If you're seeing this message, it means we're having trouble loading external resources on our website.
If you're behind a web filter, please make sure that the domains *.kastatic.org and *.kasandbox.org are unblocked.
To log in and use all the features of Khan Academy, please enable JavaScript in your browser.
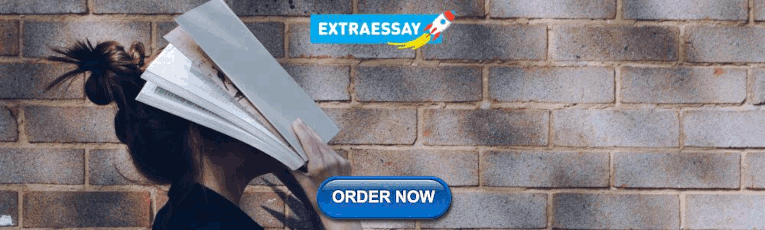
Biology library
Course: biology library > unit 1, the scientific method.
- Controlled experiments
- The scientific method and experimental design

Introduction
- Make an observation.
- Ask a question.
- Form a hypothesis , or testable explanation.
- Make a prediction based on the hypothesis.
- Test the prediction.
- Iterate: use the results to make new hypotheses or predictions.
Scientific method example: Failure to toast
1. make an observation., 2. ask a question., 3. propose a hypothesis., 4. make predictions., 5. test the predictions..
- If the toaster does toast, then the hypothesis is supported—likely correct.
- If the toaster doesn't toast, then the hypothesis is not supported—likely wrong.
Logical possibility
Practical possibility, building a body of evidence, 6. iterate..
- If the hypothesis was supported, we might do additional tests to confirm it, or revise it to be more specific. For instance, we might investigate why the outlet is broken.
- If the hypothesis was not supported, we would come up with a new hypothesis. For instance, the next hypothesis might be that there's a broken wire in the toaster.
Want to join the conversation?
- Upvote Button navigates to signup page
- Downvote Button navigates to signup page
- Flag Button navigates to signup page

Browse Course Material
Course info, instructors.
- Prof. Hazel Sive
- Prof. Tyler Jacks
- Dr. Diviya Sinha
Departments
As taught in.
- Biochemistry
- Cell Biology
- Developmental Biology
- Molecular Biology
Learning Resource Types
Introductory biology, teaching students to solve problems.
In this section, Prof. Hazel Sive describes this course’s focus on problem solving.
Problem Solving at MIT
I think the unofficial motto of MIT is “We solve problems.” Everything that we do here is to prepare our students to be problem solvers in the world. This idea permeates all the disciplines at MIT: engineering; science; business; architecture and urban planning; and humanities, arts, and social sciences. No matter what degree students earn at MIT, they leave with the ability to solve hard problems. When faced with a new problem, they know how to understand it, think about ways to solve it, try those ways, and ultimately get some kind of solution. That kind of philosophical and also real power gives students a big edge when they leave MIT and enter the workforce, go to graduate school, or go to medical school and become a physician.
It’s a difficult way to learn, but it’s a fantastic way to learn. I believe that learning should be a struggle; without struggle, you don’t get anywhere new. I think the courses at MIT are very challenging, and the introductory courses here are much harder than the introductory courses at most other universities.
Learning Terminology and Facts in Order to Solve Problems
Our course does include some rote learning, but the purpose of this rote learning is for our students to develop enough background to be able to speak the subject and understand and tackle challenging problems. They have to know what DNA is, what a gene is, and what a cell is. Very often, I’ll give them a term and I’ll say, “This is the scientific term. You should know it because it’s in your book, it’s in the scientific literature, and you’ll hear it on the news. But what’s most important is the concept underlying the term.” If you look at our problem sets and exams, you’ll see that there are no questions where students have to label a diagram, give a definition, or regurgitate facts.
Learning to Problem Solve through Practice
"It’s a terrific moment when a student realizes that this is different from any way they’ve been taught before, and they’re going to be challenged in ways in which they never knew they could be challenged."
In this course, students learn to solve problems through practice. Every two weeks, we give the students a problem set with six long problems. The problems are all about problem solving. The students look at the problems and realize that this isn’t just a matter of taking the lecture material and giving it back to us; we assume they know that information, and they’re expected to build from there. It’s very challenging for the students.
This is a shock to many of our students. In most high schools and even universities, biology is about learning facts. This was the case for me. I went to a very good university in South Africa. I learned all about the anatomy of the skull. I learned all about bones. I could classify fish. I learned many things that are very useful, but no one ever taught me how to solve a problem. Many of our students arrive at MIT having gotten the highest possible mark on the Advanced Placement ® biology exam, and when they get the first problem set in our course, they are stunned. They haven’t encountered biology as a kind of detective story where there’s a problem that they need to understand and solve. We explain that biology is a rigorous problem solving discipline; in fact, biology is all about using information to solve problems. It’s a terrific moment when a student realizes that this is different from any way they’ve been taught before, and they’re going to be challenged in ways in which they never knew they could be challenged.
The first problem set has to do with biochemistry. By the time they get the problem set, we’ve taught them about the various classes of molecules and macro-molecules that are found in living cells. We give them a problem set where not only do they have to be able to recognize something about the macro-molecules we present to them, they also have to recognize something about how the macro-molecules are put together, about bonding between the different parts of the macro-molecules, and about what that means for the structure of the macro-molecule, especially proteins. We do that both on paper and then also using a visualization program that was developed in the biology department called StarBiochem . In this program, the students are given a 3-dimensional structure of a protein, and they have to be able to understand what they’re looking at and what it means for the actual function of the protein, which is usually an enzyme that can catalyze a particular reaction. As soon as they see that problem set, they realize that this is going to be different from their high school biology experience.
As another example, when students learn about medical disorders, we don’t ask them to regurgitate the typical symptoms. Instead, we might say, “Here’s a patient that’s presenting with a funny disorder, and if she tries to move too quickly, she collapses. Her muscles look normal. Her nerves look normal, but if you do certain tests to them, you can see they’re not firing properly. Here’s what the trace of their firing pattern looks like. Suggest what’s wrong with the patient.”
The Problem Set Process
I tell the students that they have to practice these problems on their own. We can give pointers about how to solve the problems, but they need to think through the material. I tell them that when I’m thinking hard, I get a headache. For them, it might come as some other manifestation, but they should be getting their own personal version of a headache when they’re doing their problem sets. It shouldn’t be easy, but once they learn how to do a problem and get somewhere with a problem, it’s powerful. It empowers them to then go and tackle another one.
"Through this process, students show themselves that they can triumph over the work, and they come out actually having some power over the material."
For each problem set, I tell the students to print out three copies. First, students should take one copy and attempt the problem set all by themselves, without their notes and without help from others. They can identify what they don’t understand right away. They might get halfway through the problem set and panic upon realizing that they don’t know very much, that they went to lecture but didn’t absorb a lot of the material.
At that point, they can review their notes and their textbook, or go to the library, or search for information on the web. They learn what they can, then try the second problem set copy. Again, this is without help from other people; they need to personally struggle with the material. They always get farther the second time. The headache, the struggle, and then the triumph with bits of the problems is really powerful. Through this process, students show themselves that they can triumph over the work, and they come out actually having some power over the material.
Usually there will still be some holes in what they’re able to do and understand. Then, they can go and talk to their friends, their teaching assistants, and me or my co-instructor. They have to hand in their own work, in their own words, but they can work together and their work can have all these inputs. If they work as a group initially, they may miss getting that headache because they’re relying on their friends and people who may get it more quickly or in a different way than they do. I really discourage them from working together initially because I think they just don’t learn the material properly that way. They need to learn by doing. As they do more and more problems, they get better at addressing these questions. Students get substantial practice throughout the semester, and they come out really knowing something about how to solve problems in this particular area of life science.
Crafting Good Problems for 7.013
A critical part of our job as teachers is crafting good problems. We aim to create problems that have the following characteristics:
- Rooted in problem solving . A good problem should challenge students to think and to apply their knowledge in novel ways.
- Clearly written and easily understandable . The point of the problem should be clear.
- Built upon multiple aspects of the course material . Although the course is taught in a modular way, students cannot forget the earlier material as they learn new material. The early, fundamental material is used for all of the later lectures and problem sets. The best problems not only address the current module that they’re learning, but also draw upon and integrate past modules. For example, while learning about neurobiology, students should still remember that proteins only function properly if they’re put in the correct place in a cell.
- Informed by current literature . When possible, we like to draw upon real, current examples from the news and/or scientific literature. We usually take just one aspect of it and use it in a problem. When possible, we try to pick topics that we think students can relate to. This way, our problems are fresh, current, and interesting, and we never run out of ideas for problems.

You are leaving MIT OpenCourseWare
Problem-Solving in Biology Teaching: Students’ Activities and Their Achievement
- Published: 21 July 2023
- Volume 22 , pages 765–785, ( 2024 )
Cite this article
- Nataša Nikolić ORCID: orcid.org/0000-0001-8460-0430 1 &
- Radovan Antonijević ORCID: orcid.org/0000-0003-4959-376X 1
383 Accesses
1 Altmetric
Explore all metrics
Problem-solving is, by nature, a creative process which, by teaching through the implementation of research and discovery activities, allows students to create their knowledge, revise it and link it to broader systems. The aim of the research was to describe and analyse the process of solving biological problems through activities that are performed during the process of solving them, as well as to study how the implementation of these activities affects the level and quality of student achievement in biology. This study employed a quantitative method research strategy to describe the problem-solving process in biology teaching and determine student achievement. Data collection was by means of survey and testing. A Likert-scale survey and a biology knowledge test were constructed for the purposes of the research. For data analysis, descriptive statistics, factor analysis and the Pearson correlation coefficient were used. The data of eighth-grade students were collected from September 2016 to February 2017, in 72 schools in Serbia (565 students). The factor analysis confirmed that problem-solving activities could be grouped into the following five areas: (1) analysing and planning problem-solving; (2) discovering solution(s) to the problem; (3) problem-solving evaluation activities; (4) additional activities involving the discussion of the problem; (5) the degree of student independence in the process of discovering a solution to a problem. The results show that with the increasing frequency of the realisation of the research problem-solving activities, the achievement of students also increases. With regard to achievement quality, a positive but low correlation was found in all three domains—knowledge acquisition, understanding and application.
This is a preview of subscription content, log in via an institution to check access.
Access this article
Price includes VAT (Russian Federation)
Instant access to the full article PDF.
Rent this article via DeepDyve
Institutional subscriptions
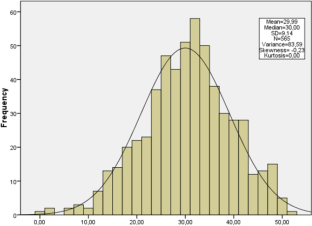
Similar content being viewed by others
The use of cronbach’s alpha when developing and reporting research instruments in science education.
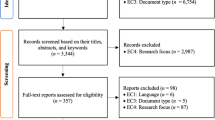
Trends in mathematics education and insights from a meta-review and bibliometric analysis of review studies
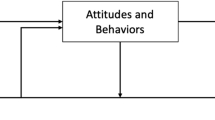
The impact of gamification in educational settings on student learning outcomes: a meta-analysis
Ali, A. R., Toriman, M. E., & Gasim, M. B. (2014). Academic achievement in biology with suggested solutions in selected secondary schools in Kano State, Nigeria. International Journal of Education and Research, 2 (11), 215–224.
Google Scholar
Anderson, J. R. (2005). Cognitive Psychology and Its Implications: Sixth Edition . Macmillan.
Aryulina, D., & Riyanto, R. (2016). A problem-based learning model in biology education courses to develop inquiry teaching competency of preservice teachers. Cakrawala Pendidikan, 35 (1), 47–57. https://doi.org/10.21831/cp.v1i1.8364
Article Google Scholar
Antonijević, R., & Nikolić, N. (2019). The role of problem-oriented teaching in the process of the development of critical and creative thinking. In V. Orlović Lovren, J. Peeters & N. Matović (Eds.), Quality of education: Global development goals and local strategies (pp. 49–63). Institute for Pedagogy and Andragogy.
Bowden, E. M. (1997). The effect of reportable and unreportable hints on anagram solution and the Aha! experience. Consciousness & Cognition, 6 (4), 545–573. https://doi.org/10.1006/ccog.1997.0325
Article CAS Google Scholar
Bruner, J., Goodnow, J., & Austin, A. (1956). A study of thinking . Wiley.
Cai, J., & Brook, M. (2006). Looking back in problem-solving. Mathematics Teaching, 196 , 42–45.
Chin, C., & Chia, L. G. (2006). Problem-based learning: Using ill-structured problems in biology project work. Science Education, 90 (1), 44–67. https://doi.org/10.1002/sce.20097
Article ADS Google Scholar
Chin, C., & Osborne, J. (2008). Students’ questions: A potential resource for teaching and learning science. Studies in Science Education, 44 (1), 1–39. https://doi.org/10.1080/03057260701828101
Costa, V., & Sarmento, R. P (2019). Confirmatory factor analysis–A case study . Retrieved from https://arxiv.org/ftp/arxiv/papers/1905/1905.05598.pdf
DeVellis, R. F. (1991). Scale development: Theory and applications . Sage Publications Inc.
Dunlap, J. C. (2005). Problem-based learning and self-efficacy: How a capstone course prepares students for a profession. Educational Technology Research and Development, 53 (1), 65–85. https://doi.org/10.1007/BF02504858
Etherington, M. B. (2011). Investigative primary science: A problem-based learning approach. Australian Journal of Teacher Education, 36 (9), 53–74. https://doi.org/10.3316/ielapa.328484780726539
Gagné, E. D., Yekovich, C. W., & Yekovich, F. R. (1993). The cognitive psychology of school learning (2nd ed.). HarperCollins College Publishers.
Gbore, L. O., & Daramola, C. A. (2013). Relative contributions of selected teachers’ variables and students’ attitudes toward academic achievement in biology among senior secondary schools students in Ondo State. Nigeria. Current Issues in Education, 16 (1), 1–11.
Gijbels, D., Dochy, F., Van den Bossche, P., & Segers, M. (2005). Effects of problem-based learning: A meta-analysis from the angle of assessment. Review of Educational Research, 75 (1), 27–61. https://doi.org/10.3102/00346543075001027
Harun, N. G., Yusof, K. H., Jamaludin, M. Z., Helmi, S. A., & Hassan, S. (2012). Motivation in problem-based learning implementation. Procedia-Social and Behavioral Sciences, 56 (2012), 233–242.
Hoskinson, A. M., Caballero, M. D., & Knight, J. K. (2013). How can we improve problem-solving in undergraduate biology? Applying lessons from 30 years of physics education research. CBE Life Sciences Education, 12 (2), 153–161.
Article PubMed PubMed Central Google Scholar
Hurst, R. W., & Milkent, M. M. (1996). Facilitating successful prediction problem-solving in biology through application of skill theory. Journal of Research in Science Teaching, 33 (5), 541–552.
Jamari, D., Mohamed, H., Abdullah, Z., Mohd Zaid, N., & Aris, B. (2018). Biology problem-solving: The high achiever students. European Proceedings of Social and Behavioural Sciences, 68 , 831–842.
Kapa, E. (2001). A metacognitive support during the process of problem solving in a computerized environment. Educational Studies in Mathematics, 47 (3), 317–336.
Kirui, J. M., & Kaluyu, V. (2018). Influence of selected psychosocial factors on learners’ performance in science subjects: A case of public secondary schools in Moyale Sub-County, Kenya. International Journal of Education and Research, 6 (1), 15–28.
Kolber, B. J. (2011). Extended problem-based learning improves scientific communication in senior biology students. Journal of College Science Teaching, 41 (1), 32–39.
Meiring, S. P. (1980). Problem solving. A basic mathematics goal, parts 1 and 2 . GLC Publishers, Agincourt.
Montague, M. (2005). Math problem solving for upper elementary students with disabilities (p. 8). The Access Center: Improving Outcomes for All Students K.
Nehm, R. H. (2010). Understanding undergraduates’ problem-solving processes. Journal of Microbiology & Biology Education, 11 (2), 119–122. https://doi.org/10.1128/jmbe.v11i2.203
Okoye, N. S., & Okechukwu, R. N. (2006). The effect of concept mapping and problem-solving teaching strategies on achievement in genetics among Nigerian Secondary School Students. African Journal of Educational Studies in Mathematics and Sciences, 4 , 93–98.
Polya, G. (1973). How to solve it: A new aspect of mathematical method (2nd ed.). Princeton University Press.
Ranjanie, B. (2017). Impact of problem-based learning on teaching biology for higher secondary students. International Journal of Current Research, 9 (12), 62932–62934.
Sabella, M. S., & Redish, E. F. (2007). Knowledge organization and activation in physics problem solving. American Journal of Physics, 75 (11), 1017–1029.
Squires, J. E., Estabrooks, C. A., Newburn-Cook, C. V., & Gierl, M. (2011). Validation of the conceptual research utilization scale: An application of the standards for educational and psychological testing in healthcare. BMC Health Services Research, 11 (1), 1–14.
Stanisavljević, J. D., & Đurić, D. Z. (2012). Efekat primene problemske nastave biologije na trajnost i kvalitet stečenih znanja [The effect of the application of problem-based biology teaching on the durability and quality of acquired knowledge]. Uzdanica, 9 (1), 303–312.
Sungur, S., Tekkaya, C., & Geban, O. (2006). Improving achievement through problem-based learning. Journal of Biological Education, 40 (4), 155–160.
Syafii, W., & Yasin, M. R. (2013). Problem-solving skills and learning achievements through problem-based module in teaching and learning biology in high school. Asian Social Science, 9 (12), 220–228. https://doi.org/10.5539/ass.v9n12p220
Thakur, P., & Dutt, S. (2017). Problem-based learning in biology: Its effect on achievement motivation of students of 9th standard. International Journal of Multidisciplinary Education and Research, 2 (2), 99–104.
Trauth Nare, A., Buck, G., & Beeman-Cadwallader, N. (2016). Promoting student agency in scientific inquiry: A self-study of relational pedagogical practices in science teacher education. In G. Buck & V. Akerson (Eds). Allowing our professional knowledge of pre-service science teacher education to be enhanced by self-study research: Turning a critical eye on our practice (pp. 43–67). Springer.
Woolfolk, A., Hughes, M., and Walkup, V. (2013). Psychology in Education (2nd ed.). Pearson Education.
Xun, G., & Land, S. M. (2004). A conceptual framework for scaffolding III-structured problem-solving processes using question prompts and peer interactions. Educational Technology Research and Development, 52 (2), 5–22. https://doi.org/10.1007/BF02504836
Download references
Author information
Authors and affiliations.
Department of Pedagogy and Andragogy, Faculty of Philosophy, University of Belgrade, Belgrade, Serbia
Nataša Nikolić & Radovan Antonijević
You can also search for this author in PubMed Google Scholar
Corresponding author
Correspondence to Nataša Nikolić .
Rights and permissions
Springer Nature or its licensor (e.g. a society or other partner) holds exclusive rights to this article under a publishing agreement with the author(s) or other rightsholder(s); author self-archiving of the accepted manuscript version of this article is solely governed by the terms of such publishing agreement and applicable law.
Reprints and permissions
About this article
Nikolić, N., Antonijević, R. Problem-Solving in Biology Teaching: Students’ Activities and Their Achievement. Int J of Sci and Math Educ 22 , 765–785 (2024). https://doi.org/10.1007/s10763-023-10407-5
Download citation
Received : 29 June 2021
Accepted : 07 July 2023
Published : 21 July 2023
Issue Date : April 2024
DOI : https://doi.org/10.1007/s10763-023-10407-5
Share this article
Anyone you share the following link with will be able to read this content:
Sorry, a shareable link is not currently available for this article.
Provided by the Springer Nature SharedIt content-sharing initiative
- Biology teaching
- Problem-solving
- Problem-solving activities
- Students’ achievement
- Find a journal
- Publish with us
- Track your research
Step by Step: Biology Undergraduates' Problem-Solving Procedures during Multiple-Choice Assessment
Affiliations.
- 1 Department of Integrative Biology, University of South Florida, Tampa, FL 33620.
- 2 Department of Biochemistry and Molecular Biology, University of Georgia, Athens, GA 30602 [email protected].
- PMID: 27909021
- PMCID: PMC5132368
- DOI: 10.1187/cbe.15-12-0255
This study uses the theoretical framework of domain-specific problem solving to explore the procedures students use to solve multiple-choice problems about biology concepts. We designed several multiple-choice problems and administered them on four exams. We trained students to produce written descriptions of how they solved the problem, and this allowed us to systematically investigate their problem-solving procedures. We identified a range of procedures and organized them as domain general, domain specific, or hybrid. We also identified domain-general and domain-specific errors made by students during problem solving. We found that students use domain-general and hybrid procedures more frequently when solving lower-order problems than higher-order problems, while they use domain-specific procedures more frequently when solving higher-order problems. Additionally, the more domain-specific procedures students used, the higher the likelihood that they would answer the problem correctly, up to five procedures. However, if students used just one domain-general procedure, they were as likely to answer the problem correctly as if they had used two to five domain-general procedures. Our findings provide a categorization scheme and framework for additional research on biology problem solving and suggest several important implications for researchers and instructors.
© 2016 L. B. Prevost and P. P. Lemons. CBE—Life Sciences Education © 2016 The American Society for Cell Biology. This article is distributed by The American Society for Cell Biology under license from the author(s). It is available to the public under an Attribution–Noncommercial–Share Alike 3.0 Unported Creative Commons License (http://creativecommons.org/licenses/by-nc-sa/3.0).
- Biology / education*
- Educational Measurement / methods*
- Likelihood Functions
- Probability
- Problem Solving*
Change Password
Your password must have 8 characters or more and contain 3 of the following:.
- a lower case character,
- an upper case character,
- a special character
Password Changed Successfully
Your password has been changed
- Sign in / Register
Request Username
Can't sign in? Forgot your username?
Enter your email address below and we will send you your username
If the address matches an existing account you will receive an email with instructions to retrieve your username
Variations in Student Approaches to Problem Solving in Undergraduate Biology Education
- Jeremy L. Hsu
- Rou-Jia Sung
- Su L. Swarat
- Alexandra J. Gore
- Stephanie Kim
- Stanley M. Lo
Schmid College of Science and Technology, Chapman University, Orange, CA 92866
Search for more papers by this author
Department of Biology, Carleton College, Northfield, MN 55057
Office of Institutional Effectiveness and Planning, California State University, Fullerton, CA 92831
Program in Mathematical Methods in Social Sciences, and
Program in Biological Sciences, Northwestern University, Evanston, IL 60201
*Address correspondence to: Stanley M. Lo ( E-mail Address: [email protected] )
Department of Cell and Developmental Biology
Joint Doctoral Program in Mathematics and Science Education, and
Research Ethics Program University of California San Diego, La Jolla, CA 92093
Existing research has investigated student problem-solving strategies across science, technology, engineering, and mathematics; however, there is limited work in undergraduate biology education on how various aspects that influence learning combine to generate holistic approaches to problem solving. Through the lens of situated cognition, we consider problem solving as a learning phenomenon that involves the interactions between internal cognition of the learner and the external learning environment. Using phenomenography as a methodology, we investigated undergraduate student approaches to problem solving in biology through interviews. We identified five aspects of problem solving (including knowledge, strategy, intention, metacognition, and mindset) that define three qualitatively different approaches to problem solving; each approach is distinguishable by variations across the aspects. Variations in the knowledge and strategy aspects largely aligned with previous work on how the use or avoidance of biological knowledge informed both concept-based and nonconcept-based strategies. Variations in the other aspects revealed intentions spanning complete disengagement to deep interest with the course material, different degrees of metacognitive reflections, and a continuum of fixed to growth mindsets. We discuss implications for how these characterizations can improve instruction and efforts to support development of problem-solving skills.
INTRODUCTION
Creating learning opportunities for students to engage with complex, real-world problems is a major goal for undergraduate science, technology, engineering, and math (STEM) education ( Harper, 2006 ; Klegeris and Hurren, 2011 ; Hoskinson et al. , 2013 ; Conana et al. , 2020 ; Avena et al. , 2021 ; Frey et al. , 2022 ). There have been multiple calls to align learning and teaching with real-world problems ( Jacob, 2004 ; American Association for the Advancement of Science, 2011 ). One important component is problem solving, where students apply concepts and think through a series of decisions that allow them to define, interpret, and solve a problem ( Martinez, 1998 ; Carlson and Bloom, 2005 ; Price et al. , 2021 ).
Multiple studies have characterized the knowledge and strategies that students use when solving problems in STEM ( Carlson and Bloom, 2005 ; Jones, 2009 ; Fredlund et al ., 2015 ; Price et al. , 2021 ; Frey et al. , 2022 ). Both domain-general knowledge (which can be applied across any discipline) and domain-specific knowledge (which are skills unique to a given discipline) can influence problem solving, suggesting the existence of discipline-related variations ( Alexander et al. , 1989 ; Jones, 2009 ; Fredlund et al. , 2015 ; Prevost and Lemons, 2016 ). In the context of undergraduate biology education, students utilize a variety of conceptual strategies (that rely on biological reasoning) and nonconceptual strategies (that are algorithmic or heuristic in nature but without connecting to biological principles) to solve problems ( Brumby, 1982 ; Hoskinson et al. , 2013 ; Avena and Knight, 2019 ; Avena et al. , 2021 ; Sung et al. , 2022 ). Similarly, a previous case study on problem solving identified one conceptual strategy grounded in biological understanding and two nonconceptual strategies based on algorithms or patterns ( Sung et al. , 2022 ). While our previous work aligns well with other existing literature, its focus on knowledge and strategies alone limits our understanding of how other aspects of student learning can impact problem solving.
A number of aspects that influence learning are correlated to how students solve problems in STEM. For example, students’ intentions for choosing particular problem-solving strategies inform the specific actions that they perform in engineering ( Case and Marshall, 2004 ). Similarly, the level of metacognitive reflection has been found to correlate with problem-solving outcomes in mathematics and medical sciences ( Safari and Meskini, 2016 ; Izzati and Mahmudi, 2018 ). Students’ mindsets, or their beliefs about their ability to improve, may also influence their problem-solving potential in mathematics ( Callejo and Vila, 2009 ). However, little work has been done to investigate how these aspects of intention, metacognition, and mindset interact with strategies to inform students’ problem-solving approaches, particularly in undergraduate biology education.
To complement existing work in the literature, we identify and characterize student approaches to problem solving in undergraduate biology education, while accounting for multiple aspects that influence learning. This current study significantly extends previous work ( Sung et al. , 2022 ) beyond considering only knowledge and strategies in problem solving, providing an opportunity to develop a more comprehensive model of how multiple aspects of problem solving are integrated in a particular approach. Specifically, our research question is: What are the qualitatively different approaches that undergraduate students use to solve problems in biology?
THEORETICAL FRAMEWORKS
In discipline-based education research across STEM, the term framework or theoretical framework is used inconsistently to convey a combination of conceptual perspectives that inform the overall interpretation of the results, methodological rationale that guides the research process from data collection to analysis, and existing literature on the research topic that situates the novel contributions of the conclusions ( Bussey et al. , 2020 ; Luft et al. , 2022 ). To distinguish these overlapping elements for our current study, we articulate situated cognition as a conceptual framework for understanding problem solving as a learning phenomenon, explain the utility of phenomenography as the methodology, and describe relevant literature on problem solving in STEM.
Situated cognition
Research on problem solving in STEM included cognitive, metacognitive, affective, and contextual dimensions ( Lee et al. , 1996 ; Taconis et al. , 2001 ; Shin et al. , 2003 ; Reigosa and Jiménez‐Aleixandre, 2007 ; Taasoobshirazi and Glynn, 2009 ; Jonassen, 2010 ; Löffler et al. , 2018 ; Akben, 2020 ). To account for these intersecting dimensions, we use situated cognition as the conceptual framework for this study ( Table 1 ). Situated cognition is a sociocultural learning theory positing that knowledge is not simply a product of cognition but is further situated in the activities, contexts, and cultures in which the knowledge is produced and used ( Brown et al. , 1989 ; Cakmakci et al. , 2020 ). Correspondingly, learning is viewed not just as constructing an understanding of disciplinary knowledge but rather more expansively as the interactions between the learner and the environment as learning occurs ( Lave and Wenger, 1991 ; Cakmakci et al. , 2020 ). Past work examining problem solving through the lens of situated cognition has identified that contextual and situational factors can impact students’ problem-solving approaches ( Kirsh, 2008 ; Roth and Jornet, 2013 ). By considering problem solving as a learning phenomenon from the perspective of situated cognition, we expect to identify a coherent way to understand student approaches to problem solving that can likely accommodate the different aspects in the existing literature, as well as additional dimensions related to course and disciplinary contexts that may emerge from the data as we observe students interacting with the problem-solving process.
Developed from situated cognition as a learning theory, cognitive apprenticeship describes six processes that can facilitate learning: modeling, coaching, scaffolding, articulation, reflection, and exploration ( Table 1 ; Hennessy, 1993 ). Modeling, coaching, and scaffolding are processes that involve an expert making explicit their tacit knowledge and thought processes, providing feedback as learners engage in a task, and designing increasingly complex tasks that successively expands on the understanding of a concept or development of a skill, respectively ( Hennessy, 1993 ; Cakmakci et al. , 2020 ). Articulation, reflection, and exploration are processes that involve learners describing their knowledge and reasoning, comparing their own thought processes with those of other people or a model, and entering a mode of learning on their own, respectively ( Hennessy, 1993 ; Cakmakci et al. , 2020 ), all of which relate to metacognition or a student’s ability to reflect on their own thinking ( Tanner, 2012 ). Because cognitive apprenticeship is a pedagogical articulation of situated cognition, fundamental insights about student approaches to problem solving emerging from this study can likely be translated into tangible teaching implications.
Phenomenography
We use phenomenography as the methodological framework to guide our research process ( Table 1 ). Phenomenography is the empirical examination of the variations in how people think about the world, with the goal of identifying and characterizing the qualitatively different ways of experiencing a specific phenomenon ( Figure 1 ; Marton, 1981 , 1986; Hajar, 2021 ). Variation theory in phenomenography further organizes the results of a research study into a two-dimensional outcome space , which articulates the aspects or specific features of a phenomenon that individuals are aware of and pay attention to, as well as the variations or distinctions in how each of these aspects are experienced by different individuals ( Table 1 ; Marton and Tsui, 2004 ; Åkerlind, 2018 ). Together across multiple aspects, the outcome space represents a hypothesis based on a set of logically related descriptions that define the qualitatively different ways of experiencing the phenomenon by different individuals ( Marton and Booth, 1997 ), that is, distinct approaches to problem solving.
FIGURE 1. Phenomenography as methodology. We use phenomenography to investigate the qualitatively different ways that undergraduate students approach problem solving in biology education. Instead of directly studying problem solving as a phenomenon, we are examining how different study participants experience and understand problem solving. The research team interacts empirically with study participants in terms of data collection and conceptually with the phenomenon of problem solving situated as positionality.
Variation theory can be further applied to explain how instructors and students may experience the same learning phenomenon differently ( Bussey et al. , 2013 ). Previously, this application of variation theory was used to articulate why it is important to examine instructors’ definition of understanding in biology education ( Hsu et al. , 2021 ) and students’ strategies in solving biology problems ( Sung et al. , 2022 ). Furthermore, variation theory can be used as a framework to examine how individual students may experience the same learning phenomenon differently. For example, when presented with an exam problem, students could attend to the features of the problem itself, patterns of the problem that are similar to other practice problems, or biological concepts embedded within the problem, thus resulting in a variety of conceptual and nonconceptual problem-solving strategies ( Sung et al. , 2022 ).
Phenomenography is a research methodology developed within higher education research by higher education researchers ( Tight, 2016 ) and has been used extensively to study learning and teaching across disciplines ( Akerlind, 2005 ; Booth, 1997 ; Entwistle, 1997 ), including STEM as well as medical and nursing education ( Swarat et al. , 2011 ; Stenfors-Hayes et al. , 2013 ; Barry et al. , 2017 ; Han and Ellis, 2019 ). Within this literature, an approach to learning was initially defined as having two aspects, including the strategy (plans for doing something) and the intention (motivation for doing it) ( Table 1 ; Marton, 1988 ; Case and Marshall, 2004 ). Three general student approaches to learning have been identified: (1) a surface approach that utilizes rote memorization to reproduce details of the course, (2) a procedural approach that relies on study strategies to achieve course outcomes, and (3) a deep approach that internalizes and assimilates meaning to understand disciplinary content ( Biggs, 1979 ; Entwistle et al. , 1979 ; Case and Marshall, 2009 ). Subsequent research highlighted the importance of affect and metacognition in student approaches to learning ( Case and Gunstone, 2002 ; Pintrich, 2004 ; Case, 2008 ). More recently, a few studies have specifically examined approaches to problem solving in biology, engineering, physics, and physiotherapy using phenomenography, further highlighting the potential connections between different aspects in problem solving across STEM ( Walsh et al. , 2007 ; Lönngren et al. , 2017 ; Dringenberg and Purzer, 2018 ; Dahlgren et al. , 2021 ; Sung et al. , 2022 ).
Problem solving in STEM
Existing literature on problem solving in undergraduate biology education primarily focus on knowledge and strategies. In addition to domain-general knowledge that can be applied across disciplines, students need to utilize declarative knowledge such as disciplinary concepts, procedural knowledge such as problem-solving strategies specific to the discipline, and conditional knowledge such as metacognitive awareness on when to use certain strategies ( Prevost and Lemons, 2016 ). Problem-solving strategies can be algorithmic, when students memorize a pattern or formula and utilize it without recognizing the underlying biological concepts ( Avena et al. , 2021 ; Sung et al. , 2022 ), or can include more complex sets of actions, where students organize biological concepts into a mental framework to solve the problem ( Nehm, 2010 ; Prevost and Lemons, 2016 ). Furthermore, students often rely on domain-general knowledge and nonbiological reasoning, particularly when approaching problems with higher-order cognitive demands in biology ( Prevost and Lemons, 2016 ; Sung et al. , 2022 ).
Beyond knowledge and strategies, approaches to learning and problem solving include intention , or motivation to use a certain strategy ( Table 1 ; Marton, 1988 ; Case and Marshall, 2004 ). Motivation is a complex term encompassing distinct and related constructs from multiple theoretical traditions ( Conradi et al. , 2014 ; Murphy et al. , 2019 ; Richardson et al. , 2020 ). One motivational factor that can influence student learning and persistence across STEM is interest ( Schiefele, 1991 ; Wang, 2013 ), a term that has been applied in many contexts in biology education research ( Rowland et al. , 2019 ). Development of interest as motivation for learning can be serendipitous (e.g., trigged by an unplanned event), promoted by other people (e.g., in response to external demands as imposed by course structure), or self-generated by students (e.g., internally recognizing connections among different concepts; Renninger and Hidi, 2022 ). Interest can drive student learning, leading to more efforts and reflections ( Zimmerman, 2002 ). While we acknowledge that there are entire bodies of research focused on motivation and interest, we use the term intention in this paper, operationalized as a combination of motivation and interest, to be consistent with prior literature in phenomenography that has studied student approaches to learning and problem solving.
Metacognition is another aspect that can influence problem solving and learning in undergraduate biology education ( Table 1 ; Tanner, 2012 ). There are multiple definitions of metacognition ( Schraw and Moshman, 1995 ; Pintrich, 2004 ; Veenman et al. , 2006 ; Dinsmore et al. , 2008 ). Here, we refer to metacognition based on self-regulated learning (SRL), where metacognition in conjunction with motivational and behavioral processes can influence the ability of learners to regulate their own learning ( Zimmerman, 2002 ; Sebasta and Bray Speth, 2017 ). In this definition, the term metacognition encompasses metacognitive knowledge (understanding and awareness of one’s own thinking and learning) and metacognitive processes or regulation (activation and utilization of specific reflective skills such as planning, monitoring, and evaluating to support learning; Pintrich, 2004 ; Dinsmore et al. , 2008 ; Stanton et al. , 2021 ). Past work has identified that increased metacognitive knowledge and processes can drive regulation of learning and improve problem solving ( Swanson, 1990 ; Antonietti et al. , 2000 ; Aşık and Erktin, 2019 ); thus, we hypothesize that different problem-solving approaches may be associated with specific forms of metacognition.
Mindset can refer to several distinct and related constructs that shape student beliefs about the nature of their abilities, which can impact learning; most work in this area has focused specifically on student beliefs about their ability to improve, which is the definition that we use in this study ( Table 1 ; Dweck and Yeager, 2019 ). Students who possess a growth mindset and believe that they are able to improve their skills tend to have more success at developing those skills, compared with students who possess a fixed mindset and believe that abilities are static and unmalleable ( Limeri et al. , 2020 ; Miller and Srougi, 2021 ). The impact of such mindsets on student learning is further influenced the instructor’s mindset as well as the sociocultural and institutional contexts of the learning environment ( Muenks et al. , 2020 ; Muenks et al. , 2021 ; Canning and Limeri, 2023 ). Because of these complex interactions among mindset, student learning, and the learning environment, we believe that this study with situated cognition as the conceptual framework can provide insights into how mindsets may impact student approaches to problem solving.
Taken together, the existing literature suggests that multiple aspects can influence student learning and problem solving. In a previous study, case studies were developed to describe the knowledge and strategies used by three participants in solving problems, with a focus on implications for assessments, and the results were largely aligned with those in the existing literature ( Sung et al. , 2022 ). However, there was additional richness to these participants’ experiences in other aspects such as intention, metacognition, and mindset that were left unexplored. More broadly, there is a need in the literature for studies that examine how these various aspects integrate together to form different approaches to problem solving, specifically in biology. Consequently, the goal of this study is to develop a comprehensive model for how students approach problem solving in undergraduate biology education.
Context and participants
This study took place at a 4-y, private not-for-profit, doctoral university with very high research activity in the United States, with an undergraduate profile described as 4-y, full-time, more selective, lower transfer-in, large, and primarily residential, according to the Carnegie Classification of Institutions of Higher Education ( McCormick and Zhao, 2005 ). We recruited 22 study participants over three academic years from an introductory course on genetics and molecular biology using a purposeful stratified sampling plan that included exam performance and gender ( Sung et al. , 2022 ). The sampling variables were included to maximize the potential number of aspects and variations in the outcome space for student approaches to problem solving ( Han and Ellis, 2019 ) but not to determine whether these variables correlated with the different approaches identified in the study. Additional descriptions on the study context and participants were reported previously ( Sung et al. , 2022 ).
We chose genetics as the disciplinary context because genetics has been identified as a critical component of undergraduate biology education ( Smith and Wood, 2016 ). Furthermore, there has been a long history of other work examining a variety of issues related to problem solving in genetics ( Tolman, 1982 ; Smith and Good, 1984 ; Stewart and Kirk, 1990 ; Cavallo, 1996 ; Avena et al. , 2021 ), providing an existing literature to build upon. Finally, a variety of qualitative and quantitative problems are available in genetics coursework that can be used as interview tasks ( Sung et al. , 2022 ).
Data collection and analysis
We used clinical interview originating from Piagetian constructivist traditions as a method ( Piaget, 2007 ) to explore students’ approaches to problem solving in biology. Additional descriptions on this method were reported previously ( Sung et al. , 2022 ). Here, we highlight that the interview protocol was semistructured, a common feature for phenomenography as a methodology ( Han and Ellis, 2019 ). Participants were asked to explain, elaborate, or confirm their problem solving approaches by verbalizing their thought processes and drawing diagrams ( diSessa, 2007 ) in interview tasks with problems that involved nondisjunction and genetic recombination, with possible follow-up prompts that further explore ideas brought up by participants (Supplemental Material). Participants were also asked if and how the course could be structured differently to support their learning, an interview question that was not analyzed in the previous study ( Sung et al. , 2022 ). The interviewers (S.L.S. and S.M.L.) had no prior contacts with the participants and were not involved with the courses that served as the recruitment site for this study. Interviews were audio-recorded and transcribed semiverbatim to remove verbal nods such as “um” and “ah” by a professional service, and Transcripts were spot-checked. Drawings also were collected as artifacts. Additional descriptions on the data collection process were reported previously ( Sung et al. , 2022 ).
Transcripts were analyzed using qualitative methodologies in three stages ( Saldana, 2021 ) as described previously ( Zuckerman and Lo, 2022 ). First, preliminary codes were developed through iterative close reading of the transcripts to provide a large corpus of thought processes emerging from the data (S.L.S., A.J.G., S.K., and S.M.L.). Second, codes were condensed to identify different aspects of approaches to problem solving, leading to the creation of a preliminary outcome space (J.L.H., R.J.S., and S.M.L.). Using constant comparative method ( Glaser, 1965 ), excerpts that describe each aspect and variations across the different approaches were contrasted with previously analyzed transcripts, allowing for the confirmation or disconfirmation of working conjectures. Third, data for each intersection in the preliminary outcome space were revisited to guard against biases, maintain consistency, and further refine specific variations from one approach to the next within each aspect, resulting in the final outcome space (J.L.H., R.J.S., and S.M.L.). We note that as the outcome space is emergent from the data, the interview protocol was not constructed around the aspects identified through this analysis process, nor were the participants asked to discuss them in the follow-up prompts. Finally, results are reported as excerpts associated with pseudonyms for study participants.
The data analyses in this current study were expanded from the set of interviews conducted previously ( Sung et al. , 2022 ). Revisiting prior data in what is called secondary analysis has emerged as common practice in qualitative methodologies in the past two decades ( Bishop and Kuula-Luumi, 2017 ). In general, data sources for such secondary analyses include formal sharing through public repositories, informal sharing among collaborators, and reuse of previously self-collected data to investigate additional research questions ( Heaton, 2008 ). This study falls under the third category of reusing our own data that were collected previously. Furthermore, there are five purposes to secondary analyses of qualitative data; specifically, this study falls under the category of supra analysis, where the focus of the new study transcends that of the previous work to address novel research questions and to reach new theoretical, empirical, and/or methodological insights ( Heaton, 2008 ).
Compared to the previous study ( Sung et al. , 2022 ), the results reported in this study represent a novel analyses of: (1) study participants who were not previously included, (2) additional data with previously unreported excerpts from the three study participants in the prior study, and (3) additional data from an interview question that had not been analyzed. We are also investigating a supra research question that asks how the previously identified strategies ( Sung et al. , 2022 ) are more comprehensively situated in an outcome space with other aspects of problem solving. Given that the goals of this study are to extend our previous work, we believe that it was of value to reexamine the previous interview data as well. Consequently, the excerpts reported in this study for the previous three case-study participants ( Sung et al. , 2022 ) represent an extension of existing work specific to the new research question outlined in this study.
Reliability, validity, and trustworthiness
In phenomenography, reliability focuses on dialogic agreement “through discussion and mutual critique of the data and of each researcher’s interpretive hypotheses” ( Åkerlind, 2005 ). The research team met regularly to examine the preliminary codes, the emergent aspects and their variations, and iterations of the outcome space, providing checks against personal biases and supporting reliability throughout the process. All disagreements were resolved through dialogic discussions in the form of argumentation ( Schoenfeld, 1992 ) to reach consensus on the structural relationships between the various aspects and approaches of the final outcome space.
Research in phenomenography is also expected to yield an outcome space considered to be appropriate and useful by relevant communities ( Entwistle, 1997 ; Åkerlind, 2005 ; van Rossum and Hamer, 2010 ; Hajar, 2021 ). Throughout data analyses, our results were presented to various communities of biology education researchers and practitioners, including faculty, staff, postdoctoral scholars, and students, for feedback. Validity stems from these repeated cycles of critique and refinement as well as the perceived usefulness of the outcome space, resulting in potentially new understanding of student learning and educational interventions ( Åkerlind, 2005 ).
Theoretical saturation was achieved by collecting and analyzing data through the constant comparative method over a sufficient length of time ( Aldiabat and Le Navenec, 2018 ) as described previously ( Sung et al. , 2022 ). In short, preliminary descriptions were developed from data in the first year and were confirmed by data in the second year. While refinement of the outcome space was supported by data in the third year, the overall structure of the outcome space did not change. Furthermore, the number of interviews conducted ( n = 22) are within the estimated range that would typically result in theoretical saturation ( Hennink et al. , 2017 ).
We recognize that our own experiences can influence the way we collected and analyzed the data, and we include a statement of positionality to situate our identities as researchers and educators in the context of this study to enhance the trustworthiness of our findings ( Bourke, 2014 ). J.L.H. and R.J.S. are pretenure faculty in biology at a comprehensive university and a primarily undergraduate institution, respectively, and both have programs in biology education research. A.J.G. and S.K. were undergraduate students majoring in quantitative methods in social sciences and biological sciences, respectively, when they contributed to the data analyses. S.L.S. has an M.S. in evolutionary biology and Ph.D. in learning sciences and was a senior research associate focused on STEM education at a teaching and learning center at the time of the study. S.M.L. is tenured teaching faculty in biology who primarily works in discipline-based education research at a research-intensive university. Together, our collective professional experiences with complementary expertise and career stages provide enriched interpretations of the data and guard against potential biases.
We identified the following five aspects with variations that distinguish different approaches to problem solving: (1) Knowledge: What is the knowledge base that students are drawing from as they work on problems? (2) Strategies: How are students connecting and applying their knowledge to reach an answer to the problem? (3) Intention: Why are students engaging with problems from this course and/or the discipline? (4) Metacognition: How do students reflect on their experiences when solving problems in biology? (5) Mindset: What are the beliefs that students have in their abilities to solve the problems?
Variations in each of these aspects revealed three hypothesized student approaches to problem solving in biology that aligned with approaches to learning previously identified in higher education ( Biggs, 1979 ; Entwistle et al. , 1979 ; Case and Marshall, 2009 ). To maintain continuity with this existing work, we have named our three approaches as the surface approach, procedural approach, and deep approach. Here, the surface approach to problem solving is primarily characterized by the use of nonbiological concepts in knowledge and strategy, a desire to complete the course as the primary intention, minimal metacognitive reflection, and a fixed mindset about biology. In the procedural approach, students develop pattern-based processes from prior experience with course-specific material but do not rely on a conceptual understanding of biology; the metacognition, intention, and mindset aspects of this approach reflect complex dynamics between interest in biology as a discipline but a fixed mindset with regards to the specific genetics course in the study. The deep approach is characterized by a strong emphasis on biological concepts and pronounced interest in the intellectual challenges afforded by participation in biology and/or genetics.
In the sections below, we detail the variations in each aspect along with the three hypothesized approaches to problem solving in undergraduate biology education. Although most participants displayed variations in all aspects (i.e., knowledge, strategies, intention, metacognition, and mindset) that correlated within one approach (i.e., surface, procedural, or deep), we also identified some participants who displayed hybrid combinations of variations related to distinct approaches in different aspects. Such inconsistencies within an outcome space can occur when individuals are transitioning from one approach to another or are at a liminal state in the learning process ( Cousin, 2006 ; Land et al. , 2014 ). Therefore, as we will further elaborate in the Discussion section, we do not necessarily view these approaches as fixed for a given participant in time and, in fact, propose that the variations we identified may be opportunities for fluidity in supporting how students can shift between approaches and towards the deep approach in the classroom.
Existing studies generally distinguished the knowledge underlying different problem-solving strategies as being conceptual (grounded in disciplinary concepts) or nonconceptual (not utilizing concepts in the discipline; Walsh et al. , 2007 ; Lönngren et al. , 2017 ; Sung et al. , 2022 ). In this study, we find that the binary distinction to be insufficient to capture the observed variations. While the surface and deep approaches are aligned with nonconceptual and conceptual cognitive knowledge, respectively, participants using the procedural approach cannot be fully characterized by either variation and instead demonstrate elements from both conceptual and nonconceptual knowledge. We can only fully capture the range of variations and describe all three approaches by considering two dimensions to knowledge emerging from the data: whether the knowledge involves biological concepts and what the participants consider as their source of the knowledge.
Key features of the surface approach in the knowledge aspect are a lack of biology, both in concept and in the source of the knowledge. For example, William described:
Usually what I do whenever I start a problem [is to] try to look at which ones are bogus answers. I look for the ones that don’t really make much sense and then from there I try to think about it. If I don’t necessarily know it, like with one of the questions, I kind of try to logically figure it out.
The knowledge used in William’s approach is devoid of biological concepts, and the primary source of knowledge is prior experience with the format and/or style of the question. In the excerpt, William focused on identifying self-described “bogus” answers in the question without mentioning any biological concepts for his initial attempts to solve the problem. William also hinted at a shift in his approach to use logic in figuring out the answer, and we will further explore this type of shift in the Discussion section.
The knowledge aspect in the procedural approach, similarly to that in the surface approach, does not rely on conceptual understanding of biology. However, participants characterized by the procedural approach drew on a different source of knowledge. Whereas in the surface approach, participants used knowledge based on the question itself irrespective of the course from which those experiences are drawn, in the procedural approach, students used their prior experiences with information specifically from this genetics course. For example, Martin described:
I worked enough problems that I saw, and also like in the solutions manual, I remember it stated that like a simple way you can tell the order of the genes was if there is only one type of like recombination that occurs, you can look at whatever occurs together.
Although Martin was clearly relying on knowledge sourced from other genetics problems in the course, he made no attempt to meaningfully connect this information to biological concepts. Therefore, even though participants using both the surface and procedural approaches did not incorporate biological concepts in their knowledge base when solving problems, superficial connections to biology may make an appearance in the procedural approach in the form of specific references to the course material. These examples highlight the variation in the knowledge aspect that distinguishes the surface approach from the procedural approach: Participants can draw on knowledge that is unrelated to biology (surface approach) or draw on information related to course material but use it in a way unconnected to biological concepts (procedural approach).
The knowledge aspect in the deep approach is characterized by its grounding in biological concepts, in contrast to that in the surface approach and the procedural approach. For example, Evan described:
Translocation is when a chunk from one chromosome breaks off and joins another chromosome and then the opposite chunk joins. So it’s just when chromosomes switch sections of DNA. I don’t how it’d affect map distance. It gets smaller, so it can’t be it. Yeah, that makes sense. It can’t be a translocation because it should do the opposite. It should be bigger. And there would be a new phenotype. Or usually there’s a new phenotype in translocation, when translocation happens because where the chromosome breaks, a new phenotype is created because the protein that’s encoded for at that breakage point gets messed up. So that’s why it’s not a translocation. It’s an inversion.
Evan used biological concepts and his internal conceptualization of this knowledge. Based on Evan’s logical reasoning, we argue that the source of knowledge went beyond simple recall of concepts from course material, as Evan was drawing inferences and making connections using his conceptual understanding.
Participants using the deep approach similarly drew on knowledge that originated from the course material and was grounded in their own understanding of biological concepts, reflecting a deep engagement between the participants as learners in the course and the course material as part of the learning environment. In contrast, while participants in the procedural approach also draw on knowledge that originated from the course material, this knowledge was grounded in processes that allowed the participants to bypass biological concepts as they solve the problem.
Variations in the knowledge aspect across the three distinct approaches provide a valuable lens with which to situate our current and prior work related to the strategy aspect. Contextualizing the strategy aspect with variations in the knowledge aspect allows us to identify connections between these two aspects within each of the three distinct approaches. Below, we apply this analysis to data from new participants specific to the current study as well as unreported data for Samuel, Kylie, and Michael (2022) from the previous case-study paper.
In the surface approach, the absence of biological concepts in the knowledge aspect likely reinforces a strategy that relies on information from a nonbiological source, such as the format, style, and/or language of the question itself. For example, William shared:
Generally, I don’t usually go with the nonpossible, so I pretty much cross that out from the beginning, because logically, I think there is something that goes on between one of the first couple of choices.
William’s strategy is based on the logic that it is necessary to eliminate nonpossible answers first, and from previous experience, those are typically the first few choices. Consequently, this strategy could potentially be applied to questions of a similar style in different contexts, not necessarily just in the genetics course. Similarly, Samuel explained how he eliminated one of the options in a multiple-choice question based on the structure of the options:
You realize that out of these four [options], like three of the four, like “describe four progeny being created”, so this one is two and two, this one is two and two, and this one is one, one, one, one, one, and this one is only one. So that’s another reason why [Option] A seems wrong, because it’s the odd one out in that sense, which again is not like super good related to biology skills.
The strategy aspect in both the surface and procedural approaches is characterized by patterns of process based on prior experience with similar problems. However, the knowledge base for the procedural approach relies on course-specific information such as practice problems, examples from class, and even (unintentional) instructor cues. Nonetheless, participants characterized by the procedural approach still focused on the nonconceptual features to support a strategy that allowed them to reason through the problems without conceptual understanding. For example, Martin shared:
I just looked at the numbers to see which was a double recombination and which one was a single recombination, and that is the way it was set up, the largest one was the parental and then smallest one was like the most recombinations. I think the most [the instructor] did was two. So I look at these numbers, and the smallest number is usually the double, and the largest is the parental. Again, I don’t really know why. I just know that the higher the frequency, the further away they are from each other, or the more like normal they are.
Martin acknowledged an explicit awareness of how to do the problem without any understanding of the concepts. Similarly, as seen below, Kylie explained her elaborate process for how to solve a recombination problem by identifying pair-wise additions that arithmetically summed to an even round number while also gauging the complexity of the problem based on the point value assigned to it. She further implied toward the end of this excerpt that other students in the course also used this type of strategy:
Also, it really helps because [the instructor] makes the numbers add up really nicely together, and you know if you’re doing it right, if you get an even number. So like here, you get 100. (…) So I knew that like you add 26 and 24, and you add 27 and 23, you get 50 each. So like that helped too. I mean, he said that out loud to us once. He was like, I’m not going to ask you to, it’s not a math test. It’s not like an understanding, but it’s a clue. And also, the point values tell you how difficult the problem is going to, like how difficult the explanation is. So like, sometimes he asks if, are these genes linked, and it’s like two points, then you know that it’s yeah they’re all linked, or they’re not linked. And if it’s more than that, then you know there’s something else going on. And it’s true every time. Don’t tell [the instructor] that, ’cause all the [other students] will hate me!
As opposed to processes based solely on the question itself, and absent any course context as seen in the surface approach, participants using the procedural approach were reliant on information related to the specific course material. The processes ranged in complexity from a simple single-step recall from course material to connecting multiple pieces of information and/or steps to form a more complex set of associations, as respectively exemplified by Martin’s and Kylie’s descriptions above.
The deep approach is also characterized by a range in complexity, from a single-step conceptual connection to an elaborate process involving multiple concepts. Instead of connecting course-based information and perceived rules to generate essentially a heuristic strategy as seen in the procedural approach, the strategy in the deep approach relies on connecting conceptual ideas grounded in biological understanding. For example, in a single-step conceptual connection, Evan described nondisjunction:
[Nondisjunction is] when the, in meiosis whenever all the chromosomes line up, and then the spindles attach, and pull them apart to separate them, haploid gametes. If for some reason, it doesn’t do that correctly, and it doesn’t split the two homologues up then, and say one gamete gets both, and one gets none, that’s a nondisjunction.
While it is possible that Evan could have memorized such a definition from a textbook, there was a certain fluency in how he described the idea using language grounded in biological concepts. In contrast, in the procedural approach, Cara described homologous chromosomes explicitly through references to visualizations provided in class: “In lecture, the visual [the instructor] put up made it seem like they, they’re similar, and so I’m like, and they look according in the visual [the instructor] put up in lecture, it looked like they were similar in size.” Evan further elaborated on how he solved a recombination problem, connecting multiple concepts and processes:
First, what you’d do is just draw the two chromosomes next to each other, and then you have to figure out which genes in what order. You knew from these numbers that these are the two wild-type phenotypes, and so in that worm, you know that the two chromosomes have these alleles, and you know the order, and you know which ones are on it, and it’s asking you what crossover would give rise to a wild-type progeny. You know that you have to get all, you have to get big C, big W, and big D. So the only way that happens is a crossover right there, which would give you all dominant alleles. So the hardest part is just figuring out what the gene order is, and which alleles are on which chromosome. And the way you get that information is from these two parental classes.
Even though Evan seemingly used what appears to be a heuristic process with sequential steps, it was also evident that this strategy involved connecting each step together using his conceptual understanding of the biological system. This aligns with the knowledge base identified for the deep approach, which is centered on the participant’s own understanding of biological concepts. It is interesting to note that Evan shifted to a formula-based strategy only when he did not understand or remember the meaning of a concept, i.e., interference in the excerpt below. We will further explore this type of shift in the Discussion section.
The last part is where applicable calculate a value for interference. And that’s just an equation. It’s one minus d, number of double recombinants, divided by the expected number of double recombinants. (…) That’s just the equation for interference. (…) Just whenever the number of double recombinants you get deviates from what you expect. Then interference is happening. I don’t know exactly why, or I don’t remember.
As we consider the connections between the two cognitive aspects of knowledge and strategy through the lens of situated cognition, we see that each approach begins to capture distinct interactions between the learner and the environment as learning occurs. For example, the near absolute avoidance of conceptual understanding and even in relation to the course material seen in the surface approach is suggestive of a lack of interaction, or disengagement, between the learner and the learning environment. The procedural approach is characterized by an almost transactional interaction; the selective knowledge that is observed and retained from the course is specific information such as formulas and patterns in previous questions that allows the learner to solve the problem without application of concepts. In the deep approach, the strong conceptual connections within both the knowledge and the strategy aspects suggests a deeper engagement in the interaction, in which the learner is internalizing and integrating concepts seen in the learning environment into their own understanding.
We characterize the intention aspect as the self-identified reasons why participants were interested and motivated to engage with problems from this course and/or the discipline. To describe the intention aspect, we consider two dimensions emerging from the data: desire and demand. We describe desire as being representative of the participant’s interest in being intellectually challenged and/or connected to the subject matter, likely correlating with interest in biological concepts and/or problem solving. On the other hand, demand captures the participant’s underlying motivation in the educational enterprise at hand, either specifically in the genetics course from which these problems originated or in the discipline of biology as a whole. Similar to our analysis of the knowledge aspect, the use of two dimensions allows us to more fully describe the variations in the intention aspect among the three distinct approaches.
Broadly, the intention aspect in the surface approach shows a disinterested perspective towards biology as a discipline, suggesting low interest in the underlying biological concepts and/or problem solving. There is a lack of desire to connect with the course material; consistently, the primary demand is motivation to complete the course and move past the content, external structures set forth by other people such as requirements for future careers or the instructor. For example, Samuel described:
I wish I was learning biology, but at some point, I have to square with the fact that I’m not. I hate this class, and I don’t think I like these kinds of premed sciences that much. You just have to get through it and then, ‘cause when you’re a doctor, I’m not going to be dealing with biology. So you just have to get through it, which is why I’m not giving it up just yet.
Similarly, William said:
I think I have never been like a cheat-for-the-test kind of person. I think learning it is probably better, but sometimes I don’t see things coming at all, and if I could be exposed to that during a review session or during class sometimes. We get taught how it works, but I don’t exactly see how that translates to problems at times, so I think getting exposed to problems more would probably help. I was trying to focus on what [the instructor] told us to study on.
In both examples, there was a lack of desire to connect to the material: Samuel did not consider the course learning biology and consequently hated the course, and for William, the perceived disconnection between what was taught and what was tested in the course frustrated him so much that he focused his efforts on what he was told to study. For both Samuel and William, this lack of connection to the course material reinforced the motivation to just finish the course.
The procedural approach is characterized by a combination of desire that is different from that of the surface approach and demand that is similar to that of the surface approach. For example, the demand of focusing on motivation to complete the course was also reflected in Kylie’s experience:
I thought it was kind of ridiculous that I knew all the information, yet I couldn’t apply it to anything, and I just think it’s a really bad way to test. [The instructor] did go through practice problems, but they were always straightforward. Then they threw you a curveball at you on the test. I’m used to tests being challenging and not presenting the information as you see it when you study, and that’s fine, but I feel like this had to do a lot with like logic and just like. I don’t know, I just, it’s not a logic class, you know. It’s biology. You just memorize how it’s done. I’m a little bit bitter about it. I kind of felt really frustrated, and the only reason why I would’ve bothered to play the game is because I’m competing with everyone else to do well, so I knew everyone else would.
Kylie’s response demonstrated her frustration that the genetics course material included on the exam did not reflect her preconceived idea of what biology should be, instead consisting of questions that were more akin to logic questions to her. This frustration underscored her need to play the game, with her primary demand also focused on just finishing the course. However, what distinguishes Kylie’s response from those of Samuel and William is her desire to be challenged on the test and/or see things presented differently in the course, suggesting interest in the underlying biological concepts and/or problem solving. This distinction is particularly striking given that William cited those exact reasons for not intellectually engaging with the class. This variation in desire, in this case a demonstrated interest to be intellectually challenged, is what distinguishes the procedural approach from the surface approach.
Similarly, Martin described:
Genetics was not my favorite. I was just like, I have to get through it to be honest. I just like really tried to cover as many problems as I could until I found a trend in the problems. And I don’t like that it is not really a way of learning, I don’t feel like. In molecular biology, because there were not so many problems per se, you really had to get an understanding of the concepts and like all the basics to really understand that, and I like that a lot better than just working problems to find an answer. Even in math the trend was always there, but you actually had to understand the basics. You couldn’t just memorize a typical pattern in the problems, because you know that wouldn’t help you all the time. These [genetics problems], if you found a pattern nine times out of ten, it would be the same, which is okay, but if the basic understanding of the concept isn’t there, I don’t feel like it is very helpful. I like learning a lot. That is why I am in college, I don’t like just doing problems and finishing it up there. I memorized how to do it, and applied it on the tests, and then pretty much forgot about it, because I wasn’t very interested in it.
Here, Martin expresses their feelings about genetics through comparisons to other courses he has taken, highlighting features that simultaneously underscored his sense of learning in those spaces and his sense of not learning in genetics. Similar to Kylie’s experience, Martin had a strong desire to be intellectually challenged. However, the seeming mismatch between how he expected those intellectual challenges to manifest in the course and how he actually did on the exam resulted in focusing the demand on his motivation to just move past the course.
Both procedural and deep approaches share the desire to be intellectually challenged; what distinguishes between the two is the variation in demand, where the deep approach is characterized by a self-generated motivation to learn both for themselves and in the course. For example, Evan shared:
I mean the test wasn’t, [the instructor] didn’t make it as hard as he could’ve, could’ve made it a lot trickier. But it’s just, there’s a lot of different things that could happen, and you have to really understand what causes those things to happen so that you, ’cause you can’t just memorize every scenario. You just have to logically work your way through it. It’s just a long process, and if you do that, you can get the right answer, but a lot of people struggled with that ’cause they don’t get that initial foundation to build off of.
Similarly, Sean explained:
I also appreciate the fact that there are some more difficult questions, such as these ones, that actually require you to, you know, work out a calculation or think of something in a new way that actually involves some sort of thought process, not just simple memorization of the notes, and then go to the test, and recognize the pattern, and just circle it. So, I appreciate the challenge. The bio class doesn’t really have that rule that you have to get a certain percentage right, it just encourages you, not just to, you know, focus on getting the right answer, and stress about that. It doesn’t matter if I get it right or wrong, as long as I learn.
These responses from Evan and Sean demonstrate that both participants appreciated intellectual challenges, having scenarios that were possibly beyond what had already been shown and citing the questions that required some sort of thought process compared with memorization of the notes. In turn, these participants indicated that their intention supported a demand that is largely driven by an internal motivation to deeply engage with course material. Moreover, the mechanism of this deeper engagement aligns with both the knowledge and strategy aspects already identified for the deep approach, where participants drew on their own conceptual understanding and form connections between these concepts to deduce a solution to the problem.
Similar to how we observed interactions between the knowledge and strategy aspects, it is possible that the intention aspect also aligns with other aspects within each approach. For example, avoiding the use of biological concepts in both knowledge and strategy in the surface approach could reinforce the notion that the participants were not learning biology, and the lack of desire or demand in their intention could in turn reinforce a deliberate choice to avoid the use of biological concepts to solve problems. Participants described above in the procedural approach commented on intellectual challenges when working through the problems; however, these challenges were sources of frustration that ultimately led to intellectual disengagement, reinforcing the use of biological concepts in strategies that supported completing course requirements rather than deeper conceptual learning.
Metacognition
Based on ideas emerging from the data, we focused this aspect on how participants reflected on their experience solving problems based on metacognitive knowledge and metacognitive process or regulation. Specifically, we examined how much participants were aware of their own knowledge and strategies in relation to the problems (metacognitive knowledge) and how the features of the course may influence connections between such awareness and problem solving by participants (metacognitive process or regulation).
The surface approach captures a largely passive engagement with the reflective process used by participants, with limited metacognitive knowledge and metacognitive process in their reflections. For example, Samuel described: “I think you’re gonna expose how superficial my understanding is. I’m not sure if that’s right. So I guess again, in explaining it, I’m realizing that I don’t know it as well as I thought I knew it.” Similarly, William states: “I know the thought process behind it. I think my biggest issue with genetics so far has been like I know what is going on, [but] I don’t know how it [is] going on.”
Both participants were clearly metacognitively aware of the limitations of their biological knowledge, recognizing that they had significant gaps in their conceptual understanding. Samuel named his understanding as superficial, whereas William identified his lack of understanding by distinguishing between his familiarity with the topic of what was going on versus his lack of the conceptual knowledge needed to solve a problem and explain how it was going on. In Samuel’s case, beyond identifying a sense that the course was not biology, there was limited reflection on metacognitive processes, with very little reflection on the additional factors that contributed to his experience. Similarly, as described earlier, William proffered only a superficial reflection on factors that would shape his metacognitive processes: “We get taught how it works, but I don’t exactly see how that translates to problems at times, so I think getting exposed to problems more would probably help.” In both cases, metacognition in the surface approach reflects some level of metacognitive knowledge and almost no metacognitive process, reflection that connects the knowledge and strategy aspects with broader contextual factors that may influence problem solving.
The procedural approach is characterized by a degree of reflection that captures a greater amount of metacognitive knowledge though with still a limited amount of metacognitive process, resulting in disconnections or incompatibilities between their increased metacognitive knowledge and their limited recognition of metacognitive processes. Specifically, participants using the strategic approach can recognize their own content knowledge and a set of expectations of what is necessary to achieve success in problem solving. While participants reflected on expectations of how the course should support them as learners, the contextual factors that shape the limited metacognitive processes that emerged as part of this reflection were often viewed as oppositions to their success. For example, Kylie indicated:
I have a friend that took this over the summer and is really good at logic problems and puzzles. Like he’s the best and he got like 95 [percent] on all his exams because of it. Even though I know for a fact I studied way harder than he did, and I probably knew the information better. It’s just like, you shouldn’t have a test that people do well because they’re good at taking it. It should be because you know the information better, you should do well, better than others, I guess.
Here, Kylie implicitly recognized that she was not as proficient as her friend in logic problems, conveying limited metacognitive processes. She explicitly laid out contextual reasons in her reflection for this limited metacognitive process, suggesting that her performance was due to the tests being a measure of logic rather than knowledge. In doing so, the reflection provided a more complex lens into her experience of problem solving than participants using the surface approach. Similarly, Bailey described:
I couldn’t get some of [the concepts]. I’m not sure. Some of these really screwed me up. I think my problem was that I never actually learned what was happening or like, why it would happen. It was like, okay, I see these numbers and so [the instructor] kinda asked the same questions, so I would try to figure out how to solve them instead of actually learning it. It definitely put me in a robot mode a little bit, maybe practice problems in class or explanation, like step-by-step what conceptually I guess is happening. I know [the instructor] did a little bit of that, but like I feel like a lot of people struggle with this concept.
Here, Bailey demonstrated her metacognitive knowledge by explicitly recognizing that she never learned some of the concepts and instead developed algorithmic strategies to solve the problems. Although she was less direct than Kylie, Bailey also highlighted limited ability for metacognitive processes, instead focusing on contextual reasons that would explain her lack of content knowledge and her reliance on nonconceptual problem-solving strategies. For instance, she cited how being asked similar questions and the lack of opportunity to review the conceptual basis for problems contributed to her not learning the biological concepts.
In contrast, the deep approach is characterized by reflections that recognize and connect metacognitive knowledge related to the expectations necessary to achieve success and a high degree of metacognitive processes that are essential for problem solving. These participants were able to distinguish specific patterns and processes that they used to improve their conceptual understanding and problem-solving capacities. For example, as described earlier, Evan noted that on the tests there were “a lot of different things that could happen, and you have to really understand what causes those things to happen” and as a result “you can’t just memorize every scenario.” Evan reflected on a difference in lower-order cognitive problems that required recall and higher-order problems that required application, and then he provided a strategy for how to solve the latter type of problems, stating that “[y]ou have to break it down, and you have to start from the beginning in terms of like segregation and stuff like that.” Similarly, Sean noted that:
There were questions on the test that I found to be very fair, very straightforward. If you had studied the notes and gone over the material and done the reading you would be in a good position to breeze through them, but I also appreciate the fact that there are some more difficult questions that actually require you to work out a calculation or think of something in a new way that actually involves some sort of thought process, not just simple memorization of the notes and then go to the test and recognize the pattern and just circle it.
Similar to Evan, Sean distinguished between cognitive levels of problems and reflected on his own metacognitive processes on what he needed to do to succeed, such as going over the notes and reading. Both participants indicated that the primary expectation of the course was to provide opportunities for developing problem-solving processes, typically in the form of higher-order cognitive problems. The alignment of metacognitive knowledge and metacognitive processes in these participants reflects a more cooperative relationship between the participants and the learning environment, whereas the reflections in the procedural approach highlight a more combative relationship or tension between these factors. In contrast, the minimal reflection seen in the surface approach represents a more passive engagement with metacognitive knowledge and metacognitive processes for problem solving.
Variations in the metacognition aspect also correlate with those in the aspects described earlier. The surface approach suggests a lack of connections to biological concepts and also a passive relationship to the course, both in intention and metacognition. The procedural approach focuses on a transactional view that uses course-based knowledge and strategies to complete the course requirements, correlating with greater metacognitive knowledge but still limited metacognitive processes. The deep approach emphasizes biological concepts in the knowledge and strategy aspects, an intention to be intellectually challenged and to engage with course material, and a connection between both metacognitive knowledge and processes.
Students can operate on a continuum of attitudes and beliefs between fixed mindset, believing that their intelligence and ability to do well cannot be improved, and growth mindset, believing that such qualities are malleable ( Yeager and Dweck, 2020 ). However, our data indicate that this nearly binary distinction of either a fixed or growth mindset as the two ends of the spectrum is not sufficient to describe the variations within this aspect for the three approaches. Instead, we articulate an additional dimension that further defines an intermediate point in the continuum between fixed and growth mindsets, i.e., whether participants viewed their learning from a deficit-based or asset-based perspective ( Denton et al. , 2020 ; Denton and Borrego, 2021 ).
The surface approach is characterized by attitudes and beliefs that learning cannot be improved as predetermined by certain factors, classic features of a fixed mindset. This variation also adopts a deficit-based perspective. For example, Michael shares:
I think people who have a natural aptitude towards biology maybe can figure [this problem] out. I have a friend who does really well on the tests, because she literally goes to the test and figures out the test. Like, she can read this and understand, think, and figure out what a homologous chromosome is. But I don’t have that kind of natural aptitude towards biology.
Michael then compares himself to another student and explicitly states that he believes that he will not be able to develop the needed aptitude for problem solving in biology, showing a fixed mindset toward his abilities to solve biological problems. He implies from a deficit perspective that he does not have an aptitude for biology, consistent with a fixed mindset that he will not be able to acquire the skills needed to solve the problem. He further characterizes this natural aptitude (or lack thereof) as something that is relevant for the entire field of biology. Michael’s reflections correlate with the idea of brilliance, that success requires innate, natural talent that cannot be taught ( Rattan et al. , 2012 , 2018).
Whereas participants using the surface approach show an alignment with fixed mindset inherently consistent with a deficit-based perspective, we found that the procedural approach can be described by a growth mindset but also coming from a deficit-based perspective. For example, Bailey explains:
I didn’t have the background to understand it in class. I took AP Bio sophomore year in high school, so it wasn’t recent to me anymore. I could’ve done this [problem] five years ago, easily. Trying to learn it in the short time I had, I like, you know, I just didn’t have it down.
Bailey viewed her lack of success as due to her missing some foundational background or ability in terms of a deficit. However, what distinguishes the procedural from the surface approach is the attitude and belief that she could have been able to succeed if she had had more time to learn and/or if the knowledge from her AP Biology experience had been more recent. Bailey also situated this deficit as specific to this genetics course, not necessarily to biology as a discipline, unlike Michael as illustrated in the surface approach. Consequently, this view that there are some specific deficits particular for this one course preventing success implies that these participants believe that they would be able to improve if they were able to rectify the deficit. Bailey further ascribed the path to success and thus improvement as one influenced by external factors, such as time because the prerequisite course was taken, rather than how her work and effort in the course could influence her ability to improve and successfully solve genetics problems. Therefore, participants using the procedural approach share elements of a growth mindset but from a deficit-based perspective.
The deep approach is similar to the procedural approach in that both are described by a growth mindset; however, the deep approach focuses on an asset-based perspective instead of a deficit-based perspective. Participants using the deep approach conveyed the attitudes and beliefs that they and their peers could take specific steps to gain skills and become better at solving problems in biology, focusing on internal factors on what they could do to improve rather than external constraints that limit their success. For example, Evan shares that “[y]ou just have to logically work your way through [problems], and it’s just a long process, and if you do that, you can get the right answer.” Similarly, Sean mentioned that he enjoyed doing practice problems for the following reason: “[i]t just encourages you, not just to focus on getting the right answer and stress about that, and instead it doesn’t matter if I get it right or wrong, as long as, like, I learn.” Both Evan and Sean described that working through problems will aid them in learning, improving, and gaining the ability to become better, aligning with a growth mindset from an asset-based perspective.
As described earlier, the connections between the cognitive aspects of knowledge and strategy suggest that each approach encapsulates distinct degrees of interactions with the learning environment on the basis of what knowledge was used in each strategy. Here, by considering multiple other aspects beyond knowledge and strategy, we can begin to formulate a more coherent understanding of each approach. For example, we can see that the lack of connections to biological concepts in knowledge and strategy correlated with a passive relationship to the course, both in intention and metacognition, and subsequently with a fixed mindset of not being able to improve in learning biology. In contrast, a transactional view as described by the knowledge and strategy aspects for the procedural approach fails to capture the sense of internal conflict between the student and the course environment as reflected in the intention, metacognition, and mindset aspects. On the other extreme, the depth of interactions between the learner and the learning environment seen in the knowledge and strategy aspects for the deep approach also correlate with the depth of engagement and cooperative relationship between internal factors of the participants and contextual factors in the course. By considering multiple dimensions of a learner’s experience with their environment through the lens of situated cognition, we see that individuals characterized by each of the approaches can have complex relationships with the course and course material. As we will discuss later, this creates potential opportunities for the instructor to acknowledge and address features of this interaction to potentially shift individuals’ problem-solving from one approach to another.
Our work provides one of the first examples to examine student approaches to problem solving in a broader and more holistic perspective that can potentially integrate multiple different aspects. The outcome space emerging from our data ( Figure 2 ) represents a hypothesis for how variations in each of the five aspects (i.e., knowledge, strategies, intention, metacognition, and mindset) are connected to one of the three approaches (i.e., surface, procedural, or deep). As we considered problem solving as a learning phenomenon through the lens of situated cognition, it is perhaps not surprising that we identified three approaches that are aligned with the previously characterized student approaches to learning ( Biggs, 1979 ; Entwistle et al. , 1979 ; Case and Marshall, 2009 ), even though we did not set out to constrain ourselves to a specific number of approaches in the outcome space. Classically, approaches as defined in phenomenography consisted only of strategies and intentions ( Marton, 1988 ; Case and Marshall, 2004 ). Here, our outcome space expands the number of aspects to include knowledge, metacognition, and mindset in addition to strategies and intentions.
FIGURE 2. Outcome space for different student approaches to problem solving. This outcome space articulates hypothesized relationships among the three approaches illustrated by specific variations across five aspects: the aspects of knowledge (blue), strategy (red), intention (green), metacognition (yellow), and mindset (purple). Under each aspect, variations are described to distinguish between two neighboring approaches, either surface versus procedural or procedural versus deep.
The three approaches to problem solving span a continuum from deliberate avoidance of using conceptual information to intentional application of biological concepts. The knowledge and strategy aspects align with other studies that have observed both biological and nonbiological strategies for problem solving ( Schoenfeld, 2016 ; Sung et al. , 2022 ). Moreover, we show that the knowledge and strategy aspects may be connected with other aspects including intention, metacognition, and mindset. These results provide an expanded view of factors that may impact how students solve problems beyond considering the domain-specific factors of declarative, procedural, and conditional knowledge ( Prevost and Lemons, 2016 ; Avena et al. , 2021 ).
Previous phenomenographic studies
Our current work is further situated in other phenomenographic studies characterizing problem solving across STEM disciplines ( Walsh et al. , 2007 ; Lönngren et al. , 2017 ; Dringenberg and Purzer, 2018 ; Dahlgren et al. , 2021 ; Sung et al. , 2022 ), which previously identified variations in problem solving in a limited number of aspects ( Figure 3 ). Therefore, our results extend these previous findings by providing an outcome space that incorporates a larger number of aspects and synthesizes the different aspects into a coherent hypothesis as a model for student approaches to problem solving.
FIGURE 3. Outcome space with mapping of prior work characterizing approaches to problem solving in STEM. Our outcome space is shown encompassing different approaches identified in previous phenomenographic studies, which are highlighted by different colors: sustainability in engineering education ( Lönngren et al. , 2017 ) in blue, physics education ( Walsh et al. , 2007 ) in red, collaborative problem solving in engineering education ( Dringenberg and Purzer, 2018 ) in green, physiology education ( Dahlgren et al. , 2021 ) in yellow, and biology education ( Sung et al. , 2022 ) in purple. The highlighted text represents the approaches as named and described in each of the original studies. Some of these approaches span multiple aspects. For example, the cognitive-based clinical reasoning approach in yellow aligns with the knowledge, strategy, and mindset aspects but not the intention and metacognition aspects.
In engineering education focusing on sustainability development, four problem-solving approaches were identified ( Lönngren et al. , 2017 ) that overlap with the variations in the strategy aspect in our outcome space ( Figure 3 , blue). In the simplify-and-avoid approach, students worked on the problems without structure or meaning; in the divide-and-control approach, students did not consider the context of the problem or draw upon knowledge of the field ( Lönngren et al. , 2017 ). This lack of conceptual application in both approaches parallels the strategies seen in the surface approach in our current study. In the isolate-and-succumb approach, students show some understanding of the concepts needed to solve the problem, but their responses remain superficial, disjointed, and lack recognition of the true complexity of engineering systems ( Lönngren et al. , 2017 ). This superficial and limited understanding of the system, despite drawing on information from the course, aligns with strategies in the procedural approach. In the integrate-and-balance approach, students show the most thorough understanding of the problem and apply appropriate engineering concepts to solve the problem ( Lönngren et al. , 2017 ), aligning with strategies in the deep approach.
In physics education, approaches to problem solving were identified ( Walsh et al. , 2007 ) with variations corresponding to the knowledge and strategy aspects in our outcome space ( Figure 3 , red). In the approach named no clear approach, students relied on features of the problem with no conceptual knowledge from the course to solve the problem ( Walsh et al. , 2007 ), aligning with knowledge and strategies in the surface approach in our current study. In two plug-and-chug approaches, students used heuristics that were either devoid of meaning in physics (memory based) or incorporated some conceptual understanding (structured), but this conceptual understanding was applied in a formulaic manner with limited meaning of the complexity of the system ( Walsh et al. , 2007 ). These features correspond to the knowledge and strategies in the procedural approach. In the scientific approach, students drew upon their own understanding and knowledge of physics principles to address each problem, aligning with knowledge and strategies in the deep approach.
Similarly, the previous study on student problem solving in biology ( Sung et al. , 2022 ) identified variations only in the knowledge and strategies aspects in the new outcome space presented in this paper ( Figure 3 , purple). In the nonconceptual approach, students utilized clues identified in the formatting of the question itself or in the language of the question to arrive at their final answer through a process of eliminating seemingly incorrect answers ( Sung et al. , 2022 ). These features correspond to the knowledge and strategies of the surface approach in our current study. In another nonconceptual approach, students relied on algorithmic processes that lacked conceptual understanding and were instead based on patterns identified from either instructor cues or previous problems ( Sung et al. , 2022 ). These features correspond to the knowledge and strategies of the procedural approach in our current study. Lastly, in the conceptual approach, students drew upon their own conceptual knowledge and understanding of biology in order to address the problem ( Sung et al. , 2022 ), aligning with the knowledge and strategy aspects of the deep approach identified in our current study.
Collaborative problem-solving approaches in engineering centered on two aspects ( Dringenberg and Purzer, 2018 ) aligned with variations in the intention and metacognition aspects in our outcome space ( Figure 3 , green). In one extreme, students using a completion approach focused primarily on completing the problem as quickly as possible and did not view their work as authentic engineering ( Dringenberg and Purzer, 2018 ), aligning with our surface approach characterized by a motivation to just complete the course (intention) and limited self-reflection that viewed the coursework as not biology (metacognition). In the middle, students using a transition approach recognized problem solving as a needed and fulfilling process that could lead them to success in future engineering classes but resisted working through the ambiguity inherent in complex engineering systems ( Dringenberg and Purzer, 2018 ), aligning with the conflicting desire and demand (intention) we observed in our procedural approach. In the other extreme, students using a growth approach viewed problem solving as an integral part of personal growth for becoming an engineer ( Dringenberg and Purzer, 2018 ), aligning with our deep approach characterized by sustained interest and motivation in the discipline (intention) and the ability to reflect on the specific concepts and steps needed to solve a problem (metacognition).
Two approaches to problem solving identified in physiotherapy education ( Dahlgren et al. , 2021 ) span the knowledge, strategy, and mindset aspects in our outcome space ( Figure 3 , yellow). In one approach called cognitive-based clinical reasoning, students relied on a step-by-step problem-solving process that was algorithmic in nature and only depended on a superficial understanding of physiotherapy; these students also centered their own experiences rather than the patient experience in physiotherapy, thus limiting how they adapt their learning to respond to new situations ( Dahlgren et al. , 2021 ). These features share similarities with the variations in the knowledge, strategy, and mindset aspects characterized by the procedural approach in our outcome space. In the second approach called relational understanding of clinical reasoning, students used understanding and reasoning in physiotherapy, which provided a more complex view that connected the student and patient; these students also indicated learning was an ongoing process and acknowledged the need to continually adapt to patient needs ( Dahlgren et al. , 2021 ). These features all align with the variations in knowledge, strategy, and mindset in the deep approach.
Our study expands the current literature on student problem-solving approaches by providing a more comprehensive outcome space that include many different aspects of problem solving. The other outcome spaces for problem solving in biology, engineering, physics, and physiotherapy education can be largely correlated within the one from our study, with some minor exceptions only in the strategy aspect ( Figure 3 ). In previous studies, strategies such as simplify-and-avoid versus divide-and-control ( Lönngren et al. , 2017 ) or memory-based versus structured plug-and-chug ( Walsh et al. , 2007 ) were separately identified within those outcome spaces; here, our data do not allow us to distinguish among these different strategies. Nonetheless, the similarities identified across these disciplines and in our work, which focuses on biology, illustrates that the outcome space emerging from this study may be applicable to other disciplines. Finally, by considering problem solving through the lens of situated cognition, our results allowed for an integration of various aspects that influence problem-solving, thus supporting the importance of sociocultural learning theories beyond cognition.
Implications for teaching
We observed some instances of participants switching approaches even within the same problem, such as Evan and William in this study and Michael in the previous study ( Sung et al. , 2022 ). Therefore, we posit that approaches to problem solving are malleable and that students can learn to adopt the deep approach. Prior work has indicated that changes in how a question is framed or how knowledge of biological concepts is activated can lead to differences in problem-solving strategies used by students ( Weston et al. , 2015 ; Avena and Knight, 2019 ). Similarly, student metacognition and mindset can shift based on the use of specific prompts ( Case and Gunstone, 2002 ; Berthold et al. , 2007 ; Tanner, 2012 ; Limeri et al. , 2020 ); However, much of this work has focused within a limited number of aspects, with little to no exploration on how shifts in one aspect may impact other aspects.
By identifying variations in each of the five aspects (i.e., knowledge, strategies, intention, metacognition, and mindset), our results provide additional context for considering interventions that could potentially shift students between approaches (i.e., surface, procedural, or deep), for example, by targeting transitions from one variation to another within one aspect. As demonstrated by other studies in phenomenography on learning in higher education ( Åkerlind, 2005 , 2018), we hypothesize that interventions shifting students from one variation to another within some aspects could also lead to changes in other aspects. In biology education research, interventions promoting metacognition can shift students’ intention from the surface approach towards the variations seen in the procedural or deep approaches while improving cognitive learning outcomes ( Conner, 2007 ; Tanner, 2012 ; Avargil et al. , 2018 ; Dang et al. , 2018 ). Across STEM disciplines, interventions that guide students away from fixed mindset toward growth mindset can positively influence intention and also cognitive learning outcomes ( Fink et al. , 2018 ; Muenks et al. , 2020 , 2021). Similarly, we speculate that providing students with structured guidance on how to become more aware of and to shape the thinking of others ( Halmo et al. , 2022 ) may spark changes in students’ intention from surface to procedural or deep approaches. For example, instructors who rely on small-group activities in class may wish to provide instructions, so that students have guidance on how to evaluate, question, and challenge their group members’ thinking or to elicit greater explanations from their peers ( Halmo et al. , 2022 ). Instructors may also wish to refer to recent evidence-based teaching guides on metacognition ( Stanton et al. , 2021 ) and problem-solving in biology ( Frey et al. , 2022 ).
To further consider implications for teaching, we draw upon the framework of cognitive apprenticeship ( Hennessy, 1993 ). Instructors could utilize interventions based on modeling and coaching that encourage intended and/or actual adoption of knowledge and strategies based on biological concepts and scaffolding in higher-order cognitive questions that promote understanding of biological concepts ( Tanner and Allen, 2005 ; Jensen et al. , 2014 ; Cleveland et al. , 2021 ), all of which are aligned with variations in the knowledge and strategy aspects of the deep approach. For example, when teaching problem solving, instructors can first link various concepts relating to the problem by explaining their connections and then demonstrate the conceptual steps needed to solve the problem (modeling). Next, instructors can provide time for students to work on similar problems and provide feedback during this process (coaching), before iterating through small-group activities with complex problems designed to expand students’ thinking (scaffolding). By directly modeling, coaching, and scaffolding the thinking process, instructors can encourage students to utilize conceptual strategies and draw upon biological knowledge when solving problems.
Similarly, instructors can take steps to promote student learning and metacognition through articulation, reflection, and exploration. Students who are able to internalize concepts and produce a mental model of the new information are more successful at learning ( Bierema et al. , 2017 ). As an example, instructors teaching problem solving in genetics can consider implementing a variant of the jigsaw intervention ( Aronson, 1978 ; Premo et al. , 2018 ; Baken et al. , 2022 ). For example, instructors can provide several scenarios that are related but require distinct solutions; students can work in small groups on one scenario, with different groups solving different scenarios. Next, the class forms new groups that merge students who worked on different scenarios. In these new groups, students can work together to share their solutions and tackle new problems that synthesize ideas from these different scenarios. In such a manner, students describe their knowledge and reasoning to each other (articulation), compare different approaches to related scenarios (reflection), and solve broader problems that require the integration of different concepts and strategies (exploration). Throughout this time, students develop their conceptual understanding of the relevant biological concepts and familiarity with different approaches to problem solving. In addition, structured reflection prompts from instructors throughout this process can be used to spark metacognition.
The relationship among different aspects influencing each other as well as impacting student learning has been empirically demonstrated in other contexts ( Case and Marshall, 2004 ; Limeri et al. , 2020 ). Theoretically, within phenomenography, the outcome space represents descriptions that coherently define the different ways of experiencing a phenomenon across the identified aspects ( Marton and Booth, 1997 ). Therefore, it is reasonable to hypothesize that by developing student metacognition or fostering an asset-based growth mindset in the classroom, we may be able to shift students toward the deep approach to problem solving. Together, we further speculate that there may be a complex, linked relationship among the five aspects of approaches to problem solving, and more work is needed to investigate the interaction among these different aspects.
LIMITATIONS AND FUTURE DIRECTIONS
We acknowledge that our results may not be broadly generalizable to biology education. Our study population includes students at a single institution who were enrolled in one specific course, albeit across several years. Furthermore, we chose genetics to be the disciplinary context for specific methodological reasons, and there may be differences in how students approach problem solving in other subdisciplines of biology. Nonetheless, the contribution of this study is the novel phenomenographic outcome space on student approaches to problem solving that incorporates many aspects previously observed in the education research literature across STEM disciplines.
Within our study sample, only a small number of participants described all five aspects of the outcome space, making it more challenging to draw inferences about the intersections between aspects and approaches. This limitation may be due to the variability in how loquacious participants were when reflecting upon one or more of the aspects. The interview protocol did not directly ask about each aspect, as they emerged from data analysis as part of the methodology in phenomenography ( Åkerlind, 2005 , 2018), Instead, our results illustrate how participants organically provided insights into these aspects when discussing their thought processes. Future work will be able to build upon this study by directly exploring the different aspects identified in the outcome space of approaches to solving problems.
We also did not explore what specific steps or sequential actions that students took to solve problems, as others have studied such heuristics ( Prevost and Lemons, 2016 ; Price et al. , 2021 ). Instead, this study focused on the aspects and variations in how students approach problem solving to generate complementary insights in the existing literature. Future work can explore how variations in these aspects intersect to influence each step of the problem-solving process.
ACKNOWLEDGMENTS
We are grateful to the study participants for their time, and we thank the course instructors for their support in providing the exam problems. We also thank R. Holmgren and G. Light for thoughtful discussions in the early phases of the study. This project was initiated with support by an institutional award from the Howard Hughes Medical Institute for undergraduate biology education under award number 52006934 and the Hewlett Fund for Curricular Innovation from the Weinberg College of Arts and Sciences at Northwestern University. S.K. and A.J.G. were supported by the Undergraduate Research Assistant Program in the Office of Undergraduate Research at Northwestern University. S.M.L. was supported in part by the Faculty Career Development Program at the University of California San Diego.
- Akben, N. ( 2020 ). Effects of the problem-posing approach on students’ problem-solving skills and metacognitive awareness in science education . Research in Science Education , 50 (3), 1143–1165. https://doi.org/10.1007/s11165-018-9726-7 Google Scholar
- Åkerlind, G. S. ( 2005 ). Variation and commonality in phenomenographic research methods . Higher Education Research & Development , 24 (4), 321–334. https://doi.org/10.1080/07294360500284672 Google Scholar
- Åkerlind, G. S. ( 2018 ). What future for phenomenographic research? On continuity and development in the phenomenography and variation theory research tradition . Scandinavian Journal of Educational Research , 62 (6), 949–958. https://doi.org/10.1080/00313831.2017.1324899 Google Scholar
- Aldiabat, K. M., & Le Navenec, C.-L. ( 2018 ). Data saturation: The mysterious step in grounded theory methodology . The Qualitative Report , 23 (1), 245–261. https://doi.org/10.46743/2160-3715/2018.2994 Google Scholar
- Alexander, P. A., Pate, P. E., Kulikowich, J. M., Farrell, D. M., & Wright, N. L. ( 1989 ). Domain-specific and strategic knowledge: Effects of training on students of differing ages or competence levels . Learning and Individual Differences , 1 (3), 283–325. https://doi.org/10.1016/1041-6080(89)90014-9 Google Scholar
- American Association for the Advancement of Science . ( 2011 ). Vision and change in undergraduate biology education: A call to action . Washington, DC: American Association for the Advancement of Science. Google Scholar
- Antonietti, A., Ignazi, S., & Perego, P. ( 2000 ). Metacognitive knowledge about problem-solving methods . British Journal of Educational Psychology , 70 (1), 1–16. https://doi.org/10.1348/000709900157921 Medline , Google Scholar
- Aronson, E. ( 1978 ). The Jigsaw Classroom . Thousand Oaks, CA: SAGE Publications. Google Scholar
- Aşık, G., & Erktin, E. ( 2019 ). Metacognitive experiences: Mediating the relationship between metacognitive knowledge and problem solving . Egitim ve Bilim/Education and Science , 44 (197), 85–103. Google Scholar
- Avargil, S., Lavi, R., & Dori, Y. J. ( 2018 ). Students’ metacognition and metacognitive strategies in science education . In Dori, Y. J.Mevarech, Z. R.Baker, D. R. Eds.), Cognition, Metacognition, and Culture in STEM Education: Learning, Teaching and Assessment (pp. 33–64). Cham, Switzerland: Springer International Publishing. Google Scholar
- Avena, J. S., & Knight, J. K. ( 2019 ). Problem solving in genetics: Content hints can help . CBE—Life Sciences Education , 18 (2), ar23. https://doi.org/10.1187/cbe.18-06-0093 Link , Google Scholar
- Avena, J. S., McIntosh, B. B., Whitney, O. N., Wiens, A., & Knight, J. K. ( 2021 ). Successful problem solving in genetics varies based on question content . CBE—Life Sciences Education , 20 (4), ar51. https://doi.org/10.1187/cbe.21-01-0016 Medline , Google Scholar
- Baken, E. K., Adams, D. C., & Rentz, M. S. ( 2022 ). Jigsaw method improves learning and retention for observation-based undergraduate biology laboratory activities . Journal of Biological Education , 56 (3), 317–322. https://doi.org/10.1080/00219266.2020.1796757 Google Scholar
- Barry, S., Ward, L., & Walter, R. ( 2017 ). Exploring nursing students’ experiences of learning using phenomenography: A literature review . Journal of Nursing Education , 56 (10), 591–598. https://doi.org/10.3928/01484834-20170918-03 Medline , Google Scholar
- Berthold, K., Nückles, M., & Renkl, A. ( 2007 ). Do learning protocols support learning strategies and outcomes? The role of cognitive and metacognitive prompts . Learning and Instruction , 17 (5), 564–577. https://doi.org/10.1016/j.learninstruc.2007.09.007 Google Scholar
- Bierema, A. M.-K., Schwartz, R. S., & Gill, S. A. ( 2017 ). To what extent does current scientific research and textbook content align? A methodology and case study . Journal of Research in Science Teaching , 54 (8), 1097–1118. https://doi.org/10.1002/tea.21399 Google Scholar
- Biggs, J. ( 1979 ). Individual differences in study processes and the quality of learning outcomes . Higher Education , 8 (4), 381–394. https://doi.org/10.1007/BF01680526 Google Scholar
- Bishop, L., & Kuula-Luumi, A. ( 2017 ). Revisiting qualitative data reuse: A decade on . SAGE Open , 7 (1), 2158244016685136. https://doi.org/10.1177/2158244016685136 Google Scholar
- Booth, S. ( 1997 ). On phenomenography, learning, and teaching . Higher Education Research & Development , 16 (2), 135–158. https://doi.org/10.1080/0729436970160203 Google Scholar
- Bourke, B. ( 2014 ). Positionality: Reflecting on the research process . The Qualitative Report , 19 (33), 1–9. https://doi.org/10.46743/2160-3715/2014.1026 Google Scholar
- Brown, J. S., Collins, A., & Duguid, P. ( 1989 ). Situated cognition and the culture of learning . Educational Researcher , 18 (1), 32–42. https://doi.org/10.3102/0013189X018001032 Google Scholar
- Brumby, M. N. ( 1982 ). Consistent differences in cognitive styles shown for qualitative biological problem-solving . British Journal of Educational Psychology , 52 (2), 244–257. https://doi.org/10.1111/j.2044-8279.1982.tb00833.x Google Scholar
- Bussey, T. J., Lo, S. M., & Rasmussen, C. ( 2020 ). Theoretical frameworks for STEM education research . In Johnson, C. C.Mohr-Schroeder, M. J.Moore, T. J.English, L. D. (Eds.), Handbook of Research on STEM Education (pp. 51–62). New York, NY: Routledge. Google Scholar
- Bussey, T. J., Orgill, M., & Crippen, K. J. ( 2013 ). Variation theory: A theory of learning and a useful theoretical framework for chemical education research . Chemistry Education Research and Practice , 14 (1), 9–22. https://doi.org/10.1039/C2RP20145C Google Scholar
- Cakmakci, G., Aydeniz, M., Brown, A., & Makokha, J. M. ( 2020 ). Situated cognition and cognitive apprenticeship learning . In Akpan, B.Kennedy, T. J. (Eds.), Science Education in Theory and Practice: An Introductory Guide to Learning Theory (pp. 293–310). Cham, Switzerland: Springer International Publishing. Google Scholar
- Callejo, M. L., & Vila, A. ( 2009 ). Approach to mathematical problem solving and students’ belief systems: Two case studies . Educational Studies in Mathematics , 72 (1), 111–126. https://doi.org/10.1007/s10649-009-9195-z Google Scholar
- Canning, E. A., & Limeri, L. B. ( 2023 ). Theoretical and methodological directions in mindset intervention research . Social and Personality Psychology Compass , 17 (6), e12758. https://doi.org/10.1111/spc3.12758 Google Scholar
- Carlson, M. P., & Bloom, I. ( 2005 ). The cyclic nature of problem solving: An emergent multidimensional problem-solving framework . Educational Studies in Mathematics , 58 (1), 45–75. https://doi.org/10.1007/s10649-005-0808-x Google Scholar
- Case, J. M. ( 2008 ). Alienation and engagement: Development of an alternative theoretical framework for understanding student learning . Higher Education , 55 (3), 321–332. https://doi.org/10.1007/s10734-007-9057-5 Google Scholar
- Case, J. M., & Marshall, D. ( 2009 ). Approaches to Learning . In Tight, M.Mok, K. H.Huisman, J.Morphew, C. (Eds.), The Routledge International Handbook of Higher Education (pp. 9–22). New York, NY: Routledge. Google Scholar
- Case, J., & Gunstone, R. ( 2002 ). Metacognitive development as a shift in approach to learning: An in-depth study . Studies in Higher Education , 27 (4), 459–470. https://doi.org/10.1080/0307507022000011561 Google Scholar
- Case, J., & Marshall, D. ( 2004 ). Between deep and surface: Procedural approaches to learning in engineering education contexts . Studies in Higher Education , 29 (5), 605–615. https://doi.org/10.1080/0307507042000261571 Google Scholar
- Cavallo, A. M. L. ( 1996 ). Meaningful learning, reasoning ability, and students’ understanding and problem solving of topics in genetics . Journal of Research in Science Teaching , 33 (6), 625–656. https://doi.org/10.1002/(SICI)1098-2736(199608)33:6<625::AID-TEA3>3.0.CO;2-Q Google Scholar
- Cleveland, A., Sezen-Barrie, A., & Marbach-Ad, G. ( 2021 ). The conceptualization of quantitative reasoning among introductory biology faculty . Journal of Microbiology & Biology Education , 22 (3), e00203–21. https://doi.org/10.1128/jmbe.00203-21 Medline , Google Scholar
- Conana, H., Marshall, D., & Case, J. ( 2020 ). A semantics analysis of first-year physics teaching: Developing students’ use of representations in problem-solving . In Winberg, C.McKenna, S.Wilmot, K. (Eds.), Building Knowledge in Higher Education: Enhancing Teaching and Learning with Legitimation Code Theory (pp. 162–179). New York, NY: Routledge. Google Scholar
- Conner, L. N. ( 2007 ). Cueing metacognition to improve researching and essay writing in a final year high school biology class . Research in Science Education , 37 (1), 1–16. https://doi.org/10.1007/s11165-004-3952-x Google Scholar
- Conradi, K., Jang, B. G., & McKenna, M. C. ( 2014 ). Motivation terminology in reading research: A conceptual review . Educational Psychology Review , 26 , 127–164. https://doi.org/10.1007/s10648-013-9245-z Google Scholar
- Cousin, G. ( 2006 ). An introduction to threshold concepts . Planet , 17 (1), 4–5. https://doi.org/10.11120/plan.2006.00170004 Google Scholar
- Dahlgren, M. A., Valeskog, K., Johansson, K., & Edelbring, S. ( 2021 ). Understanding clinical reasoning: A phenomenographic study with entry-level physiotherapy students . Physiotherapy Theory and Practice , 38 (13), 2817–2826. https://doi.org/10.1080/09593985.2021.1976332 Medline , Google Scholar
- Dang, N. V., Chiang, J. C., Brown, H. M., & McDonald, K. K. ( 2018 ). Curricular activities that promote metacognitive skills impact lower-performing students in an introductory biology course . Journal of Microbiology & Biology Education , 19 (1), 19.1.5. https://doi.org/10.1128/jmbe.v19i1.1324 Medline , Google Scholar
- Denton, M., & Borrego, M. ( 2021 ). Funds of knowledge in STEM education: A scoping review . Studies in Engineering Education , 1 (2), 71092. https://doi.org/10.21061/see.19 Google Scholar
- Denton, M., Borrego, M., & Boklage, A. ( 2020 ). Community cultural wealth in science, technology, engineering, and mathematics education: A systematic review . Journal of Engineering Education , 109 (3), 556–580. https://doi.org/10.1002/jee.20322 Google Scholar
- Dinsmore, D. L., Alexander, P. A., & Loughlin, S. M. ( 2008 ). Focusing the conceptual lens on metacognition, self-regulation, and self-regulated learning . Educational Psychology Review , 20 , 391–409. https://doi.org/10.1007/s10648-008-9083-6 Google Scholar
- diSessa, A. A. ( 2007 ). An interactional analysis of clinical interviewing . Cognition and Instruction , 25 (4), 523–565. https://doi.org/10.1080/07370000701632413 Google Scholar
- Dringenberg, E., & Purzer, Ş. ( 2018 ). Experiences of first-year engineering students working on ill-structured problems in teams . Journal of Engineering Education , 107 (3), 442–467. https://doi.org/10.1002/jee.20220 Google Scholar
- Dweck, C. S., & Yeager, D. S. ( 2019 ). Mindsets: A view from two eras . Perspectives on Psychological Science , 14 (3), 481–496. https://doi.org/10.1177/1745691618804166 Medline , Google Scholar
- Entwistle, N. ( 1997 ). Introduction: Phenomenography in higher education . Higher Education Research & Development , 16 (2), 127–134. https://doi.org/10.1080/0729436970160202 Google Scholar
- Entwistle, N., Hanley, M., & Hounsell, D. ( 1979 ). Identifying distinctive approaches to studying . Higher Education , 8 (4), 365–380. https://doi.org/10.1007/BF01680525 Google Scholar
- Fink, A., Cahill, J. M., McDaniel, M. A., Hoffman, A., & Frey, F. R. ( 2018 ). Improving general chemistry performance through a growth mindset intervention: Selective effects on underrepresented minorities . Chemistry Education Research and Practice , 19 (3), 783–806. https://doi.org/10.1039/C7RP00244K Google Scholar
- Fredlund, T., Airey, J., & Linder, C. ( 2015 ). Enhancing the possibilities for learning: Variation of disciplinary-relevant aspects in physics representations . European Journal of Physics , 36 (5), 055001. https://doi.org/10.1088/0143-0807/36/5/055001 Google Scholar
- Frey, R. F., Brame, C. J., Fink, A., & Lemons, P. P. ( 2022 ). Teaching discipline-based problem solving . CBE—Life Sciences Education , 21 (2), fe1. https://doi.org/10.1187/cbe.22-02-0030 Medline , Google Scholar
- Glaser, B. G. ( 1965 ). The constant comparative method of qualitative analysis . Social Problems , 12 (4), 436–445. https://doi.org/10.2307/798843 Google Scholar
- Hajar, A. ( 2021 ). Theoretical foundations of phenomenography: A critical review . Higher Education Research & Development , 40 (7), 1421–1436. https://doi.org/10.1080/07294360.2020.1833844 Google Scholar
- Halmo, S. M., Bremers, E. K., Fuller, S., & Stanton, J. D. ( 2022 ). “Oh, that makes sense”: Social metacognition in small-group problem solving . CBE—Life Sciences Education , 21 (3), ar58. https://doi.org/10.1187/cbe.22-01-0009 Medline , Google Scholar
- Han, F., & Ellis, R. A. ( 2019 ). Using phenomenography to tackle key challenges in science education . Frontiers in Psychology , 10 , 1414. https://doi.org/10.3389/fpsyg.2019.01414 Medline , Google Scholar
- Harper, K. A. ( 2006 ). Student problem-solving behaviors . The Physics Teacher , 44 (4), 250–251. https://doi.org/10.1119/1.2186244 Google Scholar
- Heaton, J. ( 2008 ). Secondary analysis of qualitative data: An overview . Historical Social Research/Historische Sozialforschung , 33 (3125), 33–45. https://doi.org/10.12759/hsr.33.2008.3.33-45 Google Scholar
- Hennessy, S. ( 1993 ). Situated cognition and cognitive apprenticeship: Implications for classroom learning . Studies in Science Education , 22 (1), 1–41. https://doi.org/10.1080/03057269308560019 Google Scholar
- Hennink, M. M., Kaiser, B. N., & Marconi, V. C. ( 2017 ). Code saturation versus meaning saturation: How many interviews are enough? Qualitative Health Research , 27 (4), 591–608. https://doi.org/10.1177/1049732316665344 Medline , Google Scholar
- Hoskinson, A.-M., Caballero, M. D., & Knight, J. K. ( 2013 ). How can we improve problem solving in undergraduate biology? Applying lessons from 30 years of physics education research . CBE—Life Sciences Education , 12 (2), 153–161. https://doi.org/10.1187/cbe.12-09-0149 Link , Google Scholar
- Hsu, J. L., Lo, S. M., & Sato, B. K. ( 2021 ). Defining understanding: Perspectives from biology instructors & biology education researchers . The American Biology Teacher , 83 (6), 372–376. https://doi.org/10.1525/abt.2021.83.6.372 Google Scholar
- Izzati, L. R., & Mahmudi, A. ( 2018 ). The influence of metacognition in mathematical problem solving . Journal of Physics: Conference Series , 1097 , 012107. https://doi.org/10.1088/1742-6596/1097/1/012107 Google Scholar
- Jacob, C. ( 2004 ). Critical thinking in the chemistry classroom and beyond . Journal of Chemical Education , 81 (8), 1216–1223. https://doi.org/10.1021/ed081p1216 Google Scholar
- Jensen, J. L., McDaniel, M. A., Woodard, S. M., & Kummer, T. A. ( 2014 ). Teaching to the test…or testing to teach: Exams requiring higher order thinking skills encourage greater conceptual understanding . Educational Psychology Review , 26 (2), 307–329. https://doi.org/10.1007/s10648-013-9248-9 Google Scholar
- Jonassen, D. H. ( 2010 ). Learning to Solve Problems: A Handbook for Designing Problem-Solving Learning Environments . New York, NY: Routledge. https://doi.org/10.4324/9780203847527 Google Scholar
- Jones, A. ( 2009 ). Redisciplining generic attributes: The disciplinary context in focus . Studies in Higher Education , 34 (1), 85–100. https://doi.org/10.1080/03075070802602018 Google Scholar
- Kirsh, D. ( 2008 ). Problem solving and situated cognition . In Robbins, P.Aydede, M. (Eds.), The Cambridge Handbook of Situated Cognition , (pp. 264–306). Cambridge, United Kingdom: Cambridge University Press. Google Scholar
- Klegeris, A., & Hurren, H. ( 2011 ). Impact of problem-based learning in a large classroom setting: Student perception and problem-solving skills . Advances in Physiology Education , 35 (4), 408–415. https://doi.org/10.1152/advan.00046.2011 Medline , Google Scholar
- Land, R., Rattray, J., & Vivian, P. ( 2014 ). Learning in the liminal space: A semiotic approach to threshold concepts . Higher Education , 67 , 199–217. https://doi.org/10.1007/s10734-013-9705-x Google Scholar
- Lave, J., & Wenger, E. ( 1991 ). Situated Learning: Legitimate Peripheral Participation . Cambridge, United Kingdom: Cambridge University Press. https://doi.org/10.1017/CBO9780511815355 Google Scholar
- Lee, K.-W. L., Goh, N.-K., Chia, L.-S., & Chin, C. ( 1996 ). Cognitive variables in problem solving in chemistry: A revisited study . Science Education , 80 (6), 691–710. https://doi.org/10.1002/(SICI)1098-237X(199611)80:6<691::AID-SCE4>3.0.CO;2-E Google Scholar
- Limeri, L. B., Carter, N. T., Choe, J., Harper, H. G., Martin, H. R., Benton, A., & Dolan, E. L. ( 2020 ). Growing a growth mindset: Characterizing how and why undergraduate students’ mindsets change . International Journal of STEM Education , 7 (1), 35. https://doi.org/10.1186/s40594-020-00227-2 Google Scholar
- Löffler, P., Pozas, M., & Kauertz, A. ( 2018 ). How do students coordinate context-based information and elements of their own knowledge? An analysis of students’ context-based problem-solving in thermodynamics . International Journal of Science Education , 40 (16), 1935–1956. https://doi.org/10.1080/09500693.2018.1514673 Google Scholar
- Lönngren, J., Ingerman, Å., & Svanström, M. ( 2017 ). Avoid, control, succumb, or balance: Engineering students’ approaches to a wicked sustainability problem . Research in Science Education , 47 (4), 805–831. https://doi.org/10.1007/s11165-016-9529-7 Google Scholar
- Luft, J. A., Jeong, S., Idsardi, R., & Gardner, G. ( 2022 ). Literature reviews, theoretical frameworks, and conceptual frameworks: An introduction for new biology education researchers . CBE—Life Sciences Education , 21 (3), rm33. https://doi.org/10.1187/cbe.21-05-0134 Medline , Google Scholar
- Martinez, M. E. ( 1998 ). What is problem solving? The Phi Delta Kappan , 79 (8), 605–609. www.jstor.org/stable/20439287 Google Scholar
- Marton, F. ( 1981 ). Phenomenography: Describing conceptions of the world around us . Instructional Science , 10 (2), 177–200. https://doi.org/10.1007/BF00132516 Google Scholar
- Marton, F. ( 1986 ). Phenomenography: A research approach to investigating different understandings of reality . Journal of Thought , 21 (3), 28–49. www.jstor.org/stable/42589189 Google Scholar
- Marton, F. ( 1988 ). Describing and improving learning . In Schmeck, R. R. (Ed.), Learning Strategies and Learning Styles (pp. 53–82). Boston, MA: Springer. Google Scholar
- Marton, F., & Booth, S. ( 1997 ). Learning and awareness . Hillsdale, NJ: Lawrence Erlbaum. https://doi.org/10.4324/9780203053690 Google Scholar
- Marton, F., & Tsui, A. B. ( 2004 ). Classroom Discourse and the Space of Learning . Hillsdale, NJ: Lawrence Erlbaum. https://doi.org/10.4324/9781410609762 Google Scholar
- McCormick, A. C., & Zhao, C.-M. ( 2005 ). Rethinking and reframing the Carnegie classification . Change: The Magazine of Higher Learning , 37 (5), 51–57. https://doi.org/10.3200/CHNG.37.5.51-57 Google Scholar
- Miller, H. B., & Srougi, M. C. ( 2021 ). Growth mindset interventions improve academic performance but not mindset in biochemistry . Biochemistry and Molecular Biology Education , 49 (5), 748–757. https://doi.org/10.1002/bmb.21556 Medline , Google Scholar
- Muenks, K., Canning, E. A., LaCosse, J., Green, D. J., Zirkel, S., Garcia, J. A., & Murphy, M. C. ( 2020 ). Does my professor think my ability can change? Students’ perceptions of their STEM professors’ mindset beliefs predict their psychological vulnerability, engagement, and performance in class . Journal of Experimental Psychology: General , 149 (11), 2119–2144. https://doi.org/10.1037/xge0000763 Medline , Google Scholar
- Muenks, K., Yan, V. X., Woodward, N. R., & Frey, S. E. ( 2021 ). Elaborative learning practices are associated with perceived faculty growth mindset in undergraduate science classrooms . Learning and Individual Differences , 92 , 102088. https://doi.org/10.1016/j.lindif.2021.102088 Google Scholar
- Murphy, S., MacDonald, A., Wang, C. A., & Danaia, L. ( 2019 ). Towards an understanding of STEM engagement: A review of the literature on motivation and academic emotions . Canadian Journal of Science, Mathematics and Technology Education , 19 , 304–320. https://doi.org/10.1007/s42330-019-00054-w Google Scholar
- Nehm, R. H. ( 2010 ). Understanding undergraduates’ problem-solving processes . Journal of Microbiology & Biology Education , 11 (2), 119–122. https://doi.org/10.1128/jmbe.v11i2.203 Medline , Google Scholar
- Piaget, J. ( 2007 ). The Child’s Conception of the World . Lanham, MD: Rowman & Littlefield. Google Scholar
- Pintrich, P. R. ( 2004 ). A conceptual framework for assessing motivation and self-regulated learning in college students . Educational Psychology Review , 16 (4), 385–407. https:/doi.org/10.1007/s10648-004-0006-x Google Scholar
- Premo, J., Cavagnetto, A., & Davis, W. B. ( 2018 ). Promoting collaborative classrooms: The impacts of interdependent cooperative learning on undergraduate interactions and achievement . CBE—Life Sciences Education , 17 (2), ar32. https://doi.org/10.1187/cbe.17-08-0176 Link , Google Scholar
- Prevost, L. B., & Lemons, P. P. ( 2016 ). Step by step: Biology undergraduates’ problem-solving procedures during multiple-choice assessment . CBE—Life Sciences Education , 15 (4), ar71. https://doi.org/10.1187/cbe.15-12-0255 Link , Google Scholar
- Price, A. M., Kim, C. J., Burkholder, E. W., Fritz, A. V., & Wieman, C. E. ( 2021 ). A detailed characterization of the expert problem-solving process in science and engineering: Guidance for teaching and assessment . CBE—Life Sciences Education , 20 (3), ar43. https://doi.org/10.1187/cbe.20-12-0276 Medline , Google Scholar
- Rattan, A., Savani, K., Komarraju, M., Morrison, M. M., Boggs, C., & Ambady, N. ( 2018 ). Meta-lay theories of scientific potential drive underrepresented students’ sense of belonging to science, technology, engineering, and mathematics (STEM) . Journal of Personality and Social Psychology , 115 (1), 54–75. https://doi.org/10.1037/pspi0000130 Medline , Google Scholar
- Rattan, A., Savani, K., Naidu, N. V. R., & Dweck, C. S. ( 2012 ). Can everyone become highly intelligent? Cultural differences in and societal consequences of beliefs about the universal potential for intelligence . Journal of Personality and Social Psychology , 103 (5), 787–803. https://doi.org/10.1037/a0029263 Medline , Google Scholar
- Reigosa, C., & Jiménez-Aleixandre, M. ( 2007 ). Scaffolded problem-solving in the physics and chemistry laboratory: Difficulties hindering students’ assumption of responsibility . International Journal of Science Education , 29 (3), 307–329. https://doi.org/10.1080/09500690600702454 Google Scholar
- Renninger, K. A., & Hidi, S. E. ( 2022 ). Interest development, self-related information processing, and practice . Theory into Practice , 61 (1), 23–34. https://doi.org/10.1080/00405841.2021.1932159 Google Scholar
- Richardson, D. S., Bledsoe, R. S., & Cortez, Z. ( 2020 ). Mindset, motivation, and teaching practice: Psychology applied to understanding teaching and learning in STEM disciplines . CBE—Life Sciences Education , 19 (3), ar46. https://doi.org/10.1187/cbe.19-11-0238 Link , Google Scholar
- Roth, W.-M., & Jornet, A. ( 2013 ). Situated cognition . WIREs Cognitive Science , 4 (5), 463–478. https://doi.org/10.1002/wcs.1242 Google Scholar
- Rowland, A. A., Knekta, E., Eddy, S., & Corwin, L. A. ( 2019 ). Defining and measuring students’ interest in biology: An analysis of the biology education literature . CBE—Life Sciences Education , 18 (3), ar34. https://doi.org/10.1187/cbe.19-02-0037 Link , Google Scholar
- Safari, Y., & Meskini, H. ( 2016 ). The effect of metacognitive instruction on problem solving skills in Iranian students of health sciences . Global Journal of Health Science , 8 (1), 150–156. https://doi.org/10.5539/gjhs.v8n1p150 Google Scholar
- Saldana, J. ( 2021 ). The Coding Manual for Qualitative Researchers 4th ed., Thousand Oaks, CA: SAGE Publications. Google Scholar
- Schiefele, U. ( 1991 ). Interest, learning, and motivation . Educational Psychologist , 26 (3-4), 299–323. https://doi.org/10.1207/s15326985ep2603&4_5 Google Scholar
- Schoenfeld, A. H. ( 1992 ). On paradigms and methods: What do you do when the ones you know don’t do what you want them to? Issues in the analysis of data in the form of videotapes . Journal of the Learning Sciences , 2 (2), 179–214. https://doi.org/10.1207/s15327809jls0202_3 Google Scholar
- Schoenfeld, A. H. ( 2016 ). Learning to think mathematically: Problem solving, metacognition, and sense making in mathematics . Journal of Education , 196 (2), 1–38. https://doi.org/10.1177/002205741619600202 Google Scholar
- Schraw, G., & Moshman, D. ( 1995 ). Metacognitive theories . Educational Psychology Review , 7 , 351–371. https://doi.org/10.1007/BF02212307 Google Scholar
- Sebasta, A. J., & Bray Speth, E. ( 2017 ). How should I study for the exam? Self-regulated learning strategies and achievement in introductory biology. CBE—Life Sciences Education , 16 (2), ar30. https://doi.org/10.1187/cbe.16-09-0269 Medline , Google Scholar
- Shin, N., Jonassen, D. H., & McGee, S. ( 2003 ). Predictors of well-structured and ill-structured problem solving in an astronomy simulation . Journal of Research in Science Teaching , 40 (1), 6–33. https://doi.org/10.1002/tea.10058 Google Scholar
- Smith, M. K., & Wood, W. B. ( 2016 ). Teaching genetics: Past, present, and future . Genetics , 204 (1), 5–10. https://doi.org/10.1534/genetics.116.187138 Medline , Google Scholar
- Smith, M. U., & Good, R. ( 1984 ). Problem solving and classical genetics: Successful versus unsuccessful performance . Journal of Research in Science Teaching , 21 (9), 895–912. https://doi.org/10.1002/tea.3660210905 Google Scholar
- Stanton, J. D., Sebasta, A. J., & Dunlosky, J. ( 2021 ). Fostering metacognition to support student learning and performance . CBE—Life Sciences Education , 20 (2), fe13. https://doi.org/10.1187/cbe.20-12-0289 Google Scholar
- Stenfors-Hayes, T., Hult, H., & Dahlgren, M. A. ( 2013 ). A phenomenographic approach to research in medical education . Medical Education , 47 (3), 261–270. https://doi.org/10.1111/medu.12101 Medline , Google Scholar
- Stewart, J., & Kirk, J. V. ( 1990 ). Understanding and problem-solving in classical genetics . International Journal of Science Education , 12 (5), 575–588. https://doi.org/10.1080/0950069900120509 Google Scholar
- Sung, R.-J., Swarat, S. L., & Lo, S. M. ( 2022 ). Doing coursework without doing biology: Undergraduate students’ non-conceptual strategies to problem solving . Journal of Biological Education , 56 (3), 271–283. https://doi.org/10.1080/00219266.2020.1785925 Google Scholar
- Swanson, H. L. ( 1990 ). Influence of metacognitive knowledge and aptitude on problem solving . Journal of Educational Psychology , 82 (2), 306. https://doi.org/10.1037/0022-0663.82.2.306 Google Scholar
- Swarat, S., Light, G., Park, E. J., & Drane, D. ( 2011 ). A typology of undergraduate students’ conceptions of size and scale: Identifying and characterizing conceptual variation . Journal of Research in Science Teaching , 48 (5), 512–533. https://doi.org/10.1002/tea.20403 Google Scholar
- Taasoobshirazi, G., & Glynn, S. M. ( 2009 ). College students solving chemistry problems: A theoretical model of expertise . Journal of Research in Science Teaching , 46 (10), 1070–1089. https://doi.org/10.1002/tea.20301 Google Scholar
- Taconis, R., Ferguson-Hessler, M., & Broekkamp, H. ( 2001 ). Teaching science problem solving: An overview of experimental work . Journal of Research in Science Teaching , 38 (4), 442–468. https://doi.org/10.1002/tea.1013 Google Scholar
- Tanner, K. D. ( 2012 ). Promoting student metacognition . CBE—Life Sciences Education , 11 (2), 113–120. https://doi.org/10.1187/cbe.12-03-0033 Link , Google Scholar
- Tanner, K., & Allen, D. ( 2005 ). Approaches to biology teaching and learning: Understanding the wrong answers and teaching toward conceptual change . Cell Biology Education , 4 (2), 112–117. https://doi.org/10.1187/cbe.05-02-0068 Link , Google Scholar
- Tight, M. ( 2016 ). Phenomenography: The development and application of an innovative research design in higher education research . International Journal of Social Research Methodology , 19 (3), 319–338. https://doi.org/10.1080/13645579.2015.1010284 Google Scholar
- Tolman, R. R. ( 1982 ). Difficulties in genetics problem solving . American Biology Teacher , 44 (9), 525–527. https://doi.org/10.2307/4447599 Google Scholar
- van Rossum, E. J., & Hamer, R. ( 2010 ). A pragmatic view on phenomenography and issues of validity and reliability . In van Rossum, E. J.Hamer, R. (Eds.), The Meaning of Learning and Knowing (pp. 33–53). Leiden, Netherlands: Brill. Google Scholar
- Veenman, M. V., Van Hout-Wolters, B. H., & Afflerbach, P. ( 2006 ). Metacognition and learning: Conceptual and methodological considerations . Metacognition and Learning , 1 , 3–14. https://doi.org/10.1007/s11409-006-6893-0 Google Scholar
- Yeager, D. S., & Dweck, C. S. ( 2020 ). What can be learned from growth mindset controversies? American Psychologist , 75 (9), 1269–1284. https://doi.org/10.1037/amp0000794 Medline , Google Scholar
- Walsh, L. N., Howard, R. G., & Bowe, B. ( 2007 ). Phenomenographic study of students’ problem-solving approaches in physics . Physical Review Special Topics-Physics Education Research , 3 (2), 020108. https://doi.org/10.1103/PhysRevSTPER.3.020108 Google Scholar
- Wang, X. ( 2013 ). Why students choose STEM majors: Motivation, high school learning, and postsecondary context of support . American Educational Research Journal , 50 (5), 1081–1121. https://doi.org/10.3102/0002831213488622 Google Scholar
- Weston, M., Haudek, K. C., Prevost, L., Urban-Lurain, M., & Merrill, J. ( 2015 ). Examining the impact of question surface features on students’ answers to constructed-response questions on photosynthesis . CBE—Life Sciences Education , 14 (2), ar19. https://doi.org/10.1187/cbe.14-07-0110 Link , Google Scholar
- Zimmerman, B. J. ( 2002 ). Becoming a self-regulated learner: An overview . Theory Into Practice , 41 (2), 64–70. https://doi.org/10.1207/s15430421tip4102_2 Google Scholar
- Zuckerman, A. L., & Lo, S. M. ( 2022 ). Examining the variations in undergraduate students’ conceptions of successful researchers: A phenomenographic study . CBE—Life Sciences Education , 21 (3), ar55. https://doi.org/10.1187/cbe.21-10-0295 Medline , Google Scholar
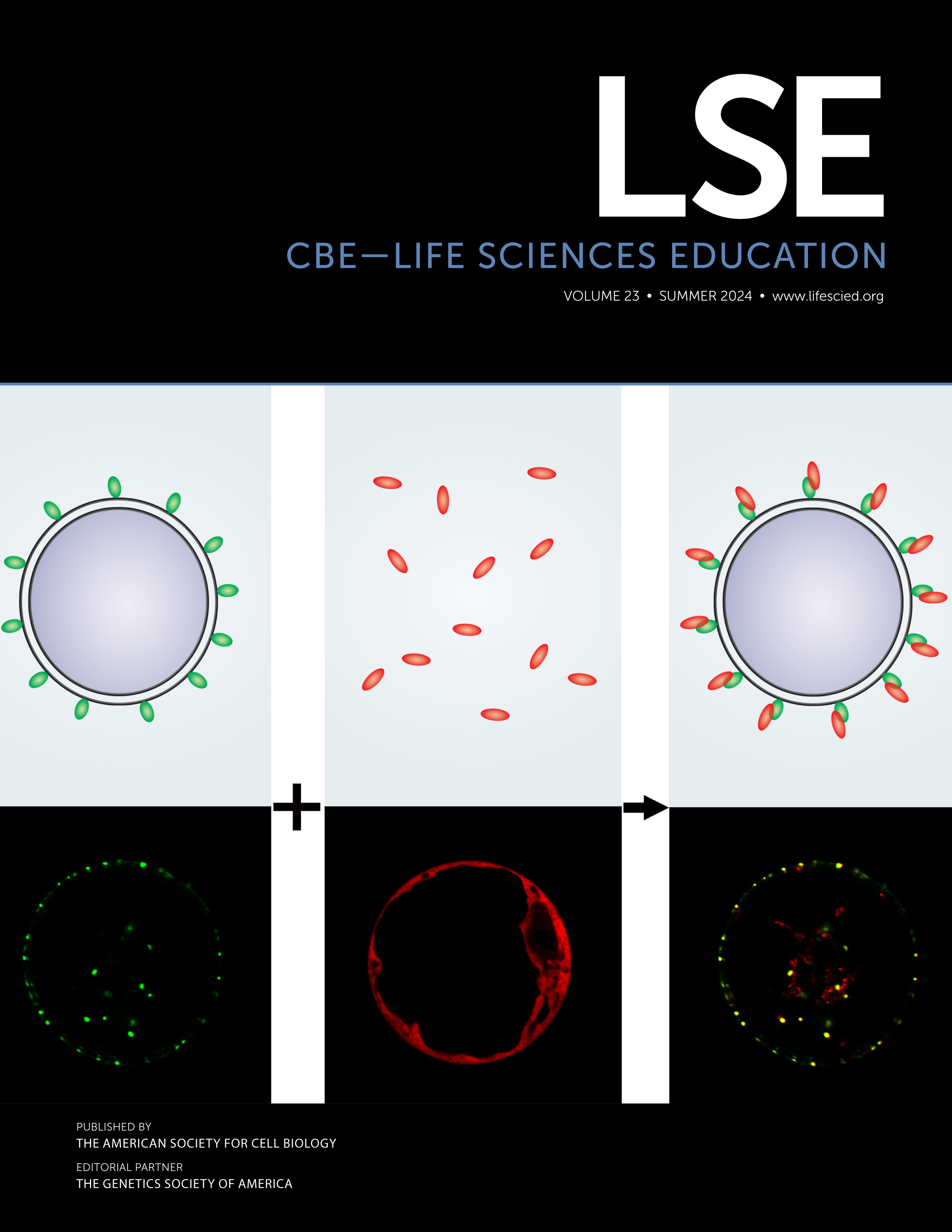
Submitted: 21 February 2023 Revised: 19 January 2024 Accepted: 9 February 2024
© 2024 J. L. Hsu et al. CBE—Life Sciences Education © 2024 The American Society for Cell Biology. This article is distributed by The American Society for Cell Biology under license from the author(s). It is available to the public under an Attribution–Noncommercial–Share Alike 3.0 Unported Creative Commons License (http://creativecommons.org/licenses/by-nc-sa/3.0).
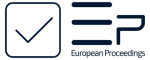
- Publishing Policies
- For Organizers/Editors
- For Authors
- For Peer Reviewers

Biology Problem-Solving: The High Achiever Students

Problem-solving has been acknowledged as one of the compulsory skills needed to face and overcome challenges of the modern world in either learning or everyday life. However, there is limited information regarding the level of problem-solving skills demonstrated by school students in learning biology subject compared to physics and mathematics. This study aims to identify the problem-solving level of 16-year old high achievers in selected boarding school in the Southern and Central Regions of Malaysia. The problem-solving skills of 70 students were measured using a validated open-ended test, UKPM, which consists of general and topic-specific problem-solving questions for biology. These questions focus on the different steps in the problem-solving processes. High achievers from boarding schools were chosen to ensure the homogenous background of the participants. The data were descriptively analysed and the overall score was used to determine the students’ problem-solving level based on the classification in Programme of International Students Assessment (PISA). The result showed that the majority of the participants are low (35%) and intermediate (64%) problem solvers and they showed incompetence in manipulating information and making justifications. They possess high tendency to find the absolute answer, but lack the reflecting ability when answering the test. The criteria and limitations showed that the participants are prone to practise a converged thinking pattern. In this, educators should introduce innovative alternative teaching and learning approach need to enhance the students’ problem-solving skills. Keywords: Problem-solving Skills Problem-solving Processes Biology School
Introduction
Problem-solving (PS) skills refer to a person’s ability to make critical judgment and decision based on the appropriate justification of the problem’s situation and its surrounding ( Kivunja, 2014 ). Solving a problem requires an individual to explore the root cause of a problem and create potential solutions pragmatically by using logic, lateral, and creative thinking ( Ismail & Atan, 2011 ). This approach is parallel with the 21st-century learning that emphasised on the construction of new knowledge, a shift from focusing solely on rote memorisation and classroom knowledge transfer in schools that have become habitual over the years. Problem-solving (PS) PS is not an innate skill ( Bal & Esen, 2016 ) therefore, providing the students with the chance to solve the problem is actually an effective way to develop this skill ( Shute, Ventura, & Ke, 2015 ; Shute & Wang, 2013 ). Learning instruction that emphasises on the understanding of core concept helps in developing students’ PS as this skill is best learnt through the use of domain-specific problem-solving activities that are challenging for students to learn ( Prevost & Lemons, 2016 ). During the process, increasing students’ understanding of the topic will help them to create and relate to the new knowledge. In the aspect of learning and education, the repetition cycle of the PS process through practices will equip them with PS skills that can be applied in different problems regardless of the context, discipline, or situation ( Yang, 2012 ). In this light, PS skills can have long-term benefits and subsequently, help the students to take charge of their profession, personal encounter and everyday hurdles ( Bal & Esen, 2016 ; Syafii & Yasin, 2013 ). Moreover, biologists agreed that students should acquire PS skills in order to learn biology better ( Hoskinson, Caballero, & Knight, 2013 ).
The numerous proposed models on PS stipulate that the basic component of the PS process is to identify problems, to suggest solutions, to apply solutions, and to reflect at the end of the process. One may interpret that the problem-solving process is the sequential steps in a linear process. However, in reality, most individuals demonstrate flexible and inventive approaches based on the different circumstance and they do not adhere to an perpetual linear PS process ( Yu, Fan, & Lin, 2014 ). PS skills are taught through the integration with the teaching of different subjects, fields, domains, or contents, the steps, process, or stages remained unchanged, therefore, understanding the meanings and function of each PS step is crucial for the success of problem-solving. PS step can be used as a guideline on what to observe and measure in evaluating the proficiency of PS. Besides that, teachers will know the types of learning support or scaffolding that have to be given to the students during the teaching and learning process.
PS requires a variety of mental skills, including interpreting information, planning, trying alternative strategies, reflecting and decision-making. However, studies have found that students are not aware of the processes taking place in problem solving ( Yu et al., 2014 ). Early PS studies demonstrated that students have difficulties in PS steps, especially when tackling the orientation stage, which is to identify the problem. In this regard, the first step of PS is vital and students should be taught for a better understanding of this component. Hence, this component should be set as the rubric or benchmark during PS measurement and assessment. Studies done in both general and domain specific PS affirmed that the students’ incapability in solving a problem does not stemmed from their lack of knowledge or skills specific domain, rather, it is due to the failure of properly identifying the source of the problem and its details. This can be seen in PS studies that compared the differences between novice and expert problem solvers where PS performance is highly influenced by individual ability to understand the problem, as well as analysing the potential answer or solution ( Prevost & Lemons, 2016 ). Nevertheless, enhancing students’ PS skills is one of the prior goals of all educational institutions therefore, developing PS skills is necessary in order to improve students’ ability in scientific thinking especially in science subjects, such as biology ( Ulusoy, Turan, Tanriverdi, & Kolayis, 2012 ; Yenice, Ozden, & Evren, 2012 ). In other words, PS skills should be developed early as in the students’ schooling years.
Previous studies have shown that Malaysia students face difficulty in problem-solving ( Abd Razak, Mohd Johar, Andriani, & Yong, 2014 ; Johnny, Abdullah, Abu, Mokhtar, & Atan, 2017 ; Kaus, Phang, Ali, Abu Samah, & Ismail, 2017 ). On the other hand, the term ‘problem-solving’ is commonly synonymous with obvious calculation and this resulted in the lack of study on problem-solving associated with Biology. In this regard, despite the differences in problems’ structure and contents between science subjects, the instructional purpose, which is to elucidate the patterns and processes in the natural world and systems, align comparatively with each other. Research noted that solving biology problem requires the engagement of the same skill practised by physicists and biologists ( Hoskinson et al., 2013 ). Nevertheless, compared to mathematics or physics there is still a prominent gap in the research of PS skills in biology for the past three decades( Kim, Prevost, & Lemons, 2015 ).
Problem Statement
The implementation of PS in pedagogical activities has led to the measurement of PS skills among the students. Studies have shown that there is a significant positive relationship between academic achievement, career success, and certain habits of mind or behaviour with skills competencies ( OECD, 2014 ; Stecher & Hamilton, 2014 ; Wüstenberg, Greiff, & Funke, 2012 ). In this light, it is more challenging to measure the competency level of PS skills compared interpersonal skills, therefore, there are guidelines that can be used in developing the instrument or selecting the rubrics to measure PS skills. On the other hand, these higher-order PS skills are arduous to be measured and the discrepancies on relevant and credible measurement scales are still debatable among the researchers ( McCoy, Braun-Monegan, Bettesworth, & Tindal, 2015 ; Stecher & Hamilton, 2014 ). By observing and measuring these PS processes, this study will obtain valuable information related to the cognitive habit in ones’ mind when solving a task. Observing and measuring these processes during intervention study will provide formative information as well as evidence of the student’s development of PS skills. There is a lack of information about problem-solving skills for biology and how students solve biology problems, among school students is still in its formative stages.
Research Questions
The research questions that lead this study are as follows:
What is the students’ problem-solving competency level for Biology?
How is the students’ performance in non-routine Biology questions in terms of the problem-solving steps in problem-solving process?
Purpose of the Study
For the purposes of this article, domain-specific problem-solving refers to topic Cell Division of secondary school biology syllabus investigate the PS level of the students. Therefore, this study aims to identify the students’ abilities regarding the steps in PS as well as their problem-solving competency level for Biology.
Research Methods
This study was participated by 70 science stream students (39 females and 31 males) who are 16 years old from three high-achieving fully residential schools located in the Central and Southern Regions of Malaysia. The students were chosen to ensure the homogenous background of the participants. Their PS skills for the biology subject were measured using the UKPM, which is a validated open-ended test with 20 topic-specific questions in Section A and 20 general questions in Section B. The topic-specific PS questions are related to cell division, while the general PS questions are related to biology or science as well as questions adapted from the problem-solving domain in Programme for International Student Assessment (PISA). This study referred to the Ge & Land PS Model that comprises four problem-solving steps, which are identifying problems (PS1), giving suggestions and options to solve the problem (PS2), making justification (PS3), and reflecting the action (PS4) ( Bixler, 2007 ). A total of 10 questions were allocated for each PS step and each question focuses on the different steps in the PS process. The maximum score for the UKPM is 120 and the data were analysed descriptively to identify the participants’ performance for each step in the PS process. The assessment rubric was adapted from previous research ( Bixler, 2007 ) while the PS classification of the competency level was done by referring to the OECD or ‘Organisation for Economic Cooperation and Development’ classification that was used in PISA ( OECD, 2014 ). The UKPM was validated prior to the research by experts in the PS domain, as well as against the biology syllabus for the Malaysian secondary school.
Table 2 summarises the participants’ scores. The results show that neither the female nor male participants score excellently in the UKPM test. The mean score for female participants was 42.92, and the difference is not distinct with the male participants with the mean score of 42.42. The overall achievement did not reach 50% of the overall score with only 42.70. The dispersion of the score patterns for all groups is almost similar, with the average of 8. The highest score is 62/120, while the lowest is 26/120. Both extreme scores were obtained by female participants.
Each individual score was compared and classified according to the six PS competency level as presented by Organisation for Economic Co-operation and Development (2014). Diagram 1 shows the percentage of the number of participants in each competency level; only 1% of the participants could be classified as possessing level 4 competencies. The majority of the participants (64%) could be categorised as level 3 problem solvers, while 35% could be classified as having level 2 competencies.
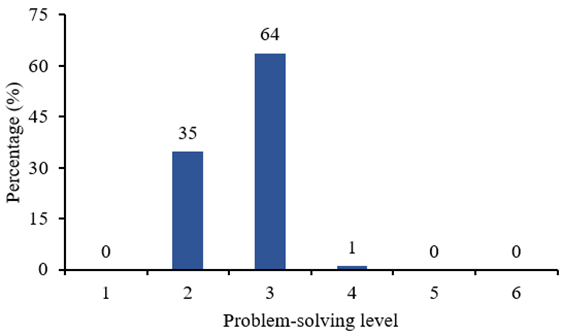
The level of problem-skill for each gender was compared against and there are only minor differences. Diagram 2 shows that 2% of the female participants possess level 4 competence. Moreover, there is a 4% difference between the number of females (36%) and males (32%) who demonstrated level 2 competence. The same difference was observed in level 3 and there is only a 6% difference between both groups.
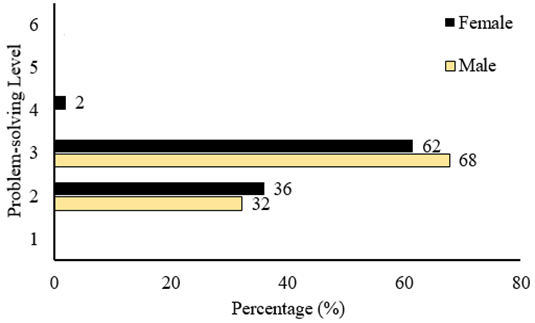
Table 3 describes the score of each PS step in details. Out of the four steps in the PS process, making reflection (PS4) has the lowest mean score of 9.33 ± 3.90 for females and 9.39 ± 3.35 for males. The mean scores show that most participants are capable to obtain at least 10 out of the total 40 marks allocated for PS4 in UKPM. Although the other three steps have higher mean scores, they are still considered to be in the low range as none of the PS steps are able to reach at least 50% of the mean score compared to the allocated marks. The minimum and maximum scores for each PS step are in the lower range as the highest score is 21 (PS4) and the lowest score is 3 (PS2). Diagram 3 summarises the findings related to the PS steps. In this light, there are no major differences in the overall achievement each PS steps between each gender.
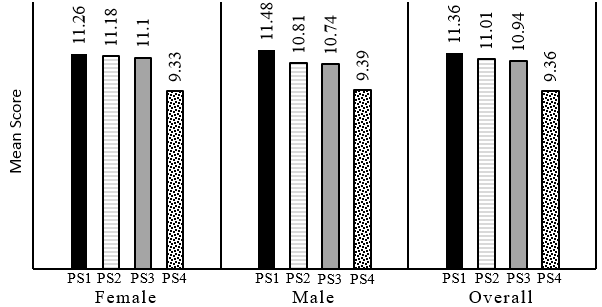
The results provide the insights on students’ behaviour when solving problems during the biology subject. The biology subject is different from physics and mathematics; this is because, the calculations only play minimal roles compared to reading and understanding the fact. It was discovered that the participants from both genders have poor knowledge and capabilities in all the PS steps, and consequently, they obtained poor results in the PS domains based on the Programme International Student Assessment (PISA).
It was discovered that the participants did not plan well and did not evaluate the situation in the questions. In one of the PS1 questions, the participants were asked to list all the barriers and factors that they should consider before choosing the most appropriate option and only a small percentage of students managed to list the appropriate answers beyond the question given, while the rest only listed down a few factors that could be found in the question. In another PS1 question, the participants were required to propose an arrangement plan regarding the number of people to be placed in eight rooms. For this question, the participants should consider the criteria given when proposing the arrangement. The researcher expected the participants to perform some calculations, however, the majority of them presented wrong answers even though a draft table was provided to assist them in planning and evaluating the problem by providing specific directions for the key stages. They only provided answers that they are familiar despite the expectation that they would be able to find the solution when they delve deeper into the question. This shows excellent achievement in public school examination will not ensure good competency in non-routine PS as most school examination revolves around routine problem (Abd Razak et al., 2014)
In the meantime, the planning process is seldom practised in answering open-ended problems even though it is commonly used in routine and algorithmic problems. Hence, it should be considered in developing the skills to solve open-ended problems ( Reid & Yang, 2002 ). In this light, most past PS studies only focused on the earlier PS steps, which are identifying the root cause of the problem and planning the solutions and actual success in PS is actually determined based on the capability to determine what is needed to be solved and how to do it effectively ( Ulusoy et al., 2012 ).
Identifying the root cause of the problem and planning the solutions are categorised as knowledge acquisition according to the PS framework in PISA ( OECD, 2014 ) or rule identification and application ( Schweizer, Wüstenberg, & Greiff, 2013 ; Wüstenberg et al., 2012 ). It is assumed that these particular steps are more utilised in higher-order thinking skills (HOTS) compared to the later steps in PS model. Meanwhile, reflections and monitoring require judgement and deep thinking and reflections can be done in most of the PS steps. Due to the huge influence in PS stages, some studies have divided the ‘Identify Problem’ and ‘Solution Planning’ steps into smaller sub-steps (E.g., ‘Gather Info’). Some studies also added other indicators that are relative to these steps (E.g., ‘Avoiding Problem’ and ‘Flexibility’) with a specific checklist of criteria that has to be observed in the study. These additions were based on the researchers’ perspectives and research needs.
The results revealed that the students are confused and facing difficulties in linking the function of spindle fibre with mitosis or meiosis failure (Figure 4 ). The participants’ lack of understanding of the key concepts has contributed to the poor results for the PS steps. As an example, the question related to the concepts of meiosis and its functions in producing haploid gamete cell which are different in terms of numbers of chromosomes, genetic content due to random desegregation, and crossing over processes. In this light, the students were unable to answer the question even though it is just slightly different from the examination format questions (Figure 5 ). This shows that the students were confused and the students had come out with varied segments of inaccurate response and totally incorrect answers. On the other hand, the success rate was improved when the same question was modified with additional explicit hints or organised to be similar to the pattern of the examination format questions. Without this explicit linkage, the participants had difficulties in linking what they had learnt on the idea of cell division with examination questions. It seems that solving previous exam questions and drill practices are common in a biology lesson so that the students could ace their examination. In this light, despite their impressive results, the students tend to have limitations in terms of their level of thinking skills. These students tend to answer the questions through memorisation, and the drilling practices create a mind model and schema that will be stored in their minds, rather than creating understanding of the principles. In other words, the students memorise the content and they face difficulties when presented with questions in a new context or structure.
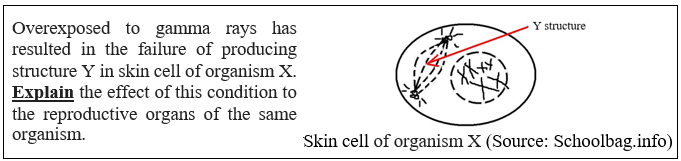
Step four in the PS process is making reflection. In UKPM, questions in PS4 prompt the participants to present their agreement on the topic (Figure 6 ). For example, the students are provided with a formula as a guide for their answers and to answer PS4 questions, students are expected to review and identify the formula and they have to suggest the correct formula when giving their justifications. Unfortunately, there were not many participants who were able to present a sound reflection. Most of them only provide their answers by referring to the given calculation without reflecting and they also provided incorrect answers. As a result, they scored very low for PS4 which affected their overall score. This shows that the learners have low abilities and face difficulties in creating a link between skills and knowledge ( Reid & Yang, 2002 ). Moreover, this study also observed the habit and pattern related to how the participants answer the test. Besides that, at the end of the UKPM test, the researcher had obtained verbal feedbacks from students who seemed to not prefer lengthy questions as they only glanced through the instruction and provide answers without any description, explanation, or justification. It was found that these students are more familiar with routine questions, which only require one right answer and they looked uncomfortable when asked to answer non-routine abstract questions that require giving opinions and justifications and consequently, gave opinions that did not reflect the lesson that they had learnt.
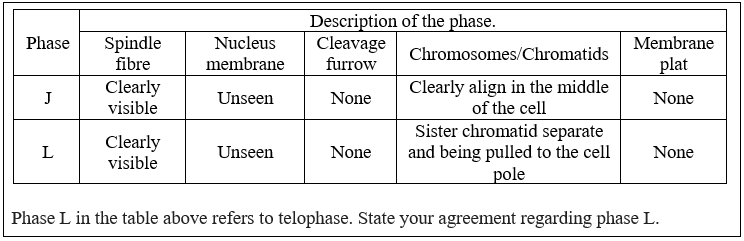
In the meantime, a good problem solver has three characteristics, which are having a good conceptual understanding of the domain involved, including domain-specific skills and being able to adjust wisely to the use of automated skills. This is because PS requires two types of knowledge namely declarative and procedural knowledge that are interdependent during the PS activity ( Yu et al., 2014 ). Expert problem solvers are more mature when performing an integrated mental representation of the problem, as well as demonstrating a better understanding of the core concepts, nature, and form of the problem ( Prevost & Lemons, 2016 ). They need more time to define and understand the issues compared to novice problem solvers who prefer to complete the task impatiently and often ignore the PS steps, including the first and most important step, which is problem identification ( Yu et al., 2014 ). The same pattern could also be observed among primary, secondary, and college students. Novice problem solvers usually seek the solution without the definite understanding of the problem and they lacked the ability to reflect their own performances. They tend to overlook the analysis and reflection process, even though they knew that they were stuck with an inappropriate solution during the process.
The flexible, linear, and sequential PS processes can be practised differently according to the problem solvers’ creativity and needs, as well as the situation. However, the students’ lack of understanding on these PS processes will affect their perceptions on the processes’ progression and nature. Furthermore, research ( Yu et al., 2014 ) reported a similar pattern in the early phase of their study. They found that students lacked the flexibility and creativity as they opted for the linear mode of an incomplete PS process. Therefore, it is important to incorporate effective teaching strategies to enhance the students' understanding on the meaning and function of each PS step, and the students can develop individual skills when solving problems. A good problem solver that practises effective monitoring step would consistently reflect the chosen strategy to ensure that they are on the right track, as well as checking for other solutions. The students should be able to monitor and steer the direction of their own progress, to ask questions among themselves that could help to maximise the effective strategies and to prevent themselves from constantly using the unproductive approach in generating solutions (Jamari, Mohamed, Abdullah, Mohd Zaid, & Aris, 2017b). Choosing an effective strategy without making revisions or having self-correcting mechanisms to monitor the progress of PS is comparable to those who fail to choose good method and strategy right from the start. This issue can contribute to the failure in PS. Therefore, students must be encouraged to make verification by monitoring and reflecting their choice that could increase their HOTS and PS level.
Previous studies suggested that instructional scaffolding is necessary in aiding the students’ problem-solving processes (Jamari, Mohd Zaid, Abdullah, Mohamed, & Aris, 2017a; Kim et al., 2015 ). In this light, it is important to focus on content specific scaffolding, which is also known as conceptual scaffolding in school. This is because mastering the content of the lesson is the ultimate goal of having learning assistance, either with or without instructional materials or the presence of a teacher. Therefore, the teacher is responsible to help students in understanding the function of each step involved in the PS in class regardless of subject or domain ( Yenice et al., 2012 ). The action of mentioning these processes during the teaching and learning process without giving the students with the opportunity to perform activities that require them to think, learn and practise each step will not enhance the students’ PS skills ( Yu et al., 2014 ). The similarities between ill-structured task and common everyday problems make it worthwhile to inculcate and develop the students’ PS skills. PS skills helps to cater the needs of solving multiple tasks in a short term which refers to schooling and learning and at the same time shaping an individual to be a capable problem solver later in life as a long term goal. Since an ill-structured task usually has complex structure and may have numerous potential solutions, this type of task requires more cognitive activity to process all the problems’ information in the attempt to find the best solution.
Promising instructional strategies to enhance HOTS and PS have been widely studied and including the inquiry learning approach and focused on STEM education (Jamari et al., 2017b). However, there are still not much studies being done on these approaches for the Malaysian context although there are plenty of studies done in other countries. Examples of teaching strategies that emphasised on authentic ill-structured problem that can be applied by teachers include case-based learning (CBL) and problem-based learning (PBL). The problem or task does not stem from the textbook, but from the everyday problems which require the application of the similar concepts or principles. CBL and PBL are categorised under inquiry and they are suitable to be used in the environment of science learning due to their potential to attract the interest of students, to spark inquiry, and to encourage them to continue exploring the task ( Herreid, Schiller, & Herreid, 2012 ; Pai et al., 2010 ). Teacher’s face to face or online involvement provides the suitable guidance to help them interpret and accelerate active information transfer processes by providing a learning environment that can develop HOTS and PS skills ( Kivunja, 2014 ; McCoy et al., 2015 ).
Although time is a factor that can affect the development of PS in an individual, it is important to expose the students to PS steps and processes so that they can learn and practise these skills to become a competent citizen. Therefore, teaching approaches and strategies that emphasise on authentic problem and active learning such as Inquiry Learning and STEM (Science, Technology, Engineering and Mathematics) education should be combined with appropriate instructional scaffolding that focuses on the students’ ability to master the lesson, as well as nurturing and developing their PS skills ( Bybee, 2010 ; Moore, Johnson, Peters-Burton, & Guzey, 2016 ; Tseng, Chang, Lai, & Chen, 2013 ). In the meantime, this study has several limitations. One of the limitations is the small number of participants as the study was focused on high achievers. Hence, the sample for this study may not represent the whole population. Nevertheless, the sample provides insights on how high achieving students conduct PS. This information will add to the body of knowledge on problem solving in the context of Malaysian school students. It is assumed that other students are facing the same PS problems as shown by the high achievers, therefore, future research can be implemented on students from different categories and backgrounds. A PS research with more focus on the biology subject can be conducted by replicating this research to other topics in the biology syllabus..
- Abd Razak, N. N. F., Mohd Johar, A., Andriani, D., & Yong, C. Y. (2014). Mathematical Problem Solving Ability Among Form Two Students. Jurnal Pendidikan Matematik, 2(2), 1-13.
- Belgin Bal, İ., & Esen, E. (2016). Problem Solving Skills of Secondary School Students. China-USA Business Review, 15(6). doi:10.17265/1537-1514/2016.06.002
- Bixler, B. A. (2007). The Effects of Scaffolding Students' Problem-Solving Pocess Via Question Prompts on Problem Solving and Intrinsic Motivation In An Online Learning Environment. (Doctor of Philosophy phd thesis), Pennsylvania State University,
- Bybee, R. W. (2010). Advancing STEM Education A 2020 Vision. Technology And Engineering Teacher, 70(1), 30-35.
- Herreid, C. F., Schiller, N. A., & Herreid, K. F. (2012). Science Stories: Using Case Studies to Teach Critical Thinking. VA, USA: National Science Teachers Association.
- Hoskinson, A. M., Caballero, M. D., & Knight, J. K. (2013). How can we improve problem solving in undergraduate biology? Applying lessons from 30 years of physics education research. CBE Life Sci Educ, 12(2), 153-161. doi:10.1187/cbe.12-09-0149
- Ismail, S., & Atan, A. (2011). Aplikasi Pendekatan Penyelesaian Masalah Dalam pengajaran Mata Pelajaran Teknikal dan Vokasional di Fakulti Pendidikan UTM. Journal of Educational Psychology and Counseling, 2, 113-144.
- Jamari, D., Mohamed, H., Abdullah, Z., Mohd Zaid, N., & Aris, B. (2017b). Fostering Higher Order Thinking And Problem Solving Skills Through Social Media. Man In India, 97(12), 1-10.
- Jamari, D., Mohd Zaid, N., Abdullah, Z., Mohamed, H., & Aris, B. (2017a). Instructional Scaffolding To Support Ill-Structured Problem Solving A Review. Sains Humanika, 9(1-4), 33-39.
- Johnny, J., Abdullah, A. H., Abu, M. S., Mokhtar, M., & Atan, N. A. (2017). Difficulties In Reasoning Among High Achievers When Doing Problem Solving In Mathematics. Man In India, 97(12), 61-70.
- Kaus, M. A., Phang, F. A., Ali, M. B., Abu Samah, N., & Ismail, A. K. (2017). Problem Solving And Social Supports: The Roles of Parents. Man In India, 97(12), 279-287.
- Kim, H. S., Prevost, L., & Lemons, P. P. (2015). Students' usability evaluation of a Web-based tutorial program for college biology problem solving. Journal of Computer Assisted Learning, 31(4), 362-377. doi:10.1111/jcal.12102
- Kivunja, C. (2014). Do You Want Your Students to Be Job-Ready with 21st Century Skills? Change Pedagogies: A Pedagogical Paradigm Shift from Vygotskyian Social Constructivism to Critical Thinking, Problem Solving and Siemens’ Digital Connectivism. International Journal of Higher Education, 3(3). doi:10.5430/ijhe.v3n3p81
- McCoy, J. D., Braun-Monegan, J., Bettesworth, L., & Tindal, G. (2015). Do Scaffolded Supports between Aspects of Problem Solving Enhance Assessment Usability? Journal of Education and Practice, 6(36), 175-185.
- Moore, T., Johnson, C. C., Peters-Burton, E. E., & Guzey, S. S. (2016). The need for a STEM road map: A framework for integrated STEM education. In (pp. 33 -12). NY: Routledge Taylor & Francis Froup.
- OECD. (2014). PISA 2012 Results: Creative Problem Solving Students’ skills in tackling real-life problems Volume V. Retrieved from La rue André-Pascal, PARIS
- Pai, A., Benning, T., Woods, N., McGinnis, G., Chu, J., Netherton, J., & Bauerle, C. (2010). The Effectiveness of a Case Study-Based First-Year Biology Class at a Black Women's College. Journal of College Science Teaching, 40(2), 32.
- Prevost, L. B., & Lemons, P. P. (2016). Step by Step: Biology Undergraduates' Problem-Solving Procedures during Multiple-Choice Assessment. CBE Life Sci Educ, 15(4). doi:10.1187/cbe.15-12-0255
- Reid, N., & Yang, M.-J. (2002). Open-ended problem solving in school chemistry: A preliminary investigation. International Journal of Science Education, 24(12), 1313-1332. doi:10.1080/09500690210163189
- Schweizer, F., Wüstenberg, S., & Greiff, S. (2013). Validity of the MicroDYN approach: Complex problem solving predicts school grades beyond working memory capacity. Learning and Individual Differences, 24, 42-52. doi:10.1016/j.lindif.2012.12.011
- Shute, V. J., Ventura, M., & Ke, F. (2015). The power of play: The effects of Portal 2 and Lumosity on cognitive and noncognitive skills. Computers & Education, 80, 58-67. doi:10.1016/j.compedu.2014.08.013
- Shute, V. J., & Wang, L. (2013). Measuring Peoblem Solving Skills In Portal 2. In IADIS International Conference on Cognition and Exploratory Learning in Digital Age (CELDA 2013) (pp. 33-39). Fort Worth, Texas, USA: International Assn for Development of the Information Society (IADIS).
- Stecher, B. M., & Hamilton, L. S. (2014). Measuring Hard-to-Measure Student Competencies A Research and Development Plan (13 978-0-8330-8806-2). Retrieved from Santa Monica, California:
- Syafii, W., & Yasin, R. M. (2013). Problem Solving Skills and Learning Achievements through Problem-Based Module in teaching and learning Biology in High School. Asian Social Science, 9(12). doi:10.5539/ass.v9n12p220
- Tseng, K. H., Chang, C. H., Lai, S. J., & Chen, W. P. (2013). Attitudes towards science, technology, engineering and mathematics (STEM) in a project-based learning (PjBL) environment. International Journal of Technology Design Education, 23, 87-102.
- Ulusoy, Y. O., Turan, H., Tanriverdi, B., & Kolayis, H. (2012). Comparison of Perceived Problem Solving Skills of Trainee Students Graduated from Different. Procedia - Social and Behavioral Sciences, 46, 2099-2103. doi:10.1016/j.sbspro.2012.05.435
- Wüstenberg, S., Greiff, S., & Funke, J. (2012). Complex problem solving — More than reasoning? Intelligence, 40(1), 1-14. doi:10.1016/j.intell.2011.11.003
- Yang, Y. T. C. (2012). Building virtual cities, inspiring intelligent citizens: Digital games for developing students’ problem solving and learning motivation. Computers & Education, 59(2), 365-377. doi:10.1016/j.compedu.2012.01.012
- Yenice, N., Ozden, B., & Evren, B. (2012). Examining of Problem Solving Skills According to Different Variables for Science Teachers Candidates. Procedia - Social and Behavioral Sciences, 46, 3880-3884. doi:10.1016/j.sbspro.2012.06.165
- Yu, K.-C., Fan, S.-C., & Lin, K.-Y. (2014). Enhancing Students’ Problem-Solving Skills through Context-Based Learning. International Journal of Science and Mathematics Education, 13(6), 1377-1401. doi:10.1007/s10763-014-9567-4
Copyright information

About this article
Publication date.
01 May 2018
Article Doi
https://doi.org/10.15405/epsbs.2018.05.68
978-1-80296-039-6
Future Academy
Print ISBN (optional)
Edition number.
1st Edition
Business, innovation, sustainability, environment, green business, environmental issues
Cite this article as:
Jamari, D., Mohamed, H., Abdullah, Z., Zaid, N. M., & Aris, B. (2018). Biology Problem-Solving: The High Achiever Students. In M. Imran Qureshi (Ed.), Technology & Society: A Multidisciplinary Pathway for Sustainable Development, vol 40. European Proceedings of Social and Behavioural Sciences (pp. 831-842). Future Academy. https://doi.org/10.15405/epsbs.2018.05.68
We care about your privacy
We use cookies or similar technologies to access personal data, including page visits and your IP address. We use this information about you, your devices and your online interactions with us to provide, analyse and improve our services. This may include personalising content or advertising for you. You can find out more in our privacy policy and cookie policy and manage the choices available to you at any time by going to ‘Privacy settings’ at the bottom of any page.
Manage My Preferences
You have control over your personal data. For more detailed information about your personal data, please see our Privacy Policy and Cookie Policy .
These cookies are essential in order to enable you to move around the site and use its features, such as accessing secure areas of the site. Without these cookies, services you have asked for cannot be provided.
Third-party advertising and social media cookies are used to (1) deliver advertisements more relevant to you and your interests; (2) limit the number of times you see an advertisement; (3) help measure the effectiveness of the advertising campaign; and (4) understand people’s behavior after they view an advertisement. They remember that you have visited a site and quite often they will be linked to site functionality provided by the other organization. This may impact the content and messages you see on other websites you visit.
SUNY ESF Problem Solving

Problem-Solving in Conservation Biology and Wildlife Management:
Exercises for Class, Field, and Laboratory (2nd Edition)
Supporting materials (data sets, images, maps, and important websites)
Note: components of first edition website are still available: Guidance and resources document and the Java applets: These three – habitat loss and fragmentation, dispersal, and PVA - are still very useful!
To receive an electronic copy of the instructor’s manual please send a message to James Gibbs at [email protected] indicating (1) your institution and position, (2) the course name and number, and (3) number of students in your class. With this information we will arrange a web download or email transmission of the manual.
SECOND EDITION
Part 1. Introduction
1. What Is Biodiversity? Spiders as Exemplars of the Biodiversity Concept
Higher resolution images of spider collections to print and work with:
Site 1 , Site 2 , Site 3 , Site 4 , Site 5 (.tif) (right click to “save as…”)
Spider phylogram (.tif) (right click to “save as…”)
Erratum: P. 8, 1st para, line 3, values of “2” should be superscripted, e.g., 1/((0.5)2+ (0.5)2) = 1/0.5 or 2
2. What Is Conservation Biology? An Analysis of the Critical Ecosystem Partnership Fund’s Strategies and Funding Priorities
Critical Ecosystem Partnership Fund
3. Why Is Biodiversity Important? Why Is It Threatened? An Exploration with the IUCN “Red List” of Threatened Species
IUCN “Red List” of Threatened Species
Part 2. Genes
4. Population Genetics: Diversity Within Versus Among Populations
[No additional resources required]
5. Genetic Drift: Establishing Population Management Targets to Limit Loss of Genetic Diversity
6. Pedigree Management: Controlling the Effects of Inbreeding as Indicated by Fluctuating Asymmetry
Bat faces to print and work with: first set & second set (.tif) (right click to “save as…”)
7. Landscape Genetics: Identifying Movement Corridors in the Landscape
Higher resolution version of map used in exercise (.tif) to work with (right click to “save as…”)
Part 3. Populations
8. Life Table Analysis: Balancing Commercial Fisheries with Sea Bird “By-Catch”
Longevity data (.txt) (right click to “save as…”)
Fecundity data (.txt) (right click to “save as…”)
Erratum: Table 8.4 values are nx, not mx as printed 9. Population Viability Analysis: El Niño Frequency and Penguin Population Persistence
[No supporting materials required]
Erratum/clarification: Page 67: the average penguin population growth rate should be 1.188 or 18% (consistent with Fig. 9.3) not the 88% nor the 1.88 factor listed elsewhere.
Also: p 70: 2/3 way down right side, “C47” should be “C7”
10. Habitat Loss and Fragmentation: Ecological Traps, Connectivity and Issues of Scale
Maps with small-scale fragmentation to print and work with: set 1 & 2 (.tif) (right click to “save as…”)
Maps with large-scale fragmentation to print and work with: set 1 & 2 (.tif) (right click to “save as…”)
An important note from an astute book user on the weighting procedure
11. Diagnosing Declining Populations: Assessing Monitoring Data to Better Understand Causes of a Rarity in an Endangered Cactus
Monitoring data (.xls) (right click to “save as…”)
Ecological data (.xls) (right click to “save as…”)
12. Estimating Population Size with Line Transects and DISTANCE
Program DISTANCE
Program DISTANCE input files (.zip) (right click to “save as…”)
13. Analyzing Camera Trap Data with PRESENCE
Latest version of Program PRESENCE (Version 2.2)
Version of Program PRESENCE used to develop exercise (Version 2.1)
Program PRESENCE input files (.zip) (right click to “save as…”)
Updated Procedure section revised for Exercise 13 to correspond to PRESENCE 2.1 (.pdf) (right click to “save as…”)
14. Estimating Population Size with Mark-Recapture Data and MARK
Program MARK
Program MARK input files (.zip) (right click to “save as…”)
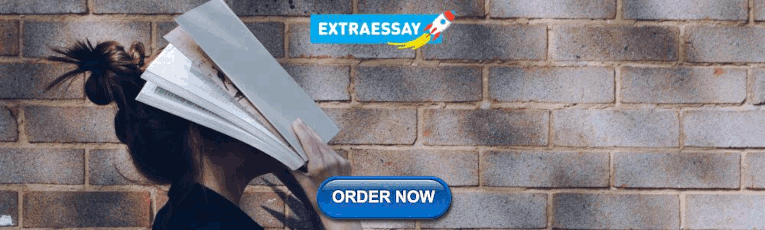
Part 4. Species
15. Estimating "Biodiversity": Indices, Effort and Inference
Program ESTIMATES
Program ESTIMATES input files (.zip) (right click to “save as…”)
16. Designing a Zoo: Ex-Situ Centers for Conservation, Research, and Education
Full list of species and supporting information (.xls) (right click to “save as…”)
17. Plant Reintroductions: Reestablishing Extirpated Populations
18. Edge Effects: Designing a Nest Predation Experiment
Part 5. Ecosystems and Landscapes
19. Ecosystem Fragmentation: Patterns and Consequences for Biodiversity
Base landscape map for scenario building (.tif) (right click to “save as…”)
20. Forest Harvesting: Balancing Timber Production and Parrot Habitat
21. Protected Areas: A Systematic Conservation Planning Approach for Ecoregions
puspec.txt (right click to “save as…”)
puinfo.txt (right click to “save as…”)
22. Island Biogeography: How Park Size and Condition Affect the Number of Species Protected
Bird and plant species list by protected area in the US (.xls file) (right click to “save as…”)
23. GIS For Conservation: Mapping and Analyzing Distributions of Wild Potato Species for Reserve Design
DIVA-GIS program
Datasets (.zip) (right click to “save as…”)
24. Global Change: Will a Cold-Adapted Frog Survive in a Warmer World?
Map of the current range of mink frogs in NY state (.tif) (right click to “save as…”)
Map of the temperature in July in NY state (.tif) (right click to “save as…”)
Map of annual precipitation in NY state (.tif) (right click to “save as…”)
ArcExplorer9.2
WinRAR archiver
Arc data set (right click to “save as…”) (note: modified Dec 1 2011 to accommodate Arc software updates).
DIVA-GIS dataset (right click to “save as…”)
Excel climate dataset (right click to “save as…”)
25. Climate Envelope Modeling: Inferring the Ranges of Species
DIVA-GIS program
Datasets (.zip) (right click to “save as…”)
Part 6. Policy and Organizations
26. Population, Consumption, Or Governance?: Which Drives Species Imperilment Most in Africa and Europe?
Bird species, population, consumption, and governance data set (.xls file) (right click to “save as…”)
28. Overconsumption: Who’s Smarter... Students or Their Professors? [No supporting materials required]
28. Conservation Values: Assessing Public Attitudes
[No supporting materials required]
29. Priority Setting: Where Around the Globe Should We Invest Our Conservation Efforts?
CI hotspots and associated data (.xls file) (right click to “save as…”)
30. An International Debate: Commercial Fishing in Galápagos National Park
GALAPAGOS ISLANDS (ECUADOR) REPORT OF THE REACTIVE MONITORING MISSION 8 TO 13 APRIL 2007
REPORT
http://www.galapagos.org/
http://www.gct.org/
http://www.darwinfoundation.org/
http://www.galapagospark.org/png/index.php
http://www.igtoa.org/
31. Conservation Law: Should the Polar Bear Be Listed as a Threatened Species?
Proposed Rule to List the Polar Bear (Ursus maritimus) (.pdf file)
Range-Wide Polar Bear (Ursus maritimus) Status Review (.pdf file)
32. Conservation Policy: Shaping Your Government
National 5 Biology Revision
Welcome to your interactive National 5 Biology Revision site. Here you will find past papers and marking instructions, summary notes, problem solving activities and much more.
Each section has a link to BBC Bitesize, a selection of past paper questions and a youtube video.
Course Content
National 5 Biology consists of 3 units of work: Cell Biology, Multicellular Organisms and Life on Earth.
Cell Structure
Transport across the cell membrane
Proteins and Enzymes
Genetic engineering, respiration.
Producing new cells
Control and communication
Reproduction
Variation and inheritance, transport in plants, transport in animals, absorption of materials.
Photosynthesis
Food production.
Evolution of Species
Cells are the most basic units of life. There are a few cell types you need to be able to identify and state the functions of their organelles. Below you will find links to BBC bitesize and a google document with past paper questions.

Transport Across the Cell Membrane
All cells are enclosed by a cell membrane, which is selectively permeable. Molecules can move into or out of cells by diffusion and active transport. Cells can gain or lose water by osmosis.
DNA carries the genetic information in the cells of all living organisms. It contains codes for the assembly of amino acids into all the proteins required in the body.
Proteins consist of combinations of amino acids. Body proteins include structural proteins, enzymes, hormones and antibodies. The shape of an enzyme allows it to speed up a biological reaction.
Bacteria exchange DNA using plasmids; viruses invade cells by first inserting their genetic material. Genetic engineering is the transfer of DNA between organisms using biotechnology.
Most cell activity requires chemical energy. Respiration is the cellular process of releasing energy from food and storing it as ATP.
Producing New Cells
Cell division allows for growth and replacement of dead cells. Most multicellular organisms are made of different cell types that are specialised to carry out specific functions.
Control and Communication
Communication between cells in a multicellular organism occurs by use of nerve impulses or hormones. The central nervous system produces electrical impulses for rapid response.
New organisms are produced when male and female haploid gametes fuse. In mammals, gametes are produced in the testes or ovaries of individuals but anthers and ovaries are on the same flowering plant.
The phenotype for a characteristic like eye colour is the result of the combination of alleles. If the alleles in the parental genotype are dominant or recessive, probable outcomes can be predicted.
Multicellular organisms require transport systems to supply their cells and remove waste products. Plants transport substances through xylem and phloem.
Multicellular organisms require transport systems to supply their cells and remove waste products. The heart uses blood vessels to transport these substances around the body.
Humans require transport systems to supply their cells and remove waste products. Gas exchange occurs in the lungs and food molecules are absorbed by the digestive system.
An ecosystem is a community of animals, plants, micro-organisms, non-living things and their shared environment. Find out about energy transfer, niches and competition in ecosystems.
Abiotic factors are non-living variables. Biotic factors are the interactions between organisms. Both affect diversity and distribution. Sampling helps us to estimate numbers of organisms in an area.
Photosynthesis is the process by which green plants use sunlight energy to make their own food. It takes place inside the chloroplasts of plant cells.
All organisms require energy. The feeding relationship in an ecosystem can be shown in a foodchain. Learn about pyramids of biomass, energy and numbers.
A growing human population demands increased food production. Farmers try to meet that demand by intensive farming, using fertilisers and pesticides that are impacting on the environment.
Mutations can alter the genes of individuals in a beneficial or detrimental way and introduce variety into a species.
Problem Solving
Below you will find examples of scientific literacy questions, problem solving and extended response questions.

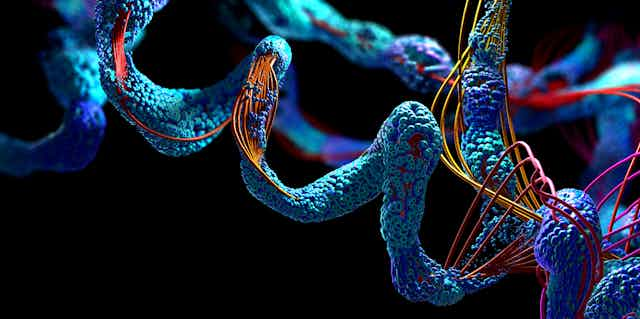
AI system can predict the structures of life’s molecules with stunning accuracy – helping to solve one of biology’s biggest problems
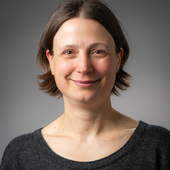
Senior Lecturer in Drug Discovery, Department of Life Sciences, University of Bath
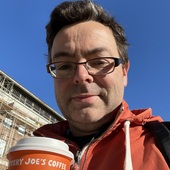
Professor of Molecular Medicine, School of Molecular and Cellular Biology, University of Leeds
Disclosure statement
The authors do not work for, consult, own shares in or receive funding from any company or organisation that would benefit from this article, and have disclosed no relevant affiliations beyond their academic appointment.
University of Leeds provides funding as a founding partner of The Conversation UK.
University of Bath provides funding as a member of The Conversation UK.
View all partners
AlphaFold 3, unveiled to the world on May 9 , is the latest version of an algorithm designed to predict the structures of proteins – vital molecules used by all life – from the “instruction code” in their building blocks.
Predicting protein structures and the way they interact with other molecules has been one of the biggest problems in biology. Yet, AI developer Google DeepMind has gone some way to solving it in the last few years. This new version of the AI system features improved function and accuracy over its predecessors.
Like the next release in a video-game franchise, structural biologists – and most recently – chemists have been waiting with impatience to see what it can do. DNA is widely understood as the instruction book for a living organism but, inside our cells, proteins are the molecules that actually carry out most of the work.
It is proteins that enable our cells to sense the world outside, to integrate information from different signals, to make new molecules within the cell, to decide to grow or to stop growing.
It is also proteins that enable the body to distinguish between foreign invaders (bacteria, viruses) and itself. And it is proteins that are the targets of most drugs that you or I take to treat disease.
Protein Lego
Why does protein structure matter? Proteins are large molecules consisting of thousands of atoms in very specific orders. The order of these atoms, and the way that they are arranged in 3D space, is crucial to a protein being able to carry out its biological function.
This same 3D arrangement also determines the way in which a drug molecule binds to its protein target and treats disease.
Imagine having a Lego set in which the bricks are not based on cuboids, but can be any shape. In order to put two bricks together in this set, each brick will need to fit snugly against the other without any holes. But this isn’t enough – the two bricks will also need to have the right combination of bumps and holes for the bricks to stay in place.
Designing a new drug molecule is a bit like playing with this new Lego set. Someone has built an enormous model already (the protein target found in our cells), and the job of the drug discovery chemist is to use their tool-kit to put a handful of bricks together that will bind to a particular part of the protein and – in biological terms – stop it carrying out its normal function.
So what does AlphaFold do? Based on knowing exactly which atoms are in any protein, how these atoms have evolved differently in different species, and what other protein structures look like, AlphaFold is very good at predicting the 3D structure of any protein.
AlphaFold 3, the most recent iteration, has expanded capabilities to model nucleic acids, for example, pieces of DNA. It can also predict the shapes of proteins that have been modified with chemical groups that may turn the protein on or off, or with sugar molecules. This gives scientists more than just a bigger, more colourful Lego set to play with. It means they can develop more detailed models of reading and correcting the genetic code and of cellular control mechanisms.
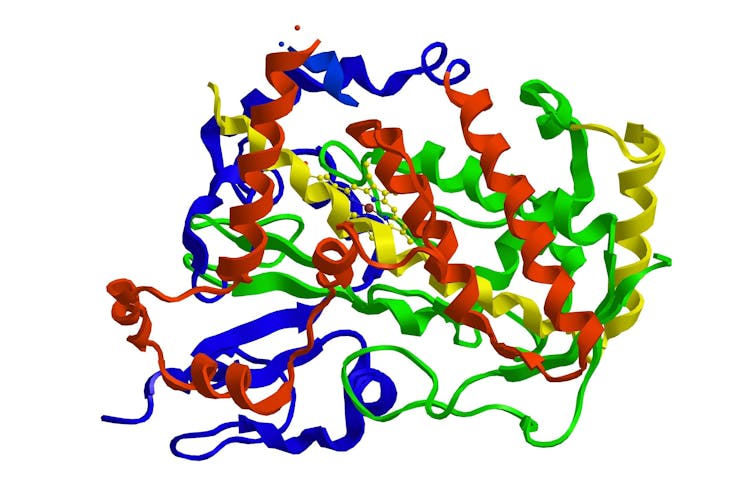
This is important in understanding disease processes at a molecular level and in developing drugs that target proteins whose biological role is regulating which genes are turned on or off. The new version of AlphaFold also predicts antibodies with greater accuracy than previous versions.
Antibodies are important proteins in biology in their own right, forming a vital part of the immune system. They are also used as biological drugs such as trastuzumab , for breast cancer, and infliximab , for diseases such as inflammatory bowel disease and rheumatoid arthritis.
The latest version of AlphaFold can predict the structure of proteins bound to drug-like small molecules. Drug discovery chemists can already predict the way in which a potential drug binds to its protein target if the 3D structure of the target has been identified through experiments. The downside is this process can take months or even years.
Predicting the way in which potential drugs and protein targets bind to each other is used to help decide which potential drugs to synthesise and test in the laboratory. AlphaFold 3 can not only predict drug binding in the absence of an experimentally identified protein structure but, in testing, it outperformed existing software predictions, even if the target structure and drug binding site were known.
These new capabilities make AlphaFold 3 an exciting addition to the repertoire of tools used to discover new therapeutic drugs. More accurate predictions will enable better decisions to be taken about which potential drugs to test in the lab (and which are unlikely to be effective).
Time and money
This saves both time and money . AlphaFold 3 also provides the opportunity to make predictions about drug binding to modified forms of the protein target which are biologically relevant but currently difficult – or impossible – to do using existing software. Examples of this are proteins modified by chemical groups such as phosphates or sugars.
Of course, as with any new potential drug, extensive experimental testing for safety and efficacy – including in human volunteers – is always needed before approval as a licensed medicine.
AlphaFold 3 does have some limitations. Like its predecessors, it is poor at predicting the behaviour of protein areas that lack a fixed or ordered structure. It is poor at predicting multiple conformations of a protein (which may change shape due to drug binding or as part of its normal biology) and cannot predict protein dynamics.
It can also make some slightly embarrassing chemical mistakes such as putting atoms on top of each other (physically impossible), and in replacing some details of a structure with its mirror images (biologically or chemically impossible).
A more substantial limitation is that the code will – for now at least – be unavailable so it will have to be used on the DeepMind server on a purely non-commercial basis. Although many academic users will not be put off by this, it will limit the enthusiasm of expert modellers, biotechnologists and many applications in drug discovery.
Despite this, the release of AlphaFold 3 looks certain to stimulate a new wave of creativity in both drug discovery and structural biology more widely – and we’re already looking forward to AlphaFold 4.
- Artificial intelligence (AI)
- Drug discovery
- Give me perspective

Data Manager

Research Support Officer

Director, Social Policy

Head, School of Psychology

Senior Research Fellow - Women's Health Services

Solving the problems of proton-conducting perovskites for next-generation fuel cells
As a newly developed perovskite with a large amount of intrinsic oxygen vacancies, BaSc 0.8 W 0.2 O 2.8 achieves high proton conduction at low and intermediate temperatures, report scientists at Tokyo Tech. By the donor doping of large W 6+ , this material can take up more water to increase its proton concentration, as well as reduce the proton trapping through electrostatic repulsion between the dopant and proton. These findings could pave the way to the rational design of novel perovskites for protonic ceramic fuel cells (PCFCs) and electrolysis cells (PCECs).
In line with global efforts towards cleaner energy technologies, fuel cells may soon become an indispensable tool for converting chemical energy -- stored in the form of hydrogen or other fuels -- into electrical energy. Among the various types of fuel cells being actively researched, those that use solid electrolytes rather than liquid ones have inherent safety and stability advantages.
In particular, protonic ceramic fuel cells (PCFCs) have attracted special attention among scientists. These devices do not operate via the conduction of oxide ions (O 2− ) but light protons (H + ) with smaller valence. A key feature of PCFCs is their ability to function at low and intermediate temperatures in the range of 50-500 °C. However, PCFCs based on perovskite electrolytes reported thus far suffer from low proton conductivity at low and intermediate temperatures.
In a recent study, a research team led by Professor Masamoto Yashima from Tokyo Institute of Technology (Tokyo Tech), in collaboration with High Energy Accelerator Research Organization (KEK), has set out to address this limitation of perovskite-based proton conductors. Their findings were published in the Journal of Materials Chemistry A on May 3, 2024.
But why is the conductivity of the conventional perovskite-type proton conductors so low? "A major problem with the conventional proton conductors is a phenomenon known as proton trapping, in which protons are trapped by acceptor dopant via electrostatic attraction between the dopant and proton," explains Yashima. "Another major problem among such proton conductors would also be their low proton concentration due to the small amount of oxygen vacancies."
To tackle these issues, the researchers developed a highly oxygen-deficient perovskite, namely BaScO 2.5 doped with W 6+ cations, or BaSc 0.8 W 0.2 O 2.8 . Thanks to its large amounts of oxygen vacancies, this material has a higher proton concentration than other proton-conducting perovskites. However, since proton hopping occurs between oxygen atoms, the oxygen vacancies would lower proton conductivity rather than increase it.
This problem was solved by full hydration of the perovskite, turning it into BaSc 0.8 W 0.2 O 3 H 0.4 . Because of the large size of the W 6+ dopant, the perovskite has a larger lattice volume, which means it can take up more water molecules than those doped with other cations such as small Mo 6+ . The high water uptake facilitates high proton conductivity by further increasing the proton concentration.
As for proton trapping, the high positive charge of the W 6+ dopant leads to a stronger repulsion with protons, which are also positively charged. This effect was confirmed through ab initio molecular dynamics simulations, which revealed the migration pathways of protons near the Sc cation when transporting across the material. The repulsion indicates reduced proton trapping by the W 6+ dopant, which leads to the high proton conductivity at low and intermediate temperatures.
Taken together, the insights provided by this study could help establish fundamental design principles for proton-conducting perovskites. "The stabilization of perovskites with disordered intrinsic oxygen vacancies and full hydration enabled by doping of large donor dopant could be an effective strategy towards next-generation proton conductors," remarks Yashima.
In addition to PCFCs, proton conductors are also needed in proton-conducting electroysis cells (PCECs), which can efficiently utilize electricity. Both of these technologies will be essential in the near future as we collectively strive towards sustainability through novel proton conductors.
Overall, the present findings may open new avenues to realize clean energy societies.
- Organic Chemistry
- Energy and Resources
- Alternative Fuels
- Electricity
- Ethanol fuel
- Quantum dot
- Fossil fuel
- Tensile strength
Story Source:
Materials provided by Tokyo Institute of Technology . Note: Content may be edited for style and length.
Journal Reference :
- Kei Saito, Kensei Umeda, Kotaro Fujii, Kazuhiro Mori, Masatomo Yashima. High proton conduction by full hydration in highly oxygen deficient perovskite . Journal of Materials Chemistry A , 2024; DOI: 10.1039/d4ta01978d
Cite This Page :
Explore More
- How Statin Therapy May Prevent Cancer
- Origins of 'Welsh Dragons' Exposed
- Resting Brain: Neurons Rehearse for Future
- Observing Single Molecules
- A Greener, More Effective Way to Kill Termites
- One Bright Spot Among Melting Glaciers
- Martian Meteorites Inform Red Planet's Structure
- Volcanic Events On Jupiter's Moon Io: High Res
- What Negative Adjectives Mean to Your Brain
- 'Living Bioelectronics' Can Sense and Heal Skin
Trending Topics
Strange & offbeat.

- SUGGESTED TOPICS
- The Magazine
- Newsletters
- Managing Yourself
- Managing Teams
- Work-life Balance
- The Big Idea
- Data & Visuals
- Reading Lists
- Case Selections
- HBR Learning
- Topic Feeds
- Account Settings
- Email Preferences
Share Podcast

A Better Framework for Solving Tough Problems
Start with trust and end with speed.
- Apple Podcasts
When it comes to solving complicated problems, the default for many organizational leaders is to take their time to work through the issues at hand. Unfortunately, that often leads to patchwork solutions or problems not truly getting resolved.
But Anne Morriss offers a different framework. In this episode, she outlines a five-step process for solving any problem and explains why starting with trust and ending with speed is so important for effective change leadership. As she says, “Let’s get into dialogue with the people who are also impacted by the problem before we start running down the path of solving it.”
Morriss is an entrepreneur and leadership coach. She’s also the coauthor of the book, Move Fast and Fix Things: The Trusted Leader’s Guide to Solving Hard Problems .
Key episode topics include: strategy, decision making and problem solving, strategy execution, managing people, collaboration and teams, trustworthiness, organizational culture, change leadership, problem solving, leadership.
HBR On Strategy curates the best case studies and conversations with the world’s top business and management experts, to help you unlock new ways of doing business. New episodes every week.
- Listen to the full HBR IdeaCast episode: How to Solve Tough Problems Better and Faster (2023)
- Find more episodes of HBR IdeaCast
- Discover 100 years of Harvard Business Review articles, case studies, podcasts, and more at HBR.org .
HANNAH BATES: Welcome to HBR On Strategy , case studies and conversations with the world’s top business and management experts, hand-selected to help you unlock new ways of doing business.
When it comes to solving complicated problems, many leaders only focus on the most apparent issues. Unfortunately that often leads to patchwork or partial solutions. But Anne Morriss offers a different framework that aims to truly tackle big problems by first leaning into trust and then focusing on speed.
Morriss is an entrepreneur and leadership coach. She’s also the co-author of the book, Move Fast and Fix Things: The Trusted Leader’s Guide to Solving Hard Problems . In this episode, she outlines a five-step process for solving any problem. Some, she says, can be solved in a week, while others take much longer. She also explains why starting with trust and ending with speed is so important for effective change leadership.
This episode originally aired on HBR IdeaCast in October 2023. Here it is.
CURT NICKISCH: Welcome to the HBR IdeaCast from Harvard Business Review. I’m Curt Nickisch.
Problems can be intimidating. Sure, some problems are fun to dig into. You roll up your sleeves, you just take care of them; but others, well, they’re complicated. Sometimes it’s hard to wrap your brain around a problem, much less fix it.
And that’s especially true for leaders in organizations where problems are often layered and complex. They sometimes demand technical, financial, or interpersonal knowledge to fix. And whether it’s avoidance on the leaders’ part or just the perception that a problem is systemic or even intractable, problems find a way to endure, to keep going, to keep being a problem that everyone tries to work around or just puts up with.
But today’s guest says that just compounds it and makes the problem harder to fix. Instead, she says, speed and momentum are key to overcoming a problem.
Anne Morriss is an entrepreneur, leadership coach and founder of the Leadership Consortium and with Harvard Business School Professor Francis Frei, she wrote the new book, Move Fast and Fix Things: The Trusted Leaders Guide to Solving Hard Problems . Anne, welcome back to the show.
ANNE MORRISS: Curt, thank you so much for having me.
CURT NICKISCH: So, to generate momentum at an organization, you say that you really need speed and trust. We’ll get into those essential ingredients some more, but why are those two essential?
ANNE MORRISS: Yeah. Well, the essential pattern that we observed was that the most effective change leaders out there were building trust and speed, and it didn’t seem to be a well-known observation. We all know the phrase, “Move fast and break things,” but the people who were really getting it right were moving fast and fixing things, and that was really our jumping off point. So when we dug into the pattern, what we observed was they were building trust first and then speed. This foundation of trust was what allowed them to fix more things and break fewer.
CURT NICKISCH: Trust sounds like a slow thing, right? If you talk about building trust, that is something that takes interactions, it takes communication, it takes experiences. Does that run counter to the speed idea?
ANNE MORRISS: Yeah. Well, this issue of trust is something we’ve been looking at for over a decade. One of the headlines in our research is it’s actually something we’re building and rebuilding and breaking all the time. And so instead of being this precious, almost farbege egg, it’s this thing that is constantly in motion and this thing that we can really impact when we’re deliberate about our choices and have some self-awareness around where it’s breaking down and how it’s breaking down.
CURT NICKISCH: You said break trust in there, which is intriguing, right? That you may have to break trust to build trust. Can you explain that a little?
ANNE MORRISS: Yeah, well, I’ll clarify. It’s not that you have to break it in order to build it. It’s just that we all do it some of the time. Most of us are trusted most of the time. Most of your listeners I imagine are trusted most of the time, but all of us have a pattern where we break trust or where we don’t build as much as could be possible.
CURT NICKISCH: I want to talk about speed, this other essential ingredient that’s so intriguing, right? Because you think about solving hard problems as something that just takes a lot of time and thinking and coordination and planning and designing. Explain what you mean by it? And also, just how we maybe approach problems wrong by taking them on too slowly?
ANNE MORRISS: Well, Curt, no one has ever said to us, “I wish I had taken longer and done less.” We hear the opposite all the time, by the way. So what we really set out to do was to create a playbook that anyone can use to take less time to do more of the things that are going to make your teams and organizations stronger.
And the way we set up the book is okay, it’s really a five step process. Speed is the last step. It’s the payoff for the hard work you’re going to do to figure out your problem, build or rebuild trust, expand the team in thoughtful and strategic ways, and then tell a real and compelling story about the change you’re leading.
Only then do you get to go fast, but that’s an essential part of the process, and we find that either people under emphasize it or speed has gotten a bad name in this world of moving fast and breaking things. And part of our mission for sure was to rehabilitate speed’s reputation because it is an essential part of the change leader’s equation. It can be the difference between good intentions and getting anything done at all.
CURT NICKISCH: You know, the fact that nobody ever tells you, “I wish we had done less and taken more time.” I think we all feel that, right? Sometimes we do something and then realize, “Oh, that wasn’t that hard and why did it take me so long to do it? And I wish I’d done this a long time ago.” Is it ever possible to solve a problem too quickly?
ANNE MORRISS: Absolutely. And we see that all the time too. What we push people to do in those scenarios is really take a look at the underlying issue because in most cases, the solution is not to take your foot off the accelerator per se and slow down. The solution is to get into the underlying problem. So if it’s burnout or a strategic disconnect between what you’re building and the marketplace you’re serving, what we find is the anxiety that people attach to speed or the frustration people attach to speed is often misplaced.
CURT NICKISCH: What is a good timeline to think about solving a problem then? Because if we by default take too long or else jump ahead and we don’t fix it right, what’s a good target time to have in your mind for how long solving a problem should take?
ANNE MORRISS: Yeah. Well, we’re playful in the book and talking about the idea that many problems can be solved in a week. We set the book up five chapters. They’re titled Monday, Tuesday, Wednesday, Thursday, Friday, and we’re definitely having fun with that. And yet, if you count the hours in a week, there are a lot of them. Many of our problems, if you were to spend a focused 40 hours of effort on a problem, you’re going to get pretty far.
But our main message is, listen, of course it’s going to depend on the nature of the problem, and you’re going to take weeks and maybe even some cases months to get to the other side. What we don’t want you to do is take years, which tends to be our default timeline for solving hard problems.
CURT NICKISCH: So you say to start with identifying the problem that’s holding you back, seems kind of obvious. But where do companies go right and wrong with this first step of just identifying the problem that’s holding you back?
ANNE MORRISS: And our goal is that all of these are going to feel obvious in retrospect. The problem is we skip over a lot of these steps and this is why we wanted to underline them. So this one is really rooted in our observation and I think the pattern of our species that we tend to be overconfident in the quality of our thoughts, particularly when it comes to diagnosing problems.
And so we want to invite you to start in a very humble and curious place, which tends not to be our default mode when we’re showing up for work. We convince ourselves that we’re being paid for our judgment. That’s exactly what gets reinforced everywhere. And so we tend to counterintuitively, given what we just talked about, we tend to move too quickly through the diagnostic phase.
CURT NICKISCH: “I know what to do, that’s why you hired me.”
ANNE MORRISS: Exactly. “I know what to do. That’s why you hired me. I’ve seen this before. I have a plan. Follow me.” We get rewarded for the expression of confidence and clarity. And so what we’re inviting people to do here is actually pause and really lean into what are the root causes of the problem you’re seeing? What are some alternative explanations? Let’s get into dialogue with the people who are also impacted by the problem before we start running down the path of solving it.
CURT NICKISCH: So what do you recommend for this step, for getting to the root of the problem? What are questions you should ask? What’s the right thought process? What do you do on Monday of the week?
ANNE MORRISS: In our experience of doing this work, people tend to undervalue the power of conversation, particularly with other people in the organization. So we will often advocate putting together a team of problem solvers, make it a temporary team, really pull in people who have a particular perspective on the problem and create the space, make it as psychologically safe as you can for people to really, as Chris Argyris so beautifully articulated, discuss the undiscussable.
And so the conditions for that are going to look different in every organization depending on the problem, but if you can get a space where smart people who have direct experience of a problem are in a room and talking honestly with each other, you can make an extraordinary amount of progress, certainly in a day.
CURT NICKISCH: Yeah, that gets back to the trust piece.
ANNE MORRISS: Definitely.
CURT NICKISCH: How do you like to start that meeting, or how do you like to talk about it? I’m just curious what somebody on that team might hear in that meeting, just to get the sense that it’s psychologically safe, you can discuss the undiscussable and you’re also focusing on the identification part. What’s key to communicate there?
ANNE MORRISS: Yeah. Well, we sometimes encourage people to do a little bit of data gathering before those conversations. So the power of a quick anonymous survey around whatever problem you’re solving, but also be really thoughtful about the questions you’re going to ask in the moment. So a little bit of preparation can go a long way and a little bit of thoughtfulness about the power dynamic. So who’s going to walk in there with license to speak and who’s going to hold back? So being thoughtful about the agenda, about the questions you’re asking about the room, about the facilitation, and then courage is a very infectious emotion.
So if you can early on create the conditions for people to show up bravely in that conversation, then the chance that you’re going to get good information and that you’re going to walk out of that room with new insight in the problem that you didn’t have when you walked in is extraordinarily high.
CURT NICKISCH: Now, in those discussions, you may have people who have different perspectives on what the problem really is. They also bear different costs of addressing the problem or solving it. You talked about the power dynamic, but there’s also an unfairness dynamic of who’s going to actually have to do the work to take care of it, and I wonder how you create a culture in that meeting where it’s the most productive?
ANNE MORRISS: For sure, the burden of work is not going to be equitably distributed around the room. But I would say, Curt, the dynamic that we see most often is that people are deeply relieved that hard problems are being addressed. So it really can create, and more often than not in our experience, it does create this beautiful flywheel of action, creativity, optimism. Often when problems haven’t been addressed, there is a fair amount of anxiety in the organization, frustration, stagnation. And so credible movement towards action and progress is often the best antidote. So even if the plan isn’t super clear yet, if it’s credible, given who’s in the room and their decision rights and mandate, if there’s real momentum coming out of that to make progress, then that tends to be deeply energizing to people.
CURT NICKISCH: I wonder if there’s an organization that you’ve worked with that you could talk about how this rolled out and how this took shape?
ANNE MORRISS: When we started working with Uber, that was wrestling with some very public issues of culture and trust with a range of stakeholders internally, the organization, also external, that work really started with a campaign of listening and really trying to understand where trust was breaking down from the perspective of these stakeholders?
So whether it was female employees or regulators or riders who had safety concerns getting into the car with a stranger. This work, it starts with an honest internal dialogue, but often the problem has threads that go external. And so bringing that same commitment to curiosity and humility and dialogue to anyone who’s impacted by the problem is the fastest way to surface what’s really going on.
CURT NICKISCH: There’s a step in this process that you lay out and that’s communicating powerfully as a leader. So we’ve heard about listening and trust building, but now you’re talking about powerful communication. How do you do this and why is it maybe this step in the process rather than the first thing you do or the last thing you do?
ANNE MORRISS: So in our process, again, it’s the days of the week. On Monday you figured out the problem. Tuesday you really got into the sandbox in figuring out what a good enough plan is for building trust. Wednesday, step three, you made it better. You created an even better plan, bringing in new perspectives. Thursday, this fourth step is the day we’re saying you got to go get buy-in. You got to bring other people along. And again, this is a step where we see people often underinvest in the power and payoff of really executing it well.
CURT NICKISCH: How does that go wrong?
ANNE MORRISS: Yeah, people don’t know the why. Human behavior and the change in human behavior really depends on a strong why. It’s not just a selfish, “What’s in it for me?” Although that’s helpful, but where are we going? I may be invested in a status quo and I need to understand, okay, if you’re going to ask me to change, if you’re going to invite me into this uncomfortable place of doing things differently, why am I here? Help me understand it and articulate the way forward and language that not only I can understand, but also that’s going to be motivating to me.
CURT NICKISCH: And who on my team was part of this process and all that kind of stuff?
ANNE MORRISS: Oh, yeah. I may have some really important questions that may be in the way of my buy-in and commitment to this plan. So certainly creating a space where those questions can be addressed is essential. But what we found is that there is an architecture of a great change story, and it starts with honoring the past, honoring the starting place. Sometimes we’re so excited about the change and animated about the change that what has happened before or what is even happening in the present tense is low on our list of priorities.
Or we want to label it bad, because that’s the way we’ve thought about the change, but really pausing and honoring what came before you and all the reasonable decisions that led up to it, I think can be really helpful to getting people emotionally where you want them to be willing to be guided by you. Going back to Uber, when Dara Khosrowshahi came in.
CURT NICKISCH: This is the new CEO.
ANNE MORRISS: The new CEO.
CURT NICKISCH: Replaced Travis Kalanick, the founder and first CEO, yeah.
ANNE MORRISS: Yeah, and had his first all-hands meeting. One of his key messages, and this is a quote, was that he was going to retain the edge that had made Uber, “A force of nature.” And in that meeting, the crowd went wild because this is also a company that had been beaten up publicly for months and months and months, and it was a really powerful choice. And his predecessor, Travis was in the room, and he also honored Travis’ incredible work and investment in bringing the company to the place where it was.
And I would use words like grace to also describe those choices, but there’s also an incredible strategic value to naming the starting place for everybody in the room because in most cases, most people in that room played a role in getting to that starting place, and you’re acknowledging that.
CURT NICKISCH: You can call it grace. Somebody else might call it diplomatic or strategic. But yeah, I guess like it or not, it’s helpful to call out and honor the complexity of the way things have been done and also the change that’s happening.
ANNE MORRISS: Yeah, and the value. Sometimes honoring the past is also owning what didn’t work or what wasn’t working for stakeholders or segments of the employee team, and we see that around culture change. Sometimes you’ve got to acknowledge that it was not an equitable environment, but whatever the worker, everyone in that room is bringing that pass with them. So again, making it discussable and using it as the jumping off place is where we advise people to start.
Then you’ve earned the right to talk about the change mandate, which we suggest using clear and compelling language about the why. “This is what happened, this is where we are, this is the good and the bad of it, and here’s the case for change.”
And then the last part, which is to describe a rigorous and optimistic way forward. It’s a simple past, present, future arc, which will be familiar to human beings. We love stories as human beings. It’s among the most powerful currency we have to make sense of the world.
CURT NICKISCH: Yeah. Chronological is a pretty powerful order.
ANNE MORRISS: Right. But again, the change leaders we see really get it right, are investing an incredible amount of time into the storytelling part of their job. Ursula Burns, the Head of Xerox is famous for the months and years she spent on the road just telling the story of Xerox’s change, its pivot into services to everyone who would listen, and that was a huge part of her success.
CURT NICKISCH: So Friday or your fifth step, you end with empowering teams and removing roadblocks. That seems obvious, but it’s critical. Can you dig into that a little bit?
ANNE MORRISS: Yeah. Friday is the fun day. Friday’s the release of energy into the system. Again, you’ve now earned the right to go fast. You have a plan, you’re pretty confident it’s going to work. You’ve told the story of change the organization, and now you get to sprint. So this is about really executing with urgency, and it’s about a lot of the tactics of speed is where we focus in the book. So the tactics of empowerment, making tough strategic trade-offs so that your priorities are clear and clearly communicated, creating mechanisms to fast-track progress. At Etsy, CEO Josh Silverman, he labeled these projects ambulances. It’s an unfortunate metaphor, but it’s super memorable. These are the products that get to speed out in front of the other ones because the stakes are high and the clock is sticking.
CURT NICKISCH: You pull over and let it go by.
ANNE MORRISS: Yeah, exactly. And so we have to agree as an organization on how to do something like that. And so we see lots of great examples both in young organizations and big complex biotech companies with lots of regulatory guardrails have still found ways to do this gracefully.
And I think we end with this idea of conflict debt, which is a term we really love. Leanne Davey, who’s a team scholar and researcher, and anyone in a tech company will recognize the idea of tech debt, which is this weight the organization drags around until they resolve it. Conflict debt is a beautiful metaphor because it is this weight that we drag around and slows us down until we decide to clean it up and fix it. The organizations that are really getting speed right have figured out either formally or informally, how to create an environment where conflict and disagreements can be gracefully resolved.
CURT NICKISCH: Well, let’s talk about this speed more, right? Because I think this is one of those places that maybe people go wrong or take too long, and then you lose the awareness of the problem, you lose that urgency. And then that also just makes it less effective, right? It’s not just about getting the problem solved as quickly as possible. It’s also just speed in some ways helps solve the problem.
ANNE MORRISS: Oh, yeah. It really is the difference between imagining the change you want to lead and really being able to bring it to life. Speed is the thing that unlocks your ability to lead change. It needs a foundation, and that’s what Monday through Thursday is all about, steps one through four, but the finish line is executing with urgency, and it’s that urgency that releases the system’s energy, that communicates your priorities, that creates the conditions for your team to make progress.
CURT NICKISCH: Moving fast is something that entrepreneurs and tech companies certainly understand, but there’s also this awareness that with big companies, the bigger the organization, the harder it is to turn the aircraft carrier around, right? Is speed relative when you get at those levels, or do you think this is something that any company should be able to apply equally?
ANNE MORRISS: We think this applies to any company. The culture really lives at the level of team. So we believe you can make a tremendous amount of progress even within your circle of control as a team leader. I want to bring some humility to this and careful of words like universal, but we do think there’s some universal truths here around the value of speed, and then some of the byproducts like keeping fantastic people. Your best people want to solve problems, they want to execute, they want to make progress and speed, and the ability to do that is going to be a variable in their own equation of whether they stay or they go somewhere else where they can have an impact.
CURT NICKISCH: Right. They want to accomplish something before they go or before they retire or finish something out. And if you’re able to just bring more things on the horizon and have it not feel like it’s going to be another two years to do something meaningful.
ANNE MORRISS: People – I mean, they want to make stuff happen and they want to be around the energy and the vitality of making things happen, which again, is also a super infectious phenomenon. One of the most important jobs of a leader, we believe, is to set the metabolic pace of their teams and organizations. And so what we really dig into on Friday is, well, what does that look like to speed something up? What are the tactics of that?
CURT NICKISCH: I wonder if that universal truth, that a body in motion stays in motion applies to organizations, right? If an organization in motion stays in motion, there is something to that.
ANNE MORRISS: Absolutely.
CURT NICKISCH: Do you have a favorite client story to share, just where you saw speed just become a bit of a flywheel or just a positive reinforcement loop for more positive change at the organization?
ANNE MORRISS: Yeah. We work with a fair number of organizations that are on fire. We do a fair amount of firefighting, but we also less dramatically do a lot of fire prevention. So we’re brought into organizations that are working well and want to get better, looking out on the horizon. That work is super gratifying, and there is always a component of, well, how do we speed this up?
What I love about that work is there’s often already a high foundation of trust, and so it’s, well, how do we maintain that foundation but move this flywheel, as you said, even faster? And it’s really energizing because often there’s a lot of pent-up energy that… There’s a lot of loyalty to the organization, but often it’s also frustration and pent-up energy. And so when that gets released, when good people get the opportunity to sprint for the first time in a little while, it’s incredibly energizing, not just for us, but for the whole organization.
CURT NICKISCH: Anne, this is great. I think finding a way to solve problems better but also faster is going to be really helpful. So thanks for coming on the show to talk about it.
ANNE MORRISS: Oh, Curt, it was such a pleasure. This is my favorite conversation. I’m delighted to have it anytime.
HANNAH BATES: That was entrepreneur, leadership coach, and author Anne Morriss – in conversation with Curt Nickisch on HBR IdeaCast.
We’ll be back next Wednesday with another hand-picked conversation about business strategy from Harvard Business Review. If you found this episode helpful, share it with your friends and colleagues, and follow our show on Apple Podcasts, Spotify, or wherever you get your podcasts. While you’re there, be sure to leave us a review.
When you’re ready for more podcasts, articles, case studies, books, and videos with the world’s top business and management experts, you’ll find it all at HBR.org.
This episode was produced by Mary Dooe, Anne Saini, and me, Hannah Bates. Ian Fox is our editor. Special thanks to Rob Eckhardt, Maureen Hoch, Erica Truxler, Ramsey Khabbaz, Nicole Smith, Anne Bartholomew, and you – our listener. See you next week.
- Subscribe On:
Latest in this series
This article is about strategy.
- Decision making and problem solving
- Strategy execution
- Leadership and managing people
- Collaboration and teams
- Trustworthiness
- Organizational culture
Partner Center

An official website of the United States government
The .gov means it’s official. Federal government websites often end in .gov or .mil. Before sharing sensitive information, make sure you’re on a federal government site.
The site is secure. The https:// ensures that you are connecting to the official website and that any information you provide is encrypted and transmitted securely.
- Publications
- Account settings
Preview improvements coming to the PMC website in October 2024. Learn More or Try it out now .
- Advanced Search
- Journal List
- CBE Life Sci Educ
- v.15(4); Winter 2016
Step by Step: Biology Undergraduates’ Problem-Solving Procedures during Multiple-Choice Assessment
Luanna b. prevost.
† Department of Integrative Biology, University of South Florida, Tampa, FL 33620
Paula P. Lemons
‡ Department of Biochemistry and Molecular Biology, University of Georgia, Athens, GA 30602
Associated Data
Findings from a mixed-methods investigation of undergraduate biology problem solving are reported. Students used a variety of problem-solving procedures that are domain general and domain specific. This study provides a model for research on alternative problem types and can be applied immediately in the biology classroom.
This study uses the theoretical framework of domain-specific problem solving to explore the procedures students use to solve multiple-choice problems about biology concepts. We designed several multiple-choice problems and administered them on four exams. We trained students to produce written descriptions of how they solved the problem, and this allowed us to systematically investigate their problem-solving procedures. We identified a range of procedures and organized them as domain general, domain specific, or hybrid. We also identified domain-general and domain-specific errors made by students during problem solving. We found that students use domain-general and hybrid procedures more frequently when solving lower-order problems than higher-order problems, while they use domain-specific procedures more frequently when solving higher-order problems. Additionally, the more domain-specific procedures students used, the higher the likelihood that they would answer the problem correctly, up to five procedures. However, if students used just one domain-general procedure, they were as likely to answer the problem correctly as if they had used two to five domain-general procedures. Our findings provide a categorization scheme and framework for additional research on biology problem solving and suggest several important implications for researchers and instructors.
INTRODUCTION
The call to reform undergraduate education involves shifting the emphasis in science classes away from rote memorization of facts toward learning core concepts and scientific practices ( National Research Council [NRC], 2003 ; American Association for the Advancement of Science [AAAS], 2011 ). To develop instruction that focuses on core concepts and scientific practices, we need more knowledge about the concepts that are challenging for students to learn. For example, biology education research has established that students struggle with the concepts of carbon cycling (e.g., Anderson et al. , 1990 ; Hartley et al ., 2011 ) and natural selection (e.g., Nehm and Reilly, 2007 ), but we know much less about students’ conceptual difficulties in ecology and physiology. Researchers and practitioners also need to discover how students develop the ability to use scientific practices. Although these efforts are underway (e.g., Anderson et al. , 2012 ; Gormally et al. , 2012 ; Dirks et al. , 2013 ; Brownell et al. , 2014 ), many research questions remain. As research accumulates, educators can create curricula and assessments that improve student learning for all. We investigate one key scientific practice that is understudied in biology education, problem solving ( AAAS, 2011 ; Singer et al. , 2012 ).
For the purposes of this article, we define problem solving as a decision-making process wherein a person is presented with a task, and the path to solving the task is uncertain. We define a problem as a task that presents a challenge that cannot be solved automatically ( Martinez, 1998 ). Problem-solving research began in the 1940s and 1950s and focused on problem-solving approaches that could be used to solve any problem regardless of the discipline ( Duncker and Lees, 1945 ; Polya, 1957 ; Newell and Simon, 1972 ; Jonassen, 2000 , 2012 ; Bassok and Novick, 2012 ). Despite the broad applicability of these domain-general problem-solving approaches, subsequent research has shown that the strongest problem-solving approaches derive from deep knowledge of a domain ( Newell and Simon, 1972 ; Chi et al. , 1981 ; Pressley et al. , 1987 ). Domain is a term that refers to a body of knowledge that can be broad, like biology, or narrow, like ecosystem structure and function. This body of literature has developed into a theoretical framework called domain-specific problem solving. We situate our research within this theoretical framework.
THE THEORETICAL FRAMEWORK OF DOMAIN-SPECIFIC PROBLEM SOLVING
Domain-specific problem solving has its origins in information-processing theory (IPT; Newell and Simon, 1972 ). IPT focuses on the cognitive processes used to reach a problem solution and emphasizes the general thinking processes people use when they attempt problem solving, such as brainstorming ( Runco and Chand, 1995 ; Halpern, 1997 ) and working backward by beginning with the problem goal and working in reverse toward the initial problem state ( Newell et al. , 1958 ; Chi and Glaser, 1985 ). Despite the empirical evidence for general thinking processes, one of IPT’s shortcomings as a comprehensive view of human cognition ( Dawson, 1998 ) is that the knowledge base of the problem solver is not considered.
Domain-specific problem solving expands IPT to recognize that experts in a particular domain have a relatively complete and well-organized knowledge base that enables them to solve the complex problems they face (e.g., Chase and Simon, 1973 ). One of the landmark studies showing the differences between the knowledge base of experts and nonexperts, or novices, was conducted in science, specifically in physics. Chi and colleagues (1981) compared the classification of physics problems by advanced physics PhD students (i.e., experts) and undergraduates who had just completed a semester of mechanics (i.e., novices), identifying fundamental differences. Chemistry researchers built on Chi’s work to identify differences in how experts and novices track their problem solving and use problem categorization and multiple representations ( Bunce et al ., 1991 ; Kohl and Finkelstein, 2008 ; Catrette and Bodner, 2010 ). Biology researchers built upon this work by conducting similar problem-solving studies among experts and novices in evolution and genetics ( Smith, 1992 ; Smith et al. , 2013 ; Nehm and Ridgway, 2011 ). Taken together, these studies established that experts tend to classify problems based on deep, conceptual features, while novices classify problems based on superficial features that are irrelevant to the solution.
Domain-specific problem-solving research within biology also has revealed important individual differences within groups of problem solvers. These studies show that wide variation in problem-solving performance exists. For example, some novices who solve problems about evolution classify problems and generate solutions that are expert-like, while others do not ( Nehm and Ridgway, 2011 ). This research points to the importance of studying variations in problem solving within novice populations.
Given the centrality of the knowledge base for domain-specific problem solving, it is necessary to describe the components of that knowledge base. Domain-specific problem-solving research recognizes three types of knowledge that contribute to expertise. Declarative knowledge consists of the facts and concepts about the domain. Procedural knowledge represents the how-to knowledge that is required to carry out domain-specific tasks. Conditional knowledge describes the understanding of when and where to use one’s declarative and procedural knowledge ( Alexander and Judy, 1988 ). Note that the field of metacognition also uses this three-type structure to describe metacognitive knowledge, or what you know about your own thinking ( Brown, 1978 ; Jacobs and Paris, 1987 ; Schraw and Moshman, 1995 ). However, for this paper, we use these terms to describe knowledge of biology, not metacognitive knowledge. More specifically, we focus on procedural knowledge.
Procedural knowledge consists of procedures. Procedures are tasks that are carried out automatically or intentionally during problem solving ( Alexander and Judy, 1988 ). Procedures exist on a continuum. They can be highly specific to the domain, such as analyzing the evolutionary relationships represented by a phylogenetic tree, or general and applicable to problems across many domains, such as paraphrasing a problem-solving prompt ( Pressley et al. , 1987 , 1989 ; Alexander and Judy, 1988 ).
APPLYING DOMAIN-SPECIFIC PROBLEM SOLVING TO MULTIPLE-CHOICE ASSESSMENT IN BIOLOGY
We used domain-specific problem solving to investigate the most common form of assessment in the college biology classroom, multiple-choice assessment ( Zheng et al ., 2008 ; Momsen et al ., 2013 ). College biology and science, technology, engineering, and mathematics (STEM) courses rely on multiple-choice assessment due to large enrollments, limited teaching assistant support, and ease of scoring. Outside the classroom, multiple-choice assessment is used on high-stakes exams that determine acceptance to professional schools, like the Medical College Admissions Test and Graduate Record Exam. To our knowledge, the framework of domain-specific problem solving has not been applied previously to investigate multiple-choice assessment in college biology.
It has become common practice within the biology education community to think about assessment, including multiple-choice assessment, by determining the Bloom’s taxonomy ranking of assessment items (e.g., Bissell and Lemons, 2006 ; Crowe et al. , 2008 ; Momsen et al. , 2010 , 2013 ). Bloom’s Taxonomy of Educational Objectives was built to facilitate the exchange of test items among faculty; it was not based primarily on the evaluation of student work ( Bloom, 1956 ; Anderson and Krathwohl, 2001 ). Bloom’s taxonomy helps educators think about the range of cognitive processes they could ask their students to perform and has served as an invaluable resource enabling educators to improve alignment between learning objectives, assessments, and classroom curricula (e.g., Crowe et al. , 2008 ). When applying Bloom’s taxonomy to assessment items, items are ranked as remembering, understanding, applying, analyzing, evaluating, and synthesizing. Items ranked as remembering and understanding are grouped as lower-order items; and items ranked as applying, analyzing, evaluating, and synthesizing are grouped as higher-order items ( Zoller, 1993 ; Crowe et al. , 2008 ). Despite the value of Bloom’s taxonomy for instructors, what is not known is the relationship between the procedural knowledge of domain-specific problem solving and the Bloom’s ranking of biology assessments. This is a critical gap in the literature, because efforts to improve student learning in college science classrooms may be stymied if critical insights about student work from domain-specific problem solving are not linked to our understanding of assessment and curricular design.
In the study reported here, we used the theoretical lens of domain-specific problem solving to describe the procedural knowledge of nonmajors in an introductory biology course. We addressed the following research questions:
- What are the domain-general and domain-specific procedures students use to solve multiple-choice biology problems?
- To what extent do students use domain-general and domain-specific procedures when solving lower-order versus higher-order problems?
- To what extent does the use of domain-general or domain-specific procedures influence the probability of answering problems correctly?
Setting and Participants
We recruited participants from a nonmajors introductory biology course at a southeastern public research university in the Spring 2011 semester. One of the authors (P.P.L.) was the course instructor. The course covered four major areas in biology: evolution, ecology, physiology, and organismal diversity. The instructor delivered course content using lecture interspersed with clicker questions and additional opportunities for students to write and discuss. Students also completed five in-class case studies during the semester; students completed cases in self-selected small groups and turned in one completed case study per group for grading. In addition to group case studies, the instructor assessed student learning via individual exams. Students also received points toward their final grades based on clicker participation.
In the second week of the semester, the instructor announced this research study in class and via the course-management system, inviting all students to participate. Students who volunteered to participate by completing an informed consent form were asked to produce written think-alouds for problems on course exams throughout the semester. One hundred sixty-four students completed an informed consent form. Of the 164 consenting students, 140 students actually produced a written think-aloud for at least one of 13 problems; of the 140 students, 18 did written think-alouds for all 13 problems. The remainder of students did written think-alouds for one to 13 problems. On average, research participants provided written think-alouds for 7.76 problems.
The 164 consenting students represented 73.9% of the course enrollment ( n = 222). The 164 consenting students included 70.8% females and 29.2% males; 20.4% freshmen, 40.9% sophomores, 24.1% juniors, and 13.9% seniors. The 164 students were majoring in the following areas: 3.7% business, 1.5% education, 4.4% humanities, 11.0% life and physical sciences, 5.9% engineering, and 72.3% social sciences.
This research was conducted under exempt status at the University of Georgia (UGA; IRB project 201110340).
Data Collection
Problem development..
We wrote 16 multiple-choice problems to include in this study. All problems related to material dealt with during class and focused specifically on ecosystems, evolution, and structure–function relationships. On data analysis, three problems were excluded, because most students were confused by the wording or visual representations or were able to solve the problem correctly with a superficial strategy. Each problem was preceded by a prompt for students to provide their written think-aloud (see Written Think-Alouds section). Each problem was also labeled with a preliminary Bloom’s taxonomy categorization ( Anderson and Krathwohl, 2001 ). A summary of all problems, including a description, the preliminary Bloom’s ranking, and the faculty consensus Bloom’s ranking, is provided in Table 1 . As an example, one of the final 13 problems is shown in Figure 1 . All other problems are shown in Supplemental Figure S1.

Sample problem from the domain of evolution used to probe students’ problem-solving procedures. The preliminary ranking that students saw for this question was Applying and Analyzing based on Bloom’s taxonomy. Experts ranked this problem as Analyzing. The correct answer is E. Images of benthic and limnetic males are courtesy of Elizabeth Carefoot, Simon Fraser University.
Summary of problems used for data collection
For each problem, a description is included along with the preliminary Bloom’s ranking, and the final consensus Bloom’s ranking. The actual problems are included in Supplemental Figure S1.
Ranking of Problems by Bloom’s Level.
We wanted to investigate the use of domain-general or domain-specific procedures in lower-order versus higher-order problems. We asked three biology faculty members who were not investigators in this study to rank the Bloom’s levels of the problems we developed. The biology faculty members were selected because they have extensive teaching experience in college biology and also have experience ranking assessment items using Bloom’s taxonomy. The faculty used a protocol similar to one described previously ( Momsen et al. , 2010 ). To assist with Bloom’s ranking, we provided them with class materials relevant to the problems, including lecture notes and background readings. This is necessary, because the ranking of a problem depends on the material that students have encountered in class previously. The faculty members independently ranked each problem. Interrater reliability of independent rankings was determined using an intraclass coefficient (0.82). The faculty members met to discuss their rankings and settled disagreements by consensus. The preliminary Bloom’s rankings and the faculty consensus Bloom’s rankings for problems are reported in Table 1 . For the remainder of the paper, we use the consensus Bloom’s rankings to describe problems as either lower order or higher order.
Administration of Problems to Students.
The 13 problems included in this study were administered to students on exams 1, 2, 3, and the final exam as follows: three on exam 1, three on exam 2 four on exam 3, and three on the final exam. Students’ multiple-choice responses were part of the actual exam score. They received 0.5 extra-credit points for providing satisfactory documentation of their thought processes. Students did not receive extra credit if we judged their documentation to be insufficient. Insufficient responses were those in which students made only one or two brief statements about their problem-solving process (e.g., “I chose C”). Students could answer the multiple-choice problem and opt not to provide documentation of their thinking for extra credit. Students could receive up to 6.5 points of extra credit for documentation of the problem set. The total points possible for the semester were 500, so extra credit for this research could account for up to 1.3% of a student’s grade.
Written Think-Alouds.
We developed a protocol to capture students’ written descriptions of their thought processes while solving problems on exams based on a think-aloud interview approach. In the think-aloud interview approach, research participants are given a problem to solve and are asked to say aloud everything they are thinking while solving the problem ( Ericsson and Simon, 1984 ; Keys, 2000 ). In the written think-aloud, students are asked to write, rather than say aloud, what they are thinking as they solve a problem. To train students to perform a written think-aloud, the course instructor modeled the think-aloud in class. She then assigned a homework problem that required students to answer a multiple-choice problem and construct written think-alouds recounting how they solved the problem. We then reviewed students’ homework and provided feedback. We selected examples of good documentation and poor documentation and published these anonymously on the online course-management system. After this training and feedback, we included four problems on every exam for which we asked students to provide a written think-aloud description. We collected 1087 written think-alouds from 140 students (63% of course enrollment, n = 222) for 13 problems. Figure 2 shows a typical example of a student written think-aloud.

Written think-aloud from an introductory biology student who had been instructed to write down her procedures for solving a multiple-choice biology problem. This document describes the student’s procedures for solving the problem shown in Figure 1 .
Data Analysis
We analyzed students’ written think-alouds using a combination of qualitative and quantitative methods. We used qualitative content analysis ( Patton, 1990 ) to identify and categorize the primary patterns of student thinking during problem solving. We used quantitative analysis to determine the relationship between use of domain-general, hybrid, and domain-specific procedures and problem type and to investigate the impact of domain-general/hybrid and domain-specific procedure use on answering correctly.
Qualitative Analyses of Students’ Written Think-alouds.
The goal of our qualitative analysis was to identify the cognitive procedures students follow to solve multiple-choice biology problems during an exam. Our qualitative analysis took place in two phases.
Phase 1: Establishing Categories of Student Problem-Solving Procedures.
Independently, we read dozens of individual think-alouds for each problem. While we read, we made notes about the types of procedures we observed. One author (P.P.L.) noted, for example, that students recalled concepts, organized their thinking, read and ruled out multiple-choice options, explained their selections, and weighed the pros and cons of multiple-choice options. The other author (L.B.P.) noted that students recalled theories, interpreted a phylogenetic tree, identified incomplete information, and refuted incorrect information. After independently reviewing the written think-alouds, we met to discuss what we had found and to build an initial list of categories of problem-solving procedures. Based on our discussion, we built a master list of categories of procedures (Supplemental Table S1).
Next, we compared our list with Bloom’s Taxonomy of Educational Objectives ( Anderson and Krathwohl, 2001 ) and the Blooming Biology Tool ( Crowe et al. , 2008 ). We sought to determine whether the cognitive processes described in these sources corresponded to the cognitive processes we observed in our initial review of students’ written think-alouds. Where there was overlap, we renamed our categories to use the language of Bloom’s taxonomy. For the categories that did not overlap, we kept our original names.
Phase 2: Assigning Student Problem-Solving Procedures to Categories.
Using the list of categories developed in phase 1, we categorized every problem-solving procedure articulated by students in the written think-alouds. We analyzed 1087 documents for 13 problems. For each of the 13 problems, we followed the same categorization process. In a one-on-one meeting, we discussed a few written think-alouds. While still in the same room, we categorized several written think-alouds independently. We then compared our categorizations and discussed any disagreements. We then repeated these steps for additional think-alouds while still together. Once we reached agreement on all categories for a single problem, we independently categorized a common subset of written think-alouds to determine interrater reliability. When interrater reliability was below a level we considered acceptable (0.8 Cronbach’s alpha), we went through the process again. Then one author (either L.B.P. or P.P.L.) categorized the remainder of the written think-alouds for that problem.
At the end of phase 2, after we had categorized all 1087 written think-alouds, we refined our category list, removing categories with extremely low frequencies and grouping closely related categories. For example, we combined the category Executing with Implementing into a category called Analyzing Visual Representations.
Phase 3: Aligning Categories with Our Theoretical Framework.
Having assigned student problem-solving procedures to categories, we determined whether the category aligned best with domain-general or domain-specific problem solving. To make this determination, we considered the extent to which the problem-solving procedures in a category depended on knowledge of biology. Categories of procedures aligned with domain-general problem solving were carried out without drawing on content knowledge (e.g., Clarifying). Categories aligned with domain-specific problem solving were carried out using content knowledge (e.g., Checking). We also identified two categories of problem solving that we labeled hybrids of domain-general and domain-specific problem solving, because students used content knowledge in these steps, but they did so superficially (e.g., Recognizing).
Supplemental Table S1 shows the categories that resulted from our analytical process, including phase 1 notes, phase 2 categories, and phase 3 final category names as presented in this paper. Categories are organized into the themes of domain-general, hybrid, and domain-specific problem solving (Supplemental Table S1).
Quantitative Analyses of Students’ Written Think-Alouds.
To determine whether students used domain-general/hybrid or domain-specific problem solving preferentially when solving problems ranked by faculty as lower order or higher order, we used generalized linear mixed models (GLMM). GLMM are similar to ordinary linear regressions but take into account nonnormal distributions. GLMM can also be applied to unbalanced repeated measures ( Fitzmaurice et al. , 2011 ). In our data set, an individual student could provide documentation to one or more problems (up to 13 problems). Thus, in some but not all cases, we have repeated measures for individuals. To account for these repeated measures, we used “student” as our random factor. We used the problem type (lower order or higher order) as our fixed factor. Because our independent variables, number of domain-general/hybrid procedures and number of domain-specific procedures, are counts, we used a negative binomial regression. For this analysis and subsequent quantitative analyses, we grouped domain-general and hybrid procedures. Even though hybrid procedures involve some use of content knowledge, the content knowledge is used superficially; we specifically wanted to investigate the impact of weak content-knowledge use compared with strong content-knowledge use. Additionally, the number of hybrid procedures in our data set is relatively low compared with domain-general and domain-specific.
To determine whether students who used more domain-general/hybrid procedures or domain-specific procedures were more likely to have correct answers to the problems, we also used GLMM. We used the number of domain-general/hybrid procedures and the number of domain-specific procedures as our fixed factors and student as our random factor. In this analysis, our dependent variable (correct or incorrect response) was dichotomous, so we used a logistic regression ( Fitzmaurice et al. , 2011 ). We also explored the correlations between the average number of domain-general/hybrid and domain-specific procedures used by students and their final percentage of points for the course.
In this section, we present the results of our analyses of students’ procedures while solving 13 multiple-choice, biology problems ( Figure 1 and Supplemental Figure S1). We used the written think-aloud protocol to discover students’ problem-solving procedures for all 13 problems.
Students Use Domain-General and Domain-Specific Procedures to Solve Multiple-Choice Biology Problems
We identified several categories of procedures practiced by students during problem solving, and we organized these categories based on the extent to which they drew upon knowledge of biology. Domain-general procedures do not depend on biology content knowledge. These procedures also could be used in other domains. Hybrid procedures show students assessing multiple-choice options with limited and superficial references to biology content knowledge. Domain-specific procedures depend on biology content knowledge and reveal students’ retrieval and processing of correct ideas about biology.
Domain-General Procedures.
We identified five domain-general problem-solving procedures that students practiced ( Table 2 ). Three of these have been described in Bloom’s taxonomy ( Anderson and Krathwohl, 2001 ). These include Analyzing Domain-General Visual Representations, Clarifying, and Comparing Language of Options. In addition, we discovered two other procedures, Correcting and Delaying, that we also categorized as domain general ( Table 2 ).
Students’ problem-solving procedures while solving multiple-choice biology problems
The procedures are categorized as domain-general, hybrid, and domain-specific. Superscripts indicate whether the problem-solving procedure aligns with previously published conceptions of student thinking or was newly identified in this study: a , Anderson and Krathwohl (2001) ; b identified in this study; c , Crowe et al . (2008) .
During Correcting, students practiced metacognition. Broadly defined, metacognition occurs when someone knows, is aware of, or monitors his or her own learning ( White, 1998 ). When students corrected, they identified incorrect thinking they had displayed earlier in their written think-aloud and mentioned the correct way of thinking about the problem.
When students Delayed, they described their decision to postpone full consideration of one multiple-choice option until they considered other multiple-choice options. We interpreted these decisions as students either not remembering how the option connected with the question or not being able to connect that option to the question well enough to decide whether it could be the right answer.
Hybrid Procedures.
We identified two problem-solving procedures that we categorized as hybrid, Comparing Correctness of Options and Recognizing. Students who compared correctness of options stated that one choice appeared more correct than the other without giving content-supported reasoning for their choice. Similarly, students who recognized an option as correct did not support this conclusion with a content-based rationale.
Domain-Specific Procedures.
In our data set, we identified six domain-specific problem-solving procedures practiced by students ( Table 2 ). Four of these have been previously described. Specifically, Analyzing Domain-Specific Visual Representations, Checking, and Recalling were described in Bloom’s taxonomy ( Anderson and Krathwohl, 2001 ). Predicting was described by Crowe and colleagues (2008) . We identified two additional categories of domain-specific problem-solving procedures practiced by students who completed our problem set, Adding Information and Asking a Question.
Adding Information occurred when students recalled material that was pertinent to one of the multiple-choice options and incorporated that information into their explanations of why a particular option was wrong or right.
Asking a Question provides another illustration of students practicing metacognition. When students asked a question, they pointed out that they needed to know some specific piece of content that they did not know yet. Typically, students who asked a question did so repeatedly in a single written think-aloud.
Students Make Errors While Solving Multiple-Choice Biology Problems
In addition to identifying domain-general, hybrid, and domain-general procedures that supported students’ problem-solving, we identified errors in students’ problem solving. We observed six categories of errors, including four that we categorized as domain general and two categorized as domain specific ( Table 3 ).
Students’ errors while solving multiple-choice biology problems
The errors are presented in alphabetical order, described, and illustrated with example quotes from different students’ documentation of their solutions to the problem shown in Figure 1 (except for Misreading, which is from problem 13 in Supplemental Figure S1).
The domain-general errors include Contradicting, Disregarding Evidence, Misreading, and Opinion-Based Judgment. In some cases, students made statements that they later contradicted; we called this Contradicting. Disregarding Evidence occurred when students’ failed to indicate use of evidence. Several problems included data in the question prompt or in visual representations. These data could be used to help students select the best multiple-choice option, yet many students gave no indication that they considered these data. When students’ words led us to believe that they did not examine the data, we assigned the category Disregarding Evidence.
Students also misread the prompt or the multiple-choice options, and we termed this Misreading. For example, Table 3 shows the student Misreading; the student states that Atlantic eels are in the presence of krait toxins, whereas the question prompt stated there are no krait in the Atlantic Ocean. In other cases, students stated that they arrived at a decision based on a feeling or because that option just seemed right. For example, in selecting option C for the stickleback problem ( Figure 1 ), one student said, “E may be right, but I feel confident with C. I chose Answer C.” These procedures were coded as Opinion-Based Judgment.
We identified two additional errors that we classified as domain specific, Making Incorrect Assumptions and Misunderstanding Content. Making Incorrect Assumptions was identified when students made faulty assumptions about the information provided in the prompt. In these cases, students demonstrated in one part of their written think-aloud that they understood the conditions for or components of a concept. However, in another part of the written think-aloud, students assumed the presence or absence of these conditions or components without carefully examining whether they held for the given problem. In the example shown in Table 3 , the student assumed additional information on fertility that was not provided in the problem.
We classified errors that showed a poor understanding of the biology content as Misunderstanding Content. Misunderstanding Content was exhibited when students stated incorrect facts from their long-term memory, made false connections between the material presented and biology concepts, or showed gaps in their understanding of a concept. In the Misunderstanding Content example shown in Table 3 , the student did not understand that the biological species concept requires two conditions, that is, the offspring must be viable and fertile. The student selected the biological species concept based only on evidence of viability, demonstrating misunderstanding.
To illustrate the problem-solving procedures described above, we present three student written think-alouds ( Table 4, A–C ). All three think-alouds were generated in response to the stickleback problem; pseudonyms are used to protect students’ identities ( Figure 1 ). Emily correctly solved the stickleback problem using a combination of domain-general and domain-specific procedures ( Table 4A ). She started by thinking about the type of answer she was looking for (Predicting). Then she analyzed the stickleback drawings and population table (Analyzing Domain-General Visual Representations) and explained why options were incorrect or correct based on her knowledge of species concepts (Checking). Brian ( Table 4B ) took an approach that included domain-general and hybrid procedures. He also made some domain-general and domain-specific errors, which resulted in an incorrect answer; Brian analyzed some of the domain-general visual representations presented in the problem but disregarded others. He misunderstood the content, incorrectly accepting the biological species concept. He also demonstrated Recognizing when he correctly eliminated choice B without giving a rationale for this step. In our third example ( Table 4C ), Jessica used domain-general, hybrid, and domain-specific procedures, along with a domain-specific error, and arrived at an incorrect answer.
Students’ written think-alouds describing their processes for solving the stickleback problem
Different types of problem-solving processes are indicated with different font types: Domain-general problem-solving steps: blue lowercase font; domain-specific problem-solving steps: blue uppercase font, hybrid problem-solving steps: blue italics; domain-general errors: orange lowercase font; domain-specific errors: orange uppercase font. The written think-alouds are presented in the exact words of the students. A, Emily, all domain-general and domain-specific steps; correct answer: E; B, Brian, domain-general and hybrid steps, domain-general and domain-specific errors; incorrect answer: C; C, Jessica, domain-general, hybrid, and domain-specific steps; domain-specific errors; incorrect answer: C.
Domain-Specific Procedures Are Used More Frequently for Higher-Order Problems Than Lower-Order Problems
To determine the extent to which students use domain-general and domain-specific procedures when solving lower-order versus higher-order problems, we determined the frequency of domain-general and hybrid procedures and domain-specific procedures for problems categorized by experts as lower order or higher order. We grouped domain-general and hybrid procedures, because we specifically wanted to examine the difference between weak and strong content usage. As Table 5, A and B , shows, students frequently used both domain-general/hybrid and domain-specific procedures to solve all problems. For domain-general/hybrid procedures, by far the most frequently used procedure for lower-order problems was Recognizing ( n = 413); the two most frequently used procedures for higher-order problems were Analyzing Domain-General Representations ( n = 153) and Recognizing ( n = 105; Table 5A ). For domain-specific procedures, the use of Checking dominated both lower-order ( n = 903) and higher-order problems ( n = 779). Recalling also was used relatively frequently for lower-order problems ( n = 207), as were Analyzing Domain-Specific Visual Representations, Predicting, and Recalling for higher-order problems ( n = 120, n = 106, and n = 107, respectively). Overall, students used more domain-general and hybrid procedures when solving lower-order problems (1.43 ± 1.348 per problem) than when solving higher-order problems (0.74 ± 1.024 per problem; binomial regression B = 0.566, SE = 0.079, p < 0.005). Students used more domain-specific procedures when solving higher-order problems (2.57 ± 1.786 per problem) than when solving lower-order problems (2.38 ± 2.2127 per problem; binomial regression B = 0.112, SE = 0.056, p < 0.001).
Frequency of each problem-solving procedure for lower-order and higher-order problems
Procedures are presented from left to right in alphabetical order. A color scale is used to represent the frequency of each procedure, with the lowest-frequency procedures shown in dark blue, moderate-frequency procedures shown in white, and high-frequency procedures shown in dark red.
Most Problem-Solving Errors Made by Students Involve Misunderstanding Content
We also considered the frequency of problem-solving errors made by students solving lower-order and higher-order problems. As Table 6 shows, most errors were categorized with the domain-specific category Misunderstanding Content, and this occurred with about equal frequency in lower-order and higher-order problems. The other categories of errors were less frequent. Interestingly, the domain-general errors Contradicting and Opinion-Based Judgment both occurred more frequently with lower-order problems. In contrast, the domain-specific error Making Incorrect Assumptions occurred more frequently with higher-order problems.
Frequency of errors for lower-order and higher-order problems
Categories of errors are presented from left to right in alphabetical order. A color scale is used to represent the frequency of each type of error, with the lowest-frequency errors shown in dark blue, moderate-frequency errors shown in white, and high-frequency errors shown in dark red.
Using Multiple Domain-Specific Procedures Increases the Likelihood of Answering a Problem Correctly
To examine the extent to which the use of domain-general or domain-specific procedures influences the probability of answering problems correctly, we performed a logistic regression. Predicted probabilities of answering correctly are shown in Figure 3 for domain-general and hybrid procedures and Figure 4 for domain-specific procedures. Coefficients of the logistic regression analyses are presented in Supplemental Tables S2 and S3. As Figure 3 shows, using zero domain-general or hybrid procedures was associated with a 0.53 predicted probability of being correct. Using one domain-general or hybrid procedure instead of zero increased the predicted probability of correctly answering a problem to 0.79. However, students who used two or more domain-general or hybrid procedures instead of one did not increase the predicted probability of answering a problem correctly. In contrast, as Figure 4 shows, using zero domain-specific procedures was associated with only a 0.34 predicted probability of answering the problem correctly, and students who used one domain-specific procedure had a 0.54 predicted probability of success. Strikingly, the more domain-specific procedures used by students, the more likely they were to answer a problem correctly up to five procedures; students who used five domain-specific procedures had a 0.97 probability of answering correctly. Predicted probabilities for students using seven and nine domain-specific codes show large confidence intervals around the predictions due to the low sample size ( n = 8 and 4, respectively). Also, we examined the extent to which the use of domain-general or domain-specific procedures correlates with course performance. We observed a weak positive correlation between the average number of domain-specific procedures used by students for a problem and their final percentage of points in the course (Spearman’s rho = 0.306; p < 0.001). There was no correlation between the average number of domain-general/hybrid procedures used by students for a problem and their final percentage of points in the course (Spearman’s rho = 0.015; p = 0.857).

Predicted probability of a correct answer based on the number of domain-general and hybrid procedures.

Predicted probability of a correct answer based on the number of domain-specific procedures.
We have used the theoretical framework of domain-specific problem solving to investigate student cognition during problem solving of multiple-choice biology problems about ecology, evolution, and systems biology. Previously, research exploring undergraduate cognition during problem solving has focused on problem categorization or students’ solutions to open-response problems ( Smith and Good, 1984 ; Smith, 1988 ; Lavoie, 1993 ; Nehm and Ridgway, 2011 ; Smith et al. 2013 ). Our goal was to describe students’ procedural knowledge, including the errors they made in their procedures. Below we draw several important conclusions from our findings and consider the implications of this research for teaching and learning.
Domain-Specific Problem Solving Should Be Used for Innovative Investigations of Biology Problem Solving
Students in our study used a variety of procedures to solve multiple-choice biology problems, but only a few procedures were used at high frequency, such as Recognizing and Checking. Other procedures that biology educators might most want students to employ were used relatively infrequently, including Correcting and Predicting. Still other procedures that we expected to find in our data set were all but absent, such as Stating Assumptions. Our research uncovers the range of procedures promoted by multiple-choice assessment in biology. Our research also provides evidence for the notion that multiple-choice assessments are limited in their ability to prompt some of the critical types of thinking used by biologists.
We propose that our categorization scheme and the theoretical framework of domain-specific problem solving should be applied for further study of biology problem solving. Future studies could be done to understand whether different ways of asking students to solve a problem at the same Bloom’s level could stimulate students to use different procedures. For example, if the stickleback problem ( Figure 1 ) were instead presented to students as a two-tier multiple-choice problem, as multiple true–false statements, or as a constructed-response problem, how would students’ procedures differ? Additionally, it would be useful to investigate whether the more highly desired, but less often observed procedures of Correcting and Predicting are used more frequently in upper-level biology courses and among more advanced biology students.
We also propose research to study the interaction between procedure and content. With our focus on procedural knowledge, we intentionally avoided an analysis of students’ declarative knowledge. However, our process of analysis led us to the conclusion that our framework can be expanded for even more fruitful research. For example, one could look within the procedural category Checking to identify the declarative knowledge being accessed. Of all the relevant declarative knowledge for a particular problem, which pieces do students typically access and which pieces are typically overlooked? The answer to this question may tell us that, while students are using an important domain-specific procedure, they struggle to apply a particular piece of declarative knowledge. As another example, one could look within the procedural category Analyzing Visual Representations to identify aspects of the visual representation that confuse or elude students. Findings from this type of research would show us how to modify visual representations for clarity or how to scaffold instruction for improved learning. We are suggesting that future concurrent studies of declarative and procedural knowledge will reveal aspects of student cognition that will stay hidden if these two types of knowledge are studied separately. Indeed, problem-solving researchers have investigated these types of interactions in the area of comprehension of science textbooks ( Alexander and Kulikowich, 1991 , 1994 ).
Lower-Order Problems May Not Require Content Knowledge, While Higher-Order Problems Promote Strong Content Usage
Because of the pervasive use among biology educators of Bloom’s taxonomy to write and evaluate multiple-choice assessments, we decided it was valuable to examine the relationship between domain-general and domain-specific procedures and lower-order versus higher-order problems.
For both lower-order and higher-order problems, domain-specific procedures were used much more frequently than domain-general procedures ( Table 5, A and B ). This is comforting and unsurprising. We administered problems about ecosystems, evolution, and structure–function relationships, so we expected and hoped students would use their knowledge of biology to solve these problems. However, two other results strike us as particularly important. First, domain-general procedures are highly prevalent ( Table 5A , n = 1108 across all problems). The use of domain-general procedures is expected. There are certain procedures that are good practice in problem solving regardless of content, such as Analyzing Domain-General Visual Representations and Clarifying. However, students’ extensive use of other domain-general/hybrid categories, namely Recognizing, is disturbing. Here we see students doing what all biology educators who use multiple-choice assessment fear, scanning the options for one that looks right based on limited knowledge. It is even more concerning that students’ use of Recognizing is nearly four times more prevalent in lower-order problems than higher-order problems and that overall domain-general procedures are more prevalent in lower-order problems ( Table 5A ). As researchers have discovered, lower-order problems, not higher-order problems, are the type most often found in college biology courses ( Momsen et al ., 2010 ). That means biology instructors’ overreliance on lower-order assessment is likely contributing to students’ overreliance on procedures that do not require biology content knowledge.
Second, it is striking that domain-specific procedures are more prevalent among higher-order problems than lower-order problems. These data suggest that higher-order problems promote strong content usage by students. As others have argued, higher-order problems should be used in class and on exams more frequently ( Crowe et al. , 2008 ; Momsen et al. , 2010 ).
Using Domain-Specific Procedures May Improve Student Performance
Although it is interesting in and of itself to learn the procedures used by students during multiple-choice assessment, the description of these categories of procedures begs the question: does the type of procedure used by students make any difference in their ability to choose a correct answer? As explained in the Introduction , the strongest problem-solving approaches stem from a relatively complete and well-organized knowledge base within a domain ( Chase and Simon, 1973 ; Chi et al. , 1981 ; Pressley et al. , 1987 ; Alexander and Judy, 1998). Thus, we hypothesized that use of domain-specific procedures would be associated with solving problems correctly, but use of domain-general procedures would not. Indeed, our data support this hypothesis. While limited use of domain-general procedures was associated with improved probability of success in solving multiple-choice problems, students who practiced extensive domain-specific procedures almost guaranteed themselves success in multiple-choice problem solving. In addition, as students used more domain-specific procedures, there was a weak but positive increase in the course performance, while use of domain-general procedures showed no correlation to performance. These data reiterate the conclusions of prior research that successful problem solvers connect information provided within the problem to their relatively strong domain-specific knowledge ( Smith and Good, 1984 ; Pressley et al. , 1987 ). In contrast, unsuccessful problem solvers heavily depend on relatively weak domain-specific knowledge ( Smith and Good, 1984 ; Smith, 1988 ). General problem-solving procedures can be used to make some progress in reaching a solution to domain-specific problems, but a problem solver can get only so far with this type of thinking. In solving domain-specific problems, at some point, the solver has to understand the particulars of a domain to reach a legitimate solution (reviewed in Pressley et al. , 1987 ; Bassok and Novick, 2012 ). Likewise, problem solvers who misunderstand key conceptual pieces or cannot identify the deep, salient features of a problem will generate inadequate, incomplete, or faulty solutions ( Chi et al. , 1981 ; Nehm and Ridgway, 2011 ).
Our findings strengthen the conclusions of previous work in two important ways. First, we studied problems from a wider range of biology topics. Second, we studied a larger population of students, which allowed us to use both qualitative and quantitative methods.
Limitations of This Research
Think-aloud protocols typically take place in an interview setting in which students verbally articulate their thought processes while solving a problem. When students are silent, the interviewer is there to prompt them to continue thinking aloud. We modified this protocol and taught students how to write out their procedures. However, one limitation of this study and all think-aloud studies is that it is not possible to analyze what students may have been thinking but did not state. Despite this limitation, we were able to identify a range of problem-solving procedures and errors that inform teaching and learning.
Implications for Teaching and Learning
There is general consensus among biology faculty that students need to develop problem-solving skills ( NRC, 2003 ; AAAS, 2011 ). However, problem solving is not intuitive to students, and these skills typically are not explicitly taught in the classroom ( Nehm, 2010 ; Hoskinson et al. , 2013 ). One reason for this misalignment between faculty values and their teaching practice is that biology problem-solving procedures have not been clearly defined. Our research presents a categorization of problem-solving procedures that faculty can use in their teaching. Instructors can use these well-defined problem-solving procedures to help students manage their knowledge of biology; students can be taught when and how to apply knowledge and how to restructure it. This gives students the tools to become more independent problem solvers ( Nehm, 2010 ).
We envision at least three ways that faculty can encourage students to become independent problem solvers. First, faculty can model the use of problem-solving procedures described in this paper and have students write out their procedures, which makes them explicit to both the students and instructor. Second, models should focus on domain-specific procedures, because these steps improve performance. Explicit modeling of domain-specific procedures would be eye-opening for students, who tend to think that studying for recognition is sufficient, particularly for multiple-choice assessment. However, our data and those of other researchers ( Stanger-Hall, 2012 ) suggest that studying for and working through problems using strong domain-specific knowledge can improve performance, even on multiple-choice tests. Third, faculty should shift from the current predominant use of lower-order problems ( Momsen et al. , 2010 ) toward the use of more higher-order problems. Our data show that lower-order problems prompt for domain-general problem solving, while higher-order problems prompt for domain-specific problem solving.
We took what we learned from the investigation reported here and applied it to develop an online tutorial called SOLVEIT for undergraduate biology students ( Kim et al. , 2015 ). In SOLVEIT, students are presented with problems similar to the stickleback problem shown in Figure 1 . The problems focus on species concepts and ecological relationships. In brief, SOLVEIT asks students to provide an initial solution to each problem, and then it guides students through the problem in a step-by-step manner that encourages them to practice several of the problem-solving procedures reported here, such as Recalling, Checking, Analyzing Visual Representations, and Correcting. In the final stages of SOLVEIT, students are asked to revise their initial solutions and to reflect on an expert’s solution as well as their own problem-solving process ( Kim et al. , 2015 ). Our findings of improved student learning with SOLVEIT ( Kim et al. , 2015 ) are consistent with the research of others that shows scaffolding can improve student problem solving ( Lin and Lehman, 1999 ; Belland, 2010 ; Singh and Haileselassie, 2010 ). Thus, research to uncover the difficulties of students during problem solving can be directly applied to improve student learning.
Supplementary Material
Acknowledgments.
We thank the students who participated in this study and the biology faculty who served as experts by providing Bloom’s rankings for each problem. We also thank the Biology Education Research Group at UGA, who improved the quality of this work with critical feedback on the manuscript. Finally, we thank the reviewers, whose feedback greatly improved the manuscript. Resources for this research were provided by UGA and the UGA Office of STEM Education.
- Alexander PA, Judy JE. The interaction of domain-specific and strategic knowledge and academic performance. Rev Educ Res. 1988; 58 :375–404. [ Google Scholar ]
- Alexander PA, Kulikowich JM. Domain-specific and strategic knowledge as predictors of expository text comprehension. J Reading Behav. 1991; 23 :165–190. [ Google Scholar ]
- Alexander PA, Kulikowich JM. Learning from physics text: a synthesis of recent research. J Res Sci Teach. 1994; 31 :895–911. [ Google Scholar ]
- American Association for the Advancement of Science. Vision and Change in Undergraduate Biology Education: A Call to Action. Washington, DC: 2011. [ Google Scholar ]
- Anderson C, Sheldon TH, Dubay J. The effects of instruction on college non-majors’ conceptions of respiration and photosynthesis. J Res Sci Teach. 1990; 27 :761–776. [ Google Scholar ]
- Anderson LW, Krathwohl DR. A Taxonomy for Learning, Teaching, and Assessing: A Revision of Bloom’s Taxonomy of Educational Objectives. Boston, MA: Allyn & Bacon; 2001. [ Google Scholar ]
- Anderson TR, Schönborn KJ, du Plessis L, Gupthar AS, Hull TL. Identifying and developing students’ ability to reason with concepts and representations in biology. In: Treagust DF, Tsui C, editors. Multiple Representations in Biological Education, vol. 7. Dordrecht, Netherlands: Springer; 2012. pp. 19–38. [ Google Scholar ]
- Bassok M, Novick LR. Problem solving. In: Holyoak KJ, Morrison RG, editors. Oxford Handbook of Thinking and Reasoning. New York: Oxford University Press; 2012. pp. 413–432. [ Google Scholar ]
- Belland BR. Portraits of middle school students constructing evidence-based arguments during problem-based learning: the impact of computer-based scaffolds. Educ Technol Res Dev. 2010; 58 :285–309. [ Google Scholar ]
- Bissell AN, Lemons PP. A new method for assessing critical thinking in the classroom. BioScience. 2006; 56 :66–72. [ Google Scholar ]
- Bloom BS. Taxonomy of Educational Objectives: The Classification of Educational Goals. New York: McKay; 1956. [ Google Scholar ]
- Brown AL. Knowing when, where, and how to remember: a problem of metacognition. In: Glaser R, editor. Advances in Instructional Psychology, vol. 1. Hillsdale, NJ: Erlbaum; 1978. pp. 77–165. [ Google Scholar ]
- Brownell SE, Wenderoth MP, Theobald R, Okoroafor N, Koval M, Freeman S, Walcher-Chevillet CL, Crowe AJ. How students think about experimental design: novel conceptions revealed by in-class activities. BioScience. 2014; 64 :125–137. [ Google Scholar ]
- Bunce DM, Gabel DL, Samuel JV. Enhancing chemistry problem-solving achievement using problem categorization. J Res Sci Teach. 1991; 28 :505–521. [ Google Scholar ]
- Cartrette DP, Bodner GM. Non-mathematical problem solving in organic chemistry. J Res Sci Teach. 2010; 47 :643–660. [ Google Scholar ]
- Chase WG, Simon HA. The mind’s eye in chess. In: Chase WG, editor. Visual Information Processing. New York: Academic; 1973. pp. 115–181. [ Google Scholar ]
- Chi MTH, Feltovich PJ, Glaser R. Categorization and representation of physics problems by experts and novices. Cogn Sci. 1981; 5 :121–152. [ Google Scholar ]
- Chi MTH, Glaser R. Problem-solving ability. In: Sternberg RJ, editor. Human Abilities: An Information-Processing Approach. New York: Freeman; 1985. [ Google Scholar ]
- Crowe A, Dirks C, Wenderoth MP. Biology in Bloom: implementing Bloom’s taxonomy to enhance student learning in biology. CBE Life Sci Educ. 2008; 7 :368–381. [ PMC free article ] [ PubMed ] [ Google Scholar ]
- Dawson MRW. Understanding Cognitive Science, 1st ed. Malden, MA: Wiley-Blackwell; 1998. [ Google Scholar ]
- Dirks C, Leroy C, Wenderoth MP. Science Process and Reasoning Skills Test (SPARST): development and early diagnostic results. 2013. Presented at the Society for the Advancement of Biology Education Research (SABER) annual meeting, held 11–14 July 2013, in Minneapolis, MN.
- Duncker K, Lees LS. On problem-solving. Psychol Monogr. 1945; 58 :i–113. [ Google Scholar ]
- Ericsson KA, Simon HA. Protocol Analysis: Verbal Reports as Data, rev. ed. Cambridge, MA: MIT Press; 1984. [ Google Scholar ]
- Fitzmaurice GM, Laird NM, Ware JH. Applied Longitudinal Analysis, 2nd ed. Hoboken, NJ: Wiley; 2011. [ Google Scholar ]
- Gormally C, Brickman P, Lutz M. Developing a test of scientific literacy skills (TOSLS): measuring undergraduates’ evaluations of scientific information and arguments. CBE Life Sci Educ. 2012; 11 :364–377. [ PMC free article ] [ PubMed ] [ Google Scholar ]
- Halpern DE. Critical Thinking across the Curriculum: A Brief Edition of Thought and Knowledge. Mahwah, NJ: Erlbaum; 1997. [ Google Scholar ]
- Hartley LM, Wilke BJ, Schramm JW, D’Avanzo C, Anderson CW. College students’ understanding of the carbon cycle: contrasting principle-based and informal reasoning. BioScience. 2011; 61 :65–75. [ Google Scholar ]
- Hoskinson A-M, Caballero MD, Knight JK. How can we improve problem solving in undergraduate biology? Applying lessons from 30 years of physics education research. CBE Life Sci Educ. 2013; 12 :153–161. [ PMC free article ] [ PubMed ] [ Google Scholar ]
- Jacobs JE, Paris SG. Children’s metacognition about reading—issues in definition, measurement, and instruction. Educ Psychol. 1987; 22 :255–278. [ Google Scholar ]
- Jonassen D. In: Trends and Issues in Instructional Design and Technology. 3rd ed., ed. RA Reiser and JV Dempsey, Boston, MA: Pearson Education; 2012. Designing for problem solving; pp. 64–74. [ Google Scholar ]
- Jonassen DH. Toward a design theory of problem solving. Educ Technol Res Dev. 2000; 48 :63–85. [ Google Scholar ]
- Keys CW. Investigating the thinking processes of eighth grade writers during the composition of a scientific laboratory report. J Res Sci Teach. 2000; 37 :676–690. [ Google Scholar ]
- Kim HS, Prevost L, Lemons PP. Students’ usability evaluation of a Web-based tutorial program for college biology problem solving. J Comput Assist Learn. 2015; 31 :362–377. [ Google Scholar ]
- Kohl PB, Finkelstein ND. Patterns of multiple representation use by experts and novices during physics problem solving. Phys Rev Spec Top Phys Educ Res. 2008; 4 :010111. [ Google Scholar ]
- Lavoie DR. The development, theory, and application of a cognitive-network model of prediction problem solving in biology. J Res Sci Teach. 1993; 30 :767–785. [ Google Scholar ]
- Lin X, Lehman JD. Supporting learning of variable control in a computer-based biology environment: effects of prompting college students to reflect on their own thinking. J Res Sci Teach. 1999; 36 :837–858. [ Google Scholar ]
- Martinez ME. What is problem solving. Phi Delta Kappan. 1998; 79 :605–609. [ Google Scholar ]
- Momsen J, Offerdahl E, Kryjevskaia M, Montplaisir L, Anderson E, Grosz N. Using assessments to investigate and compare the nature of learning in undergraduate science courses. CBE Life Sci Educ. 2013; 12 :239–249. [ PMC free article ] [ PubMed ] [ Google Scholar ]
- Momsen JL, Long TM, Wyse SA, Ebert-May D. Just the facts? Introductory undergraduate biology courses focus on low-level cognitive skills. CBE Life Sci Educ. 2010; 9 :435–440. [ PMC free article ] [ PubMed ] [ Google Scholar ]
- National Research Council. BIO2010: Transforming Undergraduate Education for Future Research Biologists. Washington, DC: National Academies Press; 2003. [ PubMed ] [ Google Scholar ]
- Nehm RH. Understanding undergraduates’ problem-solving processes. J Microbiol Biol Educ. 2010; 11 :119–122. [ PMC free article ] [ PubMed ] [ Google Scholar ]
- Nehm RH, Reilly L. Biology majors’ knowledge and misconceptions of natural selection. BioScience. 2007; 57 :263–272. [ Google Scholar ]
- Nehm RH, Ridgway J. What do experts and novices “see” in evolutionary problems. Evol Educ Outreach. 2011; 4 :666–679. [ Google Scholar ]
- Newell A, Shaw JC, Simon HA. Elements of a theory of human problem solving. Psychol Rev. 1958; 65 :151–166. [ Google Scholar ]
- Newell A, Simon HA. Human Problem Solving. Upper Saddle River, NJ: Prentice-Hall; 1972. [ Google Scholar ]
- Patton MQ. Qualitative Evaluation and Research Methods. Thousand Oaks, CA: Sage; 1990. [ Google Scholar ]
- Polya G. How to Solve It. Garden City, NY: Doubleday; 1957. [ Google Scholar ]
- Pressley M, Borkowski JG, Schneider W. Cognitive strategies: good strategy users coordinate metacognition and knowledge. Ann Child Dev. 1987; 4 :89–129. [ Google Scholar ]
- Pressley M, Goodchild F, Fleet J, Zajchowski R, Evans ED. The challenges of classroom strategy instruction. Elem Sch J. 1989; 89 :301–342. [ Google Scholar ]
- Runco MA, Chand I. Cognition and creativity. Educ Psychol Rev. 1995; 7 :243–267. [ Google Scholar ]
- Schraw G, Moshman D. Metacognitive theories. Educ Psychol Rev. 1995; 7 :351–371. [ Google Scholar ]
- Singer SR, Nielsen NR, Schweingburger HA, Committee on the Status, Contributions, and Future Directions of Discipline-Based Education Research . Discipline-Based Education Research: Understanding and Improving Learning in Undergraduate Science and Engineering. Washington, DC: National Academies Press; 2012. [ Google Scholar ]
- Singh C, Haileselassie D. Developing problem-solving skills of students taking introductory physics via Web-based tutorials. J Coll Sci Teach. 2010; 39 :42–49. [ Google Scholar ]
- Smith JI, Combs ED, Nagami PH, Alto VM, Goh HG, Gourdet MA, Hough CM, Nickell AE, Peer AG, Coley JD, et al. Development of the biology card sorting task to measure conceptual expertise in biology. CBE Life Sci Educ. 2013; 12 :628–644. [ PMC free article ] [ PubMed ] [ Google Scholar ]
- Smith MU. Successful and unsuccessful problem solving in classical genetic pedigrees. J Res Sci Teach. 1988; 25 :411–433. [ Google Scholar ]
- Smith MU. Expertise and the organization of knowledge: unexpected differences among genetic counselors, faculty, and students on problem categorization tasks. J Res Sci Teach. 1992; 29 :179–205. [ Google Scholar ]
- Smith MU, Good R. Problem solving and classical genetics: successful versus unsuccessful performance. J Res Sci Teach. 1984; 21 :895–912. [ Google Scholar ]
- Stanger-Hall KF. Multiple-choice exams: an obstacle for higher-level thinking in introductory science classes. CBE Life Sci Educ. 2012; 11 :294–306. [ PMC free article ] [ PubMed ] [ Google Scholar ]
- White RT. Decisions and problems in research on metacognition. In: Fraser B, Tobin KG, editors. International Handbook of Science Education. Dordrecht, Netherlands: Springer; 1998. pp. 1207–1213. [ Google Scholar ]
- Zheng AY, Lawhorn JK, Lumley T, Freeman S. Application of Bloom’s taxonomy debunks the “MCAT myth.” Science. 2008; 319 :414–415. [ PubMed ] [ Google Scholar ]
- Zoller U. Are lecture and learning compatible? Maybe for LOCS: unlikely for HOCS. J Chem Educ. 1993; 70 :195–197. [ Google Scholar ]
MATH3397 - Mathematical Problem Solving
MATH 3397 Mathematical Problem Solving (3 semester credit hours) Problem solving techniques including graphs, combinatorial techniques, induction, number theory, geometry and symmetry and heuristics. Students will learn to maintain good control of the problem-solving process, and will gain proficiency in presenting solutions in both oral and written form. Prerequisite: MATH 2413 or MATH 2417 or MATH 3321 . (3-0) Y
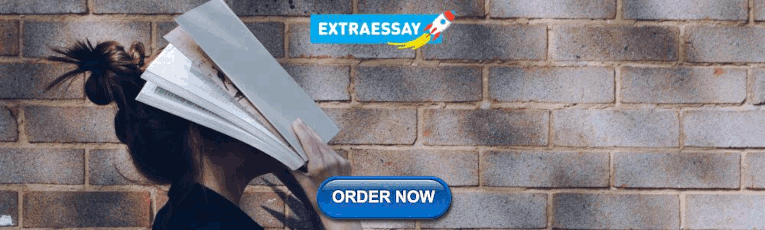
IMAGES
VIDEO
COMMENTS
The scientific method. At the core of biology and other sciences lies a problem-solving approach called the scientific method. The scientific method has five basic steps, plus one feedback step: Make an observation. Ask a question. Form a hypothesis, or testable explanation. Make a prediction based on the hypothesis.
A critical part of our job as teachers is crafting good problems. We aim to create problems that have the following characteristics: Rooted in problem solving. A good problem should challenge students to think and to apply their knowledge in novel ways. Clearly written and easily understandable. The point of the problem should be clear.
1.3: Problem Solving is shared under a not declared license and was authored, remixed, and/or curated by LibreTexts. The ability to solve problems is a skill like any other. It is NOT an innate (i.e. you've either got it or you don't) aptitude. Problem solving can be broken down into a set of skills ….
This study uses the theoretical framework of domain-specific problem solving to explore the procedures students use to solve multiple-choice problems about biology concepts. We designed several multiple-choice problems and administered them on four exams. We trained students to produce written descriptions of how they solved the problem, and this allowed us to systematically investigate their ...
Problem-based Learning in Biologywith 20 Case Examples. Problem-based Learning in Biology. Problem-based learning (PBL) is an exciting way to learn biology and is readily incorporated into large classes in a lecture hall environment. PBL engages students in solving authentic biological case problems, stimulating discussion among students and ...
chrome_reader_mode Enter Reader Mode ... { }
Problem-solving in biology teaching involves introducing students to problems and encouraging them to solve them. It can be a stimulating environment of learning in which students take risks, draw on prior knowledge and experience, discover new knowledge and test ideas (Etherington, 2011). In this article, the implementation of problem-solving ...
The phrase "problem solving" appears regularly in the biology education research (BER) literature, and there is near-universal agreement that problem solving is a valuable skill for biology students to learn and practice (AAAS, 2011; NAS, 2011). Research about defining relevant biology problems, how students solve biology problems, and what ...
We investigate one key scientific practice that is understudied in biology education, problem solving (AAAS, 2011; Singer et al., 2012). For the purposes of this article, we define problem solving as a decision-making process wherein a person is presented with a task, and the path to solving the task is uncertain.
This study uses the theoretical framework of domain-specific problem solving to explore the procedures students use to solve multiple-choice problems about biology concepts. We designed several multiple-choice problems and administered them on four exams. We trained students to produce written descriptions of how they solved the problem, and ...
Problem Solving. Educators and employers alike have all argued strongly in recent years that the ability to solve problems is one of the most important skills that should be taught to and nurtured in university students. Problem solving ability consistently ranks as one of the most sought after traits employers want from their hires.
This video focuses on the problem-solving skills needed for National 5 Biology. It supports learners to have a clear understanding of key problem-solving ski...
You can access the full National 5 Problem Solving Booklet below: National 5 Problem Solving Booklet Questions. National 5 Problem Solving Booklet Marking Scheme. You can access problem solving questions by category using the links below: Biological Keys Questions | Biological Keys Marking Scheme. Calculation Questions | Calculations Marking ...
Existing research has investigated student problem-solving strategies across science, technology, engineering, and mathematics; however, there is limited work in undergraduate biology education on how various aspects that influence learning combine to generate holistic approaches to problem solving. Through the lens of situated cognition, we consider problem solving as a learning phenomenon ...
The purpose of this study was to examine the effects of using problem-based module (PBM) in the subject of Biology on high school students' problem-solving skill and achievement.
Biology problem solving. 6 terms. b3cca8. N5 Biology: Problem solving tips. 19 terms. Mr_Houston25 Teacher. Nat 5 Biology Unit 1. 100 terms. anissaleh Teacher. MES - S4 French (Environment) 45 terms. MrsMcBratney Teacher. Sets found in the same folder. National 5 Biology: EVERYTHING! 244 terms. Images.
Problem-solving has been acknowledged as one of the compulsory skills needed to face and overcome challenges of the modern world in either learning or everyday life. However, there is limited information regarding the level of problem-solving skills demonstrated by school students in learning biology subject compared to physics and mathematics.
Problem Solving. Problem-Solving in Conservation Biology and Wildlife Management: Exercises for Class, Field, and Laboratory (2nd Edition) Supporting materials (data sets, images, maps, and important websites) Note: components of first edition website are still available: Guidance and resources document and the Java applets: These three ...
National 5 Biology Revision. Welcome to your interactive National 5 Biology Revision site. Here you will find past papers and marking instructions, summary notes, problem solving activities and much more. Each section has a link to BBC Bitesize, a selection of past paper questions and a youtube video. Unit One.
Calculating an average: An average is the result that you get when you add two or more numbers together and divide the total by the number of numbers you added together. Add (total) all of the numbers in the set you want to average. Count how many individual values are in the set of numbers. Divide step 1 by step 2.
Globular & Fibrous Proteins. Download File. Key Area 4 Cellular Differentiation. Download File. Key Area 5 Structure of the Genome.
The results showed that the percentage of students' problem-solving skills was 30.67% in the lack category, and the ability to solve problems can be improved by innovating teachers in choosing learning strategies such as using approaches, methods, models, and media that can improve problem-Solving abilities, especially in learning biology. Expand.
Predicting protein structures and the way they interact with other molecules has been one of the biggest problems in biology. Yet, AI developer Google DeepMind has gone some way to solving it in ...
Solving the problems of proton-conducting perovskites for next-generation fuel cells. ScienceDaily . Retrieved May 31, 2024 from www.sciencedaily.com / releases / 2024 / 05 / 240529144022.htm
A: a table B: an equation C: a tree or chain D: a matrix PrOACT Model Problem Objectives Alternatives Consequences Tradeoffs Seven Steps of Problem Solving 1. Define the problem 2. Disaggregating the problem (develop the hypothesis) 3. Prioritize what to do (and what not to do) 4. Create a work plan 5.
Start with trust and end with speed. May 22, 2024. When it comes to solving complicated problems, the default for many organizational leaders is to take their time to work through the issues at hand.
Domain-Specific Problem Solving Should Be Used for Innovative Investigations of Biology Problem Solving. Students in our study used a variety of procedures to solve multiple-choice biology problems, but only a few procedures were used at high frequency, such as Recognizing and Checking. Other procedures that biology educators might most want ...
MATH3397 - Mathematical Problem Solving. MATH 3397 Mathematical Problem Solving (3 semester credit hours) Problem solving techniques including graphs, combinatorial techniques, induction, number theory, geometry and symmetry and heuristics. Students will learn to maintain good control of the problem-solving process, and will gain proficiency in presenting solutions in both oral and written form.
Get a three-pack from Amazon for $12.99. 3. A magnetic towel since the last thing you wanna do after a tough workout at the gym is wipe your face with a towel that's been sitting on the dirty ...
Aiming at the problem of non-initially specifying a fixed single starting point for the Multiple Travelling Salesman Problem and the longest path for a traveling salesman in a closed loop and the shortest total path, this paper combines the fireworks algorithm with the uniparental genetic algorithm, invokes the idea of uniparental genetic algorithm to improve the explosion strategy in the ...