
An official website of the United States government
The .gov means it’s official. Federal government websites often end in .gov or .mil. Before sharing sensitive information, make sure you’re on a federal government site.
The site is secure. The https:// ensures that you are connecting to the official website and that any information you provide is encrypted and transmitted securely.
- Publications
- Account settings
Preview improvements coming to the PMC website in October 2024. Learn More or Try it out now .
- Advanced Search
- Journal List
- Brain Neurosci Adv
- v.2; Jan-Dec 2018
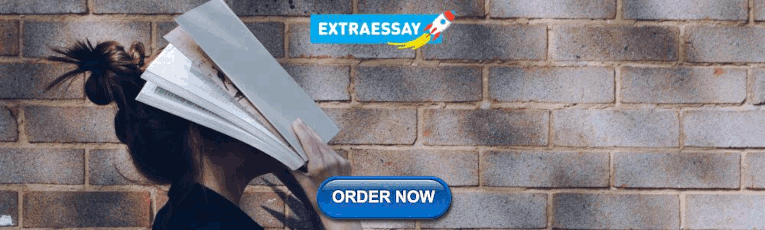
Memory: Looking back and looking forward
John p. aggleton.
1 School of Psychology, Cardiff University, Cardiff, UK
Richard G. M. Morris
2 Centre for Cognitive and Neural Systems, The University of Edinburgh, Edinburgh, UK
This review brings together past and present achievements in memory research, ranging from molecular to psychological discoveries. Despite some false starts, major advances include our growing understanding of learning-related neural plasticity and the characterisation of different classes of memory. One striking example is the ability to reactivate targeted neuronal ensembles so that an animal will seemingly re-experience a particular memory, with the further potential to modify such memories. Meanwhile, human functional imaging studies can distinguish individual episodic memories based on voxel activation patterns. While the hippocampus continues to provide a rich source of information, future progress requires broadening our research to involve other sites. Related challenges include the need to understand better the role of glial–neuron interactions and to look beyond the synapse as the sole site of experience-dependent plasticity. Unmet goals include translating our neuroscientific knowledge in order to optimise learning and memory, especially among disadvantaged populations.
Miss Prism: Memory, my dear Cecily, is the diary that we all carry about us.
Cecily: Yes, but it usually chronicles the things that have never happened, and couldn’t possibly have happened. ( Oscar Wilde (1895) , The Importance of Being Earnest)
This conversation anticipates two complementary themes that run throughout memory research. In the 1960s, when the British Neuroscience Association (BNA - the then Brain Research Asssociation or BRA) was founded, the ‘cognitive revolution’ was underway and the principal goal was to understand how the ‘diary’ of our memories was written and how the correct page became available when looking back to remember past events. A parallel goal was to understand how memory errors occur. Over the intervening period, there has been a growing emphasis on neural circuits, rather than specific brain areas, combined with increasingly detailed analyses of synaptic plasticity. Now, in the 21st century, the traditional representational framework of cognitive neuroscience is under attack from those arguing for ‘embodied cognition’ ( Claxton, 2015 ), a debate that is yet to be resolved. Meanwhile, we can now appreciate that memory not only allows us to look back but can also help us look forward, making the past a platform for future thinking.
Memory: at the birth of the BNA
With the aid of an old textbook ( Physiological Psychology by Peter Milner, 1970 ), it is possible to see how far research in learning and memory has come in 50 years. The answer is a long, long way.
Attempts in the 1960s to understand learning and memory were heavily influenced by animal research. One leading approach for studying memory mechanisms was (and still is) the behavioural analysis of how lesions affect learning, with related research examining the extinction of that learning ( Grey, 2000 ; Milner, 1970 ). At the time, analyses of animal learning were dominated by ideas and experimental procedures taken from ‘Behaviourism’. ‘Reinforcement’ was regarded as a kind of stamping-in operation for learning, something that increased the probability of a certain class of behaviour, with many ideas about learning inevitably shaped by notions of reinforcement. That said, the pioneers of modern animal learning theory in the 1970s were among the first to recognise that the old ideas of reinforcement and ‘drive reduction’ had to be replaced by the concept of ‘reward-expectancy’. The important discovery of ‘blocking’ ( Kamin, 1968 ) heralded new thinking ( Figure 1(a) ), and experiments by Robert Rescorla and by Nick Mackintosh led to the idea that learning occurred when expectancies about reward availability were violated. This idea was encapsulated in the famous ‘Rescorla and Wagner’ equation of 1972, which subsequently helped to interpret the actions of dopamine in the brain ( Schultz and Dickinson, 2000 ). The concept of ‘reward-expectancy’ was born and remains a major focus of current thinking.

Memory: At the birth of the BNA. (a) Reward predictability : A major trigger for changes in the way learning was studied was the discovery that reinforcement was only effective for learning, even in motivated animals, if it was unexpected. One phenomenon that led to this discovery is that of ‘blocking’, first reported by Leon Kamin. A conditional stimulus (white box, CS) paired with reinforcement (R) fails to be conditioned (X) if the reinforcement is already predicted by another stimulus (black box, CS). (b) Protein synthesis and memory retention : The role of protein synthesis in memory followed the discovery that post-training administration of blockers of protein synthesis (e.g. cyclohexamide) selectively interrupted long-term memory retention (after Davis and Squire, 1984 ). (c) Memory dissociations in amnesia : The apparently selective nature of ‘global amnesia’ followed observations by Brenda Milner that patient H.M. could successfully copy but was unable to remember the Rey–Ostereith figure for any length of time (top), but could learn the motor task of mirror drawing (bottom). Similar dissociations in other patients soon followed.
Students of animal learning went on to develop numerous training regimes, sometimes of such esoteric precision that their advances risked becoming impenetrable to others. Happily, many influential ideas did emerge from these analyses, including Tony Dickinson’s distinction between learned actions and learned habits, a distinction that now stimulates research on the striatum and provides a framework for understanding aspects of drug addiction ( Everitt and Robbins, 2005 ). There remained, however, a clear gap between those who sought to carry on working with laboratory rats and others who believed that to understand the human brain it was necessary to conduct invasive work in nonhuman primates. In addition, the separation between disciplines, such as that between physiology and psychology, was also deeply rooted. The interdisciplinary nature of contemporary neuroscience remained far in the future.
Beyond primates and rats, a different approach was to examine learning in simpler organisms. In St Andrews, Graham Horridge published studies of learning in arthropods. Although not influential at the time, it is salutary to reflect on his prescience in writing a book in the 1960s on ‘interneurons’ ( Horridge, 1968 ) – long before Peter Somogyi’s anatomical studies revealed both their complexity and importance ( Somogyi and Klausberger, 2005 ). Also, just as the BRA/BNA was being formed, Eric Kandel first described his work in France on the conditioning of single neurons in the sea slug ( Aplysia depilans ; Kandel and Tauc, 1965 ), followed by the programme of research on the neural basis of habituation and sensitisation in Aplysia californica that was to win him the Nobel Prize in 2000. A key feature of this research was the detailed understanding of functional circuitry, leading the way to investigate molecular mechanisms of plasticity ( Kandel, 2001 ).
Another focus was on memory consolidation – what made memory traces last – with protein synthesis emerging as a key idea. However, this concept was not without curious precursors. One idea was that DNA, or at least RNA, might be modified by the patterns of electrical activity affecting a neuron ( Milner, 1970 ), an idea made fashionable by the dramatic breakthroughs in genetic coding and transcription. Certain infamous cannibalism experiments with flatworms ( Planaria ) suggested that memories could be transferred by the process of feeding minced but ‘educated’ flatworms to ‘naïve’ flatworms. Closely related work involved the transfer of RNA from trained to naïve rats. The textbook Physiological Psychology stated that ‘the most popular analogy for memory at present … is the mechanism of genetic storage’ ( Milner, 1970 : 426) before, wisely, questioning this conclusion. The memory transfer experiments did, nonetheless, stimulate research that revealed a role for protein synthesis in long-term retention ( Davis and Squire, 1984 ). Consequently, Milner (1970) could conclude that the search for molecular changes in learning should be directed to proteins and lipids, a sentiment that has stood the test of time ( Figure 1(b) ). It is, however, worth adding that the emerging field of epigenetics now examines how environmental factors can have long-term effects on gene expression.
But what of humans, or, as they were then described, of ‘man’ [sic]? During that same period, new information emerged from neuropsychological studies of patients suffering from memory loss. It was known that damage in one of two regions, the medial diencephalon and the medial temporal lobe, was consistently linked with the failure to retain new information, that is, with anterograde amnesia ( Mair et al., 1979 ; Scoville and Milner, 1957 ). More novel was the realisation that anterograde amnesia was not as ‘global’ as first thought, as perceptual-motor learning is preserved ( Milner et al., 1968 ; Figure 1(c) ). The full significance of this dissociation between memory types (subsequently termed explicit vs implicit, or declarative vs nondeclarative) would not emerge for another decade or more ( Squire and Zola-Morgan, 1991 ; Weiskrantz and Warrington, 1979 ). These same neuropsychological studies were, however, severely limited in one key respect: it was impossible to visualise the particular patterns of brain injury in those individuals being studied. Researchers had to make educated guesses based on separate postmortem findings. Remarkably, the first study to combine detailed neuropsychological investigations of memory loss in amnesia along with subsequent postmortem data from the same patient would not be published for another decade ( Mair et al., 1979 ), with the studies of Squire’s group later setting an exacting standing in postmortem neuroanatomical analyses ( Zola-Morgan et al., 1986 ). Even now, such studies remain rare, though they include a postmortem description of the brain of the famous amnesic patient H.M. ( Annese et al., 2014 ).
Finally, in a fascinating section in Physiological Psychology , Milner (1970) considered hippocampal lesion studies in animals. He described the perplexing finding that bilateral medial temporal lesions in monkeys did not produce the expected anterograde amnesic syndrome ( Orbach et al., 1960 ), an apparent anomaly that has taken decades to resolve ( Murray and Wise, 2010 ). Milner (1970) also briefly described the outcome of hippocampal lesions in rats in just a single sentence, which mentioned that spatial learning is usually impaired ( Kaada et al., 1961 ; Kimble, 1963 ). With hindsight, it seems incredible that there was so much uncertainty about the importance of the hippocampus for memory in animals other than humans. In 1970, however, Peter Milner felt forced to infer that there must be an ‘evolutionary discontinuity’, leaving the hippocampus important for only human memory. We now know that this is incorrect.
Memory: what happened next (1970s, 1980s and 1990s)?
These decades brought a series of remarkable discoveries that form the bedrock of much current thinking. At the beginning of the period, our understanding of brain circuitry was rudimentary – a problem exacerbated by how older ‘lesion’ techniques did not distinguish between damage to cells of origin and to fibres of passage. A crucial first step was the introduction of axonal tracing techniques such as autoradiography and horseradish peroxidase ( Cowan et al., 1972 ; LaVail and LaVail, 1972 ), which heralded a quiet revolution that continues to this day with new single-cell connectional techniques ( Figure 2(a) ).

Memory: What happened next (1970s, 1980s and 1990s)? (a) Neuroanatomy : The development of axonal tract-tracing techniques such as HRP and autoradiography replaced older degeneration-based lesion techniques that were unable to distinguish cell–cell connections from damage to fibres of passage. Image shows evidence of dense projections from the monkey hippocampus (subiculum) to the mammillary bodies, a pattern that helped make sense of patients being studied at the National Hospital, Queen Square, by Elizabeth Warrington. (b) Electrophysiology : The presence of hippocampus place-cells was first revealed by John O’Keefe and Jonathan Dostrovsky through single-cell recording of neurons in area CA1 of freely moving rats in simple T- and X-mazes. Later work by Robert Muller and John Kubie used open-arenas and automated tracking of the animal and recording of cell-firing to realise much greater objectivity. (c) Electrophysiology : The discovery of long-term potentiation by Bliss and Lomo in 1973 was rapidly followed by a developing understanding of the interaction of glutamatergic and GABAergic transmission in mediating and regulating synaptic plasticity. This was summarised by Tim Bliss and Graham Collingridge in 1993 in one of the most cited papers in neuroscience. (d) Behaviour : The water maze was one of a variety of new behavioural paradigms designed to test the ostensibly spatial functions of the hippocampus realised through the discovery of place-cells (a, b). The water maze was developed at the Gatty Marine Laboratory of the University of St Andrews by Richard Morris. Hippocampal lesions caused severe deficits in learning (c). (e) Neuropsychological dissociations : Studies of brain injured patients helped to dissociate separate processes associated with full recall of an event and its context (‘who, what and where’) from the mere sense of familiarity of prior occurrence (‘I’ve seen this before’). Building on a body of experimental work, John Aggleton and Malcolm Brown developed a theoretical framework implicating different brain regions in these distinct forms of memory retrieval ( Aggleton & Brown, 1999 , 2006 ). Consistent with this dissociation, patients with greater pathology in the mammillary bodies and fornix (SMB) show a loss of recollective-based recognition but preservation of familiarity-based recognition (LMB, matched patient controls) ( Vann et al., 2009 ).
Meanwhile, some of the most influential breakthroughs involved research on the hippocampus, with two electrophysiological discoveries taking centre stage. The first was the description of place-cells in the rat hippocampus ( O’Keefe and Dostrovsky, 1971 ), research that led to a Nobel Prize for John O’Keefe ( Figure 2(b) ). The activity of a single place cell is tuned to a particular location, as defined by distal landmarks (i.e. by allocentric space), with different cells firing in different locations. The significance of this discovery was further enhanced by publication of The Hippocampus as a Cognitive Map ( O’Keefe and Nadel, 1978 ), which provided a comprehensive spatial theory of hippocampal function. The second discovery was long-term potentiation (LTP), first described in the rabbit dentate gyrus ( Bliss and Collingridge, 1993 ; Bliss and Lomo, 1973 ; Figure 2(c) ). While models of learning based on prolonged changes in synaptic strength had been postulated, the discovery of LTP revealed direct evidence that lasting changes in synaptic strength could be observed in mammals. Critical issues included the physiological and pharmacological basis of LTP (and its companion, long-term depression) as well as the need to determine whether LTP was, in fact, necessary for hippocampal-based learning.
At the same time, new behavioural tests of spatial memory, most notably those using the radial-arm maze ( Olton and Samuelson, 1976 ), the Barnes Maze ( Barnes, 1979 ) and the water maze ( Morris, 1981 ), provided more sophisticated ways of analysing behaviour. These advances then coalesced when studies using the water maze not only confirmed the importance of the rat hippocampus for allocentric spatial learning ( Morris et al., 1982 ) but also offered the opportunity to study the link between hippocampal LTP and spatial memory ( Morris et al., 1986 ; Figure 2(d) ). Successive studies revealed that blocking LTP impaired learning, as demonstrated by specific drugs that act as antagonists of the N -methyl- d -aspartate receptor, by the physiological saturation of LTP and later by gene targeting that affected LTP induction and expression. Interestingly, disruption of LTP can occur without affecting memory retrieval – an important dissociation. Despite such findings, there has long been debate over the true relevance of LTP for memory formation ( Stevens, 1998 ), while work with genetically modified mice (such as Grin1 ΔDGCA1 developed by Peter Seeburg in Germany) points to a more complex picture than often appreciated ( Bannerman et al., 2014 ). Specifically, a distinction must be made between the likely necessity for N-methyl-D-aspartate (NMDA) receptor-dependent LTP in the neocortex to store lasting ‘engrams’ and the sometimes lack of its necessity in the hippocampus during learning (e.g. for straightforward spatial tasks that lack ambiguity). Meanwhile, recent advances in using optogenetics to study fear conditioning have arguably provided some of the most compelling evidence of a causal link between LTP and memory ( Nabavi et al., 2014 ). It should also be remembered that this extensive body of work on neural plasticity stands on the shoulders of neural models of memory developed by the British mathematician David Marr (1971) , followed by later work on distributed associative memory ( Dayan and Abbott, 2001 ; McNaughton and Morris, 1987 ; Rolls, 2016 ).
Novel ideas in cognitive psychology also transformed the landscape. In 1972, Endel Tulving introduced the concept of ‘episodic memory’, which together with the companion notion of semantic memory make up explicit (or declarative) memory. Episodic memory concerns the recollection of autobiographic events that are set in a particular time and place ( Tulving, 1972 ). In contrast, semantic memory concerns factual knowledge, highlighting fundamental differences between ‘knowing’ (semantic) and ‘remembering’ (episodic). As already noted, there was prior evidence from amnesics that explicit memory and implicit memory rely on different neural substrates. Evidence accumulated showing how phenomena such as priming, perceptual-motor learning and classical conditioning should all be considered as examples of implicit learning, that is, memory without conscious remembering ( Schacter, 1992 ; Schacter et al., 1993 ).
One of many consequences was a reappraisal of the cognitive demands in memory tests given to humans and other animals. In particular, if the animal task taxes implicit memory, while the corresponding human task taxes explicit memory, then cross-species generalisations are confounded. A related consequence was the protracted debate over how recognition memory, which can readily be tested in both humans and other animals, should be categorised. One group argued that recognition and recall are two sides of the same coin ( Squire et al., 2007 ), while others argued that recognition memory comprises two independent elements – one based on recall processes, the other on a sense of familiarity ( Yonelinas, 2002 ). A key first step was the discovery of electrophysiological responses in the rhinal cortices that distinguish whether a stimulus is novel or familiar ( Brown et al., 1987 ), revealing that activity in areas such as perirhinal cortex might be sufficient to guide recognition judgements based on familiarity ( Aggleton and Brown, 1999 , 2006 ; Eichenbaum et al., 1994 ). Both clinical and monkey lesion evidence further supported the notion that the hippocampus is needed for recollective-based recognition while extra-hippocampal processes support familiarity-based recognition ( Aggleton et al., 2000 ; Murray and Mishkin, 1998 ). The gradual resolution of this debate about the nature of recognition memory ( Figure 2(e) ) had important implications for animal models of amnesia and for how we consider hippocampal function ( Murray and Wise, 2010 ).
In these same decades, Loftus and Palmer (1974) highlighted the malleability of memory by first showing that the words you use when interrogating someone’s memory can seemingly change that memory. Numerous subsequent studies confirmed the unreliability of eye-witness testimony ( Loftus, 1979 ). These findings not only have enormous practical importance but are also of theoretical significance. Some recall errors reveal that specific memories, even when ostensibly ‘consolidated’, can change after their initial formation ( Wright and Loftus, 1998 ). This simple but powerful idea has fundamentally altered the concept of memory consolidation. Rather than it being a fixing process, whereby memory is written down and later read from a ‘diary’, it appears that accessing a memory can lift it from the pages of the notional diary, creating an unstable state in which the memory representation can be altered. This rewriting or updating process is called ‘reconsolidation’. How consolidation and reconsolidation work at circuit and molecular levels is now an active area of current research ( Lee, 2009 ; Lee et al., 2017 ).
Memory: the contemporary scene
Three approaches have dominated memory research over the last quarter century. The first relates to the search for plastic mechanisms at the synapse, where a key focus has been on glutamate receptors and the downstream biochemical pathways that regulate their expression at the synapse. The second arises from the ability to genetically modify brain cells and organisms in order to test the importance of specific molecules for learning and memory. The third reflects the extraordinary advances in noninvasive imaging, which now make it possible to reveal structural detail (magnetic resonance imaging (MRI)), measure markers of neuronal and neurochemical activity (positron emission tomography, PET; functional MRI, fMRI; magnetoncephalography, MEG) and reconstruct white matter (e.g. diffusion tensor imaging (DTI)).
The focus on plasticity began, as mentioned above, with work on LTP ( Figure 2(c) ). The discovery that glutamate is the principal excitatory neurotransmitter of the brain stimulated numerous efforts to understand the fundamental mechanisms by which ionotropic and metabotropic glutamate receptors work together to regulate synaptic efficacy. The basic idea is that there may be alterations in the release of glutamate on the presynaptic side, alongside changes in the trafficking of a sub-type of glutamate receptor, AMPA receptors, on the post-synaptic side. Coupled to this concept have been advances in molecular-genetic technology, enabling specific genes of interest to be deleted or expressed in transgenic mice. Within the field of memory research, this work began with a global deletion of the alpha subunit of calcium-calmodulin kinase II (aCaMKII) that is greatly enriched in the post-synaptic density of glutamatergic synapses ( Silva et al., 1992 ). Later work used Cre–Lox technology to restrict the gene deletion of interest to specific neural circuits and brain regions, which when coupled to temporally inducible manipulations created opportunities for imaginative interventions, as typified by the research of Nobel Laureate Susumu Tonegawa at MIT.
The application of this molecular technology has reinvigorated systems neuroscience. Already, ‘gene knock-out’ is becoming a dated technology with new viral techniques for monitoring and selectively activating the brain in a cell-type and regionally specific manner becoming the new ‘state-of-the-art’. Viral approaches have made optogenetics more viable as an approach, with the potential to allow physiology and behaviour to move from correlation to causation ( Figure 3(b) ). However, for all the excitement about these new approaches, they come at a price. Much of the work has been conducted with mice, which offer a more limited repertoire of behavioural analyses than rats, although comparable genetic and viral methodologies for rats are gradually emerging. One consequence is an ever-greater focus on rodents, such that many with comparative interests, or loyalty to the idea that the primate brain is the one we should always have in mind, are becoming concerned. Nonhuman primate work has simultaneously become more restricted.

Memory: The contemporary scene. (a) Use of multi-voxel pattern recognition in fMRI to distinguish distinct episodic memories. fMRI has traditionally been used to reveal that a brain area is ‘activated’ by distinct forms of experience. The introduction of high-field imaging coupled to analysis of individual voxels reveals the capacity to distinguish the ‘hot-spot’ representations of different memories. This advance takes human imaging to a new level that, while not yet capable of decoding the nature of the memory, can nonetheless distinguish one memory trace from another within a brain area devoted to memory (after Chadwick et al., 2010 ). (b) Use of molecular engineering tools to address systems issues in memory research. Gene knock-out techniques are gradually being replaced by viral expression techniques in which, for example, an adenovirus expressing the optogenetic construct channelrhodopsin (ChR2), with a red marker called mCherry, is introduced into specific brain areas (here the dentate gyrus (DG) of the hippocampus and the basolateral amygdala (BLA)) in such a way it is only expressed when a specific immediate early gene (e.g. c- fos ) is expressed. Administering doxycycline to the drinking water prevents the virus from being expressed, but when this is removed, brain cells that are active during a specific learning experience can be ‘tagged’ with ChR2 at the time of learning. Later application of blue light via miniature light guides implanted above these brain areas enables only those specific neurons that participated in a learning experience to be activated. By doing this in hippocampus and amygdala, and combining their activation with different forms of positive or negative reinforcement, Susumu Tonegawa et al. were able to investigate the neural mechanisms by which the valence of a memory (good or bad) could be transformed (after Redondo et al., 2014 ). (c) Cognition and deprivation : Vocabulary scores of Ecuadorian children aged 36 to 72 divided by wealth, showing the divergent performances of children associated with poverty (after Grantham-McGregor et al., 2007 ).
Turning back to humans, cognitive neuroscience in the 1960s and 1970s was severely hampered by the lack of imaging methods. The first clinical computerised tomography (CT) scan took place in 1971, with the first MRI scan of the human body published in 1977 by Raymond Damadian. Subsequently, Paul Lauterbur and Peter Mansfield shared a Nobel Prize for further developing the MRI technique. The resulting proliferation of noninvasive imaging techniques reinvigorated cognitive neuroscience, beginning with PET studies by Marcus Raichle et al. ( Posner and Raichle, 1994 ). Perhaps the most salutary lesson is how classical neuropsychology, based on patients with brain injury, had only highlighted the limited number of brain structures necessary for a particular form of memory, while PET and MRI techniques could reveal the multiple brain sites and pathways whose activity is associated with the same classes of memory. An example of the latter concerns the many parietal, frontal and parahippocampal areas now implicated in episodic memory ( Cabeza et al., 2008 ; Ranganath and Ritchey, 2012 ). These imaging findings, some using multi-voxel pattern-recognition techniques for analysis ( Figure 3(a) ), promote an integrated, systems-based approach to memory that not only considers encoding but also the control processes involved in memory formation and retrieval.
New ideas also emerged about how memory processes are used. One insight was that episodic memory not only provides access to past events but also aids imagining future events ( Ingvar, 1984 ; Schacter et al., 2012 ). Evidence includes the finding that amnesics, who lack episodic memory, have an impoverished ability to imagine fictitious events ( Hassabis et al., 2007 ), that is, to imagine ‘things that couldn’t possible have happened’ (to quote Cecily from the ‘Importance of Being Earnest’). One possibility is that their inability to construct past scenes in episodic memory reflects a more general failure of scene representation, so affecting imagination ( Zeidman and Maguire, 2016 ).
Memory: current needs and future challenges
The big challenges appear to lie at polar ends of the research spectrum. At one end are studies into the molecular basis of memory, closely combined with unravelling the precise details of how ensembles of neurons interact. At the other end of the spectrum are unmet translational needs concerned with how to apply our understanding of neural mechanisms of learning to medical and societal issues.
Research into neuronal mechanisms of plasticity faces numerous obstacles. One example stems from how different forms of memory (e.g. explicit vs implicit) depend on different anatomical substrates, creating the challenge of determining the generality of any given plastic mechanism. Even within one form of memory, the situation is incredibly complex. To take the case of episodic memory, it is not all about the hippocampus, as other structures (e.g. within the medial diencephalon and frontal cortex) are also vital – but we do not know why. Added complexities stem from the need to decipher the role of local and more global oscillations as conveyors of episodic information, as well as the assimilation of newly learned information into existing semantic networks.
In recent years, novel techniques, such as those using designed receptors exclusively activated by designer drugs (DREADDS) and optogenetics, have provided new levels of specificity with which to manipulate neuronal assemblies in vivo. It already appears possible to identify neurons in rodents that are activated by a particular experience and then subsequently reactivate those same neurons to re-create a representation of the original experience ( Liu et al., 2014 ; Tonegawa et al., 2015 ). This work is presented in the context of searching for the ‘engram’, such that the goal of visualising learning in complex organisms in real time at the cellular level is now becoming a reality ( Figure 3(b) ). This ability is clearly just the beginning of research into the encoding, storage, consolidation and retrieval of memory at levels of temporal and anatomical specificity not previously realised. A challenge is to ensure appropriate levels of sophistication in the behavioural analyses that are intrinsic to interpreting these emerging methods. Clinical applications could include the removal (or at least lowered levels of expression) of specific distressing memories, notwithstanding the concomitant ethical issues ( Parsons and Ressler, 2013 ). At the same time, the search for mechanisms of plasticity will extend beyond the synapse. It is already known that white matter changes following experience may contribute to cognition ( Zatorre et al., 2012 ). One priority is to know just how little experience is required to initiate alterations in axonal properties, with current evidence suggesting that changes might occur after just a few learning trials ( Hofstetter et al., 2013 ). Related issues centre on the possible role of glia in memory. The last few years have seen extraordinary interest in glial–neuronal interactions that might influence CNS plasticity.
The study of individual differences will become a major focus. This task is aided by improved, noninvasive imaging, set alongside comprehensive genetic analyses. These techniques will be informed by the identification of numerous gene variants that can affect different aspects of learning and memory. Epigenetics will also increasingly aid our understanding of individual differences. These same advances will, however, also raise new ethical issues. At present, individual episodic memories can be detected and distinguished from their pattern of fMRI signals across different voxels ( Figure 3(a)) ( Bonnici et al., 2013 ; Rissman et al., 2016 ). Further advances at the individual level will create even greater concerns about mind reading and lie detection ( Evers and Sigman, 2013 ).
One major obstruction to progress is our woeful lack of knowledge about human brain connectivity ( Rockland, 2015 ). This long-standing problem arises principally from the need to inject axonal tracers in vivo and to visualise postmortem in order to confirm neuronal connectivity, that is, methods that can only be used in other animals. Although MRI is helping to reduce the species gulf, there are major limitations. Initiatives such as the Human Connectome Project will undoubtedly help, but the methods still fall short on anatomical resolution. Furthermore, while DTI can visualise white matter, it cannot distinguish between afferent or efferent connections, nor identify unmyelinated pathways. There also remains the problem of transposing and validating findings from other animals to the human brain. Despite the many genetic and molecular discoveries showing how conservative neural mechanisms often remain, it is also worth remembering that the human brain is unique. It is, for example, three times the size it should be for a primate of our body size.
A major challenge concerns how we apply our knowledge of memory mechanisms to improve quality of life. Naturally, there is great interest in drugs that enhance cognitive performance ( Greely et al., 2008 ; Sahakian and Morein-Zamir, 2015 ), but, at present, these are typically of relatively modest impact in those with a healthy CNS. More realistically, findings from neuroscience should be informing education in schools, yet progress in this area is still in its infancy. For this to happen, memory researchers need to collaborate more with those in other disciplines, as well as to understand the demands on teachers and others in educational settings. There also remains the ever-growing challenge of how to prevent and treat memory loss in an ageing world population, and how to counteract the consequences of chronic disease and deprivation on cognition, including memory, in developing countries. In 2007, it was estimated that 200 million children under 5 years of age are not fulfilling their cognitive development potential ( Grantham-McGregor et al., 2007 ). Strategies to reduce these inequalities ( Engle et al., 2011 ) will require neuroscientists to adopt completely different approaches, with these challenges representing some of the most important goals for the discipline.
Acknowledgments
Richard G. M. Morris thanks John O’Keefe and Lynn Nadel for inspiration and keeping me on track in the early years, and the many students and post-docs with whom he has been privileged to have worked over 30+ years, including colleagues in Edinburgh Neuroscience. Thanks also to the Medical Research Council who provided many years of Programme Grant support, and latterly the European Research Council. John P. Aggleton thanks Mort Mishkin and Malcolm Brown for their wisdom and guidance, along with numerous colleagues at Cardiff University, Durham University and NIH. The Wellcome Trust and Medical Research Council have provided invaluable support.
Declaration of conflicting interests: The author(s) declared no potential conflicts of interest with respect to the research, authorship, and/or publication of this article.
Funding: The author(s) received no financial support for the research, authorship, and/or publication of this article.
- Aggleton JP, Brown MW. (1999) Episodic memory, amnesia, and the hippocampal–anterior thalamic axis . Behavioral and Brain Sciences 22 : 425–444. [ PubMed ] [ Google Scholar ]
- Aggleton JP, Brown MW. (2006) Interleaving brain systems for episodic and recognition memory . Trends in Cognitive Sciences 10 : 455–463. [ PubMed ] [ Google Scholar ]
- Aggleton JP, McMackin D, Carpenter K, et al. (2000) Differential cognitive effects of colloid cysts in the third ventricle that spare or compromise the fornix . Brain 123 : 800–815. [ PubMed ] [ Google Scholar ]
- Annese J, Schenker-Ahmed NM, Bartsch H, et al. (2014) Postmortem examination of patient H.M.’s brain based on histological sectioning and digital 3D reconstruction . Nature Communications 5 : 3122. [ PMC free article ] [ PubMed ] [ Google Scholar ]
- Bannerman DM, Sprengel R, Sanderson DJ, et al. (2014) Hippocampal synaptic plasticity, spatial memory and anxiety . Nature Reviews Neuroscience 15 : 181–192. [ PubMed ] [ Google Scholar ]
- Barnes CA. (1979) Memory deficits associated with senescence: A neurophysiological and behavioral study in the rat . Journal of Comparative and Physiological Psychology 93 : 74–104. [ PubMed ] [ Google Scholar ]
- Bliss TV, Collingridge GL. (1993) A synaptic model of memory: Long-term potentiation in the hippocampus . Nature 361 : 31–39. [ PubMed ] [ Google Scholar ]
- Bliss TV, Lomo T. (1973) Long-lasting potentiation of synaptic transmission in the dentate area of the anaesthetized rabbit following stimulation of the perforant path . The Journal of Physiology 232 : 331–356. [ PMC free article ] [ PubMed ] [ Google Scholar ]
- Bonnici HM, Chadwick MJ, Maguire EA. (2013) Representations of recent and remote autobiographical memories in hippocampal subfields . Hippocampus 23 : 849–854. [ PMC free article ] [ PubMed ] [ Google Scholar ]
- Brown MW, Wilson FAW, Riches IP. (1987) Neuronal evidence that inferomedial temporal cortex is more important than hippocampus in certain processes underlying recognition memory . Brain Research 409 : 158–162. [ PubMed ] [ Google Scholar ]
- Cabeza R, Ciaramelli E, Olson IR, et al. (2008) The parietal cortex and episodic memory: An attentional account . Nature Reviews Neuroscience 9 : 613–625. [ PMC free article ] [ PubMed ] [ Google Scholar ]
- Chadwick MJ, Hassabis D, Weiskopf N, et al. (2010) Decoding individual episodic memory traces in the human hippocampus . Current Biology 20 : 544–547. [ PMC free article ] [ PubMed ] [ Google Scholar ]
- Claxton G. (2015) Intelligence in the Flesh: Why Your Mind Needs Your Body Much More Than It Thinks . New Haven, CT: Yale University Press. [ Google Scholar ]
- Cowan WM, Gottlieb DI, Hendrickson AE, et al. (1972) The autoradiographic demonstration of axonal connections in the central nervous system . Brain Research 37 : 21–51. [ PubMed ] [ Google Scholar ]
- Davis HP, Squire LR. (1984) Protein synthesis and memory: A review . Psychological Bulletin 96 : 518–559. [ PubMed ] [ Google Scholar ]
- Dayan P, Abbott LF. (2001) Theoretical Neuroscience , vol. 10 Cambridge, MA: The MIT Press, pp. S0306–S4522. [ Google Scholar ]
- Eichenbaum H, Otto T, Cohen NJ. (1994) Two functional components of the hippocampal memory system . Behavioral and Brain Sciences 17 : 449–472. [ Google Scholar ]
- Engle PL, Fernald LC, Alderman H, et al. (2011) Strategies for reducing inequalities and improving developmental outcomes for young children in low-income and middle-income countries . The Lancet 378 : 1339–1353. [ PubMed ] [ Google Scholar ]
- Everitt BJ, Robbins TW. (2005) Neural systems of reinforcement for drug addiction: From actions to habits to compulsion . Nature Neuroscience 8 : 1481–1489. [ PubMed ] [ Google Scholar ]
- Evers K, Sigman M. (2013) Possibilities and limits of mind-reading: A neurophilosophical perspective . Consciousness and Cognition 22 : 887–897. [ PubMed ] [ Google Scholar ]
- Grantham-McGregor S, Cheung YB, Cueto S, et al. (2007) Developmental potential in the first 5 years for children in developing countries . The Lancet 369 : 60–70. [ PMC free article ] [ PubMed ] [ Google Scholar ]
- Gray JA. (2000) The Neuropsychology of Anxiety: An Enquiry into the Functions of the Septo-Hippocampal System . Oxford: Oxford University Press. [ Google Scholar ]
- Greely H, Sahakian B, Harris J, et al. (2008) Towards responsible use of cognitive-enhancing drugs by the healthy . Nature 456 : 702–705. [ PubMed ] [ Google Scholar ]
- Hassabis D, Kumaran D, Vann SD, et al. (2007) Patients with hippocampal amnesia cannot imagine new experiences . Proceedings of the National Academy of Sciences 104 : 1726–1731. [ PMC free article ] [ PubMed ] [ Google Scholar ]
- Hofstetter S, Tavor I, Moryosef ST, et al. (2013) Short-term learning induces white matter plasticity in the fornix . Journal of Neuroscience 33 : 12844–12850. [ PMC free article ] [ PubMed ] [ Google Scholar ]
- Horridge GA. (1968) Interneurons . Dallas, TX: Freeman. [ Google Scholar ]
- Ingvar DH. (1984) ‘Memory of the future’: An essay on the temporal organization of conscious awareness . Human Neurobiology 4 : 127–136. [ PubMed ] [ Google Scholar ]
- Kaada BR, Rasmussen EW, Kveim O. (1961) Effects of hippocampal lesions on maze learning and retention in rats . Experimental Neurology 3 : 333–355. [ PubMed ] [ Google Scholar ]
- Kamin LJ. (1968) ‘Attention-like’ processes in classical conditioning . In: Jones MR. (ed.) Miami Symposium on the Prediction of Behavior: Aversive Stimulation . Miami, FL: University of Miami Press, pp. 9–31. [ Google Scholar ]
- Kandel ER. (2001) The molecular biology of memory storage: A dialogue between genes and synapses . Science 294 : 1030–1038. [ PubMed ] [ Google Scholar ]
- Kandel ER, Tauc L. (1965) Heterosynaptic facilitation in neurones of the abdominal ganglion of Aplysia depilans . The Journal of Physiology 181 : 1–27. [ PMC free article ] [ PubMed ] [ Google Scholar ]
- Kimble DP. (1963) The effects of bilateral hippocampal lesions in rats . Journal of Comparative and Physiological Psychology 56 : 273–283. [ PubMed ] [ Google Scholar ]
- LaVail JH, LaVail MM. (1972) Retrograde axonal transport in the central nervous system . Science 176 : 1416–1417. [ PubMed ] [ Google Scholar ]
- Lee JL. (2009) Reconsolidation: Maintaining memory relevance . Trends in Neurosciences 32 : 413–420. [ PMC free article ] [ PubMed ] [ Google Scholar ]
- Lee JLC, Nader K, Schiller D. (2017) An update on memory reconsolidation updating . Trends in Cognitive Sciences 21 : 531–545. [ PMC free article ] [ PubMed ] [ Google Scholar ]
- Liu X, Ramirez S, Tonegawa S. (2014) Inception of a false memory by optogenetic manipulation of a hippocampal memory engram . Philosophical Transactions of the Royal Society B 369 : 20130142. [ PMC free article ] [ PubMed ] [ Google Scholar ]
- Loftus EF. (1979) Eyewitness Testimony . Cambridge, MA: Harvard University Press. [ Google Scholar ]
- Loftus EF, Palmer JC. (1974) Reconstruction of automobile destruction: An example of the interaction between language and memory . Journal of Verbal Learning and Verbal Behavior 13 : 585–589. [ Google Scholar ]
- McNaughton BL, Morris RGM. (1987) Hippocampal synaptic enhancement and information storage within a distributed memory system . Trends in Neuroscience 10 : 408–415. [ Google Scholar ]
- Mair WG, Warrington EK, Weiskrantz L. (1979) Memory disorder in Korsakoff’s psychosis: A neuropathological and neuropsychological investigation of two cases . Brain 102 : 749–783. [ PubMed ] [ Google Scholar ]
- Marr D. (1971) Simple memory: A theory for archicortex . Philosophical Transactions of the Royal Society B 262 : 23–28. [ PubMed ] [ Google Scholar ]
- Milner B, Corkin S, Teuber H-L. (1968) Further analysis of the hippocampal amnesic syndrome: 14-year follow-up study of H.M . Neuropsychologia 6 : 215–234. [ Google Scholar ]
- Milner PM. (1970) Physiological Psychology . New York: Holt, Rinehart & Winston. [ Google Scholar ]
- Morris RG. (1981) Spatial localization does not require the presence of local cues . Learning and Motivation 12 : 239–260. [ Google Scholar ]
- Morris RG, Anderson E, Lynch GS, et al. (1986) Selective impairment of learning and blockade of long-term potentiation by an N-methyl-D-aspartate receptor antagonist, AP5 . Nature 319 : 774–776. [ PubMed ] [ Google Scholar ]
- Morris RG, Garrud P, Rawlins JN, et al. (1982) Place navigation impaired in rats with hippocampal lesions . Nature 297 : 681–683. [ PubMed ] [ Google Scholar ]
- Murray EA, Mishkin M. (1998) Object recognition and location memory in monkeys with excitotoxic lesions of the amygdala and hippocampus . Journal of Neuroscience 18 : 6568–6582. [ PMC free article ] [ PubMed ] [ Google Scholar ]
- Murray EA, Wise SP. (2010) What, if anything, can monkeys tell us about human amnesia when they can’t say anything at all? Neuropsychologia 48 : 2385–2405. [ PMC free article ] [ PubMed ] [ Google Scholar ]
- Nabavi S, Fox R, Proulx CD, et al. (2014) Engineering a memory with LTD and LTP . Nature 511 : 348–352. [ PMC free article ] [ PubMed ] [ Google Scholar ]
- O’Keefe J, Dostrovsky J. (1971) The hippocampus as a spatial map: Preliminary evidence from unit activity in the freely-moving rat . Brain Research 34 : 171–175. [ PubMed ] [ Google Scholar ]
- O’Keefe J, Nadel L. (1978) The Hippocampus as a Cognitive Map . Oxford: Oxford University Press. [ Google Scholar ]
- Olton DS, Samuelson RJ. (1976) Remembrance of places passed: Spatial memory in rats . Journal of Experimental Psychology: Animal Behavior Processes 2 : 97–116. [ PubMed ] [ Google Scholar ]
- Orbach J, Milner B, Rasmussen T. (1960) Learning and retention in monkeys after amygdala-hippocampus resection . Archives of Neurology 3 : 230–251. [ PubMed ] [ Google Scholar ]
- Parsons RG, Ressler KJ. (2013) Implications of memory modulation for post-traumatic stress and fear disorders . Nature Neuroscience 16 : 146–153. [ PMC free article ] [ PubMed ] [ Google Scholar ]
- Posner MI, Raichle ME. (1994) Images of Mind . New York: Scientific American Library/Scientific American Books. [ Google Scholar ]
- Ranganath C, Ritchey M. (2012) Two cortical systems for memory-guided behavior . Nature Reviews Neuroscience 13 : 713–726. [ PubMed ] [ Google Scholar ]
- Redondo RL, Kim J, Arons AL, et al. (2014) Bidirectional switch of the valence associated with a hippocampal contextual memory engram . Nature 513 : 426–430. [ PMC free article ] [ PubMed ] [ Google Scholar ]
- Rissman J, Chow TE, Reggente N, et al. (2016) Decoding fMRI signatures of real-world autobiographical memory retrieval . Journal of Cognitive Neuroscience 28 : 604–620. [ PubMed ] [ Google Scholar ]
- Rockland KS. (2015) About connections . Frontiers in Neuroanatomy 9 : 61 DOI: 10.3389/fnana.2015.00061. [ PMC free article ] [ PubMed ] [ CrossRef ] [ Google Scholar ]
- Rolls ET. (2016) Cerebral Cortex: Principles of Operation . Oxford: Oxford University Press. [ Google Scholar ]
- Sahakian BJ, Morein-Zamir S. (2015) Pharmacological cognitive enhancement: Treatment of neuropsychiatric disorders and lifestyle use by healthy people . The Lancet Psychiatry 2 : 357–362. [ PubMed ] [ Google Scholar ]
- Schacter DL. (1992) Priming and multiple memory systems: Perceptual mechanisms of implicit memory . Journal of Cognitive Neuroscience 4 : 244–256. [ PubMed ] [ Google Scholar ]
- Schacter DL, Addis DR, Hassabis D, et al. (2012) The future of memory: Remembering, imagining, and the brain . Neuron 76 : 677–694. [ PMC free article ] [ PubMed ] [ Google Scholar ]
- Schacter DL, Chiu CYP, Ochsner KN. (1993) Implicit memory: A selective review . Annual Review of Neuroscience 16 : 159–182. [ PubMed ] [ Google Scholar ]
- Schultz W, Dickinson A. (2000) Neuronal coding of prediction errors . Annual Review Neuroscience 23 : 473–500. [ PubMed ] [ Google Scholar ]
- Scoville WB, Milner B. (1957) Loss of recent memory after bilateral hippocampal lesions . Journal of Neurology, Neurosurgery, and Psychiatry 20 : 11–21. [ PMC free article ] [ PubMed ] [ Google Scholar ]
- Silva AJ, Stevens CF, Tonegawa S, et al. (1992) Deficient hippocampal long-term potentiation in alpha-calcium-calmodulin kinase II mutant mice . Science 257 : 201–206. [ PubMed ] [ Google Scholar ]
- Somogyi P, Klausberger T. (2005) Defined types of cortical interneurone structure space and spike timing in the hippocampus . Journal of Physiology 562 : 9–26. [ PMC free article ] [ PubMed ] [ Google Scholar ]
- Squire LR, Zola-Morgan S. (1991) The medial temporal lobe memory system . Science 253 : 1380–1386. [ PubMed ] [ Google Scholar ]
- Squire LR, Wixted JT, Clark RE. (2007) Recognition memory and the medial temporal lobe: A new perspective . Nature Reviews Neuroscience 8 : 872–883. [ PMC free article ] [ PubMed ] [ Google Scholar ]
- Stevens CF. (1998) A million dollar question: Does LTP = memory? Neuron 20 : 1–2. [ PubMed ] [ Google Scholar ]
- Tonegawa S, Liu X, Ramirez S, et al. (2015) Memory engram cells have come of age . Neuron 87 : 918–931. [ PubMed ] [ Google Scholar ]
- Tulving E. (1972) Episodic and semantic memory . In: Tulving E, Donaldson W. (eds) Organization of Memory . New York: Academic Press, pp. 381–403. [ Google Scholar ]
- Vann SD, Tsivilis D, Denby CE, et al. (2009) Impaired recollection but spared familiarity in patients with extended hippocampal system damage revealed by 3 convergent methods . Proceedings of the National Academy of Sciences 106 : 5442–5447. [ PMC free article ] [ PubMed ] [ Google Scholar ]
- Weiskrantz L, Warrington EK. (1979) Conditioning in amnesic patients . Neuropsychologia 17 : 187–194. [ PubMed ] [ Google Scholar ]
- Wilde O. (1895) The Importance of Being Earnest, a Trivial Comedy for Serious People . [ Google Scholar ]
- Wright DB, Loftus EF. (1998) How misinformation alters memories . Journal of Experimental Child Psychology 71 : 155–164. [ PubMed ] [ Google Scholar ]
- Yonelinas AP. (2002) The nature of recollection and familiarity: A review of 30 years of research . Journal of Memory and Language 46 : 441–517. [ Google Scholar ]
- Zatorre RJ, Fields RD, Johansen-Berg H. (2012) Plasticity in gray and white: Neuroimaging changes in brain structure during learning . Nature Neuroscience 15 : 528–536. [ PMC free article ] [ PubMed ] [ Google Scholar ]
- Zeidman P, Maguire EA. (2016) Anterior hippocampus: The anatomy of perception, imagination and episodic memory . Nature Reviews Neuroscience 17 : 173–182. [ PMC free article ] [ PubMed ] [ Google Scholar ]
- Zola-Morgan S, Squire LR, Amaral DG. (1986) Human amnesia and the medial temporal region: Enduring memory impairment following a bilateral lesion limited to field CA1 of the hippocampus . Journal of Neuroscience 6 : 2950–2967. [ PMC free article ] [ PubMed ] [ Google Scholar ]
- UB Directory
- News Center >
- News Releases >
Research into the nature of memory reveals how cells that store information are stabilized over time

Dheeraj Roy, PhD, assistant professor in the Department of Physiology and Biophysics in the Jacobs School of Medicine and Biomedical Sciences at UB, is a senior author on a new paper that explains aspects of how memory works at the cellular level. ( Photo: Sandra Kicman)
Neuroscientists demonstrate how the brain improves its ability to distinguish between similar experiences, findings that could lead to treatments for Alzheimer’s disease and other memory disorders
By Ellen Goldbaum
Release Date: January 19, 2024
BUFFALO, N.Y. – Think of a time when you had two different but similar experiences in a short period. Maybe you attended two holiday parties in the same week or gave two presentations at work. Shortly afterward, you may find yourself confusing the two, but as time goes on that confusion recedes and you are better able to differentiate between these different experiences.
New research published in Nature Neuroscience today reveals how this process occurs on a cellular level, findings that are critical to the understanding and treatment of memory disorders, such as Alzheimer’s disease.
Dynamic engrams store memories
The research focuses on engrams, which are neuronal cells in the brain that store memory information. “Engrams are the neurons that are reactivated to support memory recall,” says Dheeraj S. Roy, PhD, one of the paper’s senior authors and an assistant professor in the Department of Physiology and Biophysics in the Jacobs School of Medicine and Biomedical Sciences at the University at Buffalo. “When engrams are disrupted, you get amnesia.”
In the minutes and hours that immediately follow an experience, he explains, the brain needs to consolidate the engram to store it. “We wanted to know: What is happening during this consolidation process? What happens between the time that an engram is formed and when you need to recall that memory later?”
The researchers developed a computational model for learning and memory formation that starts with sensory information, which is the stimulus. Once that information gets to the hippocampus, the part of the brain where memories form, different neurons are activated, some of which are excitatory and others that are inhibitory.
When neurons are activated in the hippocampus, not all are going to be firing at once. As memories form, neurons that happen to be activated closely in time become a part of the engram and strengthen their connectivity to support future recall.
“Activation of engram cells during memory recall is not an all or none process but rather typically needs to reach a threshold (i.e., a percentage of the original engram) for efficient recall,” Roy explains. “Our model is the first to demonstrate that the engram population is not stable: The number of engram cells that are activated during recall decreases with time, meaning they are dynamic in nature, and so the next critical question was whether this had a behavioral consequence.”
Dynamic engrams are needed for memory discrimination
“Over the consolidation period after learning, the brain is actively working to separate the two experiences and that’s possibly one reason why the numbers of activated engram cells decrease over time for a single memory,” he says. “If true, this would explain why memory discrimination gets better as time goes on. It’s like your memory of the experience was one big highway initially but over time, over the course of the consolidation period on the order of minutes to hours, your brain divides them into two lanes so you can discriminate between the two.”
Roy and the experimentalists on the team now had a testable hypothesis, which they carried out using a well-established behavioral experiment with mice. Mice were briefly exposed to two different boxes that had unique odors and lighting conditions; one was a neutral environment but in the second box, they received a mild foot shock.
A few hours after that experience, the mice, who typically are constantly moving, exhibited fear memory recall by freezing when exposed to either box. “That demonstrated that they couldn’t discriminate between the two,” Roy says. “But by hour twelve, all of a sudden, they exhibited fear only when they were exposed to the box where they were uncomfortable during their very first experience. They were able to discriminate between the two. The animal is telling us that they know this box is the scary one but five hours earlier they couldn’t do that.”
Using a light-sensitive technique, the team was able to detect active neurons in the mouse hippocampus as the animal was exploring the boxes. The researchers used this technique to tag active neurons and later measure how many were reactivated by the brain for recall. They also conducted experiments that allowed a single engram cell to be tracked across experiences and time. “So I can tell you literally how one engram cell or a subset of them responded to each environment across time and correlate this to their memory discrimination,” explains Roy.”
The team’s initial computational studies had predicted that the number of engram cells involved in a single memory would decrease over time, and the animal experiments bore that out.
“When the brain learns something for the first time, it doesn’t know how many neurons are needed and so on purpose a larger subset of neurons is recruited,” he explains. “As the brain stabilizes neurons, consolidating the memory, it cuts away the unnecessary neurons, so fewer are required and in doing so helps separate engrams for different memories.”
What is happening with memory disorders?
The findings have direct relevance to understanding what is going wrong in memory disorders, such as Alzheimer’s disease. Roy explains that to develop treatments for such disorders, it is critical to know what is happening during the initial memory formation, consolidation and activation of engrams for recall.
“This research tells us that a very likely candidate for why memory dysfunction occurs is that there is something wrong with the early window after memory formation where engrams must be changing,” says Roy.
He is currently studying mouse models of early Alzheimer’s disease to find out if engrams are forming but not being correctly stabilized. Now that more is known about how engrams work to form and stabilize memories, researchers can examine which genes are changing in the animal model when the engram population decreases.
“We can look at mouse models and ask, are there specific genes that are altered? And if so, then we finally have something to test, we can modulate the gene for these ‘ refinement’ or ‘consolidation’ processes of engrams to see if that has a role in improving memory performance,” he says.
Now at the Jacobs School, Roy conducted the research while a McGovern Fellow at the Broad Institute of Massachusetts Institute of Technology (MIT) and Harvard University. Roy is one of three neuroscientists recruited to the Jacobs School this year to launch a new focus on systems neuroscience in the school’s Department of Physiology and Biophysics.
Co-authors on the paper are from Imperial College in London; the Institute of Science and Technology in Austria; the McGovern Institute for Brain Research at MIT; and the Center for Life Sciences & IDG/McGovern Institute for Brain Research at Tsinghua University in China.
The work was funded by the President’s PhD Scholarship from Imperial College London; Wellcome Trust; the Biotechnology and Biological Sciences Research Council; the Simons Foundation; the Engineering and Physical Sciences Research Council; the School of Life Sciences and the IDG/McGovern Institute for Brain Research. Roy was supported by the Warren Alpert Distinguished Scholar Award and the National Institutes of Health.
Media Contact Information
Ellen Goldbaum News Content Manager Medicine Tel: 716-645-4605 [email protected]
Read the latest in your favorite channels.

Take UB With You. Wherever.
REVIEW article
Working memory from the psychological and neurosciences perspectives: a review.
- 1 Department of Neurosciences, School of Medical Sciences, Universiti Sains Malaysia, Kubang Kerian, Malaysia
- 2 Center for Neuroscience Services and Research, Universiti Sains Malaysia, Kubang Kerian, Malaysia
Since the concept of working memory was introduced over 50 years ago, different schools of thought have offered different definitions for working memory based on the various cognitive domains that it encompasses. The general consensus regarding working memory supports the idea that working memory is extensively involved in goal-directed behaviors in which information must be retained and manipulated to ensure successful task execution. Before the emergence of other competing models, the concept of working memory was described by the multicomponent working memory model proposed by Baddeley and Hitch. In the present article, the authors provide an overview of several working memory-relevant studies in order to harmonize the findings of working memory from the neurosciences and psychological standpoints, especially after citing evidence from past studies of healthy, aging, diseased, and/or lesioned brains. In particular, the theoretical framework behind working memory, in which the related domains that are considered to play a part in different frameworks (such as memory’s capacity limit and temporary storage) are presented and discussed. From the neuroscience perspective, it has been established that working memory activates the fronto-parietal brain regions, including the prefrontal, cingulate, and parietal cortices. Recent studies have subsequently implicated the roles of subcortical regions (such as the midbrain and cerebellum) in working memory. Aging also appears to have modulatory effects on working memory; age interactions with emotion, caffeine and hormones appear to affect working memory performances at the neurobiological level. Moreover, working memory deficits are apparent in older individuals, who are susceptible to cognitive deterioration. Another younger population with working memory impairment consists of those with mental, developmental, and/or neurological disorders such as major depressive disorder and others. A less coherent and organized neural pattern has been consistently reported in these disadvantaged groups. Working memory of patients with traumatic brain injury was similarly affected and shown to have unusual neural activity (hyper- or hypoactivation) as a general observation. Decoding the underlying neural mechanisms of working memory helps support the current theoretical understandings concerning working memory, and at the same time provides insights into rehabilitation programs that target working memory impairments from neurophysiological or psychological aspects.
Introduction
Working memory has fascinated scholars since its inception in the 1960’s ( Baddeley, 2010 ; D’Esposito and Postle, 2015 ). Indeed, more than a century of scientific studies revolving around memory in the fields of psychology, biology, or neuroscience have not completely agreed upon a unified categorization of memory, especially in terms of its functions and mechanisms ( Cowan, 2005 , 2008 ; Baddeley, 2010 ). From the coining of the term “memory” in the 1880’s by Hermann Ebbinghaus, to the distinction made between primary and secondary memory by William James in 1890, and to the now widely accepted and used categorizations of memory that include: short-term, long-term, and working memories, studies that have tried to decode and understand this abstract concept called memory have been extensive ( Cowan, 2005 , 2008 ). Short and long-term memory suggest that the difference between the two lies in the period that the encoded information is retained. Other than that, long-term memory has been unanimously understood as a huge reserve of knowledge about past events, and its existence in a functioning human being is without dispute ( Cowan, 2008 ). Further categorizations of long-term memory include several categories: (1) episodic; (2) semantic; (3) Pavlovian; and (4) procedural memory ( Humphreys et al., 1989 ). For example, understanding and using language in reading and writing demonstrates long-term storage of semantics. Meanwhile, short-term memory was defined as temporarily accessible information that has a limited storage time ( Cowan, 2008 ). Holding a string of meaningless numbers in the mind for brief delays reflects this short-term component of memory. Thus, the concept of working memory that shares similarities with short-term memory but attempts to address the oversimplification of short-term memory by introducing the role of information manipulation has emerged ( Baddeley, 2012 ). This article seeks to present an up-to-date introductory overview of the realm of working memory by outlining several working memory studies from the psychological and neurosciences perspectives in an effort to refine and unite the scientific knowledge concerning working memory.
The Multicomponent Working Memory Model
When one describes working memory, the multicomponent working memory model is undeniably one of the most prominent working memory models that is widely cited in literatures ( Baars and Franklin, 2003 ; Cowan, 2005 ; Chein et al., 2011 ; Ashkenazi et al., 2013 ; D’Esposito and Postle, 2015 ; Kim et al., 2015 ). Baddeley and Hitch (1974) proposed a working memory model that revolutionized the rigid and dichotomous view of memory as being short or long-term, although the term “working memory” was first introduced by Miller et al. (1960) . The working memory model posited that as opposed to the simplistic functions of short-term memory in providing short-term storage of information, working memory is a multicomponent system that manipulates information storage for greater and more complex cognitive utility ( Baddeley and Hitch, 1974 ; Baddeley, 1996 , 2000b ). The three subcomponents involved are phonological loop (or the verbal working memory), visuospatial sketchpad (the visual-spatial working memory), and the central executive which involves the attentional control system ( Baddeley and Hitch, 1974 ; Baddeley, 2000b ). It was not until 2000 that another component termed “episodic buffer” was introduced into this working memory model ( Baddeley, 2000a ). Episodic buffer was regarded as a temporary storage system that modulates and integrates different sensory information ( Baddeley, 2000a ). In short, the central executive functions as the “control center” that oversees manipulation, recall, and processing of information (non-verbal or verbal) for meaningful functions such as decision-making, problem-solving or even manuscript writing. In Baddeley and Hitch (1974) ’s well-cited paper, information received during the engagement of working memory can also be transferred to long-term storage. Instead of seeing working memory as merely an extension and a useful version of short-term memory, it appears to be more closely related to activated long-term memory, as suggested by Cowan (2005 , 2008 ), who emphasized the role of attention in working memory; his conjectures were later supported by Baddeley (2010) . Following this, the current development of the multicomponent working memory model could be retrieved from Baddeley’s article titled “Working Memory” published in Current Biology , in Figure 2 ( Baddeley, 2010 ).
An Embedded-Processes Model of Working Memory
Notwithstanding the widespread use of the multicomponent working memory model, Cowan (1999 , 2005 ) proposed the embedded-processes model that highlights the roles of long-term memory and attention in facilitating working memory functioning. Arguing that the Baddeley and Hitch (1974) model simplified perceptual processing of information presentation to the working memory store without considering the focus of attention to the stimuli presented, Cowan (2005 , 2010 ) stressed the pivotal and central roles of working memory capacity for understanding the working memory concept. According to Cowan (2008) , working memory can be conceptualized as a short-term storage component with a capacity limit that is heavily dependent on attention and other central executive processes that make use of stored information or that interact with long-term memory. The relationships between short-term, long-term, and working memory could be presented in a hierarchical manner whereby in the domain of long-term memory, there exists an intermediate subset of activated long-term memory (also the short-term storage component) and working memory belongs to the subset of activated long-term memory that is being attended to ( Cowan, 1999 , 2008 ). An illustration of Cowan’s theoretical framework on working memory can be traced back to Figure 1 in his paper titled “What are the differences between long-term, short-term, and working memory?” published in Progress in Brain Research ( Cowan, 2008 ).
Alternative Models
Cowan’s theoretical framework toward working memory is consistent with Engle (2002) ’s view, in which it was posited that working memory capacity is comparable to directed or held attention information inhibition. Indeed, in their classic study on reading span and reading comprehension, Daneman and Carpenter (1980) demonstrated that working memory capacity, which was believed to be reflected by the reading span task, strongly correlated with various comprehension tests. Surely, recent and continual growth in the memory field has also demonstrated the development of other models such as the time-based resource-sharing model proposed by several researchers ( Barrouillet et al., 2004 , 2009 ; Barrouillet and Camos, 2007 ). This model similarly demonstrated that cognitive load and working memory capacity that were so often discussed by working memory researchers were mainly a product of attention that one receives to allocate to tasks at hand ( Barrouillet et al., 2004 , 2009 ; Barrouillet and Camos, 2007 ). In fact, the allocated cognitive resources for a task (such as provided attention) and the duration of such allocation dictated the likelihood of success in performing the tasks ( Barrouillet et al., 2004 , 2009 ; Barrouillet and Camos, 2007 ). This further highlighted the significance of working memory in comparison with short-term memory in that, although information retained during working memory is not as long-lasting as long-term memory, it is not the same and deviates from short-term memory for it involves higher-order processing and executive cognitive controls that are not observed in short-term memory. A more detailed presentation of other relevant working memory models that shared similar foundations with Cowan’s and emphasized the roles of long-term memory can be found in the review article by ( D’Esposito and Postle, 2015 ).
In addition, in order to understand and compare similarities and disparities in different proposed models, about 20 years ago, Miyake and Shah (1999) suggested theoretical questions to authors of different models in their book on working memory models. The answers to these questions and presentations of models by these authors gave rise to a comprehensive definition of working memory proposed by Miyake and Shah (1999 , p. 450), “working memory is those mechanisms or processes that are involved in the control, regulation, and active maintenance of task-relevant information in the service of complex cognition, including novel as well as familiar, skilled tasks. It consists of a set of processes and mechanisms and is not a fixed ‘place’ or ‘box’ in the cognitive architecture. It is not a completely unitary system in the sense that it involves multiple representational codes and/or different subsystems. Its capacity limits reflect multiple factors and may even be an emergent property of the multiple processes and mechanisms involved. Working memory is closely linked to LTM, and its contents consist primarily of currently activated LTM representations, but can also extend to LTM representations that are closely linked to activated retrieval cues and, hence, can be quickly activated.” That said, in spite of the variability and differences that have been observed following the rapid expansion of working memory understanding and its range of models since the inception of the multicomponent working memory model, it is worth highlighting that the roles of executive processes involved in working memory are indisputable, irrespective of whether different components exist. Such notion is well-supported as Miyake and Shah, at the time of documenting the volume back in the 1990’s, similarly noted that the mechanisms of executive control were being heavily investigated and emphasized ( Miyake and Shah, 1999 ). In particular, several domains of working memory such as the focus of attention ( Cowan, 1999 , 2008 ), inhibitory controls ( Engle and Kane, 2004 ), maintenance, manipulation, and updating of information ( Baddeley, 2000a , 2010 ), capacity limits ( Cowan, 2005 ), and episodic buffer ( Baddeley, 2000a ) were executive processes that relied on executive control efficacy (see also Miyake and Shah, 1999 ; Barrouillet et al., 2004 ; D’Esposito and Postle, 2015 ).
The Neuroscience Perspective
Following such cognitive conceptualization of working memory developed more than four decades ago, numerous studies have intended to tackle this fascinating working memory using various means such as decoding its existence at the neuronal level and/or proposing different theoretical models in terms of neuronal activity or brain activation patterns. Table 1 offers the summarized findings of these literatures. From the cognitive neuroscientific standpoint, for example, the verbal and visual-spatial working memories were examined separately, and the distinction between the two forms was documented through studies of patients with overt impairment in short-term storage for different verbal or visual tasks ( Baddeley, 2000b ). Based on these findings, associations or dissociations with the different systems of working memory (such as phonological loops and visuospatial sketchpad) were then made ( Baddeley, 2000b ). It has been established that verbal and acoustic information activates Broca’s and Wernicke’s areas while visuospatial information is represented in the right hemisphere ( Baddeley, 2000b ). Not surprisingly, many supporting research studies have pointed to the fronto-parietal network involving the dorsolateral prefrontal cortex (DLPFC), the anterior cingulate cortex (ACC), and the parietal cortex (PAR) as the working memory neural network ( Osaka et al., 2003 ; Owen et al., 2005 ; Chein et al., 2011 ; Kim et al., 2015 ). More precisely, the DLPFC has been largely implicated in tasks demanding executive control such as those requiring integration of information for decision-making ( Kim et al., 2015 ; Jimura et al., 2017 ), maintenance and manipulation/retrieval of stored information or relating to taxing loads (such as capacity limit) ( Osaka et al., 2003 ; Moore et al., 2013 ; Vartanian et al., 2013 ; Rodriguez Merzagora et al., 2014 ), and information updating ( Murty et al., 2011 ). Meanwhile, the ACC has been shown to act as an “attention controller” that evaluates the needs for adjustment and adaptation of received information based on task demands ( Osaka et al., 2003 ), and the PAR has been regarded as the “workspace” for sensory or perceptual processing ( Owen et al., 2005 ; Andersen and Cui, 2009 ). Figure 1 attempted to translate the theoretical formulation of the multicomponent working memory model ( Baddeley, 2010 ) to specific regions in the human brain. It is, however, to be acknowledged that the current neuroscientific understanding on working memory adopted that working memory, like other cognitive systems, involves the functional integration of the brain as a whole; and to clearly delineate its roles into multiple components with only a few regions serving as specific buffers was deemed impractical ( D’Esposito and Postle, 2015 ). Nonetheless, depicting the multicomponent working memory model in the brain offers a glimpse into the functional segregation of working memory.
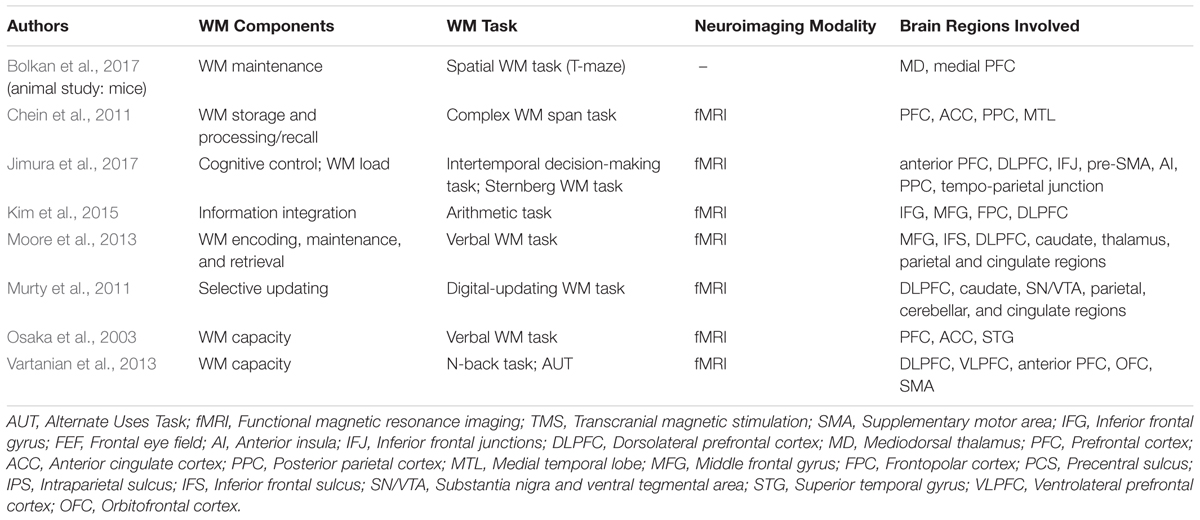
TABLE 1. Working memory (WM) studies in the healthy brain.
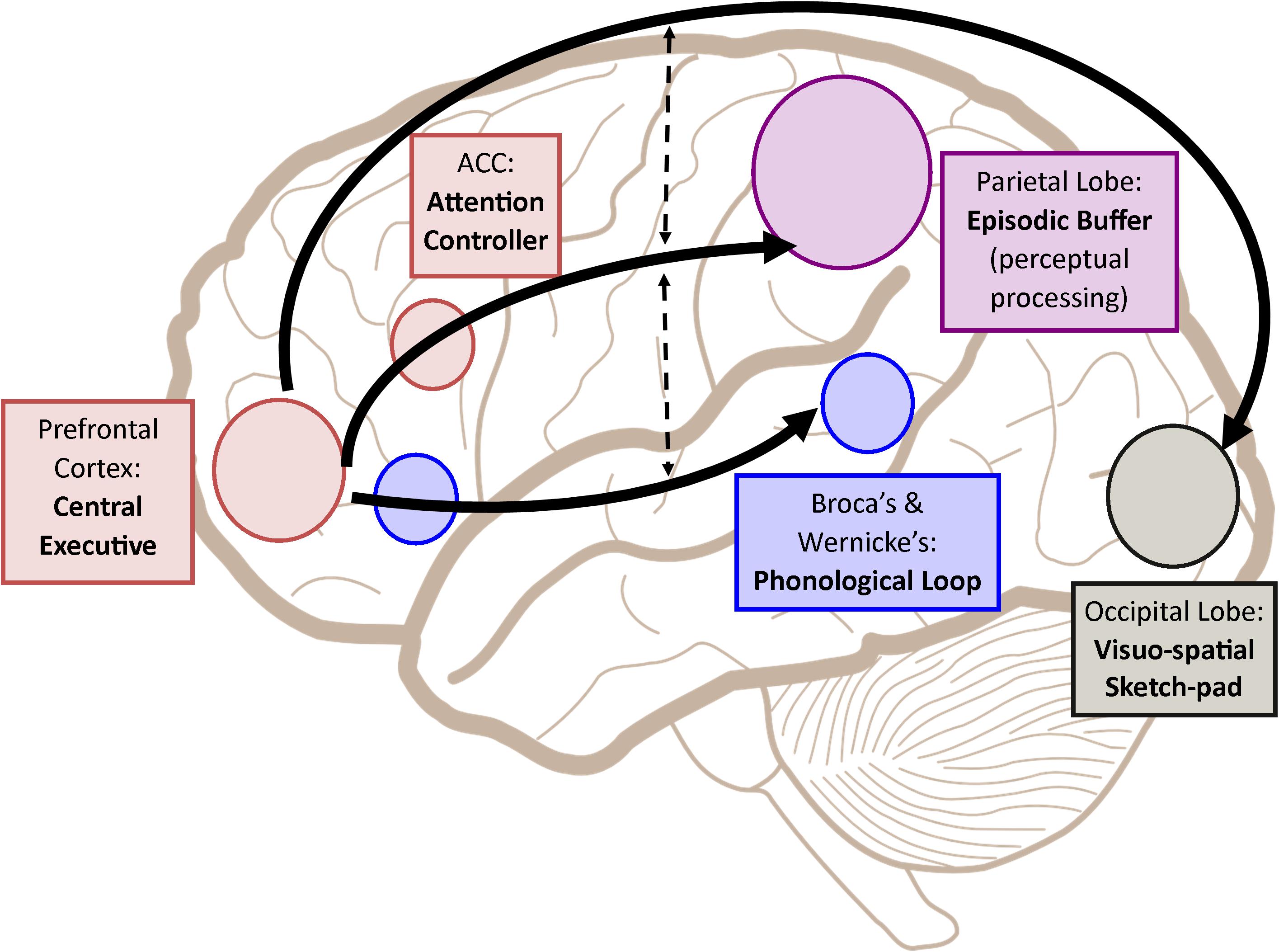
FIGURE 1. A simplified depiction (adapted from the multicomponent working memory model by Baddeley, 2010 ) as implicated in the brain, in which the central executive assumes the role to exert control and oversee the manipulation of incoming information for intended execution. ACC, Anterior cingulate cortex.
Further investigation has recently revealed that other than the generally informed cortical structures involved in verbal working memory, basal ganglia, which lies in the subcortical layer, plays a role too ( Moore et al., 2013 ). Particularly, the caudate and thalamus were activated during task encoding, and the medial thalamus during the maintenance phase, while recorded activity in the fronto-parietal network, which includes the DLPFC and the parietal lobules, was observed only during retrieval ( Moore et al., 2013 ). These findings support the notion that the basal ganglia functions to enhance focusing on a target while at the same time suppressing irrelevant distractors during verbal working memory tasks, which is especially crucial at the encoding phase ( Moore et al., 2013 ). Besides, a study conducted on mice yielded a similar conclusion in which the mediodorsal thalamus aided the medial prefrontal cortex in the maintenance of working memory ( Bolkan et al., 2017 ). In another study by Murty et al. (2011) in which information updating, which is one of the important aspects of working memory, was investigated, the midbrain including the substantia nigra/ventral tegmental area and caudate was activated together with DLPFC and other parietal regions. Taken together, these studies indicated that brain activation of working memory are not only limited to the cortical layer ( Murty et al., 2011 ; Moore et al., 2013 ). In fact, studies on cerebellar lesions subsequently discovered that patients suffered from impairments in attention-related working memory or executive functions, suggesting that in spite of the motor functions widely attributed to the cerebellum, the cerebellum is also involved in higher-order cognitive functions including working memory ( Gottwald et al., 2004 ; Ziemus et al., 2007 ).
Shifting the attention to the neuronal network involved in working memory, effective connectivity analysis during engagement of a working memory task reinforced the idea that the DLPFC, PAR and ACC belong to the working memory circuitry, and bidirectional endogenous connections between all these regions were observed in which the left and right PAR were the modeled input regions ( Dima et al., 2014 ) (refer to Supplementary Figure 1 in Dima et al., 2014 ). Effective connectivity describes the attempt to model causal influence of neuronal connections in order to better understand the hidden neuronal states underlying detected neuronal responses ( Friston et al., 2013 ). Another similar study of working memory using an effective connectivity analysis that involved more brain regions, including the bilateral middle frontal gyrus (MFG), ACC, inferior frontal cortex (IFC), and posterior parietal cortex (PPC) established the modulatory effect of working memory load in this fronto-parietal network with memory delay as the driving input to the bilateral PPC ( Ma et al., 2012 ) (refer to Figure 1 in Ma et al., 2012 ).
Moving away from brain regions activated but toward the in-depth neurobiological side of working memory, it has long been understood that the limited capacity of working memory and its transient nature, which are considered two of the defining characteristics of working memory, indicate the role of persistent neuronal firing (see Review Article by D’Esposito and Postle, 2015 ; Zylberberg and Strowbridge, 2017 ; see also Silvanto, 2017 ), that is, continuous action potentials are generated in neurons along the neural network. However, this view was challenged when activity-silent synaptic mechanisms were found to also be involved ( Mongillo et al., 2008 ; Rose et al., 2016 ; see also Silvanto, 2017 ). Instead of holding relevant information through heightened and persistent neuronal firing, residual calcium at the presynaptic terminals was suggested to have mediated the working memory process ( Mongillo et al., 2008 ). This synaptic theory was further supported when TMS application produced a reactivation effect of past information that was not needed or attended at the conscious level, hence the TMS application facilitated working memory efficacy ( Rose et al., 2016 ). As it happens, this provided evidence from the neurobiological viewpoint to support Cowan’s theorized idea of “activated long-term memory” being a feature of working memory as non-cued past items in working memory that were assumed to be no longer accessible were actually stored in a latent state and could be brought back into consciousness. However, the researchers cautioned the use of the term “activated long-term memory” and opted for “prioritized long-term memory” because these unattended items maintained in working memory seemed to employ a different mechanism than items that were dropped from working memory ( Rose et al., 2016 ). Other than the synaptic theory, the spiking working memory model proposed by Fiebig and Lansner (2017) that borrowed the concept from fast Hebbian plasticity similarly disagreed with persistent neuronal activity and demonstrated that working memory processes were instead manifested in discrete oscillatory bursts.
Age and Working Memory
Nevertheless, having established a clear working memory circuitry in the brain, differences in brain activations, neural patterns or working memory performances are still apparent in different study groups, especially in those with diseased or aging brains. For a start, it is well understood that working memory declines with age ( Hedden and Gabrieli, 2004 ; Ziaei et al., 2017 ). Hence, older participants are expected to perform poorer on a working memory task when making comparison with relatively younger task takers. In fact, it was reported that decreases in cortical surface area in the frontal lobe of the right hemisphere was associated with poorer performers ( Nissim et al., 2017 ). In their study, healthy (those without mild cognitive impairments [MCI] or neurodegenerative diseases such as dementia or Alzheimer’s) elderly people with an average age of 70 took the n-back working memory task while magnetic resonance imaging (MRI) scans were obtained from them ( Nissim et al., 2017 ). The outcomes exhibited that a decrease in cortical surface areas in the superior frontal gyrus, pars opercularis of the inferior frontal gyrus, and medial orbital frontal gyrus that was lateralized to the right hemisphere, was significantly detected among low performers, implying an association between loss of brain structural integrity and working memory performance ( Nissim et al., 2017 ). There was no observed significant decline in cortical thickness of the studied brains, which is assumed to implicate neurodegenerative tissue loss ( Nissim et al., 2017 ).
Moreover, another extensive study that examined cognitive functions of participants across the lifespan using functional magnetic resonance imaging (fMRI) reported that the right lateralized fronto-parietal regions in addition to the ventromedial prefrontal cortex (VMPFC), posterior cingulate cortex, and left angular and middle frontal gyri (the default mode regions) in older adults showed reduced modulation of task difficulty, which was reflective of poorer task performance ( Rieck et al., 2017 ). In particular, older-age adults (55–69 years) exhibited diminished brain activations (positive modulation) as compared to middle-age adults (35–54 years) with increasing task difficulty, whereas lesser deactivation (negative modulation) was observed between the transition from younger adults (20–34 years) to middle-age adults ( Rieck et al., 2017 ). This provided insights on cognitive function differences during an individual’s lifespan at the neurobiological level, which hinted at the reduced ability or efficacy of the brain to modulate functional regions to increased difficulty as one grows old ( Rieck et al., 2017 ). As a matter of fact, such an opinion was in line with the Compensation-Related Utilization of Neural Circuits Hypothesis (CRUNCH) proposed by Reuter-Lorenz and Cappell (2008) . The CRUNCH likewise agreed upon reduced neural efficiency in older adults and contended that age-associated cognitive decline brought over-activation as a compensatory mechanism; yet, a shift would occur as task loads increase and under-activation would then be expected because older adults with relatively lesser cognitive resources would max out their ‘cognitive reserve’ sooner than younger adults ( Reuter-Lorenz and Park, 2010 ; Schneider-Garces et al., 2010 ).
In addition to those findings, emotional distractors presented during a working memory task were shown to alter or affect task performance in older adults ( Oren et al., 2017 ; Ziaei et al., 2017 ). Based on the study by Oren et al. (2017) who utilized the n-back task paired with emotional distractors with neutral or negative valence in the background, negative distractors with low load (such as 1-back) resulted in shorter response time (RT) in the older participants ( M age = 71.8), although their responses were not significantly more accurate when neutral distractors were shown. Also, lesser activations in the bilateral MFG, VMPFC, and left PAR were reported in the old-age group during negative low load condition. This finding subsequently demonstrated the results of emotional effects on working memory performance in older adults ( Oren et al., 2017 ). Further functional connectivity analyses revealed that the amygdala, the region well-known to be involved in emotional processing, was deactivated and displayed similar strength in functional connectivity regardless of emotional or load conditions in the old-age group ( Oren et al., 2017 ). This finding went in the opposite direction of that observed in the younger group in which the amygdala was strongly activated with less functional connections to the bilateral MFG and left PAR ( Oren et al., 2017 ). This might explain the shorter reported RT, which was an indication of improved working memory performance, during the emotional working memory task in the older adults as their amygdala activation was suppressed as compared to the younger adults ( Oren et al., 2017 ).
Interestingly, a contrasting neural connection outcome was reported in the study by Ziaei et al. (2017) in which differential functional networks relating to emotional working memory task were employed by the two studied groups: (1) younger ( M age = 22.6) and (2) older ( M age = 68.2) adults. In the study, emotional distractors with positive, neutral, and negative valence were presented during a visual working memory task and older adults were reported to adopt two distinct networks involving the VMPFC to encode and process positive and negative distractors while younger adults engaged only one neural pathway ( Ziaei et al., 2017 ). The role of amygdala engagement in processing only negative items in the younger adults, but both negative and positive distractors in the older adults, could be reflective of the older adults’ better ability at regulating negative emotions which might subsequently provide a better platform for monitoring working memory performance and efficacy as compared to their younger counterparts ( Ziaei et al., 2017 ). This study’s findings contradict those by Oren et al. (2017) in which the amygdala was found to play a bigger role in emotional working memory tasks among older participants as opposed to being suppressed as reported by Oren et al. (2017) . Nonetheless, after overlooking the underlying neural mechanism relating to emotional distractors, it was still agreed that effective emotional processing sustained working memory performance among older/elderly people ( Oren et al., 2017 ; Ziaei et al., 2017 ).
Aside from the interaction effect between emotion and aging on working memory, the impact of caffeine was also investigated among elders susceptible to age-related cognitive decline; and those reporting subtle cognitive deterioration 18-months after baseline measurement showed less marked effects of caffeine in the right hemisphere, unlike those with either intact cognitive ability or MCI ( Haller et al., 2017 ). It was concluded that while caffeine’s effects were more pronounced in MCI participants, elders in the early stages of cognitive decline displayed diminished sensitivity to caffeine after being tested with the n-back task during fMRI acquisition ( Haller et al., 2017 ). It is, however, to be noted that the working memory performance of those displaying minimal cognitive deterioration was maintained even though their brain imaging uncovered weaker brain activation in a more restricted area ( Haller et al., 2017 ). Of great interest, such results might present a useful brain-based marker that can be used to identify possible age-related cognitive decline.
Similar findings that demonstrated more pronounced effects of caffeine on elderly participants were reported in an older study, whereas older participants in the age range of 50–65 years old exhibited better working memory performance that offset the cognitive decline observed in those with no caffeine consumption, in addition to displaying shorter reaction times and better motor speeds than observed in those without caffeine ( Rees et al., 1999 ). Animal studies using mice showed replication of these results in mutated mice models of Alzheimer’s disease or older albino mice, both possibly due to the reported results of reduced amyloid production or brain-derived neurotrophic factor and tyrosine-kinase receptor. These mice performed significantly better after caffeine treatment in tasks that supposedly tapped into working memory or cognitive functions ( Arendash et al., 2006 ). Such direct effects of caffeine on working memory in relation to age was further supported by neuroimaging studies ( Haller et al., 2013 ; Klaassen et al., 2013 ). fMRI uncovered increased brain activation in regions or networks of working memory, including the fronto-parietal network or the prefrontal cortex in old-aged ( Haller et al., 2013 ) or middle-aged adults ( Klaassen et al., 2013 ), even though the behavioral measures of working memory did not differ. Taken together, these outcomes offered insight at the neurobiological level in which caffeine acts as a psychoactive agent that introduces changes and alters the aging brain’s biological environment that explicit behavioral testing might fail to capture due to performance maintenance ( Haller et al., 2013 , 2017 ; Klaassen et al., 2013 ).
With respect to physiological effects on cognitive functions (such as effects of caffeine on brain physiology), estradiol, the primary female sex hormone that regulates menstrual cycles, was found to also modulate working memory by engaging different brain activity patterns during different phases of the menstrual cycle ( Joseph et al., 2012 ). The late follicular (LF) phase of the menstrual cycle, characterized by high estradiol levels, was shown to recruit more of the right hemisphere that was associated with improved working memory performance than did the early follicular (EF) phase, which has lower estradiol levels although overall, the direct association between estradiol levels and working memory was inconclusive ( Joseph et al., 2012 ). The finding that estradiol levels modified brain recruitment patterns at the neurobiological level, which could indirectly affect working memory performance, presents implications that working memory impairment reported in post-menopausal women (older aged women) could indicate a link with estradiol loss ( Joseph et al., 2012 ). In 2000, post-menopausal women undergoing hormone replacement therapy, specifically estrogen, were found to have better working memory performance in comparison with women who took estrogen and progestin or women who did not receive the therapy ( Duff and Hampson, 2000 ). Yet, interestingly, a study by Janowsky et al. (2000) showed that testosterone supplementation counteracted age-related working memory decline in older males, but a similar effect was not detected in older females who were supplemented with estrogen. A relatively recent paper might have provided the explanation to such contradicting outcomes ( Schöning et al., 2007 ). As demonstrated in the study using fMRI, the nature of the task (such as verbal or visual-spatial) might have played a role as a higher level of testosterone (in males) correlated with activations of the left inferior parietal cortex, which was deemed a key region in spatial processing that subsequently brought on better performance in a mental-rotation task. In contrast, significant correlation between estradiol and other cortical activations in females in the midluteal phase, who had higher estradiol levels, did not result in better performance of the task compared to women in the EF phase or men ( Schöning et al., 2007 ). Nonetheless, it remains premature to conclude that age-related cognitive decline was a result of hormonal (estradiol or testosterone) fluctuations although hormones might have modulated the effect of aging on working memory.
Other than the presented interaction effects of age and emotions, caffeine, and hormones, other studies looked at working memory training in the older population in order to investigate working memory malleability in the aging brain. Findings of improved performance for the same working memory task after training were consistent across studies ( Dahlin et al., 2008 ; Borella et al., 2017 ; Guye and von Bastian, 2017 ; Heinzel et al., 2017 ). Such positive results demonstrated effective training gains regardless of age difference that could even be maintained until 18 months later ( Dahlin et al., 2008 ) even though the transfer effects of such training to other working memory tasks need to be further elucidated as strong evidence of transfer with medium to large effect size is lacking ( Dahlin et al., 2008 ; Guye and von Bastian, 2017 ; Heinzel et al., 2017 ; see also Karbach and Verhaeghen, 2014 ). The studies showcasing the effectiveness of working memory training presented a useful cognitive intervention that could partially stall or delay cognitive decline. Table 2 presents an overview of the age-related working memory studies.
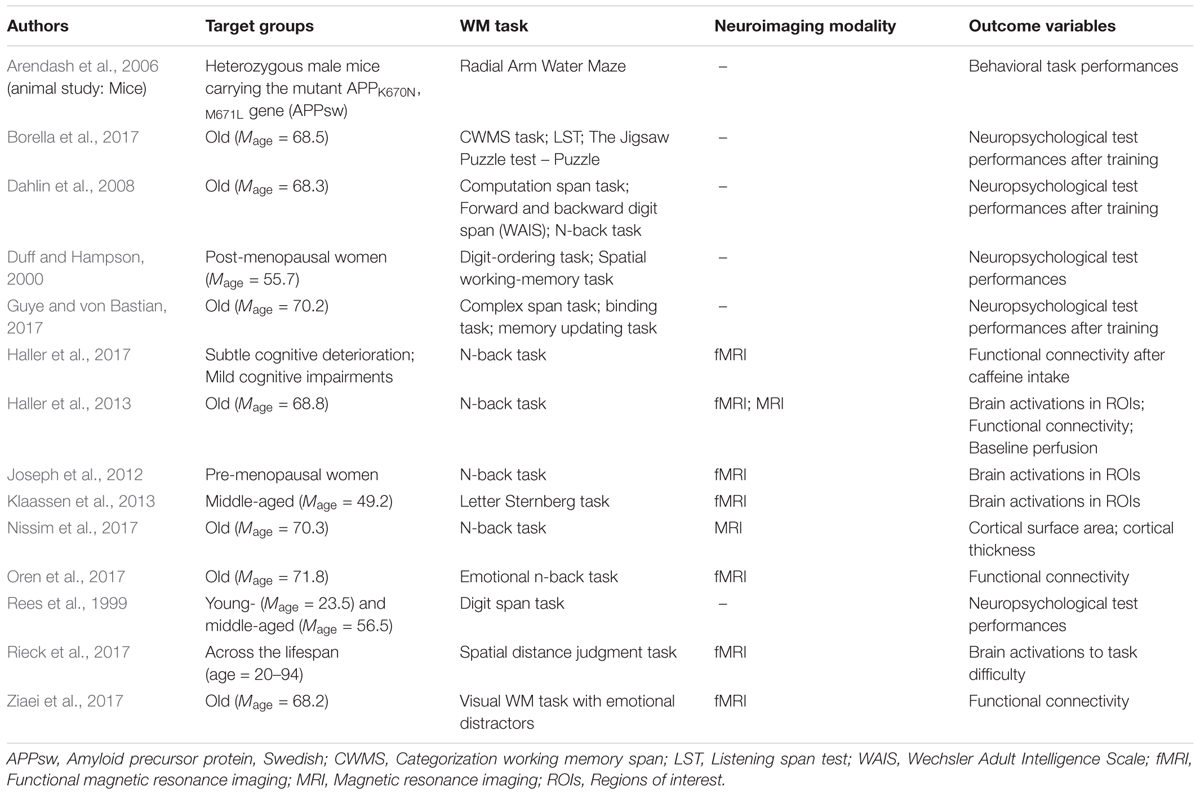
TABLE 2. Working memory (WM) studies in relation to age.
The Diseased Brain and Working Memory
Age is not the only factor influencing working memory. In recent studies, working memory deficits in populations with mental or neurological disorders were also being investigated (see Table 3 ). Having identified that the working memory circuitry involves the fronto-parietal region, especially the prefrontal and parietal cortices, in a healthy functioning brain, targeting these areas in order to understand how working memory is affected in a diseased brain might provide an explanation for the underlying deficits observed at the behavioral level. For example, it was found that individuals with generalized or social anxiety disorder exhibited reduced DLPFC activation that translated to poorer n-back task performance in terms of accuracy and RT when compared with the controls ( Balderston et al., 2017 ). Also, VMPFC and ACC, representing the default mode network (DMN), were less inhibited in these individuals, indicating that cognitive resources might have been divided and resulted in working memory deficits due to the failure to disengage attention from persistent anxiety-related thoughts ( Balderston et al., 2017 ). Similar speculation can be made about individuals with schizophrenia. Observed working memory deficits might be traced back to impairments in the neural networks that govern attentional-control and information manipulation and maintenance ( Grot et al., 2017 ). The participants performed a working memory binding task, whereby they had to make sure that the word-ellipse pairs presented during the retrieval phase were identical to those in the encoding phase in terms of location and verbal information; results concluded that participants with schizophrenia had an overall poorer performance compared to healthy controls when they were asked to actively bind verbal and spatial information ( Grot et al., 2017 ). This was reflected in the diminished activation in the schizophrenia group’s ventrolateral prefrontal cortex and the PPC that were said to play a role in manipulation and reorganization of information during encoding and maintenance of information after encoding ( Grot et al., 2017 ).
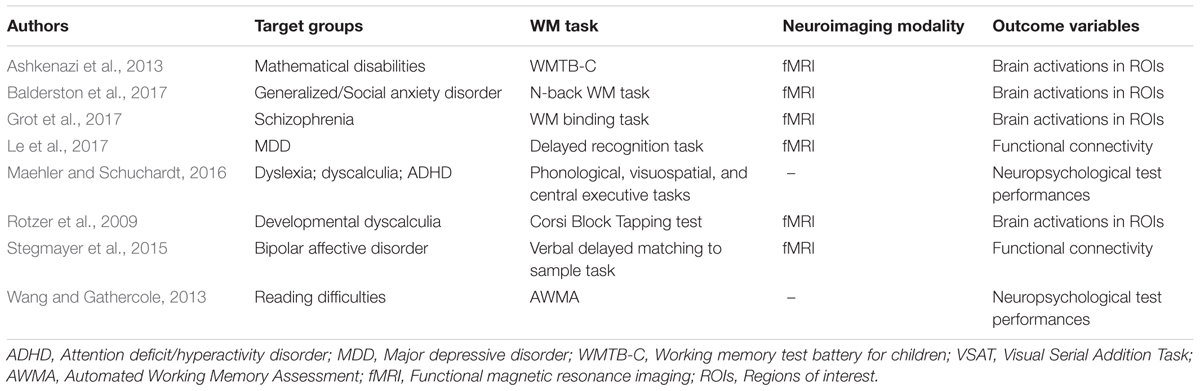
TABLE 3. Working memory (WM) studies in the diseased brain.
In addition, patients with major depressive disorder (MDD) displayed weaker performance in the working memory updating domain in which information manipulation was needed when completing a visual working memory task ( Le et al., 2017 ). The working memory task employed in the study was a delayed recognition task that required participants to remember and recognize the faces or scenes as informed after stimuli presentation while undergoing fMRI scan ( Le et al., 2017 ). Subsequent functional connectivity analyses revealed that the fusiform face area (FFA), parahippocampal place area (PPA), and left MFG showed aberrant activity in the MDD group as compared to the control group ( Le et al., 2017 ). These brain regions are known to be the visual association area and the control center of working memory and have been implicated in visual working memory updating in healthy adults ( Le et al., 2017 ). Therefore, altered visual cortical functions and load-related activation in the prefrontal cortex in the MDD group implied that the cognitive control for visual information processing and updating might be impaired at the input or control level, which could have ultimately played a part in the depressive symptoms ( Le et al., 2017 ).
Similarly, during a verbal delayed match to sample task that asked participants to sub-articulatorly rehearse presented target letters for subsequent letter-matching, individuals with bipolar affective disorder displayed aberrant neural interactions between the right amygdala, which is part of the limbic system implicated in emotional processing as previously described, and ipsilateral cortical regions often concerned with verbal working memory, pointing out that the cortico-amygdalar connectivity was disrupted, which led to verbal working memory deficits ( Stegmayer et al., 2015 ). As an attempt to gather insights into previously reported hyperactivation in the amygdala in bipolar affective disorder during an articulatory working memory task, functional connectivity analyses revealed that negative functional interactions seen in healthy controls were not replicated in patients with bipolar affective disorder ( Stegmayer et al., 2015 ). Consistent with the previously described study about emotional processing effects on working memory in older adults, this reported outcome was suggestive of the brain’s failed attempts to suppress pathological amygdalar activation during a verbal working memory task ( Stegmayer et al., 2015 ).
Another affected group with working memory deficits that has been the subject of research interest was children with developmental disorders such as attention deficit/hyperactivity disorder (ADHD), developmental dyscalculia, and reading difficulties ( Rotzer et al., 2009 ; Ashkenazi et al., 2013 ; Wang and Gathercole, 2013 ; Maehler and Schuchardt, 2016 ). For instance, looking into the different working memory subsystems based on Baddeley’s multicomponent working memory model in children with dyslexia and/or ADHD and children with dyscalculia and/or ADHD through a series of tests, it was reported that distinctive working memory deficits by groups could be detected such that phonological loop (e.g., digit span) impairment was observed in the dyslexia group, visuospatial sketchpad (e.g., Corsi block tasks) deficits in the dyscalculia group, while central executive (e.g., complex counting span) deficits in children with ADHD ( Maehler and Schuchardt, 2016 ). Meanwhile, examination of working memory impairment in a delayed match-to-sample visual task that put emphasis on the maintenance phase of working memory by examining the brainwaves of adults with ADHD using electroencephalography (EEG) also revealed a marginally significantly lower alpha band power in the posterior regions as compared to healthy individuals, and such an observation was not significantly improved after working memory training (Cogmed working memory training, CWMT Program) ( Liu et al., 2016 ). The alpha power was considered important in the maintenance of working memory items; and lower working memory accuracy paired with lower alpha band power was indeed observed in the ADHD group ( Liu et al., 2016 ).
Not dismissing the above compiled results, children encountering disabilities in mathematical operations likewise indicated deficits in the working memory domain that were traceable to unusual brain activities at the neurobiological level ( Rotzer et al., 2009 ; Ashkenazi et al., 2013 ). It was speculated that visuospatial working memory plays a vital role when arithmetic problem-solving is involved in order to ensure intact mental representations of the numerical information ( Rotzer et al., 2009 ). Indeed, Ashkenazi et al. (2013) revealed that Block Recall, a variant of the Corsi Block Tapping test and a subtest of the Working Memory Test Battery for Children (WMTB-C) that explored visuospatial sketchpad ability, was significantly predictive of math abilities. In relation to this, studies investigating brain activation patterns and performance of visuospatial working memory task in children with mathematical disabilities identified the intraparietal sulcus (IPS), in conjunction with other regions in the prefrontal and parietal cortices, to have less activation when visuospatial working memory was deemed involved (during an adapted form of Corsi Block Tapping test made suitable for fMRI [ Rotzer et al., 2009 ]); in contrast the control group demonstrated correlations of the IPS in addition to the fronto-parietal cortical activation with the task ( Rotzer et al., 2009 ; Ashkenazi et al., 2013 ). These brain activity variations that translated to differences in overt performances between healthily developing individuals and those with atypical development highlighted the need for intervention and attention for the disadvantaged groups.
Traumatic Brain Injury and Working Memory
Physical injuries impacting the frontal or parietal lobes would reasonably be damaging to one’s working memory. This is supported in studies employing neuropsychological testing to assess cognitive impairments in patients with traumatic brain injury; and poorer cognitive performances especially involving the working memory domains were reported (see Review Articles by Dikmen et al., 2009 ; Dunning et al., 2016 ; Phillips et al., 2017 ). Research on cognitive deficits in traumatic brain injury has been extensive due to the debilitating conditions brought upon an individual daily life after the injury. Traumatic brain injuries (TBI) refer to accidental damage to the brain after being hit by an object or following rapid acceleration or deceleration ( Farrer, 2017 ). These accidents include falls, assaults, or automobile accidents and patients with TBI can be then categorized into three groups; (1) mild TBI with GCS – Glasgow Coma Scale – score of 13–15; (2) moderate TBI with GCS score of 9–12; and (3) severe TBI with GCS score of 3–8 ( Farrer, 2017 ). In a recently published meta-analysis that specifically looked at working memory impairments in patients with moderate to severe TBI, patients displayed reduced cognitive functions in verbal short-term memory in addition to verbal and visuospatial working memory in comparison to control groups ( Dunning et al., 2016 ). It was also understood from the analysis that the time lapse since injury and age of injury were deciding factors that influenced these cognitive deficits in which longer time post-injury or older age during injury were associated with greater cognitive decline ( Dunning et al., 2016 ).
Nonetheless, it is to be noted that such findings relating to age of injury could not be generalized to the child population since results from the pediatric TBI cases showed that damage could negatively impact developmental skills that could indicate a greater lag in cognitive competency as the child’s frontal lobe had yet to mature ( Anderson and Catroppa, 2007 ; Mandalis et al., 2007 ; Nadebaum et al., 2007 ; Gorman et al., 2012 ). These studies all reported working memory impairment of different domains such as attentional control, executive functions, or verbal and visuospatial working memory in the TBI group, especially for children with severe TBI ( Mandalis et al., 2007 ; Nadebaum et al., 2007 ; Gorman et al., 2012 ). Investigation of whether working memory deficits are domain-specific or -general or involve one or more mechanisms, has yielded inconsistent results. For example, Perlstein et al. (2004) found that working memory was impaired in the TBI group only when complex manipulation such as sequential coding of information is required and not accounted for by processing speed or maintenance of information, but two teams of researchers ( Perbal et al., 2003 ; Gorman et al., 2012 ) suggested otherwise. From their study on timing judgments, Perbal et al. (2003) concluded that deficits were not related to time estimation but more on generalized attentional control, working memory and processing speed problems; while Gorman et al. (2012) also attributed the lack of attentional focus to impairments observed during the working memory task. In fact, in a later study by Gorman et al. (2016) , it was shown that processing speed mediated TBI effects on working memory even though the mediation was partial. On the other hand, Vallat-Azouvi et al. (2007) reported impairments in the working memory updating domain that came with high executive demands for TBI patients. Also, Mandalis et al. (2007) similarly highlighted potential problems with attention and taxing cognitive demands in the TBI group.
From the neuroscientific perspective, hyper-activation or -connectivity in the working memory circuitry was reported in TBI patients in comparison with healthy controls when both groups engaged in working memory tasks, suggesting that the brain attempted to compensate for or re-establish lost connections upon the injury ( Dobryakova et al., 2015 ; Hsu et al., 2015 ; Wylie et al., 2015 ). For a start, it was observed that participants with mild TBI displayed increased activation in the right prefrontal cortex during a working memory task when comparing to controls ( Wylie et al., 2015 ). Interestingly, this activation pattern only occurred in patients who did not experience a complete recovery 1 week after the injury ( Wylie et al., 2015 ). Besides, low activation in the DMN was observed in mild TBI patients without cognitive recovery, and such results seemed to be useful in predicting recovery in patients in which the patients did not recover when hypoactivation (low activation) was reported, and vice versa ( Wylie et al., 2015 ). This might be suggestive of the potential of cognitive recovery simply by looking at the intensity of brain activation of the DMN, for an increase in activation of the DMN seemed to be superseded before cognitive recovery was present ( Wylie et al., 2015 ).
In fact, several studies lent support to the speculation mentioned above as hyperactivation or hypoactivation in comparison with healthy participants was similarly identified. When sex differences were being examined in working memory functional activity in mild TBI patients, hyperactivation was reported in male patients when comparing to the male control group, suggesting that the hyperactivation pattern might be the brain’s attempt at recovering impaired functions; even though hypoactivation was shown in female patients as compared to the female control group ( Hsu et al., 2015 ). The researchers from the study further explained that such hyperactivation after the trauma acted as a neural compensatory mechanism so that task performance could be maintained while hypoactivation with a poorer performance could have been the result of a more severe injury ( Hsu et al., 2015 ). Therefore, the decrease in activation in female patients, in addition to the observed worse performance, was speculated to be due to a more serious injury sustained by the female patients group ( Hsu et al., 2015 ).
In addition, investigation of the effective connectivity of moderate and severe TBI participants during a working memory task revealed that the VMPFC influenced the ACC in these TBI participants when the opposite was observed in healthy subjects ( Dobryakova et al., 2015 ). Moreover, increased inter-hemispheric transfer due to an increased number of connections between the left and right hemispheres (hyper-connectivity) without clear directionality of information flow (redundant connectivity) was also reported in the TBI participants ( Dobryakova et al., 2015 ). This study was suggestive of location-specific changes in the neural network connectivity following TBI depending on the cognitive functions at work, other than providing another support to the neural compensatory hypothesis due to the observed hyper-connectivity ( Dobryakova et al., 2015 ).
Nevertheless, inconsistent findings should not be neglected. In a study that also focused on brain connectivity analysis among patients with mild TBI by Hillary et al. (2011) , elevated task-related connectivity in the right hemisphere, in particular the prefrontal cortex, was consistently demonstrated during a working memory task while the control group showed greater left hemispheric activation. This further supported the right lateralization of the brain to reallocate cognitive resources of TBI patients post-injury. Meanwhile, the study did not manage to obtain the expected outcome in terms of greater clustering of whole-brain connections in TBI participants as hypothesized ( Hillary et al., 2011 ). That said, no significant loss or gain of connections due to the injury could be concluded from the study, as opposed to the hyper- or hypoactivation or hyper-connectivity frequently highlighted in other similar researches ( Hillary et al., 2011 ). Furthermore, a study by Chen et al. (2012) also failed to establish the same results of increased brain activation. Instead, with every increase of the working memory load, increase in brain activation, as expected to occur and as demonstrated in the control group, was unable to be detected in the TBI group ( Chen et al., 2012 ).
Taken all the insightful studies together, another aspect not to be neglected is the neuroimaging techniques employed in contributing to the literature on TBI. Modalities other than fMRI, which focuses on localization of brain activities, show other sides of the story of working memory impairments in TBI to offer a more holistic understanding. Studies adopting electroencephalography (EEG) or diffusor tensor imaging (DTI) reported atypical brainwaves coherence or white matter integrity in patients with TBI ( Treble et al., 2013 ; Ellis et al., 2016 ; Bailey et al., 2017 ; Owens et al., 2017 ). Investigating the supero-lateral medial forebrain bundle (MFB) that innervates and consequently terminates at the prefrontal cortex, microstructural white matter damage at the said area was indicated in participants with moderate to severe TBI by comparing its integrity with the control group ( Owens et al., 2017 ). Such observation was backed up by evidence showing that the patients performed more poorly on attention-loaded cognitive tasks of factors relating to slow processing speed than the healthy participants, although a direct association between MFB and impaired attentional system was not found ( Owens et al., 2017 ).
Correspondingly, DTI study of the corpus callosum (CC), which described to hold a vital role in connecting and coordinating both hemispheres to ensure competent cognitive functions, also found compromised microstructure of the CC with low fractional anisotropy and high mean diffusivity, both of which are indications of reduced white matter integrity ( Treble et al., 2013 ). This reported observation was also found to be predictive of poorer verbal or visuospatial working memory performance in callosal subregions connecting the parietal and temporal cortices ( Treble et al., 2013 ). Adding on to these results, using EEG to examine the functional consequences of CC damage revealed that interhemispheric transfer time (IHTT) of the CC was slower in the TBI group than the control group, suggesting an inefficient communication between the two hemispheres ( Ellis et al., 2016 ). In addition, the TBI group with slow IHTT as well exhibited poorer neurocognitive functioning including working memory than the healthy controls ( Ellis et al., 2016 ).
Furthermore, comparing the working memory between TBI, MDD, TBI-MDD, and healthy participants discovered that groups with MDD and TBI-MDD performed poorer on the Sternberg working memory task but functional connectivity on the other hand, showed that increased inter-hemispheric working memory gamma connectivity was observed in the TBI and TBI-MDD groups ( Bailey et al., 2017 ). Speculation provided for the findings of such neuronal state that was not reflected in the explicit working memory performance was that the deficits might not be detected or tested by the utilized Sternberg task ( Bailey et al., 2017 ). Another explanation attempting to answer the increase in gamma connectivity in these groups was the involvement of the neural compensatory mechanism after TBI to improve performance ( Bailey et al., 2017 ). Nevertheless, such outcome implies that behavioral performances or neuropsychological outcomes might not always be reflective of the functional changes happening in the brain.
Yet, bearing in mind that TBI consequences can be vast and crippling, cognitive improvement or recovery, though complicated due to the injury severity-dependent nature, is not impossible (see Review Article by Anderson and Catroppa, 2007 ; Nadebaum et al., 2007 ; Dikmen et al., 2009 ; Chen et al., 2012 ). As reported by Wylie et al. (2015) , cognitive improvement together with functional changes in the brain could be detected in individuals with mild TBI. Increased activation in the brain during 6-week follow-up was also observed in the mild TBI participants, implicating the regaining of connections in the brain ( Chen et al., 2012 ). Administration of certain cognitively enhancing drugs such as methylphenidate was reported to be helpful in improving working memory performance too ( Manktelow et al., 2017 ). Methylphenidate as a dopamine reuptake inhibitor was found to have modulated the neural activity in the left cerebellum which subsequently correlated with improved working memory performance ( Manktelow et al., 2017 ). A simplified summary of recent studies on working memory and TBI is tabulated in Table 4 .
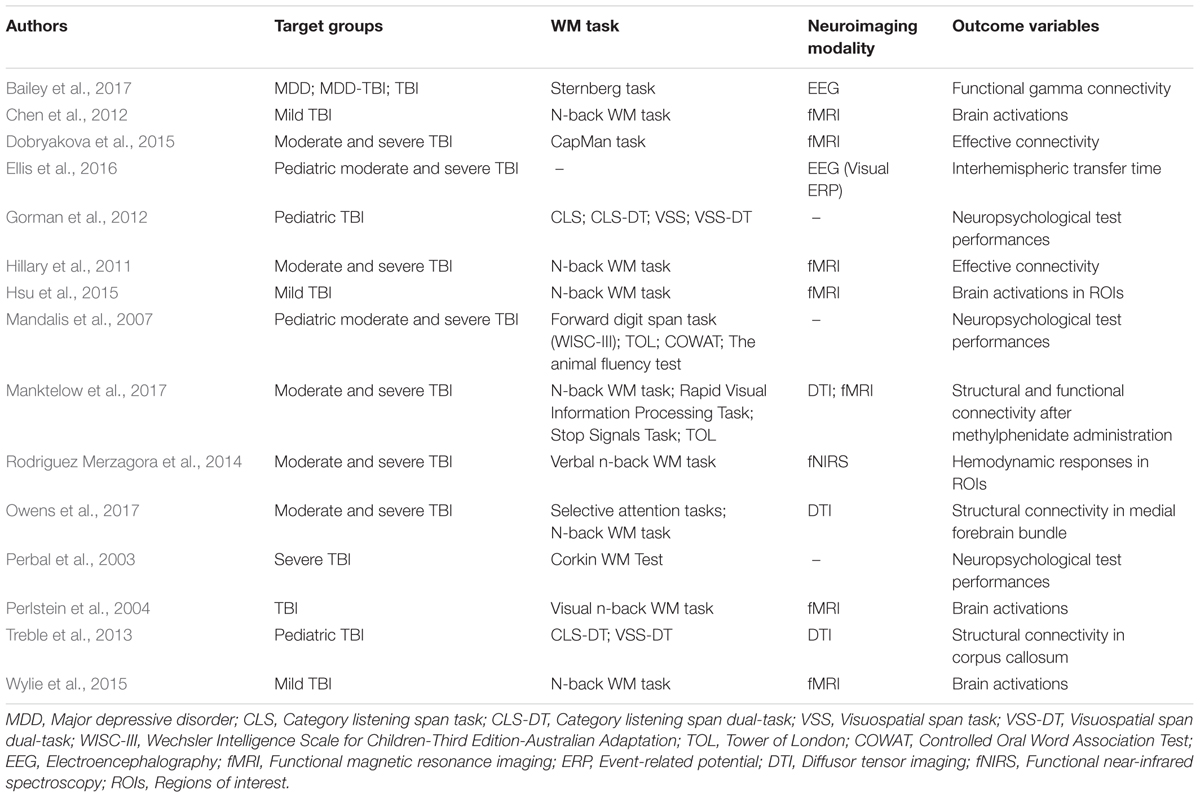
TABLE 4. Working memory (WM) studies in the TBI group.
General Discussion and Future Direction
In practice, all of the aforementioned studies contribute to the working memory puzzle by addressing the topic from different perspectives and employing various methodologies to study it. Several theoretical models of working memory that conceptualized different working memory mechanisms or domains (such as focus of attention, inhibitory controls, maintenance and manipulation of information, updating and integration of information, capacity limits, evaluative and executive controls, and episodic buffer) have been proposed. Coupled with the working memory tasks of various means that cover a broad range (such as Sternberg task, n-back task, Corsi block-tapping test, Wechsler’s Memory Scale [WMS], and working memory subtests in the Wechsler Adult Intelligence Scale [WAIS] – Digit Span, Letter Number Sequencing), it has been difficult, if not highly improbable, for working memory studies to reach an agreement upon a consistent study protocol that is acceptable for generalization of results due to the constraints bound by the nature of the study. Various data acquisition and neuroimaging techniques that come with inconsistent validity such as paper-and-pen neuropsychological measures, fMRI, EEG, DTI, and functional near-infrared spectroscopy (fNIRS), or even animal studies can also be added to the list. This poses further challenges to quantitatively measure working memory as only a single entity. For example, when studying the neural patterns of working memory based on Cowan’s processes-embedded model using fMRI, one has to ensure that the working memory task selected is fMRI-compatible, and demands executive control of attention directed at activated long-term memory (domain-specific). That said, on the one hand, there are tasks that rely heavily on the information maintenance such as the Sternberg task; on the other hand, there are also tasks that look into the information manipulation updating such as the n-back or arithmetic task. Meanwhile, the digit span task in WAIS investigates working memory capacity, although it can be argued that it also encompasses the domain on information maintenance and updating-. Another consideration involves the different natures (verbal/phonological and visuospatial) of the working memory tasks as verbal or visuospatial information is believed to engage differing sensory mechanisms that might influence comparison of working memory performance between tasks of different nature ( Baddeley and Hitch, 1974 ; Cowan, 1999 ). For instance, though both are n-back tasks that includes the same working memory domains, the auditory n-back differs than the visual n-back as the information is presented in different forms. This feature is especially crucial with regards to the study populations as it differentiates between verbal and visuospatial working memory competence within individuals, which are assumed to be domain-specific as demonstrated by vast studies (such as Nadler and Archibald, 2014 ; Pham and Hasson, 2014 ; Nakagawa et al., 2016 ). These test variations undeniably present further difficulties in selecting an appropriate task. Nevertheless, the adoption of different modalities yielded diverging outcomes and knowledge such as behavioral performances, functional segregation and integration in the brain, white matter integrity, brainwave coherence, and oxy- and deoxyhaemoglobin concentrations that are undeniably useful in application to different fields of study.
In theory, the neural efficiency hypothesis explains that increased efficiency of the neural processes recruit fewer cerebral resources in addition to displaying lower activation in the involved neural network ( Vartanian et al., 2013 ; Rodriguez Merzagora et al., 2014 ). This is in contrast with the neural compensatory hypothesis in which it attempted to understand diminished activation that is generally reported in participants with TBI ( Hillary et al., 2011 ; Dobryakova et al., 2015 ; Hsu et al., 2015 ; Wylie et al., 2015 ; Bailey et al., 2017 ). In the diseased brain, low activation has often been associated with impaired cognitive function ( Chen et al., 2012 ; Dobryakova et al., 2015 ; Wylie et al., 2015 ). Opportunely, the CRUNCH model proposed within the field of aging might be translated and integrated the two hypotheses here as it suitably resolved the disparity of cerebral hypo- and hyper-activation observed in weaker, less efficient brains as compared to healthy, adept brains ( Reuter-Lorenz and Park, 2010 ; Schneider-Garces et al., 2010 ). Moreover, other factors such as the relationship between fluid intelligence and working memory might complicate the current understanding of working memory as a single, isolated construct since working memory is often implied in measurements of the intelligence quotient ( Cowan, 2008 ; Vartanian et al., 2013 ). Indeed, the process overlap theory of intelligence proposed by Kovacs and Conway (2016) in which the constructs of intelligence were heavily scrutinized (such as general intelligence factors, g and its smaller counterparts, fluid intelligence or reasoning, crystallized intelligence, perceptual speed, and visual-spatial ability), and fittingly connected working memory capacity with fluid reasoning. Cognitive tests such as Raven’s Progressive Matrices or other similar intelligence tests that demand complex cognition and were reported in the paper had been found to correlate strongly with tests of working memory ( Kovacs and Conway, 2016 ). Furthermore, in accordance with such views, in the same paper, neuroimaging studies found intelligence tests also activated the same fronto-parietal network observed in working memory ( Kovacs and Conway, 2016 ).
On the other hand, even though the roles of the prefrontal cortex in working memory have been widely established, region specificity and localization in the prefrontal cortex in relation to the different working memory domains such as manipulation or delayed retention of information remain at the premature stage (see Review Article by D’Esposito and Postle, 2015 ). It has been postulated that the neural mechanisms involved in working memory are of high-dimensionality and could not always be directly captured and investigated using neurophysiological techniques such as fMRI, EEG, or patch clamp recordings even when comparing with lesion data ( D’Esposito and Postle, 2015 ). According to D’Esposito and Postle (2015) , human fMRI studies have demonstrated that a rostral-caudal functional gradient related to level of abstraction required of working memory along the frontal cortex (in which different regions in the prefrontal cortex [from rostral to caudal] might be associated with different abstraction levels) might exist. Other functional gradients relating to different aspects of working memory were similarly unraveled ( D’Esposito and Postle, 2015 ). These proposed mechanisms with different empirical evidence point to the fact that conclusive understanding regarding working memory could not yet be achieved before the inconsistent views are reconciled.
Not surprisingly, with so many aspects of working memory yet to be understood and its growing complexity, the cognitive neuroscience basis of working memory requires constant research before an exhaustive account can be gathered. From the psychological conceptualization of working memory as attempted in the multicomponent working memory model ( Baddeley and Hitch, 1974 ), to the neural representations of working memory in the brain, especially in the frontal regions ( D’Esposito and Postle, 2015 ), one important implication derives from the present review of the literatures is that working memory as a psychological construct or a neuroscientific mechanism cannot be investigated as an isolated event. The need for psychology and neuroscience to interact with each other in an active feedback cycle exists in which this cognitive system called working memory can be dissected at the biological level and refined both empirically, and theoretically.
In summary, the present article offers an account of working memory from the psychological and neuroscientific perspectives, in which theoretical models of working memory are presented, and neural patterns and brain regions engaging in working memory are discussed among healthy and diseased brains. It is believed that working memory lays the foundation for many other cognitive controls in humans, and decoding the working memory mechanisms would be the first step in facilitating understanding toward other aspects of human cognition such as perceptual or emotional processing. Subsequently, the interactions between working memory and other cognitive systems could reasonably be examined.
Author Contributions
WC wrote the manuscript with critical feedback and consultation from AAH. WC and AAH contributed to the final version of the manuscript. JA supervised the process and proofread the manuscript.
This work was supported by the Transdisciplinary Research Grant Scheme (TRGS) 203/CNEURO/6768003 and the USAINS Research Grant 2016.
Conflict of Interest Statement
The authors declare that the research was conducted in the absence of any commercial or financial relationships that could be construed as a potential conflict of interest.
The reviewer EB and handling Editor declared their shared affiliation.
Andersen, R. A., and Cui, H. (2009). Review intention, action planning, and decision making in parietal-frontal circuits. Neuron 63, 568–583. doi: 10.1016/j.neuron.2009.08.028
PubMed Abstract | CrossRef Full Text | Google Scholar
Anderson, V., and Catroppa, C. (2007). Memory outcome at 5 years post-childhood traumatic brain injury. Brain Inj. 21, 1399–1409. doi: 10.1080/02699050701785070
Arendash, G. W., Schleif, W., Rezai-Zadeh, K., Jackson, E. K., Zacharia, L. C., Cracchiolo, J. R., et al. (2006). Caffeine protects Alzheimer’s mice against cognitive impairment and reduces brain β-amyloid production. Neuroscience 142, 941–952. doi: 10.1016/j.neuroscience.2006.07.021
Ashkenazi, S., Rosenberg-lee, M., Metcalfe, A. W. S., Swigart, A. G., and Menon, V. (2013). Neuropsychologia visuo – spatial working memory is an important source of domain-general vulnerability in the development of arithmetic cognition. Neuropsychologia 51, 2305–2317. doi: 10.1016/j.neuropsychologia.2013.06.031
Baars, B. J., and Franklin, S. (2003). How conscious experience and working memory interact. Trends Cogn. Sci. 7, 166–172. doi: 10.1016/S1364-6613(03)00056-1
CrossRef Full Text | Google Scholar
Baddeley, A. (1996). Exploring the central executive. Q. J. Exp. Psychol. A 49, 5–28. doi: 10.1080/713755608
Baddeley, A. (2010). Working memory. Curr. Biol. 20, R136–R140. doi: 10.1016/j.cub.2009.12.014
Baddeley, A. (2012). Working memory: theories, models, and controversies. Annu. Rev. Psychol. 63, 1–29. doi: 10.1146/annurev-psych-120710-100422
Baddeley, A., and Hitch, G. (1974). Working memory. Psychol. Learn. Motiv. 8, 47–89. doi: 10.1016/j.cub.2009.12.014
Baddeley, A. D. (2000a). The episodic buffer : a new component of working memory? Trends Cogn. Sci. 4, 417–423. doi: 10.1016/S1364-6613(00)01538-2
Baddeley, A. D. (2000b). Short-Term and Working Memory. The Oxford Handbook of Memory. Oxford: Oxford University Press.
Google Scholar
Bailey, N. W., Rogasch, N. C., Hoy, K. E., Maller, J. J., Segrave, R. A., Sullivan, C. M., et al. (2017). Increased gamma connectivity during working memory retention following traumatic brain injury. Brain Inj. 31, 379–389. doi: 10.1080/02699052.2016.1239273
Balderston, N. L., Vytal, K. E., O’Connell, K., Torrisi, S., Letkiewicz, A., Ernst, M., et al. (2017). Anxiety patients show reduced working memory related dlPFC activation during safety and threat. Depress. Anxiety 34, 25–36. doi: 10.1002/da.22518
Barrouillet, P., Bernardin, S., and Camos, V. (2004). Time constraints and resource sharing in adults’ working memory spans. J. Exp. Psychol. Gen. 133, 83–100. doi: 10.1037/0096-3445.133.1.83
Barrouillet, P., and Camos, V. (2007). “The time-based resource-sharing model of working memory,” in The Cognitive Neuroscience of Working Memory , ed. N. Osaka (Oxford: Oxford University Press), 59–80. doi: 10.1093/acprof:oso/9780198570394.003.0004
Barrouillet, P., Gavens, N., Vergauwe, E., Gaillard, V., and Camos, V. (2009). Working memory span development: a time-based resource-sharing model account. Dev. Psychol. 45, 477–490. doi: 10.1037/a0014615
Bolkan, S. S., Stujenske, J. M., Parnaudeau, S., Spellman, T. J., Rauffenbart, C., Abbas, A. I., et al. (2017). Thalamic projections sustain prefrontal activity during working memory maintenance. Nat. Neurosci. 20, 987–996. doi: 10.1038/nn.4568
Borella, E., Carretti, B., Sciore, R., Capotosto, E., Taconnat, L., Cornoldi, C., et al. (2017). Training working memory in older adults: is there an advantage of using strategies? Psychol. Aging 32, 178–191. doi: 10.1037/pag0000155
Chein, J. M., Moore, A. B., and Conway, A. R. A. (2011). NeuroImage domain-general mechanisms of complex working memory span. Neuroimage 54, 550–559. doi: 10.1016/j.neuroimage.2010.07.067
Chen, C. J., Wu, C. H., Liao, Y. P., Hsu, H. L., Tseng, Y. C., Liu, H. L., et al. (2012). Working memory in patients with mild traumatic brain injury: functional MR imaging analysis. Radiology 264, 844–851. doi: 10.1148/radiol.12112154
Cowan, N. (1999). “An embedded-processes model of working memory,” in Models of Working Memory: Mechanisms of Active Maintenance and Executive Control , eds A. Miyake and P. Shah (Cambridge: Cambridge University Press). doi: 10.1017/S0140525X01003922
Cowan, N. (2005). Working memory capacity. Exp. Psychol. 54, 245–246. doi: 10.1027/1618-3169.54.3.245
Cowan, N. (2008). What are the differences between long-term, short-term, and working memory? Prog. Brain Res. 169, 323–338. doi: 10.1016/S0079-6123(07)00020-9
Cowan, N. (2010). The magical mystery four. Curr. Dir. Psychol. Sci. 19, 51–57. doi: 10.1177/0963721409359277
Dahlin, E., Nyberg, L., Bäckman, L., and Neely, A. S. (2008). Plasticity of executive functioning in young and older adults: immediate training gains, transfer, and long-term maintenance. Psychol. Aging 23, 720–730. doi: 10.1037/a0014296
Daneman, M., and Carpenter, P. A. (1980). Individual differences in working memory and reading. J. Verbal Learn. Verbal Behav. 19, 450–466. doi: 10.1016/S0022-5371(80)90312-6
D’Esposito, M., and Postle, B. R. (2015). The cognitive neuroscience of working memory. Annu. Rev. Psychol. 66, 115–142. doi: 10.1146/annurev-psych-010814-015031
Dikmen, S. S., Corrigan, J. D., Levin, H. S., Machamer, J., Stiers, W., and Weisskopf, M. G. (2009). Cognitive outcome following traumatic brain injury. J. Head Trauma Rehabil. 24, 430–438. doi: 10.1097/HTR.0b013e3181c133e9
Dima, D., Jogia, J., and Frangou, S. (2014). Dynamic causal modeling of load-dependent modulation of effective connectivity within the verbal working memory network. Hum. Brain Mapp. 35, 3025–3035. doi: 10.1002/hbm.22382
Dobryakova, E., Boukrina, O., and Wylie, G. R. (2015). Investigation of information flow during a novel working memory task in individuals with traumatic brain injury. Brain Connect. 5, 433–441. doi: 10.1089/brain.2014.0283
Duff, S. J., and Hampson, E. (2000). A beneficial effect of estrogen on working memory in postmenopausal women taking hormone replacement therapy. Horm. Behav. 38, 262–276. doi: 10.1006/hbeh.2000.1625
Dunning, D. L., Westgate, B., and Adlam, A.-L. R. (2016). A meta-analysis of working memory impairments in survivors of moderate-to-severe traumatic brain injury. Neuropsychology 30, 811–819. doi: 10.1037/neu0000285
Ellis, M. U., DeBoard Marion, S., McArthur, D. L., Babikian, T., Giza, C., Kernan, C. L., et al. (2016). The UCLA study of children with moderate-to-severe traumatic brain injury: event-related potential measure of interhemispheric transfer time. J. Neurotrauma 33, 990–996. doi: 10.1089/neu.2015.4023
Engle, R. W. (2002). Working memory capacity as executive attention. Curr. Dir. Psychol. Sci. 11, 19–23. doi: 10.1111/1467-8721.00160
Engle, R. W., and Kane, M. J. (2004). “Executive attention, working memory capacity, and a two-factor theory of cognitive control,” in The Psychology of Learning and Motivation: Advances in Research and Theory , ed. B. H. Ross (New York, NY: Elsevier), 145–199. doi: 10.1016/S0079-7421(03)44005-X
Farrer, T. J. (2017). Encyclopedia of Geropsychology , ed. N. A. Pachana. Singapore: Springer. doi: 10.1007/978-981-287-080-3
CrossRef Full Text
Fiebig, F., and Lansner, A. (2017). A spiking working memory model based on hebbian short-term potentiation. J. Neurosci. 37, 83–96. doi: 10.1523/JNEUROSCI.1989-16.2016
Friston, K., Moran, R., and Seth, A. K. (2013). Analysing connectivity with granger causality and dynamic causal modelling. Curr. Opin. Neurobiol. 23, 172–178. doi: 10.1016/j.conb.2012.11.010
Gorman, S., Barnes, M. A., Swank, P. R., Prasad, M., Cox, C. S., and Ewing-Cobbs, L. (2016). Does processing speed mediate the effect of pediatric traumatic brain injury on working memory? Neuropsychology 30, 263–273. doi: 10.1037/neu0000214
Gorman, S., Barnes, M. A., Swank, P. R., Prasad, M., and Ewing-Cobbs, L. (2012). The effects of pediatric traumatic brain injury on verbal and visual-spatial working memory. J. Int. Neuropsychol. Soc. 18, 29–38. doi: 10.1017/S1355617711001251
Gottwald, B., Wilde, B., Mihajlovic, Z., and Mehdorn, H. M. (2004). Evidence for distinct cognitive deficits after focal cerebellar lesions. J. Neurol. Neurosurg. Psychiatry 75, 1524–1531. doi: 10.1136/jnnp.2003.018093
Grot, S., Légaré, V. P., Lipp, O., Soulières, I., Dolcos, F., and Luck, D. (2017). Abnormal prefrontal and parietal activity linked to deficient active binding in working memory in schizophrenia. Schizophr. Res. 188, 68–74. doi: 10.1016/j.schres.2017.01.021
Guye, S., and von Bastian, C. C. (2017). Working memory training in older adults: bayesian evidence supporting the absence of transfer. Psychol. Aging 32, 732–746. doi: 10.1037/pag0000206
Haller, S., Montandon, M.-L., Rodriguez, C., Moser, D., Toma, S., Hofmeister, J., et al. (2017). Caffeine impact on working memory-related network activation patterns in early stages of cognitive decline. Neuroradiology 59, 387–395. doi: 10.1007/s00234-017-1803-5
Haller, S., Rodriguez, C., Moser, D., Toma, S., Hofmeister, J., Sinanaj, I., et al. (2013). Acute caffeine administration impact on working memory-related brain activation and functional connectivity in the elderly: a BOLD and perfusion MRI study. Neuroscience 250, 364–371. doi: 10.1016/j.neuroscience.2013.07.021
Hedden, T., and Gabrieli, J. D. E. (2004). Insights into the ageing mind: a view from cognitive neuroscience. Nat. Rev. Neurosci. 5, 87–96. doi: 10.1038/nrn1323
Heinzel, S., Rimpel, J., Stelzel, C., and Rapp, M. A. (2017). Transfer effects to a multimodal dual-task after working memory training and associated neural correlates in older adults – a pilot study. Front. Hum. Neurosci. 11:85. doi: 10.3389/fnhum.2017.00085
Hillary, F. G., Medaglia, J. D., Gates, K., Molenaar, P. C., Slocomb, J., Peechatka, A., et al. (2011). Examining working memory task acquisition in a disrupted neural network. Brain 134, 1555–1570. doi: 10.1093/brain/awr043
Hsu, H.-L., Chen, D. Y.-T., Tseng, Y.-C., Kuo, Y.-S., Huang, Y.-L., Chiu, W.-T., et al. (2015). Sex differences in working memory after mild traumatic brain injury: a functional MR imaging study. Radiology 276, 828–835. doi: 10.1148/radiol.2015142549
Humphreys, M. S., Bain, J. D., and Pike, R. (1989). Different ways to cue a coherent memory system: a theory for episodic, semantic, and procedural tasks. Psychol. Rev. 96, 208–233. doi: 10.1037/0033-295X.96.2.208
Janowsky, J. S., Chavez, B., and Orwoll, E. (2000). Sex steroids modify working memory. J. Cogn. Neurosci. 12, 407–414. doi: 10.1162/089892900562228
Jimura, K., Chushak, M. S., Westbrook, A., and Braver, T. S. (2017). Intertemporal decision-making involves prefrontal control mechanisms associated with working memory. Cereb. Cortex doi: 10.1093/cercor/bhx015 [Epub ahead of print].
Joseph, J. E., Swearingen, J. E., Corbly, C. R., Curry, T. E., and Kelly, T. H. (2012). Influence of estradiol on functional brain organization for working memory. Neuroimage 59, 2923–2931. doi: 10.1016/j.neuroimage.2011.09.067
Karbach, J., and Verhaeghen, P. (2014). Making working memory work: a meta-analysis of executive control and working memory training in younger and older adults. Psychol. Sci. 25, 2027–2037. doi: 10.1177/0956797614548725
Kim, C., Kroger, J. K., Calhoun, V. D., and Clark, V. P. (2015). The role of the frontopolar cortex in manipulation of integrated information in working memory. Neurosci. Lett. 595, 25–29. doi: 10.1016/j.neulet.2015.03.044
Klaassen, E. B., De Groot, R. H. M., Evers, E. A. T., Snel, J., Veerman, E. C. I., Ligtenberg, A. J. M., et al. (2013). The effect of caffeine on working memory load-related brain activation in middle-aged males. Neuropharmacology 64, 160–167. doi: 10.1016/j.neuropharm.2012.06.026
Kovacs, K., and Conway, A. R. A. (2016). Process overlap theory: a unified account of the general factor of intelligence. Psychol. Inq. 27, 151–177. doi: 10.1080/1047840X.2016.1153946
Le, T. M., Borghi, J. A., Kujawa, A. J., Klein, D. N., and Leung, H.-C. (2017). Alterations in visual cortical activation and connectivity with prefrontal cortex during working memory updating in major depressive disorder. Neuroimage 14, 43–53. doi: 10.1016/j.nicl.2017.01.004
Liu, Z.-X., Glizer, D., Tannock, R., and Woltering, S. (2016). EEG alpha power during maintenance of information in working memory in adults with ADHD and its plasticity due to working memory training: a randomized controlled trial. Clin. Neurophysiol. 127, 1307–1320. doi: 10.1016/j.clinph.2015.10.032
Ma, L., Steinberg, J. L., Hasan, K. M., Narayana, P. A., Kramer, L. A., and Moeller, F. G. (2012). Working memory load modulation of parieto-frontal connections: evidence from dynamic causal modeling. Hum. Brain Mapp. 33, 1850–1867. doi: 10.1002/hbm.21329
Maehler, C., and Schuchardt, K. (2016). Working memory in children with specific learning disorders and/or attention deficits. Learn. Individ. Differ. 49, 341–347. doi: 10.1016/j.lindif.2016.05.007
Mandalis, A., Kinsella, G., Ong, B., and Anderson, V. (2007). Working memory and new learning following pediatric traumatic brain injury. Dev. Neuropsychol. 32, 683–701. doi: 10.1080/87565640701376045
Manktelow, A. E., Menon, D. K., Sahakian, B. J., and Stamatakis, E. A. (2017). Working memory after traumatic brain injury: the neural basis of improved performance with methylphenidate. Front. Behav. Neurosci. 11:58. doi: 10.3389/fnbeh.2017.00058
Miller, G. A., Galanter, E., and Pribram, K. H. (1960). Plans and the Structure of Behavior. New York, NY: Henry Holt and Company. doi: 10.1037/10039-000
Miyake, A., and Shah, P. (eds). (1999). Models of Working Memory: Mechanisms of Active Maintenance and Executive Control. New York, NY: Cambridge University Press. doi: 10.1017/CBO9781139174909
Mongillo, G., Barak, O., and Tsodyks, M. (2008). Synaptic theory of working memory. Science 319, 1543–1546. doi: 10.1126/science.1150769
Moore, A. B., Li, Z., Tyner, C. E., Hu, X., and Crosson, B. (2013). Bilateral basal ganglia activity in verbal working memory. Brain Lang. 125, 316–323. doi: 10.1016/j.bandl.2012.05.003
Murty, V. P., Sambataro, F., Radulescu, E., Altamura, M., Iudicello, J., Zoltick, B., et al. (2011). Selective updating of working memory content modulates meso-cortico-striatal activity. Neuroimage 57, 1264–1272. doi: 10.1016/j.neuroimage.2011.05.006
Nadebaum, C., Anderson, V., and Catroppa, C. (2007). Executive function outcomes following traumatic brain injury in young children: a five year follow-up. Dev. Neuropsychol. 32, 703–728. doi: 10.1080/87565640701376086
Nadler, R. T., and Archibald, L. M. D. (2014). The assessment of verbal and visuospatial working memory with school age canadian children. Can. J. Speech Lang. Pathol. Audiol. 38, 262–279.
Nakagawa, S., Takeuchi, H., Taki, Y., Nouchi, R., Sekiguchi, A., Kotozaki, Y., et al. (2016). Sex-related differences in the effects of sleep habits on verbal and visuospatial working memory. Front. Psychol. 7:1128. doi: 10.3389/fpsyg.2016.01128
Nissim, N. R., O’Shea, A. M., Bryant, V., Porges, E. C., Cohen, R., and Woods, A. J. (2017). Frontal structural neural correlates of working memory performance in older adults. Front. Aging Neurosci. 8:328. doi: 10.3389/fnagi.2016.00328
Oren, N., Ash, E. L., Tarrasch, R., Hendler, T., Giladi, N., and Shapira-Lichter, I. (2017). Neural patterns underlying the effect of negative distractors on working memory in older adults. Neurobiol. Aging 53, 93–102. doi: 10.1016/j.neurobiolaging.2017.01.020
Osaka, M., Osaka, N., Kondo, H., Morishita, M., Fukuyama, H., Aso, T., et al. (2003). The neural basis of individual differences in working memory capacity: an fMRI study. Neuroimage 18, 789–797. doi: 10.1016/S1053-8119(02)00032-0
Owen, A. M., McMillan, K. M., Laird, A. R., and Bullmore, E. (2005). N-back working memory paradigm: a meta-analysis of normative functional neuroimaging studies. Hum. Brain Mapp. 25, 46–59. doi: 10.1002/hbm.20131
Owens, J. A., Spitz, G., Ponsford, J. L., Dymowski, A. R., Ferris, N., and Willmott, C. (2017). White matter integrity of the medial forebrain bundle and attention and working memory deficits following traumatic brain injury. Brain Behav. 7:e00608. doi: 10.1002/brb3.608
Perbal, S., Couillet, J., Azouvi, P., and Pouthas, V. (2003). Relationships between time estimation, memory, attention, and processing speed in patients with severe traumatic brain injury. Neuropsychologia 41, 1599–1610. doi: 10.1016/S0028-3932(03)00110-6
Perlstein, W. M., Cole, M. A., Demery, J. A., Seignourel, P. J., Dixit, N. K., Larson, M. J., et al. (2004). Parametric manipulation of working memory load in traumatic brain injury: behavioral and neural correlates. J. Int. Neuropsychol. Soc. 10, 724–741. doi: 10.1017/S1355617704105110
Pham, A. V., and Hasson, R. M. (2014). Verbal and visuospatial working memory as predictors of children’s reading ability. Arch. Clin. Neuropsychol. 29, 467–477. doi: 10.1093/arclin/acu024
Phillips, N. L., Parry, L., Mandalis, A., and Lah, S. (2017). Working memory outcomes following traumatic brain injury in children: a systematic review with meta-analysis. Child Neuropsychol. 23, 26–66. doi: 10.1080/09297049.2015.1085500
Rees, K., Allen, D., and Lader, M. (1999). The influences of age and caffeine on psychomotor and cognitive function. Psychopharmacology 145, 181–188. doi: 10.1007/s002130051047
Reuter-Lorenz, P. A., and Cappell, K. A. (2008). Neurocognitive ageing and the compensation hypothesis. Curr. Dir. Psychol. Sci. 17, 177–182. doi: 10.1111/j.1467-8721.2008.00570.x
Reuter-Lorenz, P. A., and Park, D. C. (2010). Human neuroscience and the aging mind : a new look at old problems. J. Gerontol. Psychol. Sci. 65, 405–415. doi: 10.1093/geronb/gbq035
Rieck, J. R., Rodrigue, K. M., Boylan, M. A., and Kennedy, K. M. (2017). Age-related reduction of BOLD modulation to cognitive difficulty predicts poorer task accuracy and poorer fluid reasoning ability. Neuroimage 147, 262–271. doi: 10.1016/j.neuroimage.2016.12.022
Rodriguez Merzagora, A. C., Izzetoglu, M., Onaral, B., and Schultheis, M. T. (2014). Verbal working memory impairments following traumatic brain injury: an fNIRS investigation. Brain Imaging Behav. 8, 446–459. doi: 10.1007/s11682-013-9258-8
Rose, N. S., LaRocque, J. J., Riggall, A. C., Gosseries, O., Starrett, M. J., Meyering, E. E., et al. (2016). Reactivation of latent working memories with transcranial magnetic stimulation. Science 354, 1136–1139. doi: 10.1126/science.aah7011
Rotzer, S., Loenneker, T., Kucian, K., Martin, E., Klaver, P., and von Aster, M. (2009). Dysfunctional neural network of spatial working memory contributes to developmental dyscalculia. Neuropsychologia 47, 2859–2865. doi: 10.1016/j.neuropsychologia.2009.06.009
Schneider-Garces, N. J., Gordon, B. A., Brumback-Peltz, C. R., Shin, E., Lee, Y., Sutton, B. P., et al. (2010). Span, CRUNCH, and beyond: working memory capacity and the aging brain. J. Cogn. Neurosci. 22, 655–669. doi: 10.1162/jocn.2009.21230
Schöning, S., Engelien, A., Kugel, H., Schäfer, S., Schiffbauer, H., Zwitserlood, P., et al. (2007). Functional anatomy of visuo-spatial working memory during mental rotation is influenced by sex, menstrual cycle, and sex steroid hormones. Neuropsychologia 45, 3203–3214. doi: 10.1016/j.neuropsychologia.2007.06.011
Silvanto, J. (2017). Working memory maintenance: sustained firing or synaptic mechanisms? Trends Cogn. Sci. 21, 152–154. doi: 10.1016/j.tics.2017.01.009
Stegmayer, K., Usher, J., Trost, S., Henseler, I., Tost, H., Rietschel, M., et al. (2015). Disturbed cortico–amygdalar functional connectivity as pathophysiological correlate of working memory deficits in bipolar affective disorder. Eur. Arch. Psychiatry Clin. Neurosci. 265, 303–311. doi: 10.1007/s00406-014-0517-5
Treble, A., Hasan, K. M., Iftikhar, A., Stuebing, K. K., Kramer, L. A., Cox, C. S., et al. (2013). Working memory and corpus callosum microstructural integrity after pediatric traumatic brain injury: a diffusion tensor tractography study. J. Neurotrauma 30, 1609–1619. doi: 10.1089/neu.2013.2934
Vallat-Azouvi, C., Weber, T., Legrand, L., and Azouvi, P. (2007). Working memory after severe traumatic brain injury. J. Int. Neuropsychol. Soc. 13, 770–780. doi: 10.1017/S1355617707070993
Vartanian, O., Jobidon, M.-E., Bouak, F., Nakashima, A., Smith, I., Lam, Q., et al. (2013). Working memory training is associated with lower prefrontal cortex activation in a divergent thinking task. Neuroscience 236, 186–194. doi: 10.1016/j.neuroscience.2012.12.060
Wang, S., and Gathercole, S. E. (2013). Working memory deficits in children with reading difficulties: memory span and dual task coordination. J. Exp. Child Psychol. 115, 188–197. doi: 10.1016/j.jecp.2012.11.015
Wylie, G. R., Freeman, K., Thomas, A., Shpaner, M., OKeefe, M., Watts, R., et al. (2015). Cognitive improvement after mild traumatic brain injury measured with functional neuroimaging during the acute period. PLoS One 10:e0126110. doi: 10.1371/journal.pone.0126110
Ziaei, M., Salami, A., and Persson, J. (2017). Age-related alterations in functional connectivity patterns during working memory encoding of emotional items. Neuropsychologia 94, 1–12. doi: 10.1016/j.neuropsychologia.2016.11.012
Ziemus, B., Baumann, O., Luerding, R., Schlosser, R., Schuierer, G., Bogdahn, U., et al. (2007). Impaired working-memory after cerebellar infarcts paralleled by changes in bold signal of a cortico-cerebellar circuit. Neuropsychologia 45, 2016–2024. doi: 10.1016/j.neuropsychologia.2007.02.012
Zylberberg, J., and Strowbridge, B. W. (2017). Mechanisms of persistent activity in cortical circuits: possible neural substrates for working memory. Annu. Rev. Neurosci. 40, 603–627. doi: 10.1146/annurev-neuro-070815-014006
Keywords : working memory, neuroscience, psychology, cognition, brain, central executive, prefrontal cortex, review
Citation: Chai WJ, Abd Hamid AI and Abdullah JM (2018) Working Memory From the Psychological and Neurosciences Perspectives: A Review. Front. Psychol. 9:401. doi: 10.3389/fpsyg.2018.00401
Received: 24 November 2017; Accepted: 09 March 2018; Published: 27 March 2018.
Reviewed by:
Copyright © 2018 Chai, Abd Hamid and Abdullah. This is an open-access article distributed under the terms of the Creative Commons Attribution License (CC BY) . The use, distribution or reproduction in other forums is permitted, provided the original author(s) and the copyright owner are credited and that the original publication in this journal is cited, in accordance with accepted academic practice. No use, distribution or reproduction is permitted which does not comply with these terms.
*Correspondence: Aini Ismafairus Abd Hamid, [email protected]
Disclaimer: All claims expressed in this article are solely those of the authors and do not necessarily represent those of their affiliated organizations, or those of the publisher, the editors and the reviewers. Any product that may be evaluated in this article or claim that may be made by its manufacturer is not guaranteed or endorsed by the publisher.
- Alzheimer's disease & dementia
- Arthritis & Rheumatism
- Attention deficit disorders
- Autism spectrum disorders
- Biomedical technology
- Diseases, Conditions, Syndromes
- Endocrinology & Metabolism
- Gastroenterology
- Gerontology & Geriatrics
- Health informatics
- Inflammatory disorders
- Medical economics
- Medical research
- Medications
- Neuroscience
- Obstetrics & gynaecology
- Oncology & Cancer
- Ophthalmology
- Overweight & Obesity
- Parkinson's & Movement disorders
- Psychology & Psychiatry
- Radiology & Imaging
- Sleep disorders
- Sports medicine & Kinesiology
- Vaccination
- Breast cancer
- Cardiovascular disease
- Chronic obstructive pulmonary disease
- Colon cancer
- Coronary artery disease
- Heart attack
- Heart disease
- High blood pressure
- Kidney disease
- Lung cancer
- Multiple sclerosis
- Myocardial infarction
- Ovarian cancer
- Post traumatic stress disorder
- Rheumatoid arthritis
- Schizophrenia
- Skin cancer
- Type 2 diabetes
- Full List »
share this!
May 15, 2024
This article has been reviewed according to Science X's editorial process and policies . Editors have highlighted the following attributes while ensuring the content's credibility:
fact-checked
peer-reviewed publication
trusted source
The crystallization of memory: Study reveals how practice forms new memory pathways in the brain
by Rockefeller University
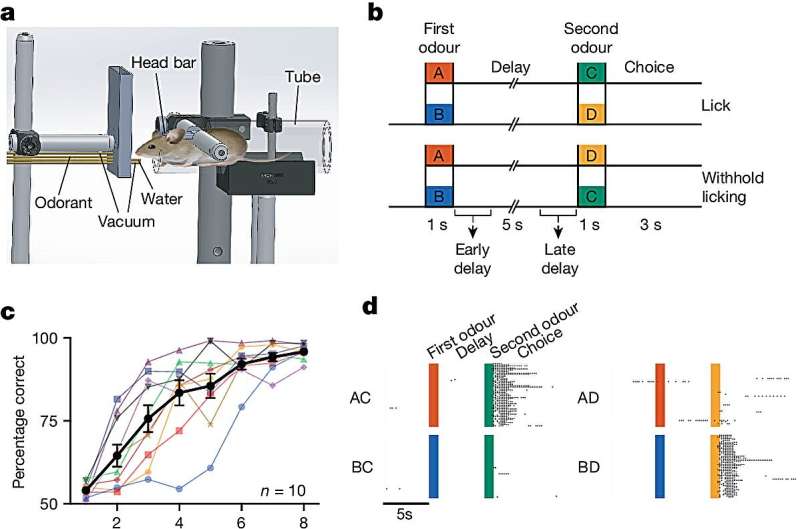
"Practice makes perfect" is no mere cliché, according to a new study from researchers at The Rockefeller University and UCLA. Instead, it's the recipe for mastering a task, because repeating an activity over and over solidifies neural pathways in your brain.
As they describe in Nature , the scientists used a cutting-edge technology developed by Rockefeller's Alipasha Vaziri to simultaneously observe 73,000 cortical neurons in mice as the animals learned and repeated a given task over two weeks. The study revealed that memory representations transform from unstable to solid in working memory circuits, giving insights into why performance becomes more accurate and automatic following repetitive practice.
"In this work we show how working memory—the brain's ability to hold and process information—improves through practice," says Vaziri, head of Rockefeller's Laboratory of Neurology and Biophysics. "We expect that these insights will not only advance our understanding of learning and memory but also have implications for addressing memory-related disorders."
Imagining challenges
Working memory is essential to a variety of cognitive functions, and yet the mechanisms underlying memory formation, retention, and recall—which enable us to perform a task we've done before without having to learn it anew—remain unclear over long timescales.
For the current study, the researchers wanted to observe the stability of working memory representations over time, and what role these representations played in the ability to skillfully perform the task on cue. To do so, they sought to record neuronal populations repeatedly in mice over a relatively long period while the animals learned and became experts in a given task.
But they faced a daunting challenge: technical limitations have hampered the ability to image the activity of large population of neurons across the brain in real time, over longer periods, and at any tissue depth in the cortex.
The UCLA researchers turned to Vaziri, who has developed brain imaging techniques that are among the only tools capable of capturing the majority of the mouse cortex in real time at a high resolution and speed.
Vaziri suggested they use light-beads microscopy (LBM), a high-speed volumetric imaging technology he developed that allows for cellular resolution in vivo recording of activity of neuronal populations up to 1 million neurons—a 100-fold increase in the number of neurons that can be simultaneously recorded.
Neural transformations
In the current study, the researchers used LBM to image the cellular activity of 73,000 neurons in mice simultaneously throughout various depths of the cortex and tracked the activity of the same neurons over two weeks as the animals identified, recalled, and repeated a sequence of odors.
They found that the working memory circuits transformed as the mice mastered the proper sequences. Initially, the circuits were unstable, but as the mice practiced the task repeatedly, the circuits began to stabilize and solidify.
"This is what we refer to as 'crystallization,'" Vaziri says. "The findings essentially illustrate that repetitive training not only enhances skill proficiency but also leads to profound changes in the brain's memory circuits, making performance more accurate and automatic."
"If one imagines that each neuron in the brain is sounding a different note, the melody that the brain is generating when it is doing the task was changing from day to day, but then became more and more refined and similar as animals kept practicing the task," adds corresponding author and UCLA Health neurologist Peyman Golshani.
Crucially, some aspects of these discoveries were uniquely enabled by the large-scale and deep tissue imaging capabilities of LBM. Initially, the researchers used standard two-photon imaging of smaller neuronal populations in upper cortical layers, but they failed to find evidence for memory stabilization. But once they employed LBM to record from over 70,000 neurons in deeper cortical regions, they were able to observe the crystallization of working memory representations that accompanied the mice's increasing mastery of the task.
"In the future, we may tackle the role of different neuronal cell types involved in mediating this mechanism, and in particular the interaction of different types of interneurons with excitatory cells," Vaziri says. "We're also interested in understanding how learning is implemented and could be transferred into a new context—that is, how the brain could generalize from a learned task to some new unknown problems."
Explore further
Feedback to editors

Study suggests AI may soon be able to detect cancer
15 minutes ago

Walking brings huge benefits for low back pain, study finds
5 hours ago

Combination targeted treatment produces lasting remissions in people with resistant aggressive B-cell lymphoma
7 hours ago

Study finds one copy of protective genetic variant helps stave off early-onset Alzheimer's disease

Drugs for enlarged prostate may also protect against dementia with Lewy bodies
8 hours ago

New study establishes best practices for supervised psilocybin

During a heat wave, high indoor temperatures can also prove dangerous
9 hours ago

Scientists reveal how an unstructured protein traps cancer-promoting molecules

Addressing maternal prenatal depression can lead to longer gestation, researchers say
10 hours ago

Researchers develop portable serological test for rapid COVID-19 immunity monitoring
Related stories.

Working memory depends on reciprocal interactions across the brain
Jul 27, 2022

Task-specific modulation of corticospinal neuron activity during motor learning in mice
May 15, 2023

Researchers unravel how the brain remembers
Dec 4, 2019

For people with memory struggles after brain injuries, the brain's processing speed is the main culprit: Study
Feb 8, 2024

Computational model reveals how the brain manages short-term memories
Dec 17, 2020

Learning skills of mice help researchers pinpoint brain areas where acquired knowledge is stored
Sep 23, 2021
Recommended for you

NG2 glia cells shown to protect against prion-induced neurotoxicity and neurodegeneration
15 hours ago

Open-source project maps the biology of spinal cord injury in unprecedented detail
13 hours ago

'Time cells' in the brain are critical for complex learning, study shows
14 hours ago

Thalamocortical connectivity crucial for functional brain networks, study finds

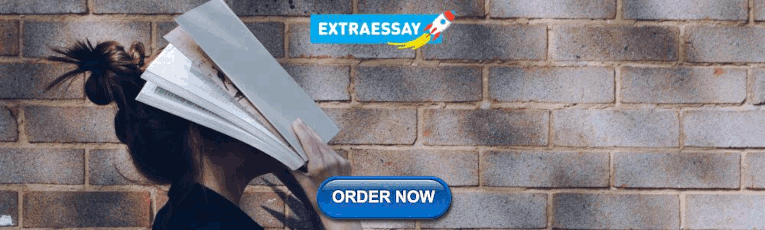
Researchers discover a significant problem in brain imaging and identify a fix
19 hours ago

Existing high blood pressure drugs may prevent epilepsy, study finds
Jun 18, 2024
Let us know if there is a problem with our content
Use this form if you have come across a typo, inaccuracy or would like to send an edit request for the content on this page. For general inquiries, please use our contact form . For general feedback, use the public comments section below (please adhere to guidelines ).
Please select the most appropriate category to facilitate processing of your request
Thank you for taking time to provide your feedback to the editors.
Your feedback is important to us. However, we do not guarantee individual replies due to the high volume of messages.
E-mail the story
Your email address is used only to let the recipient know who sent the email. Neither your address nor the recipient's address will be used for any other purpose. The information you enter will appear in your e-mail message and is not retained by Medical Xpress in any form.
Newsletter sign up
Get weekly and/or daily updates delivered to your inbox. You can unsubscribe at any time and we'll never share your details to third parties.
More information Privacy policy
Donate and enjoy an ad-free experience
We keep our content available to everyone. Consider supporting Science X's mission by getting a premium account.
E-mail newsletter

- June 19, 2024 | Cosmic Winds at Warp Speed: How Black Holes Propel Galaxy Evolution
- June 19, 2024 | Are Plants Smarter Than We Think? Cornell Scientists Uncover Form of Intelligence in Goldenrod Plants
- June 19, 2024 | How AI and 3D Printing Are Changing the Way We Grow Food
- June 19, 2024 | The Venom Cure: Ancient Predator Offers New Hope for Kidney Patients
- June 19, 2024 | Mysterious Pink Sand Deposits in Australia Reveal Existence of Previously Unknown Ancient Mountains
Reimagining Memory: New Research Reveals That Superconducting Loops Mimic the Brain
By University of California - San Diego April 19, 2024
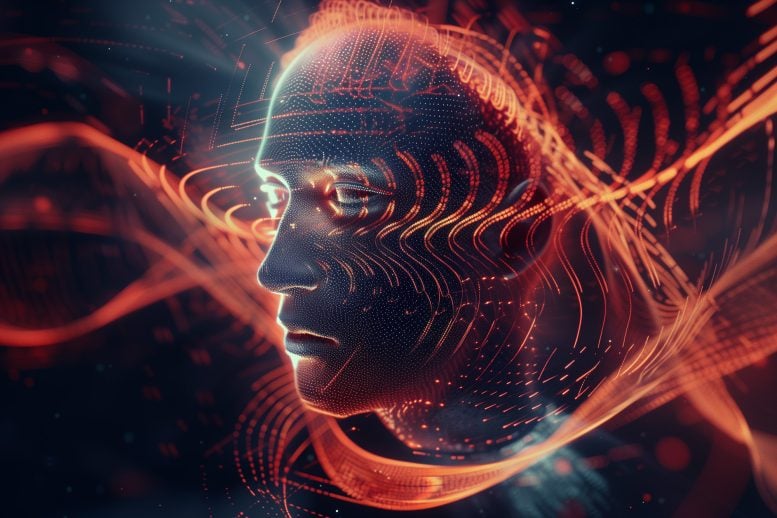
Researchers are exploring analog approaches to neuromorphic computing to address the high power demands of digital systems. A promising method from the University of California involves using disordered superconducting loops to store and transmit information, potentially enabling energy-efficient associative memory similar to human brain function.
Superconducting loops could allow computers to retain and retrieve information more efficiently.
Computers work in digits — 0s and 1s to be exact. Their calculations are digital; their processes are digital; even their memories are digital. All of which requires extraordinary power resources. As we look to the next evolution of computing and developing neuromorphic or “brain-like” computing, those power requirements are unfeasible.
To advance neuromorphic computing, some researchers are looking at analog improvements. In other words, not just advancing software, but advancing hardware too. Research from the University of California San Diego and UC Riverside shows a promising new way to store and transmit information using disordered superconducting loops.
The team’s research, which appears in the Proceedings of the National Academy of Sciences , offers the ability of superconducting loops to demonstrate associative memory, which, in humans, allows the brain to remember the relationship between two unrelated items.
“I hope what we’re designing, simulating and building will be able to do that kind of associative processing really fast,” stated UC San Diego Professor of Physics Robert C. Dynes, who is one of the paper’s co-authors.
Creating lasting memories
Picture it: you’re at a party and run into someone you haven’t seen in a while. You know their name but can’t quite recall it. Your brain starts to root around for the information: where did I meet this person? How were we introduced? If you’re lucky, your brain finds the pathway to retrieve what was missing. Sometimes, of course, you’re unlucky.
Dynes believes that short-term memory moves into long-term memory with repetition. In the case of a name, the more you see the person and use the name, the more deeply it is written into memory. This is why we still remember a song from when we were ten years old but can’t remember what we had for lunch yesterday.
“Our brains have this remarkable gift of associative memory, which we don’t really understand,” stated Dynes, who is also president emeritus of the University of California and former UC San Diego chancellor. “It can work through the probability of answers because it’s so highly interconnected. This computer brain we built and modeled is also highly interactive. If you input a signal, the whole computer brain knows you did it.”
Staying in the loop
How do disordered superconducting loops work? You need a superconducting material — in this case, the team used yttrium barium copper oxide (YBCO). Known as a high-temperature superconductor, YBCO becomes superconducting around 90 Kelvin (-297 F), which in the world of physics, is not that cold. This made it relatively easy to modify. The YBCO thin films (about 10 microns wide) were manipulated with a combination of magnetic fields and currents to create a single flux quantum on the loop. When the current was removed, the flux quantum stayed in the loop. Think of this as a piece of information or memory.
This is one loop, but associative memory and processing require at least two pieces of information. For this, Dynes used disordered loops, meaning the loops are different sizes and follow different patterns — essentially random.
A Josephson juncture, or “weak link,” as it is sometimes known, in each loop acted as a gate through which the flux quanta could pass. This is how information is transferred and the associations are built.
Although traditional computing architecture has continuous high-energy requirements, not just for processing but also for memory storage, these superconducting loops show significant power savings — on the scale of a million times less. This is because the loops only require power when performing logic tasks. Memories are stored in the physical superconducting material and can remain there permanently, as long as the loop remains superconducting.
The number of memory locations available increases exponentially with more loops: one loop has three locations, but three loops have 27. For this research, the team built four loops with 81 locations. Next, Dynes would like to expand the number of loops and the number memory locations.
“We know these loops can store memories. We know that associative memory works. We just don’t know how stable it is with a higher number of loops,” he said.
This work is not only noteworthy to physicists and computer engineers; it may also be important to neuroscientists. Dynes talked to another University of California president emeritus, Richard Atkinson, a world-renowned cognitive scientist who helped create a seminal model of human memory called the Atkinson-Shiffrin model.
Atkinson, who is also former UC San Diego chancellor and professor emeritus in the School of Social Sciences, was excited about the possibilities he saw: “Bob and I have had some great discussions trying to determine if his physics-based neural network could be used to model the Atkinson-Shiffrin theory of memory. His system is quite different from other proposed physics-based neural networks, and is rich enough that it could be used to explain the workings of the brain’s memory system in terms of the underlying physical process. It’s a very exciting prospect.”
Reference: “Collective neural network behavior in a dynamically driven disordered system of superconducting loops” by Uday S. Goteti, Shane A. Cybart and Robert C. Dynes, 12 March 2024, Proceedings of the National Academy of Sciences . DOI: 10.1073/pnas.2314995121
This work was primarily supported as part of the Quantum Materials for Energy Efficient Neuromorphic Computing (Q-MEEN-C) (Department of Energy DE-SC0019273). Other support was provided by the Department of Energy National Nuclear Security Agency (DE-NA0004106) and the Air Force Office of Scientific Research (FA9550-20-1-0144).
More on SciTechDaily
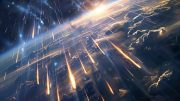
When Earth’s Magnetic Shield Faltered: Cosmic Ray Invasion 41,000 Years Ago
Fly your name around the moon on nasa’s artemis i.
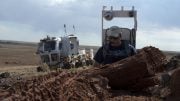
NASA to Practice Artemis Moonwalking, Roving Operations in Arizona Desert
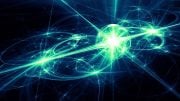
Standard Model of Particle Physics: The Absolutely Amazing Theory of Almost Everything
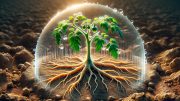
Rooted in Survival: Tomato Plants’ Ingenious Drought Defense Uncovered
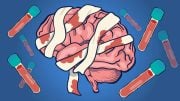
Early Blood Tests for Traumatic Brain Injury Can Accurately Predict Which Patients Are Likely To Die
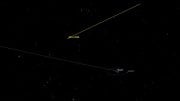
Near-Earth Asteroid 2014 JO25 Set to Fly Past Earth on April 19
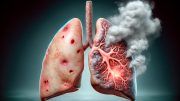
Warning: Study Shows Vaping Additives Harm a Vital Membrane in the Lungs
Be the first to comment on "reimagining memory: new research reveals that superconducting loops mimic the brain", leave a comment cancel reply.
Email address is optional. If provided, your email will not be published or shared.
Save my name, email, and website in this browser for the next time I comment.
The brain can store nearly 10 times more data than previously thought, study confirms
Scientists harnessed a new method to precisely measure the amount of information the brain can store, and it could help advance our understanding of learning.
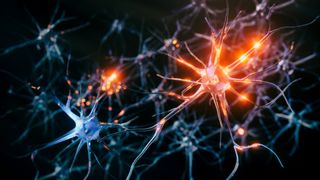
The brain may be able to hold nearly 10 times more information than previously thought, a new study confirms.
Similar to computers, the brain's memory storage is measured in "bits," and the number of bits it can hold rests on the connections between its neurons, known as synapses. Historically, scientists thought synapses came in a fairly limited number of sizes and strengths, and this in turn limited the brain's storage capacity. However, this theory has been challenged in recent years — and the new study further backs the idea that the brain can hold about 10-fold more than once thought.
In the new study, researchers developed a highly precise method to assess the strength of connections between neurons in part of a rat's brain. These synapses form the basis of learning and memory , as brain cells communicate at these points and thus store and share information.
By better understanding how synapses strengthen and weaken, and by how much, the scientists more precisely quantified how much information these connections can store. The analysis, published April 23 in the journal Neural Computation , demonstrates how this new method could not only increase our understanding of learning but also of aging and diseases that erode connections in the brain..
Related: The brain has a 'tell' for when it's recalling a false memory, study suggests
"These approaches get at the heart of the information processing capacity of neural circuits," Jai Yu , an assistant professor of neurophysiology at the University of Chicago who was not involved in the research, told Live Science in an email. "Being able to estimate how much information can potentially be represented is an important step towards understanding the capacity of the brain to perform complex computations."
In the human brain , there are more than 100 trillion synapses between neurons. Chemical messengers are launched across these synapses, facilitating the transfer of information across the brain. As we learn, the transfer of information through specific synapses increases. This "strengthening" of synapses enables us to retain the new information. In general, synapses strengthen or weaken in response to how active their constituent neurons are — a phenomenon called synaptic plasticity .
Sign up for the Live Science daily newsletter now
Get the world’s most fascinating discoveries delivered straight to your inbox.
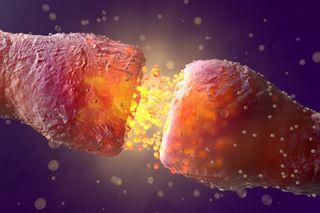
However, as we age or develop neurological diseases, such as Alzheimer's , our synapses become less active and thus weaken, reducing cognitive performance and our ability to store and retrieve memories.
Scientists can measure the strength of synapses by looking at their physical characteristics . Additionally, messages sent by one neuron will sometimes activate a pair of synapses, and scientists can use these pairs to study the precision of synaptic plasticity. In other words, given the same message, does each synapse in the pair strengthen or weaken in exactly the same way?
Measuring the precision of synaptic plasticity has proven difficult in the past, as has measuring how much information any given synapse can store. The new study changes that.
To measure synaptic strength and plasticity, the team harnessed information theory , a mathematical way of understanding how information is transmitted through a system. This approach also enables scientists to quantify how much information can be transmitted across synapses, while also taking account of the "background noise" of the brain.
— 'Short-term memory illusions' can warp human recollections just seconds after events, study suggests
— Neurons aren't the only cells that make memories in the brain, rodent study reveals
— 'Secret code' behind key type of memory revealed in new brain scans
This transmitted information is measured in bits, such that a synapse with a higher number of bits can store more information than one with fewer bits, Terrence Sejnowski , co-senior study author and head of the Computational Neurobiology Laboratory at The Salk Institute for Biological Studies, told Live Science in an email. One bit corresponds to a synapse sending transmissions at two strengths, while two bits allows for four strengths, and so on.
The team analyzed pairs of synapses from a rat hippocampus , a region of the brain that plays a major role in learning and memory formation. These synapse pairs were neighbors and they activated in response to the same type and amount of brain signals. The team determined that, given the same input, these pairs strengthened or weakened by exactly the same amount — suggesting the brain is highly precise when adjusting a given synapse's strength.
The analysis suggested that synapses in the hippocampus can store between 4.1 and 4.6 bits of information. The researchers had reached a similar conclusion in an earlier study of the rat brain, but at that time, they'd crunched the data with a less-precise method. The new study helps confirm what many neuroscientists now assume — that synapses carry much more than one bit each, Kevin Fox , a professor of neuroscience at Cardiff University in the U.K. who was not involved in the research, told Live Science in an email.
The findings are based on a very small area of the rat hippocampus, so it's unclear how they'd scale to a whole rat or human brain. It would be interesting to determine how this capacity for information storage varies across the brain and between species, Yu said.
In the future, the team's method could also be used to compare the storage capacity of different areas of the brain, Fox said. It could also be used to study a single area of the brain when it's healthy and when it's in a diseased state.
Ever wonder why some people build muscle more easily than others or why freckles come out in the sun ? Send us your questions about how the human body works to [email protected] with the subject line "Health Desk Q," and you may see your question answered on the website!
Emily is a health news writer based in London, United Kingdom. She holds a bachelor's degree in biology from Durham University and a master's degree in clinical and therapeutic neuroscience from Oxford University. She has worked in science communication, medical writing and as a local news reporter while undertaking journalism training. In 2018, she was named one of MHP Communications' 30 journalists to watch under 30. ( [email protected] )
Scientists inserted a window in a man's skull to read his brain with ultrasound
Why can't we see colors well in the dark?
'1st of its kind': NASA spots unusually light-colored boulder on Mars that may reveal clues of the planet's past
Most Popular
- 2 Y chromosome is evolving faster than the X, primate study reveals
- 3 Ming dynasty shipwrecks hide a treasure trove of artifacts in the South China Sea, excavation reveals
- 4 Gulf Stream's fate to be decided by climate 'tug-of-war'
- 5 Earth's rotating inner core is starting to slow down — and it could alter the length of our days
- 2 Newly deciphered papyrus describes 'miracle' performed by 5-year-old Jesus
- 3 Long-lost Assyrian military camp devastated by 'the angel of the Lord' finally found, scientist claims
- 4 Tuberculosis triggered giant, crusty wart to sprout on man's hand
- 5 Is Earth really getting too hot for people to survive?
Thank you for visiting nature.com. You are using a browser version with limited support for CSS. To obtain the best experience, we recommend you use a more up to date browser (or turn off compatibility mode in Internet Explorer). In the meantime, to ensure continued support, we are displaying the site without styles and JavaScript.
- View all journals
- Explore content
- About the journal
- Publish with us
- Sign up for alerts
- 12 June 2024
Sleep deprivation disrupts memory: here’s why
You can also search for this author in PubMed Google Scholar
You have full access to this article via your institution.
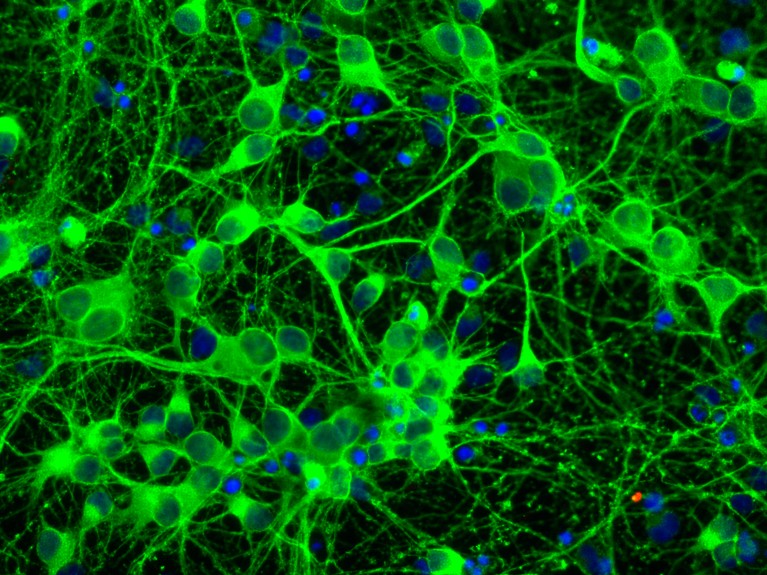
Neurons (artificially coloured) in the hippocampus play a part in learning and memory. Credit: Cell Applications Inc/Science Photo Library
A crucial brain signal linked to long-term memory falters in rats when they are deprived of sleep — which might help to explain why poor sleep disrupts memory formation 1 . Even a night of normal slumber after a poor night’s sleep isn’t enough to fix the brain signal .
These results, published today in Nature , suggest that there is a “critical window for memory processing”, says Loren Frank, a neuroscientist at the University of California, San Francisco, who was not involved with the study. “Once you’ve lost it, you’ve lost it.”
In time, these findings could lead to targeted treatments to improve memory , says study co-author Kamran Diba, a computational neuroscientist at the University of Michigan Medical School in Ann Arbor.
Firing in lockstep
Neurons in the brain seldom act alone; they are highly interconnected and often fire together in a rhythmic or repetitive pattern. One such pattern is the sharp-wave ripple , in which a large group of neurons fire with extreme synchrony, then a second large group of neurons does the same and so on, one after the other at a particular tempo. These ripples occur in a brain area called the hippocampus, which is key to memory formation . The patterns are thought to facilitate communication with the neocortex, where long-term memories are later stored.
One clue to their function is that some of these ripples are accelerated re-runs of brain-activity patterns that occurred during past events. For example, when an animal visits a particular spot in its cage, a specific group of neurons in the hippocampus fires in unison , creating a neural representation of that location. Later, these same neurons might participate in sharp-wave ripples — as if they were rapidly replaying snippets of that experience.
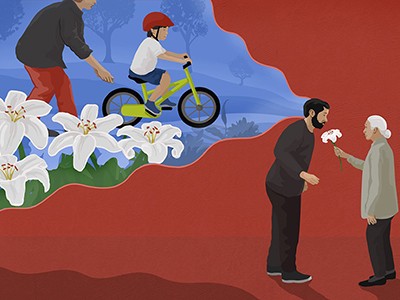
Unpicking the link between smell and memories
Previous research 2 found that, when these ripples were disturbed, mice struggled on a memory test. And when the ripples were prolonged, their performance on the same test improved 3 , leading György Buzsáki, a systems neuroscientist at NYU Langone Health in New York City, who has been researching these bursts since the 1980s, to call the ripples a ‘cognitive biomarker’ for memory and learning.
Researchers also noticed 4 that sharp-wave ripples tend to occur during deep sleep as well as during waking hours, and that those bursts during slumber seem to be particularly important for transforming short-term knowledge into long-term memories 5 . These links between the ripples, sleep and memory are well-documented, but there have been few studies that have directly manipulated sleep to determine how it affects these ripples, and in turn memory, Diba says.
Wake-up call
To understand how poor sleep affects memory, Diba and his colleagues recorded hippocampal activity in seven rats as they explored mazes over the course of several weeks. The researchers regularly disrupted the sleep of some of the animals and let others sleep at will.
To Diba’s surprise, rats that were woken up repeatedly had similar, or even higher, levels of sharp-wave-ripple activity than the rodents that got normal sleep did. But the firing of the ripples was weaker and less organized, showing a marked decrease in repetition of previous firing patterns. After the sleep-deprived animals recovered over the course of two days, re-creation of previous neural patterns rebounded, but never reached levels found in those which had normal sleep.
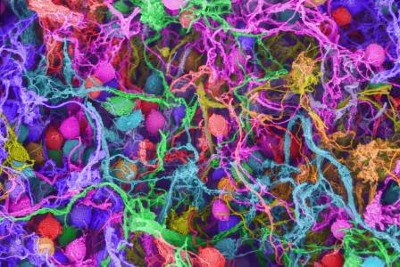
Memories are made by breaking DNA — and fixing it
This study makes clear that “memories continue to be processed after they’re experienced, and that post-experience processing is really important”, Frank says. He adds that it could explain why cramming before an exam or pulling an all-nighter might be an ineffective strategy.
It also teaches researchers an important lesson: the content of sharp-wave ripples is more important than its quantity, given that rats that got normal sleep and rats that were sleep-deprived had a similar number of ripples, he says.
Ripple effects
Buzsáki says that these findings square with data his group published in March 6 that found that sharp-wave ripples that occur while an animal is awake might help to select which experiences enter long-term memory.
It’s possible, he says, that the disorganized sharp-wave ripples of sleep-deprived rats don’t allow them to effectively flag experiences for long-term memory. As a result, the animals might be unable to replay the neural firing of those experiences at a later time.
This means that sleep disruption could be used to prevent memories from entering long-term storage, which could be useful for people who have recently experienced something traumatic, such as those with post-traumatic stress disorder, Buzsáki says.
Nature 630 , 542 (2024)
doi: https://doi.org/10.1038/d41586-024-01732-y
Giri, B. et al. Nature https://doi.org/10.1038/s41586-024-07538-2 (2024).
Article Google Scholar
Jadhav, S. P., Kemere, C., German, P. W. & Frank, L. M. Science 336 , 1454–1458 (2012).
Fernández-Ruiz, A. et al. Science 364 , 1082–1086 (2019).
Eschenko, O., Ramadan, W., Mölle, M., Born, J. & Sara, S. J. Learn. Mem. 15 , 222–228 (2008).
Article PubMed Google Scholar
Ramadan, W., Eschenko, O. & Sara, S. J. PLoS ONE 4 , e6697 (2009).
Yang, W. et al. Science 383 , 1478–1483 (2024).
Download references
Reprints and permissions
Related Articles
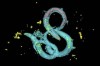
- Neuroscience
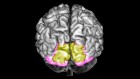
How the ‘mind’s eye’ calls up visual memories from the brain
News 14 JUN 24
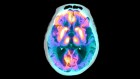
Alzheimer’s drug with modest benefits wins backing of FDA advisers
News 10 JUN 24
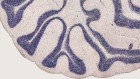
This injectable gel can help to diagnose brain injury — then it disappears
News 05 JUN 24
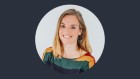
Put people at the heart of schizophrenia research
World View 19 JUN 24
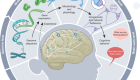
Large-scale neurophysiology and single-cell profiling in human neuroscience
Perspective 19 JUN 24
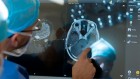
Human neuroscience is entering a new era — it mustn’t forget its human dimension
Editorial 19 JUN 24
Spatial & Single-Cell Computational Immunogenomics for Cancer Diagnosis
(Level A) $75,888 to $102,040 per annum plus an employer contribution of 17% superannuation applies. Fixed term, full time position available for ...
Adelaide (Suburb), Metropolitan Adelaide (AU)
University of Adelaide
Software Engineer for R and High-Performance Computing
Ai large-language models of single-cell data for cancer immunogenomics.
(Level B) $107,276 to $126,894 per annum plus an employer contribution of 17% superannuation applies. Fixed term, full time position available for...
Senior Research Associate (m/f/d) - permanent position - Institute of Neuropathology
Main topics of scientific projects are: Kallikrein-8 as an early biomarker and therapeutic Target for Alzheimer’s Disease Epigenetic effects of e...
Essen, Nordrhein-Westfalen (DE)
Universitätsklinikum (AöR)
Department of Health and Human Services (DHHS), is seeking exceptional candidates for the position of Director
Bethesda, Maryland (US)
National Institutes of Health
Sign up for the Nature Briefing newsletter — what matters in science, free to your inbox daily.
Quick links
- Explore articles by subject
- Guide to authors
- Editorial policies
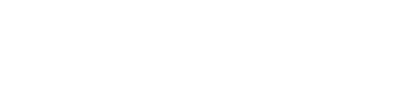
Along with Stanford news and stories, show me:
- Student information
- Faculty/Staff information
We want to provide announcements, events, leadership messages and resources that are relevant to you. Your selection is stored in a browser cookie which you can remove at any time using “Clear all personalization” below.
The smartphones that are now ubiquitous were just gaining popularity when Anthony Wagner became interested in the research of his Stanford colleague, Clifford Nass, on the effects of media multitasking and attention. Though Wagner, a professor of psychology at Stanford University and director of the Stanford Memory Laboratory , wasn’t convinced by the early data, he recommended some cognitive tests for Nass to use in subsequent experiments. More than 11 years later, Wagner was intrigued enough to write a review on past research findings, published in Proceedings of the National Academy of Sciences , and contribute some of his own.
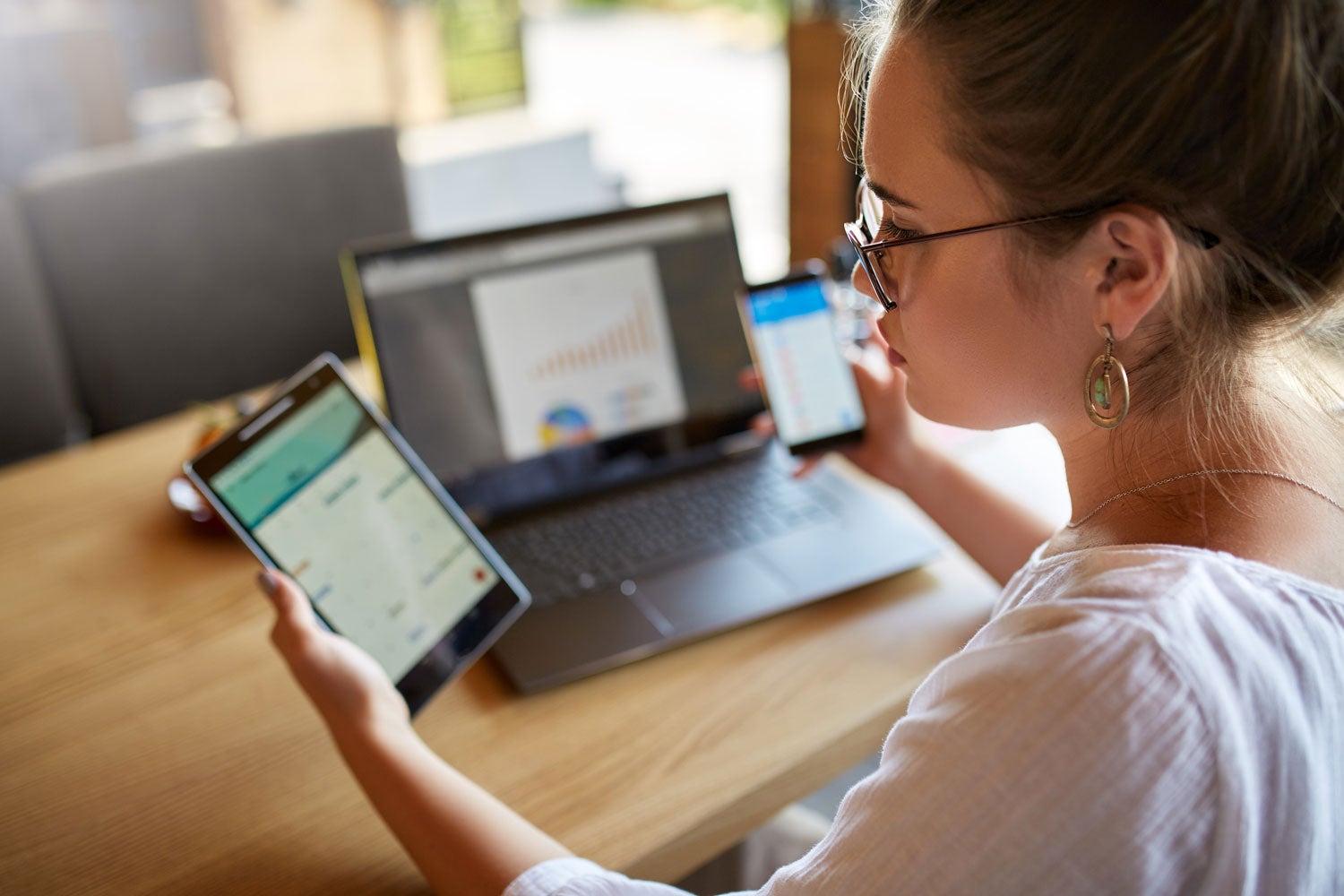
A decade’s worth of research has shown that people who frequently use many types of media at once performed significantly worse on simple memory tasks. (Image credit: Getty Images)
The paper , co-authored with neuroscientist Melina Uncapher of the University of California, San Francisco, summarizes a decade’s worth of research on the relationship between media multitasking and various domains of cognition, including working memory and attention. In doing that analysis, Wagner noticed a trend emerging in the literature: People who frequently use many types of media at once, or heavy media multitaskers, performed significantly worse on simple memory tasks.
Wagner spoke with Stanford Report to explain the findings from his review on media multitasking and cognition, and discuss why it’s premature to determine the impact of these results.
How did you become interested in researching media multitasking and memory?
I was brought into a collaboration with Cliff Nass, a Stanford faculty member in communication who passed away a few years ago, and his master’s student, Eyal Ophir. They had this question: With the explosion of media technologies that has resulted in there being multiple simultaneous channels available that we can switch between, how might this relate to human cognition? Eyal and Cliff would come chat with me about their early findings and – I have to say – I thought it was complete hooey. I was skeptical. But, after a few experiments, the data were increasingly pointing to a link between media multitasking and attention. Their findings struck me as potentially important given the way we’re living as humans in this attention economy. Years later, as a memory scientist my interests continued to grow. Given that attention and cognitive control are so fundamental for memory, I wanted to see if there was a relationship between media multitasking and memory.
How do you define media multitasking, and can you give hypothetical examples of people that would be “heavy” and “light” media multitaskers?
Well, we don’t multitask. We task switch. The word “multitasking” implies that you can do two or more things at once, but in reality our brains only allow us to do one thing at a time and we have to switch back and forth.
Heavy media multitaskers have many media channels open at once and they switch between them. A heavy media multitasker might be writing an academic paper on their laptop, occasionally checking the Stanford basketball game on TV, responding to texts and Facebook messages, then getting back to writing – but then an email pops up and they check it. A light media multitasker would only be writing the academic paper or may only switch between a couple of media. They may turn off Wi-Fi, put away their phone or change their settings so they only get notified every hour. Those are some extreme examples, but they provide a sense of how people differ in their media use. Moreover, because our media landscape has continued to accelerate and change, those who are considered a heavy or light media multitasker today may not be the same as those a decade ago.
How do scientists assess someone’s memory?
There are many forms of memory, and thus many ways of probing memory in the lab. For working memory – the ability to keep a limited amount of information active in mind – we often use simple short-delay memory tasks. For example, in one test we show a set of oriented blue rectangles, then remove them from the screen and ask the subject to retain that information in mind. Then we’ll show them another set of rectangles and ask if any have changed orientation. To measure memory capacity, we do this task with a different number of rectangles and determine how performance changes with increasing memory loads. To measure the ability to filter out distraction, sometimes we add distractors, like red rectangles that the subjects are told to ignore.
What overall trends did you notice when you were looking through the literature to write this review?
In about half of the studies, the heavy media multitaskers are significantly underperforming on tasks of working memory and sustained attention. The other half are null results; there’s no significant difference. It strikes me as pretty clear that there is a negative relationship between media multitasking and memory performance – that high media multitasking is associated with poor performance on cognitive memory tasks. There’s not a single published paper that shows a significant positive relationship between working memory capacity and multitasking.
In the review we noticed an interesting potential emerging story. One possibility is that reduced working memory occurs in heavy media multitaskers because they have a higher probability of experiencing lapses of attention. When demands are low, they underperform. But, when the task demands are high, such as when the working memory tasks are harder, there’s no difference between the heavy and light media multitaskers. This observation, combined with the negative relationship between multitasking and performance on sustained attention tasks, prompted us to start looking at intrasubject variability and moment-to-moment fluctuations in a person’s ability to use task goals to direct attention in a sustained manner.
How do these findings affect how people should engage with media, or should they at all?
I would never tell anyone that the data unambiguously show that media multitasking causes a change in attention and memory. That would be premature. It’s too early to definitively determine cause and effect.
One could choose to be cautious, however. Many of us have felt like our technology and media are controlling us – that email chime or text tone demands our attention. But we can control that by adopting approaches that minimize habitual multitasking; we can decide to be more thoughtful and reflective users of media.
That said, multitasking isn’t efficient. We know there are costs of task switching. So that might be an argument to do less media multitasking – at least when working on a project that matters academically or professionally. If you’re multitasking while doing something significant, like an academic paper or work project, you’ll be slower to complete it and you might be less successful.
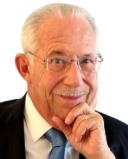
How Brain Waves Influence Your Memory
A new study discovers how the brain holds information in your working memory..
Posted June 2, 2024 | Reviewed by Lybi Ma
- Brain waves influence cognitive control and memory formation, informing how the brain manages memory.
- Working memory stores and manipulates information temporarily for tasks like learning and decision-making.
- Phase-amplitude coupling neurons synchronize with brain waves, aiding cognitive control and memory retrieval.
- New findings have implications for therapies and cognitive enhancement strategies.
Have you ever put your keys down and then completely forgotten where to find them? The brain has to work hard to protect information in your working memory from distractions. How this process works has, until recently, been unclear. A study published in Nature looked at the interactions between the front part of the brain that controls thoughts and the hippocampus, which is important for memory. They found that individual brain cells are influenced by the theta and gamma brain waves passing between these two parts of the brain and those cells have a role in cognitive control. These findings give fresh insights into how the brain manages memory, with implications for those who wish to improve their attention , decision-making , or memory retrieval.
What is working memory?
Working memory is a type of short-term memory that temporarily stores and manipulates information needed for cognitive tasks such as learning, reasoning, and comprehension. It acts as a mental workspace where information is held and processed before being encoded into long-term memory or forgotten.
Working memory is limited to holding a small amount of information (around seven items) for a brief period ranging from a few seconds to several minutes, depending on the task and the individual's cognitive abilities. Working memory coordinates the simultaneous storage and processing of information from multiple sources and plays a crucial role in higher cognitive functions like problem-solving, decision-making, and following instructions.
How does the brain make memories?
The brain makes memories through a complex process involving multiple brain regions and mechanisms. Memories are initially encoded in the hippocampus region of the brain. This process involves strengthening the connections (synapses) between neurons through repeated stimulation.
The hippocampus and the prefrontal cortex are two important brain regions in memory formation. During memory formation, the hippocampus and prefrontal cortex communicate via brain waves of different frequencies (beta and theta). Beta waves reinforce correct associations, while theta waves weaken incorrect ones, guiding what the brain learns.
The coordination of interactions between the hippocampus and prefrontal cortex is called theta-gamma phase-amplitude coupling. They work together, creating a certain rhythm. Theta waves are slower while gamma waves are faster. They are synched so that when one gets stronger, so does the other. This coordination may facilitate neural dynamics for memory and cognitive processing, integrating local sensory information processing with brain-wide cognitive control.
Studying working memory and memory retrieval
To understand the ways neurons involved in theta-gamma phase-amplitude coupling influence memory and thinking, a team of researchers at Cedars-Sinai Medical Center, Toronto Western Hospital, and Johns Hopkins Hospital, conducted a study on 36 epilepsy patients undergoing surgery for drug-resistant epilepsy using magnetic resonance imaging and electroencephalograms.
In the task, participants observed 140 rounds of different pictures. Each round started with a cross, and then one or three pictures appeared for the patient to remember. They had to hold them in memory when prompted, and later identify if a new picture matched any from that round by pressing a button.
Each session used new pictures from various categories like faces, animals, and cars, ensuring freshness. Harder rounds challenged patients with three pictures instead of one, maintaining consistent time limits for memory recall. Mixing categories prevented reliance on familiarity, encouraging active memory use over recognition.

Electrodes with at least eight wires recorded brain activity across various frequencies. The researchers examined how phase-amplitude coupling varied from trial to trial; they identified specific neurons that were sensitive to different categories of visual stimuli presented during the task that helped explain how individual neurons responded to specific types of images.
Findings and Implications
The study reveals a direct link between theta-gamma phase-amplitude coupling and the firing patterns of single neurons. Neurons showing phase-amplitude coupling synchronize with frontal theta waves, particularly when working memory load is higher, leading to faster reaction times. This suggests that phase-amplitude coupling neurons play a role in cognitive control.
To accurately retain and access memories is the main goal of cognitive control. The study demonstrated that phase-amplitude coupling neurons contribute to this process by introducing noise correlations, which improves information content at the population level. Noise correlations enhance the decodability of working memory content, particularly when involving phase-amplitude coupling neurons.
The findings of this study support a model where frontal control processes regulate working memory maintenance in brain areas like the hippocampus. The interactions between phase-amplitude coupling neurons in the broader theta-gamma phase-amplitude coupling phenomenon represent a general mechanism for top-down control in various cognitive functions beyond working memory such as attention, decision-making, speech comprehension, and long-term memory retrieval.
Understanding the memory process can lead to the development of therapies for conditions involving memory deficits or those suffering from neurological disorders. It can also lead to strategies for enhancing cognitive performance by targeting specific neural mechanisms involved in memory and cognitive control and optimizing learning and memory retention in academic settings. In summary, the study examines the connection between brain waves, cognitive control, and memory, offering promising ways to understand the neurological basis of cognition as well as opportunities to develop innovative interventions to enhance memory and cognitive function.
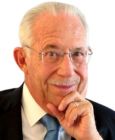
William A. Haseltine, Ph.D., is known for his pioneering work on cancer, HIV/AIDS, and genomics. He is Chair and President of the global health think tank Access Health International. His recent books include My Lifelong Fight Against Disease.
- Find a Therapist
- Find a Treatment Center
- Find a Psychiatrist
- Find a Support Group
- Find Online Therapy
- International
- New Zealand
- South Africa
- Switzerland
- Asperger's
- Bipolar Disorder
- Chronic Pain
- Eating Disorders
- Passive Aggression
- Personality
- Goal Setting
- Positive Psychology
- Stopping Smoking
- Low Sexual Desire
- Relationships
- Child Development
- Self Tests NEW
- Therapy Center
- Diagnosis Dictionary
- Types of Therapy
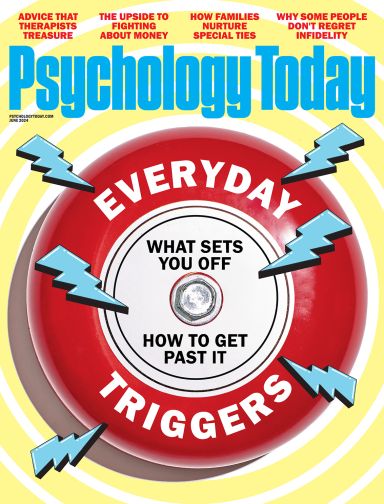
At any moment, someone’s aggravating behavior or our own bad luck can set us off on an emotional spiral that threatens to derail our entire day. Here’s how we can face our triggers with less reactivity so that we can get on with our lives.
- Emotional Intelligence
- Gaslighting
- Affective Forecasting
- Neuroscience
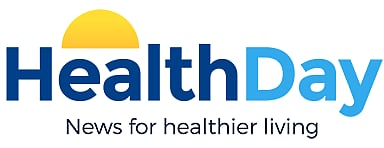
- Health A to Z
- Alternative Medicine
- Blood Disorders
- Bone and Joint
- Cardiovascular Diseases
- Child Health
- Coronavirus
- Dental Care
- Digestive System
- Disabilities
- Drug Center
- Ear, Nose, and Throat
- Environmental Health
- Exercise And Fitness
- First Aid and Emergencies
- General Health
- Health Technology
- Hearing Loss
- Hypertension
- Infectious Disease
- LGBTQ Health
- Liver Health
- Men's Health
- Mental Health
- Pain Management
- Public Health
- Pulmonology
- Senior Health
- Sexual Health
- Skin Health
- Women's Health
- News For Medical Professionals
- Our Products
- Consumer News
- Physician's Briefing
- HealthDay TV
- Wellness Library
- HealthDay Living
- Conference Coverage
- Custom Products
- Health Writing
- Health Editing
- Video Production
- Medical Review
Depression Could Take Toll on Memory With Age
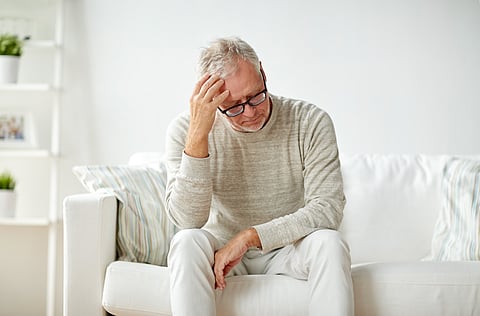
Key Takeaways
New research finds that depression and memory decline may be strongly linked
Having one upped the odds for the other, and numerous neurological mechanisms could explain the connection
Depression treatment could be key to preserving memory with age
WEDNESDAY, June 12, 2024 (HealthDay News) -- Depression and memory declines may be closely linked in older people, new research suggests.
“Our study shows that the relationship between depression and poor memory cuts both ways, with depressive symptoms preceding memory decline and memory decline linked to subsequent depressive symptoms," said senior study author Dr. Dorina Cadar , of University College London.
The study suggests that effective depression treatment could help preserve memory function with age, she added in a university news release.
The new findings come from an analysis of data from the ongoing English Longitudinal Study of Ageing, where people are tracked by questionnaires every two years.
In this sample, 16 years of data were collected from nearly 8,300 adults averaging 64 years of age.
In contrast to the finding that memory and depression were closely intertwined, the research did not show a strong relationship between depression and another marker of brain health, verbal fluency.
Cadar, who is a research fellow in dementia at UCL, said it's not surprising that battles with depression could affect memory over time.
“Depression can cause changes in brain structures, such as the hippocampus, which is critical for memory formation and retrieval," she explained. "Chronic stress and high levels of [the stress hormone] cortisol associated with depression can damage neurons in these areas."
The researchers also believe that depression upsets the balance of serotonin and dopamine in the brain, and disrupts the brain's ability to repair and reorganize vital connections.
Memory troubles might also be linked to the tendency in depression to ruminate -- repeatedly thinking about certain things or dwelling on negative feelings.
On the flip side, a life spent dealing with memory lapses could trigger insecurity, frustration and other feelings that can be triggers for depressive episodes, Cadar's group reasoned. Memory troubles might also lead one to more social isolation.
“These findings underscore the importance of monitoring memory changes in older adults with increasing depressive symptoms, to identify memory loss early and prevent further worsening of depressive function," said study lead author Jiamin Yin , who has since graduated from UCL and is now a doctoral student at the University of Rochester in New York.
So, if and when depressive symptoms arise, it is "critical" to treat them to prevent folks "from developing depression and memory dysfunction," Yin said.
The findings were published June 11 in the journal JAMA Network Open .
More information
Find out more about treating depression at the Cleveland Clinic .
SOURCE: University College London, news release, June 11, 2024
What This Means For You
If you are battling depression, be sure to get help: Research suggests depression can speed declines in memory.
Related Stories


Sound Therapy May Improve Sleep
Summary: A new study reveals that stimulating alpha brain waves using sound may improve sleep in individuals with dementia. The technique, known as Alpha Closed-Loop Auditory Stimulation (aCLAS), can speed up or slow down alpha rhythms depending on the timing of the sound. This non-invasive approach could offer a new therapeutic avenue for sleep disturbances in dementia.
- Sound stimulation can modify alpha brain waves, which are linked to memory and perception.
- aCLAS technique can speed up or slow down alpha rhythms depending on the timing of the sound.
- This approach could potentially improve sleep in individuals with dementia.
Source: University of Surrey
Using sound to stimulate certain brain waves has the potential to help those with dementia or cognitive decline sleep better, reveals a new study. Sleep disturbances are a common feature in dementia and may affect up to half of people living with the condition.
During the study, the research team from the University of Surrey and the UK Dementia Research Institute Centre for Care Research & Technology at Imperial College London, used sound stimulation to target alpha rhythms, a type of brainwave, at precise timings of the wave to investigate how the brain responds.
Alpha rhythms have been associated with memory and perception, and changes to the rhythms have been observed in those experiencing cognitive decline and dementia.
Senior author Dr Ines Violante, Senior Lecturer in Psychological Neuroscience at the University of Surrey, said:
“Alpha oscillations are a defining characteristic of our brain’s electrical activity, but we still do not fully understand their role in shaping fundamental brain functions.
“Using sound is a powerful, non-invasive approach to stimulate certain oscillations within the brain. It is important that we find ways of manipulating these oscillations to create tools for treatment applications, as we know that brain oscillations are slower in diseases, such as Alzheimer’s disease.”
In a series of experiments, researchers used an innovative brain modulation technique known as Alpha Closed-Loop Auditory Stimulation (aCLAS) , in which sounds are timed to the precise phase of alpha rhythms. To monitor the effect of stimulation, measurements of electrical activity from the brain were continuously read in real-time, and when a brain wave reached a particular phase, a sound (a burst of pink noise) was played on the participant.
Researchers observed that depending on the phase at which the sound was played, the alpha rhythm became faster or slower. The effect was also dependent on where the alpha oscillations were coming from in the brain.
Dr Henry Hebron, a former doctoral student at the University of Surrey and first author of the publication, said:
“What we have found is that alpha oscillations can be manipulated via sound when we address this rhythm on its own terms, using a closed-loop approach. Surprisingly, when we performed our aCLAS experiment as participants were falling asleep, we observed that sounds at a particular phase prevented them from reaching deeper stages of sleep (without waking them), while the same sounds at a different phase were not disruptive.
“There is a lot more to be explored regarding neural oscillations-dependent behaviours, and we believe closed-loop approaches, such as the one we implemented here, could be key.”
According to researchers, now they have shown they are able to influence Alpha waves with sound, the next steps will be to explore if they can modify the waves in such a way that enhances cognition and sleep, which could ultimately benefit dementia patients.
Professor Derk-Jan Dijk, Director of the Surrey Sleep Research Centre and Group Leader at the UK Dementia Research Institute Centre for Care Research & Technology Centre, said:
“There is much to be uncovered about the role of the alpha rhythm in sleep and cognition. This technique could be influential in pushing our understanding and improving sleep functions in those with dementia. We are now investigating the effects of this closed-loop auditory stimulation approach in REM sleep, where alpha rhythms are present but their role still unknown.”
The research contributes to the United Nations’ Sustainable Development Goal 3 – Good Health and Well-being.
About this auditory neuroscience and sleep research news
Author: Natasha Meredith Source: University of Surrey Contact: Natasha Meredith – University of Surrey Image: The image is credited to Neuroscience News
Original Research: Open access. “ A closed-loop auditory stimulation approach selectively modulates alpha oscillations and sleep onset dynamics in humans ” by Ines Violante et al. PLOS Biology
A closed-loop auditory stimulation approach selectively modulates alpha oscillations and sleep onset dynamics in humans
Alpha oscillations play a vital role in managing the brain’s resources, inhibiting neural activity as a function of their phase and amplitude, and are changed in many brain disorders.
Developing minimally invasive tools to modulate alpha activity and identifying the parameters that determine its response to exogenous modulators is essential for the implementation of focussed interventions.
We introduce Alpha Closed-Loop Auditory Stimulation (αCLAS) as an EEG-based method to modulate and investigate these brain rhythms in humans with specificity and selectivity, using targeted auditory stimulation.
Across a series of independent experiments, we demonstrate that αCLAS alters alpha power, frequency, and connectivity in a phase, amplitude, and topography-dependent manner.
Using single-pulse-αCLAS, we show that the effects of auditory stimuli on alpha oscillations can be explained within the theoretical framework of oscillator theory and a phase-reset mechanism.
Finally, we demonstrate the functional relevance of our approach by showing that αCLAS can interfere with sleep onset dynamics in a phase-dependent manner.

Time Cells Play Key Role in Learning Complex Tasks

AI Decodes Emotions in Tennis Players
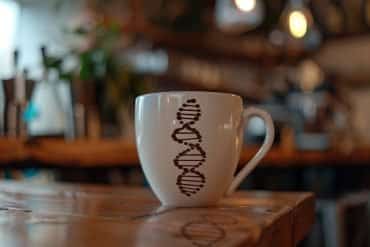
Your Caffeine Craving: Genetic or Learned?
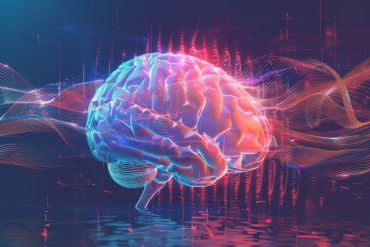
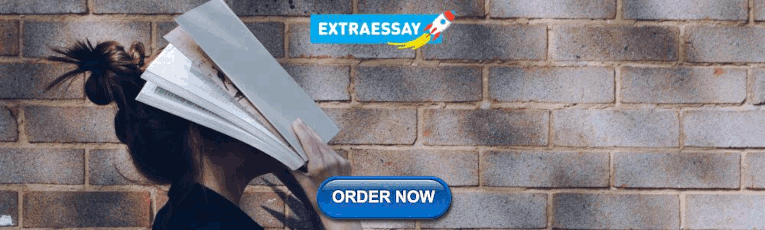
IMAGES
VIDEO
COMMENTS
Working memory. Working memory is primarily associated with the prefrontal and posterior parietal cortex (Sarnthein et al., 1998; Todd and Marois, 2005).Working memory is not localized to a single brain region, and research suggests that it is an emergent property arising from functional interactions between the prefrontal cortex (PFC) and the rest of the brain (D'Esposito, 2007).
New research published in Nature Neuroscience published on January 19, reveals that this process occurs on a cellular level, findings that are critical to the understanding and treatment of memory ...
The research focuses on engrams, which are neuronal cells in the brain that store memory information. "Engrams are the neurons that are reactivated to support memory recall," says Dheeraj S. Roy, one of the paper's senior authors and an assistant professor in the Department of Physiology and Biophysics, Jacobs School of Medicine and Biomedical Sciences.
Memory is the process of retaining of knowledge over a period for the function of affecting future actions.[] From a historical standpoint, the area of memory research from 1870 to 1920 was focused mainly on human memory.[] The book: The Principles of Psychology written by famous psychologist William James suggested that there is a difference between memory and habit.[]
The memory transfer experiments did, nonetheless, stimulate research that revealed a role for protein synthesis in long-term retention (Davis and Squire, 1984). Consequently, Milner (1970) could conclude that the search for molecular changes in learning should be directed to proteins and lipids, a sentiment that has stood the test of time ...
New research published in Nature Neuroscience today reveals how this process occurs on a cellular level, findings that are critical to the understanding and treatment of memory disorders, such as Alzheimer's disease. Dynamic engrams store memories. The research focuses on engrams, which are neuronal cells in the brain that store memory ...
McGovern Institute for Brain Research, Massachusetts Institute of Technology, Cambridge, MA, 02139, USA ... Park, YG., Kim, M.E. et al. Brain-wide mapping reveals that engrams for a single memory ...
Dynamic engrams store memories. The research focuses on engrams, which are neuronal cells in the brain that store memory information. "Engrams are the neurons that are reactivated to support ...
In this study, 27 epilepsy patients who had the electrodes implanted at UT Southwestern and a Pennsylvania hospital participated in memory tasks to generate data for brain research.
European Journal of Psychology of Education (2024) Memory retrieval involves the interaction between external sensory or internally generated cues and stored memory traces (or engrams) in a ...
By University of Basel September 27, 2023. Researchers at the University of Basel found a direct link between activity in certain brain regions like the hippocampus and memory performance, using the world's largest functional imaging study on memory, involving nearly 1,500 participants. The findings, which revealed stronger brain activation ...
Further investigation has recently revealed that other than the generally informed cortical structures involved in verbal working memory, basal ganglia, which lies in the subcortical layer, plays a role too (Moore et al., 2013).Particularly, the caudate and thalamus were activated during task encoding, and the medial thalamus during the maintenance phase, while recorded activity in the fronto ...
The crystallization of memory: Study reveals how practice forms new memory pathways in the brain. The effects of optogenetic inhibition on WM task performance. a, The experimental set-up. b, The ...
The research focuses on engrams, which are neuronal cells in the brain that store memory information. "Engrams are the neurons that are reactivated to support memory recall," says Dheeraj S. Roy, PhD, one of the paper's senior authors and an assistant professor in the Department of Physiology and Biophysics in the Jacobs School of ...
A recent study conducted by UCLA Health has revealed that repetitive practice not only enhances skill acquisition but also significantly alters the brain's pathways for memory. The research, published in the journal Nature and co-led by Rockefeller University, sought to unravel how the brain's ability to retain and process information ...
The team's research, which appears in the Proceedings of the National Academy of Sciences, offers the ability of superconducting loops to demonstrate associative memory, which, in humans, allows the brain to remember the relationship between two unrelated items. "I hope what we're designing, simulating and building will be able to do that ...
The prevalent object-based theory in cognitive research predicts that memory manipulation is performed on the level of objects. ... Awh E. (2021). Perceptual grouping reveals distinct roles for sustained slow wave activity and alpha oscillations in working memory. Journal of Cognitive Neuroscience, 33(7), 1354-1364. Crossref. PubMed. Google ...
—'Short-term memory illusions' can warp human recollections just seconds after events, study suggests — Neurons aren't the only cells that make memories in the brain, rodent study reveals
Encoded. Changes in the strength of neural connection. Consolidation. Research on memory consolidation reveals that memories: Are aided by sleep. Uchenna. Get some sleep. Recent research suggest that negative memories may be erased through: Blocking of reconsolidation.
A crucial brain signal linked to long-term memory falters in rats when they are deprived of sleep — which might help to explain why poor sleep disrupts memory formation 1. Even a night of normal ...
A decade of data reveals that heavy multitaskers have reduced memory, Stanford psychologist says. People who frequently engage with multiple types of media at once performed worse on simple memory ...
Research on memory construction reveals that memories A) are stored as exact copies of experience. B) reflect a person's biases and assumptions. C) may be chemically transferred from one organism to another. ... In Sperling's memory experiment, research participants were shown three rows of three letters, followed immediately by a low, medium ...
Study with Quizlet and memorize flashcards containing terms like 1) In the store model, input in the information-processing system A) enters the sensory register and is stored momentarily. B) simply flows on its own through the various stores. C) is automatically transferred into long-term memory. D) enters the sensory register and is stored for days or weeks, 2) Individual differences on ...
It reveals current areas of agreement and contention among scientists, and it may therefore serve as a signpost of areas of research that need further study. ... Nine experts voiced concerns about the applicability of eyewitness memory research to actual policies governing the collection of eyewitness evidence. Six experts asked about the best ...
Working memory is limited to holding a small amount of information (around seven items) for a brief period ranging from a few seconds to several minutes, depending on the task and the individual's ...
Dec. 23, 2022 — A research group has revealed that a transcription cofactor gene called CREB-Regulated Transcription Coactivator 1 (CRTC1) mediates the obesity-suppressing effects of ...
WEDNESDAY, June 12, 2024 (HealthDay News) -- Depression and memory declines may be closely linked in older people, new research suggests. "Our study shows that the relationship between depression and poor memory cuts both ways, with depressive symptoms preceding memory decline and memory decline linked to subsequent depressive symptoms," said senior study author Dr. Dorina Cadar, of ...
A new study reveals that stimulating alpha brain waves using sound may improve sleep in individuals with dementia. ... which are linked to memory and perception. ... the research team from the University of Surrey and the UK Dementia Research Institute Centre for Care Research & Technology at Imperial College London, used sound stimulation to ...
The Remembering Gene Wilder documentary was an insightful look at an extraordinary actor's life in front of and away from the camera that revealed many details viewers might not have been aware of ...
The doctor is Rep. Ronny Jackson, a Republican from Texas, today an open Trumpist in Congress. In the regime of contemplating the weekend episode, Setoodeh wrote, 'Donald Trump had severe memory ...