- Search Menu
- Sign in through your institution
- Advance Articles
- Case of the Year
- Competition Winners
- Grand Rounds
- ESC Content Collections
- Author Guidelines
- Submission Site
- Open Access Options
- Self-Archiving Policy
- About European Heart Journal - Case Reports
- About European Society of Cardiology
- ESC Publications
- Editorial Board
- Editor and Reviewer Programmes
- Advertising & Corporate Services
- Journals Career Network
- Code of Conduct
- Publons: Recognising Review
- Journals on Oxford Academic
- Books on Oxford Academic

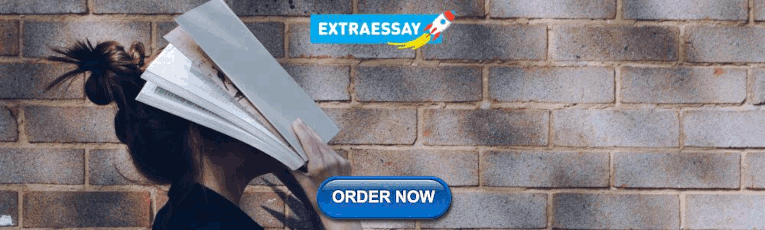
Article Contents
Introduction, case presentation, lead author biography, supplementary material.
- < Previous
Atrial fibrillation in heart failure with reduced ejection fraction: a case report of exercise training

- Article contents
- Figures & tables
- Supplementary Data
Guilherme Veiga Guimarães, Jean Marcelo Roque, Alexander D T Machado, Miguel Morita Fernandes-Silva, Paul Roberto Chizzola, Edimar Alcides Bocchi, Atrial fibrillation in heart failure with reduced ejection fraction: a case report of exercise training, European Heart Journal - Case Reports , Volume 4, Issue 5, October 2020, Pages 1–5, https://doi.org/10.1093/ehjcr/ytaa364
- Permissions Icon Permissions
Heart failure (HF) and atrial fibrillation (AF) are often concomitant and act in a vicious cycle. Atrial fibrillation is associated with greater functional limitations and increased morbidity and mortality in patients with HF. Moreover, AF associated with HF increases patients' physical inactivity, worsening their clinical condition, and prognosis. Exercise training is safe and has clear benefits in HF. However, these benefits have not been demonstrated when AF is associated with HF.
We present the case of a 57-year-old man with permanent AF and HF with reduced ejection fraction, who underwent 12 weeks of exercise training that included cardiopulmonary exercise testing, neuromuscular sympathetic activity (NMSA), and muscle blood flow (MBF) before and after training.
Exercise training was shown to have a potential benefit in reducing the activity of the sympathetic nerve and increasing muscle blood flow, as well as increasing VO 2peak and decreasing the VE/VCO 2 slope in a patient with AF associated with HF with reduced ejection fraction. These results may indicate favourable clinical implications in this group of patients.
Exercise training increases cardiopulmonary performance improving muscle blood flow. It should be recommended to patients with atrial fibrillation associated with heart failure.
The increased sensitivity of the arterial baroreflex re-establishing control over sympathetic activity, leading to improved muscle flow and exercise capacity.
These results may indicate favourable clinical implications in this group of patients.
Heart failure (HF) and atrial fibrillation (AF) are often concurrent clinical conditions, and they may be the cause or consequence of each other, progressing in a vicious cycle. 1 Approximately 40% of patients with HF present AF, leading to greater functional impairment and increased morbidity and mortality. 2 Restoration and maintenance of sinus rhythm is challenging in these patients, with a low long-term success rate of cardioversion and ablation in patients with AF and HF. 1 , 2
Regular physical activity is an evidence-based adjuvant therapy for HF. Exercise training attenuates the activity of the sympathetic nervous system and improves functional capacity, leading to lower rates of hospitalization and better quality of life. 3–5 However, studies evaluating the effects of exercise in patients with HF and AF are lacking.
Physical inactivity may worsen the exercise intolerance in patients with AF and HF, aggravating the clinical condition, increasing the risk of hospitalization, and worsening quality of life. 1 , 2 Physical activity can help break the vicious cycle of low functional capacity resulting from the binary AF and HF and should be recommended to these patients, as long as they are clinically stable, with optimized clinical treatment according to guidelines, with a resting heart rate ≤80 b.p.m. and ≤110% of the predicted for their maximum exercise age. 6
This case report shows the changes on physical capacity, neuromuscular sympathetic activity (NMSA), and muscular blood flow (MBF) after a supervised exercise training guided by the Borg scale in a patient with AF associated with HF and reduced left ventricular ejection fraction (LVEF).
A 57-year-old Caucasian man diagnosed with HF due to hypertensive dilated cardiomyopathy associated with permanent AF was referred to an exercise training program. He had non-insulin-dependent diabetes mellitus and LVEF was 35%. The functional class was III [(New York Heart Association (NYHA)] and drug therapy was according to the III Brazilian Guideline on Heart Failure and II Brazilian Guideline on Atrial Fibrillation: Losartan 100 mg, Furosemide 40 mg, Carvedilol 150 mg, Warfarin 5 mg, Simvastatin 20 mg, Metformin 1700 mg, and Omeprazole 20 mg. Because of a long history of AF, rate control strategy was implemented. The patient did not have a history of neuromuscular or pulmonary disease. He never smoke and did not drink alcohol. According to his level of physical activity, he led a sedentary life, defined as self-reported less than 1 day/week of planned, structured, and repetitive exercise. His body mass index was 36.8 kg/m 2 and resting blood pressure was 115/80 mmHg. Resting electrocardiogram showed AF with an average heart rate of 75 b.p.m.
The supervised exercise training program was carried out three times a week, for a period of 12 weeks in a closed environment, with a controlled temperature between 21°C and 24°C in the afternoon (3:30 pm to 4:30 pm). The exercise training protocol consisted of 5 min of warm-up exercises, 30 min of aerobic training performed on a cycle ergometer at 60 rpm, 20 min of resistance exercises with lower and upper limbs and 5 min of relaxation exercises. The workload was adjusted during the stimulus phase of the aerobic training to keep the perceived effort intensity between 11 (relatively easy) and 13 (slightly tiring) of the Borg Scale. 7 Resistance training was performed in a weight training station and consisted of 2 sets of 10 repetitions, and the following sequence of exercises: leg extension, leg curl, lat pull-down, peck deck, and press chest. The initial workload was set at 60% of 1 repetition maximum. 8 He was encouraged to complete the sets of 10 prescribed repetitions, performing the appropriate movement and avoiding Valsalva manoeuvre. He was instructed to rest for 30–60 s between sets. The intensity of the exercise was increased from 5% to 10% whenever the patient adapted to the exercise workload thorough the 12 weeks of training.
After 12 weeks of supervised sessions, the patient became asymptomatic (NYHA I) and was instructed to continue exercising at home.
Table 1 displays the results of the cardiopulmonary exercise test before and after 12 weeks of exercise training, performed on a cycle ergometer, using the modified Balke protocol (25 w/min). 4 Comparing the exercise test before and after 12 weeks, we observed that the rest HR reduced and the peak HR increased, and that systolic blood pressure increased, and diastolic blood pressure decreased at the peak of exercise. Peak oxygen consumption (VO 2peak ) increased and VE/VCO 2 slope decreased after training. Figure 1 shows data on muscle sympathetic nerve activity (NMSA) before and after the intervention, obtained by a microelectrode implanted in the fibular nerve and recorded by the shots per minute and every 100 b.p.m. 6 We observed that the shots decreased in both registers, indicating a reduction in NMSA. The muscle blood flow (MBF), obtained by plethysmography venous occlusion, 6 increased after exercise intervention as shown in Figure 2 .

Muscle sympathetic nerve activity (NMSA) per minute and every 100 b.p.m. before and after the intervention.

Muscle blood flow (MBS) before and after the intervention.

Electrocardiogram pre- and post-12 weeks of exercise training. ( A ) Pre-rest; ( B ) pre-peak; ( C ) post-rest, and ( D ) post-peak.
Cardiopulmonary exercise pre- and post-12 weeks of exercise training
RER, respiratory exchange ratio; VO 2peak , peak oxygen consumption; VE/VCO 2 slope, the slope of the line between pulmonary ventilation (mL/min) and carbon dioxide production (mL/min).
This case study highlights the known benefits of physical exercise in heart failure with reduced ejection fraction and permanent atrial fibrillation. To the best of our knowledge, this is the first report of the benefits of exercise training guided by the Borg scale on physical capacity, neuromuscular sympathetic activity, and muscle blood flow in a patient with AF and HF with reduced ejection fraction.
Muscle sympathetic nerve activity is an independent predictor of prognosis in HF. 9 The reduction in the frequency of NMSA shots after exercise reinforces the idea that physical training contributes to improve prognosis in these patients. The present report shows a reduction in NMSA in AF associated with HF, but it does not elucidate its mechanisms. Nevertheless, it is reasonable to speculate that exercise training promotes increased sensitivity of the arterial baroreflex, restoring control of sympathetic nerve activity, and heart rate, which may be associated with improved sensitivity of the aortic depressor. 10 , 11 In addition, exercise training may have reduced peripheral chemoreflex sensitivity, improving the ergoreflex control of NMSA in AF associated with HF. 12
Evidence shows that exercise training increases muscle blood flow in HF, 13 which was also observed in our report of a patient with AF associated with HF. This observation may have useful clinical implications because muscle blood flow is an independent predictor of mortality in HF. 9 The increase in muscle blood flow after exercise training may have resulted from better endothelial function, shifting the balance between vasodilation and vasoconstriction. 12
The presence of AF is related to lower exercise capacity, which is associated with reduced VO 2peak and increased VE/VCO 2 slope. 14 The association of AF with HF has important clinical implications, with a higher risk of hospitalization and death. 13 Studies have shown that exercise training increases the VO 2peak and a decrease the VE/VCO 2 slope in patients with HF. 3 , 15 The findings of this case report are consistent with these studies, highlighting how the benefits of exercise training can be extended to patients with AF and HF, as long as they are clinically stable, with optimized drug therapy, using beta-blockers and with controlled heart rate at rest and exercise.
On the other hand, a sub-analysis of the HF-ACTION suggested a lack of efficacy of aerobic exercise in improving the prognosis of patients with HF and AF. 16 Nevertheless, the HF-ACTION study was not designed for patients with HF and AF, and the low compliance and different aspects of exercise protocol may have contributed to an only mild increase in VO 2peak . Also, the intensity of aerobic exercise using 60–70% of the heart rate reserve does not apply to patients with AF. 17 , 18
Finally, although restoration of sinus rhythm may improve haemodynamics and prognosis in patients with HF and AF, as recent trials with catheter ablation have suggested, maintaining sinus rhythm is challenging in clinical practice and it needs to be compared with rate-control strategy in large double-blind clinical trials. 19 , 20 Importantly, despite this patient became asymptomatic, VO 2peak remained low, and his prognosis can be further improved by therapies that showed to improve survival in symptomatic patients with HFrEF, such as changing losartan to sacubitril/valsartan and adding spironolactone. 21 More recently, a sodium-glucose co-transporter-2 (SGLT2) inhibitor showed to improve survival in this population. 22
Exercise training is key to improve functional capacity and quality of life, with low risk of side effects and complications, being an essential and safe tool for treating patients with HF. The present case showed that exercise using the Borg scale of perceived exertion to guide intensity can improve the tolerance to exercise, increase the MBF, decrease the resting HR, and increase maximum HR, which may be related to better balance in the autonomic nervous system. 23
These favourable results must be confirmed by future studies and by the replication of research that examines the impact of exercise training in patients with AF and HF with both reduced and preserved ejection fraction. They should evaluate the effects on different outcomes, such as muscle sympathetic nerve activity, muscle blood flow, and functional capacity, and include other modalities of exercise, such as interval aerobic training, water-based exercises, inspiratory muscle training, and yoga. This will help better understand the role and potential mechanisms of exercise training in patients with HF and AF, and provide specific recommendations for this population.
Exercise training showed potential benefits in a patient with AF associated with HF with reduced ejection fraction, reducing sympathetic nerve activity, increasing muscle blood flow and the VO 2peak , and decreasing the VE/VCO 2 slope and resting heart rate.

Guilherme Veiga Guimarães, Phd., Researcher, School of Medicine, University of São Paulo. He is currently a cardiac rehabilitation researcher at the Heart Institute of Hospital das Clínicas, University of São Paulo, with an interest in exercise physiology.
Supplementary material is available at European Heart Journal - Case Reports online.
Fundação de Amparo à Pesquisa do Estado de São Paulo (FAPESP # 2013/17031-6); and Conselho Nacional de Desenvolvimento Científico e Tecnologico (CNPq # 40137/2016-5). Conselho Nacional de Desenvolvimento Cientıfico e Tecnologico (CNPq # 301957/2017-7) during this project to G.V.G.
Slide sets: A fully edited slide set detailing this case and suitable for local presentation is available online as Supplementary data .
Consent: The author/s confirm that written consent for submission and publication of this case report including image(s) and associated text has been obtained from the patient in line with COPE guidelines.
Conflict of interest : none declared.
Kotecha D , Piccini JP. Atrial fibrillation in heart failure: what should we do? Eur Heart J 2015 ; 36 : 3250 – 3257 .
Google Scholar
Lam CS , Rienstra M , Tay WT , Liu LC , Hummel YM , van der Meer P et al. Atrial fibrillation in heart failure with preserved ejection fraction: association with exercise capacity, left ventricular filling pressures, natriuretic peptides, and left atrial volume . JACC: Heart Failure 2017 ; 5 : 92 – 98 .
Taylor RS , Sagar VA , Davies EJ , Briscoe S , Coats AJ , Dalal H et al. Exercise-based rehabilitation for heart failure . Cochrane Database Syst Rev 2014 ; CD003331 .
Fernandes-Silva MM , Guimarães GV , Rigaud VO , Lofrano-Alves MS , Castro RE , Cruz LGDB et al. Inflammatory biomarkers and effect of exercise on functional capacity in patients with heart failure: Insights from a randomized clinical trial . Eur J Prev Cardiol 2017 ; 24 : 808 – 817 .
Guimarães GV , Ciolac EG. Physical activity: practice this idea . Am J Cardiovasc Dis 2014 ; 4 : 1 – 121 .
Wyse G, Anderson JL, Antman EM, Cooper ES, Dalquist JE, Davis KB et al. Atrial fibrillation follow-up investigation of rhythm management—the AFFIRM study design . Am J Cardiol 1997 ; 9 : 1198 – 1202 .
Carvalho VO , Bocchi EA , Guimarães GV. The Borg scale as an important tool of self-monitoring and self-regulation of exercise prescription in heart failure patients during hydrotherapy. A randomized blinded controlled trial . Circ J 2009 ; 73 : 1871 – 1876 .
Abdul-Hameed U , Rangra P , Shareef MY , Hussain ME. Reliability of 1-Repetition maximum estimation for upper and lower body muscular strength measurement in untrained middle aged type 2 diabetic patients . Asian J Sports Med 2012 ; 3 : 267 – 273 .
Barretto AC , Santos AC , Munhoz R , Rondon MU , Franco FG , Trombetta IC et al. Increased muscle sympathetic nerve activity predicts mortality in heart failure patients . Int J Cardiol 2009 ; 135 : 302 – 307 .
Piepoli M , Volterrani M , Ponikowski P , Giordano A , Capucci A , Coats A. The ergoreflex activity during exercise: predictor of impaired heart rate variability in chronic heart failure . Eur J Heart Fail 2000 ; 2 : 50 – 56 .
Guimarães GV , Belli JFC , Bacal F , Bocchi EA. Behavior of central and peripheral chemoreflex in heart failure . Arq Bras Cardiol 2011 ; 96 : 161 – 167 .
Belli JFC , Bacal F , Bocchi EA , Guimarães GV. Ergoreflex activity in heart failure . Arq Bras Cardiol 2011 ; 97 : 171 – 178 .
Pearson M , Smart N. Effect of exercise training on endothelial function in heart failure patients: a systematic review meta-analysis . Int J Cardiol 2017 ; 231 : 234 – 243 .
Guimarães GV , Silva MSVD , D'avila VM , Ferreira SMA , Silva CP , Bocchi EA. Peak VO 2 and VE/VCO 2 slope in the beta-blockers era in patients with heart failure: a Brazilian experience . Arq Bras Cardiol 2008 ; 91 : 42 – 48 .
Downing J , Balady GJ. The role of exercise training in heart failure . J Am Coll Cardiol 2011 ; 58 : 561 – 569 .
Luo N , Merrill P , Parikh KS , Whellan DJ , Piña IL , Fiuzat M et al. Exercise training in patients with chronic heart failure and atrial fibrillation . J Am Coll Cardiol 2017 ; 69 : 1683 – 1691 .
O’Connor CM , Whellan DJ , Lee KL , Keteyian SJ , Cooper LS , Ellis SJ et al. Efficacy and safety of exercise training in patients with chronic heart failure: HF-ACTION randomized controlled trial . JAMA 2009 ; 301 : 1439 – 1450 .
Cornelis J , Myers J , Heidbuchel H , Vrints C , Beckers P. Exercise training in heart failure patients with persistent atrial fibrillation: a practical approach . Card Fail Rev 2018 ; 4 : 107 – 111 .
Prabhu S , Taylor AJ , Costello BT , Kaye DM , McLellan AJA , Voskoboinik A et al. Catheter ablation versus medical rate control in atrial fibrillation and systolic dysfunction: the CAMERA-MRI study . J Am Coll Cardiol 2017 ; 70 : 1949 – 1961 .
Marrouche NF , Brachmann J , Andresen D , Siebels J , Boersma L , Jordaens L et al. Catheter ablation for atrial fibrillation with heart failure . N Engl J Med 2018 ; 378 : 417 – 427 .
Ponikowski P , Voors AA , Anker SD , Bueno H , Cleland JGF , Coats AJS , Falk V et al. 2016 ESC Guidelines for the diagnosis and treatment of acute and chronic heart failure: the Task Force for the diagnosis and treatment of acute and chronic heart failure of the European Society of Cardiology (ESC)Developed with the special contribution of the Heart Failure Association (HFA) of the ESC [published correction appears in Eur Heart J. 2016 Dec 30] . Eur Heart J 2016 ; 37 : 2129 –‐ 2200 .
Tamargo J. Sodium-glucose cotransporter 2 inhibitors in heart Failure: potential mechanisms of action, adverse effects and future developments . Eur Cardiol 2019 ; 14 : 23 – 32 .
Malmo V , Nes BM , Amundsen BH , Tjonna AE , Stoylen A , Rossvoll O et al. Aerobic interval training reduces the burden of atrial fibrillation in the short term: a randomized trial . Circulation 2016 ; 133 : 466 – 473 .
- atrial fibrillation
- vascular flow
- heart failure
- heart failure with reduced ejection fraction
Supplementary data
Email alerts, related articles in pubmed, citing articles via.
- General Instructions
- Advertising and Corporate Services
Affiliations
- Online ISSN 2514-2119
- Copyright © 2024 European Society of Cardiology
- About Oxford Academic
- Publish journals with us
- University press partners
- What we publish
- New features
- Open access
- Institutional account management
- Rights and permissions
- Get help with access
- Accessibility
- Advertising
- Media enquiries
- Oxford University Press
- Oxford Languages
- University of Oxford
Oxford University Press is a department of the University of Oxford. It furthers the University's objective of excellence in research, scholarship, and education by publishing worldwide
- Copyright © 2024 Oxford University Press
- Cookie settings
- Cookie policy
- Privacy policy
- Legal notice
This Feature Is Available To Subscribers Only
Sign In or Create an Account
This PDF is available to Subscribers Only
For full access to this pdf, sign in to an existing account, or purchase an annual subscription.
Atrial Fibrillation and Heart Failure
- First Online: 30 March 2023
Cite this chapter
- Tara U. Mudd 3
509 Accesses
Atrial fibrillation is the most common cardiac arrhythmia in the world and is something that will be encountered in the primary care setting. While not a life-threatening arrhythmia, patients with atrial fibrillation are at significantly higher risk of cardiovascular complications including stroke and heart failure. In those individuals who have both atrial fibrillation and heart failure, their symptoms may be closely intertwined and difficult to attribute to only one diagnosis. Furthermore, the presence of one diagnosis will increase the likelihood of the other. This is the case in heart failure patients with both reduced and preserved ejection fraction. Treatment of patients with atrial fibrillation and heart failure should be aimed at rhythm management whenever possible. This includes, but is not limited to, atrioventricular nodal blocking agents, antiarrhythmic therapy, and catheter ablation. Patients are best served by a multidisciplinary approach involving primary care, cardiology, and other specialists as indicated. Engagement with appropriate lifestyle modification for the patient is imperative to the optimal control of both comorbid conditions.
This is a preview of subscription content, log in via an institution to check access.
Access this chapter
- Available as EPUB and PDF
- Read on any device
- Instant download
- Own it forever
- Compact, lightweight edition
- Dispatched in 3 to 5 business days
- Free shipping worldwide - see info
Tax calculation will be finalised at checkout
Purchases are for personal use only
Institutional subscriptions
Colilla S, Crow A, Petkun W, Singer D, Simon T, Liu X. Estimates of current and future incidence and prevalence of atrial fibrillation in the U.S. adult population. Am J Cardiol. 2013;112(8):1142–7.
Article PubMed Google Scholar
Cha Y, Redfield M, Shen W, Gersh B. Atrial fibrillation and ventricular dysfunction; a vicious electromechanical cycle. Circulation. 2004;109(23):2839.
Santhanakrishnan R, Wang N, Larson M, Magnani J, McManus D, Lubitz S, et al. Atrial fibrillation begets heart failure and vice versa: temporal associations and differences in preserved versus reduced ejection fraction. Circulation. 2016;133(5):484.
Article PubMed PubMed Central Google Scholar
Howard R, Stopps T, Moe G, Gotlieb A, Armstrong P. Recovery from heart failure: structural and functional analysis in a canine model. Can J Physiol Pharmacol. 1988;66(12):1505.
Article CAS PubMed Google Scholar
Morgan D, Tomlinson C, Qayumi A, Toleikis P, McConville B, Jamieson WR. Evaluation of ventricular contractility indexes in the dog with left ventricular dysfunction induced by rapid atrial pacing. J Am Coll Cardiol. 1989;14(2):489.
Gopinathannair R, Etheridge S, Marchlinski F, Spinale F, Lakkireddy D, Olshansky B. Arrhythmia-induced cardiomyopathies; mechanisms, recognition, and management. J Am Coll Cardiol. 2015;66(15):1714–28.
Nakashima H, Kumagai K, Urata H, Gondo N, Ideishi M, Arakawa K. Angiotensin II antagonist prevents electrical remodeling in atrial fibrillation. Circulation. 2000;101(22):2612.
Goette A, Arndt M, Röcken C, Spiess A, Staack T, Geller J, et al. Regulation of angiotensin II receptor subtypes during atrial fibrillation in humans. Circulation. 2000;101(23):2678.
Nattel S, Burstein B, Dobrev D. Atrial remodeling and atrial fibrillation: mechanisms and implications. Circ Arrhythm Electrophysiol. 2008;1(1):62.
Kalifa J, Jalife J, Zaitsev A, Bagwe S, Warren M, Moreno J, et al. Intra-atrial pressure increases rate and organization of waves emanating from the superior pulmonary veins during atrial fibrillation. Circulation. 2003;108(6):668.
Yoneda Z, Anderson K, Quintana J, O’Neill M, Sims R, Glazer A, et al. Early-onset atrial fibrillation and the prevalence of rare variants in cardiomyopathy and arrhythmia genes. JAMA Cardiol. 2021;6(12):1371.
Chugh S, Havmoeller R, Narayanan K, Singh D, Rienstra M, Benjamin E, et al. Worldwide epidemiology of atrial fibrillation: a Global Burden of Disease 2010 Study. Circulation. 2014;129:837–47.
Lau D, Nattel S, Kalman J, Sanders P. Modifiable risk factors and atrial fibrillation. Circulation. 2017;136:583–96.
Gosselink A, Crijns H, Hamer H, Hillege H, Lie K. Changes in left and right atrial size after cardioversion of atrial fibrillation: role of mitral valve disease. J Am Coll Cardiol. 1993;22(6):1999–672.
Article Google Scholar
Jagadish P, Kabra R. Stroke risk in atrial fibrillation: beyond the CHA2DS2-VASc Score. Curr Cardiol Rep. 2019;21:95.
Lip G, Nieuwlaat R, Pisters R, Lane D, Crijns H. Refining clinical risk stratification for predicting stroke and thromboembolism in atrial fibrillation using a novel risk factor-based approach. Chest. 2010;137(2):263–72.
Lee C, Toft-Petersen A, Ozenne B, Phelps M, Olesen J, Ellinor P, et al. Assessing absolute stroke risk in patients with atrial fibrillation using a risk factor-based approach. Eur Heart J Cardiovasc Pharmacother. 2021;7(FI1):f3–f10.
Nielsen PB, Skjøth F, Overvad TF, Larsen TB, Lip GYH. Female sex is a risk modifier rather than a risk factor for stroke in atrial fibrillation: should we use a CHA2DS2-VA score rather than CHA2DS2-VASc? Circulation. 2018;137(8):832.
Olesen J, Lip G, Lindhardsen J, Lane D, Ahlehoff O, Hansen M, et al. Risks of thromboembolism and bleeding with thromboprophylaxis in patients with atrial fibrillation: a net clinical benefit analysis using a 'real world' nationwide cohort study. Thromb Haemost. 2011;106(4):739.
CAS PubMed Google Scholar
January C, Wann L, Calkins H, Chen L, Cigarroa J, Cleveland Jr J, et al. 2019 AHA/ACC/HRS focused update of the 2014 AHA/ACC/HRS guideline for the management of patients with atrial fibrillation. 2019. https://doi.org/10.1016/j.hrthm.2019.01.024 .
Pisters R, Lane D, Nieuwlaat R, de Vos C, Crijns H, Lip G. A novel user-friendly score (HAS-BLED) to assess 1-year risk of major bleeding in patients with atrial fibrillation. Chest. 2010;138(5):1093–100.
Zhu W, He W, Guo L, Wang X, Hong K. The HAS-BLED score for predicting major bleeding risk in anticoagulated patients with atrial fibrillation: a systematic review and meta-analysis. Clin Cardiol. 2015;38(9):555–61.
Kotecha D, Piccini J. Atrial fibrillation in heart failure: what should we do? Eur Heart J. 2015;36(46):3250–7.
CAS PubMed PubMed Central Google Scholar
Crijns H, Van Den Berg M, Van Gelder I, Van Veldhuisen D. Management of atrial fibrillation in the setting of heart failure. Eur Heart J. 1997;18(Supplement C):C45–9.
Manning W, Silverman D, Katz S, Riley M, Come P, Doherty R, et al. Impaired left atrial mechanical function after cardioversion: relation to the duration of atrial fibrillation. J Am Coll Cardiol. 1994;23(7):1535.
Klein A, Murray R, Grimm R. Role of transesophageal echocardiography-guided cardioversion of patients with atrial fibrillation. J Am Coll Cardiol. 2001;37(3):691–704.
Van Gelder I, Rienstra M, Crijns H, Olshansky B. Rate control in atrial fibrillation. Lancet. 2016;388:818–28.
Dixon K, Thanavaro J, Thais A, Lavin M. Amiodarone surveillance in primary care. J Nurse Pract. 2003;9(1):46–54.
Piccini J, Fauchier L. Rhythm control in atrial fibrillation. Lancet. 2016;388:829–40.
Kelly J, DeVore A, Wu J, Hammill B, Sharma A, Cooper L, et al. Rhythm control versus rate control in patients with atrial fibrillation and heart failure with preserved ejection fraction: insights from get with the guidelines – heart failure. J Am Heart Assoc. 2019;8(24):e011560. https://doi.org/10.1161/JAHA.118.011560 .
Patel R, Vaduganathan M, Shah S, Butler J. Atrial fibrillation in heart failure with preserved ejection fraction: insights into mechanisms and therapeutics. Pharmacol Ther. 2017;176:32–9.
Brachmann J, Sohns C, Andresen D, Siebels J, Sehner S, Boersma L. Atrial fibrillation burden and clinical outcomes in heart failure: the CASTLE-AF trial. JACC Clin Electrophysiol. 2021;7(5):594–603.
Joseph P, Swedberg K, Leong D, DPhil S. The evolution of β-blockers in coronary artery disease and heart failure (part 1/5). J Am Coll Cardiol. 2019;74(5):672–82.
Boriani G, Biffi M, Diemberger I, Martignani C, Branzi A. Rate control in atrial fibrillation; choice of treatment and assessment of efficacy. Drugs. 2003;63:1489–509.
Download references
Author information
Authors and affiliations.
Norton Heart & Vascular Institute Heart Rhythm Center AFib Clinic, Louisville, KY, USA
Tara U. Mudd
You can also search for this author in PubMed Google Scholar
Corresponding author
Correspondence to Tara U. Mudd .
Editor information
Editors and affiliations.
Assistant Professor, Vanderbilt University School of Nursing, Nashville, TN, USA
K. Melissa Smith Hayes
Director, Structural Heart Program, Director, Center for Advanced Heart Failure Therapy, Centennial Heart, Nashville, TN, USA
Nicole R. Dellise
Rights and permissions
Reprints and permissions
Copyright information
© 2023 The Author(s), under exclusive license to Springer Nature Switzerland AG
About this chapter
Mudd, T.U. (2023). Atrial Fibrillation and Heart Failure. In: Hayes, K.M.S., Dellise, N.R. (eds) Managing Heart Failure in Primary Care: A Case Study Approach. Springer, Cham. https://doi.org/10.1007/978-3-031-20193-6_10
Download citation
DOI : https://doi.org/10.1007/978-3-031-20193-6_10
Published : 30 March 2023
Publisher Name : Springer, Cham
Print ISBN : 978-3-031-20192-9
Online ISBN : 978-3-031-20193-6
eBook Packages : Medicine Medicine (R0)
Share this chapter
Anyone you share the following link with will be able to read this content:
Sorry, a shareable link is not currently available for this article.
Provided by the Springer Nature SharedIt content-sharing initiative
- Publish with us
Policies and ethics
- Find a journal
- Track your research
Heart failure outcomes in low-risk patients with atrial fibrillation: a case-control study of 680 523 Swedish individuals
Affiliations.
- 1 Department of Molecular and Clinical Medicine, Institute of Medicine, Sahlgrenska Academy, University of Gothenburg, Diagnosvägen 11, 41650, Gothenburg, Sweden.
- 2 Region Västra Götaland, Department of Medicine Geriatric and Emergency Medicine, Sahlgrenska University Hospital/Östra, Gothenburg, Sweden.
- PMID: 37139589
- PMCID: PMC10375091
- DOI: 10.1002/ehf2.14375
Aims: Knowledge of long-term outcomes in patients with atrial fibrillation (AF) remains limited. We sought to evaluate the risk of new-onset heart failure (HF) in patients with AF and a low cardiovascular risk profile.
Methods and results: Data from the Swedish National Patient Register were used to identify all patients with a first-time diagnosis of AF without underlying cardiovascular disease at baseline between 1987 and 2018. Each patient was compared with two controls without AF from the National Total Population Register. In total, 227 811 patients and 452 712 controls were included. During a mean follow-up of 9.1 (standard deviation 7.0) years, the hazard ratio (HR) for new-onset HF was 3.55 [95% confidence interval (CI) 3.51-3.60] in patients compared with controls. Women with AF (18-34 years) had HR for HF onset 24.6 (95% CI 7.59-80.0) and men HR 9.86 (95% CI 6.81-14.27). The highest risk was within 1 year in patients 18-34 years, HR 103.9 (95% CI 46.3-233.1). The incidence rate within 1 year increased from 6.2 (95% CI 4.5-8.6) per 1000 person-years in young patients (18-34 years) to 142.8 (95% CI 139.4-146.3) per 1000 person-years among older patients (>80 years).
Conclusions: Patients studied had a three-fold higher risk of developing HF compared with controls. Young patients, particularly women, carry up to 100-fold increased risk to develop HF within 1 year after AF. Further studies in patients with AF and low cardiovascular risk profile are needed to prevent serious complications such as HF.
Keywords: Atrial fibrillation; Case-control study; Heart failure; Registry-based study.
© 2023 The Authors. ESC Heart Failure published by John Wiley & Sons Ltd on behalf of European Society of Cardiology.
Publication types
- Research Support, Non-U.S. Gov't
- Atrial Fibrillation* / complications
- Atrial Fibrillation* / diagnosis
- Atrial Fibrillation* / epidemiology
- Case-Control Studies
- Heart Failure* / diagnosis
- Heart Failure* / epidemiology
- Heart Failure* / etiology
- Risk Factors
- Stroke Volume
- Sweden / epidemiology

An official website of the United States government
The .gov means it's official. Federal government websites often end in .gov or .mil. Before sharing sensitive information, make sure you're on a federal government site.
The site is secure. The https:// ensures that you are connecting to the official website and that any information you provide is encrypted and transmitted securely.
- Publications
- Account settings
- Browse Titles
NCBI Bookshelf. A service of the National Library of Medicine, National Institutes of Health.
Lord J, Willis S, Eatock J, et al. Economic modelling of diagnostic and treatment pathways in National Institute for Health and Care Excellence clinical guidelines: the Modelling Algorithm Pathways in Guidelines (MAPGuide) project. Southampton (UK): NIHR Journals Library; 2013 Dec. (Health Technology Assessment, No. 17.58.)

Economic modelling of diagnostic and treatment pathways in National Institute for Health and Care Excellence clinical guidelines: the Modelling Algorithm Pathways in Guidelines (MAPGuide) project.
Chapter 5 case study 2: full guideline model for atrial fibrillation.
This chapter presents a case study showing the development of a full guideline model to evaluate multiple decision problems across the AF pathway.
- Introduction
Introduction to the context of the case study
Atrial fibrillation is a condition characterised by irregular and rapid heart rhythm. 134 , 135 It can cause a range of symptoms including chest pains, palpitations, angina, shortness of breath and fatigue, and can sometimes present as a critical condition with haemodynamic instability requiring urgent treatment, although in contrast some patients do not experience symptoms at all and might be unaware of their condition. AF is associated with a greatly increased risk of death from stroke and other thromboembolic events, heart failure and cardiovascular disease. Three types of AF have been distinguished: paroxysmal, persistent and permanent AF. Paroxysmal AF is characterised by short episodes of irregular heart rhythm lasting < 7 days, normally < 48 hours. Persistent AF is associated with longer episodes, which do not terminate without intervention. In permanent AF there is a perpetual fibrillation of the atria. The ‘natural’ course of AF is generally progressive, with the frequency and duration of symptomatic episodes usually increasing over time.
Atrial fibrillation is common, affecting 1–2% of the general population, and is associated with age (a European study estimated the prevalence at 0.7% for people aged 55–59 years, rising to 17.8% for people aged ≥ 85 years). 135 Recent increases in AF prevalence have been attributed to improvements in survival for cardiovascular conditions associated with AF, and the ageing population. Resources consumed in the treatment of AF are estimated to account for nearly 1% of the UK NHS expenditure. The impact of AF on mortality and quality of life, and the associated economic burden led to the commissioning of a CG by NICE.
The scope for the NICE CG (CG36), 136 published in 2006, covered the processes of patient care including identification and diagnosis, treatment for the prevention of stroke and TE, electrical and pharmacological methods to correct heart rhythm (‘cardioversion’ to achieve sinus rhythm), drugs to maintain heart rhythm or to control heart rate, monitoring and referral for specialist electrophysiological interventions such as pacing or ablation. 137 The guideline also covered acute treatment for haemodynamically unstable patients, and the prevention and treatment of AF in patients undergoing cardiac surgery.
Aims of the case study
The aim of this case study was to develop a model to reflect the course of AF for a cohort of patients diagnosed and treated in accordance with CG36. 136 The model was designed to predict the incidence of AF-related risks and associated health outcomes and expenditure, so as to provide a platform to address a range of cost-effectiveness questions across the care pathway. To test the model, we conducted economic evaluations of some changes to the current pathway related to the potential update topics identified in Chapter 3 . The purpose of this analysis was to illustrate the process of developing a full guideline model, and to test its ability to evaluate cost-effectiveness questions. The results are indicative of topic areas where further investigation is likely to be of value. We did not conduct systematic reviews to inform estimates of effectiveness or other model parameters, and so the results should not be used directly to inform clinical policy or practice.
Preliminary literature review
To inform model development, we conducted an initial review of literature on published economic models for the disease area, related models from NICE guidance (e.g. TAs) and other HTA bodies and guideline developers. We searched the following secondary databases, using general disease/patient group search terms: (a) CRD NHS Economic Evaluation Database; (b) CRD HTA Database; (c) NHS Evidence; and (d) the G-I-N database. This search was intended as a rapid means of identifying appropriate model structures and sources of data. We did not conduct formal critical appraisal of published economic evaluations or summarise their findings.
Several documents were identified that were very influential in the development of our model structure. These included economic evaluations and models that covered different aspects of the diagnosis and treatment of AF that we sought to bring together in a model of the whole service pathway and disease process:
- Case finding: A HTA-funded project by Hobbs and colleagues 138 included a clinical trial and economic evaluation of methods for screening for AF. This provided data on the accuracy of diagnostic methods, as well as informing the design of the decision tree in the diagnostic section of our model.
- Antithrombotic therapy: Various models have been developed to evaluate the cost-effectiveness of antithrombotic therapy. 139 – 144 Drug treatments include antiplatelet agents (aspirin and clopidogrel) and anticoagulants (warfarin, dabigatran, rivaroxaban and apixaban). These drugs are all effective at reducing the risk of TE, but at the risk of causing dangerous bleeds. In addition, the mainstay of oral anticoagulation (OAC), warfarin, requires regular monitoring which is difficult, inconvenient for the patient and expensive. The available models estimate the balance between these various risks and their health and financial consequences. For this element of our model, we drew particularly on the models developed for the recent NICE TAs of dabigatran and rivaroxaban, and the critique of these models provided by the Evidence Review Groups and the Appraisal Committee considerations. 139 , 140 , 145 – 148 The NICE TA of another OAC drug (apixaban, Eliquis ® , Bristol-Myers Squibb) was published after completion of our model (www.nice.org.uk/TA275), and so did not influence our work.
- Antiarrhythmic therapy: Another recent NICE TA that provided valuable information for the construction of our model was TA197, which compared dronedarone (Multaq ® , Sanofi-Aventis) with other drugs for the maintenance of sinus rhythm [amiodarone and the class 1c antiarrhythmic drugs (AADs)]. 149 – 151 The report of the sponsor’s model was particularly useful, as they used a DES technique. The detailed critique provided by the Evidence Review Group and by the Appraisal Committee was also very helpful in identifying important factors to include in our model.
- Ablation: Finally, we considered an HTA review and economic evaluation that compared antiarrhythmic drug (AAD) therapy with radio frequency catheter ablation for the curative treatment of AF and flutter. 152
This list illustrates the wide range of models evaluating different parts of the AF service pathway, but we did not find any models that brought together all of these elements in sufficient detail to provide a platform for economic evaluation across the whole pathway.
Conceptual model development
Before constructing the computer model, a conceptual understanding and definition of the problem area was developed. This comprised two key elements: (1) a model of the service pathway defined in CG36 and (2) a model of the disease processes.
The design of the service pathway model began with detailed consideration of the full guideline documentation for CG36 to develop an understanding of the recommendations, the available evidence and the GDG rationale for decisions. 135 The NICE QRG document was also useful, as this contains a set of flow charts and other illustrations that put the recommendations together into a connected pathway. 55 For CG36, this included: an overview of the whole process from diagnosis to follow-up; strategies for cardioversion in acute and non-acute situations; a decision tree defining the criteria for selecting rate or rhythm control strategies; risk stratification and choice of drugs for prevention of stroke; and sequencing of rhythm and rate control drugs. These QRG ‘algorithms’ were developed into much more detailed and formalised flow charts necessary to provide a foundation for the simulation model. This involved in-depth review of the full guideline and of the precise wording of the recommendations.
The conceptual service pathway model was drafted using flow charts, which were then checked with clinical experts to identify errors or lack of clarity. Four clinicians, including a GP, two cardiologists specialising in AF and an interventional electro-physiologist provided advice. The purpose of consulting experts was to help the modellers to understand and interpret the pathway of care defined in CG36, rather than to elicit information about how services are organised in practice, or the experts’ views on how services should be organised. This process was essential to resolve some ‘gaps’ and ambiguities in the guideline algorithms and documentation. We also sought information from the experts on sources of data to inform the model parameters.
Another essential component of the conceptual model was an understanding of the disease course, how this varies between individuals and how it can be modified over time by interventions and events. The initial design of this disease process model for this guideline was informed by the preliminary literature review described above, and again clinical experts were invited to comment on this approach.
Boundary and scope of the model
The aim of this case study was to model the service pathway recommended in the NICE AF CG (CG36) 136 to estimate associated patient flows, health outcomes and cost, to assess the incremental cost-effectiveness of possible changes in the service pathway, and to estimate the value of updating selected topics within the guideline. We therefore took the scope of CG36 as the starting point for defining the model boundaries. 137 However, there were some differences between the scope and model boundaries which are described below.
The CG scope 137 included people with new-onset or acute AF, chronic AF (CAF; including recurrent paroxysmal, persistent and permanent/sustained AF), comorbidities that impact on AF, post-operative AF, and atrial flutter that is indistinguishable from AF in terms of aim of treatment. The scope was also longitudinally broad, covering the spectrum of care for patients with all stages of the condition and associated adverse events in primary and secondary NHS health-care settings, as well as referral to tertiary care. The guideline group considered evidence and made recommendations on:
- identification of AF, including active case finding but not population screening
- investigations required to confirm diagnosis and to assess comorbidity
- treatment of acute-onset AF episodes with haemodynamic instability
- prevention and treatment of post-operative AF
- risk stratification and prophylactic antithrombotic treatment
- electrical and pharmacological interventions to promote and maintain heart rhythm
- pharmacological methods to control heart rate
- referral for specialist assessment
- reviewing and monitoring of patients with AF.
The model was also broad in scope, including most of the patient groups and interventions covered in the guideline, although there were some exceptions. Owing to a lack of clarity and evidence about atrial flutter this was not explicitly modelled as a separate group. We also chose not to model post-operative AF. This was a pragmatic decision, due to anticipated difficulties in reviewing a separate body of epidemiological and clinical evidence with limited time and resources. To evaluate preventative treatments for post-operative AF would also have meant introducing a very different cohort of patients into the model, including patients undergoing cardiac surgery who did not go on to develop AF.
Although we considered adopting a population approach to modelling, to reflect costs and outcomes for both prevalent and incident cases across time, this was not possible in the time available. Instead, the model took a more conventional incident cohort approach, starting with a group of individuals being tested for suspected AF, and following these individuals through until death. Information about the demographic and clinical characteristics of individuals entering the model, which governs their risks of adverse events and health outcomes, was taken from a primary care database (described below). A data set of patients not diagnosed with AF was also obtained to allow modelling of case finding approaches and to capture consequences of false-positive and false-negative test results. (In the event, these data were not used as we did not identify sufficient information on the effectiveness of case finding or diagnostic test accuracy to model these questions.) Extension of the model to post-operative AF would require a similar individual-patient data set for this population, or sufficient information to generate such a data set.
CG36 did not review evidence relating to specialist interventions to identify and correct structural heart abnormalities or electrophysiological problems, which might be the underlying cause of AF for some individuals. Evaluation of implantable devices was explicitly excluded from the scope, as was evaluation of ‘novel/experimental’ arrhythmia surgery. The guideline group did recommend referral to a specialist if symptoms could not be adequately controlled with conventional rate or rhythm control strategies, but they did not recommend which further treatment options should be considered for which patients. There is currently a lot of interest in various ablation techniques that are potentially curative of AF refractory to AAD treatment. 152 – 157 However, we did not explicitly include this within the model as it was considered outside the scope. The model therefore stopped, as did the guideline, at the point at which patients were referred to a tertiary specialist. This issue is discussed further below.
Another common boundary issue for guideline models is the evolution of adjacent and sometimes overlapping NICE guidance. During the course of this project, three NICE TAs within the model boundary were published: two related to new OACs (dabigatran 146 and rivaroxaban 147 ) and one to a new AAD (dronedarone 150 ). As NICE CGs are expected to integrate current NICE TAs unchanged, we reviewed the evidence from these published appraisals and attempted to integrate their recommendations in the CG36 pathway. However, we did not include apixaban because the NICE TA on this drug was not published until after completion of our model.
The service pathway
Outline of pathway.
Figure 15 gives a broad view of the flow of patients through the service pathway.
Overview of AF service pathway model. FN, false-negative; p, prevalence of AF in patients tested for suspected AF; TN, true-negative; TP, true-positive.
To enable an evaluation of case finding and screening strategies, two cohorts of patients can be fed into the model (a cohort with AF and a cohort without AF). The proportion of patients with AF (p) can be manipulated to represent more or less targeted case finding strategies, with different rates of prevalence in the population being tested. In the analyses presented below, however, we model results only for patients presenting with ‘true’ AF.
Patients enter via the diagnostic module where they undergo a series of tests. If the tests are negative, patients leave the diagnostic module and wait for the next event. Patients with false-negative results, undiagnosed AF, are then at risk of another symptomatic AF episode or an AF-related event, such as a stroke or TE. If this event is not fatal, they will then present again and return to the diagnostic module. Patients with false-negative results who do not experience a symptomatic episode or AF-related event wait in the model until they die from other causes.
Patients diagnosed with AF enter the treatment pathway, where they have their risk assessed and are allocated treatments based on their personal characteristics and the guideline recommendations. These treatments may include antithrombotic drugs, interventions to promote and maintain sinus rhythm, and/or drugs to control their heart rate. These options are discussed below.
After treatment allocation, patients enter the ongoing management (OM) module, where they wait for the next event. This can be a routine follow-up appointment, in which case they will cycle back through the treatment pathway, and possibly have their treatment changed. Alternatively, they may experience an event, which may include recurrence of arrhythmia, stroke or TE or an increase in another risk factor, such as onset of hypertension or diabetes. Unless the event is fatal, the patient then returns to the treatment pathway, and his or her treatment is reassessed.
Patients may cycle between the treatment pathway and OM modules many times over their lifetime, reflecting the chronic nature of AF. The rate at which patients experience events and return for reassessment is governed by their initial characteristics on model entry, their history of events within the model, and the treatments that they receive. Patients can also leave the model at any time, due to death from non-AF related causes.
Figure 16 expands on the contents of the diagnostic and treatment pathways. This contains eight blocks related to eight main aspects of the pathway, and each linked to a chapter in CG36. Each block is also further expanded into a detailed flow chart (see Appendix 6 ).
Overview of AF service pathway model. BB, beta blocker; i.v., intravenous; N, no; Y, yes.
Patients can enter the model through two routes: (1) primary care referral and (2) emergency attendance due to an acute onset of AF. The acute onset pathway is described later. Patients presenting routinely may be symptomatic, or they may have asymptomatic AF detected incidentally (e.g. by pulse palpitation in a consultation for another purpose). AF symptoms range from breathlessness or palpitations through to acute medical problems such as heart failure, stroke or TE. The precipitating trigger for an AF test is not modelled, although patients may arrive with a history of AF-related conditions and an average utility reduction is applied to reflect other symptoms.
Patients entering the model with suspected AF are referred to a specialist for an electrocardiogram (ECG) (D1). AF can be missed by an ECG test, as it is often intermittent in nature (paroxysmal AF). If AF is not confirmed by the ECG and the patient is not suspected of having paroxysmal AF, they will be discharged (D13). However, a negative ECG might be accompanied by suspicion of paroxysmal AF (e.g. if the patient reports symptoms such a fast heartbeat). In this case, an ambulatory ECG test might be performed, either: (a) event-recorder related electrocardiogram ECG (ER ECG) (D8); or (b) a 24-hour ambulatory ECG (D9). In general, the 24-hour ambulatory ECG would be used in patients with suspected asymptomatic episodes or symptomatic episodes < 24 hours apart, whereas the ER ECG would be used in those with symptomatic episodes > 24 hours apart. If an ambulatory ECG test is negative, and the doctor has a high index of suspicion, a further ER ECG might be requested. The model assumes that patients can receive up to three negative ambulatory ECG tests before being discharged from the system (D13).
After diagnosis of AF (by standard or ambulatory ECG), the patient might be referred for additional tests, including a transthoracic echocardiogram (TTE) (D4) and possibly also a transoesophageal echocardiogram (TOE) (D6). TTE and TOE may be used to diagnose structural heart defects or to plan cardioversion. However, these treatments were not included in the model, as this was outside the scope of CG36.
The diagnostic pathway is further illustrated in the decision tree in Figure 17 . This made use of data on the diagnostic accuracy of ECG from the HTA report by Hobbs and colleagues, 138 although data to populate this decision tree were sparse.
Decision tree showing detail of diagnostic pathway. FN, false-negative; p, prevalence of AF in patients tested for suspected AF; THIN, The Health Improvement Network; TN, true-negative.
Classification
After diagnosis, patients are classified into the three types of AF: paroxysmal (spontaneous termination < 7 days and most often < 48 hours); persistent (not self-terminating and lasting > 7 days or prior cardioversion); or permanent (not terminated, terminated but relapsed, or failed cardioversion attempt). The main significance of this classification is that it is used, along with other criteria, to choose the AF treatment strategy.
- Patients with paroxysmal AF will usually follow a rhythm control strategy, with AADs used to reduce the frequency of subsequent AF episodes.
- Patients with permanent AF will follow a rate control strategy, in which no attempt is made to regain sinus rhythm, but instead drugs are used to control heart rate and avoid symptoms and potentially dangerous tachycardia.
- Patients with persistent AF may follow either a rate or rhythm control strategy: CG36 defined criteria to inform this choice based on the patient’s age, whether they have a history of coronary artery disease or left ventricular dysfunction (LVD), or if they are unsuitable for cardioversion or contraindicated to AADs.
Regardless of the strategy for treating AF, it is recommended that all patients should have their SR assessed.
Assess stroke risk
If patients are contraindicated to the OACs (SR1), then they are prescribed aspirin (SR4). Patients not contraindicated to an OAC (SR1) have their SR assessed (SR2). There are three levels of risk defined in CG36:
- low risk (aged < 65 years with no moderate- or high-risk factors)
- moderate risk (aged ≥ 65 years with no high-risk factors or aged < 75 years with hypertension, diabetes or vascular disease)
- high risk [previous ischaemic stroke/transient ischaemic attack (TIA) or thromboembolic event, aged ≥ 75 years with hypertension, diabetes, vascular disease, heart failure, or impaired left ventricular function on echocardiography].
Patients at low risk are recommended for aspirin (SR4). Patients at moderate SR may be treated with either aspirin (SR4) or warfarin (SR6). If patients are assessed as high risk then they will get treated either with warfarin (SR6) or dabigatran/rivaroxaban (SR7).
After SR assessment, patients proceed to either rate or rhythm control treatment.
Rhythm control for paroxysmal atrial fibrillation
Patients assigned to a rhythm control strategy for paroxysmal AF (RYpx) might choose at first not to receive any AAD treatment (RY1b) or to use a ‘pill-in-the pocket’ approach (RY1c) if this is suitable. The first line of regular AAD treatment is a standard beta blocker (BB) (RY2). After failure of treatment with a BB, the next line of treatment is either a class 1c agent (RY5), sotalol (RY6), or amiodarone (RY9), depending on whether the patient has a history of coronary heart failure or coronary artery disease. In addition, NICE TA197 150 recommends dronedarone as a second-line treatment for patients with additional risk factors. Once AAD treatment has failed, the guideline recommends that patients are referred to a tertiary specialist (RY11) for consideration for ablative treatment.
When patients have been allocated to an AAD treatment, they progress to OM.
Cardioversion
Patients with persistent AF following a rhythm control strategy will undergo a trial of cardioversion. If the onset of AF was > 48 hours before the cardioversion (C1), electrical cardioversion (ECV) is recommended (C7), preceded by 3 weeks of warfarin and/or TOE-guided ECV to reduce the risk of TE (C3). Patients with a high risk of cardioversion failure will also receive 4 weeks of sotalol or amiodarone (C8) before ECV. Patients with AF onset < 48 hours ago would benefit from speedier treatment, so prophylaxis with heparin administered by injection (C2) is used prior to cardioversion. In these patients, the guideline recommends use of either ECV or pharmacological cardioversion (PCV). Patients with structural heart disease (SHD; coronary artery disease or left ventricular dysfunction) undergoing PCV will be treated with intravenous amiodarone, otherwise a class 1c agent is recommended. If cardioversion (PCV or ECV) is not successful, the procedure can be repeated. The model assumes a maximum of two cardioversion attempts.
After an attempt at cardioversion, patients will have their SR assessed, before proceeding to the rate or rhythm control modules.
Rhythm control for persistent atrial fibrillation
The sequencing of AADs for patients with persistent AF following a rhythm control strategy is similar to that for patients with paroxysmal AF, except that a pill-in-the-pocket approach or no treatment is not usually considered appropriate. After initiation of an AAD, patients enter the OM strategy.
Rate control for persistent and permanent atrial fibrillation
The rate control strategy contains three lines of drug treatment, followed by referral to a tertiary specialist if the heart rate remains uncontrolled [> 80 beats per minute (b.p.m.)]. The first line is a rate-limiting calcium antagonist (RLCA) (RA3) if heart rate control during exercise is required, or otherwise BBs (RA2). If these treatments are unsuccessful at controlling the heart rate, digoxin is added (RA6 and RA7), followed by amiodarone (RA10).
Acute-onset atrial fibrillation
Patients presenting with an acute arrhythmia associated with haemodynamic instability will first undergo ECG, radiography and check of electrolytes (A1) to establish the cause of the haemodynamic instability if possible. If the situation is life-threatening, an emergency ECV will be performed (A13). If the haemodynamic instability is not life-threatening, patients not already taking anticoagulants will be given heparin (if not contraindicated) before proceeding to treatment.
For patients known to have permanent AF, then urgent treatment with an intravenous rate-control drug will be used to reduce the heart rate. This will usually be either a BB (A17) or RLCA (A18), although amiodarone (A24) may also be tried. If the AF is not known to be permanent, then urgent rhythm control with cardioversion will be tried (A9). ECV (A14) is recommended in this context, although PCV (A15 and A16) may be used if there is a delay in organising ECV. For PCV, the guideline recommends intravenous flecainide if the patient is known to have Wolff–Parkinson–White syndrome, or intravenous amiodarone otherwise.
After treatment, patients with onset of AF < 48 hours previously or at high risk of recurrence will be offered 4 weeks of warfarin, before being routed to further treatment.
Ongoing management
On entering the OM module, an appointment with the GP or specialist (OM1) is scheduled, according to the recommended follow-up frequencies from the guideline (CG36, chapter 12). 135 Patients then wait (OM2) until the next one of five types of event occurs:
- Loss of AF control (OM3). For patients on a rhythm control strategy, this will be an AF recurrence (loss of sinus rhythm). A recurrence may be ‘undocumented’, which is not sufficiently serious to trigger a consultation and the patient continues to wait until the next event, or ‘documented’ which causes the patient to seek medical attention. Documented recurrences can be acute, in which case the patient is routed to the acute onset module (A). Otherwise, patients are routed to classification (CL) to be allocated to the appropriate treatment strategy. In patients on a rate control strategy, loss of control is defined as having a resting heart rate > 80 b.p.m., which may be of acute onset (route to module A) or non-acute (route to CL).
- Major adverse event (OM4). Events included in the model are thromboembolic events (ischaemic stroke, TIA or other) and bleeds (haemorrhagic stroke or major bleed). These events may be fatal. If the patient survives, they will be routed to the classification module (CL), where their treatment will be reassessed.
- New risk factor (OM5): The onset of new risk factors, such as hypertension, diabetes or passing an age threshold, can have two effects. First, it can increase individuals’ risk of major events, reducing the time to their next major event within the OM module. Second, additional risk factors might trigger a change in treatment, as patients meet criteria that they would have previously failed. In this case patients are routed back to classification (CL) to have their treatment adjusted.
- Drug withdrawal (OM6). Patients might stop taking a drug, either due to an adverse effect or for some other reason. After a drug withdrawal, patients are sent to classification (CL), and will pass again through the pathway to have alternative treatment considered.
- Routine follow-up (OM7). It is assumed that previously undocumented AF recurrence will be detected at this time, when patients are asked about symptoms and have further tests. In such cases, patients are returned to classification (CL) and have their treatment reconsidered. Otherwise, patients have their next routine visit scheduled, and then wait for the next event (OM2).
The disease process model
The above rules were based on a model of the risks associated with AF, as illustrated in Figure 18 . This is built around the five types of outcome shown in the column on the far right:
Atrial fibrillation disease process model. Definitions for the risk factor variables are given in Table 23 . NSAID, non-steroidal anti-inflammatory drug; THIN, The Health Improvement Network.
- Loss of AF control. This was defined as loss of sinus rhythm for patients being treated with a rhythm control strategy (paroxysmal and some persistent). In patients with paroxysmal AF, this loss of sinus rhythm could be documented or undocumented, depending on whether or not the symptoms were sufficient for the patient to seek medical attention. For patients being treated under a rate control strategy, AF control was defined as maintenance of a resting heart rate < 80 b.p.m.
- TE events. These were defined to include ischaemic strokes, TIAs and other major thromboembolic events. The risk of TE is greatly increased with AF, and so it is an important outcome to include within the model.
- Bleeds. Including haemorrhagic strokes and other major bleeds. These events are included as an outcome, because drug treatment to prevent TE increases the risk of bleeding.
- Other related risks. The incidences of several other conditions (hypertension, diabetes, CHD and heart failure) were modelled as risk factors for the above directly AF-related outcomes.
- Death. Mortality unrelated to AF was modelled independently of the other risk factors (other than age and sex). Mortality related to AF was modelled by applying case-fatality rates to acute-onset arrhythmias, thromboembolic events and bleeds.
Loss of AF control, TEs and bleeds impact directly on health status (and hence on QALYs) in two ways: (1) they can be fatal; and (2) in patients who survive the event, they can reduce quality of life (utility). Patients who survive also incur additional treatment costs. We did not include costs or QALY losses for other conditions modelled as risk factors (hypertension, diabetes, CHD and heart failure). We assumed that ischaemic and haemorrhagic strokes would have a lasting impact on utility and health-care costs, owing to the high potential for disability. Other events were assumed to have more transient consequences, incurring costs and utility decrements over a short initial period.
The risk equations or algorithms listed in the second column of Figure 18 were used to calculate individuals’ risks of the included outcomes in the absence of treatment. There are five main classes of risk calculation used in the model, based on the five types of outcome. The risks of loss of AF control and progression between the types of AF (paroxysmal, persistent and permanent) were defined according a model that we developed. The risks of TEs and bleeds were defined by published risk algorithms for patients with AF: CHA 2 DS 2 -VASc and HAS-BLED respectively. 158 – 160 Rates of incidence for the other risk factors were also based on published sources: Framingham equations for CHD, type 2 diabetes and hypertension. 161 – 163 and simple age- and sex-based incidence from a cohort study for heart failure. 164 Mortality rates from non-AF-related causes were based on national life table data. 87
The inputs required for these risk calculations define the set of individual risk factor information that is required for the model (listed in the first column of Figure 18 ). The risk factors in bold were defined in advance of model entry, as variables from our individual-patient data set from The Health Improvement Network (THIN) (see Table 23 ). The factors in grey are assigned as patients move through the simulation model.
Risk factors drawn from THIN data
Finally, the third column in Figure 18 lists the treatment effects that are used to modify individuals’ baseline risks in accordance with any treatments that they receive. Treatments are grouped into four classes, defined by their major outcome targets: cardioversion (aim to regain sinus rhythm); rhythm control drugs (aim to prevent AF recurrence); rate control (aim to achieve control of heart rate); and antithrombotic (aim to reduce the risk of TE, while minimising impacts on bleeding). In addition, a withdrawal rate was defined for each drug.
Atrial fibrillation progression and control
The process by which patients pass through the different types of AF is illustrated in Figure 19 . If the first diagnosed episode terminates without intervention within 7 days, patients are classified as having ‘paroxysmal’ AF. They pass through the service pathway, as described above, are prescribed appropriate antithrombotic and antiarrhythmic therapy, and move into the OM module. If they have an AF recurrence, this will be one of three types: an ‘undocumented’ recurrence for which they do not seek medical attention and remain in OM; a documented recurrence that is self-terminating within 7 days but leads to a reassessment of their antithrombotic and antiarrhythmic medication; or onset of CAF that does not self-terminate within 7 days. The latter defines a transition from paroxysmal to persistent AF.
Model of AF progression and control.
Patients with an episode of AF that is not self-terminating in 7 days (either a first episode or a CAF recurrence of paroxysmal AF) are considered for cardioversion. If they are suitable for this procedure, it is scheduled, and if successful the patient is classified as having ‘persistent’ AF. Patients with persistent AF are prescribed appropriate antithrombotic and antiarrhythmic therapy, before going to OM. If they have a recurrence, it is assumed that they would require cardioversion to move back into sinus rhythm. This is a simplification, as in reality patients with persistent AF may also experience paroxysmal episodes.
A patient with AF that has not terminated within 7 days, who is not suitable for cardioversion, or for whom cardioversion has failed, is classified as having ‘permanent’ AF. Patients with permanent AF are given appropriate antithrombotic and rate control drugs, before going to OM. If the rate control drugs are insufficient to bring their resting heart rate below the threshold of 80 b.p.m., their treatment will be reassessed at their next scheduled appointment.
When patients experience an AF recurrence (paroxysmal or persistent) or uncontrolled heart rate (permanent), this may be of acute onset, necessitating an emergency cardioversion or rate control intervention.
Risk of thromboembolism (CHA 2 DS 2 -VASc)
Atrial fibrillation is associated with a substantial risk of stroke and other TE. This risk is not homogeneous and various risk factors have been identified that are predictive of stroke in AF. 165 These risk factors have been formulated into various SR stratification schemes. 159 , 165 – 170
A well-known and simple risk assessment scheme is the CHADS 2 score. 171 This evolved from the AF investigators’ and Stroke Prevention in Atrial Fibrillation Investigators’ criteria, 172 and is based on a point system, in which two points are assigned for a history of stroke or TIA and one point each is assigned for age > 75 years and a history of hypertension, diabetes, or recent cardiac failure. The CHA 2 DS 2 -VASc extends the CHADS 2 scheme by adding vascular disease (MI, peripheral arterial disease or aortic plaque) as a risk factor. 159 The score is calculated by adding one for each risk factor, and an additional point each for age > 75 years and prior stroke.
Risk of bleeding (HAS-BLED)
Various risk stratification systems have been proposed for the assessment of the risk of bleeding. 160 , 173 , 174 Individuals’ risk of bleeding in the model is assigned on the basis of the HAS-BLED risk algorithm. 160 This was developed from a cohort study of 3978 European subjects with AF from the Euro Heart Survey. The HAS-BLED score is calculated by adding one for each of the following risk factors: hypertension, abnormal renal/liver function, stroke, bleeding history or predisposition, labile international normalised ratio (INR), elderly (aged > 65 years), drugs that increase the risk of bleeding [e.g. non-steroidal anti-inflammatory drugs (NSAIDs) or aspirin], and alcohol (≥ 8 units/week). Coefficient calculations exclude data on labile INR as they were not available for the cohort. We also excluded antiplatelet therapy when calculating individual HAS-BLED scores in the model, to avoid double-counting the effect of aspirin on bleeding rates (as we applied relative risks of bleeding with aspirin and OACs).
Other related risks
The risks of new-onset CHD, hypertension and diabetes were calculated using multivariate risk equations estimated from the Framingham cohort study. 161 – 163 Finally, the risk of onset of heart failure was estimated based on age/sex specific rates from a general population cohort. 164 These sources are not ideal for our purpose, as they are estimated from a general population cohort, rather than from people with AF.
- Model design
Discrete event simulation model
The DES model combines the conceptual service pathway (see Figure 16 ) and the disease process model (see Figure 18 ) into a single dynamic incident cohort model that incorporates time. The patients are modelled as individual entities. Each has labels attached, which record their personal characteristics, including the risk factors listed in the first column of Figure 18 , as well as a record of their treatment and event history that accumulates within the model. The values assigned to each label may change as the model runs.
The patients travel through the model accruing costs and QALYs as they receive treatment and experience events. The patient’s route may be determined by the values stored in their labels (criteria-based routing), or by sampling from a defined distribution (probability-based routing). For instance, in the classification module the decision to adopt a rate or rhythm control strategy for a particular patient is informed by the contents of the label that records whether they have paroxysmal, persistent or permanent AF, whereas in the diagnosis module, the choice between a 24-hour or ER ECG is randomly decided according to defined probabilities. In this way, each patient’s route through the model is tailored to their individual characteristics, but also depends to some extent on chance.
Selection of the patient cohort
The model contains information on 12,776 patients with newly diagnosed AF, drawn from THIN primary care database (see Data sources below). The database contains the characteristics listed in Table 23 for each patient.
When the model is run, it is possible to randomly select patients from a subset based on their initial characteristics. For example, it would be possible to select a group of patients within a specific age range who have a history of hypertension. Sampling from the list of eligible patients is random ‘with replacement’. The cohort of patients arrives in the system at time zero, and the model runs until all patients from the cohort have died.
Set up attributes
When the sampled patients arrive in the system, information about their starting state is read from the database and copied to the appropriate labels. Patients are assigned a label to specify that they have AF that is currently undiagnosed (CurrentAFstate = 5). All patients are also assigned a label (Non_AFdeathAge) which specifies their age of death, unless they die from an AF-related cause prior to this. National life table data were used to generate probability distributions for life expectancy, based on the patient’s initial age and sex. Patients’ starting utility is assigned (CurrentUtility) based on their age and sex. This is obtained from a look-up table, so all patients of the same age and sex will have the same starting utility. Labels are also set to indicate that patients are not initially taking any medication. Each patient has a random number (U) assigned for each of the events to be modelled (e.g. U_bleed, U_diabetes). These are used when calculating, and updating, the time to each event. Finally all patients arriving have a label i_NextEvent initialised to ‘First Event’. This next event label is used to control the routing of the patient through the OM section of the model.
The diagnostic section of the model contains more probability-based decisions than the rest of the model. This reflects the difficulty that we experienced in obtaining data for this module. The intermittent nature of AF makes it impossible to establish false-negative rates for the diagnostic tests, and there is no ‘gold standard’ for assessment of diagnostic accuracy. The model does include the facility to add patients without AF, and to include false-positive test results for these patients, incurring unnecessary expenditure. However, this was not applied in the analyses presented below.
Diagnosis is one of the two sections of the pathway in which time elapses as the patient progresses. Patients arrive and have to wait a number of days for their ECG based on a defined distribution [mean 14 days, standard deviation (SD) 3 days]. Costs associated with ECG are added to the patient’s tally of costs. For patients with AF, there are four possible routes after ECG: (1) the patient has confirmed AF and there is no need for more tests; (2) the patient has confirmed AF but TTE/TOE is required to assess underlying physical problems; (3) the patient’s ECG was negative but there is still a suspicion of AF so either 24-hour ambulatory ECG or ER ECG is required; or (4) ECG is negative and there is no suspicion of AF (false-negatives). Patients in whom ambulatory ECG is necessary are randomly allocated to either 24-hour or ER ECG. Those patients in whom results are negative may then undergo ER ECG or be incorrectly discharged (false-negatives); again this is randomly allocated.
After a positive diagnosis, patients are randomly allocated to have paroxysmal or persistent AF, in accordance with a defined probability, and their CurrentAFstatus label is updated to 1 (paroxysmal), or 2 (persistent). It is assumed that no patients present for the first time with permanent AF.
False-negatives
The patients who receive a false-negative diagnosis remain in the system to ensure a penalty for missing cases is incorporated. These patients loop through a similar (but reduced version) of the OM module (described below). These untreated AF patients have their risk factors and characteristics updated in the same way as treated patients, and incur the costs and consequences of any major events that occur. If these patients experience an arrhythmic event then, depending on the severity, they will either present again for an ECG (effectively restarting this process after a delay), or they will present at the accident and emergency department for cardioversion. As these patients will not be taking medication for their AF, they do not receive any protective benefit from antithrombotic or rhythm or rate control drugs.
All patients arriving in the classification section of the model will have the CurrentAFstate label set to 1 (paroxysmal), 2 (persistent), or 3 (permanent). Only those who have cycled through the system at least once can be classified as having permanent AF. Patients with paroxysmal AF are assigned to a rhythm control treatment strategy, patients with permanent AF are assigned to rate control treatment strategy and patients with persistent AF are assigned to either a rate control or rhythm control strategy depending on their attributes in accordance with the guidelines. Patients have their TreatmentOption label updated to reflect the strategy that is adopted, and are directed to cardioversion or SR classification as appropriate.
Patients with persistent AF who are assigned to a rhythm control strategy, and who are not in sinus rhythm when they pass through the classification process, receive non-emergency cardioversion. The number of cardioversion attempts per episode is limited, currently set to a maximum of two attempts, though this parameter may be changed. If cardioversion is unsuccessful, then the patient is considered to be in permanent AF, their i_CurrentAFstatus label is updated and they are assigned to a rate control strategy. If cardioversion is successful then they remain on a rhythm control strategy and remain labelled as having persistent AF. Following cardioversion, patients are routed to have their SR assessed.
Stroke risk assessment and antithrombotic therapy
When patients enter this module, their SR scores are recalculated (CHADS 2 and CHA2DS 2 -VASc) and their antithrombotic therapy is reassessed on the basis of criteria set out in the NICE AF CG.
The NICE criteria for assignment of anticoagulation depend on age and various other risk factors (CG36, 136 TA249 146 and TA256 147 ). In many cases patients are eligible for more than one drug, so some assumptions are needed to determine the split of patients between these options. An example of the percentage split between assumptions used for the 65–74-year-old age group is shown in Table 24 . This shows the assumed percentage split between the eligible drugs for each risk group. If a patient is contraindicated to a drug or has previously withdrawn from taking it, the percentages are revised to ensure that the total equals 100% across all eligible options. The patient is then randomly allocated a suitable anticoagulant based on these revised percentages.
Choice of antithrombotic drugs by risk factors: 65–74-year-old age group
Rate and rhythm control
Each patient has two labels in which their current medication is recorded, one for antithrombotic drugs and one for rate or rhythm control drugs. These record the row number associated with the particular drug, as shown in Table 25 . The order of the rows for all the tables associated with the drugs (such as utilities, costs, etc.) is the same throughout the model. This allows the addition of other drugs at a later stage.
Drugs currently included in the model
The selection of the next line of rate or rhythm control treatment is dictated partially by the guideline and, where there is a choice of medication, by a similar random process as described above for anticoagulants. There are three tables – one each for paroxysmal, persistent (rhythm control), and rate control – that record the recommended sequencing of medications. These tables contain 21 medications on both the horizontal and vertical axes. The cells within the tables detail the percentage chance of changing from any given drug (vertical axis) to another (horizontal axis). Similarly to the anticoagulants, the percentages are read from the table and adjusted to take account of any existing contraindications for the patient, before patients are randomly assigned to one of the remaining drugs for which they are eligible. On a change of medication, the previous drug is marked as contraindicated to prevent selection again in the future
Once a patient has been prescribed a new drug they are routed to the OM section of the model. Patients may pass through the rate and rhythm control sections of the model many times, as their AF progresses, or if they withdraw from a drug. A similar process of drug selection is followed for each line of treatment. Once patients have exhausted all lines of treatment they are referred to the tertiary specialist for consideration for an interventional procedure. We assume that they will continue to take the final line of treatment following referral.
Patients travel through the model and after being diagnosed and receiving their first-line treatment options, arrive in the OM section of the model ( Figure 20 ). This section is the main driver behind the model. When patients enter, all of their risk scores and risk values are updated based on their current characteristics and any treatment effects. From these updated risks, a ‘time-to-event’ is calculated for each event of interest. These times, and the time to non-AF death, are compared to find the minimum, and this event is designated as the next event. The patient then waits until the time designated for this event to occur. This means that each patient moves through the model in time increments defined by the events that they experience, as opposed to all moving at pre-defined time intervals.
Illustration of OM process.
If the event is not related to AF, and would not cause a change in rate or rhythm control treatment, the patient’s antithrombotic risk is reassessed and changed if appropriate. The patient’s risk factors are updated, the effects of the new anticoagulant applied, and the ‘time-to-event’ recalculated. If, however, the patient’s AF has progressed, they are routed back through the classification module, from where they may change from rhythm control to rate control, be referred for cardioversion, and/or move to the next line of treatment. Following this, they return to the OM, where the process repeats.
Modelling risk
The chance of not having a particular event until a time t , given an adjusted hazard rate of λ is modelled by an exponential survival function ( F ( t ;λ) = 1 – e –λ t ). This assumes that the hazard remains constant over the period of time modelled. However, as patients move through the model, their risk of a particular type of event may change in response to other associated risks, or as they age. We modelled this using a piecemeal exponential distribution, in which the hazard changes at defined points in time (when an event has occurred), but is constant in between events.
The times at which events occur are determined by random numbers. At model entry, each patient is assigned a random number for each of the main types of event in the model. This could be considered as a proxy for unknown factors that influence a patient’s propensity for that particular type of event. TE events, bleeds and AF recurrences are composite events, each consisting of a number of subtypes of event. TE events comprise ischaemic strokes, TIAs and other thromboembolic events, bleeds comprise haemorrhagic strokes and other major bleeds, and recurrence events comprise undocumented events, self-terminating events, non-terminating events requiring cardioversion, and acute arrhythmic events requiring emergency cardioversion. For each composite event, patients are assigned another random number, which determines which subtype of event will occur next. This approach ensures that related groups of events are not treated as independent. 61
Once an event has occurred, care is needed in adjusting the times for competing events to avoid counterintuitive effects. The process for sampling time to event is illustrated in Figure 21 . For simplicity, suppose initially that there are only two types of event, TEs and bleeds. On entry to the model, an individual is assigned two random numbers between zero and one: one for TEs U 1 T and one for bleeding U 1 B . The person’s starting attributes are used to calculate CHA 2 DS 2 -VASc and HAS-BLED and initial hazard rates for TE λ 1 T and bleeding λ 1 B are estimated.
Illustration of the sampling process for the AF model. (a) TE first event; (b) TE second event; (c) bleeding first event; (d) bleeding second event.
The times to events are calculated using the inverse of the exponential survival function: T 1 k = F − 1 ( U 1 k , λ 1 k ) = − ln ( 1 − U 1 k ) / λ 1 k , where k = T , B . Suppose that T 1 T = 10 and T 1 B = 20 , so that a TE is the first event to occur. At year 10, having had one TE, the person is now at higher risk of a second TE and also at higher risk of a bleed. The CHA 2 DS 2 -VASc and HAS-BLED scores are updated, the patient is assessed for any changes in treatment and revised estimates of the hazard rates: λ 2 T and λ 2 B are obtained. The time of the next TE T 2 T is calculated as before, using a new random number U 2 T . However, if we were to do the same for bleeding, there is a chance that we could draw a random number such that the first bleed would occur later than had been originally expected: T 2 B > T 1 B . This would be counterintuitive, as the time to a bleed would appear to have been increased by the occurrence of a TE. To avoid this, instead of drawing a new random number for bleeding, the original number U 1 B is adjusted to reflect the remaining probability of a bleed, conditional on no bleed having occurred up to time T 1 T : U 2 B = ( U 1 B − a ) / ( 1 − a ) , where a = F ( T 1 T , λ 1 B ) . The time of the next bleed is then calculated as T 2 B = T 1 T + F − 1 ( U 2 B , λ 2 B ) . Thus the random number used to calculate the time to an event is only resampled when that particular type of event has occurred. This procedure is easily extended to include other types and subtypes of events, and also age thresholds that increase estimated risks or trigger new treatments.
Calculating costs and quality-adjusted life-years
For each outcome event there may be short- and long-term consequences, affecting costs and QALYs. For instance, an ischaemic stroke incurs a mean cost of £11,646 associated with the initial hospitalisation and treatment during the first 90 days, followed by an ongoing cost of £22.61 per day for continuing health and social care. The model assumes that a patient experiencing the event incurs the initial cost at the time of the event (regardless of whether or not they survive for the whole 90 days), and schedules the daily cost to start after the initial period, if they survive that long. The ongoing cost continues for the remaining lifetime of the patient.
A similar method is used to apply short- and long-term modifications to patient’s utility values. A short-term utility multiplier is applied during a defined initial period after the event, followed by a long-term multiplier. The adjusted utilities are used to estimate patients’ QALYs for as long as they survive. The duration of the initial period can differ between events, and between the costs and utilities. Some types of event are assumed not to incur any lasting cost or utility effect after the initial period.
Costs and QALYs are updated every time the patient passes through a section of the pathway where a cost is applied, as well as whenever a change in treatment occurs. In addition, if the TTNE is more than the ‘Frequency of Update’ variable (currently 90 days) then an update of costs and QALYs is scheduled. Costs and QALYs are discounted using a continuous time approach to the time of model entry for each patient.
- Data sources
A list of all parameters needed to populate the model was compiled. Potentially relevant sources of information to define these parameters were first identified from the searches conducted for CG36. 135 This provided a base of evidence of clinical effectiveness, which we knew had been identified through systematic searches and quality appraised. In extracting this evidence, we paid particular attention to the commentary on the quality and interpretation of the evidence base in the ‘from evidence to recommendations’ sections of the full guideline. Clinical papers that had informed GDG decisions were obtained and relevant data were extracted.
However, a recurring problem with CG36 135 as a source of data for modelling was the lack of meta-analysis in this document. When multiple studies related to a question were identified, the results were presented in a narrative fashion, with no attempt to statistically pool the available data. We were also aware that for some interventions the evidence base reported in CG36 135 was significantly out of date. It was not possible to conduct our own systematic reviews within the resources available, so where necessary we relied on other published systematic reviews and meta-analyses. Reviews conducted for NICE TA reviews were prioritised as sources of evidence for the model, as we knew that they will have undergone rigorous review and public consultation. Where NICE TA reviews were not available, we sought to identify information from other HTA reports, Cochrane reviews or other high-quality sources. However, it should be emphasised that effectiveness estimates were not all based on up-to-date systematic reviews, and that the results should therefore not be used to inform clinical decisions.
In addition to evidence about clinical effectiveness, we needed data to inform other model parameters, including background rates of the adverse events defined in the disease process model, utilities and costs. The other major data requirement for the model was information to define a representative cohort of patients to feed into the model. Individual patient data were obtained for a cohort of patients newly diagnosed with AF from a primary care database. These data are described below.
Individual patient data set
The Health Improvement Network (THIN) is a research database comprising anonymised patient records uploaded from primary care information systems. THIN data collection began in 2003, and the database currently contains data from 479 practices with a total of 9.1 million patients. We obtained an extract of data for patients registered on an index date (1 May 2008), aged ≥ 30 years, without any record of an AF diagnosis during the 2-year period prior to the index date. Individuals within this group with a record of an AF diagnosis during the 2-year period after the index date were then identified, defining an incident cohort of 12,776 patients who were used to populate the model. Demographic and medical information was collated for these patients for the 2 years before and 2 years after the index date (from 1 May 2006 to 30 April 2010), although some patients left the system during the 2 years after the index date. The list of patient-level variables used in the model is shown in Table 23 .
Some variables were recorded more than once over the 4-year follow-up period (e.g. blood pressure and lipid levels had often been measured several times during this time). In such cases, we took the average of the three readings closest to the date of diagnosis. Some individuals did not have a record of blood pressure, body mass index (BMI), lipids (total or high-density lipoprotein cholesterol) or smoking status. These missing data were imputed using a multivariate regression approach, to provide a full data set for use in the model. Before imputation, the incident cases of AF were on average 73.6 years old, and 47% female. Around 5% had a family history of CHD. Their average blood pressure was 78/137 mmHg, their average BMI was 28.5 kg/m 2 and 12% were current smokers. In terms of medication, around 40% were on antiplatelet or lipid lowering medication and 65% were on antihypertensive medication. Twenty-one per cent had a history of haemorrhage, such as an ulcer or bleed. Imputed values for missing data items had very similar means and SDs to those in the non-imputed data.
Risks of bleeding and thromboembolism
The model uses data from the Swedish AF cohort study to estimate incidence rates for thromboembolic and haemorrhagic events. 158 This was a nationwide cohort study containing 182,678 individuals with a diagnosis of AF [ International Classification of Diseases , Tenth Edition (ICD-10; code I489:A–F)] who were treated as an inpatient or outpatient at Swedish hospitals between July 2005 and December 2008. Average follow-up was 1.5 years. This is a very large cohort, likely to be reflective of the general Swedish population with AF (although the sampling methods did exclude patients with ‘silent AF’ and patients managed only in primary care and open clinics). The other advantage of this study as a source of data for the model was that thromboembolic and bleeding events were reported for the same cohort, providing coherent estimates of these two related risks. The applicability of these data to the UK AF population is discussed below.
One-year incidence rates for TE (stroke/TIA/peripheral embolism) stratified by CHA 2 DS 2 -VASc scores were reported for 90,490 patients not treated with warfarin ( Table 26 ). Figures used in the model were adjusted for aspirin use to provide estimate rates for an untreated cohort. Figures for CHA 2 DS 2 -VASc scores ≥ 7 were pooled, as estimated rates were uncertain above this value due to small numbers of events.
Rates of TE from the Swedish AF cohort study
Similarly, incidence rates of major bleeds (intracranial and major extracranial) were reported by HAS-BLED scores ( Table 27 ). Rates used in the model were for 33,486 patients who were not on oral anticoagulation or aspirin at baseline. Event rates for HAS-BLED scores ≥ 4 were pooled, due to small numbers of events.
Rates of bleeding from the Swedish AF cohort study
We assumed that the incidence of different types of thromboembolic event would be in the same proportions as observed in the Swedish AF cohort: 70% ischaemic strokes, 25% TIA, and 5% other embolisms. Similarly, the relative incidence of bleeds was also based on the observed rates in the Swedish AF cohort: 28% intracranial and 72% major extracranial. Minor bleeds were excluded from the model.
Atrial fibrillation control and cardioversion
Data to populate the AF progression and control model (see Figure 19 ) were drawn from two main sources. Euro Heart Survey data 175 were used to derive estimates of the proportion of first episodes that are paroxysmal (42%), recurrence rates for paroxysmal AF (54% per year), rates of progression from paroxysmal to persistent AF (20% of recurrences), and the proportion of AF recurrences that are of acute onset (64%). The proportion of recurrences for patients with paroxysmal AF that are undocumented (68%) was taken from the Canadian Registry of Atrial Fibrillation study. 176
Rates of recurrence for patients with persistent AF and progression from persistent to permanent AF were determined by effectiveness data for cardioversion (CG36, chapter 5). 135 These studies reported on the initial success rate of cardioversion (reversion to sinus rhythm within 24 hours) ( Table 28 ), early recurrences (up to 1 month) and late recurrences (> 1 month) ( Table 29 ).
Cardioversion success rates
Atrial fibrillation recurrence after cardioversion
Diagnostic accuracy
Sensitivity of the initial 12-lead ECG (78%) was based on the HTA trial by Hobbs and colleagues, 138 and it was assumed that 5–24% of patients with suspected paroxysmal AF would be diagnosed following a positive 24-hour or ER ECG. 177 Other probabilities within the diagnostic pathway (including the proportion of patients with a negative ECG referred for ambulatory assessment on the basis of suspicion of paroxysmal AF, the ratio of 24-hour to ER ambulatory ECGs, referral rates for TTE and TOE) were estimated by informal elicitation from experts.
Treatment effectiveness
Estimates of the effectiveness of antithrombotic medications (aspirin, warfarin, dabigatran and rivaroxaban) were taken from a network meta-analysis conducted by the British Medical Journal Technology Assessment Group for the NICE TA on rivaroxaban. 145 This study estimated odds ratios for thromboembolic events (ischaemic stroke and systemic embolisms), bleeding (intracranial and major extracranial bleeds) and treatment withdrawals in comparison with warfarin. As inputs to the model, we estimated relative risks compared with placebo ( Table 30 ), using assumed control risks from the warfarin arm of the ROCKET AF trial (Rivaroxaban Once Daily Oral Direct Factor Xa Inhibition Compared with Vitamin K Antagonist for Prevention of Stroke and Embolism Trial in Atrial Fibrillation). 178
Treatment effects of antithrombotic drugs
Treatment effects for the rhythm control drugs were taken from a published network meta-analysis, funded by Sanofi-Aventis to inform their submission to NICE for the appraisal of dronedarone. 179 For the model, we estimated relative risks for AF recurrence and treatment withdrawals ( Table 31 ).
Treatment effects of AADs
The effectiveness of the rate control drugs was estimated by simulating an initial resting heart rate for each individual patient (sampled from a normal distribution with a mean of 109 and SD of 31). 180 Mean reductions in heart rate with rate control drugs ( Table 32 ) were estimated from four randomised cross-over studies reported in CG36 (p. 59). 180 – 183 Data were pooled using a simple inverse-variance method of meta-analysis. Estimates of the standardised mean difference were obtained for first-line treatment (BB or RLCA) compared with no treatment, and for second-line treatment (BB and digoxin or RLCA and digoxin) compared with first-line treatment. No data were found to estimate the effect of the third-line of treatment recommended in CG36 (amiodarone). We therefore assumed that this gives the same additional reduction in heart rate as second-line treatment.
Treatment effects of rate control drugs
Case fatality rates
The proportion of patients admitted for acute AF dying within 30 days of admission was estimated at 2.56% (147 out of 5735 cases in a study of all patients admitted with a diagnosis of AF in Scotland in 1996). 184 The case fatality for ischaemic stroke was estimated from a large population-based cohort study (the Oxford Vascular Study; OXVASC). 185 Fatality rates for bleeding were estimated from a pooled analysis of data from the Sport Prevention Using Oral Thrombin Inhibitor in Atrial Fibrillation (SPORTIF) III and V trials of ximelagatran compared with warfarin for treatment of non-valvular AF. 186 This estimated a case-fatality rate for major bleeding of 8.1% (very similar for the two study arms). The fatality rate among patients experiencing an intracranial bleed was much higher (10 out of 18 cases, 56%). A similar case-fatality rate was observed for haemorrhagic strokes in the OXVASC (8 out of 17 cases, 47%).
Health utility estimates were drawn from three sources. First, baseline utility values for members of the public with no history of heart problems by 5-year age band were taken from the analysis of Health Survey for England data reported by Ara and Wailoo 187 ( Table 33 ).
General population utility by age (no other heart problems)
Utilities were then adjusted for patient’s AF status, using data from the Real-life global survey evaluating patients with Atrial Fibrillation (RealiseAF) study, 188 which is an international observation cross-sectional study of patients with any history of AF in the previous year. Out of 9665 patients evaluated, 26.5% were in sinus rhythm, 32.5% had an arrhythmia but with a heart rate of ≤ 80 b.p.m. and 41% had uncontrolled AF (neither in sinus rhythm nor heart rate ≤ 80 b.p.m.). EQ-5D™ scores were available for 9644 of these patients. We used these data to estimate utility multipliers for AF status ( Table 34 ).
Utility multipliers for AF health states, RealiseAF
Finally, we needed estimates of utility losses for the thromboembolic and bleeding events included in the model. These were obtained from the Medical Expenditure Panel Survey, 189 which collected EQ-5D scores for a nationally representative sample of 38,678 non-institutionalised adults between 2000 and 2002. These data were analysed using regression methods to estimate the marginal disutilities associated with 95 chronic conditions. Estimates of utility multipliers for adverse events included in the model are listed in Table 35 .
Utility multipliers for adverse events, Medical Expenditure Panel Survey
Finally, estimates of costs for tests and treatments administered in the service pathway, drug costs, and costs for adverse events are shown in Tables 36 , 37 and 38 respectively.
Costs for consultations and tests on the pathway
Daily drug costs (NHS net price, BNF)
Cost of adverse events included in model
- Verification and validation
The model was coded using SIMUL8 software by one of the authors (JE). The data spreadsheet was prepared by another author (MTB), who worked closely with JE to ensure that the data interface worked correctly. Data entry was checked by another author (JL), by comparison of data from the original papers with the numbers in the spread sheet. The modelling team (MTB, JE and JL) met regularly to discuss and resolve problems arising. At several stages in development, the model was discussed at meetings of the wider MAPGuide team, and issues about model structure, coding and data sources were considered.
Various steps were taken during model development to avoid potential errors. This included double coding of some of the more complicated formulae in Excel and SIMUL8, to check that they were being applied correctly. This included: the method for sampling time to event and for dealing with competing risks; the formulae used to calculate individuals’ risk of CHD, diabetes and hypertension (the Framingham formulae 161 – 163 ); and the formula for continuous discounting of costs and QALYs. A patient diary was also created to collect information about the events and timelines for individual patients running through the model, and to present this in the form of individual ‘case histories’. These diaries were used throughout development, and towards the end of this process case histories were checked for 500 patients by members of the modelling team, and any apparent inconsistencies or errors were identified, discussed and if necessary investigated.
Verification of the coding was commissioned to an external modeller based at the University of Sheffield. This comprised three tasks:
- checking that the SIMUL8 logic correctly reflected the AF pathway
- checking the coding of costs, QALYs and discounting calculations
- checking the model logic via the patient diaries.
Finally, the model outputs were compared with the model inputs to ensure that the rates of thromboembolic events and bleeds observed for a modelled cohort matched the input data (‘internal validation’). The results of the model were also analysed to verify that the relative risks for different antithrombotic drugs correctly reflected the data built into the model.
The following list summarises the set of assumptions that were agreed during model development.
Summary of model simplifications
- Patients’ individual blood pressure and cholesterol levels remain constant throughout.
- Patients have hypertension if they are taking antihypertensive drugs at the start (regardless of their blood pressure).
- As the Office for National Statistics data only cover ages up to 100 years, patients aged > 99 years are not considered eligible for this model.
- ER and 24-hour ambulatory ECG tests have a specificity of 1 (i.e. no false-positives as it is unlikely that an arrhythmia will be picked up if it does not exist), but have varying sensitivities to allow for false-negatives.
- By definition, no one can be classified as in permanent AF state on initial diagnosis as they will not have had the opportunity to try cardioversion at this point.
- The contraindicated label encapsulates any reason that the patient cannot take the particular drug, so includes reactions, treatment failure, and ineligibility due to personal characteristics.
- Patients continue with the final line of treatment after referral to the tertiary specialist, but continue in the model accumulating costs and QALYs until their death. This section is outside the scope of the guideline, but it was felt that the costs and QALYs should be included in the analysis.
- No time passes while patients pass through the classification, SR classification, acute onset, cardioversion, rate control and rhythm control sections of the model. The reason for this assumption is that we are not currently modelling resources explicitly, and as costs are accumulated on a daily basis, times of < 1 day will not change the results.
- We are not considering the costs, impact and effects of medications for comorbidities within the model, so only the medications and anticoagulants that are prescribed as part of the treatment for AF are considered.
- Modelling pathway changes
The approach to evaluating the selected topics by the AF model are outlined in Table 39 , and discussed in more detail below.
Topics for AF model
Topic A: prophylaxis for prevention of post-operative atrial fibrillation
Onset of AF following cardiothoracic surgery is a common problem. This is sometimes transient, but AF can persist and, if so, is associated with potentially serious effects (including haemodynamic instability, ischaemia, heart failure and stroke and TE). 135 CG36 135 recommends the use of prophylaxis to prevent post-operative AF (amiodarone, BBs, sotalol or RLCA) and management with an initial rhythm-control strategy (chapter 10). The 2011 review 195 highlighted new evidence related to the choice of prophylactic treatment (including statins and corticosteroids as well as AADs), the timing of prophylaxis (pre, intra- or post-operative), and subsequent treatment.
However, the modelling team did not include post-operative AF in the base-case model because the clinical and demographic characteristics of patients undergoing cardiothoracic surgery and their risks of adverse events differ from those of the general AF population. Although the base-case model could be adapted to reflect post-operative AF, this would require further consultation with experts, identification of suitable evidence sources for both baseline risks and treatment effects and possibly restructuring of the model. Consequently, this topic was not evaluated.
Topic B: drugs for pharmacological cardioversion
Background to topic.
CG36 135 made recommendations for the cardioversion of patients in AF, including patients presenting as an emergency with haemodynamic instability (chapter 7) and stable patients for whom a rhythm control strategy is being pursued (chapter 5). For the latter more common situation, the evidence suggested that ECV and PCV were of comparable efficacy. Based on clinical practice and opinion, the guideline group recommended ECV for prolonged AF (episode lasting for ≥ 48 hours), and either PCV or ECV for AF of more recent onset. Evidence on the relative effectiveness of drugs for PCV suggested that although the class 1c AADs (flecainide and propafenone) are more effective than amiodarone in the short term, they achieve a similar rate of conversion to sinus rhythm by 24 hours. The guideline group noted concerns over the safety of class 1c drugs in patients with SHD (coronary artery disease or LVD). They therefore recommended amiodarone for PCV in patients with SHD, and a class 1c drug for other patients. The group also reviewed evidence relating to adjuncts to ECV, including concomitant use of AADs to increase the chance of success and reduce recurrence, and anticoagulation and TOE to reduce the risk of stroke and TE associated with the ECV procedure.
The 2011 review 195 identified new evidence relating to the choice of methods for cardioversion as part of a rhythm control strategy. This included studies comparing drugs for PCV, ECV with concomitant AAD treatment and different ECV techniques. Four RCTs were found relating to a new class III agent, vernakalant (Brinavess ® , Cardiome), which is licensed for cardioversion of AF episodes lasting for ≤ 7 days. This drug was referred to NICE as a Single Technology Appraisal, but the process was suspended due to information about the timing of launch in the UK. Vernakalant is not yet available in the UK and does not have an NHS price, so evaluation is not currently possible. None of the new evidence relating to other drugs for PCV identified in the review document provides a suitable basis for economic evaluation, as it has have not demonstrated superiority over current recommendations. Similarly, it is difficult to identify a clear evidence base to test potential changes in CG36 recommendations on concomitant AAD treatment with ECV, or strategies to prevent TE associated with ECV.
Approach to economic evaluation
We therefore decided to use existing evidence included in CG36 to illustrate how the model could be adapted for evaluation of different methods of cardioversion. As an example, we focus on the choice of PCV drugs in patients with AF onset of < 48 hours, without haemodynamic instability or SHD (recommendation R8, p. 40, CG36 135 ): comparing the use of class 1c drugs (as is currently recommended) with amiodarone.
Key factors likely to drive the cost-effectiveness of different methods of cardioversion are: the frequency and speed of conversion to sinus rhythm; the frequency and severity of adverse events; the risks of future recurrence; and the cost of the procedure, consumables and associated hospital stay. The efficacy and speed of cardioversion is clearly important for patients (as faster resolution of symptoms will alleviate discomfort, distress and anxiety). The short duration of this benefit and the lack of quality-of-life data make it difficult to capture in a QALY metric. However, speed of cardioversion is likely to impact on the use of NHS resources and costs, which should be easier to estimate. Although we have not identified any direct evidence that more rapid cardioversion translates to a shorter hospital stay, this is a reasonable inference. We therefore modelled this effect by applying an additional cost for patients not converted to sinus rhythm by a given time, to reflect the likelihood of an extra bed-day.
In addition to the efficacy and speed of cardioversion, the risk of adverse events is a major factor that influences clinicians’ choice of drugs for PCV. In particular, with antiarrhythmic therapy there is the risk of proarrythmia, where the treatment itself can precipitate the onset of a new arrhythmia, including bradycardia, tachycardia or prolongation of the QRS or QT interval. As with any drugs, AADs may be associated with a range of other adverse events, including headache, nausea, dizziness and ocular disturbances. Though most adverse events have no long-term consequences, they can be unpleasant and are potentially harmful. As is often the case, modelling of adverse events is difficult because of the wide variety of types and severity of events associated with the rhythm control drugs. Data on adverse events is also sparse and difficult to collate due to variations in how it is reported. Nevertheless, it is important that they can be included in evaluations of AADs, both in the context of short-term use for PCV (as in this topic) and in ongoing treatment (as in the following two topics). In the illustrative analysis presented below, we included an additional cost to reflect a longer length of stay for patients experiencing a complication during PCV. We did not include any QALY loss for complications, as none of the complications observed in the identified trials had any lasting effect.
We are not aware of any evidence to suggest that the choice of drug for PCV would influence the risk of recurrence in patients successfully converted to sinus rhythm, and so did not include this in our illustrative evaluation on this question. CG36 did present evidence that concomitant use of AADs alongside ECV can reduce the rate of relapse to AF (tables 5.5 and 5.6, p. 42). 135 It would be easy to incorporate any such impacts by applying appropriate relative risks to the early and late recurrence rates following successful cardioversion.
Sources of data
The guideline recommendations on the choice of PCV drug were informed by a meta-analysis 190 comparing amiodarone, class 1c drugs (propafenone or flecainide) and placebo. This review concluded that the class 1c drugs were more effective than amiodarone at achieving sinus rhythm by 8 hours (63% vs. 42%; p < 0.001), but that there was no significant difference by 24 hours (71% vs. 66%; p = 0.50). We assumed that after 8 hours, one extra bed-day would be incurred at a cost of £338 [the excess bed-day cost for an elective inpatient stay for Healthcare Resource Group (HRG) EB07I 109 ]. For comparison, a randomised trial of cardioversion 196 reported a mean length of stay of 1 day (SD = 2) for 72 patients randomised to an initial PCV strategy, and 2 days (SD = 2) for 67 randomised to initial ECV.
The review by Chevalier and colleagues 190 also considered reports of adverse events, but found insufficient data to quantify the risks. Another review 191 conducted by the manufacturer of the new drug vernakalant, collated safety data from 22 trials of a range of PCV drugs, including amiodarone, class 1c drugs, as well as vernakalant. They reported an overall adverse event rate within 2 hours of 40% (188 of 472 patients), and a serious adverse event rate of 4% (25 of 637). Four deaths were reported during this initial period and a further eight after 24 hours, although it was reported that none of these deaths were related to treatment. There were insufficient data to estimate relative adverse event rates for different drugs. For the PCV evaluation, we assumed that 4% of patients undergoing PCV would experience a serious complication, incurring an additional 1.78 days in hospital at a cost of £338 per day (total cost £602 per complication). This estimate is based on the difference in average length of stay for elective inpatient episodes for arrhythmia or conduction disorders (EB07) for patients with/without complications, and the cost of an excess bed-day for these patients. 109 For simplicity we assumed that other more minor side effects would not have any significant health or resource impact. A relative risk parameter was added to the model to allow sensitivity analysis of the impact of a difference in the percentage of patients experiencing complications with different drugs.
We ran the model twice, once assuming that patients without SHD undergoing PCV would receive an intravenous class 1c drug, and once assuming that they would receive intravenous amiodarone. The difference in NB between these strategies illustrates the potential for gain from identifying faster acting PCV drugs for use in this context.
Topic C: rhythm versus rate control for persistent atrial fibrillation
The choice of treatment strategy for patients with persistent AF is controversial. There is no clear evidence that patients with persistent AF benefit from attempts at regaining and maintaining sinus rhythm through cardioversion and use of AADs, or whether or not they would achieve better outcomes by moving straight to a rate control strategy. CG36 reported that no study had demonstrated rhythm control to be superior to rate control (or vice versa) for the outcomes of mortality or quality of life, although the GDG concluded that there was ‘generally consistent’ evidence that rates of adverse events and hospital admissions were higher with a rhythm control strategy. The GDG also considered subgroup analyses of the Atrial Fibrillation Follow-up Investigation of Rhythm Management (AFFIRM) trial, 193 which identified some factors associated with a lower risk of death with rate control. More recent Cochrane reviews 197 , 198 have reached similar overall conclusions.
The GDG highlighted difficulties in interpreting the evidence base in this field because of confounding with antithrombotic therapy. Trials comparing rate and rhythm control suffered from an imbalance between the arms in the proportion of patients treated with an OAC, as patients allocated to rhythm control were often withdrawn from OAC treatment if they remained in sinus rhythm. The use of composite outcome measures makes it difficult to tease out the effects of rate/rhythm control from the effects of antithrombotic therapy. The primary outcome for most rate versus rhythm trials has been all-cause mortality, which includes deaths from thrombotic, haemorrhagic and arrhythmic events. Rates of hospitalisation are also confounded, since they often included admissions for cardioversion, as well as for treatment of adverse events and treatment-related side effects.
Given this equivocal evidence, CG36 recommended that the choice of strategy should be tailored for individual patients, and suggested criteria to guide this choice. The criteria for initial rate control were age (≥ 65 years), coronary artery disease, absence of congestive heart failure (CHF), and suitability for cardioversion and AADs. Additional criteria for rhythm control were symptomatic AF, first presentation of lone AF, and AF secondary to a treated/corrected precipitant.
The review of CG36 195 identified some evidence that could potentially be used to revise criteria for rate versus rhythm control in defined patient groups:
- An analysis of data from the Rate Control versus Electrical cardioversion for atrial fibrillation (RACE) trial 199 reported that hypertensive patients randomised to rhythm control were at greater risk of cardiovascular morbidity and mortality than those randomised to rate control. This difference was not seen for non-hypertensive patients.
- The AF-CHF trial 200 reported that patients with heart failure randomised to rhythm control had a similar risk of death from cardiovascular causes compared with those randomised to rate control.
- The VALsartan In Acute myocardial iNfarcTion (VALIANT) study 201 compared rate or rhythm control strategies in patients following a MI. This found an excess mortality associated with AADs over the first 45 days, but no increased mortality after this initial period.
It is not possible to use the direct rate versus rhythm evidence in the MAPGuide model, because of the problems of confounding with OAC treatment and composite outcomes. The model design separates non-AF-related mortality, which is defined at model entry and independent of interventions and events in the pathway, and AF-related mortality, which is defined as a case-fatality rate, consequent on the occurrence of certain events (acute arrhythmia, TE or bleeding), as shown in Figure 18 . The model is therefore incompatible with data on all-cause mortality effects. Similar problems apply to data on rates of hospitalisation when it is not possible to separate the reasons for admissions. This problem applies to the subgroup analyses highlighted in the guideline review, as well as to the overall comparison of rate versus rhythm control.
There are two mechanisms through which rhythm and rate control interventions impact on health outcomes in the model: through acute arrhythmic episodes that can be fatal and through the reduced quality of life associated with uncontrolled AF (lack of sinus rhythm or heart rate > 80 b.p.m.). It is straightforward to use these existing modelled mechanisms to compare the overall cost and QALY impact of directing all patients with persistent AF to rhythm control or to rate control. The results of this analysis are reported below. However, this analysis suffers from some important limitations, particularly the rather limited incorporation of adverse drug effects within the current base-case model. These issues are addressed in the Discussion section below.
Topic D: antiarrhythmic drugs
CG36 136 recommended an escalating sequence of drugs, starting treatment with a standard BB. In patients without SHD for whom a BB is ineffective, contraindicated or not tolerated, the GDG recommended use of a class 1c agent or sotalol. For patients with SHD, or in patients for whom other treatment options have failed, amiodarone was recommended. This sequence was largely based on concerns over adverse effects, rather than on efficacy or cost. Evidence suggests that amiodarone is the most effective drug for maintaining sinus rhythm, but it is associated with some potentially serious adverse effects, including pulmonary, hepatic, ophthalmic and thyroid toxicity. The guideline review 195 highlighted recent evidence relating to new and existing antiarrhythmic agents. New treatments included dronedarone, which had been the subject of a NICE TA, 150 angiotensin-converting enzyme inhibitors and angiotensin receptor blockers (ARBs), and rosuvastatin (Crestor ® , AstraZeneca).
Similar issues arise for the evaluation of particular AADs as for the comparison of rate with rhythm control strategies discussed above. In particular, adverse effects are expected to have an important influence on the choice of AAD. However, although the current version of the base-case model includes treatment withdrawals, it does not account for any significant or lasting costs or health impacts for adverse effects. We therefore consider that the base-case version of the model is not a suitable platform for evaluation of this topic. Adaptation of the model to incorporate these effects is possible (see Discussion ), but there was insufficient time in this project to make the necessary changes. Consequently, we did not attempt to use the model to evaluate this topic.
Topic E: risk factor scoring systems for stroke and embolism risk
CG36 135 includes a SR stratification algorithm that prioritises patients for oral anticoagulation. It groups patients into high-, medium- and low-risk groups on the basis of their age and history of ischaemic stroke/TIA or other thromboembolic event, hypertension, diabetes, vascular disease, valve disease, heart failure or LVD. This was based on a review of evidence on individual risk factors, and of existing risk stratification algorithms.
The guideline review 195 identified various new studies defining and testing different SR stratification schemes for patients with AF. The European Society of Cardiology (ESC) published its guideline on AF in 2010. 134 This recommended the CHADS 2 scheme as a simple initial method for assessing SR, with patients scoring ≥ 2 recommended for oral anticoagulation. For patients with a CHADS 2 score of 0 or 1, they recommend more detailed assessment with the CHA 2 DS 2 -VASc scoring system.
The base-case model incorporated CHA 2 DS 2 -VASc as the means of stratifying stroke and TE risk, and applied the NICE criteria as the means of identifying patients suitable for OACs. The model also included calculation of CHADS 2 scores in order that they could be used for comparison. It is therefore straightforward to compare these different scoring systems, and to compare different thresholds for prescription of OACs.
Topic F: stratification tools to assess bleeding risk
The review group also considered systems for assessing patients’ risk of bleeding, which could be used to identify patients who would not be suitable for OACs. CG36 136 listed criteria for assessing the risk of bleeding including age, use of antiplatelet drugs or NSAIDs, multiple other drugs treatments, uncontrolled hypertension, history of bleeding or poorly controlled anticoagulation therapy. However, recommendations about how these factors should be combined or evaluated were unclear. Since publication of the NICE guideline, more formal systems for assessing individuals’ risk of bleeding have been developed, including the HAS-BLED scoring system, which was used in the model.
As with SR assessment, it is straightforward to use the model to evaluate the application of HAS-BLED thresholds to limit the use of oral anticoagulation in patients at high risk of a bleed.
Topic G: anticoagulant drugs
Since publication of CG36, two new OACs have been recommended by NICE TAs: dabigatran (TA249) issued in March 2012 146 and rivaroxaban (TA256) issued in May 2012. 147 Both drugs were recommended with certain restrictions. Rivaroxaban was recommended as a treatment option for AF without underlying heart valve disease and at least one of the following additional risk factors:
- high blood pressure
- aged ≥ 75 years
- or history of stroke or TIA.
Criteria for access to dabigatran were similar, non-valvular AF and at least one of the following:
- stroke, TIA or embolism in the past
- heart failure of class 2 or above
- aged ≥ 65 years with diabetes, coronary artery disease or high blood pressure.
The model already includes the above criteria, so the addition of dabigatran and rivaroxaban in line with NICE TA recommendations to the CG36 treatment pathway is straightforward, and does not require any additional data. The model makes certain assumptions about the proportion of patients receiving the different antithrombotic drugs when they are eligible for more than one drug (as illustrated in Table 24 ). In particular, it assumes equal use of aspirin and warfarin when both are recommended (e.g. for patients at medium risk), it assumes equal use of warfarin and of either dabigatran or rivaroxaban when all three are recommended, and it assumes equal use of rivaroxaban and dabigatran when both are appropriate. These criteria are easily changed, and a wide range of other strategies for the use of OACs could be tested within the current model structure.
We did not attempt to model apixaban for use as an OAC for AF, as the NICE TA on this indication 202 was not published until April 2013, after completion of our research.
Topic H: catheter ablation for paroxysmal and persistent atrial fibrillation
CG36 135 includes recommendations for the referral of patients for consideration for various interventional procedures after failure of medical therapies for rate or rhythm control. This included referral for pulmonary vein isolation, which includes catheter-based procedures. However, the guideline did not review evidence for the clinical effectiveness or cost-effectiveness of any specialist invasive procedures, or make recommendations for their use per se.
The guideline review identified new evidence relating to a range of ablation techniques, indicating an increased interest in this approach. Ablation is potentially curative of AF, although it is associated with a range of complications, including cardiac tamponade, pulmonary stenosis and thrombotic and haemorrhagic events. The HTA systematic review and CEA 152 concluded that radio frequency catheter ablation is more effective than AAD therapy in patients with refractory paroxysmal AF over a period of up to 12 months. However, evidence beyond 12 months is lacking, as is evidence relating to use of the technique in patients with persistent or permanent AF. The HTA economic analysis found that treatment would be cost-effective if quality-of-life improvements are maintained over the remaining lifetime of the patient, but that cost-effectiveness is unclear if the benefits are only maintained for 5 years. Other uncertainties relate to the effect of ablation on the risk of TE.
The costs and outcomes of ablation were not included in the base-case model – as this was outside the original scope of CG36. Adaptation of the model to evaluate this procedure would be relatively straightforward, and would involve the addition of a pathway for selection of individuals for treatment, inclusion of the costs of treatment and follow-up, the costs and QALY impacts of complications, and adaptation of the AF recurrence rate calculations. As noted above, there might be some difficulty in identifying evidence of the longer-term impact of ablation on AF recurrence and TE risk.
Base-case scenario: deterministic results
Results for 10,000 patients sampled from the THIN AF cohort are shown in Table 40 and in Figures 22 – 26 . At diagnosis, this sample had an average age of 74 years, a mean CHA 2 DS 2 -VASc score of 3.3 and a mean HAS-BLED score of 2.3. The health outcomes and costs shown are the results of one run of the base-case simulation model, following the CG36 recommendations and using point estimates for all model parameters. These illustrate the magnitude and range of key outputs under the base-case scenario. Mean predicted survival was 11 years, with a range from 0 to 66 years. Adjusting for utility during this time (mean 0.64), resulted in an estimated mean lifetime accumulation of around 7 QALYs (undiscounted). Over their lifetime, the simulated patients experienced a mean 0.31 thromboembolic events and 0.79 haemorrhagic events. Their AF treatment included a mean of 0.36 attempts at cardioversion, 0.16 acute admissions, and 2.68 tertiary consultations. The model predicted wide variations in costs, with the overall lifetime cost of AF-related care amounting to a mean of £28,230 per patient, rising to a maximum of £678,741. The cost of medication made up around 21% of this total cost. On an annual basis, the mean cost of medications was £538 per patient and the mean cost of other health care was £1856 per patient (including costs for AF admissions and consultations, and treatment and care following AF-related adverse events).
Results for 10,000 patients: base-case model, deterministic
Age at diagnosis: 10,000 patients from THIN AF cohort.
Simulated health-care costs: 10,000 patients from THIN AF cohort.
Simulated life-years: 10,000 patients from THIN AF cohort.
Simulated QALYs: 10,000 patients from THIN AF cohort.
Simulated medication costs: 10,000 patients from THIN AF cohort.
To inform the decision over the number of patients to include per iteration in the probabilistic sensitivity analysis, we estimated the cumulative means of key output parameters for increasing numbers of patients. Figure 27 shows how the volatility of the estimated NB per patient decreases as the number of patients is increased to 10,000. The estimate is reasonably stable at 1000 patients and there is very little change in the estimate above 2000 patients. For the analyses below, we used 1000 patients per probabilistic iteration to limit the runtime of the model, as we wanted to compare a large number of scenarios.
Cumulative NB per patient with increasing sample size.
Base-case scenario: probabilistic results
Table 41 compares the deterministic and probabilistic results for the base-case scenario. With 500 probabilistic iterations and 1000 patients per iteration, the results of the probabilistic and deterministic analyses were quite similar. The probabilistic sensitivity analysis produced an estimate of 5.22 QALYs per patient (discounted), compared with 5.20 QALYs from the deterministic analysis. There was rather more of a difference in estimated costs (£21,048 from the probabilistic analysis, compared with £19,494 from the deterministic analysis). This is not unexpected, as a large proportion of costs in the model related to treatment of relatively rare but expensive events (mainly ischaemic and haemorrhagic strokes). Overall NBs were similar when estimated from the probabilistic and deterministic analyses: £83,441 compared with £84,497 respectively (assuming a cost-effectiveness threshold of £20,000 per QALY).
Base-case model results (per 1000 incident cases)
The number of probabilistic iterations used for the analyses presented below was based on observation of the volatility of key output estimates with increasing numbers of iterations. Figure 28 shows how the expected NB per patient changed as the number of probabilistic iterations was increased from 0 to 500 for three illustrative strategies for antithrombotic therapy. It can be seen that the expected NB per patient is lowest with no antithrombotic treatment, the base-case NICE strategy gives the next highest expected NB, and prescription of aspirin for all patients gives the highest expected NB. The ranking and relative differences between these strategies are very stable after only 100 probabilistic iterations. Unnecessary variation between strategies was removed by the following procedures:
Cumulative NB by probabilistic iteration (1000 patients per iteration).
- For each probabilistic iteration, the same patient sample was used across all of the scenarios compared. Thus the set of patients used in probabilistic loop n for the base-case scenario, was the same as for probabilistic loop n for the no antithrombotic and aspirin scenarios. For probabilistic loop n + 1, the patient sample was different from that in loop n , but again the same across all three scenarios.
- Similarly, the n ’th probabilistic iteration used the same set of values for all of the population parameters that did not differ between the scenarios. So, for example, the cost of treating a TE was the same for the n ’th probabilistic loop under the base-case scenario and for the no thromboprophylaxis and aspirin scenarios. The only parameters that differed between the scenarios for a given iteration were the cost of the antithrombotic treatment and the relative risk reductions on rates of TE and bleeds.
The analyses reported below used 500 probabilistic iterations with 1000 patients per iteration.
Topic B: pharmacological cardioversion for patients without structural heart disease
The results of the analysis comparing amiodarone with class 1c drugs for PCV in patients without SHD is shown in Table 42 . These suggest that the current guideline recommendation (class 1c drugs) is likely to be more cost-effective than amiodarone. Amiodarone is dominated as it gives a higher expected cost (£10 per patient) and fewer expected QALYs (–0.0018 per patient). At a cost-effectiveness threshold of £20,000 per QALY, this gave an expected INB of £46 more per patient with class 1c drugs than with amiodarone. However, there is a high degree of uncertainty over this result. The estimated probability that class 1c drugs are more cost-effective than amiodarone is 57% at a cost-effectiveness threshold of £20,000 per QALY. The results were very similar at a cost-effectiveness threshold of £30,000 per QALY. Sensitivity analysis over the relative incidence of complications also made very little difference to these results.
Model results: PCV drugs for patients without SHD (per 1000 patients)
Topic C: rate versus rhythm control for patients with persistent atrial fibrillation
The results for the illustrative comparison of rate and rhythm control strategies for patients with persistent AF are shown in Table 43 .
Model results: rate vs. rhythm control for persistent AF (per 1000 incident cases)
These suggest that referring all patients with persistent AF straight to rate control dominates both the current guideline recommendations and the strategy of rhythm control. Overall, the model estimates that rate control would save £256 and yield an additional 0.017 life-years and 0.133 QALYs on average per patient. The estimated differences in NB between the strategies are large. For example, rate control gives an expected INB of £2908 per patient compared with the base case at a cost-effectiveness threshold of £20,000 per QALY. This estimate increases to £4223 at £30,000 per QALY. The model also suggests that there is a high degree of certainty over this result: in all 500 probabilistic iterations, rate control was more cost-effective than either of the other strategies. However, as noted above, it is not clear that this analysis properly accounts for the full range of adverse effects associated with both rate and rhythm control drugs. Further analysis is required before conclusions should be drawn regarding the relative clinical effectiveness or cost-effectiveness of rate and rhythm control.
Topic E: thromboembolism risk thresholds for oral anticoagulation
The results of the comparison of CHA 2 DS 2 -VASc thresholds for warfarin are shown in Table 44 . For this analysis, we assumed that patients would receive warfarin if their CHA 2 DS 2 -VASc score was greater than or equal to a defined threshold X and aspirin if their CHA 2 DS 2 -VASc score was less than X . With X = 0, all patients would be prescribed warfarin. As X is increased fewer patients are prescribed warfarin, until with X = 10 all patients are prescribed aspirin. The model assumes that patients who have a bleed while on warfarin will switch to aspirin. All other model parameters are held at their base-case values. The results presented are means across 500 probabilistic iterations, each including 1000 patients.
Model results: CHA 2 DS 2 -VASc thresholds for warfarin (per 1000 incident cases)
It can be seen that as the threshold for warfarin is increased (restricting use of anticoagulation), the mean number of thromboembolic events rises, and the mean number of bleeds falls. Both life-years and QALYs are at a maximum when all patients are prescribed aspirin. This is because of the relatively high health loss associated with bleeds. However, costs are at a minimum with a CHA 2 DS 2 -VASc threshold of 5, and at a cost-effectiveness threshold of £20,000 per QALY gained, NBs reach a maximum at a CHA 2 DS 2 -VASc threshold of 6.
The results of a similar analysis using CHADS 2 thresholds for warfarin are shown in Table 45 . Here the maximum NB is reached at a CHADS 2 threshold of 4.
Model results: CHADS 2 thresholds for warfarin (per 1000 incident cases)
The above results are illustrated in Figure 29 . This shows estimated costs and QALYs relative to the base-case results. As the CHADS 2 and CHA 2 DS 2 -VASc risk thresholds are increased, the estimated QALY gain increases, and the estimated cost-effectiveness point moves to the right. This graph shows the similarity of results based on CHA 2 DS 2 -VASc and on the simpler CHADS 2 scoring system.
Costs and effects for CHADS 2 and CHA 2 DS 2 -VASc thresholds for warfarin.
Topic F: bleeding risk thresholds for oral anticoagulation
Results for the HAS-BLED threshold analysis are shown in Table 46 . This analysis assumes that a patient would receive warfarin only if their HAS-BLED score was less than a defined threshold Y and aspirin otherwise. As in the preceding analyses, we assumed that patients who have a bleed while on warfarin will switch to aspirin, and all other model parameters were held at their base-case values. The figures are presented as mean values across 500 probabilistic iterations, each including 1000 patients.
Model results: HAS-BLED thresholds for warfarin (per 1000 incident cases)
As the HAS-BLED threshold is reduced, fewer patients receive warfarin and the mean rate of TEs increases, whereas the mean rate of bleeding declines. Health outcomes (life-years and QALYs) are at a maximum and costs are at a minimum when the bleeding risk threshold is set so that no patients receive warfarin (all receive aspirin).
Topic G: choice of oral anticoagulation drugs
For this analysis ( Table 47 ), we changed the oral anticoagulation drugs available within the modelled treatment pathway. Strategy G0 is the base-case analysis, which includes CG36 criteria for allocation of patients to aspirin or warfarin, as well as TA249 criteria for access to dabigatran and TA256 for access to rivaroxaban. G1 excludes dabigatran and rivaroxaban from the treatment options.
Model results: comparison of OAC drugs (per 1000 incident cases)
Estimated health outcomes are rather better with the addition of the new drugs (with fewer thromboembolic events and bleeds and more life-years and QALYs for G0 compared with G1). However, as might be expected, costs are increased with dabigatran and rivaroxaban. At a cost-effectiveness threshold of £20,000 per QALY, this results in a very similar expected NB for these two strategies and the estimated probability that G0 is more cost-effective than G1 is only 45%. However, with NICE’s upper threshold for cost-effectiveness (£30,000 per QALY), the expected NB is higher with dabigatran and rivaroxaban: £135,685 for G0 and £128,712 for G1 (51% probability that G0 is more cost-effective than G1).
- Interactions: risk thresholds and drugs for oral anticoagulation
The model results for simultaneous changes in thresholds for stroke (CHA 2 DS 2 -VASc) and bleeding (HAS-BLED) for allocation of patients to warfarin or aspirin are shown in Table 48 . The optimum health outcome (QALY maximum) occurs with a CHA 2 DS 2 -VASc threshold of ≥ 2 combined with a HAS-BLED threshold of < 1 for warfarin. However, the greatest cost savings are attained with a CHA 2 DS 2 -VASc threshold of ≥ 5 and no HAS-BLED threshold. Overall, the model predicts a maximum INB compared with the base case of £2510 per patient (at £20,000 per QALY), which is achieved with a CHA 2 DS 2 -VASc threshold of ≥ 6, with no HAS-BLED threshold. This finding contrasts with the separate analyses of the HAS-BLED threshold shown above in Table 46 , which suggests that a very high HAS-BLED threshold would be optimum in the absence of a threshold for thromboembolic risk.
Model results: HAS-BLED and CHA 2 DS 2 -VASc thresholds for warfarin
The results for a similar analysis using CHADS 2 as the stratification system for stroke and thromboembolic risk are shown in Table 49 . It can be seen that the pattern of results is very similar with CHADS 2 and CHA 2 DS 2 -VASc, and that the optimum INB is actually greater with CHADS 2 than with CHA 2 DS 2 -VASc (£2538). This suggests that the simple CHADS 2 risk scoring system is at least as good, if not better, than the more complex alternative, CHA 2 DS 2 -VASc.
Model results: HAS-BLED and CHADS 2 thresholds for warfarin
Tables 50 and 51 show estimated QALYs, costs and NBs by CHADS 2 and HAS-BLED thresholds for selection of dabigatran and rivaroxaban respectively. These matrices illustrate how the optimal treatment thresholds can change with the anticoagulation drug: CHADS 2 ≥ 4 and HAS-BLED< 4 for warfarin; CHADS 2 ≥ 4 with no HAS-BLED threshold for dabigatran; and CHADS 2 ≥ 3 and HAS-BLED < 2 for rivaroxaban. As might be expected, there is a high level of interaction between the choice of antithrombotic drug, and the thromboembolic and bleeding risk thresholds for treatment.
Model results: HAS-BLED and CHADS 2 thresholds for dabigatran
Model results: HAS-BLED and CHADS 2 thresholds for rivaroxaban
Scope of the model
This chapter has presented the methods and results of a DES model developed to estimate the costs and health effects associated with the main processes of care for people with AF: diagnosis, cardioversion, antithrombotic therapy, rate and rhythm control, and ongoing monitoring. The model covered most of the service pathway in the NICE CG on AF, although we did not attempt to include the prevention and treatment of post-operative AF, which is a rather separate clinical question. The model does not currently include tests or interventional procedures for patients with structural heart defects, or for people with AF refractory to medical treatment (e.g. ablation or implantable devices), though these were also excluded from the scope of the original NICE guideline. As an individual-level simulation, the MAPGuide AF model reflects heterogeneity in the patient population. It contains rich information about correlated risk factors and retains information about individuals’ history as they pass through the modelled pathway, which allows greater flexibility and realism in representing variations between patients.
Overall, the model benefits from the availability of strong data sources to inform many of its key parameters. The THIN database provided individual-level data on demographic and clinical risk factors for over 12,000 individuals close to the time of their AF diagnosis. These data were derived from routine primary care data and are broadly representative of the UK population. However, there may be flaws in the recording of information in the database, as this was collected for primary patient management purposes rather than for research. We also had to make a number of assumptions to associate the recorded data with the risk factors that we required for the model.
Estimates of the rates of TE and bleeding were taken from a large population cohort study. 158 The applicability of these Swedish data to the UK AF population is open to question. However, as the data used in the model were stratified by risk score (CHA 2 DS 2 -VASc and HAS-BLED) and adjusted for estimated treatment effects of antithrombotic therapy, this would have corrected to some extent for national differences in the distribution of risk factors and differences in treatment. Other key inputs to the model were estimates of the effects of antithrombotic and antiarrhythmic therapy, which were drawn from network meta-analyses conducted to inform recent NICE TAs. 145 , 179 These analyses provide coherent estimates of the relative impacts of the available medications in these two key areas of AF treatment, although it should be noted that they do not necessarily reflect all current evidence as we have not updated the reviews on which they were based. The cost estimates for the various interventions and outcomes along the pathway were based on standard UK sources, 109 – 111 and estimates of the costs of stroke in people with AF were also supported by an unusually large and well-conducted UK population-based study. 185 The utility data underlying the QALY estimates may also be seen as a strength, as they came from large sample surveys using the EQ-5D instrument: starting with UK population utilities, 187 with adjustment for AF status from an international cohort study of patients with AF, 188 and adjustment for adverse events from a US panel of patients with a range of chronic conditions. 189 Although their applicability to a UK population can be questioned, 203 these sources provided consistent estimates of the utility impacts of AF control, and of the AF-related adverse effects included in the model.
There are, however, some weaknesses in the quality of data in some other areas (most notably around the accuracy of diagnostic tests for AF, the effectiveness of different methods of cardioversion and of rate control medications). Another potentially important weakness is the sparse data on the case fatality rates for haemorrhagic strokes and other major bleeds. The high estimated rate of mortality from bleeds compared with mortality from TEs (21% vs. 11%) in the model, impacts on the relative cost-effectiveness of anticoagulant drugs. The analysis would be strengthened by a more robust source of data on the case-fatality rates associated with the modelled events.
Modelling antithrombotic treatment strategies
The model provided a good foundation for comparison of strategies for the prevention of stroke and TE. It provided stable and consistent estimates of costs and health effects for many strategies, including changes in SR and bleeding thresholds for anticoagulation, and the choice of anticoagulant medication. The structure of the model offers great flexibility to model a wide variety of strategies.
As mentioned above, although the data underlying the model are of a generally good quality, there are some model inputs in particular that require further consideration before the results should be used to inform treatment decisions. Essentially, the model weighs up the relative frequency and severity of thromboembolic and haemorrhagic events. One key factor in this equation is the mortality associated with these different events, and the case-fatality rate for haemorrhagic strokes was only based on a very small number of cases, so might not be reliable.
The model relies on two scoring systems to stratify individuals on the basis of the risk of TE (CHA 2 DS 2 -VASc) and bleeding (HAS-BLED). At the individual patient level, the predictive validity of these systems is limited. 168 However, alternative scoring systems or simple pragmatic rules (such as relying on age alone) are no more likely to be correct. Also across the population of people with AF, both CHA 2 DS 2 -VASc and HAS-BLED are strongly predictive of the related outcomes. They therefore represent the best available foundation for modelling treatment outcomes and cost-effectiveness of oral anticoagulation for people with AF.
Modelling rate versus rhythm
Adaptation of the base-case model to address questions related to the choice of rate or rhythm control strategy, and the choice of particular rhythm control drugs was less successful. The key problem was that the direct trial evidence on rate compared with rhythm control was incompatible with the model structure. This might be seen as a problem with the trials in this field, which were confounded with between-arm differences in rates of anticoagulant therapy and presented results using composite outcomes (such as all-cause mortality and hospitalisation) which were difficult to interpret. However, some choices made in designing the base-case model did limit our ability to capture all of the potentially important impacts of rate and rhythm control strategies, and some reprogramming would be required to produce a convincing evaluation of this topic.
First, the model does not currently allow for any independent effect of sinus rhythm on risk of TE. It is controversial whether or not any such effect exists, although it might appear logical that it should (if a cardiac arrhythmia increases the chance of the development of an embolism, reversion to sinus rhythm might be expected to reduce this chance). However, evidence for this hypothesis is sparse. The only evidence that we found came from a multivariate analysis of data from the AFFIRM trial. 194 This was a cox-proportional hazards regression of ‘on treatment’ data (i.e. not intention to treat). It corrected for a number of covariates, including patient characteristics (age, coronary artery disease, CHF, LVD, mitral valve disease, diabetes, prior stroke or TIA, and smoking) and concomitant treatments (warfarin, digoxin and AADs). The estimated hazard ratio for mortality with sinus rhythm was 0.53 (95% CI 0.39 to 0.72). This was of a similar magnitude to the hazard ratio for warfarin use (0.50, 95% CI 0.37 to 0.69), and use of AADs were associated with increased mortality (1.49, 95% CI 1.11 to 2.01). The authors concluded that one hypothesis that would explain these effects is that AADs have a beneficial effect on survival through maintenance of sinus rhythm, but that this benefit might be offset by their adverse effects. However, it is difficult to draw firm conclusions from this essentially observational study, as important confounders might have been omitted. It is also difficult for us to apply these data to the model, since the results are reported in terms of an impact on all-cause mortality. However, the model could easily be modified to allow a ‘what-if’ sensitivity analysis on this point, by adding a relative risk multiplier for the risk of TE.
A second limitation of our analysis on the rate versus rhythm question, relates to the way in which we modelled adverse treatment effects. The model includes treatment discontinuation rates for all drugs, but it does not differentiate discontinuation due to adverse effects, or attach any health consequences or treatment costs for adverse effects other than bleeds for antithrombotic therapy and acute-onset arrhythmias. This is appropriate for the majority of adverse effects that patients tolerate and for the more minor adverse effects that might prompt treatment withdrawal, as these will not have significant or lasting consequences. However, the omission of more serious adverse effects is a problem. This could be rectified with some relatively straightforward reprogramming, and data is available to inform this extension of the model. As always with adverse effect data, the large number of different types of effects that patients experience and differences in reporting present a challenge. However, data is available to estimate rates for three broad categories of adverse effects for the rate and rhythm control drugs: pro-arrhythmic events (new cardiac arrhythmias potentially provoked by antiarrhythmic therapy), other serious adverse events and minor adverse events. 179 , 192 Estimates of the QALY impacts and costs associated with these types of adverse events are also available from the economic analysis produced by Sanofi-Aventis for the NICE TA of dronedarone. 149 – 151
A third limitation of the use of the model to compare rate and rhythm control strategies is the weakness of evidence on the effectiveness of rate control drugs. The model currently uses data from five small randomised cross-over trials reported in CG36 (55 patients in total), meta-analysed using a simple inverse-variance method. An alternative source of data was identified during the search for evidence to inform the rate versus rhythm analysis. This comes from a post hoc analysis of AFFIRM trial data, 192 which estimated the effects of BBs, calcium-channel blockers and digoxin alone or in combination on the achievement of adequate ventricular rate control (defined as average heart rate ≤ 80 b.p.m., with additional criteria for maximum heart rate during exercise and on 24-hour ECG monitoring). Though observational, this data set is larger than that included in the model (361 patients), it directly compares single drug and combination therapies and it is presented alongside adverse effect rates. The model could be adapted to make use of this data set, and to provide a firmer footing for comparison of rate and rhythm control strategies.
Finally, the omission of interventional techniques from the end of the rhythm control pathway in the model might have biased the results.
Comparison across the potential update topics
In the time available, we succeeded in conducting analyses for five of the eight potential update topics.
A simple two-drug comparison for topic B supported the current recommendation for the use of class 1c drugs, rather than amiodarone, for PCV in patients without SHD. This finding was highly uncertain, and the estimated INB was relatively modest (< £50 per patient at a threshold of £20,000 per QALY). This is not unexpected, first because there is little evidence of any difference in effectiveness between these drugs (class 1c drugs may be faster acting than amiodarone, but overall success rates are similar). Second, this topic is also only relevant for a subset of patients.
The analysis for topic C was speculative, as we believe it might have omitted some important factors. Nevertheless, the findings do suggest that the balance between rate and rhythm control is potentially of high economic importance. The model suggested that an overall rate control strategy could be more effective and cost-effective than the current recommendation of selecting patients for rhythm control. The size of the estimated INB for rate control compared with the base-case strategy was £2908 per patient (at a threshold of £20,000 per QALY).
Taken together, the analyses of topics E and F suggest that there is also good potential for improving the cost-effectiveness of the guideline pathway by better targeting of anticoagulation therapy on the basis of stroke and bleeding risk scores. The model estimated that the optimum strategy for selecting patients for warfarin (CHADS 2 score ≥ 4 and HAS-BLED score < 4) would save money and improve patient outcomes, yielding an INB of about £2500 per patient compared with the current guideline recommendations at the £20,000 per QALY threshold.
In contrast, the model estimated more modest gains in cost-effectiveness from the use of newer OACs rather than warfarin. Adding NICE TA recommendations for the use of dabigatran and rivaroxaban to the CG36 recommendations for warfarin made no difference to the overall NB at NICE’s lower cost-effectiveness threshold of £20,000 per QALY. Even at the upper threshold of £30,000 per QALY, a small incremental gain in NB of £358 per patient was estimated.
The above analyses suggest that of the topics analysed, the targeting of rhythm control therapy and of oral anticoagulation are the highest economic priorities for inclusion in an update of the guideline, as they both show the potential for significant improvement in the NB of the treatment pathway. The choice of drugs for oral anticoagulation and for PCV of patients without SHD are relatively lower priorities from an economic perspective, as they appear to add less to overall NBs.
Included under terms of UK Non-commercial Government License .
- Cite this Page Lord J, Willis S, Eatock J, et al. Economic modelling of diagnostic and treatment pathways in National Institute for Health and Care Excellence clinical guidelines: the Modelling Algorithm Pathways in Guidelines (MAPGuide) project. Southampton (UK): NIHR Journals Library; 2013 Dec. (Health Technology Assessment, No. 17.58.) Chapter 5, Case study 2: full guideline model for atrial fibrillation.
- PDF version of this title (5.9M)
In this Page
Other titles in this collection.
- Health Technology Assessment
Recent Activity
- Case study 2: full guideline model for atrial fibrillation - Economic modelling ... Case study 2: full guideline model for atrial fibrillation - Economic modelling of diagnostic and treatment pathways in National Institute for Health and Care Excellence clinical guidelines: the Modelling Algorithm Pathways in Guidelines (MAPGuide) project
Your browsing activity is empty.
Activity recording is turned off.
Turn recording back on
Connect with NLM
National Library of Medicine 8600 Rockville Pike Bethesda, MD 20894
Web Policies FOIA HHS Vulnerability Disclosure
Help Accessibility Careers
- Open access
- Published: 04 May 2024
Factors associated with non-fatal heart failure and atrial fibrillation or flutter within the first 30 days post COPD exacerbation: a nested case-control study
- Emily L. Graul 1 ,
- Clementine Nordon 2 ,
- Kirsty Rhodes 2 ,
- Shruti Menon 3 ,
- Mahmoud Al Ammouri 1 ,
- Constantinos Kallis 1 ,
- Anne E. Ioannides 1 ,
- Hannah R. Whittaker 1 ,
- Nicholas S. Peters 4 &
- Jennifer K. Quint 1
BMC Pulmonary Medicine volume 24 , Article number: 221 ( 2024 ) Cite this article
467 Accesses
1 Altmetric
Metrics details
An immediate, temporal risk of heart failure and arrhythmias after a Chronic Obstructive Pulmonary Disease (COPD) exacerbation has been demonstrated, particularly in the first month post-exacerbation. However, the clinical profile of patients who develop heart failure (HF) or atrial fibrillation/flutter (AF) following exacerbation is unclear. Therefore we examined factors associated with people being hospitalized for HF or AF, respectively, following a COPD exacerbation.
We conducted two nested case-control studies, using primary care electronic healthcare records from the Clinical Practice Research Datalink Aurum linked to Hospital Episode Statistics, Office for National Statistics for mortality, and socioeconomic data (2014-2020). Cases had hospitalization for HF or AF within 30 days of a COPD exacerbation, with controls matched by GP practice (HF 2:1;AF 3:1). We used conditional logistic regression to explore demographic and clinical factors associated with HF and AF hospitalization.
Odds of HF hospitalization (1,569 cases, 3,138 controls) increased with age, type II diabetes, obesity, HF and arrhythmia history, exacerbation severity (hospitalization), most cardiovascular medications, GOLD airflow obstruction, MRC dyspnea score, and chronic kidney disease. Strongest associations were for severe exacerbations (adjusted odds ratio (aOR)=6.25, 95%CI 5.10-7.66), prior HF (aOR=2.57, 95%CI 1.73-3.83), age≥80 years (aOR=2.41, 95%CI 1.88-3.09), and prior diuretics prescription (aOR=2.81, 95%CI 2.29-3.45).
Odds of AF hospitalization (841 cases, 2,523 controls) increased with age, male sex, severe exacerbation, arrhythmia and pulmonary hypertension history and most cardiovascular medications. Strongest associations were for severe exacerbations (aOR=5.78, 95%CI 4.45-7.50), age≥80 years (aOR=3.15, 95%CI 2.26-4.40), arrhythmia (aOR=3.55, 95%CI 2.53-4.98), pulmonary hypertension (aOR=3.05, 95%CI 1.21-7.68), and prescription of anticoagulants (aOR=3.81, 95%CI 2.57-5.64), positive inotropes (aOR=2.29, 95%CI 1.41-3.74) and anti-arrhythmic drugs (aOR=2.14, 95%CI 1.10-4.15).
Conclusions
Cardiopulmonary factors were associated with hospitalization for HF in the 30 days following a COPD exacerbation, while only cardiovascular-related factors and exacerbation severity were associated with AF hospitalization. Understanding factors will help target people for prevention.
Peer Review reports
Exacerbations of Chronic Obstructive Pulmonary Disease (COPD) are associated with an increased risk of cardiovascular disease (CVD) [ 1 ] likely due to linked pathophysiology, hypoxic state, and an amplified systemic inflammatory response [ 2 , 3 ]. Previous studies have demonstrated an increased, immediate period of risk for hospitalized cardiovascular events following a COPD exacerbation compared with non-exacerbating patients, [ 1 , 4 ] with the risk highest within the first month and following a severe (hospitalized) exacerbation [ 1 , 5 , 6 ]. The 30 day risk of arrhythmias and heart failure (HF) following an exacerbation, [ 6 , 7 , 8 ] approach [ 4 , 6 ] or even surpass [ 7 , 9 ] 3-fold, compared to those who did not have an exacerbation.
Globally, the prevalence of HF in COPD is high, [ 10 ] and, of patients hospitalized for exacerbation, 20% have existing, undertreated HF [ 11 ]. Incident HF attributed to exacerbations is thought to come from the increase in pulmonary arterial pressures, low blood oxygen levels [ 12 ] and activation of adrenoceptors of the autonomic nervous system [ 12 , 13 ]. However, shared symptomology of HF and COPD exacerbations makes new HF diagnosis difficult, with HF often missed [ 3 , 12 , 14 ] or occurring in tandem; approximately 8% of people primarily diagnosed with HF also have a secondary diagnosis of exacerbation [ 15 ]. Studies have investigated COPD progression in HF [ 12 ] and conversely, HF progression across COPD phenotypes, [ 12 , 16 ] but few have focused on exacerbating COPD alone [ 15 ] and no studies have examined factors associated with hospitalization with a HF diagnosis following a COPD exacerbation.
Arrhythmias are also common among people with COPD, with prevalence ranging from 5 to 15% globally, [ 10 ] and atrial fibrillation (AF) is the most common arrhythmia [ 17 ]. AF diagnoses at the time of an exacerbation are also common, with estimates around 17% [ 18 ], but due to shared, nonspecific symptoms between AF and COPD, differential diagnosis can be difficult [ 19 ]. During a COPD exacerbation, changes in blood gases from hypoxia and rising pulmonary pressure can lead to electrophysiological and structural changes of the atrium, and together are thought to contribute to exacerbation-related disturbances in rhythm and progression of AF [ 6 , 10 , 19 , 20 , 21 ] The understanding of COPD progression alongside AF progression is unclear [ 19 ]. Few studies have examined factors associated with hospitalization for incident AF after an exacerbation, with the focus on the short-term [ 21 , 22 ]. Several have however investigated factors associated with concurrent AF at time of an exacerbation [ 18 , 23 ].
Therefore, using a primary-care defined COPD cohort from the Clinical Practice Research Datalink (CPRD) Aurum database linked with hospital, mortality, and socioeconomic deprivation data, we explored factors associated with incident hospitalization for non-fatal HF, or AF or atrial flutter, within the 30 days following a COPD exacerbation.
Study design and methods
Data source.
We obtained pseudonymized, routinely-collected electronic healthcare record data from CPRD Aurum, [ 24 ] a primary care database broadly representative by age, sex, deprivation, and regional distribution, [ 25 ] and covering approximately 20% of GP-registered patients in England [ 24 ]. Primary care data from the May 2022 build [ 24 ] were linked to the Office for National Statistics (ONS) for mortality, socioeconomic data from the 2015 Index of Multiple Deprivation (IMD), and secondary care data from NHS England’s Hospital Episode Statistics (HES) Admitted Patient Care (APC) database.
Source population
The source population were people with a COPD diagnosis who had been included in the Exacerbations of COPD and their OutcomeS on CardioVascular diseases (EXACOS-CV) observational open cohort study [ 4 ]. People were eligible for inclusion in that original cohort study if they met the following criteria: 1) aged 40 years or older, 2) had a COPD diagnosis using a validated algorithm (86.5% PPV) [ 26 ] 3) were eligible for linkage with HES, ONS, and IMD data, 4) had a smoking history (i.e., current or ex-smoker), 5) had continuous GP practice registration with acceptable quality data in the year before start of follow up, and 6) had data recorded after 1 st of January 2014.
Study design and population
We conducted two nested-case control studies, in parallel. The study population for each study consisted of COPD patients in the EXACOS-CV source population, who 1) experienced an exacerbation within cohort follow-up, 2) among the pool of eligible controls had at least 30 days of contributing data post-exacerbation, and 3) had no evidence of HF or arrhythmias in the year before exacerbation. People were excluded from the AF/flutter study population if, within the 30 days post-exacerbation, they had evidence of other non-AF arrhythmias (e.g., cardiac arrest) (Fig. 1 for study designs; Supplementary Figures 1 and 2 for HF and AF/flutter eligibility diagram respectively).
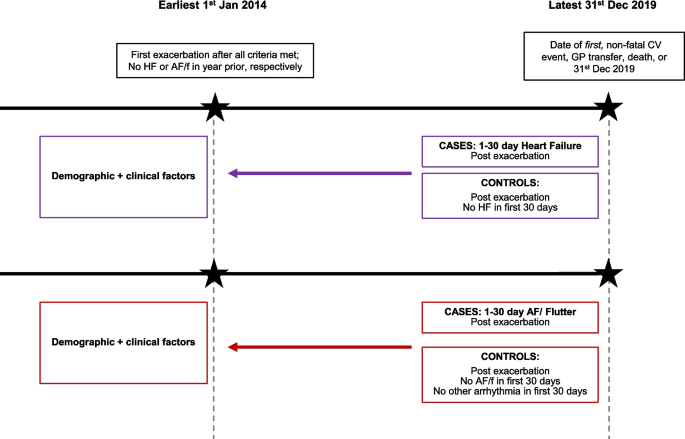
Study design for the parallel, nested case control studies. Abbreviations: HF=heart failure, AF/f = atrial fibrillation/flutter, GP=general practice, CV=cardiovascular. Two nested case control studies, for HF and AF/f respectively, were conducted among all patients who experienced an exacerbation at start of cohort follow up. Full definitions of demographic and clinical factors of interest are in the Supplementary materials
Cases were individuals with a hospitalized, cardiovascular event (HF or AF/flutter, respectively) within 1-30 days post COPD exacerbation. Cases were determined in the HES database using ICD-10 codes in the primary diagnostic position across all episodes in a single hospitalization. The date of admission was the date of the case (index date, HF or AF/flutter, respectively). Extended case definitions are in Supplementary Table 3 .
The set of controls per case was drawn among individuals in the study population who had no in-hospital diagnosis of the cardiovascular event-of-interest (HF or AF/flutter, respectively) and who had 30 days of contributing data post-exacerbation. Controls were assigned a pseudo-end date (i.e., 30 days post-exacerbation) on which to match. Controls were individually matched to cases on GP practice to account for unmeasurable potential clinical differences in disease management by clinicians, within a 30-day window of the index date, and could be used as a control for more than one case. For the HF analysis, controls were matched 2:1 and for the AF/flutter analysis, 3:1.
The choice of matching factors and ratios were chosen based on considerations of 1) maximizing the ratio itself for reasons of power and precision while 2) minimizing cases lost without a full set of controls. Only case sets with the full ratio of controls per case were included for analysis.
We examined factors potentially associated with hospitalization for HF or AF/flutter in the 30 days following an exacerbation of COPD. Factors included demographic characteristics: age (categorized into four age bands; 40-69, 70-74, 75-79, ≥80), sex, IMD quintiles, and ethnicity; and smoking status. Comorbidities included (hypertension, anxiety, depression and depressive symptoms, type II diabetes, chronic kidney disease (CKD), BMI [body mass index; using World Health Organization classification]). COPD factors included GOLD grade of airflow limitation (defined as 1 mild, 2 moderate, 3-4 severe/very severe), Medical Research Council (MRC) dyspnea score (1-2; 3; 4-5), exacerbation severity at cohort entry (moderate/severe) prior exacerbation frequency (infrequent (≤1) versus frequent (≥2) history in a year window preceding one year to cohort entry). Prior CVD history was evidence of the following anytime preceding the year before exacerbation date [acute coronary syndrome (ACS), arrhythmias, HF, ischemic stroke, pulmonary hypertension PH], COPD inhaled therapies, and major classes of cardiovascular medications (prescriptions defined two years before cohort entry). Covariate definitions are in Supplementary Table 4 .
We used validated definitions for COPD exacerbations. A moderate exacerbation was defined as a COPD-related primary care (GP) visit with either a code for exacerbation (including Lower Respiratory Tract Infection (LRTI) SNOMED-CT codes) and/or prescription for respiratory antibiotics and oral systemic corticosteroids not on the same day as an annual review, as validated in CPRD [ 27 ]. A severe exacerbation was defined as a hospitalization with an acute respiratory event code including COPD or bronchitis as a primary diagnosis, or a secondary diagnosis of COPD, as validated in HES [ 28 ]. We considered exacerbations to be the same event if recorded within 14 days in which case the highest level of severity was chosen.
We checked covariate missingness to assess use in adjusted models. Imputation was not considered for covariates with missing data, given the missingness mechanism was Missing-Not-At-Random (MNAR), violating the Missing-Completely-At-Random (MCAR) assumption premising imputation [ 29 ].
Codelists for primary care factors were generated using SNOMED-CT and British National Formulary ontologies; we used our standardizable, reproducible methodology, available on GitHub: for drug [ 30 ] and medical/phenotype codelists , respectively. Codelists for hospitalizations used ICD-10 codes. Codelists are available on our EXACOS-CV GitHub repository .
Statistical analysis
We compared separately the odds of hospitalization for HF or AF/flutter between the comparator groups for each factor, using conditional logistic regression. Our final model was adjusted for all covariates without substantial amount of missing data, including demographic factors, comorbidities, and COPD inhaled therapies and cardiovascular medications. In three sensitivity analyses, we repeated main analyses additionally adjusting for variables-of-interest with substantial missing data, respectively: GOLD, MRC, and CKD.
Analyses were performed using STATA v17.
These data were collected and provided by CPRD. Ethical approval was obtained through CPRD’s Research Data Governance Process (protocol number: 22_002377). The RECORD checklist for observational studies is in Supplementary Table 5 .
Characteristics of study participants
The HF dataset consisted of 1,569 cases experiencing an HF event within the first 30 days post-exacerbation, matched to 3,138 controls. The AF/flutter dataset consisted of 841 cases experiencing an AF/flutter event within the first 30 days post-exacerbation, matched to 2,523 controls. Tables 1 and 2 show the characteristics of the participants for HF and AF/flutter, respectively.
Across both study populations, cases tended to have had a severe exacerbation, were more likely to be older, male, an ex-smoker, have comorbidities including prior prevalent cardiovascular disease, and be prescribed cardiovascular medications. Cases and controls both tended to have infrequent exacerbation history, have prescriptions for long-acting therapies, but tended to not have prescriptions for short-acting inhaled therapies.
Factors associated with HF hospitalization 1-30 days post exacerbation
Age, type II diabetes, obesity, prior HF diagnosis, prior arrhythmia diagnosis, having a severe exacerbation, and most cardiovascular medications were associated with increased odds of being hospitalized for HF within 30 days of a COPD exacerbation (Table 1 ). The factors most strongly associated with HF were exacerbation severity (aOR=6.25, 95%CI 5.10-7.66), a prior HF diagnosis (aOR=2.57, 95%CI 1.73-3.83), age at least 80 years (≥80 vs. 40-69; aOR=2.41, 95%CI 1.88-3.09), and, of the cardiovascular medications, diuretics (aOR=2.81, 95%CI 2.29-3.45).
In sensitivity analyses, GOLD grade, MRC score, and history of CKD were all associated with an increased odds of being hospitalized for HF within the month post exacerbation.(Supplementary Table 1 ) The strongest associations were for CKD (aOR=1.85, 95%CI 1.46-2.35) and higher levels of airflow limitation and breathlessness (GOLD grade 3-4 Severe/Very Severe aOR=1.83, 95%CI 1.32-2.54, versus GOLD grade 1 Mild) (Score 4-5 MRC aOR=1.87, 95%CI 1.42-2.46, versus MRC 1-2).
Factors associated with AF/flutter hospitalization 1-30 days post exacerbation
Age, male sex, prior arrhythmia, prior PH, and having a severe exacerbation were associated with AF/flutter in the 30 days following an exacerbation. Most cardiovascular medications were also associated with AF/flutter (Table 2 ). The factors most strongly associated with AF were exacerbation severity (aOR=5.78 95%CI 4.45-7.50), age ≥80 years (aOR=3.15 95%CI 2.26-4.40), prior arrhythmia and PH (aOR=3.55, 95%CI 2.53-4.98; aOR=3.05, 95%CI 1.21-7.68), and of the cardiovascular medications, anticoagulants (aOR=3.81, 95%CI 2.57-5.64), positive inotropes (aOR=2.29, 95%CI 1.41-3.74) and anti-arrhythmic drugs (aOR=2.14, 95%CI 1.10-4.15).
In sensitivity analyses, GOLD grade, MRC score, and CKD had no association with AF/flutter (Supplementary Table 2 ).
In a primary care defined COPD population, this study examined the clinical profiles of people hospitalized for HF and for AF within a month post exacerbation. We observed that the odds of HF and of AF hospitalization were higher for people with severe, hospitalized exacerbations and with cardiovascular-related history. For HF analyses only, the odds of HF were also higher for people with microvascular factors (i.e., type II diabetes; obesity; CKD) and for people with pulmonary factors, namely those with worse GOLD grade of airflow limitation and higher levels of MRC breathlessness scores.
Heart failure
The pathophysiological links between COPD exacerbations and HF are recognized [ 31 , 32 , 33 ]. Upon exacerbation, dynamic lung hyperinflation from airflow limitation alongside heightened inflammation and hypoxia, can lead to increased strain on both the lungs and heart. The increased cardiopulmonary pressure can then lead to impaired contraction or filling of the left ventricle, namely HF with preserved or with reduced ejection fraction, respectively [ 31 , 32 , 33 ].
Few studies have investigated factors associated with HF in COPD alone, [ 15 , 34 , 35 , 36 ] of which only one [ 15 ] investigated hospitalized exacerbation with concurrent HF, but did not quantify this relationship with ORs, and was conducted in the US National Inpatient Sample (NIS) database. The remaining were post-hoc analyses of trials focused on stable COPD [ 34 , 35 , 36 ].
The strong magnitude of the association for exacerbation severity (hospitalization) but not for exacerbation frequency, suggests two points. First, from a healthcare service-level standpoint, patients hospitalized for exacerbations are more likely to be hospitalized for a future HF (i.e., re-admission) compared with patients whose exacerbation was managed in primary care. Second, clinically, exacerbation severity (e.g., greater intensity of inflammation) has a greater indication of a patients’ future cardiac state, rather than past exacerbation occurrence and management. Findings for older age were anticipated and align with previous studies [ 15 , 34 , 35 , 36 ].
The associations for history of HF, arrhythmia, type II diabetes, and CKD with post-exacerbation HF are unsurprising given their known independent relationships each with HF and exacerbations alone. Chronic, unmanaged HF can lead to future health service utilization for HF [ 16 , 37 ]. Arrhythmia-attributed cardiac remodeling can contribute to development of cardiomyopathy [ 38 ]. Diabetes is a risk factor for substantial HF progression [ 16 , 39 ], and separately a population-based study in COPD patients demonstrated an increased risk of cardiovascular mortality with type II diabetes [ 40 ]. Impaired renal hemodynamics and activation of the renin-angiotensin-aldosterone system (RAAS) can lead to HF, [ 41 ] and separately reduced kidney function is associated with future HF [ 42 ].
Our findings for cardiovascular medications indicate a certain treatment profile in primary care, leading up to the post-exacerbation HF hospitalization. The strongest association for diuretics suggests that leading up to future HF, patients perhaps are receiving treatment indicated for uncontrolled edema from existing HF, diabetic cardiomyopathy, or CKD for example.
GOLD and MRC as factors for post-exacerbation HF likewise were expected. Increased breathlessness and reduced lung function are not only symptoms of an imminent exacerbation or HF; equally, these factors can also indicate delayed diagnosis of unstable COPD or HF, [ 16 , 43 ] given their shared symptomology [ 3 , 12 , 14 ]. Reduced lung function can contribute to worsening prognosis and precipitate a future exacerbation or HF [ 16 , 43 ].
Atrial fibrillation
The pathophysiological mechanisms implicating AF post COPD exacerbation are also established [ 19 , 44 ]. At time of exacerbation, drastic increases in lung hyperinflation and impaired intrathoracic pressures can cause increased pulmonary vascular resistance and damage, leading to alterations to atrial electrophysiology [ 19 , 44 ]. Compromised gas exchange in the lungs can induce systemic inflammation and oxidative stress too, and also put strain on pulmonary vasculature, leading to abnormal atrial structure and ion-channel remodeling, [ 19 , 44 ] while certain treatments prescribed upon exacerbation are arrhythmogenic [ 19 , 44 ].
Only four studies have investigated patient profiles for AF development in unstable COPD, all hospital-based [ 18 , 21 , 22 , 23 ], of which two conducted in the US National Inpatient Sample (NIS) database [ 18 , 22 ]. Two examined factors associated with AF diagnosis after exacerbation [ 21 , 22 ] one of which patients had existing AF [ 21 ]. Two failed to quantify with ORs, only comparing baseline characteristics of exacerbating patients by status of concurrent AF [ 18 , 23 ].
Our findings for older age and male gender are not unexpected; studies similarly found these associations in exacerbating [ 18 , 22 ] and in stable COPD [ 45 ]. Unsurprisingly, exacerbation severity associated with future AF, again adding to the existing evidence of stronger associations for hospitalized exacerbation [ 1 , 6 ] and again, likewise to HF, suggests a distinction between healthcare service-level patient pathways, and intensity versus frequency.
The associations we found for history of PH and arrhythmias aligns with what was anticipated clinically. Electrophysiological and structural changes to the atrium over time, from either AF itself [ 20 ] or from chronic atrial stretching and fibrosis attributed to PH, [ 46 ] can lead to future AF. While a study using Euro Heart Survey data showed COPD as a factor for progression of paroxysmal to persistent AF (aOR=1.51, 95%CI 0.95-2.39) [ 20 ], neither of the two studies looking at patient profiles for post-exacerbation AF, looked at chronic, prevalent arrhythmias itself as a factor [ 21 , 22 ]. No studies have looked at PH, although the study among end-stage COPD patients in the NIS database found a weak association for pulmonary circulatory disorders (aOR=1.44, 95%CI 1.37-1.52) [ 22 ], compared the OR of about 3 for PH. A study found raised pulmonary artery pressure to be associated with AF (p<0.05), but failed to quantify, and it was small, underpowered, and not generalizable as it restricted to hospitalized COPD patients with existing AF [ 21 ].
The lack of associations for prior ACS, ischemic stroke, HF, and hypertension somewhat contradict the study among hospitalized, end-stage COPD patients, [ 22 ] where an association was found for HF (aOR=2.42; 95%CI: 2.36-2.48) and coagulopathy (aOR=1.23; 95%CI:1.16-1.31), but again this may reflect the more severe prognosis of these patients versus those in our study.
Likewise to HF, findings for cardiovascular medications indicate a certain treatment profile in primary care, leading up to the post-exacerbation AF hospitalization. Although we were unable to adjudicate by specific subtype of AF,(e.g., paroxysmal, persistent) the strongest associations for positive inotropes, anti-arrhythmic drugs, and anticoagulants may suggest that leading up to future AF, patients perhaps are receiving treatment to manage abnormal heart rate and/or rhythm, and/or to prevent clotting. Future research could investigate the respective treatment profile relative to paroxysmal AF and to persistent AF [ 47 ] to confirm and extend our findings.
Our null results for other comorbidities (i.e., depression and depressive symptoms, anxiety, BMI, CKD, and type-II diabetes) goes against studies finding an association for diabetes, [ 18 , 22 ] mixed findings for depression, [ 18 , 22 ] among other comorbidities. Yet these observed associations could be due to differing context; using the NIS database in a study population of only hospitalized, exacerbating, insured payors. GOLD airflow obstruction and MRC dyspnea score were not associated with post-exacerbation AF hospitalization, possibly as AF is often associated with vague symptoms of onset and not necessarily immediately thought about as a cause of increasing breathlessness in someone with COPD [ 44 , 48 , 49 ].
Methodological considerations
A key strength is our generalizable COPD cohort, defined within the electronic healthcare record with detailed data to examine and adjust for a range of factors. Unlike other studies, this allowed us to look at two patient pathways: cardiovascular-related hospitalizations post primary-care exacerbation, and re-admissions post hospitalized exacerbation. Our exclusion criteria allowed us to quantify the odds of new onset HF and AF hospitalizations following exacerbation, by ensuring no evidence of AF or HF in the year prior to exacerbation. We chose to study two common cardiovascular conditions in COPD, AF and HF. We could not subdivide HF and AF more granularly, because of insufficient statistical power and the inability to obtain electrocardiogram or echocardiogram results to adjudicate.
We used validated codes to define COPD [ 26 ] and COPD exacerbations [ 27 , 28 ] so misclassification is unlikely. Where possible, we used previously tested methods [ 30 ] and codes to define our factors-of-interest and codes were checked by a pulmonologist and/or cardiologist. The nested case control matched design allowed us to control for unmeasurable potential clinical differences in disease management by clinicians, by matching patients on GP practice.
To minimize selection bias among patients with measured factors only, we adjusted only for covariates without substantial missing data. We reserved GOLD, MRC, and CKD for sensitivity analyses; the associations of these factors with HF are generalizable only to patients with measurements (e.g., patients with greater healthcare monitoring, provision, or access). For this reason, the relationship for ethnicity could not be quantified, and given the data sparsity. For the HF analysis, we were unable to quantify B-type natriuretic peptide testing as a factor (BNP or NT-proBNP) because of 90% missing data for BNP (data not shown).
Confounding by indication is possible, particularly for the associations observed for cardiovascular medications (cases could have been more likely prescribed cardiovascular medications to manage a prevalent co-morbidity (perhaps with delayed diagnosis) that posed future cardiovascular risk, compared with controls) [ 50 ]. For example, although 12.8% of HF cases had prevalent HF diagnoses at baseline (201/1569), over 70% of HF cases were prescribed diuretics (1122/1569). Diuretics, particularly extended use of loop diuretics, can indicate possible, pre-HF diagnoses [ 51 ], given HF diagnoses tend to be delayed in COPD patients [ 14 , 37 , 51 ]. Taking this information together, this suggests a substantial proportion of cases could have been prescribed diuretics to manage possible-yet-undiagnosed HF, in which case, the later case-defining hospitalization was the delayed, first-time diagnosis of HF.
Our results for cardiovascular medications do not imply these medications are increasing the cardiovascular risk, rather they add to an understanding of the exacerbating patient profile. Furthermore, although these medications could indicate delayed CVD diagnosis, alternatively they could be medically indicated for management of a co-morbidity we did not adjust for, e.g., beta-blockers can be prescribed for thyroid conditions [ 52 ].
Implications for clinical practice
Within the month-window following an exacerbation, largely exacerbation intensity and cardiovascular-related management and disease history were associated with odds of incident HF and AF. For HF specifically, existing type II diabetes, CKD, lung function (GOLD grade), and levels of breathlessness (MRC) had an association too—but not for AF. These factors can help better identify patients most at-risk for HF and AF, to streamline efforts to allocate screening, vigilant monitoring, and prevention.
At the time of a COPD exacerbation, particularly hospitalized exacerbation, we recommend preemptively monitoring markers of possible HF, through taking medication history of loop diuretics, [ 51 ] and through BNP testing [ 53 ]. At present however, HF prevention is narrow in scope, with guidelines for early identification of HF not explicitly considering unstable COPD [ 53 , 54 ]. Our results suggest that HF monitoring should widen to include patients with COPD exacerbations.
Upon exacerbation, particularly hospitalized exacerbation, we recommend proactively screening for AF (e.g., electrocardiogram) [ 19 ] as AF commonly presents subclinically [ 17 ]. Still, at present, AF screening is narrow in scope; it is primarily conducted in patients with existing or suspected AF with the goal of preventing stroke, with AF guidelines not explicitly considering unstable COPD [ 17 , 55 ]. Our results suggest that AF screening should widen to include patients with COPD exacerbations, to help prevent future AF—even before stroke.
Cardiopulmonary factors were associated with hospitalization for HF in the 30 days following a COPD exacerbation, while only cardiovascular-related factors and exacerbation severity were associated with AF hospitalization. Understanding risk factors will help to target people for prevention. Future studies with the intent of improving risk prediction for HF and AF should consider exacerbations of COPD.
Availability of data and materials
Data are available on request from the CPRD. Their provision requires the purchase of a license, and this license does not permit the authors to make them publicly available to all. This work used data from the CPRD Aurum version collected in May 2022 and have clearly specified the data selected within the Methods section, and linked data in the Supplementary Materials . To allow identical data to be obtained by others, via the purchase of a license, the code lists will be provided upon request. Licenses are available from the CPRD ( http://www.cprd.com ): The Clinical Practice Research Datalink Group, The Medicines and Healthcare products Regulatory Agency, 10 South Colonnade, Canary Wharf, London E14 4PU.
Abbreviations
Chronic Obstructive Pulmonary Disease
Clinical Practice Research Datalink
- Cardiovascular Disease
General practice
Hospital Episode Statistics
Index of Multiple Deprivation
Medical Research Council
- Heart Failure
- Atrial Fibrillation
Chronic Kidney Disease
Müllerová H, Marshall J, de Nigris E, Varghese P, Pooley N, Embleton N, et al. Association of COPD exacerbations and acute cardiovascular events: a systematic review and meta-analysis. Ther Adv Respir Dis [Internet]. 2022 Jan;16:175346662211136. Available from: https://doi.org/10.1177/17534666221113647 .
Morgan AD, Zakeri R, Quint JK. Defining the relationship between COPD and CVD: what are the implications for clinical practice? Ther Adv Respir Dis [Internet]. 2018 Jan 1;12:175346581775052. Available from: https://doi.org/10.1177/1753465817750524 .
Balbirsingh V, Mohammed AS, Turner AM, Newnham M. Cardiovascular disease in chronic obstructive pulmonary disease: a narrative review. Thorax [Internet]. 2022 Sep;77(9):939–45. Available from: https://thorax.bmj.com/lookup/doi/ https://doi.org/10.1136/thoraxjnl-2021-218333 .
Graul EL, Nordon C, Rhodes K, Marshall J, Menon S, Kallis C, et al. Temporal risk of non-fatal cardiovascular events post COPD exacerbation: a population-based study [Accepted 20 December 2023]. Am J Respir Crit Care Med [Internet]. 2023 Dec; Available from: https://doi.org/10.1164/rccm.202307-1122OC .
Rothnie KJ, Connell O, Müllerová H, Smeeth L, Pearce N, Douglas I, et al. Myocardial Infarction and Ischemic Stroke after Exacerbations of Chronic Obstructive Pulmonary Disease. Ann Am Thorac Soc [Internet]. 2018 Aug;15(8):935–46. Available from: https://doi.org/10.1513/AnnalsATS.201710-815OC .
Hirayama A, Goto T, Shimada YJ, Faridi MK, Camargo CA, Hasegawa K. Acute Exacerbation of Chronic Obstructive Pulmonary Disease and Subsequent Risk of Emergency Department Visits and Hospitalizations for Atrial Fibrillation. Circ Arrhythm Electrophysiol [Internet]. Sep; 11(9):e006322. Available from: 2018. https://doi.org/10.1161/CIRCEP.118.006322 .
Article Google Scholar
Halpin DMG, Decramer M, Celli B, Kesten S, Leimer I, Tashkin DP. Risk of Nonlower Respiratory Serious Adverse Events Following COPD Exacerbations in the 4-year UPLIFT® Trial. Lung [Internet]. 2011 Aug [cited 2022 Oct 11];189(4):261–8. Available from: https://doi.org/10.1007/s00408-011-9301-8 .
Goto T, Shimada YJ, Faridi MK, Camargo CA, Hasegawa K. Incidence of Acute Cardiovascular Event After Acute Exacerbation of COPD. J Gen Intern Med [Internet]. 2018 Sep;33(9):1461–8. Available from: https://doi.org/10.1007/s11606-018-4518-3 .
Swart KMA, Baak BN, Lemmens L, Penning-van Beest FJA, Bengtsson C, Lobier M, Risk of cardiovascular events after an exacerbation of chronic obstructive pulmonary disease: results from the EXACOS-CV cohort study using the PHARMO Data Network in the Netherlands. Respir Res [Internet]., et al. 21;24(1):293. Available from: 2023 Nov. https://doi.org/10.1186/s12931-023-02601-4 .
Chen W, Thomas J, Sadatsafavi M, FitzGerald JM. Risk of cardiovascular comorbidity in patients with chronic obstructive pulmonary disease: a systematic review and meta-analysis. Lancet Respir Med [Internet]. 2015 Aug;3(8):631–9. Available from: https://doi.org/10.1016/S2213-2600(15)00241-6 .
Hesse K, Bourke S, Steer J. Heart failure in patients with COPD exacerbations: Looking below the tip of the iceberg. Respir Med [Internet]. May; 196:106800. Available from: 2022. https://doi.org/10.1016/j.rmed.2022.106800 .
Cuthbert JJ, Pellicori P, Optimal Clark AL., Management of Heart Failure and Chronic Obstructive Pulmonary Disease: Clinical Challenges. Int J Gen Med [Internet]. [cited 2023 Aug 9];Volume 15:7961–75. Available from: 2022Oct. https://doi.org/10.2147/IJGM.S295467 .
MacDonald DM, Ji Y, Adabag S, Alonso A, Chen LY, Henkle BE, et al. Cardiovascular Autonomic Function and Incident COPD Hospitalizations in ARIC. Ann Am Thorac Soc [Internet]. 2023 Jun 26;AnnalsATS.202211-964OC. Available from: https://doi.org/10.1513/annalsats.202211-964oc .
Hayhoe B, Kim D, Aylin PP, Majeed FA, Cowie MR, Bottle A. Adherence to guidelines in management of symptoms suggestive of heart failure in primary care. Heart [Internet]. 2019 May;105(9):678–85. Available from: https://doi.org/10.1136/heartjnl-2018-313971 .
Kichloo A, Minhas AMK, Jamal S, Shaikh AT, Albosta M, Singh J, Trends and Inpatient Outcomes of Primary Heart Failure Hospitalizations with a Concurrent Diagnosis of Acute Exacerbation of Chronic Obstructive Pulmonary Disease (from The National Inpatient Sample Database from 2004 to 2014). Am J Cardiol [Internet]., et al. Jul; 150:69–76. Available from: 2021. https://doi.org/10.1016/j.amjcard.2021.03.054 .
Axson EL, Ragutheeswaran K, Sundaram V, Bloom CI, Bottle A, Cowie MR, Hospitalisation and mortality in patients with comorbid COPD and heart failure: a systematic review and meta-analysis. Respir Res [Internet]., et al. [cited 2023 Apr 27];21(1):54. Available from: 2020Dec. https://doi.org/10.1186/s12931-020-1312-7 .
US Preventive Services Task Force, Davidson KW, Barry MJ, Mangione CM, Cabana M, Caughey AB, et al. Screening for Atrial Fibrillation: US Preventive Services Task Force Recommendation Statement. JAMA [Internet]. 2022 Jan 25;327(4):360. Available from: https://doi.org/10.1001/jama.2021.23732 .
Abdullah AS, Eigbire G, Ali M, Mohanad A, Abdul W, Hisham I, et al. Relationship of Atrial Fibrillation to Outcomes in Patients Hospitalized for Chronic Obstructive Pulmonary Disease Exacerbation. J Atr Fibrillation [Internet]. 2019 Aug [cited 2023 Aug 7];12(2):2117. Available from: https://doi.org/10.4022/jafib.2117 . .
Simons SO, Elliott A, Sastry M, Hendriks JM, Arzt M, Rienstra M, et al. Chronic obstructive pulmonary disease and atrial fibrillation: an interdisciplinary perspective. Eur Heart J [Internet]. 2021 Feb 1 [cited 2023 Aug 11];42(5):532–40. Available from: https://doi.org/10.1093/eurheartj/ehaa822 .
De Vos CB, Pisters R, Nieuwlaat R, Prins MH, Tieleman RG, Coelen RJS, et al. Progression From Paroxysmal to Persistent Atrial Fibrillation. J Am Coll Cardiol [Internet]. 2010 Feb;55(8):725–31. Available from: https://doi.org/10.1016/j.jacc.2009.11.040 .
Terzano C, Romani S, Conti V, Paone G, Oriolo F, Vitarelli A. Atrial fibrillation in the acute, hypercapnic exacerbations of COPD. Eur Rev Med Pharmacol Sci [Internet]. 2014 Oct;18(19):2908–17. Available from: https://www.europeanreview.org/article/7899 .
Xiao X, Han H, Wu C, He Q, Ruan Y, Zhai Y, et al. Prevalence of Atrial Fibrillation in Hospital Encounters With End-Stage COPD on Home Oxygen. Chest [Internet]. 2019 May [cited 2023 Aug 7];155(5):918–27. Available from: https://doi.org/10.1016/j.chest.2018.12.021 .
Rusinowicz T, Zielonka TM, Zycinska K. Cardiac Arrhythmias in Patients with Exacerbation of COPD. In: Clinical Management of Pulmonary Disorders and Diseases [Internet]. Cham: Springer International Publishing; 2017 [cited 2023 Aug 7]. p. 53–62. (Advances in Experimental Medicine and Biology; vol. 1022). Available from: http://link.springer.com/ https://doi.org/10.1007/5584_2017_41 .
Clinical Practice Research Datalink. CPRD Aurum May 2022 (Version 2022.05.001) [Internet]. Clinical Practice Research Datalink; 2022 [cited 2022 Nov 30]. Available from: https://doi.org/10.48329/t89s-kf12 .
Wolf A, Dedman D, Campbell J, Booth H, Lunn D, Chapman J, et al. Data resource profile: Clinical Practice Research Datalink (CPRD) Aurum. Int J Epidemiol [Internet]. 2019 Dec 1;48(6):1740–1740g. Available from: https://doi.org/10.1093/ije/dyz034 .
Quint JK, Müllerova H, DiSantostefano RL, Forbes H, Eaton S, Hurst JR, et al. Validation of chronic obstructive pulmonary disease recording in the Clinical Practice Research Datalink (CPRD-GOLD). BMJ Open [Internet]. 2014 Jul 1;4(7):e005540. Available from: https://doi.org/10.1136/bmjopen-2014-005540 .
Rothnie KJ, Müllerová H, Hurst JR, Smeeth L, Davis K, Thomas SL, et al. Validation of the Recording of Acute Exacerbations of COPD in UK Primary Care Electronic Healthcare Records. Chotirmall SH, editor. PLOS ONE [Internet]. 2016 Mar 9;11(3):e0151357. Available from: https://doi.org/10.1371/journal.pone.0151357 .
Rothnie KJ, Müllerová H, Thomas SL, Chandan JS, Smeeth L, Hurst JR, Recording of hospitalizations for acute exacerbations of COPD in UK electronic health care records. ClinEpidemiol [Internet]., et al. Nov;Volume 8:771–82. Available from: 2016. https://doi.org/10.2147/CLEP.S117867 .
Carpenter JR, Smuk M. Missing data: A statistical framework for practice. Biom J [Internet]. 2021 Jun [cited 2023 Feb 28];63(5):915–47. Available from: https://doi.org/10.1002/bimj.202000196 .
Graul EL, Stone PW, Massen GM, Hatam S, Adamson A, Denaxas S, et al. Determining prescriptions in electronic healthcare record data: methods for development of standardized, reproducible drug codelists. JAMIA Open [Internet]. 2023 Jul 4 [cited 2023 Sep 1];6(3):ooad078. Available from: https://academic.oup.com/jamiaopen/article/doi/ https://doi.org/10.1093/jamiaopen/ooad078/7252957 .
Axson EL, Sundaram V, Bloom CI, Bottle A, Cowie MR, Quint JK. Temporal Trends in the Incidence of Heart Failure among Patients with Chronic Obstructive Pulmonary Disease and Its Association with Mortality. Ann Am Thorac Soc. 2020;17(8):939–48.
Article PubMed Google Scholar
de Miguel Díez J, Chancafe Morgan J, Jiménez García R. The association between COPD and heart failure risk: a review. Int J Chron Obstruct Pulmon Dis. 2013;8:305–12.
Google Scholar
Stringer WW. Are We Treating Heart Failure in Patients with Chronic Obstructive Pulmonary Disease Appropriately? Ann Am Thorac Soc. 2020;17(8):932–4.
Article PubMed PubMed Central Google Scholar
Ehteshami-Afshar S, Mooney L, Dewan P, Desai AS, Lang NN, Lefkowitz MP, Characteristics Clinical, Outcomes of Patients With Heart Failure With Reduced Ejection Fraction and Chronic Obstructive Pulmonary Disease: Insights From PARADIGM-HF. J Am Heart Assoc [Internet]., et al. 16;10(4):e019238. Available from: 2021Feb. https://doi.org/10.1161/jaha.120.019238 .
Mentz RJ, Schmidt PH, Kwasny MJ, Ambrosy AP, O’Connor CM, Konstam MA, et al. The Impact of Chronic Obstructive Pulmonary Disease in Patients Hospitalized for Worsening Heart Failure With Reduced Ejection Fraction: An Analysis of the EVEREST Trial. J Card Fail [Internet]. 2012 Jul [cited 2023 Aug 15];18(7):515–23. Available from: https://doi.org/10.1016/j.cardfail.2012.04.010 .
Mentz RJ, Schulte PJ, Fleg JL, Fiuzat M, Kraus WE, Piña IL, et al. Clinical characteristics, response to exercise training, and outcomes in patients with heart failure and chronic obstructive pulmonary disease: Findings from Heart Failure and A Controlled Trial Investigating Outcomes of Exercise TraiNing (HF-ACTION). Am Heart J [Internet]. 2013 Feb;165(2):193–9. Available from: https://doi.org/10.1016/j.ahj.2012.10.029 .
Taylor CJ. Diagnosing heart failure: challenges in primary care. Heart [Internet]. 2019 May;105(9):663–4. Available from: https://doi.org/10.1136/heartjnl-2018-314396 .
Santhanakrishnan R, Wang N, Larson MG, Magnani JW, McManus DD, Lubitz SA, et al. Atrial Fibrillation Begets Heart Failure and Vice Versa: Temporal Associations and Differences in Preserved Versus Reduced Ejection Fraction. Circulation [Internet]. 2016 Feb 2;133(5):484–92. Available from: https://doi.org/10.1161/CIRCULATIONAHA.115.018614 .
Echouffo-Tcheugui JB, Ndumele CE, Zhang S, Florido R, Matsushita K, Coresh J, et al. Diabetes and Progression of Heart Failure. J Am Coll Cardiol [Internet]. 2022 Jun;79(23):2285–93. Available from: https://doi.org/10.1016/j.jacc.2022.03.378 .
Raslan AS, Quint JK, Cook S. All-Cause, Cardiovascular and Respiratory Mortality in People with Type 2 Diabetes and Chronic Obstructive Pulmonary Disease (COPD) in England: A Cohort Study Using the Clinical Practice Research Datalink (CPRD). Int J Chron Obstruct Pulmon Dis [Internet]. 2023 Jun [cited 2023 Aug 15];Volume 18:1207–18. Available from: https://www.dovepress.com/all-cause-cardiovascular-and-respiratory-mortality-in-people-with-type-peer-reviewed-fulltext-article-COPD .
Ryan DK, Banerjee D, Jouhra F. Management of Heart Failure in Patients with Chronic Kidney Disease. Eur Cardiol Rev [Internet]. 2022 Jul 26;17:e17. Available from: https://doi.org/10.15420/ecr.2021.33 .
Kottgen A, Russell SD, Loehr LR, Crainiceanu CM, Rosamond WD, Chang PP, et al. Reduced Kidney Function as a Risk Factor for Incident Heart Failure: The Atherosclerosis Risk in Communities (ARIC) Study. J Am Soc Nephrol [Internet]. 2007 Apr;18(4):1307–15. Available from: https://doi.org/10.1681/asn.2006101159 .
Axson EL, Bottle A, Cowie MR, Quint JK. Relationship between heart failure and the risk of acute exacerbation of COPD. Thorax. 2021 Apr 29;thoraxjnl-2020-216390.
Ioannides AE, Tayal U, Quint JK. Spirometry in atrial fibrillation: what’s the catch? Expert Rev Respir Med. 2023;17(10):937–50.
Article CAS PubMed Google Scholar
Huang Q, Xiong H, Shuai T, Zhang M, Zhang C, Wang Y, et al. Risk factors for new-onset atrial fibrillation in patients with chronic obstructive pulmonary disease: a systematic review and meta-analysis. PeerJ [Internet]. 2020 Dec 2;8:e10376. Available from: https://doi.org/10.7717/peerj.10376 .
Vahdatpour CA, Luebbert JJ, Palevsky HI. Atrial arrhythmias in chronic lung disease‐associated pulmonary hypertension. Pulm Circ [Internet]. 2020 Jan;10(1):1–13. Available from: https://doi.org/10.1177/2045894020910685 .
Camm AJ, Naccarelli GV, Mittal S, Crijns HJGM, Hohnloser SH, Ma CS, et al. The Increasing Role of Rhythm Control in Patients With Atrial Fibrillation. J Am Coll Cardiol [Internet]. 2022 May [cited 2024 Apr 12];79(19):1932–48. Available from: https://linkinghub.elsevier.com/retrieve/pii/S0735109722044886 .
van der Velden RMJ, Hermans ANL, Pluymaekers NAHA, Gawalko M, Elliott A, Hendriks JM, et al. Dyspnea in patients with atrial fibrillation: Mechanisms, assessment and an interdisciplinary and integrated care approach. Int J Cardiol Heart Vasc. 2022;42: 101086.
PubMed PubMed Central Google Scholar
Guerra F, Brambatti M, Nieuwlaat R, Marcucci M, Dudink E, Crijns HJGM, et al. Symptomatic atrial fibrillation and risk of cardiovascular events: data from the Euro Heart Survey. Eur Eur Pacing Arrhythm Card Electrophysiol J Work Groups Card Pacing Arrhythm Card Cell Electrophysiol Eur Soc Cardiol. 2017;19(12):1922–9.
Kyriacou DN, Lewis RJ. Confounding by Indication in Clinical Research. JAMA [Internet]. 1;316(17):1818. Available from: 2016. https://doi.org/10.1001/jama.2016.16435 .
Axson EL, Bottle A, Cowie MR, Quint JK. Relationship between heart failure and the risk of acute exacerbation of COPD. Thorax [Internet]. 2021 Aug;76(8):807–14. Available from: https://thorax.bmj.com/lookup/doi/ https://doi.org/10.1136/thoraxjnl-2020-216390 .
National Institute for Health and Care Excellence. British National Formulary. 2023. Beta-adrenoceptor blocking drugs: treatment summaries. Available from: https://bnf.nice.org.uk/treatment-summaries/beta-adrenoceptor-blocking-drugs/ .
Heidenreich PA, Bozkurt B, Aguilar D, Allen LA, Byun JJ, Colvin MM, et al. 2022 AHA/ACC/HFSA Guideline for the Management of Heart Failure: A Report of the American College of Cardiology/American Heart Association Joint Committee on Clinical Practice Guidelines. Circulation [Internet]. 2022 May 3;145(18). Available from: https://doi.org/10.1161/CIR.0000000000001063 .
National Institute for Health and Care Excellence. Chronic heart failure in adults: diagnosis and management [NG106] [Internet]. 2018. Available from: https://www.nice.org.uk/guidance/ng106/resources/chronic-heart-failure-in-adults-diagnosis-and-management-pdf-66141541311685 .
National Institute for Health and Care Excellence. Atrial fibrillation: diagnosis and management [NG196] [Internet]. 2021. Available from: https://www.nice.org.uk/guidance/ng196/resources/atrial-fibrillation-diagnosis-and-management-pdf-66142085507269 .
Download references
Acknowledgements
This study is based in part on data from the Clinical Practice Research Datalink obtained under license from the UK Medicines and Healthcare products Regulatory Agency. The data is provided by patients and collected by the National Health Service (NHS) as part of their care and support. The interpretation and conclusions contained in this study are those of the author/s alone.
This study was funded by AstraZeneca UK. CN, KR, and SM of the funding source took part in initial conceptualization and protocol design and the interpretation of results.
Author information
Authors and affiliations.
School of Public Health, Imperial College London, 86 Wood Lane, London, W12 0BZ, UK
Emily L. Graul, Mahmoud Al Ammouri, Constantinos Kallis, Anne E. Ioannides, Hannah R. Whittaker & Jennifer K. Quint
Biopharmaceuticals Medical, AstraZeneca, Academy House, 136 Hills Rd, Cambridge, CB2 8PA, UK
Clementine Nordon & Kirsty Rhodes
Medical and Scientific Affairs, AstraZeneca, 2 Pancras Sq, London, N1C 4AG, UK
Shruti Menon
National Heart and Lung Institute, Imperial College London, 86 Wood Lane, London, W12 0BZ, UK
Nicholas S. Peters
You can also search for this author in PubMed Google Scholar
Contributions
JQ takes responsibility for the content of the manuscript, including the data and analysis. EG, JQ, CN, KR, and SM conceptualized the study and designed the protocol. JQ, NP and EG contributed to the development of the codelists that defined the study variables. EG, CK contributed to the methodology. EG, HW, CK and AI accessed and verified the data. EG, CK and AI were responsible for data curation and management. EG, CK were responsible for formal analysis. EG wrote the original draft of the manuscript. EG, MA, JQ contributed to the literature review and clinical implications. All authors contributed and approved the final manuscript. All authors had final responsibility for the decision to submit for publication.
Corresponding author
Correspondence to Jennifer K. Quint .
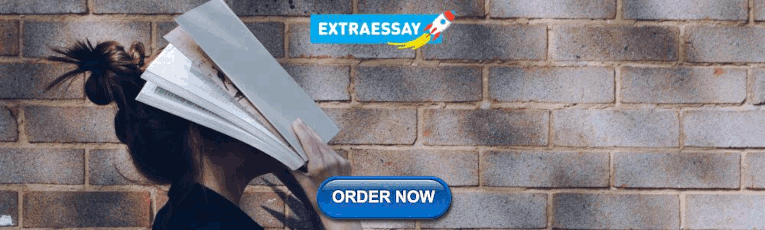
Ethics declarations
Ethics approval and consent to participate.
CPRD has NHS Health Research Authority (HRA) Research Ethics Committee (REC) approval to allow the collection and release of anonymized primary care data for observational research [NHS HRA REC reference number: 05/MRE04/87]. Each year CPRD obtains Section 251 regulatory support through the HRA Confidentiality Advisory Group (CAG), to enable patient identifiers, without accompanying clinical data, to flow from CPRD contributing GP practices in England to NHS Digital, for the purposes of data linkage [CAG reference number: 21/CAG/0008]. The protocol for this research was approved by CPRD’s Research Data Governance (RDG) Process (protocol number: 22_002377 ) and the approved protocol is available upon request. Linked pseudonymized data was provided for this study by CPRD. Data is linked by NHS Digital, the statutory trusted third party for linking data, using identifiable data held only by NHS Digital. Select general practices consent to this process at a practice level with individual patients having the right to opt-out.
Patient consent for publication
Not applicable.
Competing interests
JQ reports grants from GlaxoSmithKline, Health Data Research UK, MRC, Asthma+Lung UK, Bayer, BI, AZ and Chiesi, outside this work and AZ for the conduct of this study. JQ has received personal fees for advisory board participation, consultancy or speaking fees from GlaxoSmithKline, Evidera, AstraZeneca, and Insmed. CN, KR, and SM are employees of AZ and hold stock and/or options in the company. HW reports grants from Health Data Research UK outside the submitted work. EG, CK, AI, and MA have nothing to disclose.
Additional information
Publisher's note.
Springer Nature remains neutral with regard to jurisdictional claims in published maps and institutional affiliations.
Supplementary Information
Supplementary material 1., rights and permissions.
Open Access This article is licensed under a Creative Commons Attribution 4.0 International License, which permits use, sharing, adaptation, distribution and reproduction in any medium or format, as long as you give appropriate credit to the original author(s) and the source, provide a link to the Creative Commons licence, and indicate if changes were made. The images or other third party material in this article are included in the article's Creative Commons licence, unless indicated otherwise in a credit line to the material. If material is not included in the article's Creative Commons licence and your intended use is not permitted by statutory regulation or exceeds the permitted use, you will need to obtain permission directly from the copyright holder. To view a copy of this licence, visit http://creativecommons.org/licenses/by/4.0/ . The Creative Commons Public Domain Dedication waiver ( http://creativecommons.org/publicdomain/zero/1.0/ ) applies to the data made available in this article, unless otherwise stated in a credit line to the data.
Reprints and permissions
About this article
Cite this article.
Graul, E.L., Nordon, C., Rhodes, K. et al. Factors associated with non-fatal heart failure and atrial fibrillation or flutter within the first 30 days post COPD exacerbation: a nested case-control study. BMC Pulm Med 24 , 221 (2024). https://doi.org/10.1186/s12890-024-03035-4
Download citation
Received : 22 December 2023
Accepted : 24 April 2024
Published : 04 May 2024
DOI : https://doi.org/10.1186/s12890-024-03035-4
Share this article
Anyone you share the following link with will be able to read this content:
Sorry, a shareable link is not currently available for this article.
Provided by the Springer Nature SharedIt content-sharing initiative
- Chronic Obstructive Pulmonary Disease Exacerbations
- Electronic Health Records
- Epidemiology
BMC Pulmonary Medicine
ISSN: 1471-2466
- Submission enquiries: [email protected]
- General enquiries: [email protected]
Predicting Outcomes in Atrial Fibrillation and Heart Failure (PrOAF-HF)
Guy's and St Thomas' NHS Foundation Trust
Funder types
Identifier s
Details and patient eligibility
PrOAF-HF will aim to test if rhythm control delivered through catheter ablation in patients in whom it is not clear whether atrial fibrillation or heart failure were the first disease results in a greater improvement in left ventricular ejection fraction (LVEF) compared with patients where heart failure was diagnosed first with no evidence of AF.
Full description
Heart failure is a chronic disease affecting half a million patients in the UK. Up to 40% of patients with heart failure also suffer from atrial fibrillation. A subgroup of patients with atrial fibrillation and heart failure have tachycardia induced cardiomyopathy and benefit from significant improvement of the left ventricular function with restoration of sinus rhythm. Currently those patients can only be identified based on their response to treatment. Investigators aim to improve the treatment of AF-HF patients. The group will develop a robust modelling framework for simulating the hearts of AF-HF patients' hearts to predict patients' response to therapy and infer patient history. This modelling framework will enable integration of predictive simulations into clinical study design where the inferred initiating disease and predicted patient response to therapy are tested for selecting either rate or rhythm control in AF-HF patients. The primary objective is to test if rhythm control delivered through catheter ablation in patients where either the index disease is unclear or where AF was diagnosed prior to the onset of HF improves LVEF more than in patients where HF was diagnosed first with no evidence of AF. The secondary objectives are to examine whether the ability to terminate AF during pulmonary vein isolation is influenced by the identified index disease and to determine whether the identified index disease affects AF recurrence rate at 6 months and/or burden post ablation. This study will also identify whether the index disease affects pressure measurements as predictors of change in ejection fraction, hospitalisation, and death rates during the follow up period. This will be a non-interventional cohort study. Routinely acquired clinical data collected during pre-procedure work up will be accessed for research purposes including: 24-48 hour ambulatory (Holter) monitoring, electrocardiograph (ECG) in AF and in sinus rhythm if available; echocardiography, and clinical history and examination details. Additionally, an atrial cardiac magnetic resonance imaging (MRI) scan will be performed including assessment of atrial structure, function, fibrosis and epicardial fat burden. Symptom questionnaires will be performed During the ablation procedure, additional time will be taken to perform electrophysiological assessment in all four chambers of the heart.
Inclusion criteria
- Symptomatic persistent AF
- LVEF ≤40% prior to commencement of treatment
- NYHA class ≥2
- Patient must have been commenced on guideline directed medical therapy (GDMT)
- Patient planning to proceed to radiofrequency atrial fibrillation ablation
- Age 18-80 years
- Able and willing to provide written informed consent
Exclusion criteria
- Any clinical contra-indication to ablation
- Any disease limiting life expectancy to <1 year
- Potential participant currently pregnant or breast feeding
- Contraindication to MRI
- Paroxysmal or permanent AF
- Unable to understand verbal or written explanations given in English
Trial design
80 participants in 2 patient groups
Trial contacts and locations
Central trial contact
Magdalena Klis, MD
Data sourced from clinicaltrials.gov
Clinical trials
Research sites
Thank you for visiting nature.com. You are using a browser version with limited support for CSS. To obtain the best experience, we recommend you use a more up to date browser (or turn off compatibility mode in Internet Explorer). In the meantime, to ensure continued support, we are displaying the site without styles and JavaScript.
- View all journals
- My Account Login
- Explore content
- About the journal
- Publish with us
- Sign up for alerts
- Open access
- Published: 21 May 2024
Deep learning of left atrial structure and function provides link to atrial fibrillation risk
- James P. Pirruccello ORCID: orcid.org/0000-0001-6088-4037 1 , 2 , 3 , 4 ,
- Paolo Di Achille ORCID: orcid.org/0000-0001-9256-0678 5 , 6 ,
- Seung Hoan Choi ORCID: orcid.org/0000-0002-2797-3190 7 ,
- Joel T. Rämö 5 , 8 ,
- Shaan Khurshid 5 , 9 , 10 , 11 , 12 ,
- Mahan Nekoui ORCID: orcid.org/0000-0001-7466-4801 5 , 12 ,
- Sean J. Jurgens ORCID: orcid.org/0000-0002-1605-9782 5 , 13 , 14 ,
- Victor Nauffal ORCID: orcid.org/0000-0001-7199-299X 5 , 15 ,
- Shinwan Kany 5 , 16 ,
- Kenney Ng ORCID: orcid.org/0000-0003-0792-070X 17 ,
- Samuel F. Friedman ORCID: orcid.org/0000-0002-0688-2169 5 , 6 ,
- Puneet Batra ORCID: orcid.org/0000-0001-6822-0593 6 ,
- Kathryn L. Lunetta ORCID: orcid.org/0000-0002-9268-810X 18 ,
- Aarno Palotie ORCID: orcid.org/0000-0002-2527-5874 8 , 19 , 20 ,
- Anthony A. Philippakis ORCID: orcid.org/0000-0001-6953-3794 6 ,
- Jennifer E. Ho ORCID: orcid.org/0000-0002-7987-4768 6 , 12 , 21 ,
- Steven A. Lubitz ORCID: orcid.org/0000-0002-9599-4866 5 , 9 , 10 , 12 &
- Patrick T. Ellinor ORCID: orcid.org/0000-0002-2067-0533 5 , 9 , 10 , 12
Nature Communications volume 15 , Article number: 4304 ( 2024 ) Cite this article
2 Altmetric
Metrics details
- Cardiovascular genetics
- Genome-wide association studies
- Machine learning
Increased left atrial volume and decreased left atrial function have long been associated with atrial fibrillation. The availability of large-scale cardiac magnetic resonance imaging data paired with genetic data provides a unique opportunity to assess the genetic contributions to left atrial structure and function, and understand their relationship with risk for atrial fibrillation. Here, we use deep learning and surface reconstruction models to measure left atrial minimum volume, maximum volume, stroke volume, and emptying fraction in 40,558 UK Biobank participants. In a genome-wide association study of 35,049 participants without pre-existing cardiovascular disease, we identify 20 common genetic loci associated with left atrial structure and function. We find that polygenic contributions to increased left atrial volume are associated with atrial fibrillation and its downstream consequences, including stroke. Through Mendelian randomization, we find evidence supporting a causal role for left atrial enlargement and dysfunction on atrial fibrillation risk.
Similar content being viewed by others
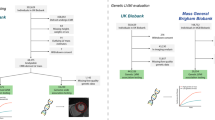
Clinical and genetic associations of deep learning-derived cardiac magnetic resonance-based left ventricular mass
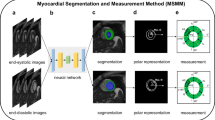
Genome-wide association analysis of left ventricular imaging-derived phenotypes identifies 72 risk loci and yields genetic insights into hypertrophic cardiomyopathy
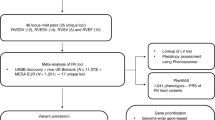
Genome-wide association analysis reveals insights into the genetic architecture of right ventricular structure and function
Introduction.
Atrial fibrillation (AF) is a common arrhythmia that is projected to affect up to 12 million Americans by 2050 1 . As a leading cause of stroke 2 , 3 , the risk factors for AF have been the subject of extensive investigation 4 , 5 , 6 . Enlargement of left atrial (LA) volumes is commonly observed with hypertension 7 , heart failure 8 , or after a diagnosis of AF 9 , 10 —and AF plays a causal role in this process 11 . Enlargement of the LA and decreased LA function have also been identified as independent risk factors for AF 10 , 12 , 13 , 14 , 15 , 16 , 17 and stroke 18 , 19 , 20 . Together, these atrial structural, contractile, or electrophysiological changes that have clinical consequences have been termed atrial cardiomyopathies 21 , 22 .
The link between LA function and AF risk has prompted interest in determining the heritability and common genetic basis for variation in LA measurements. A large-scale genome-wide association study (GWAS) in 30,201 individuals with LA measurements ascertained by echocardiography did not identify any loci with P < 5E-08 23 . Recently, a GWAS of deep learning-derived diastolic measurements in 34,245 UK Biobank participants identified one variant associated with LA volume near NPR3 24 , 25 , and a GWAS of a biplanar estimate of LA volume and function identified 14 unique loci in 35,658 participants 26 .
Taking advantage of the precision of cardiovascular magnetic resonance imaging (MRI), we developed deep learning models to produce two-dimensional measurements of the LA in 40,558 participants in the UK Biobank 27 , 28 , and applied a surface reconstruction technique to integrate these data into three-dimensional LA volume estimates. We reproduced prior observational associations between LA measurements and AF, heart failure, hypertension, and stroke. We then undertook analyses to identify common genetic variants associated with LA volumes in over 35,000 UK Biobank participants. Finally, using common genetic variants as instruments for Mendelian randomization, we performed bidirectional causal analyses between LA volume and AF.
Reconstruction of LA volumes from cardiovascular magnetic resonance images
We trained deep learning models to annotate the LA and left ventricular blood pools in four views (distinct models for the short axis view, and the two-, three-, and four-chamber long axis views). We then applied these models to all available UK Biobank cardiovascular magnetic resonance imaging (MRI) data (Methods) 27 , 28 , 29 . The quality of the deep learning models for measuring the LA was higher for the long axis views and lower for the short-axis views, which were not designed to capture the LA ( Supplementary Note ). We integrated the data from these separate cross-sections to compute the surface of a 3-dimensional representation of the LA ( Supplementary Note ), yielding LA volume estimates at 50 timepoints throughout the cardiac cycle for 40,558 participants (Fig. 1 ). We conducted analyses on the maximum LA volume (LAmax), the minimum LA volume (LAmin), the difference between those two volumes (stroke volume; LASV), and the emptying fraction (LASV/LAmax; LAEF), as well as their body surface area (BSA)-indexed counterparts (Supplementary Fig. 1 ).
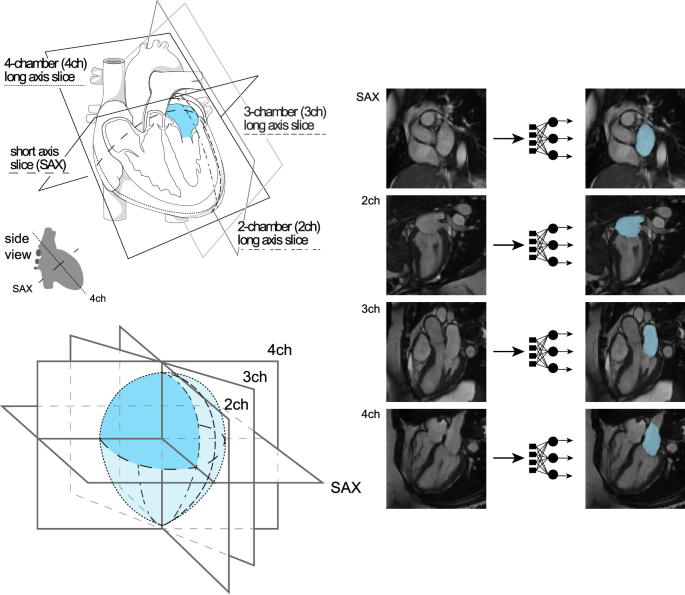
Study overview. Top left panel: orientation of the different planes in which images of the atrium were captured. The art in this panel is derived from Servier Medical Art (licensed under creativecommons by attribution, CC-BY-4.0 [ https://creativecommons.org/licenses/by/4.0/ ]). Right panel: Example images from each of the four imaging planes; after interpretation with the deep learning model, the left atrium is colored blue. Reproduced by kind permission of UK Biobank ©. Bottom left panel: schematic overview representing reconstruction of the left atrium based on information obtained from the deep learning output from the four imaging planes.
LA traits are associated with AF, heart failure, hypertension, and stroke
We analyzed the pattern of cardiac chamber volumes throughout the cardiac cycle in order to identify individuals with abnormal atrial contraction ( Supplementary Note ; Supplementary Fig. 2 ). Interestingly, a subset of 1013 participants with abnormal cardiac filling patterns had markedly elevated LA volumes, similar to those with pre-existing AF (Fig. 2 ), and were excluded from downstream analyses.
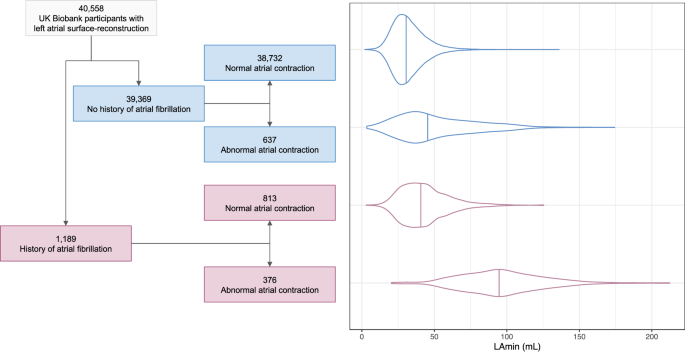
In the left panel, a flow diagram breaks down the imaged population into groups with and without AF, and then further into groups that do and do not appear to have normal cardiac filling patterns. In the right panel, the LAmin volume is depicted for these groups with violin plots; the median for each group is demarcated with a vertical line. Source data are provided as a Source Data file.
In the remaining 39,545 participants, we evaluated the association between LA measurements and prevalent or incident AF ( Supplementary Note ). The LA phenotype most strongly associated with AF was the LA minimal volume (LAmin). The 813 individuals with pre-existing AF had a greater LAmin (+8.8 mL, P = 9.2E-117). In the 2.2 years of follow-up time (mean) available on average after MRI acquisition, the risk of incident AF was increased among those with greater LAmin (293 cases; HR 1.73 per standard deviation [SD] increase; 95% CI 1.60–1.88; P = 4.0E-39). We also observed significant associations between LA measurements and hypertension, heart failure, and stroke (Fig. 3 and Supplementary Tables 1 – 3 ), as well as continuous traits such as blood pressure, creatinine, and pack years of tobacco use (Supplementary Data 1 ).
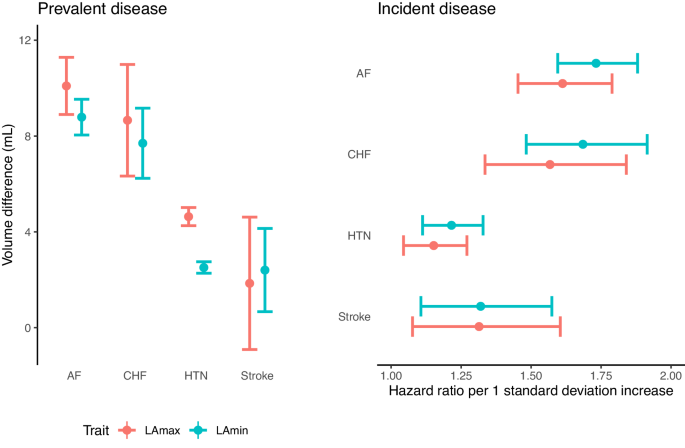
Left panel (“Prevalent disease”): the difference in LA volumes ( Y axis) between UK Biobank participants with atrial fibrillation (“AF”), heart failure (“CHF”), hypertension (“HTN”), or stroke occurring prior to MRI compared to participants without disease ( X axis). N = 39,545 participants; 813 with AF, 149 with stroke, 210 with CHF, and 11,852 with HTN. Right panel (“Incident disease”): hazard ratios for incidence of AF, CHF, HTN, and stroke ( Y axis) occurring after MRI per 1 standard deviation increase in LA volumes ( X axis). N = 36,900 (fewer due to prevalent disease for CHF and HTN; Supplementary Table 3 ); 293 with incident AF, 98 with stroke, 125 with CHF, 469 with HTN. Mean volume difference (left panel) or hazard ratio per standard deviation (right panel) estimates are represented by a circle; 95% confidence intervals for the estimate are represented by error bars. Source data are provided as a Source Data file.
Common genetic variant analysis of LA size and function identifies 20 loci
After establishing that the LA measurements replicated previously established clinical associations, we then examined the association between common genetic variants and seven LA traits: LAmax, LAmin, LAEF, and LASV, as well as for BSA-indexed LA volumes. We conducted these analyses in 35,049 participants with genetic data and without a history of AF, coronary artery disease, or heart failure (Table 1 ; Supplementary Fig. 3 ). First, we examined the SNP-heritability of the LA traits, which ranged from 0.14 (LAEF) to 0.37 (LAmax; Supplementary Table 4 ). Genetic correlation between the LA measurements ranged from −0.72 (between LAmin and LAEF) to 0.95 (between LAmax and LAmin; Supplementary Table 4 ).
Next, we performed GWAS for all seven LA traits (Table 2 ), and as a sensitivity analysis, we also performed GWAS of LA volumes after indexing on left ventricular end-diastolic volume ( Supplementary Materials and Supplementary Fig. 4 ). For all analyses, linkage disequilibrium score regression intercepts were near 1, indicating no significant evidence of inflation due to population stratification (Supplementary Table 5 ) 30 . No lead SNPs deviated from Hardy-Weinberg equilibrium (HWE) at a threshold of P < 1E-06 (Supplementary Data 2 ) 31 .
In the GWAS of LA traits conducted without indexing to BSA, we identified five loci associated with LAmax, eight with LAmin, four with LAEF, and two with LASV (Fig. 4 ). Four loci were shared between LAmax and LAmin, with lead SNPs near HLA-B , IRAK1BP1 , BEND3 , and FBXO32 / RSPH6A . LAmax was additionally associated with SNPs at the HMGA2 locus, and LAmin was associated with SNPs near ANKRD1 , SSSCA1 , IGF1R , and MYO18B . The four LAEF loci were located near FAF1 , CASQ2 , MYH6 , and MYO18B . The two LASV-associated loci included SNPs near HLA-C and MYH6 .
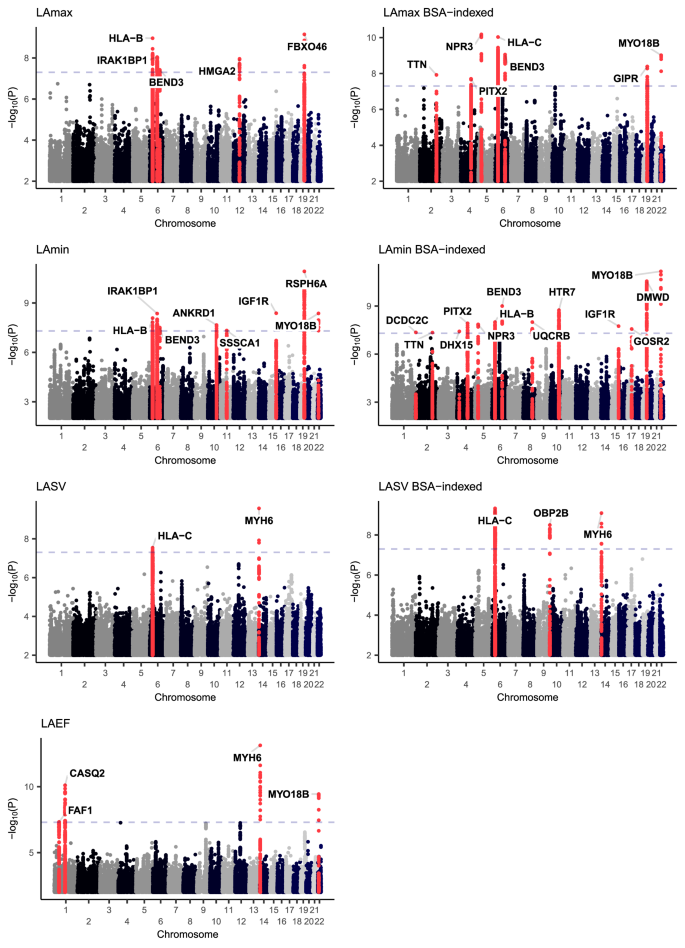
Manhattan plots showing the chromosomal position ( X axis) and the strength of association (−log10 of the P value, Y axis) for all LA measurements and the BSA-indexed counterparts (except for LAEF, which is dimensionless). Loci that contain SNPs with two-tailed BOLT-LMM P < 5E-08 are colored red and labeled with the name of the nearest gene to the most strongly associated variant.
Indexing on BSA yielded three additional loci shared by both LAmax and LAmin ( TTN , PITX2 , and NPR3 ), as well as MYO18B for LAmax, UQCRB , HTR7 , and GOSR2 for LAmin, and OBP2B for LASV. Additional loci were identified in a sensitivity analysis that accounted for left ventricular end diastolic volume (LVEDV; Supplementary Data 3 ). Because adjustment for heritable covariates can induce spurious association signals, interpretation of these loci requires caution 32 . Other sensitivity analyses (retaining participants with abnormal cardiac filling patterns; retaining only individuals with inlier genetic identities) are detailed in the Supplementary Note .
Genetic relationship between AF risk and LA dysfunction
To gain more insight into the genetic relationship between LA measurements and AF, we first evaluated their genetic correlations. Using ldsc , the strongest genetic correlation was found between LAmin and AF (rg 0.37, P = 2.0E-10), a direction of effect that corresponds to a positive correlation between LA dysfunction (i.e., increased LAmin) and risk for AF (Supplementary Table 6 ) 33 , 34 . This relationship was minimally attenuated after indexing on BSA (rg 0.33, P = 7.7E-09). We also tested for association between LA measurements and stroke (all-cause or cardioembolic) from MEGASTROKE; the strongest association was between LAmin and all-cause stroke with nominal significance (rg 0.21, P = 0.01), which was directionally concordant with increased AF risk 35 .
We then assessed the overlap between the 20 distinct LA loci identified in our study and 134 loci previously found to be associated with AF 34 . We found that 8 of the 20 LA loci overlapped with an AF locus, which was a significant enrichment based on permutation testing ( P = 1E-04, which was the minimum possible P value; see Methods) 36 . The 8 loci found in both the LA GWAS and the AF GWAS are nearest to FAF1/C1orf85 , CASQ2 , TTN , PITX2 , MYH6/MYH7 , IGF1R , GOSR2 , and MYO18B . At all 8 loci, the effect of each SNP on AF risk was in opposition to its effect on LAEF, and in most cases the effect of each SNP on AF was concordant with its effect on LAmin (Fig. 5 ). None of the loci that were linked with both LA measurements and AF were associated at genome-wide significance with LAmax.
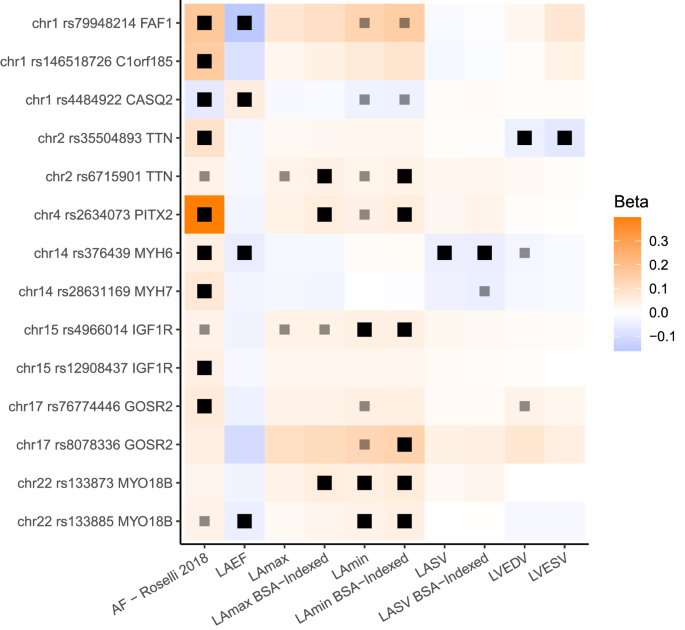
The 8 loci associated with LA measurements and AF are displayed. All loci (except those near CASQ2 and PITX2 ) have multiple patterns of linkage disequilibrium and are therefore represented multiple times. Black boxes represent an association with two-tailed BOLT-LMM P < 5E-8; lighter gray boxes represent P < 5E-6. Effect sizes are oriented with respect to the minor allele. Effect size for AF loci represents the logarithm of the odds ratio. Source data are provided as a Source Data file.
Causal link between LA minimum volume and disease risk
Because the genetic correlation analysis suggested that the strongest cross-trait association was between LAmin and AF, we performed bidirectional Mendelian randomization (MR) analyses to assess whether this relationship was causal. First, we assessed the causal effects of LAmin on the risk for AF. Variants that were associated with LAmin with P < 1E-06 were clumped and ambiguous alleles were excluded, leaving 19 SNPs. These variants were cross-referenced in summary statistics from a prior AF GWAS without UK Biobank participants to model the outcome 37 . The inverse variance weighted (IVW) model identified a significant association between LAmin and AF (OR 1.77 per SD increase in LAmin, 95% CI 1.3–2.3, P = 4.7E-05). Simple median, weighted median and MR-Egger showed the same direction of effects (Supplementary Fig. 5 ). There was significant effect heterogeneity ( P = 2.9E-05 by Cochran Q), so the contamination mixture model approach and MR-PRESSO were applied, both of which showed a significant, positive relationship between LAmin and AF with the same direction of effects (Supplementary Data 4 ; Supplementary Fig. 5 ). MR-Egger results did not reach nominal significance, nor did they yield evidence for horizontal pleiotropy (intercept P = 0.48). Within the GWAS participants, three of the 19 SNPs had evidence for pleiotropic association with AF risk factors that were derived from the CHARGE-AF risk score (Supplementary Fig. 6 ) 4 ; a sensitivity analysis excluding these three variants yielded similar results (IVW OR 1.89 per SD increase in LAmin, P = 7.3E-06; Supplementary Data 4 ; Supplementary Fig. 7 ).
Analyses treating each LA measurement as an exposure, using only instruments with P < 5E-08, revealed that the strongest statistical relationship was between LAEF and AF (OR 0.36 per SD increase in LAEF, P = 1.6E-06; Supplementary Data 5 ). Expanding the tested outcomes to heart failure 38 and stroke 35 revealed a nominal relationship between greater LAmin and increased risk for heart failure (OR 1.23 per SD increase in LAmin, P = 0.03), and between greater LAEF and reduced risk for cardioembolic stroke (OR 0.56 per SD increase in LAEF, P = 5.3E-03) but not all ischemic stroke ( P = 0.5; Supplementary Data 5 ).
We then tested the causal effect of AF on LAmin. 38 instruments that were also present in the LAmin summary statistics were taken from the 2017 AF GWAS that was conducted without UK Biobank participants 37 . Increasing genetic risk of AF was significantly associated with LAmin (0.086 SD increase per unit increase of log of odds of AF liability, 95% CI 0.049–0.123 SD, P = 6.2E-06) using the IVW approach. The simple median, weighted median, MR-Egger bootstrap, MR-PRESSO, and contamination mixture models exhibited similar directional effects and nominal significance (Supplementary Data 4 ). The intercept of the MR-Egger and MR-Egger bootstrap were not significantly different from zero (MR-Egger intercept P = 0.83, MR-Egger bootstrap intercept P = 0.39; Supplementary Data 4 , Supplementary Fig. 8 ).
A polygenic risk score for AF is associated with LA phenotypes
We constructed a 1.1-million SNP polygenic risk score (PRS) with PRScs using summary statistics from the Christophersen et al. AF GWAS, and applied this score in the 35,049 LA GWAS participants 37 , 39 . The AF PRS was statistically significantly associated with all measures of LA size and function, with a small effect size (Supplementary Table 7 ). The strongest association was with LAmin (0.052 SD increase in LAmin per SD increase in the PRS; 95% CI 0.042–0.061; P = 1.1E-25).
Polygenic estimates of LA volume predict AF, stroke, and heart failure
We created a 1.1-million SNP genome-wide polygenic score for each LA trait using PRScs 39 and tested each score in up to 423,821 UK Biobank participants who did not participate in the LA GWAS, of whom 417,881 did not have an AF diagnosis at enrollment and 21,147 developed AF afterwards. The strongest association was with the BSA-indexed LAmin polygenic score, which was linked to a modestly increased risk for incident AF or atrial flutter (HR = 1.09 per 1 SD increase in the score; P = 7.4E-32) (Fig. 6 ; Supplementary Table 8 ). This score was also associated with small increases in risks of incident all-cause stroke (7753 cases; HR = 1.04 per SD; P = 4.7E-04), ischemic stroke (5,444 cases; HR = 1.04 per SD; P = 4.7E-03), and heart failure (11,035 cases; HR = 1.05 per SD; P = 7.9E-08). Those in the top 5% of the score had a greater risk of AF (HR = 1.19, P = 7.9E-10), ischemic stroke (HR = 1.12, P = 0.06), and heart failure (HR = 1.14, P = 1.2E-03; Supplementary Data 6 ). In a sensitivity analysis that censored participants who developed AF prior to a diagnosis of heart failure, the magnitude of effect and strength of association between the LAmin score and heart failure was attenuated (7,888 cases; HR = 1.03 per SD; P = 0.01; Supplementary Data 6 ). Sensitivity analyses using lead SNP scores, different covariate adjustments, or different population subgroups yielded similar results (Supplementary Data 6 ).
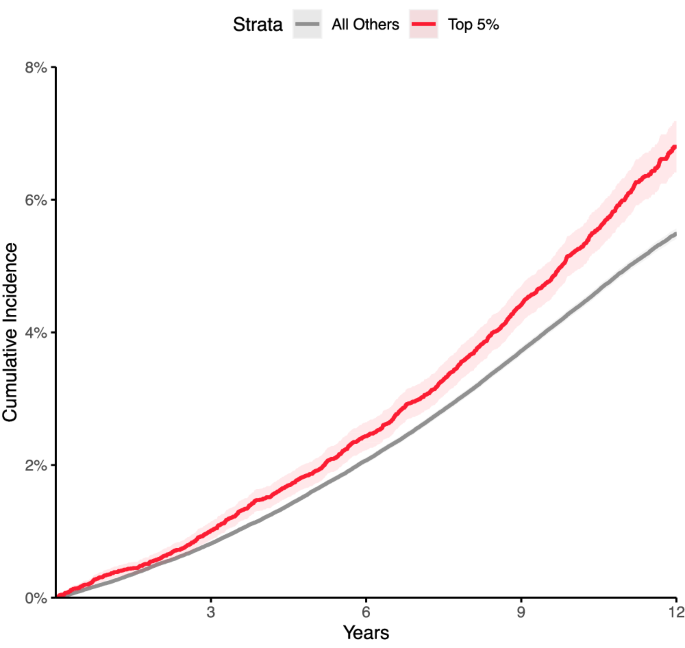
Disease incidence curves for the 417,881 participants who were unrelated to within three degrees of the participants who underwent MRI in the UK Biobank. Those in the top 5% for the BSA-indexed LAmin PRS are depicted in red; the remaining 95% are in gray. The lighter-shaded bands around each line represent the 95% confidence interval. X axis: years since enrollment in the UK Biobank. Y axis: cumulative incidence of AF (19,875 cases in the bottom 95% and 1272 cases in the top 5%). Those in the top 5% of genetically predicted LAmin indexed had an increased risk of AF (Cox HR 1.19, P = 7.9E-10) compared with those in the remaining 95% in up to 12 years of follow-up time after UK Biobank enrollment.
External validation of the LAmin polygenic score in FinnGen and All of Us
In FinnGen 40 study participants (Supplementary Data 7 ), comparable associations were observed for association between the BSA-indexed LAmin polygenic score and incident AF or atrial flutter (20,422 cases, HR = 1.08 per SD, P = 2.4E-30), ischemic stroke excluding subarachnoid hemorrhage (13,392 cases, HR = 1.03 per SD, P = 3.0E-03), ischemic stroke excluding all hemorrhage (11,822 cases, HR = 1.03 per SD, P = 5.6E-04), and heart failure (13,771 cases, HR = 1.04 per SD, P = 4.4E-06). Compared with the remaining 95% of FinnGen participants, those in the top 5% of genetically predicted LAmin indexed had an increased risk of AF (HR = 1.19 per SD, P = 8.4E-09). Those in the top 5% also had elevations in risk that were not statistically significant for ischemic stroke excluding subarachnoid hemorrhages (HR = 1.04 per SD, P = 0.36) and heart failure (HR = 1.07, P = 0.08).
In the US national biobank, All of Us 41 , the BSA-indexed LAmin polygenic score remained significantly associated with AF (4859 incident cases, HR = 1.06 per SD, P = 1.7E-04) and heart failure (5712 incident cases, HR = 1.04 per SD, P = 2.0E-02), but not ischemic stroke (66 cases, P = 0.3; Supplementary Data 8 ). In logistic models that included all cases regardless of biobank enrollment date, more cases were identified and the statistical evidence was stronger (13,399 AF cases, OR = 1.10 per SD, P = 4.9E-19; 14,572 heart failure cases, OR = 1.04 per SD, P = 1.5E-04).
In addition, 680 participants in All of Us with genetic data had BSA-indexed LAmin volume measurements. The BSA-indexed LAmin polygenic score was associated with these measurements (0.10 SD per SD of the polygenic score, P = 8.5E-03). This relationship remained nominally significant when restricted to only the largest subset of participants by genetic identity ( N = 619 participants with genetic identity similar to Europeans; 0.09 SD per SD, P = 1.5E-2).
We used a unique resource of more than 40,000 cardiac MRI studies available in the UK Biobank to enable a large, high-resolution assessment of LA structure and function. We trained deep learning models to segment LA cross-sections from cardiovascular MRI data and then derived estimates of LA volume from their 3-dimensional reconstructions. In turn, we performed an extensive series of epidemiological, genetic, polygenic, and Mendelian randomization analyses to link these LA traits to cardiovascular outcomes. Our findings permit at least five primary conclusions.
First, we were able to replicate previous observations demonstrating associations between greater LA volume and cardiovascular diseases 7 , 8 , 9 , 10 , 19 , 20 . Participants with a history of AF had larger LA volumes; and participants with larger LA volumes were more likely to be subsequently diagnosed with AF, stroke, or heart failure.
Second, these measurements enabled a large genetic analysis of LA measurements. In this work, 20 distinct genetic loci were associated with LAmax, LAmin, LAEF, LASV, or the BSA-indexed versions of these phenotypes. To our knowledge, one locus (near NPR3 ) has previously been associated at genome-wide significance with LA measurements in a study of diastolic function 25 , while 14 were recently identified in association with LA structure and function 26 . Examining the genetic findings in the present study and in Ahlberg et al. six loci were shared across both studies (near CASQ2 , MYO18B , TTN , UQCRB , ANKRD1 , and RSPH6A / FBXO46 / SIX5 ); eight were unique to Ahlberg et al. (near CITED4 , C9orf3 , BEND7 , MGAT1 , DSP , CILP , COL8A1 , and EIF2D ); and fourteen were unique to the present study (near HLA-B , IRAK1BP1 , BEND3 , HMGA2 , PITX2 , NPR3 , FAF1 , MYH6 , SSSCA1 , IGF1R , DCDC2C , DHX15 , GOSR2 , and OBP2B ). We considered this overlap in loci to be substantial, particularly since the studies used completely different deep learning models to identify the LA, and different formulas to compute LA volume from the deep learning model output (biplane vs surface reconstruction). Forty percent of the loci in our study (eight of 20) were previously associated with AF 34 , significantly more than expected by chance. At all eight loci, the allele associated with increased AF risk was directionally associated with a lower LAEF, and generally with greater LA volumes (Fig. 5 ). The opposed effect directions of these SNPs for AF risk and LAEF may be consistent with the concept of atrial cardiomyopathy 22 .
As an example of the pattern of opposed SNP effects on LAEF and AF risk, we identified a missense variant within CASQ2 (rs4074536; p.Thr66Ala) as a lead SNP for LAEF on chromosome 1. The T allele of this SNP (encoding Thr66) corresponds with a reduced LAEF in our GWAS, and with reduced expression of CASQ2 in the right atrial appendage and left ventricle in GTEx 42 . This variant is also in LD ( r 2 = 1.0) in non-African 1KG populations for the AF lead SNP rs4484922 34 , 43 . In the study by Roselli and colleagues, the rs4484922-G allele was associated with an increased risk for AF; notably, that risk-increasing allele corresponds to the LAEF-reducing T allele of rs4074536. The rs4074536-T allele has also previously been associated with a longer QRS complex duration 44 , 45 . CASQ2 encodes calsequestrin 2, which resides in the sarcoplasmic reticulum in abundance and binds to calcium ions during the cardiac cycle. Missense variants in this gene have also been associated with catecholamine-induced polymorphic ventricular tachycardia, typically following a recessive inheritance pattern 46 , 47 .
Even among LA-associated loci that were not previously associated with AF, several showed the same consistent pattern of inverse effect between AF risk and LAEF (e.g., near NPR3 , SSSCA1 , and HMGA2 ). However, this pattern did not uniformly hold. For example, at the gene-dense locus near FBXO46 / DMWD / RPSH6A , the LA volume-increasing (and LAEF-decreasing) variants were weakly associated with decreased AF risk.
Also notable was the PITX2 locus, which was the first locus associated with AF. In the present GWAS, SNPs at that locus were associated with BSA-indexed LAmax and LAmin. The lead SNP for AF (rs2129977 from Roselli et al. 2018) was in close LD with the lead SNP for LAmax and LAmin (rs2634073; r 2 = 0.85) 34 , 43 . Consistent with clinical expectations, the AF risk allele was associated with greater LA maximum and minimum volumes. These analyses excluded participants with a history of AF or abnormal cardiac filling patterns on MRI; therefore, these results support the hypothesis that the PITX2 locus may be associated with an increase in LA volume that occurs prior to AF onset, which would be consistent with experimental data showing atrial enlargement during embryonic development in mice with knocked-down PITX2 48 .
Fourth, we developed polygenic scores to gain additional insight into the relationship between LA volumes and cardiovascular diseases. A genome-wide 1.1-million variant AF PRS derived from Christophersen et al. 2017 was associated with all of the LA phenotypes—and most strongly with LAmin—even after excluding participants known to have AF 37 . This genetic evidence is consistent with and extends prior observational evidence, and suggests that some of the genetic drivers of AF risk may manifest in ways that are detectable in LA size and function.
A 1.1-million variant polygenic predictor of BSA-indexed LAmin was modestly associated with incident AF (Fig. 6 ), and weakly with stroke, in the UK Biobank. The score was also associated with heart failure—an association that was almost completely attenuated after excluding participants who were diagnosed with AF prior to heart failure. This attenuation suggests that much of the heart failure association may be mediated through AF. The association between greater genetically predicted BSA-indexed LAmin volume, heart failure, and atrial fibrillation was validated externally in FinnGen and All of Us , and the weak but statistically significant increased risk of ischemic stroke was also confirmed in FinnGen.
Finally, we found evidence of substantial genetic correlation between LA phenotypes and AF. We pursued Mendelian randomization analyses to more formally assess the hypothesis of bidirectional causation between LA phenotypes and AF. These revealed strong evidence of a causal effect of AF on LAmin, as has been previously observed 11 . There was also evidence that LA volumes, particularly LAmin, may be causal for AF. The causal effect persisted even after excluding three variants associated with at least one risk factor from CHARGE-AF 4 . However, because AF can be paroxysmal and remain undiagnosed, we cannot exclude the possibility of cryptic reverse causation: namely, that some participants may have had larger atria because of undiagnosed paroxysmal AF, such that AF itself induced the genetic association with LA volumes.
In future work, it will be interesting to determine if targeting the genes and pathways associated with abnormalities in LA function will be helpful to reduce the risk of AF, heart failure, and stroke.
This study has several limitations. All LA measurements were derived from deep learning models of cardiovascular MRI. Because a complete trans-axial stack of atrial images was not part of the UK Biobank imaging protocol, the LA measurements are estimates that are interpolated from cross-sections of the LA. Because contrast protocols were not used during image acquisition, we were not able to ascertain atrial fibrosis. The deep learning models have not been tested outside of the specific devices and imaging protocols used by the UK Biobank and are unlikely to generalize to other data sets without fine tuning. Disease labels were determined by diagnostic and procedural codes; because AF can be paroxysmal and may go undetected, it is likely that a subset of the participants had undiagnosed AF prior to MRI, which would bias causal estimates of the impact of LA volume on disease risk away from the null. The study population was largely composed of people of European ancestries, limiting generalizability of the findings to global populations. The participants who underwent MRI in the UK Biobank tended to be healthier than the remainder of the UK Biobank population, which itself is likely to be healthier than the general population. At present, there is little follow-up time subsequent to the first MRI visit for most UK Biobank participants.
In conclusion, measures of LA structure and function are heritable traits that are associated with AF, stroke, and heart failure. Genetic predictors of LA volume are linked to an elevated risk of AF and, to a lesser extent, stroke and heart failure.
Study design
Access to UK Biobank was provided under application #7089 and approved by the Partners HealthCare institutional review board (protocol 2019P003144). All UK Biobank participants provided written informed consent 49 . Analysis of All of Us was considered exempt by the UCSF IRB (#22-37715). Each All of Us biobank participant provided written informed consent 41 . The FinnGen analysis and approvals are detailed in the Supplementary Note. Study protocols complied with the tenets of the Declaration of Helsinki. Except where otherwise stated, all analyses were conducted in the UK Biobank, which is a richly phenotyped, prospective, population-based cohort that recruited 500,000 participants aged 40–69 years in the UK via mailer from 2006 to 2010 50 . We analyzed 487,283 participants with genetic data who had not withdrawn consent as of February 2020.
Statistical analyses were conducted with R version 3.6 (R Foundation for Statistical Computing, Vienna, Austria). All statistical tests were two-tailed unless otherwise specified.
Definitions of diseases and medications
We defined disease status based on self-report, ICD codes, death records, and procedural codes from the UK Biobank’s hospital episode statistics data (Supplementary Data 9 ). These data were obtained from the UK Biobank in June 2020, at which time the recommended phenotype censoring date was March 31, 2020. The UK Biobank defines that date as the last day of the month for which the number of records is greater than 90% of the mean of the number of records for the previous three months ( https://biobank.ndph.ox.ac.uk/ukb/exinfo.cgi?src=Data_providers_and_dates ).
We identified participants taking antihypertensive medications based on the Anatomical Therapeutic Classification (ATC) 51 . Medications taken by UK Biobank participants were previously mapped to ATC codes 52 . We considered medications with ATC codes beginning with C02, C09, C08CA, C03AA, C08CA01, or C03BA04 to be antihypertensives (medication names enumerated in Supplementary Data 10 ).
Cardiovascular MRI protocols
At the time of this study, the UK Biobank had released images in over 45,000 participants of an imaging substudy that is ongoing 27 , 28 . Cardiovascular MRI was performed with 1.5 Tesla scanners (Syngo MR D13 with MAGNETOM Aera scanners; Siemens Healthcare, Erlangen, Germany), and electrocardiographic gating for synchronization 28 . Several cardiac views were obtained. For this study, four views (the long axis two-, three-, and four-chamber views, as well as the short axis view) were used. In these views, balanced steady-state free precession CINEs, consisting of a series of 50 images throughout the cardiac cycle for each view, were acquired for each participant 28 . For the three long-axis views, only one imaging plane was available for each participant, with an imaging plane thickness of 6 mm and an average pixel width and height of 1.83 mm. For the short-axis view, several imaging planes were acquired. Starting at the base of the heart, 8-mm-thick imaging planes were acquired with ~2 mm gaps between each plane, forming a stack perpendicular to the longitudinal axis of the left ventricle to capture the ventricular volume. For the short axis images, the average pixel width and height was 1.86 mm.
Semantic segmentation
We labeled pixels using a process similar to that described in our prior work evaluating the thoracic aorta and which we describe here 53 . Cardiac structures were manually annotated in images from the short axis view and the two-, three-, and four-chamber long axis views from the UK Biobank by a cardiologist (JPP) using the traceoverlay software v0.1.0 54 . When present, the LA appendage was excluded, as were the pulmonary vein openings; the atrial and ventricular blood pools were distinguished by tracing a linear boundary at the base of the atrioventricular ring. To produce the models used in this manuscript, 714 short axis images were chosen, manually segmented, and used to train a deep learning model with PyTorch and fastai v1.0.61 29 , 55 . The same was done separately with 98 two-chamber images, 66 three-chamber images, and 445 four-chamber images. The models were based on a U-Net-derived architecture constructed with a ResNet34 encoder that was pre-trained on ImageNet 56 , 57 , 58 , 59 . The Adam optimizer was used 60 . The models were trained with a cyclic learning rate training policy 61 . 80% of the samples were used to train the model, and 20% were used for validation. Held-out test sets with images that were not used for training or validation were used to assess the final quality of all models.
Four separate models were trained: one for each of the three long axis views, and one for the short axis view. During training, random perturbations of the input images (augmentations) were applied, including affine rotation, zooming, and modification of the brightness and contrast.
For the short axis images, all images were resized initially to 104 × 104 pixels during the first half of training, and then to 224 × 224 pixels during the second half of training. The model was trained with a mini-batch size of 16 (with small images) or 8 (with large images). Maximum weight decay was 1E-03. The maximum learning rate was 1E-03, chosen based on the learning rate finder 29 , 62 . A focal loss function was used (with alpha 0.7 and gamma 0.7), which can improve performance in the case of imbalanced labels 63 . When training with small images, 60% of iterations were permitted to have an increasing learning rate during each epoch, and training was performed over 30 epochs while keeping the weights for all but the final layer frozen. Then, all layers were unfrozen, the learning rate was decreased to 1E-07, and the model was trained for an additional 10 epochs. When training with large images, 30% of iterations were permitted to have an increasing learning rate, and training was done for 30 epochs while keeping all but the final layer frozen. Finally, all layers were unfrozen, the learning rate was decreased to 1E-07, and the model was trained for an additional 10 epochs.
For the two-chamber long axis images, all images were resized initially to 104 × 92 pixels during the first half of training, and then to 208 × 186 pixels during the second half of training. The model was trained with a mini-batch size of 8 (with small images) or 4 (with large images). Maximum weight decay was 1E-03. Per-pixel cross entropy loss was minimized 64 . 30% of iterations were permitted to have an increasing learning rate during each epoch. When training with small images, the maximum learning rate was initially 1E-03, and training was performed over 30 epochs while keeping all weights frozen except for the final layer. When training with large images, the maximum learning rate was set to 1E-03, and the model was trained for 12 epochs while keeping all but the final layer frozen. Finally, all layers were unfrozen, the learning rate was decreased to 1E-06, and the model was retrained for an additional 8 epochs.
For the three-chamber long axis images, all images were resized initially to 128 × 128 pixels during the first half of training, and then to 256 × 256 pixels during the second half of training. The model was trained with a mini-batch size of 4 (with small images) or 2 (with large images). Maximum weight decay was 1E-02. Per-pixel cross entropy loss was minimized 64 . 30% of iterations were permitted to have an increasing learning rate during each epoch. When training with small images, the maximum learning rate was initially 1E-03, and training was performed over 20 epochs while keeping all weights frozen except for the final layer. Then, all layers were unfrozen, the learning rate was decreased to 3E-05, and the model was trained for an additional 20 epochs, with 80% of iterations permitted to have an increasing learning rate during each epoch. When training with large images, the maximum learning rate was set to 3E-04, and the model was trained for 15 epochs while keeping all but the final layer frozen; 20% of iterations were permitted to have an increasing learning rate during each epoch. Finally, all layers were unfrozen, the learning rate was decreased to 1E-07, and the model was retrained for an additional 7 epochs.
For the four-chamber long axis images, all images were resized initially to 76 × 104 pixels during the first half of training, and then to 150 × 208 pixels during the second half of training. The model was trained with a mini-batch size of 4 (with small images) or 2 (with large images). Maximum weight decay was 1E-02. Per-pixel cross entropy loss was minimized 64 . 30% of iterations were permitted to have an increasing learning rate during each epoch. When training with small images, the maximum learning rate was initially 1E-03, and training was performed over 50 epochs while keeping all weights frozen except for the final layer. Then, all layers were unfrozen, the learning rate was decreased to 3E-05, and the model was trained for an additional 15 epochs. When training with large images, the maximum learning rate was set to 3E-04, and the model was trained for 50 epochs while keeping all but the final layer frozen. Finally, all layers were unfrozen, the learning rate was decreased to 1E-07, and the model was retrained for an additional 15 epochs.
Each model was applied to all available images from its respective view that were available in the UK Biobank as of November 2020.
Semantic segmentation model quality assessment
The quality of the deep learning segmentation output was assessed against manually annotated segmentations in held-out test samples using the Sørensen-Dice coefficient, the Hausdorff distance, and the mean contour distance 65 , 66 . The Sørensen-Dice coefficient addresses the total segmentation area of the left atrium, and is a dimensionless value that ranges from 0 for an image where no pixels overlap between human and machine labels, to 1 for an image with perfect overlap between human and machine labels. The Sørensen-Dice was calculated by dividing twice the number of overlapping pixels between the two sets (the intersection) by the sum of the individual pixels considered to be left atrium in each set.
The Hausdorff distance and the mean contour distance address the perimeter of the manual and automated segmentations, and to obtain this perimeter the binary_erosion function from the python3 library scikit-image version 0.19.3 was used. The Hausdorff distance represents the maximum distance in millimeters (mm) for any point in the perimeter of the automated segmentation output to its nearest point in the perimeter of the manually annotated segmentation. The Hausdorff distance was calculated using the directed_hausdorff function from the scipy.spatial.distance python3 library, version 1.11.4. The mean contour distance represents the average distance in mm of each point on the automated segmentation output to its nearest point in the perimeter of the manually annotated segmentation. The mean contour distance was calculated for each point in the automated segmentation perimeter by testing the distance to every point in the perimeter of the manually annotated data; retaining the minimum distance for each point; and then taking the average for all points in the automated segmentation perimeter.
Poisson surface reconstruction
To integrate the output from each of the four models into one LA volume estimate, Poisson surface reconstruction was performed 67 , 68 . Among the views included in the UK Biobank cardiac MRI data set, none fully captures the 3-D anatomical structure of the LA. The short axis stack only occasionally included the lower portion of the chamber, while the three long axis (i.e., two-, three-, and four-chamber) views provided only single-slice cross-sections of the LA at different orientations. To integrate information from the four incomplete MRI views into a consistent 3D representation of the LA anatomy, we followed a procedure similar to Pirruccello et al. (2021) 69 . Briefly, we first co-rotated the LA segmentation maps from the MRI views into the same reference system (shared 3D space) using standard DICOM metadata from the Image Position (Patient) [0020,0032] and Image Orientation (Patient) [0020,0037] tags. Then, the perimeters of each 2D atrial segmentation map were extracted, yielding a sparse 3D point cloud. In addition to the point coordinates, the reconstruction algorithm requires as input a vector representing the local normal directions for each point, which is used to constrain the curvature of the reconstructed surface. In our approach, we assumed that each perimeter point’s normal vector lay on the MRI view plane and was radially oriented outwards from the center of gravity of the LA segmentation from which the point was extracted. Using three inputs, consisting of the points, the normals, and the depth argument of 16 (representing the maximum depth of the tree that the library will use for reconstruction), we applied the Poisson surface reconstruction algorithm 67 with the pypoisson python binding for the Screened Poisson Surface Reconstruction C++ library v6.13 68 . This yielded interpolated 3-D surfaces from the sparse 3D point cloud. This approach is tolerant to missing segmentation data (e.g., from the frequently missing SAX data) as long as not all available points are coplanar. 3D surfaces of the LA were reconstructed for each of the 50 MRI frames acquired during the cardiac cycle. At each timepoint, the volume of the LA was computed from the reconstructed surface model using the GetVolume routine for triangulated meshes included in the VTK library (Kitware Inc.). From the reconstructed volume traces, we estimated the maximum and minimum LA volumes, as well as LA stroke volume and emptying fraction.
Quality control after segmentation and reconstruction
Automated quality control was performed on the segmentation output to flag putatively invalid segmentations separately for each view. Studies were flagged based on the following heuristics: (a) if they had more than 1 connected component (i.e., if there were pixels in more than one connected surface that were being labeled as left atrium); (b) if the maximum single frame-to-frame change in pixels segmented as left atrium during the 50-frame CINE sequence was greater than five standard deviations beyond the population mean; (c) if no pixels were segmented as the left atrium; or (d) if the number of images in the CINE was not 50. The presence or absence of these flags was then tested for association with 3D surface reconstruction failure using logistic regression.
Identification of abnormal cardiac filling patterns
In order to focus our analyses on normal variation, we sought to exclude participants from the GWAS if they had an abnormal atrial contraction at the time of acquisition of the MRI. Although MRI uses an electrocardiographic (ECG) signal for image acquisition, the underlying ECG signal from the time of MRI signal acquisition is not available for analysis. Therefore, we sought to identify participants who appeared to have abnormal cardiac filling patterns during the MRI as a proxy for this. We trained a deep-learning model to identify the presence or absence of typical patterns of cardiac filling throughout the cardiac cycle.
To create a training set for such a model, we first fetched CINE videos from the 2-, 3-, and 4-chamber long axis views of all participants with a history of atrial fibrillation. A cardiologist (JPP) evaluated whether the videos appeared to represent a typical cardiac cycle including an atrial contraction. A deep learning model was then trained to classify filling patterns as representing normal cardiac filling or not based on the segmentation output from the semantic segmentation deep learning models. Each input channel represented the pixel counts of a cardiac chamber from a different long axis view, normalized by the maximum number of pixels seen for each channel for that participant, over the entire cardiac cycle. The normalization step prevented the model from accessing information about the absolute size of the chambers, forcing it instead to identify patterns based on relative size changes throughout the cardiac cycle. In total, 8 channels were used as input: four from the 4-chamber long axis images (left atrium, right atrium, left ventricle, right ventricle), two from the 3-chamber long axis images (left atrium, left ventricle), and two from the 2-chamber long axis images (left atrium, left ventricle). Cases were excluded if all 8 channels were not available. Therefore, the shape of the input was 50×8 (8 channels for 50 time steps). Training was performed with FastAI version 2.2.5 29 , using the TimeseriesAI library version 0.2.15 (github.com/timeseriesAI/tsai) to train an InceptionTime model 70 . The Ranger optimization function was used with cross entropy loss, and the number of filters in the InceptionTime model was 32, all of which are the software defaults in the TimeseriesAI library. Ranger incorporates RAdam and Lookahead to improve training stability early and later during training, respectively 71 , 72 . 20% of samples were randomly chosen as the validation set. The model was trained with a batch size of 32. Variable learning rates from 5E-06 to 5E-03 were permitted during training. Training was conducted using the One-Cycle policy for 20 epochs 61 , 62 .
To evaluate the accuracy of the deep learning model, manual evaluation of the cardiac filling patterns was conducted by one cardiologist (JPP) for 100 participants flagged as having abnormal cardiac filling patterns and 100 flagged as having normal cardiac filling patterns, sampled at random from participants without a history of atrial fibrillation. Sensitivity and specificity and their confidence intervals were calculated with the binom.test function in R.
Evaluation of the relationship between the LA, phenotypes, and cardiovascular diseases
For epidemiologic analyses of continuous traits, we performed linear regression, with the LA phenotypes as the dependent variable in a model with the phenotype of interest adjusted for sex, the first five principal components of ancestry, the genotyping array, the MRI scanner, and a third-degree spline of age at the time of imaging to account for possible nonlinear effects of age.
For the disease-based analyses, we focused on four disease definitions related to LA structure and function: AF or flutter, ischemic stroke, hypertension, and heart failure (defined below). For prevalent disease that was diagnosed prior to the time of imaging, linear models were used to test for an association between each disease (as a binary independent variable) and LA phenotypes (as the dependent variables), adjusting for the MRI serial number to account for inter-site differences, sex, age, and the interaction between sex and age.
For incident disease, participants with pre-existing diagnoses prior to the MRI were excluded from the analysis. A Cox proportional hazards model was used, with survival defined as the time between MRI and either the time of censoring, or disease diagnosis. The model was adjusted for the MRI serial number, sex, age, the interaction between sex and age, the cubic natural spline of height, the cubic natural spline of weight, and the cubic natural spline of BMI. As a sensitivity analysis, adjustment was additionally made for heart rate, P duration, QRS duration, P-Q interval, QTc interval, left ventricular end-systolic volume, left ventricular end diastolic volume, and left ventricular ejection fraction.
Genotyping, imputation, and genetic quality control
UK Biobank samples were genotyped on either the UK BiLEVE or UK Biobank Axiom arrays and imputed into the Haplotype Reference Consortium panel and the UK10K + 1000 Genomes panel 73 . Variant positions were keyed to the GRCh37 human genome reference. Genotyped variants with genotyping call rate <0.95 and imputed variants with INFO score <0.3 or minor allele frequency ≤ 0.005 in the analyzed samples were excluded. After variant-level quality control, 11,253,549 imputed variants remained for analysis.
Participants without imputed genetic data, or with a genotyping call rate <0.98, mismatch between self-reported sex and sex chromosome count, sex chromosome aneuploidy, excessive third-degree relatives, or outliers for heterozygosity were excluded from genetic analysis 73 . Participants were also excluded from genetic analysis if they had a history of AF or flutter, hypertrophic cardiomyopathy, dilated cardiomyopathy, heart failure, myocardial infarction, or coronary artery disease documented prior to the time they underwent cardiovascular MRI at a UK Biobank assessment center. Our definitions of these diseases in the UK Biobank are provided in Supplementary Data 9 .
GWAS of the left atrium
We analyzed the four unadjusted LA phenotypes, as well as LAmax, LAmin, and LASV estimates that were adjusted for BSA or LVEDV (rationale detailed in the Supplementary Note ), yielding 10 traits that underwent GWAS. Before conducting genetic analyses, a rank-based inverse normal transformation was applied 74 . All traits were adjusted for sex, age at enrollment, age and age 2 at the time of MRI, the first 10 principal components of ancestry, the genotyping array, and the MRI scanner’s unique identifier.
BOLT-REML v2.3.4 was used to assess the SNP-heritability of the phenotypes, as well as their genetic correlation with one another using the directly genotyped variants in the UK Biobank 75 . GWAS for each phenotype were conducted using BOLT-LMM version 2.3.4 to account for cryptic population structure and sample relatedness 75 , 76 . We used the full autosomal panel of 714,577 directly genotyped SNPs that passed quality control (minor allele frequency ≥0.001; maximum genotype missingness ≤5% for each variant; maximum sample missingness ≤2%) to construct the genetic relationship matrix (GRM), with covariate adjustment as noted above. Associations on the X chromosome were also analyzed, using all autosomal SNPs and X chromosomal SNPs to construct the GRM ( N = 732,214 SNPs), with the same covariate adjustments and significance threshold as in the autosomal analysis. In this analysis mode, BOLT treats individuals with one X chromosome as having an allelic dosage of 0/2 and those with two X chromosomes as having an allelic dosage of 0/1/2. Variants with association P < 5 × 10 −8 were considered to be genome-wide significant 77 .
We identified lead SNPs for each trait. Linkage disequilibrium (LD) clumping was performed with PLINK-1.9 31 using the same participants used for the GWAS. We outlined a 5-megabase window (--clump-kb 5000) and used a stringent LD threshold (-- r 2 0.001) in order to account for long LD blocks. With the independently significant clumped SNPs, distinct genomic loci were then defined by starting with the SNP with the strongest P value, excluding other SNPs within 500 kb, and iterating until no SNPs remained. Independently significant SNPs that defined each genomic locus are termed the lead SNPs.
HWE for GWAS lead variants was tested using the statistical library available at https://github.com/chrchang/stats (commit @67c3f71), which was written as part of Plink 31 .
Linkage disequilibrium (LD) score regression analysis was performed using ldsc version 1.0.0 30 . With ldsc , the genomic control factor (lambda GC) was partitioned into components reflecting polygenicity and inflation, using the software’s defaults.
Genetic correlation with atrial fibrillation
We used ldsc version 1.0.1 to perform cross-trait LD score regression to estimate genetic correlation between the LA measurements, atrial fibrillation (from Roselli et al. 2018), and all-cause or cardioembolic stroke (from Malik et al. 2018) 33 , 34 , 35 . Summary stats were pre-processed with the munge_sumstats.py script from ldsc 1.0.1 using the default settings, filtering out variants with imputation INFO scores less than 0.9 or minor allele frequencies below 0.01, as well as strand-ambiguous variants.
Overlap of LA loci with atrial fibrillation loci
We identified the lead SNPs associated with AF from Supplementary Table 16 of Roselli et al. 34 . For this exercise, we used each of the 134 SNPs that achieved association P < 5E-8 in the primary GWAS (column ‘I’) or in the meta-analysis (column ‘AD’). We counted the number of AF lead SNPs that fell within 500 kb of the LA lead SNP from our study. We used SNPsnap to generate 10,000 sets of SNPs that matched the LA lead SNPs based on parameters including minor allele frequency, SNPs in linkage disequilibrium, distance from the nearest gene, and gene density 36 . We then repeated the same counting procedure for each of the 10,000 synthetic SNPsnap lead SNP lists, to set a neutral expectation for the number of overlapping AF lead SNPs based on chance. This allowed us to compute a one-tailed permutation P value (with the most extreme possible P value based on 10,000 randomly chosen sets of SNPs being 1E-04).
Mendelian randomization
We sought to assess a potential causal relationship between LAmin and AF using Mendelian randomization (MR). We considered LAmin as the exposure and AF as the outcome. The genetic instruments for LAmin were generated using the genome-wide association results from this analysis. The variants from the exposure summary statistics were clumped with P < 1E-06, r 2 < 0.001, and a radius of 5 megabases using the TwoSampleMR package v0.5.7 in R 78 . These stringent clumping thresholds were intended to reduce the risk of including modestly correlated variants as if they were truly distinct instruments despite tagging the same underlying signal (e.g., having an r 2 0.1 with one another). The variants with ambiguous alleles were removed. 19 variants were harmonized with a large AF GWAS that did not include UK Biobank participants 37 . The inverse variance weighted (IVW) method was performed as the primary MR analysis. We also performed simple median, weighted median, MR-Egger, and MR-PRESSO to account for violations of the instrumental variable assumptions 79 , 80 . Since MR-Egger provides robust estimates under the InSIDE (Instrument Strength Independent of Direct Effect) assumption, we additionally conducted the MR-Egger bootstrap method to confirm the results from MR-Egger. Heterogeneity was tested with Cochran Q 81 . Because of effect heterogeneity, the contamination mixture model approach—which performs robust Mendelian randomization in the presence of invalid instruments—was also employed 82 .
To assess risk of pleiotropy of the LA genetic instruments through known pathways, each SNP was tested for association with risk factors from CHARGE-AF 4 , an atrial fibrillation risk score, within the same participants in which the GWAS was conducted. Association between each of the 19 variants and seven risk factors (height, weight, systolic blood pressure, diastolic blood pressure, use of antihypertensive medications, diagnosis of diabetes, and current smoking) was tested in a linear regression model that accounted for age and age 2 at the time of MRI, sex, the MRI serial number, the genotyping array, and genetic principal components 1–10. Associations were considered significant if they exceeded Bonferroni significance ( P < 3.8E-04).
To understand the bidirectional causal effects, we also performed an MR analysis using AF variants from the 2017 GWAS as the exposure and LA measurements as the outcome. After applying the same clumping threshold and filtering methods to AF summary statistics, 38 remaining variants were harmonized with the LAmin association results and used to construct the instrumental variable. The primary and sensitivity analyses were then conducted in the same manner as described above.
Additional Mendelian randomization analyses were conducted using each LA measurement as an exposure constructed from SNPs with P < 5E-08, tested against AF 37 , heart failure from HERMES 38 , and the trans-ancestry ischemic and cardioembolic stroke summary statistics from MEGASTROKE 35 .
Polygenic score for atrial fibrillation
We constructed a 1.1-million SNP PRS using PRScs based on summary statistics from Christophersen et al. 2017—a large AF GWAS that did not incorporate UK Biobank participants 37 , 39 . The score was constructed from 1,108,410 sites from the summary statistics that overlapped with the HapMap3 sites available in the UK Biobank as precomputed by the PRScs authors. The score was applied to the GWAS participants with LA measurements and tested for association using linear regression (Supplementary Table 7 ). For comparability, the score and the LA measurements were both standardized to a mean of zero and a standard deviation of 1.
Derivation of LA measurement polygenic scores
A polygenic score for each LA GWAS was computed using PRScs with a UK Biobank European ancestry linkage disequilibrium panel 39 . This method applies a continuous shrinkage prior to the SNP weights. PRScs was run in ‘auto’ mode on a per-chromosome basis. This mode places a standard half-Cauchy prior on the global shrinkage parameter and learns the global scaling parameter from the data; as a consequence, PRScs-auto does not require a validation data set for tuning. Based on the software default settings, only the 1.1-million SNPs found at HapMap3 sites that were also present in the UK Biobank were permitted to contribute to the score. Other polygenic scores were produced as sensitivity analyses ( Supplementary Note ).
Internal validation of LA polygenic scores in non-imaging participants
The LA polygenic scores were applied to the entire UK Biobank. Participants who had undergone MRI or related within 3 degrees of kinship to those who had undergone MRI, based on the precomputed relatedness matrix from the UK Biobank, were excluded from analysis 73 . We analyzed the relationship between this polygenic prediction of each LA measurement and incident disease (defined by self-report and diagnostic and procedural codes) in the UK Biobank using a Cox proportional hazards model as implemented by the R survival package 83 . The primary disease analyzed was atrial fibrillation. For each tested disease, we excluded participants with disease that was diagnosed prior to enrollment in the UK Biobank. We counted survival as the number of years between enrollment and disease diagnosis (for those with disease) or until death, loss to follow-up, or end of follow-up time (for those without disease).
We adjusted for covariates including sex, the cubic basis spline of age at enrollment, the interaction between the cubic basis spline of age at enrollment and sex, the genotyping array, the first five principal components of ancestry, and the cubic basis splines of height (cm), weight (kg), BMI (kg/m2), diastolic blood pressure (mmHg), and systolic blood pressure (mmHg). Sensitivity analyses included restriction participants to a genetic inlier population with European genetic identity (precomputed by the UK Biobank); adjusting for genetic principal components derived from the GWAS samples instead of the entire cohort; adjusting only for age and sex; applying score weights derived from the clumped lead variants with P < 5E-08 from each trait instead of PRScs; and thresholding the cohort into the top 5% for each polygenic score compared to the bottom 95% for the score.
External validation of the BSA-indexed LAmin polygenic score in FinnGen
FinnGen is a collection of prospective Finnish epidemiological and disease-based cohorts and hospital biobank samples 40 . The FinnGen data used here comprise 377,277 individuals from FinnGen Data Freeze 9 ( https://www.finngen.fi/en ). The data were linked by unique national personal identification numbers to the registries of national hospital discharges (available from 1968), cause of death (1969-), medication reimbursement (1964-) and purchase (1995-), specialist outpatient visits (1998-) and primary care visits (2011-). Data comprised in FinnGen Data Freeze 9 are administered by regional biobanks (Auria Biobank, Biobank of Central Finland, Biobank of Eastern Finland, Borealis Biobank, Helsinki Biobank, Tampere Biobank), the Blood Service Biobank, the Terveystalo Biobank, and biobanks administered by the Finnish Institute for Health and Welfare (THL) for the following studies: Botnia, Corogene, FinHealth 2017, FinIPF, FINRISK 1992–2012, GeneRisk, Health 2000, Health 2011, Kuusamo, Migraine, Super, T1D, and Twins). Consortium members are listed in Supplementary Note .
Patients and control subjects in FinnGen provided informed consent for biobank research, based on the Finnish Biobank Act. Alternatively, separate research cohorts, collected prior the Finnish Biobank Act came into effect (in September 2013) and start of FinnGen (August 2017), were collected based on study-specific consents and later transferred to the Finnish biobanks after approval by Fimea (Finnish Medicines Agency), the National Supervisory Authority for Welfare and Health. Recruitment protocols followed the biobank protocols and were approved by Fimea. The Coordinating Ethics Committee of the Hospital District of Helsinki and Uusimaa (HUS) statement number for the FinnGen study is Nr HUS/990/2017.
The FinnGen study is approved by Finnish Institute for Health and Welfare (permit numbers: THL/2031/6.02.00/2017, THL/1101/5.05.00/2017, THL/341/6.02.00/2018, THL/2222/6.02.00/2018, THL/283/6.02.00/2019, THL/1721/5.05.00/2019 and THL/1524/5.05.00/2020), Digital and population data service agency (permit numbers: VRK43431/2017-3, VRK/6909/2018-3, VRK/4415/2019-3), the Social Insurance Institution (permit numbers: KELA 58/522/2017, KELA 131/522/2018, KELA 70/522/2019, KELA 98/522/2019, KELA 134/522/2019, KELA 138/522/2019, KELA 2/522/2020, KELA 16/522/2020), Findata permit numbers THL/2364/14.02/2020, THL/4055/14.06.00/2020,,THL/3433/14.06.00/2020, THL/4432/14.06/2020, THL/5189/14.06/2020, THL/5894/14.06.00/2020, THL/6619/14.06.00/2020, THL/209/14.06.00/2021, THL/688/14.06.00/2021, THL/1284/14.06.00/2021, THL/1965/14.06.00/2021, THL/5546/14.02.00/2020, THL/2658/14.06.00/2021, THL/4235/14.06.00/202, Statistics Finland (permit numbers: TK-53-1041-17 and TK/143/07.03.00/2020 (earlier TK-53-90-20) TK/1735/07.03.00/2021, TK/3112/07.03.00/2021) and Finnish Registry for Kidney Diseases permission/extract from the meeting minutes on 4th July 2019.
The Biobank Access Decisions for FinnGen samples and data utilized in FinnGen Data Freeze 9 include: THL Biobank BB2017_55, BB2017_111, BB2018_19, BB_2018_34, BB_2018_67, BB2018_71, BB2019_7, BB2019_8, BB2019_26, BB2020_1, Finnish Red Cross Blood Service Biobank 7.12.2017, Helsinki Biobank HUS/359/2017, HUS/248/2020, Auria Biobank AB17-5154 and amendment #1 (August 17 2020), AB20-5926 and amendment #1 (April 23 2020) and it´s modification (Sep 22 2021), Biobank Borealis of Northern Finland_2017_1013, Biobank of Eastern Finland 1186/2018 and amendment 22 § /2020, Finnish Clinical Biobank Tampere MH0004 and amendments (21.02.2020 & 06.10.2020), Central Finland Biobank 1-2017, and Terveystalo Biobank STB 2018001 and amendment 25th Aug 2020.
FinnGen samples were genotyped using Illumina and Affymetrix arrays (Illumina Inc., San Diego, and Thermo Fisher Scientific, Santa Clara, CA, USA). Genotype imputation was performed using a population-specific SISu v3 imputation reference panel comprised high-coverage (25-30x) whole genome sequences from 3775 participants as described in a separate protocol ( https://doi.org/10.17504/protocols.io.xbgfijw ).
PRS weights were applied using PLINK v1.9 31 , 84 . Case and control statuses for atrial fibrillation or flutter, ischemic stroke excluding subarachnoid hemorrhage, ischemic stroke excluding all hemorrhages and heart failure were defined based on events in the hospital, cause of death, specialist outpatient, primary care, and medication reimbursement registries at any point during registry follow-up as detailed in Supplementary Data 7 . The association of PRS with each outcome was assessed using Cox proportional hazards models with follow-up time scale using sex, baseline age, baseline age squared, 5 genomic principal components, and the genotyping array as fixed-effects covariates.
External validation of the BSA-indexed LAmin polygenic score in All of Us
All of Us is an ongoing, diverse national biobank project in the United States 41 . Data include those from physical examination, biospecimen collection, the electronic health record (EHR), and surveys. All participants provided written, informed consent. At the time of analysis, the controlled-access data release version was 7. Within this release, we identified 245,149 participants with whole genome sequencing data.
At the time of analysis, whole genome sequencing (WGS) had been completed in 245,400 participants. Sequencing and sample quality control in All of Us has been detailed previously 85 , 86 . In brief, sequencing was performed with Illumina NovaSeq 6000, aligned GRCh38 and variants called by DRAGEN v3.4.12. A joint call set was prepared centrally by All of Us . Sample-level quality control was performed centrally by All of Us : exclusion criteria included fingerprint concordance log likelihood ratio ≤−3; sex discordance between self-report and WGS-based chromosomal sex call (if sex reported at birth was either “Male” or “Female”); contamination rate ≥ 3%; or mean coverage <30×, or <90% of bases at 20× coverage, or <8E10 aligned Q30 bases, or <95% of bases in 59 hereditary disease risk genes with 20× coverage. Fingerprint concordance was checked at 114 sites using Picard v2.23.9. Variant-level filtration removed sites with no high-quality genotypes, with ExcessHet <54.69, or with QUAL < 60 for SNPs or <69 for Indels. Ancestry prediction was performed centrally by All of Us ; briefly, Human Genome Diversity Project and 1000 Genomes samples were used to train a random forest to identify ancestry labels based on PCA from high-quality variant sites, and these loadings were then applied in All of Us .
PRScs-based polygenic score weights from the UK Biobank were lifted over from GRCh37 to GRCh38 87 . Polygenic scores were then applied to all participants with WGS as an allelic sum, with an average taken over all of the weights. The UK Biobank GWAS in-sample PCA loadings were applied to the All of Us participants in the same way. These were then tested for association with the presence or absence of disease at any point prior to enrollment or during follow-up in a logistic regression model after adjustment for age at enrollment, whether the individual’s self-reported sex was male, and the first five principal components of ancestry. Similarly, the association with incident disease was tested with a Cox model with the same covariate adjustments after excluding individuals with disease prior to enrollment. All individuals with available data were analyzed. Sensitivity analyses examining only individuals with the “EUR” ancestry label were also conducted.
Atrial fibrillation was defined to be present starting on the first date any of the following diagnostic or procedural codes were reported:
ICD10-CM: I48, I48.0, I48.1, I48.11, I48.19, I48.2, I48.20, I48.21, I48.3, I48.4, I48.9, I48.91, I48.92;
ICD9-CM: 427.31;
SNOMED: 49436004, 282825002, 426749004, 440059007, 440028005;
CPT4: 92960.
Heart failure was defined by the following codes:
SNOMED: 84114007, 42343007, 441530006, 441481004, 194779001, 15781000119107, 88805009, 5148006, 92506005, 10633002, 698296002, 426263006, 82523003, 96311000119109, 194781004, 698594003, 426611007, 15629541000119106, 23341000119109, 48447003, 10335000, 7411000175102, 424404003, 418304008, 443343001, 46113002, 417996009, 443254009, 120871000119108, 120861000119102, 56675007, 49584005, 359617009, 7421000175106, 722095005, 443344007, 153951000119103, 153931000119109, 85232009, 367363000, 83291003, 79955004, 16838951000119100, 44313006, 446221000, 703272007, 703273002
Ischemic stroke was defined by the following codes:
SNOMED: 371041009, 9901000119100, 422504002
The only volumetric LA measurement available in All of Us was the BSA-indexed LAmin volume (labeled “Left atrial End-systolic volume/Body surface area [Volume/Area] by US.2D+Calculated by area-length method”). This was analyzed as a continuous trait and was tested for association with the BSA-indexed LAmin polygenic score with adjustment for age at the time of measurement acquisition, sex, and the first five principal components of ancestry.
Reporting summary
Further information on research design is available in the Nature Portfolio Reporting Summary linked to this article.
Data availability
GWAS summary statistics have been deposited in the GWAS Catalog under accession #GCP000842. Polygenic score weights have been deposited at doi:10.5281/zenodo.10814404 88 . LA measurements have been returned to the UK Biobank for use by any approved researcher. UK Biobank data are made available to researchers from research institutions with genuine research inquiries, following IRB and UK Biobank approval. All of Us data are available for analysis to qualified researchers on the All of Us research platform. FinnGen Freeze 9 GWAS summary statistics are available at https://www.finngen.fi/en/access_results . All other data are contained within the article and its supplementary information. Source data are provided with this paper.
Code availability
Manual annotation for semantic segmentation was performed using traceoverlay v0.1.0 54 . The deep learning models have been returned to the UK Biobank for use by other researchers. The mri_la_poisson.py script used to perform Poisson surface reconstruction from segmentation output may be downloaded from Zenodo (doi:10.5281/zenodo.10811233) and is actively developed at https://github.com/broadinstitute/ml4h , available under an open-source BSD license 89 .
Miyasaka, Y. et al. Secular trends in incidence of atrial fibrillation in Olmsted County, Minnesota, 1980 to 2000, and implications on the projections for future prevalence. Circulation 114 , 119–125 (2006).
Article PubMed Google Scholar
Marini, Carmine et al. Contribution of atrial fibrillation to incidence and outcome of ischemic stroke. Stroke 36 , 1115–1119 (2005).
Wolf, P. A., Abbott, R. D. & Kannel, W. B. Atrial fibrillation as an independent risk factor for stroke: the Framingham Study. Stroke 22 , 983–988 (1991).
Article CAS PubMed Google Scholar
Alonso, A. et al. Simple risk model predicts incidence of atrial fibrillation in a racially and geographically diverse population: the CHARGE-AF consortium. J. Am. Heart Assoc. 2 , e000102 (2013).
Article PubMed PubMed Central Google Scholar
Hulme, O. L. et al. Development and validation of a prediction model for atrial fibrillation using electronic health records. JACC Clin Electrophysiol 5 , 1331–1341 (2019).
Li, Y.-G. et al. A simple clinical risk score (C2HEST) for predicting incident atrial fibrillation in Asian subjects: derivation in 471,446 Chinese subjects, with internal validation and external application in 451,199 Korean subjects. Chest 155 , 510–518 (2019).
Vaziri, S. M., Larson, M. G., Lauer, M. S., Benjamin, E. J. & Levy, D. Influence of blood pressure on left atrial size. Hypertension 25 , 1155–1160 (1995).
Cioffi, G. et al. Left atrial size and force in patients with systolic chronic heart failure: comparison with healthy controls and different cardiac diseases. Exp. Clin. Cardiol. 15 , e45–e51 (2010).
PubMed PubMed Central Google Scholar
Sanfilippo, A. J. et al. Atrial enlargement as a consequence of atrial fibrillation. A prospective echocardiographic study. Circulation 82 , 792–797 (1990).
Sardana, Mayank et al. Association of left atrial function index with atrial fibrillation and cardiovascular disease: the Framingham offspring study. J. Am. Heart Assoc. 7 , e008435 (2018).
van de Vegte, Y. J., Siland, J. E., Rienstra, M. & van der Harst, P. Atrial fibrillation and left atrial size and function: a Mendelian randomization study. Sci. Rep. 11 , 8431 (2021).
Henry, W. L. et al. Relation between echocardiographically determined left atrial size and atrial fibrillation. Circulation 53 , 273–279 (1976).
Jin, X., Pan, J., Wu, H. & Xu, D. Are left ventricular ejection fraction and left atrial diameter related to atrial fibrillation recurrence after catheter ablation? A meta-analysis. Medicine (Baltimore) 97 , e10822 (2018).
Lim, D. J. et al. Change in left atrial function predicts incident atrial fibrillation: the multi-ethnic study of atherosclerosis. Eur. Heart. J. Cardiovasc. Imag. 20 , 979–987 (2019).
Article Google Scholar
Park, J. J. et al. Left atrial strain as a predictor of new-onset atrial fibrillation in patients with heart failure. JACC Cardiovasc. Imaging 13 , 2071–2081 (2020).
Tsang, T. S. et al. Left atrial volume: important risk marker of incident atrial fibrillation in 1655 older men and women. Mayo Clin. Proc. 76 , 467–475 (2001).
Vaziri, S. M., Larson, M. G., Benjamin, E. J. & Levy, D. Echocardiographic predictors of nonrheumatic atrial fibrillation. The Framingham Heart Study. Circulation 89 , 724–730 (1994).
Benjamin, E. J., D’Agostino, R. B., Belanger, A. J., Wolf, P. A. & Levy, D. Left atrial size and the risk of stroke and death. The Framingham Heart Study. Circulation 92 , 835–841 (1995).
Bouzas-Mosquera, A. et al. Left atrial size and risk for all-cause mortality and ischemic stroke. CMAJ 183 , E657–E664 (2011).
Xu, Y. et al. Left atrial enlargement and the risk of stroke: a meta-analysis of prospective cohort studies. Front. Neurol. 11 , 26 (2020).
Fatkin, D., Huttner, I. G. & Johnson, R. Genetics of atrial cardiomyopathy. Curr. Opin. Cardiol. 34 , 275–281 (2019).
Goette, A. et al. EHRA/HRS/APHRS/SOLAECE expert consensus on atrial cardiomyopathies: definition, characterization, and clinical implication. Heart Rhythm 14 , e3–e40 (2017).
Wild, P. S. et al. Large-scale genome-wide analysis identifies genetic variants associated with cardiac structure and function. J. Clin. Invest. 127 , 1798–1812 (2017).
Bai, W. et al. A population-based phenome-wide association study of cardiac and aortic structure and function. Nat. Med. 1–9 https://doi.org/10.1038/s41591-020-1009-y (2020).
Thanaj, M. et al. Genetic and environmental determinants of diastolic heart function. medRxiv 2021.06.07.21257302 https://doi.org/10.1101/2021.06.07.21257302 (2021).
Ahlberg, G. et al. Genome-wide association study identifies 18 novel loci associated with left atrial volume and function. Eur. Heart J. https://doi.org/10.1093/eurheartj/ehab466 (2021).
Petersen, S. E. et al. Imaging in population science: cardiovascular magnetic resonance in 100,000 participants of UK Biobank - rationale, challenges and approaches. J. Cardiovasc. Magn. Reson. 15 , 46 (2013).
Petersen, S. E. et al. UK Biobank’s cardiovascular magnetic resonance protocol. J. Cardiovasc. Magn. Reson. 18 , 8 (2016).
Howard, J. & Gugger, S. Fastai: a layered API for deep learning. Information 11 , 108 (2020).
Bulik-Sullivan, B. K. et al. LD Score regression distinguishes confounding from polygenicity in genome-wide association studies. Nature Genetics 47 , 291–295 (2015).
Article CAS PubMed PubMed Central Google Scholar
Chang, C. C. et al. Second-generation PLINK: rising to the challenge of larger and richer datasets. Gigascience 4 , 7 (2015).
Aschard, H., Vilhjálmsson, B. J., Joshi, A. D., Price, A. L. & Kraft, P. Adjusting for heritable covariates can bias effect estimates in genome-wide association studies. Am. J. Hum. Genet. 96 , 329–339 (2015).
Bulik-Sullivan, B. et al. An atlas of genetic correlations across human diseases and traits. Nat. Genet. 47 , 1236–1241 (2015).
Roselli, C. et al. Multi-ethnic genome-wide association study for atrial fibrillation. Nat. Genet. 50 , 1225–1233 (2018).
Malik, R. et al. Multiancestry genome-wide association study of 520,000 subjects identifies 32 loci associated with stroke and stroke subtypes. Nat. Genet. 50 , 524–537 (2018).
Pers, T. H., Timshel, P. & Hirschhorn, J. N. SNPsnap: a web-based tool for identification and annotation of matched SNPs. Bioinformatics 31 , 418–420 (2015).
Christophersen, I. E. et al. Large-scale analyses of common and rare variants identify 12 new loci associated with atrial fibrillation. Nat. Genet. 49 , 946–952 (2017).
Shah, S. et al. Genome-wide association and Mendelian randomisation analysis provide insights into the pathogenesis of heart failure. Nat. Commun. 11 , 1–12 (2020).
Ge, T., Chen, C.-Y., Ni, Y., Feng, Y.-C. A. & Smoller, J. W. Polygenic prediction via Bayesian regression and continuous shrinkage priors. Nat. Commun. 10 , 1776 (2019).
Kurki, M. I. et al. FinnGen: unique genetic insights from combining isolated population and national health register data. medrxiv https://doi.org/10.1101/2022.03.03.22271360 (2022).
Denny, J. C. et al. The “All of Us” research program. N. Engl. J. Med. 381 , 668–676 (2019).
Lonsdale, J. et al. The genotype-tissue expression (GTEx) project. Nature Genetics 45 , 580–585 (2013).
Article CAS Google Scholar
Machiela, M. J. & Chanock, S. J. LDlink: a web-based application for exploring population-specific haplotype structure and linking correlated alleles of possible functional variants. Bioinformatics 31 , 3555–3557 (2015).
Prins, B. P. et al. Exome-chip meta-analysis identifies novel loci associated with cardiac conduction, including ADAMTS6. Genome Biol 19 , 87 (2018).
Sotoodehnia, N. et al. Common variants in 22 loci are associated with QRS duration and cardiac ventricular conduction. Nat. Genet. 42 , 1068–1076 (2010).
Lahat, H. et al. A missense mutation in a highly conserved region of CASQ2 is associated with autosomal recessive catecholamine-induced polymorphic ventricular tachycardia in bedouin families from Israel. Am. J. Hum. Genet. 69 , 1378–1384 (2001).
Ng, Kevin et al. An international multicenter evaluation of inheritance patterns, arrhythmic risks, and underlying mechanisms of CASQ2-catecholaminergic polymorphic ventricular tachycardia. Circulation 142 , 932–947 (2020).
Chinchilla, A. et al. PITX2 insufficiency leads to atrial electrical and structural remodeling linked to arrhythmogenesis. Circ. Cardiovasc. Genet. 4 , 269–279 (2011).
Collins, R. UK Biobank Protocol. https://www.ukbiobank.ac.uk/media/gnkeyh2q/study-rationale.pdf (2007).
Sudlow, C. et al. UK biobank: an open access resource for identifying the causes of a wide range of complex diseases of middle and old age. PLoS Med. 12 , e1001779 (2015).
Santos, R. et al. A comprehensive map of molecular drug targets. Nat. Rev. Drug Discov. 16 , 19–34 (2017).
Wu, Y. et al. Genome-wide association study of medication-use and associated disease in the UK Biobank. Nat. Commun. 10 , (2019).
Pirruccello, J. P. et al. Deep learning enables genetic analysis of the human thoracic aorta. bioRxiv https://doi.org/10.1101/2020.05.12.091934 (2020).
Pirruccello, J. carbocation/traceoverlay: traceoverlay v0.1.0. Zenodo https://doi.org/10.5281/zenodo.10811511 (2024).
Paszke, A. et al. PyTorch: an imperative style, high-performance deep learning library. arXiv https://arxiv.org/abs/1912.01703 (2019).
Deng, J. et al. ImageNet: a large-scale hierarchical image database. In: 2009 IEEE Conference on Computer Vision and Pattern Recognition 248–255. https://doi.org/10.1109/CVPR.2009.5206848 (2009).
He, K., Zhang, X., Ren, S. & Sun, J. Deep residual learning for image recognition. arXiv https://ieeexplore.ieee.org/document/7780459 (2015).
Krizhevsky, A., Sutskever, I. & Hinton, G. E. ImageNet classification with deep convolutional neural networks. Commun. ACM 60 , 84–90 (2017).
Ronneberger, O., Fischer, P. & Brox, T. U-Net: convolutional networks for biomedical image segmentation. arXiv https://arxiv.org/abs/1505.04597 (2015).
Kingma, D. P. & Ba, J. Adam: a method for stochastic optimization. arXiv https://arxiv.org/abs/1412.6980 (2017).
Smith, L. N. Cyclical learning rates for training neural networks. arXiv https://arxiv.org/abs/1506.01186 (2015).
Smith, L. N. A disciplined approach to neural network hyper-parameters: Part 1 -- learning rate, batch size, momentum, and weight decay. arXiv https://arxiv.org/abs/1803.09820 (2018).
Lin, T.-Y., Goyal, P., Girshick, R., He, K. & Dollár, P. Focal loss for dense object detection. arXiv https://arxiv.org/abs/1708.02002 (2018).
D. R. Cox. The regression analysis of binary sequences. J. R. Stat. Soc. Ser. B Methodol. 20 , 215–232 (1958).
Dice, L. R. Measures of the amount of ecologic association between species. Ecology 26 , 297–302 (1945).
Huttenlocher, D. P., Klanderman, G. A. & Rucklidge, W. J. Comparing images using the Hausdorff distance. IEEE Trans. Pattern Anal. Mach. Intell. 15 , 850–863 (1993).
Kazhdan, M., Bolitho, M. & Hoppe, H. Poisson surface reconstruction. (The Eurographics Association). https://doi.org/10.2312/SGP/SGP06/061-070 (2006).
Kazhdan, M. & Hoppe, H. Screened poisson surface reconstruction. ACM Trans. Graph. 32 , 29:1–29:13 (2013).
Pirruccello, J. P. et al. Genetic analysis of right heart structure and function in 40,000 people. bioRxiv https://doi.org/10.1101/2021.02.05.429046 (2021).
Fawaz, H. I. et al. InceptionTime: finding AlexNet for time series classification. Data Min. Knowl. Discov. 34 , 1936–1962 (2020).
Article MathSciNet Google Scholar
Liu, L. et al. On the variance of the adaptive learning rate and beyond. arXiv https://arxiv.org/abs/1908.03265 (2020).
Zhang, M. R., Lucas, J., Hinton, G. & Ba, J. Lookahead optimizer: k steps forward, 1 step back. arXiv https://arxiv.org/abs/1907.08610 (2019).
Bycroft, C. et al. The UK Biobank resource with deep phenotyping and genomic data. Nature 562 , 203 (2018).
Yang, J. et al. FTO genotype is associated with phenotypic variability of body mass index. Nature 490 , 267–272 (2012).
Loh, P.-R. et al. Efficient Bayesian mixed-model analysis increases association power in large cohorts. Nat. Genet. 47 , 284–290 (2015).
Loh, P.-R., Kichaev, G., Gazal, S., Schoech, A. P. & Price, A. L. Mixed-model association for biobank-scale datasets. Nature Genetics 50 , 906–908 (2018).
Risch, N. & Merikangas, K. The future of genetic studies of complex human diseases. Science 273 , 1516–1517 (1996).
Hemani, G. et al. The MR-Base platform supports systematic causal inference across the human phenome. eLife 7 , e34408 (2018).
Bowden, J., Davey Smith, G. & Burgess, S. Mendelian randomization with invalid instruments: effect estimation and bias detection through Egger regression. Int. J. Epidemiol. 44 , 512–525 (2015).
Verbanck, M., Chen, C.-Y., Neale, B. & Do, R. Detection of widespread horizontal pleiotropy in causal relationships inferred from Mendelian randomization between complex traits and diseases. Nat. Genet. 50 , 693–698 (2018).
Cochran, J. D. et al. Clonal hematopoiesis in clinical and experimental heart failure with preserved ejection fraction. Circulation 148 , 1165–1178 (2023).
Burgess, S., Foley, C. N., Allara, E., Staley, J. R. & Howson, J. M. M. A robust and efficient method for Mendelian randomization with hundreds of genetic variants. Nat. Commun. 11 , 376 (2020).
Therneau, T. M. & Grambsch, P. M. Modeling survival data: extending the Cox model. (Springer-Verlag, New York). https://doi.org/10.1007/978-1-4757-3294-8 (2000).
Purcell, S. et al. PLINK: a tool set for whole-genome association and population-based linkage analyses. Am. J. Hum. Genet. 81 , 559–575 (2007).
Venner, E. et al. Whole-genome sequencing as an investigational device for return of hereditary disease risk and pharmacogenomic results as part of the All of Us research program. Genome Med. 14 , 34 (2022).
Bick, A. G. et al. Genomic data in the All of Us research program. Nature 1–7 https://doi.org/10.1038/s41586-023-06957-x (2024).
Hinrichs, A. S. et al. The UCSC genome browser database: update 2006. Nucleic Acids Res. 34 , D590–D598 (2006).
Pirruccello, J. Left atrial polygenic scores for ‘deep learning of left atrial structure and function provides link to atrial fibrillation risk’. Zenodo https://doi.org/10.5281/zenodo.10814404 (2024).
Di Achille, P. LA GWAS checkpoint of Poisson surface reconstruction with mri_la_poisson.py. Zenodo https://doi.org/10.5281/zenodo.10811233 (2024).
Download references
Acknowledgements
This work was supported by the Fondation Leducq (14CVD01), and by grants from the National Institutes of Health to Dr. Ellinor (1RO1HL092577, K24HL105780) and Dr. Ho (R01HL134893, R01HL140224, K24HL153669). This work was supported by a John S LaDue Memorial Fellowship, the Sarnoff Cardiovascular Research Foundation Scholar Award, and NIH K08HL159346 to Dr. Pirruccello. Dr. Kany was supported by the Walter Benjamin Fellowship from the Deutsche Forschungsgemeinschaft (521832260). Dr. Jurgens was supported by the Junior Clinical Scientist Fellowship from the Dutch Heart Foundation (grant no. 03-007-2022-0035). Dr. Nauffal is supported by NIH grant 5T32HL007604-35. Dr. Khurshid is supported by NIH grant K23HL169839 and American Heart Association 23CDA1050571. Dr. Lubitz was supported by NIH grants R01HL139731, R01HL157635, and American Heart Association 18SFRN34250007. This work was supported by a grant from the American Heart Association Strategically Focused Research Networks to Dr. Ellinor. This work was funded by a collaboration between the Broad Institute and IBM Research. We would like to thank Mary O’Reilly from the Broad Institute PATTERN Team for contributing to the graphical overview in Fig. 1 . We want to acknowledge the participants and investigators of FinnGen study. The FinnGen project is funded by two grants from Business Finland (HUS 4685/31/2016 and UH 4386/31/2016) and the following industry partners: AbbVie Inc., AstraZeneca UK Ltd, Biogen MA Inc., Bristol Myers Squibb (and Celgene Corporation & Celgene International II Sàrl), Genentech Inc., Merck Sharp & Dohme LCC, Pfizer Inc., GlaxoSmithKline Intellectual Property Development Ltd., Sanofi US Services Inc., Maze Therapeutics Inc., Janssen Biotech Inc, Novartis AG, and Boehringer Ingelheim International GmbH. Following biobanks are acknowledged for delivering biobank samples to FinnGen: Arctic Biobank ( https://www.oulu.fi/medicine/node/207208 ), Auria Biobank ( www.auria.fi/biopankki ), THL Biobank ( www.thl.fi/biobank ), Helsinki Biobank ( www.helsinginbiopankki.fi ), Biobank Borealis of Northern Finland ( https://www.ppshp.fi/Tutkimus-ja-opetus/Biopankki/Pages/Biobank-Borealis-briefly-in-English.aspx ), Finnish Clinical Biobank Tampere ( www.tays.fi/en-US/Research_and_development/Finnish_Clinical_Biobank_Tampere ), Biobank of Eastern Finland ( www.ita-suomenbiopankki.fi/en ), Central Finland Biobank ( www.ksshp.fi/fi-FI/Potilaalle/Biopankki ), Finnish Red Cross Blood Service Biobank ( www.veripalvelu.fi/verenluovutus/biopankkitoiminta ), Terveystalo Biobank ( www.terveystalo.com/fi/Yritystietoa/Terveystalo-Biopankki/Biopankki/ ) and The Finnish Hematology Registry and Clinical Biobank ( https://www.fhrb.fi/ ). All Finnish Biobanks are members of BBMRI.fi infrastructure ( www.bbmri.fi ). Finnish Biobank Cooperative -FINBB ( https://finbb.fi/ ) is the coordinator of BBMRI-ERIC operations in Finland. The Finnish biobank data can be accessed through the Fingenious® services ( https://site.fingenious.fi/en/ ) managed by FINBB. The All of Us Research Program is supported by the National Institutes of Health, Office of the Director: Regional Medical Centers: 1 OT2 OD026549; 1 OT2 OD026554; 1 OT2 OD026557; 1 OT2 OD026556; 1 OT2 OD026550; 1 OT2 OD 026552; 1 OT2 OD026553; 1 OT2 OD026548; 1 OT2 OD026551; 1 OT2 OD026555; IAA #: AOD 16037; Federally Qualified Health Centers: HHSN 263201600085U; Data and Research Center: 5 U2C OD023196; Biobank: 1 U24 OD023121; The Participant Center: U24 OD023176; Participant Technology Systems Center: 1 U24 OD023163; Communications and Engagement: 3 OT2 OD023205; 3 OT2 OD023206; and Community Partners: 1 OT2 OD025277; 3 OT2 OD025315; 1 OT2 OD025337; 1 OT2 OD025276. The All of Us Research Program would not be possible without the partnership of its participants.
Author information
A full list of members and their affiliations appears in the Supplementary Information.
Authors and Affiliations
Division of Cardiology, University of California San Francisco, San Francisco, CA, USA
James P. Pirruccello
Institute for Human Genetics, University of California San Francisco, San Francisco, CA, USA
Bakar Computational Health Sciences Institute, University of California San Francisco, San Francisco, CA, USA
Cardiovascular Genetics Center, University of California San Francisco, San Francisco, CA, USA
Cardiovascular Disease Initiative, Broad Institute of MIT and Harvard, Cambridge, MA, USA
Paolo Di Achille, Joel T. Rämö, Shaan Khurshid, Mahan Nekoui, Sean J. Jurgens, Victor Nauffal, Shinwan Kany, Samuel F. Friedman, Steven A. Lubitz & Patrick T. Ellinor
Data Sciences Platform, Broad Institute of MIT and Harvard, Cambridge, MA, USA
Paolo Di Achille, Samuel F. Friedman, Puneet Batra, Anthony A. Philippakis & Jennifer E. Ho
Cardiovascular Disease Initiative, Broad Institute, Cambridge, MA, USA
Seung Hoan Choi
Institute for Molecular Medicine Finland (FIMM), Helsinki Institute of Life Science (HiLIFE), University of Helsinki, Helsinki, Finland
Joel T. Rämö & Aarno Palotie
Cardiology Division, Massachusetts General Hospital, Boston, MA, USA
Shaan Khurshid, Steven A. Lubitz & Patrick T. Ellinor
Cardiovascular Research Center, Massachusetts General Hospital, Boston, MA, USA
Demoulas Center for Cardiac Arrhythmias, Massachusetts General Hospital, Boston, MA, USA
Shaan Khurshid
Harvard Medical School, Boston, MA, USA
Shaan Khurshid, Mahan Nekoui, Jennifer E. Ho, Steven A. Lubitz & Patrick T. Ellinor
Department of Experimental Cardiology, Amsterdam UMC, University of Amsterdam, Amsterdam, NL, Netherlands
Sean J. Jurgens
Amsterdam Cardiovascular Sciences, Heart Failure & Arrhythmias, University of Amsterdam, Amsterdam, NL, Netherlands
Division of Cardiovascular Medicine, Brigham and Women’s Hospital, Boston, MA, USA
Victor Nauffal
Department of Cardiology, University Heart and Vascular Center Hamburg-Eppendorf, Hamburg, Germany
Shinwan Kany
IBM Research, Cambridge, MA, USA
Department of Biostatistics, Boston University School of Public Health, Boston, MA, USA
Kathryn L. Lunetta
Analytic and Translational Genetics Unit, Massachusetts General Hospital and Harvard Medical School, Boston, MA, USA
Aarno Palotie
Stanley Center for Psychiatric Research, Broad Institute of MIT and Harvard, Boston, MA, USA
CardioVascular Institute, Department of Medicine, Beth Israel Deaconess Medical Center, Boston, MA, USA
Jennifer E. Ho
You can also search for this author in PubMed Google Scholar
- Joel T. Rämö
- & Aarno Palotie
Contributions
P.T.E. and J.P.P. conceived of the study. S. Kurshid, K.L.L. and S.A.L. provided input into the analysis plan. J.P.P. annotated images. J.P.P. trained the deep learning models. P.D. performed surface reconstruction. J.P.P., P.D., S.J. and S.H.C. conducted bioinformatic analyses for UK Biobank data. J.P.P. conducted bioinformatic analyses for All of Us data. FinnGen and A.P. facilitated the FinnGen analyses, and J.T.R. conducted them. J.P.P., S.H.C., J.T.R. and P.T.E. wrote the paper. MN, S. Kany, V.N., K.N., S.F.F., P.B., A.A.P. and J.E.H. provided critical revisions.
Corresponding author
Correspondence to James P. Pirruccello .
Ethics declarations
Competing interests.
Dr. Pirruccello has served as a consultant for Maze Therapeutics. Dr. Lubitz is an employee of Novartis as of July 2022. Dr. Lubitz received sponsored research support from Bristol Myers Squibb, Pfizer, Boehringer Ingelheim, Fitbit, Medtronic, Premier, and IBM, and has consulted for Bristol Myers Squibb, Pfizer, Blackstone Life Sciences, and Invitae. Dr. Ng is employed by IBM Research. Dr. Ho is supported by a grant from Bayer AG focused on machine learning and cardiovascular disease and a research grant from Gilead Sciences. Dr. Ho has received research supplies from EcoNugenics. Dr. Philippakis is employed as a Venture Partner at GV; he is also supported by a grant from Bayer AG to the Broad Institute focused on machine learning for clinical trial design. Dr. Ellinor is supported by a grant from Bayer AG to the Broad Institute focused on the genetics and therapeutics of cardiovascular diseases. Dr. Ellinor has also served on advisory boards or consulted for Bayer AG, Quest Diagnostics, MyoKardia and Novartis. The remaining authors report no disclosures.
Peer review
Peer review information.
Nature Communications thanks the anonymous reviewer(s) for their contribution to the peer review of this work. A peer review file is available.
Additional information
Publisher’s note Springer Nature remains neutral with regard to jurisdictional claims in published maps and institutional affiliations.
Supplementary information
Supplementary information, peer review file, description of additional supplementary files, supplementary data 1, supplementary data 2, supplementary data 3, supplementary data 4, supplementary data 5, supplementary data 6, supplementary data 7, supplementary data 8, supplementary data 9, supplementary data 10, supplementary data 11, reporting summary, source data, source data, rights and permissions.
Open Access This article is licensed under a Creative Commons Attribution 4.0 International License, which permits use, sharing, adaptation, distribution and reproduction in any medium or format, as long as you give appropriate credit to the original author(s) and the source, provide a link to the Creative Commons licence, and indicate if changes were made. The images or other third party material in this article are included in the article’s Creative Commons licence, unless indicated otherwise in a credit line to the material. If material is not included in the article’s Creative Commons licence and your intended use is not permitted by statutory regulation or exceeds the permitted use, you will need to obtain permission directly from the copyright holder. To view a copy of this licence, visit http://creativecommons.org/licenses/by/4.0/ .
Reprints and permissions
About this article
Cite this article.
Pirruccello, J.P., Di Achille, P., Choi, S.H. et al. Deep learning of left atrial structure and function provides link to atrial fibrillation risk. Nat Commun 15 , 4304 (2024). https://doi.org/10.1038/s41467-024-48229-w
Download citation
Received : 03 September 2021
Accepted : 24 April 2024
Published : 21 May 2024
DOI : https://doi.org/10.1038/s41467-024-48229-w
Share this article
Anyone you share the following link with will be able to read this content:
Sorry, a shareable link is not currently available for this article.
Provided by the Springer Nature SharedIt content-sharing initiative
By submitting a comment you agree to abide by our Terms and Community Guidelines . If you find something abusive or that does not comply with our terms or guidelines please flag it as inappropriate.
Quick links
- Explore articles by subject
- Guide to authors
- Editorial policies
Sign up for the Nature Briefing newsletter — what matters in science, free to your inbox daily.


SARAH HOLDER, DO, AND PAULA AMIN, MD
Am Fam Physician. 2024;109(5):398-404
Author disclosure: No relevant financial relationships.
Atrial fibrillation is a supraventricular arrhythmia that increases the risk of stroke and all-cause mortality. It is the most common cardiac dysrhythmia in adults in the primary care setting, and its prevalence increases with age. The U.S. Preventive Services Task Force concluded that there is insufficient evidence to assess the benefits and harms of screening asymptomatic adults older than 50 years for atrial fibrillation. Many patients with atrial fibrillation are asymptomatic, but symptoms can include palpitations, exertional dyspnea, fatigue, and chest pain. Diagnosis is based on history and physical examination findings and should be confirmed with 12-lead electrocardiography or other recording device. The initial evaluation should include transthoracic echocardiography; serum electrolyte levels; complete blood count; and thyroid, kidney, and liver function tests. Stroke risk should be assessed in patients with atrial fibrillation using the CHA 2 DS 2 -VASc score. Warfarin and direct oral anticoagulants reduce the risk of stroke by preventing atrial thrombus formation and subsequent cerebral or systemic emboli. Hemodynamically unstable patients, including those with decompensated heart failure, should be evaluated and treated emergently. Most hemodynamically stable patients should be treated initially with rate control and anticoagulation. Rhythm control, using medications or procedures, should be considered in patients with hemodynamic instability or in some patients based on risk factors and shared decision-making. Electrical cardioversion may be appropriate as first-line rhythm control. Conversion to sinus rhythm with catheter ablation may be considered in patients who are unable or unwilling to take rate or rhythm control medications long-term or if medications have been ineffective.
Atrial fibrillation (AF) is a supraventricular arrhythmia characterized by uncoordinated electrical activation of the atria leading to ineffective atrial contractions. 1 It is the most common cardiac dysrhythmia in adults in the primary care setting. 2 It is estimated that 3 million to 6 million adults in the United States are living with AF. 2 , 3 Older adults are more commonly affected. 1 – 3 Patients with AF have a higher risk of stroke, heart failure, and all-cause mortality. 1 , 2
What Clinical History or Symptoms Suggest AF?
Many patients with AF are asymptomatic, but the most common presenting symptom is palpitations. 1 , 4 Other common symptoms include dyspnea on exertion, fatigue, dizziness, and chest pain. 4 , 5 Patients with symptoms and associated risk factors should be evaluated for AF. The U.S. Preventive Services Task Force concluded that there is insufficient evidence to assess the benefits and harms of screening for AF in asymptomatic adults older than 50 years .
EVIDENCE SUMMARY
Approximately 50% to 87% of patients with AF are asymptomatic. 5 The most common presenting symptom in patients with AF is palpitations, which occur in approximately one-third of symptomatic patients. 1 , 4 , 5 Dyspnea on exertion, fatigue, dizziness, and chest pain are other common symptoms. 5 Patients may be hemodynamically unstable and present with symptoms that include syncope, hypotension, heart failure, and myocardial ischemia due to a very rapid heartbeat caused by AF. 5
The U.S. Preventive Services Task Force concluded that there is insufficient evidence to assess the benefits and harms of screening for AF with electrocardiography (ECG), continuous monitors such as a Holter monitor, or blood pressure monitoring devices in adults older than 50 years. 6 A Cochrane review showed that systematic screening (i.e., screening all patients with ECG) and opportunistic screening (i.e., pulse palpation during an office visit for any reason, followed by ECG if pulse is irregular) are more effective in patients older than 65 years than the regular practice of diagnosing AF based on clinical presentation, but systematic screening is less cost-effective. 7
Age is the most important risk factor for the development of AF, with the greatest risk in those older than 50 years. 3 , 5 Patients older than 70 years are seven times more likely to develop AF than those 50 years or younger. 3 , 4 Other common risk factors include obesity, hypertension, diabetes mellitus, smoking, myocardial infarction, heart failure, and obstructive sleep apnea. 3 , 5
What Is Included in the Initial Evaluation of AF?
Diagnosis of AF is based on history and physical examination findings and should be confirmed with 12-lead ECG or other recording device. 1 , 5 , 8 The initial evaluation should include transthoracic echocardiography; serum electrolyte levels; complete blood count; and thyroid, kidney, and liver function tests. 1
A thorough history should be obtained from patients with suspected AF to characterize the pattern and duration of symptoms; determine potential causes; assess thromboembolic risk; review prior treatment, family history, and associated conditions; and identify reversible risk factors. 1 , 5 The physical examination can verify the diagnosis with palpation or auscultation of an irregularly irregular heartbeat. Detection of an irregularly irregular radial pulse has a sensitivity and specificity of 92% and 82%, respectively, for diagnosing AF. 9
The diagnostic standard for confirming AF is 12-lead ECG 1 , 5 , 10 ( Figure 1 11 ) . Patients with paroxysmal AF who are in normal sinus rhythm at the time of presentation may require ambulatory ECG, such as a Holter monitor. Other options for ambulatory ECG monitoring include post-event, implantable, or external loop recorders. 9 Newer detection methods include handheld ECG devices, wearable wrist devices (e.g., Apple Watch), and automatic blood pressure monitors. 10 One meta-analysis found that the most accurate devices for detecting pulse irregularities caused by AF are non–12-lead ECG (91% sensitivity and 95% specificity) and blood pressure monitors (98% sensitivity and 92% specificity). 9 Smartphones (94% sensitivity and 96% specificity) and smartwatches (94% sensitivity and 93% specificity) have similar diagnostic accuracy in detecting AF. 12

Transthoracic echocardiography should be performed to evaluate for underlying structural heart disease and to assess cardiac function and atrial size. 1 , 5 Transesophageal echocardiography is the most sensitive and specific modality for the evaluation of left atrial thrombus but is not routinely indicated. 1 A new diagnosis of AF should prompt measurements of serum electrolyte levels; complete blood count; and thyroid, kidney, and liver function tests to assess for potential causes and guide treatment. 1 , 5
How Is Stroke Risk Assessed and Reduced With Anticoagulation?
In patients with AF, stroke risk should be assessed with the CHA 2 DS 2 -VASc (congestive heart failure, hypertension, age 75 years or older [doubled], diabetes mellitus, stroke/transient ischemic attack/thromboembolism [doubled], vascular disease, age 65 to 74 years, sex category [female]) score 1 , 2 , 5 , 13 ( Table 1 1 ). Warfarin and direct oral anticoagulants (DOACs) reduce the risk of stroke by preventing atrial thrombus formation and subsequent cerebral or systemic emboli. 1
The CHA 2 DS 2 -VASc score should be used to determine the risk of embolic stroke and the need for anticoagulation. 1 , 5 , 8 , 14 – 16 Males with a score of 2 or greater and females with a score of 3 or greater generally benefit from oral anticoagulation. 1 , 14 – 16 Before initiation of anticoagulation therapy, bleeding risk should be assessed. Traditionally, the HAS-BLED (hypertension, abnormal renal and liver function, stroke, bleeding risk, labile international normalized ratio, elderly [older than 65 years], drug and alcohol use) score is used. 1 , 5 A score of more than 3 indicates high risk of bleeding. A DOAC score has been developed to predict bleeding risk for patients with AF who are using DOACs. Although the DOAC score showed superior performance to the HAS-BLED score in two validation cohorts, it has not been incorporated into AF guidelines. 17 Bleeding risk should be part of the discussion of risks vs. benefits of anticoagulation, and a high risk score alone should not prohibit use. 5 , 16
Warfarin, a vitamin K antagonist, and DOACs, including direct thrombin inhibitors and factor Xa inhibitors, reduce stroke risk in AF. Warfarin is the only oral anticoagulant recommended for the treatment of AF in patients with moderate to severe mitral stenosis or a mechanical heart valve. 5 , 14 , 18 DOACs are recommended over warfarin in patients with AF who are eligible for treatment. 5 , 14 – 16 , 19 , 20 A 2018 Cochrane review concluded that DOACs significantly decreased the risk of stroke, systemic embolic events, major bleeding, and all-cause deaths compared with warfarin. 21 The recommended DOACs for use in nonvalvular AF are apixaban (Eliquis), dabigatran (Pradaxa), edoxaban (Savaysa), and rivaroxaban (Xarelto). 2 , 16 Aspirin is not recommended for reduction of stroke risk in patients with AF. Table 2 lists the pharmacologic properties of anticoagulants used for the prevention of thromboembolism in patients with AF. 11 Left atrial appendage occlusion (e.g., with the Watchman device) is an alternative for patients with an increased risk of stroke who have contraindications to long-term anticoagulation. 16
What Is the Initial Management of AF?
Patients who are hemodynamically unstable, including those with decompensated heart failure, should be evaluated and treated emergently with airway and blood pressure support. Admission to the intensive care unit and cardiology consultation should be considered . 1 , 2 , 5 Most hemodynamically stable patients should be treated initially with rate control and anticoagulation, with consideration of rhythm control for some patients based on risk factors and shared decision-making . 1 , 2 , 5 , 8 , 22 , 23
Hospitalization is indicated for patients with unstable arrhythmia, acute myocardial infarction, altered mental status, decompensated heart failure, hypotension, or intolerable symptoms. 1 , 2 , 5 Nondihydropyridine calcium channel blockers and beta blockers are preferred for achieving rate control. 1 , 5 , 22 Lenient rate control (less than 110 beats per minute at rest) has similar benefits as strict control (less than 80 beats per minute at rest) in patients without symptoms at higher heart rates and with normal left ventricular ejection fraction (LVEF). 1 , 5 , 24 Digoxin may be considered as a second-line agent for rate control, particularly in patients with heart failure. 1 , 5 , 25
Goals of treatment are to control symptoms and to prevent thromboembolism and long-term cardiovascular morbidity from tachycardia. Addressing risk factors such as obesity, sleep apnea, hypertension, diabetes, and alcohol consumption can reduce episodes and symptoms. 5 , 26 – 28
Which Patients Are Good Candidates for Pharmacologic or Electrical Cardioversion?
Rhythm control may be preferred in patients with hemodynamic instability, younger patients, and those with a shorter duration of AF, reduced LVEF, or persistent symptoms despite treatment with rate control . 1 , 2 , 5 , 8 Electrical cardioversion may be appropriate as first-line rhythm control . 8
Select patients with new-onset AF (i.e., less than 48 hours and therefore lower risk of left atrial thrombus) or those with conditions including decompensated heart failure, acute coronary syndrome, hypotension, or acute stroke may benefit from immediate pharmacologic or electrical cardioversion. In hemodynamically stable patients, delaying cardioversion until after 4 weeks of anticoagulation is noninferior to immediate cardioversion for a return to sinus rhythm. 29 However, emergent cardioversion should not be delayed in patients with hemodynamic instability. 16 , 18 , 21
Older studies have found that a rhythm control strategy had similar outcomes as rate control for cardiovascular mortality, stroke, and all-cause mortality. 1 , 2 , 5 , 23 More recent data suggest that cardioversion within 1 year of AF onset may be associated with lower mortality and no increase in adverse effects that require hospitalization. 30 Additionally, antiarrhythmic medication may reduce cardiovascular morbidity and mortality in patients younger than 65 years compared with rate control. 31 A variety of antiarrhythmic medications with equal effectiveness are available; therefore, adverse effects should guide choice.
Class IC antiarrhythmics, including flecainide and propafenone, are contraindicated in patients with ischemic heart disease, structural heart disease, and reduced LVEF. During initiation of these medications, QRS intervals should be monitored continuously. Class III antiarrhythmics, including sotalol and dofetilide, can prolong QT intervals and cause torsades de pointes. Patients must be monitored in the hospital for the first five doses of these medications. ECG should be repeated at least every 6 months. Beta blockers and calcium channel blockers should be used with caution when taken with class III antiarrhythmics due to an increased effect of medication at lower heart rates. Kidney function and potassium and magnesium levels should be monitored in all patients taking antiarrhythmic medications.
Amiodarone is the most prescribed antiarrhythmic in the United States, although it is considered a second-line agent due to its numerous adverse effects, including permanent liver and lung toxicity, thyroid dysfunction, and skin sensitivity. In patients taking amiodarone for longer than 3 months, ECG; thyroid, liver, and pulmonary function tests; chest radiography; and ophthalmologic and skin examinations should be performed. Thyroid and liver functions should be reassessed every 6 months. ECG, chest radiography, ophthalmologic examination, and pulmonary function tests should be repeated every 6 to 12 months. A pill in pocket approach, in which a single dose of propafenone or flecainide is taken with onset of symptoms, can be considered for some patients with infrequent episodes of paroxysmal AF. 1 , 5 , 32 – 34
Which Patients Are Good Candidates for Catheter Ablation?
In younger patients with few comorbidities who have paroxysmal AF and desire rhythm control, catheter ablation may be used as first-line therapy. 8 Conversion to sinus rhythm with catheter ablation may be considered in patients who are unable or unwilling to take long-term medications for rate or rhythm control or if medications have been ineffective. 1 , 5 , 16 , 35
In some patients with AF and heart failure, catheter ablation may improve LVEF. 16 , 35 , 36 Ablation is more effective at reducing recurrence of AF compared with medication alone. 37 , 38 Up to 50% of patients may experience a temporary recurrence of AF within 3 months due to irritation from the procedure. Up to 40% of patients have continued symptoms after 3 months and may require repeat ablation. More than 50% of patients remain in sinus rhythm 3 years after ablation. The procedure is more likely to be successful in younger patients, those with paroxysmal AF, and those without structural heart disease. Patients must be able to tolerate pharmacologic anticoagulation for at least 4 weeks before and 2 months after ablation regardless of their stroke risk. 35 Although ablation may eliminate the need for rate or rhythm control medications, anticoagulation should be continued indefinitely if indicated based on stroke risk. 1 , 16 , 18 , 35
Indications for referral to cardiology include failure of rate control (in which case rhythm control should be considered) or uncontrolled symptomatic AF burden. 1
This article updates previous articles on this topic by Gutierrez and Blanchard 11 ; Gutierrez and Blanchard 39 ; King, et al. 40 ; King, et al. 41 ; Dell’Orfano, et al. 42 ; and Akhtar, et al. 43
Data Sources: A PubMed search was completed in Clinical Queries using the terms atrial fibrillation, rate control, rhythm control, ablation therapy on nonvalvular atrial fibrillation, and anticoagulation therapy for nonvalvular atrial fibrillation. We also searched Essential Evidence Plus, the Cochrane Library, and guidelines on the management of atrial fibrillation from the American Heart Association/American College of Cardiology/ Heart Rhythm Society and European Society of Cardiology. The search focused on randomized controlled trials, systematic reviews, meta-analyses, and reviews. Search dates: April to July 2023, November 2023, and February 2024.
January CT, Wann LS, Alpert JS, et al. 2014 AHA/ACC/HRS guideline for the management of patients with atrial fibrillation: a report of the American College of Cardiology/American Heart Association Task Force on Practice Guidelines and the Heart Rhythm Society [published correction appears in Circulation . 2014; 130(23): e272–274]. Circulation. 2014;130(23):e199-e267.
Frost JL, Campos-Outcalt D, Hoelting D, et al. Atrial fibrillation guideline summary. Ann Fam Med. 2017;15(5):490-491.
Kornej J, Börschel CS, Benjamin EJ, et al. Epidemiology of atrial fibrillation in the 21st century: novel methods and new insights. Circ Res. 2020;127(1):4-20.
Gahungu N, Trueick R, Coopes M, et al. Paroxysmal atrial fibrillation. BMJ. 2021;375:e058568.
Hindricks G, Potpara T, Dagres N, et al.; ESC Scientific Document Group. 2020 ESC guidelines for the diagnosis and management of atrial fibrillation developed in collaboration with the European Association for Cardio-Thoracic Surgery (EACTS) [published corrections appear in Eur Heart J 2021; 42(5): 507, Eur Heart J . 2021; 42(5): 546–547, and Eur Heart J . 2021; 42(40): 4194]. Eur Heart J. 2021;42(5):373-498.
Davidson KW, Barry MJ, Mangione CM, et al. Screening for atrial fibrillation: US Preventive Services Task Force recommendation statement. JAMA. 2022;327(4):360-367.
Moran PS, Teljeur C, Ryan M, et al. Systematic screening for the detection of atrial fibrillation. Cochrane Database Syst Rev. 2016(6):CD009586.
Joglar JA, Chung MK, Armbruster AL, et al. 2023 ACC/AHA/ACCP/HRS guideline for the diagnosis and management of atrial fibrillation: a report of the American College of Cardiology/American Heart Association Joint Committee on Clinical Practice Guidelines [published corrections appear in Circulation . 2024; 149(1): e167 and Circulation . 2024; 149(9): e936]. Circulation. 2024;149(1):e1-e156.
Taggar JS, Coleman T, Lewis S, et al. Accuracy of methods for detecting an irregular pulse and suspected atrial fibrillation: a systematic review and meta-analysis. Eur J Prev Cardiol. 2016;23(12):1330-1338.
Zungsontiporn N, Link MS. Newer technologies for detection of atrial fibrillation. BMJ. 2018;363:k3946.
Gutierrez C, Blanchard DG. Diagnosis and treatment of atrial fibrillation. Am Fam Physician. 2016;94(6):442-452.
Prasitlumkum N, Cheungpasitporn W, Chokesuwattanaskul A, et al. Diagnostic accuracy of smart gadgets/wearable devices in detecting atrial fibrillation: a systematic review and meta-analysis. Arch Cardiovasc Dis. 2021;114(1):4-16.
Rivera-Caravaca JM, Roldán V, Esteve-Pastor MA, et al. Long-term stroke risk prediction in patients with atrial fibrillation: comparison of the ABC-Stroke and CHA 2 DS 2 -VASc scores. J Am Heart Assoc. 2017;6(7):e006490.
Andrade JG, Wells GA, Deyell MW, et al.; EARLY-AF Investigators. Cryoablation or drug therapy for initial treatment of atrial fibrillation. N Engl J Med. 2021;384(4):305-315.
Perry M, Kemmis Betty S, Downes N, et al.; Guideline Committee. Atrial fibrillation: diagnosis and management-summary of NICE guidance. BMJ. 2021;373:n1150.
January CT, Wann LS, Calkins H, et al. 2019 AHA/ACC/HRS focused update of the 2014 AHA/ACC/HRS guideline for the management of patients with atrial fibrillation: a report of the American College of Cardiology/American Heart Association Task Force on Clinical Practice Guidelines and the Heart Rhythm Society [published correction appears in J Am Coll Cardiol . 2019; 74(4): 599]. J Am Coll Cardiol. 2019;74(1):104-132.
Aggarwal R, Ruff CT, Virdone S, et al. Development and validation of the DOAC score: a novel bleeding risk prediction tool for patients with atrial fibrillation on direct-acting oral anticoagulants. Circulation. 2023;148(12):936-946.
Lip GYH, Banerjee A, Boriani G, et al. Antithrombotic therapy for atrial fibrillation: CHEST guideline and expert panel report. Chest. 2018;154(5):1121-1201.
Carnicelli AP, Hong H, Connolly SJ, et al.; COMBINE AF (A Collaboration Between Multiple Institutions to Better Investigate Non-Vitamin K Antagonist Oral Anticoagulant Use in Atrial Fibrillation) Investigators. Direct oral anticoagulants versus warfarin in patients with atrial fibrillation: patient level network meta-analyses of randomized clinical trials with interaction testing by age and sex [published correction appears in Circulation 2022; 145(8): e640]. Circulation. 2022;145(4):242-255.
López-López JA, Sterne JAC, Thom HHZ, et al. Oral anticoagulants for prevention of stroke in atrial fibrillation: systematic review, network meta-analysis, and cost effectiveness analysis [published corrections appear in BMJ . 2017; 359: j5631 and BMJ . 2018; 361: k2295]. BMJ. 2017;359:j5058.
Bruins Slot KM, Berge E. Factor Xa inhibitors versus vitamin K antagonists for preventing cerebral or systemic embolism in patients with atrial fibrillation. Cochrane Database Syst Rev. 2018(3):CD008980.
Olshansky B, Rosenfeld LE, Warner AL, et al.; AFFIRM Investigators. The Atrial Fibrillation Follow-up Investigation of Rhythm Management (AFFIRM) study: approaches to control rate in atrial fibrillation. J Am Coll Cardiol. 2004;43(7):1201-1208.
Van Gelder IC, Hagens VE, Bosker HA, et al.; Rate Control versus Electrical Cardioversion for Persistent Atrial Fibrillation Study Group. A comparison of rate control and rhythm control in patients with recurrent persistent atrial fibrillation. N Engl J Med. 2002;347(23):1834-1840.
Van Gelder IC, Groenveld HF, Crijns HJGM, et al.; RACE II Investigators. Lenient versus strict rate control in patients with atrial fibrillation. N Engl J Med. 2010;362(15):1363-1373.
Kotecha D, Bunting KV, Gill SK, et al.; Rate Control Therapy Evaluation in Permanent Atrial Fibrillation (RATE-AF) Team. Effect of digoxin vs bisoprolol for heart rate control in atrial fibrillation on patient-reported quality of life: the RATE-AF randomized clinical trial. JAMA. 2020;324(24):2497-2508.
Chung MK, Eckhardt LL, Chen LY, et al.; American Heart Association Electrocardiography and Arrhythmias Committee and Exercise, Cardiac Rehabilitation, and Secondary Prevention Committee of the Council on Clinical Cardiology; Council on Arteriosclerosis, Thrombosis and Vascular Biology; Council on Cardiovascular and Stroke Nursing; and Council on Lifestyle and Cardiometabolic Health. Lifestyle and risk factor modification for reduction of atrial fibrillation: a scientific statement from the American Heart Association. Circulation. 2020;141(16):e750-e772.
Abed HS, Wittert GA, Leong DP, et al. Effect of weight reduction and cardiometabolic risk factor management on symptom burden and severity in patients with atrial fibrillation: a randomized clinical trial. JAMA. 2013;310(19):2050-2060.
Voskoboinik A, Kalman JM, De Silva A, et al. Alcohol abstinence in drinkers with atrial fibrillation. N Engl J Med. 2020;382(1):20-28.
Pluymaekers NAHA, Dudink EAMP, Luermans JGLM, et al.; RACE 7 ACWAS Investigators. Early or delayed cardioversion in recent-onset atrial fibrillation. N Engl J Med. 2019;380(16):1499-1508.
Kirchhof P, Camm AJ, Goette A, et al.; EAST-AFNET 4 Trial Investigators. Early rhythm-control therapy in patients with atrial fibrillation. N Engl J Med. 2020;383(14):1305-1316.
Chen S, Yin Y, Krucoff MW. Should rhythm control be preferred in younger atrial fibrillation patients?. J Interv Card Electrophysiol. 2012;35(1):71-80.
Bhatia S, Sugrue A, Asirvatham S. Atrial fibrillation: beyond rate control. Mayo Clin Proc. 2018;93(3):373-380.
Tseng AS, Kowlgi GN, DeSimone CV. Antiarrhythmic drugs for atrial fibrillation in the outpatient setting: common clinical scenarios and pearls for the primary care clinician. Mayo Clin Proc. 2021;96(8):2230-2242.
Zimetbaum P. Antiarrhythmic drug therapy for atrial fibrillation. Circulation. 2012;125(2):381-389.
Calkins H, Hindricks G, Cappato R, et al. 2017 HRS/EHRA/ECAS/APHRS/SOLAECE expert consensus statement on catheter and surgical ablation of atrial fibrillation: executive summary. J Arrhythm. 2017;33(5):369-409.
Marrouche NF, Brachmann J, Andresen D, et al.; CASTLE-AF Investigators. Catheter ablation for atrial fibrillation with heart failure. N Engl J Med. 2018;378(5):417-427.
Chen HS, Wen JM, Wu SN, et al. Catheter ablation for paroxysmal and persistent atrial fibrillation. Cochrane Database Syst Rev. 2012(4):CD007101.
Nyong J, Amit G, Adler AJ, et al. Efficacy and safety of ablation for people with non-paroxysmal atrial fibrillation. Cochrane Database Syst Rev. 2016(11):CD012088.
Gutierrez C, Blanchard DG. Atrial fibrillation: diagnosis and treatment. Am Fam Physician. 2011;83(1):61-68.
King DE, Dickerson LM, Sack JL. Acute management of atrial fibrillation: part I. Rate and rhythm control. Am Fam Physician. 2002;66(2):249-256.
King DE, Dickerson LM, Sack JL. Acute management of atrial fibrillation: part II. Prevention of thromboembolic complications. Am Fam Physician. 2002;66(2):261-264.
Dell’Orfano JT, Luck JC, Wolbrette DL, et al. Drugs for conversion of atrial fibrillation. Am Fam Physician. 1998;58(2):471-480.
Akhtar W, Reeves WC, Movahed A. Indications for anticoagulation in atrial fibrillation [published correction appears in Am Fam Physician . 1999; 59(5): 1122]. Am Fam Physician. 1998;58(1):130-136.
Continue Reading
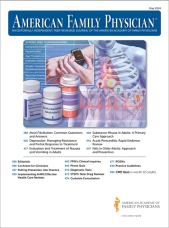
More in AFP
Copyright © 2024 by the American Academy of Family Physicians.
This content is owned by the AAFP. A person viewing it online may make one printout of the material and may use that printout only for his or her personal, non-commercial reference. This material may not otherwise be downloaded, copied, printed, stored, transmitted or reproduced in any medium, whether now known or later invented, except as authorized in writing by the AAFP. See permissions for copyright questions and/or permission requests.
Copyright © 2024 American Academy of Family Physicians. All Rights Reserved.
Log in using your username and password
- Search More Search for this keyword Advanced search
- Latest content
- Publish with us
- About the journal
- Meet the editors
- Specialist reviews
- BMJ Journals More You are viewing from: Google Indexer
You are here
- Volume 3, Issue 1
- Regular use of fish oil supplements and course of cardiovascular diseases: prospective cohort study
- Article Text
- Article info
- Citation Tools
- Rapid Responses
- Article metrics

- Ge Chen 1 ,
- Zhengmin (Min) Qian 2 ,
- Junguo Zhang 1 ,
- Shiyu Zhang 1 ,
- http://orcid.org/0000-0002-7003-6565 Zilong Zhang 1 ,
- Michael G Vaughn 3 ,
- Hannah E Aaron 2 ,
- Chuangshi Wang 4 ,
- Gregory YH Lip 5 , 6 and
- http://orcid.org/0000-0002-3643-9408 Hualiang Lin 1
- 1 Department of Epidemiology , Sun Yat-Sen University , Guangzhou , China
- 2 Department of Epidemiology and Biostatistics, College for Public Health and Social Justice , Saint Louis University , Saint Louis , Missouri , USA
- 3 School of Social Work, College for Public Health and Social Justice , Saint Louis University , Saint Louis , Missouri , USA
- 4 Medical Research and Biometrics Centre , Fuwai Hospital, National Centre for Cardiovascular Diseases, Peking Union Medical College , Beijing , China
- 5 Liverpool Centre for Cardiovascular Science , University of Liverpool and Liverpool Heart and Chest Hospital , Liverpool , UK
- 6 Department of Clinical Medicine , Aalborg University , Aalborg , Denmark
- Correspondence to Dr Hualiang Lin, Department of Epidemiology, Sun Yat-Sen University, Guangzhou, Guangdong 510080, China; linhualiang{at}mail.sysu.edu.cn
Objective To examine the effects of fish oil supplements on the clinical course of cardiovascular disease, from a healthy state to atrial fibrillation, major adverse cardiovascular events, and subsequently death.
Design Prospective cohort study.
Setting UK Biobank study, 1 January 2006 to 31 December 2010, with follow-up to 31 March 2021 (median follow-up 11.9 years).
Participants 415 737 participants, aged 40-69 years, enrolled in the UK Biobank study.
Main outcome measures Incident cases of atrial fibrillation, major adverse cardiovascular events, and death, identified by linkage to hospital inpatient records and death registries. Role of fish oil supplements in different progressive stages of cardiovascular diseases, from healthy status (primary stage), to atrial fibrillation (secondary stage), major adverse cardiovascular events (tertiary stage), and death (end stage).
Results Among 415 737 participants free of cardiovascular diseases, 18 367 patients with incident atrial fibrillation, 22 636 with major adverse cardiovascular events, and 22 140 deaths during follow-up were identified. Regular use of fish oil supplements had different roles in the transitions from healthy status to atrial fibrillation, to major adverse cardiovascular events, and then to death. For people without cardiovascular disease, hazard ratios were 1.13 (95% confidence interval 1.10 to 1.17) for the transition from healthy status to atrial fibrillation and 1.05 (1.00 to 1.11) from healthy status to stroke. For participants with a diagnosis of a known cardiovascular disease, regular use of fish oil supplements was beneficial for transitions from atrial fibrillation to major adverse cardiovascular events (hazard ratio 0.92, 0.87 to 0.98), atrial fibrillation to myocardial infarction (0.85, 0.76 to 0.96), and heart failure to death (0.91, 0.84 to 0.99).
Conclusions Regular use of fish oil supplements might be a risk factor for atrial fibrillation and stroke among the general population but could be beneficial for progression of cardiovascular disease from atrial fibrillation to major adverse cardiovascular events, and from atrial fibrillation to death. Further studies are needed to determine the precise mechanisms for the development and prognosis of cardiovascular disease events with regular use of fish oil supplements.
- Health policy
- Nutritional sciences
- Public health
Data availability statement
Data are available upon reasonable request. UK Biobank is an open access resource. Bona fide researchers can apply to use the UK Biobank dataset by registering and applying at http://ukbiobank.ac.uk/register-apply/ .
This is an open access article distributed in accordance with the Creative Commons Attribution 4.0 Unported (CC BY 4.0) license, which permits others to copy, redistribute, remix, transform and build upon this work for any purpose, provided the original work is properly cited, a link to the licence is given, and indication of whether changes were made. See: https://creativecommons.org/licenses/by/4.0/ .
https://doi.org/10.1136/bmjmed-2022-000451
Statistics from Altmetric.com
Request permissions.
If you wish to reuse any or all of this article please use the link below which will take you to the Copyright Clearance Center’s RightsLink service. You will be able to get a quick price and instant permission to reuse the content in many different ways.
WHAT IS ALREADY KNOWN ON THIS TOPIC
Findings of the effects of omega 3 fatty acids or fish oil on the risk of cardiovascular disease are controversial
Most previous studies focused on one health outcome and did not characterise specific cardiovascular disease outcomes (eg, atrial fibrillation, myocardial infarction, stroke, heart failure, and major adverse cardiovascular events)
Whether fish oil could differentially affect the dynamic course of cardiovascular diseases, from atrial fibrillation to major adverse cardiovascular events, to other specific cardiovascular disease outcomes, or even to death, is unclear
WHAT THIS STUDY ADDS
In people with no known cardiovascular disease, regular use of fish oil supplements was associated with an increased relative risk of atrial fibrillation and stroke
In people with known cardiovascular disease, the beneficial effects of fish oil supplements were seen on transitions from atrial fibrillation to major adverse cardiovascular events, atrial fibrillation to myocardial infarction, and heart failure to death
HOW THIS STUDY MIGHT AFFECT RESEARCH, PRACTICE, OR POLICY
Regular use of fish oil supplements might have different roles in the progression of cardiovascular disease
Further studies are needed to determine the precise mechanisms for the development and prognosis of cardiovascular disease events with regular use of fish oil supplements
Introduction
Cardiovascular disease is the leading cause of death worldwide, accounting for about one sixth of overall mortality in the UK. 1 2 Fish oil, a rich source of omega 3 fatty acids, containing eicosapentaenoic acid and docosahexaenoic acid, has been recommended as a dietary measure to prevent cardiovascular disease. 3 The UK National Institute for Health and Care Excellence recommends that people with or at high risk of cardiovascular disease consume at least one portion of oily fish a week, and the use of fish oil supplements has become popular in the UK and other western countries in recent years. 4 5
Although some epidemiological and clinical studies have assessed the effect of omega 3 fatty acids or fish oil on cardiovascular disease and its risk factors, the findings are controversial. The Agency for Healthcare Research and Quality systematically reviewed 37 observational studies and 61 randomised controlled trials, and found evidence indicating the beneficial effects of higher consumption of fish oil supplements on ischaemic stroke, whereas no beneficial effect was found for atrial fibrillation, major adverse cardiovascular events, myocardial infarction, total stroke, or all cause death. 6 In contrast, the Reduction of Cardiovascular Events with Icosapent Ethyl-Intervention Trial (REDUCE-IT) reported a decreased risk of major adverse cardiovascular events with icosapent ethyl in patients with raised levels of triglycerides, regardless of the use of statins. 7 Most of these findings, however, tended to assess the role of fish oil at a certain stage of cardiovascular disease. For example, some studies restricted the study population to people with a specific cardiovascular disease or at a high risk of cardiovascular disease, 8 9 whereas others evaluated databases of generally healthy populations. 10 All of these factors might preclude direct comparison of the effects of omega 3 fatty acids on atrial fibrillation events or on further deterioration of cardiovascular disease. Few studies have fully characterised specific cardiovascular disease outcomes or accounted for differential effects based on the complex disease characteristics of participants. Hence, in this study, we hypothesised that fish oil supplements might have harmful, beneficial, or no effect on different cardiovascular disease events in patients with varying health conditions.
Most previous studies on the association between fish oil and cardiovascular diseases generally focused on one health outcome. Also, no study highlighted the dynamic progressive course of cardiovascular diseases, from healthy status (primary stage), to atrial fibrillation (secondary stage), major adverse cardiovascular events (tertiary stage), and death (end stage). Clarifying this complex pathway in relation to the detailed progression of cardiovascular diseases would provide substantial insights into the prevention or treatment of future disease at critical stages. Whether fish oil could differentially affect the dynamic course of cardiovascular disease (ie, from atrial fibrillation to major adverse cardiovascular events, to other specific cardiovascular disease outcomes, or even to death) is unclear.
To deal with this evidence gap, we conducted a longitudinal cohort study to estimate the associations between fish oil supplements and specific clinical cardiovascular disease outcomes, including atrial fibrillation, major adverse cardiovascular events, and all cause death in people with no known cardiovascular disease or at high risk of cardiovascular disease for the purpose of primary prevention. We also assessed the modifying effects of fish oil supplements on the disease process, from atrial fibrillation to other outcomes, in people with known cardiovascular disease for the purpose of secondary prevention.
The UK Biobank is a community based cohort study with more than half a million UK inhabitants aged 40-69 years at recruitment. 11–13 Participants were invited to participate in this study if they were registered with the NHS and lived within 35 km of one of 22 Biobank assessment centres. Between 1 March 2006 and 31 July 2010, a baseline survey was conducted, based on a touch screen questionnaire and face-to-face interviews, to collect detailed personal, socioeconomic, and lifestyle characteristics, and information on diseases. 11–13
We excluded patients who had a diagnosis of atrial fibrillation (n=8326), heart failure (n=2748), myocardial infarction (n=11 949), stroke (n=7943), or cancer (n=48 624) at baseline; who withdrew from the study during follow-up (n=1299); or who had incomplete or outlier data for the main information (n=11 748). Because we focused only on a specific sequence of progression of cardiovascular disease (ie, from healthy status to atrial fibrillation, to major adverse cardiovascular events, and then to death), we excluded 1983 participants with other transition patterns. The remaining 415 737 participants were included in this analysis ( figure 1 ).
- Download figure
- Open in new tab
- Download powerpoint
Flowchart of selection of participants in study. The count of diagnosed diseases does not equate to the total number of individuals, because each person could have multiple diagnoses
Determining use of fish oil supplements
Information on regular use of fish oil supplements was collected from a self-reported touchscreen questionnaire during the baseline survey. 14 15 Each participant was asked whether they regularly used any fish oil supplement. Trained staff conducted a verbal interview with participants, asking if they were currently receiving treatments or taking any medicines, including omega 3 or fish oil supplements. Based on this information, we classified participants as regular users of fish oil supplements and non-users.
Follow-up and outcomes
Participants were followed up from the time of recruitment to death, loss to follow-up, or the end date of follow-up (31 March 2021), whichever came first. Incident cases of interest, including atrial fibrillation, heart failure, stroke, and myocardial infarction, were identified by linkage to death registries, primary care records, and hospital inpatient records. 11 Information on deaths was obtained from death registries of the NHS Information Centre, for participants in England and Wales, and from the NHS Central Register Scotland, for participants in Scotland. 11 Outcomes were defined by a three character ICD-10 (international classification of diseases, 10th revision) code. In this study, atrial fibrillation was defined by ICD-10 code I48, and major adverse cardiovascular events was determined by a combination of heart failure (I50, I11.0, I13.0, and I13.2), stroke (I60-I64), and myocardial infarction (I21, I22, I23, I24.1, and I25.2) codes.
We collected baseline data on age (<65 years and ≥65 years), sex (men and women), ethnic group (white and non-white), Townsend deprivation index (with a higher score indicating higher levels of deprivation), smoking status (never, previous, and current smokers), and alcohol consumption (never, previous, and current drinkers). Data for sex were taken from information in UK Biobank rather than from patient reported gender. Baseline dietary data were obtained from a dietary questionnaire completed by the patient or by an interviewer. The questionnaire was established for each nation (ie, England, Scotland, and Wales) to assess an individual's usual food intake (oily fish, non-oily fish, vegetables, fruit, and red meat). Diabetes mellitus was defined by ICD-10 codes E10-E14, self-reported physician's diagnosis, self-reported use of antidiabetic drugs, or haemoglobin A1c level ≥6.5% at baseline. Hypertension was defined by ICD-10 code I10 or I15, self-reported physician's diagnosis, self-reported use of antihypertensive drugs, or measured systolic and diastolic blood pressure ≥130/85 mm Hg at baseline. Information on other comorbidities (obesity (ICD-10 code E66), chronic obstructive pulmonary disease (J44), and chronic renal failure (N18)) was extracted from the first occurrence (UKB category ID 1712). Information on the use of drugs, including antihypertensive drugs, antidiabetic drug, and statins, was extracted from treatment and drug use records. Biochemistry markers were measured immediately at the central laboratory from serum samples collected at baseline. Binge drinking was defined as consumption of ≥6 standard drinks/day for women or ≥8 standard drinks/day for men. Detailed information on alcohol consumption and binge drinking in the UK Biobank was reported previously. 16
Statistical analysis
Characteristics of participants are summarised as number (percentages) for categorical variables and mean (standard deviation (SD)) for continuous variables. Comparisons between regular users of fish oil supplements and non-users were made with the χ 2 test or Student's t test.
We used a multi-state regression model to assess the role of regular use of fish oil supplements in the temporal disease progression from healthy status to atrial fibrillation, to major adverse cardiovascular events, and subsequently to death. The multi-state model is an extension of competing risks survival analysis. 17–19 The model allows simultaneous estimation of the role of risk factors in transitions from a healthy state to atrial fibrillation (transition A), healthy state to major adverse cardiovascular events (transition B), healthy state to death (transition C), atrial fibrillation to major adverse cardiovascular events (transition D), atrial fibrillation to death (transition E), and major adverse cardiovascular events to death (transition F) (transition pattern I, figure 2 ). The focus on these six transitions rather than on all possible health state transitions was preplanned and evidence based. If participants entered different states on the same date, we used the date of the theoretically previous state as the entry date of the latter state minus 0.5 days.
Numbers of participants in transition pattern I, from baseline to atrial fibrillation, major adverse cardiovascular events, and death
We further examined the effects of regular use of fish oil supplements on other pathways. For example, we divided major adverse cardiovascular events into three individual diseases (heart failure, stroke, and myocardial infarction), resulting in three independent pathways (transition patterns II, III, and IV, online supplemental figures S1–S3 ). All models were adjusted for age, sex, ethnic group, Townsend deprivation index, consumption of oily fish, consumption of non-oily fish, smoking status, alcohol consumption, obesity, hypertension, diabetes mellitus, chronic obstructive pulmonary disease, chronic renal failure, and use of statins, antidiabetic drugs, and antihypertensive drugs.
Supplemental material
We conducted several sensitivity analyses for the multi-state analyses of transition pattern A: additionally adjusting for setting (urban and rural), body mass index (underweight, normal, overweight, and obese), and physical activity (low, moderate, and high) in the model; adjusting for binge drinking rather than alcohol consumption; additionally adjusting for other variables of dietary intake (consumption of vegetables, fruit, and red meat); calculating participants' entry date into the previous state with different time intervals (0.5 years, one year, and two years); excluding participants who entered different states on the same date; excluding events occurring in the first two years of follow-up; restricting the follow-up date to 31 March 2020 to evaluate the influence of the covid-19 pandemic; and the use of the inverse probability weighted method to deal with biases between the regular users and non-users of fish oil supplements. Also, we conducted grouped analyses for sex, age group, ethnic group, smoking status, consumption of oily fish, consumption of non-oily fish, hypertension, and drug use, to examine effect modification. The interactions were tested with the likelihood ratio test. All analyses were carried out with R software (version 4.0.3), and the multi-model analysis was performed with the mstate package. A two tailed P value <0.05 was considered significant.
Patient and public involvement
Patients and/or the public were not involved in the design, or conduct, or reporting, or dissemination plans of this research. Participants were involved in developing the ethics and governance framework for UK Biobank and have been engaged in the progress of UK Biobank through follow-up questionnaires and additional assessment visits. UK Biobank keeps participants informed of all research output through the study website ( https://www.ukbiobank.ac.uk/explore-your-participation ), participant events, and newsletters.
A total of 415 737 participants (mean age 55.9 (SD 8.1) years; 55% women), aged 40-69 years, were analysed, and 31.4% (n=1 30 365) of participants reported regular use of fish oil supplements at baseline ( figure 1 ). Table 1 shows the characteristics of regular users (n=130 365) and non-users (n=285 372) of fish oil supplements. In the group of regular users of fish oil supplements, we found higher proportions of elderly people (22.6% v 13.9%), white people (95.1% v 94.2%), and women (57.6% v 53.9%), and higher consumption of alcohol (93.1% v 92.0%), oily fish (22.1% v 15.4%), and non-oily fish (18.0% v 15.4%) than non-users. The Townsend deprivation index (mean −1.5 (SD 3.0) v −1.3 (3.0)) and the proportion of current smokers (8.1% v 11.4%) were lower in regular users of fish oil supplements. Online supplemental table S1 provides more details on patient characteristics and online supplemental table S2 compares the basic characteristics of included and excluded people.
- View inline
Baseline characteristics of study participants grouped by use of fish oil supplements
Over a median follow-up time of of 11.9 years, 18 367 participants had atrial fibrillation (transition A) and 17 826 participants had major adverse cardiovascular events (transition B); 14 902 participants died without having atrial fibrillation or major adverse cardiovascular events (transition C). Among patients with incident atrial fibrillation, 4810 developed major adverse cardiovascular events (transition D) and 1653 died (transition E). Among patients with incident major adverse cardiovascular events, 5585 died during follow-up (transition F, figure 2 ). In separate analyses for individual diseases (transition patterns II, III, and IV, online supplemental figures S1–S3 ), in patients with atrial fibrillation, 3085 developed heart failure, 1180 had a stroke, and 1415 had a myocardial infarction. During follow-up, 2436, 2088, and 2098 deaths occurred in patients with heart failure, stroke, and myocardial infarction, respectively.
Multi-state regression results
Table 2 shows the different roles of regular use of fish oil supplements in transitions from healthy status to atrial fibrillation, to major adverse cardiovascular events, and then to death. For individuals in the primary stage (healthy status), we found that the use of fish oil supplements had a harmful effect on the transition from health to atrial fibrillation, with an adjusted hazard ratio of 1.13 (95% CI 1.10 to 1.17, transition A). The hazard ratio for transition B (from health to major adverse cardiovascular events) was 1.00 (95% CI 0.97 to 1.04) and for transition C (from health to death) was 0.98 (0.95 to 1.02).
Hazard ratios (95% confidence intervals) for each transition, for different transition patterns for progressive cardiovascular disease by regular use of fish oil supplements
For individuals in the secondary stage (atrial fibrillation) at the beginning of the study, regular use of fish oil supplements decreased the risk of major adverse cardiovascular events (transition D, hazard ratio 0.92, 95% CI 0.87 to 0.98), and had a borderline protective effect on the transition from atrial fibrillation to death (transition E, 0.91, 0.82 to 1.01). For transition F, from major adverse cardiovascular events to death, after adjusting for covariates, the hazard ratio was 0.99 (0.94 to 1.06, transition pattern I, table 2 ).
We divided major adverse cardiovascular events into three individual diseases (ie, heart failure, stroke, and myocardial infarction) and found that regular use of fish oil supplements was marginally associated with an increased risk of stroke in people with a healthy cardiovascular state (hazard ratio 1.05, 95% CI 1.00 to 1.11), whereas a protective effect was found in transitions from healthy cardiovascular states to heart failure (0.92, 0.86 to 0.98). For patients with atrial fibrillation, we found that the beneficial effects of regular use of fish oil supplements were for transitions from atrial fibrillation to myocardial infarction (0.85, 0.76 to 0.96), and from atrial fibrillation to death (0.88, 0.81 to 0.95) for transition pattern IV. For patients with heart failure, we found a protective effect of regular use of fish oil supplements on the risk of mortality (0.91, 0.84 to 0.99) (transition patterns II, III, and IV, table 2 ).
Stratified and sensitivity analyses
We found that age, sex, smoking, consumption of non-oily fish, prevalent hypertension, and use of statins and antihypertensive drugs modified the associations between regular use of fish oil supplements and the transition from healthy states to atrial fibrillation ( online supplemental figure S4 ). We found that the association between regular use of fish oil supplements and risk of transition from healthy states to major adverse cardiovascular events was greater in women (hazard ratio 1.06, 95% CI 1.00 to 1.11, P value for interaction=0.005) and non-smoking participants (1.06, 1.06 to 1.11, P value for interaction=0.001) ( online supplemental figure S4 ). The protective effect of regular use of fish oil supplements on the transition from healthy states to death was greater in men (hazard ratio 0.93, 95% CI 0.89 to 0.98, P value for interaction=0.003) and older participants (0.91, 0.86 to o 0.96, P value for interaction=0.002) ( online supplemental figures S5 and S6 ). The results were not substantially changed in the sensitivity analyses ( online supplemental table S3 ).
Principal findings
Our study characterised the regular use of fish oil supplements on the progressive course of cardiovascular disease, from a healthy state (primary stage), to atrial fibrillation (secondary stage), major adverse cardiovascular events (tertiary stage), and death (end stage). In this prospective analysis of more than 400 000 UK adults, we found that regular use of fish oil supplements could have a differential role in the progression of cardiovascular disease. For people with a healthy cardiovascular profile, regular use of fish oil supplements, a choice of primary prevention, was associated with an increased risk of atrial fibrillation. For participants with a diagnosis of atrial fibrillation, however, regular use of fish oil supplements, as secondary prevention, had a protective effect or no effect on transitions from atrial fibrillation to major adverse cardiovascular events, atrial fibrillation to death, and major adverse cardiovascular events to death. When we divided major adverse cardiovascular events into three individual diseases (ie, heart failure, stroke, and myocardial infarction), we found associations that could suggest a mildly harmful effect between regular use of fish oil supplements and transitions from a healthy cardiovascular state to stroke, whereas potential beneficial associations were found between regular use of fish oil supplements and transitions from atrial fibrillation to myocardial infarction, atrial fibrillation to death, and heart failure to death.
Comparison with other studies
Primary prevention.
The cardiovascular benefits of regular use of fish oil supplements have been examined in numerous studies but the results are controversial. Extending previous reports, our study estimated the associations between regular use of fish oil supplements and specific clinical cardiovascular disease outcomes in people with no known cardiovascular disease. Our findings are in agreement with the results of several previous randomised controlled trials and meta-analyses. The Long-Term Outcomes Study to Assess Statin Residual Risk with Epanova in High Cardiovascular Risk Patients with Hypertriglyceridaemia (STRENGTH) reported that consumption of 4 g/day of marine omega 3 fatty acids was associated with a 69% higher risk of new onset atrial fibrillation in people at high risk of cardiovascular disease. 20 A meta-analysis of seven randomised controlled trials showed that users of marine omega 3 fatty acids supplements had a higher risk of atrial fibrillation events, with a hazard ratio of 1.25 (95% CI 1.07 to 1.46, P=0.013). 21 The Vitamin D and Omega-3 Trial (VITAL Rhythm study), a large trial of omega 3 fatty acids for the primary prevention of cardiovascular disease in adults aged ≥50 years, however, found no effects on incident atrial fibrillation, major adverse cardiovascular events, or cardiovascular disease mortality among those treated with 840 mg/day of marine omega 3 fatty acids compared with placebo. 10 22
One possible explanation for the inconsistent results in these studies is that adverse effects might be related to dose and composition. Higher doses of omega 3 fatty acids used in previous studies might have had an important role in causing an adverse effect on atrial fibrillation. 21 One study found that high concentrations of fish oil altered cell membrane properties and inhibited Na-K-ATPase pump activity, whereas a low concentration of fish oil minimised peroxidation potential and optimised activity. 23 In another study, individuals with atrial fibrillation or flutter had higher percentages of total polyunsaturated fatty acids, and n-3 and n-6 polyunsaturated fatty acids, on red blood cell membranes than healthy controls. 24
In terms of composition of omega 3 fatty acids, a recent meta-analysis showed that eicosapentaenoic acid alone can be more effective at reducing the risk of cardiovascular disease than the combined effect of eicosapentaenoic acid and docosahexaenoic acid. 25 Similar outcomes were reported in the INSPIRE study, which showed that higher levels of docosahexaenoic acid reduced the cardiovascular benefits of eicosapentaenoic acid when given as a combination. 26 Another possible explanation is that age, sex, ethnic group, smoking status, dietary patterns, and use of statins and antidiabetic drugs by participants might modify the effects of regular use of fish oil supplements on cardiovascular disease events. Despite these differences in risk estimates, our findings do not support the use of fish oil or omega 3 fatty acid supplements for the primary prevention of incident atrial fibrillation or other specific clinical cardiovascular disease events in generally healthy individuals. Caution might be warranted when fish oil supplements are used for primary prevention because of the uncertain cardiovascular benefits.
Secondary prevention
Our large scale cohort study assessed the role of regular use of fish oil supplements on the disease process, from atrial fibrillation to more serious cardiovascular disease stages, to death, in people with known cardiovascular disease. Contrary to the observations for primary prevention, we found associations that could suggest beneficial effects between regular use of fish oil supplements and most cardiovascular disease transitions. No associations were found between regular use of fish oil supplements and transitions from atrial fibrillation to death, or from major adverse cardiovascular events to death.
Consistent with our hypothesis, the Gruppo Italiano per lo Studio della Sopravvivenza nell'Infarto Miocardico (GISSI) Prevenzione study reported an association between administration of low dose prescriptions of n-3 polyunsaturated fatty acids and reduced cardiovascular events in patients with recent myocardial infarction. 27 A meta-analysis of 16 randomised controlled trials also reported a tendency towards a greater beneficial effect for secondary prevention in patients with cardiovascular disease. 28 Why patients with previous atrial fibrillation benefit is unclear. These findings indicate that triglyceride independent effects of omega 3 fatty acids might in part be responsible for the benefits in cardiovascular disease seen in previous trials. 29–31 No proven biological mechanism for this explanation exists, however, and the dose and formulation of omega 3 fatty acids used in clinical practice are not known.
For the disease process, from cardiovascular disease to death, our findings are consistent with the results of secondary prevention trials of omega 3 fatty acids, which have mostly shown a weak or neutral preventive effect in all cause mortality with oil fish supplements. The GISSI heart failure trial (GISSI-HF), conducted in 6975 patients with chronic heart failure, reported that supplemental omega 3 fatty acids reduced the risk of all cause mortality by 9% (hazard ratio 0.91, 95% CI 0.833 to 0.998, P=0.041). 32 Zelniker et al showed that omega 3 fatty acids were inversely associated with a lower incidence of sudden cardiac death in patients with non-ST segment elevation acute coronary syndrome. 33 A meta-analysis found that use of omega 3 supplements of ≤1 capsule/day was not associated with all cause mortality, but among participants with a risk of cardiovascular disease, taking a higher dose was associated with a reduction in cardiac death and sudden death. 28 Individuals who might benefit the most from fish oil or omega 3 fatty acid supplements are possibly more vulnerable individuals, such as those with previous cardiovascular diseases and those who can no longer live in the community. How fish oil supplements stop further deterioration of cardiovascular disease is unclear, but the theory that supplemental omega 3 fatty acids might protect the coronary artery is biologically plausible, suggesting that omega 3 fatty acids have anti-inflammatory and anti-hypertriglyceridaemia effects, contributing to a reduction in thrombosis and improvement in endothelial function. 34–41 Nevertheless, the effects of omega 3 fatty acids vary according to an individual's previous use of statins, which might partly explain the different effects of fish oil supplements in people with and without cardiovascular disease.
Many studies of omega 3 fatty acids, including large scale clinical trials and meta-analyses, have not produced entirely consistent results. 21 25 42 Our study mainly explored the varied potential effects of regular use of fish oil supplements on progression of cardiovascular disease, offering an initial overview of this ongoing discussion. Our findings suggest caution in the use of fish oil supplements for primary prevention because of the uncertain cardiovascular benefits and adverse effects. Further studies are needed to determine whether potential confounders modify the effects of oil fish supplements and the precise mechanisms related to the development and prognosis of cardiovascular disease events.
Strengths and limitations of this study
The strengths of our study were the large sample size, long follow-up period, which allowed us to analyse clinically diagnosed incident diseases, and complete data on health outcomes. Another strength was our analytical strategy. The multi-state model gives less biased estimates than the conventional Cox model, and distinguished the effect of regular use of fish oil supplements on each transition in the course of cardiovascular disease.
Our study had some limitations. Firstly, as an observational study, no causal relations can be drawn from our findings. Secondly, although we adjusted for multiple covariates, residual confounding could still exist. Thirdly, information on dose and formulation of the fish oil supplements was not available in this study, so we could not evaluate potential dose dependent effects or differentiate between the effects of different fish oil formulations. Fourthly, the use of hospital inpatient data for determining atrial fibrillation events could have excluded some events triggered by acute episodes, such as surgery, trauma, and similar conditions, resulting in underestimation of the true risk because undiagnosed atrial fibrillation is a common occurrence. 43 Fifthly, most of the participants in this study were from the white ethnic group and whether the findings can be generalised to other ethnic groups is not known. Finally, our study did not consider behavioural changes in populations with different cardiovascular profiles because of limited information, and variations in outcomes for different cardiovascular states merits further exploration.
Conclusions
This large scale prospective study of a UK cohort suggested that regular use of fish oil supplements might have differential roles in the course of cardiovascular diseases. Regular use of fish oil supplements might be a risk factor for atrial fibrillation and stroke among the general population but could be beneficial for disease progression, from atrial fibrillation to major adverse cardiovascular events, and from atrial fibrillation to death. Further studies are needed to determine whether potential confounders modify the effects of oil fish supplements and the precise mechanisms for the development and prognosis of cardiovascular disease events.
Ethics statements
Patient consent for publication.
Consent obtained directly from patients.
Ethics approval
The UK Biobank study obtained ethical approval from the North West Multicentre Research ethics committee, Information Advisory Group, and the Community Health Index Advisory Group (REC reference for UK Biobank 11/NW/0382). Participants gave informed consent to participate in the study before taking part.
Acknowledgments
This study was conducted with UK Biobank Resource (application No: 69550). We appreciate all participants and professionals contributing to UK Biobank.
- Mensah GA ,
- Johnson CO , et al
- Gao MM , et al
- Saravanan P ,
- Davidson NC ,
- Schmidt EB , et al
- National Institute for Health and Care Excellence
- Lichtenstein AH ,
- Vadiveloo M , et al
- Djuricic I ,
- Miller M , et al
- Kesse-Guyot E ,
- Czernichow S , et al
- Klemsdal TO ,
- Sandvik L , et al
- Manson JE ,
- Lee I-M , et al
- Gallacher J ,
- Allen N , et al
- Littlejohns TJ ,
- Sudlow C , et al
- Allen NE , et al
- Zhong W-F ,
- Liu S , et al
- Wu Z , et al
- Gallagher C ,
- Elliott AD , et al
- Qian SE , et al
- Nicholls SJ ,
- Lincoff AM ,
- Garcia M , et al
- Djousse L ,
- Al-Ramady OT , et al
- Bassuk SS ,
- Cook NR , et al
- Cazzola R ,
- Della Porta M ,
- Castiglioni S , et al
- Viviani Anselmi C ,
- Ferreri C ,
- Novelli V , et al
- Khan MS , et al
- Knowlton K , et al
- Marchioli R ,
- Bomba E , et al
- Olmastroni E ,
- Gazzotti M , et al
- Al Rifai M , et al
- Tavazzi L ,
- Maggioni AP ,
- Marchioli R , et al
- Zelniker TA ,
- Morrow DA ,
- Scirica BM , et al
- Limonte CP ,
- Zelnick LR ,
- Ruzinski J , et al
- Nelson JR ,
- Miller PE ,
- Van Elswyk M ,
- Alexander DD
- Mozaffarian D ,
- Bornfeldt KE
- Harris WS ,
- Ginsberg HN ,
- Arunakul N , et al
- Markozannes G ,
- Tsapas A , et al
- Svennberg E ,
- Engdahl J ,
- Al-Khalili F , et al
Supplementary materials
Supplementary data.
This web only file has been produced by the BMJ Publishing Group from an electronic file supplied by the author(s) and has not been edited for content.
- Data supplement 1
- Data supplement 2
GYL and HL are joint senior authors.
Contributors HL supervised the whole project and designed the work. GC and HL directly accessed and verified the underlying data reported in the manuscript. GC contributed to data interpretation and writing of the report. ZQ, SZ, JZ, ZZ, MGV, HEA, CW, and GYHL contributed to the discussion and data interpretation, and revised the manuscript. All authors had full access to all of the data in the study and had final responsibility for the decision to submit for publication. The corresponding author attests that all listed authors meet authorship criteria and that no others meeting the criteria have been omitted. HL is the guarantor. Transparency: The lead author (guarantor) affirms that the manuscript is an honest, accurate, and transparent account of the study being reported; that no important aspects of the study have been omitted; and that any discrepancies from the study as planned (and, if relevant, registered) have been explained.
Funding This work was supported by the Bill and Melinda Gates Foundation (grant No INV-016826). Under the grant conditions of the foundation, a creative commons attribution 4.0 generic license has already been assigned to the author accepted manuscript version that might arise from this submission. The funder had no role in considering the study design or in the collection, analysis, interpretation of data, writing of the report, or decision to submit the article for publication.
Competing interests All authors have completed the ICMJE uniform disclosure form at www.icmje.org/disclosure-of-interest/ and declare: support from Bill and Melinda Gates Foundation for the submitted work; no financial relationships with any organisations that might have an interest in the submitted work in the previous three years; no other relationships or activities that could appear to have influenced the submitted work.
Provenance and peer review Not commissioned; externally peer reviewed.
Supplemental material This content has been supplied by the author(s). It has not been vetted by BMJ Publishing Group Limited (BMJ) and may not have been peer-reviewed. Any opinions or recommendations discussed are solely those of the author(s) and are not endorsed by BMJ. BMJ disclaims all liability and responsibility arising from any reliance placed on the content. Where the content includes any translated material, BMJ does not warrant the accuracy and reliability of the translations (including but not limited to local regulations, clinical guidelines, terminology, drug names and drug dosages), and is not responsible for any error and/or omissions arising from translation and adaptation or otherwise.
Read the full text or download the PDF:
The Heart Rhythm Society (HRS) announced the findings of three new studies demonstrating the safety and efficacy of pulsed field ablation (PFA), a non-thermal ablation treatment for patients with atrial fibrillation (AF).
BOSTON, MA, MAY 18, 2024 – Today, the Heart Rhythm Society (HRS) announced the findings of three new studies demonstrating the safety and efficacy of pulsed field ablation (PFA), a non-thermal ablation treatment for patients with atrial fibrillation (AF). PFA shortens operating times and may reduce the chance of damage to surrounding tissues with high safety margins. Researchers presented these findings as late-breaking clinical science during Heart Rhythm 2024.
Atrial fibrillation is the most common type of arrhythmia, and by 2050, up to 16 million Americans are projected to have AF 1 . Contemporary guidelines recommend antiarrhythmic drugs as the initial therapy for the maintenance of sinus rhythm, but these medications are associated with serious adverse events 2 . Catheter ablation (thermal ablation or PFA) is an effective nonpharmacological alternative that is often, but not always, the second-line treatment if long-term medications or electrical cardioversion are not preferred or are not effective. PFA is different from thermal ablation—which has stood as the standard ablation method for AF for decades—in that it disables cardiac cells using electricity instead of heat or extreme cold. Recent technological advancements, such as catheter design and energy delivery, can pave the way for the wider adoption of PFA in clinical practice to improve patient outcomes 3 .
The findings of the following studies contribute to the growing body of evidence supporting PFA as an effective treatment option for patients with AF.
- Real-world data collection in subjects treated with the FARAPULSE™ Pulsed Field Ablation system (FARADISE): FARADISE is a global, prospective, post-market registry that studied real-world outcomes in more than 1,000 patients to demonstrate the safety and effectiveness of the FARAPULSE™ PFA System, which uses an over-the-wire catheter with variable distal shapes (basket and flower). At 3 and 6 months after ablation in patients with paroxysmal and non-paroxysmal AF, researchers did not observe any reported deaths or certain serious complications, including coronary spasm, persistent phrenic nerve palsy, atrio-esophageal fistula, or pulmonary vein stenosis. Compared to traditional thermal catheter ablation, the FARAPULSE PFA System requires a significantly shorter duration of energy application. Pulmonary vein isolation (PVI) procedure, left atrial dwell, and fluoroscopy times were 51, 32, and 12 minutes, respectively. For patients with additional ablation beyond PVI, average procedure, left atrial dwell, and fluoroscopy times were 68, 42, and 16 minutes, respectively. "Acute outcomes presented from more than 1,000 patients in the global FARADISE registry reinforce the safety, efficacy and efficiency profile of the FARAPULSE PFA System for the treatment of atrial fibrillation, with no reports of coronary spasm, pulmonary vein stenosis, persistent phrenic nerve palsy or esophageal fistula," said Prof. Lucas Boersma, electrophysiologist, St. Antonius Hospital, Netherlands, professor of electrophysiology, Amsterdam UMC, and lead investigator of the FARADISE registry. "We look forward to gathering long-term evidence from this registry for this particular PFA therapy, which continues to generate promising outcomes in real-world use."
- Long-term Safety and Effectiveness After Paroxysmal Atrial Fibrillation Pulsed Field Ablation from the U.S. Multicenter admIRE Study: The admIRE study, a prospective, multicenter, single-arm clinical trial, sought to demonstrate the safety and efficacy of the VARIPULSE™ PFA Platform when used for isolation of pulmonary veins in treatment of patients in the United States with symptomatic drug refractory paroxysmal AF. All patients in the study achieved acute success. Participants also saw a significant improvement in quality of life and reduced healthcare utilizations in terms of cardiovascular hospitalizations, cardioversion, and antiarrhythmic drug use. These results demonstrate the VARIPULSE™ PFA Platform can offer safe, consistent, and effective therapy, which may expand accessibility for patients seeking AF ablation and allow better early rhythm control. "The admIRE study demonstrated good safety and effectiveness results. The primary effectiveness success was 74.6%, and among the subset of participants receiving 73-96 PFA applications for PVI, 85% achieved success. Primary safety events occurred in 2.9%, demonstrating promising evidence of the capabilities of the VARIPLUSE Platform," said Vivek Reddy, M.D., director of electrophysiology, Mount Sinai Fuster Heart Hospital, New York. "Differentiated by its unique CARTO™ 3 System integration, this was the first PFA investigational device exemption study to support a substantial number of fluoro-free procedures; 25% of procedures were performed with zero fluoroscopy."
- Impact of Pulsed Field vs Thermal Ablation on One-Year Atrial Arrhythmia Burden – Sub-analysis of the ADVENT Trial: Recent evidence indicates that post-ablation atrial arrhythmia (AA) burden – the total duration of atrial fibrillation patients experience – is a better predictor of clinical outcomes than the standard 30-second definition. Data from the ADVENT randomized controlled trial, which compared the safety and effectiveness of the FARAPULSE PFA System to standard thermal ablation, sought to determine if residual AA burden affected quality of life (QoL), clinical outcomes, and if these outcomes differed between ablation modalities. After weekly post-ablation transtelephonic ECG monitoring (TTM) and 72-hour Holter monitor readings at 6 and 12 months, researchers found that patients treated with the FARAPULSE PFA System demonstrated a statistically superior reduction in arrhythmia burden (<0.1%) than thermal ablation. Across all ablation modalities, data demonstrated significantly greater QoL improvement in patients with AA burden <0.1% and an increased risk for redo ablation, cardioversion and hospitalization in patients with >0.1% AA burden. "Treatment with the FARAPULSE PFA System achieved a clinically meaningful, low residual AA burden compared to traditional thermal ablation, with positive impacts on quality of life and clinical outcomes for patients," said Dr. Reddy. "This data provides novel, helpful evidence for predicting the patient clinical experience and comparing the effectiveness of this therapy to standard thermal ablation for pulmonary vein isolation."
Researchers presented these trials at respective late-breaking clinical trial sessions on Friday, May 17, and Saturday, May 18.
Session Details
"Late Breaking Clinical Trials and Science - Ablation: Real world data collection in subjects treated with the FARAPULSE Pulsed Field Ablation system (FARADISE) " [Friday, May 17, 2024 at 4:30 pm ET]
"Late Breaking Clinical Trials and Science - Ablation: Long-term Safety and Effectiveness After Paroxysmal Atrial Fibrillation Pulsed Field Ablation from the U.S. Multicenter admIRE Study "[Friday, May 17, 2024 at 4:45 pm ET]
"Late Breaking Clinical Trials and Science - Ablation: Impact of Pulsed Field vs Thermal Ablation on One-Year Atrial Arrhythmia Burden – Sub-analysis of the ADVENT Trial " [Saturday, May 18, 2024 at 2:15 pm ET]
1 Go AS, Hylek EM, Phillips KA, Chang Y, Henault LE, Selby JV, Singer DE. Prevalence of diagnosed atrial fibrillation in adults: national implications for rhythm management and stroke prevention: the AnTicoagulation and Risk Factors in Atrial Fibrillation (ATRIA) Study. Journal of American Medical Association, 2001; 285:2370- 2370. doi:10.1001/jama.285.18.2370 2 Andrade JG. Ablation as First-line Therapy for Atrial Fibrillation. Eur Cardiol. 2023;18:e46. doi: 10.15420/ecr.2023.04. 3 Iyengar SK, Iyengar S, Srivathsan K. The promise of pulsed field ablation and the challenges ahead. Front Cardiovasc Med., 2023;10:1235317. doi:10.3389/fcvm.2023.1235317.
About the Heart Rhythm Society
The Heart Rhythm Society is the international leader in science, education, and advocacy for cardiac arrhythmia professionals and patients and the primary information resource on heart rhythm disorders. Its mission is to improve the care of patients by promoting research, education, and optimal healthcare policies and standards. Incorporated in 1979 and based in Washington, D.C., it has a membership of more than 8,200 heart rhythm professionals from 94 countries. For more information, visit HRSonline.org .
Press Contact
About heart rhythm 2024.
The Heart Rhythm Society's annual Heart Rhythm meeting convenes 8,400+ of the world’s finest clinicians, scientists, researchers, and innovators in the field of cardiac pacing and electrophysiology. More than 1,200 international experts in the field will serve as faculty for the 200+ educational sessions, forums, symposia, and ceremonies, while 110+ exhibitors will showcase innovative products and services. For more information, visit www.HeartRhythm.com .
Membership Reminder
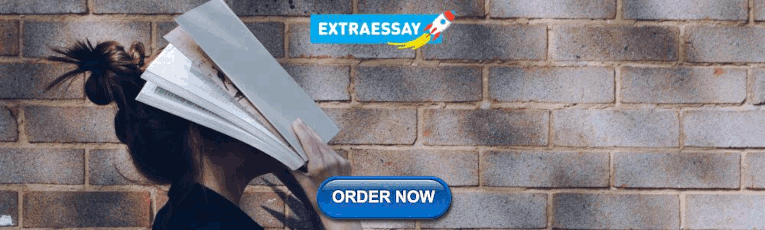
IMAGES
VIDEO
COMMENTS
Atrial fibrillation (AF) and heart failure have emerged as new cardiovascular epidemics over the last decade. 1 Heart failure affects ≈5 million patients in the United States, ... 30 The incidence of tachycardia-induced cardiomyopathy is unknown; most reports have been small, retrospective series or case studies involving mostly patients with AF.
Introduction. Atrial fibrillation (AF) and heart failure (HF) are the 2 significant epidemics in cardiovascular medicine, leading to increased morbidity and mortality. 1 AF and HF frequently coexist and impact reciprocally on each other. 2 The management of patients with AF and HF is challenging, as many medications used for AF in the absence of HF are contraindicated in the presence of HF ...
Atrial fibrillation (AF) and heart failure (HF) frequently coexist and complicate the course of treatment of each other. AF with rapid ventricular conduction can lead to tachycardia-mediated cardiomyopathy, which is a reversible cause of cardiomyopathy. However, in most cases, AF is the manifestation of various underlying cardiomyopathies. Guideline-directed pharmacological and device therapy ...
Abstract. Atrial fibrillation (AF) and heart failure (HF) are omnipresent cardiovascular disorders with a substantial impact on morbidity and mortality. As both share common risk factors, their pathophysiology is highly interrelated and a lot of patients present with both conditions. Surprisingly, despite their high prevalence, there is a ...
Atrial fibrillation (AF) and heart failure (HF) are omnipresent cardiovascular disorders with a substantial impact on morbidity and mortality. As both share common risk factors, their pathophysiology is highly interrelated and a lot of patients present with both conditions. Surprisingly, despite their high prevalence, there is a paucity of evidence regarding the optimal combined management of ...
This case study highlights the known benefits of physical exercise in heart failure with reduced ejection fraction and permanent atrial fibrillation. To the best of our knowledge, this is the first report of the benefits of exercise training guided by the Borg scale on physical capacity, neuromuscular sympathetic activity, and muscle blood flow ...
Reducing the burden of disease in patients with heart failure can be achieved by preventing atrial fibrillation. The cornerstones of prevention are lifestyle modifications, screening for and treatment of co-morbidities, and optimal guideline-directed medical therapy [3,4]. Newer therapies, such as sodium-glucose cotransporter-2 inhibitors ...
Atrial fibrillation and atrial flutter (AFF) are common in heart failure with preserved ejection fraction (HFpEF), with incidences of 5% to 32% (over 3.1-3.7 years), 1, 2 and prevalence reported of 15% to 65%. 3, 4 Atrial fibrillation in HFpEF contributes to increased risk of adverse outcomes. 5 In a post hoc analysis of the TOPCAT (Treatment ...
Introduction. Management of patients with atrial fibrillation (AF) and concomitant heart failure with preserved ejection fraction (HFpEF) remains complex. 1 Because the 2 share lots of the same pathologic mechanisms, AF can either precede or follow HFpEF, and there are differences in the temporal associations. In clinical trials, AF is an independent predictor of HFpEF, aggravating the ...
Case presentation: atrial fibrillation in heart failure. Session: Guidelines in Daily Practice I: atrial fibrillation in heart failure. Topic: Acute Heart Failure. Speaker: Professor D. Kotecha (Birmingham, GB) Event: Heart Failure 2017 - 4th World Congress on Acute Heart Failure. Congress Presentation. Slides.
In clinical practice, atrial fibrillation (AF) is the most common cardiac arrhythmia. By 2030, it is anticipated that the incidence and prevalence of AF will more than double, to 2.6 million and 12.1 million respectively [].Atrial fibrillation is a cardiac dysrhythmia that is characterized by abnormal electrical signals originating in the atria that fire rapidly with uncoordinated atrial ...
Study with Quizlet and memorize flashcards containing terms like Knowing that the client has two risk factors that cannot be modified, which intervention is most important for the nurse to include in the client's plan of care?, Which assessment finding provides the earliest indication that the client is experiencing right-sided heart failure?, Based on the electrocardiogram rhythm strip, what ...
Atrial fibrillation (AF) is the most common type of cardiac arrhythmia seen in clinical practice. The prevalence of AF increases with age: approximately 1% of individuals with AF are <60 years of ...
Introduction. The prevalence of heart failure (HF) is projected to increase 46% and its costs double to $70 billion by 2030. Atrial fibrillation (AF) is the most common sustained arrhythmia encountered in HF, with an average prevalence of 25% .Although there has been a significant increase in research targeting HF and AF, it remains unclear what approaches offer the best long-term outcomes.
Aims: Knowledge of long-term outcomes in patients with atrial fibrillation (AF) remains limited. We sought to evaluate the risk of new-onset heart failure (HF) in patients with AF and a low cardiovascular risk profile. Methods and results: Data from the Swedish National Patient Register were used to identify all patients with a first-time diagnosis of AF without underlying cardiovascular ...
Introduction to the context of the case study. Atrial fibrillation is a condition characterised by irregular and rapid heart rhythm. 134, 135 It can cause a range of symptoms including chest pains, palpitations, angina, shortness of breath and fatigue, and can sometimes present as a critical condition with haemodynamic instability requiring urgent treatment, although in contrast some patients ...
Study with Quizlet and memorize flashcards containing terms like Meet the Client: Jonathan StevensSixty-three-year-old Jonathan Stevens visits the healthcare clinic complaining of increasing fatigue and difficulty breathing. Physical assessment findings include a rapid, irregular heart rate of 138 beats/min, BP of 140/86 mmHg, and a respiratory rate of 28 breaths/min. His breath sounds are ...
Study design for the parallel, nested case control studies. Abbreviations: HF=heart failure, AF/f = atrial fibrillation/flutter, GP=general practice, CV=cardiovascular. Two nested case control studies, for HF and AF/f respectively, were conducted among all patients who experienced an exacerbation at start of cohort follow up.
Case Study: New Onset Atrial Fibrillation. A 74-year-old woman presents for her annual physical examination and notes increasing fatigue over the prior 3 months. Her history is notable for ...
PrOAF-HF will aim to test if rhythm control delivered through catheter ablation in patients in whom it is not clear whether atrial fibrillation or heart failure were the first disease results in a greater improvement in left ventricular ejection fraction (LVEF) compared with patients where heart failure was diagnosed first with no evidence of AF.
Case and control statuses for atrial fibrillation or flutter, ischemic stroke excluding subarachnoid hemorrhage, ischemic stroke excluding all hemorrhages and heart failure were defined based on ...
The following is a summary of "Factors associated with non-fatal heart failure and atrial fibrillation or flutter within the first 30 days post COPD exacerbation: a nested case-control study," published in the May 2024 issue of Pulmonology by Graul et al. Emerging evidence suggests a heightened risk of heart failure (HF) and atrial fibrillation/flutter (AF)
Atrial fibrillation is a supraventricular arrhythmia that increases the risk of stroke and all-cause mortality. It is the most common cardiac dysrhythmia in adults in the primary care setting, and ...
The cardiac manifestations observed in cases of leptospirosis infection are diverse, encompassing arrhythmias and abnormalities in electrocardiographic readings, pericarditis, myocarditis, congestive heart failure, aortitis, acute coronary arteritis, and involvement of heart valves. 6 Based on the studies, various causes for cardiac involvement ...
Twice as many platelets accumulated very high SH in the LVAD + AF case, resulting in a 36% increase in thrombogenic potential score, relative to the LVAD + SR case. ... Wang TJ, Larson MG, Levy D, et al. Temporal relations of atrial fibrillation and congestive heart failure and their joint influence on mortality: the Framingham heart study ...
Objective To examine the effects of fish oil supplements on the clinical course of cardiovascular disease, from a healthy state to atrial fibrillation, major adverse cardiovascular events, and subsequently death. Design Prospective cohort study. Setting UK Biobank study, 1 January 2006 to 31 December 2010, with follow-up to 31 March 2021 (median follow-up 11.9 years). Participants 415 737 ...
BOSTON, MA, MAY 18, 2024 - Today, the Heart Rhythm Society (HRS) announced the findings of three new studies demonstrating the safety and efficacy of pulsed field ablation (PFA), a non-thermal ablation treatment for patients with atrial fibrillation (AF).PFA shortens operating times and may reduce the chance of damage to surrounding tissues with high safety margins.