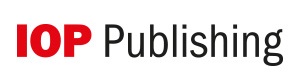
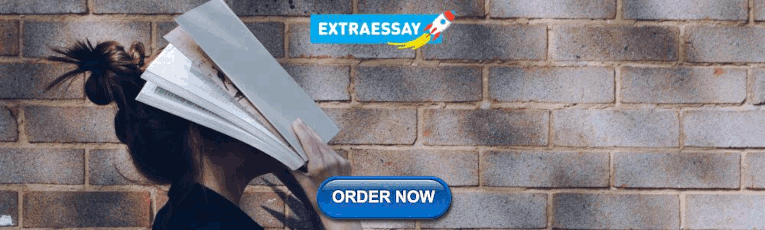
We apologize for the inconvenience...
To ensure we keep this website safe, please can you confirm you are a human by ticking the box below.
If you are unable to complete the above request please contact us using the below link, providing a screenshot of your experience.
https://ioppublishing.org/contacts/
An Open Access Journal
- Original Paper
- Open access
- Published: 20 December 2021
Integrative analysis of multimodal traffic data: addressing open challenges using big data analytics in the city of Lisbon
- Carlos Lemonde 1 ,
- Elisabete Arsenio ORCID: orcid.org/0000-0002-8520-8665 2 &
- Rui Henriques 3
European Transport Research Review volume 13 , Article number: 64 ( 2021 ) Cite this article
5146 Accesses
10 Citations
1 Altmetric
Metrics details
Worldwide cities are establishing efforts to collect urban traffic data from various modes and sources. Integrating traffic data, together with their situational context, offers more comprehensive views on the ongoing mobility changes and supports enhanced management decisions accordingly. Hence, cities are becoming sensorized and heterogeneous sources of urban data are being consolidated with the aim of monitoring multimodal traffic patterns, encompassing all major transport modes—road, railway, inland waterway—, and active transport modes such as walking and cycling. The research reported in this paper aims at bridging the existing literature gap on the integrative analysis of multimodal traffic data and its situational urban context. The reported work is anchored on the major findings and contributions from the research and innovation project Integrative Learning from Urban Data and Situational Context for City Mobility Optimization (ILU), a multi-disciplinary project on the field of artificial intelligence applied to urban mobility, joining the Lisbon city Council, public carriers, and national research institutes. The manuscript is focused on the context-aware analysis of multimodal traffic data with a focus on public transportation, offering four major contributions. First, it provides a structured view on the scientific and technical challenges and opportunities for data-centric multimodal mobility decisions. Second, rooted on existing literature and empirical evidence, we outline principles for the context-aware discovery of multimodal patterns from heterogeneous sources of urban data. Third, Lisbon is introduced as a case study to show how these principles can be enacted in practice, together with some essential findings. Finally, we instantiate some principles by conducting a spatiotemporal analysis of multimodality indices in the city against available context. Concluding, this work offers a structured view on the opportunities offered by cross-modal and context-enriched analysis of traffic data, motivating the role of Big Data to support more transparent and inclusive mobility planning decisions, promote coordination among public transport operators, and dynamically align transport supply with the emerging urban traffic dynamics.
1 Introduction
In the last decade, road traffic and mobility needs have increased significantly, especially in urban and metropolitan areas, a result of the socioeconomic growth and recent pandemic pressures [ 4 ]. This scenario is further affected by the relevance of pursuing climate objectives to reach carbon neutrality, operationalizing norms of social distancing, and the decentralization of activities and services to the periphery of urban centers. The heavy use of cars as private transport compromises the sustainability of modern cities [ 25 ]. To reach climate goals set by the Paris Agreement, the European Commission has already recognised the importance of multimodal passenger transport to increase the use of public transport, shared mobility options, and active modes of transport such as walking and cycling [ 10 , 27 ]. Multimodality, the use of different modes of transport in a single trip, can support the shift to a low carbon economy by taking advantage of the benefits of using different transport modes, such as convenience, safety, speed, cost, and reliability.
Mobility in major European capitals is not yet sustainable, prompting those capitals to reevaluate their public transport systems to meet societal goals [ 9 ]. Lisbon’s City Council is making efforts in collecting heterogeneous urban data for a better understanding of the multimodal mobility patterns [ 36 , 44 ]. Multimodal mobility patterns offer data-centric views of major traffic bottlenecks ensuring:
the city mobility planning dynamically responds to the ongoing changes in traffic;
fully transparent decisions to the citizens, enhancing the accountability of authorities;
supportive and objective coordination among public carriers and authorities involved in urban mobility planning.
In this context, heterogeneous sources of urban data are currently being consolidated in the Intelligent Management Platform of the City of Lisbon (PGIL) to meet various purposes [ 1 ]. Still, the potentialities of exploring the multiplicity of available urban data sources in an integrative manner for reaching sustainable mobility goals are still largely untapped [ 11 ].
This work aims at bridging the existing gap on the integrative analysis of multimodal traffic data and its situational urban context. To this end, we first provide a structured view on its major challenges. Second, rooted on existing literature and ongoing initiatives in major urban centers, we propose principles to address the listed challenges combining advances from urban computing, data science and intelligent transportation systems. Third, Lisbon is introduced as a reference case study to illustrate how the introduced principles can be operationalized in practice. In particular, we show how the city Council and public carriers are tackling the major obstacles to context-aware and multimodal mobility decisions. Finally, a spatiotemporal analysis of multimodality indices is conducted for the city of Lisbon using the available urban data, offering an initial practical characterization of cross-modal mobility restrictions and social equity aspects.
The remainder of this paper is organized as follows. Section 2 presents essential background on multimodality, and identifies opportunities and major technical obstacles to data-centric multimodal mobility decisions. Section 3 introduces principles for multimodal data analysis, offering guidelines to overcome the highlighted challenges. Section 4 introduces the city of Lisbon as the case study, instantiating the outlined principles using both qualitative and quantitative analyses. Final remarks are presented in Sect. 5 .
2 Background
Multimodality can be simultaneously understood as a property of the transport system, as a transport policy strategy and as a dimension of individual travel behaviour, forming a tridimensional perspective [ 26 ]. Within the later dimension, multimodality is commonly defined as the use of more than one transport mode to complete a trip.
This section first recovers essential concepts and literature on multimodal mobility (Sect. 2.1 ), and introduces state-of-the-art multimodality indices (Sect. 2.2 ) as those provide the basis for our practical study. Finally, in Sect. 2.3 , the major challenges to the context-aware and multimodal analysis of big traffic data are enumerated.
2.1 Multimodality
Buehler and Hamre [ 8 ] observed that multimodality is a subfield of a larger body of research on intrapersonal variability of travel behaviour, consisting of four dimensions: temporal, spatial, purpose and modal. The “modal” dimension describes the variability in the use of means of transport over time. Nobis [ 46 ] emphasizes the fact that the general definition of multimodality must be observed along individual trips to ensure its separation from the monomodality concept.
This distinction relates to the chosen time period, the longer the time period is, the higher is the probability that a person uses more than one mode of transport. For instance, Nobis [ 46 ] uses in her study a loose definition of multimodality, where any person who uses more than one mode of transport within one week is a multimodal transport user. In contrast, monomodal users tend to exclusively rely on a single mode of transport.
As highlighted by Tsirimpa et al. [ 64 ], one of the main goals of multimodal passenger transportation is to increase the use of public transport modes along with sustainable mobility options (i.e. cycling, walking) and emerging transport modes (e.g., shared mobility) such that a modal shift could be promoted and the use of private vehicles reduced. Zannat et al. [ 72 ] conducted a systematic review of research works using big data sources for public transport planning which covers three main areas: trip pattern analysis, modelling and performance analysis. Previous work conducted by Tympakianaki et al. [ 65 ] acknowledged the need to use multimodal traffic data sources for a more comprehensive analysis of the spatiotemporal impacts of localized disruptions on public transport demand and network performance. In their review work, Zannat et al. [ 72 ] concluded that the emergence of multimodal data is a promising research direction, as these data can be leveraged to optimize a transport network as an integrated system and be used to infer the latent public transport demand that can be attracted from enhanced connectivity between modes (e.g., public transport and shared bicycles).
Comparison of findings about multimodality across studies is challenging given the inherently different transportation systems across geographies, target data sources, temporal frames, and definitions of multimodality. However, some relevant results are common among studies: the percentage of multimodal persons decreases with advancing age [ 14 , 33 , 46 ],car availability is negatively correlated with multimodal behaviour, and positively correlated with monomodal driving [ 19 , 33 , 46 ],and having a driver’s license is negatively associated with multimodal users [ 33 , 46 ]. Multimodality is generally measured by considering the fraction of users that use a given number of travel modes. For example, Nobis [ 46 ] shows that car and public transportation users tend to be between 10 and 25 years old, with the largest group consisting of people aged 18–25, in Germany. While Buehler and Hamre [ 8 ] indicate that 87% of all trips in the United States are made by car and 90% of Americans use automobiles in their commuting trips for work purposes.
In his research work, Reichenbach [ 50 ] noted that more research is required to understand how public transport suppliers can assess the dynamics of multimodal behaviour at the user side and how synergies between modes can be enhanced.
2.2 Indices of multimodality
Despite the relevance of previous findings, most of the existing works neglect the intensity of use per transportation mode. In this context, the spatiotemporal analysis of multimodality indices from traffic records is important to dynamically detect zones with the lack of adequate transport supply along specific time periods, as well as urban zones that, despite the presence of different transport modes, are characterized by heightened imbalanced preferences towards specific modes of transport.
Diana and Pirra [ 20 ] targeted the problem of measuring multimodality at the individual level, by finding a multimodality index that comprises both descriptive statistics on the number of travel means, and the intensity of use of each mode. One of those measures is the Herfindahl–Hirschman ( \(HH\) ) index, a measure of market concentration for determining market competitiveness based on the size of firms in relation to the industry [ 53 ]. HH ranges from 0 to 1, from a perfectly competitive market with a high number of small firms to a monopoly. According to Diana and Pirra [ 20 ], in the context of transportation, the index approaches zero when a multiple balanced travel means is observed, whereas the value increases when a small number of modes tends to dominate. The original HH index is extended as follows:
where \(f_{i}\) is the intensity of use of i th transport mode, \(\overline{f}\) is the mean value of the intensities of all n modes, and m either corresponds to the total number of modes, n , in the original definition or to the number of modes offering transportation (demand different from zero) in the revised definition [ 20 ]. Susilo and Axhausen [ 58 ] used \({\text{HH}}_{m}\) to measure the repetitiveness of identical combinations of individual’s spatial–activity–travel mode choices within an observed period. In their study, higher index values were associated with periodic behavior and lower index values with less repetitive or variety-seeking behavior. In this context, \({\text{HH}}_{m}\) is also suggested to characterize the level of repetition of activity–travel patterns.
A comparable multimodal index is the Gini coefficient [ 2 ], which is classically used as a measure of income inequality in a population. A Gini coefficient of zero expresses perfect equality, while a value of one expresses maximal inequality. In the context of multimodality, it behaves similarly to the previous index. The Gini coefficient is defined as:
where \(f_{i}\) is the intensity of use of the i th mode assuming that modes are sorted in ascending order according to a target criterion (e.g., passenger demand), and \(n\) is the total number of modes. Tahmasbi et al. [ 59 ] used the Gini coefficient to evaluate the distribution of urban public facilities and accessibility level of different groups of people. This work presents a similar methodology (see Sect. 5 ).
Diana and Mokhtarian [ 18 ] reinterpreted the concept of Shannon Entropy [ 55 ] by considering a hypothetical mode choice experiment, where the uncertainty of the outcome is proportional to past multimodality behaviors of the traveler, i.e.:
When \({\text{OM}}_{PI}\) tends to 0 the individual uses only one mode among those being considered, whereas when \({\text{OM}}_{PI}\) = 1 the individual uses all these modes with the same intensity. Diana and Mokhtarian [ 18 ] proposed a variant of \({\text{OM}}_{PI}\) that is sensitive to the mean mobility level of individuals. Let \(M\) be the absolute maximum reported frequency of any mode, then:
Diana and Pirra [ 20 ] established an analogy between income inequality and multimodality, where individuals and their income respectively map into travel means and their intensities of use. An additional inequality measure, the Dalton Index [ 17 ], is proposed:
where \(\epsilon\) parameter represents the decreasing influence of more intensely used modes to determine the degree of a traveler’s multimodality. In their study, Diana and Pirra [ 20 ] showed that there is not an index that outperforms the others, still, some measures give best results in specific cases. For example, if the goal is comparing multimodal behaviors of different social groups, an index that is not replication invariant is recommended, i.e., \({\text{HH}}_{m}\) , \({\text{OM}}_{PI}\) or \({\text{OM}}_{MI}\) . Otherwise, if the mean intensities of use of the different modes vary across respondents, yet some modes in the set are never used, the application of the \({\text{DAL}}_{m}\) index is more appropriate.
2.3 Challenges to multimodal traffic data analysis
Despite the relevance of multimodal transportation to promote modal shifts from private vehicles towards public, shared and active transport modes, most urban centers still encounter major obstacles preventing the comprehensive monitoring and analysis of multimodal traffic dynamics. In accordance with these needs, this section groups the ongoing challenges in two major axes: challenges pertaining to the acquisition of consolidation of relevant urban data sources; and challenges pertaining to their integrative analysis for descriptive, predictive, and prescriptive ends.
Along the first axis, urban data acquisition and consolidation is challenged by three major needs:
the presence of an integrated automated fare collection system within the public transportation network for tracing the movements of passengers throughout the multiple carriers and modes of transport;
the relevance of city traffic sensorization initiatives, as well as standardized protocols for urban data acquisition and consolidation . Essential sources of traffic data include road traffic data from stationary and/or mobile devices; individual trip record data in the public transportation system given by smart card validations at stations or public vehicles; or pedestrian traffic data from privacy-compliant sensor technologies;
the incorporation of sources of context information. Traffic dynamics are situated, meaning that these are dependent on a high multiplicity of situational context factors. The presence of large-scale events creates irregular peaks of demand; road traffic interdictions condition mobility; weather impacts transportation mode decisions, especially active modes of transport; changes to the city urban planning affect the way traffic is generated and attracted to different parts of the city throughout the day [ 12 , 54 ]. Important sources of context data with impact on urban traffic include historical and prospective public events, ongoing and planned traffic interdictions,weather records and forecasts; geographical distribution of traffic generation-attraction pole; among others.
Along the data analytics axis, the integrative mining of traffic data produced from heterogeneous modes of transportation is challenged by four major factors:
the inherent spatiotemporal and multimodal nature of traffic data . The rich spatial, calendrical and modal content of traffic data should be properly explored, and the available sources of urban traffic data soundly processed and consolidated [ 44 ]. In addition, the stochastic nature of traffic, with considerable variability, further challenges the modeling of multimodal traffic dynamics,
the massive size of traffic data produced by mobile, ticketing and stationary devices. Exemplifying, in Lisbon, over 50 million smart card validations are observed within public carriers per month [ 4 ]. Analyzing massive individual traffic data requires the incorporation of strict scalability requirements along the pursued processing and learning algorithms,
the presence of emerging changes in urban traffic caused by shifting transport preferences, new traffic poles, as well as disruptive changes such as those triggered by mobility reforms and pandemics [ 45 ]. The value of static studies is thus of limited relevance as their findings can easily become depreciated. Instead, multimodal traffic data analysis should be fully automated and updatable once more recent data becomes available. In this context, there is the need to guarantee that the ongoing mobility changes are reflected in the computational models, as well as the ability to learn from traffic data streams and detect emerging traffic patterns,
the context-dependent nature of traffic . Despite their well-recognized impact on urban mobility, principles for context-aware traffic data analysis remain largely dispersed [ 11 ]. In fact, state-of-the-art contributions for context-aware descriptive and predictive tasks generally fail to model the joint impact that these multiple sources of context exert on urban mobility. In addition, existing works generally fail to separate the important role of both historical and prospective sources of context.
Context-aware multimodal traffic models are essential to aid mobility decisions, including operational, tactical, and strategic planning initiatives. In this context, the actionability and statistical significance of the found multimodal associations need to be robustly assessed. Decisions grounded on these associations can be linked to reforms in the transportation network, the exploitation of cost synergies, or incentives for eco-friendly transport modes (walking and cycling). As such, and irrespectively of the ends, the impact of mobility decisions should be additionally monitored and assessed to identify necessary revisions to the ongoing mobility reforms and initiatives.
In addition to the above technical challenges, environmental, social, economic and political dimensions need to be comprehensively accounted in the subsequent decision-making process to guarantee ecological and social equity issues in mobility reforms and, moreover, that these reforms are able to address the true causal factors underlying individual’s preferences and mode choice determinants [ 31 , 48 , 57 ].
Finally, governance principles are necessary to guarantee an effective multimodal coordination of efforts among the public transport operators, as well as between operators, city Councils and authorities [ 3 , 35 ]. To this end, multimodal patterns can be seen as an objective and transparent ground to facilitate cross-carrier planning and explore route-and-schedule synergies for the benefit of the citizens.
3 Multimodal big data analysis: principles
Moved by the need to address the set of challenges introduced in previous Sect. 2.3 , this section proposes a set of principles for the context-aware and multimodal analysis of Big Data produced from urban traffic sensorization initiatives. The identified principles are rooted on well-established contributions in literature and lessons from ongoing urban mobility projects, and are later confronted, in Sect. 4 , with extensive practical evidence gathered at the city of Lisbon. For simplicity, the principles are enumerated in line with the ordering of challenges along Sect. 2.3 .
Integrated multimodal fare collection system
The integration of Automated Fare Collection (AFC) systems from the different carriers operating on a given urban center provides the possibility to trace cross-carrier and multimodal trips along the public network, revealing bottlenecks such as points with heavy transfer demands [ 30 ]. Alternatives based on shared passenger identifiers are available [ 47 ], yet their use is discouraged as it does not enforce standards on the recording of individual trips, challenging subsequent consolidation, auditing and cross-carrier tariffs. Integrative ACF systems or alternative strategies to identify cross-carrier passenger flows offer an essential means to: (1) assess the efficacy of transport mode transfers in urban interfaces; (2) infer multimodal origin–destination (OD) matrices in accordance with the complete (instead of partial) commuting travel patterns of individuals; (3) discover multimodal traffic patterns to assess the needs and modal preferences of the citizens; (4) model and understand demand; and (5) support the multimodal planning of routes and schedules with the aim of reducing commuting needs and transfer waiting times.
Worldwide, different strategies for integrating AFCs across carriers, with the most common solution being based on unique smart cards validated at the stations, stops or vehicles from a transport network [ 47 , 70 ]. Trip records generally offer information pertaining to the user’s card, validation time, and associated station, vehicle, and/or route. Tariffs are generally dependent on the used modes, number of transfers, or crossed geographies. In contrast, in distance-based AFCs, the fare is usually calculated based on the total distance within a (multimodal) trip from boarding to alighting. Illustrating, the integrated AFC at Lisbon is an example of the former (Sect. 4.2 ), while the integrated AFC in Seoul is a distance-based one [ 30 ]. Buses and subway trains in Seoul are equipped with smart card readers located at the doors for boarding and alighting, thus offering the possibility to record the whole itinerary of each individual trip from the departing location to the destination, including intermediate transfers.
Urban data acquisition and consolidation
Heterogeneous sources of urban traffic data, including those generated by mobile devices, inductive loop counters, and integrative AFC systems, provide important complementary views on traffic dynamics. Following the principles initially set forth by Papadias et al. [ 49 ], these sources can be consolidated under a multi-dimensional scheme by identifying shared dimensions between sources, including time-and-date dimensions, spatial dimension (whether point, origin–destination, or trajectory information) and, when available, user and carrier dimensions. This modeling enables a coherent cross-modal navigation throughout the records of specific users, carriers, geographies, and time periods.
Given the massive size of urban data, data extraction facilities should properly index spatial, temporal and modal information for the efficient retrieval of information [ 32 , 41 ]. In this context, the target data centric recommendation systems should be equipped with efficient slicing and dicing procedures. Particular attention should be further paid to avoid unnecessary inefficiencies—for example, the characteristics of the stations, users or carriers should be decoupled from the trip records. In addition, data cleaning procedures should be available to ensure the absence of duplicates and gross errors, and further treat outlier and missing values whenever necessary. Finally, updating routines are necessary for the automatic extraction, transformation and loading of the continuously arriving data records into the consolidated database.
Context data incorporation
Recent attention has been paid on how to incorporate context to enhance traffic data analysis [ 11 ]. Two major principles are suggested for the automated acquisition of situational context. First, social media, public administration repositories, weather portals, online calendars of festivities, cultural agendas, theatre sites, and online news can be periodically explored with the aim of retrieving specific context sources of interest. Wibisono et al. [ 66 ], Tempelmeier et al. [ 61 ] and Tang et al. [ 60 ] gather principles towards this end. Despite the importance of web data mining, the acquisition of situational context data from the web is generally subjected to uncertainties related with data quality and availability. Second, in cities with well-established efforts towards the gathering and provision of situational context, the acquisition step can be simplified. In this context, periodic routines can be executed to extract context from structured or/and semi-structured sources maintained by the city Councils and other entities [ 36 ].
Multimodal traffic data analysis
Numerous principles have been suggested in the literature for the integrative analysis of traffic data from heterogeneous modes of transport:
descriptive analysis : (1) inference of multimodal origin–destination matrices by consolidating trip record data and tracing the complete movements of individual users throughout the public transport network [ 42 , 68 ],(2) mining of actionable traffic patterns, including frequent, periodic, emerging and anomalous patterns [ 37 , 39 , 71 ],(3) discovery of bottlenecks to multimodal mobility (waiting times, number of commutes, walking distances within and outside commutes) from trip record data [ 42 , 51 ],and (4) modelling traffic expectations by exploring the rich spatiotemporal content of the available traffic data and taking into consideration user-specific commutes in interface areas. State-of-the-art principles on spatiotemporal pattern mining, urban data fusion and analytics, and relational data mining can be pursued towards these ends [ 5 , 21 , 73 ],
predictive analysis : traffic forecasting is the predominant prediction task [ 40 ]. Following breakthroughs from deep learning along the last decade, we observed a shift from classic statistical approaches towards recurrent neural networks [ 22 ] and graph neural networks [ 69 ], some sensitive to transfers and other associations between different modes of transport [ 63 ], to better support both short-term and long-term forecasts,
prescriptive analysis : comprises advances on simulation, control and optimization to support decisions related with both individual and multimodal planning of the public transportation network (schedule-, vehicle- and route-wise) and urban traffic positive conditioning. Model-based multi-agent reinforcement learning [ 67 ], hierarchical network agent structures [ 15 ] and the use of deep neural networks as the underlying representation of the control problem [ 24 ] have been proposed towards these ends.
Emerging traffic changes
To account for ongoing urban mobility changes, traffic data analysis should be an automated process taking an arbitrary period of urban traffic data as input. In this context, the following principles should be pursued:
principles from incremental data mining and online learning, including those brought forth by Nallaperuma et al. [ 43 ], should be placed to guarantee the ability to learn from data streams, where new traffic records are continuously arriving. These principles guarantee the updatability of the models in the presence of more recent data without the need to compute descriptive and predictive models fully from scratch,
an additional important principle is the early discovery of emerging mobility patterns. Neves et al. [ 45 ] introduced principles for the timely discovery of emerging traffic dynamics, generally corresponding to new traffic flows or road/station/vehicle (de)congestions, creating the possibility to anticipate potential mobility bottlenecks that are critical knowledge for tactical and strategic mobility planning. In addition, trends and abrupt changes should be further identified for a proper understanding of non-seasonal changes in the city traffic.
Context-aware learning
Different principles have been placed to incorporate and learn from different sources of context, namely weather records, planned events, and occurrences of potential relevance from social media data [ 34 , 52 , 56 , 60 , 66 ]. Two major classes of context-sensitive approaches can be identified from the existing literature. First, approaches that aim to describe and predict traffic dynamics by segmenting data in slices according to the available situational context and using only context-resembling slices for understanding and forecasting demand [ 34 , 38 ]. Second, approaches able to embed the context directly in the models by capturing correlations with the context and using these correlations as correction factors to automatically adjust descriptive and predictive models [ 23 , 52 ].
Assessing multimodal decisions
Robust assessments are necessary to guarantee the adequacy of decisions placed from multimodal models of urban traffic. In this context, they should be pursued at three major levels:
data analysis level : the aforementioned descriptive, predictive and prescriptive multimodal models should be equipped with robust evaluation criteria to assess their proper decision translation. Multimodal traffic associations in descriptive model associations should be subjected to strict actionability and statistical significance testing [ 44 ]. In the context of predictive models, residual analysis and inference of upper and lower statistical bounds should be pursued using a sound evaluation setting, such as cross-validation schema on a rolling basis [ 54 ],
decision level : passenger’s modal preferences, receptivity for mode-commutes and endurable walking distances should be firstly identified on a user-by-user basis in accordance with historical data [ 16 , 28 ]. Once these assumptions are defined, the properties of the affected passenger trips can be quantified to estimate the decision’s impact on the mobility dynamics,
post-decision level : it is the easiest assessment level since the mobility dynamics before and after a decision can be objectively compared. Illustrating, the new patterns of multimodality can be measured to assess the impact of changes in the public transportation network for specific groups of users or the overall population in terms of waiting times, number of commutes, and adherence towards active and public modes of transport [ 12 ].
Multimodal planning
The data-centric analysis of the traffic demand and public transport supply provides a ground truth for the transparent and objective coordination between carriers. In this context, it is important to satisfy the following principles:
guarantee the interpretability of the learned models and the traceability of the recommendations [ 6 , 7 ]. The models should be easily auditable in order to guarantee that there is no preference towards specific carries in detriment of others,
offer a robust statistical frame. Given the stochastic nature of mobility dynamics, it is essential to assess whether the found patterns of multimodality occur by chance in order to strictly guarantee statistically significant outputs [ 29 ]. In this context, statistical tests can be placed to assess the trustworthy degree of decisions, and new heuristics incorporated within the learning process to minimize false positive and false negative discoveries,
comprehensively compare alternative decisions (e.g., suboptimal routing and scheduling plans) in order to assess complementary scenarios and further validate the quality of the suggested recommendations [ 3 ].
4 Results: addressing the challenges in the city of Lisbon
This section introduces Lisbon as our case study to show how ongoing efforts have been established to answer the introduced challenges. Section 4.1 describes the public transportation system of the Lisbon Metropolitan Area. Sections 4.2 and 4.3 describe the undertaken initiatives for exploring opportunties and addressing the major obstacles to the multimodal traffic data analysis.
4.1 Lisbon city as our study case
This work is anchored in the research and innovation project ILU—Integrative Learning from Urban Data and Situational Context for City Mobility Optimization—, a project that joins the Lisbon city Council and two research institutes, bridging the ongoing research on urban mobility with recent advances from artificial intelligence. The available traffic data comes from various heterogeneous sources collected for the Lisbon Metropolitan Area (LMA). The LMA is an administrative regional division in Portugal that covers the municipality of Lisbon and an additional set of 17 surrounding municipalities (Fig. 1 ). Although the reported research is directed towards the municipality of Lisbon, its contribution and results can be extended and applied to the nearby municipalities to enable more comprehensive analysis of inter-municipal commuting mobility patterns.
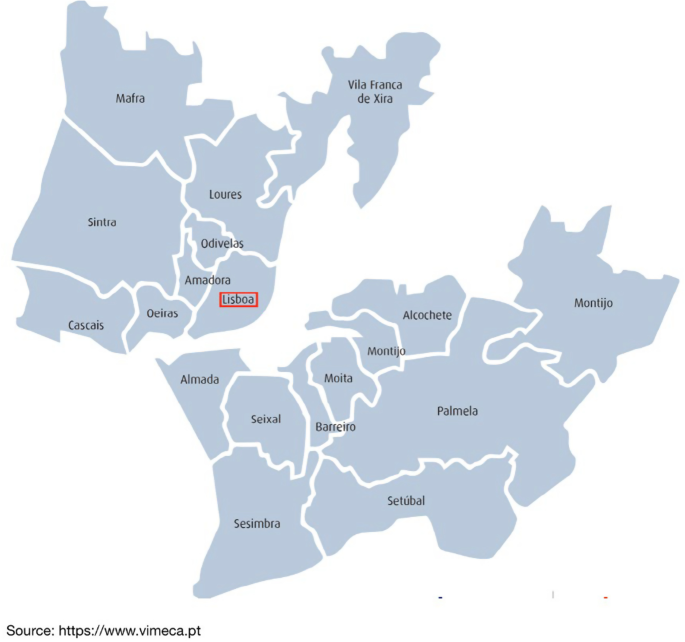
Lisbon Metropolitan Area and its municipalities
The public transport network in the Lisbon Metropolitan Area (LMA) is composed by twelve carriers. Information pertaining to the network of the largest public carriers is provided in Table 1 . Passenger transport run by the mentioned public transport operators are equipped with smart card readers for boarding and, depending on the type of vehicle, alighting.
Amongst the listed public carriers, only two—CARRIS (bus and tram operator) and METRO (subway operator)—offer a comprehensive footprint coverage along the city of Lisbon. All remaining carriers operate within the broader metropolitan geographies to offer accesses from nearby municipalities into the Lisbon city, but do not offer city-wide coverage, being limited to specific locations outside the city center (Sete Rios, Campo Grande, Areeiro, Entrecampos, Oriente, Benfica and Santa Apolónia). In this context, our focus is primarily placed on smart card validations at CARRIS and METRO operators, accounting for over 80% of the validations within the city of Lisbon.
In addition to bus, tram and subway modes of transport, we further combine validations from the Lisbon’s public bike sharing system (GIRA), corresponding to bike pick-ups and drop-offs. METRO and GIRA validations are performed at stations. In contrast, smart card validations in CARRIS are performed at the entry of buses. As such, we make use of alight stop inference principles proposed by Cerqueira et al. [ 12 ] to estimate exits.
4.2 Multimodal traffic data analysis in Lisbon
4.2.1 integrated fare collection systems.
The providers of bus, tram, subway, railway and inland waterway modes of transport in the city of Lisbon are currently operating under an integrated fare collection system, enabled through the VIVA card initiative. The VIVA card initiative, firstly established between the subway operator (METRO) and the major bus operator (CARRIS), was in 2017 extended to further encompass railway operator, Comboios de Portugal (CP), and in 2019 extended towards the remaining major carriers operating within (or interfacing with) the city of Lisbon. Footnote 1 To this end, the early individual ticketing systems were consolidated into a unique ticketing system coordinated by OTLIS, the responsible entity for managing the information resources shared among carriers. In 2019 multimodal tariff plans were also released to create incentives towards a multimodal use of the public transportation system.
4.2.2 Urban data acquisition and consolidation
Among the diverse initiatives established by the Lisbon City Council towards sustainable mobility, focal efforts are being placed on the city sensorization, and subsequent data acquisitions and consolidation [ 1 ]. Numerous sources of urban data—covering areas such as mobility, security, decarbonisation, urban planning, local development and civil protection—are currently being consolidated in the Intelligent Management Platform of Lisbon (PGIL). Footnote 2 In particular, the following sources of traffic data are currently already consolidated:
road traffic data from three major types of sensors: (1) inductive loop detectors in major road junctions in the city, offering discrete views on traffic flow; (2) mobile devices with global positioning systems (GPS) and active applications such as WAZE Footnote 3 or TomTom, Footnote 4 offering aggregated views of traffic congestion along specific road segments (geolocalized speed data); and (3) privacy-compliant cameras in major roads;
aggregated views of public transport data, including passengers’ card validations and the GPS positioning of public vehicles. Due to privacy and security aspects, only aggregated views of card validations along the public transport network are maintained by the city Council. The raw trip records are maintained separately by each operator and consolidated by OTLIS to collect statistics and ensure the sound interoperability of ticketing systems;
bike sharing data from the Lisbon’s public bike sharing system (GIRA), including trip records per user, user feedback on bicycle’s condition, bike charging information, bike malfunction and repair status, among others;
other sources: emerging modes of transportation, including private scooter traffic data, are being also consolidated. An entry requirement for new private operators is precisely the full disclosure of trip records.
4.2.3 Context data incorporation
The Lisbon city Council further established protocols to collect diversified sources of situational context information with potential impact on traffic for guiding mobility decisions. Some of the available sources of context data include:
public events, including conventions, festivals, concerts, and sport events. The historic and prospective events are currently sourced from the cultural city agenda and planned usage of halls, stadiums and open areas;
urban planning of the city with the localization of traffic generator-attractor poles, including: commercial poles (malls, commercial permits, markets, terminals), education facilities (public and private schools, universities, institutes), health-related facilities, sport facilities, cultural poles (concert halls, museums, theatres), recreational spaces, parks, or citizen spaces;
ongoing and planned construction road works (traffic conditioning events) characterized by a set of trajectories with (possibly non-convex) interval of obstruction and accompanying details (including the number of affected ways and whether interruption is spasmodic);
weather record data sourced from three meteorological stations maintained by Instituto Português do Mar e da Atmosfera (Portuguese weather agency);
other sources of interest, including details on traffic and transport networks (mostly walking, road and cycling infrastructures), zoning information (including traffic analysis zones), city occurrences (including road accidents and incidents, medical emergencies, fires and floods, logistical help and falling structures, transport requests, conservation and complaints, and rescue and civil protection), and other calendric information with impact on traffic patterns (e.g., bank holidays).
The city Council standardly stores these different context data sources using semi-structured data representations (JSON) at the Lisboa Aberta (Open Lisbon) portal. The repositories are periodically updated in order to facilitate administrative tasks, as well as to potentiate complementary strategic and research initiatives.
4.2.4 Multimodal analysis of massive traffic data
Multiple contributions on multimodal traffic data analysis have been undertaken in the context of the ILU project, rooted on the interdisciplinary triaxial lens: data science and statistics—urban mobility planning—artificial intelligence. Cerqueira et al. [ 13 ] proposed an approach for inferring dynamic and multimodal origin–destination matrices using bus, tram and subways modes. Approximately 20% of journeys in the Lisbon’s transportation network require one or more transfers. The approach supports dynamic OD inference along parameterizable calendrical rules, spatial criteria. Traffic flows can be further decomposed in accordance with the user profile and the nature of trips. Finally, the target ODs gather several statistics that support traffic flow analysis, helping CARRIS, METRO and the Lisbon city Council to detect vulnerabilities throughout the transport network, including statistics pertaining to commutation needs, walking distances and trip durations. On the same work, we further proposed alight bus stop inference models in the absence and presence of multimodal views. The gathered results show that the multimodal model successfully estimated the exits of 85% of trip segments, + 10 pp than the monomodal counterpart.
On the previous work of Neves et al. [ 44 ], we tackled the problem of mining actionable patterns of road mobility from heterogeneous sources of traffic data. To this end, we proposed the combined use of data transformations and pattern-based biclustering searches to comprehensively explore spatiotemporal associations within road traffic data. Results using geolocalized speed data from mobile devices and inductive loop counter data from stationary devices at major arteries in the city of Lisbon confirm the role of the proposed integrative data mining methodology to discover actionable traffic patterns.
These earlier contributions, together with additional predictive approaches for multimodal traffic data analysis [ 54 ] and online Big Data visualization facilities, are currently integrated within a recommendation system, termed ILU App. The deployment of this set of urban analytics tools within the PGIL managed by the city of Lisbon, is expected to support urban mobility planning giving priority for public transport options and the integration of active travel modes (walking, shared public bicycles) with bus and/or metro/subway. Moreover, the full scalability and online nature of the devised tools can be enriched by targeting other dimensions of the city dynamics in the post-pandemic era.
4.2.5 Emerging traffic changes
In urban mobility, emerging patterns reveal ongoing changes in city traffic dynamics, whose growth along time may indicate the establishment of new congestion trends along roads, stations or routes. Those trends can evolve to create traffic bottlenecks if timely precautions are not taken. As such, the early detection of emerging patterns offers urban planners the opportunity to make the necessary provisions to urban mobility.
In the earlier works of Neves et al. [ 44 ], we proposed the E2PAT method to discover emerging patterns from heterogoeneous traffic data sources in linear time. E2PAT combines spatiotemporal data mappings with simple yet effective time series differencing operations to find emerging traffic behaviors. E2PAT further provides statistical guarantees of pattern growth, support and accuracy, as well as visualization and navigation facilities, to safeguard the soundness and usability of the multimodal pattern analysis process. An integrative score is considered to measure the relevance of emerging patterns, offering a sound criterion to control the false positive and false negative discovery rates. E2PAT has been both applied to the Lisbon's road traffic monitoring system and public transport network. Results confirm their relevance to retrieve all emerging (de)congestions in the road, stations and public vehicles in accordance with flexible spatial criteria and calendrical constraints.
4.2.6 Context-aware learning
Historical and prospective sources of context data are maintained by the Lisbon city Council in semi-structured repositories that can be standardly accessed, facilitating structured retrieval of information in accordance with spatial and temporal criteria of interest [ 36 , 54 ]. In some of the previous works conducted in the context of the ILU project [ 11 , 36 ], traffic data has been segmented in accordance with the available situational context—comparable events and calendrical, meteorological and spatial context. In addition, correlations between urban traffic in Lisbon and their situational context have been comprehensively computed with the aim of producing correction factors to automatically adjust descriptive and predictive models [ 23 , 62 ]. Illustrating, the effect of extreme weather conditions on the public cycling demand demand has been assessed for a superior modelling of traffic dynamics [ 11 ]. In this same work [ 11 ], we also show that the available context, whether static or temporal, can be used to augment traffic data. We show that the application of these three groups of context-aware learning principles—context-driven corrections, context-driven data segmentation and context-driven data augmentation—can be pursued irrespectively of the underlying spatiotemporal data structure. In particular, the impact of road interdictions, public events (including sport matches and large-scale concerts), and traffic generation-attraction poles on traffic is quantified.
In an alternative work, Sardinha et al. [ 54 ] extended recurrent neural network layering to incorporate both historical and prospective sources of context with the aim of improving traffic forecasts. To this end, a sequential composition of long short term memory (LSTM) components and/or gated recurrent units (GRU) is proposed, where historical sources of context data are considered at the initial layers and prospective sources of context data at the latter layers. Historical context can be combined at the input layer to guide the learning task by relying on masking principles. For instance, calendric masks can mark weekdays or academic periods and breaks, situational masks mark periods where events of interest may impact the demand observed at a given geography, and weather masks are associated with multivariate time series with as many variables as weather attributes of interests. Prospective context data, such as weather forecasts and planned events, can be complementarily inputted into the last LSTM component to adjust predictions. Using public cycling traffic data in Lisbon, we show the role of historic and prospective sources of context to guide predictive tasks.
5 Results: multimodality indices in the city of Lisbon
Section 4 provided a general view on some of the ongoing initiatives and contributions towards multimodal traffic data analysis in the city of Lisbon. This section instantiates some of the principles enumerated in Sect. 3 with a specific purpose at hands: performing a spatiotemporal analysis of multimodality indices along the city of Lisbon to assess social equity aspects on the access to different transport modes.
Data . To conduct this study, we primarily rely on trip record data from CARRIS (bus and tram operator), METRO (subway operator) and EMEL/GIRA (bike sharing operator) given their comprehensive footprint coverage along the city. As introduced in Sect. 4.1 , additional public carriers operate within the broader Lisbon Metropolitan Area (LMA) to offer accesses from nearby municipalities into the Lisbon city peripheries. Although the inclusion of trip records from these additional carriers are not considered in this work, they are relevant to provide a more comprehensive view on multimodality.
Figure 2 identifies the routes of the major public carriers in Lisbon. Figure 2 A, B provide respectively the routes of CARRIS and METRO carriers. In Fig. 2 B, the public bike sharing stations (green) and stationary road sensors (blue) are also displayed. Figure 2 C complement this view with the routes of train operators (CP and Fertagus) and inland waterway operators (Transtejo and Soflusa), while Fig. 2 D confronts the station footprint of CARRIS (yellow) against the additional public bus carriers (TST, RodLisboa, Sulfertagus).
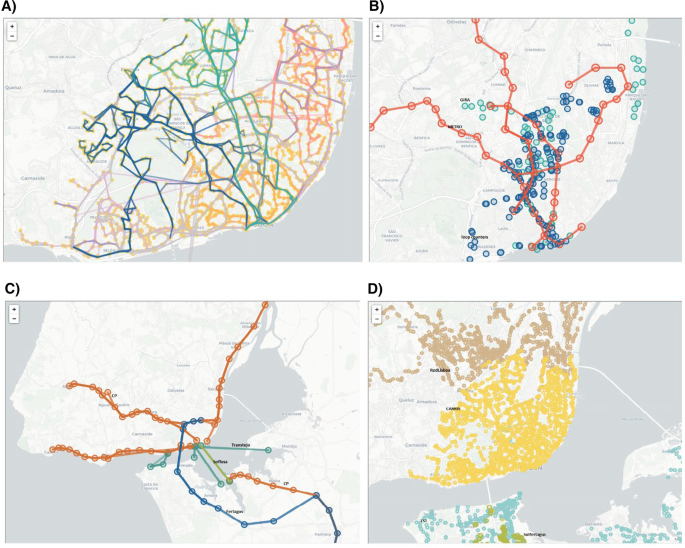
A Routes of CARRIS (major bus operator) by class: night (cobalt), red, green, blue, yellow, orange, pink buses. B Subway METRO stations and lines (red), GIRA bike stations (green) and road sensors (blue). C Stations and routes of railway and inland waterway operators: CP (orange), Fertagus (blue), Transtejo (dark green) and Soflusa (lime). D Stations of four major bus operators: CARRIS (yellow), RodLisboa (brown), TST (light blue) and Sulfertagus (lime)
For the analysis, we have considered all trip records from October 2019. A total 32.786.326 trips were recorded in the METRO network (65 million smart card validations at entry and exit stations), 11.360.894 trips were recorded at the entry of trams and buses in the CARRIS network, and 146.232 bicycles were picked up at the public GIRA’s bike sharing network during this period.
Figure 3 provides general statistics pertaining to average daily use of each mode during weekdays and weekends, while Fig. 4 offers a zoom-in on the METRO and CARRIS network to decompose the validations per subway line (Fig. 4 A) and per cluster of routes in the bus-tram network (Fig. 4 B).
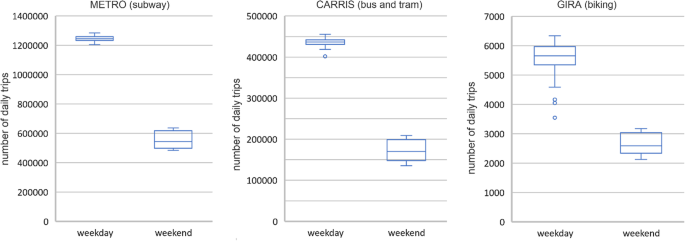
Number of daily trips along subway (METRO), bus-tram (CARRIS), and cycling (GIRA) modes of transport in Lisbon on working days and weekends, October 2019
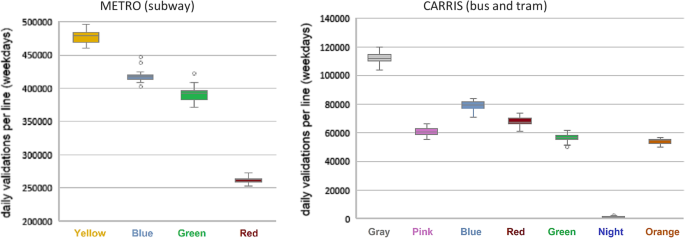
Number of daily trips along the four METRO lines (subway) and seven CARRIS clusters of routes (bus-tram) on working days, October 2019
Spatial and temporal criteria. Multimodal pattern analysis can be conducted at different spatial granularities. Two major possibilities are considered. First, the user can manually specify the target geographical region of interest using polygon and circular marking facilities. Second, the user can select predefined regions. We provide the following zoning maps for the Lisbon Metropolitan Area:
Traffic Analysis Zones (TAZ): geographical unit used in transportation planning models to assess socio-economic indicators (Fig. 5 a);
Municipalities: coarsest geographical unit for the city. Currently, this work uses city parishes as the administrative criterion of division (Fig. 5 b);
Sections: finest geographical unit, comprising small districts and neighbourhoods (Fig. 5 c);
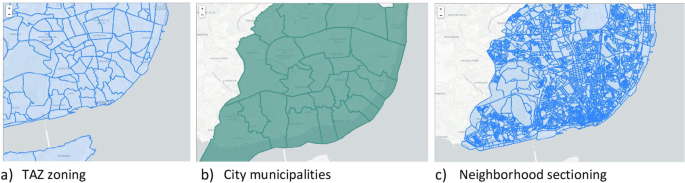
Zoning: geographical decomposition of the Lisbon city at different granularities
Under the selected spatial granularity, traffic events, such as smart card validations and individuals’ trajectories, as well as the accompanying situational context data, are then linked to one or more Lisbon’s zones in accordance with their spatial extent.
Calendrical constraints—such as day of the week (e.g., Mondays), weekdays, holidays or on/off-academic period calendars—can be placed to segment the available traffic data. The introduced principles for multimodal pattern analysis (Sect. 4 ) can then be applied per calendar or, alternatively, correction factors can be learned from calendrical annotations to guide the target tasks. Second, time intervals (e.g., on/off-peak hour intervals) or a fixed time granularity (e.g., 15-min) can be optionally specified to guide traffic data descriptors or predictors. For instance, passenger volume series in public transport can be resampled from card validations. In the absence of a minimum time granularity, the data analysis can be conducted at the raw event level or under multiple time aggregations.
Once spatiotemporal constraints are fixed, multi-dimensional querying and subsequent data mappings are provided to retrieve the desirable spatiotemporal data structures in accordance with the principles introduced along Sect. 4 .
Mode distribution. Considering traffic analysis zones (TAZ) as the spatial criteria, Fig. 6 provides a comprehensive view of the quota of the three targeted modes of transport. It shall be noted that not all TAZ are covered by subway or bike stations, hence the predominance of the bus mode (CARRIS) for a significant number of zones. The adherence towards the cycling mode of transport is considerably smaller in magnitude for most of the zones.
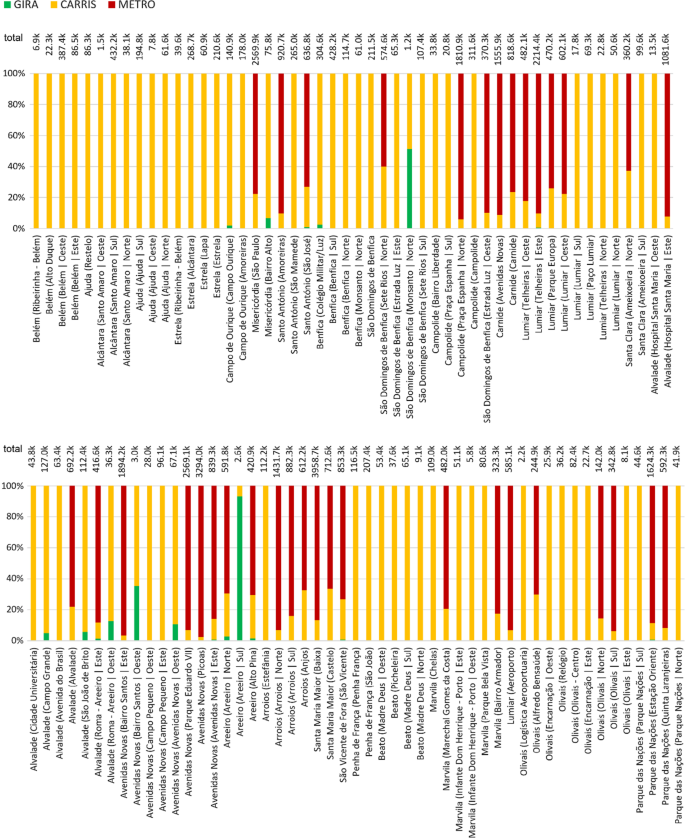
Cycling-bus-subway quota (modal trip share) across the major traffic analysis zones (TAZ) in Lisbon, October 2019
To assess how the modes are distributed in specific regions of interest, we consider the Entrecampos urban area. Entrecampos is an interface area that encompasses all modes of transport and is further characterized by the presence of business and cultural traffic generation poles.
Figure 7 A, B provide a zoom-in into this area, showing the subway, bus and cycling stations, and further highlighting some of the commercial, healthcare, educational and cultural poles contained within this area.
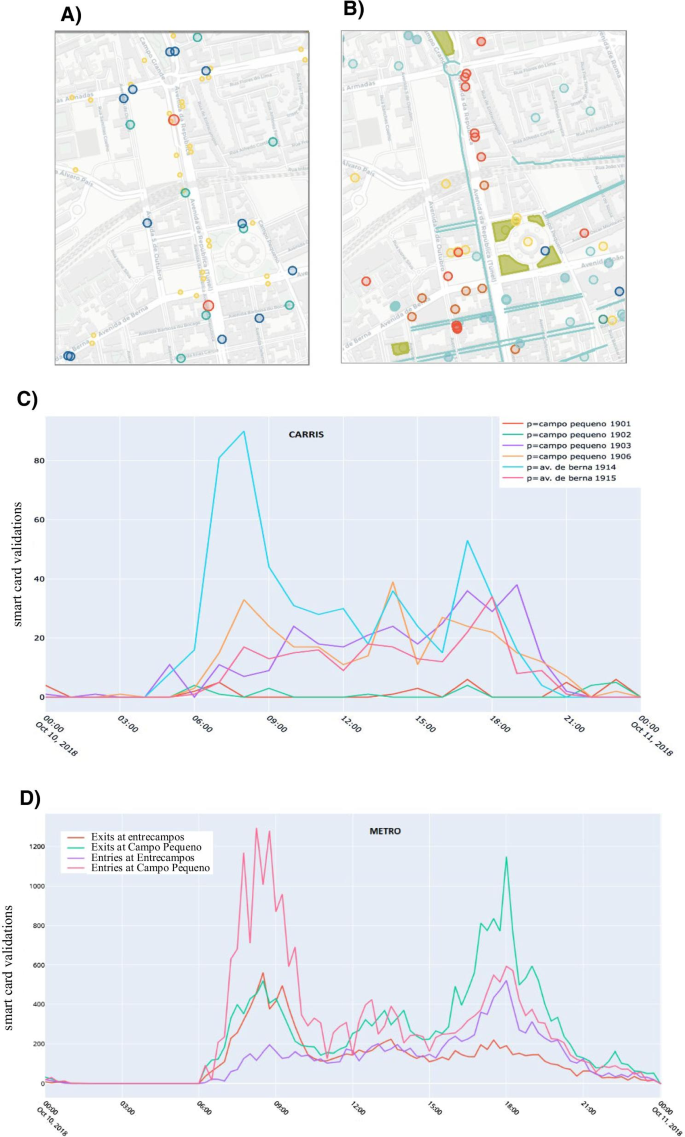
A Subway stations (red), bus stops (yellow), bike sharing stations (green) and road sensors (blue) in the Entrecampos district. B Cycling roads, green parks, commercial poles (yellow), schools and institutes (light blue), health centres (red) and cultural poles (blue) in the Entrecampos district. C Hourly check-in validations in CARRIS buses at six stops in the Entrecampos area on a typical weekday. D Check-in and check-out validations at METRO stations in Entrecampos on a typical weekday
In October 2019, we find a total of 19,033 bike pick-ups in this area, 1,786,568 smart card entry validations at the Entrecampos subway station, and 201,441 smart card validations at the bus stops in this area. Figure 7 C depicts the hourly volume of check-in validations on Entrecampos’ bus stops, while Fig. 7 D shows both check-in and check-out card validations for the two subway stations situated in this area under 15-min intervals. Generally, we observe that the amount and pattern of card validations strongly vary across stations.
Multimodality. For detecting vulnerabilities associated with multimodal transportation, two major options are made available. The user can select one of the introduced indices of multimodality and use them to assess them at the passenger level or, in alternative, at a geographical level by assessing the multimodal offering associated with a given regions.
Considering passenger level views, Fig. 8 provides a comprehensive view on the intensity of subway and bus usage per passenger. Passengers are distributed in accordance with the number of validations in METRO and CARRIS operators throughout October 2019.
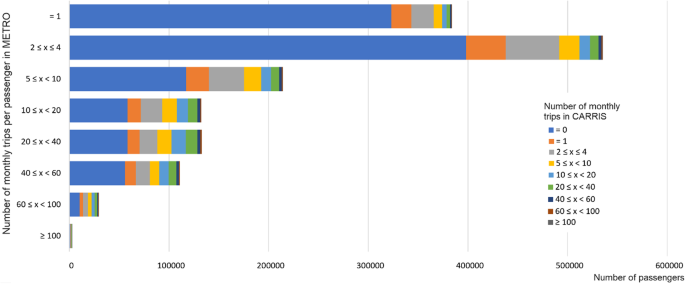
Number of passengers in accordance with the intensity of usage per subway and bus-tram modes in October 2019. Groups of passengers are defined according to the number of entry smart card validations in METRO and CARRIS networks
Considering geographical level views, Fig. 9 (and the corresponding Table 2 in “ Appendix ”) presents the spatial distribution of the Herfindahl Hirschman index (Eq. 1 ) and multimodality Gini index (Eq. 2 ) for the traffic analysis zones (TAZ) of the Lisbon city. To this end, we rely on the volume of passenger entries and exits within the bus, tram, subway and cycling modes of transport along October 2019. These state-of-the-art indices of multimodality are selected due to their sensitivity to the intensity of use per mode, bounded score ranges, and inherent simplicity.
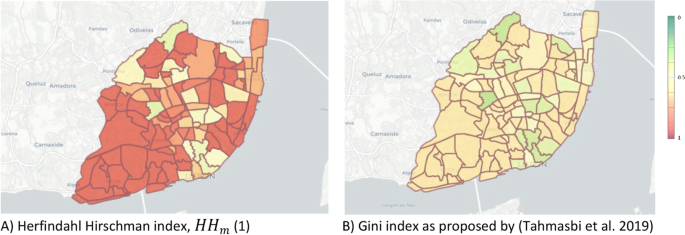
TAZ distribution of multimodality indices of bus-subway-cycling modes of transport in October 2019. A Herfindahl Hirschman index, HH m (1). B Gini index as proposed by (Tahmasbi et al. 2019)
Generally, we can observe two major sources of multimodal penalties: the presence of many zones with only mode of transport (generally bus on the periphery), as well as the intense preference towards subway transport in the center of the city. Despite the concordance of views offered by both indices, the gathered results further underline the presence of some significant differences, highlighting the importance of selecting each multimodality index aligned with the end purpose of the study.
Considering the revised HH index sensitive to the absence of traffic generated by modes without stations on a given zone, we can observe that the peripherical zones of the city are not as penalized by this index as they are by the original HH index which is normally used for equity assessment.
Figure 10 (and the corresponding Table 3 in “ Appendix ”) extends the previous analysis for Lisbon municipalities, highlighting differences as the coarser zones are now able to encompass new stations and further suggesting the importance of identifying a proper spatial criterion for the analysis of multimodal indices.
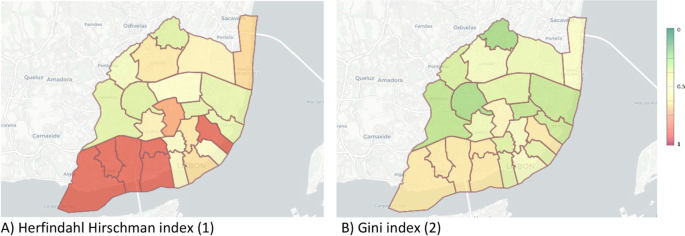
Distribution of multimodality indices (HH and Gini indices) along Lisbon municipalities considering bus-subway-cycling modes of transport in October 2019. A Herfindahl Hirschman index (1). B Gini index (2)
Multimodality indices at passenger and geographical levels offer an initial characterization of modal preferences when multiple modes are available, mobility restrictions, and social equity aspects. The comprehensive analysis of these indices is expected to assist the municipality of Lisbon and comparable cities in moving towards urban mobility plans where active modes of transportations are prioritized. As these indices are grounded on trip record data, they provide an objective means to establish coordination efforts among municipalities and carriers; and offer the possibility to monitor reforms and continuously align decisions with the ongoing city traffic transformations, ensuring that the public transport system responds to emerging multimodal traffic vulnerabilities, a growing need given the transformations and changing regulations observed in a pandemic context.
Incorporating situational context . The analysis of multimodality indices is only meaningful in the presence of situational context. In this work, we consider the role of traffic generation poles to this end. Traffic generation and attraction poles generally refers to commercial areas, employment centres such as business parks and enterprises, and collective equipment like hospitals, schools and stadiums, that generate or attract a significant volume of vehicle trips, either from contributors, visitors or providers at different times of the days. We currently maintain a complete localization of traffic generation poles for the city of Lisbon, as well as major city events (such as large concerts, congresses and soccer matches). Figure 11 provides maps of the city with some poles with impact on the city traffic.
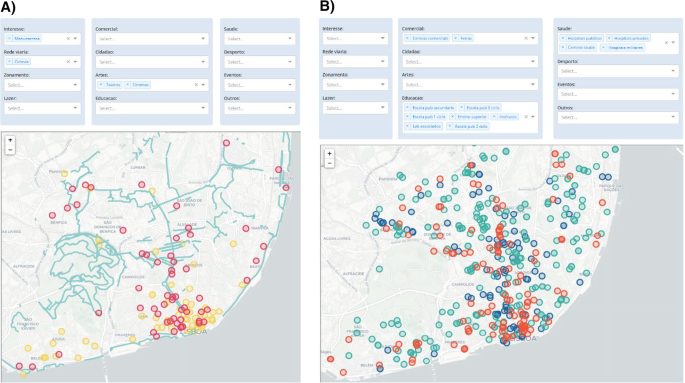
A Cycling roads (green), art and cultural poles (red), and tourist attraction poles (yellow). B Major traffic generation poles: commercial (blue), schools and institutes (green) and health centres (red)
The combined analysis of the computed multimodality indices against the above traffic generation/attraction poles’, as well as station-route maps, is essential to guarantee the presence of multiple options of transport in areas with high density of traffic generation poles. The combined analysis of these poles and individual traffic dynamics offers the unique opportunity to comprehensively model the spatiotemporal distribution of traffic along the city. Complementarily, the surveyed indices can be revised to further measure how the volume of passengers generated and attracted by nearby poles are being currently satisfied by the co-located modes of public transport.
6 Conclusions
The research work offers a structured view on the opportunities and challenges for the analysis of big traffic data produced from heterogeneous sources and passenger transport modes for supporting a more inclusive mobility planning. A set of guidelines to address existing challenges, while leveraging on opportunities, were sourced from the ongoing advances in the fields of artificial intelligence and data science which were applied to urban mobility through a real-life case study engaging the City of Lisbon and its major public passenger transport operators.
The established initiatives by the Lisbon City Council towards the consolidation of relevant sources of urban data on its intelligent management platform, together with the integrative fare collection system and entry requirements for carriers operating in the Lisbon metropolitan area, offers unique opportunities for multimodal pattern analysis and cross-carrier coordination. Still, the inherent nature of multimodal traffic data—heterogeneous, massive in size, rich in spatiotemporal dynamics, subjected to variable aspects, and context-dependent—together with the increasing disruptive changes in urban traffic poses challenges towards the pursue of data-centric multimodal decisions. To tackle these challenges, the outlined work combines a comprehensive set of principles from context-aware, spatiotemporal, distributed and relational data mining. In particular, the spatiotemporal analysis of multimodaility indices against available situational context offers an initial simplistic way of detecting urban zones with the lack of adequate transport in specific time periods and imbalanced preferences towards specific modes of transport. This is a relevant first step for the city of Lisbon to comprehensively diagnose vulnerabilities in the multimodal public transport network and to assess causal factors for the skewed distributions of demand, whether caused by the inadequacy of transport supply at specific time periods, lack of multimodal integration at destination areas, or reveal the domination of another mode of transport. Although the work represents a valuable contribution for the city to advance towards sustainable mobility, complementary qualitative research is recommended to better understand the complex interactions between human behaviour, the specific socioeconomic context of individuals and the range of traffic patterns found in each city area.
The conducted analysis of multimodal aspects pertaining to the Lisbon case suggest that decisions grounded in available traffic data provide an objective and transparent means to improve the cross-modal cooperation of public passenger transport operators and explore untapped synergies for multimodal and sustainable mobility planning.
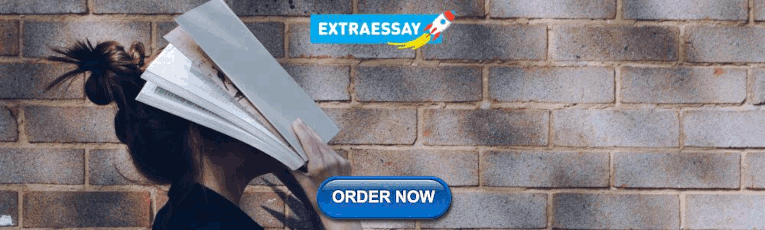
Availability of data and materials
The traffic data are available for collaborations in the context of the ILU project under non-disclosure agreements (NDAs) with CML, CARRIS and METRO.
https://www.portalviva.pt/pt/homepage/sobre-a-otlis/empresas-aderentes.aspx .
https://lisboainteligente.cm-lisboa.pt/ .
https://www.waze.com/en-GB/ .
https://www.tomtom.com/engb .
Abbreviations
Integrative Learning from Urban Data and Situational Context for City Mobility Optimization (project)
Herfindahl–Hirschman
Lisbon's Metropolitan Area
Metropolitan of Lisbon (subway operator)
Companhia Carris de Ferro de Lisboa (Bus and tram operator)
Comboios de Portugal (Train operator)
Operadores de Transportes da região de Lisboa (Public carriers in Lisbon's metropolitan area)
Global Positioning System
Lisbon's public bike sharing system
General Transit Feed Specification
Origin–Destination
Traffic Analysis Zones
Albuquerque, V., Oliveira, A., Barbosa, J. L., Rodrigues, R. S., Andrade, F., Dias, M. S., & Ferreira, J. C. (2021). Smart cities: Data-driven solutions to understand disruptive problems in transportation—The Lisbon Case Study. Energies, 14 (11), 3044.
Google Scholar
Allison, P. D. (1978). Measures of inequality. American Sociological Review, 43 , 865–880.
Almasi, M. H., Sadollah, A., Oh, Y., Kim, D. K., & Kang, S. (2018). Optimal coordination strategy for an integrated multimodal transit feeder network design considering multiple objectives. Sustainability, 10 (3), 734.
Aparicio, J. T., Arsenio, E., & Henriques, R. (2021). Understanding the impacts of the COVID-19 pandemic on public transportation travel patterns in the City of Lisbon. Sustainability, 13 (15), 8342.
Atluri, G., Karpatne, A., & Kumar, V. (2018). Spatiotemporal data mining: A survey of problems and methods. ACM Computing Surveys (CSUR), 51 (4), 1–41.
Barredo-Arrieta, A., Laña, I., & Del Ser, J. (2019). What lies beneath: A note on the explainability of black-box machine learning models for road traffic forecasting. In IEEE intelligent transportation systems conference (ITSC) (pp. 2232–2237).
Bendriss, S., & Benabdelhafid, A. (2011). Multimodal transport information system: Modelling approach for goods traceability. International Journal of Business Information Systems, 7 (4), 365–387.
Buehler, R., & Hamre, A. (2016). An examination of recent trends in multimodal travel behavior among american motorists. International Journal of Sustainable Transportation, 10 (4), 354–364.
Canitez, F. (2019). Pathways to sustainable urban mobility in developing megacities: A socio-technical transition perspective. Technological Forecasting and Social Change, 141 , 319–329.
Cepeliauskaite, G., Keppner, B., Simkute, Z., Stasiskiene, Z., Leuser, L., Kalnina, I., Kotovica, N., Andiņš, J., & Muiste, M. (2021). Smart-mobility services for climate mitigation in urban areas: Case studies of Baltic Countries and Germany. Sustainability, 13 (8), 4127.
Cerqueira, S., Arsénio, E., & Henriques, R. (2020). Integrative analysis of traffic and situational context data to support urban mobility planning. In European Transport Conference 2020 (ETC 2020) .
Cerqueira, S., Arsénio, E., & Henriques, R. (2021) Inference of multimodal and dynamic origin-destination matrices from incomplete smart-card data: A case study in the City of Lisbon. In European Transport Conference 2021 (ETC 2021) .
Cerqueira, S., Arsenio, E., & Henriques, R. (2021). Inference of dynamic origin-destination matrices with trip and transfer status from individual smart card data. In European Transport Conference 2021 (ETC 2021) .
Chlond, B. (2012). Making people independent from the car–multimodality as a strategic concept to reduce co 2-emissions. In Cars and carbon (pp. 269–293). Springer.
Choy, M. C., Srinivasan, D., & Cheu, R. L. (2003). Cooperative, hybrid agent architecture for real-time traffic signal control. IEEE Transactions on Systems, Man, and Cybernetics-Part A: Systems and Humans, 33 (5), 597–607.
Clark, W. A., Huang, Y., & Withers, S. (2003). Does commuting distance matter? Commuting tolerance and residential change. Regional Science and Urban Economics, 33 (2), 199–221.
Cowell, F. (2011). Measuring inequality . Oxford University Press.
Diana, M., & Mokhtarian, P. L. (2007). The individual desire to change a “modal basket” and its relationship with the actual and perceived use of different transport means. In 11th World congress on transport research .
Diana, M., & Mokhtarian, P. L. (2009). Desire to change one’s multimodality and its relationship to the use of different transport means. Transportation Research Part F: Traffic Psychology and Behaviour, 12 (2), 107–119.
Diana, M., & Pirra, M. (2016). A comparative assessment of synthetic indices to measure multimodality behaviours. Transportmetrica A: Transport Science, 12 (9), 771–793.
Dzeroski, S. (2009). Relational data mining. In Data mining and knowledge discovery handbook (pp. 887–911). Springer.
Fu, R., Zhang, Z., & Li, L. (2016). Using LSTM and GRU neural network methods for traffic flow prediction. In 31st Youth Academic annual conference of Chinese Association of Automation (YAC) (pp. 324–328). IEEE.
Gallop, C., Tse, C., & Zhao, J. (2012). A seasonal autoregressive model of Vancouver bicycle traffic using weather variables. In Transportation research board 91st annual meeting , number 12-2119.
Genders, W., & Razavi, S. (2016). Using a deep reinforcement learning agent for traffic signal control. arXiv preprint. arXiv:1611.01142 .
Groth, S. (2019). Multimodal divide: Reproduction of transport poverty in smart mobility trends. Transportation Research Part A: Policy and Practice, 125 , 56–71.
Groth, S., & Kuhnimhof, T. (2021). Multimodality in transportation. In R. Vickerman (Ed.), International encyclopedia of transportation (pp. 118–126). Elsevier.
Hackl, R., Raffler, C., Friesenecker, M., Kramar, H., Kalasek, R., Soteropoulos, A., Wolf-Eberl, S., Posch, P., & Tomschy, R. (2019). Promoting active mobility: Evidence-based decision-making using statistical models. Journal of Transport Geography, 80 , 102541.
Heinen, E., & Bohte, W. (2014). Multimodal commuting to work by public transport and bicycle: Attitudes toward mode choice. Transportation Research Record, 2468 (1), 111–122.
Henriques, R., & Madeira, S. C. (2018). BSIG: Evaluating the statistical significance of biclustering solutions. Data Mining and Knowledge Discovery, 32 (1), 124–161.
MathSciNet MATH Google Scholar
Jang, W. (2010). Travel time and transfer analysis using transit smart card data. Transportation Research Record, 2144 (1), 142–149.
MathSciNet Google Scholar
Javaid, A., Creutzig, F., & Bamberg, S. (2020). Determinants of low-carbon transport mode adoption: Systematic review of reviews. Environmental Research Letters, 15 (10), 103002.
John, A., Sugumaran, M., & Rajesh, R. S. (2016). Indexing and query processing techniques in spatio-temporal data. ICTACT Journal on Soft Computing, 6 (3).
Kuhnimhof, T., Chlond, B., & Von Der Ruhren, S. (2006). Users of transport modes and multimodal travel behavior: Steps toward understanding travelers’ options and choices. Transportation Research Record, 1985 (1), 40–48.
Kwoczek, S., Di Martino, S., & Nejdl, W. (2014). Predicting and visualizing traffic congestion in the presence of planned special events. Journal of Visual Languages & Computing, 25 (6), 973–980.
Lambert, J. H., Joshi, N. N., Peterson, K. D., & Wadie, S. M. (2007). Coordination and diversification of investments in multimodal transportation. Public Works Management & Policy, 11 (4), 250–265.
Leite, I., Finamore, A., & Henriques, R. (2020). Context-sensitive modeling of public transport data. In Transport Research Arena (TRA2020) , Helsinki.
Li, L., Jiang, R., He, Z., Chen, X. M., & Zhou, X. (2020). Trajectory data-based traffic flow studies: A revisit. Transportation Research Part C: Emerging Technologies, 114 , 225–240.
Li, Y., Zheng, Y., Zhang, H., & Chen, L. (2015). Traffic prediction in a bike-sharing system. In Proceedings of the 23rd SIGSPATIAL international conference on advances in geographic information systems, SIGSPATIAL ’15 (pp. 33:1–33:10). ACM.
Liao, Y., Gil, J., Pereira, R. H., Yeh, S., & Verendel, V. (2020). Disparities in travel times between car and transit: Spatiotemporal patterns in cities. Scientific Reports, 10 (1), 1–12.
Luo, X., Li, D., Yang, Y., & Zhang, S. (2019). Spatiotemporal traffic flow prediction with KNN and LSTM. Journal of Advanced Transportation . https://doi.org/10.1155/2019/4145353
Article Google Scholar
Mamoulis, N., Cao, H., Kollios, G., Hadjieleftheriou, M., Tao, Y., & Cheung, D. W. (2004). Mining, indexing, and querying historical spatiotemporal data. In Proceedings of the tenth ACM SIGKDD international conference on Knowledge discovery and data mining (pp. 236–245).
Munizaga, M. A., & Palma, C. (2012). Estimation of a disaggregate multimodal public transport origin–destination matrix from passive smartcard data from santiago, chile. Transportation Research Part C: Emerging Technologies, 24 , 9–18.
Nallaperuma, D., Nawaratne, R., Bandaragoda, T., Adikari, A., Nguyen, S., Kempitiya, T., De Silva, D., Alahakoon, D., & Pothuhera, D. (2019). Online incremental machine learning platform for big data-driven smart traffic management. IEEE Transactions on Intelligent Transportation Systems, 20 (12), 4679–4690.
Neves, F., Finamore, A., Madeira, S., & Henriques, R. (2021). Mining actionable patterns of road mobility from heterogeneous traffic data using biclustering. IEEE Transactions on Intelligent Transportation Systems . https://doi.org/10.1109/TITS.2021.3057240
Neves, F., Finamore, A., & Henriques, R. (2020). Efficient discovery of emerging patterns in heterogeneous spatiotemporal data from mobile sensors. In 17th EAI International conference on mobile and ubiquitous systems: Computing, networking and services (MobiQuitous).
Nobis, C. (2007). Multimodality: Facets and causes of sustainable mobility behavior. Transportation Research Record, 2010 (1), 35–44.
Olivková, I. (2017). Comparison and evaluation of fare collection technologies in the public transport. Procedia Engineering, 178 , 515–525.
Pakusch, C., Stevens, G., Boden, A., & Bossauer, P. (2018). Unintended effects of autonomous driving: A study on mobility preferences in the future. Sustainability, 10 (7), 2404.
Papadias, D., Tao, Y., Kanis, P., & Zhang, J. (2002). Indexing spatio-temporal data warehouses. In Proceedings 18th international conference on data engineering (pp. 166–175). IEEE.
Reichenbach, M. (2019). The multimodal transport user: A challenge for public transport? Transportation Research Procedia . https://doi.org/10.1016/j.trpro.2019.09.059
Rempe, F., Huber, G., & Bogenberger, K. (2016). Spatio-temporal congestion patterns in urban traffic networks. Transportation Research Procedia, 15 , 513–524. International symposium on enhancing highway performance (ISEHP) , June 14–16, 2016, Berlin. ISSN 2352-1465.
Rodrigues, F., Borysov, S. S., Ribeiro, B., & Pereira, F. C. (2017). A Bayesian additive model for understanding public transport usage in special events. IEEE Transactions on Pattern Analysis and Machine Intelligence, 39 (11), 2113–2126.
Rosenbluth, G. (1955). Measures of concentration. In Business concentration and price policy (pp. 55–99). Princeton University Press.
Sardinha, C., Finamore, A., & Henriques, R. (2021). Context-aware demand prediction in bike sharing systems: Incorporating spatial, meteorological and calendrical context. arXiv:2105.01125 .
Shannon, C. E. (1948). A mathematical theory of communication. Bell System Technical Journal, 27 (3), 379–423.
Soua, R., Koesdwiady, A. B., & Karray, F. (2016). Big-data-generated traffic flow prediction using deep learning and Dempster-Shafer theory. In International joint conference on neural networks (IJCNN) (pp. 3195–3202).
Sovacool, B. K., Kester, J., Noel, L., & de Rubens, G. Z. (2018). The demographics of decarbonizing transport: The influence of gender, education, occupation, age, and household size on electric mobility preferences in the Nordic region. Global Environmental Change, 52 , 86–100.
Susilo, Y. O., & Axhausen, K. W. (2014). Repetitions in individual daily activity–travel–location patterns: A study using the Herfindahl-Hirschman Index. Transportation, 41 (5), 995–1011.
Tahmasbi, B., Mansourianfar, M. H., Haghshenas, H., & Kim, I. (2019). Multimodal accessibility-based equity assessment of urban public facilities distribution. Sustainable Cities and Society, 49 , 101633.
Tang, L., Duan, Z., & Zhao, Y. (2019). Toward using social media to support ridesharing services: Challenges and opportunities. Transportation Planning and Technology, 42 (4), 355–379.
Tempelmeier, N., et al. (2019). Data4urbanmobility: Towards holistic data analytics for mobility applications in urban regions. In Companion Proceedings of The 2019 World Wide Web Conference .
Tomaras, D., Boutsis, I., & Kalogeraki, V. (2018). Modeling and predicting bike demand in large city situations. In 2018 IEEE International Conference on Pervasive Computing and Communications (PerCom) (pp. 1–10). IEEE.
Toqué, F., Khouadjia, M., Come, E., Trepanier, M., & Oukhellou, L. (2017). Short & long term forecasting of multimodal transport passenger flows with machine learning methods. In IEEE 20th international conference on intelligent transportation systems (ITSC) (pp. 560–566). IEEE.
Tsirimpa, A., Polydoropoulou, A., Pagoni, I., & Tsouros, I. (2019). A reward-based instrument for promoting multimodality. Transportation Research Part F: Traffic Psychology and Behaviour, 65 , 121–140.
Tympakianaki, A., Koutsopoulos, H. N., Jenelius, E., & Cebecauer, M. (2018). Impact analysis of transport network disruptions using multimodal data: A case study for tunnel closures in Stockholm. Case Studies on Transport Policy, 6 (2), 179–189.
Wibisono, A., Sina, I., Ihsannuddin, M. A., Hafizh, A., Hardjono, B., Nurhadiyatna, A., Jatmiko, W., et al. (2012) Traffic intelligent system architecture based on social media information. In International conference on advanced computer science and information systems (ICACSIS) (pp. 25–30). IEEE.
Wiering, M., Vreeken, J., Van Veenen, J., & Koopman, A. (2004). Simulation and optimization of traffic in a city. In IEEE intelligent vehicles symposium (pp. 453–458). IEEE.
Wong, K.-I., Wong, S. C., Tong, C., Lam, W., Lo, H. K., Yang, H., & Lo, H. (2005). Estimation of origin-destination matrices for a multimodal public transit network. Journal of Advanced Transportation, 39 (2), 139–168.
Wu, Z., Pan, S., Chen, F., Long, G., Zhang, C., & Philip, S. Y. (2020). A comprehensive survey on graph neural networks. IEEE Transactions on Neural Networks and Learning Systems, 32 , 4–24.
Yahya, S., & Noor, N. M. (2008). Strategic planning of an integrated smart card fare collection system–challenges and solutions. In 11th IEEE international conference on computational science and engineering-workshops (pp. 31–36). IEEE.
Yang, J., Zhang, X., Qiao, Y., Fadlullah, Z., & Kato, N. (2015). Global and individual mobility pattern discovery based on hotspots. In IEEE international conference on communications (ICC) (pp. 5577–5582). IEEE.
Zannat, K., & Choudhury, C. F. (2019). Emerging big data sources for public transport planning: A systematic review on current state of art and future research directions. Journal of the Indian Institute of Science, 99 (4), 601–619.
Zheng, Y., Capra, L., Wolfson, O., & Yang, H. (2014). Urban computing: Concepts, methodologies, and applications. ACM Transactions on Intelligent Systems and Technology (TIST), 5 (3), 1–55.
Download references
Acknowledgements
The authors thank the support of CARRIS, METRO and the Lisbon City Council ( Câmara Municipal de Lisboa ) and its Gabinete de Mobilidade and Centro de Operações Integrado for the data provision and valuable feedback.
This work is further supported by national funds through Fundação para a Ciência e Tecnologia under project ILU – Integrative Learning from Urban Data and Situational Context for City Mobility Optimization (DSAIPA/DS/0111/2018) – and INESC-ID pluriannual (UIDB/50021/2020).
Author information
Authors and affiliations.
LNEC I.P. & INESC-ID, Instituto Superior Técnico, University of Lisbon, Lisbon, Portugal
Carlos Lemonde
Department of Transport, LNEC I.P., Lisbon, Portugal
Elisabete Arsenio
INESC-ID, Instituto Superior Técnico, University of Lisbon, Lisbon, Portugal
Rui Henriques
You can also search for this author in PubMed Google Scholar
Contributions
All authors contributed to research and to writing-up present the article. All authors read and approved the final manuscript.
Corresponding author
Correspondence to Elisabete Arsenio .
Ethics declarations
Competing interests.
The authors declare that they have no competing interests.
Additional information
Publisher's note.
Springer Nature remains neutral with regard to jurisdictional claims in published maps and institutional affiliations.
See Tables 2 and 3 .
Rights and permissions
Open Access This article is licensed under a Creative Commons Attribution 4.0 International License, which permits use, sharing, adaptation, distribution and reproduction in any medium or format, as long as you give appropriate credit to the original author(s) and the source, provide a link to the Creative Commons licence, and indicate if changes were made. The images or other third party material in this article are included in the article's Creative Commons licence, unless indicated otherwise in a credit line to the material. If material is not included in the article's Creative Commons licence and your intended use is not permitted by statutory regulation or exceeds the permitted use, you will need to obtain permission directly from the copyright holder. To view a copy of this licence, visit http://creativecommons.org/licenses/by/4.0/ .
Reprints and permissions
About this article
Cite this article.
Lemonde, C., Arsenio, E. & Henriques, R. Integrative analysis of multimodal traffic data: addressing open challenges using big data analytics in the city of Lisbon. Eur. Transp. Res. Rev. 13 , 64 (2021). https://doi.org/10.1186/s12544-021-00520-3
Download citation
Received : 16 February 2021
Accepted : 11 November 2021
Published : 20 December 2021
DOI : https://doi.org/10.1186/s12544-021-00520-3
Share this article
Anyone you share the following link with will be able to read this content:
Sorry, a shareable link is not currently available for this article.
Provided by the Springer Nature SharedIt content-sharing initiative
- Multimodality
- Sustainable mobility
- Data science
- Smart cities
- Public transport
- Inclusive mobility
A review of multimodal transportation optimization model
Ieee account.
- Change Username/Password
- Update Address
Purchase Details
- Payment Options
- Order History
- View Purchased Documents
Profile Information
- Communications Preferences
- Profession and Education
- Technical Interests
- US & Canada: +1 800 678 4333
- Worldwide: +1 732 981 0060
- Contact & Support
- About IEEE Xplore
- Accessibility
- Terms of Use
- Nondiscrimination Policy
- Privacy & Opting Out of Cookies
A not-for-profit organization, IEEE is the world's largest technical professional organization dedicated to advancing technology for the benefit of humanity. © Copyright 2024 IEEE - All rights reserved. Use of this web site signifies your agreement to the terms and conditions.
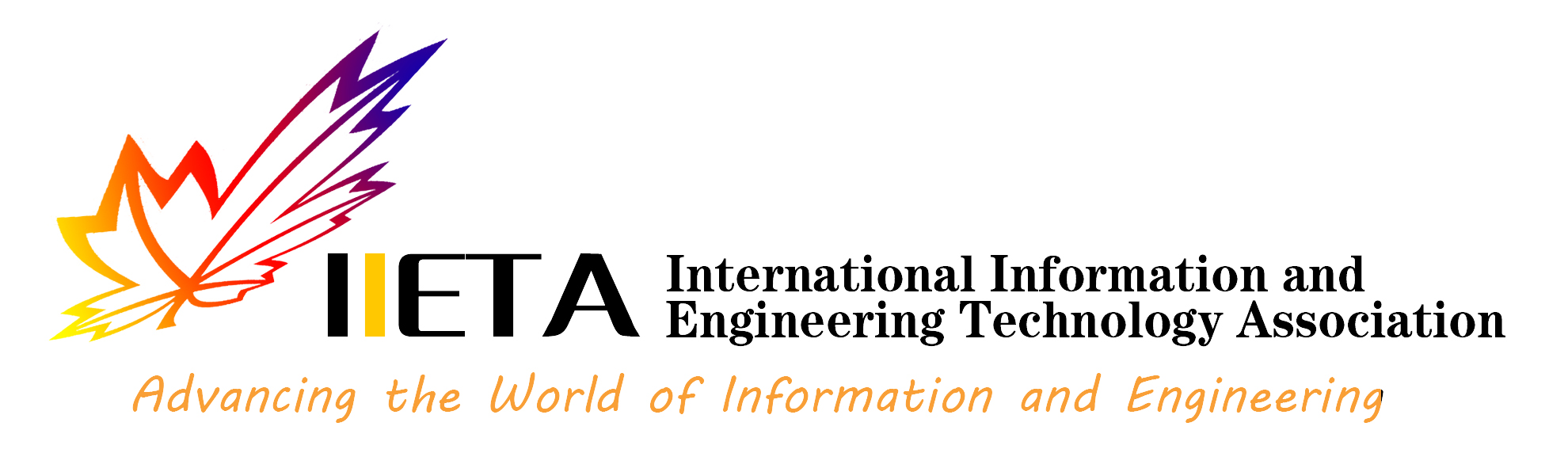
Search form
Adapting multimodal transportation infrastructure to changing transport and logistics routes.
© 2023 IIETA. This article is published by IIETA and is licensed under the CC BY 4.0 license ( http://creativecommons.org/licenses/by/4.0/ ).
OPEN ACCESS
The main purpose of the article is to study the infrastructure of multimodal transportation in the context of changes in transport and logistics routes. The object of research is the infrastructure of multimodal transportation. The main issue of the study is to determine ways to develop the infrastructure of multimodal transportation in the context of changing transport and logistics routes. For this, a modern methodology of functional modeling was used. As a result, a model was obtained that, in a graphical language, represents the main aspects of the development of the multimodal transportation infrastructure in the context of changes in transport and logistics routes. The focus of the study falls on Ukraine and its transport infrastructure. The article explores the development of multimodal transportation infrastructure in the context of changing transport and logistics routes. Showing the loss of the size of cargo transshipment by the seaports of the annexation of the area. The features of the functioning of seaports in an aggressive environment are described. Empirically, the potential volume of cargo transshipment and the volume of exports by seaports for a single country under different conditions have been established. Empirically established the volume of losses of container handling of goods. The study is limited by taking into account the multimodal transportation infrastructure of only one country taken.
multimodal transportation, multimodal terminal, logistics infrastructure, seaport, transport, Ukraine
Before the events of February 24, 2022, ≈75% of Ukraine's foreign trade turnover was accounted for by seaports. From February 24 to March 2022, they were all blocked. At the end of March, only three ports started working. Some ports were unblocked at the end of summer 2022 thanks to a grain deal. However, these ports have significantly reduced their capacity compared to the pre-war.
Before the events of February 24, Ukraine monthly exported up to 5 million tons of agricultural products through its ports. However, now due to their blockade, the country can transport only about 0.5 million tons of grain per month. This figure can be increased to 2-3 million tons by increasing the capacity of transshipment terminals, as well as railway and logistics crossings at the borders with the EU countries.
In addition, until the 24th, 33% of exports and ≈10% of GDP were provided by the mining and metallurgical complex. The metallurgical industry as a whole is export-oriented. However, in the conditions of the blocking of the Black Sea, the monthly loss of exports of metallurgical products from Ukraine reached $420 million. Today, the possibilities for exporting metallurgical products by rail are limited, and the EU seaports, to which metallurgists have reoriented, are not able to process all cargo. Consequently, the discrepancy between the infrastructure for the transportation and reloading of metallurgical products is another acute problem that needs to be addressed immediately.
Efficient logistics has a direct and close impact on the main indicators of the economic development of the state. In particular, the correlation coefficient between the Logistics Efficiency Index and GDP per capita (USD), calculated using the corresponding parameters of 40 countries of the world, is 0.873 points.
Understanding the scale of losses for the country's economy (and here we mean not only export earnings but also sales markets, foreign clients, etc.) as a result of blocking the ports of the Black and Azov Seas, the country's authorities are actively trying to look for new transport and logistics routes for export. In particular, at a meeting of experts on hydraulic engineering, they noted that in the context of the slowdown in ship inspections under the Black Sea Grain Initiative and the threat of unilateral refusal to implement the agreement, the Danube region remains the only stable route for the export of agricultural products from Ukraine and, as a result, an important element in ensuring world food security. One aspect of solving the problem is increased trade with the EU and new logistics on the western borders. Modernization and expansion of border infrastructure are one of the key elements of this strategy.
EU countries declare maximum and comprehensive support for Ukraine. Among them, they are pursuing a proactive policy in the context of finding and building new transport and logistics routes. Consequently, Ukraine should make fuller use of the opportunities that are opening up for it today. In the context of freight transport, in particular, this should manifest itself in the promotion of the development of multimodal transport infrastructure in Ukraine as part of the EU's integrated transport network.
The study considers all available types of routes within the transport infrastructure: rail, road, air transport, and sea.
The main purpose of the article is to study the features of the development of the infrastructure of multimodal transportation in the context of changes in transport and logistics routes. The object of research is the infrastructure of multimodal transportation. The structure of the article implies a review of the literature, a description of the methods used, a presentation of the main results of the study, their discussion, and the current conclusions. The main issue of the study is to determine ways to develop the infrastructure of multimodal transportation in the context of changing transport and logistics routes. For this, a modern methodology of functional modeling was used.
In the literature, studies of multimodal transportation and the development of multimodal transshipment infrastructure are represented by a wide range of fundamental and applied works. For example, theoretical issues of organizing multimodal transportation and developing the corresponding infrastructure were considered in the works of Dua and Sinha [1], Baykasotglu [2], Udo et al. [3].
The works of Fang et al. [4], Wong and Fuchs [5], Melnyk et al. [6, 7], and others are devoted to the issues of organization and management of multimodal transportation.
It is interesting to study a certain group of authors [8]. The researchers proposed mathematical modeling of the risks of multimodal transportation. The authors note that the introduction of their model in the organization of multimodal transportation, in particular, will allow for choosing the best logistics route in terms of security. However, the authors' study has not been tested on the example of real multimodal transportation. Of course, its results should be taken into account when planning new transport and logistics routes in the Ukraine-EU space. However, the possibility of taking into account the new geopolitical risk should be introduced into the mathematical model.
As it has been repeatedly indicated in the literature [9, 10], in the context of the intensive development of world trade and transport relations and global integration processes, it is important to increase the efficiency of multimodal transportation of goods between regions, countries, and continents, as well as to identify ways to introduce additional technologies on the way to creating a multimodal national company.
In the scientific and practical literature, it is noted that the creation of a multimodal company based on the principle of customer orientation, first of all, is carried out in accordance with the high standards of modern automation of all levels of logistics, technological and related processes that accompany the cycle of providing a wide range of services. The consumer to whom logistics services are provided is a component of the cargo delivery system. From these positions, the parameters of the quality of service provision are determined [11-13].
Interesting questions are raised in the scientific literature on the topic of our article. For example, various authors note [14-17] that an important component of measures to ensure the quality of a comprehensive service is a powerful technical base. Therefore, in addition to technological improvements, it is necessary to carry out certain measures for the reconstruction, and development of the production base, including through investment. Of course, there are obstacles on the way to the development of container transportation logistics in the country that does not depend on the enterprise, namely: imperfect tariffs for transportation by rail; changing customs regulations; high rates and port fees, etc. But the introduction of logistics principles into management makes it possible to integrate the enterprise into the international transport network, as well as to meet the needs of the national economy at the modern level.
Today, the transport system of Ukraine has a low level of development of transport infrastructure to ensure the proper volume of multimodal transportation. Ukraine lacks a sufficient number of multimodal transport terminals. Due to insufficient state support and the lack of an investment-friendly climate, the construction of new and the development of existing multimodal infrastructure facilities is not taking place. Multimodal transportation should become the basis for the development of the transport system of Ukraine. The transport network should be balanced, resource- and energy-efficient, taking into account the harmonious development of highways, railways, inland waterways, and other transport infrastructure based on multimodality [18, 19].
The issues of developing the infrastructure of multimodal transportation were studied in the works of Liu et al. [20], Molero et al. [21], and Lizbetin [22].
It should be noted that despite the significant scientific interest in the literature on this topic, the search for a modern methodical approach to determining ways to develop the infrastructure of multimodal transportation in the context of changing transport and logistics routes remains relevant and new. This is presented in our article.
In Ukraine, applied research on the development of the infrastructure of multimodal transportation has not yet become widespread. First of all, this is due to the insignificant involvement of Ukraine in the world's multimodal transportation in the pre-war period. And the law on such transportation in Ukraine was adopted relatively recently - in November 2022.
Today's conditions will necessitate a review of existing research on multimodal freight transport. In particular, in the context of changing transport and logistics routes and the need to ensure global food security, it is relevant to search for ways to develop and increase the capacity of the multimodal transportation infrastructure in Ukraine as part of the EU's integrated transport network.
In the study, theoretical and empirical methods of cognition are used in their dialectical combination. The main methods used in the study are statistical analysis, induction, graphical and analytical methods, methods for assessing structural dynamic shifts, comparisons, and the monographic method.
The study also uses the following assessment methods, in particular: theoretical generalization and comparison, induction and deduction are used to reveal the content of multimodal transportation, identify and generally characterize the types of infrastructure for such transportation; synthesis and economic analysis - assessed the state and trends in the development of the infrastructure of multimodal transportation in the context of changing transport and logistics routes; grouping method - to assess the loss of cargo turnover in war conditions in the context of the types of multimodal transportation infrastructure; economic, statistical and cross-border comparisons to assess the trend in the development of multimodal transport infrastructure in the EU countries; regression analysis - to study the impact of logistics efficiency (according to the Logistics Performance Index) on the economic development indicators of the state (in terms of GDP per capita, USD); cartographic and graphical method - for visual presentation of individual research results.
Data collection and analytical procedures were carried out within the framework of official statistical data of Ukraine, the country that serves as the basis of the study, and Eurostat data. The study compared countries neighboring Ukraine, such as Romania, Hungary, and Poland.
Functional and graphical modeling to represent the basic model for the development of multimodal transportation infrastructure in the context of changing transport and logistics routes. For this, IDEF technologies were used. Through the methodology of functional modeling, the system under study is presented as a set of interconnected blocks aimed at achieving the main goal of TL-0 (Development of the infrastructure of multimodal transportation in the context of changing transport and logistics routes).
The object of the transport and logistics infrastructure, which ensures the handling of goods in the process of transportation, is a cargo terminal. Depending on the type of transport used for the transportation of goods, the following types of multimodal terminals are distinguished: port/sea terminals (located on the territory (or nearby) of seaports, railway, domestic and airport terminals).
The multimodal transportation infrastructure includes a wide range of facilities: transfer stations, railway stations, airports and air terminals, sea (river) ports, container terminals, and logistics centers. To achieve the goal of the study, this paper will analyze the development and features of the functioning of:
- seaports. It is sea freight transportation that is the most popular and affordable type of multimodal transportation;
- "dry" ports - located on land, have rail and/or road links with the port (sea) terminal.
Ukraine has a wide range of multimodal transportation infrastructure facilities (Figure 1).
The largest in terms of throughput and cargo capacity are multimodal terminals located near seaports or on the territory of seaports (Figure 2).
In 2022, the EU remained the largest trading partner of Ukraine - the share of Ukrainian exports to the EU countries reached 63.2% (in 2021, the corresponding figure was 39.3%). In 2022, Ukraine increased its merchandise exports to the EU countries by 4.2% compared to 2021 (USD 26.8 billion to USD 28 billion, respectively), despite the fact that in 2022 Ukrainian exports decreased by 35.1%.
As of the beginning of 2023, in the western region of Ukraine, one of the largest (in terms of throughput and cargo capacity) infrastructure facilities for multimodal transportation is the Mostyska Container Terminal (Figure 3). Its design capacity today is 100,000 TEU. However, the operating company is working to increase the terminal's capacity to 200,000 TEU. On average, in 2022, the terminal was loaded at 65%.

Figure 1 . Infrastructure of multimodal transportation in Ukraine

Figure 2 . Infrastructure of maritime multimodal cargo transportation in Ukraine

Figure 3 . The infrastructure of overland multimodal freight transportation in Ukraine
In general, the network of inland ("dry") ports operates in close connection with the network of marine terminals. However, the challenges associated with changing transport and logistics routes, and the destruction of transport infrastructure necessitate an increase in the capacity of the western border crossings, and Danube seaports, as well as the development of infrastructure around them, including the development of multimodal transshipment terminals.
The need to develop the infrastructure of multimodal transshipment terminals at the Danube ports is argued by the need to solve the problem of establishing exports for companies in the mining and metallurgical complex, whose logistics costs as a whole increased by 4-6 times, depending on products and enterprises, among other purely military circumstances, is a consequence of increase in tariffs for freight rail transportation by 70%.
The development of the infrastructure of multimodal transportation in general for Ukraine can serve as one of the components of transport and logistics integration in the EU. Ukraine should make the most of the opportunities for the development of multimodal transportation, which will arise as a result of the implementation of infrastructure projects by the EU countries. For example Romania is renovating a wide track between Giurgiulesti and Galati. Thanks to the use of this route, Ukraine will be able to freely carry out cargo turnover with the Romanian port of Galati; Slovakia plans to create a railway transport corridor from Ukraine to the river port in Bratislava for the transportation of grain along the Danube River; Moldova has signed a Memorandum of Understanding with Ukraine on the development of railway communication. This will help increase the transportation of Ukrainian export cargo, including to the ports of the Danube region.
Achieving TL-0, according to modeling technology, involves several elements, presented in the diagram in Figure 4.
In general, the main functional model of TL-0 is shown in Figure 5.

Figure 4. Graphical diagram of the results of achieving TS-0

Figure 5 . The main functional model of TL-0
Thus, to achieve TL-0, the following processes must be followed:
TL-1. Formation of transport and logistics visa-free. It is necessary to develop the concluded agreements (the so-called “trade visa-free”, “transport visa-free”, “customs-free”, the EU initiative “Ways of Solidarity”, etc.), the operation of which, in the aggregate, will contribute to the establishment of alternative routes (both railway and automobile) export of Ukrainian agricultural products. and import of essential goods in the conditions of the blockade of seaports, speeding up the passage of Ukrainian goods across the border (including avoiding stops in the export of domestic products through automobile checkpoints), simplifying the procedure for documenting cargo at checkpoints one transit declaration and one guarantee for the movement of goods between Ukraine and 36 countries (EU countries, as well as the UK, Turkey, Serbia, North Macedonia, Iceland, Norway, Liechtenstein, and Switzerland)), simplifying the procedure for recognizing driver's documents, etc.
TL-2. Development of cooperation in the railway sector. Consultations are ongoing in government circles on the possibility of involving Ukraine in the Amber Train project and the creation of a regular rail freight line between Ukraine and the Baltic countries. For Ukraine, this may result, on the one hand, by strengthening its presence in the Baltic markets, and, on the other hand, by the possibility of using the ports of the Baltic Sea to export Ukrainian grain and metal products further to the world market. And Croatia offers its ports on the Adriatic coast for the transportation of Ukrainian grain to the countries of North Africa. However, the key point remains that the cargo to the ports of both the Baltic and Croatia will be delivered mainly by rail. Therefore, it is clear that rail freight transport will develop. This will require the modernization of the border infrastructure to increase the export capacity of Ukraine. And this will lead to an increase in demand for the services of multimodal transshipment terminals, as transport hubs and intermediaries between the Ukrainian seller and the European buyer.
TL-3. Development of multimodal transportation infrastructure on the western border. The development of multimodal transportation infrastructure on the western border of Ukraine is relevant and promising, given the possibility of including Ukraine in the New Silk Road, which connects Europe and China by container rail. In the summer of 2020, Ukrainian logistics companies have already begun to establish communications between Kyiv and other cities in China. Further from Kyiv, container trains followed through the territory of Belarus to the Brest-Malashevich border crossing on the Belarus-Poland border. However, today the transport and logistics routes that previously passed through the territory of Belarus are blocked. Obviously, containers from Asia can be reloaded in Western Ukraine and then sent to the EU countries. And in this context, Ukraine must provide European freight forwarders and customers with an efficiently functioning multimodal transportation infrastructure.
TL-4. Unblocking ports. The rapid reopening of the ports will be difficult due to the severely damaged infrastructure there. In the conditions of permanent growth of the population of the planet, and with it the demand for the products of the agro-industrial complex, the states of the world will strive to avoid a food crisis. In this context, Ukraine has a colossal export potential that needs to be realized here and now. And the economy needs foreign exchange earnings.
Firstly, to revive the functioning of the ports of the Danube and the multimodal terminals around them, it is necessary to deepen the operational bottom of the water areas of these ports. This will make it possible to receive cargo from large ships, improve the safety of navigation on the Danube, and maintain the declared depths. In addition, the renewal of the Danube fleet and port transshipment infrastructure is required.
In general, multimodal transshipment terminals in Western Ukraine are well-equipped. It provides a wide range of customs brokerage services. However, there are obstacles in the context of the revitalization of the functioning of these facilities: difficulties with crossing the border by rail due to different track widths in Ukraine (1520 mm) and EU countries (1435 mm). As a result, at checkpoints across the state border, it is necessary to replace wagon bogies or move goods to wagons with the required wheel pair width. This leads to significant downtime, and additional costs and does not allow optimal use of the existing throughput of checkpoints; limited number and throughput of checkpoints across the state border capable of serving rail freight traffic. Thus, in Ukraine, there are only 13 cargo border crossings (4 units - with Poland, 3 units - with Moldova, and 2 crossings each - with Romania, Slovakia, and Hungary). Despite the declared potential capacity of the western crossings in the amount of 6.6 million tons per month, it is actually possible to use only 3.8 million tons per month or 57.8%; higher overall dimensions of the rolling stock of Ukraine in comparison with the EU. Thus, large Ukrainian wagons can damage tunnels, bridges, platforms, and other objects of the nearby transport infrastructure, which often makes it impossible for them to move on the European railway.
We would like to note that the results of the study we obtained contain a number of parts of innovation. First of all, it concerns the fact that by analyzing the transport infrastructure of Ukraine, one can see and prove that it has all the necessary opportunities to acquire a high level in terms of logistics.
A separate result, which has elements of innovation, is the proposed functional model of ways to develop the infrastructure of multimodal transportation in the context of changing transport and logistics routes.
Discussing the results of the study, it is necessary to determine what will contribute to the revitalization of the functioning of the multimodal transportation infrastructure in Ukraine.
Of course, in order to revive the functioning of multimodal transshipment terminals, firstly, it would be worth developing and approving a strategy for the development of multimodal transportation. Discussing our results, we emphasize that in this study we empirically proved the importance and prospects of developing the infrastructure of multimodal transportation. Therefore, we believe that the next logical (natural) step should be the adoption of a strategy for the development of multimodal transportation, including the development of appropriate infrastructure.
However, today, in the absence of a state strategy for the development of multimodal transportation, the revival of the functioning of multimodal transshipment terminals can be facilitated by the full implementation of state projects for the restoration and development of transport infrastructure.
Along with the development of state multimodal transshipment terminals, the economic losses of Ukraine as a result of the loss of control over the ports of the Sea of Azov and the decrease in the congestion of the ports of Odesa may partially minimize state assistance to the development of private multimodal transshipment terminals on the Danube.
To revive the functioning of land multimodal transshipment terminals, it is important to eliminate the infrastructural constraints of rail transport. In this context, in cross-border cooperation in the western direction, the state should pursue a proactive policy in the context of the implementation of infrastructure projects related to the construction/restoration/extension of European routes through the territory of the border areas with the EU. In addition, it requires a solution to the issue of the overall dimensions of the rolling stock. Thus, large wagons can damage tunnels, bridges, platforms, and other objects of the nearby transport infrastructure, which often makes it impossible for them to move on the European railway. In May 2022, Hungary preliminary agreed on the use of several types of Ukrainian wagons on certain routes of its railway network. We believe that at the state level, activities should be intensified to coordinate similar routes for Ukrainian railcars in other neighboring countries.
The existing theories and practical aspects in the selected issues have their place, however, discussing the results we obtained, it should be noted that they have a certain value in the framework of the development of the strategy of multimodal transportation. This is manifested through the presented functional model of ways to develop the infrastructure of multimodal transportation in the context of changing transport and logistics routes. Also, it should be noted in the discussion that all results are presented within the framework of one country - Ukraine. Today, geopolitical events can continue to affect the transport routes and infrastructure needs of Ukraine, and precisely thanks to the help of countries such as Poland and Romania, this will only increase the positive impact.
The presented limitations should be discussed separately. They are mainly presented in the form of taking into account only one country and its infrastructure. In the future, the framework of the study should be expanded and other countries should be taken into account.
As part of our study, we chose the transport and logistics specifics of Ukraine. In Ukraine, the infrastructure of multimodal transportation is still in the initial stages of development. This is due, firstly, to the long absence in Ukraine of institutional support for the implementation of this type of transportation, and secondly, to the insignificant involvement of Ukraine in the world multimodal transportation in the pre-war period. However, today the country faces a number of challenges in the field of cargo transportation: the temporary loss of ports, the temporary loss of the ports of the Sea of Azov, the blocking of the work of individual ports of the Black Sea, a strict inspection of ships on the Bosphorus when passing the so-called "grain corridor". As a result of the termination of the usual logistics chains, the volume of cargo transshipment, for example, by the seaports of Ukraine in 2022 compared to 2021, almost tripled.
Redirection of the flow of goods of Ukraine towards the EU, confirming the prospects and efficiency of such transport and logistics routes (in 2022 compared to 2021: commodity exports to the EU countries increased by 4.2% with an overall reduction of 35.1%; Danube ports handled three times more cargo).
Considering the scale of the losses of the country's economy and understanding the need to realize its export potential, preserve existing sales markets and supply chains, and replenish the budget with export earnings, Ukraine should make efforts to enhance the development of multimodal transportation infrastructure in the Ukraine-EU space.
The development of multimodal transportation infrastructure, in addition to a pronounced conical effect on the national economy, will help increase the country's competitiveness in the global transport services market, develop a network of transport corridors, and integrate transport infrastructure into the global transport system.
In this context, first of all, it is necessary to accelerate the implementation of measures for the development of multimodal transshipment terminals, provided for by the Recovery Plan of Ukraine. The government's policy to eliminate infrastructural restrictions on railway transport needs to be activated (implementation of infrastructure projects related to the construction/restoration/extension of European routes by the territory of the regions bordering the EU). In addition, the development and approval of a strategy for the development of multimodal transportation is relevant.
With the constant development of transport infrastructure, it is possible to strengthen food security. For example, with access to transportation across the sea, it is possible to deliver a significant amount of grain, as Ukraine exports.
The main result of the study is the presented functional model, which characterizes the key ways to develop the infrastructure of multimodal transportation in the context of changing transport and logistics routes.
The study is limited by taking into account the multimodal transportation infrastructure of only one country taken. The prospect of further research by the authors is to study the foreign experience of state assistance to the development of multimodal transportation infrastructure in the context of its application in Ukraine.
Further research should be devoted to conducting specific case studies on the impact of geopolitical developments on multimodal infrastructure and trade flows; forecasting infrastructure needs and requirements for the transport network under different scenarios of political and economic conditions; assessing the availability of funding and partnerships to support the development of multimodal infrastructure; evaluating the effectiveness of policies and regulations that support multimodal transport.
This work was supported by the International Visegrad Fund (Visegrad+Grant No. 22220189).
[1] Dua, A., Sinha, D. (2019). Quality of multimodal freight transportation: A systematic literature review. World Review of Intermodal Transportation Research, 8(2): 167-194. https://doi.org/10.1504/WRITR.2019.099136 [2] Baykasoğlu, A. (2019). A review of fleet planning problems in single and multimodal transportation systems, Transportmetrica A: Transport Science, 15(2): 631-697. https://doi.org/10.1080/23249935.2018.1523249 [3] Udo, E.I., Huaccho Huatuco, L., Ball, P.D. (2019). Multimodal Freight Transportation: Sustainability Challenges. In: Ball, P., Huaccho Huatuco, L., Howlett, R., Setchi, R. (eds) Sustainable Design and Manufacturing 2019. KES-SDM 2019. Smart Innovation, Systems and Technologies, vol 155. Springer, Singapore. https://doi.org/10.1007/978-981-13-9271-9_12 [4] Fang, X., Cao, C., Chen, Z., Chen, W., Ni, L., Ji, Z., Gan, J. (2019). Using mixed methods to design service quality evaluation indicator system of railway container multimodal transport. Science Progress, 103(1) https://doi.org/10.1177/0036850419890491 [5] Wong, W.F., Fuchs, S. (2022). Multimodal transport networks. FRB Atlanta Working Paper No. 2022-13. https://dx.doi.org/10.29338/wp2022-13 [6] Melnyk, M., Shchehliuk, S., Leshchukh, I., Litorovych, O. (2021). Digitalization of the economies of Ukraine and Poland: National and local dimensions. Economic Annals-XXI, 191(7-8(1)): 30-42. https://doi.org/10.21003/ea.V191-03 [7] Melnyk, M., Leshchukh, I., Hlynskyy, N. (2022). The impact of the oblast center on regional socio-economic development: The center-periphery dimension. Agricultural and Resource Economics: International Scientific E-Journal, 8(1): 89-114. https://doi.org/10.51599/are.2022.08.01.05 [8] Bazyliuk, V., Shtangret, A., Sylkin, O., Bezpalko, I. (2019). Comparison of institutional dynamics of regional development publishing and printing activities in Ukraine: methodological and practical aspects. Business: Theory and Practice, 20: 116-122. https://doi.org/10.3846/btp.2019.11 [9] Sylkin, O., Shtangret, A., Ogirko, O., Melnikov, A. (2018). Assessing the financial security of the engineering enterprises as preconditions of application of anti-crisis management: Practical aspect. Business and Economic Horizons, 14(4): 926-940. http://dx.doi.org/10.22004/ag.econ.287238 [10] Sylkin, O., Kryshtanovych, M., Zachepa, A., Bilous, S., Krasko, A. (2019). Modeling the process of applying anti-crisis management in the system of ensuring financial security of the enterprise. Business: Theory and Practice, 20: 446-455. https://doi.org/10.3846/btp.2019.41 [11] Rushchyshyn, N., Medynska, T., Nikonenko, U., Kostak, Z., Ivanova, R. (2021). Regulatory and legal component in ensuring state’s financial security. Business: Theory and Practice, 22(2): 232-240. https://doi.org/10.3846/btp.2021.13580 [12] Nikonenko, U., Shtets, T., Kalinin, A., Dorosh, I., Sokolik, L. (2022). Assessing the policy of attracting investments in the main sectors of the economy in the context of introducing aspects of industry 4.0. International Journal of Sustainable Development and Planning, 17(2): 497-505. https://doi.org/10.18280/ijsdp.170214 [13] Yemelyanov, V., Nikonenko, U., Sytnyk, Y., Okhrimenko, I., Shulga, A. (2022). A model for countering the information and technical threats of intellectual capital management of innovation-oriented systems in the engineering sector. Ingénierie des Systèmes d’Information, 27(5): 799-806. https://doi.org/10.18280/isi.270513 [14] Indriastiwi, F., Hadiwardoyo, S.P., Nahry. (2023). The integrated strategic planning of multimodal freight transport network under infrastructure budget limitation. International Journal of Transport Development and Integration, 7(1): 1-11. https://doi.org/10.18280/ijtdi.070101 [15] Rindone, C., Panuccio, P. (2023). Planning for risk reduction in the transport system at urban level. International Journal of Transport Development and Integration, 7(1): 27-34. https://doi.org/10.18280/ijtdi.070104 [16] Boviatsis, M., Vlachos, G. (2023). Proactive steps towards sustainable shipping: Assessing maritime accidents of the past to prevent similar incidents in the future. International Journal of Transport Development and Integration, 7(1): 13-20. https://doi.org/10.18280/ijtdi.070102 [17] Alazzam, F.A.F., Saleh, A.J., Aldrou, K.K.A.R. (2020). Impact of pandemic COVID-19 on the legal regulation of world trade activity using the example of the medical supplies. Wiadomości Lekarskie, 73(7): 1521-1527. https://doi.org/10.36740/WLek202007139 [18] Shakhatreh, H. (2023) Payment of canal dues by carriers carrying out international overseas transportation - A case of legal discrimination. Studia Iuridica Lublinensia. 32(1). https://doi.org/10.17951/sil.2023.32.1.251-273 [19] Al Azzam, F.A.F. (2019). The adequacy of the international cooperation means for combating cybercrime and ways to modernize it. JANUS. NET E-Journal of International Relations, 10(1): 64-81. https://doi.org/10.26619/1647-7251.10.1.5 [20] Liu, Z., Wang, Z., Cheng, Q., Yin, R., Wang, M. (2021). Estimation of urban network capacity with second-best constraints for multimodal transport systems, Transportation Research Part B: Methodological, 152: 276-294. https://doi.org/10.1016/j.trb.2021.08.011 [21] Molero, G.D., Santarremigia, F.E., Poveda-Reyes, S. (2019). Key factors for the implementation and integration of innovative ICT solutions in SMEs and large companies involved in the multimodal transport of dangerous goods. European Transport Research Review, 11: 28. https://doi.org/10.1186/s12544-019-0362-8 [22] Ližbetin, J. (2019). Methodology for determining the location of intermodal transport terminals for the development of sustainable transport systems: A case study from Slovakia. Sustainability, 11(5): 1230. https://doi.org/10.3390/su11051230
Phone: + 1 825 436 9306
Email: [email protected]
Subscription
Language support
Please sign up to receive notifications on new issues and newsletters from IIETA
Select Journal/Journals:
Copyright © 2024 IIETA. All Rights Reserved.
- Help & FAQ
Multimodal freight transportation planning : a literature review
- Operations Planning Accounting & Control
- Process Analytics
Research output : Contribution to journal › Article › Academic › peer-review
Access to Document
- 10.1016/j.ejor.2013.06.055
Fingerprint
- Transport Social Sciences 100%
- Planning Social Sciences 100%
- Literature Reviews Social Sciences 100%
- Literature Social Sciences 25%
- Paper Social Sciences 25%
- Operations Research Social Sciences 25%
T1 - Multimodal freight transportation planning : a literature review
AU - Steadie Seifi, M.
AU - Dellaert, N.P.
AU - Nuijten, W.P.M.
AU - Woensel, van, T.
AU - Raoufi, R.
N2 - Multimodal transportation offers an advanced platform for more efficient, reliable, flexible, and sustainable freight transportation. Planning such a complicated system provides interesting areas in Operations Research. This paper presents a structured overview of the multimodal transportation literature from 2005 onward. We focus on the traditional strategic, tactical, and operational levels of planning, where we present the relevant models and their developed solution techniques. We conclude our review paper with an outlook to future research directions.
AB - Multimodal transportation offers an advanced platform for more efficient, reliable, flexible, and sustainable freight transportation. Planning such a complicated system provides interesting areas in Operations Research. This paper presents a structured overview of the multimodal transportation literature from 2005 onward. We focus on the traditional strategic, tactical, and operational levels of planning, where we present the relevant models and their developed solution techniques. We conclude our review paper with an outlook to future research directions.
U2 - 10.1016/j.ejor.2013.06.055
DO - 10.1016/j.ejor.2013.06.055
M3 - Article
SN - 0377-2217
JO - European Journal of Operational Research
JF - European Journal of Operational Research
Academia.edu no longer supports Internet Explorer.
To browse Academia.edu and the wider internet faster and more securely, please take a few seconds to upgrade your browser .
Enter the email address you signed up with and we'll email you a reset link.
- We're Hiring!
- Help Center
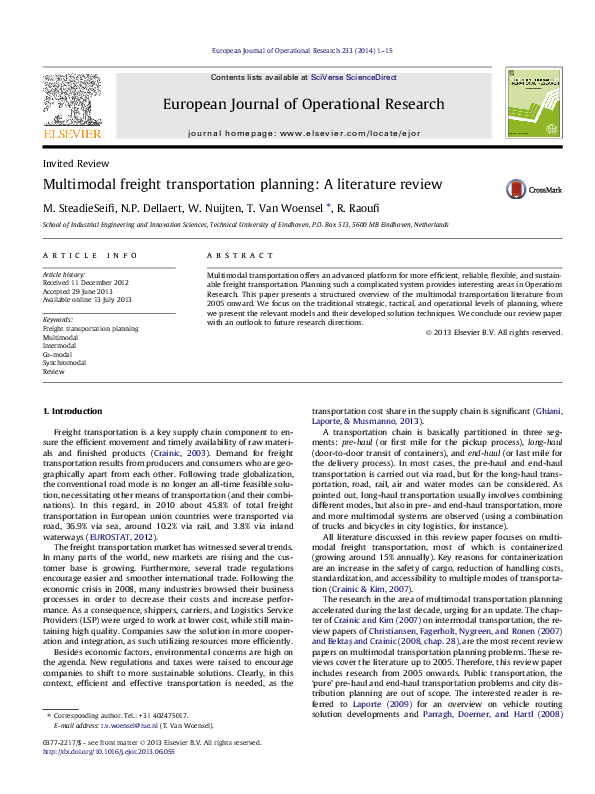
Multimodal freight transportation planning: A literature review

Related Papers
Transportation Planning and Technology
Cathy Macharis
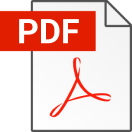
Cathy Macharis , Ethem Pekin
Bart Jourquin
Jaouad Boukachour
The exponential growth of the flow of goods and passengers, fragility of certain products and the need for the optimization of transport costs impose on carriers to use more and more multimodal transport. In addition, the need for intermodal transport policy has been strongly driven by environmental concerns and to benefit from the combination of different modes of transport to cope with the increased economic competition.This research is mainly concerned with the Intermodal Terminal Location Problem introduced recently in scientific literature which consists to determine a set of potential sites to open and how to route requests to a set of customers through the network while minimizing the total cost of transportation. We begin by presenting a description of the problem. Then, we present a mathematical formulation of the problem and discuss the sense of its constraints. The objective function to minimize is the sum of road costs and railroad combined transportation costs.As the pr...
Astrīda Rijkure
Jean-paul Rodrigue
Agunbiade Olutayo
Erim Report Series Research in Management Erasmus Research Institute of Management
Amir Gharehgozli
RELATED PAPERS
Journal of Transport Geography
S. Limbourg
Tom van Lier
Tomislav Rozic , Kristijan Rogić , Ivona Bajor
European Transport Research Review
Transportation Research Part E: Logistics and Transportation Review
Isabelle Thomas
Ellen J . Eftestöl , Mervi Rajahonka
paul rodrigues
Transportation Science
Gianfranco Guastaroba
In the course of globalization, the pressure on today's …
Lorena Sales
Transportation Research Part E-logistics and Transportation Review
Stratos Papadimitriou
Transportation Research Part E: Logistics and …
Shahin Gelareh
Development of Dry Ports
Waldo Krugell
Applied Soft Computing
Nenad Mladenović
Transportation Research Record
Tschangho Kim
James Amdal
Jhr Van Duin
European Journal of Operational Research
Marielle Christiansen , Teodor Crainic
Transportation Research Part E: Logistics …
Transportation
Inge Vierth
Ioannis Mallidis
Matin Bagherpour
OLADIPO ADEWUMI
Rickard Bergqvist
Francisco Sanchez
Journal of Business Logistics
Stanley Griffis
Takaaki Ohishi , Anibal T Azevedo , Andréa Leda de Oliveira Ramos
Nimesha Indika
Metin Türkay , Metin Türkay
IJESRT Journal
Maritime Economics & Logistics
Olcay Polat , Hans-Otto Guenther
guolei tang
Violeta Roso
Maritime Policy and Management
ATHANASIOS PALLIS
Wenjing Guo
RELATED TOPICS
- We're Hiring!
- Help Center
- Find new research papers in:
- Health Sciences
- Earth Sciences
- Cognitive Science
- Mathematics
- Computer Science
- Academia ©2024

Journal of Materials Chemistry A
Nanoscale spatial and time-resolved mapping in perovskite and organic solar cells: a multimodal technique to visualize the photoinduced charge dynamics.
Recently, perovskite solar cells (PSCs) and organic solar cells (OSCs) have emerged as solution-processable photovoltaic (PV) technologies with certified power conversion efficiencies (PCE) of about 26.1%, and 19.4% respectively. However, some challenges such as charge generation, separation, transport, collection, and recombination at the nanoscale still exist in fully controlling and understanding the performance-limiting factors in the photoactive layers of OSC and PSC devices. Although several efforts to address the above phenomena have been reported, the origin of the nanoscale defects and how they lead to performance losses have not yet been fully understood. This review aims to discuss in-depth nanoscale spatial and time-resolved mapping for OSCs and PSCs and understand their roles in improving PV performance. A comprehensive multimodal technique to map the charge carrier photodynamics at nanoscale such as charge generation, separation, transport, collection, and recombination in OSCs and PSCs is presented, which have potential to evaluate the photodynamics related to local heterogeneities in the active layers of corresponding devices.
- This article is part of the themed collection: Journal of Materials Chemistry A Recent Review Articles
Article information

Download Citation
Permissions.

M. B. Faheem, Y. Zhang, M. B. Saud, H. Li, P. I. Kaswekar and Q. Qiao, J. Mater. Chem. A , 2024, Accepted Manuscript , DOI: 10.1039/D4TA01773K
This article is licensed under a Creative Commons Attribution 3.0 Unported Licence . You can use material from this article in other publications without requesting further permissions from the RSC, provided that the correct acknowledgement is given.
Read more about how to correctly acknowledge RSC content .
Social activity
Search articles by author.
This article has not yet been cited.
Advertisements
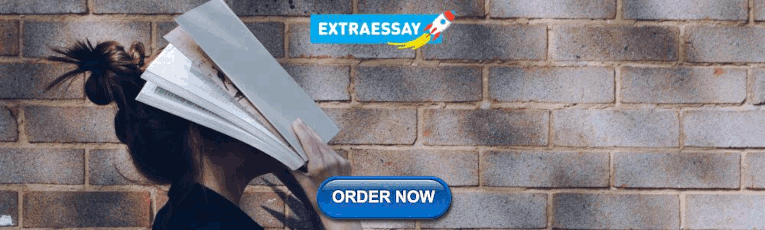
IMAGES
VIDEO
COMMENTS
Abstract. Multimodal transportation offers an advanced platform for more efficient, reliable, flexible, and sustainable freight transportation. Planning such a complicated system provides interesting areas in Operations Research. This paper presents a structured overview of the multimodal transportation literature from 2005 onward.
PDF | On Oct 1, 2015, Aman Dua and others published The Multimodal Transportation : Research Trend and Literature Review | Find, read and cite all the research you need on ResearchGate
According to EUROSTAT, in 2018, across the 28 countries members of the EU, 76.50% of freight was transported by road, 18.00% by rail and the remaining 5.50% by inland waterways. The literature on multimodal transportation is very wide and in constant growth. The goal of this paper is to provide a review of the literature on optimization models ...
The COVID-19 pandemic has severely impacted international economics and trade, including cargo transportation. As a result, enhancing the resilience of transport and logistics in the post-COVID-19 era has become a general trend. Multimodal transport, with its advantages of speed, large volume and multiple modes, has increasingly gained attention from countries worldwide. However, multimodal ...
The transport sector considerably impacts global warming because it accounts for over a quarter of all greenhouse gas (GHG) and a third of all final energy consumption [].These circumstances, combined with a growing demand for green solutions, have led to increasing interest in multimodal freight transport (MFT), which combines two or more transport modes and is considered a strategy for ...
The literature review introduced in the next section aims to update the last reviews with the large amount of research that has been conducted since 2008. Furthermore, the current state of research on stochastic service network design and network flow problems is also explicitly included. ... can support multimodal freight transport systems ...
A Literature Review On Multimodal Freight Transportation Planning Under Disruptions. E E Rosyida 1,2, B Santosa 1 and I N Pujawan 1. Published under licence by IOP Publishing Ltd IOP Conference Series: Materials Science and Engineering, Volume 337, International Conference on Industrial and Systems Engineering (IConISE) 2017 29-30 August 2017, Denpasar, Bali, Indonesia Citation E E Rosyida ...
Worldwide public transport systems are exposed to disruptions caused by malfunctions, accidents, maintenance, reduced fleet, and disasters, compromising mobility. Transport networks' multimodal planning and management can be explored to increase their robustness against these events. In this context, this research paper proposes and empirically compares methods to assess the robustness of a ...
Efforts are made to reduce cost and time, but there are other dimensions of multimodal transportation as well. In this paper authors has analysed literature with a focus on quality perspective multimodal transportation using systematic literature review method. The research in area of multimodal transportation is in nascent stage.
Abstract: This paper offers a literature review on supply chain and multimodal transportation risk management system with the. aim of to provide a comp rehensive concept for the management of ...
Multimodality can be simultaneously understood as a property of the transport system, as a transport policy strategy and as a dimension of individual travel behaviour, forming a tridimensional perspective [].Within the later dimension, multimodality is commonly defined as the use of more than one transport mode to complete a trip. This section first recovers essential concepts and literature ...
Abstract and Figures. Purpose: Multimodal freight transport developed in the transportation sector as an alternative to unimodal transport faced with the challenges brought by the growing global ...
Using a network science approach, multimodal transport systems can be described as multilayer networks, where the networks associated to different transport modes are not considered in isolation, but as a set of interconnected layers. Despite the importance of multimodality in modern cities, a unified view of the topic is currently missing.
Business, Economics. 2017. 45. Purpose: Multimodal freight transport developed in the transportation sector as an alternative to unimodal transport faced with the challenges brought by the growing global demand for transporting goods. Multimodal transport is the transportation of goods using at least two modes of transport, usually door-to-door.
Synchromodal transportation is a novel multimodal transportation concept. It builds on a collaboration of shippers and logistic service providers to enable real-time switching between transport modes and mode-free transport bookings, enabling more flexible and sustainable freight transportation. This paper summarizes the current state of research since 2010 by means of a systematic literature ...
The major component of logistic system is multimodal transportation. This paper presents the various alternative methodologies available to multimodal transportation planning. There is an application need for network modeling approach the optimization of people and freight transportation with different techniques. In the process of selecting the most efficient transportation modes, a ...
The literature review has identified challenges and opportunities associated with multimodal cargo transport, but these findings need to be further explored in the Middle East, particularly the UAE. This study will shed light on the impact of multimodal cargo transport strategies on the local economy of the UAE, as well as on international ...
Literature Review. In the literature, studies of multimodal transportation and the development of multimodal transshipment infrastructure are represented by a wide range of fundamental and applied works. ... Estimation of urban network capacity with second-best constraints for multimodal transport systems, Transportation Research Part B ...
This paper presents a structured overview of the multimodal transportation literature from 2005 onward. We focus on the traditional strategic, tactical, and operational levels of planning, where we present the relevant models and their developed solution techniques. We conclude our review paper with an outlook to future research directions.
All literature discussed in this review paper focuses on multimodal freight transportation, most of which is containerized (growing around 15% annually). Key reasons for containerization are an increase in the safety of cargo, reduction of handling costs, standardization, and accessibility to multiple modes of transportation (Crainic & Kim, 2007).
METHODOLOGY. This literature review is conducted using a desktop research methodology; i.e. our study reviews articles related to multimodal transportation published in major academic journals and conference papers addressing multimodal transportation. Published papers are collected from 2000 to 2017.
The evolving economic and technological landscape has brought about significant changes in travel behaviors and traffic patterns. These changes have led to the emergence of complex, multi-modal travel demands that interact with transportation networks, posing new challenges in transportation analysis and control. The primary objective of this study is to address these challenges by improving ...
This study aims to explore key aspects contributing to the successful applications of the SE concept across diverse industries by conducting a systematic literature review (SLR). Out of 4848 articles, 57 peer-reviewed articles in two databases published between 2013 and 2022 were subjected to descriptive and content analysis.
In this article, we aim to review contemporary literature on multimodal freight transport ation network design (MFTND), which is a most common concern of sc holars, practitioners, and policymakers ...
Recently, perovskite solar cells (PSCs) and organic solar cells (OSCs) have emerged as solution-processable photovoltaic (PV) technologies with certified power conversion efficiencies (PCE) of about 26.1%, and 19.4% respectively. However, some challenges such as charge generation, separation, transport, coll Journal of Materials Chemistry A Recent Review Articles