| | ( ) and Additional contact information Ercan Oztemel: Marmara University Engineering Faculty Samet Gursev: Marmara University Engineering Faculty , 2020, vol. 31, issue 1, No 9, 127-182 Abstract Manufacturing industry profoundly impact economic and societal progress. As being a commonly accepted term for research centers and universities, the Industry 4.0 initiative has received a splendid attention of the business and research community. Although the idea is not new and was on the agenda of academic research in many years with different perceptions, the term “Industry 4.0” is just launched and well accepted to some extend not only in academic life but also in the industrial society as well. While academic research focuses on understanding and defining the concept and trying to develop related systems, business models and respective methodologies, industry, on the other hand, focuses its attention on the change of industrial machine suits and intelligent products as well as potential customers on this progress. It is therefore important for the companies to primarily understand the features and content of the Industry 4.0 for potential transformation from machine dominant manufacturing to digital manufacturing. In order to achieve a successful transformation, they should clearly review their positions and respective potentials against basic requirements set forward for Industry 4.0 standard. This will allow them to generate a well-defined road map. There has been several approaches and discussions going on along this line, a several road maps are already proposed. Some of those are reviewed in this paper. However, the literature clearly indicates the lack of respective assessment methodologies. Since the implementation and applications of related theorems and definitions outlined for the 4th industrial revolution is not mature enough for most of the reel life implementations, a systematic approach for making respective assessments and evaluations seems to be urgently required for those who are intending to speed this transformation up. It is now main responsibility of the research community to developed technological infrastructure with physical systems, management models, business models as well as some well-defined Industry 4.0 scenarios in order to make the life for the practitioners easy. It is estimated by the experts that the Industry 4.0 and related progress along this line will have an enormous effect on social life. As outlined in the introduction, some social transformation is also expected. It is assumed that the robots will be more dominant in manufacturing, implanted technologies, cooperating and coordinating machines, self-decision-making systems, autonom problem solvers, learning machines, 3D printing etc. will dominate the production process. Wearable internet, big data analysis, sensor based life, smart city implementations or similar applications will be the main concern of the community. This social transformation will naturally trigger the manufacturing society to improve their manufacturing suits to cope with the customer requirements and sustain competitive advantage. A summary of the potential progress along this line is reviewed in introduction of the paper. It is so obvious that the future manufacturing systems will have a different vision composed of products, intelligence, communications and information network. This will bring about new business models to be dominant in industrial life. Another important issue to take into account is that the time span of this so-called revolution will be so short triggering a continues transformation process to yield some new industrial areas to emerge. This clearly puts a big pressure on manufacturers to learn, understand, design and implement the transformation process. Since the main motivation for finding the best way to follow this transformation, a comprehensive literature review will generate a remarkable support. This paper presents such a review for highlighting the progress and aims to help improve the awareness on the best experiences. It is intended to provide a clear idea for those wishing to generate a road map for digitizing the respective manufacturing suits. By presenting this review it is also intended to provide a hands-on library of Industry 4.0 to both academics as well as industrial practitioners. The top 100 headings, abstracts and key words (i.e. a total of 619 publications of any kind) for each search term were independently analyzed in order to ensure the reliability of the review process. Note that, this exhaustive literature review provides a concrete definition of Industry 4.0 and defines its six design principles such as interoperability, virtualization, local, real-time talent, service orientation and modularity. It seems that these principles have taken the attention of the scientists to carry out more variety of research on the subject and to develop implementable and appropriate scenarios. A comprehensive taxonomy of Industry 4.0 can also be developed through analyzing the results of this review. ; ; ; ; ; (search for similar items in EconPapers) 2020 (110) (external link) Abstract (text/html) Access to the full text of the articles in this series is restricted. This item may be available elsewhere in EconPapers: for items with the same title. BibTeX RIS (EndNote, ProCite, RefMan) HTML/Text This journal article can be ordered from for this article Journal of Intelligent Manufacturing is currently edited by in Journal of Intelligent Manufacturing from Bibliographic data for series maintained by Sonal Shukla ( ) and Springer Nature Abstracting and Indexing ( ). | | Browse Econ Literature- Working papers
- Software components
- Book chapters
- JEL classification
More features- Subscribe to new research
RePEc BiblioAuthor registration. - Economics Virtual Seminar Calendar NEW!
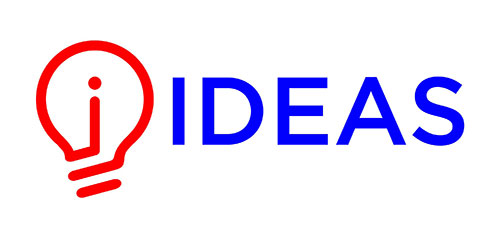 Literature review of Industry 4.0 and related technologies- Author & abstract
- 109 Citations
- Related works & more
Corrections(Marmara University Engineering Faculty) Suggested CitationDownload full text from publisher. Follow serials, authors, keywords & more Public profiles for Economics researchers Various research rankings in Economics RePEc GenealogyWho was a student of whom, using RePEc Curated articles & papers on economics topics Upload your paper to be listed on RePEc and IDEAS New papers by emailSubscribe to new additions to RePEc EconAcademicsBlog aggregator for economics research Cases of plagiarism in Economics About RePEcInitiative for open bibliographies in Economics News about RePEc Questions about IDEAS and RePEc RePEc volunteers Participating archivesPublishers indexing in RePEc Privacy statementFound an error or omission? Opportunities to help RePEc Get papers listedHave your research listed on RePEc Open a RePEc archiveHave your institution's/publisher's output listed on RePEc Get RePEc dataUse data assembled by RePEc IEEE Account- Change Username/Password
- Update Address
Purchase Details- Payment Options
- Order History
- View Purchased Documents
Profile Information- Communications Preferences
- Profession and Education
- Technical Interests
- US & Canada: +1 800 678 4333
- Worldwide: +1 732 981 0060
- Contact & Support
- About IEEE Xplore
- Accessibility
- Terms of Use
- Nondiscrimination Policy
- Privacy & Opting Out of Cookies
A not-for-profit organization, IEEE is the world's largest technical professional organization dedicated to advancing technology for the benefit of humanity. © Copyright 2024 IEEE - All rights reserved. Use of this web site signifies your agreement to the terms and conditions. To read this content please select one of the options below:Please note you do not have access to teaching notes, industry 4.0 as a data-driven paradigm: a systematic literature review on technologies. Journal of Manufacturing Technology Management ISSN : 1741-038X Article publication date: 21 June 2019 Issue publication date: 24 March 2021 The purpose of this paper is to identify current technologies related to Industry 4.0 and to develop a rationale to enhance the understanding of their functions within a data-driven paradigm. Design/methodology/approachA systematic literature review of 119 papers published in journals included in the Journal Citation Report (JCR) was conducted to identify Industry 4.0 technologies. A descriptive analysis characterizes the corpus, and a content analysis identifies the technologies. The content analysis identified 111 technologies. These technologies perform four functions related to data: data generation and capture, data transmission, data conditioning, storage and processing and data application. The first three groups consist of enabling technologies and the fourth group of value-creating technologies. Results show that Industry 4.0 publications focus on enabling technologies that transmit and process data. Value-creating technologies, which apply data in order to develop new solutions, are still rare in the literature. Research limitations/implicationsThe proposed framework serves as a structure for analysing the focus of publications over time, and enables the classification of new technologies as the paradigm evolves. Practical implicationsBecause the technical side of the new production paradigm is complex and represents an evolving field, managers benefit from a simplified and data-driven approach. The proposed framework suggests that Industry 4.0 should be approached by looking at how data can create value and at what role each technology plays in this task. Originality/valueThe study makes a direct link between Industry 4.0 technologies and the key resource of this revolution, i.e. data. It provides a rationale that not only establishes relationships between technologies and data, but also highlights their roles as enablers or creators of value. Beyond showing the current focus of Industry 4.0 publications, this paper proposes a framework that is useful for tracking the evolution of the paradigm. AcknowledgementsThe authors thank the Research Coordination of the Brazilian Ministry of Education (CAPES), for the financial support received to conduct this research (PDSE Process No. 88881.187062/2018-01). Klingenberg, C.O. , Borges, M.A.V. and Antunes Jr, J.A.V. (2021), "Industry 4.0 as a data-driven paradigm: a systematic literature review on technologies", Journal of Manufacturing Technology Management , Vol. 32 No. 3, pp. 570-592. https://doi.org/10.1108/JMTM-09-2018-0325 Emerald Publishing Limited Copyright © 2019, Emerald Publishing Limited Related articlesAll feedback is valuable. Please share your general feedback Report an issue or find answers to frequently asked questionsContact Customer Support - DOI: 10.1016/j.jclepro.2024.143023
- Corpus ID: 270747046
Industry 4.0 technologies for sustainability within small and medium enterprises: A systematic literature review and future directions- André de Mendonça Santos , Ângelo Márcio Oliveira Sant’Anna
- Published in Journal of Cleaner Production 1 June 2024
- Environmental Science, Business, Engineering
82 ReferencesInvestigating digital technologies’ implementation in circular businesses: evidence from the going circular path, significance of industry 4.0 technologies in major work functions of manufacturing for sustainable development of small and medium‐sized enterprises, is this time different how industry 4.0 affects firms’ labor productivity, industry 4.0 as an enabler of circular economy practices: evidence from european smes, artificial intelligence-driven supply chain resilience in vietnamese manufacturing small- and medium-sized enterprises, unveiling the relationship between sustainable development and industry 4.0: a text mining literature analysis, developing an iot framework for industry 4.0 in malaysian smes: an analysis of current status, practices, and challenges, do we consider sustainability when we measure small and medium enterprises’ (smes’) performance passing through digital transformation, adoption of information and digital technologies for sustainable smart manufacturing systems for industry 4.0 in small, medium, and micro enterprises (smmes), machine learning algorithms applied to intelligent tyre manufacturing, related papers. Showing 1 through 3 of 0 Related Papers Insights from Deploying Industry 4.0 Technologies Toward Sustainable Business Performance: A Study Based on Applied Methodology of SLR- Conference paper
- First Online: 01 July 2024
- Cite this conference paper
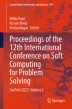 - Pritesh Shukla ORCID: orcid.org/0009-0009-1857-1956 12 ,
- Kumar Rohit ORCID: orcid.org/0000-0003-3349-3799 12 ,
- Avadhesh Dalpati ORCID: orcid.org/0000-0003-0909-4990 12 &
- Ramesh Chandra Gupta ORCID: orcid.org/0000-0002-6545-6362 12
Part of the book series: Lecture Notes in Networks and Systems ((LNNS,volume 995)) Included in the following conference series: - International conference on soft computing for problem-solving
This research focuses on the applicability of Industry 4.0 (I 4.0) technologies exploring their relevance to sustainable business performance. A study was conducted to portray the impact of I 4.0 technologies underlining their effects on business operations. This research aims to determine the linkage between I 4.0 applications and sustainable business performance addressing the barriers to I 4.0 deployment in the context of Indian manufacturing industries. Previous research indicates that sustainable business performance can be categorized into environmental performance, operational performance, and economic performance. On the other hand, Industry 4.0 implementation can be evaluated by finding the crucial enablers and barriers. In this study, a systematic literature review was performed on 68 primary articles published in the year ranging from 2015 to 2022 accumulating crucial insights from diverse publication database repositories. The findings of this article will determine the linkage between “Industry 4.0 deployment” and “sustainable business performance” based on crucial inferences from this study contributing valuable and vital insights into the current research, potential future studies, and key managerial implications in this study domain. This is a preview of subscription content, log in via an institution to check access. Access this chapterSubscribe and save. - Get 10 units per month
- Download Article/Chapter or Ebook
- 1 Unit = 1 Article or 1 Chapter
- Cancel anytime
- Available as PDF
- Read on any device
- Instant download
- Own it forever
- Available as EPUB and PDF
- Compact, lightweight edition
- Dispatched in 3 to 5 business days
- Free shipping worldwide - see info
Tax calculation will be finalised at checkout Purchases are for personal use only Institutional subscriptions Niemeyer JF, Rudolf S, Kvaratskhelia L, Mennenga M, Herrmann C (2022) A creativity-driven case-based reasoning approach for the systematic engineering of sustainable business models. In: 29th CIRP conference on life cycle engineering Google Scholar Beier G, Matthess M, Guan T, Grudzien DI, Xue B, de Lima EP, Chen L (2022) Impact of Industry 4.0 on corporate environmental sustainability: comparing practitioners’ perceptions from China, Brazil and Germany. Sustain Prod Consumption 287–300 Muhmad SN, Muhamad R (2020) Sustainable business practices and financial performance during pre- and post-SDG adoption periods: a systematic review. J Sustain Finance & Invest Stock GST (2016) Opportunities of sustainable manufacturing in Industry 4.0. In: 13th global conference on sustainable manufacturing Lopes de Sousa Jabbour AB, Jabbour CJ, Godinho Filho M, Roubaud D (2018) Industry 4.0 and the circular economy: a proposed research agenda and original roadmap for sustainable operations. Big data analytics in operations & supply chain management Jamwal A, Agrawal R, Sharma M, Kumar V, Kumar S (2021) Developing a sustainability framework for Industry 4.0. In: 28th CIRP conference on life cycle engineering Kumar P, Singh RK, Kumar V (2021) Managing supply chains for sustainable operations in the era of industry 4.0 and circular economy: analysis of barriers. Resour, Conserv Recycling 1–12 Sun X, Yu H, Solvang WD, Wang Y, Wang K (2022) The application of Industry 4.0 technologies in sustainable logistics: a systematic literature review (2012–2020) to explore future research opportunities. Environ Sci Pollut Res 9560–9591 Grant MJ, Booth A (2009) A typology of reviews: an analysis of 14 review types and associated methodologies. Health Inf Libraries J 91–108 Gunasekaran A, Papadopoulos T, Dubey R, Wamba SF, Childe SJ, Hazen B, Akter S (2016) Big data and predictive analytics for supply chain and organizational performance. J Bus Res 1–8 Ghobakhloo M (2020) Industry 4.0, digitization, and opportunities for sustainability. J Clean Prod 1–21 Luthra S, Mangla SK (2018) Evaluating challenges to Industry 4.0 initiatives for supply chain sustainability in emerging economies. Process Safety Environ Protection Bai C, Dallasega P, Orzes G, Sarkis J (2020) Industry 4.0 technologies assessment: A sustainability perspective. Int J Prod Econ Raj A, Dwivedi G, Sharma A, de Sousa Jabbour AB, Rajak S (2019) Barriers to the adoption of industry 4.0 technologies in the manufacturing sector: an inter-country comparative perspective. Int J Prod Econ Dubey R, Gunasekaran A, Childe SJ, Luo Z, Wamba SF, Roubaud D, Foropon C (2018) Examining the role of big data and predictive analytics on collaborative performance. J Clean Prod Haseeb M, Hussain HI, Ślusarczyk B, Jermsittiparsert K (2019) Industry 4.0: a solution towards technology challenges of sustainable business performance. Social Sci Rosa P, Sassanelli C, Urbinati A, Chiaroni D, Terzi S (2020) Assessing relations between circular economy and industry 4.0: a systematic literature review. Int J Prod Res 1–27 Maxim Ruff MW (2020) Industry 4.0 as an enabler of servitization in the plant engineering business: literature review and development of a conceptual research model. In: 3rd international conference on industry 4.0 and smart manufacturing Zulu SL, Zulu E, Chabala M, Chunda N (2022) Drivers and barriers to sustainability practices in the Zambian construction industry. Int J Construct Manage 1–11 Lamé G (2019) Systematic literature reviews: an introduction. In: International conference on, Delft, Netherlands Nightingale A (2009) A guide to systematic. Determining Surgical Efficacy, pp 381–384 Fernando Y, Jabbour CJ, Wah WX (2019) Pursuing green growth in technology firms through the connections between environmental innovation and sustainable business performance: does service capability matter? Resour, Conserv Recycling Cui Y, Liu W, Rani P, Alrasheedi M (2021) Internet of Things (IoT) adoption barriers for the circular economy using Pythagorean fuzzy SWARA-CoCoSo decision-making approach in the manufacturing sector. Technol Forecast Soc Change Weking J, Stocker M, Kowalkiewicz M, Bohm M, Krcmar H (2020) Leveraging industry 4.0—a business model pattern framework. Int J Prod Econ 1–17 Sharma VK, Chandna P, Bhardwaj A (2016) Green supply chain management related performance indicators in agro industry: a review. J Clean Prod 1–31 Tripathi V, Chattopadhyaya S, Mukhopadhyay AK, Sharma S, Singh J, Pimenov DY, Giasin K (2021) An innovative agile model of smart lean–green approach for sustainability enhancement in industry 4.0. J Open Innov Technol 1–20 Narwane VS, Raut RD, Yadav VS, Singh AR (2020) Barriers in sustainable industry 4.0: a case study of the footwear industry. Int J Sustain Eng 1–15 Bag S, Yadav G, Dhamija P, Kataria KK (2021) Key resources for industry 4.0 adoption and its effect on sustainable production and circular economy: an empirical study. J Clean Prod Luthra S, Mangla SK, Yadav G (2019) An analysis of causal relationships among challenges impeding redistributed manufacturing in emerging economies. J Clean Prod 1–36 Jayashree S, Reza MN, Malarvizhi CA, Gunasekaran A, Rauf MA (2022) Testing an adoption model for Industry 4.0 and sustainability: a Malaysian scenario. Sustain Prod Consumption 313–330 Sony M (2020) Pros and cons of implementing Industry 4.0 for the organizations: a review and synthesis of evidence. Prod Manuf Res 244–272 Rajput S, Singh SP (2019) Industry 4.0—challenges to implement circular economy. Benchmarking: Int J Chauhan C, Singh A (2019) A review of Industry 4.0 in supply chain management studies. J Manuf Technol Manage Rajput S, Singh SP (2019) Connecting circular economy and industry 4.0. Int J Inf Manage 98–113 Kumar S, Suhaib M, Asjad M (2020) Narrowing the barriers to industry 4.0 practices through PCA-Fuzzy AHP-K means. J Adv Manage Res Kamble S, Gunasekaran A, Dhone NC (2020) Industry 4.0 and lean manufacturing practices for sustainable organisational performance in Indian manufacturing companies. Int J Prod Res 1–20 Caiado RG, Scavarda LF, Azevedo BD, de Mattos Nascimento DL, Quelhas OL (2022) Challenges and benefits of sustainable industry 4.0 for operations and supply chain management—a framework headed toward the 2030 agenda. Sustainability 1–26 Kumar R, Singh RK, Dwivedi YK (2020) Application of industry 4.0 technologies in SMEs for ethical and sustainable operations: analysis of challenges. J Clean Prod Kaswan MS, Rathi R (2021) Investigation of life cycle assessment barriers for sustainable development in manufacturing using grey relational analysis and best worst method. Int J Sustain Eng 1–15 Raut RD, Mangla SK, Narwane VS, Gardas BB, Priyadarshinee P, Narkhede BE (2019) Linking big data analytics and operational sustainability practices for sustainable business management. J Clean Prod 10–24 Bag S, Pretorius JH (2022) Relationships between industry 4.0, sustainable manufacturing and circular economy: proposal of a research framework. Int J Organizational Anal Soto-Acosta P, Cismaru DM, Vătămănescu EM, Ciochină RS (2016) Sustainable entrepreneurship in SMEs: a business performance perspective. Sustainability Dafflon B, Moalla N, Ouzrout Y (2021) The challenges, approaches, and used techniques of CPS for manufacturing in Industry 4.0: a literature review. The Int J Adv Manuf Technol Nhamo G, Nhemachena C, Nhamo S (2019) Using ICT indicators to measure readiness of countries to implement industry 4.0 and the SDGs. Environ Econ Policy Stud Sony M, Naik S (2020) Critical factors for the successful implementation of industry 4.0: a review and future research direction. Prod Plan Control 1–18 Harikannan N, Vinodh S, Gurumurthy A (2020) Sustainable industry 4.0—an exploratory study for uncovering the drivers for integration. J Model Manage Javaid M, Haleem A, Singh RP, Suman R (2022) Understanding the adoption of Industry 4.0 technologies in improving environmental sustainability. Sustain Oper Comput 203–217 Beltrami M, Orzes G, Sarkis J, Sartor M (2021) Industry 4.0 and sustainability: towards conceptualization and theory. J Clean Prod 1–21 Goni FA, Gholamzadeh Chofreh A, Estaki Orakani Z, Klemeš JJ, Davoudi M, Mardani A (2021) Sustainable business model: a review and framework development. Clean Technol Environ Policy Sharma M, Kamble S, Mani V, Sehrawat R, Belhadi A, Sharma V (2021) Industry 4.0 adoption for sustainability in multi-tier manufacturing supply chain in emerging economies. J Clean Prod Cezarino LO, Liboni LB, Oliveira Stefanelli N, Oliveira BG, Stocco LC (2019) Diving into emerging economies bottleneck: industry 4.0 and implications for circular economy. Diving into emerging economies bottleneck Lacasa E, Santolaya JL, Biedermann A (2016) Obtaining sustainable production from the product design analysis. J Clean Prod 1–22 Ejsmont K, Gladysz B, Kluczek A (2020) Impact of industry 4.0 on sustainability—bibliometric literature review. Sustainability1–29 Contador JC, Satyro WC, Contador JL, Spinola MD (2020) Flexibility in the Brazilian industry 4.0: challenges and opportunities. Global J Flexible Syst Manage Reis JS, Espuny M, Nunhes TV, Sampaio NA, Isaksson R, Campos FC, Oliveira OJ (2021) Striding towards sustainability: a framework to overcome challenges and explore opportunities through industry 4.0. Sustainability1–28 Gupta H, Kumar A, Wasan P (2021) Industry 4.0, cleaner production and circular economy: an integrative framework for evaluating ethical and sustainable business performance of manufacturing organizations. J Clean Prod 1–18 Yadav G, Luthra S, Jakhar SK, Mangla SK, Rai DP (2020) A framework to overcome sustainable supply chain challenges through solution measures of industry 4.0 and circular economy: an automotive case. J Clean Prod 2–15 Rad FF, Oghazi P, Palmié M, Chirumalla K, Pashkevich N, Patel PC, Sattari S (2022) Industry 4.0 and supply chain performance: a systematic literature review of the benefits, challenges, and critical success factors of 11 core technologies. Indus Market Manage 268–293 Nara EO, da Costa MB, Baierle IC, Schaefer JL, Benitez GB, do Santos LM, Benitez LB (2021) Expected impact of industry 4.0 technologies on sustainable development: a study in the context of Brazil’s plastic industry. Sustain Prod Consumption 102–122 Machado E, Scavarda LF, Caiado RG, Thomé AM (2021) Barriers and enablers for the integration of industry 4.0 and sustainability in supply chains of MSMEs. Sustainability1–31 Seth D (2016) An empirical investigation of critical success factors and performance measures for green manufacturing in cement industry. J Manuf Technol Manage Mio C, Costantini A, Panfilo S (2021) Performance measurement tools for sustainable business: a systematic literature review on the sustainability balanced scorecard use. Corporate Soc Responsibility Environ Manage 367–384 Castagnoli R, Büchi G, Coeurderoy R, Cugno M (2021) Evolution of industry 4.0 and international business: a systematic literature. Eur Manage J 1–18 Machado CG, Winroth M, Carlsson D, Almström P, Centerholt V, Hallin M (2019) Industry 4.0 readiness in manufacturing companies: challenges and enablers towards increased digitalization. In: 52nd CIRP conference on manufacturing systems Pasi BN, Mahajan SK, Rane SB (2021) The current sustainability scenario of industry 4.0 enabling technologies in Indian manufacturing industries. Industry 4.0 Enabling Technol 1–32 Kayapinar Kaya S, Aycin E (2021) An integrated interval type 2 fuzzy AHP and COPRAS-G methodologies for supplier selection in the era of industry 4.0. Neural Comput Appl 10515–10535 Khanzode AG, Sarma PR, Mangla SK, Yuan H (2020) Modeling the industry 4.0 adoption for sustainable production in micro, small & medium enterprises. J Clean Prod Ullah H, Wang Z, Mohsin M, Jiang W, Abbas H (2022) Multidimensional perspective of green financial innovation between green intellectual capital on sustainable business: the case of Pakistan. Environ Sci Pollut Res Yadav G, Kumar A, Luthra S, Garza-Reyes JA, Kumar V, Batista L (2020) A framework to achieve sustainability in manufacturing organisations of developing economies using industry 4.0 technologies’ enablers. Comput Industry 1–13 Download references Author informationAuthors and affiliations. Department of Industrial and Production Engineering (IPE), SGSITS, Indore, MP, India Pritesh Shukla, Kumar Rohit, Avadhesh Dalpati & Ramesh Chandra Gupta You can also search for this author in PubMed Google Scholar Corresponding authorCorrespondence to Pritesh Shukla . 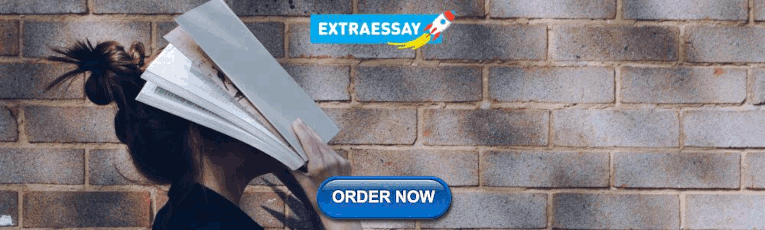 Editor informationEditors and affiliations. Department of Applied Mathematics and Scientific Computing, IIT Roorkee, Roorkee, Uttarakhand, India Millie Pant Department of Mathematics, Indian Institute of Technology Roorkee, Roorkee, India School of Mathematics, Computer Science and Engineering, Liverpool Hope University, LIVERPOOL, UK Atulya Nagar Rights and permissionsReprints and permissions Copyright information© 2024 The Author(s), under exclusive license to Springer Nature Singapore Pte Ltd. About this paperCite this paper. Shukla, P., Rohit, K., Dalpati, A., Gupta, R.C. (2024). Insights from Deploying Industry 4.0 Technologies Toward Sustainable Business Performance: A Study Based on Applied Methodology of SLR. In: Pant, M., Deep, K., Nagar, A. (eds) Proceedings of the 12th International Conference on Soft Computing for Problem Solving. SocProS 2023. Lecture Notes in Networks and Systems, vol 995. Springer, Singapore. https://doi.org/10.1007/978-981-97-3292-0_34 Download citationDOI : https://doi.org/10.1007/978-981-97-3292-0_34 Published : 01 July 2024 Publisher Name : Springer, Singapore Print ISBN : 978-981-97-3291-3 Online ISBN : 978-981-97-3292-0 eBook Packages : Intelligent Technologies and Robotics Intelligent Technologies and Robotics (R0) Share this paperAnyone you share the following link with will be able to read this content: Sorry, a shareable link is not currently available for this article. Provided by the Springer Nature SharedIt content-sharing initiative Policies and ethics - Find a journal
- Track your research
Academia.edu no longer supports Internet Explorer. To browse Academia.edu and the wider internet faster and more securely, please take a few seconds to upgrade your browser . Enter the email address you signed up with and we'll email you a reset link. 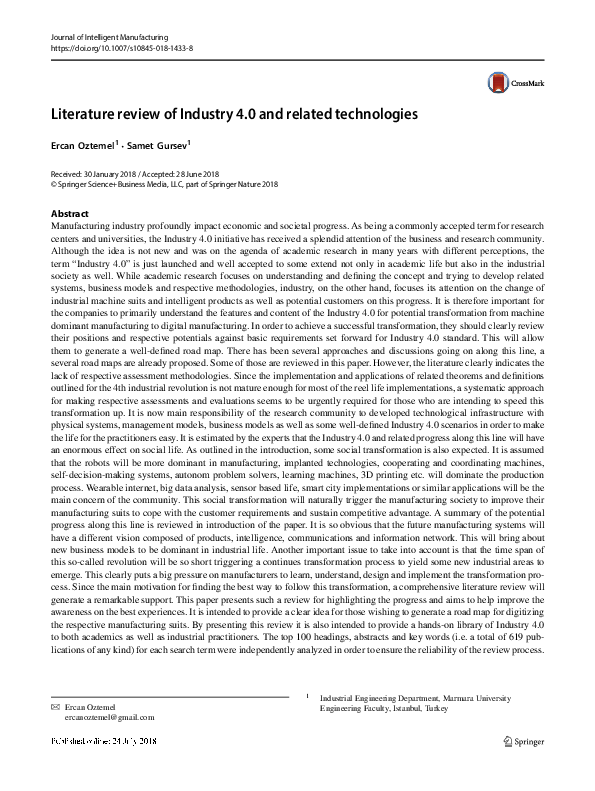 Literature review of Industry 4.0 and related technologies 2018, Journal of Intelligent Manufacturing Related PapersSamrudhi Deshmukh The manufacturing industry is a sector of our society which constantly faces challenges with the variation in demand and supply. Optimization in such a dynamic environment becomes difficult for any industry. Advanced approaches constantly attempt to balance the profit of the supplier as well as contentment level of the customer. Automation in the industry assures that mass production will maintain the supply proportional to the demand. In order to take the automation to the next level, Industry 4.0 allows for a more flexible production environment by introducing the concept of digitalization through a cyber-physical system or CPS. It also becomes an attempt to keep the manufacturing sector updated with the advances in technology of this 21st Cy. This article attempts to list out the concepts of this next generation approach towards a more sustainable model of the manufacturing industry. Moreover, the research will also investigate the advantages and limitations of such a concept in ... 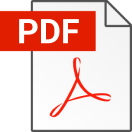 International Journal for Research in Applied Science and Engineering Technology IJRASET IJRASET Publication The objective of this paper is to visualize and to show the direction of Industry 4.0 to develop smart factories in future. The application of new techniques and technologies which are based upon the Internet of things (IOT) ,block chain technology, cloud computing and cyber physical system have paved the path of significant improvement such as increase of automation, quality of production and reduced the time between the development of new products and its launch. Ahmed Elkaseer The arrival of Industry 4.0 has popularised the concept of smart interactions between humans and the physical world that could realise the synergistic integration of intelligent manufacturing assets. However, a systematic and cogent approach to the practical and profitable application of Industry 4.0 is still missing. This paper presents practical approaches to the application of Industry 4.0 to manufacture with the aim of strengthening its competitiveness and meeting the growing serious challenges that threaten its profitability and survival. Precision Additive Metal Manufacturing is utilised in this study for demonstration purposes. A conceptual framework combined with two practical modules available in the market, a "native-design" and "Plug and Play", is proposed. These approaches offer flexible prototypes with sequential procedures that ultimately would allow for easy employment of Industry 4.0, and will help remove technical barriers to the development of m... BEATRICE SANTOS The evolution of information and communication technologies and its introduction into production processes are transforming traditional industry, bringing it to a new level of organizational development. In order to avail the benefits of these technologies to strengthen competitiveness in the global market, a new paradigm is being discussed worldwide. Industry 4.0 or 4th Industrial Revolution are some of the terms used to describe the implementation of "smart" devices that can communicate autonomously along the value chain. In this approach, machines use self-optimization, self-configuration, and artificial intelligence to provide better quality goods and services. In this context, the overall goal of the study is to intensify the discussion and provide an overview of Industry 4.0 to show how combination of advanced technologies and internet can create new opportunities to overcome the current industrial challenges. MOHD SUHAIB Received: 25 January 2019 Abstract Accepted: 14 August 2019 Low cost manufacturing of quality products remains an essential part of present economy and technological advances made it possible. Advances and amalgamation of information technology bring the production systems at newer level. Industry 4.0, factory for future, smart factory, digital manufacturing, and industrial automation are the new buzz words of industry stalwarts and academicians. These new technological revolutions bound to change not only the complete manufacturing scenarios but many other sectors of the society. In this paper an attempt has been made to capture the essence of Industry 4.0 by redefining it in simple words, further its complex, disruptive nature and inevitability along with technologies backing it has been discussed. Its enabling role in manufacturing philosophies like Lean Manufacturing, and Flexible Manufacturing are also reported. At last the challenges its adoption and future research areas are ... The Journal of Engineering and Exact Sciences LIZANDRA VERGARA The concept of Industry 4.0 emerged in Germany as a strategy for innovation and recovery of economic performance and has spread worldwide. The digital transformation proposed by Industry 4.0 is driven by intelligent manufacturing processes, digitalization, flexibility, integration of systems, and real-time analysis of big data generating intelligent processes and services oriented to customer needs. However, despite the opportunities produced by technological innovation, the fourth industrial revolution has established an environment of uncertainty in the labor market and business models reflected throughout the social sphere. Thus, this study aimed to identify the potential impacts and challenges of Industry 4.0 through a systematic literature review (SLR). Our findings pointed to seven potential impacts of Industry 4.0 on manufacturing: (i) environmental, (ii) competitive, (iii) economic, (iv) education, (v) labor market, (vi) business models, and (vii) social. Additionally, six p... Renewable and Nonrenewable Energy M.vijaya Lakshmi , Dr. Ramachandran Manickam , Sathiyaraj Chinnasamy , A PAVITHRA Industry 4.0 Wireless Supported by connectivity and sensors Factories has machines. These sensors Display the entire production line and monitor and can make its own decisions are integrated into one system. Industry 4.0 Smart production Uses processes to produce essential waste products to meet the COVID-19 epidemic shortage. Patients need themselves Essential medical supplies to get on time, during this crisis Medical disposable items and Smart supply chain of equipment it provides Industry is 4.0 as a flexible product line Smart system used. Intelligence (I) by RTC, real-time information on Internet of Things (Yoda) and almost all other digital technologies for the entire production process. Any medical area Design and upgrade pre-designed Using software is done quickly and to print the required components Digital production such as 3D printing Uses technologies. Industrial 4.0 Technologies COVID-19Diagnose symptoms of, It is related to this disease Helps to avoid the chances of getting sick Can predict. Possible health problems and expected recovery Helps keep track of opportunities. Govt for 19 explosions Assistive Industry 4.0 Notable Technologies. Cross New Business Opportunities Is open. However, flexibility, Performance and product customization the requirements of Real to handle value creation Procedures no longer apply. Industrial 4.0, (Fourth Industrial Revolution) is a term coined in Germany; more important ideas were first published in 2011.It is the internet of future technologies Physical systems (CPS), Integration in the Internet and production systems as defined. Control computers in real time, Plan and predict Helps. In the field of technology, in the production of this concept causing revolution and related services have been discussed in research for many years. One of the basic principles for creating an industrial 4.0 environment is to connect organizations and Working value chain individually and Control each other arbitrarily. Research Expression is an interdisciplinary biannual and bilingual research journal published by Government VYT Post Graduate Autonomous College Dr. Ashok D . Gaur Technology has changed the face of the industrial and manufacturing world. This is the age of the fourth industrial revolution, commonly known as Industry 4.0. It means the introduction of automation and data exchange in manufacturing technologies like Cyber-Physical Systems, Internet of Things, big data and analytics, augmented reality, additive manufacturing, simulation, horizontal and vertical system integration, autonomous robots as well as cloud computing. It links between man and machine by integrating and combining artificial intelligence in machines. The main aims of Industry 4.0 to initiate transformation in industrial manufacturing through digitalization and exploitation of the potentials of new technologies. In essence, Industry 4.0 is related to the creation of creates "smart factory". Amazon, Uber, Facebook, "smart factories" and 3D printing, are among modern pioneers of the new industrial revolution. But with a great opportunity, comes great challenges. The aim of this paper is to present and facilitate an understanding of Industry 4.0 concepts, its drivers, enablers, goals, and limitations. Sarah El Hamdi The industrial sector has historically been linked to the prosperity, development and evolution of nations, hence to the genesis of the philosophy of competitiveness, mainly at the manufacturing level. An environment is in perpetual adaptation to trends, and the best example is the change of industrial ideology, as was the case in the past. The global society is facing the same challenges. Customer demands are becoming more and more specific and personalized, which means that factories have to innovate in terms of production and management of mudas in an eco-environmental context closely followed by several global organizations. Information and communication technologies have become the joker to master in order to stay in a market that is global and very competitive. At the heart of this evolution is the emergence of the Industry 4.0 concept, which includes a key element that is intelligent production in factories of the future that can meet different obstacles. The purpose of the c... Industry 4.0 - Current Status and Future Trends Ercan Oztemel Loading Preview Sorry, preview is currently unavailable. You can download the paper by clicking the button above. RELATED PAPERSIOP Conference Series: Earth and Environmental Science Shahryar Sorooshian Belmont Press Dr.adel aneiba Annual Reviews in Control Hervé Panetto International Journal of Online Engineering (iJOE) juan david diaz IntechOpen Limited - London Meisam Gordan International Journal of Scientific Research in Science and Technology International Journal of Scientific Research in Science and Technology IJSRST Mobility and Vehicle Mechanics edina karabegovic Dimitrios Koulouriotis THE IJES Editor Zenodo (CERN European Organization for Nuclear Research) RACH MERVEILLE Journal of Intelligent Manufacturing Ahmad Barari IFAC-PapersOnLine Václav Kaczmarczyk IJAERS Journal IRJET Journal , Dr. Surabhi shanker IRJET Journal Technical, Economic and Societal Effects of Manufacturing 4.0 karl-erik michelsen GIS SCIENCE JOURNAL shimol philip Aparna Unnikrishnan RELATED TOPICS- We're Hiring!
- Help Center
- Find new research papers in:
- Health Sciences
- Earth Sciences
- Cognitive Science
- Mathematics
- Computer Science
- Academia ©2024
Login to your accountChange password, your password must have 8 characters or more and contain 3 of the following:. - a lower case character,
- an upper case character,
- a special character
Password Changed SuccessfullyYour password has been changed Create a new accountCan't sign in? Forgot your password? Enter your email address below and we will send you the reset instructions If the address matches an existing account you will receive an email with instructions to reset your password Request UsernameCan't sign in? Forgot your username? Enter your email address below and we will send you your username If the address matches an existing account you will receive an email with instructions to retrieve your username 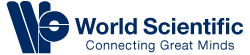 - This Journal
-
- Institutional Access
Cookies NotificationOur site uses javascript to enchance its usability. you can disable your ad blocker or whitelist our website www.worldscientific.com to view the full content., select your blocker:, adblock plus instructions. - Click the AdBlock Plus icon in the extension bar
- Click the blue power button
- Click refresh
Adblock Instructions- Click the AdBlock icon
- Click "Don't run on pages on this site"
uBlock Origin Instructions- Click on the uBlock Origin icon in the extension bar
- Click on the big, blue power button
- Refresh the web page
uBlock Instructions- Click on the uBlock icon in the extension bar
Adguard Instructions- Click on the Adguard icon in the extension bar
- Click on the toggle next to the "Protection on this website" text
Brave Instructions- Click on the orange lion icon to the right of the address bar
- Click the toggle on the top right, shifting from "Up" to "Down
Adremover Instructions- Click on the AdRemover icon in the extension bar
- Click the "Don’t run on pages on this domain" button
- Click "Exclude"
Adblock Genesis Instructions- Click on the Adblock Genesis icon in the extension bar
- Click on the button that says "Whitelist Website"
Super Adblocker Instructions- Click on the Super Adblocker icon in the extension bar
- Click on the "Don’t run on pages on this domain" button
- Click the "Exclude" button on the pop-up
Ultrablock Instructions- Click on the UltraBlock icon in the extension bar
- Click on the "Disable UltraBlock for ‘domain name here’" button
Ad Aware Instructions- Click on the AdAware icon in the extension bar
- Click on the large orange power button
Ghostery Instructions- Click on the Ghostery icon in the extension bar
- Click on the "Trust Site" button
Firefox Tracking Protection Instructions- Click on the shield icon on the left side of the address bar
- Click on the toggle that says "Enhanced Tracking protection is ON for this site"
Duck Duck Go Instructions- Click on the DuckDuckGo icon in the extension bar
- Click on the toggle next to the words "Site Privacy Protection"
Privacy Badger Instructions- Click on the Privacy Badger icon in the extension bar
- Click on the button that says "Disable Privacy Badger for this site"
Disconnect Instructions- Click on the Disconnect icon in the extension bar
- Click the button that says "Whitelist Site"
Opera Instructions- Click on the blue shield icon on the right side of the address bar
- Click the toggle next to "Ads are blocked on this site"
 System Upgrade on Tue, May 28th, 2024 at 2am (EDT)Artificial intelligence applications for industry 4.0: a literature-based study. - Mohd Javaid ,
- Abid Haleem ,
- Ravi Pratap Singh , and
- Rajiv Suman
https://orcid.org/0000-0001-8871-2886 Department of Mechanical Engineering, Jamia Millia Islamia, New Delhi, India E-mail Address: [email protected] Corresponding author. Search for more papers by this author https://orcid.org/0000-0002-3487-0229 Department of Industrial and Production Engineering, Dr B R Ambedkar National Institute of Technology, Jalandhar, Punjab, India Department of Industrial and Production Engineering, G. B. Pant University of Agriculture and Technology, Pantnagar, Uttarakhand, India Artificial intelligence (AI) contributes to the recent developments in Industry 4.0. Industries are focusing on improving product consistency, productivity and reducing operating costs, and they want to achieve this with the collaborative partnership between robotics and people. In smart industries, hyperconnected manufacturing processes depend on different machines that interact using AI automation systems by capturing and interpreting all data types. Smart platforms of automation can play a decisive role in transforming modern production. AI provides appropriate information to take decision-making and alert people of possible malfunctions. Industries will use AI to process data transmitted from the Internet of things (IoT) devices and connected machines based on their desire to integrate them into their equipment. It provides companies with the ability to track their entire end-to-end activities and processes fully. This literature review-based paper aims to brief the vital role of AI in successfully implementing Industry 4.0. Accordingly, the research objectives are crafted to facilitate researchers, practitioners, students and industry professionals in this paper. First, it discusses the significant technological features and traits of AI, critical for Industry 4.0. Second, this paper identifies the significant advancements and various challenges enabling the implementation of AI for Industry 4.0. Finally, the paper identifies and discusses significant applications of AI for Industry 4.0. With an extensive review-based exploration, we see that the advantages of AI are widespread and the need for stakeholders in understanding the kind of automation platform they require in the new manufacturing order. Furthermore, this technology seeks correlations to avoid errors and eventually to anticipate them. Thus, AI technology is gradually accomplishing various goals of Industry 4.0. - Artificial intelligence (AI)
- Industry 4.0
- applications
- advancements
1. IntroductionThe applications of artificial intelligence (AI) technologies enhance the capabilities significantly in the manufacturing sector as it works across various business lines and levels, from staff planning to product design, maximizing performance, product quality and employee well-being. Advances in AI are central to various advancements, allowing robots to manage more computational tasks and make independent decisions based on environmental data in real time for Industry 4.0. It includes managing different criteria such as content types, manufacturing methods, budget limits and time constraints. The ideas and other essential tasks can be managed and evaluated using machine learning (ML), providing further insight into the latest designs. AI is used in factories to allow the predictive management of sensitive industrial machineries to predict asset failure in Industry 4.0. The administration is helped to timely rehabilitate the facilities to avoid expensive unplanned downtime ( Yao et al. , 2017 ; Lee et al. , 2018 ; Bécue et al. , 2021 ). AI algorithms help businesses to predict shifts in the markets to maximize production supply chains. This offers management an enormous benefit from a reactionary to a competitor. AI algorithms estimate market demands by searching for position trends, socio-economic and macroeconomic variables, environmental patterns, status policy, customer behavior and more. This development would allow manufacturers to reduce production downtime and optimize their manufacturing lines’ overall operating effectiveness. In addition, AI and computer training increase quality management and standardization by producing a predictive analysis of the equipment’s features and streamlining production lines ultimately. With AI implementation, industries can now take fast, data-driven decisions, simplify production processes, minimize operating costs and enhance customer service ( Dal Mas et al. , 2019 ; Haenlein et al. , 2019 ; Gupta et al. , 2021 ). With an AI-compatible smart plant, manufacturing will work unprecedentedly, reduce costs and improve customer service. Industries can avoid downtimes by forecasting delays, control inventory by tracking stocks, anticipate the delivery speed and provide the highest quality goods. In order to monitor the production process and detect mistakes such as the microscopic crack in production facilities, computing vision may be used. AI may alert companies to production line problems that may lead to quality problems. The serious ones can be avoided in the early stages of the overall development level of Industry 4.0 ( Javaid and Haleem , 2019 ; Ibrahim and Hassan , 2019 ; Sanchez et al. , 2020 ). Advanced AI algorithms in deep learning and artificial neural networks are used for repair prediction to formulate asset failure predictions. Quality requires AI algorithms to report evolving production defects to manufacture teams that can trigger product quality problems. This can analyze slight machine behavior abnormalities, changes in raw materials, etc. In order to ensure that a maximum algorithm produces values within the given interval, the product designer often establishes minimum and maximum limits. The results given are solutions that can be evaluated further with the help of ML to obtain insights into which architecture satisfies standards. AI algorithms are used for quality management to alert production units of possible production faults, leading to problems with product quality ( Cheng et al. , 2016 ; Koh et al. , 2019 ; Bousdekis et al. , 2020 ). Manufacturing industries use this technology to produce a virtual representation that replicates factory, product or physical characteristics. By using cameras, sensors and other data collection techniques, this reflects real-time knowledge. Combining interactive and physical environments makes it possible to track plants, analyze data and solve issues proactively. The flaw detecting method in production lines becomes smarter in manufacturing. A computerized device can detect various surface defects such as scratches, cracks and leaks and others with deep neural network integrations. Data scientists teach visual inspection systems to identify defects according to their mission by applying image recognition, object identification and instance segmentation algorithms ( Haleem et al. , 2020 ; Massaro et al. , 2020 ). ML modeling will forecast energy demand in the future by handling historical data on consumed energy. The popular ML method is focused on sequential data measurements to forecast energy consumption. AI can permit systems to monitor themselves to reduce downtimes, maximize resource uses and anticipate failures ( Mazurek and Małagocka , 2019 ; Chen et al. , 2020 ). It can aid decision-makers in testing environments, increase the efficiency of assets and prevent system failures. It will help organizations appreciate their products by visualizing the performance of their products in their factory environment and in real time by the workforce. The information obtained from the simulated reality will be used to turn the product concept into potential goods in the real universe ( Bortolini et al. , 2017 ; Milward et al. , 2019 ). 2. Research MethodThis is review-based research reporting from different research papers, blogs and other research platforms by searching the keyword as “artificial intelligence”, “Industry 4.0”. This paper addresses the following research objectives: • | To brief about AI for Industry 4.0 and discuss technological features and traits of AI for Industry 4.0; | • | To study significant advancements of AI and discuss various challenges in implementing the AI concept for Industry 4.0; | • | To study diversified sets/subsets of AI for Industry 4.0; | • | To identify significant applications of AI for Industry 4.0. |
3. Artificial Intelligence in Industry 4.0In Industry 4.0, AI integrates numerous technologies that enable software and machines to sense, comprehend, act and learn human operations. The industrial production system can be more efficient using this technology. The manufacturing sector is constantly growing because this technology advances with Industry 4.0. AI is one of the developing technologies used to increase efficiency, product quality and reduce operational costs. The smart factory comprised of hyperconnected production processes comprises multiple machines that all communicate with one another. Manufacturers undergo a digital transformation that manages and uses their data sets by leveraging AI and ML for better quality control, standardization and maintenance. The benefits of AI in manufacturing processes are numerous in day-to-day services in Industry 4.0 ( Ibrahim and Hassan , 2019 ; Chi-Hsien and Nagasawa , 2019 ; Zhang and Lu , 2021 ). It is utilized to speed up our job by producing more accurate results with less human effort. It makes use of digital technology, which makes Industry 4.0 smarter and more productive. AI advancements give rise to computing systems that can see, hear, learn and open innovative platforms to improve skills. 3.1. Artificial intelligenceAI refers to human-like intelligence demonstrated by machines like natural intelligence, which helps solve problems of varied nature. AI has a significant effect on the production areas that can perform various tasks just like human intelligence. The use of AI technology in the production supply chain will predict product demand’s time, geographical and socio-economic dynamics in various algorithms, accounting for macroeconomic cycles and weather patterns ( Tung , 2019 ; Xu , 2021 ). The predictive management of equipment with sensors to track working conditions and tooling efficiency is also highly advantageous to AI. This technology can address many of the industry’s internal problems, from skill shortages to decision-making complexities, deployment difficulties and overflowing knowledge. Using AI in production plants allows companies to transform their procedures entirely. The use of AI and robotics in industrial production is mainly observed since mass production is revolutionized. Robots will carry out recurring tasks, design the development model, increase competence, develop strategies for building automation, eradicate human error and provide superior quality assurance ( Nascimento and Bellini , 2018 ; Merayo et al. , 2019 ; Ammar et al. , 2021 ). AI gives companies a sophisticated level of analysis that they can use to analyze their individual components’ results. The AI database analysis can increase a facility’s total performance and improve the output quality. It allows robots or other equipment intelligent enough to sense abnormalities and track parameters. It detects, summarizes and analyses the massive data flow, then passes it to other computers to a cloud-based network. It helps to manage a large-scale flood and enables an internet of things (IoT)-scale ecosystem to be leveled. AI helps program creators and broadcasters to detect which shows they can suggest to specific consumers based on their behavior by entering the entertainment industry. ML algorithms are used for user behavior, and such algorithms become smarter with time to determine user requirements also ( Badri et al. , 2018 ; Cioffi et al. , 2020 ; Radanliev et al. , 2021a ). 3.2. Industry 4.0The word Industry 4.0 applies to the implementation of advance information and manufacturing technologies in industries. This is the term often used for the digital revolution in the industry. It is a global word that refers to AI Cyber, IoT, cloud, ML, etc. These can be intelligently interpreted and developed in the manufacturing processes. It can quickly assess the data gathered during the manufacturing process. New processes are obtained via this assessment and can constantly adjust output changes. Various processes are also not only better linked in this industrial revolution but also streamlined. Industry 4.0 is the pavement for digitization in the industrial industry, which changes how we communicate with and revolutionizes AI and ML applications ( Hofmann et al. , 2019 ; Cioffi et al. , 2020 ). One of the key objectives of Industry 4.0 is to operate the computers in a decentralized and autonomous manner in cases of exceptions, interferences or overlapping objectives requiring external feedback. The application of AI has led to positive changes in their intelligent factories that reduce maintenance costs. Furthermore, advances in industrial cybersecurity technologies often allow corporate network surveillance to tackle hacker attacks in good time. Industry 4.0 provides the latest development in industrial technology automation and data sharing. AI can be easily determining their future manufacturing with the effective storage of data. The more the machines data sets are fed, the more patterns are evolved, learned and decided with the interest of the production company. This automation helps correctly forecast errors, predict working loads, track problems and expect them ( Haleem and Javaid , 2019 ; Dudukalov et al. , 2021 ). 3.3. Need of artificial intelligence for industry 4.0Industry 4.0 needs to prepare for networked factories that are highly embedded in the supply chain, design team, production line and quality control into a smart engine that provides practical insights with the help of AI. To exploit Industry 4.0’s many opportunities, manufacturers need to develop a system that considers the whole production process as it needs cooperation across the whole supply chain cycle. Today, the main fields of AI, ML and IoT adoption are asset control, supply chain management and resource management. Combining these new tools, asset tracking precision, the visibility of the supply chain and stock utilization can be improved. Predictive maintenance can be improved using ML strategies like algorithms, processes powered by machine intelligence and quality optimization ( Shi et al. , 1995 ; Kunst et al. , 2019 ; Javaid and Haleem , 2020 ). Effective time monitoring of operating loads at the factory floor contributing to production planning efficiency can be quickly undertaken using AI. By combining ML with overall equipment effectiveness, producers can increase production, preventive maintenance and asset workloads. 4. Technological Features and Traits of Artificial Intelligence for Industry 4.0Figure 1 reflects the various technological enablers of AI, which fulfills the requirements while implementing this philosophy in Industry 4.0 culture. There are four different traits: operations technology, data technology, analytics technology and platform technology ( Chen and Li , 2019 ; van Geest et al. , 2021 ). These enablers support the AI practice to make the Industry 4.0 sphere more accurate, precise, quick, optimized and secure to make Industry 4.0 more effective and realistic. 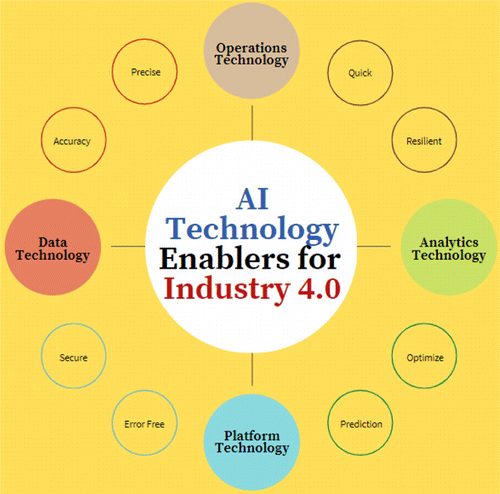 Fig. 1. Various tools and traits of AI for Industry 4.0. AI has a binding effect in manufacturing on the intelligent maintenance of the development system. This provides predictive solutions to prevent sudden harm to equipment. AI-enabled solutions for manufacturers will prevent equipment failure before it gets affected. The fact that production data is fantastic for AI drives this popularity. The production is full of empirical data that is easier to interpret for robots. Hundreds of variables affect the output process while they are challenging to analyze in human environments. The effect of individual variables in complex situations can be predicted effectively by AI models. Machines can operate under human capacity in other sectors that include language or feelings. AI enhances the quality management of industrial systems. AI-based systems spot component flaws on the manufacturing line ( Chen and Xu , 2001 ; Tang et al. , 2001 ; Romeo et al. , 2020 ; Bogoviz , 2020 ). There is unbelievable use of AI in Industry 4.0. Industrial AI robotic cooperation allows producers to supply generative materials more quickly. AI is transforming how designers design goods in the industrial sector. The AI production solutions have guidance into the suitable models. A digital twin is used to track and assess the manufacturing process and determine whether quality problems can arise or whether the product output is less than expected. Digital twins allow producers to have a clear picture of the used products and allow the refilling process to be automated. Manufacturers use AI technologies for the analysis of sensor data to detect possible downtimes. Manufacturers assist AI systems in predicting whether or when functions will malfunction so that servicing and repair can be planned for the failure ( Skobelev and Borovik , 2017 ; Sharma et al. , 2021 ). The automotive sector continues to embrace AI services to modernize its activities. It has special applications that can turn a modern production company entirely. AI can predict the competition and reliably evaluate the potential benefit of goods when they are in demand. AI software programming can allow producers to reduce electricity prices and adverse market fluctuations by enhancing demand forecasting while operating. The integration of AI algorithms in procurement, industrial sourcing and cost control. It is already underway in business and consumer goods, technical facilities and aircraft applications for various customers to enhance product requirement projections in their workstream. This technology derives its strength from the data collected from instruments or sensors installed in manufacturing machinery ( Preuveneers and Ilie-Zudor , 2017 ; Jimeno-Morenilla et al. , 2021 ). Generative programming uses the algorithms of ML to represent the approach of an engineer to design. Designers enter design parameters into the design program, and the software produces all possible results that these parameters can provide. This technology easily allows designers to create thousands of design alternatives for a component. Businesses need to adjust to the unstable raw material price to continue to compete in the market. The preservation of the optimal standard of consistency in a process or good is quality assurance. It enhances the assembly line’s capability to operate based on parameters and algorithms that provide the best final goods. AI systems can distinguish deviations from the standard performance with machine viewing technology because most defects are noticeable. If the output of an end product is less than intended, AI systems will cause a warning for users to respond ( Xu , 1999 ; Feng and Xu , 1999 ; Paolanti et al. , 2018 ; Kebisek et al. , 2020 ). This technology stepped up attempts to adopt digital transformation to meet changing customer needs. In Industry 4.0, analytics and the IoT will be instrumental in defining trends, behaviors and providing in-house evidence on the manufacturer. Manufacturers expect to optimize intelligent resources based on the gathered data from different intelligent workflows and processes of the plant. The ultimate aim in the automotive sector is a prompt and reliable distribution to a customer. However, it is impossible to develop a reliable distribution chain with several facilities in various areas. Using a process mining tool, manufacturers can compare each area’s output by each step, including time, cost and the person taking the step. These findings help streamline operations and determine the location of bottlenecks so that companies can respond ( Samarasinghe and Medis , 2020 ; Tiwari and Khan , 2020 ; Borowski , 2021 ). Artificial neural networks are ideal for the variable and continuously evolving production processes to process several parameters across many layers. It needs adequate training to demonstrate high precision in producing predictions of the mechanical properties of processed goods, which reduces the costs of raw materials. The trend of business, propelled by the modern mode of contact between human beings and the computer, has changed AI. Smart plants consume automated facilities and have digitally-enabled devices that allow machinery to communicate via IoT configuration between them and the factory systems. Industries are increasingly demanding these skills to ensure the productivity of the manufacturing plants ( Tarassov , 2018 ; Ng , 2020 ). 5. Significant Advancements in Industry 4.0 Through Artificial IntelligenceThe successful launch of autonomous vehicles and robots shows the integration of AI and ML. The use of sensors in combination with ML helps the output of each development phase be continually evaluated. The adapting of supply to demand is one of the common problems in the industry. The integration of ML helps to fulfill energy requirements optimally. AI technologies are also used to improve user service. For example, many chatbots on e-commerce sites came with AI-driven and configured so that several typical consumer questions can be answered instantaneously. The agricultural sector saw an increase in the use of sophisticated tractors and smart plucking machines. Fraud detection in the financial sector is another significant application of AI ( Chun et al. , 2018 ; Haleem et al. , 2019 ; Leng et al. , 2021 ). Nowadays, robots are an integral part of the manufacturing company’s machinery. These robots have now made intelligent decisions and work together to maximize productivity in production facilities in conjunction with AI technology. AI can be quickly operating self-driving or semiautonomous cars. It becomes part of network-linked and run-on roads depending on various circumstances. The AI systems of vehicles can forecast the driver’s actions, classify the passengers, assess conditions on the way and traffic congestions to track vehicle driving. Self-driving cars are sure to be the next major thing in the automotive business. While AI self-driving is still in the research and test stage in many countries, it can replace manual driving and move safer on roads. The use of AI in the immobilizing sector provides agents, brokers and customers all with new resources. AI-powered companies help dealers and agents to find the right solution for those looking for their assets to purchase and sell ( Kempegowda and Chaczko , 2018 ; Ruiz-Sarmiento et al. , 2020 ; Kliestik et al. , 2020 ). Industrial firms invest in automated AI vehicles to automate logistic processes to help control the delivery centers. Self-driving cars thus eliminate dependence on human drivers. The demand for products can also be effectively predicted by AI systems using predictive analytics. AI manufacturing applications gather data from different sources. Later on, it can forecast product demand correctly based on evidence. The AI app can handle order records and uninstall/install new stocks. It is one of the finest technologies for production management, market management and inventory management. Through analyzing historical product–price data, algorithms for ML will predict the price of a product. It can use neural networks and in-depth modeling to recognize images and supervise predictive model learning ( Dhanabalan and Sathish , 2018 ; Helmold , 2019 ). AI helps the robot to accurately capture tiny air bubbles and assess the position of the gas leakage. It quickly locates trouble areas and production lines and significantly reduces labor cost and detection errors, along with data extracted from the entire production chain. Sensors are built into each hardware piece in Industry 4.0 environment to communicate from machine to machine. Moreover, ML provided by data-physical systems and cloud computing makes linking humans, machinery and resources seamless. As a result, all aspects, including vehicles, manufacturing lines, factories and facilities, in the production process can be connected closely. 6. Various Challenges in Implementing Artificial Intelligence for Industry 4.0Factors related to data quality, machine-to-machine variations, cybersecurity and operational regimes are major identified barriers to effectively realizing the AI practice for enhancements in Industry 4.0. For example, Fig. 2 shows various associated challenges in implementing AI methodologies in Industry 4.0 sphere. It ultimately correlated with the perfection, reliability, error freeness and completeness of the data; variableness, controlling, capability and automation of machine-related variations; diagnosis, system analysis and assurance factors are associated with operational schemes ( Tang and Veelenturf , 2019 ; Ribeiro et al. , 2021 ). 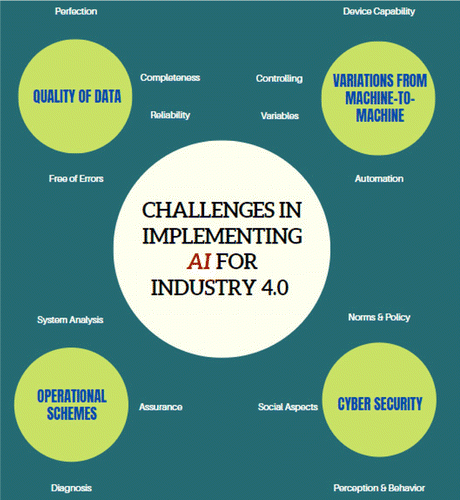 Fig. 2. Associated challenges in realizing AI for industry 4.0. AI is being used to classify and accurately diagnose and handle a wide variety of health care facilities, including data mining to identify trends, medical imaging, pharmacy management, pharmaceutical detection and robotic procedure. Retail companies are constantly looking to find trends for customer behavior as a competition space and thereby match their approach with their competitor’s intelligence. AI tools will monitor client behavior so that trends can be identified and potential results are predicted. Companies can better respond to their demands by observing the actions of current consumers. AI is the best tool for every company that changes the game. It is becoming more available to businesses as the platform matures and costs decrease. It can be helpful in production to make things safer and cheaper. The industrial sector has already looked forward and effectively adopted emerging technology ( Frank et al. , 2019 ; Benotsmane et al. , 2019 ; Singer and Cohen , 2021 ). AI can easily carry out manufacturing, quality management, shorter design time and waste reduction, improved reuse of production and predictive maintenance. Predictive maintenance enables businesses to determine whether machinery needs high precision maintenance rather than preventive maintenance. Predictive maintenance avoids the use of ML unplanned downtime. In the production equipment, technologies like sensors and advanced analytics allow for predictive maintenance by addressing system problems and managing warnings. AI experts concentrated for some time on the identification of patterns and computer pedagogy. ML is central to the identification of images, faces and words. Audio–vision processing, digital translation or transcription and driver-free vehicles are the other noteworthy applications ( Uslu and Fırat , 2019 ; Gomes et al. , 2020 ). AI may ingest a mixture of the sensor, computer and human data, refine operations or achieve production lights. The intelligent production plant consists of hyperconnections comprised of interconnected computers, which use automation platforms for AIs to capture and analyze all types of information, including images and standardized tests. Manufacturing deploys AI to incorporate automation frameworks for managing various tasks. It also encourages workers to simultaneously use the same computer to execute other tasks, thereby reducing human contact. Using AI, a manufacturer may warn workers of problems with a project in a short space of time. No longer any digital revolution is overlooked, and AI programming has a major and vital role in the industry’s future. Manufacturers will adopt and implement manufacturing-revolutionizing AI technologies ( Terziyan et al. , 2018 ; Rizvi et al. , 2021 ). The standard manufacturers can now start to adopt ML models for cost savings aggressively. While most manufacturing processes have been researched for decades, recent development has opened up a new frontier for further optimization in AI, particularly ML. It allows one to create a paradigm that takes data from various sources into account. AI means that fewer human resources are harmful and minimize the number of mistakes in the production plant. The number of injuries at the workplace will decline as robots overtake human beings and conduct regular and dangerous tasks. When AI takes over the production facility and automates repetitive and ordinary human jobs, employees concentrate on creative and complicated activities. People should concentrate on pushing creativity and driving business to advanced stages using AI ( Yan et al. , 2017 ; Pereira et al. , 2020 ). In recent years, AI and factory automation have progressed significantly. The development of deep learning algorithms and developments in sensor technology have led to a new generation of developing computer resources. AI helps machines collect and remove data, recognize models, learn and adapt through master intelligence and recognize learning and language. In addition, AI is excellent at interpreting and decoding natural language. This would make communication with the app easier for employees and managers. Inventories costs can be reduced using AI, which creates robust advancement for the manufacturing industry. The productive industry needs to be equipped for integrated manufacturing plants, which are very structured in the product line and quality management ( Lafferty , 2019 ; Lăzăroiu et al. , 2021 ). For example, a factory worker should procure raw material reserves from the shelf and immediately establish the inventory transaction using a monitoring camera. 7. Diversified Sets/Subsets of AI for Industry 4.0Figure 3 exemplifies distinct sets and subsets related to practicing AI methodologies for improving and enhancing the Industry 4.0 environment. In this perspective, ML, cognitive computing, the IoT, factory-based industrial automation, robotics and big data-related aspects are needed to be taken care of while realizing Industry 4.0 through the support of AI ( Wan et al. , 2018 ; Pires et al. , 2019 ). 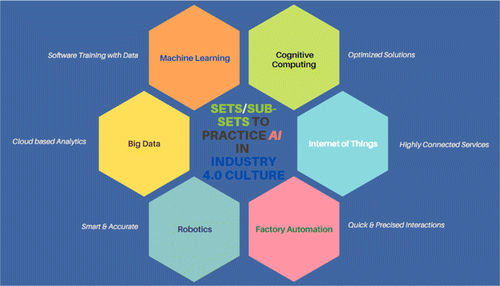 Fig. 3. Different sets/subsets of AI for industry 4.0. AI enhances Industry 4.0 to improve their demand forecasts and ensure that supply increases and decreases as needed. In the production industry, AI focuses primarily on reducing downtime and ensuring the continued effectiveness of production lines. When replacement parts must be ordered, AI may be used to forecast. This skill eliminates plant machinery downtimes and prevents costly components from being stocked. It can be done by adding vast quantities of data and modeling that indicates a possible component malfunction. ML models continuously interpret data unique to the company and production, making it more precise and preventing errors and anomalies. The whole supply chain can be controlled by AI and foresee any changes in demand that can influence production processes ( Bai et al. , 2020 ; Popkova and Sergi , 2020 ; Lee and Lim , 2021 ). Digital twin AI technology gives engineers the capability to view and test components, manufacturing lines more remotely. With today’s cloud-enabled power rental capability, small and large enterprises may use AI technologies to identify bottlenecks, weaknesses and errors and improve functionality that speeds up their time on the market. Engineers can see how everything interacts with many data and map connections between materials, devices and systems. AI can autonomously upgrade the specification based on real-world information. This technology adoption will contribute to mass personalization and significantly enhance versatility. Manufacturing may have highly ineffective procedures, which are usually refined and modified continuously ( Angelopoulos et al. , 2020 ; Hansen and Bøgh , 2021 ). AI can produce data that allow making sound, factual and data-oriented business decisions. This also helps to remove individual prejudices from the calculation, and in many ways, to provide a more detailed overview. Data from various sources can be collected through an AI platform and ML technologies. AI allows producers to remain competitive, cut prices, optimize resources and provide their workers with a better workplace and customer experience. Large production companies started using AI to make purchases of materials and allocation decisions. AI would also improve manufacturers’ standards for launch dates and capacity-based volumes along with unplanned downtime. This technology will support businesses to determine what to do with their replacement potential, such as early production of seasonal goods sold at low cost to retail stores in the year ( Candanedo et al. , 2018 ; Azizi , 2020 ; Mhlanga , 2020 ). In most businesses using standard data, early progress will generally come when supervised AI demands good data and proper preparation. In other words, as solutions start to appear, businesses follow AI. Industries of easily accessible high-quality data then provide AI applications ready for use as quickly as possible. Better vision software will facilitate quality on a mass scale with fewer workers. It is likely to affect task analyzing data and propose market optimization activities, from architecture to process and service. The architecture of the product is often severely interfered by AI. This provides generative design methods that identify the problem, compute the whole problem area and amplify cognitive skills ( Pilati and Regattieri , 2018 ; Lu , 2019 ). AI can also improve design products by proposing solutions by generative design tools based on the comprehensive overview provided by engineers and designers. In order to devise estimates of market demands, AI algorithms can research consumption, weather, socio-economic and macroeconomic and geographical trends. The data will help manufacturers anticipate shifts in the industry and optimize their energy usage, stock levels, raw material procurement and staffing to adapt more effectively to market changes. The next step in industrial technology is to link robots, computers and machinery to the IoT and improve it with ML algorithms. AI is one of the latest tools that manufacturers use to increase product consistency, effectiveness and reduce running costs ( Özdemir and Hekim , 2018 ; Zheng et al. , 2018 ; Sajid et al. , 2021 ). The transition from conventional automation focused on autonomous industrial robots to networked. Cyberphysical structures have revolutionized the functioning of manufacturing plants and introduced new competition requirements of Industry 4.0. The AI-driven production systems can tailor the production of components to the order. Sensors monitor components by ordering them to shorten lead times according to demand and algorithms. Manufacturing lines become information systems that feed decision-making in matters that are fundamental to the product line. It gives a concise view of what needs to be targeted next by drawing action items for the Industry 4.0 transformation plan. In order to improve productivity and add to the requisite multiadaptivity, the main aspects of Industry 4.0 reveal an AI for the IoT ( Radanliev et al. , 2020 ; Chetthamrongchai and Jermsittiparsert , 2020 ; Mhlanga , 2021 ). 8. Artificial Intelligence Applications for Industry 4.0AI strengthens companies’ analytical capacity to make accurate predictions and use their resources more effectively and minimize stock costs. There are many potentials to apply AI and ML in production. For a wide range of applications like production process modeling and predictive quality analytics, artificial neural networks have proven to be an incredibly efficient learning platform. AI is adopted for defect identification and decreasing waste to raise revenue projections. It also gives market managers an idea about updating business models as per the changing manufacturing sector. This technology is used for the real-time detection of defects. If several products show the same defect, the mistake can be corrected in real time ( Salkin et al. , 2018 ; Hou et al. , 2020 ). This helps businesses to schedule manufacturing lines well in advance, forecast demand and order inventory. As a result, projections in the supply chain can fluctuate depending on a range of variables that can be very difficult for man. This will save enormous time and resources because this sensitive technology limits waste from defects without human interference and further ensures that better products of extreme performance are manufactured. Instead of depending on people for time-intensive in-process testing and quality management, AI will help accelerate procedures and improve precision. The production methods, efficiency, protection, facility maintenance, logistics and manufacturing are manual, labor-intensive activities and processes ( Bibby and Dehe , 2018 ; Peres et al. , 2020 ). Table 1 shows significant applications of AI for implementing Industry 4.0. Applications of AI toward industry 4.0 implementation.S. no | Applications | Description with references |
---|
1 | Helps to perform a routine task | AI-enabled industrial robotics are used for automatic processing and performing routine tasks. This can avoid and minimize human bugs to a marginal pace using this technology. Assembly, welding, paint, stock testing, pick-up and putting, die molding, boiling, glass production and grinding are included in the applications. An industrial robot can track its accuracy and efficiency and prepare itself to improve with AI. Some production robots are equipped with this technology, which helps the robot in complex and random environments to achieve precision mobility ( , ; , ; , ; , ). | 2 | Production planning and demand forecasting | ML systems encourage resource planning, and they are good at handling production planning and demand forecasting. AI-driven demand prediction systems produce more precise outcomes than conventional demand prediction. Industries can handle inventory volumes better and thus reduce the likelihood of cash-in-stock and off-stock situations. Technology driven by AI will help companies refine processes for achieving sustainable levels of output. Manufacturers can prefer process mining software powered by AI to detect and remove bottlenecks from organizational processing ( , ; , ; , ). | 3 | Increase awareness of the product | AI helps producers to increase their product awareness and encourage companies to experiment with potential measures to improve asset efficiency. Until manufacturing its physical equivalent, manufacturers may use digital twins. This application allows companies to gather data from the virtual twin and develop the original data-driven product. Because of the change in market appetite for personalization, producers can employ digital twin devices to design different product permutations. This encourages consumers to buy the product based on output measurements instead of its architecture ( , ; , ; , ; , ). | 4 | Better monitoring and safety | AI provides better monitoring and safety and has become one of the most common causes of usage. This technology can be used for staff identification, thermal scanning or monitoring employee contacts for touch tracking and sanitation. AI had contributed to long-term protection solutions before they occurred or accelerated detection of the root cause after the occurrence. These solutions can contribute to happier staff, safer jobs and ongoing employment. AI reduces downtime and guarantees high-quality end goods in the production process. In addition, engineering firms use AI-based analytical solutions to enhance work performance in their data structures ( , ; , ; , ; , ). | 5 | Appropriate information | In a production system, information for several minutes does not appear or go unnoticed by the human eye. Advanced technology such as ML and AI helps identify microscopic deficiencies in circuit boards well beyond human vision. Therefore, these technologies can provide appropriate information in Industry 4.0. Furthermore, collaborative robots are becoming increasingly common in manufacturing firms. Robots can interact with human associates and be guided by humans, including new commands that are not expected in the original programming of the robot. Therefore, improved computer senses would result in longer-term safety ( , ; , ; , ; , ). | 6 | Designing and manufacturing | In the manufacture of goods with generative architecture, AI plays an important part. It is an iterative design process that includes feeding into the AI algorithms of comprehensive design details. This knowledge can cover many design criteria, including processing methods, nature of the product, time limits and budget constraints. The algorithm will examine any possible solution permutation by taking all these parameters into account and have the most appropriate output solutions ( , ; , ; , ; , ; , 2021b). | 7 | Detection of defects | ML and AI technologies can be beneficial here since cameras, lasers and scanning instruments can be linked to an AI program. It analyses objects as they travel down the production line. This technology is used for the real-time detection of defects. The several products show the same defect and can be corrected in real time. It will save enormous time and resources because this sensitive technology limits waste from defects without human interference. AI technology can produce valuable information that enables entrepreneurs to build innovative and robust business models. AI is beneficial at detecting patterns and phenomena where the ordinary human cannot see excessively ( , ; , ; , ; , ). | 8 | Enhance product efficiency | Automatic learning greatly enhances production efficiency by integrating predictive algorithms for maintenance into manufacturing operations. AI can quickly replace visual checks with highly precise and powerful robots. The challenge of AI usage in Industry 4.0 allows manufacturers to work with experts to find adequate and customized solutions. For the implementation of AI, the industries become more efficient. While the transition in Industry 4.0 is still in its early stages, AI has already brought us major benefits. This technology has intended to change the way for producing goods and process materials forever from the concept and manufacturing floors ( , ; , ; , ; , ). | 9 | Quality assurance | AI would have a different effect on production. For quality assurance, products are used to improve computer vision for in-time detection of product defects as manufacturers recognize the importance of AI in the reliable, timely detection and maintenance of the manufacturing line. This technology can minimize downtime and enhance product development, and continuous service is gaining momentum. In addition, AI algorithms will warn manufacturing teams to emerge failures, such as subtle anomalies in equipment and other problems, triggering product quality problems ( , ; , ; , ; , ). | 10 | Optimize processes | AI technology optimizes processes and promotes high efficiency. The future plant is modular, clean and optimally uses capital to produce everything from individual goods to mass. In terms of Industry 4.0, such versatility needs to be of high maturity; human beings work together with robotics in mixed teams and are assisted by intelligent support systems in their tasks using this technology. The use of AI in Industry 4.0 offers both actual manufacturing facility possibilities and challenges ( , ; , ; , ). | 11 | Supply chain monitoring | Machine education, natural language modeling, machine vision, robotics and language recognition make the management of supply chains more intelligent. This technology provides many supply chain monitoring applications. The demand for products can also be effectively predicted by AI systems using predictive analytics. AI production tools gather data from different sources and can precisely predict product demand based on them. In addition, the AI apps can handle order records and uninstall/install new stocks ( , ; , ; , ; , ). | 12 | Production management | AI is one of the most important technologies used for production management, market management and inventory management. Machines can be more powerful than people. This is much quicker in the performance of tasks than people. AI-powered robots execute repeated tasks without being scheduled. AI enhance production, customer support sales and quality and market efficiency through smart management. The AI production system can deliver optimization of the processes, low operating costs, high quality, rapid decision-making and customer experience improvement ( , ; , ; , ; , ; , ). | AI allows manufacturing firms to deal with unforeseen downtimes, low yields and low productivity by quick feedback loops. In supply chain management, the use of AI is growing exponentially. This technology gains traction during activities of distribution chain management. The smarter functions in supply-line management include ML and natural language processing, computer vision, robotics and speech recognition. Warehouse logistics and processes can be optimized using AI software and applications. Tools and software with AI capability can also effectively control and monitor fleet activities ( Carvajal Soto et al. , 2019 ; Ludbrook et al. , 2019 ; Villalba-Diez et al. , 2019 ; Azeem et al. , 2021 ; Massaro et al. , 2021 ). AI algorithms can easily report evolving production defects to manufacture teams that can trigger product quality problems. A high degree of consistency can be sustained by addressing these problems early. It also allows suppliers to gather information on their commodity use and success in the market. The AI algorithms can make the anticipation of demand trends in order to improve supply chains for manufacturing. AI-powered inspection tools have automatic procedures for fault detection. The smart equipment defect detecting tools in production track the equipment’s efficiency and condition ( Avishay et al. , 2019 ; O’Donovan et al. , 2019 ; Tao et al. , 2019 ; Neumann et al. , 2021 ). 9. Discussion on the FindingsAI is introduced in Industry 4.0 for the massive transition of producer firms and will provide new business models and lead to changes in productivity. Predictive repair cost control leads to less maintenance, decreasing labor costs, lower inventory and wasteful materials. Management of the supply chain through efficient stock management and a well-controlled and syncing output flow can be quickly undertaken using this technology. A mixture of refined machinery and adaptive software is visualized as the future of the industry. The simplicity and scalability ensure great data analysis and cloud computing infrastructure. Through this technology, companies will optimize manufacturing processes. In manufacturing processes, AI is utilized by original equipment makers who operate efficiently in smart factories and introduce Industry 4.0. This technology is used for better quality management, standardization and maintenance by predictive analysis of machinery functions and progressive rationalization of factory lines. Many businesses now want to incorporate AI in their manufacturing systems for better strategy and automation platforms. This makes it easy to adjust to demand and to move from the raw materials, joining the production chain to the finished product. Via knowledge networks, consumers are linked to the market and demand premium goods and interactions. Digital designs and smart development manufacturing companies can produce personalized goods without any productivity loss. The use of AI in Industry 4.0 is a development that radically changes the market in the years ahead. AI is one of the best examples of emerging technology in manufacturing. The companies are helped to include high-quality goods by developing tools that use AI capabilities. In addition, AI is the best and modern technology that combines sensor device processes, machines and data for improving the overall operation. Data is collected via the sensors, transmitted via the internet to the cloud server and analyzed via ML and AI algorithms. It is then returned to an automated robot or a service terminal to complete a complete workflow. As a result, industries create greater user understanding, production, product quality control, delivery logistics and consumer input. In today’s time, digital data must become compatible and refinable as a basis for new business models and monetization for the intensive use of AI and ML frameworks. In order to take suitable decisions, businesses need to be fully aware of their digitalization degree and Industry 4.0 willingness. Several indices have been developed for this purpose in the Industry 4.0 maturity evaluation of performance. Better quality control and feasible perspectives improved product quality continuously. Improved coordination with human machines improved safety and performance. AI enables risk analysis and businesses to identify breakdowns. The computers can be monitored in real time, downtimes prevented and total productivity increased. In all industries, AI has led to transformative developments. It is implemented in every field, including hospitals, life sciences, immobilization, education, manufacture, etc. The AI revolution will turn vast volumes of data into practical observations and forecasts that offer an incentive for fields powered by data such as biology, robots, connected and smart systems, etc. As Industry 4.0 demands a relatively precise and comprehensive data flow, the different modules used in the manufacturing phase can be refined. As a result, each part of the manufacturing line is increasingly scalable and detailed, enabling individual production to properly embody and anticipate customers’ desires, thereby producing a virtuous circle of production–sales input. AI is meant to transform the way we produce goods and process materials from the design and manufacturing floor to the supply and administration chain. Innovations have already been available to the automotive sector. Automation can enable production to achieve a high degree of precision and efficiency beyond human capacity. It can function in otherwise risky, tedious and challenging situations for human beings since large businesses rely on sizeable industrial manufacturing to achieve their competitive edge in our current stage of growth. The capacity of computers to mimic human intellectual skills is AI. They are equipped with electrical control circuits and electronic chips. These are the parts of AI that provide software mechanisms and maintain them. The system is also supposed to deal with sensory functions. The device is very convenient for AI researchers to link up on the server. ML frees corporate knowledge and the management of decisions in a greater domain. There were hundreds of variables that influence the development process, although these are very difficult to interpret for people. ML models can easily predict the effect of individual variables in dynamic scenarios. Machines also operate under human capacity in other sectors that include language or feelings. For example, the maintenance of machinery and equipment production lines is higher in the industrial industry, which significantly influences any production process that connects with assets. Computer education and predictive analytics have turned management of the supply chain into a smooth operation. For sorting and packing goods, warehouses are using AI-enhanced robots. In addition, AI algorithms are being increasingly used to identify the fastest shipping route and facilitate the distribution of goods to customers at various places. The availability of extensive data on how the goods are evaluated and how they work describes the various fields that need to be tested for AI software and machines. Predictive system management enables manufacturers to prevent overhead disruption to equipment. It can determine whether machinery requires repair services through AI-powered predictive analytical solutions. 10. Future of Artificial Intelligence in Industry 4.0AI will provide valuable information that enables corporate executives to develop innovative and robust business models. This system will become highly useful at detecting patterns and phenomena where the ordinary human cannot see excessively. AI will produce data to allow to make sound, factual and data-oriented business decisions. It will also help to remove individual prejudices from the calculation, and in many ways, provide a more detailed overview. AI and ML tools can gather data from several different sources and find growth, extension and even new market opportunities, and new products and services will be developed. Other innovations such as blockchain and edge computing have become more popular, and their fusion with IoT also provides new applications. Soon, AI will boom with new advantages that will help to create a wired, intelligent and smart society. For example, manufacturing may have highly ineffective procedures, which are usually refined and modified continuously. Robotics as a service would have the capacity to recreate specific human functions in the future, such as speech and image recognition, with the help of AI. It can track, analyze production quotas and can contribute to predictive maintenance models. 11. ConclusionIndustry 4.0, with the help of AI, completely automates the control of the different stages of the production processes. Based on the product specifications, any stage of the manufacturing procedure will be refined in real time. It can integrate the complete development chain, and the job load involved in data processes can be extended to many divisions. The data collection systems and data feedback systems can be incorporated into manufacturing processes through AI. This technology can share assembly lines with production processes to increase efficiency. Advanced AI algorithms are used for repair prediction and to formulate asset failure predictions. The integration of AI with Industry 4.0 provides various industrial developments. AI can better manage the related output processes. This technology can create meaningful perspectives that contribute to creativity in manufacturing. The physical representation of the production environment is fully visualized with data collection instruments such as sensors and cameras. The data generated by intelligent components are gathered, saved and processed using a cloud link. In the future, this will collect cloud information and make a smooth functioning of Industry 4.0. - Abubakar, AM and I Adeshola ( 2019 ) Digital exam and assessments: A riposte to industry 4.0 . In Handbook of Research on Faculty Development for Digital Teaching and Learning , pp. 245–263. IGI Global. Crossref , Google Scholar
- Ahmed, RS, ESA Ahmed and RA Saeed ( 2021 ) Machine learning in cyber-physical systems in industry 4.0 . In Artificial Intelligence Paradigms for Smart Cyber-Physical Systems , pp. 20–41. IGI Global. Crossref , Google Scholar
- Ammar, M, A Haleem, M Javaid, R Walia and S Bahl ( 2021 ) Improving material quality management and manufacturing organizations system through industry 4.0 technologies . Materials Today: Proceedings , 45 , 5089–5096. Crossref , Google Scholar
- Angelopoulos, A, ET Michailidis, N Nomikos, P Trakadas, A Hatziefremidis, S Voliotis and T Zahariadis ( 2020 ) Tackling faults in the industry 4.0 era—A survey of machine-learning solutions and key aspects . Sensors , 20 (1), 109. Crossref , Google Scholar
- Ansari, F, S Erol and W Sihn ( 2018 ) Rethinking human-machine learning in industry 4.0: How does the paradigm shift treat the role of human learning? . Procedia Manufacturing , 23 , 117–122. Crossref , Google Scholar
- Ashima, R, A Haleem, S Bahl, M Javaid, SK Mahla and S Singh ( 2021 ) Automation and manufacturing of smart materials in additive manufacturing technologies using internet of things towards the adoption of industry 4.0 . Materials Today: Proceedings , 45 , 5081–5088. Crossref , Google Scholar
- Avishay, D, V Pavlov, G Pavlova, B Petrov and N Dimitrov ( 2019 ) Industry 4.0 – Robots with distributed mobility and elements of artificial intelligence . Global Journal of Computer Science and Technology . Google Scholar
- Azeem, M, A Haleem, S Bahl, M Javaid, R Suman and D Nandan ( 2021 ) Big data applications to take up major challenges across manufacturing industries: A brief review . Materials Today: Proceedings . Google Scholar
- Azizi, A ( 2020 ) Applications of artificial intelligence techniques to enhance sustainability of industry 4.0: Design of an artificial neural network model as dynamic behavior optimizer of robotic arms . Complexity , 2020 , 8564140. Crossref , Google Scholar
- Badri, A, B Boudreau-Trudel and AS Souissi ( 2018 ) Occupational health and safety in the industry 4.0 era: A cause for major concern? . Safety Science , 109 , 403–411. Crossref , Google Scholar
- Bag, S, JH Pretorius, S Gupta and YK Dwivedi ( 2021 ) Role of institutional pressures and resources in the adoption of big data analytics powered artificial intelligence, sustainable manufacturing practices and circular economy capabilities . Technological Forecasting and Social Change , 163 , 120420. Crossref , Google Scholar
- Bahrin, MA, MF Othman, NH Azli and MF Talib ( 2016 ) Industry 4.0: A review on industrial automation and robotic . Jurnal Teknologi , 78 (6–13). Google Scholar
- Bai, C, P Dallasega, G Orzes and J Sarkis ( 2020 ) Industry 4.0 technologies assessment: A sustainability perspective . International Journal of Production Economics , 229 , 107776. Crossref , Google Scholar
- Banjanović-Mehmedović, L and F Mehmedović ( 2020 ) Intelligent manufacturing systems driven by artificial intelligence in industry 4.0 . In Handbook of Research on Integrating Industry 4.0 in Business and Manufacturing , pp. 31–52. IGI global. Crossref , Google Scholar
- Bécue, A, I Praça and J Gama ( 2021 ) Artificial intelligence, cyber-threats and industry 4.0: Challenges and opportunities . Artificial Intelligence Review , 54 , 3849–3886. Crossref , Google Scholar
- Benotsmane, R, G Kovács and L Dudás ( 2019 ) Economic, social impacts and operation of smart factories in industry 4.0 focusing on simulation and artificial intelligence of collaborating robots . Social Sciences , 8 (5), 143. Crossref , Google Scholar
- Bibby, L and B Dehe ( 2018 ) Defining and assessing industry 4.0 maturity levels–case of the defence sector . Production Planning & Control , 29 (12), 1030–1043. Crossref , Google Scholar
- Bogoviz, AV ( 2020 ) Perspective directions of state regulation of competition between human and artificial intellectual capital in industry 4.0 . Journal of Intellectual Capital , 21 (4), 583–600. Crossref , Google Scholar
- Borowski, PF ( 2021 ) Digitization, digital twins, blockchain, and industry 4.0 as elements of management process in enterprises in the energy sector . Energies , 14 (7), 1885. Crossref , Google Scholar
- Bortolini, M, E Ferrari, M Gamberi, F Pilati and M Faccio ( 2017 ) Assembly system design in the industry 4.0 era: A general framework . IFAC-PapersOnLine , 50 (1), 5700–5705. Crossref , Google Scholar
- Bourke, E ( 2019 ) Smart production systems in industry 4.0: Sustainable supply chain management, cognitive decision-making algorithms, and dynamic manufacturing processes . Journal of Self-Governance and Management Economics , 7 (2), 25–30. Crossref , Google Scholar
- Bousdekis, A, D Apostolou and G Mentzas ( 2020 ) A human cyber physical system framework for operator 4.0–artificial intelligence symbiosis . Manufacturing Letters , 25 , 10–15. Crossref , Google Scholar
- Candanedo, IS, EH Nieves, SR González, MT Martín and AG Briones ( 2018 ) Machine learning predictive model for industry 4.0 . In Int. Conf. Knowledge Management in Organizations , pp. 501–510. Cham: Springer. Crossref , Google Scholar
- Canito, A, D Mota, G Marreiros, JM Corchado and C Martins ( 2020 ) Contextual adaptative interfaces for industry 4.0 . In Int. Symp. Distributed Computing and Artificial Intelligence , pp. 149–157. Cham: Springer. Google Scholar
- Carvajal Soto, JA, F Tavakolizadeh and D Gyulai ( 2019 ) An online machine learning framework for early detection of product failures in an industry 4.0 context . International Journal of Computer Integrated Manufacturing , 32 (4–5), 452–465. Crossref , Google Scholar
- Chao, L, L Jiafei, Z Liming, G Aicheng, F Yipeng, Y Jiangpeng and L Xiu ( 2020 ) Nuclear power plants with artificial intelligence in industry 4.0 era: Top-level design and current applications—A systemic review . IEEE Access , 8 , 194315–194332. Crossref , Google Scholar
- Chen, Y and Y Li ( 2019 ) Intelligent autonomous pollination for future farming-a micro air vehicle conceptual framework with artificial intelligence and human-in-the-loop . IEEE Access , 7 , 119706–119717. Crossref , Google Scholar
- Chen, H, L Li and Y Chen ( 2020 ) Explore success factors that impact artificial intelligence adoption on telecom industry in China . Journal of Management Analytics , 8 (1), 36–68. Crossref , Google Scholar
- Chen, ZB and LD Xu ( 2001 ) An object-oriented intelligent CAD system for ceramic kiln . Knowledge-Based Systems , 14 (5–6), 263–270. Crossref , Google Scholar
- Cheng, GJ, LT Liu, XJ Qiang and Y Liu ( 2016 ) Industry 4.0 development and application of intelligent manufacturing . In 2016 Int. Conf. Information System and Artificial Intelligence (ISAI) , pp. 407–410. IEEE. Crossref , Google Scholar
- Chetthamrongchai, P and K Jermsittiparsert ( 2020 ) Ensuring environmental performance of pharmaceutical companies of Thailand: Role of robotics and Al awareness and technical content knowledge in industry 4.0 Era . Systematic Reviews in Pharmacy , 11 (1), 129–138. Google Scholar
- Chi-Hsien, K and S Nagasawa ( 2019 ) Applying machine learning to market analysis: Knowing your luxury consumer . Journal of Management Analytics , 6 (4), 404–419. Crossref , Google Scholar
- Chun, KW, H Kim and K Lee ( 2018 ) A study on research trends of technologies for industry 4.0; 3 D printing, artificial intelligence, big data, cloud computing, and internet of things . In Advanced Multimedia and Ubiquitous Engineering , pp. 397–403. Singapore: Springer. Google Scholar
- Cioffi, R, M Travaglioni, G Piscitelli, A Petrillo and F De Felice ( 2020 ) Artificial intelligence and machine learning applications in smart production: Progress, trends, and directions . Sustainability , 12 (2), 492. Crossref , Google Scholar
- Cotet, GB, NL Carutasu and F Chiscop ( 2020 ) Industry 4.0 diagnosis from an millennial educational perspective . Education Sciences , 10 (1), 21. Crossref , Google Scholar
- Da Costa, MB, LM Dos Santos, JL Schaefer, IC Baierle and EO Nara ( 2019 ) Industry 4.0 technologies basic network identification . Scientometrics , 121 (2), 977–994. Crossref , Google Scholar
- Dal Mas, F, D Piccolo, L Cobianchi, L Edvinsson, G Presch, M Massaro, M Skrap, A Ferrario di Tor Vajana, S D’Auria and C Bagnoli ( 2019 ) The effects of artificial intelligence, robotics, and industry 4.0 technologies. Insights from the healthcare sector . In Proc. First European Conf. Impact of Artificial Intelligence and Robotics , pp. 88–95. Google Scholar
- Davidson, R ( 2020 ) Cyber-physical production networks, artificial intelligence-based decision-making algorithms, and big data-driven innovation in industry 4.0-based manufacturing systems . Economics, Management, and Financial Markets , 15 (3), 16–22. Crossref , Google Scholar
- Dhanabalan, T and A Sathish ( 2018 ) Transforming Indian industries through artificial intelligence and robotics in industry 4.0 . International Journal of Mechanical Engineering and Technology , 9 (10), 835–845. Google Scholar
- Diez-Olivan, A, J Del Ser, D Galar and B Sierra ( 2019 ) Data fusion and machine learning for industrial prognosis: Trends and perspectives towards industry 4.0 . Information Fusion , 50 , 92–111. Crossref , Google Scholar
- Dudukalov, EV, VD Munister, AL Zolkin, AN Losev and AV Knishov ( 2021 ) The use of artificial intelligence and information technology for measurements in mechanical engineering and in process automation systems in industry 4.0 . Journal of Physics: Conference Series , 1889 (5), 052011. Crossref , Google Scholar
- Duft, G and P Durana ( 2020 ) Artificial intelligence-based decision-making algorithms, automated production systems, and big data-driven innovation in sustainable industry 4.0 . Economics, Management and Financial Markets , 15 (4), 9–18. Crossref , Google Scholar
- Fatima, S, A Haleem, S Bahl, M Javaid, SK Mahla and S Singh ( 2021 ) Exploring the significant applications of internet of things (IoT) with 3D printing using advanced materials in medical field . Materials Today: Proceedings , 45 (Part 6), 4844–4851. Crossref , Google Scholar
- Felstead, M ( 2019 ) Cyber-physical production systems in industry 4.0: Smart factory performance, manufacturing process innovation, and sustainable supply chain networks . Economics, Management, and Financial Markets , 14 (4), 37–43. Crossref , Google Scholar
- Feng, S and LD Xu ( 1999 ) Hybrid artificial intelligence approach to urban planning . Expert Systems , 16 (4), 248–261. Crossref , Google Scholar
- Frank, AG, LS Dalenogare and NF Ayala ( 2019 ) Industry 4.0 technologies: Implementation patterns in manufacturing companies . International Journal of Production Economics , 210 , 15–26. Crossref , Google Scholar
- García, NM ( 2019 ) Multi-agent system for anomaly detection in industry 4.0 using machine learning techniques . ADCAIJ: Advances in Distributed Computing and Artificial Intelligence Journal , 8 (4), 33–40. Crossref , Google Scholar
- Gomes, MG, VH da Silva, LF Pinto, P Centoamore, S Digiesi, F Facchini and GC Neto ( 2020 ) Economic, environmental and social gains of the implementation of artificial intelligence at dam operations toward industry 4.0 principles . Sustainability , 12 (9), 3604. Crossref , Google Scholar
- Gray-Hawkins, M, L Michalkova, P Suler and NA Zhuravleva ( 2019 ) Real-time process monitoring in industry 4.0 manufacturing systems: Sensing, smart, and sustainable technologies . Economics, Management and Financial Markets , 14 (4), 30–36. Crossref , Google Scholar
- Gupta, BB, A Tewari, I Cvitić, D Peraković and X Chang ( 2021 ) Artificial intelligence empowered emails classifier for internet of things based systems in industry 4.0 . Wireless Networks . Google Scholar
- Haenlein, M, A Kaplan, CW Tan and P Zhang ( 2019 ) Artificial intelligence (AI) and management analytics . Journal of Management Analytics , 6 (4), 341–343. Crossref , Google Scholar
- Haleem, A, M Javaid and IH Khan ( 2019 ) Current status and applications of artificial intelligence (AI) in medical field: An overview . Current Medicine Research and Practice , 9 (6), 231–237. Crossref , Google Scholar
- Haleem, A, M Javaid, RP Singh and R Suman ( 2021 ) Applications of artificial intelligence (AI) for cardiology during COVID-19 pandemic . Sustainable Operations and Computers , 2 , 71–78. Crossref , Google Scholar
- Haleem, A and M Javaid ( 2019 ) Additive manufacturing applications in industry 4.0: A review . Journal of Industrial Integration and Management , 4 (4), 1930001. Link , Google Scholar
- Haleem, A and M Javaid ( 2020 ) Medical 4.0 and its role in healthcare during COVID-19 pandemic: A review . Journal of Industrial Integration and Management , 5 (4), 531–545. Link , Google Scholar
- Haleem, A, R Vaishya, M Javaid and IH Khan ( 2020 ) Artificial intelligence (AI) applications in orthopaedics: An innovative technology to embrace . Journal of Clinical Orthopaedics & Trauma , 11 , S80–S81. Crossref , Google Scholar
- Hansen, EB and S Bøgh ( 2021 ) Artificial intelligence and internet of things in small and medium-sized enterprises: A survey . Journal of Manufacturing Systems , 58 , 362–372. Crossref , Google Scholar
- Hao, M, H Li, X Luo, G Xu, H Yang and S Liu ( 2019 ) Efficient and privacy-enhanced federated learning for industrial artificial intelligence . IEEE Transactions on Industrial Informatics , 16 (10), 6532–6542. Crossref , Google Scholar
- Hayhoe, T, I Podhorska, A Siekelova and V Stehel ( 2019 ) Sustainable manufacturing in industry 4.0: Cross-sector networks of multiple supply chains, cyber-physical production systems, and AI-driven decision-making . Journal of Self-Governance and Management Economics , 7 (2), 31–36. Crossref , Google Scholar
- Helmold, M ( 2019 ) Industry 4.0 and artificial intelligence (AI) in PM . In Progress in Performance Management , pp. 161–163. Cham: Springer. Crossref , Google Scholar
- Hofmann, E, H Sternberg, H Chen, A Pflaum and G Prockl ( 2019 ) Supply chain management and industry 4.0: Conducting research in the digital age . International Journal of Physical Distribution & Logistics Management , 49 (10), 945–955. Crossref , Google Scholar
- Hou, T, B Cheng, R Wang, W Xue and PE Chaudhry ( 2020 ) Developing industry 4.0 with systems perspectives . Systems Research and Behavioral Science , 37 (4), 741–748. Crossref , Google Scholar
- Hughes, L, YK Dwivedi, NP Rana, MD Williams and V Raghavan ( 2020 ) Perspectives on the future of manufacturing within the industry 4.0 era . Production Planning & Control , 1–21. Google Scholar
- Ibrahim, WM and R Hassan ( 2019 ) Recruitment trends in the era of industry 4.0 using artificial intelligence: Pro and cons . Asian Journal of Research in Business and Management , 1 (1), 16–21. Google Scholar
- Ivanov, D, CS Tang, A Dolgui, D Battini and A Das ( 2020 ) Researchers’ perspectives on industry 4.0: Multi-disciplinary analysis and opportunities for operations management . International Journal of Production Research , 59 (7), 2055–2078. Crossref , Google Scholar
- Javaid, M, A Haleem, R Vaishya, S Bahl, R Suman and A Vaish ( 2020 ) Industry 4.0 technologies and their applications in fighting COVID-19 pandemic . Diabetes & Metabolic Syndrome: Clinical Research & Reviews , 14 (4), 419–422. Crossref , Google Scholar
- Javaid, M and A Haleem ( 2020 ) Critical components of industry 5.0 towards a successful adoption in the field of manufacturing . Journal of Industrial Integration and Management , 5 (3), 327–348. Link , Google Scholar
- Javaid, M and A Haleem ( 2019 ) Industry 4.0 applications in medical field: A brief review . Current Medicine Research and Practice , 9 (3), 102–109. Crossref , Google Scholar
- Jimeno-Morenilla, A, P Azariadis, R Molina-Carmona, S Kyratzi and V Moulianitis ( 2021 ) Technology enablers for the implementation of industry 4.0 to traditional manufacturing sectors: A review . Computers in Industry , 125 , 103390. Crossref , Google Scholar
- Kebisek, M, P Tanuska, L Spendla, J Kotianova and P Strelec ( 2020 ) Artificial intelligence platform proposal for paint structure quality prediction within the industry 4.0 concept . IFAC-PapersOnLine , 53 (2), 11168–11174. Crossref , Google Scholar
- Kempegowda, SM and Z Chaczko ( 2018 ) Industry 4.0 complemented with EA approach: A proposal for digital transformation success . In 2018 26th Int. Conf. Systems Engineering (ICSEng) , pp. 1–6. IEEE. Crossref , Google Scholar
- Khayyam, H, B Javadi, M Jalili and RN Jazar ( 2020 ) Artificial intelligence and internet of things for autonomous vehicles . In Nonlinear Approaches in Engineering Applications , pp. 39–68. Cham: Springer. Crossref , Google Scholar
- Kliestik, T, E Nica, H Musa, M Poliak and EA Mihai ( 2020 ) Networked, smart, and responsive devices in industry 4.0 manufacturing systems . Economics, Management and Financial Markets , 15 (3), 23–29. Crossref , Google Scholar
- Koh, L, G Orzes and FJ Jia ( 2019 ) The fourth industrial revolution (industry 4.0): Technologies disruption on operations and supply chain management . International Journal of Operations & Production Management . Crossref , Google Scholar
- Kumar, A and D Gupta ( 2020 ) Challenges within the industry 4.0 setup . In A Roadmap to Industry 4.0: Smart Production, Sharp Business and Sustainable Development , pp. 187–205. Cham: Springer. Crossref , Google Scholar
- Kunst, R, L Avila, A Binotto, E Pignaton, S Bampi and J Rochol ( 2019 ) Improving devices communication in industry 4.0 wireless networks . Engineering Applications of Artificial Intelligence , 83 , 1–2. Crossref , Google Scholar
- Kushwaha, S, S Bahl, AK Bagha, KS Parmar, M Javaid, A Haleem and RP Singh ( 2020 ) Significant applications of machine learning for COVID-19 pandemic . Journal of Industrial Integration and Management , 5 (4). Link , Google Scholar
- Lafferty, C ( 2019 ) Sustainable industry 4.0: Product decision-making information systems, data-driven innovation, and smart industrial value creation . Journal of Self-Governance and Management Economics , 7 (2), 19–24. Crossref , Google Scholar
- Lăzăroiu, G, T Kliestik and A Novak ( 2021 ) Internet of things smart devices, industrial artificial intelligence, and real-time sensor networks in sustainable cyber-physical production systems . Journal of Self-Governance and Management Economics , 9 , 20–30. Google Scholar
- Lee, C and C Lim ( 2021 ) From technological development to social advance: A review of industry 4.0 through machine learning . Technological Forecasting and Social Change , 167 , 120653. Crossref , Google Scholar
- Lee, J, H Davari, J Singh and V Pandhare ( 2018 ) Industrial artificial intelligence for industry 4.0-based manufacturing systems . Manufacturing Letters , 18 , 20–23. Crossref , Google Scholar
- Lee, WJ, H Wu, H Yun, H Kim, MB Jun and JW Sutherland ( 2019 ) Predictive maintenance of machine tool systems using artificial intelligence techniques applied to machine condition data . Procedia CIRP , 80 , 506–511. Crossref , Google Scholar
- Leng, J, G Ruan, Y Song, Q Liu, Y Fu, K Ding and X Chen ( 2021 ) A loosely-coupled deep reinforcement learning approach for order acceptance decision of mass-individualized printed circuit board manufacturing in industry 4.0 . Journal of Cleaner Production , 280 , 124405. Crossref , Google Scholar
- Li, BH, BC Hou, WT Yu, XB Lu and CW Yang ( 2017 ) Applications of artificial intelligence in intelligent manufacturing: A review . Frontiers of Information Technology & Electronic Engineering , 18 (1), 86–96. Crossref , Google Scholar
- Lu, Y ( 2019 ) Artificial intelligence: A survey on evolution, models, applications and future trends . Journal of Management Analytics , 6 (1), 1–29. Crossref , Google Scholar
- Ludbrook, F, KF Michalikova, Z Musova and P Suler ( 2019 ) Business models for sustainable innovation in industry 4.0: Smart manufacturing processes, digitalization of production systems, and data-driven decision making . Journal of Self-Governance and Management Economics , 7 (3), 21–26. Crossref , Google Scholar
- Masood, T and P Sonntag ( 2020 ) Industry 4.0: Adoption challenges and benefits for SMEs . Computers in Industry , 121 , 103261. Crossref , Google Scholar
- Massaro, A, N Contuzzi and A Galiano ( 2020 ) Intelligent processes in automated production involving industry 4.0 technologies and artificial intelligence . In Advanced Robotics and Intelligent Automation in Manufacturing , pp. 97–122. IGI Global. Crossref , Google Scholar
- Massaro, A, V Maritati, A Galiano, V Birardi and L Pellicani ( 2018 ) ESB platform integrating KNIME data mining tool oriented on industry 4.0 based on artificial neural network predictive maintenance . International Journal of Artificial Intelligence and Applications , 9 (3), 1–7. Crossref , Google Scholar
- Massaro, M, S Secinaro, F Dal Mas, V Brescia and D Calandra ( 2021 ) Industry 4.0 and circular economy: An exploratory analysis of academic and practitioners’ perspectives . Business Strategy and the Environment , 30 (2), 1213–1231. Crossref , Google Scholar
- Mazurek, G and K Małagocka ( 2019 ) Perception of privacy and data protection in the context of the development of artificial intelligence . Journal of Management Analytics , 6 (4), 344–364. Crossref , Google Scholar
- Merayo, D, A Rodriguez-Prieto and AM Camacho ( 2019 ) Comparative analysis of artificial intelligence techniques for material selection applied to manufacturing in industry 4.0 . Procedia Manufacturing , 41 , 42–49. Crossref , Google Scholar
- Mhlanga, D ( 2021 ) Artificial intelligence in the industry 4.0, and its impact on poverty, innovation, infrastructure development, and the sustainable development goals: Lessons from emerging economies? . Sustainability , 13 (11), 5788. Crossref , Google Scholar
- Mhlanga, D ( 2020 ) Industry 4.0 in finance: The impact of artificial intelligence (ai) on digital financial inclusion . International Journal of Financial Studies , 8 (3), 45. Crossref , Google Scholar
- Milward, R, GH Popescu, KF Michalikova, Z Musova and V Machova ( 2019 ) Sensing, smart, and sustainable technologies in industry 4.0: Cyber-physical networks, machine data capturing systems, and digitized mass production . Economics, Management and Financial Markets , 14 (3), 37–43. Crossref , Google Scholar
- Nascimento, AM and CG Bellini ( 2018 ) Artificial intelligence and industry 4.0: The next frontier in organizations . BAR-Brazilian Administration Review , 15 (4). Crossref , Google Scholar
- Neumann, WP, S Winkelhaus, EH Grosse and CH Glock ( 2021 ) Industry 4.0 and the human factor – A systems framework and analysis methodology for successful development . International Journal of Production Economics , 233 , 107992. Crossref , Google Scholar
- Ng, HS ( 2020 ) Opportunities, challenges, and solutions for industry 4.0 . In Business Management and Communication Perspectives in Industry 4.0 , pp. 32–51. IGI Global. Crossref , Google Scholar
- O’Donovan, P, C Gallagher, K Leahy and DT O’Sullivan ( 2019 ) A comparison of fog and cloud computing cyber-physical interfaces for industry 4.0 real-time embedded machine learning engineering applications . Computers in Industry , 110 , 12–35. Crossref , Google Scholar
- Olsen, TL and B Tomlin ( 2020 ) Industry 4.0: Opportunities and challenges for operations management . Manufacturing & Service Operations Management , 22 (1), 113–122. Crossref , Google Scholar
- Özdemir, V and N Hekim ( 2018 ) Birth of industry 5.0: Making sense of big data with artificial intelligence, “the internet of things” and next-generation technology policy . Omics: A Journal of Integrative Biology , 22 (1), 65–76. Crossref , Google Scholar
- Oztemel, E and S Gursev ( 2020 ) Literature review of industry 4.0 and related technologies . Journal of Intelligent Manufacturing , 31 (1), 127–182. Crossref , Google Scholar
- Paolanti, M, L Romeo, A Felicetti, A Mancini, E Frontoni and J Loncarski ( 2018 ) Machine learning approach for predictive maintenance in industry 4.0 . In 2018 14th IEEE/ASME Int. Conf. Mechatronic and Embedded Systems and Applications (MESA) , pp. 1–6. IEEE. Crossref , Google Scholar
- Pereira, AG, TM Lima and F Charrua-Santos ( 2020 ) Industry 4.0 and society 5.0: Opportunities and threats . International Journal of Recent Technology and Engineering , 8 (5), 3305–3308. Google Scholar
- Peres, RS, X Jia, J Lee, K Sun, AW Colombo and J Barata ( 2020 ) Industrial artificial intelligence in industry 4.0 - Systematic review, challenges and outlook . IEEE Access , 8 , 220121–220139. Crossref , Google Scholar
- Petrasch, R and R Hentschke ( 2016 ) Process modeling for industry 4.0 applications: Towards an industry 4.0 process modeling language and method . In 2016 13th Int. Joint Conf. Computer Science and Software Engineering (JCSSE) , pp. 1–5. IEEE. Crossref , Google Scholar
- Pilati, F and A Regattieri ( 2018 ) The impact of digital technologies and artificial intelligence on production systems in today industry 4.0 environment . Network Industries Quarterly , 20 (2), 16–20. Google Scholar
- Pires, F, A Cachada, J Barbosa, AP Moreira and P Leitão ( 2019 ) Digital twin in industry 4.0: Technologies, applications and challenges . In 2019 IEEE 17th Int. Conf. Industrial Informatics (INDIN) , Vol. 1 , pp. 721–726. IEEE. Crossref , Google Scholar
- Popkova, EG and BS Sergi ( 2020 ) Human capital and AI in industry 4.0. Convergence and divergence in social entrepreneurship in Russia . Journal of Intellectual Capital . Crossref , Google Scholar
- Popkova, EG ( 2019 ) Preconditions of formation and development of industry 4.0 in the conditions of knowledge economy . In Industry 4.0: Industrial Revolution of the 21st Century , pp. 65–72. Cham: Springer. Crossref , Google Scholar
- Preuveneers, D and E Ilie-Zudor ( 2017 ) The intelligent industry of the future: A survey on emerging trends, research challenges and opportunities in industry 4.0 . Journal of Ambient Intelligence and Smart Environments , 9 (3), 287–298. Crossref , Google Scholar
- Radanliev, P, D De Roure, R Nicolescu, M Huth and O Santos ( 2021a ) Artificial intelligence and the internet of things in industry 4.0 . CCF Transactions on Pervasive Computing and Interaction . Crossref , Google Scholar
- Radanliev, P, D De Roure, R Nicolescu, M Huth and O Santos ( 2021b ) Digital twins: Artificial intelligence and the IoT cyber-physical systems in industry 4.0 . International Journal of Intelligent Robotics and Applications . Google Scholar
- Radanliev, P, D De Roure, K Page, JR Nurse, R Mantilla Montalvo, O Santos, LT Maddox and P Burnap ( 2020 ) Cyber risk at the edge: Current and future trends on cyber risk analytics and artificial intelligence in the industrial internet of things and industry 4.0 supply chains . Cybersecurity , 3 , 1–21. Crossref , Google Scholar
- Ribeiro, J, R Lima, T Eckhardt and S Paiva ( 2021 ) Robotic process automation and artificial intelligence in industry 4.0–A literature review . Procedia Computer Science , 181 , 51–58. Crossref , Google Scholar
- Riley, C, J Vrbka and Z Rowland ( 2021 ) Internet of things-enabled sustainability, big data-driven decision-making processes, and digitized mass production in industry 4.0-based manufacturing systems . Journal of Self-Governance and Management Economics , 9 , 42–52. Google Scholar
- Rizvi, AT, A Haleem, S Bahl and M Javaid ( 2021 ) Artificial intelligence (AI) and its applications in Indian manufacturing: A review . In Current Advances in Mechanical Engineering , pp. 825–835. Singapore: Springer. Crossref , Google Scholar
- Romeo, L, J Loncarski, M Paolanti, G Bocchini, A Mancini and E Frontoni ( 2020 ) Machine learning-based design support system for the prediction of heterogeneous machine parameters in industry 4.0 . Expert Systems with Applications , 140 , 112869. Crossref , Google Scholar
- Ruiz-Sarmiento, JR, J Monroy, FA Moreno, C Galindo, JM Bonelo and J Gonzalez-Jimenez ( 2020 ) A predictive model for the maintenance of industrial machinery in the context of industry 4.0 . Engineering Applications of Artificial Intelligence , 87 , 103289. Crossref , Google Scholar
- Sajid, S, A Haleem, S Bahl, M Javaid, T Goyal and M Mittal ( 2021 ) Data science applications for predictive maintenance and materials science in context to industry 4.0 . Materials Today: Proceedings . Crossref , Google Scholar
- Salkin, C, M Oner, A Ustundag and E Cevikcan ( 2018 ) A conceptual framework for industry 4.0 . In Industry 4.0: Managing the Digital Transformation , pp. 3–23. Cham: Springer. Crossref , Google Scholar
- Samarasinghe, KR and A Medis ( 2020 ) Artificial intelligence based strategic human resource management (AISHRM) for industry 4.0 . Global Journal of Management and Business Research . Crossref , Google Scholar
- Sanchez, DO ( 2019 ) Corporate social responsibility challenges and risks of industry 4.0 technologies: A review . In Smart SysTech 2019; European Conf. Smart Objects, Systems and Technologies , pp. 1–8. IEEE. Google Scholar
- Sanchez, M, E Exposito and J Aguilar ( 2020 ) Autonomic computing in manufacturing process coordination in industry 4.0 context . Journal of Industrial Information Integration , 19 , 100159. Crossref , Google Scholar
- Sharma, M, S Kamble, V Mani, R Sehrawat, A Belhadi and V Sharma ( 2021 ) Industry 4.0 adoption for sustainability in multi-tier manufacturing supply chain in emerging economies . Journal of Cleaner Production , 281 , 125013. Crossref , Google Scholar
- Shi, S, LD Xu and B Liu ( 1995 ) Artificial neural network for combining forecasts . Journal of Systems Engineering and Electronics , 6 (2), 58–64. Google Scholar
- Sima, V, IG Gheorghe, J Subić and D Nancu ( 2020 ) Influences of the industry 4.0 revolution on the human capital development and consumer behavior: A systematic review . Sustainability , 12 (10), 4035. Crossref , Google Scholar
- Singer, G and Y Cohen ( 2021 ) A framework for smart control using machine-learning modeling for processes with closed-loop control in industry 4.0 . Engineering Applications of Artificial Intelligence , 102 , 104236. Crossref , Google Scholar
- Singh, RK, P Kumar and M Chand ( 2019 ) Evaluation of supply chain coordination index in context to industry 4.0 environment . Benchmarking: An International Journal . Google Scholar
- Skobelev, PO and SY Borovik ( 2017 ) On the way from industry 4.0 to industry 5.0: From digital manufacturing to digital society . Industry 4.0 , 2 (6), 307–311. Google Scholar
- Stentoft, J, K Adsbøll Wickstrøm, K Philipsen and A Haug ( 2020 ) Drivers and barriers for industry 4.0 readiness and practice: Empirical evidence from small and medium-sized manufacturers . Production Planning & Control , 32 (10), 811–828. Crossref , Google Scholar
- Tang, CS and LP Veelenturf ( 2019 ) The strategic role of logistics in the industry 4.0 era . Transportation Research Part E: Logistics and Transportation Review , 129 , 1–11. Crossref , Google Scholar
- Tang, C, L Xu and S Feng ( 2001 ) An agent-based geographical information system . Knowledge-Based Systems , 14 (5–6), 233–242. Crossref , Google Scholar
- Tao, F, Q Qi, L Wang and AY Nee ( 2019 ) Digital twins and cyber–physical systems toward smart manufacturing and industry 4.0: Correlation and comparison . Engineering , 5 (4), 653–661. Crossref , Google Scholar
- Tarassov, VB ( 2018 ) Enterprise total agentification as a way to industry 4.0: Forming artificial societies via goal-resource networks . In Int. Conf. Intelligent Information Technologies for Industry , pp. 26–40. Cham: Springer. Google Scholar
- Teoh, YK, SS Gill and AK Parlikad ( 2021 ) IoT and fog computing based predictive maintenance model for effective asset management in industry 4.0 using machine learning . IEEE Internet of Things Journal. Crossref , Google Scholar
- Terziyan, V, S Gryshko and M Golovianko ( 2018 ) Patented intelligence: Cloning human decision models for industry 4.0 . Journal of Manufacturing Systems , 48 , 204–217. Crossref , Google Scholar
- Tiwari, K and MS Khan ( 2020 ) Sustainability accounting and reporting in the industry 4.0 . Journal of Cleaner Production , 258 , 120783. Crossref , Google Scholar
- Tuffnell, C, P Kral, P Durana and T Krulicky ( 2019 ) Industry 4.0-based manufacturing systems: Smart production, sustainable supply chain networks, and real-time process monitoring . Journal of Self-Governance and Management Economics , 7 (2), 7–12. Crossref , Google Scholar
- Tung, K ( 2019 ) AI, the internet of legal things, and lawyers . Journal of Management Analytics , 6 (4), 390–403. Crossref , Google Scholar
- Uslu, BÇ and SÜ Fırat ( 2019 ) A comprehensive study on internet of things based on key artificial intelligence technologies and industry 4.0 . In Advanced Metaheuristic Methods in Big Data Retrieval and Analytics , pp. 1–26. IGI Global. Crossref , Google Scholar
- van Geest, M, B Tekinerdogan and C Catal ( 2021 ) Design of a reference architecture for developing smart warehouses in industry 4.0 . Computers in Industry , 124 , 103343. Crossref , Google Scholar
- Villalba-Diez, J, X Zheng, D Schmidt and M Molina ( 2019 ) Characterization of industry 4.0 lean management problem-solving behavioral patterns using EEG sensors and deep learning . Sensors , 19 (13), 2841. Crossref , Google Scholar
- Wan, J, J Yang, Z Wang and Q Hua ( 2018 ) Artificial intelligence for cloud-assisted smart factory . IEEE Access , 6 , 55419–55430. Crossref , Google Scholar
- Xu, L ( 1999 ) Preface . Expert Systems with Applications , 16 (1), 1–2. http://doi.org/10.1016/S0957-4174(98)00033-5 . Crossref , Google Scholar
- Xu, L ( 2021 ) Special issue on system research on artificial intelligence . Systems Research and Behavioral Science . https://doi-org.proxy.lib.odu.edu/10.1002/sres.2776 . Crossref , Google Scholar
- Yan, J, Y Meng, L Lu and L Li ( 2017 ) Industrial big data in an industry 4.0 environment: Challenges, schemes, and applications for predictive maintenance . IEEE Access , 5 , 23484–23491. Crossref , Google Scholar
- Yao, X, J Zhou, J Zhang and CR Boër ( 2017 ) From intelligent manufacturing to smart manufacturing for industry 4.0 driven by next generation artificial intelligence and further on . In 2017 5th Int. Conf. Enterprise Systems (ES) , pp. 311–318. IEEE. Crossref , Google Scholar
- You, Z and L Feng ( 2020 ) Integration of industry 4.0 related technologies in construction industry: A framework of cyber-physical system . IEEE Access , 8 , 122908–122922. Crossref , Google Scholar
- Zhang, C and Y Lu ( 2021 ) Study on artificial intelligence: The state of the art and future prospects . Journal of Industrial Information Integration , 23 , 100224. Crossref , Google Scholar
- Zheng, P, Z Sang, RY Zhong, Y Liu, C Liu, K Mubarok, S Yu and X Xu ( 2018 ) Smart manufacturing systems for industry 4.0: Conceptual framework, scenarios, and future perspectives . Frontiers of Mechanical Engineering , 13 (2), 137–150. Crossref , Google Scholar
- Zheng, T, M Ardolino, A Bacchetti and M Perona ( 2021 ) The applications of industry 4.0 technologies in manufacturing context: A systematic literature review . International Journal of Production Research , 1–33. Crossref , Google Scholar
- Zhou, K, T Liu and L Zhou ( 2015 ) Industry 4.0: Towards future industrial opportunities and challenges . In 2015 12th Int. Conf. Fuzzy Systems and Knowledge Discovery (FSKD) , pp. 2147–2152. IEEE. Crossref , Google Scholar
- Internet of Intelligent Things: A convergence of embedded systems, edge computing and machine learning Franklin Oliveira, Daniel G. Costa, Flávio Assis and Ivanovitch Silva 1 Jul 2024 | Internet of Things, Vol. 26
- IoT Application in Garment Manufacturing Rupayan Roy and Manoj Tiwari 29 Mar 2024
- C. Ganesh Kumar ,
- R. Jothi Basu ,
- M. Yuvaraj , and
- Arokiaraj David
- Identifying the Applications of Artificial Intelligence in Online Marketing Rasoul Abbasi and Mohadeseh Esmaili 8 Mar 2024
- Development of Physics Learning Media : A Literature Review Intan Fachrudina Maslakhah, Budi Jatmiko and I Gusti Made Sanjaya 7 March 2024 | IJORER : International Journal of Recent Educational Research, Vol. 5, No. 2
- Virtual manufacturing in Industry 4.0: A review Mohsen Soori, Behrooz Arezoo and Roza Dastres 1 Mar 2024 | Data Science and Management, Vol. 7, No. 1
- Understanding Medical 4.0 implementation through enablers: An integrated multi-criteria decision-making approach Md Nahid Akhtar, Abid Haleem, Mohd Javaid and Mohd Vasif 1 Mar 2024 | Informatics and Health, Vol. 1, No. 1
- Harmonizing sustainability in industry 5.0 era: Transformative strategies for cleaner production and sustainable competitive advantage Rishabh Sharma and Himanshu Gupta 1 Mar 2024 | Journal of Cleaner Production, Vol. 445
- Harnessing AIoT in Transforming the Education Landscape Mukesh Chaware 23 Feb 2024
- Self-organizing maps: a novel approach to identify and map business clusters Francis Bowen and Janaína Siegler 8 February 2024 | Journal of Management Analytics, Vol. 3
- Enhancing innovativeness and performance of the manufacturing supply chain through datafication: The role of resilience Shuang Tian, Lin Wu, Maria Pia Ciano, Marco Ardolino and Kulwant S. Pawar 1 Feb 2024 | Computers & Industrial Engineering, Vol. 188
- Selection of Industry 4.0 technologies for Lean Six Sigma integration using fuzzy DEMATEL approach Arish Ibrahim and Gulshan Kumar 18 January 2024 | International Journal of Lean Six Sigma, Vol. 18
- Integration of Generative AI Techniques and Applications in Student Behavior and Cognitive Achievement in Arab Higher Education Mohammed Jaboob, Manar Hazaimeh and Abdullah M. Al-Ansi 8 January 2024 | International Journal of Human–Computer Interaction, Vol. 99
- Artificial Intelligence Management in Industry 4.0 - Challenge or Opportunity Glib Mazhara and Viktoriia Melnychuk 27 September 2023
- The Impact of Artificial Intelligence on Supply Chain Management in Modern Business Mitra Madancian, Hamed Taherdoost, Maassoumeh Javadi, Inam Ullah Khan and Alaeddin Kalantari et al. 30 January 2024
- Exploring the Acceptance and User Satisfaction of AI-Driven e-Learning Platforms (Blackboard, Moodle, Edmodo, Coursera and edX): An Integrated Technology Model Raneem Rashad Saqr, Sabah Abdullah Al-Somali and Mohammad Y. Sarhan 25 December 2023 | Sustainability, Vol. 16, No. 1
- Synergizing AI and HRM Kamaal Allil 29 Dec 2023
- Linking Discrete-Event Simulation with Artificial Intelligence: A Literature-Based Analysis of Existing Approaches in the Context of Manufacturing Planning and Control M. Kranz, V. Nitsch and S. Mütze-Niewöhner 18 Dec 2023
- Application of Industry 4.0 Technologies in Climate-Smart Agricultural Practices Soumya Sucharita Panda, Sudatta Banerjee and Swati Alok 13 Dec 2023
- Keep up with the modern joneses: an empirical analysis of the digital divide in Italy Alessandra Patti and Valeria Schifilliti 11 December 2023 | Applied Economics Letters
- Antecedents of Industry 4.0 capabilities and technological innovation: a dynamic capabilities perspective Ayman wael AL-Khatib, Ahmed Shuhaiber, Ibrahim Mashal and Manaf Al-Okaily 7 December 2023 | European Business Review, Vol. 17
- Shafi Ahmad ,
- Zahid A. Khan ,
- Mohammed Ali , and
- Mohammad Asjad
- Ibrahim Haleem Khan , and
- Sanjay Mohan
- Identifying issues in adoption of AI practices in construction supply chains: towards managing sustainability Arpit Singh, Ashish Dwivedi, Dindayal Agrawal and Durgesh Singh 13 January 2023 | Operations Management Research, Vol. 16, No. 4
- Estimation of Tool Life in the Milling Process—Testing Regression Models Andrzej Paszkiewicz, Grzegorz Piecuch, Tomasz Żabiński, Marek Bolanowski and Mateusz Salach et al. 23 November 2023 | Sensors, Vol. 23, No. 23
- Impact of Machine Learning on IT Project Management Ibrahim Jobr Alfaifi and Prof. Mehmet Sabih Aksoy 9 December 2023 | Journal of Image Processing and Intelligent Remote Sensing, No. 41
- Smart Agriculture Resource Allocation and Cost Optimization Using ML in Cloud Computing Environment Pancham Singh, Mrignainy Kansal, Mili Srivastava and Muskan Gupta 24 Nov 2023
- Integration of Artificial Intelligence and Machine Learning for Internet of Things Kamal Kumar, Vinod Kumar and Seema 15 Nov 2023
- Artificial Intelligence Approaches for Studying the pp Interactions at High Energy Using Adaptive Neuro-Fuzzy Interface System Doaa Mahmoud Habashy, Mahmoud Yaseen El-Bakry, El-Sayed Ahmed El-Dahshan and Hanem Ibrahim Lebda 15 November 2023
- Explainable Artificial Intelligence for Cybersecurity P. Sharon Femi, K. Ashwini, A. Kala and V. Rajalakshmi 27 October 2023
- A study on ChatGPT for Industry 4.0: Background, potentials, challenges, and eventualities Mohd Javaid, Abid Haleem and Ravi Pratap Singh 1 Nov 2023 | Journal of Economy and Technology, Vol. 1
- AI-powered blockchain technology in industry 4.0, a review Mohsen Soori, Roza Dastres and Behrooz Arezoo 1 Nov 2023 | Journal of Economy and Technology, Vol. 1
- Drivers of generative artificial intelligence to fostering exploitative and exploratory innovation: A TOE framework Ayman wael AL-khatib 1 Nov 2023 | Technology in Society, Vol. 75
- AI Toolbox Concept for the Arrowhead Framework Dániel Ficzere, Gergely Hollósi, Attila Frankó and Pál Varga 30 Oct 2023
- Roadmap to achieving sustainable development: Does digital economy matter in industrial green transformation? Xiaodong Yang, Yang Xu, Asif Razzaq, Desheng Wu and Jianhong Cao et al. 27 October 2023 | Sustainable Development, Vol. 200
- USING ARTIFICIAL INTELLIGENCE IN EDUCATION 4.0 József UDVAROS and Norbert FORMAN 20 Oct 2023 | Journal of Technology and Information, Vol. 15, No. 1
- Adoption of industry 4.0 in different sectors: a structural review using natural language processing Shamneesh Sharma, Arun Malik, Chetan Sharma, Isha Batra and Mahender Singh Kaswan et al. 7 October 2023 | International Journal on Interactive Design and Manufacturing (IJIDeM), Vol. 56
- Chili Quality Classification using Convolution Neural Network William Adam, Muhammad Niswar and Zainal 4 Oct 2023
- Artificial intelligence applications in finance: a survey Xuemei Li, Alexander Sigov, Leonid Ratkin, Leonid A. Ivanov and Ling Li 10 August 2023 | Journal of Management Analytics, Vol. 10, No. 4
- Artificial intelligence-based solutions for climate change: a review Lin Chen, Zhonghao Chen, Yubing Zhang, Yunfei Liu and Ahmed I. Osman et al. 13 June 2023 | Environmental Chemistry Letters, Vol. 21, No. 5
- Diverse Applications of Three-Dimensional Printing in Biomedical Engineering: A Review Prachi Agarwal, Gargi Arora, Amit Panwar, Vidhi Mathur and Varadharajan Srinivasan et al. 1 Oct 2023 | 3D Printing and Additive Manufacturing, Vol. 10, No. 5
- Enhancing Counter Drone Operations Through Human-AI Collaboration: A Hierarchical Decision-Making Framework Varun Mehta, Kesav Kaza, Fardad Dadboud, Miodrag Bolic and Iraj Mantegh 1 Oct 2023
- Identifying Different Operational Regimes of an Electric Arc Furnace Using Machine Learning Based Alternative Clustering Approaches Venkata Chaitanya Kanakamedala, Allen Chacko Johny, Arya K. Bhattacharya and Kumar Chatterjee 21 Sep 2023
- Black Box Model Analysis for Industrial Fault Monitoring Sergio Galve, Xavier Vilajosana and Vicenç Puig 12 Sep 2023
- PesViT: a deep learning approach for detecting misuse of pesticides on farm Le Quang Thao, Nguyen Duy Thien, Ngo Chi Bach, Duong Duc Cuong and Le Duc Anh et al. 21 April 2023 | The Journal of Supercomputing, Vol. 79, No. 14
- Adopting Artificial Intelligence in Industry 4.0: Understanding the Drivers, Barriers and Technology Trends Fateme Dinmohammadi 30 Aug 2023
- Circular economy e-business model portfolio development for e-business applications: Impacts on ESG and sustainability performance Yun Arifatul Fatimah, Devika Kannan, Kannan Govindan and Zainal Arifin Hasibuan 1 Aug 2023 | Journal of Cleaner Production, Vol. 415
- Transforming farming with intelligence: Smart vibration monitoring and alert system Amandeep Singh, Naser Nawayseh, Yash Kumar Dhabi, Siby Samuel and Harwinder Singh 1 Aug 2023 | Journal of Engineering Research, Vol. 2
- Movable factory—A systematic literature review of concepts, requirements, applications, and gaps Zahra Kazemi, Jonas Kjaer Rask, Cláudio Gomes, Emre Yildiz and Peter Gorm Larsen 1 Aug 2023 | Journal of Manufacturing Systems, Vol. 69
- Multitasking FBG sensors for condition monitoring with a wideband (DC–MHz) interrogation system Ziv Glasser, Egor Liokumovitch, Stas Bank, Nadav Simor and Alexander Kalnitsky et al. 24 July 2023 | Applied Optics, Vol. 62, No. 22
- Cold supply chain inventory models for agricultural products with multi-stage stochastic deterioration rates Raosaheb Latpate, Maruti Bhosale and Sandesh Kurade 7 July 2023 | Journal of Management Analytics, Vol. 10, No. 3
- A review on effective alarm management systems for industrial process control: Barriers and opportunities Faizan E Mustafa, Ijaz Ahmed, Abdul Basit, Um-E-Habiba Alvi and Saddam Hussain Malik et al. 1 Jul 2023 | International Journal of Critical Infrastructure Protection, Vol. 41
- Neuro-Symbolic AI for Compliance Checking of Electrical Control Panels VITO BARBARA, MASSIMO GUARASCIO, NICOLA LEONE, GIUSEPPE MANCO and ALESSANDRO QUARTA et al. 10 July 2023 | Theory and Practice of Logic Programming, Vol. 23, No. 4
- Examining Customer Behavior Towards the Use of Contextual Commerce Powered by Artificial Intelligence Ree Chan Ho and Nelvin XeChung Leow 26 May 2023
- Shailendra Kumar ,
- Ajay Singh ,
- Mohammad Asjad ,
- Mohd. Suhaib ,
- Chitransh Kulshrestha , and
- Sandeep Sirohi
- Love Kumar and
- Rajiv Kumar Sharma
- Garment fit evaluation using neural networks technology Kaixuan Liu, Ruolin Wang, Xinyue Hao, Chun Zhu and Shunmuzi Zhou et al. 18 April 2023 | The Journal of The Textile Institute, Vol. 4
- Guest editorial for special issue – AI facets and industrial applications (Part 2) Sandeep Walia and Pradeep Kautish 4 April 2023 | foresight, Vol. 25, No. 2
- Understanding the potential applications of Artificial Intelligence in Agriculture Sector Mohd Javaid, Abid Haleem, Ibrahim Haleem Khan and Rajiv Suman 1 Mar 2023 | Advanced Agrochem, Vol. 2, No. 1
- Building Inclusive Smart Cities through Innovation Intermediaries Dominique Lepore, Niccolò Testi and Edna Pasher 22 February 2023 | Sustainability, Vol. 15, No. 5
- AI, ML and Other Bioinformatics Tools for Preclinical and Clinical Development of Drug Products Avinash Khadela, Sagar Popat, Jinal Ajabiya, Disha Valu and Shrinivas Savale et al. 7 February 2023
- Digitalization and artificial knowledge for accountability in SCM: a systematic literature review Assunta Di Vaio, Badar Latif, Nuwan Gunarathne, Manjul Gupta and Idiano D'Adamo 6 February 2023 | Journal of Enterprise Information Management, Vol. 8
- Performance Analysis Method for Robotic Process Automation Rosa Quille, Felipe Almeida, Joshua Borycz, Pedro Corrêa and Lucia Filgueiras et al. 17 February 2023 | Sustainability, Vol. 15, No. 4
- Artificial Intelligence and Blockchain Technology in the 4.0 IR Metaverse Era Mohammad Rashed Hasan Polas, Bulbul Ahamed and Md. Masud Rana 6 Jan 2023
- 3D with Artificial Intelligence Technology Contributes Science and Technology Education Development Hao Jiang, Xiaoli Liu, Mingxi Tang and Kaibin Xiang 25 March 2023
- Design Futures with GAI: Exploring the Potential of Generative AI Tools in Collaborative Speculation Yanru Lyu, Tingxuan Hao and Zhouhengyi Yi 26 November 2023
- Gegenwärtige Herausforderungen im Geschftsprozessmanagement Matthes Elstermann, Albert Fleischmann, Christoph Moser, Stefan Oppl and Werner Schmidt et al. 29 October 2023
- Künstliche Intelligenz: Chance oder Risiko für das Unternehmertum der Zukunft? Lars Heim and Sebastian Gerth 30 December 2023
- Artificial Intelligence: Benefits, Application, Ethical Issues, and Organizational Responses Khalda Ali, Maram Alzaidi, Dimah Al-Fraihat and Amir M. Elamir 1 January 2023
- Tackling IoT Security Challenge by Metaheuristics Tuned Extreme Learning Machine Luka Jovanovic, Masa Gajevic, Milos Dobrojevic, Nebojsa Budimirovic and Nebojsa Bacanin et al. 16 June 2023
- “Deep learning” for healthcare: Opportunities, threats, and challenges Russell Kabir, Madhini Sivasubramanian, Geeta Hitch, Saira Hakkim and John Kainesie et al. 1 Jan 2023
- Digital Twin applications toward Industry 4.0: A Review Mohd Javaid, Abid Haleem and Rajiv Suman 1 Jan 2023 | Cognitive Robotics, Vol. 3
- An integrated outlook of Cyber–Physical Systems for Industry 4.0: Topical practices, architecture, and applications Mohd Javaid, Abid Haleem, Ravi Pratap Singh and Rajiv Suman 1 Jan 2023 | Green Technologies and Sustainability, Vol. 1, No. 1
- Development of a risk assessment methodology for intelligent technology of diagnosing industrial equipment for complex oil and gas facilities Galina Samigulina and Zarina Samigulina 1 Jan 2023 | Procedia Computer Science, Vol. 225
- ES-SECS/GEM: An Efficient Security Mechanism for SECS/GEM Communications Shams Ul Arfeen Laghari, Selvakumar Manickam, Ayman Khallel Al-Ani, Mahmood A. Al-Shareeda and Shankar Karuppayah 1 Jan 2023 | IEEE Access, Vol. 11
- A Novel Methodology for Unsupervised Anomaly Detection in Industrial Electrical Systems Marco Carratù, Vincenzo Gallo, Salvatore Dello Iacono, Paolo Sommella and Alessandro Bartolini et al. 1 Jan 2023 | IEEE Transactions on Instrumentation and Measurement, Vol. 72
- The ChatGPT Application on Quality Management: A Comprehensive Review Abdulkadir Atalan 1 January 2023 | SSRN Electronic Journal, Vol. 5
- Charging Stations and Electromobility Development: A Cross-Country Comparative Analysis Tomasz Zema, Adam Sulich and Sebastian Grzesiak 21 December 2022 | Energies, Vol. 16, No. 1
- Blockchain—Internet of Things Applications: Opportunities and Challenges for Industry 4.0 and Society 5.0 Amit Kumar Tyagi, Sathian Dananjayan, Deepshikha Agarwal and Hasmath Farhana Thariq Ahmed 13 January 2023 | Sensors, Vol. 23, No. 2
- Artificial Intelligence: Trends and Challenges Av. Karthick and S. Gopalsamy 25 Nov 2022
- The Development of Intelligent Education and Teachers’ Professional Informatization Led by an Intelligent Ubiquitous Network Yuyuan Zhang, Hai Zhang and Jun Ye 7 Oct 2022 | Computational Intelligence and Neuroscience, Vol. 2022
- How Do Technologies Based on Cyber–Physical Systems Affect the Environmental Performance of Products? A Comparative Study of Manufacturers’ and Customers’ Perspectives Naiara Uriarte-Gallastegi, Beñat Landeta-Manzano, German Arana-Landín and Iker Laskurain-Iturbe 18 October 2022 | Sustainability, Vol. 14, No. 20
- Federico Walas Mateo and
- Andrés Redchuk
- Control System Development and Implementation of a CNC Laser Engraver for Environmental Use with Remote Imaging Hani Attar, Amer Tahseen Abu-Jassar, Ayman Amer, Vyacheslav Lyashenko and Vladyslav Yevsieiev et al. 10 Sep 2022 | Computational Intelligence and Neuroscience, Vol. 2022
- Anjee Gorkhali
- Achieving a Data-Driven Risk Assessment Methodology for Ethical AI Anna Felländer, Jonathan Rebane, Stefan Larsson, Mattias Wiggberg and Fredrik Heintz 18 August 2022 | Digital Society, Vol. 1, No. 2
- Application of Artificial Immune Systems in Advanced Manufacturing Rui Pinto and Gil Gonçalves 1 Sep 2022 | Array, Vol. 15
- MAP: Design, Development, Deployment, and Maintenance of Industrie 4.0 AI Applications Aldo Dagnino, Maciej Kolomycki and Alok Kucheria 1 Aug 2022
- Industry 4.0: A Chance or a Threat for Gen Z? The Perspective of Economics Students Bernard Bińczycki and Sławomir Dorocki 21 July 2022 | Sustainability, Vol. 14, No. 14
- The relationship between inbound open innovation and the innovative use of information technology by individuals in teams of start‐ups Meifang Yao, Dan Ye and Liyi Zhao 25 May 2022 | Systems Research and Behavioral Science, Vol. 39, No. 3
- AI‐enabled IT capability and organizational performance Fang Wang 23 May 2022 | Systems Research and Behavioral Science, Vol. 39, No. 3
- Disruptive technology identification of intelligent logistics robots in AIoT industry: Based on attributes and functions analysis Weifeng Jia, Shuo Wang, Yongping Xie, Zifeng Chen and Kaixin Gong 1 June 2022 | Systems Research and Behavioral Science, Vol. 39, No. 3
- Impact of value co‐creation in the artificial intelligence innovation ecosystem on competitive advantage and innovation intelligibility Yu Sun, Xiaobo Xu, Haiqing Yu and Hecheng Wang 1 June 2022 | Systems Research and Behavioral Science, Vol. 39, No. 3
- Knowledge chain integration of design structure matrix‐based project team: An integration model Shuo Tian, Hangeng Zhao, Xiaobo Xu, Rongchao Mu and Qiang Ma 3 June 2022 | Systems Research and Behavioral Science, Vol. 39, No. 3
- An evaluation framework of IT‐enabled service‐oriented manufacturing Yu Sun, Yuming He, Haiqing Yu and Hecheng Wang 30 May 2022 | Systems Research and Behavioral Science, Vol. 39, No. 3
- From ideas to entrepreneurial opportunity: A study on AI Baoshan Ge, Qi Wang and Meifang Yao 1 June 2022 | Systems Research and Behavioral Science, Vol. 39, No. 3
- Seizing the opportunity window of artificial intelligence in China: Towards an innovation policy mix framework for emerging technologies from an evolution perspective Jing Liu, Mengbo Wang, Xiaoling Kang, Xia Zhang and Xing Chen 9 June 2022 | Systems Research and Behavioral Science, Vol. 39, No. 3
- Millimeter-Wave Smart Antenna Solutions for URLLC in Industry 4.0 and Beyond Abdul Jabbar, Qammer H. Abbasi, Nadeem Anjum, Tahera Kalsoom and Naeem Ramzan et al. 31 March 2022 | Sensors, Vol. 22, No. 7
- Developing Instrument for Investigation of Blockchain Technology Dmitry Kushnir, Maxim Kovtsur, Ammar Muthanna, Anastasiia Kistruga and Mark Akilov et al. 29 March 2022
- Leveraging Design Thinking Towards the Convergence of AI, IoT and Blockchain: Strategic Drivers and Human-Centered Use Cases Maximilian Tigges, Chloé Ipert and René Mauer 5 October 2022
- Artificial intelligence (AI) applications for marketing: A literature-based study Abid Haleem, Mohd Javaid, Mohd Asim Qadri, Ravi Pratap Singh and Rajiv Suman 1 Jan 2022 | International Journal of Intelligent Networks, Vol. 3
- Enhancing smart farming through the applications of Agriculture 4.0 technologies Mohd Javaid, Abid Haleem, Ravi Pratap Singh and Rajiv Suman 1 Jan 2022 | International Journal of Intelligent Networks, Vol. 3
- ICTs into mathematical instructions for meaningful teaching and learning Mukesh Maharjan, Niroj Dahal and Binod Prasad Pant 1 January 2022 | Advances in Mobile Learning Educational Research, Vol. 2, No. 2
Recommended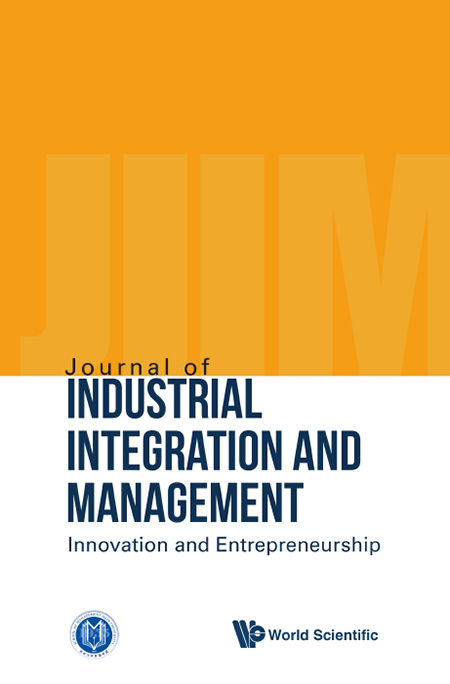 Received 7 June 2021 Revised 17 June 2021 Accepted 3 July 2021 Published: 21 October 2021 InformationInitiativesYou are accessing a machine-readable page. In order to be human-readable, please install an RSS reader. All articles published by MDPI are made immediately available worldwide under an open access license. No special permission is required to reuse all or part of the article published by MDPI, including figures and tables. For articles published under an open access Creative Common CC BY license, any part of the article may be reused without permission provided that the original article is clearly cited. For more information, please refer to https://www.mdpi.com/openaccess . Feature papers represent the most advanced research with significant potential for high impact in the field. A Feature Paper should be a substantial original Article that involves several techniques or approaches, provides an outlook for future research directions and describes possible research applications. Feature papers are submitted upon individual invitation or recommendation by the scientific editors and must receive positive feedback from the reviewers. Editor’s Choice articles are based on recommendations by the scientific editors of MDPI journals from around the world. Editors select a small number of articles recently published in the journal that they believe will be particularly interesting to readers, or important in the respective research area. The aim is to provide a snapshot of some of the most exciting work published in the various research areas of the journal. Original Submission Date Received: . - Active Journals
- Find a Journal
- Proceedings Series
- For Authors
- For Reviewers
- For Editors
- For Librarians
- For Publishers
- For Societies
- For Conference Organizers
- Open Access Policy
- Institutional Open Access Program
- Special Issues Guidelines
- Editorial Process
- Research and Publication Ethics
- Article Processing Charges
- Testimonials
- Preprints.org
- SciProfiles
- Encyclopedia
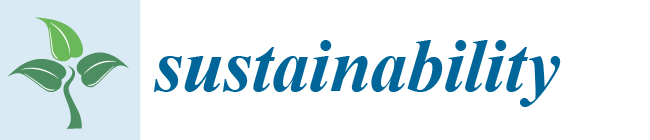 Article Menu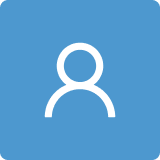 - Subscribe SciFeed
- Recommended Articles
- Google Scholar
- on Google Scholar
- Table of Contents
Find support for a specific problem in the support section of our website. Please let us know what you think of our products and services. Visit our dedicated information section to learn more about MDPI. JSmol ViewerThe role of bim in integrating digital twin in building construction: a literature review.  1. Introduction2. background, 2.1. concept of bim, 2.2. concept of digital twin, 2.3. advancement of bim to digital twin, 3. methodology, 4. literature review, 4.1. discussion on available research on digital twin with bim. - Integration of BIM and DT: Douglas et al. [ 35 ] focused on using real time data from sensors and other sources to enhance the DT, as well as using data analytics and machine learning algorithms to analyze these data and make predictions about building performance;
- Real time data analysis: Opoku et al. [ 27 ] and Deng et al. [ 11 ] focused on using real time data from sensors and other sources to enhance the DT, as well as using data analytics and machine learning algorithms to analyze these data and make predictions about building performance;
- Simulation and visualization: there has been research on using simulation and visualization technologies to enhance the DT and improve decision-making in the construction and engineering industries [ 21 , 27 ];
- Cost and resource optimization: DT and BIM potentially reduce costs, improve resource allocation, and increase overall efficiency in the building construction process [ 33 , 40 ];
- BIM/DT in the context of sustainability: the integration of BIM and DT support sustainable design and construction practices by incorporating data on energy efficiency [ 21 ], material usage [ 26 ], and environmental impact [ 18 ]; it integrates real-time data from sensors and IoT devices [ 21 ], enabling continuous monitoring [ 5 ], analysis, and proactive maintenance [ 34 ] for sustainable practices.
4.2. Evolution of Digital Twin from BIM4.3. current study to compare digital twin with bim. - Concept Origin: technology’s origin is its history, goals, and principles. Understanding the concept helps researchers evaluate their strengths, weaknesses, and applications. The concept’s origin can also indicate which technological parts are more developed or need more research.
- Purpose: to define each technology’s scope and goals. This criterion helps determine their complementary roles and the best integration strategies to improve building design, construction, and operation.
- Application focus: It highlights each technology’s primary focus. It also shows each technology’s pros and cons to guide future improvements. It is crucial to choose the right technology for a project or application.
- Features: They are an essential aspect of the scientific comparison between BIM and DT, as they help understand each technology’s capabilities and limitations and their potential for integration and interoperability.
- Level of Details: We can assess the pros and cons of integrating these technologies into building projects.
- Scalability: allows for evaluating their ability to handle different types of projects and their potential limitations regarding resource requirements and integration with other technologies.
- Main Users: Identify each technology’s primary users and how it meets their needs. This information can help stakeholders choose technology based on project needs and team expertise.
- Interoperability: enables these technologies to be integrated with other systems and software, leading to greater efficiencies and improved outcomes in the building lifecycle management process.
- Application interface: evaluates the usability and effectiveness of the software for different users and applications.
- Building life cycle stage: compares BIM and DT in building construction, as it can help determine which technology is more suitable for a given project.
4.3.1. Concept Origin4.3.2. purposes, 4.3.3. application focus, 4.3.4. features, 4.3.5. level of details (lod), 4.3.6. scalability, 4.3.7. main users, 4.3.8. interoperability, 4.3.9. application interface, 4.3.10. characteristics, 4.4. advancement of bim to improve digital twin in building construction. - Increased interoperability: BIM technology has become more interoperable, allowing seamless data exchange between platforms and systems [ 7 ]. It makes creating and updating DT easier with real time data from sensors and other sources.
- Improved data accuracy: BIM technology can offer precise and comprehensive insights into a building’s blueprint, building process, and maintenance, all of which can contribute to developing a more precise DT [ 12 ].
- Increased collaboration: BIM enables collaboration among architects, engineers, and construction professionals, leading to better decision-making and improved overall outcomes [ 25 ]. When this collaboration is applied to creating a DT, it can result in a more comprehensive and effective virtual representation of the building.
- Better visualization: BIM technology has advanced to include more realistic and interactive visualizations [ 40 ], making it easier to understand and analyze the building’s performance through the DT [ 11 ].
- More advanced simulation: BIM has also advanced to include more advanced simulation capabilities, allowing for the simulation of complex systems and analyzing building performance in real time [ 40 ].
5. Result and Discussion5.1. result and discussion, 5.2. limitation, 5.3. future study, 6. conclusions, author contributions, institutional review board statement, informed consent statement, data availability statement, conflicts of interest. # | Titles | Authors/ Years | Citation # | Journals/ Conferences | Research Methodologies | Key Findings |
---|
1 | Digital Twin: Vision, Benefits, Boundaries, and Creation for Buildings | Khajavi et al. (2019) | [ ] | IEEE | Experimentation: Testing—Sensor network used to create DT of a building. | Proposing a framework to enable a DT of a building facade. | 2 | Towards a semantic Construction Digital Twin: Directions for future research | Boje et al. (2020) | [ ] | Automation in Construction | Literature Review: The research approach is divided into three steps: reviewing BIM, analyzing DT uses, and identifying research gaps. | BIM can be used to create a construction DT concept, allowing for more efficient construction. | 3 | Characterizing the Digital Twin: A systematic literature review | Jones et al. (2020) | [ ] | CIRP-JMST | Literature Review: This paper provided a characterization of the DT, identified gaps in knowledge, and identified areas for future research. | Identifying 13 characteristics of the DT and its process of operation, as well as 7 knowledge gaps and topics for future research focus. | 4 | Construction with digital twin information systems | Sacks et al. (2020) | [ ] | Data-Centric Engineering | Conceptual analysis: Analyzes construction project management processes, digital tools, and workflow frameworks. | Four core information and control concepts for DT construction, focusing on concentric control workflow cycles and prioritizing closure. | 5 | Differentiating Digital Twin from Digital Shadow: Elucidating a Paradigm Shift to Expedite a Smart, Sustainable Built Environment | Sepasgozar (2021) | [ ] | MDPI | Literature Review: This section analyzes DT scientific research quantitatively, using scientometric analysis to identify trends, challenges, and publications in various fields. | DT applications are recommended for real-time decision-making, self-operation, and remote supervision in smart cities, engineering and construction sectors post-COVID-19. | 6 | Digital Twin in construction: An Empirical Analysis | El Jazzar et al. (2020) | [ ] | Conference Paper | Literature Review DT practice in construction: Categorizes integration into Digital Model, Digital Shadow, and DT. | Developing the framework for understanding DT implementation in the construction industry. | 7 | Digital Twins in Built Environments: An Investigation of the Characteristics, Applications, and Challenges | Shahzad et al. (2022) | [ ] | MDPI | Literature Review: Semi-structured interviews with ten industry experts. | Exploring the relationship between DTs, technologies, and implementation challenges. | 8 | SPHERE: BIM Digital Twin Platform | Alonso et al. (2019) | [ ] | MDPI | Literature Review: Collaborative practices are facilitated using the IDDS framework and PAAS platform for data integration and processing. | SPHERE platform improves building energy performance, reduces costs, and enhances the indoor environment. | 9 | From BIM to Digital Twins: A Systematic Review of the Evolution of Intelligent Building Representations in the AEC-FM industry | Deng et al. (2021) | [ ] | IT Con | Literature Review: Review of emerging technologies for BIM and DTs. | Developing a five-level ladder categorization system for reviewing studies on DT applications, focusing on the building life cycle, research domains, and technologies. | 10 | Digital twin application in the construction industry: A literature review | Opoku et al. (2021) | [ ] | Building Engineering | Systematic Review: The study analyzes DT concepts, technologies, and applications in construction using systematic review methodology and the science mapping method. | Highlighting six DT applications in construction, highlighting their development in various lifecycle phases but focusing on design and engineering over demolition and recovery. | 11 | From BIM towards Digital Twin: Strategy and Future Development for Smart Asset Management | Lu et al. (2020) | [ ] | CSIC | Literature Review: The study reviews latest research and industry standards impacting BIM and asset management. | Proposing a framework for smart asset management using DT technology and promoting smart DT-enabled asset management adoption. | 12 | Digital Twins for Construction Sites: Concepts, LoD Definition, and Applications | Zhang et al. (2022) | [ ] | ASCE | Questionnaires and interviews are used to propose a framework that enhances construction site monitoring, management, quality, efficiency, and safety. | Proposing a framework for utilizing DTs to extend BIM, IoT, data storage, integration, analytics, and physical environment interaction in construction site management. | 13 | A Proposed Framework for Construction 4.0 Based on a Review of Literature | Sawhney et al. (2020) | [ ] | ASC | Literature Review: The study reviews Industry 4.0’s impact on the construction sector, defining the framework, benefits, and barriers. | Revealing BIM and CDE are crucial for Construction 4.0 implementation, transforming the industry into efficient, quality-centered, and safe. | 14 | A Review of Digital Twin Applications in Construction | Madubuike et al. (2022) | [ ] | IT Con | Systematic Review: The study reviews literature, analyzes existing and emerging applications, and identifies limitations. | Evaluating DT technology’s benefits in construction, comparing applications, and identifying limitations. | 15 | Application of Digital Twin Technologies in Construction: An Overview of Opportunities and Challenges | Feng et al. (2021) | [ ] | ISARC | Literature Review: 23 recent publications were reviewed for DT development in construction. | DT technologies in the AEC industry face challenges in data integration, security, and funding, requiring skilled professionals and advanced technologies. | 16 | Design and Construction Integration Technology Based on Digital Twin | Zhou et al. (2021) | [ ] | PSGEC | Literature Review: Review recent papers on the application of DT in substation design and construction integration. | Improving performance, reducing construction difficulties, and simplifying maintenance by addressing low digitization intelligence issues. | 17 | Digital Twin-Driven Intelligent Construction: Features and Trends | Zhang et al. (2021) | [ ] | Tech. Science Press | Literature Review: The study reviews DT-driven IC usage, focusing on information perception, data mining, state assessment, and intelligent optimization. | Sustainable IC and DT enhance construction industry efficiency, real-time structure monitoring, and safety prediction, with four aspects proposed for digital dual-drive sustainable intelligent construction. | 18 | Towards Next Generation Cyber-Physical Systems and Digital Twins for Construction | Akanmu et al. (2021) | [ ] | IT Con | Literature Review: The paper reviews evolution, applications, limitations, next generation CPS/DTs, enabling technologies, and conclusions in construction. | Exploring opportunities for CPS and DT in construction, promoting increased deployment and workforce productivity. | 19 | Virtually Intelligent Product Systems: Digital and Physical Twins | Grieves (2019) | [ ] | Astronautics Aeronautics | Literature Review: Paper explores interconnected Physical Twin, product lifecycle, and DT concepts. | DT concept requires value-driven use cases, with new ones emerging as technology advances. | 20 | Digital twins from design to handover of constructed assets | Seaton et al. (2022) | [ ] | World Built Environment Forum | Literature Review; Case Studies; Interviews: The paper examines DTs’ dimensions, application, asset life cycle, and use cases from the perspective of professionals in the built environment sector. | DTs in the built environment require accurate definition, efficient data management, and high BIM adoption for success. | 21 | Digital Twin for Accelerating Sustainability in Positive Energy District: A Review of Simulation Tools and Applications | Zhang et al. (2021) | [ ] | Frontiers in Sustainable Cities | Literature Review: Review of DT for PEDs, discussing concepts, principles, tools, and applications. | Digital PED twin consists of virtual models, sensor network integration, data analytics, and a stakeholder layer, with limited tools for full functionality. | 22 | A Review of the Digital Twin Technology in the AEC-FM Industry | Hosamo et al. (2022) | [ ] | Hindawi Civil Engineering | Literature Review: 77 academic publications clustered around DT applications in the AEC-FM industry. | DT implementation in the AEC-FM industry requires information standardization and a conceptual framework. | 23 | BIM, Digital Twin and Cyber Physical Systems: Crossing and Blurring Boundaries | Douglas et al. (2021) | [ ] | Computing in Construction | Systematic Review: The paper reviews DT BIM and CPS concepts, promoting discussion in construction. | Identifying three distinct DT and BIM understandings, requiring further investigation. | 24 | Climate Emergency—Managing, Building, and Delivering the Sustainable Development Goals | Gorse et al. (2020) | [ ] | SEEDS | Literature Review; Interview; Case Studies: Data collection, communication, and rapid response processes. | Proposing the growth of DT as benefits realized over time and an approach to DT for BIM-enabled asset management. | 25 | Developing BIM-Based Linked Data Digital Twin Architecture to Address a Key Missing Factor: Occupants | Sobhkhiz and El-Diraby (2022) | [ ] | ASCE | Case Study: Extended the DT architecture for addressing issues. | Proposing architecture for designing DTs using semantic web technologies, linked data approaches, machine learning, and BIM integration. | 26 | Digital Twin in the Architecture, Engineering, and Construction Industry: A Bibliometric Review | Almatared et al. (2022) | [ ] | ASCE | Literature Review: Research synthesizes DT in the AEC industry using bibliometric analysis, identifying trends, challenges, and knowledge gaps. | Exposing quantitative research trends and needs for DT in the AEC industry. Future research should focus on data interoperability, AIoT, and AI. | 27 | Digital Twins: Details Of Implementation | Quirk et al. (2020) | [ ] | ASHRAE | Literature Review: This article discusses implementing a DT, validating results, and real-time calibration. | DTs enable ongoing monitoring of data center environments, enabling rapid decision-making and energy efficiency optimization, reducing surprises, and enhancing business efficiency. | 28 | Industry 4.0 for the Built Environment: The Role of Digital Twins and Their Application for the Built Environment | Bolpagni et al. (2021) | [ ] | Structural Integrity 20 | Case Study: Literature Review of DT vision, utilization, BIM specifications, and energy efficiency management in facility management. | Discussing DT concept, human–building interaction, post-construction use cases, property management, field data, and practical solutions. | 29 | The Development of a BIM-Based Interoperable Toolkit for Efficient Renovation in Buildings: From BIM to Digital Twin | Daniotti et al. (2022) | [ ] | MDPI | Literature Review: A European project validates the BIM4EEB renovation toolset using KPIs in real-world cases. | Developing the Horizon2020 Project’s BIM-based toolkit development, real-world validation, and benefits enhance the building renovation process. | 30 | Internet of Things (IoT), Building Information Modeling (BIM), and Digital Twin (DT) in Construction Industry: A Review, Bibliometric, and Network Analysis | Baghalzadeh et al. (2022) | [ ] | MDPI | Literature Review: Reviews 1879 studies in Web of Science database network on visualization, research interactions, and influential authors. | Revealing prolific authors, prominent journals, nations, popular topics, and future trends. | - Zhang, J.; Cheng, J.C.P.; Chen, W.; Chen, K. Digital Twins for Construction Sites: Concepts, LoD Definition, and Applications. J. Manag. Eng. 2022 , 38 , 04021094. [ Google Scholar ] [ CrossRef ]
- Lu, Q.; Xie, X.; Heaton, J.; Parlikad, A.K.; Schooling, J. From BIM towards Digital Twin: Strategy and Future Development for Smart Asset Management. In Service Oriented, Holonic and Multi-Agent Manufacturing Systems for Industry of the Future ; Borangiu, T., Trentesaux, D., Leitão, P., Giret Boggino, A., Botti, V., Eds.; Springer International Publishing: Cham, Switzerland, 2020; pp. 392–404. [ Google Scholar ]
- Grieves, M. Digital Twin: Manufacturing Excellence through Virtual Factory Replication ; Michael W. Grieves, LLC: Cocoa Beach, FL, USA, 2015. [ Google Scholar ]
- O’Gorman, T. How Digital Twins Optimize the Performance of Your Assets in a Sustainable Way. IBM Blog . 2023. Available online: https://www.ibm.com/blog/how-digital-twins-optimize-the-performance-of-your-assets-in-a-sustainable-way (accessed on 22 May 2023).
- Sacks, R.; Brilakis, I.; Pikas, E.; Xie, H.S.; Girolami, M. Construction with Digital Twin Information Systems. Data Cent. Eng. 2020 , 1 , e14. [ Google Scholar ] [ CrossRef ]
- Autodesk Autodesk. Available online: https://www.autodesk.com/solutions/digital-twin/architecture-engineering-construction (accessed on 15 February 2022).
- Boje, C.; Guerriero, A.; Kubicki, S.; Rezgui, Y. Towards a Semantic Construction Digital Twin: Directions for Future Research. Autom. Constr. 2020 , 114 , 103179. [ Google Scholar ] [ CrossRef ]
- Chen, Y.; Kamara, J.M. A Framework for Using Mobile Computing for Information Management on Construction Sites. Autom. Constr. 2011 , 20 , 776–788. [ Google Scholar ] [ CrossRef ]
- Fischer, M.; Ashcraft, H.W.; Reed, D.; Khanzode, A. Atul Khanzode Integrating Project Delivery ; Wiley: Hoboken, NJ, USA, 2017; ISBN 978-0470587355. Available online: https://www.amazon.com/Integrating-Project-Delivery-Martin-Fischer/dp/0470587350 (accessed on 29 October 2022).
- Eastman, C.M.; Eastman, C.; Teicholz, P.; Sacks, R.; Liston, K. BIM Handbook: A Guide to Building Information Modeling for Owners, Managers, Designers, Engineers and Contractors ; John Wiley & Sons: Hoboken, NJ, USA, 2011; ISBN 978-0-470-54137-1. [ Google Scholar ]
- Deng, M.; Menassa, C.C.; Kamat, V.R. From BIM to Digital Twins: A Systematic Review of the Evolution of Intelligent Building Representations in the AEC-FM Industry. ITcon 2021 , 26 , 58–83. [ Google Scholar ] [ CrossRef ]
- Akanmu, A.A.; Anumba, C.J.; Ogunseiju, O.O. Towards next Generation Cyber-Physical Systems and Digital Twins for Construction. ITcon 2021 , 26 , 505–525. [ Google Scholar ] [ CrossRef ]
- Building Digital Twin Association Publications: Antwerp, Belgium, 2019. White Paper, Q4 2019. p. 9. Available online: https://buildingdigitaltwin.org/wp-content/uploads/2022/02/WhitePaper1-en.pdf (accessed on 22 May 2023).
- Adhikari, S.; Collins, J.; Loreto, G.; Nguyen, T.D. The Use of Parametric Modeling to Enhance the Understanding of Concrete Formwork Structures. In Proceedings of the 2021 ASEE Virtual Annual Conference Content Access, Virtual, 26–29 July 2021. [ Google Scholar ]
- Grieves, M.W. Product Lifecycle Management: The New Paradigm for Enterprises. Int. J. Prod. Dev. 2005 , 2 , 71–84. [ Google Scholar ] [ CrossRef ]
- Grieves, M. Product Lifecycle Management: Driving the next Generation of Lean Thinking ; McGraw-Hill: New York, NY, USA, 2006; ISBN 978-0-07-145230-4. [ Google Scholar ]
- Grieves, M. Virtually Perfect: Driving Innovative and Lean Products through Product Lifecycle Management ; Space Coast Press: Cocoa Beach, FL, USA, 2011; ISBN 978-0-9821380-0-7. [ Google Scholar ]
- Seaton, H.; Savian, C.; Sepasgozar, S.; Sawhney, A. Digital Twins from Design to Handover of Constructed Assets ; Royal Institution of Chartered Surveyors: London, UK, 2022. [ Google Scholar ]
- Hardin: BIM and Construction Management: Proven Tools. Available online: https://scholar.google.com/scholar_lookup?title=BIM%20and%20Construction%20Management%3A%20Proven%20Tools%2C%20Methods&author=B.%20Hardin&publication_year=2009 (accessed on 10 May 2023).
- Zhou, L.; An, C.; Shi, J.; Lv, Z.; Liang, H. Design and Construction Integration Technology Based on Digital Twin. In Proceedings of the 2021 Power System and Green Energy Conference (PSGEC), Shanghai, China, 20–22 August 2021; pp. 7–11. [ Google Scholar ]
- Khajavi, S.H.; Motlagh, N.H.; Jaribion, A.; Werner, L.C.; Holmström, J. Digital Twin: Vision, Benefits, Boundaries, and Creation for Buildings. IEEE Access 2019 , 7 , 147406–147419. [ Google Scholar ] [ CrossRef ]
- Jones, D.; Snider, C.; Nassehi, A.; Yon, J.; Hicks, B. Characterising the Digital Twin: A Systematic Literature Review. CIRP J. Manuf. Sci. Technol. 2020 , 29 , 36–52. [ Google Scholar ] [ CrossRef ]
- Sepasgozar, S.M.E. Differentiating Digital Twin from Digital Shadow: Elucidating a Paradigm Shift to Expedite a Smart, Sustainable Built Environment. Buildings 2021 , 11 , 151. [ Google Scholar ] [ CrossRef ]
- El Jazzar, M.; Piskernik, M.; Nassereddine, H. Digital Twin in Construction: An Empirical Analysis. In Proceedings of the EG-ICE 2020 Workshop on Intelligent Computing in Engineering, Proceedings, Online, 1–4 July 2020. [ Google Scholar ]
- Shahzad, M.; Shafiq, M.T.; Douglas, D.; Kassem, M. Digital Twins in Built Environments: An Investigation of the Characteristics, Applications, and Challenges. Buildings 2022 , 12 , 120. [ Google Scholar ] [ CrossRef ]
- Alonso, R.; Borras, M.; Koppelaar, R.H.E.M.; Lodigiani, A.; Loscos, E.; Yöntem, E. SPHERE: BIM Digital Twin Platform. Proceedings 2019 , 20 , 9. [ Google Scholar ] [ CrossRef ]
- Opoku, D.-G.J.; Perera, S.; Osei-Kyei, R.; Rashidi, M. Digital Twin Application in the Construction Industry: A Literature Review. J. Build. Eng. 2021 , 40 , 102726. [ Google Scholar ] [ CrossRef ]
- Sawhney, A.; Riley, M.; Irizarry, J.; Pérez, C.T. A Proposed Framework for Construction 4.0 Based on a Review of Literature. In EPiC Series in Built Environment ; EasyChair: Liverpool, UK, 2020; Volume 1, pp. 301–309. [ Google Scholar ]
- Madubuike, O.C.; Anumba, C.J.; Khallaf, R. A Review of Digital Twin Applications in Construction. ITcon 2022 , 27 , 145–172. [ Google Scholar ] [ CrossRef ]
- Feng, H.; Chen, Q.; García de Soto, B. Application of Digital Twin Technologies in Construction: An Overview of Opportunities and Challenges. In Proceedings of the 38th International Symposium on Automation and Robotics in Construction (ISARC 2021), Dubai, United Arab Emirates, 4 August 2021. [ Google Scholar ]
- Zhang, H.; Zhou, Y.; Zhu, H.; Sumarac, D.; Cao, M. Digital Twin-Driven Intelligent Construction: Features and Trends. Struct. Durab. Health Monit. 2021 , 15 , 183–206. [ Google Scholar ] [ CrossRef ]
- Grieves, M.W. Virtually Intelligent Product Systems: Digital and Physical Twins. In Complex Systems Engineering: Theory and Practice ; Flumerfelt, S., Schwartz, K.G., Mavris, D., Briceno, S., Eds.; American Institute of Aeronautics and Astronautics, Inc.: Reston, VA, USA, 2019; pp. 175–200. ISBN 978-1-62410-564-7. [ Google Scholar ]
- Zhang, X.; Shen, J.; Saini, P.K.; Lovati, M.; Han, M.; Huang, P.; Huang, Z. Digital Twin for Accelerating Sustainability in Positive Energy District: A Review of Simulation Tools and Applications. Front. Sustain. Cities 2021 , 3 , 663269. [ Google Scholar ] [ CrossRef ]
- Hosamo, H.H.; Imran, A.; Cardenas-Cartagena, J.; Svennevig, P.R.; Svidt, K.; Nielsen, H.K. A Review of the Digital Twin Technology in the AEC-FM Industry. Adv. Civ. Eng. 2022 , 2022 , e2185170. [ Google Scholar ] [ CrossRef ]
- Douglas, D.; Kelly, G.; Kassem, K. BIM, Digital Twin and Cyber-Physical Systems: Crossing and Blurring Boundaries. In Proceedings of the 2021 European Conference on Computing in Construction, Rhodes, Greece, 26 July 2021; pp. 204–211. [ Google Scholar ]
- Gorse, C.; Booth, C.; Scott, L.; Dastbaz, M. Climate Emergency—Managing, Building, and Delivering the Sustainable Development Goals: Selected Proceedings from the International Conference of Sustainable Ecological Engineering Design for Society (SEEDS) 2020 ; Springer International Publishing: Berlin/Heidelberg, Germany, 2021; ISBN 978-3-030-79450-7. [ Google Scholar ]
- Sobhkhiz, S.; El-Diraby, T. Developing BIM-Based Linked Data Digital Twin Architecture to Address a Key Missing Factor: Occupants ; ASCE Library: Reston, VA, USA, 2022; pp. 11–20. [ Google Scholar ] [ CrossRef ]
- Almatared, M.; Liu, H.; Tang, S.; Sulaiman, M.; Lei, Z.; Li, H.X. Digital Twin in the Architecture, Engineering, and Construction Industry: A Bibliometric Review ; ASCE Library: Reston, VA, USA, 2022; p. 678. [ Google Scholar ]
- Quirk, D.; Lanni, J.; Chauhan, N. Digital Twins: Details Of Implementation. ASHRAE J. 2020 , 62 , 20–24. [ Google Scholar ]
- Bolpagni, M.; Gavina, R.; Ribeiro, D. Industry 4.0 for the Built Environment: Methodologies, Technologies and Skills ; Springer Nature: Berlin/Heidelberg, Germany, 2021; ISBN 978-3-030-82430-3. [ Google Scholar ]
- Daniotti, B.; Masera, G.; Bolognesi, C.M.; Lupica Spagnolo, S.; Pavan, A.; Iannaccone, G.; Signorini, M.; Ciuffreda, S.; Mirarchi, C.; Lucky, M.; et al. The Development of a BIM-Based Interoperable Toolkit for Efficient Renovation in Buildings: From BIM to Digital Twin. Buildings 2022 , 12 , 231. [ Google Scholar ] [ CrossRef ]
- Baghalzadeh Shishehgarkhaneh, M.; Keivani, A.; Moehler, R.C.; Jelodari, N.; Roshdi Laleh, S. Internet of Things (IoT), Building Information Modeling (BIM), and Digital Twin (DT) in Construction Industry: A Review, Bibliometric, and Network Analysis. Buildings 2022 , 12 , 1503. [ Google Scholar ] [ CrossRef ]
- Kaur, M.J.; Mishra, V.P.; Maheshwari, P. The Convergence of Digital Twin, IoT, and Machine Learning: Transforming Data into Action ; Springer: Cham, Switzerland, 2020; Available online: https://link.springer.com/chapter/10.1007/978-3-030-18732-3_1 (accessed on 14 May 2023).
- Schleich, B.; Anwer, N.; Mathieu, L.; Wartzack, S. Shaping the Digital Twin for Design and Production Engineering. CIRP Ann. 2017 , 66 , 141–144. [ Google Scholar ] [ CrossRef ] [ Green Version ]
- Qi, Q.; Tao, F. Digital Twin and Big Data Towards Smart Manufacturing and Industry 4.0: 360 Degree Comparison. IEEE Access 2018 , 6 , 3585–3593. [ Google Scholar ] [ CrossRef ]
- Carlsén, A.; Elfstrand, O. Augmented Construction: Developing a Framework for Implementing Building Information Modeling through Augmented Reality at Construction Sites ; Semantic Scholar: Stockholm, Sweden, 2018. [ Google Scholar ]
- Gubbi, J.; Buyya, R.; Marusic, S.; Palaniswami, M. Internet of Things (IoT): A Vision, Architectural Elements, and Future Directions. Future Gener. Comput. Syst. 2013 , 29 , 1645–1660. [ Google Scholar ] [ CrossRef ] [ Green Version ]
- Tang, S.; Shelden, D.R.; Eastman, C.M.; Pishdad-Bozorgi, P.; Gao, X. A Review of Building Information Modeling (BIM) and the Internet of Things (IoT) Devices Integration: Present Status and Future Trends. Autom. Constr. 2019 , 101 , 127–139. [ Google Scholar ] [ CrossRef ]
- Lee, D.; Cha, G.; Park, S. A Study on Data Visualization of Embedded Sensors for Building Energy Monitoring Using BIM. Int. J. Precis. Eng. Manuf. 2016 , 17 , 807–814. [ Google Scholar ] [ CrossRef ]
- IoT for All. ThoughtWire Blog. Digital Twins vs. Building Information Modeling (BIM). 2020. Available online: https://www.iotforall.com/digital-twin-vs-bim?ss360SearchTerm=Digital%20Twin (accessed on 16 August 2022).
- Rolle, R.; Martucci, V.; Godoy, E. Architecture for Digital Twin Implementation Focusing on Industry 4.0. IEEE Lat. Am. Trans. 2020 , 18 , 889–898. [ Google Scholar ] [ CrossRef ]
- Kritzinger, W.; Karner, M.; Traar, G.; Henjes, J.; Sihn, W. Digital Twin in Manufacturing: A Categorical Literature Review and Classification. IFAC Pap. 2018 , 51 , 1016–1022. [ Google Scholar ] [ CrossRef ]
- Lavrentyeva, A.V.; Dzikia, A.A.; Kalinina, A.E.; Frolov, D.P.; Akhverdiev, E.A.; Barakova, A.S. Artificial Intelligence and Digital Transformations in the Society. IOP Conf. Ser. Mater. Sci. Eng. 2019 , 483 , 012019. [ Google Scholar ] [ CrossRef ] [ Green Version ]
Click here to enlarge figure # | Authors/Years | | Journals/ Conferences | Methods | Broad Area |
---|
1 | Khajavi et al. (2019) | [ ] | IEEE | Experimentation Testing | Construction | 2 | Boje et al. (2020) | [ ] | Automation in Construction | Literature Review | Construction | 3 | Jones et al. (2020) | [ ] | CIRP-JMST | Literature Review | Multidisciplinary | 4 | Sacks et al. (2020) | [ ] | Data-Centric Engineering | Literature Review | Construction | 5 | Sepasgozar (2021) | [ ] | MDPI | Literature Review | Construction | 6 | El Jazzar et al. (2020) | [ ] | Conference Paper | Literature Review | Construction | 7 | Shahzad et al. (2022) | [ ] | MDPI | Literature Review Interviews | Multidisciplinary | 8 | Alonso et al. (2019) | [ ] | MDPI | Literature Review | Construction | 9 | Deng et al. (2021) | [ ] | IT Con | Literature Review | Civil Engineering | 10 | Opoku et al. (2021) | [ ] | Building Engineering | Systematic Review | Construction | 11 | Lu et al. (2020) | [ ] | CSIC | Literature Review | Construction | 12 | Zhang et al. (2022) | [ ] | ASCE | Questionnaires Interviews | Construction | 13 | Sawhney et al. (2020) | [ ] | ASC | Literature Review | Construction | 14 | Madubuike et al. (2022) | [ ] | IT Con | Systematic Review | Construction | 15 | Feng et al. (2021) | [ ] | ISARC | Literature Review | Construction | 16 | Zhou et al. (2021) | [ ] | PSGEC | Literature Review | Construction | 17 | Zhang et al. (2021) | [ ] | Tech. Science Press | Literature Review | Construction | 18 | Akanmu et al. (2021) | [ ] | IT Con | Literature Review | Construction | 19 | Grieves (2019) | [ ] | Astronautics Aeronautics | Literature Review | Engineering | 20 | Seaton et al. (2022) | [ ] | World Built Environment Forum | Literature Review Case Studies | Construction | 21 | Zhang et al. (2021) | [ ] | Frontiers in Sustainable Cities | Literature Review | Construction | 22 | Hosamo et al. (2022) | [ ] | Hindawi Civil Engineering | Literature Review | Construction | 23 | Douglas et al. (2021) | [ ] | Computing in Construction | Systematic Review | Construction | 24 | Gorse et al. (2020) | [ ] | SEEDS | Literature Review Interviews | Construction | 25 | Sobhkhiz and El-Diraby (2022) | [ ] | ASCE | Case Study | Construction | 26 | Almatared et al. (2022) | [ ] | ASCE | Literature Review | Construction | 27 | Quirk et al. (2020) | [ ] | ASHRAE | Literature Review | Construction | 28 | Bolpagni et al. (2021) | [ ] | Structural Integrity 20 | Case Study Literature Review | Construction | 29 | Daniotti et al. (2022) | [ ] | MDPI | Literature Review Experimentation Testing | Construction | 30 | Baghalzadeh et al. (2022) | [ ] | MDPI | Literature Review | Construction | # | Items | BIM | Digital Twin in Building |
---|
1 | Concept Origin | Dr. Charles Eastman (1970s) | NASA Apollo program (1960s) Dr. Michael Grieves (2000s) | 2 | Purposes | Used to enhance efficiency during design, construction, and throughout the building lifecycle | Used to enhance operational efficiency through predictive maintenance and monitoring assets | 3 | Application focus | Design visualization and consistency Class detection Time and cost estimation Lean construction Stakeholders’ interoperability | Predictive Maintenance What-if analysis Occupant satisfaction Resource consumption efficiency Closed-loop design | 4 | Features | Real time data flow is not necessarily required. | Real time data flow is not necessarily required | 5 | Level of Details | A detailed model of the building’s design and construction | Performance and optimization-focused real time building operation replica | 6 | Scalability | Depends on underlying technology and resources available for data processing and storage | More suitable for large-scale projects | 7 | Main Users | Complex and detailed, geared towards architects, engineers, contractors, and building professionals with high level of control and customization | Streamlined and intuitive, geared towards facility managers and operators with real time data and monitoring capabilities | 8 | Interoperability | 3D model, Construction Operation Building COBie, IFC, CDE | 3D Model, WSN, Data Analytics, Machine learning | 9 | Application interface | Autodek Revit, ArchiCAD, MicroStation, BIM Server, Grevit, Open Source | Autodesk Tandem, Predix, Dasher 360, Ecodomus, Siemens Digital Twin, Bentley iTwin | 10 | Building Life cycle stage | Design Construction Use (Maintenance) Demolition | Use (Operation) | # | Items | BIM | Digital Twin | Sources |
---|
1 | 3D model visualization | Yes | Yes | [ , ] | 2 | Reliance on CDE | Yes | No | [ , ] | 3 | Reliance on IFC | Yes | No | [ , ] | 4 | Reliance on WSN | No | Yes | [ , ] | 5 | Reliance on Data Analytics | No | Yes | [ , ] | 6 | Reliance on Machine Learning | No | Yes | [ , ] | 7 | APIs Interoperability | Yes | Yes | [ , ] | 8 | COBie Interoperability | Yes | Yes | [ , ] | 9 | Data standardization | Yes | Yes | [ , ] | 10 | Data exchangeability (two-way communication) | No | Yes | [ ] | 11 | Scheduling | Yes | Yes | [ , ] | 12 | Architects, Engineers, and Contractors interface | Yes | No | [ ] | 13 | Facility Manager/Operator interface | No | Yes | [ , ] | 14 | Focus on Collaboration | Yes | Yes | [ , ] | 15 | Focus on Real-time data | No | Yes | [ , ] | 16 | Focus on Design and Construction | Yes | No | [ , ] | 17 | Focus on Building Operations | No | Yes | [ , ] | 18 | Focus on Physical & Functional Aspects of Building | Yes | No | [ , ] | 19 | Inclusion of People, Processes, and Behaviors | No | Yes | [ , ] | 20 | Time management | Yes | Yes | [ , ] | 21 | Budget management | Yes | Yes | [ , ] | 22 | Project simulation analysis | Yes | Yes | [ ] | 23 | Simulation analysis in context | No | Yes | [ ] | 24 | Live monitoring of assets | No | Yes | [ , ] | 25 | Live and instant updates on equipment status | No | Yes | [ ] | 26 | Instant response to equipment failures | No | Yes | [ ] | 27 | Insights to increase building use and performance | No | Yes | [ ] | 28 | Overall project time and cost reduction | Yes | Yes | [ , ] | 29 | Easy application on existing buildings | No | Yes | [ ] | 30 | Better value for employers | Yes | Yes | [ , ] | 31 | Improved building sustainability | Yes | Yes | [ , ] | 32 | Dynamic construction risk management improved | No | Yes | [ , ] | 33 | Enhance site logistics | No | Yes | [ , ] | 34 | Use of machine learning and automated processes | No | Yes | [ , ] | 35 | Use of self-learning algorithms | No | Yes | [ , ] | | The statements, opinions and data contained in all publications are solely those of the individual author(s) and contributor(s) and not of MDPI and/or the editor(s). MDPI and/or the editor(s) disclaim responsibility for any injury to people or property resulting from any ideas, methods, instructions or products referred to in the content. |
Share and CiteNguyen, T.D.; Adhikari, S. The Role of BIM in Integrating Digital Twin in Building Construction: A Literature Review. Sustainability 2023 , 15 , 10462. https://doi.org/10.3390/su151310462 Nguyen TD, Adhikari S. The Role of BIM in Integrating Digital Twin in Building Construction: A Literature Review. Sustainability . 2023; 15(13):10462. https://doi.org/10.3390/su151310462 Nguyen, Tran Duong, and Sanjeev Adhikari. 2023. "The Role of BIM in Integrating Digital Twin in Building Construction: A Literature Review" Sustainability 15, no. 13: 10462. https://doi.org/10.3390/su151310462 Article MetricsArticle access statistics, further information, mdpi initiatives, follow mdpi. 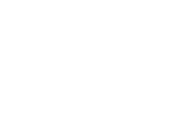 Subscribe to receive issue release notifications and newsletters from MDPI journals 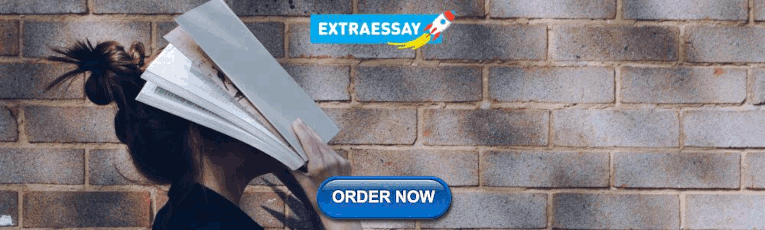 |
COMMENTS
Based on the literature review, the authors provide a definition for Industry 4.0 which specifies six design principles that should be considered when applying Industry 4.0 solutions. The article is structured as the following. Chapter 2 provides an overview of the research process and method implemented in this study.
Note that, this exhaustive literature re view provides a concrete definition of Industry 4.0 and defines its six design principles. such as interoperability, virtualization, local, real-time ...
This exhaustive literature review provides a concrete definition of Industry 4.0 and defines its six design principles such as interoperability, virtualization, local, real-time talent, service orientation and modularity. Manufacturing industry profoundly impact economic and societal progress. As being a commonly accepted term for research centers and universities, the Industry 4.0 initiative ...
AbstractManufacturing industry profoundly impact economic and societal progress. As being a commonly accepted term for research centers and universities, the Industry 4.0 initiative has received a splendid attention of the business and research community. ...
road map for implementing Industry 4.0 As can be drawn from the above recommendations, the superior quality of the manufacturing industry strictly depends on its high quality applied production technology. Industry 4.0 standards ensures this by addressing several high rank research topics including autonomy, machine to
Abstract. Industry 4.0 has become one of the most discussed subjects in academic and professional fields. The number of articles published is large and continues to increase, introducing new issues, concepts, methods, and technologies. Many review articles deal with specific issues and not always with the necessary rigor, making a more general ...
Literature review of Industry 4.0 and related technologies Oztemel Ercan; Samet, Gursev. Journal of Intelligent Manufacturing ; London Vol. 31, Iss. 1, (Jan 2020): 127-182.
(DOI: 10.1007/S10845-018-1433-8) Manufacturing industry profoundly impact economic and societal progress. As being a commonly accepted term for research centers and universities, the Industry 4.0 initiative has received a splendid attention of the business and research community. Although the idea is not new and was on the agenda of academic research in many years with different perceptions ...
Literature review of Industry 4.0 and related technologies. Abstract: Abstract Manufacturing industry profoundly impact economic and societal progress. As being a commonly accepted term for research centers and universities, the Industry 4.0 initiative has received a splendid attention of the business and research community.
Ercan Oztemel & Samet Gursev, 2020. " Literature review of Industry 4.0 and related technologies ," Journal of Intelligent Manufacturing, Springer, vol. 31 (1), pages 127-182, January. Downloadable (with restrictions)! Manufacturing industry profoundly impact economic and societal progress. As being a commonly accepted term for research centers ...
This disruptive impact on manufacturing companies will allow the smart manufacturing ecosystem paradigm. Industry 4.0 is the turning point to the end of the conventional centralized applications. The Industry 4.0 environment is scanned on this paper, describing the so-called enabling technologies and systems over the manufacturing environment.
In the era of industry 4.0, many manufacturing industries are expected to adopt and implement the fourth industrial revolution. The purpose of this study is to focus on the technologies that helps in increasing the productivity of companies by using Industry 4.0 technologies. Also, industry 4.0 has a big impact on the economic and environment which is trending nowadays. A systematic Literature ...
THE DIRECTION OF INDUSTRY: A LITERATURE REVIEW ON INDUSTRY 4.0. Wichmann, Robert Lawrence (1); Eisenbart, Boris (1); Gericke, Kilian (2) iversity of Technology; 2: University of LuxembourgABSTRACTWith the rapid success of the digital enterprises in the 21st Century, industrial manufacturing is expected to be approaching.
Industry 4.0 or smart manufacturing is an industrial revolution for the production systems that has a great impact on competitiveness and the various prompted lines of research. This new concept that allows to interconnect the machines between them (IOT) and facilitates production with a minimum of time and cost generates anomalies and research questions. In this paper, we will review the ...
The findings of this systematic literature review highlight the multifaceted role of Industry 4.0 technologies in achieving net-zero supply chain performance. However, the study also identifies challenges related to policy, technology, economy, and markets to harness these technologies effectively.
The purpose of this paper is to identify current technologies related to Industry 4.0 and to develop a rationale to enhance the understanding of their functions within a data-driven paradigm.,A systematic literature review of 119 papers published in journals included in the Journal Citation Report (JCR) was conducted to identify Industry 4.0 ...
Manufacturing industry profoundly impact economic and societal progress. As being a commonly accepted term for research centers and universities, the Industry 4.0 initiative has received a splendid attention of the business and research community. Although the idea is not new and was on the agenda of academic research in many years with different perceptions, the term Industry 4.0 is just ...
@article{Santos2024Industry4T, title={Industry 4.0 technologies for sustainability within small and medium enterprises: A systematic literature review and future directions}, author={Andr{\'e} de Mendonça Santos and {\^A}ngelo M{\'a}rcio Oliveira Sant'Anna}, journal={Journal of Cleaner Production}, year={2024}, url={https://api ...
This research focuses on the applicability of Industry 4.0 (I 4.0) technologies exploring their relevance to sustainable business performance. ... After this author creates a table based on Review Literature on Sustainability and Review Literature on Industry 4.0, further distribution is stated below. ... the recent rise of Industry 4.0-related ...
The objective of this paper is to visualize and to show the direction of Industry 4.0 to develop smart factories in future. The application of new techniques and technologies which are based upon the Internet of things (IOT) ,block chain technology, cloud computing and cyber physical system have paved the path of significant improvement such as increase of automation, quality of production and ...
Oztemel, E and S Gursev (2020) Literature review of industry 4.0 and related technologies. Journal of Intelligent Manufacturing, 31(1), 127-182. Crossref, Google Scholar; Paolanti, M, L Romeo, A Felicetti, A Mancini, E Frontoni and J Loncarski (2018) Machine learning approach for predictive maintenance in industry 4.0.
Even though the topic of Industry 4.0 in the last decade has attracted significant and multifarious attention from academics and practitioners, a structured and systematic review of Industry 4.0 in the context of contemporary logistics is currently lacking. This study attempted to address this shortcoming by performing a systematic review of the available literature of Industry 4.0 in the ...
Global distribution of case studies. 4.2. Occurrence of Industry 4.0 Technologies Figure 5 above indicates how the technologies of Industry 4.0 have been covered in the literature over the years ...
Besides the regional impact, the integration of Industry 4.0 technologies strongly affects the actual working space of employees. ... Literature review of Industry 4.0 and related technologies. Journal of Intelligent Manufacturing, 31 (1) (2020), pp. 127-182, 10.1007/s10845-018-1433-8.
Conclusions: Barriers to implementing Industry 4.0 technologies are interrelated in nature and prevent businesses from realizing the full benefit of implemented Industry 4.0 technologies. Adequate financial support, new knowledge and skills are required to be able to ensure the successful implementation of Industry 4.0 in warehousing management.
Literature Review: The study reviews Industry 4.0's impact on the construction sector, defining the framework, benefits, and barriers. Revealing BIM and CDE are crucial for Construction 4.0 implementation, transforming the industry into efficient, quality-centered, and safe. 14: A Review of Digital Twin Applications in Construction
The bibliometric analysis shows that Industry 4.0 technologies will help to improve sustainable waste management practices and shows a close association between waste management and Industry 4.0. Szpilko et al. (2023) presented a literature review on waste management in smart cities and the linking of current practices and future directions.