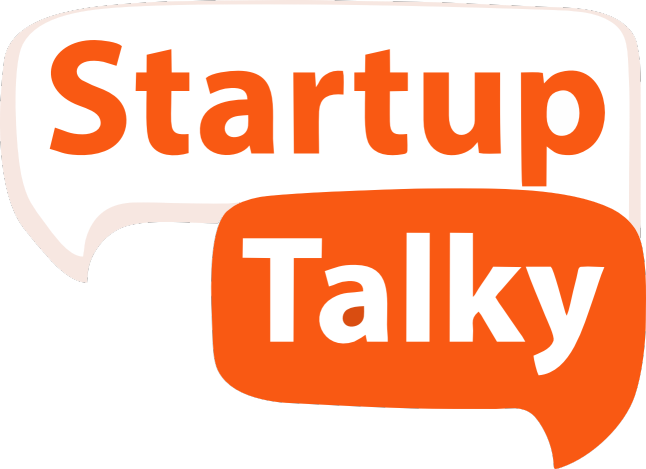
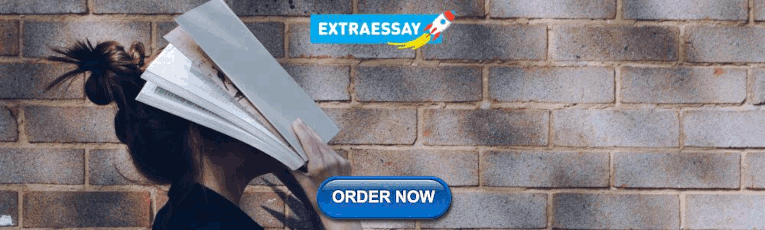
Netflix Recommendation Engine - How Netflix uses Big data and Analytics to Recommend you your Favourite Shows
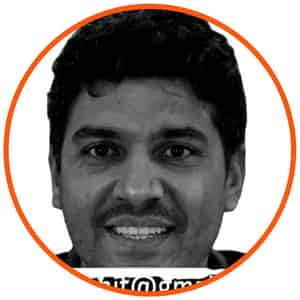
Harshit Verma
Entertainment is probably as old as the era of humans itself. We have found out different ways of getting entertained. Some of the sources include dance, singing, playing but some of the most famous and widely accepted ways of entertainment are films, theatrics and movies.
In this 21st century, as the internet penetrates every domain, it has not left the entertainment sector per se. It has boosted the domain to such heights that it is probably hard to go back to square one. The topmost entertainment provider in the world is Netflix. It uses technology to scale great heights and great revenues.
There was an old film with dialogue where the protagonist says “A film works only when it has three elements to it, Entertainment, Entertainment and Entertainment”. Well, we as viewers might be tempted to say yes it is true but is it still the same in the twenty-first century? The answer may be a little more than just entertainment. It might include promotions, marketing and more. What more you ask? Big data, Artificial intelligence, machine learning.
Netflix, the prime entertainment host, do it all to cater to your entertainment needs. We will dive deep to understand how Netflix uses its recommendation engine and how it has incorporated this super-tech to reach new heights.
A little preface about Netflix Personalised Entertainment/Content on Netflix Data Analytics of Netflix The Recommendation Engine of Netflix Business Verticals of Netflix Some Facts about Netflix that might Interest you FAQ
A little Preface about Netflix
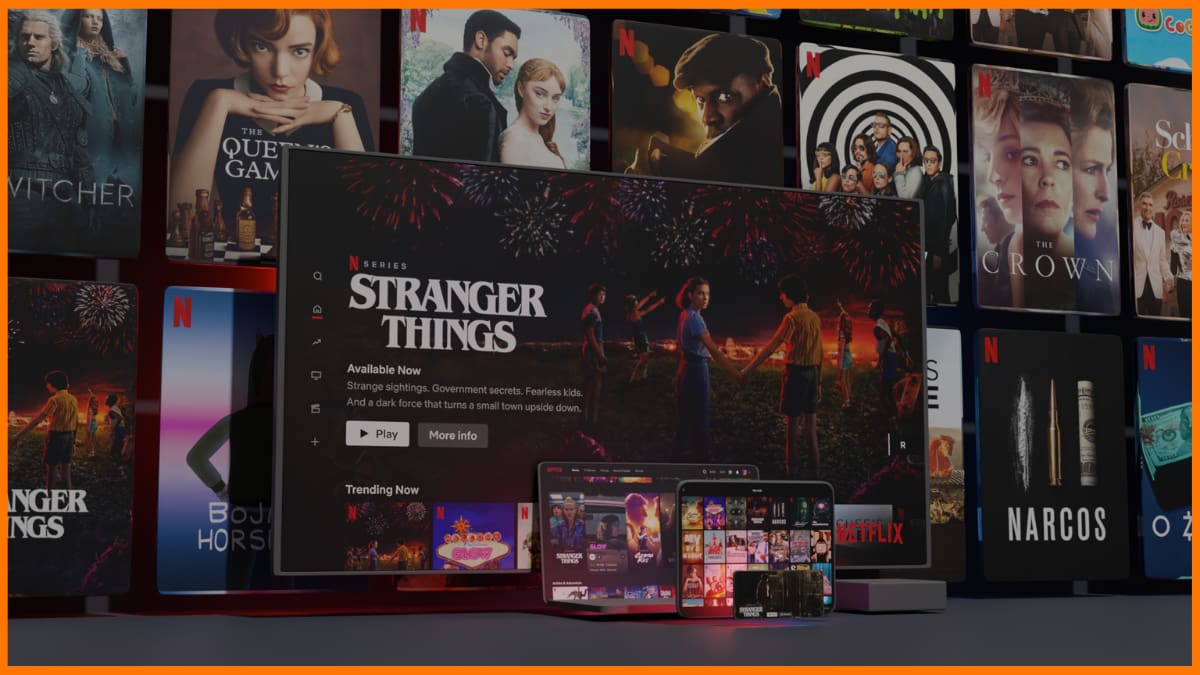
Netflix is a streaming service that offers a wide variety of movies, TV series, shows, anime, documentaries, and more. As mentioned, it is a streaming service, so it can be accessed on every possible device. You can stream Netflix via the official website, or its android or IOS app.
You can tune into it instantly on the web at netflix.com from your personal computer or on any internet-connected device that offers the Netflix app, including smart TVs, smartphones, tablets, streaming media players and game consoles . It is a monthly subscribed service, which you have to redeem monthly.
There is always something to watch on Netflix. So much so that it has a full library of entertainment. It is extensively built for the best experience in entertainment to its subscribers. That is why Netflix is the most famous streaming platform in the world.
You might wonder that entertainment is top-notch on Netflix but there is one more thing that it pays huge attention to. The thing is not hideous but is often not much talked about. That one aspect is the library and the whole management of this extensively built personalised library of content.
Netflix, for years, is able to provide personalised content recommendations to its each and every subscriber. How does it do that? What is the magic behind it? let us uncover that.
Personalised Entertainment/Content on Netflix
“If the Starbucks secret is a smile when you get your latte… ours is that the Web site adapts to the individual’s taste.” - Reed Hastings (CEO of Netflix)
Over the course of the last few years, Netflix has become the favourite destination of people who want to binge on some entertainment films and shows. Netflix started as a humble DVD rental business and it later turned into something totally different as technology kicked in.
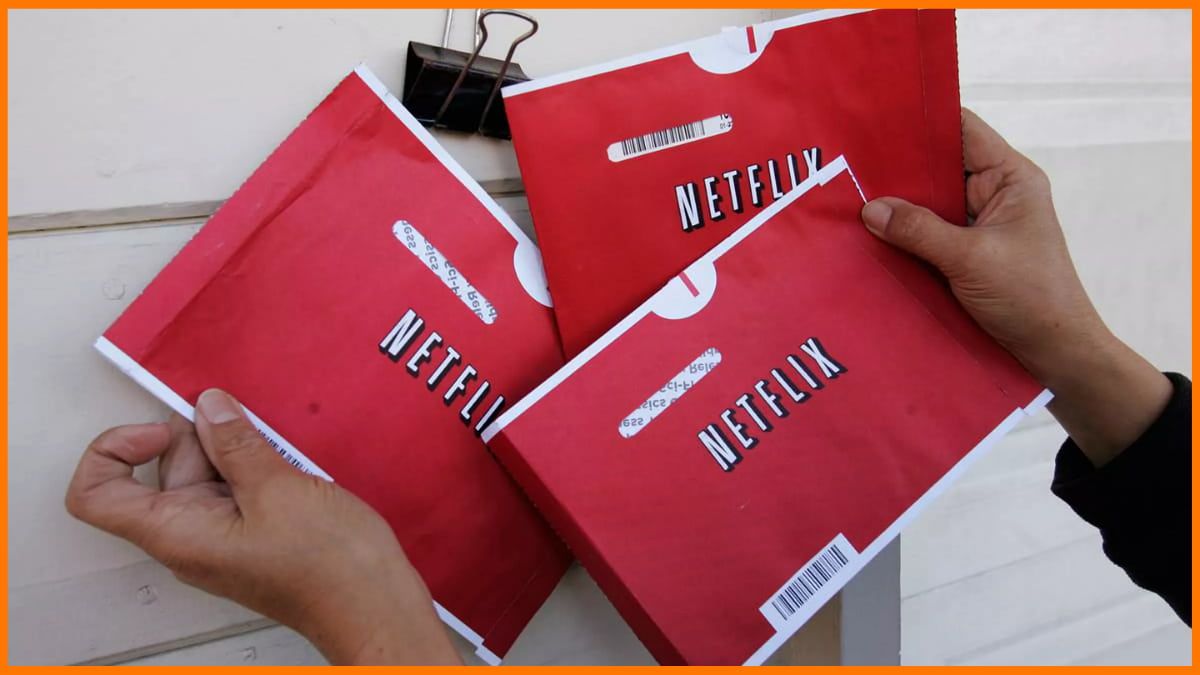
We can see the huge subscriber base of Netflix as proof of work and growth. One of the most crucial elements of this growth is personalised content. That crucial element is the underlying asset of the presence of Big Data and artificial intelligence.
Netflix doesn't just work in managing content, movies, TV shows and entertainment but it has a lot of other data to handle as well. It has user insights, their data and usage patterns and everything connected to them and of course ‘us’.
The data management part is not easy at all, especially when you have to constantly change to adapt to your surroundings. Netflix does it so well, no wonder it uses Big data to manage and make sense of huge piles of useful data.
“Where there is data smoke, there is business fire.” — Thomas Redman
If we see the graph of Netflix's memberships and subscriptions, we can see a beautiful upward direction to the moon. The reason is its personalised services and the best user interface that is available out in the whole world.
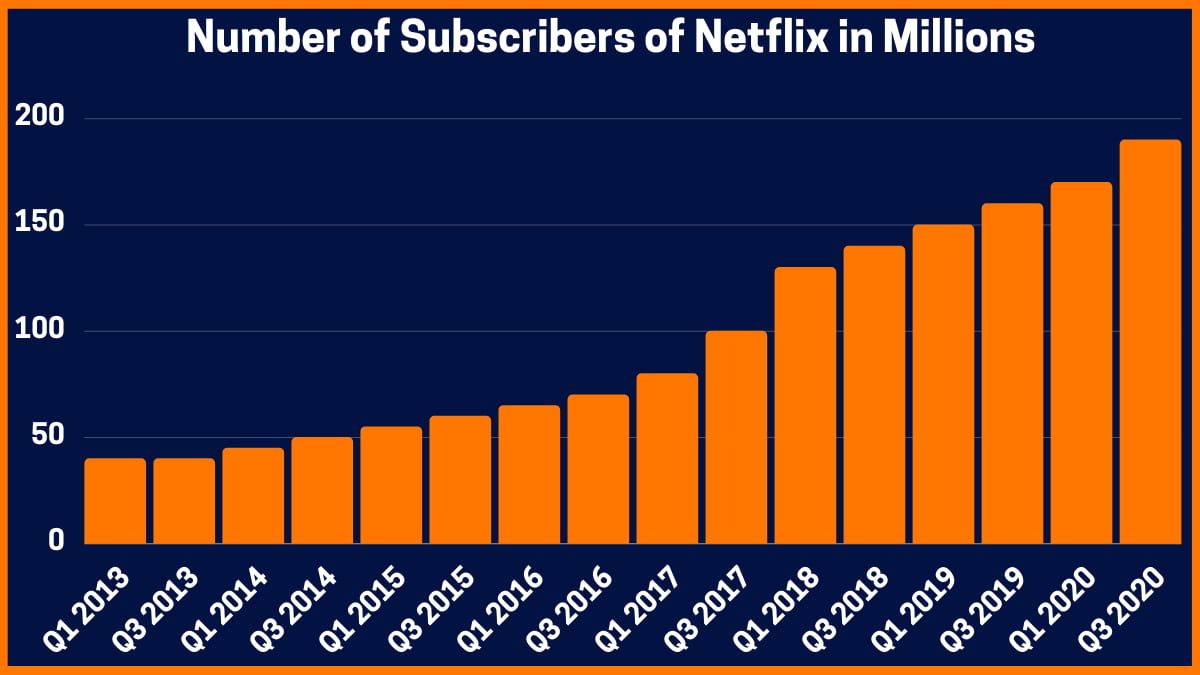
The revenue of this streaming giant is also similar to that of its subscriber base. It has grown steeply and steadily. The reason is the efficiency undoubtedly.
When it first started as a DVD rental service, it was a quite simple video provider. It used to use mails to provide DVD copies of the content. It was in 2010 when Netflix thought of rebranding and using more sophisticated technology as an aid. They began streaming online and the data that they were collecting grew many folds. This marks many years of anniversary for Netflix as a data-driven company. It has been data-driven even from its very inception.
Their “Data Analytics' team work very closely with decision-makers of the company. The data team has useful insights, metrics, predictions and analytics so that everyone can work efficiently. They have to work super closely with the product teams, content teams, studio and marketing teams and altogether with the business operations.
With the data they collect, they have to perform context-rich analysis to provide insight into their business, partners and of course their subscribers or members. This also enriches the experience for Netflix.

Data Analytics of Netflix
When you are dealing with huge amounts of data then efficient data management becomes the reason and a necessary condition for your success. At Netflix, data analytics is the backbone of every work that they do. It is the metric at which they measure their location. It is the basis to know where they are and essentially where they are going. This is where Netflix finds and experiments, it is also the place where they solve existing problems.
Even from the DVD days, they are a data-driven company first and then anything else. From its inception, they have grown their data department to new heights every now and then.
As Netflix grew, the need to manage data effectively and efficiently grew too. Every decision is fueled by the data behind it. If you are into any business in the world, you need data to do your best possible job. Netflix does it and it does it quite efficiently.
Data Science and Engineering at Netflix is primarily and supremely is directed at improving various aspects of the streaming business. Among all the other roles, research applications span many areas including Netflix's personalization algorithms, content valuation, and optimization for future streaming.
To maximise the already big impact of Netflix’s research, they do not centralise research into a separate organisation. Instead, they do it within altogether other departments. They have many teams that pursue research in collaboration with business teams , engineering teams, and other researchers. This enables closer partnerships between researchers and the business and engineering in each and every area.
In addition to that, research that applies to the same methodological area or business area is shared and highlighted in discussion and debate forums to strengthen the work and its impact.
When we think about big data and Netflix, what comes to mind? More than often you would think that it has something to do with the content recommendation algorithm or the streaming to your personal device. Yes, you are right in most senses, these two topics are the main contributors for data research and analytics but there is more.
They both are an integral part but there is more to the whole picture. So, further data is used to “make the experience even better than before”. Data has to do a lot with questions like “Which piece of content makes our customers or members most joyous” or “What are some of the areas in which Netflix can collaborate to provide 360-degree entertainment”.
Data solves the problem of finding the right market fit for the product in any sort of market. Which in turn enhances the user experience of Netflix as a whole.
The Recommendation Engine of Netflix
As we discussed previously, data is fuel for Netflix's smoothness and convenience. The motive is to constantly improve the predictions on how someone is going to react after watching a certain type of movie, genre and another basis. This helps in knowing about the customer preferences, which can be used in future for making better predictions.
This is when their recommendation algorithm comes into the picture. Netflix has, over the years, designed an algorithm that can suggest recommendations to its users. It is called the Netflix recommendation Engine or NRE. it has been reported that 80% of Netflix viewer activity is driven by personalised recommendations from the engine. Which is a pretty good number for a streaming platform like Netflix. It also saves marketing costs for the streaming giant.
In Netflix’s case, the NRE or the Netflix recommendation engine has some different factors of inputs. It collects data that will be the most relevant in the prediction of user behaviours. Some of the most commonly tracked inputs are as follows,
- The device used to stream on.
- The number of searches.
- If the show was paused or fast-forwarded.
- Whether the entire show/movie was completed watching.
- Whether the viewer gave the show or movie a thumbs up or thumbs down.
- Scenes that the user replayed.
- Time and date at user watched a show/movie.
- Profile information such as age, gender, location.
These are some most used inputs that Netflix recommendation engines use. Moreover, of all the websites that use big data and other predicting technologies, Netflix does it the smoothest. It has been reported that 47% of North Americans prefer to use Netflix with an exclamatory 93 % retention basis . This marks proof of the efficient working of the Netflix model.
Nevertheless, Netflix is not just winning because of its near-perfect prediction and recommendation technology but also good management. Let us know a little about the business verticals at the heart of this streaming giant.
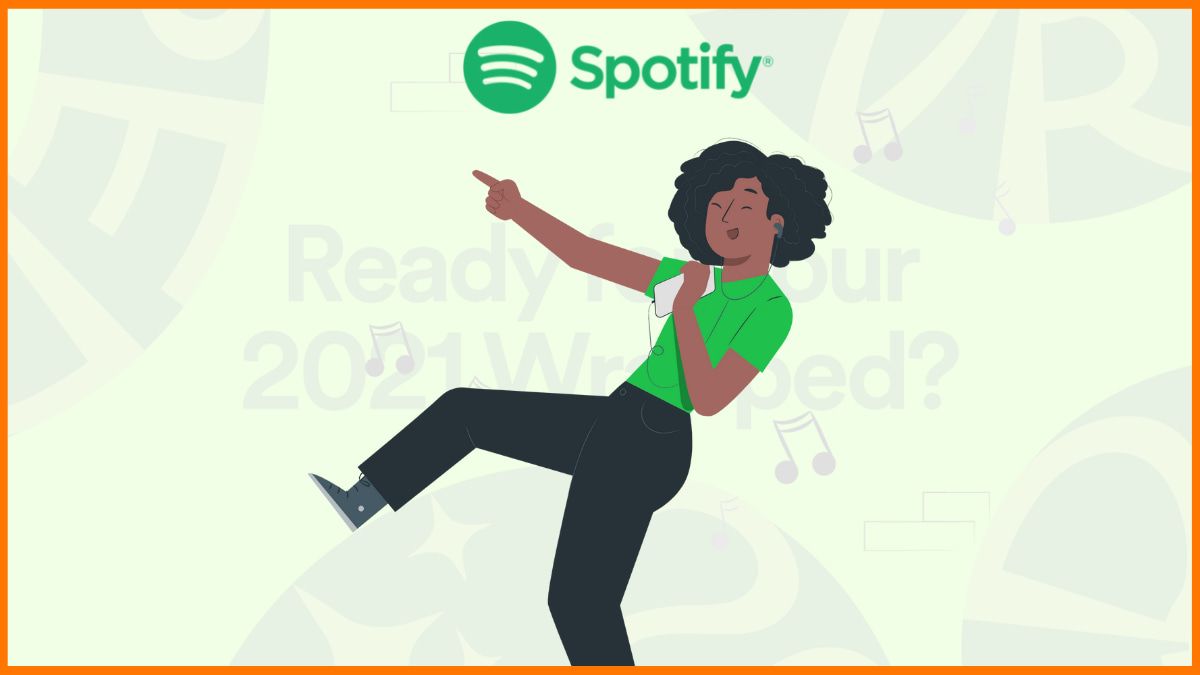
Business Verticals of Netflix
What you see is the content and recommendations, well stacked on Netflix, what you do not see is the work that goes behind curtains. There are business verticals/segments that work as a team to improve how we binge-watch content online. Let us read about them in brief words.
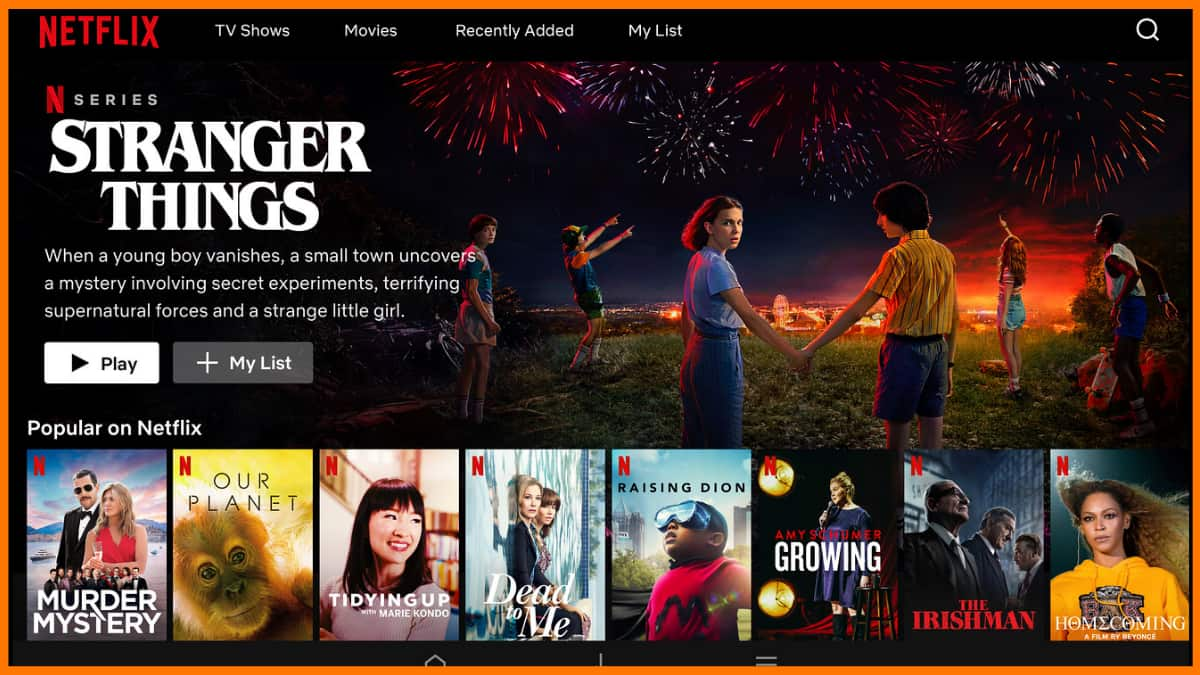
Product is the actual product that the streaming giant is providing. It is the segment that deals with the Netflix app. The motive of this department or business segment is to deliver high-quality streaming, smooth user interface , best customer service. The product segment also has to ensure that the members get the right content recommendations at the right time.
The content segment is the cream for the cake. At the heart of Netflix, it also is a content producing company. The content vertical is accountable and responsible for licensing and enabling shows and movies for Netflix. This department also works on all things that can be joyous to the public. Buying decisions at this and all other levels are done by this area of the business vertical.
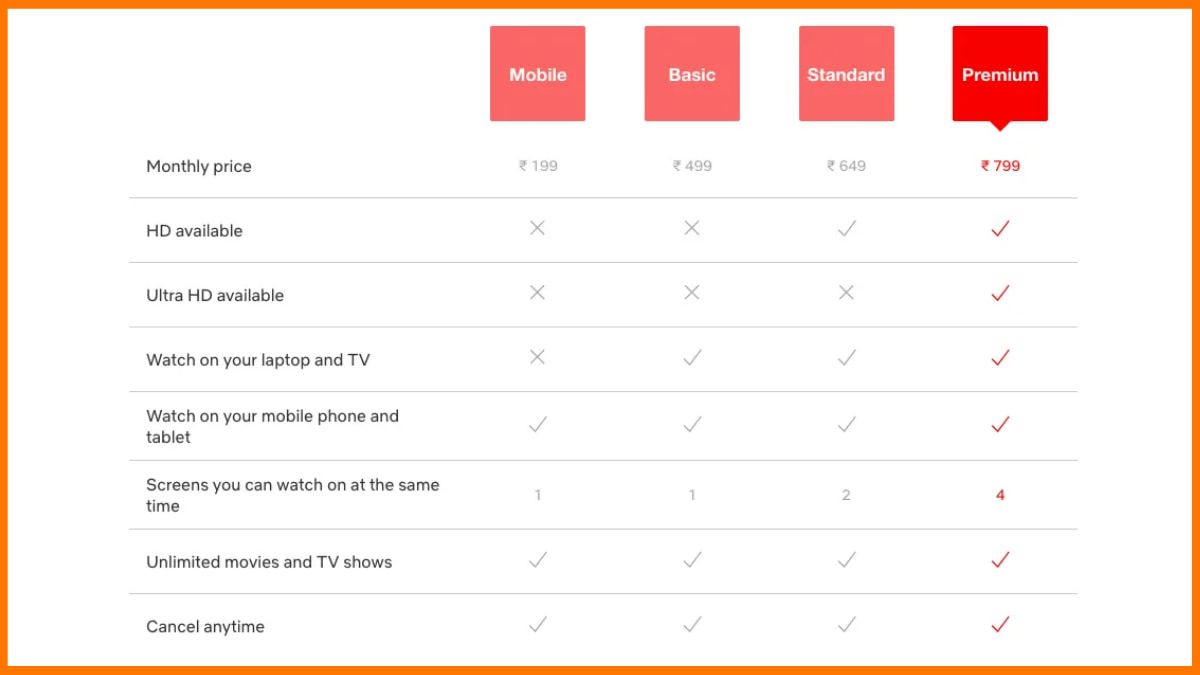
Memberships are the very fuel with which Netflix works. Anything that can increase memberships or subscriptions are managed and promoted by this business vertical. This includes marketing, sign up prompts, pricing and even partnering with other companies for promotions. They manage and handle all the incomings and welcomings at the Netflix website and app.
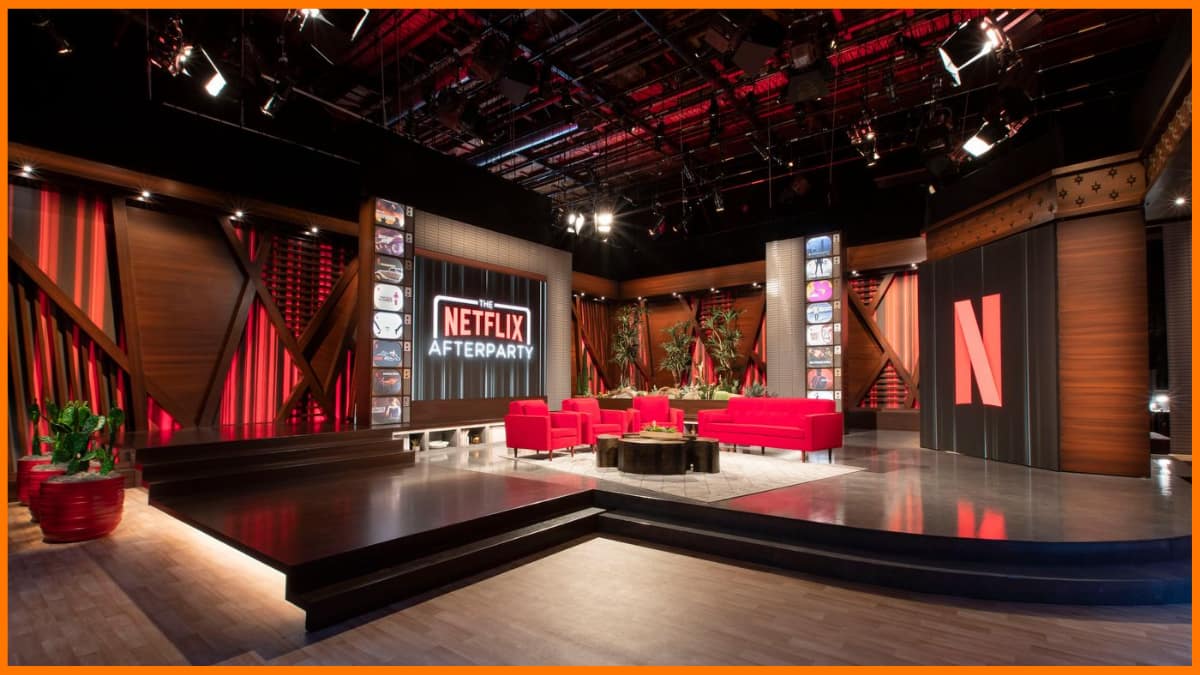
A studio is a place where a piece of content is shot. Many of the content that Netflix produces is done in already set up studios. This is also a cost-saving or cutting method . This department works at planning, development, and all the pre and post-production activities for the content. Thus, they work closely with content verticals.
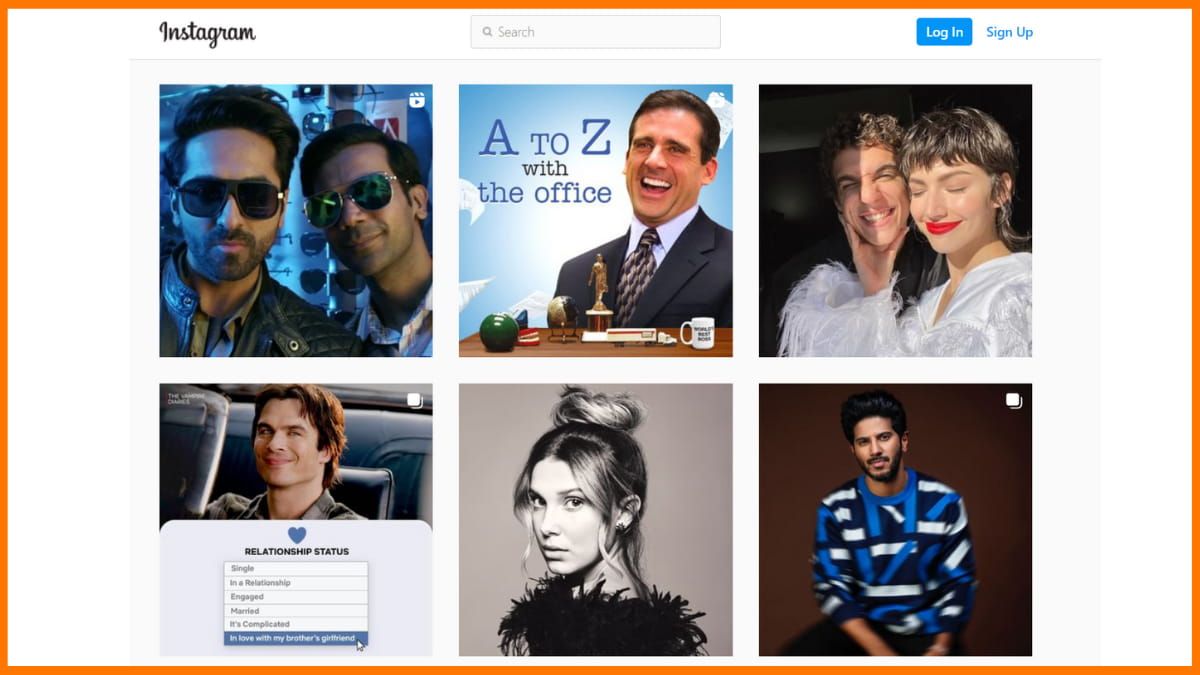
This vertical is focused to spread awareness and promotions about the content that Netflix is producing. This is done through new or traditional media or a combination of both. You must have seen advertisements for Netflix exclusive movies and tv shows, this is the department behind those.
This is the team that ensures the efficient, secure and state of art use of technology tools to manage the whole working of the platform. The data analytics and engineering tools are managed here to provide personalised content to each and every member/subscriber.
Some Facts about Netflix that might Interest you
- Despite more competition, Netflix still has the largest subscriber count in 2020.
- 60 million US adults have a Netflix subscription.
- Netflix was originally called “Kibble”.
- Netflix staffers think that you decide on a movie in two minutes.
- The company is older than most users realise.
- Netflix at its IPO sold its shares about 15 dollars, as its market grew, the share price went up to 350 dollars.
- 41% of Netflix users are watching without paying thanks to password and account sharing.
- Nearly two-thirds of US households now have Netflix.
- Netflix was one of the first streaming services available as an app on different devices.
- You'll Soon be able to Stream Netflix in a Tesla.
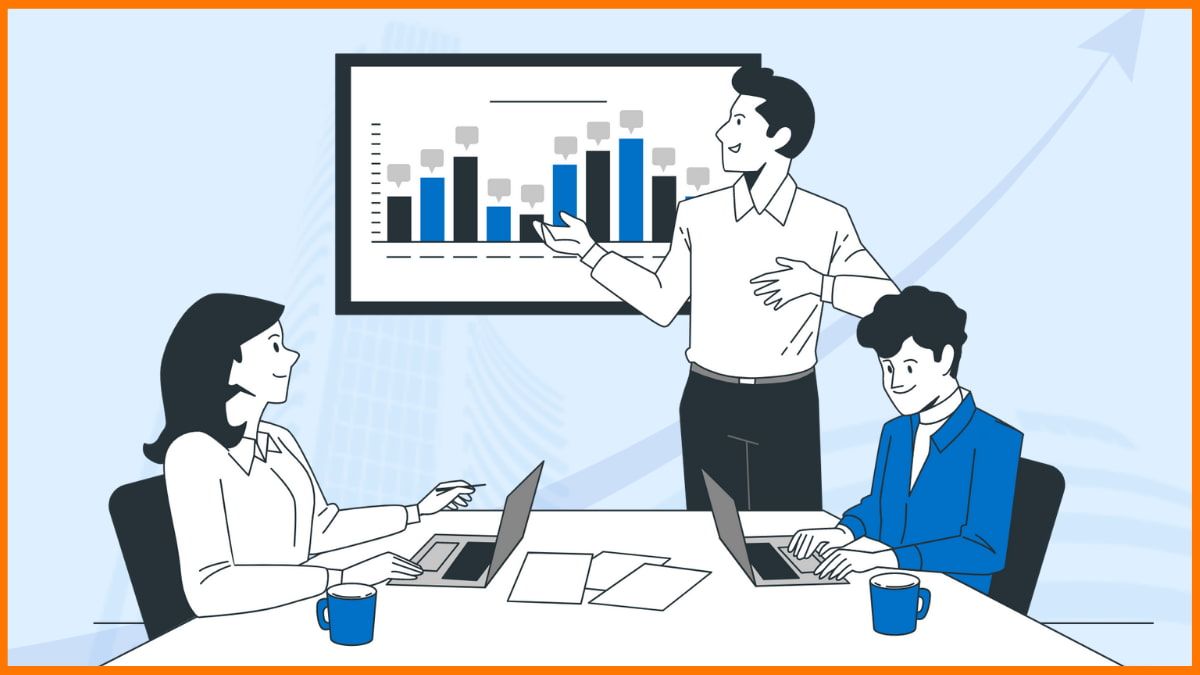
Data analytics is the fuel that powers Netflix. Netflix doesn't just work in managing content, movies, TV shows and entertainment but it has a lot of other data to handle as well. There is no efficient way other than “Big data” to handle such enormous amounts of data efficiently.
Netflix does it so well that we do not even notice that change. It cleverly posts content recommendations that are exactly matched with our likes. The data analytics at Netflix brings tailor-made and personalised content to each and every subscriber.
This makes Netflix best not only on the content basis but also on the overall user experience. That is the sole reason why we see steep spikes in Netflix viewerships over the years.
How accurate is the Netflix recommendation system?
Netflix's Recommendation Engine is so accurate that 80% of Netflix viewer activity is driven by personalised recommendations from the engine.
How do I get better recommendations on Netflix?
Whenever you watch a show on Netflix, you can give a thumbs up or thumbs down. Each time you give a show or film a thumbs up, Netflix will likely recommend similar content.
Is Netflix recommendation supervised or unsupervised?
Netflix recommendation engine is a supervised quality control algorithm.
Must have tools for startups - Recommended by StartupTalky
- Convert Visitors into Leads- SeizeLead
- Payment Gateway- Razorpay
- Spy on your Competitors- Adspyder
- Manage your business smoothly- Google Workspace
Navigating the World of Mobile SDKs: A Developer's Guide
Every app development team consists of tech architecture, UI/UX coding, front and back-end developers, a team of marketers, graphic or motion designers, and quality assurance specialists to ensure the app is running smoothly. Nevertheless, developing a mobile app can take anywhere from a few months to a full year,
Players Who Have Earned the Most Through IPL in the Last 17 Years
Run by the most affluent cricket board in the world– BCCI– the Indian Premier League is among the world’s highest-paid lucrative tournaments. As mentioned by Forbes, IPL is the sixth most valuable sports league in the world, ranking behind the NFL, the Champions League, and Europe’s four big
Indian FMCG Consumer Engagement Revolutionised by AI
The analysis by the global market research firm EY states that consumer goods companies have enormous opportunities to use AI to improve asset tracking, supply chain management, and consumer experience and engagement. The use of artificial intelligence (AI) technologies to improve customer centricity and operational efficiency is on the rise
Empowering India's MSMEs: Navigating the 45-Day Payment Rule
In India, there's a new rule called the 45-day MSME payment rule. It says big companies have to pay small businesses (MSMEs) on time. This is important for small businesses in India because it helps them stay financially healthy and run their businesses smoothly. The 45-day MSME payment rule mandates
To revisit this article, visit My Profile, then View saved stories .
- Backchannel
- Newsletters
- WIRED Insider
- WIRED Consulting
Libby Plummer
This is how Netflix's top-secret recommendation system works
More than 80 per cent of the TV shows people watch on Netflix are discovered through the platform’s recommendation system. That means the majority of what you decide to watch on Netflix is the result of decisions made by a mysterious, black box of an algorithm. Intrigued? Here's how it works.
Netflix uses machine learning and algorithms to help break viewers’ preconceived notions and find shows that they might not have initially chosen. To do this, it looks at nuanced threads within the content, rather than relying on broad genres to make its predictions. This explains how, for example, one in eight people who watch one of Netflix's Marvel shows are completely new to comic book-based stuff on Netflix.
Read more: Disney's streaming gamble is all about not getting eaten by Netflix
To help understand, consider a three-legged stool. "The three legs of this stool would be Netflix members; taggers who understand everything about the content; and our machine learning algorithms that take all of the data and put things together," says Todd Yellin, Netflix’s vice president of product innovation.
While Netflix has over 100 million users worldwide, if the multiple user profiles for each subscriber are counted, this brings the total to around 250 million active profiles. "What we see from those profiles is the following kinds of data – what people watch, what they watch after, what they watch before, what they watched a year ago, what they’ve watched recently and what time of day". This data forms the first leg of the metaphorical stool.
This information is then combined with more data aimed at understanding the content of shows. The latter – the second leg of the stool – is gathered from dozens of in-house and freelance staff who watch every minute or every show on Netflix and tag it. The tags they use range massively from how cerebral the piece is, to whether it has an ensemble cast, is set in space, or stars a corrupt cop.
"We take all of these tags and the user behaviour data and then we use very sophisticated machine learning algorithms that figure out what’s most important - what should we weigh," Yellin says. "How much should it matter if a consumer watched something yesterday? Should that count twice as much or ten times as much compared to what they watched a whole year ago? How about a month ago? How about if they watched ten minutes of content and abandoned it or they binged through it in two nights? How do we weight all that? That’s where machine learning comes in. What those three things create for us is ‘taste communities’ around the world. It’s about people who watch the same kind of things that you watch."
Viewers fit into multiple taste groups – of which there are "a couple of thousand" – and it’s these that affect what recommendations pop up to the top of your onscreen interface, which genre rows are displayed, and how each row is ordered for each individual viewer. The tags that are used for the machine learning algorithms are the same across the globe. However, a smaller sub-set of tags are used in a more outward-facing way, feeding directly into the user interface and differing depending on country, language and cultural context. "These have to be localised in ways that make sense," Yellin says. "For example, the word ‘gritty’ [as in, 'gritty drama'] may not translate into Spanish or French."
Jeremy White
Karen Williams
Read more: The next media revolution will come from driverless cars
The data that Netflix feeds into its algorithms can be broken down into two types – implicit and explicit. “Explicit data is what you literally tell us: you give a thumbs up to The Crown , we get it,” Yellin explains. "Implicit data is really behavioural data. You didn’t explicitly tell us 'I liked Unbreakable Kimmy Schmidt ', you just binged on it and watched it in two nights, so we understand that behaviourally. The majority of useful data is implicit."
To illustrate how all this data comes together to help viewers find new things to watch, Netflix looked at the patterns that led viewers towards the Marvel characters that make up The Defenders . While there were some more obvious trends, such as series with strong female leads – like Orange is the New Black – steering characters towards Jessica Jones , there were also a few less obvious sources, like the smart humour of Master of None and the psychological thrill of Making A Murderer driving people towards the wise-ass private detective. Meanwhile, "shows that expose the dark side of society" were shown to drive viewers to Luke Cage , such as the question of guilt in Amanda Knox and the examination of technology in Black Mirror .
This article was originally published by WIRED UK
Angela Watercutter
Jennifer M. Wood
Eric Ravenscraft
Marah Eakin
Search code, repositories, users, issues, pull requests...
Provide feedback.
We read every piece of feedback, and take your input very seriously.
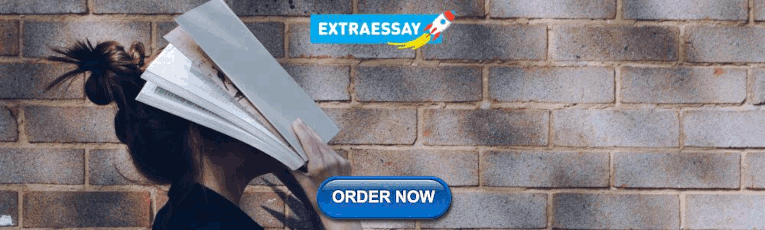
Saved searches
Use saved searches to filter your results more quickly.
To see all available qualifiers, see our documentation .
- Notifications
A Machine Learning Case Study for Recommendation System of movies based on collaborative filtering and content based filtering.
veeralakrishna/Case-Study-ML-Netflix-Movie-Recommendation-System
Folders and files, repository files navigation, case-study-ml-netflix-movie-recommendation-system, business problem.
Netflix is all about connecting people to the movies they love. To help customers find those movies, they developed world-class movie recommendation system: CinematchSM. Its job is to predict whether someone will enjoy a movie based on how much they liked or disliked other movies. Netflix use those predictions to make personal movie recommendations based on each customer’s unique tastes. And while Cinematch is doing pretty well, it can always be made better. Now there are a lot of interesting alternative approaches to how Cinematch works that netflix haven’t tried. Some are described in the literature, some aren’t. We’re curious whether any of these can beat Cinematch by making better predictions. Because, frankly, if there is a much better approach it could make a big difference to our customers and our business. Credits: https://www.netflixprize.com/rules.html
Problem Statement
Netflix provided a lot of anonymous rating data, and a prediction accuracy bar that is 10% better than what Cinematch can do on the same training data set. (Accuracy is a measurement of how closely predicted ratings of movies match subsequent actual ratings.)
- https://www.netflixprize.com/rules.html
- https://www.kaggle.com/netflix-inc/netflix-prize-data
- Netflix blog: https://medium.com/netflix-techblog/netflix-recommendations-beyond-the-5-stars-part-1-55838468f429
- surprise library: http://surpriselib.com/ (we use many models from this library)
- surprise library doc: http://surprise.readthedocs.io/en/stable/getting_started.html (we use many models from this library)
- installing surprise: https://github.com/NicolasHug/Surprise#installation
- Research paper: http://courses.ischool.berkeley.edu/i290-dm/s11/SECURE/a1-koren.pdf (most of our work was inspired by this paper)
- SVD Decomposition : https://www.youtube.com/watch?v=P5mlg91as1c
Real world/Business Objectives and constraints
Objectives:.
- Predict the rating that a user would give to a movie that he has not yet rated.
- Minimize the difference between predicted and actual rating (RMSE and MAPE)
Constraints:
- Some form of interpretability.
- There is no low latency requirement as the recommended movies can be precomputed earlier.
Type of Data:
- There are 17770 unique movie IDs.
- There are 480189 unique user IDs.
- There are ratings. Ratings are on a five star (integral) scale from 1 to 5.
- There is a date on which the movie is watched by the user in the format YYYY-MM-DD.
Getting Started
Start by downloading the project and run "NetflixMoviesRecommendation.ipynb" file in ipython-notebook.
Prerequisites
You need to have installed following softwares and libraries in your machine before running this project.
- Anaconda: It will install ipython notebook and most of the libraries which are needed like sklearn, pandas, seaborn, matplotlib, numpy, scipy.
- Python 3: https://www.python.org/downloads/
- Anaconda: https://www.anaconda.com/download/
- XGBoost: conda install -c conda-forge xgboost
- Surprise: pip install surprise
- ipython-notebook - Python Text Editor
- sklearn - Machine learning library
- seaborn, matplotlib.pyplot, - Visualization libraries
- numpy, scipy- number python library
- pandas - data handling library
- XGBoost - Used for making regression models
- Surprise - used for making recommendation system models
Veerala Hari Krishna - Complete work
Acknowledgments
Applied AI Course
- Jupyter Notebook 100.0%
Recommender Systems in Industry: A Netflix Case Study
Cite this chapter.
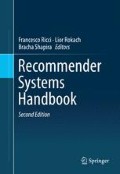
- Xavier Amatriain 4 , 5 &
- Justin Basilico 4
20k Accesses
30 Citations
The Netflix Prize put a spotlight on the importance and use of recommender systems in real-world applications. Many the competition provided many lessons about how to approach recommendation and many more have been learned since the Grand Prize was awarded in 2009. The evolution of industrial applications of recommender systems has been driven by the availability of different kinds of user data and the level of interest for the area within the research community. The goal of this chapter is to give an up-to-date overview of recommender systems techniques used in an industrial setting. We will give a high-level description the practical use of recommendation and personalization techniques. We will highlight some of the main lessons learned from the Netflix Prize. We will then use Netflix personalization as a case study to describe several approaches and techniques used in a real-world recommendation system. Finally, we will pinpoint what we see as some promising current research avenues and unsolved problems that deserve attention in this domain from an industry perspective.
This is a preview of subscription content, log in via an institution to check access.
Access this chapter
- Available as PDF
- Read on any device
- Instant download
- Own it forever
- Available as EPUB and PDF
- Compact, lightweight edition
- Dispatched in 3 to 5 business days
- Free shipping worldwide - see info
Tax calculation will be finalised at checkout
Purchases are for personal use only
Institutional subscriptions
The application of Matrix Factorization to the task of rating prediction closely resembles the technique known as Singular Value Decomposition used, for example, to identify latent factors in Information Retrieval. Therefore, it is common to see people referring to this MF solution as SVD.
For practical purposes we consider responses below a few hundred milliseconds (e.g. 200) to be real-time.
Intermediate recommendations usually represent lists of items that have been pre-selected and even ranked in advanced but need to undergo further processing such as filtering or re-ranking before being presented to the user.
Chukwa is a Hadoop subproject devoted to large-scale log collection and analysis.
Hadoop is an open-source software framework for storage and large-scale processing of data-sets on clusters of commodity hardware.
Apache Hive is a data warehouse infrastructure built on top of Hadoop for providing data summarization, query, and analysis.
Pig is a high-level platform for creating MapReduce programs used with Hadoop using a language called Pig Latin.
The Hadoop Distributed File System (HDFS) is a distributed file system designed to run on commodity hardware.
Amazon S3 (Simple Storage Service) is an online file storage web service offered by Amazon Web Services.
Apache Kafka is publish-subscribe messaging rethought as a distributed commit log.
Apache Cassandra is an open source distributed database management system designed to handle large amounts of data across many commodity servers, providing high availability with no single point of failure.
EVCache is a distributed in-memory data store for the cloud.
MySQL is one of the most popular open source relational databases.
It is important to note that the term “social recommendation” was originally used to describe collaborative filtering approaches [ 7 , 74 ].
Agarwal, D., Chen, B.C., Elango, P., Ramakrishnan, R.: Content recommendation on web portals. Commun. ACM 56 (6), 92–101 (2013). DOI 10.1145/2461256.2461277. URL http://doi.acm.org/10.1145/2461256.2461277
Agarwal, D., Chen, B.C., Pang, B.: Personalized recommendation of user comments via factor models. In: Proceedings of the Conference on Empirical Methods in Natural Language Processing, EMNLP ‘11, pp. 571–582. Association for Computational Linguistics, Stroudsburg, PA, USA (2011). URL http://dl.acm.org/citation.cfm?id=2145432.2145499
Ahmed, A., Teo, C.H., Vishwanathan, S., Smola, A.: Fair and balanced: Learning to present news stories. In: Proceedings of the Fifth ACM International Conference on Web Search and Data Mining, WSDM ‘12, pp. 333–342. ACM, New York, NY, USA (2012). DOI 10.1145/2124295.2124337. URL http://doi.acm.org/10.1145/2124295.2124337
Amatriain, X., Lathia, N., Pujol, J.M., Kwak, H., Oliver, N.: The wisdom of the few: a collaborative filtering approach based on expert opinions from the web. In: Proc. of 32nd ACM SIGIR, SIGIR ‘09, pp. 532–539. ACM, New York, NY, USA (2009). DOI 10.1145/1571941.1572033. URL http://dx.doi.org/10.1145/1571941.1572033
Amatriain, X., Pujol, J.M., Oliver, N.: I Like It…I Like It Not: Evaluating User Ratings Noise in Recommender Systems. In: G.J. Houben, G. McCalla, F. Pianesi, M. Zancanaro (eds.) User Modeling, Adaptation, and Personalization, vol. 5535, chap. 24, pp. 247–258. Springer Berlin (2009). DOI 10.1007/978-3-642-02247-0_24. URL http://dx.doi.org/10.1007/978-3-642-02247-0_24
Andersen, R., Borgs, C., Chayes, J., Feige, U., Flaxman, A., Kalai, A., Mirrokni, V., Tennenholtz, M.: Trust-based recommendation systems: an axiomatic approach. In: Proc. of the 17th WWW, WWW ‘08, pp. 199–208. ACM, New York, NY, USA (2008). DOI 10.1145/1367497.1367525. URL http://doi.acm.org/10.1145/1367497.1367525
Basu, C., Hirsh, H., Cohen, W.: Recommendation as classification: using social and content-based information in recommendation. In: Proc. of AAAI ‘98, AAAI ‘98/IAAI ‘98, pp. 714–720. American Association for Artificial Intelligence, Menlo Park, CA, USA (1998). URL http://dl.acm.org/citation.cfm?id=295240.295795
Bell, R.M., Koren, Y.: Lessons from the Netflix Prize Challenge. SIGKDD Explor. Newsl. 9 (2), 75–79 (2007). DOI 10.1145/1345448.1345465. URL http://dx.doi.org/10.1145/1345448.1345465
Berndhardsson, E.: Music recommendations at spotify (2013)
Google Scholar
Blei, D.M., Ng, A.Y., Jordan, M.I.: Latent dirichlet allocation. J. Mach. Learn. Res. 3 , 993–1022 (2003). URL http://dl.acm.org/citation.cfm?id=944919.944937
Bourke, S., McCarthy, K., Smyth, B.: Power to the people: exploring neighbourhood formations in social recommender system. In: Proc. of Recsys ‘11, RecSys ‘11, pp. 337–340. ACM, New York, NY, USA (2011). DOI 10.1145/2043932.2043997. URL http://doi.acm.org/10.1145/2043932.2043997
Breiman, L.: Random forests. Machine learning 45 (1), 5–32 (2001)
Article MATH Google Scholar
Burges, C., Shaked, T., Renshaw, E., Lazier, A., Deeds, M., Hamilton, N., Hullender, G.: Learning to rank using gradient descent. In: Proceedings of the 22nd ICML, ICML ‘05, pp. 89–96. ACM, New York, NY, USA (2005). DOI 10.1145/1102351.1102363. URL http://dx.doi.org/10.1145/1102351.1102363
Burke, R.: The adaptive web. chap. Hybrid Web Recommender Systems, pp. 377–408 (2007). DOI 10.1007/978-3-540-72079-9_12. URL http://dx.doi.org/10.1007/978-3-540-72079-9_12
Cao, Z., Liu, T.: Learning to rank: From pairwise approach to listwise approach. In: In Proceedings of the 24th ICML, pp. 129–136 (2007). URL http://citeseerx.ist.psu.edu/viewdoc/summary?doi=10.1.1.64.1518
Celma, O.: Music Recommendation and Discovery: The Long Tail, Long Fail, and Long Play in the Digital Music Space. Springer (2010)
Chapelle, O., Keerthi, S.S.: Efficient algorithms for ranking with SVMs. Information Retrieval 13 , 201–215 (2010). DOI 10.1007/s10791-009-9109-9. URL http://dx.doi.org/10.1007/s10791-009-9109-9
Chen, W.Y., Chu, J.C., Luan, J., Bai, H., Wang, Y., Chang, E.Y.: Collaborative filtering for orkut communities: Discovery of user latent behavior. In: Proceedings of the 18th International Conference on World Wide Web, WWW ‘09, pp. 681–690. ACM, New York, NY, USA (2009). DOI 10.1145/1526709.1526801. URL http://doi.acm.org/10.1145/1526709.1526801
Das, A.S., Datar, M., Garg, A., Rajaram, S.: Google news personalization: Scalable online collaborative filtering. In: Proceedings of the 16th International Conference on World Wide Web, WWW ‘07, pp. 271–280. ACM, New York, NY, USA (2007). DOI 10.1145/1242572.1242610. URL http://doi.acm.org/10.1145/1242572.1242610
Davidson, J., Liebald, B., Liu, J., Nandy, P., Van Vleet, T., Gargi, U., Gupta, S., He, Y., Lambert, M., Livingston, B., Sampath, D.: The youtube video recommendation system. In: Proceedings of the Fourth ACM Conference on Recommender Systems, RecSys ‘10, pp. 293–296. ACM, New York, NY, USA (2010). DOI 10.1145/1864708.1864770. URL http://doi.acm.org/10.1145/1864708.1864770
Diaz-Aviles, E., Georgescu, M., Nejdl, W.: Swarming to rank for recommender systems. In: Proc. of Recsys ‘12, RecSys ‘12, pp. 229–232. ACM, New York, NY, USA (2012). DOI 10.1145/2365952.2366001. URL http://doi.acm.org/10.1145/2365952.2366001
Elkan, C., Noto, K.: Learning classifiers from only positive and unlabeled data. In: Proc. of the 14th ACM SIGKDD, KDD ‘08, pp. 213–220. ACM, New York, NY, USA (2008). DOI 10.1145/1401890.1401920. URL http://dx.doi.org/10.1145/1401890.1401920
Freund, Y., Iyer, R., Schapire, R.E., Singer, Y.: An efficient boosting algorithm for combining preferences. J. Mach. Learn. Res. 4 , 933–969 (2003). URL http://portal.acm.org/citation.cfm?id=964285
Frey, B.J., Dueck, D.: Clustering by passing messages between data points. Science 315 , 2007 (2007)
Article MathSciNet Google Scholar
Friedman, J.H.: Greedy function approximation: a gradient boosting machine. Annals of Statistics pp. 1189–1232 (2001)
Fujiwara, Y., Nakatsuji, M., Yamamuro, T., Shiokawa, H., Onizuka, M.: Efficient personalized pagerank with accuracy assurance. In: Proceedings of the 18th ACM SIGKDD International Conference on Knowledge Discovery and Data Mining, KDD ‘12, pp. 15–23. ACM, New York, NY, USA (2012). DOI 10.1145/2339530.2339538. URL http://doi.acm.org/10.1145/2339530.2339538
Funk, S.: Netflix update: Try this at home. http://sifter.org/ simon/journal/20061211.html (2006). URL http://sifter.org/~simon/journal/20061211.html
Gorgoglione, M., Panniello, U., Tuzhilin, A.: The effect of context-aware recommendations on customer purchasing behavior and trust. In: Proc. of Recsys ‘11, RecSys ‘11, pp. 85–92. ACM, New York, NY, USA (2011). DOI 10.1145/2043932.2043951. URL http://doi.acm.org/10.1145/2043932.2043951
Gupta, P., Goel, A., Lin, J., Sharma, A., Wang, D., Zadeh, R.: Wtf: The who to follow service at twitter. In: Proceedings of the 22Nd International Conference on World Wide Web, WWW ‘13, pp. 505–514. International World Wide Web Conferences Steering Committee, Republic and Canton of Geneva, Switzerland (2013). URL http://dl.acm.org/citation.cfm?id=2488388.2488433
Herlocker, J.L., Konstan, J.A., Terveen, L.G., Riedl, J.T.: Evaluating collaborative filtering recommender systems. ACM Trans. Inf. Syst. 22 (1), 5–53 (2004). DOI http://doi.acm.org/10.1145/963770.963772
Hu, Y., Koren, Y., Volinsky, C.: Collaborative Filtering for Implicit Feedback Datasets. In: Proc. of the 2008 Eighth ICDM, ICDM ‘08 , vol. 0, pp. 263–272. IEEE Computer Society, Washington, DC, USA (2008). DOI 10.1109/ICDM.2008.22. URL http://dx.doi.org/10.1109/ICDM.2008.22
in the Industry, R.S.: Recommendation systems in the industry. Tutorial at Recsys 2009 (2009)
Jamali, M., Ester, M.: Trustwalker: a random walk model for combining trust-based and item-based recommendation. In: Proc. of KDD ‘09, KDD ‘09, pp. 397–406. ACM, New York, NY, USA (2009). DOI 10.1145/1557019.1557067. URL http://doi.acm.org/10.1145/1557019.1557067
Jeh, G., Widom, J.: Simrank: A measure of structural-context similarity. In: Proceedings of the Eighth ACM SIGKDD International Conference on Knowledge Discovery and Data Mining, KDD ‘02, pp. 538–543. ACM, New York, NY, USA (2002). DOI 10.1145/775047.775126. URL http://doi.acm.org/10.1145/775047.775126
Karatzoglou, A., Amatriain, X., Baltrunas, L., Oliver, N.: Multiverse recommendation: n-dimensional tensor factorization for context-aware collaborative filtering. In: Proc. of the fourth ACM Recsys, RecSys ‘10, pp. 79–86. ACM, New York, NY, USA (2010). DOI 10.1145/1864708.1864727. URL http://dx.doi.org/10.1145/1864708.1864727
Karimzadehgan, M., Li, W., Zhang, R., Mao, J.: A stochastic learning-to-rank algorithm and its application to contextual advertising. In: Proceedings of the 20th WWW, WWW ‘11, pp. 377–386. ACM, New York, NY, USA (2011). DOI 10.1145/1963405.1963460. URL http://doi.acm.org/10.1145/1963405.1963460
Karypis, G.: Evaluation of item-based top-n recommendation algorithms. In: CIKM ‘01: Proceedings of the tenth international conference on Information and knowledge management, pp. 247–254. ACM, New York, NY, USA (2001). DOI http://doi.acm.org/10.1145/ 502585.502627
Knijnenburg, B.P.: Conducting user experiments in recommender systems. In: Proceedings of the sixth ACM conference on Recommender systems, RecSys ‘12, pp. 3–4. ACM, New York, NY, USA (2012). DOI 10.1145/2365952.2365956. URL http://doi.acm.org/10.1145/2365952.2365956
Koenigstein, N., Nice, N., Paquet, U., Schleyen, N.: The xbox recommender system. In: Proceedings of the Sixth ACM Conference on Recommender Systems, RecSys ‘12, pp. 281–284. ACM, New York, NY, USA (2012). DOI 10.1145/2365952.2366015. URL http://doi.acm.org/10.1145/2365952.2366015
Kohavi, R., Deng, A., Frasca, B., Longbotham, R., Walker, T., Xu, Y.: Trustworthy online controlled experiments: five puzzling outcomes explained. In: Proceedings of KDD ‘12, pp. 786–794. ACM, New York, NY, USA (2012). DOI 10.1145/2339530.2339653. URL http://doi.acm.org/10.1145/2339530.2339653
Kohavi, R., Henne, R.M., Sommerfield, D.: Practical guide to controlled experiments on the web: Listen to your customers not to the hippo. In: Proceedings of the 13th ACM SIGKDD International Conference on Knowledge Discovery and Data Mining, KDD ‘07, pp. 959–967. ACM, New York, NY, USA (2007). DOI 10.1145/1281192.1281295. URL http://doi.acm.org/10.1145/1281192.1281295
Koren, Y.: Factorization meets the neighborhood: a multifaceted collaborative filtering model. In: Proceedings of the 14th ACM SIGKDD, KDD ‘08, pp. 426–434. ACM, New York, NY, USA (2008). DOI 10.1145/1401890.1401944. URL http://dx.doi.org/10.1145/1401890.1401944
Koren, Y.: Collaborative filtering with temporal dynamics. In: Proceedings of the 15th ACM SIGKDD, KDD ‘09, pp. 447–456. ACM, New York, NY, USA (2009). DOI 10.1145/1557019.1557072. URL http://dx.doi.org/10.1145/1557019.1557072
Koren, Y., Bell, R., Volinsky, C.: Matrix Factorization Techniques for Recommender Systems. Computer 42 (8), 30–37 (2009). DOI 10.1109/MC.2009.263. URL http://dx.doi.org/10.1109/MC.2009.263
Lagun, D., Hsieh, C.H., Webster, D., Navalpakkam, V.: Towards better measurement of attention and satisfaction in mobile search. In: Proceedings of the 37th International ACM SIGIR Conference on Research & Development in Information Retrieval, SIGIR ‘14, pp. 113–122. ACM, New York, NY, USA (2014). DOI 10.1145/2600428.2609631. URL http://doi.acm.org/10.1145/2600428.2609631
Lamere, P.B.: I’ve got 10 million songs in my pocket: Now what? In: Proceedings of the Sixth ACM Conference on Recommender Systems, RecSys ‘12, pp. 207–208. ACM, New York, NY, USA (2012). DOI 10.1145/2365952.2365994. URL http://doi.acm.org/10.1145/2365952.2365994
Li, L., Chu, W., Langford, J., Schapire, R.E.: A contextual-bandit approach to personalized news article recommendation. In: Proceedings of the 19th International Conference on World Wide Web, WWW ‘10, pp. 661–670. ACM, New York, NY, USA (2010). DOI 10.1145/1772690.1772758. URL http://doi.acm.org/10.1145/1772690.1772758
Linden, G., Smith, B., York, J.: Amazon.com recommendations: Item-to-item collaborative filtering. IEEE Internet Computing 7 (1), 76–80 (2003). DOI 10.1109/MIC.2003.1167344. URL http://dx.doi.org/10.1109/MIC.2003.1167344
Liu, J., Pedersen, E., Dolan, P.: Personalized news recommendation based on click behavior. In: 2010 International Conference on Intelligent User Interfaces (2010)
Liu, N.N., Meng, X., Liu, C., Yang, Q.: Wisdom of the better few: cold start recommendation via representative based rating elicitation. In: Proc. of RecSys ‘11, RecSys ‘11. ACM, New York, NY, USA (2011). DOI 10.1145/2043932.2043943. URL http://doi.acm.org/10.1145/2043932.2043943
McLaughlin, M.R., Herlocker, J.L.: A collaborative filtering algorithm and evaluation metric that accurately model the user experience. In: Proc. of SIGIR ‘04 (2004)
Mcnee, S.M., Riedl, J., Konstan, J.A.: Being accurate is not enough: how accuracy metrics have hurt recommender systems. In: CHI ‘06: CHI ‘06 extended abstracts on Human factors in computing systems, pp. 1097–1101. ACM Press, New York, NY, USA (2006). DOI 10.1145/ 1125451.1125659
Navalpakkam, V., Jentzsch, L., Sayres, R., Ravi, S., Ahmed, A., Smola, A.: Measurement and modeling of eye-mouse behavior in the presence of nonlinear page layouts. In: Proceedings of the 22Nd International Conference on World Wide Web, WWW ‘13, pp. 953–964. International World Wide Web Conferences Steering Committee, Republic and Canton of Geneva, Switzerland (2013). URL http://dl.acm.org/citation.cfm?id=2488388.2488471
Ning, X., Karypis, G.: Sparse linear methods with side information for top-n recommendations. In: Proc. of the 21st WWW, WWW ‘12 Companion, pp. 581–582. ACM, New York, NY, USA (2012). DOI 10.1145/2187980.2188137. URL http://doi.acm.org/10.1145/2187980.2188137
Noel, J., Sanner, S., Tran, K., Christen, P., Xie, L., Bonilla, E.V., Abbasnejad, E., Della Penna, N.: New objective functions for social collaborative filtering. In: Proc. of WWW ‘12, WWW ‘12, pp. 859–868. ACM, New York, NY, USA (2012). DOI 10.1145/2187836.2187952. URL http://doi.acm.org/10.1145/2187836.2187952
O’Donovan, J., Smyth, B.: Trust in recommender systems. In: Proc. of IUI ‘05, IUI ‘05, pp. 167–174. ACM, New York, NY, USA (2005). DOI 10.1145/1040830.1040870. URL http://doi.acm.org/10.1145/1040830.1040870
Oku, K., Nakajima, S., Miyazaki, J., Uemura, S.: Context-aware SVM for context-dependent information recommendation. In: Proc. of the 7th Conference on Mobile Data Management (2006)
Parra, D., Amatriain, X.: Walk the Talk: Analyzing the relation between implicit and explicit feedback for preference elicitation. In: J.A. Konstan, R. Conejo, J.L. Marzo, N. Oliver (eds.) User Modeling, Adaption and Personalization, Lecture Notes in Computer Science , vol. 6787, chap. 22, pp. 255–268. Springer, Berlin, Heidelberg (2011). DOI 10.1007/978-3-642-22362-4_22. URL http://dx.doi.org/10.1007/978-3-642-22362-4\_22
Parra, D., Karatzoglou, A., Amatriain, X., Yavuz, I.: Implicit feedback recommendation via implicit-to-explicit ordinal logistic regression mapping. In: Proc. of the 2011 CARS Workshop (2011)
Pizzato, L., Rej, T., Chung, T., Koprinska, I., Kay, J.: Recon: A reciprocal recommender for online dating. In: Proceedings of the Fourth ACM Conference on Recommender Systems, RecSys ‘10, pp. 207–214. ACM, New York, NY, USA (2010). DOI 10.1145/1864708.1864747. URL http://doi.acm.org/10.1145/1864708.1864747
Rabkin, A., Katz, R.: Chukwa: A system for reliable large-scale log collection. In: Proceedings of the 24th International Conference on Large Installation System Administration, LISA’10, pp. 1–15. USENIX Association, Berkeley, CA, USA (2010). URL http://dl.acm.org/citation.cfm?id=1924976.1924994
Radlinski, F., Kurup, M., Joachims, T.: How does clickthrough data reflect retrieval quality? In: Proc. of the 17th CIKM, CIKM ‘08, pp. 43–52. ACM, New York, NY, USA (2008). DOI 10.1145/1458082.1458092. URL http://dx.doi.org/10.1145/1458082.1458092
Reda, A., Park, Y., Tiwari, M., Posse, C., Shah, S.: Metaphor: A system for related search recommendations. In: Proceedings of the 21st ACM International Conference on Information and Knowledge Management, CIKM ‘12, pp. 664–673. ACM, New York, NY, USA (2012). DOI 10.1145/2396761.2396847. URL http://doi.acm.org/10.1145/2396761.2396847
Rendle, S.: Factorization Machines. In: Proc. of 2010 IEEE ICDM, pp. 995–1000. IEEE (2010). DOI 10.1109/ICDM.2010.127. URL http://dx.doi.org/10.1109/ICDM.2010.127
Rendle, S., Freudenthaler, C., Gantner, Z., Thieme, L.S.: BPR: Bayesian personalized ranking from implicit feedback. In: Proceedings of the 25th UAI, UAI ‘09, pp. 452–461. AUAI Press, Arlington, Virginia, United States (2009). URL http://portal.acm.org/citation.cfm?id=1795167
Rendle, S., Freudenthaler, C., Thieme, L.S.: Factorizing personalized Markov chains for next-basket recommendation. In: Proc. of the 19th WWW, WWW ‘10, pp. 811–820. ACM, New York, NY, USA (2010). DOI 10.1145/1772690.1772773. URL http://dx.doi.org/10.1145/1772690.1772773
Rendle, S., Gantner, Z., Freudenthaler, C., Schmidt-Thieme, L.: Fast context-aware recommendations with factorization machines. In: Proc. of the 34th ACM SIGIR, SIGIR ‘11, pp. 635–644. ACM, New York, NY, USA (2011). DOI 10.1145/2009916.2010002. URL http://doi.acm.org/10.1145/2009916.2010002
Ribeiro, M.T., Lacerda, A., Veloso, A., Ziviani, N.: Pareto-efficient hybridization for multi-objective recommender systems. In: Proceedings of the sixth ACM conference on Recommender systems, RecSys ‘12, pp. 19–26. ACM, New York, NY, USA (2012). DOI 10.1145/2365952.2365962. URL http://doi.acm.org/10.1145/2365952.2365962
Rodriguez, M., Posse, C., Zhang, E.: Multiple objective optimization in recommender systems. In: Proceedings of the sixth ACM conference on Recommender systems, RecSys ‘12, pp. 11–18. ACM, New York, NY, USA (2012). DOI 10.1145/2365952.2365961. URL http://doi.acm.org/10.1145/2365952.2365961
Salakhutdinov, R., Mnih, A., Hinton, G.E.: Restricted Boltzmann machines for collaborative filtering. In: Proc of ICML ‘07. ACM, New York, NY, USA (2007)
Science: Rockin’ to the Music Genome. Science 311 (5765), 1223d– (2006). DOI 10.1126/science.311.5765.1223d. URL http://www.sciencemag.org
Sha, X., Quercia, D., Michiardi, P., Dell’Amico, M.: Spotting trends: the wisdom of the few. In: Proc. of the Recsys ‘12, RecSys ‘12, pp. 51–58. ACM, New York, NY, USA (2012). DOI 10.1145/2365952.2365967. URL http://doi.acm.org/10.1145/2365952.2365967
Shardanand, U., Maes, P.: Social information filtering: algorithms for automating word of mouth. In: Proc. of SIGCHI ‘95, CHI ‘95, pp. 210–217. ACM Press/Addison-Wesley Publishing Co., New York, NY, USA (1995). DOI 10.1145/223904.223931. URL http://dx.doi.org/10.1145/223904.223931
Shi, Y., Karatzoglou, A., Baltrunas, L., Larson, M., Hanjalic, A., Oliver, N.: TFMAP: optimizing MAP for top-n context-aware recommendation. In: Proc. of the 35th SIGIR, SIGIR ‘12, pp. 155–164. ACM, New York, NY, USA (2012). DOI 10.1145/2348283.2348308. URL http://doi.acm.org/10.1145/2348283.2348308
Shi, Y., Karatzoglou, A., Baltrunas, L., Larson, M., Oliver, N., Hanjalic, A.: CLiMF: learning to maximize reciprocal rank with collaborative less-is-more filtering. In: Proc. of the sixth Recsys, RecSys ‘12, pp. 139–146. ACM, New York, NY, USA (2012). DOI 10.1145/2365952.2365981. URL http://dx.doi.org/10.1145/2365952.2365981
Sigurbjörnsson, B., van Zwol, R.: Flickr tag recommendation based on collective knowledge. In: Proceedings of the 17th International Conference on World Wide Web, WWW ‘08, pp. 327–336. ACM, New York, NY, USA (2008). DOI 10.1145/1367497.1367542. URL http://doi.acm.org/10.1145/1367497.1367542
Steck, H.: Training and testing of recommender systems on data missing not at random. In: Proc. of the 16th ACM SIGKDD, KDD ‘10, pp. 713–722. ACM, New York, NY, USA (2010). DOI 10.1145/1835804.1835895. URL http://dx.doi.org/10.1145/1835804.1835895
Steck, H.: Item popularity and recommendation accuracy. In: Proceedings of the fifth ACM conference on Recommender systems, RecSys ‘11, pp. 125–132. ACM, New York, NY, USA (2011). DOI 10.1145/2043932.2043957. URL http://doi.acm.org/10.1145/2043932.2043957
Steck, H.: Evaluation of recommendations: Rating-prediction and ranking. In: Proceedings of the 7th ACM Conference on Recommender Systems, RecSys ‘13, pp. 213–220. ACM, New York, NY, USA (2013). DOI 10.1145/2507157.2507160. URL http://doi.acm.org/10.1145/2507157.2507160
Stern, D.H., Herbrich, R., Graepel, T.: Matchbox: large scale online bayesian recommendations. In: Proc. of the 18th WWW, WWW ‘09, pp. 111–120. ACM, New York, NY, USA (2009). DOI 10.1145/1526709.1526725. URL http://dx.doi.org/10.1145/1526709.1526725
Takács, G., Pilászy, I., Németh, B., Tikk, D.: Major components of the gravity recommendation system. SIGKDD Explor. Newsl. 9 (2), 80–83 (2007). DOI 10.1145/1345448.1345466. URL http://doi.acm.org/10.1145/1345448.1345466
Takács, G., Tikk, D.: Alternating least squares for personalized ranking. In: Proc. of Recsys ‘12, RecSys ‘12, pp. 83–90. ACM, New York, NY, USA (2012). DOI 10.1145/2365952.2365972. URL http://doi.acm.org/10.1145/2365952.2365972
Tan, M., Xia, T., Guo, L., Wang, S.: Direct optimization of ranking measures for learning to rank models. In: Proceedings of the 19th ACM SIGKDD International Conference on Knowledge Discovery and Data Mining, KDD ‘13, pp. 856–864. ACM, New York, NY, USA (2013). DOI 10.1145/2487575.2487630. URL http://doi.acm.org/10.1145/2487575.2487630
Teh, Y.W., Jordan, M.I., Beal, M.J., Blei, D.M.: Hierarchical dirichlet processes. Journal of the American Statistical Association 101 (2004)
Valizadegan, H., Jin, R., Zhang, R., Mao, J.: Learning to Rank by Optimizing NDCG Measure. In: Proc. of SIGIR ‘00, pp. 41–48 (2000). URL http://citeseerx.ist.psu.edu/viewdoc/summary?doi=10.1.1.154.8402
Vargas, S., Castells, P.: Rank and relevance in novelty and diversity metrics for recommender systems. In: Proceedings of the fifth ACM conference on Recommender systems, RecSys ‘11, pp. 109–116. ACM, New York, NY, USA (2011). DOI 10.1145/2043932.2043955. URL http://doi.acm.org/10.1145/2043932.2043955
Wang, J., Sarwar, B., Sundaresan, N.: Utilizing related products for post-purchase recommendation in e-commerce. In: Proceedings of the Fifth ACM Conference on Recommender Systems, RecSys ‘11, pp. 329–332. ACM, New York, NY, USA (2011). DOI 10.1145/2043932.2043995. URL http://doi.acm.org/10.1145/2043932.2043995
Wang, J., Zhang, Y., Posse, C., Bhasin, A.: Is it time for a career switch? In: Proceedings of the 22Nd International Conference on World Wide Web, WWW ‘13, pp. 1377–1388. International World Wide Web Conferences Steering Committee, Republic and Canton of Geneva, Switzerland (2013). URL http://dl.acm.org/citation.cfm?id=2488388.2488509
Weston, J., Yee, H., Weiss, R.: Learning to rank recommendations with the k-order statistic loss. In: ACM International Conference on Recommender Systems (RecSys) (2013). URL http://dl.acm.org/citation.cfm?id=2507210
Xia, F., Liu, T.Y., Wang J.and Zhang, W., Li, H.: Listwise approach to learning to rank: theory and algorithm. In: Proc. of the 25th ICML, ICML ‘08, pp. 1192–1199. ACM, New York, NY, USA (2008). DOI 10.1145/1390156.1390306. URL http://dx.doi.org/10.1145/1390156.1390306
Xiong, L., Chen, X., Huang, T., J. Schneider, J.G.C.: Temporal collaborative filtering with bayesian probabilistic tensor factorization. In: Proceedings of SIAM Data Mining (2010)
Xu, J., Li, H.: AdaRank: a boosting algorithm for information retrieval. In: Proc. of SIGIR ‘07, SIGIR ‘07, pp. 391–398. ACM, New York, NY, USA (2007). DOI 10.1145/1277741.1277809. URL http://dx.doi.org/10.1145/1277741.1277809
Xu, J., Liu, T.Y., Lu, M., Li, H., Ma, W.Y.: Directly optimizing evaluation measures in learning to rank. In: Proc. of SIGIR ‘08, pp. 107–114. ACM, New York, NY, USA (2008). DOI 10.1145/1390334.1390355. URL http://dx.doi.org/10.1145/1390334.1390355
Y, K., Sill, J.: OrdRec: an ordinal model for predicting personalized item rating distributions. In: RecSys ‘11, pp. 117–124 (2011)
Yang, S., Long, B., Smola, A., Zha, H., Zheng, Z.: Collaborative competitive filtering: learning recommender using context of user choice. In: Proc. of the 34th ACM SIGIR, SIGIR ‘11, pp. 295–304. ACM, New York, NY, USA (2011). DOI 10.1145/2009916.2009959. URL http://dx.doi.org/10.1145/2009916.2009959
Yang, X., Steck, H., Guo, Y., Liu, Y.: On top-k recommendation using social networks. In: Proc. of RecSys ‘12, RecSys ‘12, pp. 67–74. ACM, New York, NY, USA (2012). DOI 10.1145/2365952.2365969. URL http://doi.acm.org/10.1145/2365952.2365969
Yi, J., Chen, Y., Li, J., Sett, S., Yan, T.W.: Predictive model performance: Offline and online evaluations. In: Proceedings of the 19th ACM SIGKDD International Conference on Knowledge Discovery and Data Mining, KDD ‘13, pp. 1294–1302. ACM, New York, NY, USA (2013). DOI 10.1145/2487575.2488215. URL http://doi.acm.org/10.1145/2487575.2488215
Download references
Author information
Authors and affiliations.
Netflix, 100 Winchester Cr., Los Gatos, CA, 95032, USA
Xavier Amatriain & Justin Basilico
Quora, 150 Castro St., Mountain View, USA
Xavier Amatriain
You can also search for this author in PubMed Google Scholar
Corresponding author
Correspondence to Xavier Amatriain .
Editor information
Editors and affiliations.
Faculty of Computer Science, Free University of Bozen-Bolzano, Bolzano - Bozen, Italy
Francesco Ricci
Information Systems Engineering, Ben-Gurion University of the Negev, Beer-Sheva, Israel
Lior Rokach
Ben-Gurion University of the Negev, Beer-Sheva, Israel
Bracha Shapira
Rights and permissions
Reprints and permissions
Copyright information
© 2015 Springer Science+Business Media New York
About this chapter
Amatriain, X., Basilico, J. (2015). Recommender Systems in Industry: A Netflix Case Study. In: Ricci, F., Rokach, L., Shapira, B. (eds) Recommender Systems Handbook. Springer, Boston, MA. https://doi.org/10.1007/978-1-4899-7637-6_11
Download citation
DOI : https://doi.org/10.1007/978-1-4899-7637-6_11
Publisher Name : Springer, Boston, MA
Print ISBN : 978-1-4899-7636-9
Online ISBN : 978-1-4899-7637-6
eBook Packages : Computer Science Computer Science (R0)
Share this chapter
Anyone you share the following link with will be able to read this content:
Sorry, a shareable link is not currently available for this article.
Provided by the Springer Nature SharedIt content-sharing initiative
- Publish with us
Policies and ethics
- Find a journal
- Track your research
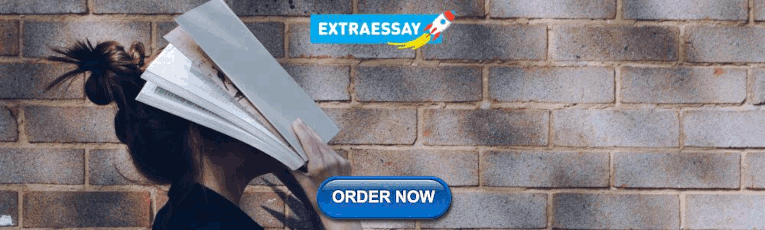
IMAGES
VIDEO
COMMENTS
It uses phrases such as 'Similar titles to watch instantly', 'More like …' etc. Search is also one of the important aspects of the Netflix recommendation system. Data Sources: According to (Netflix Technology Blog, 2017b), the data sources for the recommendation system of Netflix are: A set of several billion ratings from its members.
Figure 1 displays a Netflix homepage with red circles enumerating different recommendation tasks, each of which is powered by a different algorithm. For example, there is a dedicated algorithm (1) for choosing the first video to display prominently at the top of the homepage, another one for ranking already-watched videos that the user may want to continue watching (7), as well as others ...
In Netflix's case, the NRE or the Netflix recommendation engine has some different factors of inputs. It collects data that will be the most relevant in the prediction of user behaviours. Some of the most commonly tracked inputs are as follows, The device used to stream on. The number of searches.
The Netflix Recommender System: Algorithms, Business Value, and Innovation 13:3 Fig. 1. (Left) An example of the page of recommendations, showing two of the roughly 40 rows of recom-mendations on that page. Suspenseful Movies is an example of a genre row driven by the PVR algorithm (Section 2.1).
In this article, we outline some of the challenges encountered and lessons learned in using deep learning for recommender systems at Netflix. We first provide an overview of the various recommendation tasks on the Netflix service. We found that different model architectures excel at different tasks. Even though many deep-learning models can be ...
Chapters 3 and 4 form the second part of this book, presenting a comprehensive case-study analysis of Netflix. Chapter 3 discusses Netflix's origins and historical development since its founding in 1997. ... Frey thoroughly explores the mythology surrounding Netflix's recommendation system, focusing on the algorithm driven by the company's ...
On the practical side, integrating deep-learning toolboxes in our system has made it faster and easier to implement and experiment with both deep-learning and non-deep-learning approaches for various recommendation tasks. We conclude this article by summarizing our take-aways that may generalize to other applications beyond Netflix.
Here's how it works. Netflix uses machine learning and algorithms to help break viewers' preconceived notions and find shows that they might not have initially chosen. To do this, it looks at ...
Abstract. Deep learning has profoundly impacted many areas of machine learning. How-. ever, it took a while for its impact t o be felt in the field of recommender systems. In this article, we ...
This article discusses the various algorithms that make up the Netflix recommender system, and describes its business purpose. We also describe the role of search and related algorithms, which for us turns into a recommendations problem as well.
A Machine Learning Case Study for Recommendation System of movies based on collaborative filtering and content based filtering. Business Problem Netflix is all about connecting people to the movies they love.
In keeping with such a relational ontology of algorithms, I have adopted Bucher's (2016, 2018) flexible methodology of technography to analyse the global streaming giant Netflix and its sophisticated recommendation system, the Netflix Recommender System (NRS). Of the many algorithmic applications and practices propagating in the film and television industry, the NRS has received the most ...
Netflix's recommendation system is designed to provide each user with a personalized viewing experience. This goes beyond mere genre preferences, taking into account a myriad of factors to ...
Given an existing application, an improvement in the recommendation system can have a value of millions of dollars and can be the factor that determines the success or failure of a business. In Sect.11.2 we will review some of the typical uses of recommendation systems in industry. While a lot of work in recommendation
recommendation system as an asset. Netflix created a highly powerful data mining system that, rather than relying only on a costumer's past consumption to create recommendations, also provides cross-reference usage patterns across all users to offer unique recommendations (Gomez-Uribe & Hunt, 2016; Rayna & Striukova, 2016).
In this article, we outline some of the challenges encountered and lessons learned in using deep learning for recommender systems at Netflix. We first provide an overview of the various recommendation tasks on the Netflix service. We found that different model architectures excel at different tasks.
This chapter uses Netflix personalization as a case study to describe several approaches and techniques used in a real-world recommendation system and pinpoint what it sees as some promising current research avenues and unsolved problems that deserve attention in this domain from an industry perspective. The Netflix Prize put a spotlight on the importance and use of recommender systems in real ...
The goal of this chapter is to give an up-to-date overview of recommender systems techniques used in an industrial setting. We will give a high-level description the practical use of recommendation and personalization techniques. We will highlight some of the main lessons learned from the Netflix Prize. We will then use Netflix personalization ...
The challenges encountered and lessons learned in using deep learning for recommender systems at Netflix are outlined and some of the take-aways that may generalize to other applications beyond Netflix are summarized. Deep learning has profoundly impacted many areas of machine learning. However, it took a while for its impact to be felt in the field of recommender systems.
Here are a few tips for businesses from the Netflix case study: Collect data on your customers' actions and preferences. You can use this data to tailor your marketing messages, suggest products ...
The other challenge was to maintain the customers as competitors like hulu, espn+ etc have good content too. For this purpose, Netflix introduced the recommendation system. Netflix invested heavily into big data analytics to recommend the best content for their users. 2.8 Technical challenges
Rating Distribution: The distribution of ratings over the years offers insights into the evolving content landscape and audience reception. Data-Driven Insights: Our data analysis journey showcased the power of data in unraveling the mysteries of Netflix's content landscape, providing valuable insights for viewers and content creators.