Thank you for visiting nature.com. You are using a browser version with limited support for CSS. To obtain the best experience, we recommend you use a more up to date browser (or turn off compatibility mode in Internet Explorer). In the meantime, to ensure continued support, we are displaying the site without styles and JavaScript.
- View all journals
- My Account Login
- Explore content
- About the journal
- Publish with us
- Sign up for alerts
- Open access
- Published: 10 October 2022
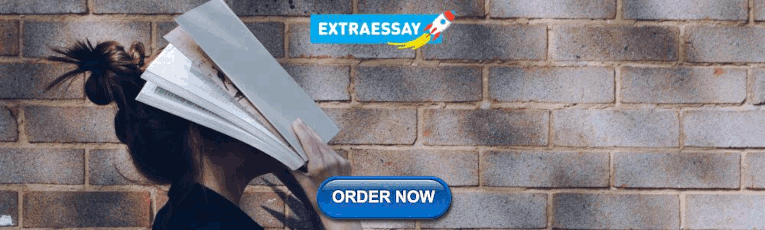
Health effects associated with smoking: a Burden of Proof study
- Xiaochen Dai ORCID: orcid.org/0000-0002-0289-7814 1 , 2 ,
- Gabriela F. Gil 1 ,
- Marissa B. Reitsma 1 ,
- Noah S. Ahmad 1 ,
- Jason A. Anderson 1 ,
- Catherine Bisignano 1 ,
- Sinclair Carr 1 ,
- Rachel Feldman 1 ,
- Simon I. Hay ORCID: orcid.org/0000-0002-0611-7272 1 , 2 ,
- Jiawei He 1 , 2 ,
- Vincent Iannucci 1 ,
- Hilary R. Lawlor 1 ,
- Matthew J. Malloy 1 ,
- Laurie B. Marczak 1 ,
- Susan A. McLaughlin 1 ,
- Larissa Morikawa ORCID: orcid.org/0000-0001-9749-8033 1 ,
- Erin C. Mullany 1 ,
- Sneha I. Nicholson 1 ,
- Erin M. O’Connell 1 ,
- Chukwuma Okereke 1 ,
- Reed J. D. Sorensen 1 ,
- Joanna Whisnant 1 ,
- Aleksandr Y. Aravkin 1 , 3 ,
- Peng Zheng 1 , 2 ,
- Christopher J. L. Murray ORCID: orcid.org/0000-0002-4930-9450 1 , 2 &
- Emmanuela Gakidou ORCID: orcid.org/0000-0002-8992-591X 1 , 2
Nature Medicine volume 28 , pages 2045–2055 ( 2022 ) Cite this article
25k Accesses
43 Citations
168 Altmetric
Metrics details
- Risk factors
Matters Arising to this article was published on 14 April 2023
As a leading behavioral risk factor for numerous health outcomes, smoking is a major ongoing public health challenge. Although evidence on the health effects of smoking has been widely reported, few attempts have evaluated the dose–response relationship between smoking and a diverse range of health outcomes systematically and comprehensively. In the present study, we re-estimated the dose–response relationships between current smoking and 36 health outcomes by conducting systematic reviews up to 31 May 2022, employing a meta-analytic method that incorporates between-study heterogeneity into estimates of uncertainty. Among the 36 selected outcomes, 8 had strong-to-very-strong evidence of an association with smoking, 21 had weak-to-moderate evidence of association and 7 had no evidence of association. By overcoming many of the limitations of traditional meta-analyses, our approach provides comprehensive, up-to-date and easy-to-use estimates of the evidence on the health effects of smoking. These estimates provide important information for tobacco control advocates, policy makers, researchers, physicians, smokers and the public.
Similar content being viewed by others
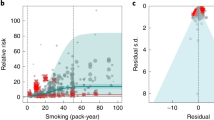
The Burden of Proof studies: assessing the evidence of risk
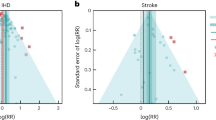
Health effects associated with exposure to secondhand smoke: a Burden of Proof study
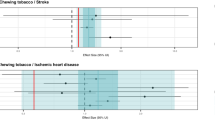
Health effects associated with chewing tobacco: a Burden of Proof study
Among both the public and the health experts, smoking is recognized as a major behavioral risk factor with a leading attributable health burden worldwide. The health risks of smoking were clearly outlined in a canonical study of disease rates (including lung cancer) and smoking habits in British doctors in 1950 and have been further elaborated in detail over the following seven decades 1 , 2 . In 2005, evidence of the health consequences of smoking galvanized the adoption of the first World Health Organization (WHO) treaty, the Framework Convention on Tobacco Control, in an attempt to drive reductions in global tobacco use and second-hand smoke exposure 3 . However, as of 2020, an estimated 1.18 billion individuals globally were current smokers and 7 million deaths and 177 million disability-adjusted life-years were attributed to smoking, reflecting a persistent public health challenge 4 . Quantifying the relationship between smoking and various important health outcomes—in particular, highlighting any significant dose–response relationships—is crucial to understanding the attributable health risk experienced by these individuals and informing responsive public policy.
Existing literature on the relationship between smoking and specific health outcomes is prolific, including meta-analyses, cohort studies and case–control studies analyzing the risk of outcomes such as lung cancer 5 , 6 , 7 , chronic obstructive pulmonary disease (COPD) 8 , 9 , 10 and ischemic heart disease 11 , 12 , 13 , 14 due to smoking. There are few if any attempts, however, to systematically and comprehensively evaluate the landscape of evidence on smoking risk across a diverse range of health outcomes, with most current research focusing on risk or attributable burden of smoking for a specific condition 7 , 15 , thereby missing the opportunity to provide a comprehensive picture of the health risk experienced by smokers. Furthermore, although evidence surrounding specific health outcomes, such as lung cancer, has generated widespread consensus, findings about the attributable risk of other outcomes are much more heterogeneous and inconclusive 16 , 17 , 18 . These studies also vary in their risk definitions, with many comparing dichotomous exposure measures of ever smokers versus nonsmokers 19 , 20 . Others examine the distinct risks of current smokers and former smokers compared with never smokers 21 , 22 , 23 . Among the studies that do analyze dose–response relationships, there is large variation in the units and dose categories used in reporting their findings (for example, the use of pack-years or cigarettes per day) 24 , 25 , which complicates the comparability and consolidation of evidence. This, in turn, can obscure data that could inform personal health choices, public health practices and policy measures. Guidance on the health risks of smoking, such as the Surgeon General’s Reports on smoking 26 , 27 , is often based on experts’ evaluation of heterogenous evidence, which, although extremely useful and well suited to carefully consider nuances in the evidence, is fundamentally subjective.
The present study, as part of the Global Burden of Diseases, Risk Factors, and Injuries Study (GBD) 2020, re-estimated the continuous dose–response relationships (the mean risk functions and associated uncertainty estimates) between current smoking and 36 health outcomes (Supplementary Table 1 ) by identifying input studies using a systematic review approach and employing a meta-analytic method 28 . The 36 health outcomes that were selected based on existing evidence of a relationship included 16 cancers (lung cancer, esophageal cancer, stomach cancer, leukemia, liver cancer, laryngeal cancer, breast cancer, cervical cancer, colorectal cancer, lip and oral cavity cancer, nasopharyngeal cancer, other pharynx cancer (excluding nasopharynx cancer), pancreatic cancer, bladder cancer, kidney cancer and prostate cancer), 5 cardiovascular diseases (CVDs: ischemic heart disease, stroke, atrial fibrillation and flutter, aortic aneurysm and peripheral artery disease) and 15 other diseases (COPD, lower respiratory tract infections, tuberculosis, asthma, type 2 diabetes, Alzheimer’s disease and related dementias, Parkinson’s disease, multiple sclerosis, cataracts, gallbladder diseases, low back pain, peptic ulcer disease, rheumatoid arthritis, macular degeneration and fractures). Definitions of the outcomes are described in Supplementary Table 1 . We conducted a separate systematic review for each risk–outcome pair with the exception of cancers, which were done together in a single systematic review. This approach allowed us to systematically identify all relevant studies indexed in PubMed up to 31 May 2022, and we extracted relevant data on risk of smoking, including study characteristics, following a pre-specified template (Supplementary Table 2 ). The meta-analytic tool overcomes many of the limitations of traditional meta-analyses by incorporating between-study heterogeneity into the uncertainty of risk estimates, accounting for small numbers of studies, relaxing the assumption of log(linearity) applied to the risk functions, handling differences in exposure ranges between comparison groups, and systematically testing and adjusting for bias due to study designs and characteristics. We then estimated the burden-of-proof risk function (BPRF) for each risk–outcome pair, as proposed by Zheng et al. 29 ; the BPRF is a conservative risk function defined as the 5th quantile curve (for harmful risks) that reflects the smallest harmful effect at each level of exposure consistent with the available evidence. Given all available data for each outcome, the risk of smoking is at least as harmful as the BPRF indicates.
We used the BPRF for each risk–outcome pair to calculate risk–outcome scores (ROSs) and categorize the strength of evidence for the association between smoking and each health outcome using a star rating from 1 to 5. The interpretation of the star ratings is as follows: 1 star (*) indicates no evidence of association; 2 stars (**) correspond to a 0–15% increase in risk across average range of exposures for harmful risks; 3 stars (***) represent a 15–50% increase in risk; 4 stars (****) refer to >50–85% increase in risk; and 5 stars (*****) equal >85% increase in risk. The thresholds for each star rating were developed in consultation with collaborators and other stakeholders.
The increasing disease burden attributable to current smoking, particularly in low- and middle-income countries 4 , demonstrates the relevance of the present study, which quantifies the strength of the evidence using an objective, quantitative, comprehensive and comparative framework. Findings from the present study can be used to support policy makers in making informed smoking recommendations and regulations focusing on the associations for which the evidence is strongest (that is, the 4- and 5-star associations). However, associations with a lower star rating cannot be ignored, especially when the outcome has high prevalence or severity. A summary of the main findings, limitations and policy implications of the study is presented in Table 1 .
We evaluated the mean risk functions and the BPRFs for 36 health outcomes that are associated with current smoking 30 (Table 2 ). Following the Preferred Reporting Items for Systematic Reviews and Meta-Analyses (PRISMA) guidelines 31 for each of our systematic reviews, we identified studies reporting relative risk (RR) of incidence or mortality from each of the 36 selected outcomes for smokers compared with nonsmokers. We reviewed 21,108 records, which were identified to have been published between 1 May 2018 and 31 May 2022; this represents the most recent time period since the last systematic review of the available evidence for the GBD at the time of publication. The meta-analyses reported in the present study for each of the 36 health outcomes are based on evidence from a total of 793 studies published between 1970 and 2022 (Extended Data Fig. 1 – 5 and Supplementary Information 1.5 show the PRISMA diagrams for each outcome). Only prospective cohort and case–control studies were included for estimating dose–response risk curves, but cross-sectional studies were also included for estimating the age pattern of smoking risk on cardiovascular and circulatory disease (CVD) outcomes. Details on each, including the study’s design, data sources, number of participants, length of follow-up, confounders adjusted for in the input data and bias covariates included in the dose–response risk model, can be found in Supplementary Information 2 and 3 . The theoretical minimum risk exposure level used for current smoking was never smoking or zero 30 .
Five-star associations
When the most conservative interpretation of the evidence, that is, the BPRF, suggests that the average exposure (15th–85th percentiles of exposure) of smoking increases the risk of a health outcome by >85% (that is, ROS > 0.62), smoking and that outcome are categorized as a 5-star pair. Among the 36 outcomes, there are 5 that have a 5-star association with current smoking: laryngeal cancer (375% increase in risk based on the BPRF, 1.56 ROS), aortic aneurysm (150%, 0.92), peripheral artery disease (137%, 0.86), lung cancer (107%, 0.73) and other pharynx cancer (excluding nasopharynx cancer) (92%, 0.65).
Results for all 5-star risk–outcome pairs are available in Table 2 and Supplementary Information 4.1 . In the present study, we provide detailed results for one example 5-star association: current smoking and lung cancer. We extracted 371 observations from 25 prospective cohort studies and 53 case–control studies across 25 locations (Supplementary Table 3 ) 5 , 6 , 32 , 33 , 34 , 35 , 36 , 37 , 38 , 39 , 40 , 41 , 42 , 43 , 44 , 45 , 46 , 47 , 48 , 49 , 50 , 51 , 52 , 53 , 54 , 55 , 56 , 57 , 58 , 59 , 60 , 61 , 62 , 63 , 64 , 65 , 66 , 67 , 68 , 69 , 70 , 71 , 72 , 73 , 74 , 75 , 76 , 77 , 78 , 79 , 80 , 81 , 82 , 83 , 84 , 85 , 86 , 87 , 88 , 89 , 90 , 91 , 92 , 93 , 94 , 95 , 96 , 97 , 98 , 99 , 100 , 101 , 102 , 103 , 104 , 105 , 106 , 107 . Exposure ranged from 1 pack-year to >112 pack-years, with the 85th percentile of exposure being 50.88 pack-years (Fig. 1a ).
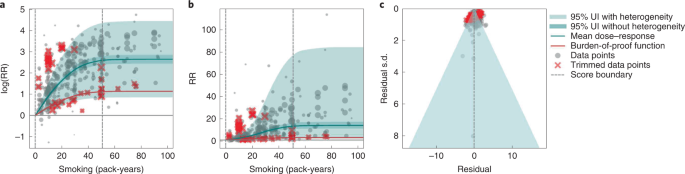
a , The log(RR) function. b , RR function. c , A modified funnel plot showing the residuals (relative to 0) on the x axis and the estimated s.d. that includes reported s.d. and between-study heterogeneity on the y axis.
We found a very strong and significant harmful relationship between pack-years of current smoking and the RR of lung cancer (Fig. 1b ). The mean RR of lung cancer at 20 pack-years of smoking was 5.11 (95% uncertainty interval (UI) inclusive of between-study heterogeneity = 1.84–14.99). At 50.88 pack-years (85th percentile of exposure), the mean RR of lung cancer was 13.42 (2.63–74.59). See Table 2 for mean RRs at other exposure levels. The BPRF, which represents the most conservative interpretation of the evidence (Fig. 1a ), suggests that smoking in the 15th–85th percentiles of exposure increases the risk of lung cancer by an average of 107%, yielding an ROS of 0.73.
The relationship between pack-years of current smoking and RR of lung cancer is nonlinear, with diminishing impact of further pack-years of smoking, particularly for middle-to-high exposure levels (Fig. 1b ). To reduce the effect of bias, we adjusted observations that did not account for more than five confounders, including age and sex, because they were the significant bias covariates identified by the bias covariate selection algorithm 29 (Supplementary Table 7 ). The reported RRs across studies were very heterogeneous. Our meta-analytic method, which accounts for the reported uncertainty in both the data and between-study heterogeneity, fit the data and covered the estimated residuals well (Fig. 1c ). After trimming 10% of outliers, we still detected publication bias in the results for lung cancer. See Supplementary Tables 4 and 7 for study bias characteristics and selected bias covariates, Supplementary Fig. 5 for results without 10% trimming and Supplementary Table 8 for observed RR data and alternative exposures across studies for the remaining 5-star pairs.
Four-star associations
When the BPRF suggests that the average exposure of smoking increases the risk of a health outcome by 50–85% (that is, ROS > 0.41–0.62), smoking is categorized as having a 4-star association with that outcome. We identified three outcomes with a 4-star association with smoking: COPD (72% increase in risk based on the BPRF, 0.54 ROS), lower respiratory tract infection (54%, 0.43) and pancreatic cancer (52%, 0.42).
In the present study, we provide detailed results for one example 4-star association: current smoking and COPD. We extracted 51 observations from 11 prospective cohort studies and 4 case–control studies across 36 locations (Supplementary Table 3 ) 6 , 8 , 9 , 10 , 78 , 108 , 109 , 110 , 111 , 112 , 113 , 114 , 115 , 116 . Exposure ranged from 1 pack-year to 100 pack-years, with the 85th percentile of exposure in the exposed group being 49.75 pack-years.
We found a strong and significant harmful relationship between pack-years of current smoking and RR of COPD (Fig. 2b ). The mean RR of COPD at 20 pack-years was 3.17 (1.60–6.55; Table 2 reports RRs at other exposure levels). At the 85th percentile of exposure, the mean RR of COPD was 6.01 (2.08–18.58). The BPRF suggests that average smoking exposure raises the risk of COPD by an average of 72%, yielding an ROS of 0.54. The results for the other health outcomes that have an association with smoking rated as 4 stars are shown in Table 2 and Supplementary Information 4.2 .
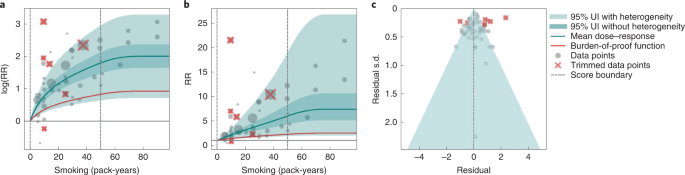
a , The log(RR) function. b , RR function. c , A modified funnel plot showing the residuals (relative to 0) on th e x axis and the estimated s.d. that includes the reported s.d. and between-study heterogeneity on the y axis.
The relationship between smoking and COPD is nonlinear, with diminishing impact of further pack-years of current smoking on risk of COPD, particularly for middle-to-high exposure levels (Fig. 2a ). To reduce the effect of bias, we adjusted observations that did not account for age and sex and/or were generated for individuals aged >65 years 116 , because they were the two significant bias covariates identified by the bias covariate selection algorithm (Supplementary Table 7 ). There was large heterogeneity in the reported RRs across studies, and our meta-analytic method fit the data and covered the estimated residuals well (Fig. 2b ). Although we trimmed 10% of outliers, publication bias was still detected in the results for COPD. See Supplementary Tables 4 and 7 for study bias characteristics and selected bias covariates, Supplementary Fig. 5 for results without 10% trimming and Supplementary Table 8 for reported RR data and alternative exposures across studies for the remaining health outcomes that have a 4-star association with smoking.
Three-star associations
When the BPRF suggests that the average exposure of smoking increases the risk of a health outcome by 15–50% (or, when protective, decreases the risk of an outcome by 13–34%; that is, ROS >0.14–0.41), the association between smoking and that outcome is categorized as having a 3-star rating. We identified 15 outcomes with a 3-star association: bladder cancer (40% increase in risk, 0.34 ROS); tuberculosis (31%, 0.27); esophageal cancer (29%, 0.26); cervical cancer, multiple sclerosis and rheumatoid arthritis (each 23–24%, 0.21); lower back pain (22%, 0.20); ischemic heart disease (20%, 0.19); peptic ulcer and macular degeneration (each 19–20%, 0.18); Parkinson's disease (protective risk, 15% decrease in risk, 0.16); and stomach cancer, stroke, type 2 diabetes and cataracts (each 15–17%, 0.14–0.16).
We present the findings on smoking and type 2 diabetes as an example of a 3-star risk association. We extracted 102 observations from 24 prospective cohort studies and 4 case–control studies across 15 locations (Supplementary Table 3 ) 117 , 118 , 119 , 120 , 121 , 122 , 123 , 124 , 125 , 126 , 127 , 128 , 129 , 130 , 131 , 132 , 133 , 134 , 135 , 136 , 137 , 138 , 139 , 140 , 141 , 142 , 143 , 144 . The exposure ranged from 1 cigarette to 60 cigarettes smoked per day, with the 85th percentile of exposure in the exposed group being 26.25 cigarettes smoked per day.
We found a moderate and significant harmful relationship between cigarettes smoked per day and the RR of type 2 diabetes (Fig. 3b ). The mean RR of type 2 diabetes at 20 cigarettes smoked per day was 1.49 (1.18–1.90; see Table 2 for other exposure levels). At the 85th percentile of exposure, the mean RR of type 2 diabetes was 1.54 (1.20–2.01). The BPRF suggests that average smoking exposure raises the risk of type 2 diabetes by an average of 16%, yielding an ROS of 0.15. See Table 2 and Supplementary Information 4.3 for results for the additional health outcomes with an association with smoking rated as 3 stars.
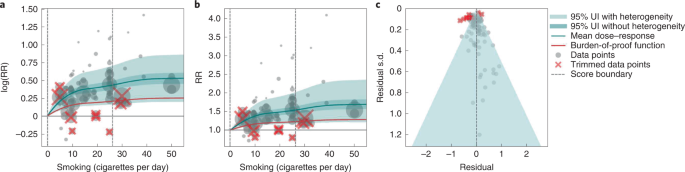
a , The log(RR) function. b , RR function. c , A modified funnel plot showing the residuals (relative to 0) on the x axis and the estimated s.d. that includes the reported s.d. and between-study heterogeneity on the y axis.
The relationship between smoking and type 2 diabetes is nonlinear, particularly for high exposure levels where the mean risk curve becomes flat (Fig. 3a ). We adjusted observations that were generated in subpopulations, because it was the only significant bias covariate identified by the bias covariate selection algorithm (Supplementary Table 7 ). There was moderate heterogeneity in the observed RR data across studies and our meta-analytic method fit the data and covered the estimated residuals extremely well (Fig. 3b,c ). After trimming 10% of outliers, we still detected publication bias in the results for type 2 diabetes. See Supplementary Tables 4 and 7 for study bias characteristics and selected bias covariates, Supplementary Fig. 5 for results without 10% trimming and Supplementary Table 8 for observed RR data and alternative exposures across studies for the remaining 3-star pairs.
Two-star associations
When the BPRF suggests that the average exposure of smoking increases the risk of an outcome by 0–15% (that is, ROS 0.0–0.14), the association between smoking and that outcome is categorized as a 2-star rating. We identified six 2-star outcomes: nasopharyngeal cancer (14% increase in risk, 0.13 ROS); Alzheimer’s and other dementia (10%, 0.09); gallbladder diseases and atrial fibrillation and flutter (each 6%, 0.06); lip and oral cavity cancer (5%, 0.05); and breast cancer (4%, 0.04).
We present the findings on smoking and breast cancer as an example of a 2-star association. We extracted 93 observations from 14 prospective cohort studies and 9 case–control studies across 14 locations (Supplementary Table 3 ) 84 , 87 , 145 , 146 , 147 , 148 , 149 , 150 , 151 , 152 , 153 , 154 , 155 , 156 , 157 , 158 , 159 , 160 , 161 , 162 , 163 , 164 , 165 . The exposure ranged from 1 cigarette to >76 cigarettes smoked per day, with the 85th percentile of exposure in the exposed group being 34.10 cigarettes smoked per day.
We found a weak but significant relationship between pack-years of current smoking and RR of breast cancer (Extended Data Fig. 6 ). The mean RR of breast cancer at 20 pack-years was 1.17 (1.04–1.31; Table 2 reports other exposure levels). The BPRF suggests that average smoking exposure raises the risk of breast cancer by an average of 4%, yielding an ROS of 0.04. See Table 2 and Supplementary Information 4.4 for results on the additional health outcomes for which the association with smoking has been categorized as 2 stars.
The relationship between smoking and breast cancer is nonlinear, particularly for high exposure levels where the mean risk curve becomes flat (Extended Data Fig. 6a ). To reduce the effect of bias, we adjusted observations that were generated in subpopulations, because it was the only significant bias covariate identified by the bias covariate selection algorithm (Supplementary Table 7 ). There was heterogeneity in the reported RRs across studies, but our meta-analytic method fit the data and covered the estimated residuals (Extended Data Fig. 6b ). After trimming 10% of outliers, we did not detect publication bias in the results for breast cancer. See Supplementary Tables 4 and 7 for study bias characteristics and selected bias covariates, Supplementary Fig. 5 for results without 10% trimming and Supplementary Table 8 for observed RR data and alternative exposures across studies for the remaining 2-star pairs.
One-star associations
When average exposure to smoking does not significantly increase (or decrease) the risk of an outcome, once between-study heterogeneity and other sources of uncertainty are accounted for (that is, ROS < 0), the association between smoking and that outcome is categorized as 1 star, indicating that there is not sufficient evidence for the effect of smoking on the outcome to reject the null (that is, there may be no association). There were seven outcomes with an association with smoking that rated as 1 star: colorectal and kidney cancer (each –0.01 ROS); leukemia (−0.04); fractures (−0.05); prostate cancer (−0.06); liver cancer (−0.32); and asthma (−0.64).
We use smoking and prostate cancer as examples of a 1-star association. We extracted 78 observations from 21 prospective cohort studies and 1 nested case–control study across 15 locations (Supplementary Table 3 ) 157 , 160 , 166 , 167 , 168 , 169 , 170 , 171 , 172 , 173 , 174 , 175 , 176 , 177 , 178 , 179 , 180 , 181 , 182 , 183 , 184 , 185 . The exposure among the exposed group ranged from 1 cigarette to 90 cigarettes smoked per day, with the 85th percentile of exposure in the exposed group being 29.73 cigarettes smoked per day.
Based on our conservative interpretation of the data, we did not find a significant relationship between cigarettes smoked per day and the RR of prostate cancer (Fig. 4B ). The exposure-averaged BPRF for prostate cancer was 0.94, which was opposite null from the full range of mean RRs, such as 1.16 (0.89–1.53) at 20 cigarettes smoked per day. The corresponding ROS was −0.06, which is consistent with no evidence of an association between smoking and increased risk of prostate cancer. See Table 2 and Supplementary Information 4.5 for results for the additional outcomes that have a 1-star association with smoking.
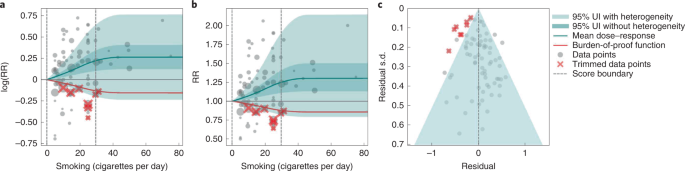
The relationship between smoking and prostate cancer is nonlinear, particularly for middle-to-high exposure levels where the mean risk curve becomes flat (Fig. 4a ). We did not adjust for any bias covariate because no significant bias covariates were selected by the algorithm (Supplementary Table 7 ). The RRs reported across studies were very heterogeneous, but our meta-analytic method fit the data and covered the estimated residuals well (Fig. 4b,c ). The ROS associated with the BPRF is −0.05, suggesting that the most conservative interpretation of all evidence, after accounting for between-study heterogeneity, indicates an inconclusive relationship between smoking exposure and the risk of prostate cancer. After trimming 10% of outliers, we still detected publication bias in the results for prostate cancer, which warrants further studies using sample populations. See Supplementary Tables 4 and 7 for study bias characteristics and selected bias covariates, Supplementary Fig. 5 for results without 10% trimming and Supplementary Table 8 for observed RR data and alternative exposures across studies for the remaining 1-star pairs.
Age-specific dose–response risk for CVD outcomes
We produced age-specific dose–response risk curves for the five selected CVD outcomes ( Methods ). The ROS associated with each smoking–CVD pair was calculated based on the reference risk curve estimated using all risk data regardless of age information. Estimation of the BPRF, calculation of the associated ROS and star rating of the smoking–CVD pairs follow the same rules as the other non-CVD smoking–outcome pairs (Table 1 and Supplementary Figs. 2 – 4 ). Once we had estimated the reference dose–response risk curve for each CVD outcome, we determined the age group of the reference risk curve. The reference age group is 55–59 years for all CVD outcomes, except for peripheral artery disease, the reference age group for which is 60–64 years. We then estimated the age pattern of smoking on all CVD outcomes (Supplementary Fig. 2 ) and calculated age attenuation factors of the risk for each age group by comparing the risk of each age group with that of the reference age group, using the estimated age pattern (Supplementary Fig. 3 ). Last, we applied the draws of age attenuation factors of each age group to the dose–response risk curve for the reference age group to produce the age group-specific dose–response risk curves for each CVD outcome (Supplementary Fig. 4 ).
Using our burden-of-proof meta-analytic methods, we re-estimated the dose–response risk of smoking on 36 health outcomes that had previously been demonstrated to be associated with smoking 30 , 186 . Using these methods, which account for both the reported uncertainty of the data and the between-study heterogeneity, we found that 29 of the 36 smoking–outcome pairs are supported by evidence that suggests a significant dose–response relationship between smoking and the given outcome (28 with a harmful association and 1 with a protective association). Conversely, after accounting for between-study heterogeneity, the available evidence of smoking risk on seven outcomes (that is, colon and rectum cancer, kidney cancer, leukemia, prostate cancer, fractures, liver cancer and asthma) was insufficient to reject the null or draw definitive conclusions on their relationship to smoking. Among the 29 outcomes that have evidence supporting a significant relationship to smoking, 8 had strong-to-very-strong evidence of a relationship, meaning that, given all the available data on smoking risk, we estimate that average exposure to smoking increases the risk of those outcomes by >50% (4- and 5-star outcomes). The currently available evidence for the remaining 21 outcomes with a significant association with current smoking was weak to moderate, indicating that smoking increases the risk of those outcomes by at least >0–50% (2- and 3-star associations).
Even under our conservative interpretation of the data, smoking is irrefutably harmful to human health, with the greatest increases in risk occurring for laryngeal cancer, aortic aneurysm, peripheral artery disease, lung cancer and other pharynx cancer (excluding nasopharynx cancer), which collectively represent large causes of death and ill-health. The magnitude of and evidence for the associations between smoking and its leading health outcomes are among the highest currently analyzed in the burden-of-proof framework 29 . The star ratings assigned to each smoking–outcome pair offer policy makers a way of categorizing and comparing the evidence for a relationship between smoking and its potential health outcomes ( https://vizhub.healthdata.org/burden-of-proof ). We found that, for seven outcomes in our analysis, there was insufficient or inconsistent evidence to demonstrate a significant association with smoking. This is a key finding because it demonstrates the need for more high-quality data for these particular outcomes; availability of more data should improve the strength of evidence for whether or not there is an association between smoking and these health outcomes.
Our systematic review approach and meta-analytic methods have numerous benefits over existing systematic reviews and meta-analyses on the same topic that use traditional random effects models. First, our approach relaxes the log(linear) assumption, using a spline ensemble to estimate the risk 29 . Second, our approach allows variable reference groups and exposure ranges, allowing for more accurate estimates regardless of whether or not the underlying relative risk is log(linear). Furthermore, it can detect outliers in the data automatically. Finally, it quantifies uncertainty due to between-study heterogeneity while accounting for small numbers of studies, minimizing the risk that conclusions will be drawn based on spurious findings.
We believe that the results for the association between smoking and each of the 36 health outcomes generated by the present study, including the mean risk function, BPRF, ROS, average excess risk and star rating, could be useful to a range of stakeholders. Policy makers can formulate their decisions on smoking control priorities and resource allocation based on the magnitude of the effect and the consistency of the evidence relating smoking to each of the 36 outcomes, as represented by the ROS and star rating for each smoking–outcome association 187 . Physicians and public health practitioners can use the estimates of average increased risk and the star rating to educate patients and the general public about the risk of smoking and to promote smoking cessation 188 . Researchers can use the estimated mean risk function or BPRF to obtain the risk of an outcome at a given smoking exposure level, as well as uncertainty surrounding that estimate of risk. The results can also be used in the estimation of risk-attributable burden, that is, the deaths and disability-adjusted life-years due to each outcome that are attributable to smoking 30 , 186 . For the general public, these results could help them to better understand the risk of smoking and manage their health 189 .
Although our meta-analysis was comprehensive and carefully conducted, there are limitations to acknowledge. First, the bias covariates used, although carefully extracted and evaluated, were based on observable study characteristics and thus may not fully capture unobserved characteristics such as study quality or context, which might be major sources of bias. Second, if multiple risk estimates with different adjustment levels were reported in a given study, we included only the fully adjusted risk estimate and modeled the adjustment level according to the number of covariates adjusted for (rather than which covariates were adjusted for) and whether a standard adjustment for age and sex had been applied. This approach limited our ability to make full use of all available risk estimates in the literature. Third, although we evaluated the potential for publication bias in the data, we did not test for other forms of bias such as when studies are more consistent with each other than expected by chance 29 . Fourth, our analysis assumes that the relationships between smoking and health outcomes are similar across geographical regions and over time. We do not have sufficient evidence to quantify how the relationships may have evolved over time because the composition of smoking products has also changed over time. Perhaps some of the heterogeneity of the effect sizes in published studies reflects this; however, this cannot be discerned with the currently available information.
In the future, we plan to include crude and partially adjusted risk estimates in our analyses to fully incorporate all available risk estimates, to model the adjusted covariates in a more comprehensive way by mapping the adjusted covariates across all studies comprehensively and systematically, and to develop methods to evaluate additional forms of potential bias. We plan to update our results on a regular basis to provide timely and up-to-date evidence to stakeholders.
To conclude, we have re-estimated the dose–response risk of smoking on 36 health outcomes while synthesizing all the available evidence up to 31 May 2022. We found that, even after factoring in the heterogeneity between studies and other sources of uncertainty, smoking has a strong-to-very-strong association with a range of health outcomes and confirmed that smoking is irrefutably highly harmful to human health. We found that, due to small numbers of studies, inconsistency in the data, small effect sizes or a combination of these reasons, seven outcomes for which some previous research had found an association with smoking did not—under our meta-analytic framework and conservative approach to interpreting the data—have evidence of an association. Our estimates of the evidence for risk of smoking on 36 selected health outcomes have the potential to inform the many stakeholders of smoking control, including policy makers, researchers, public health professionals, physicians, smokers and the general public.
For the present study, we used a meta-analytic tool, MR-BRT (metaregression—Bayesian, regularized, trimmed), to estimate the dose–response risk curves of the risk of a health outcome across the range of current smoking levels along with uncertainty estimates 28 . Compared with traditional meta-analysis using linear mixed effect models, MR-BRT relaxes the assumption of a log(linear) relationship between exposure and risk, incorporates between-study heterogeneity into the uncertainty of risk estimates, handles estimates reported across different exposure categories, automatically identifies and trims outliers, and systematically tests and adjusts for bias due to study designs and characteristics. The meta-analytic methods employed by the present study followed the six main steps proposed by Zheng et al. 28 , 29 , namely: (1) enacting a systematic review approach and data extraction following a pre-specified and standardized protocol; (2) estimating the shape of the relationship between exposure and RR; (3) evaluating and adjusting for systematic bias as a function of study characteristics and risk estimation; (4) quantifying between-study heterogeneity while adjusting for within-study correlation and the number of studies; (5) evaluating potential publication or reporting biases; and (6) estimating the mean risk function and the BPRF, calculating the ROS and categorizing smoking–outcome pairs using a star-rating scheme from 1 to 5.
The estimates for our primary indicators of this work—mean RRs across a range of exposures, BRPFs, ROSs and star ratings for each risk–outcome pair—are not specific to or disaggregated by specific populations. We did not estimate RRs separately for different locations, sexes (although the RR of prostate cancer was estimated only for males and of cervical and breast cancer only for females) or age groups (although this analysis was applied to disease endpoints in adults aged ≥30 years only and, as detailed below, age-specific estimates were produced for the five CVD outcomes).
The present study complies with the PRISMA guidelines 190 (Supplementary Tables 9 and 10 and Supplementary Information 1.5 ) and Guidelines for Accurate and Transparent Health Estimates Reporting (GATHER) recommendations 191 (Supplementary Table 11 ). The study was approved by the University of Washington Institutional Review Board (study no. 9060). The systematic review approach was not registered.
Selecting health outcomes
In the present study, current smoking is defined as the current use of any smoked tobacco product on a daily or occasional basis. Health outcomes were initially selected using the World Cancer Research Fund criteria for convincing or probable evidence as described in Murray et al. 186 . The 36 health outcomes that were selected based on existing evidence of a relationship included 16 cancers (lung cancer, esophageal cancer, stomach cancer, leukemia, liver cancer, laryngeal cancer, breast cancer, cervical cancer, colorectal cancer, lip and oral cavity cancer, nasopharyngeal cancer, other pharynx cancer (excluding nasopharynx cancer), pancreatic cancer, bladder cancer, kidney cancer and prostate cancer), 5 CVDs (ischemic heart disease, stroke, atrial fibrillation and flutter, aortic aneurysm and peripheral artery disease) and 15 other diseases (COPD, lower respiratory tract infections, tuberculosis, asthma, type 2 diabetes, Alzheimer’s disease and related dementias, Parkinson’s disease, multiple sclerosis, cataracts, gallbladder diseases, low back pain, peptic ulcer disease, rheumatoid arthritis, macular degeneration and fracture). Definitions of the outcomes are described in Supplementary Table 1 .
Step 1: systematic review approach to literature search and data extraction
Informed by the systematic review approach we took for the GBD 2019 (ref. 30 ), for the present study we identified input studies in the literature using a systematic review approach for all 36 smoking–outcome pairs using updated search strings to identify all relevant studies indexed in PubMed up to 31 May 2022 and extracted data on smoking risk estimates. Briefly, the studies that were extracted represented several types of study design (for example, cohort and case–control studies), measured exposure in several different ways and varied in their choice of reference categories (where some compared current smokers with never smokers, whereas others compared current smokers with nonsmokers or former smokers). All these study characteristics were catalogued systematically and taken into consideration during the modeling part of the analysis.
In addition, for CVD outcomes, we also estimated the age pattern of risk associated with smoking. We applied a systematic review of literature approach for smoking risk for the five CVD outcomes. We developed a search string to search for studies reporting any association between binary smoking status (that is, current, former and ever smokers) and the five CVD outcomes from 1 January 1970 to 31 May 2022, and included only studies reporting age-specific risk (RR, odds ratio (OR), hazard ratio (HR)) of smoking status. The inclusion criteria and results of the systematic review approach are reported in accordance with PRISMA guidelines 31 . Details for each outcome on the search string used in the systematic review approach, refined inclusion and exclusion criteria, data extraction template and PRISMA diagram are given in Supplementary Information 1 . Title and/or abstract screening, full text screening and data extraction were conducted by 14 members of the research team and extracted data underwent manual quality assurance by the research team to verify accuracy.
Selecting exposure categories
Cumulative exposure in pack-years was the measure of exposure used for COPD and all cancer outcomes except for prostate cancer, to reflect the risk of both duration and intensity of current smoking on these outcomes. For prostate cancer, CVDs and all the other outcomes except for fractures, we used cigarette-equivalents smoked per day as the exposure for current smoking, because smoking intensity is generally thought to be more important than duration for these outcomes. For fractures, we used binary exposure, because there were few studies examining intensity or duration of smoking on fractures. The smoking–outcome pairs and the corresponding exposures are summarized in Supplementary Table 4 and are congruent with the GBD 2019 (refs. 30 , 186 ).
Steps 2–5: modeling dose–response RR of smoking on the selected health outcomes
Of the six steps proposed by Zheng et al. 29 , steps 2–5 cover the process of modeling dose–response risk curves. In step 2, we estimated the shape (or the ‘signal’) of the dose–response risk curves, integrating over different exposure ranges. To relax the log(linear) assumption usually applied to continuous dose–response risk and make the estimates robust to the placement of spline knots, we used an ensemble spline approach to fit the functional form of the dose–response relationship. The final ensemble model was a weighted combination of 50 models with random knot placement, with the weight of each model proportional to measures of model fit and total variation. To avoid the influence of extreme data and reduce publication bias, we trimmed 10% of data for each outcome as outliers. We also applied a monotonicity constraint to ensure that the mean risk curves were nondecreasing (or nonincreasing in the case of Parkinson’s disease).
In step 3, following the GRADE approach 192 , 193 , we quantified risk of bias across six domains, namely, representativeness of the study population, exposure, outcome, reverse causation, control for confounding and selection bias. Details about the bias covariates are provided in Supplementary Table 4 . We systematically tested for the effect of bias covariates using metaregression, selected significant bias covariates using the Lasso approach 194 , 195 and adjusted for the selected bias covariates in the final risk curve.
In step 4, we quantified between-study heterogeneity accounting for within-study correlation, uncertainty of the heterogeneity, as well as small number of studies. Specifically, we used a random intercept in the mixed-effects model to account for the within-study correlation and used a study-specific random slope with respect to the ‘signal’ to capture between-study heterogeneity. As between-study heterogeneity can be underestimated or even zero when the number of studies is small 196 , 197 , we used Fisher’s information matrix to estimate the uncertainty of the heterogeneity 198 and incorporated that uncertainty into the final results.
In step 5, in addition to generating funnel plots and visually inspecting for asymmetry (Figs. 1c , 2c , 3c and 4c and Extended Data Fig. 6c ) to identify potential publication bias, we also statistically tested for potential publication or reporting bias using Egger’s regression 199 . We flagged potential publication bias in the data but did not correct for it, which is in line with the general literature 10 , 200 , 201 . Full details about the modeling process have been published elsewhere 29 and model specifications for each outcome are in Supplementary Table 6 .
Step 6: estimating the mean risk function and the BPRF
In the final step, step 6, the metaregression model inclusive of the selected bias covariates from step 3 (for example, the highest adjustment level) was used to predict the mean risk function and its 95% UI, which incorporated the uncertainty of the mean effect, between-study heterogeneity and the uncertainty in the heterogeneity estimate accounting for small numbers of studies. Specifically, 1,000 draws were created for each 0.1 level of doses from 0 pack-years to 100 pack-years or cigarette-equivalents smoked per day using the Bayesian metaregression model. The mean of the 1,000 draws was used to estimate the mean risk at each exposure level, and the 25th and 95th draws were used to estimate the 95% UIs for the mean risk at each exposure level.
The BPRF 29 is a conservative estimate of risk function consistent with the available evidence, correcting for both between-study heterogeneity and systemic biases related to study characteristics. The BPRF is defined as either the 5th (if harmful) or 95th (if protective) quantile curve closest to the line of log(RR) of 0, which defines the null (Figs. 1a , 2b , 3a and 4a ). The BPRF represents the smallest harmful (or protective) effect of smoking on the corresponding outcome at each level of exposure that is consistent with the available evidence. A BPRF opposite null from the mean risk function indicates that insufficient evidence is available to reject null, that is, that there may not be an association between risk and outcome. Likewise, the further the BPRF is from null on the same side of null as the mean risk function, the higher the magnitude and evidence for the relationship. The BPRF can be interpreted as indicating that, even accounting for between-study heterogeneity and its uncertainty, the log(RR) across the studied smoking range is at least as high as the BPRF (or at least as low as the BPRF for a protective risk).
To quantify the strength of the evidence, we calculated the ROS for each smoking–outcome association as the signed value of the log(BPRF) averaged between the 15th and 85th percentiles of observed exposure levels for each outcome. The ROS is a single summary of the effect of smoking on the outcome, with higher positive ROSs corresponding to stronger and more consistent evidence and a higher average effect size of smoking and a negative ROS, suggesting that, based on the available evidence, there is no significant effect of smoking on the outcome after accounting for between-study heterogeneity.
For ease of communication, we further classified each smoking–outcome association into a star rating from 1 to 5. Briefly, 1-star associations have an ROS <0, indicating that there is insufficient evidence to find a significant association between smoking and the selected outcome. We divided the positive ROSs into ranges 0.0–0.14 (2-star), >0.14–0.41 (3-star), >0.41–0.62 (4-star) and >0.62 (5-star). These categories correspond to excess risk ranges for harmful risks of 0–15%, >15–50%, >50–85% and >85%. For protective risks, the ranges of exposure-averaged decreases in risk by star rating are 0–13% (2 stars), >13–34% (3 stars), >34–46% (4 stars) and >46% (5 stars).
Among the 36 smoking–outcome pairs analyzed, smoking fracture was the only binary risk–outcome pair, which was due to limited data on the dose–response risk of smoking on fracture 202 . The estimation of binary risk was simplified because the RR was merely a comparison between current smokers and nonsmokers or never smokers. The concept of ROS for continuous risk can naturally extend to binary risk because the BPRF is still defined as the 5th percentile of the effect size accounting for data uncertainty and between-study heterogeneity. However, binary ROSs must be divided by 2 to make them comparable with continuous ROSs, which were calculated by averaging the risk over the range between the 15th and the 85th percentiles of observed exposure levels. Full details about estimating mean risk functions, BPRFs and ROSs for both continuous and binary risk–outcome pairs can be found elsewhere 29 .
Estimating the age-specific risk function for CVD outcomes
For non-CVD outcomes, we assumed that the risk function was the same for all ages and all sexes, except for breast, cervical and prostate cancer, which were assumed to apply only to females or males, respectively. As the risk of smoking on CVD outcomes is known to attenuate with increasing age 203 , 204 , 205 , 206 , we adopted a four-step approach for GBD 2020 to produce age-specific dose–response risk curves for CVD outcomes.
First, we estimated the reference dose–response risk of smoking for each CVD outcome using dose-specific RR data for each outcome regardless of the age group information. This step was identical to that implemented for the other non-CVD outcomes. Once we had generated the reference curve, we determined the age group associated with it by calculating the weighted mean age across all dose-specific RR data (weighted by the reciprocal of the s.e.m. of each datum). For example, if the weighted mean age of all dose-specific RR data was 56.5, we estimated the age group associated with the reference risk curve to be aged 55–59 years. For cohort studies, the age range associated with the RR estimate was calculated as a mean age at baseline plus the mean/median years of follow-up (if only the maximum years of follow-up were reported, we would halve this value and add it to the mean age at baseline). For case–control studies, the age range associated with the OR estimate was simply the reported mean age at baseline (if mean age was not reported, we used the midpoint of the age range instead).
In the third step, we extracted age group-specific RR data and relevant bias covariates from the studies identified in our systematic review approach of age-specific smoking risk on CVD outcomes, and used MR-BRT to model the age pattern of excess risk (that is, RR-1) of smoking on CVD outcomes with age group-specific excess RR data for all CVD outcomes. We modeled the age pattern of smoking risk on CVDs following the same steps we implemented for modeling dose–response risk curves. In the final model, we included a spline on age, random slope on age by study and the bias covariate encoding exposure definition (that is, current, former and ever smokers), which was picked by the variable selection algorithm 28 , 29 . When predicting the age pattern of the excess risk of smoking on CVD outcomes using the fitted model, we did not include between-study heterogeneity to reduce uncertainty in the prediction.
In the fourth step, we calculated the age attenuation factors of excess risk compared with the reference age group for each CVD outcome as the ratio of the estimated excess risk for each age group to the excess risk for the reference age group. We performed the calculation at the draw level to obtain 1,000 draws of the age attenuation factors for each age group. Once we had estimated the age attenuation factors, we carried out the last step, which consisted of adjusting the risk curve for the reference age group from step 1 using equation (1) to produce the age group-specific risk curves for each CVD outcome:
We implemented the age adjustment at the draw level so that the uncertainty of the age attenuation factors could be naturally incorporated into the final adjusted age-specific RR curves. A PRISMA diagram detailing the systematic review approach, a description of the studies included and the full details about the methods are in Supplementary Information 1.5 and 5.2 .
Estimating the theoretical minimum risk exposure level
The theoretical minimum risk exposure level for smoking was 0, that is, no individuals in the population are current or former smokers.
Model validation
The validity of the meta-analytic tool has been extensively evaluated by Zheng and colleagues using simulation experiments 28 , 29 . For the present study, we conducted two additional sensitivity analyses to examine how the shape of the risk curves was impacted by applying a monotonicity constraint and trimming 10% of data. We present the results of these sensitivity analyses in Supplementary Information 6 . In addition to the sensitivity analyses, the dose–response risk estimates were also validated by plotting the mean risk function along with its 95% UI against both the extracted dose-specific RR data from the studies included and our previous dose–response risk estimates from the GBD 2019 (ref. 30 ). The mean risk functions along with the 95% UIs were validated based on data fit and the level, shape and plausibility of the dose–response risk curves. All curves were validated by all authors and reviewed by an external expert panel, comprising professors with relevant experience from universities including Johns Hopkins University, Karolinska Institute and University of Barcelona; senior scientists working in relevant departments at the WHO and the Center for Disease Control and Prevention (CDC) and directors of nongovernmental organizations such as the Campaign for Tobacco-Free Kids.
Statistical analysis
Analyses were carried out using R v.3.6.3, Python v.3.8 and Stata v.16.
Statistics and reproducibility
The study was a secondary analysis of existing data involving systematic reviews and meta-analyses. No statistical method was used to predetermine sample size. As the study did not involve primary data collection, randomization and blinding, data exclusions were not relevant to the present study, and, as such, no data were excluded and we performed no randomization or blinding. We have made our data and code available to foster reproducibility.
Reporting summary
Further information on research design is available in the Nature Research Reporting Summary linked to this article.
Data availability
The findings from the present study are supported by data available in the published literature. Data sources and citations for each risk–outcome pair can be downloaded using the ‘download’ button on each risk curve page currently available at https://vizhub.healthdata.org/burden-of-proof . Study characteristics and citations for all input data used in the analyses are also provided in Supplementary Table 3 , and Supplementary Table 2 provides a template of the data collection form.
Code availability
All code used for these analyses is publicly available online ( https://github.com/ihmeuw-msca/burden-of-proof ).
Doll, R. & Hill, A. B. Smoking and carcinoma of the lung. Br. Med. J. 2 , 739–748 (1950).
Article CAS PubMed PubMed Central Google Scholar
Di Cicco, M. E., Ragazzo, V. & Jacinto, T. Mortality in relation to smoking: the British Doctors Study. Breathe 12 , 275–276 (2016).
Article PubMed PubMed Central Google Scholar
World Health Organization. WHO Framework Convention on Tobacco Control 36 (WHO, 2003).
Dai, X., Gakidou, E. & Lopez, A. D. Evolution of the global smoking epidemic over the past half century: strengthening the evidence base for policy action. Tob. Control 31 , 129–137 (2022).
Article PubMed Google Scholar
Dikshit, R. P. & Kanhere, S. Tobacco habits and risk of lung, oropharyngeal and oral cavity cancer: a population-based case-control study in Bhopal, India. Int. J. Epidemiol. 29 , 609–614 (2000).
Article CAS PubMed Google Scholar
Liaw, K. M. & Chen, C. J. Mortality attributable to cigarette smoking in Taiwan: a 12-year follow-up study. Tob. Control 7 , 141–148 (1998).
Gandini, S. et al. Tobacco smoking and cancer: a meta-analysis. Int. J. Cancer 122 , 155–164 (2008).
Deng, X., Yuan, C. & Chang, D. Interactions between single nucleotide polymorphism of SERPINA1 gene and smoking in association with COPD: a case–control study. Int. J. Chron. Obstruct. Pulmon. Dis. 12 , 259–265 (2017).
Leem, A. Y., Park, B., Kim, Y. S., Jung, J. Y. & Won, S. Incidence and risk of chronic obstructive pulmonary disease in a Korean community-based cohort. Int. J. Chron. Obstruct. Pulmon. Dis. 13 , 509–517 (2018).
Forey, B. A., Thornton, A. J. & Lee, P. N. Systematic review with meta-analysis of the epidemiological evidence relating smoking to COPD, chronic bronchitis and emphysema. BMC Pulmon. Med. 11 , 36 (2011).
Article Google Scholar
Tan, J. et al. Smoking, blood pressure, and cardiovascular disease mortality in a large cohort of Chinese men with 15 years follow-up. Int. J. Environ. Res. Public Health 15 , E1026 (2018).
Doll, R., Peto, R., Boreham, J. & Sutherland, I. Mortality in relation to smoking: 50 years’ observations on male British doctors. Br. Med. J. 328 , 1519 (2004).
Huxley, R. R. & Woodward, M. Cigarette smoking as a risk factor for coronary heart disease in women compared with men: a systematic review and meta-analysis of prospective cohort studies. Lancet 378 , 1297–1305 (2011).
Hbejan, K. Smoking effect on ischemic heart disease in young patients. Heart Views 12 , 1–6 (2011).
Chao, H. et al. A meta-analysis of active smoking and risk of meningioma. Tob. Induc. Dis. 19 , 34 (2021).
Shi, H., Shao, X. & Hong, Y. Association between cigarette smoking and the susceptibility of acute myeloid leukemia: a systematic review and meta-analysis. Eur. Rev. Med Pharm. Sci. 23 , 10049–10057 (2019).
CAS Google Scholar
Macacu, A., Autier, P., Boniol, M. & Boyle, P. Active and passive smoking and risk of breast cancer: a meta-analysis. Breast Cancer Res. Treat. 154 , 213–224 (2015).
Pujades-Rodriguez, M. et al. Heterogeneous associations between smoking and a wide range of initial presentations of cardiovascular disease in 1 937 360 people in England: lifetime risks and implications for risk prediction. Int. J. Epidemiol. 44 , 129–141 (2015).
Kanazir, M. et al. Risk factors for hepatocellular carcinoma: a case-control study in Belgrade (Serbia). Tumori 96 , 911–917 (2010).
Pytynia, K. B. et al. Matched-pair analysis of survival of never smokers and ever smokers with squamous cell carcinoma of the head and neck. J. Clin. Oncol. 22 , 3981–3988 (2004).
Barengo, N. C., Antikainen, R., Harald, K. & Jousilahti, P. Smoking and cancer, cardiovascular and total mortality among older adults: the Finrisk Study. Prev. Med. Rep. 14 , 100875 (2019).
Guo, Y. et al. Modifiable risk factors for cognitive impairment in Parkinson’s disease: a systematic review and meta-analysis of prospective cohort studies. Mov. Disord. 34 , 876–883 (2019).
Aune, D., Vatten, L. J. & Boffetta, P. Tobacco smoking and the risk of gallbladder disease. Eur. J. Epidemiol. 31 , 643–653 (2016).
Qin, L., Deng, H.-Y., Chen, S.-J. & Wei, W. Relationship between cigarette smoking and risk of chronic myeloid leukaemia: a meta-analysis of epidemiological studies. Hematology 22 , 193–200 (2017).
Petrick, J. L. et al. Tobacco, alcohol use and risk of hepatocellular carcinoma and intrahepatic cholangiocarcinoma: the Liver Cancer Pooling Project. Br. J. Cancer 118 , 1005–1012 (2018).
United States Department of Health, Education and Welfare. Smoking and Health. Report of the Advisory Committee on Smoking and Health to the Surgeon General of the United States Public Health Service https://www.cdc.gov/tobacco/data_statistics/sgr/index.htm (US DHEW, 1964).
United States Public Health Service Office of the Surgeon General & National Center for Chronic Disease Prevention and Health Promotion (US) Office on Smoking and Health. Smoking Cessation: A Report of the Surgeon General . (US Department of Health and Human Services, 2020).
Zheng, P., Barber, R., Sorensen, R. J. D., Murray, C. J. L. & Aravkin, A. Y. Trimmed constrained mixed effects models: formulations and algorithms. J. Comput. Graph Stat. 30 , 544–556 (2021).
Zheng, P. et al. The Burden of Proof studies: assessing the evidence of risk. Nat. Med. in press (2022).
Reitsma, M. B. et al. Spatial, temporal, and demographic patterns in prevalence of smoking tobacco use and attributable disease burden in 204 countries and territories, 1990–2019: a systematic analysis from the Global Burden of Disease Study 2019. Lancet 397 , 2337–2360 (2021).
Moher, D., Liberati, A., Tetzlaff, J. & Altman, D. G. Preferred reporting items for systematic reviews and meta-analyses: the PRISMA statement. Br. Med. J. 339 , b2535 (2009).
Liu, Z. Y., He, X. Z. & Chapman, R. S. Smoking and other risk factors for lung cancer in Xuanwei, China. Int. J. Epidemiol. 20 , 26–31 (1991).
Brownson, R. C., Reif, J. S., Keefe, T. J., Ferguson, S. W. & Pritzl, J. A. Risk factors for adenocarcinoma of the lung. Am. J. Epidemiol. 125 , 25–34 (1987).
Marugame, T. et al. Lung cancer death rates by smoking status: comparison of the Three-Prefecture Cohort study in Japan to the Cancer Prevention Study II in the USA. Cancer Sci. 96 , 120–126 (2005).
Dosemeci, M., Gokmen, I., Unsal, M., Hayes, R. B. & Blair, A. Tobacco, alcohol use, and risks of laryngeal and lung cancer by subsite and histologic type in Turkey. Cancer Causes Control 8 , 729–737 (1997).
Freedman, N. D. et al. Impact of changing US cigarette smoking patterns on incident cancer: risks of 20 smoking-related cancers among the women and men of the NIH-AARP cohort. Int. J. Epidemiol. 45 , 846–856 (2016).
Bae, J.-M. et al. Lung cancer incidence by smoking status in Korean men: 16 years of observations in the Seoul Male Cancer Cohort study. J. Korean Med. Sci. 28 , 636–637 (2013).
Everatt, R., Kuzmickienė, I., Virvičiūtė, D. & Tamošiūnas, A. Cigarette smoking, educational level and total and site-specific cancer: a cohort study in men in Lithuania. Eur. J. Cancer Prev. 23 , 579–586 (2014).
Nordlund, L. A., Carstensen, J. M. & Pershagen, G. Are male and female smokers at equal risk of smoking-related cancer: evidence from a Swedish prospective study. Scand. J. Public Health 27 , 56–62 (1999).
Siemiatycki, J., Krewski, D., Franco, E. & Kaiserman, M. Associations between cigarette smoking and each of 21 types of cancer: a multi-site case–control study. Int. J. Epidemiol. 24 , 504–514 (1995).
Chyou, P. H., Nomura, A. M. & Stemmermann, G. N. A prospective study of the attributable risk of cancer due to cigarette smoking. Am. J. Public Health 82 , 37–40 (1992).
Potter, J. D., Sellers, T. A., Folsom, A. R. & McGovern, P. G. Alcohol, beer, and lung cancer in postmenopausal women. The Iowa Women’s Health Study. Ann. Epidemiol. 2 , 587–595 (1992).
Chyou, P. H., Nomura, A. M., Stemmermann, G. N. & Kato, I. Lung cancer: a prospective study of smoking, occupation, and nutrient intake. Arch. Environ. Health 48 , 69–72 (1993).
Pesch, B. et al. Cigarette smoking and lung cancer–relative risk estimates for the major histological types from a pooled analysis of case–control studies. Int. J. Cancer 131 , 1210–1219 (2012).
Jöckel, K. H. et al. Occupational and environmental hazards associated with lung cancer. Int. J. Epidemiol. 21 , 202–213 (1992).
Jöckel, K. H., Ahrens, W., Jahn, I., Pohlabeln, H. & Bolm-Audorff, U. Occupational risk factors for lung cancer: a case-control study in West Germany. Int. J. Epidemiol. 27 , 549–560 (1998).
Lei, Y. X., Cai, W. C., Chen, Y. Z. & Du, Y. X. Some lifestyle factors in human lung cancer: a case-control study of 792 lung cancer cases. Lung Cancer 14 , S121–S136 (1996).
Pawlega, J., Rachtan, J. & Dyba, T. Evaluation of certain risk factors for lung cancer in Cracow (Poland)—a case–control study. Acta Oncol. 36 , 471–476 (1997).
Mao, Y. et al. Socioeconomic status and lung cancer risk in Canada. Int. J. Epidemiol. 30 , 809–817 (2001).
Barbone, F., Bovenzi, M., Cavallieri, F. & Stanta, G. Cigarette smoking and histologic type of lung cancer in men. Chest 112 , 1474–1479 (1997).
Matos, E., Vilensky, M., Boffetta, P. & Kogevinas, M. Lung cancer and smoking: a case–control study in Buenos Aires, Argentina. Lung Cancer 21 , 155–163 (1998).
Simonato, L. et al. Lung cancer and cigarette smoking in Europe: an update of risk estimates and an assessment of inter-country heterogeneity. Int. J. Cancer 91 , 876–887 (2001).
Risch, H. A. et al. Are female smokers at higher risk for lung cancer than male smokers? A case–control analysis by histologic type. Am. J. Epidemiol. 138 , 281–293 (1993).
Sankaranarayanan, R. et al. A case–control study of diet and lung cancer in Kerala, south India. Int. J. Cancer 58 , 644–649 (1994).
Band, P. R. et al. Identification of occupational cancer risks in British Columbia. Part I: methodology, descriptive results, and analysis of cancer risks, by cigarette smoking categories of 15,463 incident cancer cases. J. Occup. Environ. Med. 41 , 224–232 (1999).
Becher, H., Jöckel, K. H., Timm, J., Wichmann, H. E. & Drescher, K. Smoking cessation and nonsmoking intervals: effect of different smoking patterns on lung cancer risk. Cancer Causes Control 2 , 381–387 (1991).
Brockmöller, J., Kerb, R., Drakoulis, N., Nitz, M. & Roots, I. Genotype and phenotype of glutathione S-transferase class mu isoenzymes mu and psi in lung cancer patients and controls. Cancer Res. 53 , 1004–1011 (1993).
PubMed Google Scholar
Vena, J. E., Byers, T. E., Cookfair, D. & Swanson, M. Occupation and lung cancer risk. An analysis by histologic subtypes. Cancer 56 , 910–917 (1985).
Cascorbi, I. et al. Homozygous rapid arylamine N -acetyltransferase (NAT2) genotype as a susceptibility factor for lung cancer. Cancer Res. 56 , 3961–3966 (1996).
CAS PubMed Google Scholar
Chiazze, L., Watkins, D. K. & Fryar, C. A case–control study of malignant and non-malignant respiratory disease among employees of a fiberglass manufacturing facility. Br. J. Ind. Med 49 , 326–331 (1992).
CAS PubMed PubMed Central Google Scholar
Ando, M. et al. Attributable and absolute risk of lung cancer death by smoking status: findings from the Japan Collaborative Cohort Study. Int. J. Cancer 105 , 249–254 (2003).
De Matteis, S. et al. Are women who smoke at higher risk for lung cancer than men who smoke? Am. J. Epidemiol. 177 , 601–612 (2013).
He, Y. et al. Changes in smoking behavior and subsequent mortality risk during a 35-year follow-up of a cohort in Xi’an, China. Am. J. Epidemiol. 179 , 1060–1070 (2014).
Nishino, Y. et al. Cancer incidence profiles in the Miyagi Cohort Study. J. Epidemiol. 14 , S7–S11 (2004).
Papadopoulos, A. et al. Cigarette smoking and lung cancer in women: results of the French ICARE case–control study. Lung Cancer 74 , 369–377 (2011).
Shimazu, T. et al. Alcohol and risk of lung cancer among Japanese men: data from a large-scale population-based cohort study, the JPHC study. Cancer Causes Control 19 , 1095–1102 (2008).
Tindle, H. A. et al. Lifetime smoking history and risk of lung cancer: results from the Framingham Heart Study. J. Natl Cancer Inst. 110 , 1201–1207 (2018).
PubMed PubMed Central Google Scholar
Yong, L. C. et al. Intake of vitamins E, C, and A and risk of lung cancer. The NHANES I epidemiologic followup study. First National Health and Nutrition Examination Survey. Am. J. Epidemiol. 146 , 231–243 (1997).
Hansen, M. S. et al. Sex differences in risk of smoking-associated lung cancer: results from a cohort of 600,000 Norwegians. Am. J. Epidemiol. 187 , 971–981 (2018).
Boffetta, P. et al. Tobacco smoking as a risk factor of bronchioloalveolar carcinoma of the lung: pooled analysis of seven case-control studies in the International Lung Cancer Consortium (ILCCO). Cancer Causes Control 22 , 73–79 (2011).
Yun, Y. D. et al. Hazard ratio of smoking on lung cancer in Korea according to histological type and gender. Lung 194 , 281–289 (2016).
Suzuki, I. et al. Risk factors for lung cancer in Rio de Janeiro, Brazil: a case–control study. Lung Cancer 11 , 179–190 (1994).
De Stefani, E., Deneo-Pellegrini, H., Carzoglio, J. C., Ronco, A. & Mendilaharsu, M. Dietary nitrosodimethylamine and the risk of lung cancer: a case–control study from Uruguay. Cancer Epidemiol. Biomark. Prev. 5 , 679–682 (1996).
Google Scholar
Kreuzer, M. et al. Risk factors for lung cancer in young adults. Am. J. Epidemiol. 147 , 1028–1037 (1998).
Armadans-Gil, L., Vaqué-Rafart, J., Rosselló, J., Olona, M. & Alseda, M. Cigarette smoking and male lung cancer risk with special regard to type of tobacco. Int. J. Epidemiol. 28 , 614–619 (1999).
Kubík, A. K., Zatloukal, P., Tomásek, L. & Petruzelka, L. Lung cancer risk among Czech women: a case–control study. Prev. Med. 34 , 436–444 (2002).
Rachtan, J. Smoking, passive smoking and lung cancer cell types among women in Poland. Lung Cancer 35 , 129–136 (2002).
Thun, M. J. et al. 50-year trends in smoking-related mortality in the United States. N. Engl. J. Med. 368 , 351–364 (2013).
Zatloukal, P., Kubík, A., Pauk, N., Tomásek, L. & Petruzelka, L. Adenocarcinoma of the lung among women: risk associated with smoking, prior lung disease, diet and menstrual and pregnancy history. Lung Cancer 41 , 283–293 (2003).
Hansen, M. S., Licaj, I., Braaten, T., Lund, E. & Gram, I. T. The fraction of lung cancer attributable to smoking in the Norwegian Women and Cancer (NOWAC) Study. Br. J. Cancer 124 , 658–662 (2021).
Zhang, P. et al. Association of smoking and polygenic risk with the incidence of lung cancer: a prospective cohort study. Br. J. Cancer 126 , 1637–1646 (2022).
Weber, M. F. et al. Cancer incidence and cancer death in relation to tobacco smoking in a population-based Australian cohort study. Int. J. Cancer 149 , 1076–1088 (2021).
Guo, L.-W. et al. A risk prediction model for selecting high-risk population for computed tomography lung cancer screening in China. Lung Cancer 163 , 27–34 (2022).
Mezzoiuso, A. G., Odone, A., Signorelli, C. & Russo, A. G. Association between smoking and cancers among women: results from the FRiCaM multisite cohort study. J. Cancer 12 , 3136–3144 (2021).
Hawrysz, I., Wadolowska, L., Slowinska, M. A., Czerwinska, A. & Golota, J. J. Adherence to prudent and mediterranean dietary patterns is inversely associated with lung cancer in moderate but not heavy male Polish smokers: a case–control study. Nutrients 12 , E3788 (2020).
Huang, C.-C., Lai, C.-Y., Tsai, C.-H., Wang, J.-Y. & Wong, R.-H. Combined effects of cigarette smoking, DNA methyltransferase 3B genetic polymorphism, and DNA damage on lung cancer. BMC Cancer 21 , 1066 (2021).
Viner, B., Barberio, A. M., Haig, T. R., Friedenreich, C. M. & Brenner, D. R. The individual and combined effects of alcohol consumption and cigarette smoking on site-specific cancer risk in a prospective cohort of 26,607 adults: results from Alberta’s Tomorrow Project. Cancer Causes Control 30 , 1313–1326 (2019).
Park, E. Y., Lim, M. K., Park, E., Oh, J.-K. & Lee, D.-H. Relationship between urinary 4-(methylnitrosamino)-1-(3-pyridyl)-1-butanol and lung cancer risk in the general population: a community-based prospective cohort study. Front. Oncol. 11 , 611674 (2021).
De Stefani, E., Deneo-Pellegrini, H., Mendilaharsu, M., Carzoglio, J. C. & Ronco, A. Dietary fat and lung cancer: a case–control study in Uruguay. Cancer Causes Control 8 , 913–921 (1997).
Wünsch-Filho, V., Moncau, J. E., Mirabelli, D. & Boffetta, P. Occupational risk factors of lung cancer in São Paulo, Brazil. Scand. J. Work Environ. Health 24 , 118–124 (1998).
Hu, J. et al. A case-control study of diet and lung cancer in northeast China. Int. J. Cancer 71 , 924–931 (1997).
Jia, G., Wen, W., Massion, P. P., Shu, X.-O. & Zheng, W. Incorporating both genetic and tobacco smoking data to identify high-risk smokers for lung cancer screening. Carcinogenesis 42 , 874–879 (2021).
Rusmaully, J. et al. Risk of lung cancer among women in relation to lifetime history of tobacco smoking: a population-based case–control study in France (the WELCA study). BMC Cancer 21 , 711 (2021).
Jin, K. et al. Tobacco smoking modifies the association between hormonal factors and lung cancer occurrence among post-menopausal Chinese women. Transl. Oncol. 12 , 819–827 (2019).
Tse, L. A., Wang, F., Wong, M. C.-S., Au, J. S.-K. & Yu, I. T.-S. Risk assessment and prediction for lung cancer among Hong Kong Chinese men. BMC Cancer 22 , 585 (2022).
Huang, C.-C. et al. Joint effects of cigarette smoking and green tea consumption with miR-29b and DNMT3b mRNA expression in the development of lung cancer. Genes 13 , 836 (2022).
Hosseini, M. et al. Environmental risk factors for lung cancer in Iran: a case–control study. Int. J. Epidemiol. 38 , 989–996 (2009).
Naghibzadeh-Tahami, A. et al. Is opium use associated with an increased risk of lung cancer? A case–control study. BMC Cancer 20 , 807 (2020).
Shimatani, K., Ito, H., Matsuo, K., Tajima, K. & Takezaki, T. Cumulative cigarette tar exposure and lung cancer risk among Japanese smokers. Jpn J. Clin. Oncol. 50 , 1009–1017 (2020).
Lai, C.-Y. et al. Genetic polymorphism of catechol- O -methyltransferase modulates the association of green tea consumption and lung cancer. Eur. J. Cancer Prev. 28 , 316–322 (2019).
Schwartz, A. G. et al. Hormone use, reproductive history, and risk of lung cancer: the Women’s Health Initiative studies. J. Thorac. Oncol. 10 , 1004–1013 (2015).
Kreuzer, M., Gerken, M., Heinrich, J., Kreienbrock, L. & Wichmann, H.-E. Hormonal factors and risk of lung cancer among women? Int. J. Epidemiol. 32 , 263–271 (2003).
Sreeja, L. et al. Possible risk modification by CYP1A1, GSTM1 and GSTT1 gene polymorphisms in lung cancer susceptibility in a South Indian population. J. Hum. Genet. 50 , 618–627 (2005).
Siemiatycki, J. et al. Are the apparent effects of cigarette smoking on lung and bladder cancers due to uncontrolled confounding by occupational exposures? Epidemiology 5 , 57–65 (1994).
Chan-Yeung, M. et al. Risk factors associated with lung cancer in Hong Kong. Lung Cancer 40 , 131–140 (2003).
Lawania, S., Singh, N., Behera, D. & Sharma, S. Xeroderma pigmentosum complementation group D polymorphism toward lung cancer susceptibility survival and response in patients treated with platinum chemotherapy. Future Oncol. 13 , 2645–2665 (2017).
De Stefani, E. et al. Mate drinking and risk of lung cancer in males: a case-control study from Uruguay. Cancer Epidemiol. Biomark. Prev. 5 , 515–519 (1996).
Pérez-Padilla, R. et al. Exposure to biomass smoke and chronic airway disease in Mexican women. A case-control study. Am. J. Respir. Crit. Care Med. 154 , 701–706 (1996).
Zhang, X.-R. et al. Glucosamine use, smoking and risk of incident chronic obstructive pulmonary disease: a large prospective cohort study. Br. J. Nutr . https://doi.org/10.1017/S000711452100372X (2021).
Johannessen, A., Omenaas, E., Bakke, P. & Gulsvik, A. Incidence of GOLD-defined chronic obstructive pulmonary disease in a general adult population. Int. J. Tuberc. Lung Dis. 9 , 926–932 (2005).
Fox, J. Life-style and mortality: a large-scale census-based cohort study in Japan. J. Epidemiol. Community Health 45 , 173 (1991).
Article PubMed Central Google Scholar
Thomson, B. et al. Low-intensity daily smoking and cause-specific mortality in Mexico: prospective study of 150 000 adults. Int. J. Epidemiol. 50 , 955–964 (2021).
van Durme, Y. M. T. A. et al. Prevalence, incidence, and lifetime risk for the development of COPD in the elderly: the Rotterdam study. Chest 135 , 368–377 (2009).
Li, L. et al. SERPINE2 rs16865421 polymorphism is associated with a lower risk of chronic obstructive pulmonary disease in the Uygur population: a case–control study. J. Gene Med. 21 , e3106 (2019).
Ganbold, C. et al. The cumulative effect of gene-gene interactions between GSTM1 , CHRNA3 , CHRNA5 and SOD3 gene polymorphisms combined with smoking on COPD risk. Int. J. Chron. Obstruct. Pulmon. Dis. 16 , 2857–2868 (2021).
Omori, H. et al. Twelve-year cumulative incidence of airflow obstruction among Japanese males. Intern. Med. 50 , 1537–1544 (2011).
Manson, J. E., Ajani, U. A., Liu, S., Nathan, D. M. & Hennekens, C. H. A prospective study of cigarette smoking and the incidence of diabetes mellitus among US male physicians. Am. J. Med. 109 , 538–542 (2000).
Lv, J. et al. Adherence to a healthy lifestyle and the risk of type 2 diabetes in Chinese adults. Int. J. Epidemiol. 46 , 1410–1420 (2017).
Waki, K. et al. Alcohol consumption and other risk factors for self-reported diabetes among middle-aged Japanese: a population-based prospective study in the JPHC study cohort I. Diabet. Med. 22 , 323–331 (2005).
Meisinger, C., Döring, A., Thorand, B. & Löwel, H. Association of cigarette smoking and tar and nicotine intake with development of type 2 diabetes mellitus in men and women from the general population: the MONICA/KORA Augsburg Cohort Study. Diabetologia 49 , 1770–1776 (2006).
Huh, Y. et al. Association of smoking status with the risk of type 2 diabetes among young adults: a nationwide cohort study in South Korea. Nicotine Tob. Res. 24 , 1234–1240 (2022).
Sawada, S. S., Lee, I.-M., Muto, T., Matuszaki, K. & Blair, S. N. Cardiorespiratory fitness and the incidence of type 2 diabetes: prospective study of Japanese men. Diabetes Care 26 , 2918–2922 (2003).
Will, J. C., Galuska, D. A., Ford, E. S., Mokdad, A. & Calle, E. E. Cigarette smoking and diabetes mellitus: evidence of a positive association from a large prospective cohort study. Int. J. Epidemiol. 30 , 540–546 (2001).
Nakanishi, N., Nakamura, K., Matsuo, Y., Suzuki, K. & Tatara, K. Cigarette smoking and risk for impaired fasting glucose and type 2 diabetes in middle-aged Japanese men. Ann. Intern. Med. 133 , 183–191 (2000).
Sairenchi, T. et al. Cigarette smoking and risk of type 2 diabetes mellitus among middle-aged and elderly Japanese men and women. Am. J. Epidemiol. 160 , 158–162 (2004).
Hou, X. et al. Cigarette smoking is associated with a lower prevalence of newly diagnosed diabetes screened by OGTT than non-smoking in Chinese men with normal weight. PLoS ONE 11 , e0149234 (2016).
Hu, F. B. et al. Diet, lifestyle, and the risk of type 2 diabetes mellitus in women. N. Engl. J. Med. 345 , 790–797 (2001).
Teratani, T. et al. Dose-response relationship between tobacco or alcohol consumption and the development of diabetes mellitus in Japanese male workers. Drug Alcohol Depend. 125 , 276–282 (2012).
Kawakami, N., Takatsuka, N., Shimizu, H. & Ishibashi, H. Effects of smoking on the incidence of non-insulin-dependent diabetes mellitus. Replication and extension in a Japanese cohort of male employees. Am. J. Epidemiol. 145 , 103–109 (1997).
Patja, K. et al. Effects of smoking, obesity and physical activity on the risk of type 2 diabetes in middle-aged Finnish men and women. J. Intern. Med. 258 , 356–362 (2005).
White, W. B. et al. High-intensity cigarette smoking is associated with incident diabetes mellitus in Black adults: the Jackson Heart Study. J. Am. Heart Assoc. 7 , e007413 (2018).
Uchimoto, S. et al. Impact of cigarette smoking on the incidence of Type 2 diabetes mellitus in middle-aged Japanese men: the Osaka Health Survey. Diabet. Med . 16 , 951–955 (1999).
Rimm, E. B., Chan, J., Stampfer, M. J., Colditz, G. A. & Willett, W. C. Prospective study of cigarette smoking, alcohol use, and the risk of diabetes in men. Br. Med. J. 310 , 555–559 (1995).
Article CAS Google Scholar
Hilawe, E. H. et al. Smoking and diabetes: is the association mediated by adiponectin, leptin, or C-reactive protein? J. Epidemiol. 25 , 99–109 (2015).
InterAct, Consortium et al. Smoking and long-term risk of type 2 diabetes: the EPIC-InterAct study in European populations. Diabetes Care 37 , 3164–3171 (2014).
Jee, S. H., Foong, A. W., Hur, N. W. & Samet, J. M. Smoking and risk for diabetes incidence and mortality in Korean men and women. Diabetes Care 33 , 2567–2572 (2010).
Rasouli, B. et al. Smoking and the risk of LADA: results from a Swedish population-based case-control study. Diabetes Care 39 , 794–800 (2016).
Wannamethee, S. G., Shaper, A. G. & Perry, I. J., British Regional Heart Study. Smoking as a modifiable risk factor for type 2 diabetes in middle-aged men. Diabetes Care 24 , 1590–1595 (2001).
Radzeviciene, L. & Ostrauskas, R. Smoking habits and type 2 diabetes mellitus in women. Women Health 58 , 884–897 (2018).
Carlsson, S., Midthjell, K. & Grill, V., Nord-Trøndelag Study. Smoking is associated with an increased risk of type 2 diabetes but a decreased risk of autoimmune diabetes in adults: an 11-year follow-up of incidence of diabetes in the Nord-Trøndelag study. Diabetologia 47 , 1953–1956 (2004).
Akter, S. et al. Smoking, smoking cessation, and the risk of type 2 diabetes among Japanese adults: Japan Epidemiology Collaboration on Occupational Health Study. PLoS ONE 10 , e0132166 (2015).
Pirie, K. et al. The 21st century hazards of smoking and benefits of stopping: a prospective study of one million women in the UK. Lancet 381 , 133–141 (2013).
Park, C.-H. et al. [The effect of smoking status upon occurrence of impaired fasting glucose or type 2 diabetes in Korean men]. J. Prev. Med. Public Health 41 , 249–254 (2008).
Doi, Y. et al. Two risk score models for predicting incident Type 2 diabetes in Japan. Diabet. Med. 29 , 107–114 (2012).
van den Brandt, P. A. A possible dual effect of cigarette smoking on the risk of postmenopausal breast cancer. Eur. J. Epidemiol. 32 , 683–690 (2017).
Dossus, L. et al. Active and passive cigarette smoking and breast cancer risk: results from the EPIC cohort. Int. J. Cancer 134 , 1871–1888 (2014).
Kawai, M., Malone, K. E., Tang, M.-T. C. & Li, C. I. Active smoking and the risk of estrogen receptor-positive and triple-negative breast cancer among women ages 20 to 44 years. Cancer 120 , 1026–1034 (2014).
Reynolds, P. et al. Active smoking, household passive smoking, and breast cancer: evidence from the California Teachers Study. J. Natl Cancer Inst. 96 , 29–37 (2004).
Ellingjord-Dale, M. et al. Alcohol, physical activity, smoking, and breast cancer subtypes in a large, nested case-control study from the Norwegian Breast Cancer Screening Program. Cancer Epidemiol. Biomark. Prev. 26 , 1736–1744 (2017).
Arthur, R. et al. Association between lifestyle, menstrual/reproductive history, and histological factors and risk of breast cancer in women biopsied for benign breast disease. Breast Cancer Res. Treat. 165 , 623–631 (2017).
Luo, J. et al. Association of active and passive smoking with risk of breast cancer among postmenopausal women: a prospective cohort study. Br. Med. J. 342 , d1016 (2011).
White, A. J., D’Aloisio, A. A., Nichols, H. B., DeRoo, L. A. & Sandler, D. P. Breast cancer and exposure to tobacco smoke during potential windows of susceptibility. Cancer Causes Control 28 , 667–675 (2017).
Gram, I. T. et al. Breast cancer risk among women who start smoking as teenagers. Cancer Epidemiol. Biomark. Prev. 14 , 61–66 (2005).
Gammon, M. D. et al. Cigarette smoking and breast cancer risk among young women (United States). Cancer Causes Control 9 , 583–590 (1998).
Magnusson, C., Wedrén, S. & Rosenberg, L. U. Cigarette smoking and breast cancer risk: a population-based study in Sweden. Br. J. Cancer 97 , 1287–1290 (2007).
Chu, S. Y. et al. Cigarette smoking and the risk of breast cancer. Am. J. Epidemiol. 131 , 244–253 (1990).
Lemogne, C. et al. Depression and the risk of cancer: a 15-year follow-up study of the GAZEL cohort. Am. J. Epidemiol. 178 , 1712–1720 (2013).
Morabia, A., Bernstein, M., Héritier, S. & Khatchatrian, N. Relation of breast cancer with passive and active exposure to tobacco smoke. Am. J. Epidemiol. 143 , 918–928 (1996).
Conlon, M. S. C., Johnson, K. C., Bewick, M. A., Lafrenie, R. M. & Donner, A. Smoking (active and passive), N -acetyltransferase 2, and risk of breast cancer. Cancer Epidemiol. 34 , 142–149 (2010).
Ozasa, K., Japan Collaborative Cohort Study for Evaluation of Cancer. Smoking and mortality in the Japan Collaborative Cohort Study for Evaluation of Cancer (JACC). Asian Pac. J. Cancer Prev. 8 , 89–96 (2007).
Jones, M. E., Schoemaker, M. J., Wright, L. B., Ashworth, A. & Swerdlow, A. J. Smoking and risk of breast cancer in the Generations Study cohort. Breast Cancer Res. 19 , 118 (2017).
Bjerkaas, E. et al. Smoking duration before first childbirth: an emerging risk factor for breast cancer? Results from 302,865 Norwegian women. Cancer Causes Control 24 , 1347–1356 (2013).
Gram, I. T., Little, M. A., Lund, E. & Braaten, T. The fraction of breast cancer attributable to smoking: the Norwegian women and cancer study 1991–2012. Br. J. Cancer 115 , 616–623 (2016).
Li, C. I., Malone, K. E. & Daling, J. R. The relationship between various measures of cigarette smoking and risk of breast cancer among older women 65–79 years of age (United States). Cancer Causes Control 16 , 975–985 (2005).
Xue, F., Willett, W. C., Rosner, B. A., Hankinson, S. E. & Michels, K. B. Cigarette smoking and the incidence of breast cancer. Arch. Intern. Med. 171 , 125–133 (2011).
Parker, A. S., Cerhan, J. R., Putnam, S. D., Cantor, K. P. & Lynch, C. F. A cohort study of farming and risk of prostate cancer in Iowa. Epidemiology 10 , 452–455 (1999).
Sawada, N. et al. Alcohol and smoking and subsequent risk of prostate cancer in Japanese men: the Japan Public Health Center-based prospective study. Int. J. Cancer 134 , 971–978 (2014).
Hiatt, R. A., Armstrong, M. A., Klatsky, A. L. & Sidney, S. Alcohol consumption, smoking, and other risk factors and prostate cancer in a large health plan cohort in California (United States). Cancer Causes Control 5 , 66–72 (1994).
Cerhan, J. R. et al. Association of smoking, body mass, and physical activity with risk of prostate cancer in the Iowa 65+ Rural Health Study (United States). Cancer Causes Control 8 , 229–238 (1997).
Watters, J. L., Park, Y., Hollenbeck, A., Schatzkin, A. & Albanes, D. Cigarette smoking and prostate cancer in a prospective US cohort study. Cancer Epidemiol. Biomark. Prev. 18 , 2427–2435 (2009).
Butler, L. M., Wang, R., Wong, A. S., Koh, W.-P. & Yu, M. C. Cigarette smoking and risk of prostate cancer among Singapore Chinese. Cancer Causes Control 20 , 1967–1974 (2009).
Lotufo, P. A., Lee, I. M., Ajani, U. A., Hennekens, C. H. & Manson, J. E. Cigarette smoking and risk of prostate cancer in the physicians’ health study (United States). Int. J. Cancer 87 , 141–144 (2000).
Hsing, A. W. et al. Diet, tobacco use, and fatal prostate cancer: results from the Lutheran Brotherhood Cohort Study. Cancer Res. 50 , 6836–6840 (1990).
Veierød, M. B., Laake, P. & Thelle, D. S. Dietary fat intake and risk of prostate cancer: a prospective study of 25,708 Norwegian men. Int. J. Cancer 73 , 634–638 (1997).
Meyer, J., Rohrmann, S., Bopp, M. & Faeh, D. & Swiss National Cohort Study Group. Impact of smoking and excess body weight on overall and site-specific cancer mortality risk. Cancer Epidemiol. Biomark. Prev . 24 , 1516–1522 (2015).
Putnam, S. D. et al. Lifestyle and anthropometric risk factors for prostate cancer in a cohort of Iowa men. Ann. Epidemiol. 10 , 361–369 (2000).
Taghizadeh, N., Vonk, J. M. & Boezen, H. M. Lifetime smoking history and cause-specific mortality in a cohort study with 43 years of follow-up. PLoS ONE 11 , e0153310 (2016).
Park, S.-Y. et al. Racial/ethnic differences in lifestyle-related factors and prostate cancer risk: the Multiethnic Cohort Study. Cancer Causes Control 26 , 1507–1515 (2015).
Nomura, A. M., Lee, J., Stemmermann, G. N. & Combs, G. F. Serum selenium and subsequent risk of prostate cancer. Cancer Epidemiol. Biomark. Prev. 9 , 883–887 (2000).
Rodriguez, C., Tatham, L. M., Thun, M. J., Calle, E. E. & Heath, C. W. Smoking and fatal prostate cancer in a large cohort of adult men. Am. J. Epidemiol. 145 , 466–475 (1997).
Rohrmann, S. et al. Smoking and risk of fatal prostate cancer in a prospective U.S. study. Urology 69 , 721–725 (2007).
Giovannucci, E. et al. Smoking and risk of total and fatal prostate cancer in United States health professionals. Cancer Epidemiol. Biomark. Prev. 8 , 277–282 (1999).
Rohrmann, S. et al. Smoking and the risk of prostate cancer in the European Prospective Investigation into Cancer and Nutrition. Br. J. Cancer 108 , 708–714 (2013).
Lund Nilsen, T. I., Johnsen, R. & Vatten, L. J. Socio-economic and lifestyle factors associated with the risk of prostate cancer. Br. J. Cancer 82 , 1358–1363 (2000).
Hsing, A. W., McLaughlin, J. K., Hrubec, Z., Blot, W. J. & Fraumeni, J. F. Tobacco use and prostate cancer: 26-year follow-up of US veterans. Am. J. Epidemiol. 133 , 437–441 (1991).
Murray, C. J. L. et al. Global burden of 87 risk factors in 204 countries and territories, 1990–2019: a systematic analysis for the Global Burden of Disease Study 2019. Lancet 396 , 1223–1249 (2020).
Bero, L. A. & Jadad, A. R. How consumers and policymakers can use systematic reviews for decision making. Ann. Intern. Med. 127 , 37–42 (1997).
Centers for Disease Control and Prevention (CDC). Cigarette smoking among adults and trends in smoking cessation—United States, 2008. MMWR Morb. Mortal. Wkly Rep. 58 , 1227–1232 (2009).
Prochaska, J. O. & Goldstein, M. G. Process of smoking cessation: implications for clinicians. Clin. Chest Med. 12 , 727–735 (1991).
Page, M. J. et al. The PRISMA 2020 statement: an updated guideline for reporting systematic reviews. Br. Med. J. 372 , n71 (2021).
Stevens, G. A. et al. Guidelines for Accurate and Transparent Health Estimates Reporting: the GATHER statement. Lancet 388 , e19–e23 (2016).
BMJ Best Practice. What is GRADE? https://bestpractice.bmj.com/info/us/toolkit/learn-ebm/what-is-grade (BMJ, 2021).
The GRADE Working Group. GRADE handbook . https://gdt.gradepro.org/app/handbook/handbook.html (The GRADE Working Group, 2013).
Efron, B., Hastie, T., Johnstone, I. & Tibshirani, R. Least angle regression. Ann. Stat. 32 , 407–499 (2004).
Tibshirani, R. Regression shrinkage and selection via the lasso. J. R. Stat. Soc. Ser. B Stat. Methodol. 58 , 267–288 (1996).
von Hippel, P. T. The heterogeneity statistic I2 can be biased in small meta-analyses. BMC Med. Res. Methodol. 15 , 35 (2015).
Kontopantelis, E., Springate, D. A. & Reeves, D. A re-analysis of the Cochrane Library data: the dangers of unobserved heterogeneity in meta-analyses. PLoS ONE 8 , e69930 (2013).
Biggerstaff, B. J. & Tweedie, R. L. Incorporating variability in estimates of heterogeneity in the random effects model in meta-analysis. Stat. Med. 16 , 753–768 (1997).
Egger, M., Smith, G. D., Schneider, M. & Minder, C. Bias in meta-analysis detected by a simple, graphical test. Br. Med. J. 315 , 629–634 (1997).
Lee, P. N., Forey, B. A. & Coombs, K. J. Systematic review with meta-analysis of the epidemiological evidence in the 1900s relating smoking to lung cancer. BMC Cancer 12 , 385 (2012).
Rücker, G., Carpenter, J. R. & Schwarzer, G. Detecting and adjusting for small-study effects in meta-analysis. Biometr. J. 53 , 351–368 (2011).
Wu, Z.-J., Zhao, P., Liu, B. & Yuan, Z.-C. Effect of cigarette smoking on risk of hip fracture in men: a meta-analysis of 14 prospective cohort studies. PLoS ONE 11 , e0168990 (2016).
Thun, M. J. et al. in Cigarette Smoking Behaviour in the United States: changes in cigarette-related disease risks and their implication for prevention and control (eds Burns, D.M. et al.) Tobacco Control Monograph No. 8 Ch. 4 (National Cancer Institute, 1997).
Tolstrup, J. S. et al. Smoking and risk of coronary heart disease in younger, middle-aged, and older adults. Am. J. Public Health 104 , 96–102 (2014).
Jonas, M. A., Oates, J. A., Ockene, J. K. & Hennekens, C. H. Statement on smoking and cardiovascular disease for health care professionals. American Heart Association. Circulation 86 , 1664–1669 (1992).
Khan, S. S. et al. Cigarette smoking and competing risks for fatal and nonfatal cardiovascular disease subtypes across the life course. J. Am. Heart Assoc. 10 , e021751 (2021).
Download references
Acknowledgements
Research reported in this publication was supported by the Bill & Melinda Gates Foundation and Bloomberg Philanthropies. The content is solely the responsibility of the authors and does not necessarily represent the official views of the funders. The study funders had no role in study design, data collection, data analysis, data interpretation, writing of the final report or the decision to publish.
We thank the Tobacco Metrics Team Advisory Group for their valuable input and review of the work. The members of the Advisory Group are: P. Allebeck, R. Chandora, J. Drope, M. Eriksen, E. Fernández, H. Gouda, R. Kennedy, D. McGoldrick, L. Pan, K. Schotte, E. Sebrie, J. Soriano, M. Tynan and K. Welding.
Author information
Authors and affiliations.
Institute for Health Metrics and Evaluation, University of Washington, Seattle, WA, USA
Xiaochen Dai, Gabriela F. Gil, Marissa B. Reitsma, Noah S. Ahmad, Jason A. Anderson, Catherine Bisignano, Sinclair Carr, Rachel Feldman, Simon I. Hay, Jiawei He, Vincent Iannucci, Hilary R. Lawlor, Matthew J. Malloy, Laurie B. Marczak, Susan A. McLaughlin, Larissa Morikawa, Erin C. Mullany, Sneha I. Nicholson, Erin M. O’Connell, Chukwuma Okereke, Reed J. D. Sorensen, Joanna Whisnant, Aleksandr Y. Aravkin, Peng Zheng, Christopher J. L. Murray & Emmanuela Gakidou
Department of Health Metrics Sciences, School of Medicine, University of Washington, Seattle, WA, USA
Xiaochen Dai, Simon I. Hay, Jiawei He, Peng Zheng, Christopher J. L. Murray & Emmanuela Gakidou
Department of Applied Mathematics, University of Washington, Seattle, WA, USA
Aleksandr Y. Aravkin
You can also search for this author in PubMed Google Scholar
Contributions
X.D., S.I.H., S.A.M., E.C.M., E.M.O., C.J.L.M. and E.G. managed the estimation or publications process. X.D. and G.F.G. wrote the first draft of the manuscript. X.D. and P.Z. had primary responsibility for applying analytical methods to produce estimates. X.D., G.F.G., N.S.A., J.A.A., S.C., R.F., V.I., M.J.M., L.M., S.I.N., C.O., M.B.R. and J.W. had primary responsibility for seeking, cataloguing, extracting or cleaning data, and for designing or coding figures and tables. X.D., G.F.G., M.B.R., N.S.A., H.R.L., C.O. and J.W. provided data or critical feedback on data sources. X.D., J.H., R.J.D.S., A.Y.A., P.Z., C.J.L.M. and E.G. developed methods or computational machinery. X.D., G.F.G., M.B.R., S.I.H., J.H., R.J.D.S., A.Y.A., P.Z., C.J.L.M. and E.G. provided critical feedback on methods or results. X.D., G.F.G., M.B.R., C.B., S.I.H., L.B.M., S.A.M., A.Y.A. and E.G. drafted the work or revised it critically for important intellectual content. X.D., S.I.H., L.B.M., E.C.M., E.M.O. and E.G. managed the overall research enterprise.
Corresponding author
Correspondence to Xiaochen Dai .
Ethics declarations
Competing interests.
The authors declare no competing interests.
Peer review
Peer review information.
Nature Medicine thanks Frederic Sitas and the other, anonymous, reviewer(s) for their contribution to the peer review of this work. Primary Handling Editors: Jennifer Sargent and Ming Yang, in collaboration with the Nature Medicine team.
Additional information
Publisher’s note Springer Nature remains neutral with regard to jurisdictional claims in published maps and institutional affiliations.
Extended data
Extended data fig. 1 prisma 2020 flow diagram for an updated systematic review of the smoking and tracheal, bronchus, and lung cancer risk-outcome pair..
The PRISMA flow diagram of an updated systematic review on the relationship between smoking and lung cancer conducted on PubMed to update historical review from previous cycles of the Global Burden of Disease Study. Template is from: Page MJ, McKenzie JE, Bossuyt PM, Boutron I, Hoffmann TC, Mulrow CD, et al. The PRISMA 2020 statement: an updated guideline for reporting systematic reviews. BMJ 2021;372:n71. doi: 10.1136/bmj.n71. For more information, visit: http://www.prisma-statement.org/ .
Extended Data Fig. 2 PRISMA 2020 flow diagram for an updated systematic review of the Smoking and Chronic obstructive pulmonary disease risk-outcome pair.
The PRISMA flow diagram of an updated systematic review on the relationship between smoking and chronic obstructive pulmonary disease conducted on PubMed to update historical review from previous cycles of the Global Burden of Disease Study. Template is from: Page MJ, McKenzie JE, Bossuyt PM, Boutron I, Hoffmann TC, Mulrow CD, et al. The PRISMA 2020 statement: an updated guideline for reporting systematic reviews. BMJ 2021;372:n71. doi: 10.1136/bmj.n71. For more information, visit: http://www.prisma-statement.org/ .
Extended Data Fig. 3 PRISMA 2020 flow diagram for an updated systematic review of the Smoking and Diabetes mellitus type 2 risk- outcome pair.
The PRISMA flow diagram of an updated systematic review on the relationship between smoking and type 2 diabetes conducted on PubMed to update historical review from previous cycles of the Global Burden of Disease Study. Template is from: Page MJ, McKenzie JE, Bossuyt PM, Boutron I, Hoffmann TC, Mulrow CD, et al. The PRISMA 2020 statement: an updated guideline for reporting systematic reviews. BMJ 2021;372:n71. doi: 10.1136/bmj.n71. For more information, visit: http://www.prisma-statement.org/ .
Extended Data Fig. 4 PRISMA 2020 flow diagram for an updated systematic review of the Smoking and Breast cancer risk-outcome pair.
The PRISMA flow diagram of an updated systematic review on the relationship between smoking and breast cancer conducted on PubMed to update historical review from previous cycles of the Global Burden of Disease Study. Template is from: Page MJ, McKenzie JE, Bossuyt PM, Boutron I, Hoffmann TC, Mulrow CD, et al. The PRISMA 2020 statement: an updated guideline for reporting systematic reviews. BMJ 2021;372:n71. doi: 10.1136/bmj.n71. For more information, visit: http://www.prisma-statement.org/ .
Extended Data Fig. 5 PRISMA 2020 flow diagram for an updated systematic review of the Smoking and Prostate cancer risk-outcome pair.
The PRISMA flow diagram of an updated systematic review on the relationship between smoking and prostate cancer conducted on PubMed to update historical review from previous cycles of the Global Burden of Disease Study. Template is from: Page MJ, McKenzie JE, Bossuyt PM, Boutron I, Hoffmann TC, Mulrow CD, et al. The PRISMA 2020 statement: an updated guideline for reporting systematic reviews. BMJ 2021;372:n71. doi: 10.1136/bmj.n71. For more information, visit: http://www.prisma-statement.org/ .
Extended Data Fig. 6 Smoking and Breast Cancer.
a , log-relative risk function. b , relative risk function. c , A modified funnel plot showing the residuals (relative to 0) on the x-axis and the estimated standard deviation (SD) that includes reported SD and between-study heterogeneity on the y-axis.
Supplementary information
Supplementary information.
Supplementary Information 1: Data source identification and assessment. Supplementary Information 2: Data inputs. Supplementary Information 3: Study quality and bias assessment. Supplementary Information 4: The dose–response RR curves and their 95% UIs for all smoking–outcome pairs. Supplementary Information 5: Supplementary methods. Supplementary Information 6: Sensitivity analysis. Supplementary Information 7: Binary smoking–outcome pair. Supplementary Information 8: Risk curve details. Supplementary Information 9: GATHER and PRISMA checklists.
Rights and permissions
Open Access This article is licensed under a Creative Commons Attribution 4.0 International License, which permits use, sharing, adaptation, distribution and reproduction in any medium or format, as long as you give appropriate credit to the original author(s) and the source, provide a link to the Creative Commons license, and indicate if changes were made. The images or other third party material in this article are included in the article’s Creative Commons license, unless indicated otherwise in a credit line to the material. If material is not included in the article’s Creative Commons license and your intended use is not permitted by statutory regulation or exceeds the permitted use, you will need to obtain permission directly from the copyright holder. To view a copy of this license, visit http://creativecommons.org/licenses/by/4.0/ .
Reprints and permissions
About this article
Cite this article.
Dai, X., Gil, G.F., Reitsma, M.B. et al. Health effects associated with smoking: a Burden of Proof study. Nat Med 28 , 2045–2055 (2022). https://doi.org/10.1038/s41591-022-01978-x
Download citation
Received : 11 April 2022
Accepted : 28 July 2022
Published : 10 October 2022
Issue Date : October 2022
DOI : https://doi.org/10.1038/s41591-022-01978-x
Share this article
Anyone you share the following link with will be able to read this content:
Sorry, a shareable link is not currently available for this article.
Provided by the Springer Nature SharedIt content-sharing initiative
This article is cited by
Estimating effects of whole grain consumption on type 2 diabetes, colorectal cancer and cardiovascular disease: a burden of proof study.
Nutrition Journal (2024)
- Gabriela F. Gil
- Jason A. Anderson
- Emmanuela Gakidou
Nature Communications (2024)
A multi-ancestry cerebral cortex transcriptome-wide association study identifies genes associated with smoking behaviors
- Xiaohang Xu
Molecular Psychiatry (2024)
- Luisa S. Flor
Nature Medicine (2024)
Metabolic profiling of smoking, associations with type 2 diabetes and interaction with genetic susceptibility
- Sofia Carlsson
European Journal of Epidemiology (2024)
Quick links
- Explore articles by subject
- Guide to authors
- Editorial policies
Sign up for the Nature Briefing: Cancer newsletter — what matters in cancer research, free to your inbox weekly.

Click through the PLOS taxonomy to find articles in your field.
For more information about PLOS Subject Areas, click here .
Loading metrics
Open Access
Peer-reviewed
Research Article
The impact of peer pressure on cigarette smoking among high school and university students in Ethiopia: A systemic review and meta-analysis
Roles Conceptualization, Data curation, Methodology, Software, Writing – review & editing
* E-mail: [email protected]
Affiliation College of Health Sciences, Debre Markos University, Debre Markos, Ethiopia

Roles Formal analysis, Resources, Supervision
Roles Data curation, Formal analysis, Investigation, Methodology, Validation
Roles Data curation, Formal analysis, Project administration, Software, Supervision
Roles Formal analysis, Visualization, Writing – original draft
Roles Data curation, Methodology, Software, Validation, Visualization, Writing – original draft, Writing – review & editing
Affiliation Department of Nursing, College of Nursing, University of Saskatchewan, Regina, Canada
Roles Formal analysis, Funding acquisition, Investigation, Methodology, Project administration, Validation, Visualization
Roles Methodology, Project administration, Resources, Software, Supervision, Validation, Visualization
Roles Data curation, Investigation, Resources, Validation, Visualization, Writing – original draft, Writing – review & editing
Roles Investigation, Project administration, Software, Supervision, Validation, Writing – review & editing
Roles Software, Supervision, Validation, Visualization, Writing – original draft, Writing – review & editing
Roles Supervision, Validation, Visualization, Writing – original draft, Writing – review & editing
Roles Methodology, Supervision, Writing – review & editing
Affiliations Colleges of Nursing, University of Saskatchewan, Saskatoon, Canada, School of Life Sciences and Bioengineering, Nelson Mandela African Institute of Science and Technology, Arusha City, Tanzania
Roles Methodology, Software, Supervision, Visualization, Writing – original draft, Writing – review & editing
Affiliations School of Science and Health, Western Sydney University, Penrith, NSW, Australia, Translational Health Research Institute, Western Sydney University, Penrith, NSW, Australia, Discipline of Child and Adolescent Health, Sydney Medical School, Faculty of Medicine and Health, The University of Sydney, Westmead, NSW, Australia, Oral Health Services, Sydney Local Health District and Sydney Dental Hospital, NSW Health, Surry Hills, NSW, Australia
- Cheru Tesema Leshargie,
- Animut Alebel,
- Getiye Dejenu Kibret,
- Molla Yigzaw Birhanu,
- Henok Mulugeta,
- Patricia Malloy,
- Fasil Wagnew,
- Atsede Alle Ewunetie,
- Daniel Bekele Ketema,
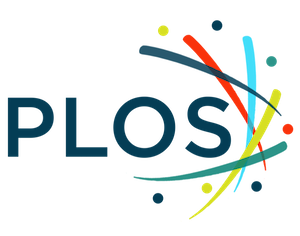
- Published: October 11, 2019
- https://doi.org/10.1371/journal.pone.0222572
- Reader Comments
Cigarettes and their by-products (i.e., smoke; ash) are a complex, dynamic, and reactive mixture of around 5,000 chemicals. Cigarette smoking potentially harms nearly every organ of the human body, causes innumerable diseases, and impacts the health of smokers and those interacting with the smokers. Smoking brings greater health problems in the long-term like increased risk of stroke and brain damage. For students, peer pressure is one of the key factors contributing to cigarette smoking. Therefore, this systematic review and meta-analysis assessed the impact of peer pressure on cigarette smoking among high school and university students in Ethiopia.
An extensive search of key databases including Cochrane Library, PubMed, Google Scholar, Hinari, Embase and Science Direct was conducted to identify and access articles published on the prevalence of cigarette smoking by high school and university students in Ethiopia. The search period for articles was conducted from 21 st September, 2018 to 25 th December 25, 2018. All necessary data were extracted using a standardized data extraction checklist. Quality and risk of bias of studies were assessed using standardized tools. Heterogeneity between the included studies was assessed using Cochrane Q-test statistic and I 2 test. To estimate the pooled prevalence of cigarette smoking, a random effects model was fitted. The impact of peer pressure on cigarette smoking was determined and was reported in Odds Ratio (OR) with 95% Confidence Interval (CI). Meta-analysis was conducted using Stata software.
From 175 searched articles, 19 studies fulfilled the eligibility criteria and were included in this study. The pooled prevalence of cigarette smoking among Ethiopian high school and university students was 15.9% (95% CI: 12.21, 19.63). Slightly higher prevalence of cigarette smoking was noted among university students [17.35% (95% CI: 13.21, 21.49)] as compared to high school students [12.77% (95% CI: 6.72%, 18.82%)]. The current aggregated meta-analysis revealed that peer pressure had a significant influence on cigarette smoking (OR: 2.68 (95% CI: 2.37, 3.03).
More than one sixth of the high school and university students in Ethiopia smoke cigarette. Students who had peer pressure from their friends were more likely to smoke cigarette. Therefore, school-based intervention programs are needed to reduce the high prevalence of cigarette smoking among students in Ethiopia.
Citation: Leshargie CT, Alebel A, Kibret GD, Birhanu MY, Mulugeta H, Malloy P, et al. (2019) The impact of peer pressure on cigarette smoking among high school and university students in Ethiopia: A systemic review and meta-analysis. PLoS ONE 14(10): e0222572. https://doi.org/10.1371/journal.pone.0222572
Editor: Wisit Cheungpasitporn, University of Mississippi Medical Center, UNITED STATES
Received: March 15, 2019; Accepted: September 3, 2019; Published: October 11, 2019
Copyright: © 2019 Leshargie et al. This is an open access article distributed under the terms of the Creative Commons Attribution License , which permits unrestricted use, distribution, and reproduction in any medium, provided the original author and source are credited.
Data Availability: All relevant data are within the manuscript and its Supporting Information files.
Funding: The authors received no specific funding for this work.
Competing interests: The authors have declared that no competing interests exist.
Abbreviations: CI, Confidence Interval; HIV, Human Immune Deficiency Virus; OR, Odd Ration; PRISMA, Preferred Reporting Items for Systematic Reviews and Meta-Analyses; SE, Standard Error; SNNPR, South Nation and Nationalities People of the Region; RR, Relative Risk; WHO, World Health Organization
Introduction
Smoking cigarettes yields a complex, dynamic and reactive mixture of around 5,000 chemicals [ 1 – 3 ]. Globally, it is one of the leading preventable causes of respiratory tract complications, disability, and early deaths related to complications [ 4 – 7 ]. It accounts for six of the eight leading causes of morbidity and mortality [ 5 ]. Essentially, it is a legal drug that kills many of its users when used exactly as intended by manufacturers. Currently, the World Health Organization (WHO) estimates that the use of both smoking and smokeless tobacco account for around 6 million deaths worldwide annually, of which 600,000 deaths were among non-smokers due to exposure to the smoke [ 8 ]. More than 30% of world’s adult population are consumers of tobacco, which leads to a warning that a billion people will die of adverse health effects related to the tobacco epidemic within the 21st century unless effective preventative measures are undertaken [ 3 ].
Smoking affects almost every organ in the human body (such as circulatory, respiratory, gastrointestinal and musculoskeletal systems), increases the risk for several diseases, and reduces the health of smokers in general [ 9 , 10 ]. The key effect of smoking cigarettes is primarily on the lungs with approximately 85% of chronic obstructive pulmonary disease (COPD) and lung cancer and about 33% of other cancers (i.e., esophagus, oral cavity, uterus, stomach, and pancreas) related to smoking [ 9 – 11 ].
Normal adolescent developmental stage is affected by high level of peer pressure that can influence risk-taking behaviors including substance use [ 12 ]. Globally, especially in low- and middle-income countries, an estimated 80% of the one billion adolescent smokers are suffering from tobacco-related morbidity and mortality [ 7 ]. Cigarette smoking negatively influences the physical and mental health of an individual [ 13 ]. This is particularly true for high school and university students who already face major health challenges such as stress [ 14 ]. Smoking is also associated with poor educational performance, high-risk drinking behavior, illegal drug use, and high-risk sexual behaviors [ 14 , 15 ]. Peer pressure is widely recognized as a crucial factor affecting young people's early experimentation with tobacco and their willingness to continue smoking [ 16 ]. Several students attending higher education institutions practice cigarette smoking for several reasons, such as a way to cope with stress [ 17 ]. Factors that contribute to the continued use of tobacco include being male, drinking alcohol, having a friend who drinks alcohol, having a friend who smokes, having family members who smoke and being older in age, to mention some [ 18 ].
In sub-Saharan Africa, the prevalence of smoking is increasing and is projected to continue to increase [ 19 , 20 ]. The current data in the region reveals substantial variation in smoking rates among countries ranging from 1.8% in Zambia to 25.8% in Sierra Leone [ 21 ]. In Ethiopia, cigarette smoking is among one of the most commonly used substances, which leads to addiction [ 22 ]. It has deleterious effects on the health of the young users, significantly reduces academic performance in students and increases risk of contracting HIV and other sexually transmitted diseases. Several primary studies on the prevalence and associated factors of cigarette smoking among high school and university students have been conducted in Ethiopia [ 23 – 37 ]. According to earlier reviews of the literature, prevalence of smoking in Ethiopia ranges from 2.99% in Addis Ababa [ 38 ] to 28.6% in Hawassa and Jima University [ 30 ]. Therefore, this systematic review and meta-analysis aimed to review the pooled prevalence of cigarette smoking among high school and university students in Ethiopia and the impact of peer pressure on cigarette smoking among high school and university students in Ethiopia.
Method and materials
This systematic review is based on the Preferred Reporting Items of Systematic Reviews and Meta-Analysis (PRISMA) checklist guidelines to ensure scientific rigor [ 39 ] ( S1 Table ). Prospective registration of systematic review and meta-analysis promotes transparency, helps reduce potential for bias, and improves review’s credibility. However, this meta-analysis and systematic review was not registered on the prosperous, and we have acknowledged this gap in the limitation section.
This systematic review and meta-analysis reports data from Ethiopia. Ethiopia is located in the north-eastern part of the African continent or what is known as the “Horn of Africa”. The country is divided into nine regional states and two administrative cities [ 40 ] containing a total of 108,386,391 million population with a national density of 94 people per square kilometer, 2019 [ 41 ]. Ethiopia shares land borders with five countries: Sudan , Somalia , Djibouti , Eritrea , and Kenya [ 42 ].
Inclusion and exclusion criteria
Eligibility criteria..
This systematic review and meta-analysis included studies only conducted in Ethiopia that assessed the prevalence of cigarette smoking. Published articles were reviewed and rated for inclusion. Full articles were retrieved if a specific outcome of interest (smoking status) was defined. This review included all observational study designs (cross-sectional studies, case-control studies, and cohort studies). However, case reports or case series, duplicate reports, and inconsistent outcome measures were excluded. Moreover, we excluded articles that were published in a language other than English. Documents that were not accessible after contacting the principal investigator three times by email were also excluded. Articles that reported measures other than Relative Risk (RR) or equivalent values, or from which an Odds Ratio (OR) could not be calculated were also excluded from consideration, The eligibility criteria for each individual article were checked by three authors independently (CT, AA1, and AA2). If there was a disagreement between the two authors, a third person (UGM) resolved the disagreement. All reviewers came together in person and discussed the assessment results.
Information sources
This systematic review and meta-analysis were conducted by considering all the available studies (both published and open grey reports), governmental and other stakeholder annual reports, and national surveys on children and adolescents which have data on cigarette smoking among high school and university students in Ethiopia. An extensive search was done from the following international databases, including Cochrane Library, PubMed, Google Scholar, Hinari , Embase, CINAHL, Web of Science, and Science Direct to access articles conducted on the prevalence of smoking cigarette. The following keywords “prevalence”, ("cigarette smoking" OR ("cigarette"[All Fields] AND "smoking"[All Fields]) OR "cigarette smoking"[All Fields]) AND substance[All Fields]) AND (high[All Fields] AND ("schools"[MeSH Terms] OR "schools"[All Fields] OR "school"[All Fields]) AND ("universities"[MeSH Terms] OR "universities"[All Fields] OR "university"[All Fields])) AND ("students"[MeSH Terms] OR "students"[All Fields]) AND ("Ethiopia"[MeSH Terms] OR "Ethiopia"[All Fields]) were used to obtain published articles. Boolean operators particularly pairing aspects of “OR” or “AND” were used as search terms to separate articles. The search for all articles was conducted from 21 st September, 2018 to 25 th December, 2018 ( S2 Table ).
This systematic review and meta-analysis had two outcomes. The first outcome was the pooled prevalence of cigarette smoking among high school and university students in Ethiopia, which was calculated by dividing the number of smokers to the total students (sample size) multiplied by 100. The second outcome was the impact of peer pressure on cigarette smoking practice. We adjusted the effect size into Odd Ratio (OR) since all the studies were cross sectional and the appropriate effect size estimate for cross sectional design is OR to estimate the impact of peer pressure on cigarette smoking.
Data extraction
The necessary data (primary author, publication year, region, study design, sample size, prevalence of cigarette smoking) were extracted from the eligible articles by two authors (CT, AA and AA1) independently using prepiloted data extraction format prepared in Microsoft ™ Excel spreadsheet ( S3 Table ). Any disagreements between the three reviewers in the review process were discussed with the three reviewer team members (GD, DB and PM) until consensus was reached. Moreover, the data of kappa of agreement during the systematic searches was also used to solve the disagreements among two independent reviewers (CT and AA4). The kappa agreement was interpreted as less than chance agreement if less than 0, slight agreement if 0.01–0.20, fair agreement if 0.21–0.40, moderate agreement if 0.41–0.60, substantial agreement if 0.61–0.80 and moderate agreement if the kappa was 0.81–0.99 [ 43 ].
The four authors (CT, FW, MA and AA1) also independently extracted data on the association of cigarette smoking and peer pressure. If studies did not report OR, RR, or equivalent measures, raw data were screened to determine whether OR could be calculated. When the studies reported both the crude OR/RRs and the adjusted OR/RRs, the adjusted figures were extracted.
Quality assessment of the included studies
We assessed the quality of the included studies according to the Newcastle-Ottawa Scale (NOS) [ 44 ] ( S4 Table ). The NOS has three main domains and uses a star-based grading system with each study scoring a maximum of 10 stars. The first domain focuses on the methodological quality of the study (sample size, response rate, and sampling technique) with the possibility of a five-star grading (1 = poor to 5 = excellent). The second domain of the tool deals with the comparability of the study cases or cohorts, with the possibility of two stars. The last domain deals with the outcomes and statistical analysis of the study with a possibility of three stars. Three authors (MA, UGM, and DB) independently assessed the quality of each included study using the NOS. Any disagreement between the three authors was resolved by requesting other two authors (MY and PP) to independently assess the methodological quality to reach a consensus. Finally, studies with stars of ≥ 7 out of 10 were considered to be of a high quality [ 45 ]. Moreover, we assessed the quality of each included articles using National Institutes of Health (NIH) ( S5 Table ) which is a more detail tool on quality assessment than NOS. The tool has 14 criteria to assess the article independently with a response of “Yes, No and Not Applicable”. Articles with NIH assessment result of 85% and more (that means number of articles with yes divided by total criteria minus not applicable) were considered as good quality.
Risk of bias
For each included study, the risk of bias was assessed independently by two authors (UGM and CT). Risk of bias assessment was carried out using Holly 2012 tool which contain 10 recommended criteria for the internal and external validity tool [ 46 ]. This tool includes: representation of the population, sampling frame, methods of participants’ selection, non-response bias, data collection directly from subjects, acceptability of case definition, reliability and validity of study tools, mode of data collection, length of prevalence period; and appropriateness of numerator and denominator. Each item was classified as low and high risk of bias. Unclear assessment was classified as high risk of bias. The overall score of the risk of bias was then categorized according to the number of high risk item scores for bias per study: low (≤ 2), moderate (3–4), and high (≥ 5) ( S6 Table ).
Statistical data analysis
Standard error for all included studies was computed using the binomial distribution formula. Heterogeneity across studies were assessed by determining the p-values of Cochrane Q-test and I 2 -test statistics [ 47 ]. For meta-analysis result with significant heterogeneity, univariate meta-regression was used to assess the source of heterogeneity across each study. A funnel plot was also used for visual assessment of the publication bias. Asymmetry of the funnel plot is an indicator of potential publication bias. Furthermore, Egger’s test was used to determine if there was significant publication bias, and a p -value less than 0.10 was considered to indicate the presence of significant publication bias [ 48 ]. We selected Egger`s test to assess the publication bias because, the value of Egger`s test is more specific than Begg`s test [ 49 , 50 ]. We conducted the log relative risk to assess the effect of peer pressure on students’ cigarette smoking status. Furthermore, sensitivity analysis using a random effects model was performed to assess the influence of a single study on the pooled prevalence estimates. Subgroup analysis was used to minimize the random variations between the point estimates of the primary study subgroup, and analysis was done based on study settings (i.e., institution). Univariable meta-regression analysis was also conducted with year of publication and the outcome variable. All data manipulation and statistical analysis were performed using Stata ™ software (Version 14; Stata Corp, College Station, TX).
The electronic database search identified a total of 179 published articles. Of these, 121 duplicate articles were removed. Furthermore, 28 articles were removed after reviewing the titles and the abstract as they were not relevant to the focus of the review. Finally, one article was excluded due to inaccessibility of the full text despite three requests to the primary author on data, and 10 articles were excluded after reviewing their full text. Finally, 19 articles met all the prior criteria and were included in this analysis ( Fig 1 ).
- PPT PowerPoint slide
- PNG larger image
- TIFF original image
https://doi.org/10.1371/journal.pone.0222572.g001
Overview of the original included articles
All of the 19 articles included in this study were published between 1999 to 2017 in peer-reviewed journals. A total of 16,486 study participants were included in this systematic review and meta-analysis. The smallest sample size was 155 from a study conducted at Bahir Dar University [ 36 ], and the largest sample size was 1,984 in a study conducted in Gondar Medical College, Amhara Region [ 34 ]. All included studies were cross-sectional in design. The characteristics of the studies included in this review are described in ( Table 1 ) .
https://doi.org/10.1371/journal.pone.0222572.t001
Quality assessment result of the included articles
The qualities of individual articles were assessed using different tools; namely NOS and NIH quality assessment tools. Accordingly, NOS assessment result all articles had good quality using the NOS criteria. However, when assessed using NIH quality assessment tool, 1 (5.3%) study [ 36 ] was categorized as poor and the rest [ 11 , 15 , 23 – 35 , 37 , 38 , 51 ] were categorized as good quality ( S5 Table ).
Kappa agreement
Disagreements between the two reviewers during data extraction process were assessed using the Kappa agreement. Therefore, a = 9 and b = 2 represent the number of times the two reviewers agreed while c = 1 and d = 7 represent the number of times the two reviewers disagree. If there are no disagreements, b and c would be zero, and the reviewers agreement (po) is 1, or 100%. If there are no agreements, a and d would be zero, and the reviewers agreement (po) is 0. Interobserver agreement was 68% that indicate a substantial agreement between the two main reviewers who extracted data.
Risk of bias was performed for each included study using the risk of bias assessment tool that includes ten different items [ 46 ]. From the 19 included studies, the risk of bias summary assessment revealed that 94.7% of the included studies had a low risk of bias [ 15 , 23 – 35 , 37 , 38 , 51 ] while only one (5.3%) of the included studies had a moderate risk of bias [ 36 ].
Prevalence of cigarette smoking
The overall pooled prevalence of cigarette smoking in Ethiopia using the 19 studies was 16.31% (95% CI: 12.17, 20.45). A random-effects model was used because of the significant heterogeneity ( I 2 = 98.1%, p-value <0.001) across the studies ( Fig 2 ). Additionally, univariate meta-regression analysis was conducted to identify possible sources of heterogeneity. The different covariates included in the analysis were publication year and sample size. However, none of these variables were found to be statistically significant.
https://doi.org/10.1371/journal.pone.0222572.g002
The existence of publication bias was assured by funnel plot asymmetry. The funnel plot graph indicates that there is a significant variability within the findings of the 19 individual primary articles included in this meta-analysis ( Fig 3 ). The publication bias checked by objective measurement namely Egger’s tests also showed a statistically significant publication bias ( Egger's test p-value = 0 . 001 ). To handle the observed publication bias, we performed the trim and fill analysis, which is a nonparametric methods for estimating the number of missing studies that might exist and helps in reducing and adjusting publication bias in meta-analysis.
https://doi.org/10.1371/journal.pone.0222572.g003
Assessment of heterogeneity
We used I 2 statistics to investigate the presence of variation across the included studies. Accordingly, the result of I 2 statistics using a random effects model revealed a significant heterogeneity across the included studies ((I 2 = 98.1%, p-value <0 . 001 ).
Subgroup analysis
The findings from the subgroup analysis showed that the highest and lowest cigarette smoking was observed among university students 17.35% (95% CI: 12.97, 22.16) and high school students 13.76% (95% CI: 7.24, 20.27), respectively ( Fig 4 ).
https://doi.org/10.1371/journal.pone.0222572.g004
Similarly, the regional subgroup analysis result revealed the pooled prevalence of smoking from highest to lowest was [20.11% (95% CI: 11.39, 28.84)] in Ethio-Somalia and Harari region, [18.96% (95% CI: -0.03, 38.01)] in Tigray region, [17.35% (95% CI: 13.21, 21.49)] in South Nation Nationality and People of Ethiopia (SNNPE), [15.34% (95% CI: 10.84, 19.83)] in Amhara region, [14.98% (95% CI: 7.37, 22.55)] in Oromia region, and [5.9% (95% CI: 0.02, 11.79)] in Addis Ababa region ( Fig 5 ).
https://doi.org/10.1371/journal.pone.0222572.g005
The linear trend of cigarette smoking status of students in Ethiopia
The cumulative univariate meta-analysis on cigarette smoking status among high school and university with the year of 1984–2017 was performed. The result from cumulative univariate meta-analysis showed the trend in prevalence estimates of cigarette smoking status among high school and university over time. The finding revealed that there is more or less constant trend ( Fig 6 ).
https://doi.org/10.1371/journal.pone.0222572.g006
The univariate meta-regression using bubble plot was also performed. The bubble plot figure indicates that the trend was slight increment ( Fig 7 ).
https://doi.org/10.1371/journal.pone.0222572.g007
The effect of peer pressure on cigarette smoking status
Five of the 19 included studies reported the effect of peer pressure on cigarette smoking. From this, three studies [ 11 , 30 , 37 ] showed a positive effect of peer pressure on cigarette smoking, while the other two studies [ 31 , 51 ] showed no relationship between peer pressure and cigarette smoking. However, the aggregated meta-analysis revealed a higher odds of cigarette smoking among students who experienced peer pressure than those who didn’t (OR: 2.68, 95% CI: 2.37, 3.03) ( Fig 8 ).
https://doi.org/10.1371/journal.pone.0222572.g008
Cigarette smoking has major health and social consequences, and it reduces the educational performance of students [ 52 , 53 ]. This systematic review and meta-analysis, therefore, was conducted to assess the pooled prevalence of cigarette smoking and its association with peer pressure among high school and university students in Ethiopia. Accordingly, the pooled prevalence of cigarette smoking among Ethiopian high school and university students was 15.92%. This finding is lower than a study conducted among students in South Africa which reported a prevalence of 16.9% [ 50 ]. Conversely, the current reported pooled prevalence of cigarette smoking was higher than a study conducted among government and private schools and college students in Bengaluru, India (12.8%) [ 54 ] and amongst university students in Iran (13.8%) [ 55 ].
In this review, the pooled prevalence of cigarette smoking was lower than a study finding observed among Kenyan secondary school students (38.6%) and Cameroon university students (93.1%) [ 56 , 57 ]. In addition, our finding was slightly lower than a study conducted among high school students in Shiraz- Iran (19.7%) [ 58 ]. This might be due to the difference between sample size and socio-demographic nature of the two study populations. There is also cultural variation among the study communities. Moreover, the higher prevalence of cigarette smoking in the current study could be due to the dominance of male participants as evidence suggests that males tend towards different types of substance abuse than females [ 59 , 60 ].
Similarly, the current pooled prevalence of cigarette smoking is also lower than a systematic review conducted in Africa [ 50 ] and the Middle East [ 61 ]. This variation might be due to the differences in the study period and sample size between these two studies. In addition, the previous review was conducted only among university students, while the current review included both high school and university students.
The current review also considered subgroup analysis to appreciate the variability or heterogenic characteristics of the included studies. Accordingly, a higher prevalence was observed among university students (17.35%) than high school students (12.77%). This could be because most high school students live with their families which may limit them from cigarette smoking because of parental control. Additionally, in most cases, students during their high school time live with families and that may not encourage smoking cigarette. On the contrary, when they join to the university, almost all students become independent of their family supervision. This independency and pressure from their friends increases the proportion of students who smokes cigarette [ 62 ]. Educational institutions can be a challenging environment and everyone copes with stress in different ways [ 17 ]. Moreover, as students enter to university, they start a new life away from their families in a different and strange environment which can contribute to their behavior or involvement in substance abuse like cigarette smoking [ 55 ]. Evidence also supports that as the level of education increase, the proportion of smoking increases [ 63 , 64 ].
A subgroup analysis by regions of the country also showed a higher prevalence of cigarette smoking among universities in other category (i.e., Harar region, Somalia region and Oromia region). This finding might be due to typical local practices of substances like cigarette and khat in these regions. Therefore, the government, school management, local communities and other concerned bodies need to implement school-based intervention programs in order to reduce the pooled prevalence of cigarette smoking.
Students who felt peer pressure were more likely to smoke cigarette than those who had no peer pressure. This finding was similar to a study conducted in Kenyan students and Shiraz- Iran [ 57 ] where peer pressure was found to have a significant (positive) effect on the likelihood of cigarette smoking [ 56 , 58 ]. Peer group pressure is widely known as a decisive factor which affects the early onset of experimentation with tobacco and the individual’s subsequent willingness to continue smoking [ 16 ]. Similarly, other systematic reviews state the most common factors influencing students’ smoking status was having smoker friends [ 55 , 65 ]. Therefore, the school management needs to implement youth association focusing on counseling and rehabilitation service for to seize students already practicing smoking and also those who are not practicing yet now.
Strengths and limitations of the study
This review has several strengths including: this review focus on the adolescent and young adult populations who are vulnerable to initiating substance use/abuse behaviors. In addition, this review rigorous adherence to the PRISMA checklist which improves its quality for the readers. Moreover, this finding will give an insight into developing a health promotion policy for the country. Whereas, on top of the above strength, this review has the following limitations: This review included studies that were published only in English language which may limit the number of studies that were reported in other languages. Moreover, the other limitation of this review was the risk of self-report bias introduced from the original studies included in the review. On top of these the protocol of this manuscript was not registered online before conducting it.
Conclusions
This systematic review and meta-analysis indicate that the prevalence of cigarette smoking among Ethiopian high school and university students was high. More than one sixth of the high school and university students smoke cigarettes. This higher cigarette smoking proportion of students was influenced by peer pressure. Variations were also observed in the prevalence of cigarette smoking by different regions in the country. Therefore, school-based intervention programs aimed at prevention of cigarette smoking is recommended. In particular, educational programs on how to resist and handle peer pressure are essential to prevent cigarette smoking among high school and university students in Ethiopia.
Supporting information
S1 table. prisma 2009 checklist..
https://doi.org/10.1371/journal.pone.0222572.s001
S2 Table. Searches for databases.
https://doi.org/10.1371/journal.pone.0222572.s002
S3 Table. Data extraction tools Smoke.
https://doi.org/10.1371/journal.pone.0222572.s003
S4 Table. Quality assessments.
https://doi.org/10.1371/journal.pone.0222572.s004
S5 Table. NIH quality assessments.
https://doi.org/10.1371/journal.pone.0222572.s005
S6 Table. Risk of bias for each study.
https://doi.org/10.1371/journal.pone.0222572.s006
Acknowledgments
The authors of this work would like to forward great and deepest gratitude for Debre Markos University for creating convenient environment and internet service. Furthermore, the authors would like also to forward special acknowledgement for authors of primary studies.
- View Article
- Google Scholar
- 7. World Health Organization. WHO Report on the global tobacco epidemic, 2015. Geneva, Switzerland: WHO. 2015.
- 8. World Health Organization: WHO Global Report on Trends in Prevalence of Tobacco Smoking. Geneva, SW: WHO 2015.
- 13. Fit for Work team: Smoking and its impact on mental health. March 6, 2017.
- PubMed/NCBI
- 22. Kebede Y, Abula T: Substance Abuse For the Ethiopian Health Center Team. 1st edition. Addis Ababa: Ethiopian Public Health training Initiative 2005.
- 25. Gezahegn T, Andualem D, Mitiku TH: Substance Use and Associated Factors among University Students in Ethiopia: A Cross-Sectional Study. Hindawi Publishing Corporation 2014.
- 40. Ethiopia—Historical Attractions, Regions, Cities and Population.
- 44. Newcastle: Newcastle-Ottawa Scale customized for cross-sectional studies. In. available from https://static-content.springer.com/esm/…/12889_2012_5111_MOESM3_ESM.doc . 2012.
- 52. US Department of Health and Human Services: How Tobacco Smoke Causes Disease: What It Means to You. Atlanta: U.S. Department of Health and Human Services, Centers for Disease Control and Prevention, National Center for Chronic Disease Prevention and Health Promotion, Office on Smoking and Health,. 2010.
- 53. US Department of Health and Human Services: The Health Consequences of Smoking—50 Years of Progress: A Report of the Surgeon General. Atlanta: U.S. Department of Health and Human Services, Centers for Disease Control and Prevention, National Center for Chronic Disease Prevention and Health Promotion, Office on Smoking and Health. 2014.
- Research article
- Open access
- Published: 01 September 2016
Association between high school students’ cigarette smoking, asthma and related beliefs: a population-based study
- Resa M. Jones 1 , 2 , 3 ,
- Kara P. Wiseman 1 &
- Marina Kharitonova 1
BMC Public Health volume 16 , Article number: 913 ( 2016 ) Cite this article
18k Accesses
9 Citations
3 Altmetric
Metrics details
Smoking has a detrimental effect on the symptoms and severity of asthma, a common chronic disease among adolescents. The purpose of this study was to examine the association between asthma and smoking among high school students and assess provider-patient communication with asthmatic adolescents regarding smoking and adolescents’ beliefs about the harms of smoking.
In fall 2014, data from high school students, ages 14–18 years, completing the 2009-2010 Virginia Youth Tobacco Survey ( N = 1796) were used in descriptive analyses and multivariable logistic regression models adjusting for model-specific confounders as appropriate.
Overall, an estimated 19 % of high school students in Virginia smoked and 16 % had asthma. Odds of smoking did not differ by asthma status; however, asthmatics had 1.5 times higher odds of being asked if they smoke (95 % CI 1.06–2.13) and being advised not to smoke by a health professional (95 % CI 1.10–2.14) compared to non-asthmatics. Asthmatics who believed second-hand smoke or smoking 1–5 cigarettes/day was not harmful had respectively 4.2 and 2.8 times higher odds of smoking than those who thought each was harmful. Further, asthmatics who thought smoking 1−2 years is safe had 3.4 times higher odds of smoking than those who did not (95 % CI 1.57–10.1).
Conclusions
While asthmatic adolescents are just as likely to smoke as non-asthmatics, less healthy beliefs about the risks of smoking increase the odds of smoking among asthmatics. Thus, targeted asthma-specific smoking prevention and education to change attitudes and beliefs could be an effective tool for adolescents.
Peer Review reports
Asthma is one of the most common chronic diseases among children and youth in the United States [ 1 ]. Approximately 14 % of children aged 17 and under have ever been diagnosed with asthma [ 2 ] Among high school students, primarily ages 14–18 years, about 11 % currently have asthma [ 3 ]. Asthma affects everyday activities, such as playing, exercise, school attendance, and sleeping, as well as emotional health; [ 1 , 4 ] however, asthma is possible to manage and control with medication and behavior modification [ 1 ].
All asthma management guidelines state that patients should be strongly advised not to smoke [ 5 ]. Adolescents with asthma are expected to avoid smoking because it might initiate or aggravate asthmatic symptoms [ 6 ]. Further, asthmatics who smoke have more asthma attacks, worse pulmonary function and higher rates of hospitalization [ 7 ]. In addition, smoking may reduce the effectiveness of daily control medicine [ 8 ]. Overall, about 20 % of U.S. high school students [ 3 ] and Virginia high school students [ 9 ] smoke, which compromises their current and future health. While one study found that asthma in childhood reduces smoking initiation in male adolescents [ 10 ], the majority of previous studies assessing smoking among asthmatic adolescents show that they are more likely to smoke than non-asthmatic adolescents [ 11 – 15 ]. In addition, a cohort study of adolescents examined smoking and asthma and found a bidirectional relationship [ 15 ]. Some studies have found that while the demographic and behavioral predictors of smoking could be the same for adolescents with and without asthma [ 11 ], some differences in motivation exist [ 16 , 17 ]. Other research suggests that asthmatic adolescents may make a more active decision to start smoking than adolescents without asthma [ 17 ].
Little is known about how provider communication might impact adolescents’ smoking or what influences smoking behavior among asthmatics. Thus, to better inform intervention development and identify areas for education, the objectives of this study are to: (1) describe the prevalence of those with and without diagnosed asthma among Virginia high school students and the sub-group who smoked in the past 30 days, (2) examine the association between the prevalence of asthma and smoking, (3) evaluate provider-patient communication regarding smoking and examine adolescents’ beliefs about the harms of smoking by asthma status, and (4) examine the association between smoking and provider-patient communication regarding smoking and adolescents’ beliefs about the harms of smoking.
Study sample
A two-stage cluster sample design was used to produce a representative sample of Virginia high school students (grades 9–12; majority are ages 14–18 years) for the Virginia Youth Tobacco Survey (Virginia YTS) [ 9 ]. Stage one of the sampling included all Virginia public schools containing grades 9, 10, 11, or 12. Schools were selected with probability proportional to school enrollment size. Stage two consisted of systematic equal probability sampling (with a random start) of classes from each participating school. All second period classes in the selected schools were included in the sampling frame. All students in these selected classes were eligible to participate in the survey.
Seventy-two percent of randomly selected high schools agreed to participate (36 of 50) and the student response rate (completed questionnaires divided by total number of students) for students in the selected class periods in participating high schools was 81.9 % ( N = 1827 of 2232) [ 9 ]. The overall response rate was 58.9 % [ 9 ]. This study includes respondents ages ≥14 years who answered the primary questions of interest ( N = 1796; n = 16 students <14 years were excluded). This study was approved by the Virginia Commonwealth University Institutional Review Board.
The Virginia YTS is a self-administered, written questionnaire based on the validated Youth Tobacco Survey and Youth Risk Behavior Survey designed by the Centers for Disease Control and Prevention (CDC) [ 3 , 9 ]. The survey, distributed in classrooms during the 2009 – 2010 academic year, included questions about tobacco use, tobacco-related psychosocial constructs, asthma, health care, and demographics.
Students were asked “During the past 30 days, on how many days did you smoke cigarettes?” Responses were combined to create a dichotomous variable indicating whether or not the student had smoked in the past 30 days. Students were also asked whether “a doctor or nurse ever told [them] that [they] have asthma?” Responses were coded to reflect three categories-Yes, and still have it now; Yes, but don’t have it anymore; and No.
Several questions measured smoking-related variables. Students provided information on whether “During the past 12 months, did any doctor, dentist, nurse, or other health professional ask you if you smoke?” and “During the past 12 months, did any doctor, dentist, nurse, or other health professional advise you not to smoke?” (“yes”, “no”, and “don’t know/not sure” were dichotomized as “yes” or “no”, which included “don’t know/not sure”). Students also answered knowledge/belief questions relating to the harms of smoking, with response options of “definitely yes”, “probably yes”, “probably not”, and “definitely not”, which were recoded as binary variables to reflect “yes” or “no” responses (i.e., “Do you think young people risk harming themselves if they smoke from 1 to 5 cigarettes per day?” “Do you think it is safe to smoke for only a year or two, as long as you quit after that?” and “Do you think the smoke from other people’s cigarettes is harmful to you?”) Students also provided information on gender and age as well as race (i.e., White, Black, and Other), whether they lived with a smoker, the disposable funds they had to spend in any way in the last 4 weeks (coded as none, $1–$10, $11–$20, $21–$50, and > $50), how many of their four closest friends smoke (coded as ≥1 or none), and rules about smoking in the home or car (coded as always/sometimes allowed or never allowed).
Statistical analysis
SAS 9.4 PROC SURVEYFREQ, PROC SURVEYREG, and PROC SURVEYLOGISTIC were used for data analysis with appropriate weights and strata given the sampling scheme of the Virginia YTS. Descriptive statistics were calculated for demographics of the overall sample and among those who smoked in the last 30 days and to determine the prevalence of asthma and smoking. Differences in smoking-related communication with health professionals and beliefs about the harms of smoking by asthma status were assessed. Logistic regression was used to assess both the association between asthma and smoking (dependent variable) among all students and the relationship between health professional communication about smoking and individual beliefs about the harms of smoking and smoking behavior (dependent variable) among asthmatics. We determined whether there was evidence of effect modification for age, race, or gender for all models. Possible confounders (i.e., student’s gender, age, race, whether they lived with a smoker, amount of disposable funds available to spend in any way in the last 4 weeks, friends’ smoking, rules about smoking in the home, and rules about smoking in the car) were assessed for the regression models using the 10 % change-in-estimate rule as conceptually appropriate [ 18 ]. The final multivariable logistic regression models provide odds ratios (OR) and confidence intervals (CI) adjusted for model-specific confounders.
Table 1 provides the estimated demographic characteristics of Virginia high school students, ages ≥14 years. About half were female students and 36.4 % indicated that they live with someone who smokes. Approximately half (55.5 %) were white students and 26.8 % were Black students. Overall, 18.1 % of female and 20.5 % of male students had smoked one or more cigarettes in the past 30 days at the time of the survey. In addition, 16.2 % of the overall sample reported currently having asthma whereas 17.9 % of youth who smoked in the past 30 days reported currently having asthma.
No evidence of effect modification was found for age, race, or gender. Taking into account asthma status, students who lived with a smoker had 2.57 times higher odds of smoking (95 % CI 1.90–3.48) compared to those not living with a smoker (data not shown in Tables). The odds of smoking among students with current asthma or a previous diagnosis were not significantly different than those who were never diagnosed with asthma after adjusting for the confounder of living with a smoker.
In the past 12 months, a greater percentage of students with current asthma were asked by a health professional about whether they smoked (43.6 %, p -value = 0.049) and advised to not smoke (35.4 %, p -value = 0.087) compared to those without asthma (Table 2 ; unadjusted analyses as no evidence of confounding). In fact, after adjustment for model-specific confounders, students with asthma had 1.5 times higher odds of being asked by a health professional whether they smoked (95 % CI 1.06–2.13) and 1.5 times higher odds of being advised to not smoke (95 % CI 1.10–2.14) compared to those who had never had asthma (data not shown in Tables.) Students’ beliefs about the harms of smoking did not significantly vary by asthma status.
Table 3 provides estimates of the association between smoking status and health provider communication and students’ beliefs about smoking for asthmatic students (adjusted for model-specific confounders detailed in Table footnotes). Among students with asthma, smoking status was associated with health professionals asking students about their smoking. Specifically, asthmatics who were asked if they smoked had 2.46 higher odds of smoking than those who were not asked if they smoked (95 % CI: 1.04–5.81). Asthmatic students who thought it was safe to smoke for only 1–2 years had 3.97 times higher odds of smoking than those who did not believe it was safe (95 % CI 1.57–10.06).
This study assessed the association between asthma and smoking status as well as high school students’ reported communication about smoking risks with their clinicians, and the association between clinician advice and students’ beliefs about smoking harms and cigarette smoking among asthmatic high school students in Virginia. While several studies have shown that adolescents with asthma are more likely to smoke than those without asthma [ 11 – 15 ], we found asthmatic students were just as likely to smoke as their non-asthmatic peers. The odds of provider communication about smoking and the potential harms of smoking were higher for asthmatic high school students compared to non-asthmatic students. Less healthy beliefs about the actual risks of smoking increase the odds of smoking among asthmatics.
Smoking prevalence and beliefs about harms of smoking
Despite asthma management guidelines and negative effects of smoking on asthma symptoms, self-reported asthmatic high school students in Virginia are just as likely to smoke as those without asthma. This finding is divergent from the majority of studies that have reported asthmatic adolescents are more apt to smoke than non-asthmatic adolescents [ 11 – 15 ]. However, one previous study found asthma in childhood reduces smoking initiation in male adolescents [ 10 ]. Further, a longitudinal cohort study of Dutch adolescents examined smoking and asthma and found a bidirectional relationship. Specifically, at 1-year follow-up, those with self-reported asthma were 1.91 times more likely to begin smoking than those without asthma and those who smoked were 2.86 times more likely to develop symptoms of asthma over the course of the study [ 15 ]. These results suggest that adolescents with asthma are more willing to smoke regardless of the unpleasant experiences associated with smoking initiation and the consequences that smoking can induce. Also, differences in motivation exist by asthma status [ 16 , 17 ]. For example, adolescents with asthma are 1.67 times more likely to report smoking initiation due to peer pressure and a greater proportion of asthmatics use smoking as a means of weight control compared to adolescents without asthma (18.8 % vs 13.1 %) [ 16 ]. Evidence also exists that asthmatic adolescents may make a more active decision to start smoking than adolescents without asthma [ 17 ].
The vast majority of respondents (~9 out of 10) demonstrated accurate knowledge of the harms of tobacco smoke and smoking. This is a positive result and shows wide and accurate awareness of the harms of cigarettes among high school students, which could be the result of concerted efforts to prevent and reduce tobacco use among youth. For example, direct health promotion activities are underway in Virginia and multiple marketing campaigns reached 648,500 in 2009 with approximately 694,000 – 731,000 youth reached annually 2004 to 2008 [ 19 ]. However, among asthmatic students, less healthy responses to questions about the knowledge and beliefs about the harms of smoking (i.e., second-hand smoke is not harmful, smoking 1–5 cigarettes a day is not harmful, smoking only a year or 2 years is safe) were positively associated with increased odds of smoking in the past 30 days; however, the only belief that had a statistically significant association was thinking that smoking for 1–2 years is safe. This finding likely reflects the belief among youth that it is not hard to quit smoking. While a general message that smoking is harmful can be received by all high school students, particular knowledge that smoking even for a relatively short period of time (only 1 or 2 years) is not safe and perhaps information about the reality of early nicotine dependence could discourage smoking initiation or occasional smoking, which could lead to regular smoking. This supports previous findings that low risk perception is a predictor of smoking among adolescents [ 20 ].
Overall, to realize the largest public health impact, targeted prevention efforts that make the short- and long-term consequences of smoking concrete and highlight how to combat peer pressure are needed to address smoking among adolescents with and without asthma. Engaging, age-appropriate materials and messages delivered through public schools, recreation centers, and faith-based institutions can effectively impact attitudes and ultimately lead to behavior change. For example, since the inception of statewide programming and partnering with the Virginia Department of Education on health promotion activities, the prevalence of smoking among Virginia high school students dropped from 28.6 % in 2001 to 8.2 % in 2015 [ 19 ]. Previous research also suggests that gender should also be considered when developing prevention and cessation interventions for asthmatic adolescents [ 21 ].
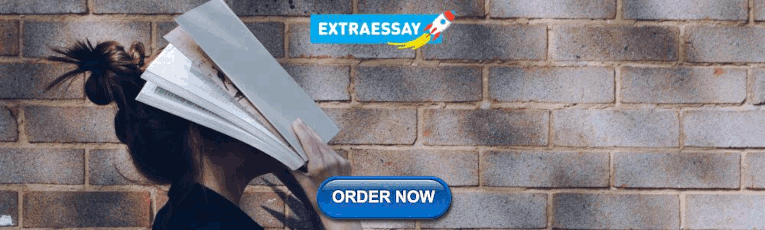
Health professional communication
To date, no studies have assessed patient-provider communication about smoking with asthmatic adolescents. In this study, high school students with asthma had more communication with health professionals regarding smoking than those without asthma. While this is a positive result, in total, less than half of all students with asthma were asked if they smoke. Using the same smoking measure, 2011 National Youth Tobacco Survey data suggest that among students ages 15 years and older, the proportion of health care providers asking about smoking ranged from 35.3 to 69.9 depending on the student’s smoking history [ 22 ]. Therefore, it appears that providers could continue to improve. Among asthmatic students, communication with a health professional regarding smoking had an inconsistent effect, as health professionals asking about smoking was significantly associated with increased odds of smoking, while advice about not smoking was not associated with smoking. The positive association between providers asking about smoking and behavior could be due to the fact that doctors are more apt to ask about smoking if they know the patient has a history of smoking and/or that smokers could be more likely to remember being asked. Previous research among adolescents from Tennessee found screening and advice about smoking from a healthcare provider had a positive impact on knowledge and quit attempts among smokers [ 23 ], which conflicts with the findings of this study. Given the high risk of smoking initiation during adolescence, smoking should continue to be addressed consistently by doctors and other providers treating adolescents with asthma. While laws exist to promote confidential discussions between medical providers and adolescent patients, one study found 64 % of adolescents seen in medical practice had documented alone time with a clinician [ 24 ] whereas another study reported almost 48 % of adolescents ages 15–17 years who had a preventive health visit did not have time alone with a clinician [ 25 ]. Further, about one-third of parents disagree that providers should see an adolescent patient alone [ 26 ]. Thus, if adolescents less than 18 years of age receive medical care with a parent present, discussing risky behaviors such as smoking may be sensitive and difficult to broach.
Then again, one of the most important aspects of asthma management is self-care, such as avoiding asthma triggers and complying with control medication. High school students are able to understand and remember self-care plans and they have enough independence to make decisions to avoid smoking. This study suggests that there are missed opportunities for health care professionals to communicate to adolescent patients with asthma the importance of being tobacco free and how it is essential in preventing acute episodes. In addition, some studies of smoking among asthmatic adolescents have found that poor medication adherence is associated with smoking [ 12 ]. Taken together, these findings could indicate that a complete asthma management plan provided by a health professional would help address smoking as well as other aspects of self-care. Further studies could examine the relationship between patient-provider communication and asthma episode severity among adolescents as well as confidence to avoid irritants such as tobacco smoke, and self-efficacy in asthma management among adolescents.
Limitations
These study findings should be considered in light of a few limitations. First, a self-report questionnaire, administered in school classrooms, was used for this study so misclassification could exist. Students could have inaccurately reported their asthma status or diagnosis; however, this is unlikely given asthmatic students generally take medications and see a primary care provider at least once a year once they are diagnosed [ 27 ]. In addition, there could be a tendency toward socially desirable answers about smoking behavior by students. However, self-reported smoking by adolescents is similar to the prevalence given cotinine testing and is considered valid for population-level estimates [ 28 – 31 ]. Also, if present, under-reporting of smoking could mean there is an even greater public health impact than estimated in the current study. Importantly, the prevalence of current asthma and smoking in the last 30 days were assessed using the identical questions to that in the CDC’s Youth Tobacco Survey and Youth Risk Behavioral Surveillance (YRBS) survey, which reportedly have high reliability [ 31 ] and facilitate comparison to national data [ 3 ]. Smoking, defined as having ≥1 cigarette in the past 30 days, was purposely coded to align with the U.S. Healthy People 2020 tobacco-related Leading Health Indicator goal to reduce the prevalence of adolescents smoking in the last 30 days [ 32 ]. Second, because the high school students in the sample are from only one state in the U.S., findings may not be generalizable to all U.S. high school students. However, current cigarette smoking prevalence in this study was comparable to the identical contemporaneous measure from the national 2009 YRBS survey (19.2 %) [ 3 ]. The prevalence of current asthma in our sample was higher than national averages from the 2005–2010 National Health and Nutrition Examination Survey (12.9 %) [ 33 ], however, Virginia is well-known for its asthma burden within the U.S. [ 34 ]. Smoking prevalence among asthmatics in this study (17.9 %) was much lower than the prevalence of current smoking among asthmatic adolescents in the nationally representative longitudinal (48 %) [ 35 ]. Participating schools were in urban, suburban, and rural communities and schools that declined to participate were not more likely to be inner city schools or schools with higher proportion of historically disadvantaged students (M. White, Virginia Foundation for Healthy Youth, personal communication, 2014). Additionally, school-based studies exclude drop-outs and these youth tend to engage in more risky health-related behaviors. Third, the study data are cross-sectional; thus, temporality regarding asthma diagnosis and smoking as well as doctors asking about or advising against smoking is not exactly known. Lastly, because this study involves secondary data analyses, potential confounders (e.g., socio-economic status, school-level health promotion activities, etc.) and associations of interest were limited by a function of the initial design and data collection.
While most high school students understand the harms of even occasional smoking, many of them still smoke, including students with asthma. In this cross-sectional study, among students with asthma, advice from health professionals does not appear to make a significant difference in the decision to smoke; however, importantly, personal beliefs and knowledge about the harms of smoking for only 1–2 years significantly increased the odds of smoking. Overall, this highlights the importance of school-based educational interventions among children and adolescents in the effort to prevent youth smoking. Both asthma and smoking remain a major health issue for adolescents in Virginia and in the U.S., and many young people are affected by both. In general, formal prevention education about the harms of smoking takes place mostly in the school [ 36 ], while patient education about asthma management takes place primarily in a clinical setting. Health education that currently focuses on either prevention or treatment/management should find an intersection, so that issues that affect the same population can be addressed. Helpful in this topic could be studies addressing provider-patient communication about smoking among adolescents with asthma and broader public health educational interventions targeting teens with asthma.
Abbreviations
Centers for Disease Control and Prevention
Confidence interval
National Center for Chronic Disease Prevention and Health Promotion. Healthy Youth! Asthma. Centers for Disease Control and Prevention. http://www.cdc.gov/healthyschools/asthma/index.htm . Accessed 6 Apr 2016. Updated June 17, 2015
Bloom B, Cohen RA, Freeman G. Summary health statistics for U.S. children: National Health Interview Survey, 2009. National Center for Health Statistics. Vital Health Stat. 2010;(10):247. http://www.cdc.gov/nchs/data/series/sr_10/sr10_247.pdf . Accessed 6 Apr 2016. Published December 2010.
Eaton DK, Kann L, Kinchen S, Shanklin S, Ross J, Hawkins J, et al. Youth Risk Behavior Surveillance - United States 2009. MMWR Surveill Summ. 2010;59(5):1–142.
PubMed Google Scholar
McQuaid EL, Kopel SJ, Nassau JH. Behavioral adjustment in children with asthma: A meta-analysis. J Dev Behav Pediatr. 2001;22(6):430–9.
Article CAS PubMed Google Scholar
National Asthma Education and Prevention Program Expert Panel Report 3. Guidelines for the Diagnosis and Management of Asthma-Summary Report 2007. National Heart, Lung, and Blood Institue. U.S. Department of Health and Human Services. National Institutes of Health. http://www.nhlbi.nih.gov/guidelines/asthma/asthsumm.pdf . Accessed 6 Apr 2016. Published October 2007
Yoo S, Kim HB, Lee SY, Kim BS, Kim JH, Yu J, et al. Effect of active smoking on asthma symptoms, pulmonary function, and BHR in adolescents. Pediatr Pulmonol. 2009;44(10):954–61.
Eisner MD, Iribarren C. The influence of cigarette smoking on adult asthma outcomes. Nicotine Tob Res. 2007;9(1):53–6.
Article PubMed Google Scholar
Livingston E, Thomson NC, Chalmers GW. Impact of smoking on asthma therapy: A critical review of clinical evidence. Drugs. 2005;65(11):1521–36.
Virginia Foundation for Healthy Youth. Tobacco Use Among Virginia’s Middle and High School Students. Results of the 2009 Youth Tobacco Survey: Including comparisons to the 2001, 2003, 2005, and 2007 survey results. Survey and Evaluation Research Laboratory, Virginia Commonwealth University. http://www.vfhy.org/sites/default/files/pdf/YTS09_report_final_0.pdf . Accessed 6 Apr 2016. Published 2010
Verlato G, Bortolami O, Accordini S, Olivieri M, Cappa V, Bugiani M, et al. Asthma in childhood reduces smoking initiation in subsequent teens among males. J Adolesc Health. 2011;48(3):253–8.
Zbikowski SM, Klesges RC, Robinson LA, Alfano CM. Risk factors for smoking among adolescents with asthma. J Adolesc Health. 2002;30(4):279–87.
Precht DH, Keiding L, Madsen M. Smoking patterns among adolescents with asthma attending upper secondary schools: A community-based study. Pediatrics. 2003;111(5 Pt 1):e562–8.
Hublet A, De Bacquer D, Boyce W, Godeau E, Schmid H, Vereecken C, et al. Smoking in young people with asthma. J Public Health. 2007;29(4):343–9.
Article CAS Google Scholar
Jones SE, Merkle S, Wheeler L, Mannino DM, Crossett L. Tobacco and other drug use among high school students with asthma. J Adolesc Health. 2006;39(2):291–4.
Van de Ven MO, Engels RC, Kerstjens HA, Van den Eijnden RJ. Bidirectionality in the relationship between asthma and smoking in adolescents: A population-based cohort study. J Adolesc Health. 2007;41(5):444–54.
Precht DH, Keiding L, Nielsen GA, Madsen M. Smoking among upper secondary pupils with asthma: Reasons for their smoking behavior: A population-based study. J Adolesc Health. 2006;39(1):141–3.
Van De Ven MO, Engels RC, Otten R, Van Den Eijnden RJ. A longitudinal test of the theory of planned behavior predicting smoking onset among asthmatic and non-asthmatic adolescents. J Behav Med. 2007;30(5):435–45.
Article Google Scholar
Greenland S, Rothman KJ. Introduction to stratified analysis. In: Rothman KJ, Greenland S, Lash TL, editors. Modern Epidemiology. Philadelphia: Lippincott Williams and Wilkins; 2008. p. 261–2.
Google Scholar
Virginia Foundation for Healthy Youth. Statistics Dashboard. http://vfhy.org/statistics . Accessed 11 July 2016. Published 2015
Van De Ven MO, Van Den Eijnden RJ, Engels RC. Smoking-specific cognitions and smoking behavior among adolescents with asthma. Psychol Health. 2006;21(6):699–716.
Guo SE, Ratner PA, Johnson JL, Okoli CT, Hassain S. Correlates of smoking among adolescents with asthma. J Clin Nurs. 2010;19(5-6):701–11.
Schauer GL, Agaku IT, King BA, Malarcher AM. Health care provider advice for adolescent tobacco use: results from the 2011 National Youth Tobacco Survey. Pediatrics. 2014;134(3):446–55.
Hum AM, Robinson LA, Jackson AA, Ali KS. Physician communication regarding smoking and adolescent tobacco use. Pediatrics. 2011;127(6):e1368–74.
Article PubMed PubMed Central Google Scholar
Duncan RE, Vandeleur M, Derks A, Sawyer S. Confidentiality with adolescents in the medical setting: what do parents think? J Adolesc Health. 2011;49(4):428–30.
Edman JC, Adams SH, Park MJ, Irwin Jr CE. Who gets confidential care? Disparities in a national sample of adolescents. J Adolesc Health. 2010;46(4):393–5.
Hutchinson JW, Stafford EM. Changing parental opinions about teen privacy through education. Pediatrics. 2005;116(4):966–71.
Chua K, Schuster MA, McWilliams JM. Differences in health care access and utilization between adolescents and young adults with asthma. Pediatrics. 2013;131(5):892–901.
Dolcini MM, Adler NE, Lee P, Bauman KE. An assessment of the validity of adolescent self-reported smoking using three biological indicators. Nicotine Tob Res. 2003;5(4):473–83.
Patrick DL, Cheadle A, Thompson DC, Diehr P, Koepsell T, Kinne S. The validity of self-reported smoking: a review and meta-analysis. Am J of Public Health. 1994;84(7):1086–93.
Brener ND, Billy JO, Grady WR. Assessment of factors affecting the validity of self-reported health-risk behavior among adolescents: evidence from the scientific literature. J Adolesc Health. 2003;33(6):436–57.
Brener ND, Kann L, McManus T, Kinchen SA, Sundberg EC, Ross JG. Reliability of the 1999 Youth Risk Behavior Survey questionnaire. J Adolesc Health. 2002;31(4):336–42.
Healthy People 2020. 2020 Topics and Objectives. Tobacco Use-Objectives. 2010. https://www.healthypeople.gov/2020/topics-objectives/topic/tobacco-use/objectives . Accessed 5 July 2016. Published December 2
Kit BK, Simon AE, Bordy DJ, Akinbami LJ. US prevalence and trends in tobacco smoke exposure among children and adolescents with asthma. Pediatrics. 2013;131:407–14.
Asthma Allergy Foundation of America. Asthma Capitals. http://www.aafa.org/page/asthma-capitals.aspx . Accessed 6 Mar 2012. Published February 2011
Tercyak KP. Psychosocial risk factors for tobacco use among adolescents with asthma. J Pediatr Psychol. 2003;28(7):495–504.
U.S. Department of Health and Human Services. Centers for Disease Control and Prevention. Results from the School Health Policies and Practices Study 2012. Centers for Disease Control and Prevention. http://www.cdc.gov/healthyyouth/shpps/2012/pdf/shpps-results_2012.pdf . Accessed 6 Apr 2016. Published 2013
Download references
Acknowledgements
Study data are from the Virginia Youth Tobacco Survey, which is sponsored by the Virginia Foundation for Healthy Youth. The Survey and Evaluation Research Laboratory at Virginia Commonwealth University, with guidance from the Centers for Disease Control and Prevention (CDC), oversaw survey administration. The Youth Tobacco Survey was designed by the CDC as part of its Youth Tobacco Surveillance and Evaluation System.
Not applicable.
Availability of data and materials
The data will not be shared as the authors obtained permission for a select dataset of Virginia Youth Tobacco Survey variables from the Virginia Foundation for Healthy Youth. Interested parties may request data from the Virginia Foundation for Healthy Youth.
Authors’ contributions
RMJ was responsible for the concept, design, and interpretation of data. She directed the data analyses, drafted sections of the manuscript, and was responsible for all edits. KPW participated in discussions about analytic model development, performed analyses, and assisted with interpretation of the data. MK drafted sections of the manuscript, and performed analyses. All authors read and approved the final manuscript.
Competing interests
The authors declare that they have no competing interests.
Consent for publication
Ethics approval and consent to participate.
Institutional Review Board approval was obtained for this study, which included de-identified, cross-sectional data.
Author information
Authors and affiliations.
Division of Epidemiology, Department of Family Medicine and Population Health, School of Medicine, Virginia Commonwealth University, 830 E. Main St., 8th Floor, Box 980212, 23298-0212, Richmond, VA, USA
Resa M. Jones, Kara P. Wiseman & Marina Kharitonova
Massey Cancer Center, Virginia Commonwealth University, Richmond, VA, USA
Resa M. Jones
Center on Health Disparities, Virginia Commonwealth University, Richmond, VA, USA
You can also search for this author in PubMed Google Scholar
Corresponding author
Correspondence to Resa M. Jones .
Rights and permissions
Open Access This article is distributed under the terms of the Creative Commons Attribution 4.0 International License ( http://creativecommons.org/licenses/by/4.0/ ), which permits unrestricted use, distribution, and reproduction in any medium, provided you give appropriate credit to the original author(s) and the source, provide a link to the Creative Commons license, and indicate if changes were made. The Creative Commons Public Domain Dedication waiver ( http://creativecommons.org/publicdomain/zero/1.0/ ) applies to the data made available in this article, unless otherwise stated.
Reprints and permissions
About this article
Cite this article.
Jones, R.M., Wiseman, K.P. & Kharitonova, M. Association between high school students’ cigarette smoking, asthma and related beliefs: a population-based study. BMC Public Health 16 , 913 (2016). https://doi.org/10.1186/s12889-016-3579-7
Download citation
Received : 19 April 2016
Accepted : 23 August 2016
Published : 01 September 2016
DOI : https://doi.org/10.1186/s12889-016-3579-7
Share this article
Anyone you share the following link with will be able to read this content:
Sorry, a shareable link is not currently available for this article.
Provided by the Springer Nature SharedIt content-sharing initiative
- Adolescents
- Smoking-related beliefs
BMC Public Health
ISSN: 1471-2458
- General enquiries: [email protected]
Log in using your username and password
- Search More Search for this keyword Advanced search
- Latest content
- Current issue
- Anniversary
- BMJ Journals More You are viewing from: Google Indexer
You are here
- Volume 12, Issue suppl 1
- Smoking on the rise among young adults: implications for research and policy
- Article Text
- Article info
- Citation Tools
- Rapid Responses
- Article metrics

- Correspondence to: Paula M Lantz, PhD, Department of Health Management & Policy, University of Michigan School of Public Health, 109 Observatory Rd. Room M3116, Ann Arbor, MI 48109-2029, USA; plantz{at}umich.edu
This paper investigates hypotheses regarding the cause of the recent apparent increase in young adult smoking, compares trends in smoking among young adults with trends in the use of other substances, and considers the implications for youth tobacco control research and policy. Time series analyses of national data suggest that the recent observed increase in smoking among young adults is primarily an artefact of the almost simultaneous increase in smoking among high school students. In addition, however, it also appears that there have been real changes in smoking patterns among young adults. While many questions remain regarding recent trends in tobacco and other drug use among adolescents and young adults, what is known leads to a clarion call for increased intervention and policy action for the prevention and control of tobacco use among young adults in the USA.
- adolescence
- young adults, high school students
- college students
- smoking patterns
- CAS, College Alcohol Study
- CPS, Current Population Survey
- NHIS, National Health Interview Survey
https://doi.org/10.1136/tc.12.suppl_1.i60
Statistics from Altmetric.com
Request permissions.
If you wish to reuse any or all of this article please use the link below which will take you to the Copyright Clearance Center’s RightsLink service. You will be able to get a quick price and instant permission to reuse the content in many different ways.
In the late 1990s, evidence from a number of different sources pointed to a disquieting trend: cigarette smoking among college students in the USA was on the rise. Wechsler et al sounded one of the first alarms, reporting that longitudinal data from 130 college campuses showed that the prevalence of self reported smoking in the past 30 days increased from 22.3% in 1993 to 28.5% in 1997, an increase of approximately 28%. 1 A 1999 follow up survey (also conducted on a nationally representative sample of four year colleges) confirmed an increase in the prevalence of cigarette smoking among college students. 2
Data from the Monitoring the Future project also demonstrate that there was an increase in the prevalence of cigarette smoking among college students in the late 1990s. This upsurge, however, was observed among young adults in general—both those enrolled and not enrolled in college. 3 Between 1993 and 1999, the 30 day prevalence of cigarette smoking rose by 25% among college students 1–4 years beyond high school, and by about 21% for young adults 19–24 who were not in college. Although year 2000–01 data suggest a decrease in smoking among both college students and young adults not in school, these trends remain quite disturbing. At the present time, it is estimated that there are approximately 11 million smokers between the ages of 19 and 25 in the USA. 4
Several questions emerging from these general trends should resonate with those interested in youth tobacco prevention and control. A primary issue is the extent to which trends among young adults are reflections of previous trends in smoking behaviour among teenagers—that is, the apparent increase among young adults may be a cohort effect reflecting increased use among adolescents a few years earlier. In addition, however, these trends raise the spectre that there has been a true increase in the number of young adults initiating cigarette smoking after high school. From this, a cascade of concerns and additional questions flows. What might explain the apparent increase in the initiation of tobacco use among young adults? Is it possible that some tobacco control strategies aimed at adolescents are merely delaying or deferring initiation rather than preventing it? Have tobacco control advocates and policymakers been remiss in not focusing more resources on young adults?
The purpose of this paper is to explore the issue of recent trends in cigarette smoking among young adults in significant depth. This paper has the following objectives: (1) to review the published literature regarding trends in smoking behaviour among young adults in the USA over the past two decades, comparing college students and non-college young adults; (2) to investigate hypotheses regarding the causes of the apparent increase in smoking in the late 1990s; (3) to explore key issues that arise from a deeper understanding of these concurrent trends and various explanations regarding the causes and driving forces behind them; and (4) to make recommendations for tobacco control research and policy.
Review of published literature and trend data
Much of the information presented and reviewed in this paper was obtained from published manuscripts and abstracts. In addition, information on trends in adolescent and young adult smoking was obtained from a number of published reports and public websites. A major source of information for this paper came from the Monitoring the Future project, including a recent report on substance use among college students and adults aged 19–40 years. 3
Trend analysis of Monitoring the Future data
Annual data from the Monitoring the Future project were analysed using time series analytic techniques to detect whether or not the trend toward increasing smoking among high school students in the mid 1990s is related to the subsequent increase in smoking among college students. The goal here was to explore the hypothesis that the recent increase in young adult smoking is an artefact (or cohort effect) of the observed increase in smoking among teenagers. Using time series modelling (with lag functions), an analysis was conducted to see if there is statistical evidence of such a cohort effect. The dependent variable used for this analysis was 30 day prevalence of cigarette smoking (that is, whether or not someone had smoked a cigarette in the past 30 days).
Secondary analysis of 2000 NHIS data
Data from the 2000 National Health Interview Survey (NHIS) were analysed to assess recent trends in smoking behaviour among young adults. Following the methods of Pierce et al , 5 adult survey respondents were classified into birth cohorts (in this case, single year birth cohorts). Analyses were performed on 18 birth cohorts from 1960 through 1977. People in these birth cohorts turned 18 from 1978 to 1995, and turned 21 from 1981 to 1998. Restricting the sample to these birth cohorts means that all subjects for these analyses were 23–40 years old at the time of the NHIS 2000 survey. Including more recent birth cohorts was possible, but given that the people in these cohorts were 18–22 years old at the time of the survey and thus had not yet completed their early adult years, results regarding tobacco use patterns during this time period would be biased (and almost certainly in the direction of underestimation).
During the NHIS interview process, respondents who report smoking 100 or more cigarettes during their lifetime are categorised as “ever smokers” and asked a series of additional questions. The main variable of interest for this analysis was the age at which “ever smokers” reported becoming regular smokers (worded as “at what age did you become a fairly regular smoker?”). Subjects responded with an age, that they never were a regular smoker, that they didn’t know, or that they refused to answer the question. Age at initiation of regular smoking was recoded to create a number of new variables including the establishing of regular smoking before age 15, before age 18, at age 18, or at ages 19–21. Other NHIS variables under analysis included ones related to current cigarette smoking status, the use of other tobacco products, and smoking cessation behaviour.
Variables of interest were analysed by birth cohort to detect any trends or changes over time. Most analyses were conducted for the entire sample and by sex. All analyses used weighted data to correct for the complex sampling design of the NHIS. The strengths of the NHIS data—which present a cross-sectional picture across a number of age groups and thus birth cohorts—are that the experiences of several cohorts can be analysed using only one year of data. A limitation, however, is that everyone is answering questions in the year 2000, when they were asked to recall specific aspects of their smoking initiation and early smoking behaviour. The further people are away from these experiences, the more likely recall bias might influence their responses. Thus, any changes observed across cohorts may reflect, in part, the fact that older cohorts are further away from the actual experiences in question.
Recent trends in smoking among young adults
Monitoring the future results.
A very useful and informative source of information on trends in tobacco and other drug use among American youth is the Monitoring the Future project, an ongoing research programme conducted since 1975 out of the Institute for Social Research at the University of Michigan. 3, 6 Since 1976, a representative sample of the high school seniors participating in the Monitoring the Future surveys has received periodic follow up surveys. Thus, the Monitoring the Future project provides information on the drug related behaviour of high school students and adults through the age of 40 at the present time.
Data available from the Monitoring the Future Project demonstrate that there has been a significant increase in cigarette use among young adults during the past decade, for both those in and not in college. Figure 1 shows trends in the 30 day prevalence of cigarette smoking (panel A) and the 30 day prevalence of daily smoking (panel B) over the past two decades, for full time college students, for others 1–4 years past high school, and high school seniors. 3 For all three groups, the trends are similar. There was a decrease in cigarette smoking behaviour during the 1980s. In the early 1990s, however, smoking prevalence began to increase among all three groups, with an especially strong increase among high school seniors. The 30 day prevalence of smoking continued to increase through the 1990s for high school seniors, peaking in 1997 and decreasing thereafter. The trend lines for full time college students and other young adults peaked in 1999, and showed a decrease for the first time in many years in 2000.
- Download figure
- Open in new tab
- Download powerpoint
Monitoring the Future trend data regarding smoking. Reproduced from Johnston et al , 3 with permission. (A) Trends in 30 day prevalence of cigarette use. (B) Trends in 30 day prevalence of daily cigarette use.
Regarding college students, Johnston et al report: “Between 1990 and 1999, the 30-day prevalence of cigarette smoking by college students rose from 23% to 31%, or by about one-third, and daily smoking rose from 14% to 19%—or by about 40%.” 3 Between 1980 and 1994, female college students had higher rates of smoking than males. However, a crossover occurred in 1995, and since this time rates of smoking among college males have been slightly higher than those for females. It is believed that this crossover reflects a similar sex crossover in smoking behaviour that occurred among high school seniors a few years earlier. 3
Young adults 1–4 years beyond high school but not in college have a higher prevalence of smoking than those in college, with 30 day prevalence rates in the mid to late 1990s ranging from 35–42%. Rates of heavy smoking are also significantly greater among young adults not in college. For example, in the year 2000, 23.7% of young adults not in college reported smoking a half pack or more a day, compared with 10.1% of full time college students. Despite the greater prevalence of smoking among non-college young adults, the relative increases in smoking were much greater among college students. For example, between 1990 and 1999, the 30 day prevalence of daily smoking increased by approximately 25% for non-college young adults, yet by almost 60% for full time college students. 3
In summary, Monitoring the Future data provide clear and credible evidence that there were significant and alarming increases in cigarette smoking among both male and female high school students and young adults during the 1990s. 3, 6 Trends in self reported smoking behaviour are paralleled by trends in the percentage of high school seniors and young adults reporting that most or all of their friends smoke. 3 While the most recent data are suggestive of a decline or at least a plateau in the trend line, the results for the 1990s are unmistakable: high school students, college students, and young adults not in college all experienced a significant upsurge in cigarette smoking during the 1990s.
Harvard School of Public Health College Alcohol Studies
Another very useful source of information regarding trends in cigarette smoking among college students in the USA is the Harvard School of Public Health College Alcohol Study (CAS). The CAS involves a random sample of undergraduates at a nationally representative sample of four year colleges. While the primary focus of the survey is alcohol behaviour, information about cigarette smoking is included as well.
Results from the 1993 CAS included that 22.3% of full time college students had smoked in the past 30 days, with an additional 25% reporting that they were former smokers. 7 Wechsler et al looked at changes in smoking prevalence between the 1993 and 1997 surveys, and discovered the alarming finding reported above. 1 Over this five year period, there had been a 27.8% increase in cigarette smoking prevalence (defined as smoking during the past 30 days). In addition, it was reported that there was a decrease in smoking rates at the “extremes” of smoking behaviour: there were fewer very light smokers (< 1 cigarette per day) and fewer heavy smokers (> 20 per day).
More recently, Rigotti et al analyzed data from a third CAS, conducted in 1999. 2 Their findings included that more than 60% of college students had tried some sort of tobacco product in their life, that almost half (45.7%) had used some sort of tobacco product in the past year, and that a third (32.9%) were current users in terms of 30 day prevalence of any tobacco use. 2 The 30 day prevalence rate of cigarette use in 1999 was similar to the rate in 1997, suggesting a plateau in the upsurge among college students. While cigarettes accounted for the majority of tobacco ingested by college students in 1999, cigars also were a significant source of tobacco for males. Even though cigarette smoking rates were similar for males and females (28.4% v 28.5%, respectively), males had a higher overall rate of tobacco use in the past 30 days because of their higher use of cigars (15.7% v 3.9%) and smokeless tobacco (8.7% v 0.4%). 2
Other studies
Selected major findings from a number of data sources are summarised in table 1. The 1995 National College Health Risk Behaviour Survey included students at both two and four year institutions. 8 Nearly three quarters of the respondents (74.8%) reported that they had ever tried a cigarette, and 29% had smoked at least one cigarette in the past 30 days. Whites and those at two year institutions were more likely to report ever and recent smoking. This study was not longitudinal. Thus it cannot offer insights regarding trends.
- View inline
Prevalence of smoking in past 30 days among young adults in major national studies
Results from the NHIS suggest that the rate of current smoking among 18–24 year olds was 23.5% in 1991. 9 This rate had risen to 28.6% in 1997, a 22% increase. 10 Although the definition of current smoking in the NHIS changed slightly in 1992 (from having ever smoked 100 or more cigarettes and currently smoking to having ever smoked 100 or more cigarettes and now smoking every day or some days), this change is not responsible for the increase in smoking observed among 18–24 year olds during the 1990s. In fact, no other adult age group experienced an increase in smoking rates over this time; the only increase in current smoking occurred in the youngest adult age group.
Data from the NHIS also signal that, starting around 1997, the prevalence of smoking among those 18–24 years old was as high as those 25–44 years (for example, 28.7% v 28.6% in 1997, and 26.8% v 27.0% in 2000) (Gary Giovino, personal communication on unpublished NHIS results, May 2002). In prior years, NHIS results consistently showed the highest prevalence of smoking in the 25–44 age group, and significantly lower rates in the youngest adult years. This changed, however, in 1997 when two important trend lines met: while smoking rates for adults 25–44 years old were declining in the early to mid 1990s, they were simultaneously increasing among younger adults. NHIS data also suggest that, between 1997 and 2000, the prevalence of smoking among young adults 18–24 years old and those 25–44 was similar and was decreasing slightly.
NHIS results from the year 2000 (data not shown) also demonstrate that young adult males are significantly more likely to use other types of tobacco products than females, and that this pattern holds across birth cohorts. The use of cigars and bidis among males appears to have increased somewhat across birth cohorts reaching age 21 between the years of 1991 and 1998, and a significant proportion of males (over 25%) report having used smokeless tobacco products. This is alarming given the finding that smokeless tobacco use is a significant predictor of cigarette smoking initiation among young adult males. 11, 12 Also alarming are data suggesting that the consumption of cigars increased dramatically between 1993 and 1998, with a slight decrease after that year. 13
Major explanations for trends in young adult smoking
The compositional change hypothesis.
There are many possible explanations for the trends in young adult cigarette smoking described above. One explanation for the increase in smoking among college students is that it is an artefact of a compositional change in the US college student population. Historically, young adults not in college have had higher rates of smoking than those of a similar age but enrolled in college. 3 If more young adults are attending college, it is possible that increases in student smoking represent a change in the types of young adults attending school rather than a true increase in the prevalence of cigarette smoking. According to data from the Current Population Survey (CPS), 14 there has been a slight increase in the proportion of high school graduating seniors who attend college. In 1995, 62.0% of graduating high school seniors were enrolled in a college or university in the fall. This proportion climbed to 67.0% in the fall of 1997, which was a record high. In 1998, the proportion dropped to 65.6%, and fell to 62.9% in 1999 and 63.0% in 2000.
The CPS statistics suggest that the increase in the proportion of high school seniors attending college occurred at the same time that college smoking rates were increasing. Thus, it is possible that part of the increase in smoking observed among college students is due to a compositional change in the types of students who are attending college. The amount of the increase explained by such a change, however, is likely to be quite small. First, the increase in college enrolment, while certainly noteworthy, is not of such a magnitude that it would have a significant compositional effect. Second, there have been increases in smoking among young adults both in and not enrolled in college. Thus, the compositional change explanation, while worth considering, is not credible as a major explanation for the increase in young adult smoking.
The cohort effect hypothesis
A second hypothesis to consider is that the recent observed increase in smoking among young adults in the USA is an artefact of the almost simultaneous increase in smoking among high school students. Between 1991 and 1997, cigarette smoking among youth increased significantly according to several different data sources. 3, 15 Monitoring the Future data suggest a 32% increase in 30 day prevalence of any smoking among high school seniors during this time period. 10 Longitudinal analysis of Monitoring the Future data have established the existence of cohort effects: “if a class (or birth) cohort establishes an unusually high rate of smoking at an early age relative to other cohorts, the rate is likely to remain high throughout the life cycle relative to that of other birth cohorts at equivalent ages.” 3
Given the significant increase in smoking among high school students in the 1990s, the observed increase in smoking among young adults is often presumed to reflect the aging of adolescent cohorts with higher smoking rates. 2, 3 There are indeed data to support the cohort hypothesis. First, Monitoring the Future results do suggest that the increase in smoking among high school seniors predates the observed increase among young adults ages 19–24. An increase in 30 day prevalence of smoking was first observed in 1993 for high school seniors, and increases were subsequently observed for 19–20 year olds in 1994, for 21–22 year olds in 1995, and for 23–24 year olds in 1996. These results are quite suggestive of an aging cohort effect.
Second, time series modelling results suggest that the two trend lines—that is, trends in the 30 day smoking prevalence rates for high school seniors and young adults 1–4 years out of high school between 1980 and 2000—are significantly related to one another. Specifically, the results showed that rates of current smoking among high school seniors explained three quarters of the variance in the rates of smoking among all 19–20 year olds over the 21 year time period under study, using a one year lag function (R 2 = 0.756, p < 0.001). In addition, smoking rates among high school seniors explained two thirds of the variance in current smoking rates among all 21–22 year olds, using a three year lag function (R 2 = 0.67, p < 0.001). Similarly, smoking rates among high school seniors explained three quarters of the variance in smoking among college students 1–4 years out of high school, using a two year lag function (R 2 = 0.765, p < 0.001). Thus, rates of smoking among high school seniors are highly correlated with and explain the majority of the variance in subsequent smoking rates among young adults.
Nonetheless, there are also some disturbing aspects of observed trends that argue against a pure cohort effect. First, when the trend lines for college students and young adults not in school are separated (fig 1), one can see that increases in 30 day prevalence of any smoking and the 30 day prevalence of daily smoking among college students really started before 1990. 3 As such, it appears that the increase in smoking among college students actually was apparent before the upsurge in smoking among high school seniors (which appears to have started in 1992–93).
Second, similar to trends in cigarette consumption, the use of illicit drugs increased dramatically among high school students and young adults in the USA during the 1990s. 16, 17 Between 1980 and 1992, most illicit drugs showed a strong decrease in use among high school students, college students and young adults not in college. 3 After 1992, however, a number of drugs—including marijuana—showed a clear increase in use among adolescents (both males and females), with smaller increases for a number of substances among young adults. Rates of any illicit drug use increased in a startlingly way between 1992 and 2000 as follows: from 27.1% to 40.9% for high school seniors, from 29.7% to 39.3% for young adults ages 19–20, and from 30.0% to 36.9% for young adults ages 21–22. 3, 18 These rates began to stabilise slightly in the late 1990s.
Monitoring the Future investigators believe that much of the increase in illicit drug use among young adults is a cohort phenomenon, the result of adolescent cohorts with significant increases in use aging into young adulthood. 3 However, increases in the use of some drugs occurred simultaneously among high school students and young adults (both those in and not in college), including increases in the use of marijuana, hallucinogens, and amphetamines. Interestingly, Gledhill-Hoyt et al report that nearly a third of college marijuana users initiated use while in college. 16
Compared with other substances, trends in alcohol use are somewhat different. During the time period that smoking was significantly increasing among high school students and young adults in general, alcohol consumption was experiencing a slight increase in terms of 30 day prevalence and binge drinking. 19, 20 These increases are especially noteworthy as they started in the mid 1990s after a nearly decade long decline. 20, 21 Thus, while the observed increases are not as dramatic as those for smoking or for illicit drug use, these small increases represent a clear and definite shift in a trend line.
In summary, while the cohort hypothesis likely has some degree of explanatory power, it is probably not the full explanation for the observed increase in smoking among young adults. The story becomes more complex when we look at some of the details in the trend lines, and we also consider the fact that a broader phenomenon regarding other substance use among adolescents and young adults was occurring at the same time. In general it appears that increases in smoking have occurred at the same time as increases in the use of other tobacco products, binge drinking, and the use of many types of illicit drugs, including marijuana. Given the strong evidence that risk taking behaviour regarding substances in general was on the rise among youth and young adults during the 1990s, it is clear that the case of cigarette smoking should not be viewed as an isolated phenomenon.
The change in age at initiation or habitual smoking hypothesis
In attempting to explain the increase in cigarette smoking among young adults in the USA, it is also important to consider whether there have been any changes in the age of smoking initiation or habitual smoking. Becoming a regular or habitual smoker is described as a process or a series of transitions through several stages, starting with the first “initiating” puff on a cigarette. 22, 23
The epidemiology of cigarette smoking initiation in the USA includes one clear and consistent finding: the majority of people who end up being habitual smokers initiate experimentation with smoking as children or adolescents. 15 Data from a variety of sources consistently have shown that the vast majority of people who try a cigarette for the first time are under 18, and that the majority who become daily smokers do so by or at age 18.
There is no doubt that, even in the face of increased smoking rates among young adults, cigarette smoking initiation remains primarily an activity of minors. Even so, there may have been changes in some aspects of the process of being a regular or habitual smoker. In particular, it may be that there have been changes in age distribution of habitual or regular smoking. Below, results from an analysis of data from the 2000 NHIS regarding smoking behaviour in early adulthood across birth cohorts (from 1960 to 1977) are presented in an attempt to shed a bit more light on the hypothesis that there have been changes in age specific smoking patterns concomitant with the observed increase in cigarette use among young adults.
Trends in ever smoking 100 cigarettes
NHIS data from 2000 show that, across the 18 age cohorts, between a third and a half of adults ages 23–40 reported that they have smoked 100 or more cigarettes in their lifetime (table 2). There does appear to have been a slight increase in the proportion of adults reporting ever smoking 100 cigarettes for more recent birth cohorts (those turning 21 between 1996 and 1998), although this change is not statistically significant. There also appears to have been a slight increase in the rate of current smokers over time, with people from younger age cohorts (those born in 1975–77) having a higher rate of current smoking than those in older cohorts. This is consistent with the findings regarding an increase in smoking prevalence among young adults described above. However, given that the NHIS findings presented here involve cross-sectional analysis only, it is possible that this pattern is explained in part by older respondents having had more time to engage in smoking cessation.
2000 National Health Interview Survey results: smoking prevalence by birth cohort
Trends in age at initiation of regular smoking
The 2000 NHIS survey data suggest that the mean and median age at which regular smoking was established did not change much over these 18 birth cohorts. On average, over time, the mean age for regular smoking has been 20.8 years (with a median of 17 years). While there was a decrease in the mean age of establishment of regular smoking for the 1973 and 1974 birth cohorts (17.5 and 18.7 years, respectively), more recent birth cohorts have average ages of initiation of regular smoking that are quite similar to the general pattern over time.
As shown in table 3, the proportion of ever smokers establishing habitual smoking habits by the age of adulthood has fluctuated some over time, but does appear to have risen somewhat for cohorts born after 1970 (that is, for those turning 18 during the early 1990s). For example, 66.8% of ever smokers in the 1970 birth cohort became regular smokers by age 18, compared with 74.5% in the 1976 birth cohort. When coupled with trends regarding the mean age at initiation, these results support a well accepted tenet in tobacco prevention circles: that most smokers initiate smoking behaviour as adolescents, and that—for the vast majority of smokers—smoking became a regular activity or habit at age 18 or younger. Data from the 2000 NHIS do not suggest any sort of significant deviation from well established and well understood patterns of regarding youth smoking in the USA.
2000 National Health Interview Survey results: patterns in the age of initiation of regular smoking by birth cohort
However, the NHIS results do suggest that the rate at which ever smokers establish regular smoking between the ages of 19–21 has experienced an increase in recent years (table 3). In particular, the proportion of ever smokers who report the establishment of habitual smoking at the ages of 19, 20, or 21 appears to have increased for more recent birth cohorts, specifically the 1975–77 birth cohorts. People in these birth cohorts turned 21 between 1996 and 1998, years in which smoking among young adults was increasing. For example, 13.5% of ever smokers in the 1974 birth cohort reported becoming regular smokers in early adulthood (as opposed to age 18 or younger), compared with 17.8% in the 1975 birth cohort and 21.7% in the 1977 birth cohort (table 3). For the most recent cohorts, approximately one out of four smokers became a regular smoker between the ages of 19 and 21. Time series analysis shows that the change in the slope of this trend line is close to being significant at the 0.05 level (p = 0.058). While more data points are needed to reach any solid conclusions, the current data do suggest that a significant proportion of smokers are making the transition to habitual smoking as young adults and that this appears to have increased in frequency among more recent birth cohorts.
It is also interesting to note that the proportion of ever smokers (100 or more cigarettes) who report that they never became a “habitual smoker” was similarly low (less than 6%) across the birth cohorts under study (table 3). Thus, it appears that most people who have ever had 100 cigarettes become what they consider to be a regular smoker at some point, and that the rate of experimenters who do not become regular smokers has not changed much over the past two decades. Even so, some people who smoke regularly can do so without smoking daily. These people are referred to as “intermittent smokers”. 24 NHIS data show that the prevalence of intermittent or non-daily smokers has increased over the birth cohorts under study, from a rate of 12.1% of ever smokers in the 1960 cohort to 20.8% in the 1977 cohort (table 3). Time series analysis revealed that there has been a significant change in the slope of this trend line (p = 0.001), suggesting that the rate of intermittent smoking among adults is indeed higher among more recent birth cohorts, or those who reached young adulthood during the years where smoking prevalence among young adults was increasing.
Sex difference
Some interesting sex differences in smoking patterns are apparent in the 2000 NHIS data (table 4). First, the proportion reporting ever smoking 100 cigarettes is higher among males than females in all birth cohorts. Second, changes in the age at regular smoking are stronger for males than females. Using the last four years of available NHIS data (representing those who turned 23 between 1997 and 2000), the proportion of males establishing regular smoking by age 18 decreased from 80.3% for the 1974 birth cohort to 67.4% for the 1977 birth cohort, compared with 77.8% and 73.6% for the 1974 and 1977 birth cohorts, respectively, for females. Similarly, the proportion of males who reported becoming a regular smoker at ages 19–21 increased by 75% comparing the 1970 birth cohort with the 1977 birth cohort (14.0% v 24.5%). The proportion for females across this same time period increased by 5.5% (with some fluctuations—see table 4). These trend data suggest that the phenomenon of an increase in the rate of habitual smoking initiation is much stronger for males.
2000 National Health Interview Survey results: age at which respondent became a regular smoker by birth cohort and sex
In summary, the epidemiology of cigarette smoking indicates that smoking initiation primarily occurs during adolescence. Evidence from a number of sources suggests that this pattern has intensified during the past two decades. The majority of smokers are still trying their first cigarette in early adolescence, and making the transition to habitual smoking by age 19. However, it is also the case that a significant proportion of smokers establish regular or habitual smoking as young adults. Analyses of NHIS survey data suggest that this proportion has been sizeable for some time, and that it increased, particularly among males, during the late 1990s. In addition, the proportion of current smokers who do not smoke daily has significantly increased among younger birth cohorts.
These findings are paralleled by data from a number of other recent surveys, including results from the 1999 National Youth Tobacco Survey, which show that the proportion of 18 and 19 year olds classified as “non-daily smokers” or “experimenters” was greater than the proportion of current smokers. 25 In addition, trend data from the National Household Survey on Drug Abuse show that the rate of initiation of daily cigarette use among both 12–17 year olds and 18–25 year olds increased during the 1990s. 26 For young adults (18–25 years), the rate of initiation for daily smoking (per 1000 person years of exposure) jumped from 28.9 in 1990 to 34.7 in 1997. 26
Along with a “cohort effect” (whereby cohorts with increased rates of adolescent smoking carried their smoking rates into their young adult years), the increase in smoking prevalence among young adults also appears to be occurring because there has been an increase in the rate at which young adults who have experimented with cigarettes become regular smokers. For those who turned 21 in 1998, approximately 1 out of 5 female smokers and 1 out of 4 male smokers established regular smoking after the age of 18. It appears that there have been some changes in smoking behaviour patterns among young adults above and beyond an increase in prevalence caused by a cohort effect.
INDIVIDUAL RISK FACTORS AND SOCIAL ENVIRONMENT CONSIDERATIONS
Recent results from the 1998–99 Tobacco-Use Supplement to the CPS suggest that, among young adults ages 18–24, current smokers (26% of the sample overall) were more likely to be male (29%), white (31%) or American Indian (35%), unemployed (36%), or blue collar (34%) or services workers (32%). 27 There is very little in the published literature regarding risk factors for smoking among young adults not in college. In contrast, the Harvard College CAS have provided valuable insights regarding individual risk factors for smoking among college students.
Multivariable analysis of the 1993 CAS data led Emmons et al to conclude that other lifestyle choices are significantly associated with cigarette smoking in the past 30 days among college students. 7 This includes using marijuana, heavy drinking, and having multiple sex partners. The attitudes that parties are a very important or important part of college life and that collegiate athletics and religion are not very important also were significantly related to smoking. In addition, Emmons et al found that white students, those belonging to a fraternity or sorority, and women living in a co-ed dorm had a higher risk of smoking. Similar to findings from the 1993 CAS data, 1999 college students who used tobacco were more likely to be white and to experiment with other risky behaviours (for example, binge drinking, marijuana use, and multiple sexual partners) than non-smokers. 2
Analysing predictors of “late onset smoking” (defined as establishment of smoking after high school), Ellickson et al found that lower parental education, worse grades in high school, and younger age relative to others in a grade cohort were significant risk factors. 28 In a longitudinal study of college bound high school students who reported never experimenting with tobacco, Choi et al found that—four years later—14% had initiated smoking. 29 Risk factors for this late initiation included being white, having more depressive symptoms, attending church less often, believing that peers approve of smoking, and believing that experimenting with cigarettes is safe.
Wee et al found that adults younger than 30—both male and female—are more likely to smoke if they are trying to lose weight. 30 Weschler et al reported that a prominent perception among health centre directors on college campuses is that students smoke for a variety of reasons—including as a response to stress and as tool for weight control—and that many students do not believe they are addicted and that they will quit upon graduation. 31
In addition, it is believed that smoking reduces and, for some people, fully relieves anxiety in a variety of social situations. Sonntag et al reported that social anxiety has been significantly associated with nicotine dependence in both cross-sectional and longitudinal studies. 32 In addition Anda et al claimed that their results from the Adverse Childhood Experiences Study contribute to a growing literature suggesting that “nicotine use is associated with self-medicating efforts to cope with negative emotional and social experiences”. 33 A significant, graded relation was found between smoking and the number of adverse childhood experiences, including emotional, physical, and sexual abuse; a battered mother; parental separation/divorce; and growing up with a substance abusing, mentally ill, or incarcerated household member.
Are adolescents and young adults smoking cigarettes more because of increased feelings of social anxiety and pressure? Are they trying to “self medicate” to relieve stress or emotional pain in some way? The myriad reasons that adolescents and young adults are smoking cigarettes and using other substances are complex and not well understood. A full review of the literature on this topic is outside of the scope of this paper. However, a prominent social environment hypothesis regarding the increase in smoking among young adults is that the tobacco industry has intensified its activity in this market segment—that is, more aggressive industry marketing activities may be partly responsible for the increase in smoking observed among college students and young adults in general.
Using tobacco industry documents that have become public in the wake of litigation, Katz and Lavack, 34 Sepe et al , 35 and Sepe and Glantz 36 have argued that changes in industry promotional tactics correspond with the increase in smoking observed among young adults. These marketing tactics have taken many forms since the late 1980s: (1) promotions in bars, nightclubs, comedy clubs, and other venues that use person-to-person interactions, free samples, free promotional accessories, contests, and games; (2) efforts to cultivate “brand presence” in bars, including company branded items (such as napkins, coasters, clothing for employees, etc), and financial incentives for owners and employees; and (3) increased use of the alternative press (especially weekly alternative newspapers in urban areas) for several purposes, including product advertisement, event promotion, and bar promotion.
Adult only facilities—such as bars and nightclubs—are exempt from the 1998 Master Settlement Agreement in terms of marketing activities. Sepe et al argue that tobacco industry bar and nightclub promotions “protect the industry from advertising regulations, clean indoor air laws, and accusations of marketing to adolescents. Bar promotions help the industry engineer peer influence to encourage tobacco use among young adults.” 35
Sepe and Glantz wrote that young adults “are not immune to ‘late’ initiation of smoking . . .. Directed marketing toward young adults in social settings such as bars and nightclubs may raise the age at initiation toward what it was in the past. Current increases in young adult smoking, in terms of both overall prevalence and first use, suggest that this directed marketing is having an impact.” 36 Thus, the argument is being made that observed increases in smoking among young adults are in part explained by tobacco industry promotional tactics.
There is a growing body of research literature reporting associations between exposure to tobacco industry marketing/promotions and smoking behaviours, particularly among youth. 37– 40 Thus, is not unreasonable to consider the hypothesis that increased efforts targeting young adults have reaped benefits for the industry. Although spending on tobacco advertising remained relatively constant between 1988 and 1998, promotional allowances tripled in size during this time period. 41, 42 As has been argued in the past, the industry’s continued investment in specific types of promotion and marketing suggests that those within in the industry itself must have some evidence or reason to believe that these tactics are effective. 43
Ling and Glantz have attempted to shed light on why the tobacco industry has intensified its marketing efforts among young adults. They explain:
“First, the industry views the transition from smoking the first cigarette to becoming a confirmed pack-a-day smoker as a series of stages that may extend to age 25, and it has developed marketing strategies not only to encourage initial experimentation (often as teens), but also to carry new smokers through each stage of this process. Second, industry marketers encourage solidification of smoking habits and increases in cigarette consumption by focusing on key transition moments when young adults adopt new behaviors, such as entering new workplaces, school, military, and especially leisure and social activities. Third, tobacco companies study young adults’ attitudes, social groups, values, aspirations, role models, and activities, and infiltrate both their physical and social environments.” 44
Evidence from industry documents confirms that the tobacco industry has invested significant time and resources into studying youth and young adult development, motivations, and social environments, and that this research has helped them to divide potential and actual smokers into different markets or segments. 22, 40, 45 As described above, a number of recent articles provide provocative new evidence and ideas regarding tobacco industry strategies and trends in smoking among young adults. A note of caution, however, needs to be raised. The evidence to date is of a simple ecological nature: smoking rates among young adults rose several years after the industry first introduced promotional activities in bars, nightclubs, and other venues targeting young adults (in the late 1980s), and shortly after these types of efforts were intensified (in the early to mid 1990s). Rigotti et al recently found that, controlling for a number of potential confounders, those college students who report exposure to bar and campus tobacco promotional events do have higher rates of smoking, and that this association is only observed among those who became smokers as adults. 46 However, showing temporal associations and establishing causation are, of course, two different things. Thus, although certainly provocative and compelling, the evidence to date does not conclusively show a causal link between industry tactics and the increase in smoking among young adults.
RESEARCH AND POLICY IMPLICATIONS
As described above, much has been written about the apparent increase in smoking among college students. Increases in smoking, however, have not been observed exclusively in the college population. Significant increases also have been witnessed among young adults in general, and importantly among high school students. There is credible evidence that some of the observed increase among young adults is an artifactual result of the aging of cohorts with increased smoking among youth. In addition, there is information indicating that other factors may be at play as well. Recent NHIS data suggest that there was an increase in the rate at which young adults became “regular” or habitual smokers at the same time as the observed increase in smoking prevalence, especially among males. In addition, the increase in cigarette smoking has occurred concomitantly with an increase in other risk taking behaviours regarding substance use, including binge drinking and the use of marijuana and other illicit drugs.
The reasons for the increase in smoking among young adults are not clear, and there are many questions that remain unanswered at this point in time. Additional research is needed in multiple areas, including research that will help to answer the following questions:
What sociodemographic and behavioural characteristics are associated with changes in the smoking behaviour of young adults? What subgroups are at higher risk for becoming habitual smokers as young adults? Are the characteristics or risk factors for habitual smoking initiation the same among college students and those not in school? Is the recent increase really largely a male phenomenon?
How is the increase in cigarette smoking among young adults related to the increase in the use of other substances? Are some of the same causal factors involved across substances?
Are there any tobacco control policies and interventions aimed at adolescents that may be delaying or deferring initiation of habitual smoking rather than preventing it?
How do we best intervene with adults who have just “come of age”? Are young adults more like adults or adolescents in terms of their knowledge and understanding of risk, their motivations, their self perceptions, their attitudes, the social influences that affect them, etc? What needs to be understood about young adults to better inform the design of smoking prevention and control interventions?
Even in the face of these and a number of other unanswered questions, we do have sufficient information and knowledge in hand to consider a number of programmatic and policy responses. The following is a list of potential policy responses and intervention strategies that need to be investigated, debated, and discussed as the tobacco control community further develops an agenda for addressing tobacco use among young adults.
Invest in smoking cessation interventions aimed at young adults
Although rates of smoking cessation have increased among adults over the past two decades, this has primarily been observed among adults ages 45 and older. Among young adults (ages 18–24), the percentage of ever smokers who have quit smoking has remained relatively stable, especially over the past 10 years (Gary Giovino, personal communication on unpublished NHIS results, May 2002). This does not mean, however, that young adult smokers are not interested in quitting. Results from the 1995 National College Health Risk Behaviour Survey included that 59% of current smokers had made at least one quit attempt, and that this rate was 82% among daily smokers. 47 Furthermore, recent results from the 2000 NHIS suggest that among those ages 18–24, over three quarters of current smokers who attempted to quit in the past still would like to quit, and that almost half (44.2%) of those who have zero quit attempts also would like to quit (Gary Giovino, personal communication on unpublished NHIS results, May 2002).
Given the prevalence of smoking and of the desire to quit among young adults, it is important that interventions and resources regarding smoking cessation be made available. According to the 2000 CPS, over 60% of young adults who graduated from high school are enrolled in a college or university; and 80% of young adults who are not full time students are in the labour force. Thus, a significant proportion of young adults can be reached with messages and resources offered through educational institutions and work sites.
Unfortunately, there is very little evaluation literature on smoking cessation interventions aimed at young adults. Thus, it is not possible at this time to make specific recommendations regarding cessation intervention approaches that have proven effective among young adults. We also should recognise that smoking cessation interventions that have been developed for adults in general may not be the best approach to take with younger adults. Those between the ages of 18–25 may be more like adolescents than older adults in their perceptions of risk, their perceptions of themselves as “smokers” or as having an addiction, their attitudes towards different types of cessation messages, and thus their responses to behavioural interventions. Thus, simply increasing the exposure of young adults to the existing arsenal of cessation tools/interventions is likely not the best way to proceed. A significant amount of formative research needs to be conducted in this area (for a start see O’Neill et al , 48 and Martinelli 49 ).
Even so, at this point in time it does seem reasonable to recommend that smoking cessation interventions that have been shown to be effective with adults in general be offered through student health services on college campuses, and that they be part of employee health benefit packages and resources, including typical employment venues of young adults not enrolled in college. Interventions should be tailored to address the attitudes and tobacco use patterns of young adults, recognising that a significant proportion have only recently become regular smokers or still may be intermittent smokers, and that a non-trivial proportion of males also use tobacco products other than cigarettes. Analysis of the impact of standard smoking cessation interventions in the young adult population need to be conducted so we can have some notion of their degree of effectiveness relative to older adults.
Unfortunately, many young adults are without health insurance, and people in this age group (especially males) do not have frequent contact with health care providers. Thus, it will admittedly be a challenge to expose young adult habitual smokers to proven cessation strategies involving clinical interventions combined with nicotine replacement therapy. In addition, data suggest that adolescents and young adults are infrequently asked about their smoking status and counselled regarding cessation during encounters with primary care providers. 50, 51 As such, interventions that do not rely on “teachable moments” with health care providers also must be designed and evaluated.
Wechsler et al conducted a survey of 393 college health centre directors to assess their attitudes about and efforts regarding student smoking. 52 The findings included that while 85% of directors considered smoking to be a serious problem, only 27% prohibit smoking in all indoor areas (which includes private offices and dormitories). In addition, almost half reported that there were no smoking cessation programmes available on their campus, and—among those who do provide cessation resources—the prominent perception was that demand was quite low. Similarly, a study conducted at 11 public colleges in Massachusetts found that “tobacco use among college students was not regarded as a high-priority problem by students or administrators”. 53 Thus, an obvious first step is to engage in efforts that will assist in making tobacco control a priority issue among college and university administrators and health care providers. It is likely that similar educational and “problem definition” efforts will have to be directed at employers and work site health managers.
Invest in smoking prevention interventions aimed at young adults
Given the epidemiology of smoking initiation, focusing prevention and control activities on youth has made great sense. 22, 37 However, the view that this focus on youth may be myopic and even dangerous in some ways has been expressed. Glantz has argued that a primary focus on youth in tobacco control efforts may be counterproductive, as it reinforces tobacco industry depictions of smoking as an “adult” behaviour, and shifts attention away from more comprehensive efforts. 54 Hill has made a similar argument, with a primary concern being that messages that youth should not smoke are likely to reinforce adolescents’ natural rebellious attitudes toward adults. 55 Even if one believes that it is essential to target serious tobacco prevention efforts toward youth, an important admission is that efforts to date, involving a wide variety of interventions, programmes and policies, have been met with limited success. 22, 37
Given current trends and the recognition that an increasing proportion of adult smokers initiate regular smoking after age 18, the time has come to increase prevention and control activities in the young adult population. It is still the case that the majority of smokers are fully engrained in this activity by the time they are 19. However, it appears that currently over 20% of smokers make the transition from occasional to habitual smoker as young adults. As Ling and Glantz have argued: “During the critical years of young adulthood, public health efforts dwindle while tobacco industry efforts intensify . . . Public health efforts should match tobacco industry interest in young adults. Each place where young adults adopt new behaviours also provides opportunities for public health interventions.” 44
Just what these prevention interventions and policies should look like is unclear at the moment. Again, there is very little literature regarding efforts to prevent tobacco use among young adults. Ling and Glantz suggest: “public health campaigns that resonate with the psychological needs and values of both smokers and nonsmokers may improve smoking prevention and cessation efforts. Interventions that affect cigarette prices, acceptance of the tobacco industry, the social acceptability of smoking, and secondhand tobacco smoke particularly threaten the industry.” 45 Jacobson et al suggested that public health practitioners and policymakers can learn a great deal from how the tobacco industry has skilfully marketed its products: “Just as tobacco marketing can influence smoking behaviour, social marketing is a promising approach to smoking prevention, although it does require significant resources and skillful execution.” The literature on social marketing suggests that mass media campaigns increase their chance for effectiveness if: (1) the campaign strategies are based on sound social marketing principles; (2) the effort is large and intense; (3) target groups are carefully differentiated; (4) messages for specific target groups are based on empirical findings regarding the attitudes, beliefs, needs and interests of the groups; and (5) the campaign is of sufficient duration. 22 Ling and Glantz recommend that media messages should not simply attempt to convince individuals not to smoke. 44 They also should support clean indoor air policies, social environments that challenge the social acceptability of smoking, and tobacco excise taxes.
Focusing prevention and control activities among young adults begs the question of the relative degree of focus on the college versus non-college populations. Given that smoking rates have increased more among college students than those not in school, and given the attention that the tobacco industry is giving to this market, one could argue that college students should be the number one priority. However, it is also the case that smoking rates are significantly higher among those not in school. It is likely that the most effective interventions will need to be tailored differently for these different groups of young adults. Tough discussions regarding the best use of limited prevention resources need to occur.
Consider potential counterproductive effects of interventions targeting adolescents
Even if one believes that a strong focus on youth is essential, it is possible that specific types of interventions and strategies are having the counterproductive effect of delaying rather than totally preventing tobacco use. Thus, it is important to consider whether any current youth focused strategies are delaying, deferring, or even encouraging smoking initiation among young adults rather than preventing it. To answer this issue, we would need to review a wide range of evaluations in which the long term effects of interventions were actually tracked into the early adult years. Unfortunately, follow up periods for youth tobacco interventions rarely extend beyond adolescence. An exception to this is the work of Rigotti et al , who analysed 1999 data regarding tobacco use among students at public colleges in Massachusetts and found that those students from this state (and thus ostensibly exposed to the Massachusetts’s youth focused tobacco control programme) had significantly lower rates of current use than those who attended high school in another state (31.5% v 42.6%). 56 These results suggest that exposure to a multiple component, comprehensive tobacco control programme as an adolescent has positive effects that last into young adulthood.
Additional information on the long term effects of youth tobacco prevention and control activities is greatly needed. In addition, it is critical that the tobacco control community invest some time and energy into considering whether or not specific types of youth focused strategies do indeed have the potential for counterproductive delaying effects. For example, it has become increasingly common for youth in possession of cigarettes to receive sanctions through the legal system (including such penalties as a ticket/fine or loss of driving privileges). 22, 54, 57 Such sanctions, of course, do not apply to adults. These negative consequences likely do not prevent experimentation with smoking, yet they may actually reduce youth access and/or persuade some minors to avoid smoking in public places. If such sanctions actually do decrease some minors’ ability and/or willingness to smoke, the transition to habitual smoking may be delayed. However, the desire to be “rebellious” and to engage in what is sanctioned as adult behaviour may have been reinforced (perhaps even intensified) and may remain strong as the adolescent reaches the “legal age” for the behaviour. As such, this may lead to increased smoking among young adults. While one might consider the proposed scenario rather far-fetched, it does seem worthwhile to contemplate potential negative side effects of various types of youth focused interventions. We need to entertain the uncomfortable possibility that strategies and tactics focusing on youth tobacco control are in part contributing to the recent changes in smoking behaviour observed among young adults.
Promote smoke-free environments
The promotion of smoke-free environments should be considered a potentially effective mechanism for decreasing smoking among young adults. These environments include work sites, campuses, restaurants, bars and nightclubs, and even homes. There is a growing amount of evidence that clean indoor air policies can have a positive effect on smokers as well as those at risk for exposure to environmental tobacco smoke. 22, 58 Such policies create social environments that reinforce messages about the negative aspects of tobacco smoke. Such environments may also encourage current smokers to quit or reduce their consumption, and in doing so may prevent some smokers from transitioning into regular, habitual smoking.
Ling and Glantz recommend the promotion of smoke-free homes among young adults: “ . . .educating young adults about the dangers of secondhand smoke may be particularly effective because they are starting new households and new families. Educating young adult parents (and parents to be) about the dangers of secondhand smoke will provide benefits not only for the new child (who will avoid the morbidity associated with involuntary smoking) but may also prompt cessation among the adults.” 44
Several people have argued for the creation of campus wide smoke-free environments (including dormitories and other residences, eating and recreation facilities, classrooms, and private offices). 5, 52 The results of their survey of college health centre directors suggest that some schools are attempting to counter trends in student smoking by implementing no-smoking policies. Such policies, however, must be promoted and enforced if they are to have any effect. Regarding smoke-free bars, Sepe et al stated that the “[c]reation of smoke-free bars—with appropriate ground-work and public education—may be a key to undermining the tobacco industry’s efforts to use bars to reestablish the social acceptability of smoking and secondhand smoke”. 35
Consider smoking in a broader context of risk taking behaviour
Adolescents and young adults will be done a great disservice if researchers and policy advocates do not consider tobacco use in the larger context of social environments and risk taking behaviour, in particular risky sexual behaviour and the use of alcohol and illicit drugs. A tobacco focused approach to policy and intervention is not likely to be the most effective strategy, since it is clear that a number of risky behaviours are linked with each other and with some identifiable attitudes and perspectives. The root causes of youth and young adult smoking are likely not tobacco specific, but rather things that motivate or drive people to engage in other forms of risky or rebellious behaviour. Malcolm Gladwell, in his book The tipping point , makes the important observation that what leads to smoking is not positive perceptions or attitudes about the act of smoking itself: “Over the past decade, the anti-smoking movement has railed against the tobacco companies for making smoking cool and has spent untold millions of dollars of public money trying to convince teenagers that smoking isn’t cool But that’s not the point. Smoking was never cool. Smokers are cool..” 59 Thus, it is possible that risk taking behaviours cluster together because adolescents (or young adults) are trying to project an overall image or persona of themselves that they view positively (a person who is rebellious, takes risk, is independent, etc). As such, the phenomenon may be more about a process of attempting to become a specific type of person than an isolated decision to engage in a specific type of behaviour (that is, cigarette smoking). Furthermore, while it is certainly reasonable to point fingers at the tobacco industry, insinuating sole blame on industry marketing tactics for the broad phenomenon of increased smoking among young adults is too simplistic. Efforts to reduce tobacco use among young adults need to include, but also have a broader vision and scope than, counteracting industry marketing/promotional activities
The recent increase in smoking among young adults should be of grave concern to those engaged in tobacco prevention and control among youth. The increase is partly a residual effect of increases in cigarette smoking that have occurred among adolescents. In addition, however, the upsurge in smoking among young adults appears to be part of a broader phenomenon involving changes in substance use and risk taking behaviours among youth making the transition to adulthood. While there are many unanswered questions about recent trends in cigarette smoking and other drug use among both adolescents and young adults, what is known to date leads to a clarion call for increased intervention and policy action regarding the prevention and control of substance abuse among young adults—both on and off campus—in the USA.
Acknowledgments
Nicole Kuiper provided valuable research assistance and support on this project. David Mendez and Harold Pollack provided guidance regarding analysis, and Gary Giovino offered useful data and comments. Nancy Rigotti, Pam Ling, Ken Warner, Peter Jacobson, and Ernest Dopp provided excellent substantive comments on earlier drafts of the paper. In addition, numerous colleagues attending the Innovations in Youth Tobacco Control Conference (July 2002) shared useful and provocative feedback.
- ↵ Wechsler H , Rigotti N, Gledhill-Hoyt J, et al. Increased levels of cigarette use among college students. JAMA 1998 ; 280 1673 –78. OpenUrl CrossRef PubMed Web of Science
- ↵ Rigotti NA , Lee JE, Wechsler H. U.S. College students’ use of tobacco products: results of a national survey. JAMA 2000 ; 248 : 699 –705.
- ↵ Johnston LD , O’Malley PM, Bachman JG. Monitoring the Future national survey results on drug use, 1975–2000 . Volume II: College students and adults ages 19–40. Bethesda, Maryland: National Institute on Drug Abuse, 2001. (NIH Publication No. 01–4925.)
- ↵ Substance Abuse and Mental Health Services Administration . Summary of findings from the 2000 National Household Survey on Drug Abuse. Rockville, Maryland: Office of Applied Studies, Report No: (SMA) 01–3549, September 2001 .
- ↵ Pierce JP , Naquin M, Gilpin E, et al. Smoking initiation in the United States: a role for worksite and college smoking bans. J Natl Cancer Inst 1991 ; 83 : 1009 –13. OpenUrl Abstract / FREE Full Text
- ↵ Monitoring the Future . University of Michigan News and Information Services: Ann Arbor, Michigan, Access date: 30 August 2002. URL: http://monitoringthefuture.org
- ↵ Emmons KM , Wechsler H, Dowdall G, et al. Predictors of smoking among U.S. college students. Am J Public Health 1998 ; 88 : 104 –7. OpenUrl CrossRef PubMed Web of Science
- ↵ Centers for Disease Control and Prevention . Tobacco use among high school students – United States, 1997. MMWR Morb Mortal Wkly Rep 1998 ; 47 : 229 –33. OpenUrl PubMed
- ↵ National Center for Health Statistics . Health, United States, 1993. Hyattsville, Maryland: Public Health Service, 1994 . (DHHS Publication Number (PHS) 94–1232.)
- ↵ Centers for Disease Control and Prevention . Tobacco use – United States, 1900–1999. MMWR Morb Mortal Wkly Rep 1999 ; 48 : 986 –93. OpenUrl PubMed
- ↵ Haddock CK , Web MV, DeBon M, et al. Evidence that smokeless tobacco use is a gateway for smoking initiation in young adult males. Prev Med 2001 ; 32 : 262 –7. OpenUrl CrossRef PubMed Web of Science
- ↵ Glover E , Laflin M, Edwards S. Age of initiation and switching patterns between smokeless tobacco and cigarettes among college students in the United States. Am J Public Health 1989 ; 79 : 207 –8. OpenUrl PubMed Web of Science
- ↵ Nyman AL , Taylor TM, Biener L. Trends in cigar smoking and perceptions of health risks among Massachusetts adults. Tobacco Control 2002 ; 11 (suppl II): ii25 –8. OpenUrl Abstract / FREE Full Text
- ↵ National Center for Education Statistics . The Condition of Education 2000. Access date: 5 February 2003. URL: http://nces.ed.gov/pubs2000/2000062.pdf
- ↵ Giovino GA . Epidemiology of tobacco use among US adolescents. Nicotine Tob Res 1999 ; 1 (supp 1): 31 –40.
- ↵ Gledhill-Hoyt J , Lee H, Strote J, et al. Increased use of marijuana and other illicit drugs at U.S. colleges in the 1990s: results of three national surveys. Addiction 2000 ; 95 : 1655 –67. OpenUrl CrossRef PubMed Web of Science
- ↵ Johnson RA , Gerstein DR. Initiating of use of alcohol, cigarettes, marijuana, cocaine and other substances in US birth cohorts since 1919. Am J Public Health 1998 ; 88 : 27 –33. OpenUrl PubMed Web of Science
- ↵ Bachman JG , Johnston LD, O’Malley PM. Explaining recent increases in students’ marijuana use: impacts of perceived risks and disapproval, 1976 through 1996. Am J Public Health 1998 ; 88 : 887 –92. OpenUrl PubMed Web of Science
- ↵ O’Malley PM , Johnston DL, Bachman JG. Alcohol use among adolescents. Alcohol Health & Research World 1998 ; 2 : 85 –93.
- ↵ Schulenberg J , Bachman JG, O’Malley PM, et al. High school educational success and subsequent substance use: a panel analysis following adolescents into young adulthood. J Health Soc Behav 1994 ; 35 : 45 –62. OpenUrl CrossRef PubMed Web of Science
- ↵ Schulenberg J , O’Malley PM, Bachman JG, et al. Getting drunk and growing up: trajectories of frequent binge drinking during the transition to young adulthood. J Stud Alcohol 1996 ; 57 : 289 –304. OpenUrl PubMed Web of Science
- ↵ Jacobson PD , Lantz PM, Warner KE, et al. Combating teen smoking: research and policy strategies . Ann Arbor, Michigan: University of Michigan Press, 2001 .
- ↵ Flay BR , Hu FB, Richardson J. Psychosocial predictors of different stages of cigarette smoking among high school students. Prev Med 1998 ; 27 : A9 –18. OpenUrl CrossRef PubMed Web of Science
- ↵ Husten CG , McCarty MC, Giovino GA, et al. Intermittent smokers: a descriptive analysis of persons who have never smoked daily. Am J Public Health 1998 ; 88 : 86 –9. OpenUrl PubMed Web of Science
- ↵ American Legacy Foundation . Legacy First Look Report 3: pathways to established smoking: results from the 1999 National Youth Tobacco Survey. Washington DC: American Legacy Foundation, October 2000 .
- ↵ SAMHSA , Office of Applied Statistics. Tobacco use in America: findings from the 1999 National Household Survey on Drug Abuse. Access date: 30 August 2002. URL: http://www.samhsa.gov/OAS/NHSDA/tobacco/appendixd.htm
- ↵ Lawrence D , Fagan P, Backinger C, et al. Smoking patterns among young adults ages 18–24 in the US: data from the Current Population Survey, 1998–1999. Abstract from the Society for Research on Nicotine and Tobacco Annual Meeting, Savannah, Georgia, February 2002 .
- ↵ Ellickson PL , McGuigan KA, Klein DJ. Predictors of late-onset smoking and cessation over 10 years. J Adolesc Health 2001 ; 29 : 101 –8. OpenUrl
- ↵ Choi WS , Harris KJ, Okuyemi K et al. Predictors of smoking initiation among college-bound high school students. Abstract from the Society for Research on Nicotine and Tobacco Annual Meeting, Savannah, Georgia, February 2002 .
- ↵ Wee CC , Rigotti NA, Davis RB, et al. Relationship between smoking and weight control efforts among adults in the United States. Arch Intern Med 2001 ; 161 : 546 –50. OpenUrl CrossRef PubMed Web of Science
- ↵ Wechsler H , Lee JE, Nelson TF, et al. Drinking levels, alcohol problems and secondhand effects in substance-free college residences: results of a national study. J Stud Alcohol 2001 ; 61 : 23 –32. OpenUrl
- ↵ Sonntag H , Wittchen HU, Hofler M, et al . Are social fears and DSM-IV social anxiety disorder associated with smoking and nicotine dependence in adolescents and young adults? Eur Psychiatry 2000 ; 15 : 67 –74. OpenUrl CrossRef PubMed Web of Science
- ↵ Anda RF , Croft JB, Felitti, VJ, et al . Adverse childhood experiences and smoking during adolescence and adulthood. JAMA 1999 ; 282 : 1652 –8. OpenUrl CrossRef PubMed Web of Science
- ↵ Katz SK , Lavack AM. Tobacco related bar promotions: insights from tobacco industry documents. Tobacco Control 2002 ; 11 (suppl I): i92 –101. OpenUrl Abstract / FREE Full Text
- ↵ Sepe E , Ling PM, Glantz SA. Smooth moves: tobacco bar and nightclub promotions target young adults. Am J Public Health 2002 : 92 : 414 –9. OpenUrl CrossRef PubMed Web of Science
- ↵ Sepe E , Glantz SA. Bar and club tobacco promotions in the alternative press: targeting young adults. Am J Public Health 2002 : 92 : 75 –8. OpenUrl PubMed Web of Science
- ↵ Lantz P , Jacobson PD, Warner KE, et al. Investing in youth tobacco control: a review of smoking prevention and control strategies. Tobacco Control 2000 ; 9 : 47 –63. OpenUrl Abstract / FREE Full Text
- Gilpin EA , Pierce JP, Rosbrook B. Are adolescents receptive to current sales promotion practices of the tobacco industry? Prev Med 1997 ; 26 : 14 –21. OpenUrl CrossRef PubMed Web of Science
- Pierce JP , Gilpin EA, Choi WS. Sharing the blame: smoking experimentation and future smoking-attributable mortality due to Joe Camel and Marlboro advertising and promotions. Tobacco Control 1999 ; 8 : 37 –44. OpenUrl Abstract / FREE Full Text
- ↵ Perry CL . The tobacco industry and underage youth smoking: tobacco industry documents from the Minnesota litigation. Pediatr Adolesc Med 1999 ; 153 : 935 –41. OpenUrl
- ↵ King C , Siegel M. The Master Settlement Agreement with the tobacco industry and cigarette advertising in magazines. N Engl J Med 2001 ; 345 : 504 –11. OpenUrl CrossRef PubMed Web of Science
- ↵ Rosenberg NJ , Siegel M. Use of corporate sponsorship as a tobacco marketing tool: A review of tobacco industry sponsorship in the USA, 1995–99. Tobacco Control 2001 ; 10 : 239 –46. OpenUrl Abstract / FREE Full Text
- ↵ Tye JB , Warner KE, Glantz SA. Tobacco advertising and consumption: evidence of a causal relationship. J Public Health Policy 1987 ; 8 : 492 –508. OpenUrl CrossRef PubMed
- ↵ Ling PM , Glantz SA. Why and how the tobacco industry sells cigarettes to young adults: evidence from industry documents. Am J Public Health 2002 ; 92 : 908 –16. OpenUrl CrossRef PubMed Web of Science
- ↵ Ling PM , Glantz SA. Using tobacco industry marketing research to design more effective tobacco control campaigns. JAMA 2002 ; 287 : 2983 –9. OpenUrl CrossRef PubMed Web of Science
- ↵ Rigotti NA , Moran SE, Wechsler H. College students’ exposure to tobacco promotions at bars, clubs and campus social events: prevalence and relationship to tobacco use. Abstract from the Society for Research on Nicotine and Tobacco Annual Meeting, New Orleans, February 2003 .
- ↵ Everett SA , Husten CG, Kann LK, et al. Smoking initiation and smoking patterns among U.S. college students. J Am Coll Health 1999 ; 48 : 55 –60. OpenUrl PubMed Web of Science
- ↵ O’Neill HK , Gillispie MA, Slobin K. Stages of change and smoking cessation: a computer-administered intervention program for young adults. Am J Health Promotion 2000 ; 15 : 93 –96. OpenUrl PubMed Web of Science
- ↵ Martinelli AM . An explanatory model of variables influencing health promotion behaviors in smoking and nonsmoking college students. Public Health Nurs 1999 ; 16 : 263 –9. OpenUrl CrossRef PubMed Web of Science
- ↵ Foote J , Harris R, Giles M, et al. Physician advice and tobacco use: a survey of 1st-year college students. J Am Coll Health 1996 ; 45 : 129 –32. OpenUrl PubMed Web of Science
- ↵ Thorndike A , Ferris T, Stafford R, et al. Rates of U.S. physicians counseling adolescents about smoking. J Natl Cancer Inst 1999 ; 91 : 1857 –62. OpenUrl Abstract / FREE Full Text
- ↵ Wechsler H , Kelley K, Seibring M, et al. College smoking policies and smoking cessation programs: results of a survey of college health center directors. J Am Coll Health 2001 : 49 : 205 –12. OpenUrl PubMed Web of Science
- ↵ Majchrzak N , Park E, Rigotti N. Tobacco use by Massachusetts public college students: discussing issues with students and administrators. Abstract from the Society for Research on Nicotine and Tobacco Annual Meeting, Savannah, Georgia, February 2002 .
- ↵ Glantz SA . Preventing tobacco use—the youth access trap. Am J Public Health 1996 ; 86 : 1156 –8. OpenUrl
- ↵ Hill D . Why we should tackle adult smoking first. Tobacco Control 1999 ; 8 : 333 –5. OpenUrl FREE Full Text
- ↵ Rigotti NA , Regan S, Majchrzak NE, et al. Tobacco use by Massachusetts public college students in 1999. Tobacco Control 2002 ; 11 (suppl II): ii20 –4. OpenUrl Abstract / FREE Full Text
- ↵ Wakefield M , Giovino G. Teen penalties for tobacco possession, use, and purchase: evidence and issues. Tobacco Control 2003 ; 12 (suppl I): 6 –13. OpenUrl FREE Full Text
- ↵ Brownson RC , Eriksen MP, Davis RM, et al. Environmental tobacco smoke: health effects and policies to reduce exposure. Ann Rev Public Health 1997 ; 18 : 163 –85. OpenUrl CrossRef PubMed Web of Science
- ↵ Gladwell M . The tipping point: how little things can make a big difference. New York: Little, Brown & Co, 2000 .
Read the full text or download the PDF:
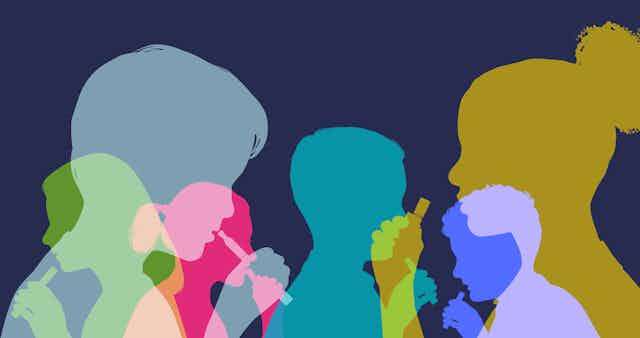
Millions of current smokers became addicted when they were teens – and nicotine marketing targets adolescents today just as it did decades ago
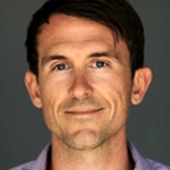
Associate Professor of Social and Behavioral Sciences, Rutgers University
Disclosure statement
Jon-Patrick Allem receives funding from the California Tobacco Control Program, and the National Institutes of Health. He has received fees for consulting services in court cases pertaining to the content on social media platforms.
View all partners
About 37 million children ages 13 to 15 around the world use tobacco , according to a 2024 report from the World Health Organization.
In 2023, e-cigarettes were the most commonly used tobacco product in the U.S., with 7.7% of middle school and high school students reporting e-cigarette use. Cigarettes were the next most common, with 1.6% of middle- and high school students saying they had consumed them in the past month.
Research shows that most people who use tobacco start in childhood .
I am a public health researcher who studies the different ways in which corporations influence adolescent health, with current projects focused on identifying sources of exposure to tobacco marketing among adolescents and young adults.
Over the past decade, I’ve researched ways in which tobacco companies market to children and young adults.
Health harms of tobacco
Smoking is harmful to every organ in the human body and is known to cause many types of cancers and heart disease, among other problems. Nicotine, by itself, in products like e-cigarettes and oral pouches , is harmful to adolescent brain development .
The adolescent brain develops into early adulthood, especially in the prefrontal cortex . This part of the brain is involved in higher cognitive development, including functions pertaining to attention, memory and cognitive flexibility . Research has shown that nicotine exposure results in long-term functional and structural changes in the brain .
Old tactics in new US products
Decades of research have documented how tobacco companies marketed tobacco products for years to attract young customers in order to sustain and grow their businesses.
In a 2021 study, my team and I showed that exposure to e-cigarette marketing with cartoon images decreased high school students’ perceptions of harm from e-cigarettes. Research makes clear that this change in perspective can make adolescents more open to trying e-cigarettes .
In an earlier study, my team and I examined the impact of e-cigarette product placement and imagery in music videos on propensity to use e-cigarettes among young adults. We found that exposure to e-cigarette product placement in music videos increased young adults’ intentions to try e-cigarettes in the future.
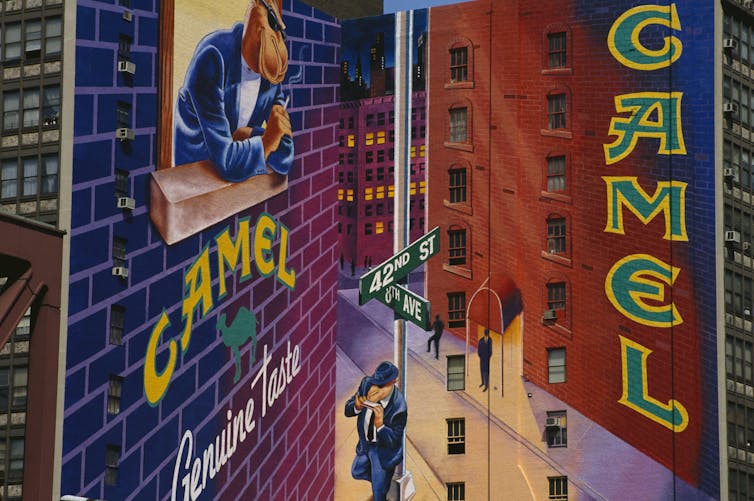
Striking at an early age
While our research has largely focused on the U.S. context, several recent studies have shown how tobacco companies continue to market to children in other parts of the world.
For example, a team of researchers conducted focus groups among Chinese adolescents to understand sources of tobacco marketing exposure and what features made ads attractive. They found that adolescents reported being exposed to tobacco ad campaigns near their home and school . These young Chinese people also reported that bright colors, product packaging and price promotions featured in e-cigarette ads and other promotional materials attracted their attention.
In England, a 2022 study provided an overview of marketing claims present on the websites of popular e-cigarette brands. Most notably, the study found that U.K websites featured medicinal claims for smoking cessation, associations with youth culture and depictions of young people using e-cigarettes.
Normalizing tobacco use
The emergence of social media platforms has led tobacco companies to shift their marketing tactics from traditional channels like magazines, billboards and retail stores to online channels, capitalizing on the time that young people spend on screens.
Tobacco companies have helped normalize tobacco use online with the help of branded social media account pages and social media influencers . For example, in 2021, researchers discovered that e-cigarettes were being used and promoted by influencers on Amazon-owned Twitch, a video-sharing platform popular among adolescent gamers.
Similarly, streaming platforms normalize tobacco use. For example, in a 2022 study, my team and I analyzed Netflix original TV shows and films for e-cigarette-related content. Most notably, we documented brief representations of teenagers holding e-cigarettes in the PG-13 film “Hubie Halloween.” Our findings contradict Netflix’s no e-cigarette pledge for PG-13 content .
This suggests a need for further outside-party monitoring of e-cigarettes in Netflix programming.
Spreading awareness
Every country will have to effectively enforce and expand restrictions on cigarette and e-cigarette marketing to protect children and teenagers from messages that encourage them to try smoking and vaping. Additional efforts are needed everywhere to help discourage young people from getting hooked on tobacco.
Most parents, teachers and other authority figures are likely unaware of the extent to which young people are exposed to tobacco content online, including tobacco ads and peer-generated posts on social media that normalize tobacco use.
Campaigns focused on tobacco-related digital media literacy could create awareness about tobacco products and describe how they are being marketed in the digital environment. There are various evidence-based resources that could be built upon to help young people evaluate tobacco advertising messages, empowering digital and social media users to actively evaluate information rather than remain a passive target for the industry’s marketing.
I am certain that increased awareness could prevent young people from consuming tobacco.
- Children's health
- Social media
- Public health
- Advertising
- E-cigarettes
- Cognitive skills
- Prefrontal cortex
- Teen smoking

Data Manager

Research Support Officer

Director, Social Policy

Head, School of Psychology

Senior Research Fellow - Women's Health Services

An official website of the United States government
The .gov means it’s official. Federal government websites often end in .gov or .mil. Before sharing sensitive information, make sure you’re on a federal government site.
The site is secure. The https:// ensures that you are connecting to the official website and that any information you provide is encrypted and transmitted securely.
- Publications
- Account settings
Preview improvements coming to the PMC website in October 2024. Learn More or Try it out now .
- Advanced Search
- Journal List
- Taylor & Francis Open Select

Tobacco smoking: Health impact, prevalence, correlates and interventions
Robert west.
a Department of Behavioural Science and Health , University College London , London, UK
Background and objectives : Despite reductions in prevalence in recent years, tobacco smoking remains one of the main preventable causes of ill-health and premature death worldwide. This paper reviews the extent and nature of harms caused by smoking, the benefits of stopping, patterns of smoking, psychological, pharmacological and social factors that contribute to uptake and maintenance of smoking, the effectiveness of population and individual level interventions aimed at combatting tobacco smoking, and the effectiveness of methods used to reduce the harm caused by continued use of tobacco or nicotine in some form.
Results and conclusions : Smoking behaviour is maintained primarily by the positive and negative reinforcing properties of nicotine delivered rapidly in a way that is affordable and palatable, with the negative health consequences mostly being sufficiently uncertain and distant in time not to create sufficient immediate concern to deter the behaviour. Raising immediate concerns about smoking by tax increases, social marketing and brief advice from health professionals can increase the rate at which smokers try to stop. Providing behavioural and pharmacological support can improve the rate at which those quit attempts succeed. Implementing national programmes containing these components are effective in reducing tobacco smoking prevalence and reducing smoking-related death and disease.
Introduction
The continued popularity of tobacco smoking appears to defy rational explanation. Smokers mostly acknowledge the harm they are doing to themselves and many report that they do not enjoy it – yet they continue to smoke (Fidler & West, 2011 ; Ussher, Brown, Rajamanoharan, & West, 2014 ). The reason is that nicotine from cigarettes generates strong urges to smoke that undermine and overwhelm concerns about the negative consequences of smoking, and the resolve not to smoke in those trying to stop (West & Shiffman, 2016 ). Progress is being made in many countries in reducing smoking prevalence but it remains one of the main causes of ill health and premature death worldwide (Gowing et al., 2015 ).
This paper provides a broad overview of smoking in terms of: the health effects, benefits of stopping, prevalence and patterns of use, psychological, pharmacological and social factors leading to uptake and maintenance of the behaviour, effectiveness of population level and individual level interventions to combat it, and methods used to reduce the harm despite continued use of tobacco or nicotine.
Definitions of smoking and smoking cessation
Tobacco smoking consists of drawing into the mouth, and usually the lungs, smoke from burning tobacco (West & Shiffman, 2016 ). The type of product smoked is most commonly cigarettes, but can also include cigarillos, cigars, pipes or water pipes. ‘Smokeless’ tobacco is also popular in some parts of the world. This typically involves using tobacco preparations for chewing, sniffing into the nose or placing as a wad in the mouth between the cheeks and gums (Critchley & Unal, 2003 ). Smokeless tobacco use has features that are similar to smoking and can carry significant health risks (Critchley & Unal, 2003 ); however, this article focuses on smoked tobacco only as this has been the subject of by far the largest volume of research and is the most harmful form of tobacco use.
Stopping smoking usually involves an intention not to smoke any more cigarettes from a given point in time (a ‘quit attempt’), followed by self-conscious resistance of urges to smoke resulting in a period of abstinence. If someone making a quit attempt smokes one or more cigarettes on an occasion but then resumes abstinence, this is usually termed a ‘lapse’. If this person resumes smoking on a regular basis s/he is said to have ‘relapsed’. ‘Short-term abstinence’ is commonly defined in terms of achieving up to 4 weeks of abstinence. ‘Long-term abstinence’ often refers to abstinence for at least 6 months but more typically involves abstinence for at least 12 months. There is no agreed criterion for deciding when someone has ‘stopped smoking’ so it is essential when using the term to be clear about how long the abstinence period has been.
Health impact of smoking and the benefits of stopping
Tobacco smoking increases the risk of contracting a wide range of diseases, many of which are fatal. Stopping smoking at any age is beneficial compared with continuing to smoke. For some diseases, the risk can be reversed while for others the risk is approximately frozen at the point when smoking stopped.
Health impact of smoking
Table Table1 1 lists the main causes of death from smoking. Tobacco smoking is estimated to lead to the premature death of approximately 6 million people worldwide and 96,000 in the UK each year (Action on Smoking and Health, 2016b ; World Health Organization, 2013 ). A ‘premature death from smoking’ is defined as a death from a smoking-related disease in an individual who would otherwise have died later from another cause. On average, these premature deaths involve 10 years of life years lost (US Department of Health and Human Services, 2004 ). Many of these deaths occur in people who have stopped smoking but whose health has already been harmed by smoking. It also happens to be the case that smokers who do not stop smoking lose an average of 10 years of life expectancy compared with never-smokers and they start to suffer diseases of old age around 10 years earlier than non-smokers (Jha & Peto, 2014 ).
Most smoking-related deaths arise from cancers (mainly lung cancer), respiratory disease (mainly chronic obstructive pulmonary disease – COPD), and cardiovascular disease (mainly coronary heart disease) (Action on Smoking and Health, 2016b ). Smoking is an important risk factor for stroke, blindness, deafness, back pain, osteoporosis, and peripheral vascular disease (leading to amputation) (US Department of Health and Human Services, 2004 ). After the age of 40, smokers on average have higher levels of pain and disability than non-smokers (US Department of Health and Human Services, 2004 ).
Smoking in both women and men reduces fertility (Action on Smoking and Health, 2013 ). Smoking in pregnancy causes underdevelopment of the foetus and increases the risk of miscarriage, neonatal death, respiratory disease in the offspring, and is probably a cause of mental health problems in the offspring (Action on Smoking and Health, 2013 ).
People used to think that smoking was protective against Alzheimer’s disease but we now know that the opposite is the case: it is a major risk factor for both Alzheimer’s and vascular dementia (Ferri et al., 2011 ; US Department of Health and Human Services, 2004 ).
There is a positive association between average daily cigarette consumption and risk of smoking-related disease, but in the case of cardiovascular disease the association is non-linear, so that low levels of cigarette consumption carry a higher risk than would be expected from a simple linear relationship (US Department of Health and Human Services, 2004 ).
Tobacco smoke contains biologically significant concentrations of known carcinogens as well as many other toxic chemicals. Some of these, including a number of tobacco-specific nitrosamines (particularly NNK and NNN) are constituents of tobacco, largely as a result of the way it is processed, while others such as benzopyrine result from combustion of tobacco (Action on Smoking and Health, 2014b ). These chemicals form part of the particulate matter in smoke. Tobacco smoke also contains the gas, carbon monoxide (CO). CO is a potent toxin, displacing oxygen from haemoglobin molecules. However, acutely the amount of CO in tobacco smoke is too small to lead to hypoxia and the body produces increased numbers of red blood cells to compensate.
The nicotine in tobacco smoke may cause a small part of the increase in cardiovascular disease but none or almost none of the increase in risk of respiratory disease or cancer (Benowitz, 1997 , 1998 ). It is the other components of cigarette smoke that do almost all the damage. It has been proposed on the basis of studies with other species that nicotine damages the adolescent brain but there is no evidence for clinically significant deficits in cognition or emotion in adults who smoked during adolescence and then stopped (US Department of Health and Human Services, 2004 ).
Exposure to second-hand smoke carries a significant risk for both children and adults. Thus, non-smokers who are exposed to a smoky environment have an increased risk of cancer, heart disease and respiratory disease (Action on Smoking and Health, 2014a ).
Benefits of stopping smoking
Table Table1 1 lists the main benefits of stopping smoking. Smokers who stop before their mid-30s have approximately the same life expectancy as never smokers (Doll, Peto, Boreham, & Sutherland, 2004 ; Pirie, Peto, Reeves, Green, & Beral, 2013 ). After the age of 35 years or so, stopping smoking recovers 2–3 months of healthy life expectancy for every year of smoking avoided, or 4–6 h for every day (Jha & Peto, 2014 ).
Stopping smoking has different effects on different smoking-related diseases. Excess risk of heart attack caused by smoking reduces by 50% within 12 months of stopping smoking. Stopping smoking returns the rate of decline in lung function to the normal age-related decline, but does not reverse this; it reduces the frequency of ‘exacerbations’ (acute attacks of breathing difficulty resulting in death or hospitalisation) in COPD patients (US Surgeon General, 1990 ). Stopping smoking ‘freezes’ the risk of smoking-related cancers at the level experienced when stopping occurs but does not decrease it in absolute terms (US Surgeon General, 1990 ).
Smokers who stop show reduced levels of stress and mood disorder than those who continue (Royal College of Physicians and Royal College of Psychiatrists, 2013 ). They also report higher levels of happiness and life satisfaction than those who continue (Shahab & West, 2009 , 2012 ). This suggests that smoking may harm mental health, though other explanations cannot be ruled out on the current evidence.
Prevalence and patterns of smoking
Smoking prevalence.
There are estimated to be approximately 1 billion tobacco smokers worldwide (Eriksen, Mackay, & Ross, 2013 ), amounting to approximately 30% of men and 7% of women (Gowing et al., 2015 ).
Cigarette smoking prevalence in Great Britain was estimated to be 16.9% in 2015, the most recent year for which figures are available at the time of writing: slightly lower in women than men (Office of National Satistics, 2016 ). Smoking in Great Britain has declined by 0.7 percentage points per year since 2001 (from 26.9% of adults in 2001). In Australia, daily cigarette smoking has declined by 0.6 percentage points per year over a similar time period (from 22.4% of adults aged 18 + years in 2001 to 14.5% in 2015) (Australian Bureau of Statistics, 2015 ). However, international comparisons are confused by different countries using a different definition of what counts as being a smoker, and different methods for assessing prevalence. Australia only counts daily smokers in their headline figures. The situation in the US is even more misleading. The headline prevalence figure for the US is below 16%, but this does not include occasional smokers and people who smoke cigarillos which are essentially cigarettes in all but name and which have become increasingly popular in recent years. So the figure for prevalence that is most comparable to the figure for Great Britain is 20% (Jamal, 2016 ).
With the above caveats in mind, the figures in Table Table2 2 for smoking prevalence in world regions in men and women provide very broad estimates (Gowing et al., 2015 ). Most noteworthy is that smoking prevalence in men is more than four times that in women globally but that the difference is much less in most parts of Europe, and that Eastern Europe as a whole has the highest smoking prevalence of any region in the world.
Note: Current smoking of any tobacco product, adults aged 15 years and older, age-standardised rate, by gender. ‘Tobacco smoking’ includes cigarettes, cigars, pipes or any other smoked tobacco products. ‘Current smoking’ includes both daily and non-daily or occasional smoking. From Gowing et al. ( 2015 ).
Smoking patterns
The most common age of first trying a cigarette in countries that have been studied is 10–15 years (Action on Smoking and Health, 2015b ; Talip, Murang, Kifli, & Naing, 2016 ); take up of regular smoking usually continues up to early 20s (Dierker et al., 2008 ).
Average daily cigarette consumption among smokers in the US and UK has declined steadily since the 1970s. In the UK, it is currently 11 cigarettes per day, and non-daily smoking is very rare (Action on Smoking and Health, 2016c ; Jarvis, Giovino, O’Connor, Kozlowski, & Bernert, 2014 ). Smokers take in an average of 1–1.5 mg of nicotine per cigarette (US Department of Health Human Services, 2014 ). The US figures on patterns of smoking are distorted by not counting ‘cigarillos’ and other smoked tobacco products which are used very much like cigarettes, whose prevalence has increased in recent years (Jamal et al., 2015 ). The reduction in daily cigarette consumption has not been accompanied by a reduction in daily nicotine intake (Jarvis et al., 2014 ). This could be due to the use of other smoked tobacco products (in the case of the US) or smokers smoking their cigarettes more intensively (taking more, deeper or longer puffs).
Smokers in England spend an average of £23 per week on cigarettes and this figure is slowly rising (West & Brown, 2015 ). In the UK, hand-rolled cigarettes have become increasingly popular with 34% of smokers currently reporting use of these products (Action on Smoking and Health, 2016c ). Men and people in more deprived socio-economic groups are more likely to smoke hand-rolled cigarettes (Action on Smoking and Health, 2016c ).
In most countries, there are strong negative associations between smoking prevalence and educational level, affluence and mental health; and positive associations with alcohol use disorder and substance use disorder (Action on Smoking and Health, 2016a , 2016c ; Royal College of Physicians and Royal College of Psychiatrists, 2013 ; Talati, Keyes, & Hasin, 2016 ). In the UK, average daily cigarette consumption is higher for men than women, and higher in smokers in more deprived socio-economic groups and those with mental health problems (Action on Smoking and Health, 2016c ).
Psychological, pharmacological and social factors involved in smoking and smoking cessation
The natural history of smoking can be modelled as states and factors that influence the transition between these. Figure Figure1 1 shows transitions that have been researched – the variables identified in the diagram are listed descriptively without attempting to explain how they may be connected.

Factors associated with transitions in the natural history of smoking (parentheses indicate negative associations).
Smoking initiation
Important factors predicting initiation in western societies are: having friends who smoke, having parents who smoke, low social grade, tendency to mental health problems and impulsivity (Action on Smoking and Health, 2015b ). Transition to daily smoking follows a highly variable pattern sometimes being very rapid and sometimes taking several years (Schepis & Rao, 2005 ). Important factors predicting transition to regular smoking are: having friends who smoke, weak academic orientation, low parental support, pro-smoking attitudes, drinking alcohol and low socio-economic status (Action on Smoking and Health, 2015b ).
Smoking initiation has a ‘heritability’ (the proportion of variance in a characteristic that is attributable to genetic rather than environmental variance) of approximately 30–50% in western societies (Vink, Willemsen, & Boomsma, 2005 ). This means that differences in genetic make-up account for almost half of the difference in likelihood of starting smoking between individuals. This does not mean that environmental factors do not also play a crucial role as is evident from the very large decline in smoking initiation since the 1970s in many western countries.
The heritability of cigarette addiction (as distinct from smoking) is approximately 70–80% in western societies (Vink et al., 2005 ). Cigarette addiction here refers to the extent to which someone experiences a strong need to smoke. It is usually indexed by a combination of number of cigarettes per day and time from waking to smoking the first cigarette of the day (Kozlowski, Porter, Orleans, Pope, & Heatherton, 1994 ). It can also be indexed by the self-reported strength of urges to smoke (Fidler, Shahab, & West, 2011 ). Heritability of cigarette addiction, as indexed by failure of attempts to stop, is higher than the heritability for smoking and for initiation of smoking. This suggests that differences in genetic inheritance play a larger role in being able to stop smoking than in starting to smoke.
Cigarette addiction
Cigarette addiction stems from the fact that smoking provides highly controllable doses of the drug, nicotine, rapidly to the brain in a form that is accessible, affordable and palatable (West, 2009 ; West & Shiffman, 2016 ). Nicotine provided more slowly, for example by the nicotine transdermal patch, is much less addictive. It is possible that one or more mono-amine oxidase inhibitors in cigarette smoke add to, or synergise, the addictive properties of nicotine (Hogg, 2016 ).
The psychopharmacology of cigarette addiction is complex and far from fully understood. The following paragraphs summarise the current narrative.
Nicotine resembles the naturally occurring neurotransmitter, acetylcholine, sufficiently to attach itself to a subset of neuronal receptors for this neurotransmitter in the brain. These are called ‘nicotinic acetylcholine receptors’. When it does this with receptors in the ventral tegmental area in the midbrain, it causes an increased rate of firing of the nerves projecting forward from that area to another part of the brain called the nucleus accumbens. This causes release of another neurotransmitter called dopamine in the nucleus accumbens.
Dopamine release and uptake by neurones in the nucleus accumbens is believed to be central to all addictive behaviours. It acts as a neural ‘teaching signal’ which causes the brain to form an association between the current situation as perceived and the impulse to engage in whatever action immediately preceded this release. In the case of smoking, this creates an urge to smoke in situations in which smoking frequently occurs. These are often referred to as ‘cue-driven smoking urges’ or ‘situational cravings’ (West, 2009 ; West & Shiffman, 2016 ). This explains why even non-daily smokers often find it difficult to stop smoking altogether.
Repeated ingestion of nicotine from cigarettes causes changes to the functioning of the ventral tegmental area and nucleus accumbens such that when brain concentrations of nicotine are lower than usual, there is an abnormally low level of neural activity in these regions. This leads to feelings of need for behaviours that have in the past restored normal functioning, typically smoking. This feeling of need can be thought of as a kind of ‘nicotine hunger’, also called ‘background craving’ (West, 2009 ; West & Shiffman, 2016 ). This is probably why time between waking and first cigarette of the day is a useful predictor of difficulty stopping smoking (Vangeli, Stapleton, Smit, Borland, & West, 2011 ). So ‘cue-driven smoking urges’ and ‘nicotine hunger’ are important factors contributing to smoking behaviour and thought to be the primary mechanisms underpinning cigarette addiction (West, 2009 ; West & Shiffman, 2016 ).
When smokers abstain from cigarettes, within a few hours many of them start to experience nicotine withdrawal symptoms. Withdrawal symptoms from a drug are temporary symptoms that arise when the drug dose is reduced or use is terminated. They arise from neural adaptation to the presence of the drug in the central nervous system. For smoking, the most common early onset symptoms are: irritability, restlessness and difficult concentrating. Depression and anxiety have also been observed in some smokers. These symptoms typically last 1 to 4 weeks (West, 2009 ; West & Shiffman, 2016 ).
After a day or two of stopping smoking, many smokers experience other symptoms: increased appetite, constipation, mouth ulcers, cough, and weight gain. Increased appetite tends to last for at least 3 months; weight gain (averaging around 6 kg) tends to be permanent; other symptoms tend to last a few weeks. The increased appetite, weight gain and constipation arise from termination of nicotine intake but the others are probably related to other effects of stopping smoking (West, 2009 ; West & Shiffman, 2016 ).
Any of the above effects of abstinence may in individual cases promote resumption of smoking following a quit attempt but statistically the association is inconsistent and weak; the main factors driving relapse appear to be cue-driven smoking urges and nicotine hunger (Fidler & West, 2011 ; West, 2009 ; West & Shiffman, 2016 ).
Many smokers report that smoking helps them cope with stress and increases their ability to concentrate. However, this appears to be because when they go for a period without smoking they experience nicotine withdrawal symptoms that are relieved by smoking. Long-term smokers who stop report lower levels of stress than when they were smoking and no reduction in ability to concentrate (West, 2009 ; West & Shiffman, 2016 ).
It is commonly thought that smokers with mental health problems are using cigarettes to ‘self-medicate’ or treat their psychological symptoms. However, the evidence indicates that neither nicotine nor smoking improves psychological symptoms, and people with serious mental health disorders who stop smoking do not experience a worsening of mental health. In fact some studies have found an improvement (Royal College of Physicians and Royal College of Psychiatrists, 2013 ).
Smoking cessation
For most smokers, cessation requires a determined attempt to stop and then sufficient resolve in the following weeks and months to overcome what are often powerful urges to smoke. Factors that predict quit attempts differ from those that predict the success of those attempts (Vangeli et al., 2011 ). Approximately 5% of unaided quit attempts succeed for at least 6 months (Hughes, Keely, & Naud, 2004 ). Relapse after this point is estimated to be around 50% over subsequent years (Stapleton & West, 2012 ).
The most common self-reported reasons for smoking are stress relief and enjoyment, with around half of smokers reporting these smoking motives. Weight control, aiding concentration and socialising are also quite commonly cited (Fidler & West, 2009 ). Smoking for supposed stress relief, improved concentration, weight control or other functions has not been found to be related to attempts to stop or success of attempts to stop (Fidler & West, 2009 ). Smokers who report enjoying smoking are less likely to try to stop but not less likely to succeed if they do try (Fidler & West, 2011 ). In addition, having a positive smoker identity (liking being a smoker) predicts not trying to quit, over and above enjoyment of smoking (Fidler & West, 2009 ).
No clear association has been found between the number of times smokers have tried to stop in the past and their chances of success the next time they try (Vangeli et al., 2011 ). However, having tried to stop in the past few months is predictive of failure of the next quit attempt (Zhou et al., 2009 ). Belief in the harm caused by smoking is predictive of smokers making quit attempts but not the success of those attempts (Vangeli et al., 2011 ).
Some clinical studies have found that women were less likely to succeed in quit attempts than men but large population studies have found no difference in success rates between the genders (Vangeli et al., 2011 ) so it may be the case that women who seek help with stopping have greater difficulty than men who seek help with stopping.
Number of cigarettes smoked per day, time between waking and the first cigarette of the day and rated strength of urges to smoke prior to a quit attempt have been found to predict success of quit attempts (Vangeli et al., 2011 ).
Quit attempts that involve gradual reduction are less likely to succeed than those that involve quitting abruptly, even after controlling statistically for measures of cigarette addiction, confidence in quitting, other methods used to quit (e.g. nicotine replacement therapy) and sociodemographic factors (Lindson-Hawley et al., 2016 ).
Interventions to combat smoking
There is extensive evidence on interventions that can reduce smoking prevalence, either by reducing initiation or promoting cessation. Table Table3 3 lists those that have the strongest evidence.
Population-level interventions
Increasing the financial cost of smoking through tax increases and control of illicit supply on average reduces overall consumption with a typical price elasticity globally of 0.4 (meaning that for every 10% increase in the real cost there is a 4% decrease in the number of cigarettes purchased). Most of the effect is in getting smokers to reduce their daily cigarette consumption so the effect on smoking prevalence has been found to be an average of a 1–2 percentage point prevalence reduction for every 10% increase in the real cost (Levy, Huang, Havumaki, & Meza, 2016 ). It has been claimed that increasing taxes on tobacco increases the amount of smuggling of cheap tobacco, but the evidence does not support this (Action on Smoking and Health, 2015a ; Joossens & Raw, 2003 ).
Social marketing campaigns (e.g. TV advertising) can prevent smoking uptake, increase the rate at which smokers try to quit and improve the chances of success. This can lead to a reduction in smoking prevalence. Their effectiveness varies considerably with intensity, type of campaign and context (Bala, Strzeszynski, Topor-Madry, & Cahill, 2013 ; Hoffman & Tan, 2015 ).
Legislating to ban smoking in all indoor public areas may have a one-off effect on reducing smoking prevalence but findings are inconsistent across different countries (Bala et al., 2013 ). For example, in countries such as France it was not possible to detect an effect while in England, there did appear to be a decline in prevalence following the ban.
Although it is hard to show conclusively, circumstantial evidence suggests that banning tobacco advertising and putting large graphic health warnings on cigarette packets may have reduced smoking prevalence in some countries (Hoffman & Tan, 2015 ; Noar et al., 2016 ).
Individual-level interventions to promote smoking cessation
Brief advice.
Brief advice to stop smoking from a physician and offer of support to all smokers, regardless of motivation to quit, has been found in randomised trials to increase rate of quitting by an average of 2 percentage points of all those receiving it, whether or not they were initially interested in quitting (Stead et al., 2013 ). The offer of support appears to be more effective in getting smokers to try to quit than just advising smokers to stop (Aveyard, Begh, Parsons, & West, 2012 ).
Pharmacotherapy
Using a form of nicotine replacement therapy (NRT: transdermal patch, chewing gum, nasal spray, mouth spray, lozenge, inhalator, dissolvable strip) for at least 6 weeks from the start of a quit attempt increases the chances of long-term success of that quit attempt by about 3–7 percentage points if the user is under the care of a health professional or provided as part of a structured support programme (Stead et al., 2012 ). Some studies have found that NRT when bought from a shop and used without any additional structured support does not improve the chances of success at stopping (Kotz, Brown, & West, 2014a , 2014b ). A small proportion of people who use NRT to stop smoking continue to use it for months or even years after stopping smoking, but NRT appears to carry minimal risk to long-term users (Royal College of Physicians, 2016 ; Stead et al., 2012 ).
Data are sparse but at present, using an electronic cigarette in a quit attempt appears to increase the chances of success at stopping on average by an amount broadly similar to that from NRT; the variety of products available and the greater similarity to smoking appear to make them more attractive to many smokers as a means of stopping than NRT (McNeill et al., 2015 ; Royal College of Physicians, 2016 ). Electronic cigarettes deliver nicotine to users by heating a liquid containing nicotine, propylene glycol or glycerol and usually flavourings to create a vapour that is inhaled. They appear to carry minimal acute risk to users. If they are used long-term, their risk is almost certainly much less than that of smoking (based on concentrations of chemicals in the vapour) (McNeill et al., 2015 ; Royal College of Physicians, 2016 ).
‘Dual-form NRT’ (combining a transdermal NRT patch and one of the other forms) increases the chances of success at stopping more than ‘single-form NRT’ (just using one of the products) (Stead et al., 2012 ). Starting to use a nicotine transdermal patch several weeks before the target quit date may improve the chances of success at quitting compared with starting on the quit date (Stead et al., 2012 ).
Taking the prescription anti-depressant, bupropion (brand name Zyban), improves the chances of success of quit attempts by a similar amount to single-form NRT (Hughes, Stead, Hartmann-Boyce, Cahill, & Lancaster, 2014 ). Bupropion often leads to sleep disturbance and carries a very small risk of seizure. Bupropion probably works by reducing urges to smoke rather than any effect on depressed mood, but how it does this is not known. It is contra-indicated in pregnant smokers and people with an elevated seizure risk or history of eating disorder (Hughes et al, 2014 ). Taking the tricyclic anti-depressant, nortriptyline also improves the chances of success of quit attempts, probably by about the same amount as bupropion and NRT (Hughes et al., 2014 ). Its mechanism of action is not known. Nortriptyline often leads to dry mouth and sleep disorder and can be fatal in overdose (Hughes et al., 2014 ).
Taking the nicotinic-acetylcholine receptor partial agonist, varenicline (brand name Chantix in the US and Champix elsewhere), improves the chances of success by about 50% more than bupropion or single-form NRT (Cahill, Lindson-Hawley, Thomas, Fanshawe, & Lancaster, 2016 ). This is true for smokers with or without a psychiatric disorder (Anthenelli et al., 2016 ). Varenicline appears to work both by reducing urges to smoke and the rewarding effect of nicotine should a lapse occur (West, Baker, Cappelleri, & Bushmakin, 2008 ). Varenicline often leads to sleep disturbance and nausea. Serious neuropsychiatric and cardiovascular adverse reactions have been reported, but in comparative studies these have not been found to be more common than placebo or NRT (Anthenelli et al., 2016 ; Cahill et al., 2016 ; Sterling, Windle, Filion, Touma, & Eisenberg, 2016 ).
Taking the nicotinic-acetylcholine receptor partial agonist, cytisine, appears to improve the chances of success at least as much as single-form NRT and probably more (Cahill et al., 2016 ). Cytisine often causes nausea. No serious adverse reactions have been reported to date (Cahill et al., 2016 ). Where it is licensed for sale, cytisine is less than 1/10th the cost of other smoking cessation medications (Cahill et al., 2016 ).
Behavioural support
There is good evidence that behavioural interventions of many kinds, delivered though several modalities can help smokers to stop. Thus, behavioural support (encouragement, advice and discussion) from a trained stop-smoking specialist, provided at least weekly until at least 4 weeks following the target quit date can increase the chances of long-term success of a quit attempt by about 3–7 percentage points, whether it is given by phone or face-to-face (Lancaster & Stead, 2005 ). Group behavioural support (specialist-led groups of smokers stopping together and engaging in a structured discussion about their experiences), involving at least weekly sessions lasting until at least 4 weeks after the target quit date can increase the chances of success of a quit attempt by a similar amount or possibly more than individual support (Stead & Lancaster, 2005 ). Scheduled, multi-session telephone support can improve rates of success at stopping smoking by a broadly similar amount (Stead, Hartmann-Boyce, Perera, & Lancaster, 2013 ) but some large studies have failed to detect an effect so contextual factors and/or the precise type of support could be crucial to success. The effects of behavioural support and medication/NRT on success at stopping smoking appear to combine roughly additively (Stead, Koilpillai, & Lancaster, 2015 ). Smoking cessation support appears to be effective in primary care, secondary care and worksite settings (Cahill & Lancaster, 2014 ; West et al., 2015 ). Financial incentives, in the form of vouchers, have been found to increase smoking cessation rates for as long as they are in place (Cahill, Hartmann-Boyce, & Perera, 2015 ; Higgins & Solomon, 2016 ). Printed self-help materials can improve the chances of success at stopping long term by around 1–2 percentage points (Hartmann-Boyce, Lancaster, & Stead, 2014 ).
There is still relatively limited evidence on the effectiveness of digital support interventions for smoking cessation. Thus, while there is evidence that tailored, interactive websites can improve the chances of success at stopping smoking compared with no support, brief written materials or static information websites, many of those tested have not been found to be effective and it is not clear what differentiates those that are effective from those that are not (Graham et al., 2016 ). Text messaging programmes have been found to increase the chances of success of quit attempts by about 2–7 percentage points (Whittaker, McRobbie, Bullen, Rodgers, & Gu, 2016 ). There is currently insufficient evidence to know whether smartphone applications can improve success rates of quit attempts, although preliminary data suggest that they might (Whittaker et al., 2016 ). Evidence on alternative and complementary therapies is not sufficient to make confident statements about their effectiveness as aids to smoking cessation (Barnes et al., 2010 ; White, Rampes, Liu, Stead, & Campbell, 2014 ).
Overall, the highest smoking cessation rates appear to be achieved using specialist face-to-face behavioural support together with either varenicline or dual form NRT. With this support, continuous abstinence rates up to 52 weeks, verified by expired-air carbon monoxide tests, of more than 40% have been achieved (Kralikova et al., 2013 ). More commonly, 52-week continuous abstinence rates with this treatment are between 15 and 25% (West et al., 2015 ).
Smoking cessation support for pregnant smokers
In pregnant smokers, there is some evidence that NRT can help promote smoking cessation but evidence for an effect sustained to end of pregnancy is not conclusive (Sterling et al., 2016 ). There is also evidence that written self-help materials and face-to-face behavioural support can aid smoking cessation (Jones, Lewis, Parrott, Wormall, & Coleman, 2016 ), and financial incentives have also been found to improve quitting rates among pregnant smokers (Tappin et al., 2015 ). Almost half of women who stop smoking during pregnancy as a result of a clinical intervention relapse to smoking within 6 months of the birth (Jones et al., 2016 ).
Effectiveness of programmes to reduce smoking uptake
School-based programmes that involve both social competence training and peer-led social influence have been found to reduce smoking uptake (Georgie, Sean, Deborah, Matthew, & Rona, 2016 ) but educational programmes have not (Thomas, McLellan, & Perera, 2013 ). Mass media campaigns and increasing the financial cost of smoking reduce smoking uptake (Brinn, Carson, Esterman, Chang, & Smith, 2012 ; van Hasselt et al., 2015 ).
Reducing the harm from tobacco and nicotine use
Smokers who report that they are reducing their cigarette consumption smoke only 1–2 fewer cigarettes per day on average than when they say they are not (Beard et al., 2013 ). Clinical trials have found that use of NRT while smoking can substantially reduce cigarette consumption compared with placebo (Royal College of Physicians, 2016 ) but national surveys show very little reduction in cigarette consumption when smokers take up use of NRT in real-world settings (Beard et al., 2013 ). The benefit from using NRT while continuing to smoke appears to be in promoting subsequent smoking cessation. Using NRT (or varenicline) to reduce cigarette smoking with no immediate plans to quit leads to increased rates of quitting subsequently (Wu, Sun, He, & Zeng, 2015 ).
‘Snus’, a form of tobacco that is placed between the gums and the cheek and which is prepared in a way that is very low in carcinogens, gives high doses of nicotine but without evidence of an increase in risk of major tobacco-related cancers and either no, or a small, increase in risk of heart disease. It does appear to increase risk of periodontal disease, however. Snus is very popular in Sweden. Sweden has very low rates of smoking and tobacco-related disease indicating that a form of nicotine intake other than smoking can become popular and suggesting that this can contribute to a substantial reduction in tobacco-related harm (Royal College of Physicians, 2016 ).
The introduction of complete bans on smoking in indoor public areas can also be considered as a harm reduction measure. In this case, the main issue is harm to non-tobacco users. The evidence shows that such bans have been rapidly followed in the UK and several other jurisdictions by a reduction in heart attacks in non-smokers (Action on Smoking and Health, 2014a ).
Conclusions
Tobacco smoking causes death and disability on a huge scale and only about half of smokers report enjoying it. Despite this, approximately 1 billion adults engage in this behaviour worldwide and only around 5% of unaided quit attempts succeed for 6 months or more. The main reason appears to be that cigarettes deliver nicotine rapidly to the brain in a form that is convenient, and palatable. Nicotine acts on the brain to create urges to smoke in situations where smoking would normally occur and when brain nicotine levels become depleted. Concern about the harm from, and financial cost of, smoking are mostly not sufficient to counter this.
Governments can reduce smoking prevalence by raising the cost of smoking through taxation, mounting sustained social marketing campaigns, ensuring that health professionals routinely advise smokers to stop and offer support for quitting, and make available pharmacological and behavioural support for stopping.
Statement of competing interests
RW has, within the past 3 years, undertaken research and consultancy for companies that develop and manufacture smoking cessation medications (Pfizer, GSK, and J&J). He is an unpaid advisor to the UK’s National Centre for Smoking cessation and Training. His salary is funded by Cancer Research UK.
Disclosure statement
No potential conflict of interest was reported by the author.
This work was supported by Cancer Research UK [grant number C1417/A22962].
- Action on Smoking and Health (2013). Smoking and reproduction . London: ASH; Retrieved from http://www.ash.org.uk/files/documents/ASH_112.pdf [ Google Scholar ]
- Action on Smoking and Health (2014a). Secondhand smoke . London: ASH; Retrieved from http://www.ash.org.uk/files/documents/ASH_113.pdf [ Google Scholar ]
- Action on Smoking and Health (2014b). What’s in a cigarette? London: ASH; Available from http://www.ash.org.uk/files/documents/ASH_117.pdf [ Google Scholar ]
- Action on Smoking and Health (2015a). Illicit trade in tobacco . London: ASH; Available from http://www.ash.org.uk/files/documents/ASH_122.pdf [ Google Scholar ]
- Action on Smoking and Health (2015b). Young people and smoking . London: ASH; Retrieve from http://www.ash.org.uk/files/documents/ASH_108.pdf [ Google Scholar ]
- Action on Smoking and Health (2016a). Smoking and mental health . London: ASH; Retrieved from http://www.ash.org.uk/files/documents/ASH_120.pdf [ Google Scholar ]
- Action on Smoking and Health (2016b). Smoking statistics: Illness and death . London: ASH; Retrieved from http://www.ash.org.uk/files/documents/ASH_107.pdf [ Google Scholar ]
- Action on Smoking and Health (2016c). Smoking statistics: Who smokes and how much? London: ASH; Retrieved from http://www.ash.org.uk/files/documents/ASH_106.pdf [ Google Scholar ]
- Anthenelli R. M., Benowitz N. L., West R., St Aubin L., McRae T., Lawrence D., & Evins A. E. (2016). Neuropsychiatric safety and efficacy of varenicline, bupropion, and nicotine patch in smokers with and without psychiatric disorders (EAGLES): A double-blind, randomised, placebo-controlled clinical trial . The Lancet , , 2507–2520. doi: 10.1016/s0140-6736(16)30272-0 [ PubMed ] [ CrossRef ] [ Google Scholar ]
- Australian Bureau of Statistics (2015). National health survey . Canberra: Author. [ Google Scholar ]
- Aveyard P., Begh R., Parsons A., & West R. (2012). Brief opportunistic smoking cessation interventions: a systematic review and meta-analysis to compare advice to quit and offer of assistance . Addiction , , 1066–1073. doi: 10.1111/j.1360-0443.2011.03770.x [ PubMed ] [ CrossRef ] [ Google Scholar ]
- Bala M. M., Strzeszynski L., Topor-Madry R., & Cahill K (2013). Mass media interventions for smoking cessation in adults . Cochrane Database of Systematic Reviews , , Cd004704. doi: 10.1002/14651858.CD004704.pub3 [ PubMed ] [ CrossRef ] [ Google Scholar ]
- Barnes J., Dong C. Y., McRobbie H., Walker N., Mehta M., & Stead L. F. (2010). Hypnotherapy for smoking cessation . Cochrane Database of Systematic Reviews , , Cd001008. doi: 10.1002/14651858.CD001008.pub2 [ PubMed ] [ CrossRef ] [ Google Scholar ]
- Beard E., McNeill A., Aveyard P., Fidler J., Michie S., & West R. (2013). Association between use of nicotine replacement therapy for harm reduction and smoking cessation: A prospective study of English smokers . Tobacco Control , , 118–122. 10.1136/tobaccocontrol-2011-050007 [ PubMed ] [ CrossRef ] [ Google Scholar ]
- Benowitz N. L. (1997). The role of nicotine in smoking-related cardiovascular disease . Preventive Medicine , , 412–417. 10.1006/pmed.1997.0175 [ PubMed ] [ CrossRef ] [ Google Scholar ]
- Benowitz N. L. (1998). Nicotine safety and toxicity . Oxford: Oxford University Press. [ Google Scholar ]
- Brinn M. P., Carson K. V., Esterman A. J., Chang A. B., & Smith B. J. (2012). Cochrane review: Mass media interventions for preventing smoking in young people . Evidence-based Child Health: A Cochrane Review Journal , , 86–144. 10.1002/ebch.v7.1 [ PubMed ] [ CrossRef ] [ Google Scholar ]
- Cahill K., Hartmann-Boyce J., & Perera R (2015). Incentives for smoking cessation . Cochrane Database of Systematic Reviews , , Cd004307. doi: 10.1002/14651858.CD004307.pub5 [ PubMed ] [ CrossRef ] [ Google Scholar ]
- Cahill K., & Lancaster T (2014). Workplace interventions for smoking cessation . Cochrane Database of Systematic Reviews , , Cd003440. doi: 10.1002/14651858.CD003440.pub4 [ PubMed ] [ CrossRef ] [ Google Scholar ]
- Cahill K., Lindson-Hawley N., Thomas K. H., Fanshawe T. R., & Lancaster T (2016). Nicotine receptor partial agonists for smoking cessation . Cochrane Database of Systematic Reviews , , Cd006103. doi: 10.1002/14651858.CD006103.pub7 [ PMC free article ] [ PubMed ] [ CrossRef ] [ Google Scholar ]
- Critchley J., & Unal B. (2003). Health effects associated with smokeless tobacco: A systematic review . Thorax , , 435–443. 10.1136/thorax.58.5.435 [ PMC free article ] [ PubMed ] [ CrossRef ] [ Google Scholar ]
- Dierker L., He J., Kalaydjian A., Swendsen J., Degenhardt L., Glantz M., & Merikangas K. (2008). The importance of timing of transitions for risk of regular smoking and nicotine dependence . Annals of Behavioral Medicine , , 87–92. doi: 10.1007/s12160-008-9051-x [ PMC free article ] [ PubMed ] [ CrossRef ] [ Google Scholar ]
- Doll R., Peto R., Boreham J., & Sutherland I. (2004). Mortality in relation to smoking: 50 years’ observations on male British doctors . British Medical Journal , , 1519. doi: 10.1136/bmj.38142.554479.AE [ PMC free article ] [ PubMed ] [ CrossRef ] [ Google Scholar ]
- Eriksen M., Mackay J., & Ross H (2013). The tobacco atlas . New York, NY: American Cancer Society. [ Google Scholar ]
- Ferri C. P., West R., Moriyama T. S., Acosta D., Guerra M., Huang Y., … Prince M. J. (2011). Tobacco use and dementia: Evidence from the 1066 dementia population-based surveys in Latin America, China and India . International Journal of Geriatric Psychiatry , , 1177–1185. doi: 10.1002/gps.2661 [ PubMed ] [ CrossRef ] [ Google Scholar ]
- Fidler J., Shahab L., & West R. (2011). Strength of urges to smoke as a measure of severity of cigarette dependence: Comparison with the fagerström test for nicotine dependence and its components . Addiction , , 631–638. 10.1111/add.2011.106.issue-3 [ PubMed ] [ CrossRef ] [ Google Scholar ]
- Fidler J., & West R. (2009). Self-perceived smoking motives and their correlates in a general population sample . Nicotine & Tobacco Research , , 1182–1188. doi: 10.1093/ntr/ntp120 [ PubMed ] [ CrossRef ] [ Google Scholar ]
- Fidler J., & West R. (2011). Enjoyment of smoking and urges to smoke as predictors of attempts and success of attempts to stop smoking: A longitudinal study . Drug and Alcohol Dependence , , 30–34. doi: 10.1016/j.drugalcdep.2010.10.009 [ PubMed ] [ CrossRef ] [ Google Scholar ]
- Georgie J. M., Sean H., Deborah M. C., Matthew H., & Rona C. (2016). Peer-led interventions to prevent tobacco, alcohol and/or drug use among young people aged 11–21 years: a systematic review and meta-analysis . Addiction , , 391–407. doi: 10.1111/add.13224 [ PMC free article ] [ PubMed ] [ CrossRef ] [ Google Scholar ]
- Gowing L. R., Ali R. L., Allsop S., Marsden J., Turf E. E., West R., & Witton J. (2015). Global statistics on addictive behaviours: 2014 status report . Addiction , , 904–919. doi: 10.1111/add.12899 [ PubMed ] [ CrossRef ] [ Google Scholar ]
- Graham A. L., Carpenter K. M., Cha S., Cole S., Jacobs M. A., Raskob M., & Cole-Lewis H. (2016). Systematic review and meta-analysis of internet interventions for smoking cessation among adults . Substance Abuse and Rehabilitation , , 55–69. doi: 10.2147/sar.s101660 [ PMC free article ] [ PubMed ] [ CrossRef ] [ Google Scholar ]
- Hartmann-Boyce J., Lancaster T., & Stead L. F (2014). Print-based self-help interventions for smoking cessation . Cochrane Database of Systematic Reviews , , Cd001118. doi: 10.1002/14651858.CD001118.pub3 [ PubMed ] [ CrossRef ] [ Google Scholar ]
- Higgins S. T., & Solomon L. J. (2016). Some recent developments on financial incentives for smoking cessation among pregnant and newly postpartum women . Current Addiction Reports , , 9–18. doi: 10.1007/s40429-016-0092-0 [ PMC free article ] [ PubMed ] [ CrossRef ] [ Google Scholar ]
- Hoffman S. J., & Tan C. (2015). Overview of systematic reviews on the health-related effects of government tobacco control policies . BMC Public Health , , 744. doi: 10.1186/s12889-015-2041-6 [ PMC free article ] [ PubMed ] [ CrossRef ] [ Google Scholar ]
- Hogg R. C. (2016). Contribution of monoamine oxidase inhibition to tobacco dependence: A review of the evidence . Nicotine & Tobacco Research , , 509–523. doi: 10.1093/ntr/ntv245 [ PubMed ] [ CrossRef ] [ Google Scholar ]
- Hughes J. R., Keely J., & Naud S. (2004). Shape of the relapse curve and long-term abstinence among untreated smokers . Addiction , , 29–38. 10.1111/add.2004.99.issue-1 [ PubMed ] [ CrossRef ] [ Google Scholar ]
- Hughes J. R., Stead L. F., Hartmann-Boyce J., Cahill K., & Lancaster T (2014). Antidepressants for smoking cessation . Cochrane Database of Systematic Reviews , , Cd000031. doi: 10.1002/14651858.CD000031.pub4 [ PMC free article ] [ PubMed ] [ CrossRef ] [ Google Scholar ]
- Jamal A. (2016). Current cigarette smoking among adults – United States, 2005–2015 . Morbidity and Mortality Weekly Report , , 1205–1211 [ PubMed ] [ Google Scholar ]
- Jamal A., Homa D., O’Connor E., Babb S., Caraballo R., Singh T., & King B. (2015). Current cigarette smoking among adults – United States, 2005–2014 . Morbidity and Mortality Weekly Report , , 1233–1240. 10.15585/mmwr.mm6444a2 [ PubMed ] [ CrossRef ] [ Google Scholar ]
- Jarvis M. J., Giovino G. A., O’Connor R. J., Kozlowski L. T., & Bernert J. T. (2014). Variation in nicotine intake among U.S. cigarette smokers during the past 25 years: Evidence from NHANES surveys . Nicotine & Tobacco Research , , 1620–1628. doi: 10.1093/ntr/ntu120 [ PubMed ] [ CrossRef ] [ Google Scholar ]
- Jha P., & Peto R. (2014). Global effects of smoking, of quitting, and of taxing tobacco . New England Journal of Medicine , , 60–68. doi: 10.1056/NEJMra1308383 [ PubMed ] [ CrossRef ] [ Google Scholar ]
- Jones M., Lewis S., Parrott S., Wormall S., & Coleman T. (2016). Re-starting smoking in the postpartum period after receiving a smoking cessation intervention: A systematic review . Addiction , , 981–990. doi: 10.1111/add.13309 [ PMC free article ] [ PubMed ] [ CrossRef ] [ Google Scholar ]
- Joossens L., & Raw M. (2003). Turning off the tap: The real solution to cigarette smuggling . International Journal of Tuberculosis and Lung Disease , , 214–222. [ PubMed ] [ Google Scholar ]
- Kotz D., Brown J., & West R. (2014a). Prospective cohort study of the effectiveness of smoking cessation treatments used in the “real world” . Mayo Clinic Proceedings , , 1360–1367. doi: 10.1016/j.mayocp.2014.07.004 [ PMC free article ] [ PubMed ] [ CrossRef ] [ Google Scholar ]
- Kotz D., Brown J., & West R. (2014b). ‘Real-world’ effectiveness of smoking cessation treatments: A population study . Addiction , , 491–499. doi: 10.1111/add.12429 [ PubMed ] [ CrossRef ] [ Google Scholar ]
- Kozlowski L. T., Porter C. Q., Orleans C. T., Pope M. A., & Heatherton T. (1994). Predicting smoking cessation with self-reported measures of nicotine dependence: FTQ, FTND, and HSI . Drug and Alcohol Dependence , , 211–216. 10.1016/0376-8716(94)90158-9 [ PubMed ] [ CrossRef ] [ Google Scholar ]
- Kralikova E., Kmetova A., Stepankova L., Zvolska K., Davis R., & West R. (2013). Fifty-two-week continuous abstinence rates of smokers being treated with varenicline versus nicotine replacement therapy . Addiction , , 1497–1502. doi: 10.1111/add.12219 [ PubMed ] [ CrossRef ] [ Google Scholar ]
- Lancaster T., & Stead L. F (2005). Individual behavioural counselling for smoking cessation . Cochrane Database of Systematic Reviews , , Cd001292. doi: 10.1002/14651858.CD001292.pub2 [ PubMed ] [ CrossRef ] [ Google Scholar ]
- Levy D. T., Huang A. T., Havumaki J. S., & Meza R. (2016). The role of public policies in reducing smoking prevalence: Results from the Michigan SimSmoke tobacco policy simulation model . Cancer Causes and Control , , 615–625. doi: 10.1007/s10552-016-0735-4 [ PMC free article ] [ PubMed ] [ CrossRef ] [ Google Scholar ]
- Lindson-Hawley N., Banting M., West R., Michie S., Shinkins B., & Aveyard P. (2016). Gradual versus abrupt smoking cessation: A randomized, controlled noninferiority trial . Annals of Internal Medicine , , 585–592. doi: 10.7326/m14-2805 [ PubMed ] [ CrossRef ] [ Google Scholar ]
- McNeill A., Brose L., Calder R., Hitchman S., Hajek P., & McRobbie H. (2015). E-cigarettes: An evidence update. A report commissioned by Public Health England . London: Public Health England. [ Google Scholar ]
- Noar S. M., Francis D. B., Bridges C., Sontag J. M., Ribisl K. M., & Brewer N. T. (2016). The impact of strengthening cigarette pack warnings: Systematic review of longitudinal observational studies . Social Science & Medicine , , 118–129. doi: 10.1016/j.socscimed.2016.06.011 [ PMC free article ] [ PubMed ] [ CrossRef ] [ Google Scholar ]
- Office of National Satistics (2016). Smoking prevalence in Great Britain . London: ONS; Retrieved from https://www.ons.gov.uk/peoplepopulationandcommunity/healthandsocialcare/drugusealcoholandsmoking/datasets/adultsmokinghabitsingreatbritain [ Google Scholar ]
- Royal College of Physicians and Royal College of Psychiatrists (2013). Smoking and mental health . London: RCP. [ Google Scholar ]
- Pirie K., Peto R., Reeves G. K., Green J., & Beral V. (2013). The 21st century hazards of smoking and benefits of stopping: A prospective study of one million women in the UK . Lancet , , 133–141. doi: 10.1016/s0140-6736(12)61720-6 [ PMC free article ] [ PubMed ] [ CrossRef ] [ Google Scholar ]
- Royal College of Physicians. (2016). Nicotine without smoke: Tobacco harm reduction . London: RCP. [ Google Scholar ]
- Schepis T. S., & Rao U. (2005). Epidemiology and etiology of adolescent smoking . Current Opinion in Pediatrics , , 607–612. 10.1097/01.mop.0000176442.49743.31 [ PubMed ] [ CrossRef ] [ Google Scholar ]
- Shahab L., & West R. (2009). Do ex-smokers report feeling happier following cessation? Evidence from a cross-sectional survey . Nicotine & Tobacco Research , , 553–557. doi: 10.1093/ntr/ntp031 [ PubMed ] [ CrossRef ] [ Google Scholar ]
- Shahab L., & West R. (2012). Differences in happiness between smokers, ex-smokers and never smokers: cross-sectional findings from a national household survey . Drug and Alcohol Dependence , , 38–44. doi: 10.1016/j.drugalcdep.2011.08.011 [ PubMed ] [ CrossRef ] [ Google Scholar ]
- Stapleton J. A., & West R. (2012). A direct method and ICER tables for the estimation of the cost-effectiveness of smoking cessation interventions in general populations: application to a new cytisine trial and other examples . Nicotine & Tobacco Research , , 463–471. doi: 10.1093/ntr/ntr236 [ PubMed ] [ CrossRef ] [ Google Scholar ]
- Stead L. F., Buitrago D., Preciado N., Sanchez G., Hartmann-Boyce J., & Lancaster T (2013). Physician advice for smoking cessation . Cochrane Database of Systematic Reviews , , Cd000165. doi: 10.1002/14651858.CD000165.pub4 [ PMC free article ] [ PubMed ] [ CrossRef ] [ Google Scholar ]
- Stead L. F., Hartmann-Boyce J., Perera R., & Lancaster T (2013). Telephone counselling for smoking cessation . Cochrane Database of Systematic Reviews , , Cd002850. doi: 10.1002/14651858.CD002850.pub3 [ PubMed ] [ CrossRef ] [ Google Scholar ]
- Stead L. F., Koilpillai P., & Lancaster T (2015). Additional behavioural support as an adjunct to pharmacotherapy for smoking cessation . Cochrane Database of Systematic Reviews , , Cd009670. doi: 10.1002/14651858.CD009670.pub3 [ PubMed ] [ CrossRef ] [ Google Scholar ]
- Stead L. F., & Lancaster T (2005). Group behaviour therapy programmes for smoking cessation . Cochrane Database of Systematic Reviews , , Cd001007. doi: 10.1002/14651858.CD001007.pub2 [ PubMed ] [ CrossRef ] [ Google Scholar ]
- Stead L. F., Perera R., Bullen C., Mant D., Hartmann-Boyce J., Cahill K., & Lancaster T. (2012). Nicotine replacement therapy for smoking cessation . Cochrane Database of Systematic Reviews , , Cd000146. doi: 10.1002/14651858.CD000146.pub4 [ PubMed ] [ CrossRef ] [ Google Scholar ]
- Sterling L. H., Windle S. B., Filion K. B., Touma L., & Eisenberg M. J. (2016). Varenicline and adverse cardiovascular events: A systematic review and meta-analysis of randomized controlled trials . Journal of the American Heart Association , , doi: 10.1161/jaha.115.002849 [ PMC free article ] [ PubMed ] [ CrossRef ] [ Google Scholar ]
- Talati A., Keyes K., & Hasin D. (2016). Changing relationships between smoking and psychiatric disorders across twentieth century birth cohorts: Clinical and research implications . Molecular Psychiatry , , 464–471. doi: 10.1038/mp.2015.224 [ PMC free article ] [ PubMed ] [ CrossRef ] [ Google Scholar ]
- Talip T., Murang Z., Kifli N., & Naing L. (2016). Systematic review of smoking initiation among Asian Adolescents, 2005–2015: Utilizing the frameworks of triadic influence and planned behavior . Asian Pacific Journal of Cancer Prevention , , 3341–3355. [ PubMed ] [ Google Scholar ]
- Tappin D., Bauld L., Purves D., Boyd K., Sinclair L., MacAskill S., & Coleman T. (2015). Financial incentives for smoking cessation in pregnancy: Randomised controlled trial . British Medical Journal , , h134. doi: 10.1136/bmj.h134 [ PubMed ] [ CrossRef ] [ Google Scholar ]
- Thomas R. E., McLellan J., & Perera R. (2013). School-based programmes for preventing smoking . Evidence-based Child Health: A Cochrane Review Journal , , 1616–2040. 10.1002/ebch.v8.5 [ PMC free article ] [ PubMed ] [ CrossRef ] [ Google Scholar ]
- US Department of Health and Human Services (2004). The health consequences of smoking: a report of the surgeon general (p. 62). Atlanta, GA: US Department of Health and Human Services, Centers for Disease Control and Prevention, National Center for Chronic Disease Prevention and Health Promotion, Office on Smoking and Health. [ Google Scholar ]
- US Department of Health Human Services (2014). The health consequences of smoking – 50 years of progress: A report of the surgeon general (p. 17). Atlanta, GA: US Department of Health and Human Services, Centers for Disease Control and Prevention, National Center for Chronic Disease Prevention and Health Promotion, Office on Smoking and Health. [ Google Scholar ]
- US Surgeon General. (1990). The health benefits of smoking cessation . Washington, DC: Department of Health and Human Services. [ Google Scholar ]
- Ussher M., Brown J., Rajamanoharan A., & West R. (2014). How do prompts for attempts to quit smoking relate to method of quitting and quit success? Annals of Behavioral Medicine , , 358–368. doi: 10.1007/s12160-013-9545-z [ PubMed ] [ CrossRef ] [ Google Scholar ]
- van Hasselt M., Kruger J., Han B., Caraballo R. S., Penne M. A., Loomis B., & Gfroerer J. C. (2015). The relation between tobacco taxes and youth and young adult smoking: What happened following the 2009 US federal tax increase on cigarettes? Addictive Behaviors , , 104–109. 10.1016/j.addbeh.2015.01.023 [ PMC free article ] [ PubMed ] [ CrossRef ] [ Google Scholar ]
- Vangeli E., Stapleton J., Smit E. S., Borland R., & West R. (2011). Predictors of attempts to stop smoking and their success in adult general population samples: A systematic review . Addiction , , 2110–2121. doi: 10.1111/j.1360-0443.2011.03565.x [ PubMed ] [ CrossRef ] [ Google Scholar ]
- Vink J. M., Willemsen G., & Boomsma D. I. (2005). Heritability of smoking initiation and nicotine dependence . Behavior Genetics , , 397–406. doi: 10.1007/s10519-004-1327-8 [ PubMed ] [ CrossRef ] [ Google Scholar ]
- West R. (2009). The multiple facets of cigarette addiction and what they mean for encouraging and helping smokers to stop . Chronic Obstructive Pulmonary Disease , , 277–283. 10.1080/15412550903049181 [ PubMed ] [ CrossRef ] [ Google Scholar ]
- West R., Baker C. L., Cappelleri J. C., & Bushmakin A. G. (2008). Effect of varenicline and bupropion SR on craving, nicotine withdrawal symptoms, and rewarding effects of smoking during a quit attempt . Psychopharmacology (Berl) , , 371–377. doi: 10.1007/s00213-007-1041-3 [ PubMed ] [ CrossRef ] [ Google Scholar ]
- West R., & Brown J. (2015). Smoking in England 2007–2014 . Retrieved from www.smokinginengland.info/latest-statistics/ [ Google Scholar ]
- West R., Raw M., McNeill A., Stead L., Aveyard P., Bitton J., & Borland R. (2015). Health-care interventions to promote and assist tobacco cessation: A review of efficacy, effectiveness and affordability for use in national guideline development . Addiction , , 1388–1403. doi: 10.1111/add.12998 [ PMC free article ] [ PubMed ] [ CrossRef ] [ Google Scholar ]
- West R., & Shiffman S (2016). Smoking cessation (3rd ed.). Abingdon: Health Press. [ Google Scholar ]
- White A. R., Rampes H., Liu J. P., Stead L.F., & Campbell J (2014). Acupuncture and related interventions for smoking cessation . Cochrane Database of Systematic Reviews , , Cd000009. doi: 10.1002/14651858.CD000009.pub4 [ PubMed ] [ CrossRef ] [ Google Scholar ]
- Whittaker R., McRobbie H., Bullen C., Rodgers A., & Gu Y. (2016). Mobile phone-based interventions for smoking cessation . Cochrane Database of Systematic Reviews , , Cd006611. doi: 10.1002/14651858.CD006611.pub4 [ PubMed ] [ CrossRef ] [ Google Scholar ]
- World Health Organization (2013). WHO report on the global tobacco epidemic, 2013: enforcing bans on tobacco advertising, promotion and sponsorship . Geneva: WHO. [ Google Scholar ]
- Wu L., Sun S., He Y., & Zeng J. (2015). Effect of smoking reduction therapy on smoking cessation for smokers without an intention to quit: An updated systematic review and meta-analysis of randomized controlled . International Journal of Environmental Research and Public Health , , 10235–10253. doi: 10.3390/ijerph120910235 [ PMC free article ] [ PubMed ] [ CrossRef ] [ Google Scholar ]
- Zhou X., Nonnemaker J., Sherrill B., Gilsenan A. W., Coste F., & West R. (2009). Attempts to quit smoking and relapse: Factors associated with success or failure from the ATTEMPT cohort study . Addictive Behaviors , , 365–373. doi: 10.1016/j.addbeh.2008.11.013 [ PubMed ] [ CrossRef ] [ Google Scholar ]

A .gov website belongs to an official government organization in the United States.
A lock ( ) or https:// means you've safely connected to the .gov website. Share sensitive information only on official, secure websites.
- About Health Effects of Cigarette Smoking
- Secondhand Smoke
- E-cigarettes (Vapes)
- Menthol Tobacco Products
- Morbidity and Mortality Weekly Reports (MMWR)
- About Surveys
- Other Tobacco Products
- Smoking and Tobacco Use Features
- Patient Care Settings and Smoking Cessation
- Patient Care
- Funding Opportunity Announcements
- State and Community Work
- National and State Tobacco Control Program
- Multimedia and Tools
Related Topics:
- Show All Home
- Tobacco - Health Equity
- Tobacco - Surgeon General's Reports
- State Tobacco Activities Tracking and Evaluation (STATE) System
- Global Tobacco Control
E-Cigarette Use Among Youth
What to know.
E-cigarettes are the most commonly used tobacco product among U.S. youth. No tobacco products, including e-cigarettes, are safe, especially for children, teens, and young adults. Learn more about e-cigarette use among youth.
- In the United States, youth use e-cigarettes, or vapes, more than any other tobacco product. 1
- No tobacco products, including e-cigarettes, are safe, especially for children, teens, and young adults. 2
- Most e-cigarettes contain nicotine, which is highly addictive. Nicotine can harm the parts of an adolescent's brain that control attention, learning, mood, and impulse control. 2
- E-cigarette marketing, the availability of flavored products, social influences, and the effects of nicotine can influence youth to start or continue vaping. 3 4
- Most middle and high school students who vape want to quit. 5
- Many people have an important role in protecting youth from vaping including parents and caregivers, educators and school administrators, health care providers, and community partners.
- States and local communities can implement evidence-based policies, programs, and services to reduce youth vaping.
E-cigarette use among U.S. youth
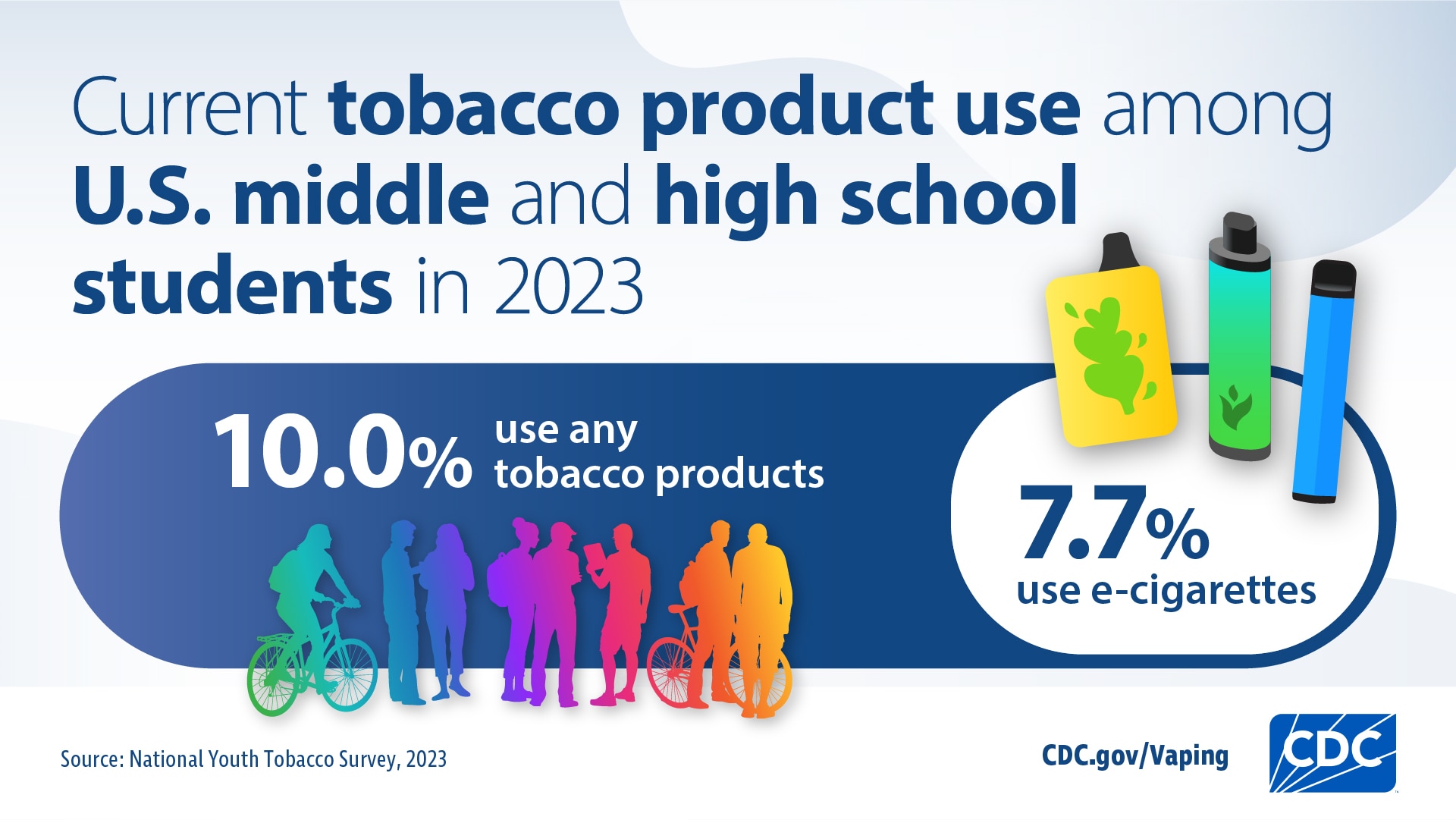
In 2023, e-cigarettes were the most commonly used tobacco product among middle and high school students in the United States. In 2023: 6
- 550,000 (4.6%) middle school students.
- 1.56 million (10.0%) high school students.
- Among students who had ever used e-cigarettes, 46.7% reported current e-cigarette use.
- 1 in 4 (25.2%) used an e-cigarette every day.
- 1 in 3 (34.7%) used an e-cigarette on at least 20 of the last 30 days.
- 9 in 10 (89.4%) used flavored e-cigarettes.
- Most often used disposable e-cigarettes (60.7%) followed by e-cigarettes with prefilled or refillable pods or cartridges (16.1%).
- Most commonly reported using the following brands: Elf Bar, Esco Bars, Vuse, JUUL, and Mr. Fog.
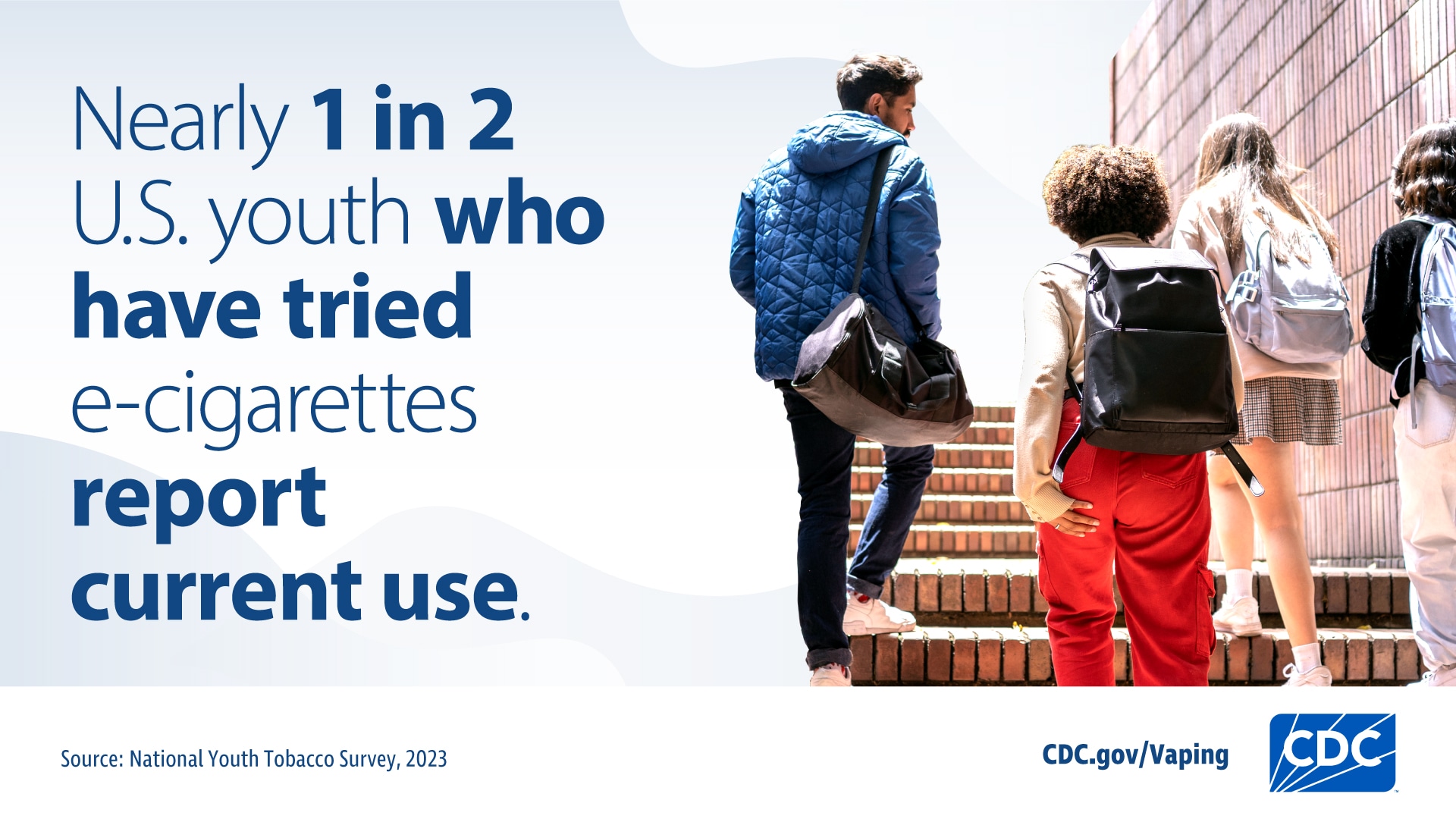
Most middle and high school students who vape want to quit and have tried to quit. 5 In 2020:
- 63.9% of students who currently used e-cigarettes reported wanting to quit.
- 67.4% of students who currently used e-cigarettes reported trying to quit in the last year.
Most tobacco use, including vaping, starts and is established during adolescence. There are many factors associated with youth tobacco product use . These include:
- Tobacco advertising that targets youth.
- Product accessibility.
- Availability of flavored products.
- Social influences.
- Adolescent brain sensitivity to nicotine.
Some groups of middle and high school students use e-cigarettes at a higher percentage than others. For example, in 2023: 6
- More females than males reported current e-cigarette use.
- Non-Hispanic multiracial students: 20.8%.
- Non-Hispanic White students: 18.4%.
- Hispanic or Latino students: 18.2%.
- Non-Hispanic American Indian and Alaska Native students: 15.4%.
- Non-Hispanic Black or African American students: 12.9%.
Many young people who vape also use other tobacco products, including cigarettes and cigars. 7 This is called dual use. In 2020: 8
- About one in three high school students (36.8%) who vaped also used other tobacco products.
- One in two middle school students (49.0%) who vaped also used other tobacco products.
E-cigarettes can also be used to deliver other substances, including cannabis. In 2016, nearly one in three (30.6%) of U.S. middle and high school students who had ever used an e-cigarette reported using marijuana in the device. 9
- Park-Lee E, Ren C, Cooper M, Cornelius M, Jamal A, Cullen KA. Tobacco product use among middle and high school students—United States, 2022 . MMWR Morb Mortal Wkly Rep. 2022;71:1429–1435.
- U.S. Department of Health and Human Services. E-cigarette Use Among Youth and Young Adults: A Report of the Surgeon General . Centers for Disease Control and Prevention; 2016. Accessed Feb 14, 2024.
- Apelberg BJ, Corey CG, Hoffman AC, et al. Symptoms of tobacco dependence among middle and high school tobacco users: results from the 2012 National Youth Tobacco Survey . Am J Prev Med. 2014;47(Suppl 1):S4–14.
- Gentzke AS, Wang TW, Cornelius M, et al. Tobacco product use and associated factors among middle and high school students—National Youth Tobacco Survey, United States, 2021 . MMWR Surveill Summ. 2022;71(No. SS-5):1–29.
- Zhang L, Gentzke A, Trivers KF, VanFrank B. Tobacco cessation behaviors among U.S. middle and high school students, 2020 . J Adolesc Health. 2022;70(1):147–154.
- Birdsey J, Cornelius M, Jamal A, et al. Tobacco product use among U.S. middle and high school students—National Youth Tobacco Survey, 2023 . MMWR Morb Mortal Wkly Rep. 2023;72:1173–1182.
- Wang TW, Gentzke AS, Creamer MR, et al. Tobacco product use and associated factors among middle and high school students—United States, 2019 . MMWR Surveill Summ. 2019;68(No. SS-12):1–22.
- Wang TW, Gentzke AS, Neff LJ, et al. Characteristics of e-cigarette use behaviors among US youth, 2020 . JAMA Netw Open. 2021;4(6):e2111336.
- Trivers KF, Phillips E, Gentzke AS, Tynan MA, Neff LJ. Prevalence of cannabis use in electronic cigarettes among U.S. youth . JAMA Pediatr. 2018;172(11):1097–1099.
Smoking and Tobacco Use
Commercial tobacco use is the leading cause of preventable disease, disability, and death in the United States.
For Everyone
Health care providers, public health.
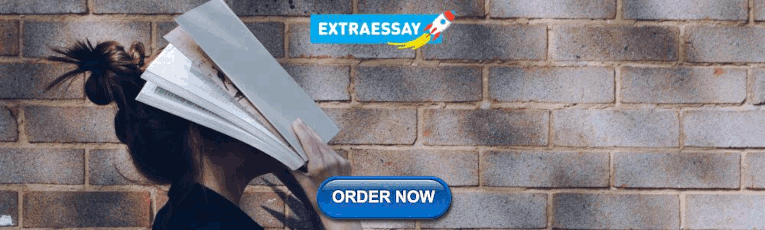
IMAGES
VIDEO
COMMENTS
Introduction. Tobacco smoking is one of the greatest threats to public health and is defined as any habitual use of the tobacco plant leaf.1 The use of tobacco is divided into combustible and non-combustible forms. Combustible tobacco products include cigarettes, cigars and water pipes, while electronic cigarettes and tobacco formulations developed for chewing or snuffing are classified as non ...
Smoking alone causes 90% of lung cancer, 80%. of chronic bronchitis, and 25% of other lung-related. diseases. None of the organs in the body are safe from. the harmful effects of tobacco. Smoking ...
Introduction. Academic performance and its relationship to adolescent smoking have been widely studied. Students who perform better academically are less likely to smoke, while those whose performance is weak smoke much more often (Bryant et al., 2000, Pennanen et al., 2011, Schnohr et al., 2009).Poor subjective health and factors related to well-being are also known to be related to ...
EFFECT OF CIGARETTE SMOKING ON THE ACADEMIC PERFORMANCE AMONG UNIVERSITY STUDENTS. September 2019. Authors: Sohayla Attalla. Mansoura University. Preprints and early-stage research may not have ...
57-63; doi 10.35198/01-2020-001-0009. Smoking Behaviour of Univ ersity. Students: a Descriptiv e Study. BACKGROUND: The theoretical background of the. study shows the importance of the issue of ...
research is to explore the effects of cigarette smoking on the academic achievement among university students. MATERIALS AND METHODS A total of 136 smoker students including 113 male and 23 female students from Management and Science University with 30 non smokers as control group are included in this research study. The total study
We identified three outcomes with a 4-star association with smoking: COPD (72% increase in risk based on the BPRF, 0.54 ROS), lower respiratory tract infection (54%, 0.43) and pancreatic cancer ...
Conclusion: Smoking status and nicotine dependence were predictive of worsening academic performance, including lower GPA, higher absenteeism rate and academic warnings. In addition, there is a substantial and unfavorable dose-response association between smoking history and cigarette consumption with impaired academic performance indicators.
Background Cigarettes and their by-products (i.e., smoke; ash) are a complex, dynamic, and reactive mixture of around 5,000 chemicals. Cigarette smoking potentially harms nearly every organ of the human body, causes innumerable diseases, and impacts the health of smokers and those interacting with the smokers. Smoking brings greater health problems in the long-term like increased risk of ...
This level of awareness is comparable to that in a study conducted in Jordan in 2012, where 92.4% of non-smoking university students were knowledgeable about the adverse effects of passive smoking on the general population. 13 Interestingly, our findings indicated that most participants recognised cigarette smoke as a significant contributor to ...
Background: Smoking behavior has been associated with poor academic performance among adult students worldwide. However, the detrimental effect of nicotine dependence on several students' academic ...
Background Smoking has a detrimental effect on the symptoms and severity of asthma, a common chronic disease among adolescents. The purpose of this study was to examine the association between asthma and smoking among high school students and assess provider-patient communication with asthmatic adolescents regarding smoking and adolescents' beliefs about the harms of smoking. Methods In fall ...
likely reflect the effects of initial withdrawal from nicotine. To examine the long-run effect of smoking cessation on mental health, we calculate the average of our distress scale and prescription drug indicators over interview years 2 through 5. We find small and statistically insignificant effects of the cessation program on these outcomes.
RESULTS: Survey results included a total of 2,714 student-responders with age range 10-20 years (mean age 13.9 years). 54% were males and 46% were females. Over-all, 31.6% of high school students have tried smoking, majority of whom experienced this in their early teens. A significantly higher incidence of smoking in the family is observed in ever-smokers compared to non-smokers.
This paper investigates hypotheses regarding the cause of the recent apparent increase in young adult smoking, compares trends in smoking among young adults with trends in the use of other substances, and considers the implications for youth tobacco control research and policy. Time series analyses of national data suggest that the recent observed increase in smoking among young adults is ...
The association of cigaret smoking by high school students with poor academic performance has been investigated in different studies. Our study conduct on the internship students of dental school ...
In 2023, e-cigarettes were the most commonly used tobacco product in the U.S., with 7.7% of middle school and high school students reporting e-cigarette use. Cigarettes were the next most common ...
Background and objectives: Despite reductions in prevalence in recent years, tobacco smoking remains one of the main preventable causes of ill-health and premature death worldwide.This paper reviews the extent and nature of harms caused by smoking, the benefits of stopping, patterns of smoking, psychological, pharmacological and social factors that contribute to uptake and maintenance of ...
Some groups of middle and high school students use e-cigarettes at a higher percentage than others. For example, in 2023: 6. More females than males reported current e-cigarette use. Current use of e-cigarettes varied by race and ethnicity. Non-Hispanic multiracial students: 20.8%. Non-Hispanic White students: 18.4%. Hispanic or Latino students ...
estimated that smoking increases the risk of. coronary heart disease about 2-4 times, stroke 2-4 times, lung cancer 25 times in. men, and 25.7 times in women. Be sides, smoking can lead to an ...