and ideas when working in a group, and make an effort to actively listen to what others have to say. Be open to new and unconventional ideas and resist the urge to dismiss them too quickly.
Finally, use brainstorming to generate a wide range of potential solutions. This is the place where you can let your imagination run wild. At this stage, don’t worry about the feasibility or practicality of the solutions; instead, focus on generating as many ideas as possible. Write down everything that comes to mind, no matter how ridiculous or unusual it may appear. This can be done individually or in groups.
Once you’ve compiled a list of potential solutions, it’s time to assess them and select the best one. This is the next step in the problem-solving process, which we’ll go over in greater detail in the following section.
Step 3 – Evaluate Options and Choose the Best Solution
Once you’ve compiled a list of potential solutions, it’s time to assess them and select the best one. This is the third step in effective problem solving, and it entails weighing the advantages and disadvantages of each solution, considering their feasibility and practicability, and selecting the solution that is most likely to solve the problem effectively.
To begin, weigh the advantages and disadvantages of each solution. This will assist you in determining the potential outcomes of each solution and deciding which is the best option. For example, a quick and easy solution may not be the most effective in the long run, whereas a more complex and time-consuming solution may be more effective in solving the problem in the long run.
Consider each solution’s feasibility and practicability. Consider the following:
- Can the solution be implemented within the available resources, time, and budget?
- What are the possible barriers to implementing the solution?
- Is the solution feasible in today’s political, economic, and social environment?
You’ll be able to tell which solutions are likely to succeed and which aren’t by assessing their feasibility and practicability.
Finally, choose the solution that is most likely to effectively solve the problem. This solution should be based on the criteria you’ve established, such as the advantages and disadvantages of each solution, their feasibility and practicability, and your overall goals.
It is critical to remember that there is no one-size-fits-all solution to problems. What is effective for one person or situation may not be effective for another. This is why it is critical to consider a wide range of solutions and evaluate each one based on its ability to effectively solve the problem.
Step 4 – Implement and Monitor the Solution

When you’ve decided on the best solution, it’s time to put it into action. The fourth and final step in effective problem solving is to put the solution into action, monitor its progress, and make any necessary adjustments.
To begin, implement the solution. This may entail delegating tasks, developing a strategy, and allocating resources. Ascertain that everyone involved understands their role and responsibilities in the solution’s implementation.
Next, keep an eye on the solution’s progress. This may entail scheduling regular check-ins, tracking metrics, and soliciting feedback from others. You will be able to identify any potential roadblocks and make any necessary adjustments in a timely manner if you monitor the progress of the solution.
Finally, make any necessary modifications to the solution. This could entail changing the solution, altering the plan of action, or delegating different tasks. Be willing to make changes if they will improve the solution or help it solve the problem more effectively.
It’s important to remember that problem solving is an iterative process, and there may be times when you need to start from scratch. This is especially true if the initial solution does not effectively solve the problem. In these situations, it’s critical to be adaptable and flexible and to keep trying new solutions until you find the one that works best.
To summarise, effective problem solving is a critical skill that can assist individuals and organisations in overcoming challenges and achieving their objectives. Effective problem solving consists of four key steps: defining the problem, generating potential solutions, evaluating alternatives and selecting the best solution, and implementing the solution.
You can increase your chances of success in problem solving by following these steps and considering factors such as the pros and cons of each solution, their feasibility and practicability, and making any necessary adjustments. Furthermore, keep in mind that problem solving is an iterative process, and there may be times when you need to go back to the beginning and restart. Maintain your adaptability and try new solutions until you find the one that works best for you.
- Novick, L.R. and Bassok, M., 2005. Problem Solving . Cambridge University Press.
Was this helpful?

Daniel Croft
Daniel Croft is a seasoned continuous improvement manager with a Black Belt in Lean Six Sigma. With over 10 years of real-world application experience across diverse sectors, Daniel has a passion for optimizing processes and fostering a culture of efficiency. He's not just a practitioner but also an avid learner, constantly seeking to expand his knowledge. Outside of his professional life, Daniel has a keen Investing, statistics and knowledge-sharing, which led him to create the website www.learnleansigma.com, a platform dedicated to Lean Six Sigma and process improvement insights.

8D (8 Disciplines)

What is the (PDCA) Plan, Do, Check, Act Cycle?
Free lean six sigma templates.
Improve your Lean Six Sigma projects with our free templates. They're designed to make implementation and management easier, helping you achieve better results.

5S Floor Marking Best Practices
In lean manufacturing, the 5S System is a foundational tool, involving the steps: Sort, Set…
How to Measure the ROI of Continuous Improvement Initiatives
When it comes to business, knowing the value you’re getting for your money is crucial,…
8D Problem-Solving: Common Mistakes to Avoid
In today’s competitive business landscape, effective problem-solving is the cornerstone of organizational success. The 8D…
The Evolution of 8D Problem-Solving: From Basics to Excellence
In a world where efficiency and effectiveness are more than just buzzwords, the need for…
8D: Tools and Techniques
Are you grappling with recurring problems in your organization and searching for a structured way…
How to Select the Right Lean Six Sigma Projects: A Comprehensive Guide
Going on a Lean Six Sigma journey is an invigorating experience filled with opportunities for…
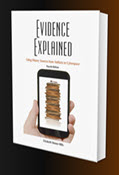
QuickTips: The Blog @ Evidence Explained
How To Solve a Research Problem
Elizabeth Shown Mills
It’s doable. Really. All it takes are two nevers and three always.
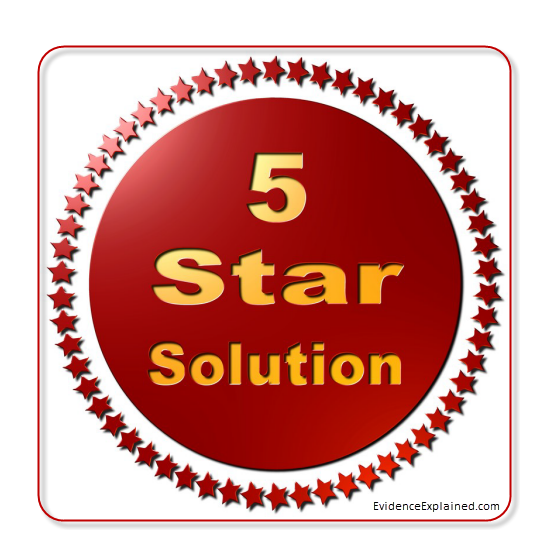
1. Never rely on a single document for any point, even when it tells you exactly what you want to know. Records often err and we won’t know it until and unless we test their assertions against other records. With any piece of research our goal should be to use all relevant records and to make certain that anything we use to “corroborate” something else has totally independent origins.
2. Never assume any type of record is reliable. Record types are like people. Some are totally dependable. Some make us cringe or roll our eyeballs. Most are a mixture. We love what’s good about them. We’re wise to look warily at the flaws.
3. Always test each finding—no matter how small it may be—against the Evidence Analysis Process Map . The ultimate “fact” in all projects is this: Our conclusion can never be better than the evidence on which we base it.
4. Always use each finding to lead you to something else. No fact is an absolute. No record is an end to itself. Facts and records are always part of something much bigger. To understand the fragment we’ve found and interpret it correctly, we need the rest of what went on.
5. Always contrast, compare, and challenge. Each new piece of information needs to be scrutinized rigorously. We study not just the details in a record but the construction of the record and the record set. We watch for anomalies in the records and discrepancies between them.
We study the behavior of the people who created each record. We study the laws that governed the record—and the degree to which participants and their communities c omplied with those laws. We constantly look for ways to measure differences and similarities—and ways to quantify actions. We watch for behavior that is aberrant to community norms—which means we may also have to study the community and its records deeply enough to define those norms.
Yes. It’s doable. I didn’t say it’s quick 'n easy. But it’s doable. The issue now is this: How badly do you want to solve that research problem?
PHOTO CREDITS: "5 Star Solution," CanStockPhoto (http://www.canstockphoto.com/images-photos/solution.html#file_view.php?id=16887728 : downloaded 7 April 2015), image csp16887728, uploaded by drcmarx, 20 November 2013; used under license.
Posted 8 April 2015
- Corroboration
- Evidence analysis
- Problem solving
- Log in or register to post comments
- 20668 views
© Evidence Explained 2011-2024.
- google plus
Educational resources and simple solutions for your research journey
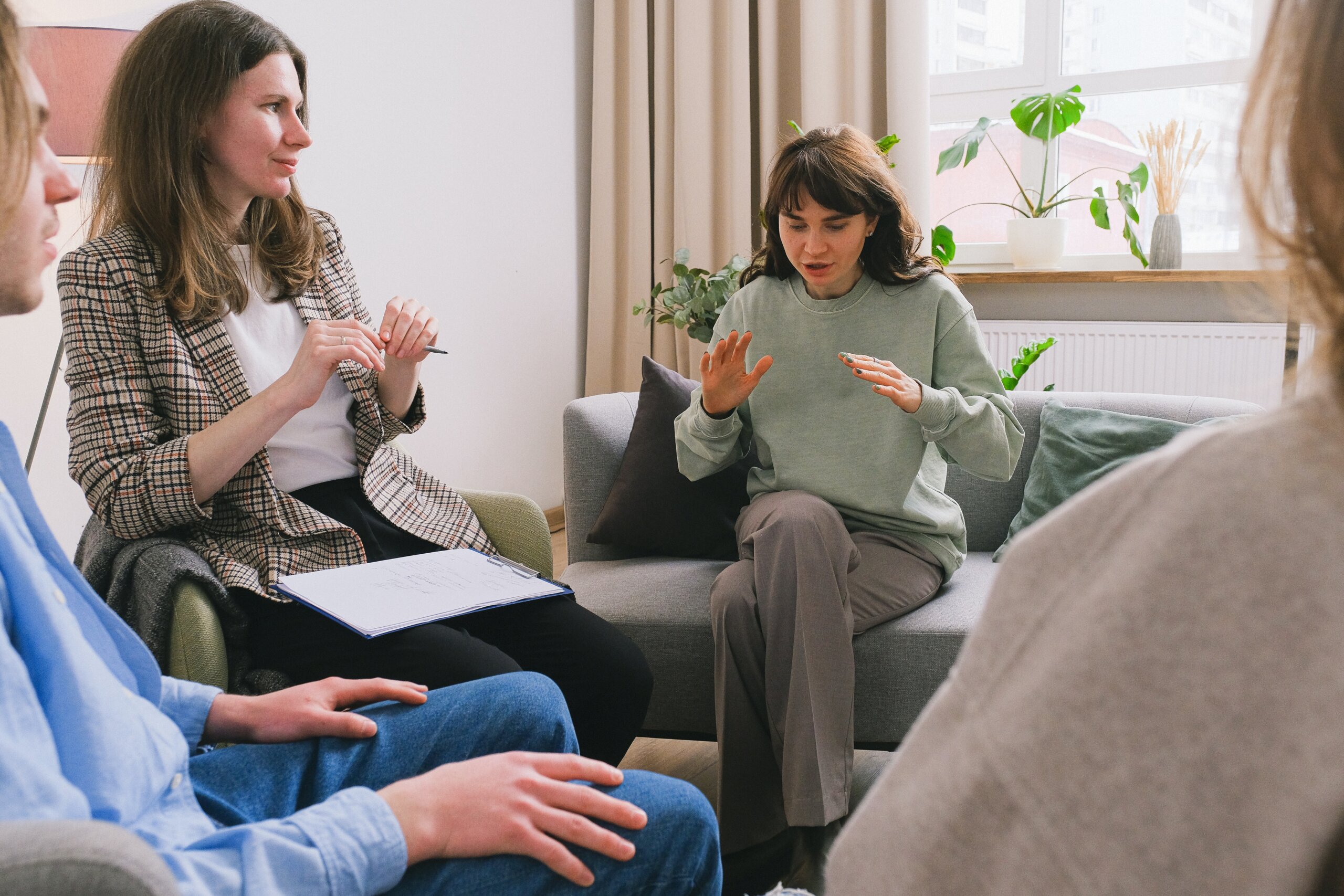
What is a Research Problem? Characteristics, Types, and Examples
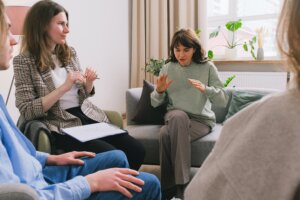
A research problem is a gap in existing knowledge, a contradiction in an established theory, or a real-world challenge that a researcher aims to address in their research. It is at the heart of any scientific inquiry, directing the trajectory of an investigation. The statement of a problem orients the reader to the importance of the topic, sets the problem into a particular context, and defines the relevant parameters, providing the framework for reporting the findings. Therein lies the importance of research problem s.
The formulation of well-defined research questions is central to addressing a research problem . A research question is a statement made in a question form to provide focus, clarity, and structure to the research endeavor. This helps the researcher design methodologies, collect data, and analyze results in a systematic and coherent manner. A study may have one or more research questions depending on the nature of the study.
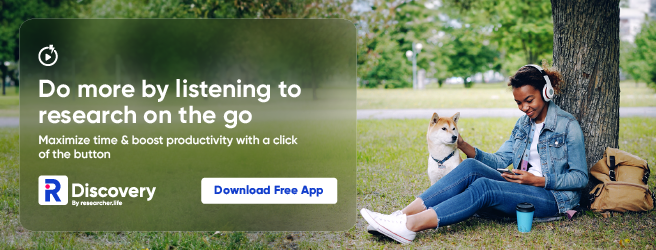
Identifying and addressing a research problem is very important. By starting with a pertinent problem , a scholar can contribute to the accumulation of evidence-based insights, solutions, and scientific progress, thereby advancing the frontier of research. Moreover, the process of formulating research problems and posing pertinent research questions cultivates critical thinking and hones problem-solving skills.
Table of Contents
What is a Research Problem ?
Before you conceive of your project, you need to ask yourself “ What is a research problem ?” A research problem definition can be broadly put forward as the primary statement of a knowledge gap or a fundamental challenge in a field, which forms the foundation for research. Conversely, the findings from a research investigation provide solutions to the problem .
A research problem guides the selection of approaches and methodologies, data collection, and interpretation of results to find answers or solutions. A well-defined problem determines the generation of valuable insights and contributions to the broader intellectual discourse.
Characteristics of a Research Problem
Knowing the characteristics of a research problem is instrumental in formulating a research inquiry; take a look at the five key characteristics below:
Novel : An ideal research problem introduces a fresh perspective, offering something new to the existing body of knowledge. It should contribute original insights and address unresolved matters or essential knowledge.
Significant : A problem should hold significance in terms of its potential impact on theory, practice, policy, or the understanding of a particular phenomenon. It should be relevant to the field of study, addressing a gap in knowledge, a practical concern, or a theoretical dilemma that holds significance.
Feasible: A practical research problem allows for the formulation of hypotheses and the design of research methodologies. A feasible research problem is one that can realistically be investigated given the available resources, time, and expertise. It should not be too broad or too narrow to explore effectively, and should be measurable in terms of its variables and outcomes. It should be amenable to investigation through empirical research methods, such as data collection and analysis, to arrive at meaningful conclusions A practical research problem considers budgetary and time constraints, as well as limitations of the problem . These limitations may arise due to constraints in methodology, resources, or the complexity of the problem.
Clear and specific : A well-defined research problem is clear and specific, leaving no room for ambiguity; it should be easily understandable and precisely articulated. Ensuring specificity in the problem ensures that it is focused, addresses a distinct aspect of the broader topic and is not vague.
Rooted in evidence: A good research problem leans on trustworthy evidence and data, while dismissing unverifiable information. It must also consider ethical guidelines, ensuring the well-being and rights of any individuals or groups involved in the study.
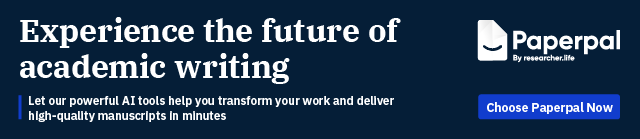
Types of Research Problems
Across fields and disciplines, there are different types of research problems . We can broadly categorize them into three types.
- Theoretical research problems
Theoretical research problems deal with conceptual and intellectual inquiries that may not involve empirical data collection but instead seek to advance our understanding of complex concepts, theories, and phenomena within their respective disciplines. For example, in the social sciences, research problem s may be casuist (relating to the determination of right and wrong in questions of conduct or conscience), difference (comparing or contrasting two or more phenomena), descriptive (aims to describe a situation or state), or relational (investigating characteristics that are related in some way).
Here are some theoretical research problem examples :
- Ethical frameworks that can provide coherent justifications for artificial intelligence and machine learning algorithms, especially in contexts involving autonomous decision-making and moral agency.
- Determining how mathematical models can elucidate the gradual development of complex traits, such as intricate anatomical structures or elaborate behaviors, through successive generations.
- Applied research problems
Applied or practical research problems focus on addressing real-world challenges and generating practical solutions to improve various aspects of society, technology, health, and the environment.
Here are some applied research problem examples :
- Studying the use of precision agriculture techniques to optimize crop yield and minimize resource waste.
- Designing a more energy-efficient and sustainable transportation system for a city to reduce carbon emissions.
- Action research problems
Action research problems aim to create positive change within specific contexts by involving stakeholders, implementing interventions, and evaluating outcomes in a collaborative manner.
Here are some action research problem examples :
- Partnering with healthcare professionals to identify barriers to patient adherence to medication regimens and devising interventions to address them.
- Collaborating with a nonprofit organization to evaluate the effectiveness of their programs aimed at providing job training for underserved populations.
These different types of research problems may give you some ideas when you plan on developing your own.
How to Define a Research Problem
You might now ask “ How to define a research problem ?” These are the general steps to follow:
- Look for a broad problem area: Identify under-explored aspects or areas of concern, or a controversy in your topic of interest. Evaluate the significance of addressing the problem in terms of its potential contribution to the field, practical applications, or theoretical insights.
- Learn more about the problem: Read the literature, starting from historical aspects to the current status and latest updates. Rely on reputable evidence and data. Be sure to consult researchers who work in the relevant field, mentors, and peers. Do not ignore the gray literature on the subject.
- Identify the relevant variables and how they are related: Consider which variables are most important to the study and will help answer the research question. Once this is done, you will need to determine the relationships between these variables and how these relationships affect the research problem .
- Think of practical aspects : Deliberate on ways that your study can be practical and feasible in terms of time and resources. Discuss practical aspects with researchers in the field and be open to revising the problem based on feedback. Refine the scope of the research problem to make it manageable and specific; consider the resources available, time constraints, and feasibility.
- Formulate the problem statement: Craft a concise problem statement that outlines the specific issue, its relevance, and why it needs further investigation.
- Stick to plans, but be flexible: When defining the problem , plan ahead but adhere to your budget and timeline. At the same time, consider all possibilities and ensure that the problem and question can be modified if needed.
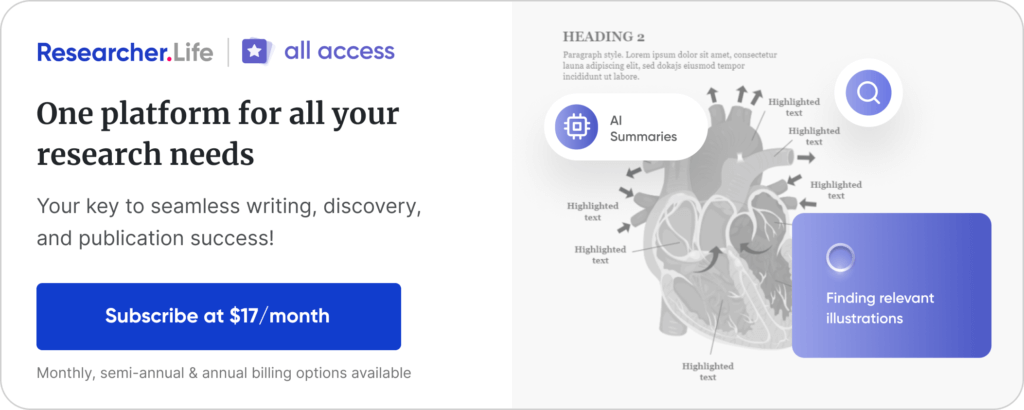
Key Takeaways
- A research problem concerns an area of interest, a situation necessitating improvement, an obstacle requiring eradication, or a challenge in theory or practical applications.
- The importance of research problem is that it guides the research and helps advance human understanding and the development of practical solutions.
- Research problem definition begins with identifying a broad problem area, followed by learning more about the problem, identifying the variables and how they are related, considering practical aspects, and finally developing the problem statement.
- Different types of research problems include theoretical, applied, and action research problems , and these depend on the discipline and nature of the study.
- An ideal problem is original, important, feasible, specific, and based on evidence.
Frequently Asked Questions
Why is it important to define a research problem?
Identifying potential issues and gaps as research problems is important for choosing a relevant topic and for determining a well-defined course of one’s research. Pinpointing a problem and formulating research questions can help researchers build their critical thinking, curiosity, and problem-solving abilities.
How do I identify a research problem?
Identifying a research problem involves recognizing gaps in existing knowledge, exploring areas of uncertainty, and assessing the significance of addressing these gaps within a specific field of study. This process often involves thorough literature review, discussions with experts, and considering practical implications.
Can a research problem change during the research process?
Yes, a research problem can change during the research process. During the course of an investigation a researcher might discover new perspectives, complexities, or insights that prompt a reevaluation of the initial problem. The scope of the problem, unforeseen or unexpected issues, or other limitations might prompt some tweaks. You should be able to adjust the problem to ensure that the study remains relevant and aligned with the evolving understanding of the subject matter.
How does a research problem relate to research questions or hypotheses?
A research problem sets the stage for the study. Next, research questions refine the direction of investigation by breaking down the broader research problem into manageable components. Research questions are formulated based on the problem , guiding the investigation’s scope and objectives. The hypothesis provides a testable statement to validate or refute within the research process. All three elements are interconnected and work together to guide the research.
R Discovery is a literature search and research reading platform that accelerates your research discovery journey by keeping you updated on the latest, most relevant scholarly content. With 250M+ research articles sourced from trusted aggregators like CrossRef, Unpaywall, PubMed, PubMed Central, Open Alex and top publishing houses like Springer Nature, JAMA, IOP, Taylor & Francis, NEJM, BMJ, Karger, SAGE, Emerald Publishing and more, R Discovery puts a world of research at your fingertips.
Try R Discovery Prime FREE for 1 week or upgrade at just US$72 a year to access premium features that let you listen to research on the go, read in your language, collaborate with peers, auto sync with reference managers, and much more. Choose a simpler, smarter way to find and read research – Download the app and start your free 7-day trial today !
Related Posts

Simple Random Sampling: Definition, Methods, and Examples

What is a Case Study in Research? Definition, Methods, and Examples
- Thesis Action Plan New
- Academic Project Planner
Literature Navigator
Thesis dialogue blueprint, writing wizard's template, research proposal compass.
- Why students love us
- Why professors love us
- Rebels Blog (Free)
- Why we are different
- All Products
- Coming Soon
Identifying a Research Problem: A Step-by-Step Guide

Identifying a research problem is a crucial first step in the research process, serving as the foundation for all subsequent research activities. This guide provides a comprehensive overview of the steps involved in identifying a research problem, from understanding its essence to employing advanced strategies for refinement.
Key Takeaways
- Understanding the definition and importance of a research problem is essential for academic success.
- Exploring diverse sources such as literature reviews and consultations can help in formulating a solid research problem.
- A clear problem statement, aligned research objectives, and well-defined questions are crucial for a focused study.
- Evaluating the feasibility and potential impact of a research problem ensures its relevance and scope.
- Advanced strategies, including interdisciplinary approaches and technology utilization, can enhance the identification and refinement of research problems.
Understanding the Essence of Identifying a Research Problem
Defining the research problem.
A research problem is the focal point of any academic inquiry. It is a concise and well-defined statement that outlines the specific issue or question that the research aims to address. This research problem usually sets the tone for the entire study and provides you, the researcher, with a clear purpose and a clear direction on how to go about conducting your research.
Importance in Academic Research
It also demonstrates the significance of your research and its potential to contribute new knowledge to the existing body of literature in the world. A compelling research problem not only captivates the attention of your peers but also lays the foundation for impactful and meaningful research outcomes.
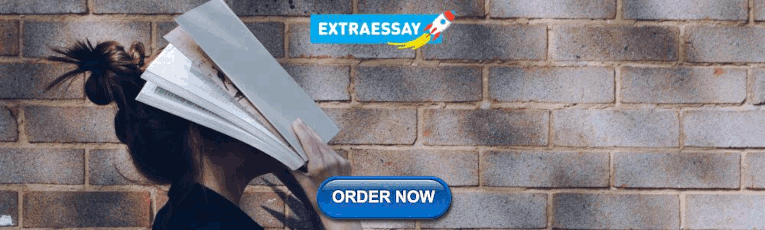
Initial Steps to Identification
To identify a research problem, you need a systematic approach and a deep understanding of the subject area. Below are some steps to guide you in this process:
- Conduct a thorough literature review to understand what has been studied before.
- Identify gaps in the existing research that could form the basis of your study.
- Consult with academic mentors to refine your ideas and approach.
Exploring Sources for Research Problem Identification
Literature review.
When you embark on the journey of identifying a research problem, a thorough literature review is indispensable. This process involves scrutinizing existing research to find literature gaps and unexplored areas that could form the basis of your research. It's crucial to analyze recent studies, seminal works, and review articles to ensure a comprehensive understanding of the topic.
Existing Theories and Frameworks
The exploration of existing theories and frameworks provides a solid foundation for developing a research problem. By understanding the established models and theories, you can identify inconsistencies or areas lacking in depth which might offer fruitful avenues for research.
Consultation with Academic Mentors
Engaging with academic mentors is vital in shaping a well-defined research problem. Their expertise can guide you through the complexities of your field, offering insights into feasible research questions and helping you refine your focus. This interaction often leads to the identification of unique and significant research opportunities that align with current academic and industry trends.
Formulating the Research Problem
Crafting a clear problem statement.
To effectively address your research problem, start by crafting a clear problem statement . This involves succinctly describing who is affected by the problem, why it is important, and how your research will contribute to solving it. Ensure your problem statement is concise and specific to guide the entire research process.
Setting Research Objectives
Setting clear research objectives is crucial for maintaining focus throughout your study. These objectives should directly align with the problem statement and guide your research activities. Consider using a bulleted list to outline your main objectives:
- Understand the underlying factors contributing to the problem
- Explore potential solutions
- Evaluate the effectiveness of proposed solutions
Determining Research Questions
The formulation of precise research questions is a pivotal step in defining the scope and direction of your study. These questions should be directly derived from your research objectives and designed to be answerable through your chosen research methods. Crafting well-defined research questions will help you maintain a clear focus and avoid common pitfalls in the research process.
Evaluating the Scope and Relevance of the Research Problem
Feasibility assessment.
Before you finalize a research problem, it is crucial to assess its feasibility. Consider the availability of resources, time, and expertise required to conduct the research. Evaluate potential constraints and determine if the research problem can be realistically tackled within the given limitations.
Significance to the Field
Ensure that your research problem has a clear and direct impact on the field. It should aim to contribute to existing knowledge and address a real-world issue that is relevant to your academic discipline.
Potential Impact on Existing Knowledge
The potential impact of your research problem on existing knowledge cannot be understated. It should challenge, extend, or refine current understanding in a meaningful way. Consider how your research can add value to the existing body of work and potentially lead to significant advancements in your field.
Techniques for Refining the Research Problem
Narrowing down the focus.
To effectively refine your research problem, start by narrowing down the focus . This involves pinpointing the specific aspects of your topic that are most significant and ensuring that your research problem is not too broad. This targeted approach helps in identifying knowledge gaps and formulating more precise research questions.
Incorporating Feedback
Feedback is crucial in the refinement process. Engage with academic mentors, peers, and experts in your field to gather insights and suggestions. This collaborative feedback can lead to significant improvements in your research problem, making it more robust and relevant.
Iterative Refinement Process
Refinement should be seen as an iterative process, where you continuously refine and revise your research problem based on new information and feedback. This approach ensures that your research problem remains aligned with current trends and academic standards, ultimately enhancing its feasibility and relevance.
Challenges in Identifying a Research Problem
Common pitfalls and how to avoid them.
Identifying a research problem can be fraught with common pitfalls such as selecting a topic that is too broad or too narrow. To avoid these, you should conduct a thorough literature review and seek feedback from peers and mentors. This proactive approach ensures that your research question is both relevant and manageable.
Dealing with Ambiguity
Ambiguity in defining the research problem can lead to significant challenges down the line. Ensure clarity by operationalizing variables and explicitly stating the research objectives. This clarity will guide your entire research process, making it more structured and focused.
Balancing Novelty and Practicality
While it's important to address a novel issue in your research, practicality should not be overlooked. A research problem should not only contribute new knowledge but also be feasible and have clear implications. Balancing these aspects often requires iterative refinement and consultation with academic mentors to align your research with real-world applications.
Advanced Strategies for Identifying a Research Problem
Interdisciplinary approaches.
Embrace the power of interdisciplinary approaches to uncover unique and comprehensive research problems. By integrating knowledge from various disciplines, you can address complex issues that single-field studies might overlook. This method not only broadens the scope of your research but also enhances its applicability and depth.
Utilizing Technology and Data Analytics
Leverage technology and data analytics to refine and identify research problems with precision. Advanced tools like machine learning and big data analysis can reveal patterns and insights that traditional methods might miss. This approach is particularly useful in fields where large datasets are involved, or where real-time data integration can lead to more dynamic research outcomes.
Engaging with Industry and Community Needs
Focus on the needs of industry and community to ensure your research is not only academically sound but also practically relevant. Engaging with real-world problems can provide a rich source of research questions that are directly applicable and beneficial to society. This strategy not only enhances the relevance of your research but also increases its potential for impact.
Dive into the world of academic success with our 'Advanced Strategies for Identifying a Research Problem' at Research Rebels. Our expertly crafted guides and action plans are designed to simplify your thesis journey, transforming complex academic challenges into manageable tasks. Don't wait to take control of your academic future. Visit our website now to learn more and claim your special offer!
In conclusion, identifying a research problem is a foundational step in the academic research process that requires careful consideration and systematic approach. This guide has outlined the essential steps involved, from understanding the context and reviewing existing literature to formulating clear research questions. By adhering to these guidelines, researchers can ensure that their studies are grounded in a well-defined problem, enhancing the relevance and impact of their findings. It is crucial for scholars to approach this task with rigor and critical thinking to contribute meaningfully to the body of knowledge in their respective fields.
Frequently Asked Questions
What is a research problem.
A research problem is a specific issue, inconsistency, or gap in knowledge that needs to be addressed through scientific inquiry. It forms the foundation of a research study, guiding the research questions, methodology, and analysis.
Why is identifying a research problem important?
Identifying a research problem is crucial as it determines the direction and scope of the study. It helps researchers focus their inquiry, formulate hypotheses, and contribute to the existing body of knowledge.
How do I identify a suitable research problem?
To identify a suitable research problem, start with a thorough literature review to understand existing research and identify gaps. Consult with academic mentors, and consider relevance, feasibility, and your own interests.
What are some common pitfalls in identifying a research problem?
Common pitfalls include choosing a problem that is too broad or too narrow, not aligning with existing literature, lack of originality, and failing to consider the practical implications and feasibility of the study.
Can technology help in identifying a research problem?
Yes, technology and data analytics can aid in identifying research problems by providing access to a vast amount of data, revealing patterns and trends that might not be visible otherwise. Tools like digital libraries and research databases are particularly useful.
How can I refine my research problem?
Refine your research problem by narrowing its focus, seeking feedback from peers and mentors, and continually reviewing and adjusting the problem statement based on new information and insights gained during preliminary research.

AI for Academia: Revolutionizing Research and Learning
How do you write a hypothesis step-by-step instructions, how to write a bachelor thesis: tips and tricks, how to write the best dissertation: a comprehensive guide, help with your master thesis: essential resources and advice.

Demystifying Research: Understanding the Difference Between a Problem and a Hypothesis

Avoiding Procrastination Pitfalls: Bachelor Thesis Progress and Weekend Celebrations

How Do You Write a Hypothesis for a Research Paper? Step-by-Step Guide

How to Write a Thesis Fast: Tips and Strategies for Success

The Note-Taking Debate: Pros and Cons of Digital and Analog Methods

Thesis Action Plan

- Rebels Blog
- Blog Articles
- Terms and Conditions
- Payment and Shipping Terms
- Privacy Policy
- Return Policy
© 2024 Research Rebels, All rights reserved.
Your cart is currently empty.
Identifying the Research Problem
- First Online: 13 April 2022
Cite this chapter
- Yanmei Li 3 &
- Sumei Zhang 4
949 Accesses
The first step of conducting research is identifying a researchable problem. This chapter explains the procedures to identify the research problem. The procedure of research involves systematically investigating a subject matter to reveal facts or reach new conclusions. Research can be theory driven or problem driven. Most of the studies in urban and regional planning attempt to investigate issues or problems either to: (1) identity and define the problem, and/or (2) probe further to answer research questions after identifying, defining, and operationalizing the problem. This chapter introduces methods, such as factual data mining, back of envelope analysis, quick research, and issues unique to a sub-discipline of planning, to identify the research problem.
This is a preview of subscription content, log in via an institution to check access.
Access this chapter
Subscribe and save.
- Get 10 units per month
- Download Article/Chapter or Ebook
- 1 Unit = 1 Article or 1 Chapter
- Cancel anytime
- Available as PDF
- Read on any device
- Instant download
- Own it forever
- Available as EPUB and PDF
- Compact, lightweight edition
- Dispatched in 3 to 5 business days
- Free shipping worldwide - see info
- Durable hardcover edition
Tax calculation will be finalised at checkout
Purchases are for personal use only
Institutional subscriptions
APA (American Planning Association). (2020). AICP Certification Exam Outline. Retrieved on 22 Sept 2020 from https://planning.org/certification/examprep/subjectmatter/
Patton, C. V., Sawicki, D. S., & Clark, J. J. (2013). Basic methods of policy analysis and planning (3rd ed.). Pearson. New York City.
Google Scholar
Sharifi, A., & Khavarian-Garmsir, A. Z. (2020). The COVID-19 pandemic: Impacts on cities and major lessons for urban planning, design, and management. Science of the Total Environment, 749 (2020).
Web Resources
American Planning Association: https://www.planning.org/
Cyburbia, Urban Planning Community: https://www.cyburbia.org/
Planetizen: Planning News, Jobs, and Education: https://www.planetizen.com/
Bloomberg City Lab: https://www.bloomberg.com/citylab
Download references
Author information
Authors and affiliations.
Florida Atlantic University, Boca Raton, FL, USA
University of Louisville, Louisville, KY, USA
Sumei Zhang
You can also search for this author in PubMed Google Scholar
Electronic Supplementary Material
(docx 14 kb), rights and permissions.
Reprints and permissions
Copyright information
© 2022 Springer Nature Switzerland AG
About this chapter
Li, Y., Zhang, S. (2022). Identifying the Research Problem. In: Applied Research Methods in Urban and Regional Planning. Springer, Cham. https://doi.org/10.1007/978-3-030-93574-0_2
Download citation
DOI : https://doi.org/10.1007/978-3-030-93574-0_2
Published : 13 April 2022
Publisher Name : Springer, Cham
Print ISBN : 978-3-030-93573-3
Online ISBN : 978-3-030-93574-0
eBook Packages : Mathematics and Statistics Mathematics and Statistics (R0)
Share this chapter
Anyone you share the following link with will be able to read this content:
Sorry, a shareable link is not currently available for this article.
Provided by the Springer Nature SharedIt content-sharing initiative
- Publish with us
Policies and ethics
- Find a journal
- Track your research
Steps for Inquiry and Problem-Solving
Although problem-solving and inquiry are technically different, they require many of the same skills. At a minimum, students need to recognize the language that they will need and the processes involved. Critical thinking skills are also crucial for successful inquiry projects. Teachers can model some critical thinking skills, such as
- analyzing arguments, claims, or evidence
- making inferences using inductive or deductive reasoning
- judging or evaluating
- making decisions or solving problems
- asking and answering questions for clarification
- defining terms
- identifying assumptions
- interpreting and explaining
- reasoning verbally
- seeing both sides of an issue (Lai, 2011, pp. 9-10)
Critical thinking skills do not necessarily come naturally with second language learning, and they are culturally situated, so students need to learn and practice them before, during, and after each step in the process. According to Molnar, Boninger, and Fogarty (2011), an environment that “encourages students to ask questions, to think about their thought processes, and thus to develop habits of mind that enable them to transfer the critical thinking skills they learn in class to other, unrelated, situations” is where critical thinking is cultivated (p. i).
Although sources describe the steps in the inquiry process differently, most sources include the same five basic steps of establishing orientation , conceptualization , investigation , conclusion , and discussion (Pedaste, et al., 2015). These steps are addressed to the learner and include:
- What are you interested in? Ask a question that has meaning, define the problem, and figure out what you need to do to answer it.
- Investigate by researching. Plan, gather resources and information, and record what you have found.
- Create new ideas, thoughts, and directions for action. Make sense of the information you have gathered by summarizing, synthesizing, and interpreting.
- Discuss with others. Interaction can shed new light on the question, the investigation, and the process. Share what you have learned and then use the feedback to return to the process.
- Reflect on the inquiry process. Did the process lead to unexpected conclusions? Is there something else that needs researching? Has the problem been solved?
For younger or less proficient learners, Freeman and Freeman (1998) present six steps that follow these same basic guidelines. They call this the “Wonderfilled Way of Learning,” and the steps are addressed to the teacher:
- Ask the students: What do we know about ______ ?
- Ask the students: What do we wonder about _____ ?
- Ask the students: How can we find out about _____ ?
- With the students, work out a plan of action, and, at the same time, work school district curriculum requirements into the unit.
- Plan an event to celebrate what you have learned together.
- Learning is continuous. From any unit, more topics and questions come up. Begin the cycle again. (pp. 138–139)
Regardless of which set of guidelines you and your students follow, inquiry projects can be used to support language and content learning.
In the chapter’s opening scenario, Ms. Petrie guides the students through learning experiences. She has planned that throughout the project, they will not only learn techniques for inquiry such as planning, brainstorming, reflecting, and evaluating, but through their interactions, they will also acquire a variety of language content and structures. These activities facilitate many of the CALL principles—for example, students have many opportunities for language input and output, they have many choices (structure/autonomy), they are motivated to learn because they are answering meaningful questions (authenticity and connection), and they interact with peers and community members (social interaction and feedback).

Share This Book
Feedback/errata.
Comments are closed.
- Increase Font Size
Problem-Solving in Business: SKILLS AND QUALIFICATIONS NEEDED IN PROBLEM-SOLVING
- ABOUT THIS LIBGUIDE
- PROBLEM-SOLVING DEFINED AND WHY IT IS IMPORTANT
- SKILLS AND QUALIFICATIONS NEEDED IN PROBLEM-SOLVING
- PROBLEM-SOLVING STEPS
- CASE STUDIES
- MORE HELPFUL RESOURCES

- RESEARCH SKILLS
- EMOTIONAL INTELLIGENCE
- CRITICAL THINKING
- RISK-TAKING
are our ability to find an answer to a question to a solution to a problem. Research skills include the ability to gather information about your topic, review that information, and analyze and interpret the information in a manner that brings us to a solution. SOURCE:
| |
1. Start broad then dive into the specifics 2. Learn how to recognize a quality source 3. Verify information from several sources 4. Be open to surprising answers | 5. Stay organized 6. Take advantage of library resources
SOURCE: |
ISBN: 9780226239736 Publication Date: 2016-10-18 With more than three-quarters of a million copies sold since its first publication, The Craft of Research has helped generations of researchers at every level--from first-year undergraduates to advanced graduate students to research reporters in business and government--learn how to conduct effective and meaningful research.
ISBN: 1137453656 Publication Date: 2015-10-19 This timely book inspires researchers to deploy relevant, effective, innovative digital methods. It explores the relationship of such methods to 'mainstream' social science; interdisciplinarity; innovations in digital research tools; the opportunities (and challenges) of digital methods in researching social life; and digital research ethics. |
ISBN: 9781446295410 Publication Date: 2016-01-05 Qualitative researchers can now connect with participants online to collect deep, rich data and generate new understandings of contemporary research phenomena. Doing Qualitative Research Online gives students and researchers the practical and scholarly foundations needed to gain digital research literacies essential for designing and conducting studies based on qualitative data collected online.
ISBN: 0262042878 Publication Date: 2019-08-01 In The Joy of Search, Daniel Russell shows us how to be great online researchers. We don't have to be computer geeks or a scholar searching out obscure facts; we just need to know some basic methods. Russell explains how to frame search queries so they will yield information and describes the best ways to use such resources as Google Earth, Google Scholar, Wikipedia, and Wikimedia. He shows when to put search terms in double quotes, how to use the operator (*), why metadata is important, and how to triangulate information from multiple sources. |
or EI is the ability to understand and manage your own emotions, and those of the people around you. People with a high degree of emotional intelligence know what they're feeling, what their emotions mean, and how these emotions can affect other people. For leaders, having emotional intelligence is essential for success. After all, who is more likely to succeed – a leader who shouts at his team when he's under stress, or a leader who stays in control, and calmly assesses the situation? SOURCE:
involves being sensitive to and perceptive of other people's emotions, and having the ability to intuitively facilitate improved performance based on this knowledge. The modern workplace is characterized by open communication, teamwork, and a mutual respect among employees and their supervisors. Possessing emotional intelligence allows managers to better understand and people they supervise. SOURCE:
| |
1. It’s knowing what your actions and reactions are going to be and how they are going to affect other people, especially when making decisions. People who can give themselves an honest self assessment are going to be better prepared to handle whatever is thrown at them. They understand not only why they make a decision, but the fallout it may have for other people. 2. The next component is self regulation which is the ability to control disruptive impulses, especially in high-stress situations. Controlling those impulsive, emotional reactions is what allows professionals to formulate a constructive and appropriate response. Think of it as the bumpers on a bowling lane. They help the ball stay on track. 3. The third key aspect of emotional intelligence is motivation. Someone who is motivated is committed to the work itself. He/she is driven to achieve, and committed to the organization. A motivated employee is going to stand out. | 4. Empathy is the fourth component and is incredibly important when thinking about emotional intelligence. It’s a driving force in every conversation and interaction, and it can make or break a relationship. Whether it’s a prospect, client, colleague, or manager, empathy is the ability to understand what someone is going through. It’s what allows the other person to feel understood, which is incredibly important when building any type of relationship. 5. The fifth and final component is social skill, which is the culmination of all of the above. It’s taking the other components and organizing them so they can be put into practice.
SOURCE: |
ISBN: 9780190698959 Publication Date: Cary Cherniss and Cornelia W. Roche [2020] "This book describes how 25 outstanding leaders used emotional intelligence to deal with critical challenges and opportunities.. The book distills the leaders' experiences into nine strategies that can help any leader or potential leader to be more effective. Each chapter concludes with activities that help readers to apply immediately each of those strategies."
ISBN: 1633697339 Publication Date: 2019-08-06 Become a Better Leader by Improving Your Emotional Intelligence Bestselling author DANIEL GOLEMAN first brought the concept of emotional intelligence (EI) to the forefront of business through his articles in Harvard Business Review, establishing EI as an indispensable trait for leaders. The Emotionally Intelligent Leader brings together three of Goleman's bestselling HBR articles. |
ISBN: 9781633696679 Publication Date: 2019-03-26 Become a mindful listener at work. Listening is a critical skill that leaders and managers often take for granted. By learning to listen mindfully, you can keep your employees more engaged, foster the discovery of new ideas, and hear what you need to hear in a discussion rather than what you expect to hear.
ISBN: 9781599186351 Publication Date: 2018-10-30 Improve Your EQ Success as an entrepreneur takes a lot more than big ideas and dedication. With threats of burnout and rejection at nearly every turn, the entrepreneurial journey is riddled with obstacles--but the staff, experts, and voices of Entrepreneur want you to know you're not alone. |
is the act of turning new and imaginative ideas into reality. Creativity is characterized by the ability to perceive the world in new ways, to find hidden patterns, to make connections between seemingly unrelated phenomena, and to generate solutions. Creativity involves two processes: thinking, then producing. SOURCE:
in business is a way of thinking that inspires, challenges, and helps people to find innovative solutions and create opportunities out of problems. It’s the reason some companies wow us with new, amazing ideas, whilst others merely follow the beaten path. It’s the source of innovation and inspiration. SOURCE: | |
Creativity is characterized by novelty, and there are two broad processes that produce effective novelty: . Divergent thinking can be described as generating ideas. Convergent thinking occurs when people evaluate their ideas to reach appropriate conclusions. In this sense, convergent thinking judges the ideas that arise during divergent thinking to determine their suitability.
What’s happening in the brain. Before we jump into our tips for improving divergent thinking to maximize your creative potential, let’s take a look at what’s happening in the brain during |
That is, think about what you want to achieve, not what you want to avoid.
One of the easiest ways to help your brain defocus and become more associative in order to bolster your divergent thinking skills is to simply take a break from what you’re doing.
In general, the best way to get better at something is to practice. There are a wide variety of methods people can use to practice divergent thinking, such as brainstorming, free writing, and playing games.
SOURCE: |
ISBN: 9781523088256 Publication Date: 2020-06-23 Creativity is the engine for innovation, but too many people associate creativity solely with the arts, even though to be an incredible scientist, engineer, or entrepreneur requires immense creativity. The process of creativity combines wonder (inspiration, awe, audacity, and asking a lot of what-if questions) with rigor (discipline, practice, skill-building, and attention to detail).
ISBN: 9780262358392 Publication Date: 2020-02-25 The definitive book on leadership in the digital era: why digital technologies call for leadership that emphasizes creativity, collaboration, and inclusivity. Certain ideas about business leadership are held to be timeless, and certain characteristics of leaders--often including a square jaw, a deep voice, and extroversion--are said to be universal.
Publication Date: New York : Oxford University Press, 2020. Trending skills include analytical thinking and innovation, active learning strategies, creativity, reasoning, and complex problem solving. |
ISBN: 9781541674042 Publication Date: 2019-09-03 More than three decades after its original publication, Conceptual Blockbusting has never been more relevant, powerful, or fresh. Integrating insights from the worlds of psychology, engineering, management, art, and philosophy, Adams identifies the key blocks (perceptual, emotional, cultural, environmental, intellectual, and expressive) that prevent us from realizing the full potential of our fertile minds. Employing unconventional exercises and other interactive elements, Adams shows individuals, teams, and organizations how to overcome these blocks, embrace alternative ways of thinking about complex problems, and celebrate the joy of creativity.
ISBN: 9781616893941 Publication Date: 2016-12-06 Long known as the go-to management consultant of the design world, Keith Granet reveals more of his clear-eyed insights about running a creative business in this follow-up to his book The Business of Design. While aimed at creative enterprises, Granet's advice, quickly summarized as "know what you do best and focus on that," applies to any organization, small or large, commercial or nonprofit.
ISBN: 0071850112 Publication Date: 2015-07-31 Successful strategies must have one key element to produce spectacular results for your business-- originality. However, pure ingenuity can be hard to produce in the noise of derivative ideas and worn out processes drowning out fresh progress--which is why you need Creative Strategy Generation to help guide you through tried-and-true business practices in a fresh, new, and exciting way. |
In a nutshell, is the ability to think reasonably, detaching yourself from personal bias, emotional responses, and subjective opinions. It involves using the data at hand to make a reasoned choice without falling prey to the temptations of doing things simply because they’ve always been done a certain way. SOURCE: | |
We live in an age if infinite information. Everyone in a business environment should scan their business ecosystem through the information gathering from reliable and relevant sources of information. This includes general news, business news, industry news, and digital sources such as groups, and experts.
“Winging it” is simply not acceptable. Critical thinking and problem solving starts with aggressive preparation which means thinking about thinking and making sure possibilities are covered and all sources of information have been used appropriately to prepare for a day, week, month, year, and all activities.
Aggressive preparation is the foundation for executing plans through proactive planning and to-do lists that are realistic. By proactively planning and being in control of information and situations, the focus can be on the tasks at hand which should be the things necessary to .
Showing up just when the day is starting or when meetings are starting isn’t proactive and doesn’t support critical thinking and problem solving; it probably makes things worse. Being 15 minutes early allows for the ability to see things that others don’t see (or hear) and that information can be used as part of the journey.
First of all, put the smartphone AWAY! Put it in your pocket. Unless you are in a critical position like sales or customer service where the execution of your strategy relies on your immediate reaction, put it AWAY. Be present and focus. Nobody can think critically if half their attention is on a leaderboard on buzzfeed. It’s ridiculous. After you put your phone away, then listen and learn. Each experience, each new piece of knowledge builds the ability to think more critically and solve problems of the business. |
People think critically and solve problems are able to clearly share their insights without talking too much, over-selling, or blathering on about non-sense. By clearly sharing insights, you are then able to listen to reactions and fine-tune your approaches.
Even the best critical thinkers don’t know everything; but they do have a process for figuring things out. Acknowledge when you don’t know something and proactively seek the right information through the right resources.
Nobody likes a smartass-know-it-all. The best critical thinkers are respectful and build resources though humility and gratitude. They build a portfolio of knowledge and accumulate approaches and methods of problem solving that rely on other people. The best critical thinkers understand a little sugar goes a lot further than a lot of vinegar.
One of the most important aspects of good, strong critical thinking and problem solving is doing it right and not cutting any corners. Cutting corners will inevitably give you the wrong solution and a poor business result.
And finally, the most important rule; take immense pride and ownership in your work. By seeking to be the best at what you do and seeking to be the best for your company, you will easily find the right paths along the journey. Pride is the most important ingredient in critical thinking and problem solving because it implicitly drives you to figure things out in ways that you didn’t think were previously possible. SOURCE: |
ISBN: 9781984878106 Publication Date: 2021-02-02 The bestselling author of Give and Take and Originals examines the critical art of rethinking: learning to question your opinions and open other people's minds, which can position you for excellence at work and wisdom in life Intelligence is usually seen as the ability to think and learn, but in a rapidly changing world, there's another set of cognitive skills that might matter more: the ability to rethink and unlearn. Organizational psychologist Adam Grant is an expert on opening other people's minds--and our own.
ISBN: 1633885984 Publication Date: 2019-09-27 Using a host of real-world examples, the authors show you how to win an argument, defend a case, recognize a fallacy, see through deception, persuade a skeptic, and turn defeat into victory. Whether you're evaluating a social media rumor or you just want to become more adept at making your points and analyzing others' arguments, The Art of Deception--now with an updated preface--will give you the intellectual tools to become a more effective thinker and speaker. Helpful exercises and discussion questions are also included. |
ISBN: 1633699218 Publication Date: 2020-06-16 We've outsourced too much of our thinking. Too often, we've stopped thinking for ourselves. What we need is a new approach for integrating these information sources more effectively, harnessing the value they provide without undermining our ability to think for ourselves.
ISBN: 1626568766 Publication Date: 2020-05-12 Smart machines will take over millions of jobs in manufacturing, office work, the service sector, the professions, you name it. We need to excel at critical, creative, and innovative thinking and at genuinely engaging with others--things machines can't do well. The crucial mindset underlying NewSmart is humility--not self-effacement but an accurate self-appraisal: acknowledging you can't have all the answers, remaining open to new ideas, and committing yourself to lifelong learning. Drawing on extensive multidisciplinary research, |
The best definition of in business involves a group of individuals working together to complete a task or a large goal. A leader's role in developing and managing the team is critical to team success. SOURCE: | |
1. Great ideas don't come from lone geniuses 2. Diverse perspectives can help you come up with winning innovations 3. Team work can make you happier 4. When you work in a team, you grow as an individual 5. Sharing the workload eases burnout | 6. Dividing the work lets you grow your skills 7. Recognition from other team members can improve your productivity 8. Working in a team helps you take risks that pay off 9. When you work in a team, you'll feel less stressed 10. Good communication boosts your creativity
SOURCE: |
ISBN: 1401958141 Publication Date: Carlsbad, California : Hay House, Inc., [2020] In this book, Mike Robbins dives deep into the ways great teams build trust, collaborate, and perform at the highest level. The book's core principles include fostering an environment of psychological safety, honoring diversity and inclusion, addressing and navigating conflict, building trust, and a maintain a balance of healthy expectations and empathy.
ISBN: 9781119550112 Publication Date: 2020-06-10 Proven methods to push your organization to its maximum potential with responsible leadership Accountable Leaders is the real-world guide to propelling your business to extraordinary levels of performance and achievement. This invaluable guide will provide the tools and knowledge to take you and your organization to incredible levels of performance and achievement. |
ISBN: 9781119551409 Publication Date: 2019-09-24 The book helps the reader assess how his/her team is performing on each of the 5Cs--context, composition, competencies, change, and collaborative leadership, and discusses options concerning how to improve team performance along each of these dimensions. This book provides the next generation of team leaders, team members, and team consultants with the knowledge and skills they need to create effective and high functioning teams.
ISBN: 9781250231581 Publication Date: 2020-03-03 What does it take to command a team of elite individuals? It requires a commitment to seven key principles: Courage, Trust, Respect, Growth, Excellence, Resiliency, and Alignment. All of these are present in an elite team which commits to them deeply in order to forge the character worthy of uncommon success. |
...Any new venture, whether business or personal, entails unknowns. And unknowns mean there’s risk. Entrepreneurs embrace this risk, not risk in general, but a special kind of risk. Researcher Sally Caird calls it “calculated risk taking” and describes it this way: “Calculated is operationally defined as the ability to deal with incomplete information and act on a risky option, that requires skill, to actualize challenging but realistic goals.” SOURCE: | |
1. Risk urges you to already existing ones 2. Fear of failure gets obliterated once you embrace a risk-taking culture 3. It inspires 4. In “fight or flight” situations you can really | 5. You 6. The team learns to when nothing else is left to do 7. It will give your employees a sense of ownership, a sense of exploration, the motivation to take it one step further, to SOURCE: |
ISBN: 9781401961800 Publication Date: 2021-03-16 Every entrepreneur is in search of their next move-that next idea with a sharp edge or fresh angle that inspires and ignites. But how do you know when to trust your gut and when to cut your losses and move on? Victoria uses principles drawn from the performing arts to prove that uncertainty is not the enemy, but a crucial step on the path, and to show that even when your plans and goals aren't clear, you can follow your curiosity, interests, and ideas forward.
|
ISBN: 9781426214721 Publication Date: 2016-03-01 Are risk-takers born or made? Why are some more willing to go out on a limb (so to speak) than others? How do we weigh the value of opportunities large or small that may have the potential to change the course of our lives? These are just a few of the questions that author Kayt Sukel tackles, applying the latest research in neuroscience and psychology to compelling real-world situations. |
| |
_________________
-- Provides full-text access to scholarly journals and articles from top-quality sources. Enter your keyword in the search box (such as "creativity and problem solving"). Results can be filtered by the categories on the right side of the page.
-- Provides full-text print, as well as audio and video materials from books, magazines, newspapers, journals, and non-print sources. Enter your keywords or phrases in the search box (such as "problem solving and teamwork"). Results can be filtered a variety of ways.
-- Provides full-text print, as well as audio and video materials from books, magazines, newspapers, journals, and non-print sources. Enter your keywords or phrases in the search box (such as "problem solving and emotional intelligence"). Results can be filtered a variety of ways.
-- Interesting collection of articles from books, journals, magazines, and videos. Enter your keywords and phrases in the search box (such as "problem solving and creativity").
-- Contains numerous articles on the various aspects of problem solving from an intellectual perspective. Results can be filtered by date, language, geography, and more. | ________________
|
- << Previous: PROBLEM-SOLVING DEFINED AND WHY IT IS IMPORTANT
- Next: PROBLEM-SOLVING STEPS >>
- Last Updated: Mar 23, 2024 4:47 PM
- URL: https://libguides.nypl.org/problem_solving_in_business
- Organizations
- Planning & Activities
- Product & Services
- Structure & Systems
- Career & Education
- Entertainment
- Fashion & Beauty
- Political Institutions
- SmartPhones
- Protocols & Formats
- Communication
- Web Applications
- Household Equipments
- Career and Certifications
- Diet & Fitness
- Mathematics & Statistics
- Processed Foods
- Vegetables & Fruits
Difference Between Inquiry and Investigation
• Categorized under Words | Difference Between Inquiry and Investigation
Inquiry vs Investigation
Man acquires knowledge through observation, inquiry, and investigation into things that interest him. In every aspect of his life, he uses these tools to provide him with information about everything in his world, especially the processes of inquiry and investigation.
Through inquiry and investigation he is able to learn about his past, his body, and about the things in his surroundings including all that has happened in the past and all that is still happening in his environment. They are very important especially in scientific studies to provide researchers information and knowledge about the subject of their research. In law, specifically criminal law as well as in police work, investigation is necessary to allow the uncovering of facts about a case.
“Inquiry” is defined as “the systematic search for information, knowledge and truth about certain things and matters of public interest.” It is the process of solving a problem through researching and probing. It involves questioning and interrogation. It is aimed not only at searching for and acquiring knowledge and information about something, but it is also meant to settle any doubt that individuals may have on the subject.
The word “inquiry” comes from the base word “inquire” which is derived from the Old French word “enquerre” meaning “to ask.” It, in turn, comes from the Latin words “in” (into) and “quaerere” (ask or seek). Its first known use was in the 15th century.
“Investigation,” on the other hand, is defined as the process of inquiring into something thoroughly. It is the systematic examination and scrutiny of the factors that have affected the subject of the investigation in order to uncover facts regarding the matter. It is synonymous with the words; examine, inquire, explore, probe, dig or delve into, and research. It comes from the Latin word “investigatus” which is the past participle of “investigare” meaning “to track or investigate.” Its first known use was in the early 16th century.
“Inquiry” and “investigation” can be used in lieu of each other since they are synonyms and mean the same thing although they have some very subtle differences. An inquiry involves investigation and an investigation involves inquiry.
One difference is how they are handled. While an inquiry is usually done through questioning and probing into the circumstances relating to the matter at hand, an investigation is done more carefully and by thoroughly inspecting details in an organized manner and assessing facts which are uncovered in the process.
1.“Inquiry” is the process of searching for knowledge and information about a problem through asking questions and probing while “investigation” is the organized process of inquiring about a problem through inspection and assessment. 2.Both an inquiry and an investigation may be used interchangeably, but an investigation is a more lengthy process than an inquiry. 3.An investigation involves an inquiry and vice versa; inquiries may be done not as painstakingly thorough as that of an investigation. 4.“Inquiry” comes from the Latin words “in” (into) and “quaerere” (ask or seek) while “investigation” comes from the Latin word “investigatus” (to track).
- Recent Posts
- Difference Between Mocha and Coffee - January 11, 2012
- Difference Between Verb and Predicate - January 2, 2012
- Difference Between Tropical Meteorology and Monsoon Meteorology - January 2, 2012
Sharing is caring!
Read More ESL Articles
Search differencebetween.net :.

- Difference Between Inquiry and Query
- Difference Between Enquiry and Inquiry
- Differences between Enquiry and Investigation
- Difference Between Data and Information
- Difference Between Intelligence and Knowledge
Cite APA 7 M, E. (2016, June 8). Difference Between Inquiry and Investigation. Difference Between Similar Terms and Objects. http://www.differencebetween.net/language/words-language/difference-between-inquiry-and-investigation/. MLA 8 M, Emelda. "Difference Between Inquiry and Investigation." Difference Between Similar Terms and Objects, 8 June, 2016, http://www.differencebetween.net/language/words-language/difference-between-inquiry-and-investigation/.
nice but wish you talked more about investigative inquiries
Leave a Response
Name ( required )
Email ( required )
Please note: comment moderation is enabled and may delay your comment. There is no need to resubmit your comment.
Notify me of followup comments via e-mail
Written by : Emelda M. and updated on 2016, June 8 Articles on DifferenceBetween.net are general information, and are not intended to substitute for professional advice. The information is "AS IS", "WITH ALL FAULTS". User assumes all risk of use, damage, or injury. You agree that we have no liability for any damages.
Advertisments
More in 'words'.
- Difference Between Center and Centre
- Difference Between Lodge and Resort
- Difference Between Authoritarian and Fascism
- Difference Between Advocate and Barrister
- Difference Between Advocacy and Lobbying
Top Difference Betweens
Get new comparisons in your inbox:, most emailed comparisons, editor's picks.
- Difference Between MAC and IP Address
- Difference Between Platinum and White Gold
- Difference Between Civil and Criminal Law
- Difference Between GRE and GMAT
- Difference Between Immigrants and Refugees
- Difference Between DNS and DHCP
- Difference Between Computer Engineering and Computer Science
- Difference Between Men and Women
- Difference Between Book value and Market value
- Difference Between Red and White wine
- Difference Between Depreciation and Amortization
- Difference Between Bank and Credit Union
- Difference Between White Eggs and Brown Eggs
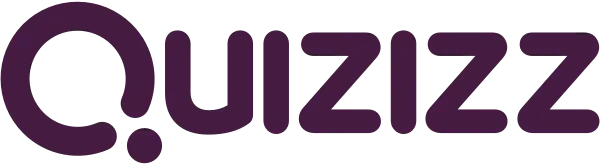
Have an account?

4th QTR_Inquiries, Investigation and Imm...
4th QTR_Inquiries, Investigation and Immersion
31 questions
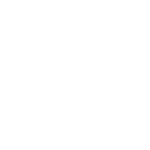
Introducing new Paper mode
No student devices needed. Know more
Immersion is the process of solving a problem through researching and probing.
Inquiry is the systematic examination to uncover facts regarding the matter.
Investigation means that the researches immerse themselves in the culture they are studying.
When we are inquiring we asked questions like what we do when interview someone.
Both inquiry and investigation may be used interchangeably but an investigation is a more lengthy process than an inquiry.
As a senior high school student it is important to us to study Inquiry, Investigation and Immersion because it will help our science skills through research and to have a clear understanding of a specific topic.
One way to understand how to collect data of a study is to describe adequately research design.
The term “ sources” refers to print, electronic or visual materials necessary for your research.
The Scope and Delimitation of the Study is a part of the research where conclusions are expressed as well as recommendation.
Descriptive Analysis. In this type of analysis, the researcher is only after describing the characteristics of the subjects under study.
In the field of Science, Technology, Engineering and Mathematics (STEM), research is helpful. Research helps to obtain significant information about diseases trends and risk factor.
A – If the two statements are correct.
B – If the first statement is correct and second statement is incorrect.
C – If the second statement is correct while the first one is not.
D – If the two statement are both incorrect.
Types of experimental research design are true, quasi- experimental and evaluation experiment. True-experimental does not control both time-related and group-related threats.
It is helpful to supplement a definition, where appropriate, to clarify further the meaning of the term. You are advised to invent a definition for any term which has already a clear and accepted definition.
The term “sources” refers to print, electronic or visual materials necessary for your research. Sources are classified into primary, secondary and tertiary.
A hypothesis is a specific proposition which is presented for testing through research. Hypothesis gives a permanent explanation that accounts for a set of facts.
Validity is traditionally defined as degree to which a test measure what it claims, to be measuring. A valid questionnaire helps to collect reliable and accurate data.
Data collection refers to the problem of a research. Thad at should not be collected, recorded and organized.
Tables present clear and organized data. A table must be clear and simple but complete.
Recommendations are based on fiction of a study. They should not be too broad to lose its relevance to the exact topic of your research study.
Choose the word that does not belong to the group.
d. television
a. introduction
b. conclusion
c. objectives
d. statement of the problem
a. summary of findings
b. conclusions
c. organization
d. recommendations
a. findings
b. sometimes
a. Pie chart
b. Bar chart
c. Circle graph
d. Countless graph
a.Experimental Research Design
b. Descriptive research design
c. Action Research Design
d. Static group comparison design
a.Chicago Style
b. MBA Style format
c. MLA Style
d. American Psychological Association (APA)
. a.Research questions
b. Sample and Population
c. Instrumentation
d. Data Gathering procedure
Explore all questions with a free account
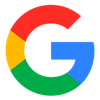
Continue with email
Continue with phone
More From Forbes
Stumped five ways to hone your problem-solving skills.
- Share to Facebook
- Share to Twitter
- Share to Linkedin
Respect the worth of other people's insights
Problems continuously arise in organizational life, making problem-solving an essential skill for leaders. Leaders who are good at tackling conundrums are likely to be more effective at overcoming obstacles and guiding their teams to achieve their goals. So, what’s the secret to better problem-solving skills?
1. Understand the root cause of the problem
“Too often, people fail because they haven’t correctly defined what the problem is,” says David Ross, an international strategist, founder of consultancy Phoenix Strategic Management and author of Confronting the Storm: Regenerating Leadership and Hope in the Age of Uncertainty .
Ross explains that as teams grapple with “wicked” problems – those where there can be several root causes for why a problem exists – there can often be disagreement on the initial assumptions made. As a result, their chances of successfully solving the problem are low.
“Before commencing the process of solving the problem, it is worthwhile identifying who your key stakeholders are and talking to them about the issue,” Ross recommends. “Who could be affected by the issue? What is the problem – and why? How are people affected?”
He argues that if leaders treat people with dignity, respecting the worth of their insights, they are more likely to successfully solve problems.
Best High-Yield Savings Accounts Of 2024
Best 5% interest savings accounts of 2024, 2. unfocus the mind.
“To solve problems, we need to commit to making time to face a problem in its full complexity, which also requires that we take back control of our thinking,” says Chris Griffiths, an expert on creativity and innovative thinking skills, founder and CEO of software provider OpenGenius, and co-author of The Focus Fix: Finding Clarity, Creativity and Resilience in an Overwhelming World .
To do this, it’s necessary to harness the power of the unfocused mind, according to Griffiths. “It might sound oxymoronic, but just like our devices, our brain needs time to recharge,” he says. “ A plethora of research has shown that daydreaming allows us to make creative connections and see abstract solutions that are not obvious when we’re engaged in direct work.”
To make use of the unfocused mind in problem solving, you must begin by getting to know the problem from all angles. “At this stage, don’t worry about actually solving the problem,” says Griffiths. “You’re simply giving your subconscious mind the information it needs to get creative with when you zone out. From here, pick a monotonous or rhythmic activity that will help you to activate the daydreaming state – that might be a walk, some doodling, or even some chores.”
Do this regularly, argues Griffiths, and you’ll soon find that flashes of inspiration and novel solutions naturally present themselves while you’re ostensibly thinking of other things. He says: “By allowing you to access the fullest creative potential of your own brain, daydreaming acts as a skeleton key for a wide range of problems.”
3. Be comfortable making judgment calls
“Admitting to not knowing the future takes courage,” says Professor Stephen Wyatt, founder and lead consultant at consultancy Corporate Rebirth and author of Antidote to the Crisis of Leadership: Opportunity in Complexity . “Leaders are worried our teams won’t respect us and our boards will lose faith in us, but what doesn’t work is drawing up plans and forecasts and holding yourself or others rigidly to them.”
Wyatt advises leaders to heighten their situational awareness – to look broadly, integrate more perspectives and be able to connect the dots. “We need to be comfortable in making judgment calls as the future is unknown,” he says. “There is no data on it. But equally, very few initiatives cannot be adjusted, refined or reviewed while in motion.”
Leaders need to stay vigilant, according to Wyatt, create the capacity of the enterprise to adapt and maintain the support of stakeholders. “The concept of the infallible leader needs to be updated,” he concludes.
4. Be prepared to fail and learn
“Organisations, and arguably society more widely, are obsessed with problems and the notion of problems,” says Steve Hearsum, founder of organizational change consultancy Edge + Stretch and author of No Silver Bullet: Bursting the Bubble of the Organisational Quick Fix .
Hearsum argues that this tendency is complicated by the myth of fixability, namely the idea that all problems, however complex, have a solution. “Our need for certainty, to minimize and dampen the anxiety of ‘not knowing,’ leads us to oversimplify and ignore or filter out anything that challenges the idea that there is a solution,” he says.
Leaders need to shift their mindset to cultivate their comfort with not knowing and couple that with being OK with being wrong, sometimes, notes Hearsum. He adds: “That means developing reflexivity to understand your own beliefs and judgments, and what influences these, asking questions and experimenting.”
5. Unleash the power of empathy
Leaders must be able to communicate problems in order to find solutions to them. But they should avoid bombarding their teams with complex, technical details since these can overwhelm their people’s cognitive load, says Dr Jessica Barker MBE , author of Hacked: The Secrets Behind Cyber Attacks .
Instead, she recommends that leaders frame their messages in ways that cut through jargon and ensure that their advice is relevant, accessible and actionable. “An essential leadership skill for this is empathy,” Barker explains. “When you’re trying to build a positive culture, it is crucial to understand why people are not practicing the behaviors you want rather than trying to force that behavioral change with fear, uncertainty and doubt.”
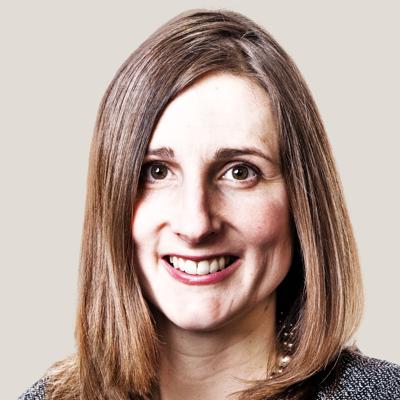
- Editorial Standards
- Reprints & Permissions
Join The Conversation
One Community. Many Voices. Create a free account to share your thoughts.
Forbes Community Guidelines
Our community is about connecting people through open and thoughtful conversations. We want our readers to share their views and exchange ideas and facts in a safe space.
In order to do so, please follow the posting rules in our site's Terms of Service. We've summarized some of those key rules below. Simply put, keep it civil.
Your post will be rejected if we notice that it seems to contain:
- False or intentionally out-of-context or misleading information
- Insults, profanity, incoherent, obscene or inflammatory language or threats of any kind
- Attacks on the identity of other commenters or the article's author
- Content that otherwise violates our site's terms.
User accounts will be blocked if we notice or believe that users are engaged in:
- Continuous attempts to re-post comments that have been previously moderated/rejected
- Racist, sexist, homophobic or other discriminatory comments
- Attempts or tactics that put the site security at risk
- Actions that otherwise violate our site's terms.
So, how can you be a power user?
- Stay on topic and share your insights
- Feel free to be clear and thoughtful to get your point across
- ‘Like’ or ‘Dislike’ to show your point of view.
- Protect your community.
- Use the report tool to alert us when someone breaks the rules.
Thanks for reading our community guidelines. Please read the full list of posting rules found in our site's Terms of Service.
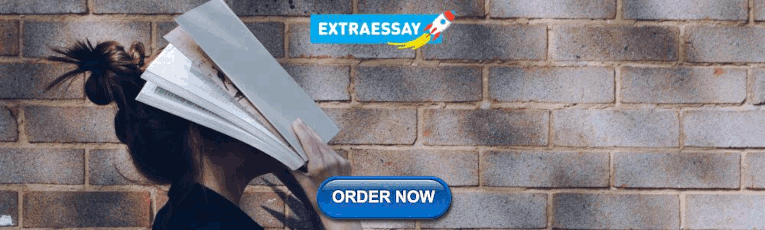
IMAGES
VIDEO
COMMENTS
Dr. Zina O'Leary explains the problem-solving process and demonstrates its usefulness in conducting research. Chapter 1: The Problem-Solving Process icon angle down. Start time: 00:00:00; End time: 00:03:27; Chapter 2: Using the Process: Research Questions and Design icon angle down. Start time: 00:03:28; End time: 00:07:08; Chapter 3: Using ...
The findings from this second experiment showed that people tend to go through two different stages when solving a series of problems. People begin their problem-solving process in a generative manner during which they explore various tactics — some successful and some not. Then they use their experience to narrow down their choices of ...
The problem-solving models must be constructed so that they can operate iteratively, can be easily accessible to users with different knowledge backgrounds and can be identified and implemented on ...
We are building on the previous work studying expert-novice differences and problem solving but taking a different direction. We sought to create an empirically grounded framework that would characterize the detailed structure of the full problem-solving process by focusing on the operational decisions that skilled practitioners make when successfully solving authentic problems in their ...
Abstract. This chapter follows the historical development of research on problem solving. It begins with a description of two research traditions that addressed different aspects of the problem-solving process: (1) research on problem representation (the Gestalt legacy) that examined how people understand the problem at hand, and (2) research on search in a problem space (the legacy of Newell ...
This article presents a four-part theoretical framework of narrative theory, self-disclosure theory, autobiographical memory theory and attribution theory, which together provide a sense-making structure for why probing works and why it is important to research interviews. I then summarise a taxonomic model, entitled the DICE approach to probing.
Research in Problem-Solving: Improving the Progression from Novice to Expert Roxi Finney Abstract: This paper presents a review of research in problem solving. The first section includes various research papers across multiple disciplines which call for the need to improve the problem solving skills of students and the need to improve the ...
The problem statement should indicate an area of conflict, concern, or controversy. 5. The problem should be something that is feasible (time, money, resources) for you to tackle. 6. You should be able to gather information and/or data to help solve the problem. (i.e., you can research the problem)
Research. ge andDefinition:Problem Solving Competency:Problem solving is the process of determining an unknown "series of actions" to take in order to find a solution (Muir et al., 2008) and involves calculations, application of various strategies, rationalization, beliefs, an. man, 2010).Essential Components for Students:Apply a ...
The relationship between problem-solving, proving and learningIn the past three decades, there has been a dramatic shift in the nature of research on mathematical problem-solving. In the 1980s, problem-solving was primarily conceived of as a goal of mathematical instruction.
Noting the strong influence that the problem finding process exerts on the outcome of the problem-solving process (Lee and Cho 2007) and how this influence increases as the degree of structure of the problem decreases (Mumford et al. 1994), understanding the problem finding process within the context of ill-structured and real-world tasks, such ...
Step 1 - Define the Problem. The definition of the problem is the first step in effective problem solving. This may appear to be a simple task, but it is actually quite difficult. This is because problems are frequently complex and multi-layered, making it easy to confuse symptoms with the underlying cause.
3. Always test each finding—no matter how small it may be—against the Evidence Analysis Process Map. The ultimate "fact" in all projects is this: Our conclusion can never be better than the evidence on which we base it. 4. Always use each finding to lead you to something else. No fact is an absolute.
A research problem is a gap in existing knowledge, a contradiction in an established theory, or a real-world challenge that a researcher aims to address in their research. It is at the heart of any scientific inquiry, directing the trajectory of an investigation. The statement of a problem orients the reader to the importance of the topic, sets ...
May 21, 2024. Identifying a research problem is a crucial first step in the research process, serving as the foundation for all subsequent research activities. This guide provides a comprehensive overview of the steps involved in identifying a research problem, from understanding its essence to employing advanced strategies for refinement.
The first step of conducting research is identifying a researchable problem. This chapter explains the procedures to identify the research problem. The procedure of research involves systematically investigating a subject matter to reveal facts or reach new conclusions. Research can be theory driven or problem driven.
analyzing arguments, claims, or evidence. making inferences using inductive or deductive reasoning. judging or evaluating. making decisions or solving problems. asking and answering questions for clarification. defining terms. identifying assumptions. interpreting and explaining. reasoning verbally.
Problem-solving is a subset of critical thinking and employs the same strategies. Although the line between the two is fuzzy, in general, the goal of problem-solving is to adduce correct solutions to well-structured problems, whereas the goal of critical thinking is to construct and defend reasonable solutions to ill-structured problems.
the process of solving a problem through researching and probing. inquiry. It involves questioning and interrogations. ... provides the best solution to some of the world problems and also enhance the knowledge of the researchers tool for building knowledge and for facilitating new learning introduces new ideas, ... The Importance of Research
Problem-Solving in Business: SKILLS AND QUALIFICATIONS NEEDED IN PROBLEM-SOLVING This guide is designed to help you approach your business problem-solving process in a systematic way through information sources that can help you understand your situation more clearly and provide a distinct direction towards the appropriate solution.
Summary: 1."Inquiry" is the process of searching for knowledge and information about a problem through asking questions and probing while "investigation" is the organized process of inquiring about a problem through inspection and assessment. 2.Both an inquiry and an investigation may be used interchangeably, but an investigation is a ...
3. solve problems, that includes the abilities to understand problems, develop mathematical models, solve them, and interpret their solutions, 4. communicate ideas using symbols, tables, diagrams ...
1. Multiple Choice. Immersion is the process of solving a problem through researching and probing. 2. Multiple Choice. Inquiry is the systematic examination to uncover facts regarding the matter. 3. Multiple Choice. Investigation means that the researches immerse themselves in the culture they are studying.
"To solve problems, we need to commit to making time to face a problem in its full complexity, which also requires that we take back control of our thinking," says Chris Griffiths, an expert ...