Have a language expert improve your writing
Run a free plagiarism check in 10 minutes, generate accurate citations for free.
- Knowledge Base
- Research paper
- How to Write a Discussion Section | Tips & Examples
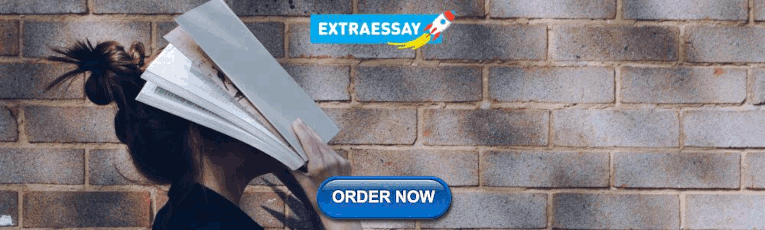
How to Write a Discussion Section | Tips & Examples
Published on August 21, 2022 by Shona McCombes . Revised on July 18, 2023.
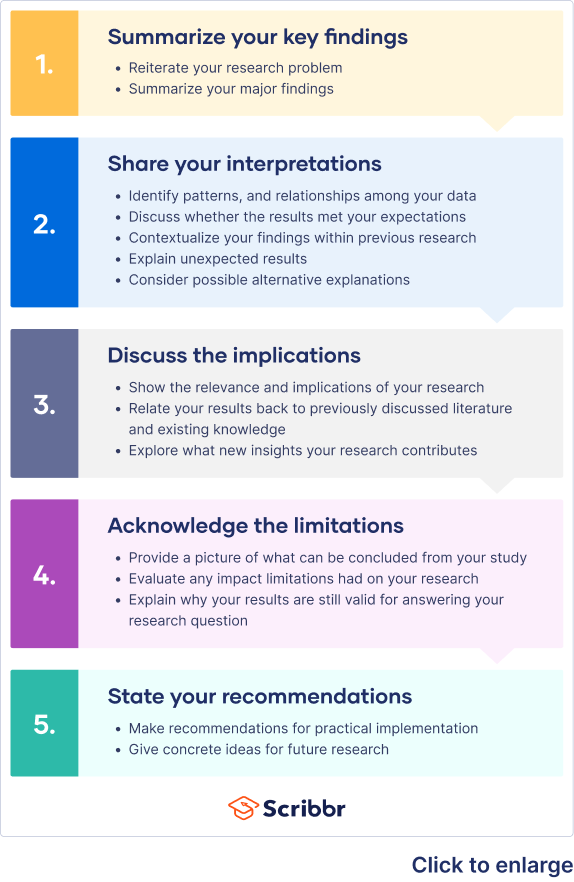
The discussion section is where you delve into the meaning, importance, and relevance of your results .
It should focus on explaining and evaluating what you found, showing how it relates to your literature review and paper or dissertation topic , and making an argument in support of your overall conclusion. It should not be a second results section.
There are different ways to write this section, but you can focus your writing around these key elements:
- Summary : A brief recap of your key results
- Interpretations: What do your results mean?
- Implications: Why do your results matter?
- Limitations: What can’t your results tell us?
- Recommendations: Avenues for further studies or analyses
Instantly correct all language mistakes in your text
Upload your document to correct all your mistakes in minutes
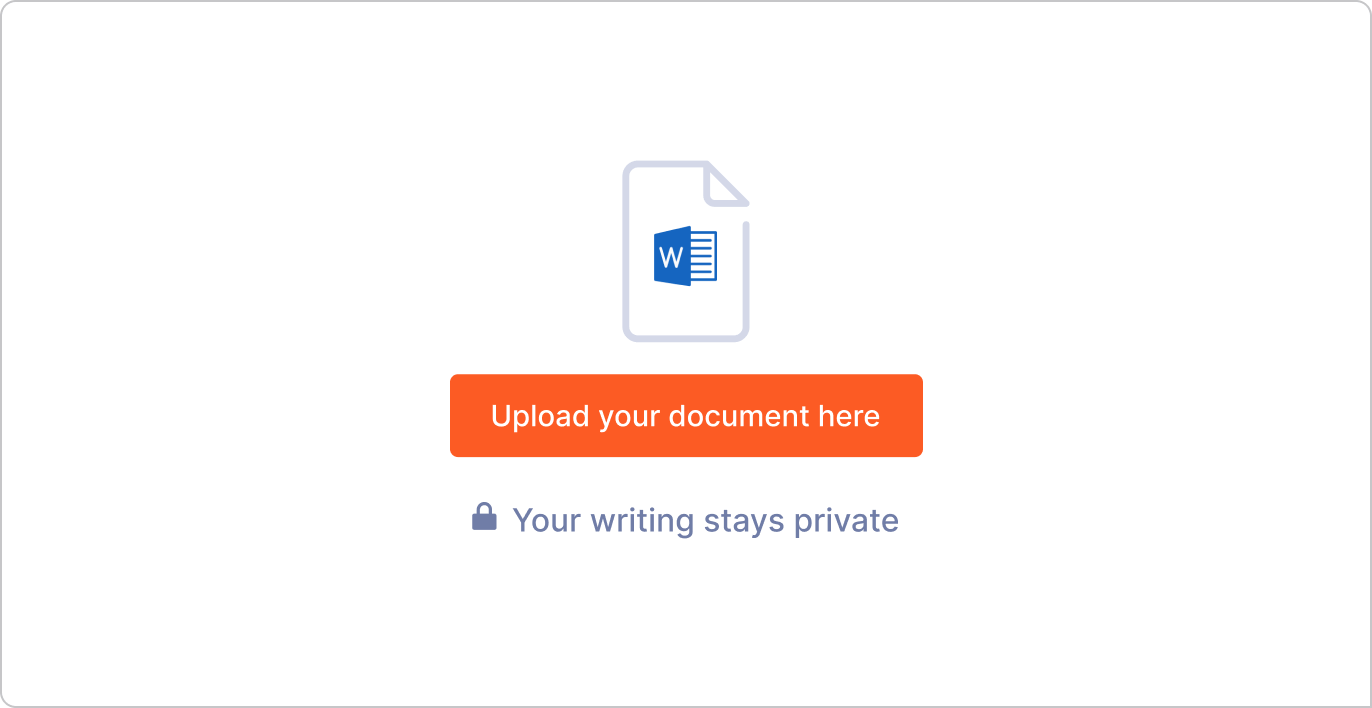
Table of contents
What not to include in your discussion section, step 1: summarize your key findings, step 2: give your interpretations, step 3: discuss the implications, step 4: acknowledge the limitations, step 5: share your recommendations, discussion section example, other interesting articles, frequently asked questions about discussion sections.
There are a few common mistakes to avoid when writing the discussion section of your paper.
- Don’t introduce new results: You should only discuss the data that you have already reported in your results section .
- Don’t make inflated claims: Avoid overinterpretation and speculation that isn’t directly supported by your data.
- Don’t undermine your research: The discussion of limitations should aim to strengthen your credibility, not emphasize weaknesses or failures.
Don't submit your assignments before you do this
The academic proofreading tool has been trained on 1000s of academic texts. Making it the most accurate and reliable proofreading tool for students. Free citation check included.
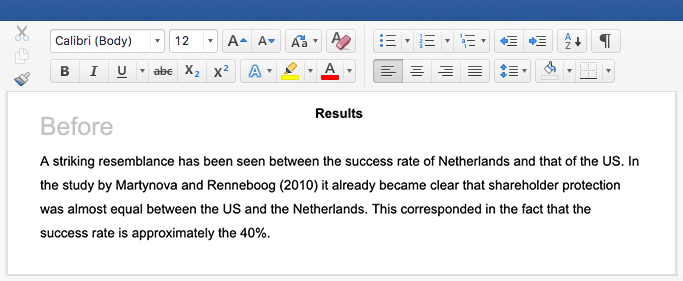
Try for free
Start this section by reiterating your research problem and concisely summarizing your major findings. To speed up the process you can use a summarizer to quickly get an overview of all important findings. Don’t just repeat all the data you have already reported—aim for a clear statement of the overall result that directly answers your main research question . This should be no more than one paragraph.
Many students struggle with the differences between a discussion section and a results section . The crux of the matter is that your results sections should present your results, and your discussion section should subjectively evaluate them. Try not to blend elements of these two sections, in order to keep your paper sharp.
- The results indicate that…
- The study demonstrates a correlation between…
- This analysis supports the theory that…
- The data suggest that…
The meaning of your results may seem obvious to you, but it’s important to spell out their significance for your reader, showing exactly how they answer your research question.
The form of your interpretations will depend on the type of research, but some typical approaches to interpreting the data include:
- Identifying correlations , patterns, and relationships among the data
- Discussing whether the results met your expectations or supported your hypotheses
- Contextualizing your findings within previous research and theory
- Explaining unexpected results and evaluating their significance
- Considering possible alternative explanations and making an argument for your position
You can organize your discussion around key themes, hypotheses, or research questions, following the same structure as your results section. Alternatively, you can also begin by highlighting the most significant or unexpected results.
- In line with the hypothesis…
- Contrary to the hypothesized association…
- The results contradict the claims of Smith (2022) that…
- The results might suggest that x . However, based on the findings of similar studies, a more plausible explanation is y .
As well as giving your own interpretations, make sure to relate your results back to the scholarly work that you surveyed in the literature review . The discussion should show how your findings fit with existing knowledge, what new insights they contribute, and what consequences they have for theory or practice.
Ask yourself these questions:
- Do your results support or challenge existing theories? If they support existing theories, what new information do they contribute? If they challenge existing theories, why do you think that is?
- Are there any practical implications?
Your overall aim is to show the reader exactly what your research has contributed, and why they should care.
- These results build on existing evidence of…
- The results do not fit with the theory that…
- The experiment provides a new insight into the relationship between…
- These results should be taken into account when considering how to…
- The data contribute a clearer understanding of…
- While previous research has focused on x , these results demonstrate that y .
Here's why students love Scribbr's proofreading services
Discover proofreading & editing
Even the best research has its limitations. Acknowledging these is important to demonstrate your credibility. Limitations aren’t about listing your errors, but about providing an accurate picture of what can and cannot be concluded from your study.
Limitations might be due to your overall research design, specific methodological choices , or unanticipated obstacles that emerged during your research process.
Here are a few common possibilities:
- If your sample size was small or limited to a specific group of people, explain how generalizability is limited.
- If you encountered problems when gathering or analyzing data, explain how these influenced the results.
- If there are potential confounding variables that you were unable to control, acknowledge the effect these may have had.
After noting the limitations, you can reiterate why the results are nonetheless valid for the purpose of answering your research question.
- The generalizability of the results is limited by…
- The reliability of these data is impacted by…
- Due to the lack of data on x , the results cannot confirm…
- The methodological choices were constrained by…
- It is beyond the scope of this study to…
Based on the discussion of your results, you can make recommendations for practical implementation or further research. Sometimes, the recommendations are saved for the conclusion .
Suggestions for further research can lead directly from the limitations. Don’t just state that more studies should be done—give concrete ideas for how future work can build on areas that your own research was unable to address.
- Further research is needed to establish…
- Future studies should take into account…
- Avenues for future research include…
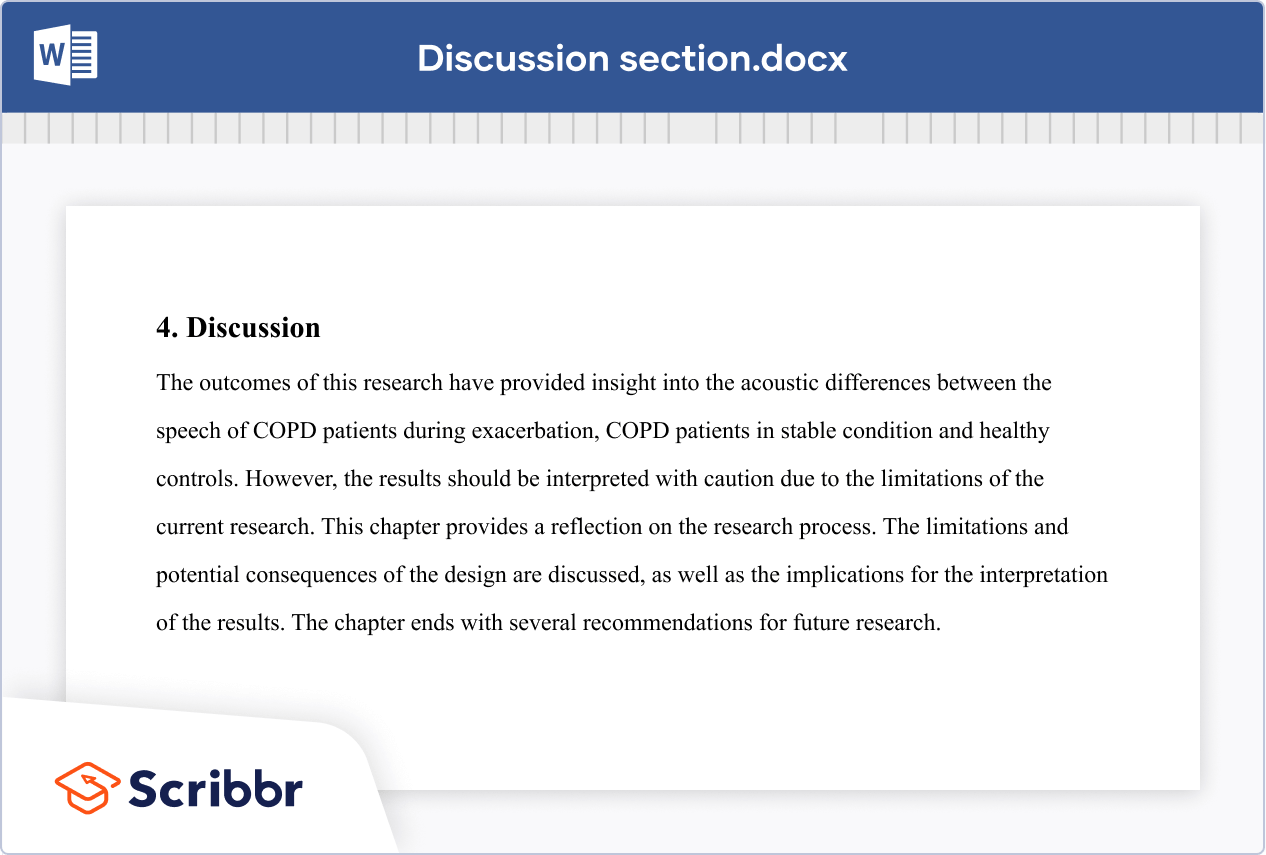
If you want to know more about AI for academic writing, AI tools, or research bias, make sure to check out some of our other articles with explanations and examples or go directly to our tools!
Research bias
- Anchoring bias
- Halo effect
- The Baader–Meinhof phenomenon
- The placebo effect
- Nonresponse bias
- Deep learning
- Generative AI
- Machine learning
- Reinforcement learning
- Supervised vs. unsupervised learning
(AI) Tools
- Grammar Checker
- Paraphrasing Tool
- Text Summarizer
- AI Detector
- Plagiarism Checker
- Citation Generator
In the discussion , you explore the meaning and relevance of your research results , explaining how they fit with existing research and theory. Discuss:
- Your interpretations : what do the results tell us?
- The implications : why do the results matter?
- The limitation s : what can’t the results tell us?
The results chapter or section simply and objectively reports what you found, without speculating on why you found these results. The discussion interprets the meaning of the results, puts them in context, and explains why they matter.
In qualitative research , results and discussion are sometimes combined. But in quantitative research , it’s considered important to separate the objective results from your interpretation of them.
In a thesis or dissertation, the discussion is an in-depth exploration of the results, going into detail about the meaning of your findings and citing relevant sources to put them in context.
The conclusion is more shorter and more general: it concisely answers your main research question and makes recommendations based on your overall findings.
Cite this Scribbr article
If you want to cite this source, you can copy and paste the citation or click the “Cite this Scribbr article” button to automatically add the citation to our free Citation Generator.
McCombes, S. (2023, July 18). How to Write a Discussion Section | Tips & Examples. Scribbr. Retrieved June 26, 2024, from https://www.scribbr.com/dissertation/discussion/
Is this article helpful?
Shona McCombes
Other students also liked, how to write a literature review | guide, examples, & templates, what is a research methodology | steps & tips, how to write a results section | tips & examples, get unlimited documents corrected.
✔ Free APA citation check included ✔ Unlimited document corrections ✔ Specialized in correcting academic texts
- USC Libraries
- Research Guides
Organizing Your Social Sciences Research Paper
- 8. The Discussion
- Purpose of Guide
- Design Flaws to Avoid
- Independent and Dependent Variables
- Glossary of Research Terms
- Reading Research Effectively
- Narrowing a Topic Idea
- Broadening a Topic Idea
- Extending the Timeliness of a Topic Idea
- Academic Writing Style
- Applying Critical Thinking
- Choosing a Title
- Making an Outline
- Paragraph Development
- Research Process Video Series
- Executive Summary
- The C.A.R.S. Model
- Background Information
- The Research Problem/Question
- Theoretical Framework
- Citation Tracking
- Content Alert Services
- Evaluating Sources
- Primary Sources
- Secondary Sources
- Tiertiary Sources
- Scholarly vs. Popular Publications
- Qualitative Methods
- Quantitative Methods
- Insiderness
- Using Non-Textual Elements
- Limitations of the Study
- Common Grammar Mistakes
- Writing Concisely
- Avoiding Plagiarism
- Footnotes or Endnotes?
- Further Readings
- Generative AI and Writing
- USC Libraries Tutorials and Other Guides
- Bibliography
The purpose of the discussion section is to interpret and describe the significance of your findings in relation to what was already known about the research problem being investigated and to explain any new understanding or insights that emerged as a result of your research. The discussion will always connect to the introduction by way of the research questions or hypotheses you posed and the literature you reviewed, but the discussion does not simply repeat or rearrange the first parts of your paper; the discussion clearly explains how your study advanced the reader's understanding of the research problem from where you left them at the end of your review of prior research.
Annesley, Thomas M. “The Discussion Section: Your Closing Argument.” Clinical Chemistry 56 (November 2010): 1671-1674; Peacock, Matthew. “Communicative Moves in the Discussion Section of Research Articles.” System 30 (December 2002): 479-497.
Importance of a Good Discussion
The discussion section is often considered the most important part of your research paper because it:
- Most effectively demonstrates your ability as a researcher to think critically about an issue, to develop creative solutions to problems based upon a logical synthesis of the findings, and to formulate a deeper, more profound understanding of the research problem under investigation;
- Presents the underlying meaning of your research, notes possible implications in other areas of study, and explores possible improvements that can be made in order to further develop the concerns of your research;
- Highlights the importance of your study and how it can contribute to understanding the research problem within the field of study;
- Presents how the findings from your study revealed and helped fill gaps in the literature that had not been previously exposed or adequately described; and,
- Engages the reader in thinking critically about issues based on an evidence-based interpretation of findings; it is not governed strictly by objective reporting of information.
Annesley Thomas M. “The Discussion Section: Your Closing Argument.” Clinical Chemistry 56 (November 2010): 1671-1674; Bitchener, John and Helen Basturkmen. “Perceptions of the Difficulties of Postgraduate L2 Thesis Students Writing the Discussion Section.” Journal of English for Academic Purposes 5 (January 2006): 4-18; Kretchmer, Paul. Fourteen Steps to Writing an Effective Discussion Section. San Francisco Edit, 2003-2008.
Structure and Writing Style
I. General Rules
These are the general rules you should adopt when composing your discussion of the results :
- Do not be verbose or repetitive; be concise and make your points clearly
- Avoid the use of jargon or undefined technical language
- Follow a logical stream of thought; in general, interpret and discuss the significance of your findings in the same sequence you described them in your results section [a notable exception is to begin by highlighting an unexpected result or a finding that can grab the reader's attention]
- Use the present verb tense, especially for established facts; however, refer to specific works or prior studies in the past tense
- If needed, use subheadings to help organize your discussion or to categorize your interpretations into themes
II. The Content
The content of the discussion section of your paper most often includes :
- Explanation of results : Comment on whether or not the results were expected for each set of findings; go into greater depth to explain findings that were unexpected or especially profound. If appropriate, note any unusual or unanticipated patterns or trends that emerged from your results and explain their meaning in relation to the research problem.
- References to previous research : Either compare your results with the findings from other studies or use the studies to support a claim. This can include re-visiting key sources already cited in your literature review section, or, save them to cite later in the discussion section if they are more important to compare with your results instead of being a part of the general literature review of prior research used to provide context and background information. Note that you can make this decision to highlight specific studies after you have begun writing the discussion section.
- Deduction : A claim for how the results can be applied more generally. For example, describing lessons learned, proposing recommendations that can help improve a situation, or highlighting best practices.
- Hypothesis : A more general claim or possible conclusion arising from the results [which may be proved or disproved in subsequent research]. This can be framed as new research questions that emerged as a consequence of your analysis.
III. Organization and Structure
Keep the following sequential points in mind as you organize and write the discussion section of your paper:
- Think of your discussion as an inverted pyramid. Organize the discussion from the general to the specific, linking your findings to the literature, then to theory, then to practice [if appropriate].
- Use the same key terms, narrative style, and verb tense [present] that you used when describing the research problem in your introduction.
- Begin by briefly re-stating the research problem you were investigating and answer all of the research questions underpinning the problem that you posed in the introduction.
- Describe the patterns, principles, and relationships shown by each major findings and place them in proper perspective. The sequence of this information is important; first state the answer, then the relevant results, then cite the work of others. If appropriate, refer the reader to a figure or table to help enhance the interpretation of the data [either within the text or as an appendix].
- Regardless of where it's mentioned, a good discussion section includes analysis of any unexpected findings. This part of the discussion should begin with a description of the unanticipated finding, followed by a brief interpretation as to why you believe it appeared and, if necessary, its possible significance in relation to the overall study. If more than one unexpected finding emerged during the study, describe each of them in the order they appeared as you gathered or analyzed the data. As noted, the exception to discussing findings in the same order you described them in the results section would be to begin by highlighting the implications of a particularly unexpected or significant finding that emerged from the study, followed by a discussion of the remaining findings.
- Before concluding the discussion, identify potential limitations and weaknesses if you do not plan to do so in the conclusion of the paper. Comment on their relative importance in relation to your overall interpretation of the results and, if necessary, note how they may affect the validity of your findings. Avoid using an apologetic tone; however, be honest and self-critical [e.g., in retrospect, had you included a particular question in a survey instrument, additional data could have been revealed].
- The discussion section should end with a concise summary of the principal implications of the findings regardless of their significance. Give a brief explanation about why you believe the findings and conclusions of your study are important and how they support broader knowledge or understanding of the research problem. This can be followed by any recommendations for further research. However, do not offer recommendations which could have been easily addressed within the study. This would demonstrate to the reader that you have inadequately examined and interpreted the data.
IV. Overall Objectives
The objectives of your discussion section should include the following: I. Reiterate the Research Problem/State the Major Findings
Briefly reiterate the research problem or problems you are investigating and the methods you used to investigate them, then move quickly to describe the major findings of the study. You should write a direct, declarative, and succinct proclamation of the study results, usually in one paragraph.
II. Explain the Meaning of the Findings and Why They are Important
No one has thought as long and hard about your study as you have. Systematically explain the underlying meaning of your findings and state why you believe they are significant. After reading the discussion section, you want the reader to think critically about the results and why they are important. You don’t want to force the reader to go through the paper multiple times to figure out what it all means. If applicable, begin this part of the section by repeating what you consider to be your most significant or unanticipated finding first, then systematically review each finding. Otherwise, follow the general order you reported the findings presented in the results section.
III. Relate the Findings to Similar Studies
No study in the social sciences is so novel or possesses such a restricted focus that it has absolutely no relation to previously published research. The discussion section should relate your results to those found in other studies, particularly if questions raised from prior studies served as the motivation for your research. This is important because comparing and contrasting the findings of other studies helps to support the overall importance of your results and it highlights how and in what ways your study differs from other research about the topic. Note that any significant or unanticipated finding is often because there was no prior research to indicate the finding could occur. If there is prior research to indicate this, you need to explain why it was significant or unanticipated. IV. Consider Alternative Explanations of the Findings
It is important to remember that the purpose of research in the social sciences is to discover and not to prove . When writing the discussion section, you should carefully consider all possible explanations for the study results, rather than just those that fit your hypothesis or prior assumptions and biases. This is especially important when describing the discovery of significant or unanticipated findings.
V. Acknowledge the Study’s Limitations
It is far better for you to identify and acknowledge your study’s limitations than to have them pointed out by your professor! Note any unanswered questions or issues your study could not address and describe the generalizability of your results to other situations. If a limitation is applicable to the method chosen to gather information, then describe in detail the problems you encountered and why. VI. Make Suggestions for Further Research
You may choose to conclude the discussion section by making suggestions for further research [as opposed to offering suggestions in the conclusion of your paper]. Although your study can offer important insights about the research problem, this is where you can address other questions related to the problem that remain unanswered or highlight hidden issues that were revealed as a result of conducting your research. You should frame your suggestions by linking the need for further research to the limitations of your study [e.g., in future studies, the survey instrument should include more questions that ask..."] or linking to critical issues revealed from the data that were not considered initially in your research.
NOTE: Besides the literature review section, the preponderance of references to sources is usually found in the discussion section . A few historical references may be helpful for perspective, but most of the references should be relatively recent and included to aid in the interpretation of your results, to support the significance of a finding, and/or to place a finding within a particular context. If a study that you cited does not support your findings, don't ignore it--clearly explain why your research findings differ from theirs.
V. Problems to Avoid
- Do not waste time restating your results . Should you need to remind the reader of a finding to be discussed, use "bridge sentences" that relate the result to the interpretation. An example would be: “In the case of determining available housing to single women with children in rural areas of Texas, the findings suggest that access to good schools is important...," then move on to further explaining this finding and its implications.
- As noted, recommendations for further research can be included in either the discussion or conclusion of your paper, but do not repeat your recommendations in the both sections. Think about the overall narrative flow of your paper to determine where best to locate this information. However, if your findings raise a lot of new questions or issues, consider including suggestions for further research in the discussion section.
- Do not introduce new results in the discussion section. Be wary of mistaking the reiteration of a specific finding for an interpretation because it may confuse the reader. The description of findings [results section] and the interpretation of their significance [discussion section] should be distinct parts of your paper. If you choose to combine the results section and the discussion section into a single narrative, you must be clear in how you report the information discovered and your own interpretation of each finding. This approach is not recommended if you lack experience writing college-level research papers.
- Use of the first person pronoun is generally acceptable. Using first person singular pronouns can help emphasize a point or illustrate a contrasting finding. However, keep in mind that too much use of the first person can actually distract the reader from the main points [i.e., I know you're telling me this--just tell me!].
Analyzing vs. Summarizing. Department of English Writing Guide. George Mason University; Discussion. The Structure, Format, Content, and Style of a Journal-Style Scientific Paper. Department of Biology. Bates College; Hess, Dean R. "How to Write an Effective Discussion." Respiratory Care 49 (October 2004); Kretchmer, Paul. Fourteen Steps to Writing to Writing an Effective Discussion Section. San Francisco Edit, 2003-2008; The Lab Report. University College Writing Centre. University of Toronto; Sauaia, A. et al. "The Anatomy of an Article: The Discussion Section: "How Does the Article I Read Today Change What I Will Recommend to my Patients Tomorrow?” The Journal of Trauma and Acute Care Surgery 74 (June 2013): 1599-1602; Research Limitations & Future Research . Lund Research Ltd., 2012; Summary: Using it Wisely. The Writing Center. University of North Carolina; Schafer, Mickey S. Writing the Discussion. Writing in Psychology course syllabus. University of Florida; Yellin, Linda L. A Sociology Writer's Guide . Boston, MA: Allyn and Bacon, 2009.
Writing Tip
Don’t Over-Interpret the Results!
Interpretation is a subjective exercise. As such, you should always approach the selection and interpretation of your findings introspectively and to think critically about the possibility of judgmental biases unintentionally entering into discussions about the significance of your work. With this in mind, be careful that you do not read more into the findings than can be supported by the evidence you have gathered. Remember that the data are the data: nothing more, nothing less.
MacCoun, Robert J. "Biases in the Interpretation and Use of Research Results." Annual Review of Psychology 49 (February 1998): 259-287; Ward, Paulet al, editors. The Oxford Handbook of Expertise . Oxford, UK: Oxford University Press, 2018.
Another Writing Tip
Don't Write Two Results Sections!
One of the most common mistakes that you can make when discussing the results of your study is to present a superficial interpretation of the findings that more or less re-states the results section of your paper. Obviously, you must refer to your results when discussing them, but focus on the interpretation of those results and their significance in relation to the research problem, not the data itself.
Azar, Beth. "Discussing Your Findings." American Psychological Association gradPSYCH Magazine (January 2006).
Yet Another Writing Tip
Avoid Unwarranted Speculation!
The discussion section should remain focused on the findings of your study. For example, if the purpose of your research was to measure the impact of foreign aid on increasing access to education among disadvantaged children in Bangladesh, it would not be appropriate to speculate about how your findings might apply to populations in other countries without drawing from existing studies to support your claim or if analysis of other countries was not a part of your original research design. If you feel compelled to speculate, do so in the form of describing possible implications or explaining possible impacts. Be certain that you clearly identify your comments as speculation or as a suggestion for where further research is needed. Sometimes your professor will encourage you to expand your discussion of the results in this way, while others don’t care what your opinion is beyond your effort to interpret the data in relation to the research problem.
- << Previous: Using Non-Textual Elements
- Next: Limitations of the Study >>
- Last Updated: Jun 18, 2024 10:45 AM
- URL: https://libguides.usc.edu/writingguide
When you choose to publish with PLOS, your research makes an impact. Make your work accessible to all, without restrictions, and accelerate scientific discovery with options like preprints and published peer review that make your work more Open.
- PLOS Biology
- PLOS Climate
- PLOS Complex Systems
- PLOS Computational Biology
- PLOS Digital Health
- PLOS Genetics
- PLOS Global Public Health
- PLOS Medicine
- PLOS Mental Health
- PLOS Neglected Tropical Diseases
- PLOS Pathogens
- PLOS Sustainability and Transformation
- PLOS Collections
- How to Write Discussions and Conclusions

The discussion section contains the results and outcomes of a study. An effective discussion informs readers what can be learned from your experiment and provides context for the results.
What makes an effective discussion?
When you’re ready to write your discussion, you’ve already introduced the purpose of your study and provided an in-depth description of the methodology. The discussion informs readers about the larger implications of your study based on the results. Highlighting these implications while not overstating the findings can be challenging, especially when you’re submitting to a journal that selects articles based on novelty or potential impact. Regardless of what journal you are submitting to, the discussion section always serves the same purpose: concluding what your study results actually mean.
A successful discussion section puts your findings in context. It should include:
- the results of your research,
- a discussion of related research, and
- a comparison between your results and initial hypothesis.
Tip: Not all journals share the same naming conventions.
You can apply the advice in this article to the conclusion, results or discussion sections of your manuscript.
Our Early Career Researcher community tells us that the conclusion is often considered the most difficult aspect of a manuscript to write. To help, this guide provides questions to ask yourself, a basic structure to model your discussion off of and examples from published manuscripts.

Questions to ask yourself:
- Was my hypothesis correct?
- If my hypothesis is partially correct or entirely different, what can be learned from the results?
- How do the conclusions reshape or add onto the existing knowledge in the field? What does previous research say about the topic?
- Why are the results important or relevant to your audience? Do they add further evidence to a scientific consensus or disprove prior studies?
- How can future research build on these observations? What are the key experiments that must be done?
- What is the “take-home” message you want your reader to leave with?
How to structure a discussion
Trying to fit a complete discussion into a single paragraph can add unnecessary stress to the writing process. If possible, you’ll want to give yourself two or three paragraphs to give the reader a comprehensive understanding of your study as a whole. Here’s one way to structure an effective discussion:
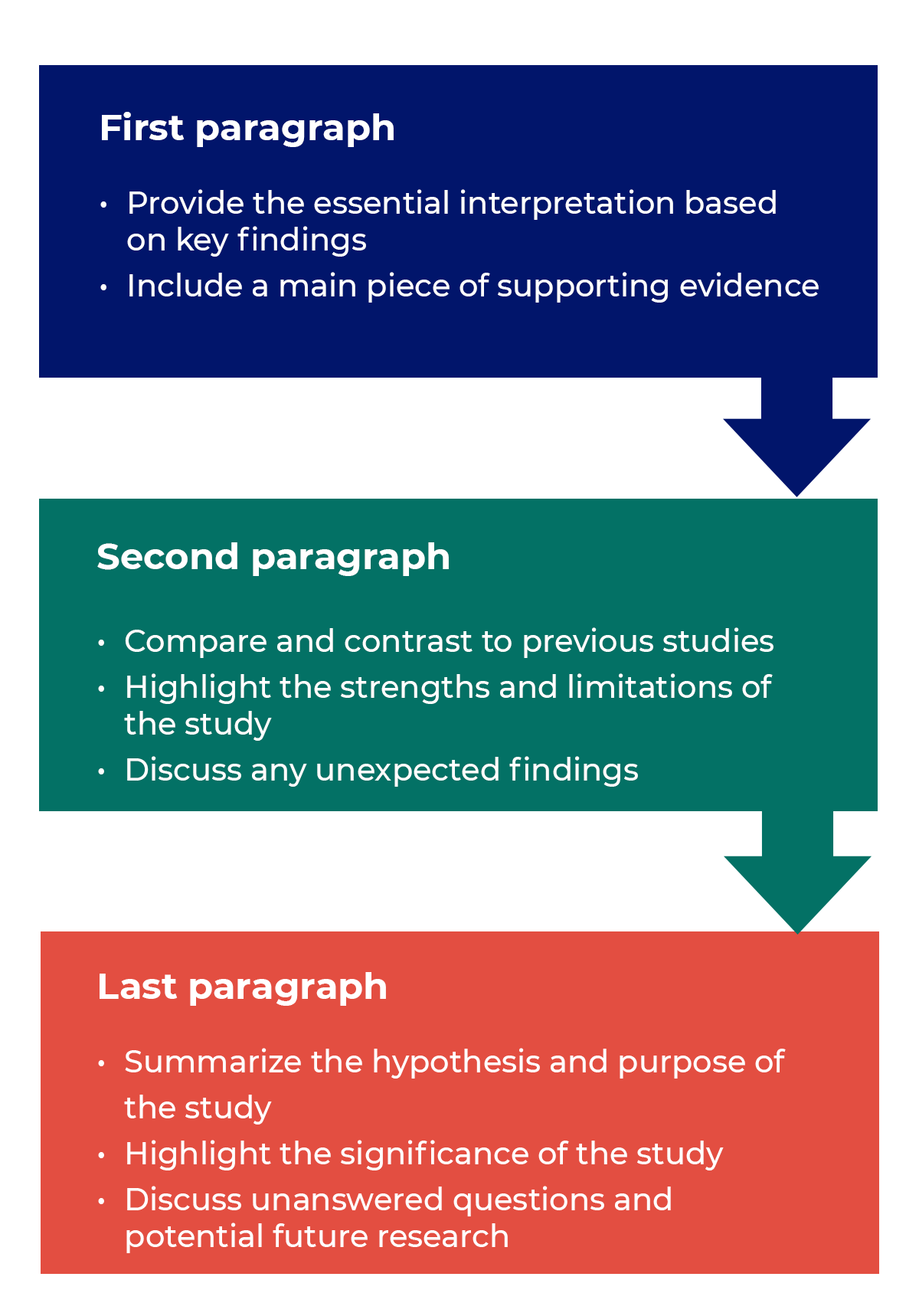
Writing Tips
While the above sections can help you brainstorm and structure your discussion, there are many common mistakes that writers revert to when having difficulties with their paper. Writing a discussion can be a delicate balance between summarizing your results, providing proper context for your research and avoiding introducing new information. Remember that your paper should be both confident and honest about the results!

- Read the journal’s guidelines on the discussion and conclusion sections. If possible, learn about the guidelines before writing the discussion to ensure you’re writing to meet their expectations.
- Begin with a clear statement of the principal findings. This will reinforce the main take-away for the reader and set up the rest of the discussion.
- Explain why the outcomes of your study are important to the reader. Discuss the implications of your findings realistically based on previous literature, highlighting both the strengths and limitations of the research.
- State whether the results prove or disprove your hypothesis. If your hypothesis was disproved, what might be the reasons?
- Introduce new or expanded ways to think about the research question. Indicate what next steps can be taken to further pursue any unresolved questions.
- If dealing with a contemporary or ongoing problem, such as climate change, discuss possible consequences if the problem is avoided.
- Be concise. Adding unnecessary detail can distract from the main findings.
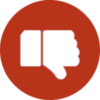
Don’t
- Rewrite your abstract. Statements with “we investigated” or “we studied” generally do not belong in the discussion.
- Include new arguments or evidence not previously discussed. Necessary information and evidence should be introduced in the main body of the paper.
- Apologize. Even if your research contains significant limitations, don’t undermine your authority by including statements that doubt your methodology or execution.
- Shy away from speaking on limitations or negative results. Including limitations and negative results will give readers a complete understanding of the presented research. Potential limitations include sources of potential bias, threats to internal or external validity, barriers to implementing an intervention and other issues inherent to the study design.
- Overstate the importance of your findings. Making grand statements about how a study will fully resolve large questions can lead readers to doubt the success of the research.
Snippets of Effective Discussions:
Consumer-based actions to reduce plastic pollution in rivers: A multi-criteria decision analysis approach
Identifying reliable indicators of fitness in polar bears
- How to Write a Great Title
- How to Write an Abstract
- How to Write Your Methods
- How to Report Statistics
- How to Edit Your Work
The contents of the Peer Review Center are also available as a live, interactive training session, complete with slides, talking points, and activities. …
The contents of the Writing Center are also available as a live, interactive training session, complete with slides, talking points, and activities. …
There’s a lot to consider when deciding where to submit your work. Learn how to choose a journal that will help your study reach its audience, while reflecting your values as a researcher…
How to Write the Discussion Section of a Research Paper
The discussion section of a research paper analyzes and interprets the findings, provides context, compares them with previous studies, identifies limitations, and suggests future research directions.
Updated on September 15, 2023

Structure your discussion section right, and you’ll be cited more often while doing a greater service to the scientific community. So, what actually goes into the discussion section? And how do you write it?
The discussion section of your research paper is where you let the reader know how your study is positioned in the literature, what to take away from your paper, and how your work helps them. It can also include your conclusions and suggestions for future studies.
First, we’ll define all the parts of your discussion paper, and then look into how to write a strong, effective discussion section for your paper or manuscript.
Discussion section: what is it, what it does
The discussion section comes later in your paper, following the introduction, methods, and results. The discussion sets up your study’s conclusions. Its main goals are to present, interpret, and provide a context for your results.
What is it?
The discussion section provides an analysis and interpretation of the findings, compares them with previous studies, identifies limitations, and suggests future directions for research.
This section combines information from the preceding parts of your paper into a coherent story. By this point, the reader already knows why you did your study (introduction), how you did it (methods), and what happened (results). In the discussion, you’ll help the reader connect the ideas from these sections.
Why is it necessary?
The discussion provides context and interpretations for the results. It also answers the questions posed in the introduction. While the results section describes your findings, the discussion explains what they say. This is also where you can describe the impact or implications of your research.
Adds context for your results
Most research studies aim to answer a question, replicate a finding, or address limitations in the literature. These goals are first described in the introduction. However, in the discussion section, the author can refer back to them to explain how the study's objective was achieved.
Shows what your results actually mean and real-world implications
The discussion can also describe the effect of your findings on research or practice. How are your results significant for readers, other researchers, or policymakers?
What to include in your discussion (in the correct order)
A complete and effective discussion section should at least touch on the points described below.
Summary of key findings
The discussion should begin with a brief factual summary of the results. Concisely overview the main results you obtained.
Begin with key findings with supporting evidence
Your results section described a list of findings, but what message do they send when you look at them all together?
Your findings were detailed in the results section, so there’s no need to repeat them here, but do provide at least a few highlights. This will help refresh the reader’s memory and help them focus on the big picture.
Read the first paragraph of the discussion section in this article (PDF) for an example of how to start this part of your paper. Notice how the authors break down their results and follow each description sentence with an explanation of why each finding is relevant.
State clearly and concisely
Following a clear and direct writing style is especially important in the discussion section. After all, this is where you will make some of the most impactful points in your paper. While the results section often contains technical vocabulary, such as statistical terms, the discussion section lets you describe your findings more clearly.
Interpretation of results
Once you’ve given your reader an overview of your results, you need to interpret those results. In other words, what do your results mean? Discuss the findings’ implications and significance in relation to your research question or hypothesis.
Analyze and interpret your findings
Look into your findings and explore what’s behind them or what may have caused them. If your introduction cited theories or studies that could explain your findings, use these sources as a basis to discuss your results.
For example, look at the second paragraph in the discussion section of this article on waggling honey bees. Here, the authors explore their results based on information from the literature.
Unexpected or contradictory results
Sometimes, your findings are not what you expect. Here’s where you describe this and try to find a reason for it. Could it be because of the method you used? Does it have something to do with the variables analyzed? Comparing your methods with those of other similar studies can help with this task.
Context and comparison with previous work
Refer to related studies to place your research in a larger context and the literature. Compare and contrast your findings with existing literature, highlighting similarities, differences, and/or contradictions.
How your work compares or contrasts with previous work
Studies with similar findings to yours can be cited to show the strength of your findings. Information from these studies can also be used to help explain your results. Differences between your findings and others in the literature can also be discussed here.
How to divide this section into subsections
If you have more than one objective in your study or many key findings, you can dedicate a separate section to each of these. Here’s an example of this approach. You can see that the discussion section is divided into topics and even has a separate heading for each of them.
Limitations
Many journals require you to include the limitations of your study in the discussion. Even if they don’t, there are good reasons to mention these in your paper.
Why limitations don’t have a negative connotation
A study’s limitations are points to be improved upon in future research. While some of these may be flaws in your method, many may be due to factors you couldn’t predict.
Examples include time constraints or small sample sizes. Pointing this out will help future researchers avoid or address these issues. This part of the discussion can also include any attempts you have made to reduce the impact of these limitations, as in this study .
How limitations add to a researcher's credibility
Pointing out the limitations of your study demonstrates transparency. It also shows that you know your methods well and can conduct a critical assessment of them.
Implications and significance
The final paragraph of the discussion section should contain the take-home messages for your study. It can also cite the “strong points” of your study, to contrast with the limitations section.
Restate your hypothesis
Remind the reader what your hypothesis was before you conducted the study.
How was it proven or disproven?
Identify your main findings and describe how they relate to your hypothesis.
How your results contribute to the literature
Were you able to answer your research question? Or address a gap in the literature?
Future implications of your research
Describe the impact that your results may have on the topic of study. Your results may show, for instance, that there are still limitations in the literature for future studies to address. There may be a need for studies that extend your findings in a specific way. You also may need additional research to corroborate your findings.
Sample discussion section
This fictitious example covers all the aspects discussed above. Your actual discussion section will probably be much longer, but you can read this to get an idea of everything your discussion should cover.
Our results showed that the presence of cats in a household is associated with higher levels of perceived happiness by its human occupants. These findings support our hypothesis and demonstrate the association between pet ownership and well-being.
The present findings align with those of Bao and Schreer (2016) and Hardie et al. (2023), who observed greater life satisfaction in pet owners relative to non-owners. Although the present study did not directly evaluate life satisfaction, this factor may explain the association between happiness and cat ownership observed in our sample.
Our findings must be interpreted in light of some limitations, such as the focus on cat ownership only rather than pets as a whole. This may limit the generalizability of our results.
Nevertheless, this study had several strengths. These include its strict exclusion criteria and use of a standardized assessment instrument to investigate the relationships between pets and owners. These attributes bolster the accuracy of our results and reduce the influence of confounding factors, increasing the strength of our conclusions. Future studies may examine the factors that mediate the association between pet ownership and happiness to better comprehend this phenomenon.
This brief discussion begins with a quick summary of the results and hypothesis. The next paragraph cites previous research and compares its findings to those of this study. Information from previous studies is also used to help interpret the findings. After discussing the results of the study, some limitations are pointed out. The paper also explains why these limitations may influence the interpretation of results. Then, final conclusions are drawn based on the study, and directions for future research are suggested.
How to make your discussion flow naturally
If you find writing in scientific English challenging, the discussion and conclusions are often the hardest parts of the paper to write. That’s because you’re not just listing up studies, methods, and outcomes. You’re actually expressing your thoughts and interpretations in words.
- How formal should it be?
- What words should you use, or not use?
- How do you meet strict word limits, or make it longer and more informative?
Always give it your best, but sometimes a helping hand can, well, help. Getting a professional edit can help clarify your work’s importance while improving the English used to explain it. When readers know the value of your work, they’ll cite it. We’ll assign your study to an expert editor knowledgeable in your area of research. Their work will clarify your discussion, helping it to tell your story. Find out more about AJE Editing.

Adam Goulston, PsyD, MS, MBA, MISD, ELS
Science Marketing Consultant
See our "Privacy Policy"
Ensure your structure and ideas are consistent and clearly communicated
Pair your Premium Editing with our add-on service Presubmission Review for an overall assessment of your manuscript.
- Research Process
- Manuscript Preparation
- Manuscript Review
- Publication Process
- Publication Recognition
- Language Editing Services
- Translation Services
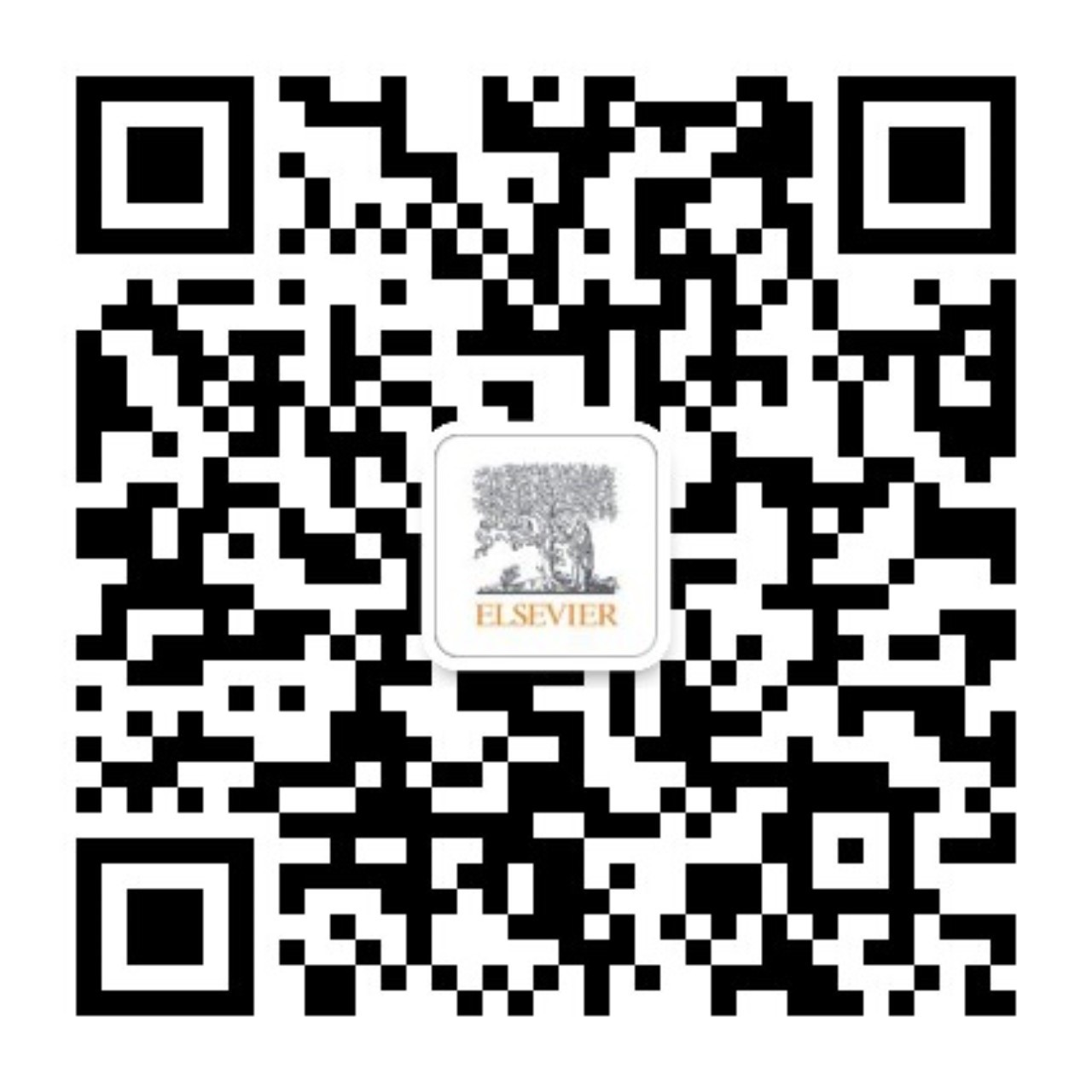
6 Steps to Write an Excellent Discussion in Your Manuscript
- 4 minute read
- 16.2K views
Table of Contents
The discussion section in scientific manuscripts might be the last few paragraphs, but its role goes far beyond wrapping up. It’s the part of an article where scientists talk about what they found and what it means, where raw data turns into meaningful insights. Therefore, discussion is a vital component of the article.
An excellent discussion is well-organized. We bring to you authors a classic 6-step method for writing discussion sections, with examples to illustrate the functions and specific writing logic of each step. Take a look at how you can impress journal reviewers with a concise and focused discussion section!
Discussion frame structure
Conventionally, a discussion section has three parts: an introductory paragraph, a few intermediate paragraphs, and a conclusion¹. Please follow the steps below:
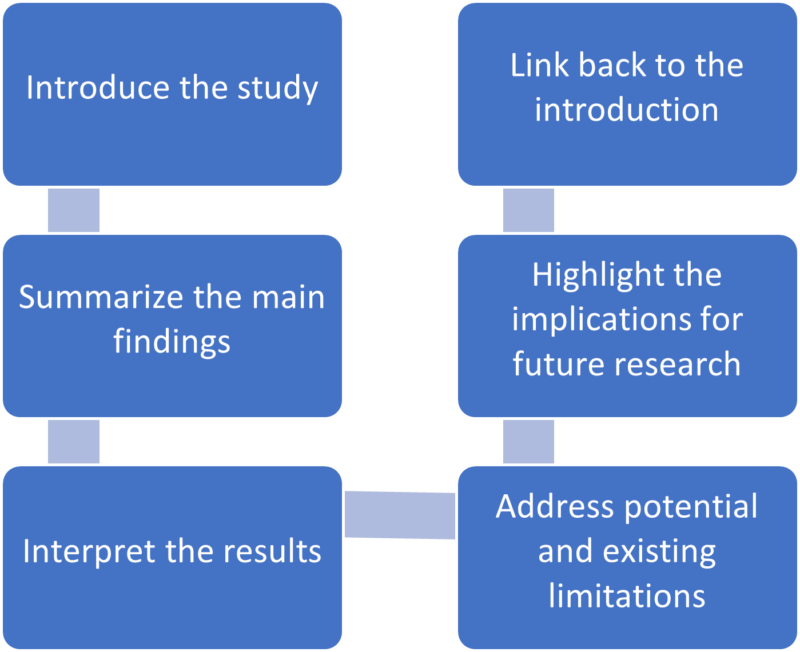
1.Introduction—mention gaps in previous research¹⁻ ²
Here, you orient the reader to your study. In the first paragraph, it is advisable to mention the research gap your paper addresses.
Example: This study investigated the cognitive effects of a meat-only diet on adults. While earlier studies have explored the impact of a carnivorous diet on physical attributes and agility, they have not explicitly addressed its influence on cognitively intense tasks involving memory and reasoning.
2. Summarizing key findings—let your data speak ¹⁻ ²
After you have laid out the context for your study, recapitulate some of its key findings. Also, highlight key data and evidence supporting these findings.
Example: We found that risk-taking behavior among teenagers correlates with their tendency to invest in cryptocurrencies. Risk takers in this study, as measured by the Cambridge Gambling Task, tended to have an inordinately higher proportion of their savings invested as crypto coins.
3. Interpreting results—compare with other papers¹⁻²
Here, you must analyze and interpret any results concerning the research question or hypothesis. How do the key findings of your study help verify or disprove the hypothesis? What practical relevance does your discovery have?
Example: Our study suggests that higher daily caffeine intake is not associated with poor performance in major sporting events. Athletes may benefit from the cardiovascular benefits of daily caffeine intake without adversely impacting performance.
Remember, unlike the results section, the discussion ideally focuses on locating your findings in the larger body of existing research. Hence, compare your results with those of other peer-reviewed papers.
Example: Although Miller et al. (2020) found evidence of such political bias in a multicultural population, our findings suggest that the bias is weak or virtually non-existent among politically active citizens.
4. Addressing limitations—their potential impact on the results¹⁻²
Discuss the potential impact of limitations on the results. Most studies have limitations, and it is crucial to acknowledge them in the intermediary paragraphs of the discussion section. Limitations may include low sample size, suspected interference or noise in data, low effect size, etc.
Example: This study explored a comprehensive list of adverse effects associated with the novel drug ‘X’. However, long-term studies may be needed to confirm its safety, especially regarding major cardiac events.
5. Implications for future research—how to explore further¹⁻²
Locate areas of your research where more investigation is needed. Concluding paragraphs of the discussion can explain what research will likely confirm your results or identify knowledge gaps your study left unaddressed.
Example: Our study demonstrates that roads paved with the plastic-infused compound ‘Y’ are more resilient than asphalt. Future studies may explore economically feasible ways of producing compound Y in bulk.
6. Conclusion—summarize content¹⁻²
A good way to wind up the discussion section is by revisiting the research question mentioned in your introduction. Sign off by expressing the main findings of your study.
Example: Recent observations suggest that the fish ‘Z’ is moving upriver in many parts of the Amazon basin. Our findings provide conclusive evidence that this phenomenon is associated with rising sea levels and climate change, not due to elevated numbers of invasive predators.
A rigorous and concise discussion section is one of the keys to achieving an excellent paper. It serves as a critical platform for researchers to interpret and connect their findings with the broader scientific context. By detailing the results, carefully comparing them with existing research, and explaining the limitations of this study, you can effectively help reviewers and readers understand the entire research article more comprehensively and deeply¹⁻² , thereby helping your manuscript to be successfully published and gain wider dissemination.
In addition to keeping this writing guide, you can also use Elsevier Language Services to improve the quality of your paper more deeply and comprehensively. We have a professional editing team covering multiple disciplines. With our profound disciplinary background and rich polishing experience, we can significantly optimize all paper modules including the discussion, effectively improve the fluency and rigor of your articles, and make your scientific research results consistent, with its value reflected more clearly. We are always committed to ensuring the quality of papers according to the standards of top journals, improving the publishing efficiency of scientific researchers, and helping you on the road to academic success. Check us out here !
Type in wordcount for Standard Total: USD EUR JPY Follow this link if your manuscript is longer than 12,000 words. Upload
References:
- Masic, I. (2018). How to write an efficient discussion? Medical Archives , 72(3), 306. https://doi.org/10.5455/medarh.2018.72.306-307
- Şanlı, Ö., Erdem, S., & Tefik, T. (2014). How to write a discussion section? Urology Research & Practice , 39(1), 20–24. https://doi.org/10.5152/tud.2013.049
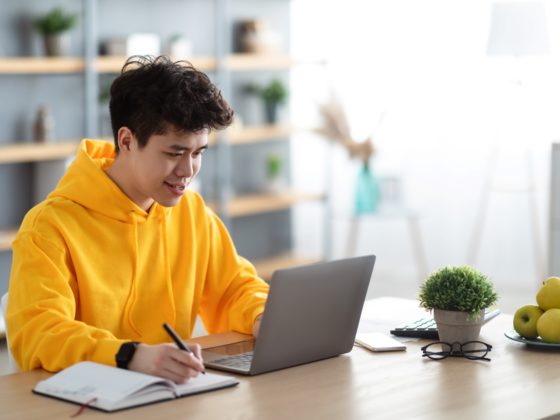
Navigating “Chinglish” Errors in Academic English Writing
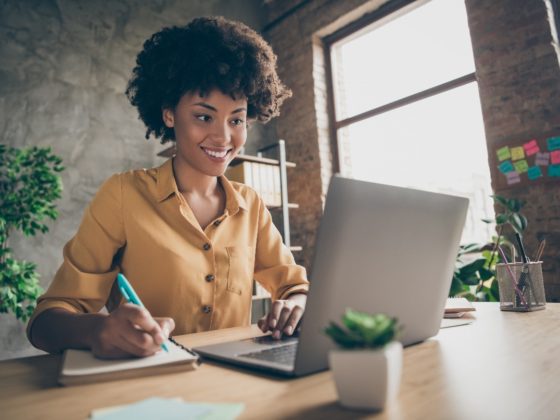
A Guide to Crafting Shorter, Impactful Sentences in Academic Writing
You may also like.
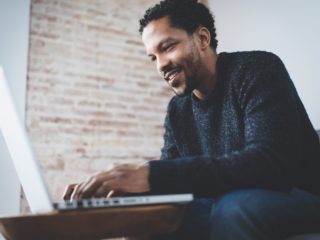
Page-Turner Articles are More Than Just Good Arguments: Be Mindful of Tone and Structure!
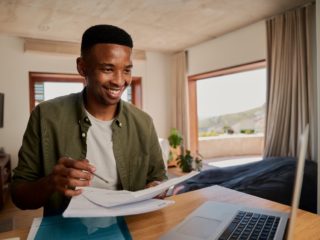
A Must-see for Researchers! How to Ensure Inclusivity in Your Scientific Writing
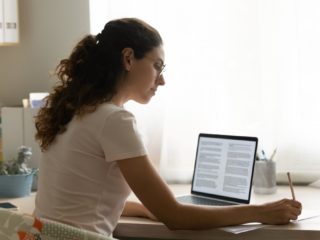
Make Hook, Line, and Sinker: The Art of Crafting Engaging Introductions
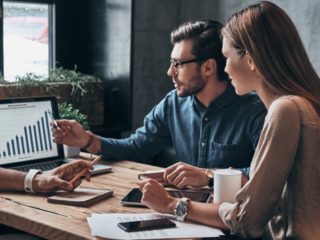
Can Describing Study Limitations Improve the Quality of Your Paper?
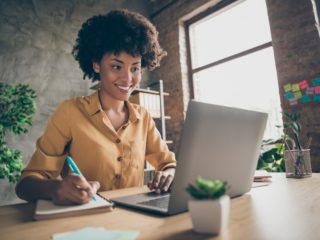
How to Write Clear and Crisp Civil Engineering Papers? Here are 5 Key Tips to Consider
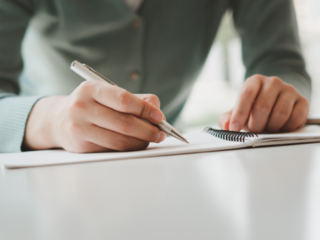
The Clear Path to An Impactful Paper: ②
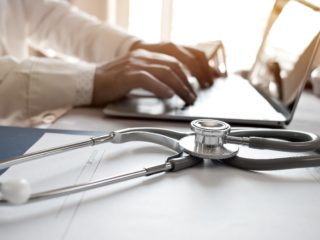
The Essentials of Writing to Communicate Research in Medicine
Input your search keywords and press Enter.

Organizing Academic Research Papers: 8. The Discussion
- Purpose of Guide
- Design Flaws to Avoid
- Glossary of Research Terms
- Narrowing a Topic Idea
- Broadening a Topic Idea
- Extending the Timeliness of a Topic Idea
- Academic Writing Style
- Choosing a Title
- Making an Outline
- Paragraph Development
- Executive Summary
- Background Information
- The Research Problem/Question
- Theoretical Framework
- Citation Tracking
- Content Alert Services
- Evaluating Sources
- Primary Sources
- Secondary Sources
- Tertiary Sources
- What Is Scholarly vs. Popular?
- Qualitative Methods
- Quantitative Methods
- Using Non-Textual Elements
- Limitations of the Study
- Common Grammar Mistakes
- Avoiding Plagiarism
- Footnotes or Endnotes?
- Further Readings
- Annotated Bibliography
- Dealing with Nervousness
- Using Visual Aids
- Grading Someone Else's Paper
- How to Manage Group Projects
- Multiple Book Review Essay
- Reviewing Collected Essays
- About Informed Consent
- Writing Field Notes
- Writing a Policy Memo
- Writing a Research Proposal
- Acknowledgements
The purpose of the discussion is to interpret and describe the significance of your findings in light of what was already known about the research problem being investigated, and to explain any new understanding or fresh insights about the problem after you've taken the findings into consideration. The discussion will always connect to the introduction by way of the research questions or hypotheses you posed and the literature you reviewed, but it does not simply repeat or rearrange the introduction; the discussion should always explain how your study has moved the reader's understanding of the research problem forward from where you left them at the end of the introduction.
Importance of a Good Discussion
This section is often considered the most important part of a research paper because it most effectively demonstrates your ability as a researcher to think critically about an issue, to develop creative solutions to problems based on the findings, and to formulate a deeper, more profound understanding of the research problem you are studying.
The discussion section is where you explore the underlying meaning of your research , its possible implications in other areas of study, and the possible improvements that can be made in order to further develop the concerns of your research.
This is the section where you need to present the importance of your study and how it may be able to contribute to and/or fill existing gaps in the field. If appropriate, the discussion section is also where you state how the findings from your study revealed new gaps in the literature that had not been previously exposed or adequately described.
This part of the paper is not strictly governed by objective reporting of information but, rather, it is where you can engage in creative thinking about issues through evidence-based interpretation of findings. This is where you infuse your results with meaning.
Kretchmer, Paul. Fourteen Steps to Writing to Writing an Effective Discussion Section . San Francisco Edit, 2003-2008.
Structure and Writing Style
I. General Rules
These are the general rules you should adopt when composing your discussion of the results :
- Do not be verbose or repetitive.
- Be concise and make your points clearly.
- Avoid using jargon.
- Follow a logical stream of thought.
- Use the present verb tense, especially for established facts; however, refer to specific works and references in the past tense.
- If needed, use subheadings to help organize your presentation or to group your interpretations into themes.
II. The Content
The content of the discussion section of your paper most often includes :
- Explanation of results : comment on whether or not the results were expected and present explanations for the results; go into greater depth when explaining findings that were unexpected or especially profound. If appropriate, note any unusual or unanticipated patterns or trends that emerged from your results and explain their meaning.
- References to previous research : compare your results with the findings from other studies, or use the studies to support a claim. This can include re-visiting key sources already cited in your literature review section, or, save them to cite later in the discussion section if they are more important to compare with your results than being part of the general research you cited to provide context and background information.
- Deduction : a claim for how the results can be applied more generally. For example, describing lessons learned, proposing recommendations that can help improve a situation, or recommending best practices.
- Hypothesis : a more general claim or possible conclusion arising from the results [which may be proved or disproved in subsequent research].
III. Organization and Structure
Keep the following sequential points in mind as you organize and write the discussion section of your paper:
- Think of your discussion as an inverted pyramid. Organize the discussion from the general to the specific, linking your findings to the literature, then to theory, then to practice [if appropriate].
- Use the same key terms, mode of narration, and verb tense [present] that you used when when describing the research problem in the introduction.
- Begin by briefly re-stating the research problem you were investigating and answer all of the research questions underpinning the problem that you posed in the introduction.
- Describe the patterns, principles, and relationships shown by each major findings and place them in proper perspective. The sequencing of providing this information is important; first state the answer, then the relevant results, then cite the work of others. If appropriate, refer the reader to a figure or table to help enhance the interpretation of the data. The order of interpreting each major finding should be in the same order as they were described in your results section.
- A good discussion section includes analysis of any unexpected findings. This paragraph should begin with a description of the unexpected finding, followed by a brief interpretation as to why you believe it appeared and, if necessary, its possible significance in relation to the overall study. If more than one unexpected finding emerged during the study, describe each them in the order they appeared as you gathered the data.
- Before concluding the discussion, identify potential limitations and weaknesses. Comment on their relative importance in relation to your overall interpretation of the results and, if necessary, note how they may affect the validity of the findings. Avoid using an apologetic tone; however, be honest and self-critical.
- The discussion section should end with a concise summary of the principal implications of the findings regardless of statistical significance. Give a brief explanation about why you believe the findings and conclusions of your study are important and how they support broader knowledge or understanding of the research problem. This can be followed by any recommendations for further research. However, do not offer recommendations which could have been easily addressed within the study. This demonstrates to the reader you have inadequately examined and interpreted the data.
IV. Overall Objectives
The objectives of your discussion section should include the following: I. Reiterate the Research Problem/State the Major Findings
Briefly reiterate for your readers the research problem or problems you are investigating and the methods you used to investigate them, then move quickly to describe the major findings of the study. You should write a direct, declarative, and succinct proclamation of the study results.
II. Explain the Meaning of the Findings and Why They are Important
No one has thought as long and hard about your study as you have. Systematically explain the meaning of the findings and why you believe they are important. After reading the discussion section, you want the reader to think about the results [“why hadn’t I thought of that?”]. You don’t want to force the reader to go through the paper multiple times to figure out what it all means. Begin this part of the section by repeating what you consider to be your most important finding first.
III. Relate the Findings to Similar Studies
No study is so novel or possesses such a restricted focus that it has absolutely no relation to other previously published research. The discussion section should relate your study findings to those of other studies, particularly if questions raised by previous studies served as the motivation for your study, the findings of other studies support your findings [which strengthens the importance of your study results], and/or they point out how your study differs from other similar studies. IV. Consider Alternative Explanations of the Findings
It is important to remember that the purpose of research is to discover and not to prove . When writing the discussion section, you should carefully consider all possible explanations for the study results, rather than just those that fit your prior assumptions or biases.
V. Acknowledge the Study’s Limitations
It is far better for you to identify and acknowledge your study’s limitations than to have them pointed out by your professor! Describe the generalizability of your results to other situations, if applicable to the method chosen, then describe in detail problems you encountered in the method(s) you used to gather information. Note any unanswered questions or issues your study did not address, and.... VI. Make Suggestions for Further Research
Although your study may offer important insights about the research problem, other questions related to the problem likely remain unanswered. Moreover, some unanswered questions may have become more focused because of your study. You should make suggestions for further research in the discussion section.
NOTE: Besides the literature review section, the preponderance of references to sources in your research paper are usually found in the discussion section . A few historical references may be helpful for perspective but most of the references should be relatively recent and included to aid in the interpretation of your results and/or linked to similar studies. If a study that you cited disagrees with your findings, don't ignore it--clearly explain why the study's findings differ from yours.
V. Problems to Avoid
- Do not waste entire sentences restating your results . Should you need to remind the reader of the finding to be discussed, use "bridge sentences" that relate the result to the interpretation. An example would be: “The lack of available housing to single women with children in rural areas of Texas suggests that...[then move to the interpretation of this finding].”
- Recommendations for further research can be included in either the discussion or conclusion of your paper but do not repeat your recommendations in the both sections.
- Do not introduce new results in the discussion. Be wary of mistaking the reiteration of a specific finding for an interpretation.
- Use of the first person is acceptable, but too much use of the first person may actually distract the reader from the main points.
Analyzing vs. Summarizing. Department of English Writing Guide. George Mason University; Discussion . The Structure, Format, Content, and Style of a Journal-Style Scientific Paper. Department of Biology. Bates College; Hess, Dean R. How to Write an Effective Discussion. Respiratory Care 49 (October 2004); Kretchmer, Paul. Fourteen Steps to Writing to Writing an Effective Discussion Section . San Francisco Edit, 2003-2008; The Lab Report . University College Writing Centre. University of Toronto; Summary: Using it Wisely . The Writing Center. University of North Carolina; Schafer, Mickey S. Writing the Discussion . Writing in Psychology course syllabus. University of Florida; Yellin, Linda L. A Sociology Writer's Guide. Boston, MA: Allyn and Bacon, 2009.
Writing Tip
Don’t Overinterpret the Results!
Interpretation is a subjective exercise. Therefore, be careful that you do not read more into the findings than can be supported by the evidence you've gathered. Remember that the data are the data: nothing more, nothing less.
Another Writing Tip
Don't Write Two Results Sections!
One of the most common mistakes that you can make when discussing the results of your study is to present a superficial interpretation of the findings that more or less re-states the results section of your paper. Obviously, you must refer to your results when discussing them, but focus on the interpretion of those results, not just the data itself.
Azar, Beth. Discussing Your Findings. American Psychological Association gradPSYCH Magazine (January 2006)
Yet Another Writing Tip
Avoid Unwarranted Speculation!
The discussion section should remain focused on the findings of your study. For example, if you studied the impact of foreign aid on increasing levels of education among the poor in Bangladesh, it's generally not appropriate to speculate about how your findings might apply to populations in other countries without drawing from existing studies to support your claim. If you feel compelled to speculate, be certain that you clearly identify your comments as speculation or as a suggestion for where further research is needed. Sometimes your professor will encourage you to expand the discussion in this way, while others don’t care what your opinion is beyond your efforts to interpret the data.
- << Previous: Using Non-Textual Elements
- Next: Limitations of the Study >>
- Last Updated: Jul 18, 2023 11:58 AM
- URL: https://library.sacredheart.edu/c.php?g=29803
- QuickSearch
- Library Catalog
- Databases A-Z
- Publication Finder
- Course Reserves
- Citation Linker
- Digital Commons
- Our Website
Research Support
- Ask a Librarian
- Appointments
- Interlibrary Loan (ILL)
- Research Guides
- Databases by Subject
- Citation Help
Using the Library
- Reserve a Group Study Room
- Renew Books
- Honors Study Rooms
- Off-Campus Access
- Library Policies
- Library Technology
User Information
- Grad Students
- Online Students
- COVID-19 Updates
- Staff Directory
- News & Announcements
- Library Newsletter
My Accounts
- Interlibrary Loan
- Staff Site Login

FIND US ON
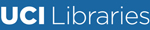
- Langson Library
- Science Library
- Grunigen Medical Library
- Law Library
- Connect From Off-Campus
- Accessibility
- Gateway Study Center
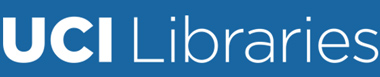
Email this link
Writing a scientific paper.
- Writing a lab report
- INTRODUCTION
Writing a "good" discussion section
"discussion and conclusions checklist" from: how to write a good scientific paper. chris a. mack. spie. 2018., peer review.
- LITERATURE CITED
- Bibliography of guides to scientific writing and presenting
- Presentations
- Lab Report Writing Guides on the Web
This is is usually the hardest section to write. You are trying to bring out the true meaning of your data without being too long. Do not use words to conceal your facts or reasoning. Also do not repeat your results, this is a discussion.
- Present principles, relationships and generalizations shown by the results
- Point out exceptions or lack of correlations. Define why you think this is so.
- Show how your results agree or disagree with previously published works
- Discuss the theoretical implications of your work as well as practical applications
- State your conclusions clearly. Summarize your evidence for each conclusion.
- Discuss the significance of the results
- Evidence does not explain itself; the results must be presented and then explained.
- Typical stages in the discussion: summarizing the results, discussing whether results are expected or unexpected, comparing these results to previous work, interpreting and explaining the results (often by comparison to a theory or model), and hypothesizing about their generality.
- Discuss any problems or shortcomings encountered during the course of the work.
- Discuss possible alternate explanations for the results.
- Avoid: presenting results that are never discussed; presenting discussion that does not relate to any of the results; presenting results and discussion in chronological order rather than logical order; ignoring results that do not support the conclusions; drawing conclusions from results without logical arguments to back them up.
CONCLUSIONS
- Provide a very brief summary of the Results and Discussion.
- Emphasize the implications of the findings, explaining how the work is significant and providing the key message(s) the author wishes to convey.
- Provide the most general claims that can be supported by the evidence.
- Provide a future perspective on the work.
- Avoid: repeating the abstract; repeating background information from the Introduction; introducing new evidence or new arguments not found in the Results and Discussion; repeating the arguments made in the Results and Discussion; failing to address all of the research questions set out in the Introduction.
WHAT HAPPENS AFTER I COMPLETE MY PAPER?
The peer review process is the quality control step in the publication of ideas. Papers that are submitted to a journal for publication are sent out to several scientists (peers) who look carefully at the paper to see if it is "good science". These reviewers then recommend to the editor of a journal whether or not a paper should be published. Most journals have publication guidelines. Ask for them and follow them exactly. Peer reviewers examine the soundness of the materials and methods section. Are the materials and methods used written clearly enough for another scientist to reproduce the experiment? Other areas they look at are: originality of research, significance of research question studied, soundness of the discussion and interpretation, correct spelling and use of technical terms, and length of the article.
- << Previous: RESULTS
- Next: LITERATURE CITED >>
- Last Updated: Aug 4, 2023 9:33 AM
- URL: https://guides.lib.uci.edu/scientificwriting
Off-campus? Please use the Software VPN and choose the group UCIFull to access licensed content. For more information, please Click here
Software VPN is not available for guests, so they may not have access to some content when connecting from off-campus.
- Affiliate Program

- UNITED STATES
- 台灣 (TAIWAN)
- TÜRKIYE (TURKEY)
- Academic Editing Services
- - Research Paper
- - Journal Manuscript
- - Dissertation
- - College & University Assignments
- Admissions Editing Services
- - Application Essay
- - Personal Statement
- - Recommendation Letter
- - Cover Letter
- - CV/Resume
- Business Editing Services
- - Business Documents
- - Report & Brochure
- - Website & Blog
- Writer Editing Services
- - Script & Screenplay
- Our Editors
- Client Reviews
- Editing & Proofreading Prices
- Wordvice Points
- Partner Discount
- Plagiarism Checker
- APA Citation Generator
- MLA Citation Generator
- Chicago Citation Generator
- Vancouver Citation Generator
- - APA Style
- - MLA Style
- - Chicago Style
- - Vancouver Style
- Writing & Editing Guide
- Academic Resources
- Admissions Resources
How to Write a Discussion Section for a Research Paper
We’ve talked about several useful writing tips that authors should consider while drafting or editing their research papers. In particular, we’ve focused on figures and legends , as well as the Introduction , Methods , and Results . Now that we’ve addressed the more technical portions of your journal manuscript, let’s turn to the analytical segments of your research article. In this article, we’ll provide tips on how to write a strong Discussion section that best portrays the significance of your research contributions.
What is the Discussion section of a research paper?
In a nutshell, your Discussion fulfills the promise you made to readers in your Introduction . At the beginning of your paper, you tell us why we should care about your research. You then guide us through a series of intricate images and graphs that capture all the relevant data you collected during your research. We may be dazzled and impressed at first, but none of that matters if you deliver an anti-climactic conclusion in the Discussion section!
Are you feeling pressured? Don’t worry. To be honest, you will edit the Discussion section of your manuscript numerous times. After all, in as little as one to two paragraphs ( Nature ‘s suggestion based on their 3,000-word main body text limit), you have to explain how your research moves us from point A (issues you raise in the Introduction) to point B (our new understanding of these matters). You must also recommend how we might get to point C (i.e., identify what you think is the next direction for research in this field). That’s a lot to say in two paragraphs!
So, how do you do that? Let’s take a closer look.
What should I include in the Discussion section?
As we stated above, the goal of your Discussion section is to answer the questions you raise in your Introduction by using the results you collected during your research . The content you include in the Discussions segment should include the following information:
- Remind us why we should be interested in this research project.
- Describe the nature of the knowledge gap you were trying to fill using the results of your study.
- Don’t repeat your Introduction. Instead, focus on why this particular study was needed to fill the gap you noticed and why that gap needed filling in the first place.
- Mainly, you want to remind us of how your research will increase our knowledge base and inspire others to conduct further research.
- Clearly tell us what that piece of missing knowledge was.
- Answer each of the questions you asked in your Introduction and explain how your results support those conclusions.
- Make sure to factor in all results relevant to the questions (even if those results were not statistically significant).
- Focus on the significance of the most noteworthy results.
- If conflicting inferences can be drawn from your results, evaluate the merits of all of them.
- Don’t rehash what you said earlier in the Results section. Rather, discuss your findings in the context of answering your hypothesis. Instead of making statements like “[The first result] was this…,” say, “[The first result] suggests [conclusion].”
- Do your conclusions line up with existing literature?
- Discuss whether your findings agree with current knowledge and expectations.
- Keep in mind good persuasive argument skills, such as explaining the strengths of your arguments and highlighting the weaknesses of contrary opinions.
- If you discovered something unexpected, offer reasons. If your conclusions aren’t aligned with current literature, explain.
- Address any limitations of your study and how relevant they are to interpreting your results and validating your findings.
- Make sure to acknowledge any weaknesses in your conclusions and suggest room for further research concerning that aspect of your analysis.
- Make sure your suggestions aren’t ones that should have been conducted during your research! Doing so might raise questions about your initial research design and protocols.
- Similarly, maintain a critical but unapologetic tone. You want to instill confidence in your readers that you have thoroughly examined your results and have objectively assessed them in a way that would benefit the scientific community’s desire to expand our knowledge base.
- Recommend next steps.
- Your suggestions should inspire other researchers to conduct follow-up studies to build upon the knowledge you have shared with them.
- Keep the list short (no more than two).
How to Write the Discussion Section
The above list of what to include in the Discussion section gives an overall idea of what you need to focus on throughout the section. Below are some tips and general suggestions about the technical aspects of writing and organization that you might find useful as you draft or revise the contents we’ve outlined above.
Technical writing elements
- Embrace active voice because it eliminates the awkward phrasing and wordiness that accompanies passive voice.
- Use the present tense, which should also be employed in the Introduction.
- Sprinkle with first person pronouns if needed, but generally, avoid it. We want to focus on your findings.
- Maintain an objective and analytical tone.
Discussion section organization
- Keep the same flow across the Results, Methods, and Discussion sections.
- We develop a rhythm as we read and parallel structures facilitate our comprehension. When you organize information the same way in each of these related parts of your journal manuscript, we can quickly see how a certain result was interpreted and quickly verify the particular methods used to produce that result.
- Notice how using parallel structure will eliminate extra narration in the Discussion part since we can anticipate the flow of your ideas based on what we read in the Results segment. Reducing wordiness is important when you only have a few paragraphs to devote to the Discussion section!
- Within each subpart of a Discussion, the information should flow as follows: (A) conclusion first, (B) relevant results and how they relate to that conclusion and (C) relevant literature.
- End with a concise summary explaining the big-picture impact of your study on our understanding of the subject matter. At the beginning of your Discussion section, you stated why this particular study was needed to fill the gap you noticed and why that gap needed filling in the first place. Now, it is time to end with “how your research filled that gap.”
Discussion Part 1: Summarizing Key Findings
Begin the Discussion section by restating your statement of the problem and briefly summarizing the major results. Do not simply repeat your findings. Rather, try to create a concise statement of the main results that directly answer the central research question that you stated in the Introduction section . This content should not be longer than one paragraph in length.
Many researchers struggle with understanding the precise differences between a Discussion section and a Results section . The most important thing to remember here is that your Discussion section should subjectively evaluate the findings presented in the Results section, and in relatively the same order. Keep these sections distinct by making sure that you do not repeat the findings without providing an interpretation.
Phrase examples: Summarizing the results
- The findings indicate that …
- These results suggest a correlation between A and B …
- The data present here suggest that …
- An interpretation of the findings reveals a connection between…
Discussion Part 2: Interpreting the Findings
What do the results mean? It may seem obvious to you, but simply looking at the figures in the Results section will not necessarily convey to readers the importance of the findings in answering your research questions.
The exact structure of interpretations depends on the type of research being conducted. Here are some common approaches to interpreting data:
- Identifying correlations and relationships in the findings
- Explaining whether the results confirm or undermine your research hypothesis
- Giving the findings context within the history of similar research studies
- Discussing unexpected results and analyzing their significance to your study or general research
- Offering alternative explanations and arguing for your position
Organize the Discussion section around key arguments, themes, hypotheses, or research questions or problems. Again, make sure to follow the same order as you did in the Results section.
Discussion Part 3: Discussing the Implications
In addition to providing your own interpretations, show how your results fit into the wider scholarly literature you surveyed in the literature review section. This section is called the implications of the study . Show where and how these results fit into existing knowledge, what additional insights they contribute, and any possible consequences that might arise from this knowledge, both in the specific research topic and in the wider scientific domain.
Questions to ask yourself when dealing with potential implications:
- Do your findings fall in line with existing theories, or do they challenge these theories or findings? What new information do they contribute to the literature, if any? How exactly do these findings impact or conflict with existing theories or models?
- What are the practical implications on actual subjects or demographics?
- What are the methodological implications for similar studies conducted either in the past or future?
Your purpose in giving the implications is to spell out exactly what your study has contributed and why researchers and other readers should be interested.
Phrase examples: Discussing the implications of the research
- These results confirm the existing evidence in X studies…
- The results are not in line with the foregoing theory that…
- This experiment provides new insights into the connection between…
- These findings present a more nuanced understanding of…
- While previous studies have focused on X, these results demonstrate that Y.
Step 4: Acknowledging the limitations
All research has study limitations of one sort or another. Acknowledging limitations in methodology or approach helps strengthen your credibility as a researcher. Study limitations are not simply a list of mistakes made in the study. Rather, limitations help provide a more detailed picture of what can or cannot be concluded from your findings. In essence, they help temper and qualify the study implications you listed previously.
Study limitations can relate to research design, specific methodological or material choices, or unexpected issues that emerged while you conducted the research. Mention only those limitations directly relate to your research questions, and explain what impact these limitations had on how your study was conducted and the validity of any interpretations.
Possible types of study limitations:
- Insufficient sample size for statistical measurements
- Lack of previous research studies on the topic
- Methods/instruments/techniques used to collect the data
- Limited access to data
- Time constraints in properly preparing and executing the study
After discussing the study limitations, you can also stress that your results are still valid. Give some specific reasons why the limitations do not necessarily handicap your study or narrow its scope.
Phrase examples: Limitations sentence beginners
- “There may be some possible limitations in this study.”
- “The findings of this study have to be seen in light of some limitations.”
- “The first limitation is the…The second limitation concerns the…”
- “The empirical results reported herein should be considered in the light of some limitations.”
- “This research, however, is subject to several limitations.”
- “The primary limitation to the generalization of these results is…”
- “Nonetheless, these results must be interpreted with caution and a number of limitations should be borne in mind.”
Discussion Part 5: Giving Recommendations for Further Research
Based on your interpretation and discussion of the findings, your recommendations can include practical changes to the study or specific further research to be conducted to clarify the research questions. Recommendations are often listed in a separate Conclusion section , but often this is just the final paragraph of the Discussion section.
Suggestions for further research often stem directly from the limitations outlined. Rather than simply stating that “further research should be conducted,” provide concrete specifics for how future can help answer questions that your research could not.
Phrase examples: Recommendation sentence beginners
- Further research is needed to establish …
- There is abundant space for further progress in analyzing…
- A further study with more focus on X should be done to investigate…
- Further studies of X that account for these variables must be undertaken.
Consider Receiving Professional Language Editing
As you edit or draft your research manuscript, we hope that you implement these guidelines to produce a more effective Discussion section. And after completing your draft, don’t forget to submit your work to a professional proofreading and English editing service like Wordvice, including our manuscript editing service for paper editing , cover letter editing , SOP editing , and personal statement proofreading services. Language editors not only proofread and correct errors in grammar, punctuation, mechanics, and formatting but also improve terms and revise phrases so they read more naturally. Wordvice is an industry leader in providing high-quality revision for all types of academic documents.
For additional information about how to write a strong research paper, make sure to check out our full research writing series !
Wordvice Writing Resources
- How to Write a Research Paper Introduction
- Which Verb Tenses to Use in a Research Paper
- How to Write an Abstract for a Research Paper
- How to Write a Research Paper Title
- Useful Phrases for Academic Writing
- Common Transition Terms in Academic Papers
- Active and Passive Voice in Research Papers
- 100+ Verbs That Will Make Your Research Writing Amazing
- Tips for Paraphrasing in Research Papers
Additional Academic Resources
- Guide for Authors. (Elsevier)
- How to Write the Results Section of a Research Paper. (Bates College)
- Structure of a Research Paper. (University of Minnesota Biomedical Library)
- How to Choose a Target Journal (Springer)
- How to Write Figures and Tables (UNC Writing Center)

- Research Paper Guides
- Basics of Research Paper Writing
How to Write a Discussion Section: Writing Guide
- Speech Topics
- Basics of Essay Writing
- Essay Topics
- Other Essays
- Main Academic Essays
- Research Paper Topics
- Miscellaneous
- Chicago/ Turabian
- Data & Statistics
- Methodology
- Admission Writing Tips
- Admission Advice
- Other Guides
- Student Life
- Studying Tips
- Understanding Plagiarism
- Academic Writing Tips
- Basics of Dissertation & Thesis Writing

- Essay Guides
- Formatting Guides
- Basics of Research Process
- Admission Guides
- Dissertation & Thesis Guides

Table of contents

Use our free Readability checker
The discussion section of a research paper is where the author analyzes and explains the importance of the study's results. It presents the conclusions drawn from the study, compares them to previous research, and addresses any potential limitations or weaknesses. The discussion section should also suggest areas for future research.
Everything is not that complicated if you know where to find the required information. We’ll tell you everything there is to know about writing your discussion. Our easy guide covers all important bits, including research questions and your research results. Do you know how all enumerated events are connected? Well, you will after reading this guide we’ve prepared for you!
What Is in the Discussion Section of a Research Paper
The discussion section of a research paper can be viewed as something similar to the conclusion of your paper. But not literal, of course. It’s an ultimate section where you can talk about the findings of your study. Think about these questions when writing:
- Did you answer all of the promised research questions?
- Did you mention why your work matters?
- What are your findings, and why should anyone even care?
- Does your study have a literature review?
So, answer your questions, provide proof, and don’t forget about your promises from the introduction.
How to Write a Discussion Section in 5 Steps
How to write the discussion section of a research paper is something everyone googles eventually. It's just life. But why not make everything easier? In brief, this section we’re talking about must include all following parts:
- Answers for research questions
- Literature review
- Results of the work
- Limitations of one’s study
- Overall conclusion
Indeed, all those parts may confuse anyone. So by looking at our guide, you'll save yourself some hassle. P.S. All our steps are easy and explained in detail! But if you are looking for the most efficient solution, consider using professional help. Leave your “ write my research paper for me ” order at StudyCrumb and get a customized study tailored to your requirements.
Step 1. Start Strong: Discussion Section of a Research Paper
First and foremost, how to start the discussion section of a research paper? Here’s what you should definitely consider before settling down to start writing:
- All essays or papers must begin strong. All readers will not wait for any writer to get to the point. We advise summarizing the paper's main findings.
- Moreover, you should relate both discussion and literature review to what you have discovered. Mentioning that would be a plus too.
- Make sure that an introduction or start per se is clear and concise. Word count might be needed for school. But any paper should be understandable and not too diluted.
Step 2. Answer the Questions in Your Discussion Section of a Research Paper
Writing the discussion section of a research paper also involves mentioning your questions. Remember that in your introduction, you have promised your readers to answer certain questions. Well, now it’s a perfect time to finally give the awaited answer. You need to explain all possible correlations between your findings, research questions, and literature proposed. You already had hypotheses. So were they correct, or maybe you want to propose certain corrections? Section’s main goal is to avoid open ends. It’s not a story or a fairytale with an intriguing ending. If you have several questions, you must answer them. As simple as that.
Step 3. Relate Your Results in a Discussion Section
Writing a discussion section of a research paper also requires any writer to explain their results. You will undoubtedly include an impactful literature review. However, your readers should not just try and struggle with understanding what are some specific relationships behind previous studies and your results. Your results should sound something like: “This guy in their paper discovered that apples are green. Nevertheless, I have proven via experimentation and research that apples are actually red.” Please, don’t take these results directly. It’s just an initial hypothesis. But what you should definitely remember is any practical implications of your study. Why does it matter and how can anyone use it? That’s the most crucial question.
Step 4. Describe the Limitations in Your Discussion Section
Discussion section of a research paper isn’t limitless. What does that mean? Essentially, it means that you also have to discuss any limitations of your study. Maybe you had some methodological inconsistencies. Possibly, there are no particular theories or not enough information for you to be entirely confident in one’s conclusions. You might say that an available source of literature you have studied does not focus on one’s issue. That’s why one’s main limitation is theoretical. However, keep in mind that your limitations must possess a certain degree of relevancy. You can just say that you haven’t found enough books. Your information must be truthful to research.
Step 5. Conclude Your Discussion Section With Recommendations
Your last step when you write a discussion section in a paper is its conclusion, like in any other academic work. Writer’s conclusion must be as strong as their starting point of the overall work. Check out our brief list of things to know about the conclusion in research paper :
- It must present its scientific relevance and importance of your work.
- It should include different implications of your research.
- It should not, however, discuss anything new or things that you have not mentioned before.
- Leave no open questions and carefully complete the work without them.
Discussion Section of a Research Paper Example
All the best example discussion sections of a research paper will be written according to our brief guide. Don’t forget that you need to state your findings and underline the importance of your work. An undoubtedly big part of one’s discussion will definitely be answering and explaining the research questions. In other words, you’ll already have all the knowledge you have so carefully gathered. Our last step for you is to recollect and wrap up your paper. But we’re sure you’ll succeed!

How to Write a Discussion Section: Final Thoughts
Today we have covered how to write a discussion section. That was quite a brief journey, wasn’t it? Just to remind you to focus on these things:
- Importance of your study.
- Summary of the information you have gathered.
- Main findings and conclusions.
- Answers to all research questions without an open end.
- Correlation between literature review and your results.
But, wait, this guide is not the only thing we can do. Looking for how to write an abstract for a research paper for example? We have such a blog and much more on our platform.

Our academic writing service is just a click away. We are proud to say that our writers are professionals in their fields. Buy a research paper and our experts can provide prompt solutions without compromising the quality.
Discussion Section of a Research Paper: Frequently Asked Questions
1. how long should the discussion section of a research paper be.
Our discussion section of a research paper should not be longer than other sections. So try to keep it short but as informative as possible. It usually contains around 6-7 paragraphs in length. It is enough to briefly summarize all the important data and not to drag it.
2. What's the difference between the discussion and the results?
The difference between discussion and results is very simple and easy to understand. The results only report your main findings. You stated what you have found and how you have done that. In contrast, one’s discussion mentions your findings and explains how they relate to other literature, research questions, and one’s hypothesis. Therefore, it is not only a report but an efficient as well as proper explanation.
3. What's the difference between a discussion and a conclusion?
The difference between discussion and conclusion is also quite easy. Conclusion is a brief summary of all the findings and results. Still, our favorite discussion section interprets and explains your main results. It is an important but more lengthy and wordy part. Besides, it uses extra literature for references.
4. What is the purpose of the discussion section?
The primary purpose of a discussion section is to interpret and describe all your interesting findings. Therefore, you should state what you have learned, whether your hypothesis was correct and how your results can be explained using other sources. If this section is clear to readers, our congratulations as you have succeeded.

Joe Eckel is an expert on Dissertations writing. He makes sure that each student gets precious insights on composing A-grade academic writing.
You may also like


How To Write The Discussion Chapter
A Simple Explainer With Examples + Free Template
By: Jenna Crossley (PhD) | Reviewed By: Dr. Eunice Rautenbach | August 2021
If you’re reading this, chances are you’ve reached the discussion chapter of your thesis or dissertation and are looking for a bit of guidance. Well, you’ve come to the right place ! In this post, we’ll unpack and demystify the typical discussion chapter in straightforward, easy to understand language, with loads of examples .
Overview: The Discussion Chapter
- What the discussion chapter is
- What to include in your discussion
- How to write up your discussion
- A few tips and tricks to help you along the way
- Free discussion template
What (exactly) is the discussion chapter?
The discussion chapter is where you interpret and explain your results within your thesis or dissertation. This contrasts with the results chapter, where you merely present and describe the analysis findings (whether qualitative or quantitative ). In the discussion chapter, you elaborate on and evaluate your research findings, and discuss the significance and implications of your results .
In this chapter, you’ll situate your research findings in terms of your research questions or hypotheses and tie them back to previous studies and literature (which you would have covered in your literature review chapter). You’ll also have a look at how relevant and/or significant your findings are to your field of research, and you’ll argue for the conclusions that you draw from your analysis. Simply put, the discussion chapter is there for you to interact with and explain your research findings in a thorough and coherent manner.
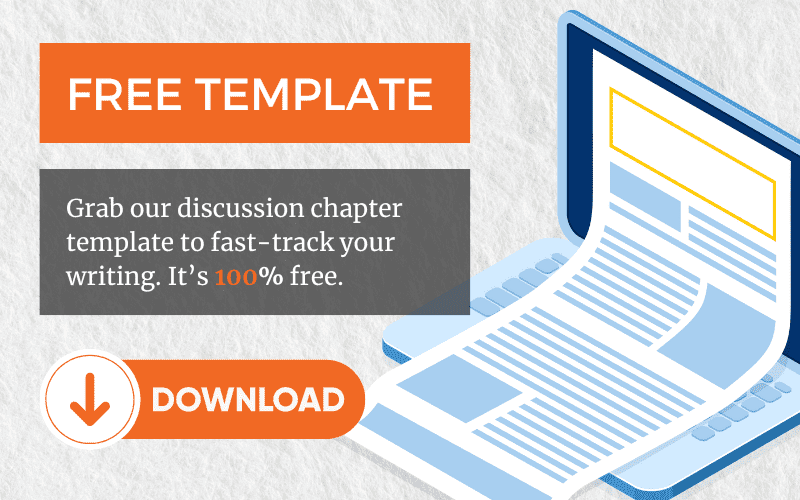
What should I include in the discussion chapter?
First things first: in some studies, the results and discussion chapter are combined into one chapter . This depends on the type of study you conducted (i.e., the nature of the study and methodology adopted), as well as the standards set by the university. So, check in with your university regarding their norms and expectations before getting started. In this post, we’ll treat the two chapters as separate, as this is most common.
Basically, your discussion chapter should analyse , explore the meaning and identify the importance of the data you presented in your results chapter. In the discussion chapter, you’ll give your results some form of meaning by evaluating and interpreting them. This will help answer your research questions, achieve your research aims and support your overall conclusion (s). Therefore, you discussion chapter should focus on findings that are directly connected to your research aims and questions. Don’t waste precious time and word count on findings that are not central to the purpose of your research project.
As this chapter is a reflection of your results chapter, it’s vital that you don’t report any new findings . In other words, you can’t present claims here if you didn’t present the relevant data in the results chapter first. So, make sure that for every discussion point you raise in this chapter, you’ve covered the respective data analysis in the results chapter. If you haven’t, you’ll need to go back and adjust your results chapter accordingly.
If you’re struggling to get started, try writing down a bullet point list everything you found in your results chapter. From this, you can make a list of everything you need to cover in your discussion chapter. Also, make sure you revisit your research questions or hypotheses and incorporate the relevant discussion to address these. This will also help you to see how you can structure your chapter logically.
Need a helping hand?
How to write the discussion chapter
Now that you’ve got a clear idea of what the discussion chapter is and what it needs to include, let’s look at how you can go about structuring this critically important chapter. Broadly speaking, there are six core components that need to be included, and these can be treated as steps in the chapter writing process.
Step 1: Restate your research problem and research questions
The first step in writing up your discussion chapter is to remind your reader of your research problem , as well as your research aim(s) and research questions . If you have hypotheses, you can also briefly mention these. This “reminder” is very important because, after reading dozens of pages, the reader may have forgotten the original point of your research or been swayed in another direction. It’s also likely that some readers skip straight to your discussion chapter from the introduction chapter , so make sure that your research aims and research questions are clear.
Step 2: Summarise your key findings
Next, you’ll want to summarise your key findings from your results chapter. This may look different for qualitative and quantitative research , where qualitative research may report on themes and relationships, whereas quantitative research may touch on correlations and causal relationships. Regardless of the methodology, in this section you need to highlight the overall key findings in relation to your research questions.
Typically, this section only requires one or two paragraphs , depending on how many research questions you have. Aim to be concise here, as you will unpack these findings in more detail later in the chapter. For now, a few lines that directly address your research questions are all that you need.
Some examples of the kind of language you’d use here include:
- The data suggest that…
- The data support/oppose the theory that…
- The analysis identifies…
These are purely examples. What you present here will be completely dependent on your original research questions, so make sure that you are led by them .
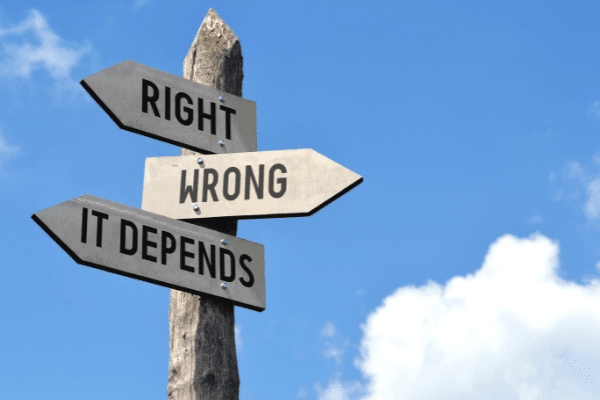
Step 3: Interpret your results
Once you’ve restated your research problem and research question(s) and briefly presented your key findings, you can unpack your findings by interpreting your results. Remember: only include what you reported in your results section – don’t introduce new information.
From a structural perspective, it can be a wise approach to follow a similar structure in this chapter as you did in your results chapter. This would help improve readability and make it easier for your reader to follow your arguments. For example, if you structured you results discussion by qualitative themes, it may make sense to do the same here.
Alternatively, you may structure this chapter by research questions, or based on an overarching theoretical framework that your study revolved around. Every study is different, so you’ll need to assess what structure works best for you.
When interpreting your results, you’ll want to assess how your findings compare to those of the existing research (from your literature review chapter). Even if your findings contrast with the existing research, you need to include these in your discussion. In fact, those contrasts are often the most interesting findings . In this case, you’d want to think about why you didn’t find what you were expecting in your data and what the significance of this contrast is.
Here are a few questions to help guide your discussion:
- How do your results relate with those of previous studies ?
- If you get results that differ from those of previous studies, why may this be the case?
- What do your results contribute to your field of research?
- What other explanations could there be for your findings?
When interpreting your findings, be careful not to draw conclusions that aren’t substantiated . Every claim you make needs to be backed up with evidence or findings from the data (and that data needs to be presented in the previous chapter – results). This can look different for different studies; qualitative data may require quotes as evidence, whereas quantitative data would use statistical methods and tests. Whatever the case, every claim you make needs to be strongly backed up.
Step 4: Acknowledge the limitations of your study
The fourth step in writing up your discussion chapter is to acknowledge the limitations of the study. These limitations can cover any part of your study , from the scope or theoretical basis to the analysis method(s) or sample. For example, you may find that you collected data from a very small sample with unique characteristics, which would mean that you are unable to generalise your results to the broader population.
For some students, discussing the limitations of their work can feel a little bit self-defeating . This is a misconception, as a core indicator of high-quality research is its ability to accurately identify its weaknesses. In other words, accurately stating the limitations of your work is a strength, not a weakness . All that said, be careful not to undermine your own research. Tell the reader what limitations exist and what improvements could be made, but also remind them of the value of your study despite its limitations.
Step 5: Make recommendations for implementation and future research
Now that you’ve unpacked your findings and acknowledge the limitations thereof, the next thing you’ll need to do is reflect on your study in terms of two factors:
- The practical application of your findings
- Suggestions for future research
The first thing to discuss is how your findings can be used in the real world – in other words, what contribution can they make to the field or industry? Where are these contributions applicable, how and why? For example, if your research is on communication in health settings, in what ways can your findings be applied to the context of a hospital or medical clinic? Make sure that you spell this out for your reader in practical terms, but also be realistic and make sure that any applications are feasible.
The next discussion point is the opportunity for future research . In other words, how can other studies build on what you’ve found and also improve the findings by overcoming some of the limitations in your study (which you discussed a little earlier). In doing this, you’ll want to investigate whether your results fit in with findings of previous research, and if not, why this may be the case. For example, are there any factors that you didn’t consider in your study? What future research can be done to remedy this? When you write up your suggestions, make sure that you don’t just say that more research is needed on the topic, also comment on how the research can build on your study.
Step 6: Provide a concluding summary
Finally, you’ve reached your final stretch. In this section, you’ll want to provide a brief recap of the key findings – in other words, the findings that directly address your research questions . Basically, your conclusion should tell the reader what your study has found, and what they need to take away from reading your report.
When writing up your concluding summary, bear in mind that some readers may skip straight to this section from the beginning of the chapter. So, make sure that this section flows well from and has a strong connection to the opening section of the chapter.
Tips and tricks for an A-grade discussion chapter
Now that you know what the discussion chapter is , what to include and exclude , and how to structure it , here are some tips and suggestions to help you craft a quality discussion chapter.
- When you write up your discussion chapter, make sure that you keep it consistent with your introduction chapter , as some readers will skip from the introduction chapter directly to the discussion chapter. Your discussion should use the same tense as your introduction, and it should also make use of the same key terms.
- Don’t make assumptions about your readers. As a writer, you have hands-on experience with the data and so it can be easy to present it in an over-simplified manner. Make sure that you spell out your findings and interpretations for the intelligent layman.
- Have a look at other theses and dissertations from your institution, especially the discussion sections. This will help you to understand the standards and conventions of your university, and you’ll also get a good idea of how others have structured their discussion chapters. You can also check out our chapter template .
- Avoid using absolute terms such as “These results prove that…”, rather make use of terms such as “suggest” or “indicate”, where you could say, “These results suggest that…” or “These results indicate…”. It is highly unlikely that a dissertation or thesis will scientifically prove something (due to a variety of resource constraints), so be humble in your language.
- Use well-structured and consistently formatted headings to ensure that your reader can easily navigate between sections, and so that your chapter flows logically and coherently.
If you have any questions or thoughts regarding this post, feel free to leave a comment below. Also, if you’re looking for one-on-one help with your discussion chapter (or thesis in general), consider booking a free consultation with one of our highly experienced Grad Coaches to discuss how we can help you.
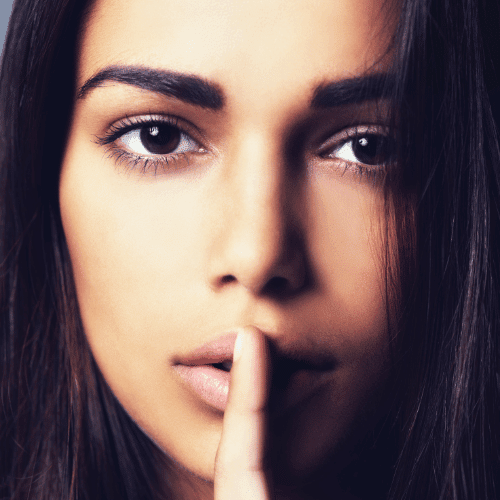
Psst... there’s more!
This post was based on one of our popular Research Bootcamps . If you're working on a research project, you'll definitely want to check this out ...
You Might Also Like:
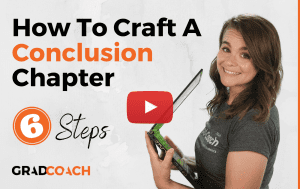
36 Comments
Thank you this is helpful!
This is very helpful to me… Thanks a lot for sharing this with us 😊
This has been very helpful indeed. Thank you.
This is actually really helpful, I just stumbled upon it. Very happy that I found it, thank you.
Me too! I was kinda lost on how to approach my discussion chapter. How helpful! Thanks a lot!
This is really good and explicit. Thanks
Thank you, this blog has been such a help.
Thank you. This is very helpful.
Dear sir/madame
Thanks a lot for this helpful blog. Really, it supported me in writing my discussion chapter while I was totally unaware about its structure and method of writing.
With regards
Syed Firoz Ahmad PhD, Research Scholar
I agree so much. This blog was god sent. It assisted me so much while I was totally clueless about the context and the know-how. Now I am fully aware of what I am to do and how I am to do it.
Thanks! This is helpful!
thanks alot for this informative website
Dear Sir/Madam,
Truly, your article was much benefited when i structured my discussion chapter.
Thank you very much!!!
This is helpful for me in writing my research discussion component. I have to copy this text on Microsoft word cause of my weakness that I cannot be able to read the text on screen a long time. So many thanks for this articles.
This was helpful
Thanks Jenna, well explained.
Thank you! This is super helpful.
Thanks very much. I have appreciated the six steps on writing the Discussion chapter which are (i) Restating the research problem and questions (ii) Summarising the key findings (iii) Interpreting the results linked to relating to previous results in positive and negative ways; explaining whay different or same and contribution to field of research and expalnation of findings (iv) Acknowledgeing limitations (v) Recommendations for implementation and future resaerch and finally (vi) Providing a conscluding summary
My two questions are: 1. On step 1 and 2 can it be the overall or you restate and sumamrise on each findings based on the reaerch question? 2. On 4 and 5 do you do the acknowlledgement , recommendations on each research finding or overall. This is not clear from your expalanattion.
Please respond.
This post is very useful. I’m wondering whether practical implications must be introduced in the Discussion section or in the Conclusion section?
Sigh, I never knew a 20 min video could have literally save my life like this. I found this at the right time!!!! Everything I need to know in one video thanks a mil ! OMGG and that 6 step!!!!!! was the cherry on top the cake!!!!!!!!!
Thanks alot.., I have gained much
This piece is very helpful on how to go about my discussion section. I can always recommend GradCoach research guides for colleagues.
Many thanks for this resource. It has been very helpful to me. I was finding it hard to even write the first sentence. Much appreciated.
Thanks so much. Very helpful to know what is included in the discussion section
this was a very helpful and useful information
This is very helpful. Very very helpful. Thanks for sharing this online!
it is very helpfull article, and i will recommend it to my fellow students. Thank you.
Superlative! More grease to your elbows.
Powerful, thank you for sharing.
Wow! Just wow! God bless the day I stumbled upon you guys’ YouTube videos! It’s been truly life changing and anxiety about my report that is due in less than a month has subsided significantly!
Simplified explanation. Well done.
The presentation is enlightening. Thank you very much.
Thanks for the support and guidance
This has been a great help to me and thank you do much
I second that “it is highly unlikely that a dissertation or thesis will scientifically prove something”; although, could you enlighten us on that comment and elaborate more please?
Sure, no problem.
Scientific proof is generally considered a very strong assertion that something is definitively and universally true. In most scientific disciplines, especially within the realms of natural and social sciences, absolute proof is very rare. Instead, researchers aim to provide evidence that supports or rejects hypotheses. This evidence increases or decreases the likelihood that a particular theory is correct, but it rarely proves something in the absolute sense.
Dissertations and theses, as substantial as they are, typically focus on exploring a specific question or problem within a larger field of study. They contribute to a broader conversation and body of knowledge. The aim is often to provide detailed insight, extend understanding, and suggest directions for further research rather than to offer definitive proof. These academic works are part of a cumulative process of knowledge building where each piece of research connects with others to gradually enhance our understanding of complex phenomena.
Furthermore, the rigorous nature of scientific inquiry involves continuous testing, validation, and potential refutation of ideas. What might be considered a “proof” at one point can later be challenged by new evidence or alternative interpretations. Therefore, the language of “proof” is cautiously used in academic circles to maintain scientific integrity and humility.
Submit a Comment Cancel reply
Your email address will not be published. Required fields are marked *
Save my name, email, and website in this browser for the next time I comment.
- Print Friendly
Training videos | Faqs

Discussion Section Examples and Writing Tips
Abstract | Introduction | Literature Review | Research question | Materials & Methods | Results | Discussion | Conclusion
In this blog, we look at how to write the discussion section of a research paper. We will go through plenty of discussion examples and understand how to construct a great discussion section for your research paper.
1. What is the purpose of the discussion section?

The discussion section is one of the most important sections of your research paper. This is where you interpret your results, highlight your contributions, and explain the value of your work to your readers. This is one of the challenging parts to write because the author must clearly explain the significance of their results and tie everything back to the research questions.
2. How should I structure my discussion section?
Generally, the discussion section of a research paper typically contains the following parts.
Research summary It is a good idea to start this section with an overall summary of your work and highlight the main findings of your research.
Interpretation of findings You must interpret your findings clearly to your readers one by one.
Comparison with literature You must talk about how your results fit into existing research in the literature.
Implications of your work You should talk about the implications and possible benefits of your research.
Limitations You should talk about the possible limitations and shortcomings of your research
Future work And finally, you can talk about the possible future directions of your work.
3. Discussion Examples
Let’s look at some examples of the discussion section. We will be looking at discussion examples from different fields and of different formats. We have split this section into multiple components so that it is easy for you to digest and understand.
3.1. An example of research summary in discussion
It is a good idea to start your discussion section with the summary of your work. The best way to do this will be to restate your research question, and then reminding your readers about your methods, and finally providing an overall summary of your results.
Our aims were to compare the effectiveness and user-friendliness of different storm detection software for storm tracking. On the basis of these aims, we ran multiple experiments with the same conditions using different storm detection software. Our results showed that in both speed and accuracy of data, ‘software A’ performed better than ‘software B’. _ Aims summary _ Methodology summary _ Results summary
This discussion example is from an engineering research paper. The authors are restating their aims first, which is to compare different types of storm-tracking software. Then, they are providing a brief summary of the methods. Here, they are testing different storm-tracking software under different conditions to see which performs the best. Then, they are finally providing their main finding which is that they found ‘software A’ better than ‘software B’. This is a very good example of how to start the discussion section by presenting a summary of your work.
3.2. An example of result interpretation in discussion
The next step is to interpret your results. You have to explain your results clearly to your readers. Here is a discussion example that shows how to interpret your results.
The results of this study indicate significant differences between classical music and pop music in terms of their effects on memory recall and cognition. This implies that as the complexity of the music increases, so does its ability to facilitate cognitive processing. This finding aligns with the well-known “Mozart effect,” which suggests that listening to classical music can enhance cognitive function. _ Result _ Interpretation _ Additional evidence
The authors are saying that their results show that there is a significant difference between pop music and classical music in terms of memory recall and cognition. Now they are providing their interpretation of the findings. They think it is because there is a link between the complexity of music and cognitive processing. They are also making a reference to a well-known theory called the ‘Mozart effect’ to back up their findings. It is a nicely written passage and the author’s interpretation sounds very convincing and credible.
3.3. An example of literature comparison in discussion
The next step is to compare your results to the literature. You have to explain clearly how your findings compare with similar findings made by other researchers. Here is a discussion example where authors are providing details of papers in the literature that both support and oppose their findings.
Our analysis predicts that climate change will have a significant impact on wheat yield. This finding undermines one of the central pieces of evidence in some previous simulation studies [1-3] that suggest a negative effect of climate change on wheat yield, but the result is entirely consistent with the predictions of other research [4-5] that suggests the overall change in climate could result in increases in wheat yield. _ Result _ Comparison with literature
The authors are saying that their results show that climate change will have a significant effect on wheat production. Then, they are saying that there are some papers in the literature that are in agreement with their findings. However, there are also many papers in the literature that disagree with their findings. This is very important. Your discussion should be two-sided, not one-sided. You should not ignore the literature that doesn’t corroborate your findings.
3.4. An example of research implications in discussion
The next step is to explain to your readers how your findings will benefit society and the research community. You have to clearly explain the value of your work to your readers. Here is a discussion example where authors explain the implications of their research.
The results contribute insights with regard to the management of wildfire events using artificial intelligence. One could easily argue that the obvious practical implication of this study is that it proposes utilizing cloud-based machine vision to detect wildfires in real-time, even before the first responders receive emergency calls. _ Your finding _ Implications of your finding
In this paper, the authors are saying that their findings indicate that Artificial intelligence can be used to effectively manage wildfire events. Then, they are talking about the practical implications of their study. They are saying that their work has proven that machine learning can be used to detect wildfires in real-time. This is a great practical application and can save thousands of lives. As you can see, after reading this passage, you can immediately understand the value and significance of the work.
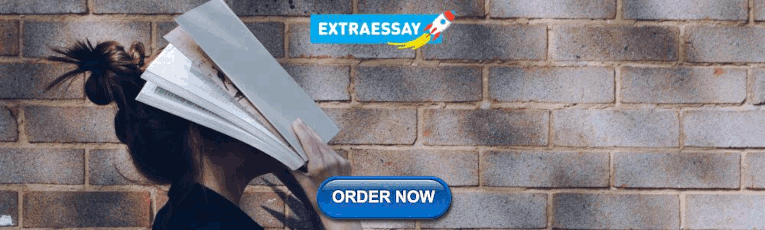
3.5. An example of limitations in discussion
It is very important that you discuss the limitations of your study. Limitations are flaws and shortcomings of your study. You have to tell your readers how your limitations might influence the outcomes and conclusions of your research. Most studies will have some form of limitation. So be honest and don’t hide your limitations. In reality, your readers and reviewers will be impressed with your paper if you are upfront about your limitations.
Study design and small sample size are important limitations. This could have led to an overestimation of the effect. Future research should reconfirm these findings by conducting larger-scale studies. _ Limitation _ How it might affect the results? _ How to fix the limitation?
Here is a discussion example where the author talks about study limitations. The authors are saying that the main limitations of the study are the small sample size and weak study design. Then they explain how this might have affected their results. They are saying that it is possible that they are overestimating the actual effect they are measuring. Then finally they are telling the readers that more studies with larger sample sizes should be conducted to reconfirm the findings.
As you can see, the authors are clearly explaining three things here:
3.6. An example of future work in discussion
It is important to remember not to end your paper with limitations. Finish your paper on a positive note by telling your readers about the benefits of your research and possible future directions. Here is a discussion example where the author talks about future work.
Our study highlights useful insights about the potential of biomass as a renewable energy source. Future research can extend this research in several ways, including research on how to tackle challenges that hinder the sustainability of renewable energy sources towards climate change mitigation, such as market failures, lack of information and access to raw materials. _ Benefits of your work _ Future work
The authors are starting the final paragraph of the discussion section by highlighting the benefit of their work which is the use of biomass as a renewable source of energy. Then they talk about future research. They are saying that future research can focus on how to improve the sustainability of biomass production. This is a very good example of how to finish the discussion section of your paper on a positive note.
4. Frequently Asked Questions
Sometimes you will have negative or unexpected results in your paper. You have to talk about it in your discussion section. A lot of students find it difficult to write this part. The best way to handle this situation is not to look at results as either positive or negative. A result is a result, and you will always have something important and interesting to say about your findings. Just spend some time investigating what might have caused this result and tell your readers about it.
You must talk about the limitations of your work in the discussion section of the paper. One of the important qualities that the scientific community expects from a researcher is honesty and admitting when they have made a mistake. The important trick you have to learn while presenting your limitations is to present them in a constructive way rather than being too negative about them. You must try to use positive language even when you are talking about major limitations of your work.
If you have something exciting to say about your results or found something new that nobody else has found before, then, don’t be modest and use flat language when presenting this in the discussion. Use words like ‘break through’, ‘indisputable evidence’, ‘exciting proposition’ to increase the impact of your findings.
Important thing to remember is not to overstate your findings. If you found something really interesting but are not 100% sure, you must not mislead your readers. The best way to do this will be to use words like ‘it appears’ and ‘it seems’. This will tell the readers that there is a slight possibility that you might be wrong.
Similar Posts
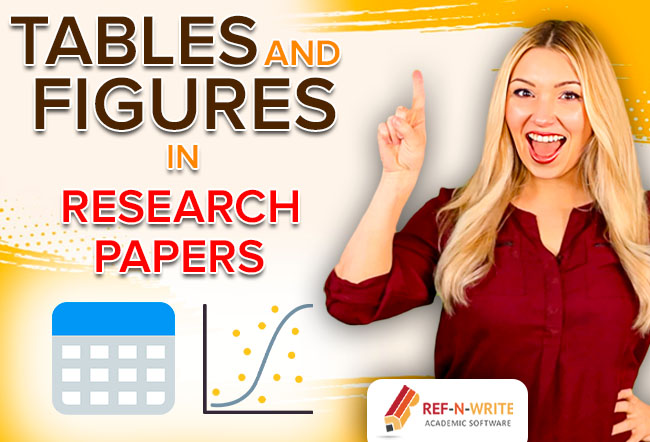
Figures and Tables in Research Papers – Tips and Examples
In this blog, we will look at best practices for presenting tables and figures in your research paper.
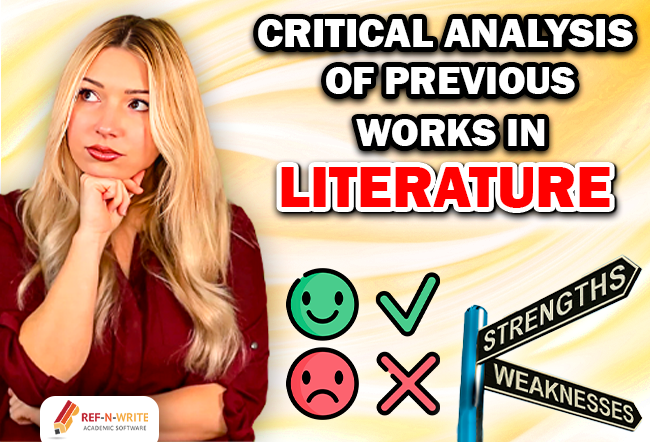
Critical Literature Review : How to Critique a Research Article?
In this blog, we will look at how to use constructive language when critiquing other’s work in your research paper.
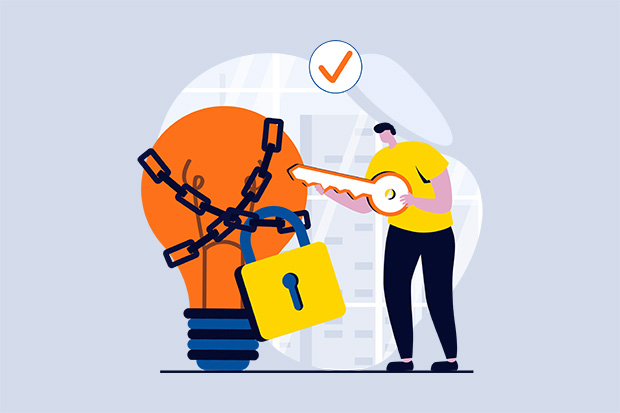
Introduction Paragraph Examples and Writing Tips
In this blog, we will go through a few introduction paragraph examples and understand how to construct a great introduction paragraph for your research paper.
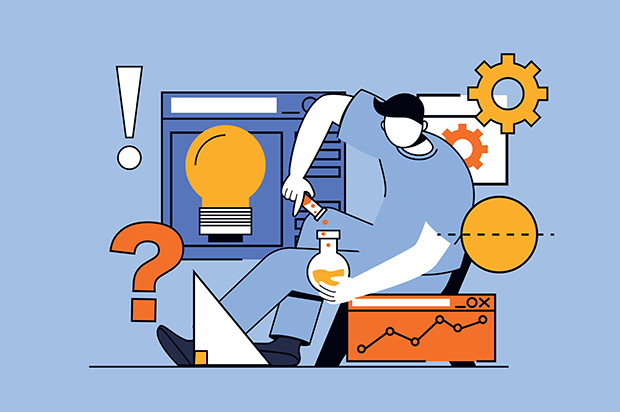
Materials and Methods Examples and Writing Tips
In this blog, we will go through many materials and methods examples and understand how to write a clear and concise method section for your research paper.
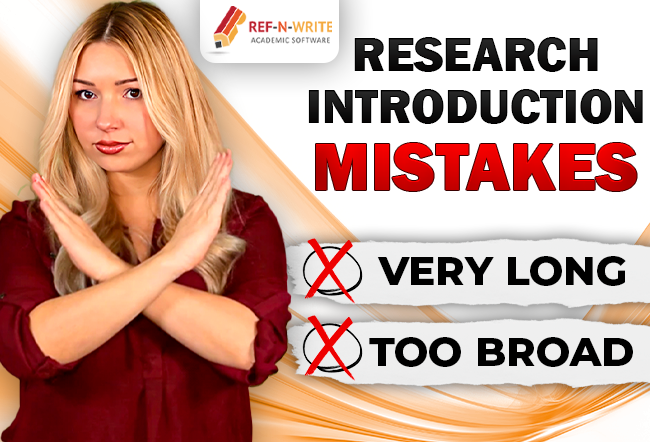
3 Costly Mistakes to Avoid in the Research Introduction
In this blog, we will discuss three common mistakes that beginner writers make while writing the research paper introduction.

Technical Terms, Notations, and Scientific Jargon in Research Papers
In this blog, we will teach you how to use specialized terminology in your research papers with some practical examples.
Leave a Reply Cancel reply
Your email address will not be published. Required fields are marked *
Save my name, email, and website in this browser for the next time I comment.
- 5 Share Facebook
- 7 Share Twitter
- 5 Share LinkedIn
- 10 Share Email

An official website of the United States government
The .gov means it’s official. Federal government websites often end in .gov or .mil. Before sharing sensitive information, make sure you’re on a federal government site.
The site is secure. The https:// ensures that you are connecting to the official website and that any information you provide is encrypted and transmitted securely.
- Publications
- Account settings
Preview improvements coming to the PMC website in October 2024. Learn More or Try it out now .
- Advanced Search
- Journal List
- BMC Med Res Methodol

Writing a discussion section: how to integrate substantive and statistical expertise
Michael höfler.
1 Institute of Clinical Psychology and Psychotherapy, Technische Universität Dresden, Dresden, Germany
5 Chair of Clinical Psychology and Behavioural Neuroscience, Institute of Clinical Psychology and Psychotherapy, Technische Universität Dresden, Dresden, Germany
2 Behavioral Epidemiology, Institute of Clinical Psychology and Psychotherapy, Technische Universität Dresden, Dresden, Germany
Sebastian Trautmann
Robert miller.
3 Faculty of Psychology, Technische Universität Dresden, Dresden, Germany
4 Department of Medical Epidemiology and Biostatistics, Karolinska Institute, Stockholm, Sweden
Associated Data
Not applicable.
When discussing results medical research articles often tear substantive and statistical (methodical) contributions apart, just as if both were independent. Consequently, reasoning on bias tends to be vague, unclear and superficial. This can lead to over-generalized, too narrow and misleading conclusions, especially for causal research questions.
To get the best possible conclusion, substantive and statistical expertise have to be integrated on the basis of reasonable assumptions. While statistics should raise questions on the mechanisms that have presumably created the data, substantive knowledge should answer them. Building on the related principle of Bayesian thinking, we make seven specific and four general proposals on writing a discussion section.
Misinterpretation could be reduced if authors explicitly discussed what can be concluded under which assumptions. Informed on the resulting conditional conclusions other researchers may, according to their knowledge and beliefs, follow a particular conclusion or, based on other conditions, arrive at another one. This could foster both an improved debate and a better understanding of the mechanisms behind the data and should therefore enable researchers to better address bias in future studies.
After a research article has presented the substantive background, the methods and the results, the discussion section assesses the validity of results and draws conclusions by interpreting them. The discussion puts the results into a broader context and reflects their implications for theoretical (e.g. etiological) and practical (e.g. interventional) purposes. As such, the discussion contains an article’s last words the reader is left with.
Common recommendations for the discussion section include general proposals for writing [ 1 ] and structuring (e.g. with a paragraph on a study’s strengths and weaknesses) [ 2 ], to avoid common statistical pitfalls (like misinterpreting non-significant findings as true null results) [ 3 ] and to “go beyond the data” when interpreting results [ 4 ]. Note that the latter includes much more than comparing an article’s results with the literature. If results and literature are consistent, this might be due to shared bias only. If they are not consistent, the question arises why inconsistency occurs – maybe because of bias acting differently across studies [ 5 – 7 ]. Recommendations like the CONSORT checklist do well in demanding all quantitative information on design, participation, compliance etc. to be reported in the methods and results section and “addressing sources of potential bias”, “limitations” and “considering other relevant evidence” in the discussion [ 8 , 9 ]. Similarly, the STROBE checklist for epidemiological research demands “a cautious overall interpretation of results” and "discussing the generalizability (external validity)" [ 10 , 11 ]. However, these guidelines do not clarify how to deal with the complex bias issue, and how to get to and report conclusions.
Consequently, suggestions on writing a discussion often remain vague by hardly addressing the role of the assumptions that have (often implicitly) been made when designing a study, analyzing the data and interpreting the results. Such assumptions involve mechanisms that have created the data and are related to sampling, measurement and treatment assignment (in observational studies common causes of factor and outcome) and, as a consequence, the bias this may produce [ 5 , 6 ]. They determine whether a result allows only an associational or a causal conclusion. Causal conclusions, if true, are of much higher relevance for etiology, prevention and intervention. However, they require much stronger assumptions. These have to be fully explicit and, therewith, essential part of the debate since they always involve subjectivity. Subjectivity is unavoidable because the mechanisms behind the data can never be fully estimated from the data themselves [ 12 ].
In this article, we argue that the conjunction of substantive and statistical (methodical) knowledge in the verbal integration of results and beliefs on mechanisms can be greatly improved in (medical) research papers. We illustrate this through the personal roles that a statistician (i.e. methods expert) and a substantive researcher should take. Doing so, we neither claim that usually just two people write a discussion, nor that one person lacks the knowledge of the other, nor that there were truly no researchers that have both kinds of expertise. As a metaphor, the division of these two roles into two persons describes the necessary integration of knowledge via the mode of a dialogue. Verbally, it addresses the finding of increased specialization of different study contributors in biomedical research. This has teared apart the two processes of statistical compilation of results and their verbal integration [ 13 ]. When this happens a statistician alone is limited to a study’s conditions (sampled population, experimental settings etc.), because he or she is unaware of the conditions’ generalizability. On the other hand, a A substantive expert alone is prone to over-generalize because he or she is not aware of the (mathematical) prerequisites for an interpretation.
The article addresses both (medical) researchers educated in basic statistics and research methods and statisticians who cooperate with them. Throughout the paper we exemplify our arguments with the finding of an association in a cross-tabulation between a binary X (factor) and a binary Y (outcome): those who are exposed to or treated with X have a statistically significantly elevated risk for Y as compared to the non-exposed or not (or otherwise) treated (for instance via the chi-squared independence test or logistic regression). Findings like this are frequent and raise the question which more profound conclusion is valid under what assumptions. Until some decades ago, statistics has largely avoided the related topic of causality and instead limited itself on describing observed distributions (here a two-by-two table between D = depression and LC = lung cancer) with well-fitting models.
We illustrate our arguments with the concrete example of the association found between the factor depression (D) and the outcome lung cancer (LC) [ 14 ]. Yet very different mechanisms could have produced such an association [ 7 ], and assumptions on these lead to the following fundamentally different conclusions (Fig. (Fig.1 1 ):
- D causes LC (e.g. because smoking might constitute “self-medication” of depression symptoms)
- LC causes D (e.g. because LC patients are demoralized by their diagnosis)
- D and LC cause each other (e.g. because the arguments in both a. and b. apply)
- D and LC are the causal consequence of the same factor(s) (e.g. poor health behaviors - HB)
- D and LC only share measurement error (e.g. because a fraction of individuals that has either depression or lung cancer denies both in self-report measures).

Different conclusions about an association between D and LC. a D causes LC, b LC causes B, c D and LC cause each other, d D and LC are associated because of a shared factor (HB), e D and LC are associated because they have correlated errors
Note that we use the example purely for illustrative purposes. We do not make substantive claims on what of a. through e. is true but show how one should reflect on mechanisms in order to find the right answer. Besides, we do not consider research on the D-LC relation apart from the finding of association [ 14 ].
Assessing which of a. through e. truly applies requires substantive assumptions on mechanisms: the temporal order of D and LC (a causal effect requires that the cause occurs before the effect), shared factors, selection processes and measurement error. Questions on related mechanisms have to be brought up by statistical consideration, while substantive reasoning has to address them. Together this yields provisional assumptions for inferring that are subject to readers’ substantive consideration and refinement. In general, the integration of prior beliefs (anything beyond the data a conclusion depends on) and the results from the data themselves is formalized by Bayesian statistics [ 15 , 16 ]. This is beyond the scope of this article, still we argue that Bayesian thinking should govern the process of drawing conclusions.
Building on this idea, we provide seven specific and four general recommendations for the cooperative process of writing a discussion. The recommendations are intended to be suggestions rather than rules. They should be subject to further refinement and adjustment to specific requirements in different fields of medical and other research. Note that the order of the points is not meant to structure a discussion’s writing (besides 1.).
Recommendations for writing a discussion section
Specific recommendations.
Consider the example on the association between D and LC. Rather than starting with an in-depth (causal) interpretation a finding should firstly be taken as what it allows inferring without doubt: Under the usual assumptions that a statistical model makes (e.g. random sampling, independence or certain correlation structure between observations [ 17 ]), the association indicates that D (strictly speaking: measuring D) predicts an elevated LC risk (strictly speaking: measuring LC) in the population that one has managed to sample (source population). Assume that the sample has been randomly drawn from primary care settings. In this case the association is useful to recommend medical doctors to better look at an individual’s LC risk in case of D. If the association has been adjusted for age and gender (conveniently through a regression model), the conclusion modifies to: If the doctor knows a patient’s age and gender (what should always be the case) D has additional value in predicting an elevated LC risk.
In the above example, a substantive researcher might want to conclude that D and LC are associated in a general population instead of just inferring to patients in primary care settings (a.). Another researcher might even take the finding as evidence for D being a causal factor in the etiology of LC, meaning that prevention of D could reduce the incidence rate of LC (in whatever target population) (b.). In both cases, the substantive researcher should insist on assessing the desired interpretation that goes beyond the data [ 4 ], but the statistician immediately needs to bring up the next point.
The explanation of all the assumptions that lead from a data result to a conclusion enables a reader to assess whether he or she agrees with the authors’ inference or not. These conditions, however, often remain incomplete or unclear, in which case the reader can hardly assess whether he or she follows a path of argumentation and, thus, shares the conclusion this path leads to.
Consider conclusion a. and suppose that, instead of representative sampling in a general population (e.g. all U.S. citizens aged 18 or above), the investigators were only able to sample in primary care settings. Extrapolating the results to another population than the source population requires what is called “external validity”, “transportability” or the absence of “selection bias” [ 18 , 19 ]. No such bias occurs if the parameter of interest is equal in the source and the target population. Note that this is a weaker condition than the common belief that the sample must represent the target population in everything . If the parameter of interest is the difference in risk for LC between cases and non-cases of D, the condition translates into: the risk difference must be equal in target and source population.
For the causal conclusion b., however, sufficient assumptions are very strict. In an RCT, the conclusion is valid under random sampling from the target population, random allocation of X, perfect compliance in X, complete participation and no measurement error in outcome (for details see [ 20 ]). In practice, on the other hand, the derivations from such conditions might sometimes be modest what may produce little bias only. For instance, non-compliance in a specific drug intake (treatment) might occur only in a few individuals to little extent through a random process (e.g. sickness of a nurse being responsible for drug dispense) and yield just small (downward) bias [ 5 ]. The conclusion of downward bias might also be justified if non-compliance does not cause anything that has a larger effect on a Y than the drug itself. Another researcher, however, could believe that non-compliance leads to taking a more effective, alternative treatment. He or she could infer upward bias instead if well-informed on the line of argument.
In practice, researchers frequently use causal language yet without mentioning any assumptions. This does not imply that they truly have a causal effect in mind, often causal and associational wordings are carelessly used in synonymous way. For example, concluding “depression increases the risk of lung cancer” constitutes already causal wording because it implies that a change in the depression status would change the cancer risk. Associational language like “lung cancer risk is elevated if depression occurs”, however, would allow for an elevated lung cancer risk in depression cases just because LC and D share some causes (“inducing” or “removing” depression would not change the cancer risk here).
Often, it is unclear where the path of argumentation from assumptions to a conclusion leads when alternative assumptions are made. Consider again bias due to selection. A different effect in target and source population occurs if effect-modifying variables distribute differently in both populations. Accordingly, the statistician should ask which variables influence the effect of interest, and whether these can be assumed to distribute equally in the source population and the target population. The substantive researcher might answer that the causal risk difference between D and LC likely increases with age. Given that this is true, and if elder individuals have been oversampled (e.g. because elderly are over-represented in primary care settings), both together would conclude that sampling has led to over-estimation (despite other factors, Fig. Fig.2 2 ).

If higher age is related to a larger effect (risk difference) of D on LC, a larger effect estimate is expected in an elder sample
However, the statistician might add, if effect modification is weak, or the difference in the age distributions is modest (e.g. mean 54 vs. 52 years), selection is unlikely to have produced large (here: upward) bias. In turn, another substantive researcher, who reads the resulting discussion, might instead assume a decrease of effect with increasing age and thus infer downward bias.
In practice, researchers should be extremely sensitive for bias due to selection if a sample has been drawn conditionally on a common consequence of factor and outcome or a variable associated with such a consequence [19 and references therein]. For instance, hospitalization might be influenced by both D and LC, and thus sampling from hospitals might introduce a false association or change an association’s sign; particularly D and LC may appear to be negatively associated although the association is positive in the general population (Fig. (Fig.3 3 ).

If hospitalization (H) is a common cause of D and LC, sampling conditionally on H can introduce a spurious association between D and LC ("conditioning on a collider")
Usually, only some kinds of bias are discussed, while the consequences of others are ignored [ 5 ]. Besides selection the main sources of bias are often measurement and confounding. If one is only interested in association, confounding is irrelevant. For causal conclusions, however, assumptions on all three kinds of bias are necessary.
Measurement error means that the measurement of a factor and/or outcome deviates from the true value, at least in some individuals. Bias due to measurement is known under many other terms that describe the reasons why such error occurs (e.g. “recall bias” and “reporting bias”). In contrast to conventional wisdom, measurement error does not always bias association and effect estimates downwards [ 5 , 6 ]. It does, for instance, if only the factor (e.g. depression) is measured with error and the errors occur independently from the outcome (e.g. lung cancer), or vice versa (“non-differential misclassification”) [22 and references therein]. However, many lung cancer cases might falsely report depression symptoms (e.g. to express need for care). Such false positives (non-cases of depression classified as cases) may also occur in non-cases of lung cancer but to a lesser extent (a special case of “differential misclassification”). Here, bias might be upward as well. Importantly, false positives cause larger bias than false negatives (non-cases of depression falsely classified as depression cases) as long as the relative frequency of a factor is lower than 50% [ 21 ]. Therefore, they should receive more attention in discussion. If measurement error occurs in depression and lung cancer, the direction of bias also depends on the correlation between both errors [ 21 ].
Note that what is in line with common standards of “good” measurement (e.g. a Kappa value measuring validity or reliability of 0.7) might anyway produce large bias. This applies to estimates of prevalence, association and effect. The reason is that while indices of measurement are one-dimensional, bias depends on two parameters (sensitivity and specificity) [ 21 , 22 ]. Moreover, estimates of such indices are often extrapolated to different kinds of populations (typically from a clinical to general population), what may be inadequate. Note that the different kinds of bias often interact, e.g. bias due to measurement might depend on selection (e.g. measurement error might differ between a clinical and a general population) [ 5 , 6 ].
Assessment of bias due to confounding variables (roughly speaking: common causes of factor and outcome) requires assumptions on the entire system of variables that affect both factor and outcome. For example, D and LC might share several causes such as stressful life events or socioeconomic status. If these influence D and LC with the same effect direction, this leads to overestimation, otherwise (different effect directions) the causal effect is underestimated. In the medical field, many unfavorable conditions may be positively related. If this holds true for all common factors of D and LC, upward bias can be assumed. However, not all confounders have to be taken into account. Within the framework of “causal graphs”, the “backdoor criterion” [ 7 ] provides a graphical rule for sets of confounders to be sufficient when adjusted for. Practically, such a causal graph must include all factors that directly or indirectly affect both D and LC. Then, adjustment for a set of confounders that meets the “backdoor criterion” in the graph completely removes bias due to confounding. In the example of Fig. Fig.4 4 it is sufficient to adjust for Z 1 and Z 2 because this “blocks” all paths that otherwise lead backwards from D to LC. Note that fully eliminating bias due to confounding also requires that the confounders have been collected without measurement error [ 5 , 6 , 23 ]. Therefore, the advice is always to concede at least some “residual” bias and reflect on the direction this might have (could be downward if such error is not stronger related to D and LC than a confounder itself).

Causal graph for the effect of D on LC and confounders Z 1 , Z 2 and Z 3
Whereas the statistician should pinpoint to the mathematical insight of the backdoor criterion, its application requires profound substantive input and literature review. Of course, there are numerous relevant factors in the medical field. Hence, one should practically focus on those with the highest prevalence (a very seldom factor can hardly cause large bias) and large assumed effects on both X and Y.
If knowledge on any of the three kinds of bias is poor or very uncertain, researchers should admit that this adds uncertainty in a conclusion: systematic error on top of random error. In the Bayesian framework, quantitative bias analysis formalizes this through the result of larger variance in an estimate. Technically, this additional variance is introduced via the variances of distributions assigned to “bias parameters”; for instance a misclassification probability (e.g. classifying a true depression case as non-case) or the prevalence of a binary confounder and its effects on X and Y. Of course, bias analysis also changes point estimates (hopefully reducing bias considerably). Note that conventional frequentist analysis, as regarded from the Bayesian perspective, assumes that all bias parameters were zero with a probability of one [ 5 , 6 , 23 ]. The only exceptions (bias addressed in conventional analyses) are adjustment on variables to hopefully reduce bias due to confounding and weighting the individuals (according to variables related to participation) to take into account bias due to selection.
If the substantive investigator understands the processes of selection, measurement and confounding only poorly, such strict analysis numerically reveals that little to nothing is known on the effect of X on Y, no matter how large an observed association and a sample (providing small random error) may be [ 5 , 6 , 23 ]). This insight has to be brought up by the statistician. Although such an analysis is complicated, itself very sensitive to how it is conducted [ 5 , 6 ] and rarely done, the Bayesian thinking behind it forces researchers to better understand the processes behind the data. Otherwise, he or she cannot make any assumptions and, in turn, no conclusion on causality.
Usually articles end with statements that only go little further than the always true but never informative statement “more research is needed”. Moreover, larger samples and better measurements are frequently proposed. If an association has been found, a RCT or other interventional study is usually proposed to investigate causality. In our example, this recommendation disregards that: (1) onset of D might have a different effect on LC risk than an intervention against D (the effect of onset cannot be investigated in any interventional study), (2) the effects of onset and intervention concern different populations (those without vs. those with depression), (3) an intervention effect depends on the mode of intervention [ 24 ], and (4) (applying the backdoor criterion) a well-designed observational study may approximatively yield the same result as a randomized study would [ 25 – 27 ]. If the effect of “removing” depression is actually of interest, one could propose an RCT that investigates the effect of treating depression in a strictly defined way and in a strictly defined population (desirably in all who meet the criteria of depression). Ideally, this population is sampled randomly, and non-participants and dropouts are investigated with respect to assumed effect-modifiers (differences in their distributions between participants and non-participants can then be addressed e.g. by weighting [ 27 ]). In a non-randomized study, one should collect variables supposed to meet the backdoor-criterion with the best instruments possible.
General recommendations
Yet when considering 1) through 7); i.e. carefully reflecting on the mechanisms that have created the data, discussions on statistical results can be very misleading, because the basic statistical methods are mis-interpreted or inadequately worded.
A common pitfall is to consider the lack of evidence for the alternative hypothesis (e.g. association between D and LC) as evidence for the null hypothesis (no association). In fact, such inference requires an a-priori calculated sample-size to ensure that the type-two error probability does not exceed a pre-specified limit (typically 20% or 10%, given the other necessary assumptions, e.g. on the true magnitude of association). Otherwise, the type-two error is unknown and in practice often large. This may put a “false negative result” into the scientific public that turns out to be “unreplicable” – what would be falsely interpreted as part of the “replication crisis”. Such results are neither positive nor negative but uninformative . In this case, the wording “there is no evidence for an association” is adequate because it does not claim that there is no association.
Frequently, it remains unclear which hypotheses have been a-priori specified and which have been brought up only after some data analysis. This, of course, is scientific malpractice because it does not enable the readership to assess the random error emerging from explorative data analysis. Accordingly, the variance of results across statistical methods is often misused to filter out the analysis that yields a significant result (“ p -hacking”, [ 28 ]). Pre-planned tests (via writing a grant) leave at least less room for p-hacking because they specify a-priori which analysis is to be conducted.
On the other hand, post-hoc analyses can be extremely useful for identifying unexpected phenomena and creating new hypotheses. Verbalization in the discussion section should therefore sharply separate between conclusions from hypothesis testing and new hypotheses created from data exploration. The distinction is profound, since a newly proposed hypothesis just makes a new claim. Suggesting new hypotheses cannot be wrong, this can only be inefficient if many hypotheses turn out to be wrong. Therefore, we suggest proposing only a limited number of new hypotheses that appear promising to stimulate further research and scientific progress. They are to be confirmed or falsified with future studies. A present discussion, however, should yet explicate the testable predictions a new hypothesis entails, and how a future study should be designed to keep bias in related analyses as small as possible.
Confidence intervals address the problem of reducing results to the dichotomy of significant and non-significant through providing a range of values that are compatible with the data at the given confidence level, usually 95% [ 29 ].
This is also addressed by Bayesian statistics that allows calculating what frequentist p -values are often misinterpreted to be: the probability that the alternative (or null) hypothesis is true [ 17 ]. Moreover, one can calculate how likely it is that the parameter lies within any specified range (e.g. the risk difference being greater than .05, a lower boundary for practical significance) [ 15 , 16 ]. To gain these benefits, one needs to specify how the parameter of interest (e.g. causal risk difference between D and LC) is distributed before inspecting the data. In Bayesian statistics (unlike frequentist statistics) a parameter is a random number that expresses prior beliefs via a “prior distribution”. Such a “prior” is combined with the data result to a “posterior distribution”. This integrates both sources of information.
Note that confidence intervals also can be interpreted from the Bayesian perspective (then called “credibility interval”). This assumes that all parameter values were equally likely (uniformly distributed, strictly speaking) before analyzing the data [ 5 , 6 , 20 ].
Testing just for a non-zero association can only yield evidence for an association deviating from zero. A better indicator for the true impact of an effect/association for clinical, economic, political, or research purposes is its magnitude. If an association between D and LC after adjusting for age and gender has been discovered, then the knowledge of D has additional value in predicting an elevated LC probability beyond age and gender. However, there may be many other factors that stronger predict LC and thus should receive higher priority in a doctor’s assessment. Besides, if an association is small, it may yet be explained by modest (upward) bias. Especially large samples often yield significant results with little practical value. The p -value does not measure strength of association [ 17 ]. For instance, in a large sample, a Pearson correlation between two dimensional variables could equal 0.1 only but with a p -value <.001. A further problem arises if the significance threshold of .05 is weakened post-hoc to allow for “statistical trends” ( p between .05 and .10) because a result has “failed to reach significance” (this wording claims that there is truly an association. If this was known, no research would be necessary).
It is usually the statistician’s job to insist not only on removing the attention from pure statistical significance to confidence intervals or even Bayesian interpretation, but also to point out the necessity of a meaningful cutoff for practical significance. The substantive researcher then has to provide this cutoff.
Researchers should not draw conclusions that have not been explicitly tested for. For example, one may have found a positive association between D and LC (e.g. p = .049), but this association is not significant (e.g. p = .051), when adjusting for “health behavior”. This does not imply that “health behavior” “explains” the association (yet fully). The difference in magnitude of association in both analyses compared here (without and with adjustment on HB) may be very small and the difference in p -values (“borderline significance” after adjustment) likely to emerge from random error. This often applies to larger differences in p as well.
Investigators, however, might find patterns in their results that they consider worth mentioning for creating hypotheses. In the example above, adding the words “in the sample”, would clarify that they refer just to the difference of two point estimates . By default, “association” in hypotheses testing should mean “statistically significant association” (explorative analyses should instead refer to “suggestive associations”).
Conclusions
Some issues of discussing results not mentioned yet appear to require only substantive reasoning. For instance, Bradford Hill’s consideration on “plausibility” claims that a causal effect is more likely, if it is in line with biological (substantive) knowledge, or if a dose-response relation has been found [ 30 ]. However, the application of these considerations itself depends on the trueness of assumptions. For instance, bias might act differently across the dose of exposure (e.g. larger measurement error in outcome among those with higher dosage). As a consequence, a pattern observed across dose may mask a true or pretend a wrong dose-response relation [ 30 ]. This again has to be brought up by statistical expertise.
There are, however, some practical issues that hinder the cooperation we suggest. First, substantive researchers often feel discomfort when urged to make assumptions on the mechanisms behind the data, presumably because they fear to be wrong. Here, the statistician needs to insist: “If you are unable to make any assumptions, you cannot conclude anything!” And: “As a scientist you have to understand the processes that create your data.” See [ 31 ] for practical advice on how to arrive at meaningful assumptions.
Second, statisticians have long been skeptical against causal inference. Still, most of them focus solely on describing observed data with distributional models, probably because estimating causal effects has long been regarded as unfeasible with scientific methods. Training in causality remains rather new, since strict mathematical methods have been developed only in the last decades [ 7 ].
The cooperation could be improved if education in both fields focused on the insight that one cannot succeed without the other. Academic education should demonstrate that in-depth conclusions from data unavoidably involve prior beliefs. Such education should say: Data do not “speak for themselves”, because they “speak” only ambiguously and little, since they have been filtered through various biases [ 32 ]. The subjectivity introduced by addressing bias, however, unsettles many researchers. On the other hand, conventional frequentist statistics just pretends to be objective. Instead of accepting the variety of possible assumptions, it makes the absurd assumption of “no bias with probability of one”. Or it avoids causal conclusions at all if no randomized study is possible. This limits science to investigating just associations for all factors that can never be randomized (e.g. onset of depression). However, the alternative of Bayesian statistics and thinking are themselves prone to fundamental cognitive biases which should as well be subject of interdisciplinary teaching [ 33 ].
Readers may take this article as an invitation to read further papers’ discussions differently while evaluating our claims. Rather than sharing a provided conclusion (or not) they could ask themselves whether a discussion enables them to clearly specify why they share it (or not). If the result is uncertainty, this might motivate them to write their next discussion differently. The proposals made in this article could help shifting scientific debates to where they belong. Rather than arguing on misunderstandings caused by ambiguity in a conclusion’s assumptions one should argue on the assumptions themselves.
Acknowledgements
We acknowledge support by the German Research Foundation and the Open Access Publication Funds of the TU Dresden. We wish to thank Pia Grabbe and Helen Steiner for language editing and the cited authors for their outstanding work that our proposals build on.
John Venz is funded by the German Federal Ministry of Education and Research (BMBF) project no. 01ER1303 and 01ER1703. He has contributed to this manuscript outside of time funded by these projects.
Availability of data and materials
Abbreviations.
D | depression |
HB | health behavior |
LC | lung cancer |
RCT | randomized clinical trial |
X | factor variable |
Y | outcome variable |
Authors’ contributions
MH and RM had the initial idea on the article. MH has taken the lead in writing. JV has contributed to the statistical parts, especially the Bayesian aspects. RM has refined the paragraphs on statistical inference. ST joined later and has added many clarifications related to the perspective of the substantive researcher. All authors have contributed to the final wording of all sections and the article’s revision. All authors read and approved the final manuscript.
Ethics approval and consent to participate
Consent for publication, competing interests.
The authors declare that they have no competing interests.
Publisher’s Note
Springer Nature remains neutral with regard to jurisdictional claims in published maps and institutional affiliations.

- Walden University
- Faculty Portal
General Research Paper Guidelines: Discussion
Discussion section.
The overall purpose of a research paper’s discussion section is to evaluate and interpret results, while explaining both the implications and limitations of your findings. Per APA (2020) guidelines, this section requires you to “examine, interpret, and qualify the results and draw inferences and conclusions from them” (p. 89). Discussion sections also require you to detail any new insights, think through areas for future research, highlight the work that still needs to be done to further your topic, and provide a clear conclusion to your research paper. In a good discussion section, you should do the following:
- Clearly connect the discussion of your results to your introduction, including your central argument, thesis, or problem statement.
- Provide readers with a critical thinking through of your results, answering the “so what?” question about each of your findings. In other words, why is this finding important?
- Detail how your research findings might address critical gaps or problems in your field
- Compare your results to similar studies’ findings
- Provide the possibility of alternative interpretations, as your goal as a researcher is to “discover” and “examine” and not to “prove” or “disprove.” Instead of trying to fit your results into your hypothesis, critically engage with alternative interpretations to your results.
For more specific details on your Discussion section, be sure to review Sections 3.8 (pp. 89-90) and 3.16 (pp. 103-104) of your 7 th edition APA manual
*Box content adapted from:
University of Southern California (n.d.). Organizing your social sciences research paper: 8 the discussion . https://libguides.usc.edu/writingguide/discussion
Limitations
Limitations of generalizability or utility of findings, often over which the researcher has no control, should be detailed in your Discussion section. Including limitations for your reader allows you to demonstrate you have thought critically about your given topic, understood relevant literature addressing your topic, and chosen the methodology most appropriate for your research. It also allows you an opportunity to suggest avenues for future research on your topic. An effective limitations section will include the following:
- Detail (a) sources of potential bias, (b) possible imprecision of measures, (c) other limitations or weaknesses of the study, including any methodological or researcher limitations.
- Sample size: In quantitative research, if a sample size is too small, it is more difficult to generalize results.
- Lack of available/reliable data : In some cases, data might not be available or reliable, which will ultimately affect the overall scope of your research. Use this as an opportunity to explain areas for future study.
- Lack of prior research on your study topic: In some cases, you might find that there is very little or no similar research on your study topic, which hinders the credibility and scope of your own research. If this is the case, use this limitation as an opportunity to call for future research. However, make sure you have done a thorough search of the available literature before making this claim.
- Flaws in measurement of data: Hindsight is 20/20, and you might realize after you have completed your research that the data tool you used actually limited the scope or results of your study in some way. Again, acknowledge the weakness and use it as an opportunity to highlight areas for future study.
- Limits of self-reported data: In your research, you are assuming that any participants will be honest and forthcoming with responses or information they provide to you. Simply acknowledging this assumption as a possible limitation is important in your research.
- Access: Most research requires that you have access to people, documents, organizations, etc.. However, for various reasons, access is sometimes limited or denied altogether. If this is the case, you will want to acknowledge access as a limitation to your research.
- Time: Choosing a research focus that is narrow enough in scope to finish in a given time period is important. If such limitations of time prevent you from certain forms of research, access, or study designs, acknowledging this time restraint is important. Acknowledging such limitations is important, as they can point other researchers to areas that require future study.
- Potential Bias: All researchers have some biases, so when reading and revising your draft, pay special attention to the possibilities for bias in your own work. Such bias could be in the form you organized people, places, participants, or events. They might also exist in the method you selected or the interpretation of your results. Acknowledging such bias is an important part of the research process.
- Language Fluency: On occasion, researchers or research participants might have language fluency issues, which could potentially hinder results or how effectively you interpret results. If this is an issue in your research, make sure to acknowledge it in your limitations section.
University of Southern California (n.d.). Organizing your social sciences research paper: Limitations of the study . https://libguides.usc.edu/writingguide/limitations
In many research papers, the conclusion, like the limitations section, is folded into the larger discussion section. If you are unsure whether to include the conclusion as part of your discussion or as a separate section, be sure to defer to the assignment instructions or ask your instructor.
The conclusion is important, as it is specifically designed to highlight your research’s larger importance outside of the specific results of your study. Your conclusion section allows you to reiterate the main findings of your study, highlight their importance, and point out areas for future research. Based on the scope of your paper, your conclusion could be anywhere from one to three paragraphs long. An effective conclusion section should include the following:
- Describe the possibilities for continued research on your topic, including what might be improved, adapted, or added to ensure useful and informed future research.
- Provide a detailed account of the importance of your findings
- Reiterate why your problem is important, detail how your interpretation of results impacts the subfield of study, and what larger issues both within and outside of your field might be affected from such results
University of Southern California (n.d.). Organizing your social sciences research paper: 9. the conclusion . https://libguides.usc.edu/writingguide/conclusion
- Previous Page: Results
- Next Page: References
- Office of Student Disability Services
Walden Resources
Departments.
- Academic Residencies
- Academic Skills
- Career Planning and Development
- Customer Care Team
- Field Experience
- Military Services
- Student Success Advising
- Writing Skills
Centers and Offices
- Center for Social Change
- Office of Academic Support and Instructional Services
- Office of Degree Acceleration
- Office of Research and Doctoral Services
- Office of Student Affairs
Student Resources
- Doctoral Writing Assessment
- Form & Style Review
- Quick Answers
- ScholarWorks
- SKIL Courses and Workshops
- Walden Bookstore
- Walden Catalog & Student Handbook
- Student Safety/Title IX
- Legal & Consumer Information
- Website Terms and Conditions
- Cookie Policy
- Accessibility
- Accreditation
- State Authorization
- Net Price Calculator
- Contact Walden
Walden University is a member of Adtalem Global Education, Inc. www.adtalem.com Walden University is certified to operate by SCHEV © 2024 Walden University LLC. All rights reserved.
Have a language expert improve your writing
Run a free plagiarism check in 10 minutes, automatically generate references for free.
- Knowledge Base
- Dissertation
- How to Write a Discussion Section | Tips & Examples
How to Write a Discussion Section | Tips & Examples
Published on 21 August 2022 by Shona McCombes . Revised on 25 October 2022.
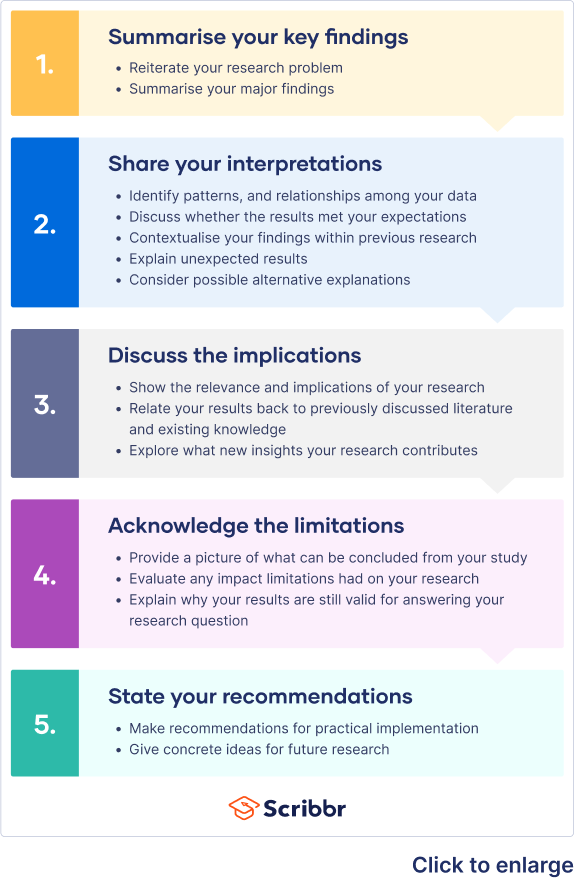
The discussion section is where you delve into the meaning, importance, and relevance of your results .
It should focus on explaining and evaluating what you found, showing how it relates to your literature review , and making an argument in support of your overall conclusion . It should not be a second results section .
There are different ways to write this section, but you can focus your writing around these key elements:
- Summary: A brief recap of your key results
- Interpretations: What do your results mean?
- Implications: Why do your results matter?
- Limitations: What can’t your results tell us?
- Recommendations: Avenues for further studies or analyses
Instantly correct all language mistakes in your text
Be assured that you'll submit flawless writing. Upload your document to correct all your mistakes.
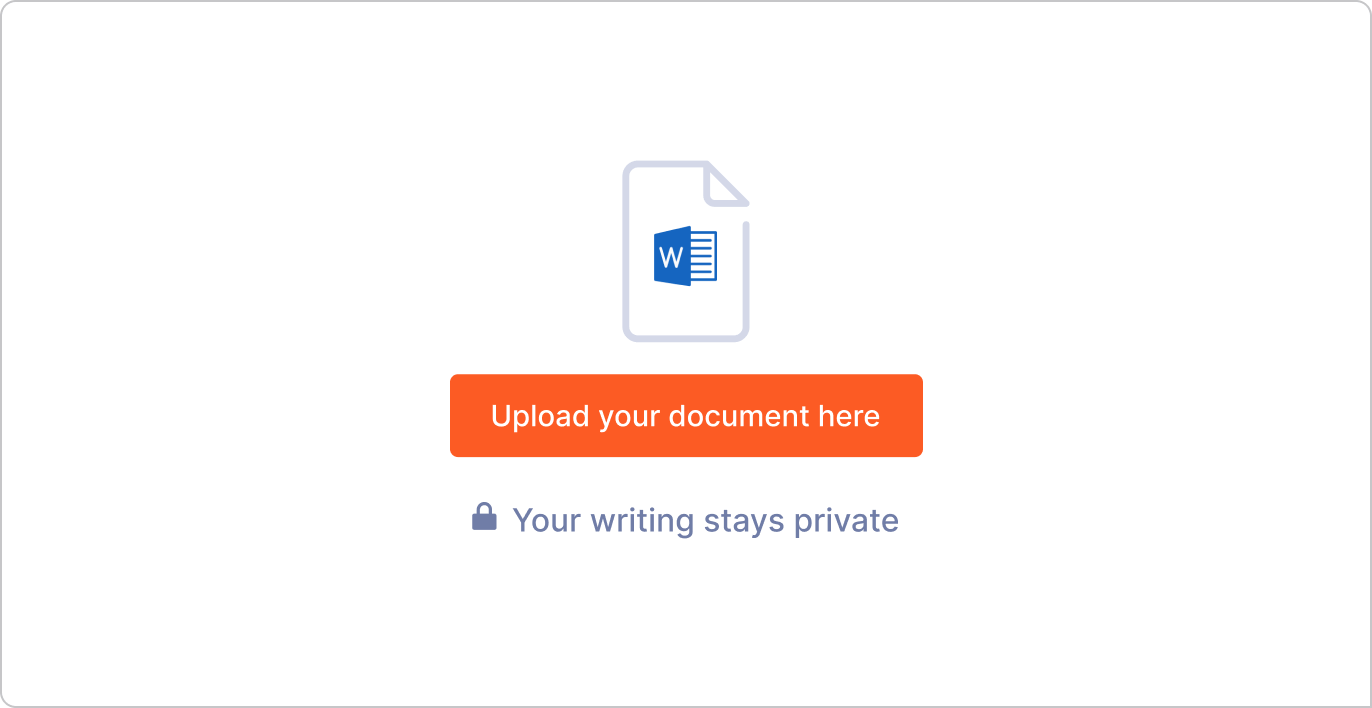
Table of contents
What not to include in your discussion section, step 1: summarise your key findings, step 2: give your interpretations, step 3: discuss the implications, step 4: acknowledge the limitations, step 5: share your recommendations, discussion section example.
There are a few common mistakes to avoid when writing the discussion section of your paper.
- Don’t introduce new results: You should only discuss the data that you have already reported in your results section .
- Don’t make inflated claims: Avoid overinterpretation and speculation that isn’t directly supported by your data.
- Don’t undermine your research: The discussion of limitations should aim to strengthen your credibility, not emphasise weaknesses or failures.
Prevent plagiarism, run a free check.
Start this section by reiterating your research problem and concisely summarising your major findings. Don’t just repeat all the data you have already reported – aim for a clear statement of the overall result that directly answers your main research question . This should be no more than one paragraph.
Many students struggle with the differences between a discussion section and a results section . The crux of the matter is that your results sections should present your results, and your discussion section should subjectively evaluate them. Try not to blend elements of these two sections, in order to keep your paper sharp.
- The results indicate that …
- The study demonstrates a correlation between …
- This analysis supports the theory that …
- The data suggest that …
The meaning of your results may seem obvious to you, but it’s important to spell out their significance for your reader, showing exactly how they answer your research question.
The form of your interpretations will depend on the type of research, but some typical approaches to interpreting the data include:
- Identifying correlations , patterns, and relationships among the data
- Discussing whether the results met your expectations or supported your hypotheses
- Contextualising your findings within previous research and theory
- Explaining unexpected results and evaluating their significance
- Considering possible alternative explanations and making an argument for your position
You can organise your discussion around key themes, hypotheses, or research questions, following the same structure as your results section. Alternatively, you can also begin by highlighting the most significant or unexpected results.
- In line with the hypothesis …
- Contrary to the hypothesised association …
- The results contradict the claims of Smith (2007) that …
- The results might suggest that x . However, based on the findings of similar studies, a more plausible explanation is x .
As well as giving your own interpretations, make sure to relate your results back to the scholarly work that you surveyed in the literature review . The discussion should show how your findings fit with existing knowledge, what new insights they contribute, and what consequences they have for theory or practice.
Ask yourself these questions:
- Do your results support or challenge existing theories? If they support existing theories, what new information do they contribute? If they challenge existing theories, why do you think that is?
- Are there any practical implications?
Your overall aim is to show the reader exactly what your research has contributed, and why they should care.
- These results build on existing evidence of …
- The results do not fit with the theory that …
- The experiment provides a new insight into the relationship between …
- These results should be taken into account when considering how to …
- The data contribute a clearer understanding of …
- While previous research has focused on x , these results demonstrate that y .
Even the best research has its limitations. Acknowledging these is important to demonstrate your credibility. Limitations aren’t about listing your errors, but about providing an accurate picture of what can and cannot be concluded from your study.
Limitations might be due to your overall research design, specific methodological choices , or unanticipated obstacles that emerged during your research process.
Here are a few common possibilities:
- If your sample size was small or limited to a specific group of people, explain how generalisability is limited.
- If you encountered problems when gathering or analysing data, explain how these influenced the results.
- If there are potential confounding variables that you were unable to control, acknowledge the effect these may have had.
After noting the limitations, you can reiterate why the results are nonetheless valid for the purpose of answering your research question.
- The generalisability of the results is limited by …
- The reliability of these data is impacted by …
- Due to the lack of data on x , the results cannot confirm …
- The methodological choices were constrained by …
- It is beyond the scope of this study to …
Based on the discussion of your results, you can make recommendations for practical implementation or further research. Sometimes, the recommendations are saved for the conclusion .
Suggestions for further research can lead directly from the limitations. Don’t just state that more studies should be done – give concrete ideas for how future work can build on areas that your own research was unable to address.
- Further research is needed to establish …
- Future studies should take into account …
- Avenues for future research include …
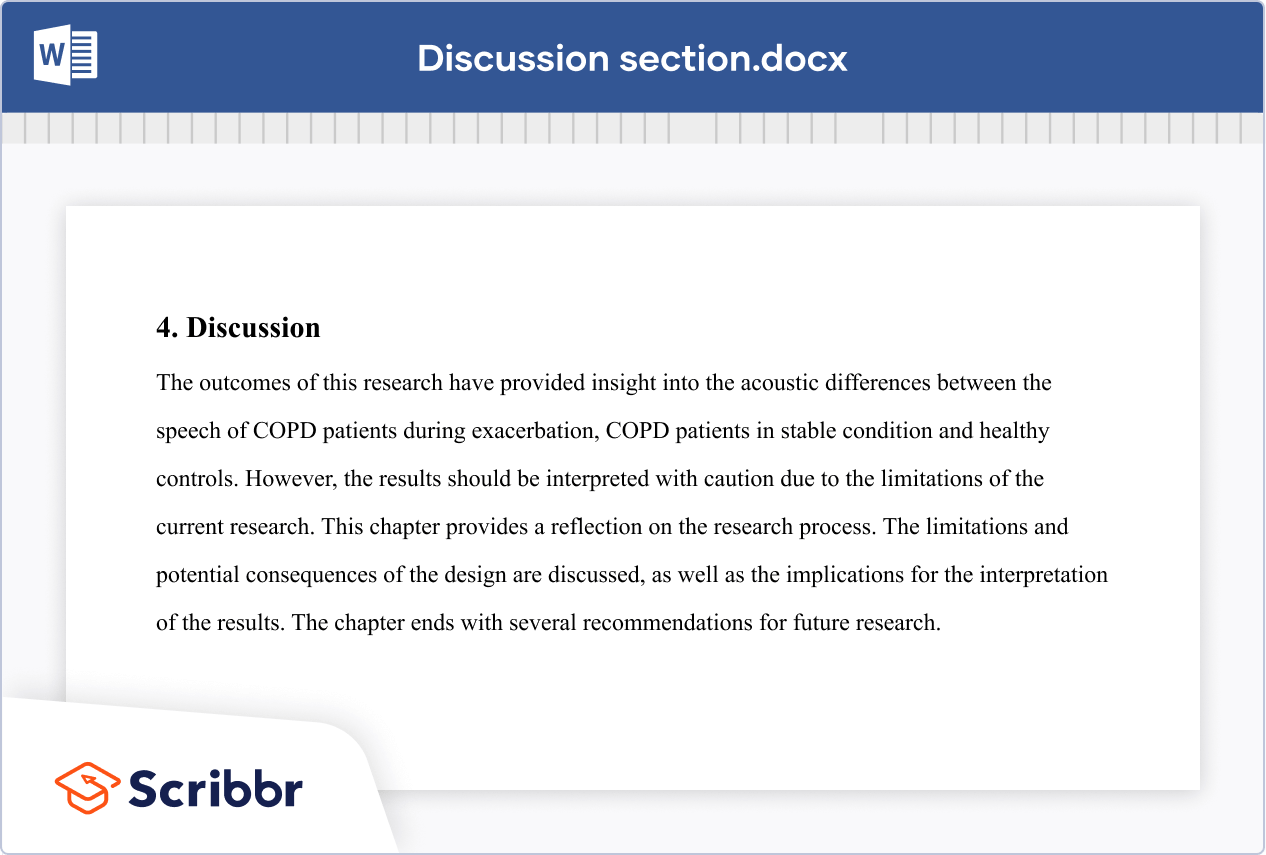
Cite this Scribbr article
If you want to cite this source, you can copy and paste the citation or click the ‘Cite this Scribbr article’ button to automatically add the citation to our free Reference Generator.
McCombes, S. (2022, October 25). How to Write a Discussion Section | Tips & Examples. Scribbr. Retrieved 24 June 2024, from https://www.scribbr.co.uk/thesis-dissertation/discussion/
Is this article helpful?
Shona McCombes
Other students also liked, how to write a results section | tips & examples, research paper appendix | example & templates, how to write a thesis or dissertation introduction.
✨ Enrol by 3 July to get access to our Summer Writing Accelerator at no additional cost! 💰 ✨
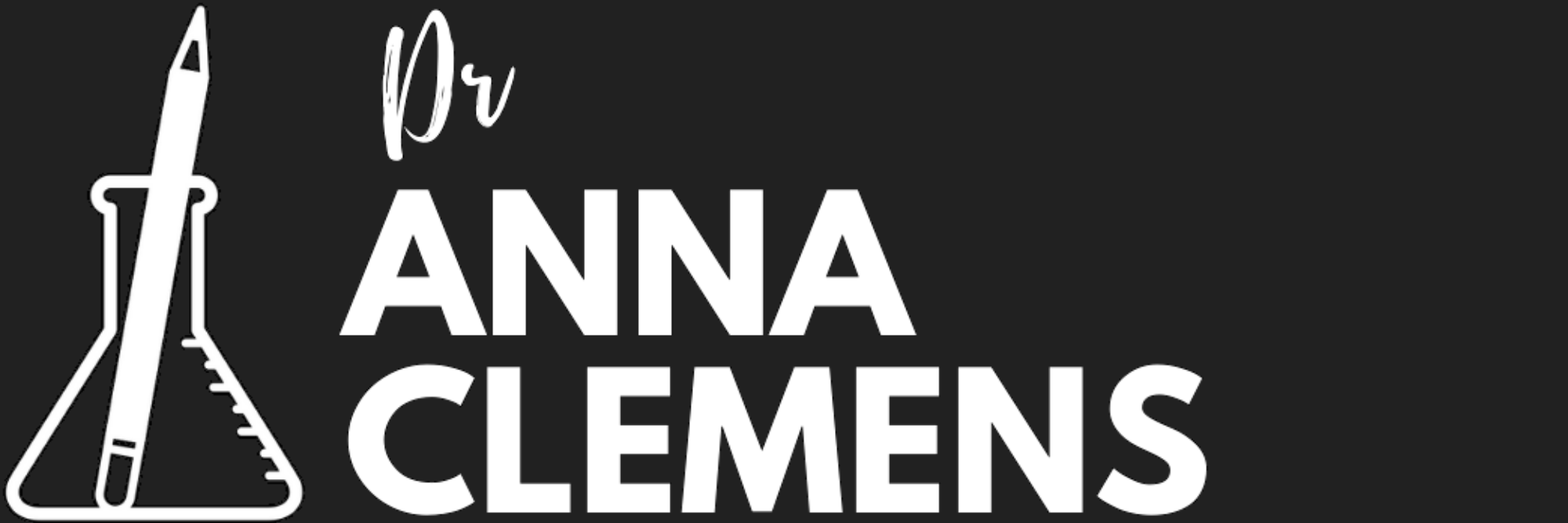
How (Not) to Write the Discussion Section of a Research Paper
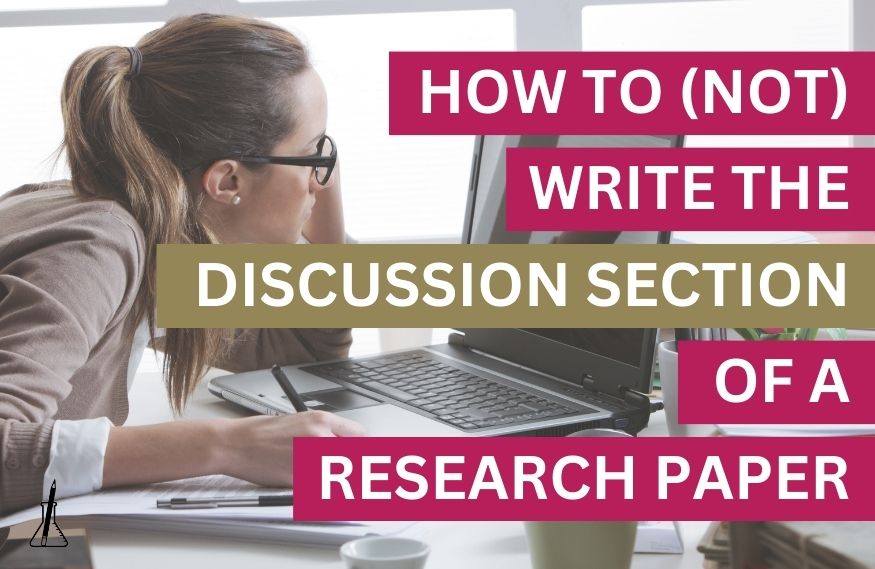
What the Discussion section of a scientific paper should and shouldn’t contain.
The Discussion section might well be the hardest part of a paper to write. Yet, so useful! In a great Discussion section, the authors tie the different findings of the paper together, analyse them in the context of existing literature, offer speculations, suggest further research and highlight the study’s significance and possible impact.
I love reading well written Discussions sections – it’s not uncommon that I only really understand the author’s contributions after I’ve read this section, especially when the research is somewhat outside my field of expertise. At the same time, I rarely come across well written discussions of research studies.
Why is that? I think a major factor is that the discussion is the least rigid part of a paper, so many authors are simply at a loss as to what to write. Another reason might be that the authors understand their results and what they mean so well that they cannot imagine what kind of discussion the reader would need in order to make sense out of the findings. It often is hard to take a step back to look at the bigger picture.
The Discussion section in a research paper: The 4 most common mistakes
Therefore, I’ve gathered the four most common mistakes I see scientists make in Discussion sections and offer some advice on how to improve them:
1. Providing paragraphs of background information
Authors who do this probably intend to put their results into context. However, the discussion section is neither the right place to reiterate background info nor to mention it for the first time. The correct place for contextualising your study is the introduction section.
This doesn’t mean that you shouldn’t reference other studies in your discussion. But instead of writing a big block containing only background information, I recommend to refer to other studies in connection with the discussion of your findings. It’s great to mention, for example, whether and why your results and hypotheses agree or disagree with the findings and hypotheses of other studies.
2. Not restating the problem you are solving
Your readers will likely either skip right to your discussion section (I often do this when I’m researching for stories I’m writing about), or they just have just read your results section. In either case, it makes sense to quickly restate why you have researched this topic. For those who skipped your “Introduction” and “Results” sections, reading a quick motivation for your study helps them to put your study into context. Chronological readers will have just deep-dived into the nitty-gritty of your results. By restating the problem you are solving, you help them to zoom out again and remind them of the “why”.
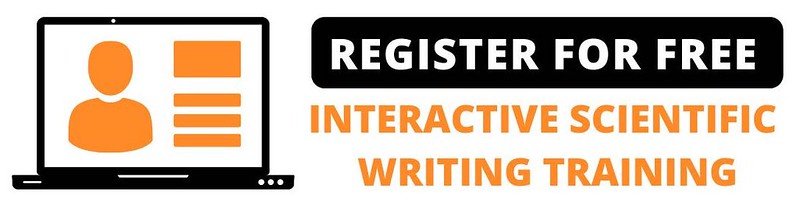
3. Describing the purpose and result of each experiment
Or in other words, don’t confuse what belongs in the “Results” and what in the “Discussion” section. So, skip the “First, we… Then, it was… In order to, we next…”. While I absolutely recommend to state the purpose and result of each experiment, hence guiding your reader through your study’s narrative, it belongs in the “Results” section. Or put differently, once the reader arrives at the discussion section, your story line should be clear. If you compare the story in a scientific paper to a drama, your discussion corresponds to the “Resolution” part of a story. The reader wants to know how things have changed for the characters of your story (aka your model system or study subject) after you obtained your results, how your study has changed the state of the art, or what implications your findings have for an application.
That said, stating the main conclusions of your results in the discussion section is absolutely recommended. But use the remaining space to actually discuss your findings.
4. Too general implication statements
Every discussion section should contain some sentences that outline the significance and importance of your findings. To be fair, most papers contain at least one sentence about this. However, all too often these statements are very general and broad. It’s okay to speculate here about possible applications or a likely impact your findings might have. But try to paint a more precise picture than just saying that your novel material “could be used in nanotechnology applications”. As a reader, I want to know what applications it will be good for specifically? And how exactly will it benefit those applications?
These are the four most common mistakes I see people make in their discussion section. At last, a little word of warning. Sometimes authors prefer to write a combined “Results and Discussion” section. While this can be appropriate in some cases, be careful to not skip discussing your results when doing this and offer an analysis beyond presenting and interpreting your findings.
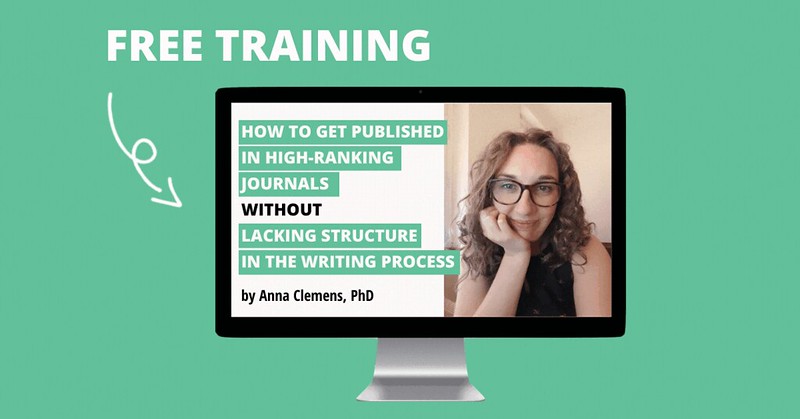
Procrastinating on your writing? Not feeling like you’re effective at communicating clearly and/or getting desk-rejected a lot?
In this free online training for science researchers, Dr Anna Clemens introduces you to her step-by-step system to write clear & concise papers for your target journals in a timely manner.
Share article
© Copyright 2018-2024 by Anna Clemens. All Rights Reserved.
Photography by Alice Dix
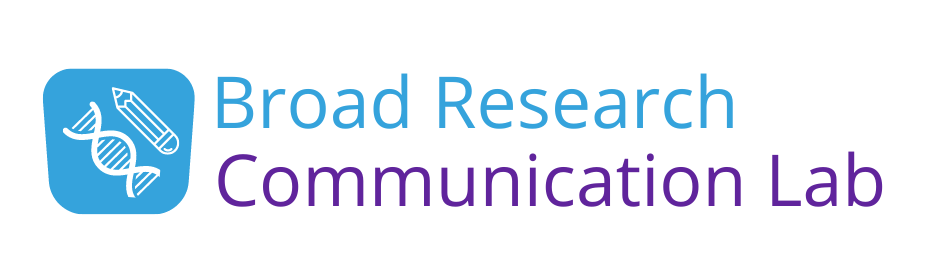
Journal Article: Discussion
Criteria for success.
A strong Discussion section:
- Tells the main conclusion of the paper in one or two sentences.
- Tells how the paper’s results contribute to answering the big questions posed in the Introduction.
- Explains how (and why) this work agrees or disagrees with other, similar work.
- Explains how the limitations of this study leave the big questions unanswered.
- Tells how extensions of this paper’s results will be useful for answering the big questions.
Structure Diagram
The Discussion is the part of your paper where you can share what you think your results mean with respect to the big questions you posed in your Introduction. The Introduction and Discussion are natural partners: the Introduction tells the reader what question you are working on and why you did this experiment to investigate it; the Discussion tells the reader what the results of that experiment have to say about the bigger question.
Imagine you explained the results in the paper to a labmate who looks confused and asks you, “Sure, but so what? Why was this cool or interesting?” Your response to your labmate should be similar to the content in the Discussion.
Analyze Your Audience
Different kinds of readers will expect different things from your Discussion. Readers who are not experts in your field might read your Discussion before your Results in the hopes that they can learn what your Results mean and why your paper is important without having to learn how to interpret your experimental results. They might also be interested to know what you think the future of your field is. Readers who are more familiar with your field will generally understand what the results of your experiments say, but they will be curious about how you interpreted confusing, conflicting, or complicated results.
As you write your Discussion, decide who will find each paragraph interesting and what you want them to take away from it. Successful Discussions can simultaneously provide the specific, nuanced information that experts want to read and the broader, more general statements that non-experts can appreciate.
The balance between expert and non-expert readers in your target audience will depend on the journal to which you submit. High-profile, general readership journals will have more non-expert readers, while more technical, field-specific journals have almost exclusively expert readers.
Tell how your paper is special
Weak Discussions begin with a summary of the results or a repetition of the main points of the Introduction. Strong Discussions immediately carve out a place for themselves in the large universe of papers by saying what makes this one interesting or special. One way to do this is to start the Discussion with one or two sentences that state the main finding from the results and what that finding means for the field.
Relate your results to existing results
In the Introduction, you probably helped motivate your study by citing previous results in your field. Now that you’ve laid out your results, you should tell whether your results agree or disagree with prior work and why. You might have extended previous work, showed how apparently conflicting results are actually harmonious, or exposed a contradiction that currently has no explanation.
Tell how your study’s limitations leave open the big questions
Every study is finite: you did some things and not others, and you used methods that can explain some phenomena but not others. How do the limitations of your study leave open the bigger questions? Do you just need to do more of the same kind of work? Have you shown that current methods are inadequate for answering the big question?
Every paper is a contribution to a larger scientific conversation. Hopefully, you think your contribution is somehow useful to that conversation: it provides new information or tools that will help you or other researchers move toward answers to the big questions. To explain this contribution, many Discussions end with a forward-looking statement that tries to place the paper in an expected future of research in that field.
This content was adapted from from an article originally created by the MIT Biological Engineering Communication Lab .
Resources and Annotated Examples
Annotated example 1.
This is the discussion for an article published in Science Translational Medicine . 6 MB
Annotated Example 2
This is the discussion for an article published in Cell . 325 KB
Pardon Our Interruption
As you were browsing something about your browser made us think you were a bot. There are a few reasons this might happen:
- You've disabled JavaScript in your web browser.
- You're a power user moving through this website with super-human speed.
- You've disabled cookies in your web browser.
- A third-party browser plugin, such as Ghostery or NoScript, is preventing JavaScript from running. Additional information is available in this support article .
To regain access, please make sure that cookies and JavaScript are enabled before reloading the page.

The Process of Writing a Research Paper Guide: The Discussion
- Types of Research Designs
- Choosing a Research Topic
- Preparing to Write
- The Abstract
- The Introduction
- The Literature Review
- The Methodology
- The Results
- The Discussion
- The Conclusion
- Proofreading Your Paper
- Citing Sources
- Annotated Bibliography
- Giving an Oral Presentation
- How to Manage Group Projects
- Writing a Book Review
- Writing a Research Proposal
- Acknowledgements
The purpose of the discussion is to interpret and describe the significance of your findings in light of what was already known about the research problem being investigated and to explain any new understanding or insights that emerged as a result of your study of the problem. The discussion will always connect to the introduction by way of the research questions or hypotheses you posed and the literature you reviewed, but the discussion does not simply repeat or rearrange the first parts of your paper; the discussion clearly explain how your study advanced the reader's understanding of the research problem from where you left them at the end of your review of prior research.
Importance of a Good Discussion
The discussion section is often considered the most important part of your research paper because this is where you:
- Most effectively demonstrates your ability as a researcher to think critically about an issue, to develop creative solutions to problems based upon a logical synthesis of the findings, and to formulate a deeper, more profound understanding of the research problem under investigation,
- Present the underlying meaning of your research, note possible implications in other areas of study, and explore possible improvements that can be made in order to further develop the concerns of your research,
- Highlight the importance of your study and how it may be able to contribute to and/or help fill existing gaps in the field. If appropriate, the discussion section is also where you state how the findings from your study revealed and helped fill gaps in the literature that had not been previously exposed or adequately described, and
- Engage the reader in thinking critically about issues based upon an evidence-based interpretation of findings; it is not governed strictly by objective reporting of information.
Annesley Thomas M. “The Discussion Section: Your Closing Argument.” Clinical Chemistry 56 (November 2010): 1671-1674; Bitchener, John and Helen Basturkmen. “Perceptions of the Difficulties of Postgraduate L2 Thesis Students Writing the Discussion Section.” Journal of English for Academic Purposes 5 (January 2006): 4-18; Kretchmer, Paul. Fourteen Steps to Writing an Effective Discussion Section . San Francisco Edit, 2003-2008.
Structure and Writing Style
I. General Rules
These are the general rules you should adopt when composing your discussion of the results :
- Do not be verbose or repetitive
- Be concise and make your points clearly
- Avoid the use of jargon or undefined technical language
- Follow a logical stream of thought; in general, interpret and discuss the significance of your findings in the same sequence you described them in your results section [a notable exception is to begin by highlighting an unexpected result or finding]
- Use the present verb tense, especially for established facts; however, refer to specific works or prior studies in the past tense
- If needed, use subheadings to help organize your discussion or to categorize your interpretations into themes
II. The Content
The content of the discussion section of your paper most often includes :
- Explanation of results : comment on whether or not the results were expected for each set of results; go into greater depth to explain findings that were unexpected or especially profound. If appropriate, note any unusual or unanticipated patterns or trends that emerged from your results and explain their meaning in relation to the research problem.
- References to previous research : either compare your results with the findings from other studies or use the studies to support a claim. This can include re-visiting key sources already cited in your literature review section, or, save them to cite later in the discussion section if they are more important to compare with your results instead of being a part of the general literature review of research used to provide context and background information. Note that you can make this decision to highlight specific studies after you have begun writing the discussion section.
- Deduction : a claim for how the results can be applied more generally. For example, describing lessons learned, proposing recommendations that can help improve a situation, or highlighting best practices.
- Hypothesis : a more general claim or possible conclusion arising from the results [which may be proved or disproved in subsequent research]. This can be framed as new research questions that emerged as a result of your analysis.
III. Organization and Structure
Keep the following sequential points in mind as you organize and write the discussion section of your paper:
- Think of your discussion as an inverted pyramid. Organize the discussion from the general to the specific, linking your findings to the literature, then to theory, then to practice [if appropriate].
- Use the same key terms, narrative style, and verb tense [present] that you used when when describing the research problem in your introduction.
- Begin by briefly re-stating the research problem you were investigating and answer all of the research questions underpinning the problem that you posed in the introduction.
- Describe the patterns, principles, and relationships shown by each major findings and place them in proper perspective. The sequence of this information is important; first state the answer, then the relevant results, then cite the work of others. If appropriate, refer the reader to a figure or table to help enhance the interpretation of the data [either within the text or as an appendix].
- Regardless of where it's mentioned, a good discussion section includes analysis of any unexpected findings. This part of the discussion should begin with a description of the unanticipated finding, followed by a brief interpretation as to why you believe it appeared and, if necessary, its possible significance in relation to the overall study. If more than one unexpected finding emerged during the study, describe each of them in the order they appeared as you gathered or analyzed the data. As noted, the exception to discussing findings in the same order you described them in the results section would be to begin by highlighting the implications of a particularly unexpected or significant finding that emerged from the study, followed by a discussion of the remaining findings.
- Before concluding the discussion, identify potential limitations and weaknesses if you do not plan to do so in the conclusion of the paper. Comment on their relative importance in relation to your overall interpretation of the results and, if necessary, note how they may affect the validity of your findings. Avoid using an apologetic tone; however, be honest and self-critical [e.g., had you included a particular question in a survey instrument, additional data could have been revealed].
- The discussion section should end with a concise summary of the principal implications of the findings regardless of their significance. Give a brief explanation about why you believe the findings and conclusions of your study are important and how they support broader knowledge or understanding of the research problem. This can be followed by any recommendations for further research. However, do not offer recommendations which could have been easily addressed within the study. This would demonstrate to the reader that you have inadequately examined and interpreted the data.
IV. Overall Objectives
The objectives of your discussion section should include the following: I. Reiterate the Research Problem/State the Major Findings
Briefly reiterate the research problem or problems you are investigating and the methods you used to investigate them, then move quickly to describe the major findings of the study. You should write a direct, declarative, and succinct proclamation of the study results, usually in one paragraph.
II. Explain the Meaning of the Findings and Why They are Important
Consider the likelihood that no one has thought as long and hard about your study as you have. Systematically explain the underlying meaning of your findings and state why you believe they are significant. After reading the discussion section, you want the reader to think critically about the results [“why didn't I think of that?”]. You don’t want to force the reader to go through the paper multiple times to figure out what it all means. If applicable, begin this part of the section by repeating what you consider to be your most significant or unanticipated finding first, then systematically review each finding. Otherwise, follow the general order you reported the findings in the results section.
III. Relate the Findings to Similar Studies
No study in the social sciences is so novel or possesses such a restricted focus that it has absolutely no relation to previously published research. The discussion section should relate your results to those found in other studies, particularly if questions raised from prior studies served as the motivation for your research. This is important because comparing and contrasting the findings of other studies helps to support the overall importance of your results and it highlights how and in what ways your study differs from other research about the topic. Note that any significant or unanticipated finding is often because there was no prior research to indicate the finding could occur. If there is prior research to indicate this, you need to explain why it was significant or unanticipated. IV. Consider Alternative Explanations of the Findings
It is important to remember that the purpose of research in the social sciences is to discover and not to prove . When writing the discussion section, you should carefully consider all possible explanations for the study results, rather than just those that fit your hypothesis or prior assumptions and biases. This is especially important when describing the discovery of significant or unanticipated findings.
V. Acknowledge the Study’s Limitations
It is far better for you to identify and acknowledge your study’s limitations than to have them pointed out by your professor! Note any unanswered questions or issues your study did not address and describe the generalizability of your results to other situations. If a limitation is applicable to the method chosen to gather information, then describe in detail the problems you encountered and why. VI. Make Suggestions for Further Research
You may choose to conclude the discussion section by making suggestions for further research [this can be done in the overall conclusion of your paper]. Although your study may offer important insights about the research problem, this is where you can address other questions related to the problem that remain unanswered or highlight previously hidden questions that were revealed as a result of conducting your research. You should frame your suggestions by linking the need for further research to the limitations of your study [e.g., in future studies, the survey instrument should include more questions that ask..."] or linking to critical issues revealed from the data that were not considered initially in your research.
NOTE: Besides the literature review section, the preponderance of references to sources is usually found in the discussion section . A few historical references may be helpful for perspective, but most of the references should be relatively recent and included to aid in the interpretation of your results or used to link to similar studies. If a study that you cited does not support your findings, don't ignore it--clearly explain why your research findings differ from theirs.
V. Problems to Avoid
- Do not waste time restating your results . Should you need to remind the reader of a finding to be discussed, use "bridge sentences" that relate the result to the interpretation. An example would be: “In the case of determining available housing to single women with children in rural areas of Texas, the findings suggest that access to good schools is important," then move on to further explaining this finding and its implications.
- Recommendations for further research can be included in either the discussion or conclusion of your paper, but do not repeat your recommendations in the both sections. Think about the overall narrative flow of your paper to determine where best to locate this information. However, if your findings raise a lot of new questions or issues, consider including suggestions for further research in the discussion section.
- Do not introduce new results in the discussion section. Be wary of mistaking the reiteration of a specific finding for an interpretation because it may confuse the reader. The description of findings [results] and the interpretation of their significance [discussion] should be distinct sections of your paper. If you choose to combine the results section and the discussion section into a single narrative, you must be clear in how you report the information discovered and your own interpretation of each finding. This approach is not recommended if you lack experience writing college-level research papers.
- Use of the first person pronoun is generally acceptable. Using first person singular pronouns can help emphasize a point or illustrate a contrasting finding. However, keep in mind that too much use of the first person can actually distract the reader from the main points [i.e., I know you're telling me this; just tell me!].
Analyzing vs. Summarizing . Department of English Writing Guide. George Mason University; Discussion . The Structure, Format, Content, and Style of a Journal-Style Scientific Paper. Department of Biology. Bates College; Hess, Dean R. "How to Write an Effective Discussion." Respiratory Care 49 (October 2004); Kretchmer, Paul. Fourteen Steps to Writing to Writing an Effective Discussion Section . San Francisco Edit, 2003-2008; The Lab Report . University College Writing Centre. University of Toronto; Sauaia, A. et al. "The Anatomy of an Article: The Discussion Section: "How Does the Article I Read Today Change What I Will Recommend to my Patients Tomorrow?” The Journal of Trauma and Acute Care Surgery 74 (June 2013): 1599-1602; Research Limitations & Future Research . Lund Research Ltd., 2012; Summary: Using it Wisely . The Writing Center. University of North Carolina; Schafer, Mickey S. Writing the Discussion . Writing in Psychology course syllabus. University of Florida; Yellin, Linda L. A Sociology Writer's Guide . Boston, MA: Allyn and Bacon, 2009.
- << Previous: The Results
- Next: The Conclusion >>
- Last Updated: Oct 1, 2019 1:26 PM
- URL: https://midlakes.libguides.com/c.php?g=972151
Thank you for visiting nature.com. You are using a browser version with limited support for CSS. To obtain the best experience, we recommend you use a more up to date browser (or turn off compatibility mode in Internet Explorer). In the meantime, to ensure continued support, we are displaying the site without styles and JavaScript.
- View all journals
- Explore content
- About the journal
- Publish with us
- Sign up for alerts
- Open access
- Published: 05 January 2024
Enhancing geometric representations for molecules with equivariant vector-scalar interactive message passing
- Yusong Wang 1 , 2 na1 ,
- Tong Wang ORCID: orcid.org/0000-0002-9483-0050 1 na1 ,
- Shaoning Li 1 na1 ,
- Xinheng He 1 , 3 , 4 ,
- Mingyu Li 1 , 5 ,
- Zun Wang ORCID: orcid.org/0000-0002-8763-8327 1 ,
- Nanning Zheng 2 ,
- Bin Shao ORCID: orcid.org/0000-0002-9790-5687 1 &
- Tie-Yan Liu ORCID: orcid.org/0000-0002-0476-8020 1
Nature Communications volume 15 , Article number: 313 ( 2024 ) Cite this article
5856 Accesses
2 Citations
4 Altmetric
Metrics details
- Chemical biology
- Computational biology and bioinformatics
- Computational models
- Molecular modelling
- Protein structure predictions
Geometric deep learning has been revolutionizing the molecular modeling field. Despite the state-of-the-art neural network models are approaching ab initio accuracy for molecular property prediction, their applications, such as drug discovery and molecular dynamics (MD) simulation, have been hindered by insufficient utilization of geometric information and high computational costs. Here we propose an equivariant geometry-enhanced graph neural network called ViSNet, which elegantly extracts geometric features and efficiently models molecular structures with low computational costs. Our proposed ViSNet outperforms state-of-the-art approaches on multiple MD benchmarks, including MD17, revised MD17 and MD22, and achieves excellent chemical property prediction on QM9 and Molecule3D datasets. Furthermore, through a series of simulations and case studies, ViSNet can efficiently explore the conformational space and provide reasonable interpretability to map geometric representations to molecular structures.
Similar content being viewed by others
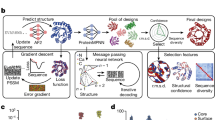
Computational design of soluble and functional membrane protein analogues
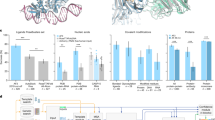
Accurate structure prediction of biomolecular interactions with AlphaFold 3
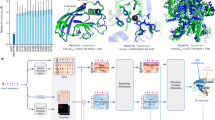
Highly accurate protein structure prediction with AlphaFold
Introduction.
Molecular modeling plays a crucial role in modern scientific and engineering fields, aiding in the understanding of chemical reactions, facilitating new drug development, and driving scientific and technological advancements 1 , 2 , 3 , 4 . One commonly used method in molecular modeling is density functional theory (DFT). DFT enables accurate calculations of energy, forces, and other chemical properties of molecules 5 , 6 . However, due to the large computational requirements, DFT calculations often demand significant computational resources and time, particularly for large molecular systems or high-precision calculations. Machine learning (ML) offers an alternative solution by learning from reference data with ab initio accuracy and high computational efficiency 7 , 8 . Gradient-domain machine learning (GDML) 9 constructs accurate molecular force fields using conservation of energy and limited samples from ab initio molecular dynamics trajectories, enabling cost-effective simulations while maintaining accuracy. Symmetric GDML (sGDML) 10 further improves force field construction by incorporating physical symmetries, achieving CCSD(T)-level accuracy for flexible molecules. An exact iterative approach (Global sGDML) 11 extends sGDML to global force fields for molecules with several hundred atoms, maintaining correlations of atomic degree and accurately describing complex molecules and materials. In recent years, deep learning (DL) has demonstrated its powerful ability to learn from raw data without any hand-crafted features in many fields and thus attracted more and more attention. However, the inherent drawback of deep learning, which requires large amounts of data, has become a bottleneck for its application to more scenarios 12 . To alleviate the dependency on data for DL potentials, recent works have incorporated the inductive bias of symmetry into neural network design, known as geometric deep learning (GDL). Symmetry describes the conservation of physical laws, i.e., the unchanged physical properties with any transformations such as translations or rotations. It allows GDL to be extended to limited data scenarios without any data augmentation.
Equivariant graph neural network (EGNN) is one of the representative approaches in GDL, which has extensive capability to model molecular geometry 12 , 13 , 14 , 15 , 16 , 17 , 18 , 19 , 20 , 21 . A popular kind of EGNN conducts equivariance from directional information and involves geometric features to predict molecular properties. GemNet 20 extends the invariant DimeNet/DimeNet++ 16 , 17 with dihedral information. They explicitly extract geometric information in the Euclidean space with first-order geometric tensor, i.e., setting l max = 1. PaiNN 18 and equivariant transformer 19 further adopt vector embedding and scalarize the angular representation implicitly via the inner product of the vector embedding itself. They reduce the complexity of explicit geometry extraction by taking the angular information into consideration. Another mainstream approach to achieving equivariance is through group representation theory, which can achieve higher accuracy but comes with large computational costs. NequIP, Allegro, and MACE 12 , 22 , 23 achieve state-of-the-art performance on several molecular dynamics simulation datasets leveraging high-order geometric tensors. On the one hand, algorithms based on group representation theory have strong mathematical foundations and are able to fully utilize geometric information using high-order geometric tensors. On the other hand, these algorithms often require computationally expensive operations such as the Clebsch–Gordan product (CG-product) 24 , making them possibly suitable for periodic systems with elaborate model design but impractical for large molecular systems such as chemical and biological molecules without periodic boundary conditions.
In this study, we propose ViSNet (short for “Vector-Scalar interactive graph neural Network"), which alleviates the dilemma between computational costs and sufficient utilization of geometric information. By incorporating an elaborate runtime geometry calculation (RGC) strategy, ViSNet implicitly extracts various geometric features, i.e., angles, dihedral torsion angles, and improper angles in accordance with the force field of classical MD with linear time complexity, thus significantly accelerating model training and inference while reducing the memory consumption. To extend the vector representation, we introduce spherical harmonics and simplify the computationally expensive Clebsch–Gordan product with the inner product. Furthermore, we present a well-designed vector–scalar interactive equivariant message passing (ViS-MP) mechanism, which fully utilizes the geometric features by interacting vector hidden representations with scalar ones. When comprehensively evaluated on some benchmark datasets, ViSNet outperforms all state-of-the-art algorithms on all molecules in MD17, revised MD17 and MD22 datasets and shows superior performance on QM9, Molecule3D dataset indicating the powerful capability of molecular geometric representation. ViSNet also has won the PCQM4Mv2 track in the OGB-LCS@NeurIPS2022 competition ( https://ogb.stanford.edu/neurips2022/results/ ). We then performed molecular dynamics simulations for each molecule on MD17 driven by ViSNet trained only with limited data (950 samples). The highly consistent interatomic distance distributions and the explored potential energy surfaces between ViSNet and quantum simulation illustrate that ViSNet is genuinely data-efficient and can perform simulations with high fidelity. To further explore the usefulness of ViSNet to real-world applications, we used an in-house dataset that consists of about 10,000 different conformations of the 166-atom mini-protein Chignolin derived from replica exchange molecular dynamics and calculated at the DFT level. When evaluated on the dataset, ViSNet also achieved significantly better performance than empirical force fields, and the simulations performed by ViSNet exhibited very close force calculation to DFT. In addition, ViSNet exhibits reasonable interpretability to map geometric representation to molecular structures. The contributions of ViSNet can be summarized as follows:
Proposing an RGC module that utilizes high-order geometric tensors to implicitly extract various geometric features, including angles, dihedral torsion angles, and improper angles, with linear time complexity.
Introducing ViS-MP mechanism to enable efficient interaction between vector hidden representations and scalar ones and fully exploit the geometric information.
Achieving state-of-the-art performance in six benchmarks for predicting energy, forces, HOMO-LUMO gap, and other quantum properties of molecules.
Performing molecular dynamics simulations driven by ViSNet on both small molecules and 166-atom Chignolin with high fidelity.
Demonstrating reasonable model interpretability between geometric features and molecular structures.
Overview of ViSNet
ViSNet is a versatile EGNN that predicts potential energy, atomic forces as well as various quantum chemical properties by taking atomic coordinates and numbers as inputs. As shown in Fig. 1 a, the model is composed of an embedding block and multiple stacked ViSNet blocks, followed by an output block. The atomic number and coordinates are fed into the embedding block followed by ViSNet blocks to extract and encode geometric representations. The geometric representations are then used to predict molecular properties through the output block. It is worth noting that ViSNet is an energy-conserving potential, i.e., the predicted atomic forces are derived from the negative gradients of the potential energy with respect to the coordinates 9 , 10 .
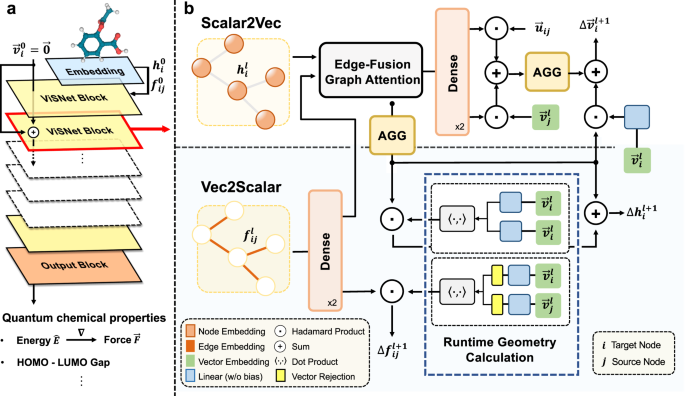
a Model sketch of ViSNet. ViSNet embeds the 3D structures of molecules and extracts the geometric information through a series of ViSNet blocks and outputs the molecule properties such as energy, forces, and HOMO–LUMO gap through an output block. b Flowchart of one ViSNet Block. One ViSNet block consists of two modules: (i) Scalar2Vec , responsible for attaching scalar embeddings to vectors.; (ii) Vec2Scalar , renovates scalar embeddings built on RGC strategy. The inputs of Scalar2Vec are the node embedding h i , edge embedding f i j , direction unit \({\overrightarrow{v}}_{i}\) and the relative positions between two atoms. The edge-fusion graph attention module (serves as \({\phi }_{{\rm {m}}}^{{\rm {s}}}\) ) takes as input h i and the output of the dense layer following f i j , and outputs scalar messages. Before aggregation, each scalar message is transformed through a dense layer, and then fused with the unit of the relative position \({\overrightarrow{u}}_{ij}\) and its own direction unit \({\overrightarrow{v}}_{j}\) . We further compute the vector messages and aggregate them all among the neighborhood. Through a gated residual connection, the final residual \({{\Delta }}{\overrightarrow{v}}_{i}\) is produced. In Vec2Scalar module, by Hadamard production of aggregated scalar messages and the output of RGC-Angle calculation and adding a gated residual connection, the final Δ h i is figured out. Likewise, combining the projected f i j and the output of RGC-Dihedral calculation, the final Δ f i j is determined.
The success of classical force fields shows that geometric features such as interatomic distances, angles, dihedral torsion angles, and improper angles in Fig. 2 are essential to determine the total potential energy of molecules. The explicit extraction of invariant geometric representations in previous studies often suffers from a large amount of time or memory consumption during model training and inference. Given an atom, the calculation of angular information scales \({{{{{{{\mathcal{O}}}}}}}}({{{{{{{{\mathcal{N}}}}}}}}}^{2})\) with the number of neighboring atoms, while the computational complexity is even \({{{{{{{\mathcal{O}}}}}}}}({{{{{{{{\mathcal{N}}}}}}}}}^{3})\) for dihedrals 20 . To alleviate this problem, inspired by Sch¨utt et al. 18 , we propose runtime geometry calculation (RGC), which uses an equivariant vector representation (termed as direction unit) for each node to preserve its geometric information. RGC directly calculates the geometric information from the direction unit which only sums the vectors from the target node to its neighbors once. Therefore, the computational complexity can be reduced to \({{{{{{{\mathcal{O}}}}}}}}({{{{{{{\mathcal{N}}}}}}}})\) . Notably, beyond employing angular information that has been used in PaiNN 18 and ET 19 , ViSNet further considers the dihedral torsion and improper angle calculation with higher geometric tensors.
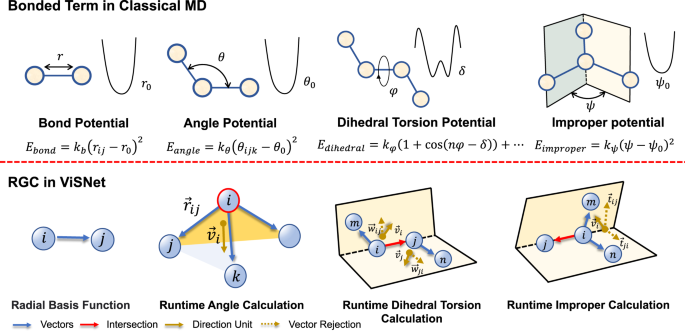
The bonded terms consist of bond length, bond angle, dihedral torsion, and improper angle. The RGC module depicts all bonded terms of classical MD as model operations in linear time complexity. Yellow arrow \({\overrightarrow{v}}_{i}\) denotes the direction unit in Eq. ( 1 ).
Considering the sub-structure of a toy molecule with four atoms shown in Fig. 2 , the angular information of the target node i could be conducted from the vector \({\overrightarrow{r}}_{ij}\) as follows:
where \({\overrightarrow{r}}_{ij}\) is the vector from node i to its neighboring node j , \({\overrightarrow{u}}_{ij}\) is the unit vector of \({\overrightarrow{r}}_{ij}\) . Here, we define the direction unit \({\overrightarrow{v}}_{i}\) as the sum of all unit vectors from node i to its all neighboring nodes j , where node i is the intersection of all unit vectors. As shown in Eq. ( 2 ), we calculate the inner product of the direction unit \({\overrightarrow{v}}_{i}\) which represents the sum of the inner products of unit vectors from node i to all its neighboring nodes. Combining with Eq. ( 1 ), the inner product of direction \({\overrightarrow{v}}_{i}\) finally stands for the sum of cosine values of all angles formed by node i and any two of its neighboring nodes.
Similar to runtime angle calculation, we also calculate the vector rejection 25 of the direction unit \({\overrightarrow{v}}_{i}\) of node i and \({\overrightarrow{v}}_{j}\) of node j on the vector \({\overrightarrow{u}}_{ij}\) and \({\overrightarrow{u}}_{ji}\) , respectively.
where \({{{{{{{{\rm{Rej}}}}}}}}}_{\overrightarrow{b}}(\overrightarrow{a})\) represents the vector component of \(\overrightarrow{a}\) perpendicular to \(\overrightarrow{b}\) , termed as the vector rejection. \({\overrightarrow{u}}_{ij}\) and \({\overrightarrow{v}}_{i}\) are defined in Eq. ( 1 ). \({\overrightarrow{w}}_{ij}\) represents the sum of the vector rejection \({{{{{{{{\rm{Rej}}}}}}}}}_{{\overrightarrow{u}}_{ij}}({\overrightarrow{u}}_{im})\) and \({\overrightarrow{w}}_{ji}\) represents the sum of the vector rejection \({{{{{{{{\rm{Rej}}}}}}}}}_{{\overrightarrow{u}}_{ji}}({\overrightarrow{u}}_{jn})\) . The inner product between \({\overrightarrow{w}}_{ij}\) and \({\overrightarrow{w}}_{ji}\) is then calculated to conduct dihedral torsion angle information of the intersecting edge e i j as follows:
The improper angle is derived from a pyramid structure forming by 4 nodes. As the last toy molecule shown in Fig. 2 , node i is the vertex of the pyramid, and the improper torsion angle is formed by two adjacent planes with an intersecting edge e i j . We can also calculate the improper angle by vector rejection:
In the same way, the inner product between \({\overrightarrow{t}}_{ij}\) and \({\overrightarrow{t}}_{ji}\) indicates the summation of improper angle information formed by e i j :
Multiple works have shown the effectiveness of high-order geometric tensors for molecular modeling 12 , 22 , 26 , 27 . However, the computational overheads of these approaches are generally expansive due to the CG-product, impeding their further application for large systems. In this work, we convert the vectors to high-order representation with spherical harmonics but discard CG-product with the inner product following the idea of RGC. We find that the extended high-order geometric tensors can still represent the above angular information in the form of Legendre polynomials according to the addition theorem:
where the P l is the Legendre polynomial of degree l , Y l , m denotes the spherical harmonics function and \({Y}_{l,m}^{*}\) denotes its complex conjugation. We sum the product of different order l to obtain the scalar angular representation, which is the same operation as the inner product. It is worth noting that such an extension does not increase the model size and keeps the model architecture unchanged. We also provide proof about the rotational invariance of the RGC strategy in the section “Proofs of the rotational invariance of RGC ”.
In order to make full use of geometric information and enhance the interaction between scalars and vectors, we designed an effective vector–scalar interactive message-passing mechanism with respect to the intersecting nodes and edges for angles and dihedrals, respectively. It is important to note that previous studies 18 , 19 primarily focused on updating node features, whereas our approach updates both node and edge features during message passing, leading to a more comprehensive geometric representation. The key operations in ViS-MP are given as follows:
where h i denotes the scalar embedding of node i , f i j stands for the edge feature between node i and node j . \({\overrightarrow{v}}_{i}\) represents the embedding of the direction unit mentioned in RGC. The superscript of variables indicates the index of the block that the variables belong to. We omit the improper angle here for brevity. A comprehensive version is depicted in Supplementary. ViS-MP extends the conventional message passing, aggregation, and update processes with vector–scalar interactions. Eqs. ( 8 ) and ( 9 ) depict our message-passing and aggregation processes. To be concrete, scalar messages m i j incorporating scalar embedding h j , h i , and f i j are passed and then aggregated to node i through a message function \({\phi }_{m}^{s}\) (Eq. ( 8 )). Similar operations are applied for vector messages \({\overrightarrow{m}}_{i}^{l}\) of node i that incorporates scalar message m i j , vector \({\overrightarrow{r}}_{ij}\) and vector embedding \({\overrightarrow{v}}_{j}\) (Eq. ( 9 )). Equations ( 10 ) and ( 11 ) demonstrate the update processes. h i is updated by the aggregated scalar message output m i while the inner product of \({\overrightarrow{v}}_{i}\) is updated through an update function \({\phi }_{un}^{s}\) . Then \({\overrightarrow{f}}_{ij}\) is updated by the inner product of the rejection of the vector embedding \({\overrightarrow{v}}_{i}\) and \({\overrightarrow{v}}_{j}\) through an update function \({\phi }_{ue}^{s}\) . Finally, the vector embedding \({\overrightarrow{v}}_{i}\) is updated by both scalar and vector messages through an update function \({\phi }_{un}^{v}\) . Notably, the vectors update function, i.e., ϕ v require to be equivariant. The detailed message and update functions can be found in the Methods section. A proof about the equivariance of ViS-MP can be found in Supplementary Methods.
In summary, the geometric features are extracted by inner products in the RGC strategy and the scalar and vector embeddings are cyclically updating each other in ViS-MP so as to learn a comprehensive geometric representation from molecular structures.
Accurate quantum chemical property predictions
We evaluated ViSNet on several prevailing benchmark datasets including MD17 9 , 10 , 28 , revised MD17 29 , MD22 30 , QM9 31 , Molecule3D 32 , and OGB-LSC PCQM4Mv2 33 for energy, force, and other molecular property prediction. MD17 consists of the MD trajectories of seven small organic molecules; the number of conformations in each molecule dataset ranges from 133,700 to 993,237. The dataset rMD17 is a reproduced version of MD17 with higher accuracy. MD22 is a recently proposed MD trajectories dataset that presents challenges with respect to larger system sizes (42–370 atoms). Large molecules such as proteins, lipids, carbohydrates, nucleic acids, and supramolecules are included in MD22. QM9 consists of 12 kinds of quantum chemical properties of 133,385 small organic molecules with up to 9 heavy atoms. Molecule3D is a recently proposed dataset including 3,899,647 molecules collected from PubChemQC with their ground-state structures and corresponding properties calculated by DFT. We focus on the prediction of the HOMO–LUMO gap following ComENet 34 . OGB-LSC PCQM4Mv2 is a quantum chemistry dataset originally curated under the PubChemQC including a DFT-calculated HOMO–LUMO gap of 3,746,619 molecules. The 3D conformations are provided for 3,378,606 training molecules but not for the validation and test sets. The training details of ViSNet on each benchmark are described in the “Methods” section.
We compared ViSNet with the state-of-the-art algorithms, including DimeNet 16 , PaiNN 18 , SpookyNet 21 , ET 19 , GemNet 20 , UNiTE 35 , NequIP 12 , SO3KRATES 36 , Allegro 22 , MACE 23 and so on. As shown in Table 1 (MD17), Table 2 (rMD17), and Table 3 (MD22), it is remarkable that ViSNet outperformed the compared algorithms for both small (MD17 and rMD17) and large molecules (MD22) with the lowest mean absolute errors (MAE) of predicted energy and forces. On the one hand, compared with PaiNN, ET, and GemNet, ViSNet incorporated more geometric information and made full use of geometric information in ViS-MP, which contributes to the performance gains. On the other hand, compared with NequIP, Allegro, SO3KRATES, MACE, etc., ViSNet testified the effect of introducing spherical harmonics in the RGC module.
As shown in Table 4 , ViSNet also achieved superior performance for chemical property predictions on QM9. It outperformed the compared algorithms for 9 of 12 chemical properties and achieved comparable results on the remaining properties. Elaborated evaluations on Molecule3D confirmed the high prediction accuracy of ViSNet as shown in Table 5 . ViSNet achieved 33.6% and 6.51% improvements than the second-best for random split and scaffold split, respectively. Furthermore, ViSNet exhibited good portability to other multimodality methods, e.g., Transformer-M 37 and outperformed other approaches on OGB-LSC PCQM4Mv2 (see Supplementary Fig. S1) . ViSNet also achieved the winners of PCQM4Mv2 track in the OGB-LCS@NeurIPS2022 competition when testing on unseen molecules 38 ( https://ogb.stanford.edu/neurips2022/results/ ).
To evaluate the computational efficiency of our ViSNet, following 23 , we compare the time latency of ViSNet with prevailing models in Supplementary Fig. S2 . The latency is defined as the time it takes to compute forces on a structure (i.e., the gradient calculation for a set of input coordinates through the whole deep neural network). As shown in Supplementary Fig. S2 , ViSNet ( L = 2) saved 42.8% time latency compared with MACE ( L = 2). Notably, despite the use of CG-product, Allegro had a significant speed improvement compared to NequIP and BOTNet. However, ViSNet still saved 6.1%, 4.1%, and 61% time latency compared to Allegro with L = 1, 2, and 3, respectively.
Efficient molecular dynamics simulations
To evaluate ViSNet as the potential for MD simulations, we incorporated ViSNet that trained only with 950 samples on MD17 into the ASE simulation framework 39 to perform MD simulations for all seven kinds of organic molecules. All simulations are run with a time step τ = 0.5 fs under the Berendsen thermostat with the other settings the same as those of the MD17 dataset. As shown in Fig. 3 , we analyzed the interatomic distance distributions derived from both AIMD simulations with ViSNet as the potential and ab initio molecular dynamics simulations at the DFT level for all seven molecules, respectively. As shown in Fig. 3 a, the interatomic distance distribution h ( r ) is defined as the ensemble average of atomic density at a radius r 9 . Figure 3 b–h illustrates the distributions derived from ViSNet are very close to those generated by DFT. We also compared the potential energy surfaces sampled by ViSNet and DFT for these molecules, respectively (Supplementary Fig. S3 ). The consistent potential energy surfaces suggest that ViSNet can recover the conformational space from the simulation trajectories. Moreover, compared to DFT, numerous groundbreaking machine learning force fields (MLFFs), including sGDML 10 , ANI 40 , DPMD 41 , and PhysNet 42 have proven their exceptional speeds in MD simulations. Similar to such algorithms, ViSNet also exhibited significant computational cost reduction compared to DFT as shown in Supplementary Fig. S4 and Table S2 .
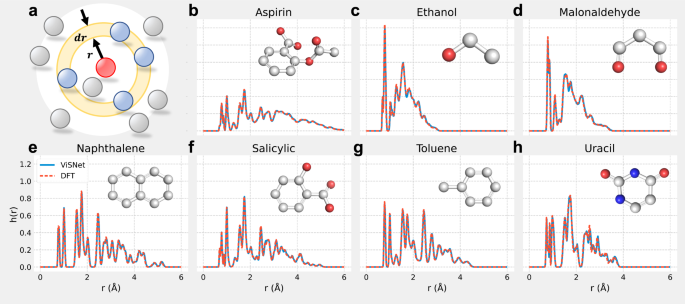
a An illustration about the atomic density at a radius r with the arbitrary atom as the center. The interatomic distance distribution is defined as the ensemble average of atomic density. b – h The interatomic distance distributions comparison between simulations by ViSNet and DFT for all seven organic molecules in MD17. The curve of ViSNet is shown using a solid blue line, while the dashed orange line is used for the DFT curve. The structures of the corresponding molecules are shown in the upper right corner. Source data are provided as a Source Data file.
To further examine the molecular properties derived from simulations driven by ViSNet, we performed 500 ps MD simulations at a constant energy ensemble (NVE) for ethanol in the MD17 dataset with a time step of τ = 0.5 fs and 200 ps Ac-Ala3-NHMe in the MD22 dataset with a time step of τ = 1 fs. The simulations were driven by ViSNet, sGDML, and DFT, respectively. For ethanol, we analyzed its vibrational spectra and the probability distribution of dihedral angles. For Ac-Ala3-NHMe, we investigated its vibrational spectra and potential energy surface (PES) via the Ramachandran plot. To analyze the Ramachandran plot of different simulations, the free energy value was estimated using the potential of mean force (PMF). ϕ and ψ were set as two reaction coordinates ( x , y ). All three ϕ and ψ dihedrals in Ac-Ala3-NHMe were calculated and plotted. The relative free energy value was calculated and referred to with the minimum value. To generate the landscape, 40 bins were used in both the x and y directions. Supplementary Fig. S5 a and b demonstrate that both ViSNet and sGDML generate similar vibrational spectra, with slight differences in peak intensities compared to DFT. The probability distribution of hydroxyl angles in ethanol (Supplementary Fig. S5 c) reveals three minima: gauche ± ( M g ± ) and trans ( M t ). Furthermore, even though ViSNet showed better performance than sGDML for various conformations in the MD22 dataset, starting from the same structure of the alanine tetrapeptide, the performance difference may not have a notable impact on the sampling efficiency for such small molecules, and thus may also lead to similar dynamics on the Ramachandran plots as shown in the Supplementary Fig. S5 d–f. These results demonstrate that with only a few training samples, ViSNet can act with the potential to perform high-fidelity molecular dynamics simulations with much less computational cost and higher accuracy.
Applications for real-world full-atom proteins
To examine the usefulness of ViSNet in real-world applications, we made evaluations on the 166-atom mini-protein Chignolin (Fig. 4 a). Based on a Chignolin dataset consisting of about 10,000 conformations that sampled by replica exchange MD 43 and calculated at DFT level by Gaussian 16 44 in our another study 45 , 46 , we split it as training, validation, and test sets by the ratio of 8:1:1. We trained ViSNet as well as other prevailing MLFFs including ET 19 , PaiNN 18 , GemNet-OC 47 , MACE 23 , NequIP 12 and Allegro 22 and compared them with molecular mechanics (MM) 48 . The DFT results were used as the ground truth. Figure 4 b shows the free energy landscape of Chignolin and is depicted by d D3−G7 (the distance between carbonyl oxygen on the D3 backbone and nitrogen on the G7 backbone) and d E5−T8 (the distance between carbonyl oxygen on the E5 backbone and nitrogen on T8 backbone). The concentrated energy basin on the left shows the folded state and the scattered energy basin on the right shows the unfolded state. We randomly selected six structures from different regions of the potential energy surface for visualization. Among them, four structures were predicted by the model with smaller errors than the MAE while the other two with larger errors. Interestingly, all models consistently performed poorly on the structures with high potential energies (low probability of sampling) and performed well on the other structures. This implies that the sampling of conformations with high potential energies could be enhanced to ensure the generalization ability of the models.
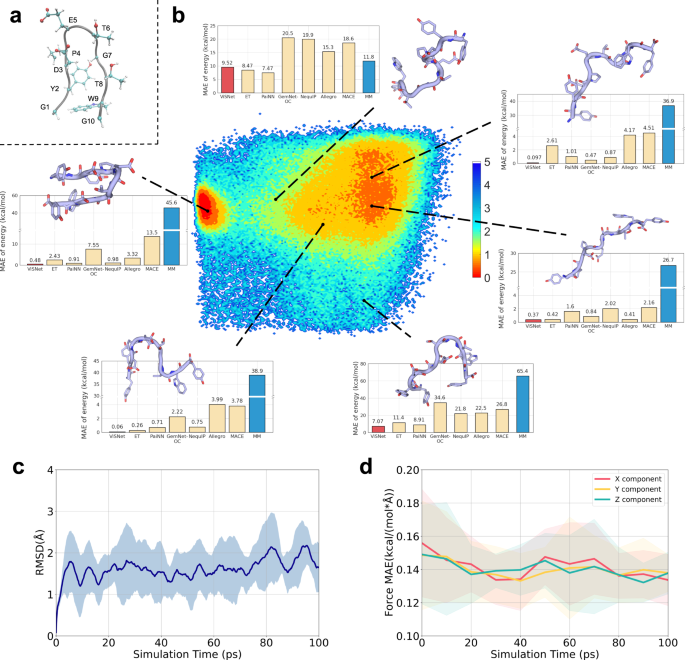
a The visualization of Chignolin structure. The backbone is colored grey while the side chains of each residue in Chignolin are highlighted with a ball and stick. b The energy landscape of Chignolin sampled by REMD. The x -axis of the landscape is the distance between carbonyl oxygen on the D3 backbone and nitrogen on the G7 backbone, while the y -axis is the distance between carbonyl oxygen on the E5 backbone and nitrogen on the T8 backbone. Six structures were then selected for visualization. Each structure is shown as a cartoon and residues are depicted in sticks. The histograms show the absolute error between the energy difference predicted by MLFFs including ViSNet, ET, PaiNN, GemNet-OC, NequIP, Allegro, and MACE or calculated by MM, and the ground truth calculated by DFT on the corresponding structure. c The average root mean square deviation (RMSD) of the Chignolin trajectories simulated by ViSNet was calculated from 10 different trajectories. The shaded areas indicate the standard deviation range. d The MAE of each component of atomic forces during the simulations driven by ViSNet. The ground truth energies and forces were calculated using Gaussian 16. The shaded areas indicate the standard deviation range. Source data are provided as a Source Data file.
Supplementary Fig. S6 shows the correlations between the energies predicted by MLFFs or MM and the ground truth values calculated by DFT for all conformations in the test set. ViSNet achieved a lower MAE and a higher R 2 score. From the violin plot of the absolute errors shown in Supplementary Fig. S7 , ViSNet, PaiNN and ET exhibited smaller errors than other MLFFs while MM got a much wider range of prediction errors. Similar results can be seen in the force correlations in each component shown in Supplementary Fig. S8 . Detailed settings about DFT and MM calculations are shown in Supplementary Materials. Furthermore, we also made a comprehensive comparison by taking model performance, training time consumption, and model size into consideration. ViSNet and other state-of-the-art algorithms such as PaiNN, ET, GemNet-OC, MACE, NequIP, and Allegro were analyzed on the Chignolin dataset and shown in Fig. 5 . Although ViSNet is marginally slower than ET and PaiNN, it introduces more geometric information, significantly enhancing its performance. When compared to GemNet, which also incorporates dihedral angles, ViSNet’s computational cost is significantly more affordable. Similarly, ViSNet proves to be computationally efficient when compared to models employing the CG-product method, such as MACE, Allegro, and NequIP.
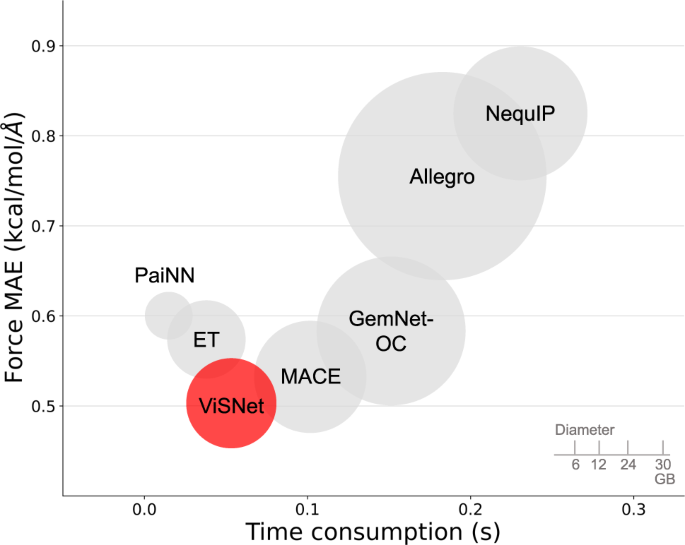
PaiNN and ET are faster and smaller as ViSNet further incorporates dihedral calculation. ViSNet outperforms GemNet-OC due to its Runtime Geometry Calculation, reducing the explicit extraction of dihedral complexity from \({{{{{{{\mathcal{O}}}}}}}}({{{{{{{{\mathcal{N}}}}}}}}}^{3})\) to \({{{{{{{\mathcal{O}}}}}}}}({{{{{{{\mathcal{N}}}}}}}})\) . Additionally, ViSNet is also faster and smaller than MACE, Allegro, and NequIP for streamlining the CG-product. ViSNet achieves the best performance for its elaborate design, i.e., runtime geometric calculation and vector–scalar interactive message passing. Source data are provided as a Source Data file.
In addition, we performed MD simulations for Chignolin driven by ViSNet. 10 conformations were randomly selected as initial structures, and 100 ps simulations were run for each. As shown in Fig. 4 c, the RMSD for 10 simulation trajectories is shown against the simulation time. In Fig. 4 d, we displayed the MAE values of each component of the atomic forces between ViSNet and those calculated by Gaussian 16 44 at the DFT level. The simulation trajectory driven by ViSNet exhibited a small force difference for each component to quantum mechanics, which implies that ViSNet has no bias towards any force component, and thus consolidates the accuracy and potential usefulness for real-world applications.
Interpretability of ViSNet on molecular structures
Prior works have shown the effectiveness of incorporating geometric features, such as angles 16 , 20 . The primary method of geometry extraction utilized by ViSNet is the distinct inner product in its runtime geometry calculation. To this end, we illustrate a reasonable model interpretability of ViSNet by mapping the angle representations derived from the inner product of direction units in the model to the atoms in the molecular structure. We aim to bridge the gap between geometric representation in ViSNet and molecular structures. We visualized the embeddings after the inner product of direction units \(\langle {\overrightarrow{v}}_{i},{\overrightarrow{v}}_{i}\rangle\) extracted from 50 aspirin samples on the validation set. The high-dimensional embeddings were reduced to 2-dimensional space using T-SNE 49 and then clustered using DBSCAN 50 without the prior of number of clusters.
Supplementary Fig. S9 exhibits the clustering results of nodes’ embeddings after the inner product of their corresponding direction units. We further map the clustered nodes to the atoms of aspirin chemical structure. Interestingly, the embeddings for these nodes could be distinctly gathered into several clusters shown in different colors. For example, although carbon atom C 11 and carbon atom C 12 possess different positions and connect with different atoms, their inner product \(\langle {\overrightarrow{v}}_{i},{\overrightarrow{v}}_{i}\rangle\) are clustered into the same class for holding similar substructures ({ C 11 − O 2 O 3 C 6 } and { C 12 − O 1 O 4 C 13 }). To summarize, ViSNet can discriminate different molecular substructures in the embedding space.
Ablation study
To further explore where the performance gains of ViSNet come from, we conducted a comprehensive ablation study. Specifically, we excluded the runtime angle calculation (w/o A), runtime dihedral calculation (w/o D), and both of them (w/o A&D) in ViSNet, in order to evaluate the usefulness of each part. ViSNet-improper denotes the additional improper angles and ViSNet l =1 uses the first-order spherical harmonics.
We designed some model variants with different message-passing mechanisms based on ViS-MP for scalar and vector interaction. ViSNet-N directly aggregates the dihedral information to intersecting nodes, and ViSNet-T leverages another form of dihedral calculation. The details of these model variants are elaborated in Supplementary. The results of the ablation study are shown in Supplementary Table S3 and Supplementary Fig. S10 . Based on the results, we can see that both kinds of directional geometric information are useful and the dihedral information contributes a little bit more to the final performance. The significant performance drop from ViSNet-N and ViSNet-T further validates the effectiveness of the ViS-MP mechanism. ViSNet-improper achieves similar performance to ViSNet for small molecules, but the contribution of improper angles is more obvious for large molecules (see Table 3 ). Furthermore, ViSNet using higher-order spherical harmonics achieves better performance.
We propose ViSNet, a geometric deep learning potential for molecular dynamics simulation. The group representation theory-based methods and the directional information-based methods are two mainstream classes of geometric deep learning potentials to enforce SE(3) equivariance 20 . ViSNet takes advantage of both sides in designing the RGC strategy and ViS-MP mechanism. On the one hand, the RGC strategy explicitly extracts and exploits the directional geometric information with computationally lightweight operations, making the model training and inference fast. On the other hand, ViS-MP employs a series of effective and efficient vector-scalar interactive operations, leading to the full use of geometric information. Furthermore, according to the many-body expansion theory 51 , 52 , 53 , the potential energy of the whole system equals the potential of each single atom plus the energy corrections from two-bodies to many-bodies. Most of the previous studies model the truncated energy correction terms hierarchically with k -hop information via stacking k message passing blocks. Different from these approaches, ViSNet encodes the angle, dihedral torsion, and improper information in a single block, which empowers the model to have a much more powerful representation ability. In addition, ViSNet’s universality or completeness is not validated by the geometric Weisfeiler–Leman (GWL) test 54 due to the inner product operation, which is computationally efficient but fails to distinguish certain atom reflection structures with the same angular information. To pass counterexamples or the GWL test, incorporating the CG-product with higher-order spherical harmonics is necessary in future studies.
Besides predicting energy, force, and chemical properties with high accuracy, performing molecular dynamics simulations with ab initio accuracy at the cost of the empirical force field is a grand challenge. ViSNet proves its usefulness in real-world ab initio molecular dynamics simulations with less computational costs and the ability of scaling to large molecules such as proteins. Extending ViSNet to support larger and more complex molecular systems will be our future research direction.
Equivariance
In the context of machine learning for atomic systems, equivariance is a pervasive concept. Specifically, the atomic vectors such as dipoles or forces must rotate in a manner consistent with the conformation coordinates. In molecular dynamics, such equivariance can be ensured by computing gradients based on a predicted conservative scalar energy. Formally, a function \({{{{{{{\mathcal{F}}}}}}}}:{{{{{{{\mathcal{X}}}}}}}}\to {{{{{{{\mathcal{Y}}}}}}}}\) is equivariant should guarantee:
where \({\rho }_{{{{{{{{\mathcal{X}}}}}}}}}(g)\) and \({\rho }_{{{{{{{{\mathcal{X}}}}}}}}}(g)\) are group representations in input and output spaces. The integration of equivariance into model parameterization has been shown to be effective, as seen in the implementation of shift-equivariance in CNNs, which is critical for enhancing the generalization capacity.
Proofs of the rotational invariance of RGC
Assume that the molecule rotates in 3D space, i.e.,
where, R ∈ S O (3) is an arbitrary rotation matrix that satisfies:
The angular information after rotation is calculated as follows:
As shown in Eq. ( 18 ), the angle information does not change after rotation. The dihedral angular and improper information is also rotationally invariant since:
As Eq. ( 18 ) proved, the inner product has rotational invariance. Then, Eq. ( 19 ) can be further simplified as
The dihedral or improper angular information after rotation is calculated as:
As a result, Eqs. ( 18 ) and ( 21 ) have proved the rotational invariance of our proposed runtime geometry calculation (RGC).
We also provide proof of the equivariance of our ViS-MP in Supplementary Methods.
Detailed operations and modules in ViSNet
ViSNet predicts the molecular properties (e.g., energy \(\hat{E}\) , forces \(\overrightarrow{F}\in {{\mathbb{R}}}^{N\times 3}\) , dipole moment μ ) from the current states of atoms, including the atomic positions \(X\in {{\mathbb{R}}}^{N\times 3}\) and atomic numbers \(Z\in {{\mathbb{N}}}^{N}\) . The architecture of the proposed ViSNet is shown in Fig. 1 . The overall design of ViSNet follows the vector–scalar interactive message passing as illustrated from Eqs. ( 8 )–( 11 ). First, an embedding block encodes the atom numbers and edge distances into the embedding space. Then, a series of ViSNet blocks update the node-wise scalar and vector representations based on their interactions. A residual connection is placed between two ViSNet blocks. Finally, stacked corresponding gated equivariant blocks proposed by 18 are attached to the output block for specific molecular property prediction.
The embedding block
ViSNet expands the direct node and edge embedding with their neighbors. It first embeds atomic chemical symbol z i , and calculates the edge representation whose distances within the cutoff through radial basis functions (RBF). Then the initial embedding of the atom i , its 1-hop neighbors j and the directly connected edge e i j within cutoff are fused together as the initial node embedding \({h}_{i}^{0}\) and edge embedding \({f}_{ij}^{0}\) . In summary, the embedding block is given by:
\({{{{{{{\mathcal{N}}}}}}}}(i)\) denotes the set of 1-hop neighboring nodes of node i , and j is one of its neighbors. The embedding process is elaborated in Supplementary. The initial vector embedding \({\overrightarrow{v}}_{i}\) is set to \(\overrightarrow{0}\) . The vector embeddings \(\overrightarrow{v}\) are projected into the embedding space by following 18 ; \(\overrightarrow{v}\in {{\mathbb{R}}}^{N\times 3\times F}\) and F is the size of hidden dimension. The advantage of such projection is to assign a unique high-dimensional representation for each embedding to discriminate from each other. Further discussions on its effectiveness and interpretability are given in the Results section.
The Scalar2Vec module
In the Scalar2Vec module, the vector embedding \(\overrightarrow{v}\) is updated by both the scalar messages derived from node and edge scalar embeddings (Eq. ( 8 )) and the vector messages with inherent geometric information (Eq. ( 9 )). The message of each atom is calculated through an Edge-Fusion Graph Attention module, which fuses the node and edge embeddings and computes the attention scores. The fusion of the node and edge embeddings could be the concatenation operation, Hadamard product, or adding a learnable bias 55 . We leverage the Hadamard product and the vanilla multi-head attention mechanism borrowed from Transformer 56 for edge-node fusion.
Following 19 , we pass the fused representations through a nonlinear activation function as shown in Eq. ( 23 ). The value ( V ) in the attention mechanism is also fused by edge features before being multiplied by attention scores weighted by a cosine cutoff as shown in Eq. ( 24 ),
where l ∈ {0, 1, 2, ⋯ , L } is the index of the block, σ denotes the activation function (SiLU in this paper), W is the learnable weight matrix, ⊙ represents the Hadamard product, ϕ ( ⋅ ) denotes the cosine cutoff and Dense( ⋅ ) refers to one learnable weight matrix with an activation function. For brevity, we omit the learnable bias for linear transformation on scalar embedding in equations, and there is no bias for vector embedding to ensure the equivariance.
Then, the computed \({m}_{ij}^{l}\) is used to produce the geometric messages \({\overrightarrow{m}}_{ij}^{l}\) for vectors:
And the vector embedding \({\overrightarrow{v}}^{l}\) is updated by:
The Vec2Scalar module
In the Vec2Scalar module, the node embedding \({h}_{i}^{l}\) and edge embedding \({f}_{ij}^{l}\) are updated by the geometric information extracted by the RGC strategy, i.e., angles (Eq. ( 10 )) and dihedrals (Eq. ( 11 )), respectively. The residual node embedding \({{\Delta }}{h}_{i}^{l+1}\) , is calculated by a Hadamard product between the runtime angle information and the aggregated scalar messages with a gated residual connection:
To compute the residual edge embedding \({{\Delta }}{f}_{ij}^{l+1}\) , we perform the Hadamard product of the runtime dihedral information with the transformed edge embedding:
After the residual hidden representations are calculated, we add them to the original input of block l and feed them to the next block.
A comprehensive version that includes improper angles is depicted in Supplementary Methods.
The output block
Following PaiNN 18 , we update the scalar embedding and vector embedding of nodes with multiple gated equivariant blocks:
where [ ⋅ , ⋅ ] is the tensor concatenation operation. The final scalar embedding \({h}_{i}^{L}\in {{\mathbb{R}}}^{N\times 1}\) and vector embedding \({\overrightarrow{v}}_{i}^{L}\in {{\mathbb{R}}}^{N\times 3\times 1}\) are used to predict various molecular properties.
On QM9, the molecular dipole is calculated as follows:
where \({\overrightarrow{r}}_{c}\) denotes the center of mass. Similarly, for the prediction of electronic spatial extent 〈 R 2 〉, we use the following equation:
For the remaining 10 properties y , we simply aggregate the final scalar embedding of nodes as follows:
For models trained on the molecular dynamics datasets including MD17, revised MD17, and Chignolin, the total potential energy is obtained as the sum of the final scalar embedding of the nodes. As an energy-conserving potential, the forces are then calculated using the negative gradients of the predicted total potential energy with respect to the atomic coordinates:
Statistics and reproducibility
For the QM9 dataset, we randomly split it into 110,000 samples as the train set, 10,000 samples as the validation set, and the rest as the test set by following the previous studies 18 , 19 . For the Molecule3D and OGB-LSC PCQM4Mv2 datasets, the splitting has been provided in their paper 32 , 33 .
To evaluate the effectiveness of ViSNet in simulation data, ViSNet was trained on MD17 and rMD17 with a limited data setting, which consists of only 950 uniformly sampled conformations for model training and 50 conformations for validation for each molecule. For the MD22 dataset, we use the same number of molecules as in ref. 30 for training and validation, and the rest as the test set.
Furthermore, the whole Chignolin dataset was randomly split into 80%, 10%, and 10% as the training, validation, and test datasets. Six representative conformations were picked from the test set for illustration.
Experimental settings
For the QM9 dataset, we adopted a batch size of 32 and a learning rate of 1e−4 for all the properties. For the Molecule3D dataset, we adopted a larger batch size of 512 and a learning rate of 2e−4. For the OGB-LSC PCQM4Mv2 dataset, we trained our model in a mixed 2D/3D mode with a batch size of 256 and a learning rate of 2e−4. The mean squared error (MSE) loss was used for model training. For the molecular dynamic dataset including MD17, rMD17, MD22, and Chignolin, we leveraged a combined MSE loss for energy and force prediction. The weight of energy loss was set to 0.05. The weight of force loss was set to 0.95. The batch size was chosen from 2, 4, 8 due to the GPU memory and the learning rate was chosen from 1e−4 to 4e−4 for different molecules. The cutoff was set to 5 for small molecules in QM9, MD17, rMD17, and Molecule3D, and changed to 4 for Chignolin in order to reduce the number of edges in the molecular graphs. For the MD22 dataset, the cutoff of relatively small molecules was set to 5, that of bigger molecules was set to 4. Cutoff was not used in the OGB-LSC PCQM4Mv2 dataset. We used the learning rate decay if the validation loss stopped decreasing. The patience was set to 5 epochs for Molecule3D, 15 epochs for QM9, and 30 epochs for MD17, rMD17, MD22, and Chignolin. The learning rate decay factor was set to 0.8 for these models. Training is stopped if a maximum number of epochs is reached, or the validation loss does not improve for a maximum number of early stopping patience. The ViSNet model trained on the molecular dynamic datasets and Molecule3D had 9 hidden layers and the embedding dimension was set to 256. We used a larger model for the QM9 dataset, i.e., the embedding dimension changed to 512. For the OGB-LSC PCQM4Mv2 dataset, we use the 12-layer and 768-dimension Transformer-M 37 as the backbone. More details about the hyperparameters of ViSNet can be found in Supplementary Table S4 . Experiments were conducted on NVIDIA 32G-V100 GPUs.
Reporting summary
Further information on research design is available in the Nature Portfolio Reporting Summary linked to this article.
Data availability
All relevant data supporting the key findings of this study are available within the article and its Supplementary Information files. MD17 dataset [ http://www.quantum-machine.org/gdml/data/npz ], MD22 dataset [ http://www.quantum-machine.org/gdml/data/npz ], rMD17 dataset [ https://archive.materialscloud.org/record/file?filename=rmd17.tar.bz2&record_id=466 ], QM9 dataset [ https://deepchemdata.s3-us-west-1.amazonaws.com/datasets/molnet_publish/qm9.zip ], Molecule3D dataset [ https://github.com/divelab/MoleculeX/tree/molx/Molecule3D ], OGB-LSC PCQM4Mv2 dataset [ https://ogb.stanford.edu/docs/lsc/pcqm4mv2 ] and Chignolin dataset [ https://github.com/microsoft/AI2BMD/tree/ViSNet/chignolin_data ]. Source data are provided with this paper.
Code availability
Most experiments were run with Python with version 3.9.15, Pytorch with version 1.11.0, Pytorch Geometric with version 2.1.0, and Pytorch Lightning with version 1.8.0. The code used to reproduce our results is available at https://github.com/microsoft/AI2BMD/tree/ViSNet 57 . Matplotlib and Seaborn were used for plotting figures.
Chow, E., Klepeis, J., Rendleman, C., Dror, R. & Shaw, D. 9.6 new technologies for molecular dynamics simulations. In Comprehensive Biophysics (ed Egelman, E.H.) 86–104 (Elsevier, Amsterdam, 2012).
Singh, S. & Singh, V. K. Molecular dynamics simulation: methods and application. In Frontiers in Protein Structure, Function, and Dynamics (eds Singh, D. B. & Tripathi, T.) 213–238 (Springer, 2020).
Lu, S. et al. Activation pathway of a g protein-coupled receptor uncovers conformational intermediates as targets for allosteric drug design. Nat. Commun. 12 , 1–15 (2021).
Article ADS Google Scholar
Li, Y. et al. Exploring the regulatory function of the n-terminal domain of sars-cov-2 spike protein through molecular dynamics simulation. Adv. Theory Simul. 4 , 2100152 (2021).
Article CAS PubMed PubMed Central Google Scholar
Kohn, W. & Sham, L. J. Self-consistent equations including exchange and correlation effects. Phys. Rev. 140 , A1133 (1965).
Article ADS MathSciNet Google Scholar
Marx, D. & Hutter, J. Ab Initio Molecular Dynamics: Basic Theory and Advanced Methods (Cambridge University Press, 2009).
Christensen, A. S., Bratholm, L. A., Faber, F. A. & Anatole von Lilienfeld, O. Fchl revisited: faster and more accurate quantum machine learning. J. Chem. Phys. 152 , 044107 (2020).
Article ADS CAS PubMed Google Scholar
Bartók, A. P., Payne, M. C., Kondor, R. & Csányi, G. Gaussian approximation potentials: the accuracy of quantum mechanics, without the electrons. Phys. Rev. Lett. 104 , 136403 (2010).
Article ADS PubMed Google Scholar
Chmiela, S. et al. Machine learning of accurate energy-conserving molecular force fields. Sci. Adv. 3 , e1603015 (2017).
Article ADS PubMed PubMed Central Google Scholar
Chmiela, S., Sauceda, H. E., Müller, K.-R. & Tkatchenko, A. Towards exact molecular dynamics simulations with machine-learned force fields. Nat. Commun. 9 , 1–10 (2018).
Article CAS Google Scholar
Chmiela, S. et al. Accurate global machine learning force fields for molecules with hundreds of atoms. Sci. Adv. 9 , eadf0873 (2023).
Article PubMed PubMed Central Google Scholar
Batzner, S. et al. E (3)-equivariant graph neural networks for data-efficient and accurate interatomic potentials. Nat. Commun. 13 , 1–11 (2022).
Article Google Scholar
Brandstetter, J., Hesselink, R., van der Pol, E., Bekkers, E. & Welling, M. Geometric and physical quantities improve e (3) equivariant message passing. In International Conference on Learning Representations (OpenReview.net, 2022).
Hutchinson, M. J. et al. Lietransformer: equivariant self-attention for lie groups. In International Conference on Machine Learning , (eds Meila, M. & Zhang, T.) 4533–4543 (PMLR, 2021).
Fuchs, F., Worrall, D., Fischer, V. & Welling, M. Se (3)-transformers: 3d roto-translation equivariant attention networks. Adv. Neural Inf. Process. Syst. 33 , 1970–1981 (2020).
Google Scholar
Gasteiger, J., Groß, J. & Günnemann, S. Directional message passing for molecular graphs. In International Conference on Learning Representations (OpenReview.net, 2019).
Gasteiger, J. et al. Fast and uncertainty-aware directional message passing for non-equilibrium molecules. arXiv preprint arXiv:2011.14115 (2020).
Schütt, K., Unke, O. & Gastegger, M. Equivariant message passing for the prediction of tensorial properties and molecular spectra. In International Conference on Machine Learning (eds Meila, M. & Zhang, T.) 9377–9388 (PMLR, 2021).
Thölke, P. & De Fabritiis, G. Torchmd-net: equivariant transformers for neural network based molecular potentials. In The International Conference on Learning Representations (OpenReview.net, 2022).
Gasteiger, J., Becker, F. & Günnemann, S. Gemnet: universal directional graph neural networks for molecules. Adv. Neural Inf. Process. Syst. 34 , 6790–6802 (2021).
Unke, O. T. et al. Spookynet: learning force fields with electronic degrees of freedom and nonlocal effects. Nat. Commun. 12 , 1–14 (2021).
Musaelian, A. et al. Learning local equivariant representations for large-scale atomistic dynamics. Nat. Commun. 14 , 579 (2023).
Batatia, I. et al. MACE: Higher order equivariant message passing neural networks for fast and accurate force fields. Adv. Neural. Inf. Process. Syst. 35 , 11423–11436 (2022).
Han, J., Rong, Y., Xu, T. & Huang, W. Geometrically equivariant graph neural networks: a survey. arXiv preprint arXiv:2202.07230 (2022).
Perwass, C., Edelsbrunner, H., Kobbelt, L. & Polthier, K. Geometric Algebra With Applications in Engineering Vol. 4 (Springer, 2009).
Zitnick, L. et al. Spherical channels for modeling atomic interactions. Adv. Neural. Inf. Process. Syst. 35 , 8054–8067 (2022).
Liao, Y.-L. & Smidt, T. Equiformer: Equivariant Graph Attention Transformer for 3D Atomistic Graphs. The Eleventh International Conference on Learning Representations. (2022).
Schütt, K. T., Arbabzadah, F., Chmiela, S., Müller, K. R. & Tkatchenko, A. Quantum-chemical insights from deep tensor neural networks. Nat. Commun. 8 , 1–8 (2017).
Christensen, A. S. & Von Lilienfeld, O. A. On the role of gradients for machine learning of molecular energies and forces. Mach. Learn.: Sci. Technol. 1 , 045018 (2020).
Chmiela, S. et al. Accurate global machine learning force fields for molecules with hundreds of atoms. Sci Adv. 9 , eadf0873 (2023).
Ramakrishnan, R., Dral, P. O., Rupp, M. & Von Lilienfeld, O. A. Quantum chemistry structures and properties of 134 kilo molecules. Sci. Data 1 , 1–7 (2014).
Xu, Z. et al. Molecule3d: a benchmark for predicting 3d geometries from molecular graphs. arXiv preprint arXiv:2110.01717 (2021).
Hu, W. et al. OGB-LSC: a large-scale challenge for machine learning on graphs. In Proc. of the 35 th Conference on Neural Information Processing Systems Datasets and Benchmarks Track (Round 2) (eds Vanschoren, J. & Yeung, S.) (Neural Information Processing Systems Foundation, Inc., 2021).
Wang, L., Liu, Y., Lin, Y., Liu, H. & Ji, S. Comenet: towards complete and efficient message passing for 3d molecular graphs. Adv. Neural Inf. Process. Syst. 35 , 650–664 (2022).
Qiao, Z. et al. Informing geometric deep learning with electronic interactions to accelerate quantum chemistry. Proc. Natl Acad. Sci. USA 119 , e2205221119 (2022).
Frank, T., Unke, O. T. & Muller, K. R. So3krates: equivariant attention for interactions on arbitrary length-scales in molecular systems. In Advances in Neural Information Processing Systems (eds Koyejo, S. et al.) (Curran Associates, Inc., 2022).
Luo, S. et al. One transformer can understand both 2d & 3d molecular data. arXiv preprint arXiv:2210.01765 (2022).
Wang, Y. et al. An ensemble of visnet, transformer-m, and pretraining models for molecular property prediction in ogb large-scale challenge@ neurips 2022. arXiv preprint arXiv:2211.12791 (2022).
Larsen, A. H. et al. The atomic simulation environment—a python library for working with atoms. J. Phys.: Condens. Matter 29 , 273002 (2017).
Smith, J. S., Isayev, O. & Roitberg, A. E. Ani-1: an extensible neural network potential with DFT accuracy at force field computational cost. Chem. Sci. 8 , 3192–3203 (2017).
Zhang, L., Han, J., Wang, H., Car, R. & Weinan, E. Deep potential molecular dynamics: a scalable model with the accuracy of quantum mechanics. Phys. Rev. Lett. 120 , 143001 (2018).
Unke, O. T. & Meuwly, M. Physnet: a neural network for predicting energies, forces, dipole moments, and partial charges. J. Chem. Theory Comput. 15 , 3678–3693 (2019).
Article CAS PubMed Google Scholar
Qi, R., Wei, G., Ma, B. & Nussinov, R. Replica exchange molecular dynamics: a practical application protocol with solutions to common problems and a peptide aggregation and self-assembly example. In Peptide Self-assembly (eds Nilsson, B. L. & Doran, T. M.) 101–119 (Springer, 2018).
Frisch, M. J. et al. Gaussian 16 Revision C.01 (Gaussian Inc. Wallingford, CT, 2016).
Wang, T., He, X., Li, M., Shao, B. & Liu, T.-Y. AIMD-Chig: exploring the conformational space of a 166-atom protein chignolin with ab initio molecular dynamics. Sci. Data 10 , 549 (2023).
Wang, Z. et al. Improving machine learning force fields for molecular dynamics simulations with fine-grained force metrics. J. Chem. Phys. 159 , 035101 (2023).
Gasteiger, J. et al. GemNet-OC: Developing Graph Neural Networks for Large and Diverse Molecular Simulation Datasets. Transactions on Machine Learning Research (2022).
Case, D. A. et al. Amber 2021 (University of California, San Francisco, 2021).
Van der Maaten, L. & Hinton, G. Visualizing data using t-sne. J. Mach. Learn. Res. 9 , 2579–2605 (2008).
Ester, M., Kriegel, H.-P., Sander, J., Xu, X. et al. A density-based algorithm for discovering clusters in large spatial databases with noise. In kdd (eds Simoudis, E., Han, J. & Fayyad, U.) Vol. 96, 226–231 (AAAI Press, 1996).
Nesbet, R. Atomic Bethe–Goldstone equations. III. correlation energies of ground states of Be, B, C, N, O, F, and Ne. Phys. Rev. 175 , 2 (1968).
Article ADS CAS Google Scholar
Hankins, D., Moskowitz, J. & Stillinger, F. Water molecule interactions. J. Chem. Phys. 53 , 4544–4554 (1970).
Gordon, M. S., Fedorov, D. G., Pruitt, S. R. & Slipchenko, L. V. Fragmentation methods: a route to accurate calculations on large systems. Chem. Rev. 112 , 632–672 (2012).
Joshi, C. K., Bodnar, C., Mathis, S. V., Cohen, T. & Lio, P. On the expressive power of geometric graph neural networks. arXiv preprint arXiv:2301.09308 (2023).
Ying, C. et al. Do transformers really perform badly for graph representation? Adv. Neural Inf. Process. Syst. 34 , (2021).
Vaswani, A. et al. Attention is all you need. Adv. Neural Inf. Process. Syst. 30 , (2017).
Wang, T. Enhancing geometric representations for molecules with equivariant vector–scalar interactive message passing. AI2BMD https://doi.org/10.5281/zenodo.10069040 (2023).
Download references
Acknowledgements
We would like to express our sincere gratitude to S. Chmiela, H.E. Sauceda, K.R. Müller, and A. Tkatchenko, for their invaluable assistance in performing the simulations and analyzing the vibrational spectra. Their extensive expertise and knowledge greatly contributed to the completion of the supplementary experiments, making our manuscript more solid.
Author information
These authors contributed equally: Yusong Wang, Tong Wang, Shaoning Li.
Authors and Affiliations
Microsoft Research AI4Science, 100080, Beijing, China
Yusong Wang, Tong Wang, Shaoning Li, Xinheng He, Mingyu Li, Zun Wang, Bin Shao & Tie-Yan Liu
National Key Laboratory of Human–Machine Hybrid Augmented Intelligence, National Engineering Research Center for Visual Information and Applications, and Institute of Artificial Intelligence and Robotics, Xi’an Jiaotong University, 710049, Xi’an, China
Yusong Wang & Nanning Zheng
The CAS Key Laboratory of Receptor Research and State Key Laboratory of Drug Research, Shanghai Institute of Materia Medica, Chinese Academy of Sciences, 201203, Shanghai, China
University of Chinese Academy of Sciences, 100049, Beijing, China
Medicinal Chemistry and Bioinformatics Center, School of Medicine, Shanghai Jiaotong University, Shanghai, 200025, China
You can also search for this author in PubMed Google Scholar
Contributions
T.W. led, conceived, and designed the study. T.W. is the lead contact. Y.W., S.L., X.H., and M.L. conducted the work when they were visiting Microsoft Research. S.L., Y.W., and T.W. carried out algorithm design. Y.W., S.L., X.H., and T.W. carried out experiments, evaluations, analysis, and visualization. Y.W. and S.L. wrote the original manuscript. T.W., X.H., M.L., Z.W., and B.S. revised the manuscript. N.Z. and T.-Y.L. contributed to the writing. All authors reviewed the final manuscript.
Corresponding authors
Correspondence to Tong Wang or Bin Shao .
Ethics declarations
Competing interests.
T.W., B.S., and T.-Y.L. have been filing a patent on ViSNet model. The remaining authors declare no competing interests.
Peer review
Peer review information.
Nature Communications thanks Zhirong Liu and the other, anonymous, reviewer(s) for their contribution to the peer review of this work. A peer review file is available.
Additional information
Publisher’s note Springer Nature remains neutral with regard to jurisdictional claims in published maps and institutional affiliations.
Supplementary information
Supplementary material, peer review file, reporting summary, source data, source data, rights and permissions.
Open Access This article is licensed under a Creative Commons Attribution 4.0 International License, which permits use, sharing, adaptation, distribution and reproduction in any medium or format, as long as you give appropriate credit to the original author(s) and the source, provide a link to the Creative Commons license, and indicate if changes were made. The images or other third party material in this article are included in the article’s Creative Commons license, unless indicated otherwise in a credit line to the material. If material is not included in the article’s Creative Commons license and your intended use is not permitted by statutory regulation or exceeds the permitted use, you will need to obtain permission directly from the copyright holder. To view a copy of this license, visit http://creativecommons.org/licenses/by/4.0/ .
Reprints and permissions
About this article
Cite this article.
Wang, Y., Wang, T., Li, S. et al. Enhancing geometric representations for molecules with equivariant vector-scalar interactive message passing. Nat Commun 15 , 313 (2024). https://doi.org/10.1038/s41467-023-43720-2
Download citation
Received : 04 May 2023
Accepted : 16 November 2023
Published : 05 January 2024
DOI : https://doi.org/10.1038/s41467-023-43720-2
Share this article
Anyone you share the following link with will be able to read this content:
Sorry, a shareable link is not currently available for this article.
Provided by the Springer Nature SharedIt content-sharing initiative
This article is cited by
Equivariant neural network force fields for magnetic materials.
- Zilong Yuan
Quantum Frontiers (2024)
By submitting a comment you agree to abide by our Terms and Community Guidelines . If you find something abusive or that does not comply with our terms or guidelines please flag it as inappropriate.
Quick links
- Explore articles by subject
- Guide to authors
- Editorial policies
Sign up for the Nature Briefing newsletter — what matters in science, free to your inbox daily.

Advertisement
Supported by
Who Won the Debate? Biden Stumbles Left Trump on Top
A halting debate performance by President Biden left Democratic strategists reeling, raising questions about his fitness to stay in the race.
- Share full article

By Alan Rappeport
Reporting from Washington
In the first presidential debate of the year between the leading Democratic and Republican candidates, President Biden and former President Donald J. Trump clashed on inflation, taxes, Ukraine and the future of democracy.
A halting performance from Mr. Biden and a relatively steady and measured delivery by Mr. Trump left Democrats deeply concerned about Mr. Biden’s prospects. Personal attacks overshadowed discussions of policy during the debate, with the candidates sparring over who had a better golf game, their respective cognitive abilities and their legal problems.
On cable news and social media, strategists from both parties wondered if Mr. Biden could continue in the race against Mr. Trump. Few Democrats could muster an upbeat assessment of the president’s performance.
Here is a sampling of the reaction.
“It was a really disappointing debate performance from Joe Biden. I don’t think there’s any other way to slice it. His biggest issue was to prove to the American people that he had the energy, the stamina — and he didn’t do that,” Kate Bedingfield, Mr. Biden’s former White House communications director, said on CNN.
“Biden is even whiffing on his easy pitches — abortion and Jan. 6. I mean, my God,” said Matt Gorman, a Republican strategist and former senior adviser to the presidential campaign for Senator Tim Scott of South Carolina.
“Look, I debated Joe 7 times in 2020. He’s a different guy in 2024,” Andrew Yang, a Democratic presidential candidate in 2020, said on the social media platform X, adding the hashtag #swapJoeout.
We are having trouble retrieving the article content.
Please enable JavaScript in your browser settings.
Thank you for your patience while we verify access. If you are in Reader mode please exit and log into your Times account, or subscribe for all of The Times.
Thank you for your patience while we verify access.
Already a subscriber? Log in .
Want all of The Times? Subscribe .
- - Google Chrome
Intended for healthcare professionals
- My email alerts
- BMA member login
- Username * Password * Forgot your log in details? Need to activate BMA Member Log In Log in via OpenAthens Log in via your institution

Search form
- Advanced search
- Search responses
- Search blogs
- Trends in...
Trends in cardiovascular disease incidence among 22 million people in the UK over 20 years: population based study
- Related content
- Peer review
- Geert Molenberghs , professor 4 ,
- Geert Verbeke , professor 4 ,
- Francesco Zaccardi , associate professor 5 ,
- Claire Lawson , associate professor 5 ,
- Jocelyn M Friday , data scientist 1 ,
- Huimin Su , PhD student 2 ,
- Pardeep S Jhund , professor 1 ,
- Naveed Sattar , professor 6 ,
- Kazem Rahimi , professor 3 ,
- John G Cleland , professor 1 ,
- Kamlesh Khunti , professor 5 ,
- Werner Budts , professor 1 7 ,
- John J V McMurray , professor 1
- 1 School of Cardiovascular and Metabolic Health, British Heart Foundation Cardiovascular Research Centre, University of Glasgow, Glasgow, UK
- 2 Department of Cardiovascular Sciences, KU Leuven, Leuven, Belgium
- 3 Deep Medicine, Nuffield Department of Women’s and Reproductive Health, University of Oxford, Oxford, UK
- 4 Interuniversity Institute for Biostatistics and statistical Bioinformatics (I-BioStat), Hasselt University and KU Leuven, Belgium
- 5 Leicester Real World Evidence Unit, Diabetes Research Centre, University of Leicester, Leicester, UK
- 6 College of Medical, Veterinary and Life Sciences, University of Glasgow, Glasgow, UK
- 7 Congenital and Structural Cardiology, University Hospitals Leuven, Belgium
- Correspondence to: N Conrad nathalie.conrad{at}kuleuven.be (or @nathalie_conrad on X)
- Accepted 1 May 2024
Objective To investigate the incidence of cardiovascular disease (CVD) overall and by age, sex, and socioeconomic status, and its variation over time, in the UK during 2000-19.
Design Population based study.
Setting UK.
Participants 1 650 052 individuals registered with a general practice contributing to Clinical Practice Research Datalink and newly diagnosed with at least one CVD from 1 January 2000 to 30 June 2019.
Main outcome measures The primary outcome was incident diagnosis of CVD, comprising acute coronary syndrome, aortic aneurysm, aortic stenosis, atrial fibrillation or flutter, chronic ischaemic heart disease, heart failure, peripheral artery disease, second or third degree heart block, stroke (ischaemic, haemorrhagic, and unspecified), and venous thromboembolism (deep vein thrombosis or pulmonary embolism). Disease incidence rates were calculated individually and as a composite outcome of all 10 CVDs combined and were standardised for age and sex using the 2013 European standard population. Negative binomial regression models investigated temporal trends and variation by age, sex, and socioeconomic status.
Results The mean age of the population was 70.5 years and 47.6% (n=784 904) were women. The age and sex standardised incidence of all 10 prespecified CVDs declined by 19% during 2000-19 (incidence rate ratio 2017-19 v 2000-02: 0.80, 95% confidence interval 0.73 to 0.88). The incidence of coronary heart disease and stroke decreased by about 30% (incidence rate ratios for acute coronary syndrome, chronic ischaemic heart disease, and stroke were 0.70 (0.69 to 0.70), 0.67 (0.66 to 0.67), and 0.75 (0.67 to 0.83), respectively). In parallel, an increasing number of diagnoses of cardiac arrhythmias, valve disease, and thromboembolic diseases were observed. As a result, the overall incidence of CVDs across the 10 conditions remained relatively stable from the mid-2000s. Age stratified analyses further showed that the observed decline in coronary heart disease incidence was largely restricted to age groups older than 60 years, with little or no improvement in younger age groups. Trends were generally similar between men and women. A socioeconomic gradient was observed for almost every CVD investigated. The gradient did not decrease over time and was most noticeable for peripheral artery disease (incidence rate ratio most deprived v least deprived: 1.98 (1.87 to 2.09)), acute coronary syndrome (1.55 (1.54 to 1.57)), and heart failure (1.50 (1.41 to 1.59)).
Conclusions Despite substantial improvements in the prevention of atherosclerotic diseases in the UK, the overall burden of CVDs remained high during 2000-19. For CVDs to decrease further, future prevention strategies might need to consider a broader spectrum of conditions, including arrhythmias, valve diseases, and thromboembolism, and examine the specific needs of younger age groups and socioeconomically deprived populations.
Introduction
Since the 1970s, the prevention of coronary disease, both primary and secondary, has improved considerably, largely attributable to public health efforts to control risk factors, such as antismoking legislation, and the widespread use of drugs such as statins. 1 2
Improvements in mortality due to heart disease have, however, stalled in several high income countries, 3 and reports suggest that the incidence of heart disease might even be increasing among younger people. 4 5 6 Conversely, along with coronary heart disease, other cardiovascular conditions are becoming relatively more prominent in older people, altering the profile of cardiovascular disease (CVD) in ageing societies. The importance of non-traditional risk factors for atherosclerotic diseases, such as socioeconomic deprivation, has also been increasingly recognised. Whether socioeconomic deprivation is as strongly associated with other CVDs as with atherosclerosis is uncertain, but it is important to understand as many countries have reported an increase in socioeconomic inequalities. 7
Large scale epidemiological studies are therefore needed to investigate secular trends in CVDs to target future preventive efforts, highlight the focus for future clinical trials, and identify healthcare resources required to manage emerging problems. Existing comprehensive efforts, such as statistics on CVD from leading medical societies or the Global Burden of Diseases studies, have helped toward this goal, but reliable age standardised incidence rates for all CVDs, how these vary by population subgroups, and changes over time are currently not available. 8 9 10
We used a large longitudinal database of linked primary care, secondary care, and death registry records from a representative sample of the UK population 11 12 to assess trends in the incidence of 10 of the most common CVDs in the UK during 2000-19, and how these differed by sex, age, socioeconomic status, and region.
Data source and study population
We used anonymised electronic health records from the GOLD and AURUM datasets of Clinical Practice Research Datalink (CPRD). CPRD contains information on about 20% of the UK population and is broadly representative of age, sex, ethnicity, geographical spread, and socioeconomic deprivation. 11 12 It is also one of the largest databases of longitudinal medical records from primary care in the world and has been validated for epidemiological research for a wide range of conditions. 11 We used the subset of CPRD records that linked information from primary care, secondary care from Hospital Episodes Statistics (HES admitted patient care and HES outpatient) data, and death certificates from the Office for National Statistics (ONS). Linkage was possible for a subset of English practices, covering about 50% of the CPRD records. Data coverage dates were 1 January 1985 to 31 December 2019 for primary care data (including drug prescription data), 1 April 1997 to 30 June 2019 for secondary care data, and 2 January 1998 to 30 May 2019 for death certificates.
Included in the study were men and women registered with a general practice for at least one year during the study period (1 January 2000 to 30 June 2019) whose records were classified by CPRD as acceptable for use in research and approved for HES and ONS linkage.
Study endpoints
The primary endpoint was the first presentation of CVD as recorded in primary or secondary care. We investigated 10 CVDs: acute coronary syndrome, aortic aneurysm, aortic stenosis, atrial fibrillation or flutter, chronic ischaemic heart disease, heart failure, peripheral artery disease, second or third degree heart block, stroke (ischaemic, haemorrhagic, or unspecified), and venous thromboembolism (deep vein thrombosis or pulmonary embolism). We defined incident diagnoses as the first record of that condition in primary care or secondary care regardless of its order in the patient’s record.
Diseases were considered individually and as a composite outcome of all 10 CVDs combined. For the combined analyses, we calculated the primary incidence (considering only the first recorded CVD in each patient, reflecting the number of patients affected by CVDs) and the total incidence (considering all incident CVD diagnoses in each patient, reflecting the cumulative number of CVD diagnoses). We performed sensitivity analyses including diagnoses recorded on death certificates.
To identify diagnoses, we compiled a list of diagnostic codes based on the coding schemes in use in each data source following previously established methods. 13 14 15 We used ICD-10 (international classification of diseases, 10th revision) codes for diagnoses recorded in secondary care, ICD-9 (international classification of diseases, ninth revision) (in use until 31 December 2000) and ICD-10 codes for diagnoses recorded on death certificates (used in sensitivity analyses only), the UK Office of Population Censuses and Surveys classification (OPCS-4) for procedures performed in secondary care settings, and a combination of Read, SNOMED, and local EMIS codes for diagnoses recorded in primary care records (see supplementary table S1). 16 Supplementary texts S1, S2, and S3 describe our approach to the generation of the diagnostic code list as well as considerations and sensitivity analyses into the validity of diagnoses recorded in UK electronic health records.
We selected covariates to represent a range of known cardiovascular risk factors. For clinical data, including systolic and diastolic blood pressure, smoking status, cholesterol (total:high density lipoprotein ratio), and body mass index (BMI), we abstracted data from primary care records as the most recent measurement within two years before the incident CVD diagnosis. BMI was categorised as underweight (<18.5), normal (18.5-24.9), overweight (25-29.9), and obesity (≥30). Information on the prevalence of chronic kidney disease, dyslipidaemia, hypertension, and type 2 diabetes was obtained as the percentage of patients with a diagnosis recorded in their primary care or secondary care record at any time up to and including the date of a first CVD diagnosis. Patients’ socioeconomic status was described using the index of multiple deprivation 2015, 17 a composite measure of seven dimensions (income, employment, education, health, crime, housing, living environment) and provided by CPRD. Measures of deprivation are calculated at small area level, covering an average population of 1500 people, and are presented in fifths, with the first 20% and last 20% representing the least and most deprived areas, respectively. We extracted information on ethnicity from both primary and secondary care records, and we used secondary care data when records differed. Ethnicity was grouped into four categories: African/Caribbean, Asian, white, and mixed/other. Finally, we extracted information on cardiovascular treatments (ie, aspirin and other antiplatelets, alpha adrenoceptor antagonists, aldosterone antagonists/mineralocorticoid receptor antagonists, angiotensin converting enzyme inhibitors, angiotensin II receptor antagonists, beta blockers, calcium channel blockers, diuretics, nitrates, oral anticoagulants, and statins) as the number of patients with at least two prescriptions of each drug class within six months after incident CVD, among patients alive and registered with a general practitioner 30 days after the diagnosis. Supplementary table S2 provides a list of substances included in each drug class. Prescriptions were extracted from primary care records up to 31 December 2019.
Statistical analyses
Categorical data for patient characteristics are presented as frequencies (percentages), and continuous data are presented as means and standard deviations (SDs) for symmetrically distributed data or medians and interquartile ranges (IQRs) for non-symmetrically distributed data, over the whole CVD cohort and stratified by age, sex, socioeconomic status, region, and calendar year of diagnosis. For variables with missing entries, we present numbers and percentages of records with missing data. For categorical variables, frequencies refer to complete cases.
Incidence rates of CVD were calculated by dividing the number of incident diagnoses by the number of patient years in the cohort. Category specific rates were computed separately for subgroups of age, sex, socioeconomic status, region, and calendar year of diagnosis. Age calculations were updated for each calendar year. To ensure calculations referred to incident diagnoses, we excluded individuals, from both the numerator and the denominator populations, with a disease of interest diagnosed before the study start date (1 January 2000), or within the first 12 months of registration with their general practice. Time at risk started at the latest of the patient’s registration date plus 12 months, 30 June of their birth year, or study start date; and stopped at the earliest of death, transfer out of practice, last collection date of the practice, incidence of the disease of interest, or linkage end date (30 June 2019). Disease incidence was standardised for age and sex 18 using the 2013 European standard population 19 in five year age bands up to age 90 years.
Negative binomial regression models were used to calculate overall and category specific incidence rate ratios and corresponding 95% confidence intervals (CIs). 20 Models were adjusted for calendar year of diagnosis, age (categorised into five years age bands), sex, socioeconomic status, and region. We chose negative binomial models over Poisson models to account for potential overdispersion in the data. Sensitivity analyses comparing Poisson and negative binomial models showed similar results.
Study findings are reported according to the RECORD (reporting of studies conducted using observational routinely collected health data) recommendations. 21 We performed statistical analyses in R, version 4.3.3 (R Foundation for Statistical Computing, Vienna, Austria).
Patient and public involvement
No patients or members of the public were directly involved in this study owing to constraints on funding and time.
A total of 22 009 375 individuals contributed data between 1 January 2000 and 30 June 2019, with 146 929 629 patient years of follow-up. Among those we identified 2 906 770 new CVD diagnoses, affecting 1 650 052 patients. Mean age at first CVD diagnosis was 70.5 (SD 15.0) years, 47.6% (n=784 904) of patients were women, and 11.6% (n=191 421), 18.0% (n=296 554), 49.7% (n=820 892), and 14.2% (n=233 833) of patients had a history of chronic kidney disease, dyslipidaemia, hypertension, and type 2 diabetes, respectively, at the time of their first CVD diagnosis ( table 1 ).
Characteristics of patients with a first diagnosis of CVD, 2000-19. Values are number (percentage) unless stated otherwise
- View inline
During 2017-19, the most common CVDs were atrial fibrillation or flutter (age-sex standardised incidence 478 per 100 000 person years), heart failure (367 per 100 000 person years), and chronic ischaemic heart disease (351 per 100 000 person years), followed by acute coronary syndrome (190 per 100 000 person years), venous thromboembolism (183 per 100 000 person years), and stroke (181 per 100 000 patient years) ( fig 1 ).

Incidence of a first diagnosis of cardiovascular disease per 100 000 person years, 2000-19. Incidence rates are age-sex standardised to the 2013 European standard population. Any cardiovascular disease refers to the primary incidence of cardiovascular disease across the10 conditions investigated (ie, number of patients with a first diagnosis of cardiovascular disease). See supplementary table S4 for crude incidence rates by age and sex groups. IRR=incidence rate ratio
- Download figure
- Open in new tab
- Download powerpoint
Temporal trends
The primary incidence of CVDs (ie, the number of patients with CVD) decreased by 20% during 2000-19 (age-sex standardised incidence rate ratio 2017-19 v 2000-02: 0.80 (95% CI 0.73 to 0.88)). However, the total incidence of CVD (ie, the total number of new CVD diagnoses) remained relatively stable owing to an increasing number of subsequent diagnoses among patients already affected by a first CVD (incidence rate ratio 2017-19 v 2000-02: 1.00 (0.91 to 1.10)).
The observed decline in CVD incidence was largely due to declining rates of atherosclerotic diseases, in particular acute coronary syndrome, chronic ischaemic heart disease, and stroke, which decreased by about 30% during 2000-19. The incidence of peripheral artery disease also declined, although more modestly (incidence rate ratio 2017-19 v 2000-02: 0.89 (0.80 to 0.98)) ( fig 1 ).
The incidence of non-atherosclerotic heart diseases increased at varying rates, with incidence of aortic stenosis and heart block more than doubling over the study period (2017-19 v 2000-02: 2.42 (2.13 to 2.74) and 2.22 (1.99 to 2.46), respectively) ( fig 1 ). These increasing rates of non-atherosclerotic heart diseases balanced the reductions in ischaemic diseases so that the overall incidence of CVD across the 10 conditions appeared to reach a plateau and to remain relatively stable from 2007-08 (incidence rate ratio 2017-19 v 2005-07: 1.00 (0.91 to 1.10)) ( fig 2 ).

Age standardised incidence of cardiovascular disease by sex, 2000-19. Any cardiovascular disease refers to the primary incidence of cardiovascular disease across the 10 conditions investigated (ie, number of patients with a first diagnosis of cardiovascular disease). IRR=incidence rate ratio
Age stratified analyses further showed that the observed decrease in incidence of chronic ischaemic heart disease, acute coronary syndrome, and stroke was largely due to a reduced incidence in those aged >60 years, whereas incidence rates in those aged <60 years remained relatively stable ( fig 3 and fig 4 ).

Sex standardised incidence of cardiovascular disease in all age groups. Any cardiovascular disease refers to the primary incidence of cardiovascular disease across the 10 conditions investigated (ie, number of patients with a first diagnosis of cardiovascular disease)

Sex standardised incidence of cardiovascular diseases by age subgroups <69 years. Any cardiovascular disease refers to the primary incidence of cardiovascular disease across the 10 conditions investigated (ie, number of patients with a first diagnosis of cardiovascular disease)
Age at diagnosis
CVD incidence was largely concentrated towards the end of the life span, with a median age at diagnosis generally between 65 and 80 years. Only venous thromboembolism was commonly diagnosed before age 45 years ( fig 5 ). Over the study period, age at first CVD diagnosis declined for several conditions, including stroke (on average diagnosed 1.9 years earlier in 2019 than in 2000), heart block (1.3 years earlier in 2019 than in 2000), and peripheral artery disease (1 year earlier in 2019 than in 2000) (see supplementary figure S1). Adults with a diagnosis before age 60 years were more likely to be from lower socioeconomic groups and to have a higher prevalence of several risk factors, including obesity, smoking, and high cholesterol levels (see supplementary table S3).

Incidence rates of cardiovascular diseases calculated by one year age bands and divided into a colour gradient of 20 quantiles to reflect incidence density by age. IQR=interquartile range
Incidence by sex
Age adjusted incidence of all CVDs combined was higher in men (incidence rate ratio for women v men: 1.46 (1.41 to 1.51)), with the notable exception of venous thromboembolism, which was similar between men and women. The incidence of aortic aneurysms was higher in men (3.49 (3.33 to 3.65)) ( fig 2 ). The crude incidence of CVD, however, was similar between men and women (1069 per 100 000 patient years and 1176 per 100 000 patient years, respectively), owing to the higher number of women in older age groups. Temporal trends in disease incidence were generally similar between men and women ( fig 2 ).
Incidence by socioeconomic status
The most deprived socioeconomic groups had a higher incidence of any CVDs (incidence rate ratio most deprived v least deprived: 1.37 (1.30 to 1.44)) ( fig 6 ). A socioeconomic gradient was observed across almost every condition investigated. That gradient did not decrease over time, and it was most noticeable for peripheral artery disease (incidence rate ratio most deprived v least deprived: 1.98 (1.87 to 2.09)), acute coronary syndrome (1.55 (1.54 to 1.57)), and heart failure (1.50 (1.41 to 1.59)). For aortic aneurysms, atrial fibrillation, heart failure, and aortic stenosis, socioeconomic inequalities in disease incidence appeared to increase over time.

Age-sex standardised incidence rates of cardiovascular diseases by socioeconomic status (index of multiple deprivation 2015). Any cardiovascular disease refers to the primary incidence of cardiovascular disease across the 10 conditions investigated (ie, number of patients with a first diagnosis of cardiovascular disease). Yearly incidence estimates were smoothed using loess (locally estimated scatterplot smoothing) regression lines
Regional differences
Higher incidence rates were seen in northern regions (north west, north east, Yorkshire and the Humber) of England for all 10 conditions investigated, even after adjusting for socioeconomic status. Aortic aneurysms and aortic stenosis had the strongest regional gradients, with incidence rates about 30% higher in northern regions compared with London. Geographical variations remained modest, however, and did not appear to change considerably over time (see supplementary figure S2).
Sensitivity analyses
In sensitivity analyses that used broader disease definitions, that included diagnoses recorded on death certificates, that relied on longer lookback periods for exclusion of potentially prevalent diagnoses, or that were restricted to diagnoses recorded during hospital admissions, temporal trends in disease incidence appeared similar (see supplementary figures S3-S6).
Secondary prevention treatments
The proportion of patients using statins and antihypertensive drugs after a first CVD diagnosis increased over time, whereas the use of non-dihydropyridines calcium channel blockers, nitrates, and diuretics decreased over time. Non-vitamin K antagonist oral anticoagulants increasingly replaced vitamin K anticoagulants (see supplementary figure S7).
The findings of this study suggest that important changes occurred in the distribution of CVDs during 2000-19 and that several areas are of concern. The incidence of non-atherosclerotic heart diseases was shown to increase, the decline in atherosclerotic disease in younger people was stalling, and socioeconomic inequalities had a substantial association across almost every CVD investigated.
Implications for clinical practice and policy
Although no causal inference can be made from our data, the decline in rates of ischaemic diseases coincided with reductions in the prevalence of risk factors such as smoking, hypertension, and raised cholesterol levels in the general population over the same period, 22 and this finding suggests that efforts in the primary and secondary prevention of atherosclerotic diseases have been successful. The decline in stroke was not as noticeable as that for coronary heart disease, which may reflect the rising incidence of atrial fibrillation. The variation in trends for peripheral artery disease could be due to differences in risk factors (eg, a stronger association with diabetes), the multifaceted presentations and causes, and the introduction of systematic leg examinations for people with diabetes. 23 24
All the non-atherosclerotic diseases, however, appeared to increase during 2000-19. For some conditions, such as heart failure, the observed increase remained modest, whereas for others, such as aortic stenosis and heart block, incidence rates doubled. All analyses in this study were standardised for age and sex, to illustrate changes in disease incidence independently of changes in population demographics. Whether these trends solely reflect increased awareness, access to diagnostic tests, or even screening (eg, for abdominal aortic aneurysm 25 ) and coding practices, is uncertain. Reductions in premature death from coronary heart disease may have contributed to the emergence of these other non-atherosclerotic CVDs. Regardless, the identification of increasing numbers of people with these problems has important implications for health services, especially the provision of more surgical and transcatheter valve replacement, pacemaker implantation, and catheter ablation for atrial fibrillation. Importantly, these findings highlight the fact that for many cardiovascular conditions such as heart block, aortic aneurysms, and non-rheumatic valvular diseases, current medical practice remains essentially focused on the management of symptoms and secondary prevention and that more research into underlying causes and possible primary prevention strategies is needed. 26 27
These varying trends also mean that the contribution of individual CVDs towards the overall burden has changed. For example, atrial fibrillation or flutter are now the most common CVDs in the UK. Atrial fibrillation is also a cause (and consequence) of heart failure, and these two increasingly common problems may amplify the incidence of each other. Venous thromboembolism and heart block also appeared as important contributors to overall CVD burden, with incidence rates similar to those of stroke and acute coronary syndrome, yet both receive less attention in terms of prevention efforts.
The stalling decline in the rate of coronary heart disease in younger age groups is of concern, has also been observed in several other high income countries, and may reflect rising rates of physical inactivity, obesity, and type 2 diabetes in young adults. 4 6 28 The stalled decline suggests prevention approaches may need to be expanded beyond antismoking legislation, blood pressure control, and lipid lowering interventions to include the promotion of physical activity, weight control, and use of new treatments shown to reduce cardiovascular risk in people with type 2 diabetes. 29 Although CVD incidence is generally low in people aged <60 years, identifying those at high risk of developing CVD at a young age and intervening before problems occur could reduce premature morbidity and mortality and have important economic implications.
Our study further found that socioeconomic inequalities may contribute to CVD burden, and that this association is not restricted to selected conditions but is visible across most CVDs. The reasons behind the observed increase in risk in relation to socioeconomic inequalities are likely to be multifactorial and to include environmental, occupational, psychosocial, and behavioural risk factors, including established cardiovascular risk factors such as smoking, obesity, nutrition, air pollution, substance misuse, and access to care. 30 How these findings apply to different countries is likely to be influenced by socioeconomic structures and healthcare systems, although health inequalities have been reported in numerous countries. 30 One important factor in the present study is that access to care is free at the point of care in the UK, 31 and yet socioeconomic inequalities persist despite universal health coverage and they did not appear to improve over time. Independently of the specificities of individual countries, our findings highlight the importance of measuring and considering health inequalities and suggest that dealing with the social determinants of health—the conditions under which people are born, live, work, and age—could potentially bring substantial health improvements across a broad range of chronic conditions.
Finally, our results reflect disease incidence based on diagnostic criteria, screening practices, availability, and accuracy of diagnostic tests in place at a particular time and therefore must be interpreted within this context. 32 Several of the health conditions investigated are likely to being sought and detected with increased intensity over the study period. For example, during the study period the definition of myocardial infarction was revised several times, 33 34 35 and high sensitivity troponins were progressively introduced in the UK from 2010. These more sensitive markers of cardiac injury are thought to have increased the detection rates for less severe disease. 36 37 Similarly, increased availability of computed tomography may have increased detection rates for stroke. 38 These changes could have masked an even greater decline in these conditions than observed in the present study. Conversely, increased use of other biochemical tests (such as natriuretic peptides) and more sensitive imaging techniques might have increased the detection of other conditions. 39 40 41 The implementation of a screening programme for aortic aneurysm and incentive programmes aimed at improving coding practices, including the documentation of CVD, associated risk factors and comorbidities, and treatment of these, are also likely to have contributed to the observed trends. 25 42 43 As a result, the difference in incidence estimates and prevalence of comorbidities over time may not reflect solely changes in the true incidence but also differences in ascertainment of people with CVD. 44 Nonetheless, long term trends in large and unconstrained populations offer valuable insights for healthcare resource planning and for the design of more targeted prevention strategies that could otherwise not be answered by using smaller cohorts, cross sectional surveys, or clinical trials; and precisely because they are based on routinely reported diagnoses they are more likely to capture the burden of disease as experienced by doctors and health services.
Strengths and limitations of this study
A key strength of this study is its statistical power, with >140 million person years of data. The large size of the cohort allowed us to perform incidence calculations for a broad spectrum of conditions, and to examine the influence of age, sex, and socioeconomic status as well as trends over 20 years. One important limitation of our study was the modest ethnic diversity in our cohort and the lack of information on ethnicity for the denominator population, which precluded us from stratifying incidence estimates by ethnic group. Our analyses were also limited by the unavailability or considerable missingness of additional variables potentially relevant to the development of CVD, such as smoking, body mass index, imaging data, women specific cardiovascular risk factors (eg, pregnancy associated hypertension and gestational diabetes), and blood biomarkers. Further research may also need to consider an even wider spectrum of CVDs, including individual types of valve disease, pregnancy related conditions, and infection related heart diseases. Research using databases with electronic health records is also reliant on the accuracy of clinical coding input by doctors in primary care as part of a consultation, or in secondary care as part of a hospital admission. We therefore assessed the validity of diagnoses in UK electronic health records data and considered it to be appropriate in accordance with the >200 independent validation studies reporting an average positive predictive value of about 90% for recorded diagnoses. 45 Observed age distributions were also consistent with previous studies and added to the validity of our approach. Nevertheless, our results must be interpreted within the context and limitations of routinely collected data from health records, diagnostic criteria, screening practices, the availability and accuracy of diagnostic tests in place at that time, and the possibility that some level of miscoding is present or that some bias could have been introduced by restricting the cohort to those patients with at least 12 months of continuous data.
Conclusions
Efforts to challenge the notion of the inevitability of vascular events with ageing, and evidence based recommendations for coronary heart disease prevention, have been successful and can serve as a model for other non-communicable diseases. Our findings show that it is time to expand efforts to improve the prevention of CVDs. Broadening research and implementation efforts in both primary and secondary prevention to non-atherosclerotic diseases, tackling socioeconomic inequalities, and introducing better risk prediction and management among younger people appear to be important opportunities to tackle CVDs.
What is already known on this topic
Recent data show that despite decades of declining rates of cardiovascular mortality, the burden from cardiovascular disease (CVD) appears to have stalled in several high income countries
What this study adds
This observational study of a representative sample of 22 million people from the UK during 2000-19 found reductions in CVD incidence to have been largely restricted to ischaemic heart disease and stroke, and were paralleled by a rising number of diagnoses of cardiac arrhythmias, valve disease, and thromboembolic events
Venous thromboembolism and heart block were important contributors to the overall burden of CVDs, with incidence rates similar to stroke and acute coronary syndromes
Improvements in rates of coronary heart disease almost exclusively appeared to benefit those aged >60 years, and the CVD burden in younger age groups appeared not to improve
Ethics statements
Ethical approval.
This study was approved by the Clinical Practice Research Datalink Independent Scientific Advisory Committee.
Data availability statement
Access to Clinical Practice Research Datalink (CPRD) data is subject to a license agreement and protocol approval process that is overseen by CPRD’s research data governance process. A guide to access is provided on the CPRD website ( https://www.cprd.com/data-access ) To facilitate the subsequent use and replication of the findings from this study, aggregated data tables are provided with number of events and person years at risk by individual condition and by calendar year, age (by five year age band), sex, socioeconomic status, and region (masking field with fewer than five events, as per CPRD data security and privacy regulations) on our GitHub repository ( https://github.com/nathalieconrad/CVD_incidence ).
Acknowledgments
We thank Hilary Shepherd, Sonia Coton, and Eleanor L Axson from the Clinical Practice Research Datalink for their support and expertise in preparing the dataset underlying these analyses.
Contributors: NC and JJVM conceived and designed the study. NC, JJVM, GM, and GV designed the statistical analysis plan and NC performed the statistical analysis. All authors contributed to interpreting the results, drafting the manuscript, and the revisions. NC, GM, and GV had permission to access the raw data and NC and GM verified the raw data. All authors gave final approval of the version to be published and accept responsibility to submit the manuscript for publication. NC and JJVM accept full responsibility for the conduct of the study, had access to aggregated data, and controlled the decision to publish. They are the guarantors. The corresponding author attests that all listed authors meet authorship criteria and that no others meeting the criteria have been omitted.
Funding: This study was funded by a personal fellowship from the Research Foundation Flanders (grant No 12ZU922N), a research grant from the European Society of Cardiology (grant No App000037070), and the British Heart Foundation Centre of Research Excellence (grant No RE/18/6/34217). The funders had no role in considering the study design or in the collection, analysis, interpretation of data, writing of the report, or decision to submit the article for publication.
Competing interests: All authors have completed the ICMJE uniform disclosure form at www.icmje.org/disclosure-of-interest/ and declare: NC is funded by a personal fellowship from the Research Foundation Flanders and a research grant from the European Society of Cardiology. JMF, PSJ, JGC, NS, and JJVM are supported by British Heart Foundation Centre of Research Excellence. PSJ and JJVM are further supported by the Vera Melrose Heart Failure Research Fund. JJVM has received funding to his institution from Amgen and Cytokinetics for his participation in the steering sommittee for the ATOMIC-HF, COSMIC-HF, and GALACTIC-HF trials and meetings and other activities related to these trials; has received payments through Glasgow University from work on clinical trials, consulting, and other activities from Alnylam, Amgen, AstraZeneca, Bayer, Boehringer Ingelheim, Bristol Myers Squibb, Cardurion, Dal-Cor, GlaxoSmithKline, Ionis, KBP Biosciences, Novartis, Pfizer, and Theracos; and has received personal lecture fees from the Corpus, Abbott, Hikma, Sun Pharmaceuticals, Medscape/Heart.Org, Radcliffe Cardiology, Alkem Metabolics, Eris Lifesciences, Lupin, ProAdWise Communications, Servier Director, and Global Clinical Trial Partners. NS declares consulting fees or speaker honorariums, or both, from Abbott Laboratories, Afimmune, Amgen, AstraZeneca, Boehringer Ingelheim, Lilly, Hanmi Pharmaceuticals, Janssen, Merck Sharp & Dohme, Novartis, Novo Nordisk, Pfizer, Roche Diagnostics, and Sanofi; and grant support paid to his university from AstraZeneca, Boehringer Ingelheim, Novartis, and Roche Diagnostics. KK has acted as a consultant or speaker or received grants for investigator initiated studies for Astra Zeneca, Bayer, Novartis, Novo Nordisk, Sanofi-Aventis, Lilly, Merck Sharp & Dohme, Boehringer Ingelheim, Oramed Pharmaceuticals, Roche, and Applied Therapeutics. KK is supported by the National Institute for Health and Care Research (NIHR) Applied Research Collaboration East Midlands (ARC EM) and the NIHR Leicester Biomedical Research Centre (BRC). CL is funded by an NIHR Advanced Research Fellowship (NIHR-300111) and supported by the Leicester BRC. PSJ has received speaker fees from AstraZeneca, Novartis, Alkem Metabolics, ProAdWise Communications, Sun Pharmaceuticals, and Intas Pharmaceuticals; has received advisory board fees from AstraZeneca, Boehringer Ingelheim, and Novartis; has received research funding from AstraZeneca, Boehringer Ingelheim, Analog Devices; his employer, the University of Glasgow, has been remunerated for clinical trial work from AstraZeneca, Bayer, Novartis, and Novo Nordisk; and is the Director of Global Clinical Trial Partners. HS is supported by the China Scholarship Council. Other authors report no support from any organisation for the submitted work, no financial relationships with any organisations that might have an interest in the submitted work in the previous three years, and no other relationships or activities that could appear to have influenced the submitted work.
Transparency: The lead author (NC) affirms that the manuscript is an honest, accurate, and transparent account of the study being reported; that no important aspects of the study have been omitted; and that any discrepancies from the study as planned (and, if relevant, registered) have been explained.
Dissemination to participants and related patient and public communities: Results from this study will be shared with patient associations and foundations dedicated to preventing cardiovascular diseases, such as the European Heart Network and the American Heart Association. To reach the public, findings will also be press released alongside publication of this manuscript. Social media (eg, X) will be used to draw attention to the work and stimulate debate about its findings. Finally, the underlying developed algorithms will be freely available for academic use at https://github.com/nathalieconrad/CVD_incidence .
Provenance and peer review: Not commissioned; externally peer reviewed.
This is an Open Access article distributed in accordance with the terms of the Creative Commons Attribution (CC BY 4.0) license, which permits others to distribute, remix, adapt and build upon this work, for commercial use, provided the original work is properly cited. See: http://creativecommons.org/licenses/by/4.0/ .
- ↵ Institute for Health Metrics and Evaluation. Gobal Burden of Diseases Viz Hub. 2023. https://vizhub.healthdata.org/gbd-compare/
- Ananth CV ,
- Rutherford C ,
- Rosenfeld EB ,
- O’Flaherty M ,
- Allender S ,
- Scarborough P ,
- Andersson C ,
- Abdalla SM ,
- Mensah GA ,
- Johnson CO ,
- GBD-NHLBI-JACC Global Burden of Cardiovascular Diseases Writing Group
- Almarzooq ZI ,
- American Heart Association Council on Epidemiology and Prevention Statistics Committee and Stroke Statistics Subcommittee
- Townsend N ,
- Atlas Writing Group, European Society of Cardiology
- Herrett E ,
- Gallagher AM ,
- Bhaskaran K ,
- ↵ Wolf A, Dedman D, Campbell J, et al. Data resource profile: Clinical Practice Research Datalink (CPRD) Aurum. International Journal of Epidemiology. 2019 Mar 11 [cited 2019 Mar 22]; https://academic.oup.com/ije/advance-article/doi/10.1093/ije/dyz034/5374844
- Verbeke G ,
- Molenberghs G ,
- Ferguson LD ,
- ↵ Medicines and Healthcare products Regulatory Agency. What coding systems are used in CPRD data? 2023. https://www.cprd.com/defining-your-study-population
- ↵ Department for Communities and Local Government (DCLG). The English Index of Multiple Deprivation 2015: Guidance. 2015; pp1-7. https://www.gov.uk/government/statistics/english-indices-of-deprivation-2015
- ↵ Kirkwood B, Sterne J. Essential medical statistics. 2010.
- ↵ Eurostat’s task force. Revision of the European Standard Population Report. 2013.
- Benchimol EI ,
- Guttmann A ,
- RECORD Working Committee
- ↵ NHS Digital. Health Survey for England, 2021 part 1. https://digital.nhs.uk/data-and-information/publications/statistical/health-survey-for-england/2021
- Criqui MH ,
- ↵ Health and Social Care Information Centre. Quality and Outcomes Framework - Indicators 2011-12. 2011. https://digital.nhs.uk/data-and-information/publications/statistical/quality-and-outcomes-framework-achievement-prevalence-and-exceptions-data/quality-and-outcomes-framework-2011-12
- Jacomelli J ,
- Summers L ,
- Stevenson A ,
- Earnshaw JJ
- Vahanian A ,
- Beyersdorf F ,
- ESC/EACTS Scientific Document Group
- Glikson M ,
- Nielsen JC ,
- Kronborg MB ,
- ESC Scientific Document Group
- Stevenson C ,
- Peeters A ,
- Federici M ,
- Schultz WM ,
- Verbakel JY ,
- Thygesen K ,
- Alpert JS ,
- Joint ESC/ACCF/AHA/WHF Task Force for the Redefinition of Myocardial Infarction
- Joint ESC/ACCF/AHA/WHF Task Force for the Universal Definition of Myocardial Infarction
- Sandoval Y ,
- High-STEACS investigators
- Camargo ECS ,
- Singhal AB ,
- Wiener RS ,
- Schwartz LM ,
- Sappler N ,
- Roalfe AK ,
- Lay-Flurrie SL ,
- Ordóñez-Mena JM ,
- Herbert A ,
- Wijlaars L ,
- Zylbersztejn A ,
- Cromwell D ,
- ↵ NHS Digital. Quality and Outcomes Framework (QOF), 2019-20. Indicator definitions. 2020. https://digital.nhs.uk/data-and-information/publications/statistical/quality-and-outcomes-framework-achievement-prevalence-and-exceptions-data/2019-20
- Pronovost PJ
- Thomas SL ,
- Schoonen WM ,
Main navigation
- Our Articles
- Dr. Joe's Books
- Media and Press
- Our History
- Public Lectures
- Past Newsletters
- Photo Gallery: The McGill OSS Separates 25 Years of Separating Sense from Nonsense
Subscribe to the OSS Weekly Newsletter!
The blood microbiome is probably not real.

- Add to calendar
- Tweet Widget
Up until recently, if bacteria were detected in your blood you would be in a world of trouble. Blood was long considered to be sterile, meaning free of viable microorganisms like bacteria. When disease-causing bacteria spread to the blood, they can cause a life-threatening septic shock.
But the use of DNA sequencing technology has allowed researchers to more easily detect something that had been reported as early as the late 1960s: bacteria can be found in the blood and not cause disease.
As we begin to map out and understand the complex microbial ecosystem that lives in our gut and elsewhere in the body, we contemplate an important question: is there such a thing as a blood microbiome?
Detecting a fingerprint in the blood
Our large intestine is not sterile; it is teeming with bacteria. But there are parts of the body that were long thought to be devoid of microorganisms. The brain. Bones. A variety of internal fluids, like our synovial fluid and peritoneal fluid. And, importantly, the blood.
Blood is made up of a liquid called plasma filled with red blood cells, whose main function is to carry oxygen to our cells. It also transports white blood cells, important to monitor for and fight off infections, as well as platelets, involved in clotting.
In the 1960s, a team of Italian researchers published multiple papers describing “mycoplasm-like forms”—meaning shapes that look like a particular type of bacteria that often contaminate cells cultured in the lab—in the blood of healthy people. This finding was confirmed in 1977 by a different team, which reported that four out of the 60 blood samples they had drawn from healthy volunteers showed bacteria growing in them. These types of tests, however, were rudimentary compared to what we have access to now. In the 2000s, they were mostly supplanted by DNA testing.
While we can sequence the entire DNA of any bacteria found in the blood, the technique most often used is 16S rRNA gene sequencing. I have always admired physicists’ penchant for quirky names: gluons, neutrinos, and charm quarks. Molecular biologists, by comparison, tend to be more sober. Yes, we have genes like Sonic hedgehog and proteins called scramblases; usually, though, we have to contend with the dryness of “16S rRNA.” You see, RNA is a molecule with many uses. Messenger RNA (or mRNA) acts as a disposable copy of a gene, a template for the production of a specific protein. Transfer RNA (or tRNA) actually brings the building blocks of a protein to where they are being assembled. And ribosomal RNA (or rRNA) is the main component of the giant protein factories in our cells known as ribosomes. One of its subunits is made up of, among others, a particular string of RNA known as the 16S rRNA.
The cool thing about the gene that codes for this 16S rRNA molecule is that it is very old and it mutates at a slow rate. By reading its precise sequence, scientists can tell which species it belongs to. Most of the studies of the putative blood microbiome use this technique to tell which species of bacteria are present in the blood being tested. The limitation of this test, however, is that dead bacteria have DNA too. The fact that DNA from the 16S rRNA gene of a precise bacterial species was detected in someone’s blood does not mean these bacteria were alive. For there to be a microbiome in the blood, these microorganisms need to live.
Which brings us to another important point of discussion. In order for scientists to agree that a blood microbiome exists, they first need to decide on the definition of a microbiome, and this is still a point of contention. In 2020, while companies were more than happy to sell hyped-up services testing your gut microbiome and claiming to interpret what it meant for your health, actual experts in the field met to agree on just what the word meant. “We are lacking,” they wrote , “a clear commonly agreed definition of the term ‘microbiome’.” For example, do viruses qualify? A microbiome implies life but viruses live on the edge, pun intended: they have the genetic blueprint for life yet they cannot reproduce on their own.
These experts proposed that the word “microbiome” should refer to the sum of two things: the microbiota, meaning the living microorganisms themselves, and their theatre of activity. It’s like saying that the Earth is not simply the life forms it houses, but also all of their individual components, and the traces they leave behind, and the environmental conditions in which they thrive or die. The microbiome is made up of bacteria and other microorganisms, yes, but also their proteins, lipids, sugars, and DNA and RNA molecules, as well as the signalling molecules and toxins that get exchanged within their theatre. (This is where viruses were sorted, by the way: not as part of the living microbiota but as belonging to the theatre of activity of the microbiome.)
The microbiome is a community, and this community has a distinct habitat.
So, what does the evidence say? Is our blood truly host to a thriving community of microorganisms or is something else going on?
Transient and sporadic
Initial studies of the alleged blood microbiome were small . The amounts of bacteria that were being reported based on DNA sequencing were tiny. If this microbiome existed, it seemed sparse, more “asteroid field in real life” than “asteroid field in the movies.”
An issue looming over this early research is that of contamination. If bacteria are detected in a blood sample, were they really in the blood… or did they contaminate supplies along the way? When blood is drawn, the skin, which has its own microbiome, is punctured. The area is usually swabbed with alcohol to kill bacteria, and the supplies used should be sterile, but suffice to say that from the blood draw to the DNA extraction to the DNA amplification to the sequencing of this DNA, bacteria can be introduced into the system. In fact, it is such common knowledge that certain bacteria are found inside of the laboratory kits used by scientists that this ecosystem has its own name: the kitome. One way to rule out these contaminants is to simultaneously run negative controls alongside samples every step of the way, to make sure that these negative controls are indeed free of bacteria. But early papers rarely reported when controls were used.
Last year, results from what purports to be the largest study ever into the question of whether the blood microbiome exists were published in Nature Microbiology . A total of 9,770 healthy individuals were tested. The conclusion? Yes, some bacteria could be found in their blood, but the evidence contradicted the claim of an ecosystem. In 84% of the samples tested, no bacteria were detected. In most of the other samples, only one species was found. In an ecosystem, you would expect to see species appearing together repeatedly, but this was not the case here. And the species they found most often in their samples were known to contaminate these types of laboratory experiments.
So, what were the few bacteria found in the blood and not recognized as contaminants doing there in the first place if they were not part of a healthy microbiome? The authors lean toward an alternative explanation that had been floated for many years: these bacteria are transient. They end up in the blood from other parts of the body, either because of some minor leak or through their active transportation into the blood by agents such as dendritic cells. Like pedestrians wandering off onto the highway, these bacteria do not normally live in the blood but they can be seen there when we look at the right moment.
Putting the diagnostic cart before the horse
This blood microbiome story could end here and simply be an interesting example of scientific research homing in on a curious finding, testing a hypothesis, and ultimately refuting it (or at the very least providing strong evidence against it). But given the incentives of modern research and the social-media spotlight cast on the academic literature, there are two slightly worrying angles here that merit discussion.
Scientists are more and more incentivized to find practical applications for their research. It’s not enough, for example, to study bacteria that survive at incredibly high temperatures; we must be assured that the DNA replication enzyme these bacteria possess will one day be used in laboratories all over the world to conduct research, identify criminals, and test samples for the presence of a pandemic-causing coronavirus.
In researching this topic, I came across many papers claiming the existence of “blood microbiome signatures” for certain diseases that are not known to be infectious. We are thus not talking about infections leaking in the blood and causing sepsis. I saw reports of signatures for cardiovascular disease , liver disease , heart attacks , even for gastrointestinal disease in dogs . The idea is that these signatures could soon be turned into (profitable) diagnostic tests. The problem, of course, is that these studies are based on the hypothesis that a blood microbiome is real; that its equilibrium can be affected by disease; and that these changes can be reliably detected and interpreted.
But if the blood microbiome is imaginary, we are just chasing ghosts. This is not unlike the time that scientists were publishing signatures of microRNAs in the blood for every possible cancer. When I looked at the published literature in grad school, I realized that the multiple signatures reported for a single cancer barely overlapped . They were just chance findings. Compare enough variables in a small sample set and you will find what appears to be an association.
My second concern is that the transitory leakage of bacteria into the blood, as evidenced by the recent Nature Microbiology paper, will be used as confirmation of a pseudoscientific entity: leaky gut syndrome. At the end of their paper, the researchers hypothesize that these bacteria end up in the blood because the integrity of certain barriers in the body are compromised during disease or during periods of stress. The “net” in our gut gets a bit porous, and some of our colon’s bacteria end up in circulation, though they are not causing disease as far as we can tell. A form of leaky gut is known to exist in certain intestinal diseases , likely to be a consequence and not a cause. But leaky gut syndrome, favoured by non-evidence-based practitioners, does not appear to be real, yet many websites portray it as the one true cause of all diseases, a real epidemic. Nuanced scientific findings have a history of being stolen, distorted, and toyed with by fake doctors to give credence to their pet theories. Though I have yet to see examples of it, I suspect work done on this hypothesized blood microbiome will similarly get weaponized.
You have been warned.
Take-home message: - Our blood was long considered to be sterile, meaning free of viable microbes, unless a dangerous infection leaked into it, causing sepsis - Studies have provided evidence for the presence of bacteria in the blood of some healthy humans, leading to the hypothesis that, much like in our gut, our blood is host to a microbiome - The largest study ever done on the topic provided strong evidence against this hypothesis. It seems that when non-disease-causing bacteria find themselves in our blood, it is temporary and occasional
@CrackedScience
What to read next
The story linking nutrition and health has unexpected twists 28 jun 2024.

A Taste of Bitter Melon 28 Jun 2024

Your Appendix May Not Be Useless After All 21 Jun 2024

On the Trail of Chemtrail Nonsense 21 Jun 2024

Facial Creams and Lotions Offer Hope in a Jar 14 Jun 2024
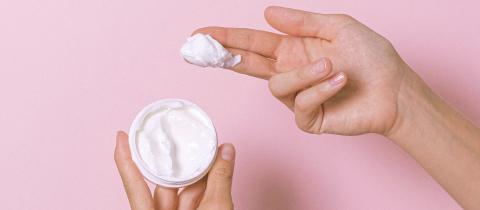
Protect Yourself Against Sunscreen Myths 14 Jun 2024

Department and University Information
Office for science and society.
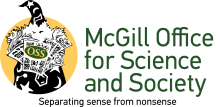
Numbers, Facts and Trends Shaping Your World
Read our research on:
Full Topic List
Regions & Countries
- Publications
- Our Methods
- Short Reads
- Tools & Resources
Read Our Research On:
Political Typology Quiz
Where do you fit in the political typology, are you a faith and flag conservative progressive left or somewhere in between.
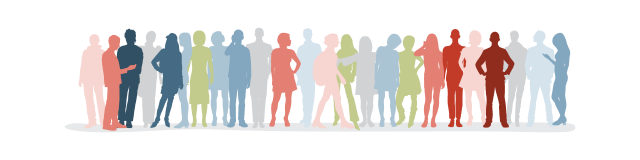
Take our quiz to find out which one of our nine political typology groups is your best match, compared with a nationally representative survey of more than 10,000 U.S. adults by Pew Research Center. You may find some of these questions are difficult to answer. That’s OK. In those cases, pick the answer that comes closest to your view, even if it isn’t exactly right.
Sign up for The Briefing
Weekly updates on the world of news & information
1615 L St. NW, Suite 800 Washington, DC 20036 USA (+1) 202-419-4300 | Main (+1) 202-857-8562 | Fax (+1) 202-419-4372 | Media Inquiries
Research Topics
- Email Newsletters
ABOUT PEW RESEARCH CENTER Pew Research Center is a nonpartisan fact tank that informs the public about the issues, attitudes and trends shaping the world. It conducts public opinion polling, demographic research, media content analysis and other empirical social science research. Pew Research Center does not take policy positions. It is a subsidiary of The Pew Charitable Trusts .
© 2024 Pew Research Center
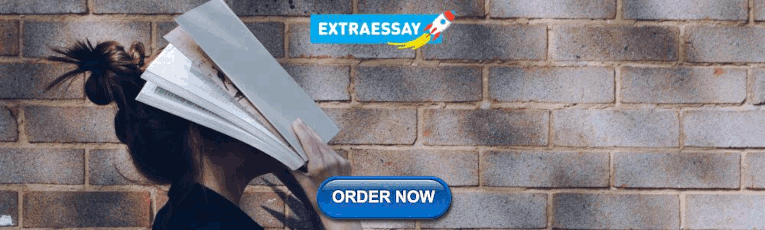
IMAGES
VIDEO
COMMENTS
The discussion section is where you delve into the meaning, importance, and relevance of your results.. It should focus on explaining and evaluating what you found, showing how it relates to your literature review and paper or dissertation topic, and making an argument in support of your overall conclusion.It should not be a second results section.. There are different ways to write this ...
The discussion section is often considered the most important part of your research paper because it: Most effectively demonstrates your ability as a researcher to think critically about an issue, to develop creative solutions to problems based upon a logical synthesis of the findings, and to formulate a deeper, more profound understanding of the research problem under investigation;
The discussion reviews the findings and puts them into the context of the overall research. It brings together all the sections that came before it and allows a reader to see the connections between each part of the research paper. In a discussion section, the author engages in three necessary steps: interpretation, analysis, and explanation.
Begin with a clear statement of the principal findings. This will reinforce the main take-away for the reader and set up the rest of the discussion. Explain why the outcomes of your study are important to the reader. Discuss the implications of your findings realistically based on previous literature, highlighting both the strengths and ...
The discussion section provides an analysis and interpretation of the findings, compares them with previous studies, identifies limitations, and suggests future directions for research. This section combines information from the preceding parts of your paper into a coherent story. By this point, the reader already knows why you did your study ...
1.Introduction—mention gaps in previous research¹⁻². 2. Summarizing key findings—let your data speak¹⁻². 3. Interpreting results—compare with other papers¹⁻². 4. Addressing limitations—their potential impact on the results¹⁻². 5. Implications for future research—how to explore further¹⁻².
This section is often considered the most important part of a research paper because it most effectively demonstrates your ability as a researcher to think critically about an issue, to develop creative solutions to problems based on the findings, and to formulate a deeper, more profound understanding of the research problem you are studying.. The discussion section is where you explore the ...
Papers usually end with a concluding section, often called the "Discussion.". The Discussion is your opportunity to evaluate and interpret the results of your study or paper, draw inferences and conclusions from it, and communicate its contributions to science and/or society. Use the present tense when writing the Discussion section.
Papers that are submitted to a journal for publication are sent out to several scientists (peers) who look carefully at the paper to see if it is "good science". These reviewers then recommend to the editor of a journal whether or not a paper should be published. Most journals have publication guidelines. Ask for them and follow them exactly.
Discussion is mainly the section in a research paper that makes the readers understand the exact meaning of the results achieved in a study by exploring the significant points of the research, its ...
The discussion section can be written in 3 parts: an introductory paragraph, intermediate paragraphs and a conclusion paragraph. For intermediate paragraphs, a "divide and conquer" approach, meaning a full paragraph describing each of the study endpoints, can be used. In conclusion, academic writing is similar to other skills, and practice ...
Begin the Discussion section by restating your statement of the problem and briefly summarizing the major results. Do not simply repeat your findings. Rather, try to create a concise statement of the main results that directly answer the central research question that you stated in the Introduction section.
The discussion section of a research paper is where the author analyzes and explains the importance of the study's results. It presents the conclusions drawn from the study, compares them to previous research, and addresses any potential limitations or weaknesses. The discussion section should also suggest areas for future research.
A discussion critically analyses and interprets the results of a scientific study, placing the results in the context of published literature and explaining how they affect the field.. In this section, you will relate the specific findings of your research to the wider scientific field. This is the opposite of the introduction section, which starts with the broader context and narrows to focus ...
Step 4: Acknowledge the limitations of your study. The fourth step in writing up your discussion chapter is to acknowledge the limitations of the study. These limitations can cover any part of your study, from the scope or theoretical basis to the analysis method (s) or sample.
An example of research summary in discussion. 3.2. An example of result interpretation in discussion. 3.3. An example of literature comparison in discussion. 3.4. An example of research implications in discussion. 3.5. An example of limitations in discussion.
After a research article has presented the substantive background, the methods and the results, the discussion section assesses the validity of results and draws conclusions by interpreting them. The discussion puts the results into a broader context and reflects their implications for theoretical (e.g. etiological) and practical (e.g ...
Discussion Section. The overall purpose of a research paper's discussion section is to evaluate and interpret results, while explaining both the implications and limitations of your findings. Per APA (2020) guidelines, this section requires you to "examine, interpret, and qualify the results and draw inferences and conclusions from them ...
Table of contents. What not to include in your discussion section. Step 1: Summarise your key findings. Step 2: Give your interpretations. Step 3: Discuss the implications. Step 4: Acknowledge the limitations. Step 5: Share your recommendations. Discussion section example.
The Discussion section might well be the hardest part of a paper to write. Yet, so useful! In a great Discussion section, the authors tie the different findings of the paper together, analyse them in the context of existing literature, offer speculations, suggest further research and highlight the study's significance and possible impact.
The discussion section, a systematic critical appraisal of results, is a key part of a research paper, wherein the authors define, critically examine, describe and interpret their findings ...
Purpose. The Discussion is the part of your paper where you can share what you think your results mean with respect to the big questions you posed in your Introduction. The Introduction and Discussion are natural partners: the Introduction tells the reader what question you are working on and why you did this experiment to investigate it; the ...
PPOG 500 R ESEARCH P APER T OPIC S ELECTION Topic A: Immigration Policy Discussion I have selected Topic A on immigration policy to research for my course research paper. Illegal immigration is a complex issue that involves balancing national security, domestic policy, and humanitarian concerns (Massey et al., 2016). This policy dilemma has sparked heated political debates and legislative ...
The discussion section is often considered the most important part of your research paper because this is where you: Most effectively demonstrates your ability as a researcher to think critically about an issue, to develop creative solutions to problems based upon a logical synthesis of the findings, and to formulate a deeper, more profound understanding of the research problem under ...
At baseline, the mean AHI was 51.5 events per hour in trial 1 and 49.5 events per hour in trial 2, and the mean body-mass index (BMI, the weight in kilograms divided by the square of the height in ...
where l ∈ {0, 1, 2, ⋯ , L} is the index of the block, σ denotes the activation function (SiLU in this paper), W is the learnable weight matrix, ⊙ represents the Hadamard product, ϕ( ⋅ ...
A halting debate performance by President Biden left Democratic strategists reeling, raising questions about his fitness to stay in the race.
Objective To investigate the incidence of cardiovascular disease (CVD) overall and by age, sex, and socioeconomic status, and its variation over time, in the UK during 2000-19. Design Population based study. Setting UK. Participants 1 650 052 individuals registered with a general practice contributing to Clinical Practice Research Datalink and newly diagnosed with at least one CVD from 1 ...
Up until recently, if bacteria were detected in your blood you would be in a world of trouble. Blood was long considered to be sterile, meaning free of viable microorganisms like bacteria. When disease-causing bacteria spread to the blood, they can cause a life-threatening septic shock. But the use of DNA sequencing technology has allowed researchers to more easily detect something that had ...
Take our quiz to find out which one of our nine political typology groups is your best match, compared with a nationally representative survey of more than 10,000 U.S. adults by Pew Research Center. You may find some of these questions are difficult to answer. That's OK.