text mining Recently Published Documents
Total documents.
- Latest Documents
- Most Cited Documents
- Contributed Authors
- Related Sources
- Related Keywords
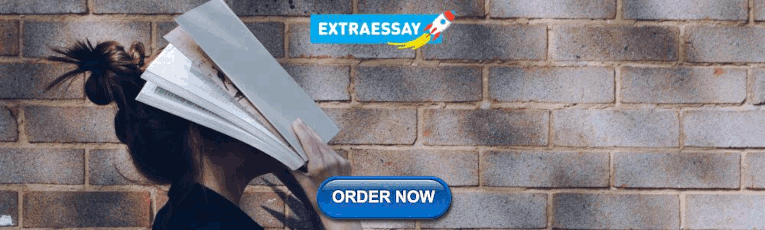
Automated Text Classification of Maintenance Data of Higher Education Buildings Using Text Mining and Machine Learning Techniques
Evaluation of the synergy degree of industrial de-capacity policies based on text mining: a case study of china's coal industry, application of informetrics on financial network text mining based on affective computing, recycling behaviour: mapping knowledge domain through bibliometrics and text mining, penerapan text mining untuk melakukan clustering data tweet akun blibli pada media sosial twitter menggunakan k-means clustering.
Social media is computer-based technology that facilitates the sharing of ideas, thoughts, and information through the building of virtual networks and communities. Twitter is one of the most popular social media in Indonesia which has 78 million users. Businesses rely heavily on Twitter for advertising. Businesses can use these types of tweet content as a means of advertising to Twitter users by Knowing the types of tweet content that are mostly retweeted by their followers . In this study, the application of Text Mining to perform clustering using the K-means clustering method with the best number of clusters obtained from the Silhouette Coefficient method on the @bliblidotcom Twitter tweet data to determine the types of tweet content that are mostly retweeted by @bliblidotcom followers. Tweets with the most retweets and favorites are discount offers and flash sales, so Blibli Indonesia could use this kind of tweet to conduct advertising on social media Twitter because the prize quiz tweets are liked by the @bliblidotcom Twitter account followers.
The Epilepsy Ontology: a community-based ontology tailored for semantic interoperability and text-mining
Abstract Motivation: Epilepsy is a multi-faceted complex disorder that requires a precise understanding of the classification, diagnosis, treatment, and disease mechanism governing it. Although scattered resources are available on epilepsy, comprehensive and structured knowledge is missing. In contemplation to promote multidisciplinary knowledge exchange and facilitate advancement in clinical management, especially in pre-clinical research, a disease-specific ontology is necessary. The presented ontology is designed to enable better interconnection between scientific community members in the epilepsy domain.Results: The Epilepsy Ontology (EPIO) is an assembly of structured knowledge on various aspects of epilepsy, developed according to Basic Formal Ontology (BFO) and Open Biological and Biomedical Ontology (OBO) Foundry principles. Concepts and definitions are collected from the latest International League against Epilepsy (ILAE) classification, domain-specific ontologies, and scientific literature. This ontology consists of 1,879 classes and 28,151 axioms (2,171 declaration axioms, 2,219 logical axioms) from several aspects of epilepsy. This ontology is intended to be used for data management and text mining purposes.
ANALISIS KECENDERUNGAN LAPORAN MASYARAKAT PADA “LAPORGUB..!” PROVINSI JAWA TENGAH MENGGUNAKAN TEXT MINING DENGAN FUZZY C-MEANS CLUSTERING
Effective communication between the government and society is essential to achieve good governance. The government makes an effort to provide a means of public complaints through an online aspiration and complaint service called “LaporGub..!”. To group incoming reports easier, the topic of the report is searched by using clustering. Text Mining is used to convert text data into numeric data so that it can be processed further. Clustering is classified as soft clustering (fuzzy) and hard clustering. Hard clustering will divide data into clusters strictly without any overlapping membership with other clusters. Soft clustering can enter data into several clusters with a certain degree of membership value. Different membership values make fuzzy grouping have more natural results than hard clustering because objects at the boundary between several classes are not forced to fully fit into one class but each object is assigned a degree of membership. Fuzzy c-means has an advantage in terms of having a more precise placement of the cluster center compared to other cluster methods, by improving the cluster center repeatedly. The formation of the best number of clusters is seen based on the maximum silhouette coefficient. Wordcloud is used to determine the dominant topic in each cluster. Word cloud is a form of text data visualization. The results show that the maximum silhouette coefficient value for fuzzy c-means clustering is shown by the three clusters. The first cluster produces a word cloud regarding road conditions as many as 449 reports, the second cluster produces a word cloud regarding covid assistance as many as 964 reports, and the third cluster produces a word cloud regarding farmers fertilizers as many as 176 reports. The topic of the report regarding covid assistance is the cluster with the most number of members.
Text visualization for geological hazard documents via text mining and natural language processing
Analysis of sebaceous gland carcinoma associated genes using network analysis to identify potentially actionable genes.
Eyelid sebaceous gland carcinoma (SGC) is a rare but life-threatening condi-tion. However, there is limited computational research associated with un-derlying protein interactions specific to eyelid sebaceous gland carcinoma. The aim of our study is to identify and analyse the genes associated with eyelid sebaceous gland carcinoma using text mining and to develop a protein-protein interaction network to predict significant biological pathways using bioinformatics tool. Genes associated with eyelid sebaceous gland carcinoma were retrieved from the PubMed database using text mining with key terms ‘eyelid’, ‘sebaceous gland carcinoma’ and excluding the genes for ‘Muir-Torre Syndrome’. The interaction partners were identified using STRING. Cytoscape was used for visualization and analysis of the PPI network. Molec-ular complexes in the network were predicted using MCODE plug-in and ana-lyzed for gene ontology terms using DAVID. PubMed retrieval process identi-fied 79 genes related to eyelid sebaceous gland carcinoma. The PPI network associated with eyelid sebaceous gland carcinoma produced 79 nodes, 1768 edges. Network analysis using Cytoscape identified nine key genes and two molecular complexes to be enriched in the protein-protein interaction net-work. GO enrichment analysis identified biological processes cell fate com-mitment, Wnt signalling pathway, retinoic acid signalling and response to cytokines to be enriched in our network. Genes identified in the study might play a pivotal role in understanding the underlying molecular pathways in-volved in the development and progression of eyelid sebaceous gland carci-noma. Furthermore, it may aid in the identification of candidate biomarkers and therapeutic targets in the treatment of eyelid sebaceous gland carcino-ma.
Determining banking service attributes from online reviews: text mining and sentiment analysis
PurposeThe current study employs text mining and sentiment analysis to identify core banking service attributes and customer sentiment in online user-generated reviews. Additionally, the study explains customer satisfaction based on the identified predictors.Design/methodology/approachA total of 32,217 customer reviews were collected across 29 top banks on bankbazaar.com posted from 2014 to 2021. In total three conceptual models were developed and evaluated employing regression analysis.FindingsThe study revealed that all variables were found to be statistically significant and affect customer satisfaction in their respective models except the interest rate.Research limitations/implicationsThe study is confined to the geographical representation of its subjects' i.e. Indian customers. A cross-cultural and socioeconomic background analysis of banking customers in different countries may help to better generalize the findings.Practical implicationsThe study makes essential theoretical and managerial contributions to the existing literature on services, particularly the banking sector.Originality/valueThis paper is unique in nature that focuses on banking customer satisfaction from online reviews and ratings using text mining and sentiment analysis.
Export Citation Format
Share document.

- Open access
- Published: 29 June 2017
Text mining and semantics: a systematic mapping study
- Roberta Akemi Sinoara ORCID: orcid.org/0000-0001-8572-2747 1 ,
- João Antunes 1 &
- Solange Oliveira Rezende 1
Journal of the Brazilian Computer Society volume 23 , Article number: 9 ( 2017 ) Cite this article
15k Accesses
30 Citations
1 Altmetric
Metrics details
As text semantics has an important role in text meaning, the term semantics has been seen in a vast sort of text mining studies. However, there is a lack of studies that integrate the different research branches and summarize the developed works. This paper reports a systematic mapping about semantics-concerned text mining studies. This systematic mapping study followed a well-defined protocol. Its results were based on 1693 studies, selected among 3984 studies identified in five digital libraries. The produced mapping gives a general summary of the subject, points some areas that lacks the development of primary or secondary studies, and can be a guide for researchers working with semantics-concerned text mining. It demonstrates that, although several studies have been developed, the processing of semantic aspects in text mining remains an open research problem.
Introduction
Text mining techniques have become essential for supporting knowledge discovery as the volume and variety of digital text documents have increased, either in social networks and the Web or inside organizations. Text sources, as well as text mining applications, are varied. Although there is not a consensual definition established among the different research communities [ 1 ], text mining can be seen as a set of methods used to analyze unstructured data and discover patterns that were unknown beforehand [ 2 ].
A general text mining process can be seen as a five-step process, as illustrated in Fig. 1 . The process starts with the specification of its objectives in the problem identification step. The text mining analyst, preferably working along with a domain expert, must delimit the text mining application scope, including the text collection that will be mined and how the result will be used. The specifications stated in the problem identification step will guide the next steps of the text mining process, which can be executed in cycles of data preparation (pre-processing step), knowledge discovery (pattern extraction step), and knowledge evaluation (post-processing step).
A general text mining process
The pre-processing step is about preparing data for pattern extraction. In this step, raw text is transformed into some data representation format that can be used as input for the knowledge extraction algorithms. The activities performed in the pre-processing step are crucial for the success of the whole text mining process. The data representation must preserve the patterns hidden in the documents in a way that they can be discovered in the next step. In the pattern extraction step, the analyst applies a suitable algorithm to extract the hidden patterns. The algorithm is chosen based on the data available and the type of pattern that is expected. The extracted knowledge is evaluated in the post-processing step. If this knowledge meets the process objectives, it can be put available to the users, starting the final step of the process, the knowledge usage. Otherwise, another cycle must be performed, making changes in the data preparation activities and/or in pattern extraction parameters. If any changes in the stated objectives or selected text collection must be made, the text mining process should be restarted at the problem identification step.
Text data are not naturally in a format that is suitable for the pattern extraction, which brings additional challenges to an automatic knowledge discovery process. The meaning of natural language texts basically depends on lexical, syntactic, and semantic levels of linguistic knowledge. Each level is more complex and requires a more sophisticated processing than the previous level. This is a common trade-off when dealing with natural language processing: expressiveness versus processing cost. Thus, lexical and syntactic components have been more broadly explored in text mining than the semantic component [ 2 ]. Recently, text mining researchers have become more interested in text semantics, looking for improvements in the text mining results. The reason for this increasing interest can be assigned both to the progress of the computing capacity, which is constantly reducing the processing time, and to developments in the natural language processing field, which allow a deeper processing of raw texts.
In order to compare the expressiveness of each level of text interpretation (lexical, syntactic, and semantic), consider two simple sentences:
Company A acquired Company B.
Company B acquired Company A.
Sentences 1 and 2 have opposite meanings, but they have the same terms (“Company”, “A”, “B”, “acquired”). Thus, if we analyze these sentences only in the lexical level, it is not possible to differentiate them. However, considering the sentence syntax, we can see that they are opposite. They have the same verb, and the subject of one sentence is the object of the other sentence and vice versa. If we analyze a little deeper, now considering the sentence semantics, we find that in sentence 1, “Company A” has the semantic role of agent regarding the verb “acquire” and “Company B” has the semantic role of theme . The same can be said to a third sentence:
Company B was acquired by Company A.
Despite the fact that syntactically sentences 1 and 3 have opposite subjects and objects, they have the same semantic roles. Thus, at the semantic level, they have the same meaning. If we go deeper and consider semantic relations among words (as the synonymy, for example), we can find that sentence 4 also expresses the same event:
Company A purchased Company B.
Besides, going even deeper in the interpretation of the sentences, we can understand their meaning—they are related to some takeover—and we can, for example, infer that there will be some impacts on the business environment.
Traditionally, text mining techniques are based on both a bag-of-words representation and application of data mining techniques. In this approach, only the lexical component of the texts are considered. In order to get a more complete analysis of text collections and get better text mining results, several researchers directed their attention to text semantics.
Text semantics can be considered in the three main steps of text mining process: pre-processing, pattern extraction and post-processing. In the pre-processing step, data representation can be based on some sort of semantic aspect of the text collection. In the pattern extraction, semantic information can be used to guide the model generation or to refine it. In the post-processing step, the extracted patterns can be evaluated based on semantic aspects. Either way, text mining based on text semantics can go further than text mining based only on lexicon or syntax. A proper treatment of text semantics can lead to more appropriate results for certain applications [ 2 ]. For example, semantic information has an important impact on document content and can be crucial to differentiate documents which, despite the use of the same vocabulary, present different ideas about the same subject.
The term semantics has been seen in a vast sort of text mining studies. However, there is a lack of studies that integrate the different branches of research performed to incorporate text semantics in the text mining process. Secondary studies, such as surveys and reviews, can integrate and organize the studies that were already developed and guide future works.
Thus, this paper reports a systematic mapping study to overview the development of semantics-concerned studies and fill a literature review gap in this broad research field through a well-defined review process. Semantics can be related to a vast number of subjects, and most of them are studied in the natural language processing field. As examples of semantics-related subjects, we can mention representation of meaning, semantic parsing and interpretation, word sense disambiguation, and coreference resolution. Nevertheless, the focus of this paper is not on semantics but on semantics-concerned text mining studies. As the term semantics appears in text mining studies in different contexts, this systematic mapping aims to present a general overview and point some areas that lack the development of primary studies and those areas that secondary studies would be of great help. This paper aims to point some directions to the reader who is interested in semantics-concerned text mining researches.
As it covers a wide research field, this systematic mapping study started with a space of 3984 studies, identified in five digital libraries. Due to time and resource limitations, except for survey papers, the mapping was done primarily through information found in paper abstracts. Therefore, our intention is to present an overview of semantics-concerned text mining, presenting a map of studies that has been developed by the research community, and not to present deep details of the studies. The papers were analyzed in relation to their application domains, performed tasks, applied methods and resources, and level of user’s interaction. The contribution of this paper is threefold: (i) it presents an overview of semantics-concerned text mining studies from a text mining viewpoint, organizing the studies according to seven aspects (application domains, languages, external knowledge sources, tasks, methods and algorithms, representation models, and user’s interaction); (ii) it quantifies and confirms some previous feelings that we had about our study subject; and (iii) it provides a starting point for those, researchers or practitioners, who are initiating works on semantics-concerned text mining.
The remainder of this paper is organized as follows. The “ Method applied for systematic mapping ” section presents an overview of systematic mapping method, since this is the type of literature review selected to develop this study and it is not widespread in the text mining community. In this section, we also present the protocol applied to conduct the systematic mapping study, including the research questions that guided this study and how it was conducted. The results of the systematic mapping, as well as identified future trends, are presented in the “ Results and discussion ” section. The “ Conclusion ” section concludes this work.
Method applied for systematic mapping
The review reported in this paper is the result of a systematic mapping study, which is a particular type of systematic literature review [ 3 , 4 ]. Systematic literature review is a formal literature review adopted to identify, evaluate, and synthesize evidences of empirical results in order to answer a research question. It is extensively applied in medicine, as part of the evidence-based medicine [ 5 ]. This type of literature review is not as disseminated in the computer science field as it is in the medicine and health care fields 1 , although computer science researches can also take advantage of this type of review. We can find important reports on the use of systematic reviews specially in the software engineering community [ 3 , 4 , 6 , 7 ]. Other sparse initiatives can also be found in other computer science areas, as cloud-based environments [ 8 ], image pattern recognition [ 9 ], biometric authentication [ 10 ], recommender systems [ 11 ], and opinion mining [ 12 ].
A systematic review is performed in order to answer a research question and must follow a defined protocol. The protocol is developed when planning the systematic review, and it is mainly composed by the research questions, the strategies and criteria for searching for primary studies, study selection, and data extraction. The protocol is a documentation of the review process and must have all the information needed to perform the literature review in a systematic way. The analysis of selected studies, which is performed in the data extraction phase, will provide the answers to the research questions that motivated the literature review. Kitchenham and Charters [ 3 ] present a very useful guideline for planning and conducting systematic literature reviews. As systematic reviews follow a formal, well-defined, and documented protocol, they tend to be less biased and more reproducible than a regular literature review.
When the field of interest is broad and the objective is to have an overview of what is being developed in the research field, it is recommended to apply a particular type of systematic review named systematic mapping study [ 3 , 4 ]. Systematic mapping studies follow an well-defined protocol as in any systematic review. The main differences between a traditional systematic review and a systematic mapping are their breadth and depth. While a systematic review deeply analyzes a low number of primary studies, in a systematic mapping a wider number of studies are analyzed, but less detailed. Thus, the search terms of a systematic mapping are broader and the results are usually presented through graphs. Systematic mapping studies can be used to get a mapping of the publications about some subject or field and identify areas that require the development of more primary studies and areas in which a narrower systematic literature review would be of great help to the research community.
This paper reports a systematic mapping study conducted to get a general overview of how text semantics is being treated in text mining studies. It fills a literature review gap in this broad research field through a well-defined review process. As a systematic mapping, our study follows the principles of a systematic mapping/review. However, as our goal was to develop a general mapping of a broad field, our study differs from the procedure suggested by Kitchenham and Charters [ 3 ] in two ways. Firstly, Kitchenham and Charters [ 3 ] state that the systematic review should be performed by two or more researchers. Although our mapping study was planned by two researchers, the study selection and the information extraction phases were conducted by only one due to the resource constraints. In this process, the other researchers reviewed the execution of each systematic mapping phase and their results. Secondly, systematic reviews usually are done based on primary studies only, nevertheless we have also accepted secondary studies (reviews or surveys) as we want an overview of all publications related to the theme.
In the following subsections, we describe our systematic mapping protocol and how this study was conducted.
Systematic mapping planning
The first step of a systematic review or systematic mapping study is its planning. The researchers conducting the study must define its protocol, i.e., its research questions and the strategies for identification, selection of studies, and information extraction, as well as how the study results will be reported. The main parts of the protocol that guided the systematic mapping study reported in this paper are presented in the following.
Research question: the main research question that guided this study was “How is semantics considered in text mining studies?” The main question was detailed in seven secondary questions, all of them related to text mining studies that consider text semantics in some way:
What are the application domains that focus on text semantics?
What are the natural languages being considered when working with text semantics?
Which external sources are frequently used in text mining studies when text semantics is considered?
In what text mining tasks is the text semantics most considered?
What methods and algorithms are commonly used?
How can texts be represented?
Do users or domain experts take part in the text mining process?
Study identification: the study identification was performed through searches for studies conducted in five digital libraries: ACM Digital Library, IEEE Xplore, Science Direct, Web of Science, and Scopus. The following general search expression was applied in both Title and Keywords fields, when allowed by the digital library search engine: semantic* AND text* AND (mining OR representation OR clustering OR classification OR association rules) .
Study selection: every study returned in the search phase went to the selection phase. Studies were selected based on title, abstract, and paper information (as number of pages, for example). Through this analysis, duplicated studies (most of them were studies found in more than one database) were identified. Besides, studies which match at least one of the following exclusion criteria were rejected: (i) one page papers, posters, presentations, abstracts, and editorials; (ii) papers hosted in services with restricted access and not accessible; (iii) papers written in languages different from English or Portuguese; and (iv) studies that do not deal with text mining and text semantics.
Information extraction: the information extraction phase was performed with papers accepted in the selection phase (papers that were not identified as duplicated or rejected). The abstracts were read in order to extract the information presented in Fig. 2 .
Information extraction form
As any literature review, this study has some bias. The advantage of a systematic literature review is that the protocol clearly specifies its bias, since the review process is well-defined. There are bias related to (i) study identification, i.e., only papers matching the search expression and returned by the searched digital libraries were selected; (ii) selection criteria, i.e., papers that matches the exclusion criteria were rejected; and (iii) information extraction, i.e., the information were mainly extracted considering only title and abstracts. It is not feasible to conduct a literature review free of bias. However, it is possible to conduct it in a controlled and well-defined way through a systematic process.
Systematic mapping conduction
The conduction of this systematic mapping followed the protocol presented in the last subsection and is illustrated in Fig. 3 . The selection and the information extraction phases were performed with support of the Start tool [ 13 ].
Systematic mapping conduction phases. The numbers in the shaded areas indicate the quantity of studies involved
This paper reports the results obtained after the execution of two cycles of the systematic mapping phases. The first cycle was executed based on searches performed in January 2014. The second cycle was an update of the first cycle, with searches performed in February 2016 2 . A total of 3984 papers were found using the search expression in the five digital libraries. In the selection phase, 725 duplicated studies were identified and 1566 papers were rejected according to the exclusion criteria, mainly based on their title and abstract. Most of the rejected papers match the last exclusion criteria ( Studies that do not deal with text mining and text semantics ). Among them, we can find studies that deal with multimedia data (images, videos, or audio) and with construction, description, or annotation of corpus.
After the selection phase, 1693 studies were accepted for the information extraction phase. In this phase, information about each study was extracted mainly based on the abstracts, although some information was extracted from the full text. The results of the accepted paper mapping are presented in the next section.
Results and discussion
The mapping reported in this paper was conducted with the general goal of providing an overview of the researches developed by the text mining community and that are concerned about text semantics. This mapping is based on 1693 studies selected as described in the previous section. The distribution of these studies by publication year is presented in Fig. 4 . We can note that text semantics has been addressed more frequently in the last years, when a higher number of text mining studies showed some interest in text semantics. The peak was in 2011, with 223 identified studies. The lower number of studies in the year 2016 can be assigned to the fact that the last searches were conducted in February 2016.
Distribution of the 1693 accepted studies by publication year. Searches for studies identification were executed in January 2014 and February 2016
The results of the systematic mapping study is presented in the following subsections. We start our report presenting, in the “ Surveys ” section, a discussion about the eighteen secondary studies (surveys and reviews) that were identified in the systematic mapping. Then, each following section from “ Application domains ” to “ User’s interaction ” is related to a secondary research question that guided our study, i.e., application domains, languages, external knowledge sources, text mining tasks, methods and algorithms, representation model, and user’s interaction. In the “ Systematic mapping summary and future trends ” section, we present a consolidation of our results and point some gaps of both primary and secondary studies.
Some studies accepted in this systematic mapping are cited along the presentation of our mapping. We do not present the reference of every accepted paper in order to present a clear reporting of the results.
In this systematic mapping, we identified 18 survey papers associated to the theme text mining and semantics [ 14 – 31 ]. Each paper exploits some particularity of this broad theme. In the following, we present a short overview of these papers, which is based on the full text of the papers.
Grobelnik [ 14 ] presents, briefly but in a very clear form, an interesting discussion of text processing in his three-page paper. The author organizes the field in three main dimensions, which can be used to classify text processing approaches: representation, technique, and task. The task dimension is about the kind of problems, we solve through the text processing. Document search, clustering, classification, summarization, trend detection, and monitoring are examples of tasks. Considering how text representations are manipulated (technique dimension), we have the methods and algorithms that can be used, including machine learning algorithms, statistical analysis, part-of-speech tagging, semantic annotation, and semantic disambiguation. In the representation dimension, we can find different options for text representation, such as words, phrases, bag-of-words, part-of-speech, subject-predicate-object triples and semantically annotated triples.
Grobelnik [ 14 ] also presents the levels of text representations, that differ from each other by the complexity of processing and expressiveness. The most simple level is the lexical level, which includes the common bag-of-words and n-grams representations. The next level is the syntactic level, that includes representations based on word co-location or part-of-speech tags. The most complete representation level is the semantic level and includes the representations based on word relationships, as the ontologies. Several different research fields deal with text, such as text mining, computational linguistics, machine learning, information retrieval, semantic web and crowdsourcing. Grobelnik [ 14 ] states the importance of an integration of these research areas in order to reach a complete solution to the problem of text understanding.
Stavrianou et al. [ 15 ] present a survey of semantic issues of text mining, which are originated from natural language particularities. This is a good survey focused on a linguistic point of view, rather than focusing only on statistics. The authors discuss a series of questions concerning natural language issues that should be considered when applying the text mining process. Most of the questions are related to text pre-processing and the authors present the impacts of performing or not some pre-processing activities, such as stopwords removal, stemming, word sense disambiguation, and tagging. The authors also discuss some existing text representation approaches in terms of features, representation model, and application task. The set of different approaches to measure the similarity between documents is also presented, categorizing the similarity measures by type (statistical or semantic) and by unit (words, phrases, vectors, or hierarchies).
Stavrianou et al. [ 15 ] also present the relation between ontologies and text mining. Ontologies can be used as background knowledge in a text mining process, and the text mining techniques can be used to generate and update ontologies. The authors conclude the survey stating that text mining is an open research area and that the objectives of the text mining process must be clarified before starting the data analysis, since the approaches must be chosen according to the requirements of the task being performed.
Methods that deal with latent semantics are reviewed in the study of Daud et al. [ 16 ]. The authors present a chronological analysis from 1999 to 2009 of directed probabilistic topic models, such as probabilistic latent semantic analysis, latent Dirichlet allocation, and their extensions. The models are classified according to their main functionality. They describe their advantages, disadvantages, and applications.
Wimalasuriya and Dou [ 17 ], Bharathi and Venkatesan [ 18 ], and Reshadat and Feizi-Derakhshi [ 19 ] consider the use of external knowledge sources (e.g., ontology or thesaurus) in the text mining process, each one dealing with a specific task. Wimalasuriya and Dou [ 17 ] present a detailed literature review of ontology-based information extraction. The authors define the recent information extraction subfield, named ontology-based information extraction (OBIE), identifying key characteristics of the OBIE systems that differentiate them from general information extraction systems. Besides, they identify a common architecture of the OBIE systems and classify existing systems along with different dimensions, as information extraction method applied, whether it constructs and updates the ontology, components of the ontology, and type of documents the system deals with. Bharathi and Venkatesan [ 18 ] present a brief description of several studies that use external knowledge sources as background knowledge for document clustering. Reshadat and Feizi-Derakhshi [ 19 ] present several semantic similarity measures based on external knowledge sources (specially WordNet and MeSH) and a review of comparison results from previous studies.
Schiessl and Bräscher [ 20 ] and Cimiano et al. [ 21 ] review the automatic construction of ontologies. Schiessl and Bräscher [ 20 ], the only identified review written in Portuguese, formally define the term ontology and discuss the automatic building of ontologies from texts. The authors state that automatic ontology building from texts is the way to the timely production of ontologies for current applications and that many questions are still open in this field. Also, in the theme of automatic building of ontologies from texts, Cimiano et al. [ 21 ] argue that automatically learned ontologies might not meet the demands of many possible applications, although they can already benefit several text mining tasks. The authors divide the ontology learning problem into seven tasks and discuss their developments. They state that ontology population task seems to be easier than learning ontology schema tasks.
Jovanovic et al. [ 22 ] discuss the task of semantic tagging in their paper directed at IT practitioners. Semantic tagging can be seen as an expansion of named entity recognition task, in which the entities are identified, disambiguated, and linked to a real-world entity, normally using a ontology or knowledge base. The authors compare 12 semantic tagging tools and present some characteristics that should be considered when choosing such type of tools.
Specifically for the task of irony detection, Wallace [ 23 ] presents both philosophical formalisms and machine learning approaches. The author argues that a model of the speaker is necessary to improve current machine learning methods and enable their application in a general problem, independently of domain. He discusses the gaps of current methods and proposes a pragmatic context model for irony detection.
The application of text mining methods in information extraction of biomedical literature is reviewed by Winnenburg et al. [ 24 ]. The paper describes the state-of-the-art text mining approaches for supporting manual text annotation, such as ontology learning, named entity and concept identification. They also describe and compare biomedical search engines, in the context of information retrieval, literature retrieval, result processing, knowledge retrieval, semantic processing, and integration of external tools. The authors argue that search engines must also be able to find results that are indirectly related to the user’s keywords, considering the semantics and relationships between possible search results. They point that a good source for synonyms is WordNet.
Leser and Hakenberg [ 25 ] presents a survey of biomedical named entity recognition. The authors present the difficulties of both identifying entities (like genes, proteins, and diseases) and evaluating named entity recognition systems. They describe some annotated corpora and named entity recognition tools and state that the lack of corpora is an important bottleneck in the field.
Dagan et al. [ 26 ] introduce a special issue of the Journal of Natural Language Engineering on textual entailment recognition, which is a natural language task that aims to identify if a piece of text can be inferred from another. The authors present an overview of relevant aspects in textual entailment, discussing four PASCAL Recognising Textual Entailment (RTE) Challenges. They declared that the systems submitted to those challenges use cross-pair similarity measures, machine learning, and logical inference. The authors also describe tools, resources, and approaches commonly used in textual entailment tasks and conclude with the perspective that in the future, the constructed entailment “engines” will be used as a basic module by the text-understanding applications.
Irfan et al. [ 27 ] present a survey on the application of text mining methods in social network data. They present an overview of pre-processing, classification and clustering techniques to discover patterns from social networking sites. They point out that the application of text mining techniques can reveal patterns related to people’s interaction behaviors. The authors present two basic pre-processing activities: feature extraction and feature selection. The authors also review classification and clustering approaches. They present different machine learning algorithms and discuss the importance of ontology usage to introduce explicit concepts, descriptions, and the semantic relationships among concepts. Irfan et al. [ 27 ] identify the main challenges related to the manipulation of social network texts (such as large data, data with impurities, dynamic data, emotions interpretations, privacy, and data confidence) and to text mining infrastructure (such as usage of cloud computing and improvement of the usability of text mining methods).
In the context of semantic web, Sheth et al. [ 28 ] define three types of semantics: implicit semantics, formal semantics, and powerful (or soft) semantics. Implicit semantics are those implicitly present in data patterns and is not explicitly represented in any machine processable syntax. Machine learning methods exploit this type of semantics. Formal semantics are those represented in some well-formed syntactic form and are machine-processable. The powerful semantics are the sort of semantics that allow uncertainty (that is, the representation of degree of membership and degree of certainty) and, therefore, allowing abductive or inductive reasoning. The authors also correlates the types of semantics with some core capabilities required by a practical semantic web application. The authors conclude their review asserting the importance of focusing research efforts in representation mechanisms for powerful semantics in order to move towards the development of semantic applications.
The formal semantics defined by Sheth et al. [ 28 ] is commonly represented by description logics, a formalism for knowledge representation. The application of description logics in natural language processing is the theme of the brief review presented by Cheng et al. [ 29 ].
The broad field of computational linguistics is presented by Martinez and Martinez [ 30 ]. Considering areas of computational linguistics that can be interesting to statisticians, the authors describe three main aspects of computational linguistics: formal language, information retrieval, and machine learning. The authors present common models for knowledge representation, addressing their statistical characteristics and providing an overview of information retrieval and machine learning methods related to computational linguistics. They describe some of the major statistical contributions to the areas of machine learning and computational linguistics, from the point of view of classification and clustering algorithms. Martinez and Martinez [ 30 ] emphasize that machine translation, part-of-speech tagging, word sense disambiguation, and text summarization are some of the identified applications that statisticians can contribute.
Bos [ 31 ] presents an extensive survey of computational semantics, a research area focused on computationally understanding human language in written or spoken form. He discusses how to represent semantics in order to capture the meaning of human language, how to construct these representations from natural language expressions, and how to draw inferences from the semantic representations. The author also discusses the generation of background knowledge, which can support reasoning tasks. Bos [ 31 ] indicates machine learning, knowledge resources, and scaling inference as topics that can have a big impact on computational semantics in the future.
As presented in this section, the reviewed secondary studies exploit some specific issues of semantics-concerned text mining researches. In contrast to them, this paper reviews a broader range of text mining studies that deal with semantic aspects. To the best of our knowledge, this is the first report of a mapping of this field. We present the results of our systematic mapping study in the following sections, organized in seven dimensions of the text mining studies derived from our secondary research questions: application domains, languages, external knowledge usage, tasks, methods and algorithms, representation model, and user’s interaction.
Application domains
Research question:
Figure 5 presents the domains where text semantics is most present in text mining applications. Health care and life sciences is the domain that stands out when talking about text semantics in text mining applications. This fact is not unexpected, since life sciences have a long time concern about standardization of vocabularies and taxonomies. The building of taxonomies and ontologies is such a common practice in health care and life sciences that World Wide Web Consortium (W3C) has an interest group specific for developing, evaluating, and supporting semantic web technologies for this field [ 32 ]. Among the most common problems treated through the use of text mining in the health care and life science is the information retrieval from publications of the field. The search engine PubMed [ 33 ] and the MEDLINE database are the main text sources among these studies. There are also studies related to the extraction of events, genes, proteins and their associations [ 34 – 36 ], detection of adverse drug reaction [ 37 ], and the extraction of cause-effect and disease-treatment relations [ 38 – 40 ].
Application domains identified in the literature mapping accepted studies
The second most frequent identified application domain is the mining of web texts, comprising web pages, blogs, reviews, web forums, social medias, and email filtering [ 41 – 46 ]. The high interest in getting some knowledge from web texts can be justified by the large amount and diversity of text available and by the difficulty found in manual analysis. Nowadays, any person can create content in the web, either to share his/her opinion about some product or service or to report something that is taking place in his/her neighborhood. Companies, organizations, and researchers are aware of this fact, so they are increasingly interested in using this information in their favor. Some competitive advantages that business can gain from the analysis of social media texts are presented in [ 47 – 49 ]. The authors developed case studies demonstrating how text mining can be applied in social media intelligence. From our systematic mapping data, we found that Twitter is the most popular source of web texts and its posts are commonly used for sentiment analysis or event extraction.
Besides the top 2 application domains, other domains that show up in our mapping refers to the mining of specific types of texts. We found research studies in mining news, scientific papers corpora, patents, and texts with economic and financial content.
Whether using machine learning or statistical techniques, the text mining approaches are usually language independent. However, specially in the natural language processing field, annotated corpora is often required to train models in order to resolve a certain task for each specific language (semantic role labeling problem is an example). Besides, linguistic resources as semantic networks or lexical databases, which are language-specific, can be used to enrich textual data. Most of the resources available are English resources. Thus, the low number of annotated data or linguistic resources can be a bottleneck when working with another language. There are important initiatives to the development of researches for other languages, as an example, we have the ACM Transactions on Asian and Low-Resource Language Information Processing [ 50 ], an ACM journal specific for that subject.
In this study, we identified the languages that were mentioned in paper abstracts. The collected data are summarized in Fig. 6 . We must note that English can be seen as a standard language in scientific publications; thus, papers whose results were tested only in English datasets may not mention the language, as examples, we can cite [ 51 – 56 ]. Besides, we can find some studies that do not use any linguistic resource and thus are language independent, as in [ 57 – 61 ]. These facts can justify that English was mentioned in only 45.0% of the considered studies.
Languages identified in the literature mapping accepted studies
Chinese is the second most mentioned language (26.4% of the studies reference the Chinese language). Wu et al. [ 62 ] point two differences between English and Chinese: in Chinese, there are no white spaces between words in a sentence and there are a higher number of frequent words (the number of frequent words in Chinese is more than twice the number of English frequent words). These characteristics motivate the development of methods and experimental evaluations specifically for Chinese.
This mapping shows that there is a lack of studies considering languages other than English or Chinese. The low number of studies considering other languages suggests that there is a need for construction or expansion of language-specific resources (as discussed in “ External knowledge sources ” section). These resources can be used for enrichment of texts and for the development of language specific methods, based on natural language processing.
External knowledge sources
Text mining initiatives can get some advantage by using external sources of knowledge. Thesauruses, taxonomies, ontologies, and semantic networks are knowledge sources that are commonly used by the text mining community. Semantic networks is a network whose nodes are concepts that are linked by semantic relations. The most popular example is the WordNet [ 63 ], an electronic lexical database developed at the Princeton University. Depending on its usage, WordNet can also be seen as a thesaurus or a dictionary [ 64 ].
There is not a complete definition for the terms thesaurus, taxonomy, and ontology that is unanimously accepted by all research areas. Weller [ 65 ] presents an interesting discussion about the term ontology , including its origin and proposed definitions. She concluded the discussion stating that: “Ontologies should unambiguously represent shared background knowledge that helps people within a community of interest to understand each other. And they should make computer-readable indexing of information possible on the Web” [ 65 ]. The same can be said about thesauruses and taxonomies. In a general way, thesauruses, taxonomies, and ontologies are normally specialized in a specific domain and they usually differs from each other by their degree of expressiveness and complexity in their relational constructions [ 66 ]. Ontology would be the most expressive type of knowledge representation, having the most complex relations and formalized construction.
When looking at the external knowledge sources used in semantics-concerned text mining studies (Fig. 7 ), WordNet is the most used source. This lexical resource is cited by 29.9% of the studies that uses information beyond the text data. WordNet can be used to create or expand the current set of features for subsequent text classification or clustering. The use of features based on WordNet has been applied with and without good results [ 55 , 67 – 69 ]. Besides, WordNet can support the computation of semantic similarity [ 70 , 71 ] and the evaluation of the discovered knowledge [ 72 ].
External sources identified in the literature mapping accepted studies
The second most used source is Wikipedia [ 73 ], which covers a wide range of subjects and has the advantage of presenting the same concept in different languages. Wikipedia concepts, as well as their links and categories, are also useful for enriching text representation [ 74 – 77 ] or classifying documents [ 78 – 80 ]. Medelyan et al. [ 81 ] present the value of Wikipedia and discuss how the community of researchers are making use of it in natural language processing tasks (in special word sense disambiguation), information retrieval, information extraction, and ontology building.
The use of Wikipedia is followed by the use of the Chinese-English knowledge database HowNet [ 82 ]. Finding HowNet as one of the most used external knowledge source it is not surprising, since Chinese is one of the most cited languages in the studies selected in this mapping (see the “ Languages ” section). As well as WordNet, HowNet is usually used for feature expansion [ 83 – 85 ] and computing semantic similarity [ 86 – 88 ].
Web pages are also used as external sources [ 89 – 91 ]. Normally, web search results are used to measure similarity between terms. We also found some studies that use SentiWordNet [ 92 ], which is a lexical resource for sentiment analysis and opinion mining [ 93 , 94 ]. Among other external sources, we can find knowledge sources related to Medicine, like the UMLS Metathesaurus [ 95 – 98 ], MeSH thesaurus [ 99 – 102 ], and the Gene Ontology [ 103 – 105 ].
Text mining tasks
The distribution of text mining tasks identified in this literature mapping is presented in Fig. 8 . Classification and clustering are the most frequent tasks. Classification corresponds to the task of finding a model from examples with known classes (labeled instances) in order to predict the classes of new examples. On the other hand, clustering is the task of grouping examples (whose classes are unknown) based on their similarities. Classification was identified in 27.4% and clustering in 17.0% of the studies. As these are basic text mining tasks, they are often the basis of other more specific text mining tasks, such as sentiment analysis and automatic ontology building. Therefore, it was expected that classification and clustering would be the most frequently applied tasks.
Text mining tasks identified in the literature mapping accepted studies
Besides classification and clustering, we can note that semantic concern are present in tasks as information extraction [ 106 – 108 ], information retrieval [ 109 – 111 ], sentiment analysis [ 112 – 115 ], and automatic ontology building [ 116 , 117 ], as well as the pre-processing step itself [ 118 , 119 ].
Methods and algorithms
A word cloud 3 of methods and algorithms identified in this literature mapping is presented in Fig. 9 , in which the font size reflects the frequency of the methods and algorithms among the accepted papers. We can note that the most common approach deals with latent semantics through Latent Semantic Indexing (LSI) [ 2 , 120 ], a method that can be used for data dimension reduction and that is also known as latent semantic analysis. The Latent Semantic Index low-dimensional space is also called semantic space. In this semantic space, alternative forms expressing the same concept are projected to a common representation. It reduces the noise caused by synonymy and polysemy; thus, it latently deals with text semantics. Another technique in this direction that is commonly used for topic modeling is latent Dirichlet allocation (LDA) [ 121 ]. The topic model obtained by LDA has been used for representing text collections as in [ 58 , 122 , 123 ].
Word cloud of methods and algorithms identified in the literature mapping studies. To enable a better reading of the word cloud, the frequency of the methods and algorithms higher than one was rounded up to the nearest ten (for example, a method applied in 75 studies is represented in the word cloud in a word size corresponding to the frequency 80)
Beyond latent semantics, the use of concepts or topics found in the documents is also a common approach. The concept-based semantic exploitation is normally based on external knowledge sources (as discussed in the “ External knowledge sources ” section) [ 74 , 124 – 128 ]. As an example, explicit semantic analysis [ 129 ] rely on Wikipedia to represent the documents by a concept vector. In a similar way, Spanakis et al. [ 125 ] improved hierarchical clustering quality by using a text representation based on concepts and other Wikipedia features, such as links and categories.
The issue of text ambiguity has also been the focus of studies. Word sense disambiguation can contribute to a better document representation. It is normally based on external knowledge sources and can also be based on machine learning methods [ 36 , 130 – 133 ].
Other approaches include analysis of verbs in order to identify relations on textual data [ 134 – 138 ]. However, the proposed solutions are normally developed for a specific domain or are language dependent.
In Fig. 9 , we can observe the predominance of traditional machine learning algorithms, such as Support Vector Machines (SVM), Naive Bayes, K-means, and k-Nearest Neighbors (KNN), in addition to artificial neural networks and genetic algorithms. The application of natural language processing methods (NLP) is also frequent. Among these methods, we can find named entity recognition (NER) and semantic role labeling. It shows that there is a concern about developing richer text representations to be input for traditional machine learning algorithms, as we can see in the studies of [ 55 , 139 – 142 ].
Text representation models
The most popular text representation model is the vector space model. In this model, each document is represented by a vector whose dimensions correspond to features found in the corpus. When features are single words, the text representation is called bag-of-words. Despite the good results achieved with a bag-of-words, this representation, based on independent words, cannot express word relationships, text syntax, or semantics. Therefore, it is not a proper representation for all possible text mining applications.
The use of richer text representations is the focus of several studies [ 62 , 79 , 97 , 143 – 148 ]. Most of the studies concentrate on proposing more elaborated features to represent documents in the vector space model, including the use of topic model techniques, such as LSI and LDA, to obtain latent semantic features. Deep learning [ 149 ] is currently applied to represent independent terms through their associated concepts, in an attempt to narrow the relationships between the terms [ 150 , 151 ]. The use of distributed word representations (word embeddings) can be seen in several works of this area in tasks such as classification [ 88 , 152 , 153 ], summarization [ 154 ], and information retrieval [ 155 ].
Besides the vector space model, there are text representations based on networks (or graphs), which can make use of some text semantic features. Network-based representations, such as bipartite networks and co-occurrence networks, can represent relationships between terms or between documents, which is not possible through the vector space model [ 147 , 156 – 158 ].
In addition to the text representation model, text semantics can also be incorporated to text mining process through the use of external knowledge sources, like semantic networks and ontologies, as discussed in the “ External knowledge sources ” section.
User’s interaction
Text mining is a process to automatically discover knowledge from unstructured data. Nevertheless, it is also an interactive process, and there are some points where a user, normally a domain expert, can contribute to the process by providing his/her previous knowledge and interests. As an example, in the pre-processing step, the user can provide additional information to define a stoplist and support feature selection. In the pattern extraction step, user’s participation can be required when applying a semi-supervised approach. In the post-processing step, the user can evaluate the results according to the expected knowledge usage.
Despite the fact that the user would have an important role in a real application of text mining methods, there is not much investment on user’s interaction in text mining research studies. A probable reason is the difficulty inherent to an evaluation based on the user’s needs. In empirical research, researchers use to execute several experiments in order to evaluate proposed methods and algorithms, which would require the involvement of several users, therefore making the evaluation not feasible in practical ways.
Less than 1% of the studies that were accepted in the first mapping cycle presented information about requiring some sort of user’s interaction in their abstract. To better analyze this question, in the mapping update performed in 2016, the full text of the studies were also considered. Figure 10 presents types of user’s participation identified in the literature mapping studies. The most common user’s interactions are the revision or refinement of text mining results [ 159 – 161 ] and the development of a standard reference, also called as gold standard or ground truth, which is used to evaluate text mining results [ 162 – 165 ]. Besides that, users are also requested to manually annotate or provide a few labeled data [ 166 , 167 ] or generate of hand-crafted rules [ 168 , 169 ].
Types of user participation identified in the literature mapping accepted studies
Systematic mapping summary and future trends
How is semantics considered in text mining studies?
Semantics is an important component in natural language texts. Consequently, in order to improve text mining results, many text mining researches claim that their solutions treat or consider text semantics in some way. However, text mining is a wide research field and there is a lack of secondary studies that summarize and integrate the different approaches. How is semantics considered in text mining studies? Looking for the answer to this question, we conducted this systematic mapping based on 1693 studies, accepted among the 3984 studies identified in five digital libraries. In the previous subsections, we presented the mapping regarding to each secondary research question. In this subsection, we present a consolidation of our results and point some future trends of semantics-concerned text mining.
As previously stated, the objective of this systematic mapping is to provide a general overview of semantics-concerned text mining studies. The papers considered in this systematic mapping study, as well as the mapping results, are limited by the applied search expression and the research questions. It is not feasible to cover all published papers in this broad field. Therefore, the reader can miss in this systematic mapping report some previously known studies. It is not our objective to present a detailed survey of every specific topic, method, or text mining task. This systematic mapping is a starting point, and surveys with a narrower focus should be conducted for reviewing the literature of specific subjects, according to one’s interests.
The quantitative analysis of the scientific production by each text mining dimension (presented from the “ Application domains ” section to the “ User’s interaction ” section) confirmed some previous feelings that we had about our study subject and highlighted other interesting characteristics of the field. Text semantics is closely related to ontologies and other similar types of knowledge representation. We also know that health care and life sciences is traditionally concerned about standardization of their concepts and concepts relationships. Thus, as we already expected, health care and life sciences was the most cited application domain among the literature accepted studies. This application domain is followed by the Web domain, what can be explained by the constant growth, in both quantity and coverage, of Web content.
It was surprising to find the high presence of the Chinese language among the studies. Chinese language is the second most cited language, and the HowNet, a Chinese-English knowledge database, is the third most applied external source in semantics-concerned text mining studies. Looking at the languages addressed in the studies, we found that there is a lack of studies specific to languages other than English or Chinese. We also found an expressive use of WordNet as an external knowledge source, followed by Wikipedia, HowNet, Web pages, SentiWordNet, and other knowledge sources related to Medicine.
Text classification and text clustering, as basic text mining tasks, are frequently applied in semantics-concerned text mining researches. Among other more specific tasks, sentiment analysis is a recent research field that is almost as applied as information retrieval and information extraction, which are more consolidated research areas. SentiWordNet, a lexical resource for sentiment analysis and opinion mining, is already among the most used external knowledge sources.
The treatment of latent semantics, through the application of LSI, stands out when looking at methods and algorithms. Besides that, traditional text mining methods and algorithms, like SVM, KNN, and K-means, are frequently applied and researches tend to enhance the text representation by applying NLP methods or using external knowledge sources. Thus, text semantics can be incorporated to the text mining process mainly through two approaches: the construction of richer terms in the vector space representation model or the use of networks or graphs to represent semantic relations between terms or documents.
In real application of the text mining process, the participation of domain experts can be crucial to its success. However, the participation of users (domain experts) is seldom explored in scientific papers. The difficulty inherent to the evaluation of a method based on user’s interaction is a probable reason for the lack of studies considering this approach.
The mapping indicates that there is space for secondary studies in areas that has a high number of primary studies, such as studies of feature enrichment for a better text representation in the vector space model; use of classification methods; use of clustering methods; and the use of latent semantics in text mining. A detailed literature review, as the review of Wimalasuriya and Dou [ 17 ] (described in “ Surveys ” section), would be worthy for organization and summarization of these specific research subjects.
Considering the development of primary studies, we identified three main future trends: user’s interaction, non-English text processing, and graph-based representation. We expect an increase in the number of studies that have some level of user’s interaction to bring his/her needs and interests to the process. This is particularly valuable for the clustering task, because a considered good clustering solution can vary from user to user [ 170 ]. We also expect a raise of resources (linguistic resources and annotated corpora) for non-English languages. These resources are very important to the development of semantics-concerned text mining techniques. Higher availability of non-English resources will allow a higher number of studies dealing with these languages. Another future trend is the development and use of graph-based text representation. Nowadays, there are already important researches in this direction, and we expect that it will increase as graph-based representations are more expressive than traditional representations in the vector space model.
As an alternative summary of this systematic mapping, additional visualizations of both the selected studies and systematic mapping results can be found online at http://sites.labic.icmc.usp.br/pinda_sm . For this purpose, the prototype of the Pinda tool was adapted for hierarchical visualization of the textual data, using K-means algorithm to group the results. The tool allows the analysis of data (title + abstract of selected studies or information extracted from them) through multiple visualization techniques (Thumbnail, Snippets, Directories, Scatterplot, Treemap, and Sunburst), coordinating the user’s interactions for a better understanding of existing relationships. Figure 11 illustrates the Scatterplot visualization of studies accepted in this systematic mapping. Some of the possible visualizations of the systematic mapping results are presented in Fig. 12 .
Scatterplot visualization of accepted studies of the systematic mapping
Directories and Treemap visualizations of the systematic mapping results
Text semantics are frequently addressed in text mining studies, since it has an important influence in text meaning. However, there is a lack of secondary studies that consolidate these researches. This paper reported a systematic mapping study conducted to overview semantics-concerned text mining literature. The scope of this mapping is wide (3984 papers matched the search expression). Thus, due to limitations of time and resources, the mapping was mainly performed based on abstracts of papers. Nevertheless, we believe that our limitations do not have a crucial impact on the results, since our study has a broad coverage.
The main contributions of this work are (i) it presents a quantitative analysis of the research field; (ii) its conduction followed a well-defined literature review protocol; (iii) it discusses the area regarding seven important text mining dimensions: application domain, language, external knowledge source, text mining task, method and algorithm, representation model, and user’s interaction; and (iv) the produced mapping can give a general summary of the subject and can be of great help for researchers working with semantics and text mining. Thus, this work filled a gap in the literature as, to the best of our knowledge, this is the first general literature review of this wide subject.
Although several researches have been developed in the text mining field, the processing of text semantics remains an open research problem. The field lacks secondary studies in areas that has a high number of primary studies, such as feature enrichment for a better text representation in the vector space model. Another highlight is about a language-related issue. We found considerable differences in numbers of studies among different languages, since 71.4% of the identified studies deal with English and Chinese. Thus, there is a lack of studies dealing with texts written in other languages. When considering semantics-concerned text mining, we believe that this lack can be filled with the development of good knowledge bases and natural language processing methods specific for these languages. Besides, the analysis of the impact of languages in semantic-concerned text mining is also an interesting open research question. A comparison among semantic aspects of different languages and their impact on the results of text mining techniques would also be interesting.
1 A simple search for “systematic review” on the Scopus database in June 2016 returned, by subject area, 130,546 Health Sciences documents (125,254 of them for Medicine) and only 5,539 Physical Sciences (1328 of them for Computer Science). The coverage of Scopus publications are balanced between Health Sciences (32% of total Scopus publication) and Physical Sciences (29% of total Scopus publication).
2 It was not possible to perform the second cycle of searches in ACM Digital Library because of a change in the interface of this search engine. However, it must be notice that only eight studies that was found only in this database was accepted in the first cycle. All other studies was also retrieved by other search engines (specially Scopus, which retrieved more than 89% of accepted studies.)
3 Word cloud created with support of Wordle [ 171 ].
Miner G, Elder J, Hill T, Nisbet R, Delen D, Fast A (2012) Practical text mining and statistical analysis for non-structured text data applications. 1st edn. Academic Press, Boston.
Google Scholar
Aggarwal CC, Zhai C (eds)2012. Mining text data. Springer, Durham.
Kitchenham B, Charters S (2007) Guidelines for performing systematic literature reviews in software engineering. EBSE Technical Report EBSE-2007-01. Keele University and Durham University Joint Report, Durham, UK.
Petersen K, Feldt R, Mujtaba S, Mattsson M (2008) Systematic mapping studies in software engineering In: EASE 2008: Proceedings of the 12th International Conference on Evaluation and Assessment in Software Engineering. EASE’08, 68–77. British Computer Society, Swinton, UK.
Brereton P, Kitchenham BA, Budgen D, Turner M, Khalil M (2007) Lessons from applying the systematic literature review process within the software engineering domain. J Syst Softw80(4): 571–583.
Article Google Scholar
Kitchenham B, Pretorius R, Budgen D, Brereton OP, Turner M, Niazi M, et al (2010) Systematic literature reviews in software engineering—a tertiary study. Inf Softw Technol52(8): 792–805.
Felizardo KR, Nakagawa EY, MacDonell SG, Maldonado JC (2014) A visual analysis approach to update systematic reviews In: EASE’14: Proceedings of the 18th International Conference on Evaluation and Assessment in Software Engineering, 4:1–4:10. ACM, New York.
Moghaddam FA, Lago P, Grosso P (2015) Energy-efficient networking solutions in cloud-based environments: a systematic literature review. ACM Comput Surv47(4): 64:1–64:32.
Pedro RWD, Nunes FLS, Machado-Lima A (2013) Using grammars for pattern recognition in images: a systematic review. ACM Comput Surv46(2): 26:1–26:34.
Pisani PH, Lorena AC (2013) A systematic review on keystroke dynamics. J Braz Comput Soc19(4): 573–587.
Park DH, Kim HK, Choi IY, Kim JK (2012) A literature review and classification of recommender systems research. Expert Syst Appl39(11): 10059–10072.
Khan K, Baharudin BB, Khan A, et al (2009) Mining opinion from text documents: a survey In: DEST’09: Proceedings of the 3rd IEEE International Conference on Digital Ecosystems and Technologies, 217–222. IEEE.
Laboratory of Research on Software Engineering (LaPES) - StArt Tool. http://lapes.dc.ufscar.br/tools/start_tool . Accessed 8 June 2016.
Grobelnik M (2011) Many faces of text processing In: WIMS’11: Proceedings of the International Conference on Web Intelligence, Mining and Semantics, 5. ACM.
Stavrianou A, Andritsos P, Nicoloyannis N (2007) Overview and semantic issues of text mining. SIGMOD Rec36(3): 23–34.
Daud A, Li J, Zhou L, Muhammad F (2010) Knowledge discovery through directed probabilistic topic models: a survey. Front Comput Sci China4(2): 280–301.
Wimalasuriya DC, Dou D (2010) Ontology-based information extraction: an introduction and a survey of current approaches. J Inf Sci36(3): 306–323.
Bharathi G, Venkatesan D (2012) Study of ontology or thesaurus based document clustering and information retrieval. J Eng Appl Sci7(4): 342–347.
Reshadat V, Feizi-Derakhshi MR (2012) Studying of semantic similarity methods in ontology. Res J Appl Sci Eng Technol4(12): 1815–1821.
Schiessl M, Bräscher M (2012) Do texto às ontologias: uma perspectiva para a ciência da informação. Ciência da Informação40(2): 301–311.
Cimiano P, Völker J, Studer R (2006) Ontologies on demand?—a description of the state-of-the-art, applications, challenges and trends for ontology learning from text. Inf Wiss Prax57(6-7): 315–320.
Jovanovic J, Bagheri E, Cuzzola J, Gasevic D, Jeremic Z, Bashash R (2014) Automated semantic tagging of textual content. IT Prof16(6): 38–46.
Wallace BC (2015) Computational irony: a survey and new perspectives. Artif Intell Rev43(4): 467–483.
Winnenburg R, Wächter T, Plake C, Doms A, Schroeder M (2008) Facts from text: can text mining help to scale-up high-quality manual curation of gene products with ontologies?Brief Bioinform9(6): 466–478.
Leser U, Hakenberg J (2005) What makes a gene name? Named entity recognition in the biomedical literature. Brief Bioinform6(4): 357–369.
Dagan I, Dolan B, Magnini B, Roth D (2009) Recognizing textual entailment: rational, evaluation and approaches. Nat Lang Eng15(04): i–xvii.
Irfan R, King CK, Grages D, Ewen S, Khan SU, Madani SA, et al. (2015) A survey on text mining in social networks. Knowl Eng Rev30(02): 157–170.
Sheth A, Ramakrishnan C, Thomas C (2005) Semantics for the semantic web: the implicit, the formal and the powerful. Int J Semant Web Inf Syst1(1): 1–18.
Cheng XY, Cheng C, Zhu Q (2011) The applications of description logics in natural language processing. Adv Mater Res204: 381–386.
Martinez A, Martinez W (2015) At the interface of computational linguistics and statistics. Wiley Interdiscip Rev Comput Stat7(4): 258–274.
Article MathSciNet Google Scholar
Bos J (2011) A survey of computational semantics: representation, inference and knowledge in wide-coverage text understanding. Lang Linguist Compass5(6): 336–366.
W, 3C - Semantic Web Health Care and Life Sciences Interest Group. https://www.w3.org/blog/hcls/ . Accessed 8 June 2016.
National Center for Biotechnology Information - PubMed. http://www.ncbi.nlm.nih.gov/pubmed/ . Accessed 8 June 2016.
Miwa M, Thompson P, McNaught J, Kell DB, Ananiadou S (2012) Extracting semantically enriched events from biomedical literature. BMC Bioinforma13(1): 1–24.
Ravikumar KE, Liu H, Cohn JD, Wall ME, Verspoor K (2011) Pattern learning through distant supervision for extraction of protein-residue associations in the biomedical literature, vol. 2. pp 59–65. IEEE, Honolulu. http://ieeexplore.ieee.org/document/6147049/ .
Xia N, Lin H, Yang Z, Li Y (2011) Combining multiple disambiguation methods for gene mention normalization. Expert Syst Appl38(7): 7994–7999.
Sarker A, Gonzalez G (2015) Portable automatic text classification for adverse drug reaction detection via multi-corpus training. J Biomed Inform53: 196–207.
Wu JL, Yu LC, Chang PC (2012) Detecting causality from online psychiatric texts using inter-sentential language patterns. BMC Med Inform Dec Making12(1): 1–10.
Abacha AB, Zweigenbaum P (2011) A hybrid approach for the extraction of semantic relations from MEDLINE abstracts. Lect Notes Comput Sci (Incl Subseries Lect Notes Artif Intell Lect Notes Bioinforma)6609 LNCS(PART 2): 139–150.
Yu LC, Wu CH, Jang FL (2007) Psychiatric consultation record retrieval using scenario-based representation and multilevel mixture model. IEEE IEEE Trans Inf Technol Biomed11(4): 415–427.
Musto C, Semeraro G, Lops P, Gemmis MD (2015) CrowdPulse: a framework for real-time semantic analysis of social streams. Inf Syst54: 127–146.
García-Moya L, Kudama S, Aramburu MJ, Berlanga R (2013) Storing and analysing voice of the market data in the corporate data warehouse. Inf Syst Front15(3): 331–349.
Eugenio BD, Green N, Subba R (2013) Detecting life events in feeds from Twitter In: ICSC 2013: Proceedings of the IEEE Seventh International Conference on Semantic Computing, 274–277. IEEE, Irvine, http://ieeexplore.ieee.org/document/6693529/ .
Chapter Google Scholar
Torunoglu D, Telseren G, Sagturk O, Ganiz MC (2013) Wikipedia based semantic smoothing for twitter sentiment classification In: INISTA 2013: Proceedings of the IEEE International Symposium on Innovations in Intelligent Systems and Applications, 1–5. IEEE, Albena.
Cao Q, Duan W, Gan Q (2011) Exploring determinants of voting for the “helpfulness” of online user reviews: a text mining approach. Decis Support Syst50(2): 511–521.
Levi A, Mokryn O, Diot C, Taft N (2012) Finding a needle in a haystack of reviews: cold start context-based hotel recommender system In: RecSys’12: Proceedings of the sixth ACM Conference on Recommender Systems, 115–122. ACM, New York.
He W, Shen J, Tian X, Li Y, Akula V, Yan G, et al (2015) Gaining competitive intelligence from social media data: evidence from two largest retail chains in the world. Ind Manag Data Syst115(9): 1622–1636.
He W, Tian X, Chen Y, Chong D (2016) Actionable social media competitive analytics for understanding customer experiences. J Comput Inf Syst56(2): 145–155.
Tian X, He W, Tao R, Akula V (2016) Mining online hotel reviews: a case study from hotels in China In: AMCIS 2016: Proceedings of the 22nd Americas Conference on Information Systems, 1–8.
ACM - Asian and Low-Resource Language Information Processing (TALLIP). http://tallip.acm.org/ . Accessed 8 June 2016.
Chen CL, Liu CL, Chang YC, Tsai HP (2011) Mining opinion holders and opinion patterns in US financial statements In: TAAI 2011: Proceedings of the International Conference on Technologies and Applications of Artificial Intelligence, 62–68. IEEE, Chung-Li,
Chen J, Liu J, Yu W, Wu P (2009) Combining lexical stability and improved lexical chain for unsupervised word sense disambiguation In: KAM’09: Proceedings of the Second International Symposium on Knowledge Acquisition and Modeling, 430–433. IEEE, Wuhan. http://ieeexplore.ieee.org/document/5362135/ .
Rusu D, Fortuna B, Grobelnik M, Mladenic D (2009) Semantic graphs derived from triplets with application in document summarization. Informatica (Slovenia)33(3): 357–362.
Krachina O, Raskin V, Triezenberg K (2007) Reconciling privacy policies and regulations: ontological semantics perspective In: Human Interface and the Management of Information. Interacting in Information Environments, 730–739. Springer, Berlin,
Mansuy T, Hilderman RJ (2006) A characterization of WordNet features in Boolean models for text classification In: AusDM 2006: Proceedings of the fifth Australasian Conference on Data Mining and Analystics, 103–109. Australian Computer Society, Inc, Darlinghurst,
Ciaramita M, Gangemi A, Ratsch E, Šaric J, Rojas I (2005) Unsupervised learning of semantic relations between concepts of a molecular biology ontology In: IJCAI’05: Proceedings of the 19th International Joint Conference on Artificial Intelligence, 659–664. Morgan Kaufmann Publishers Inc., San Francisco, CA.
Kim K, Chung BS, Choi Y, Lee S, Jung JY, Park J (2014) Language independent semantic kernels for short-text classification. Expert Syst Appl41(2): 735–743.
Gujraniya D, Murty MN (2012) Efficient classification using phrases generated by topic models In: ICPR 2012: Proceedings of the 21st International Conference on Pattern Recognition, 2331–2334. IEEE, Tsukuba,
Du C, Zhuang F, He Q, Shi Z (2012) Multi-task semi-supervised semantic feature learning for classification In: ICDM 2012: Proceedings of the IEEE 12th International Conference on Data Mining, 191–200. IEEE, Brussels, http://ieeexplore.ieee.org/document/6413903/ .
Wu Q, Zhang C, Deng X, Jiang C (2011) LDA-based model for topic evolution mining on text In: ICCSE 2011: Proceedings of the 6th International Conference on Computer Science & Education, 946–949. IEEE, Singapore,
Lu X, Zheng B, Velivelli A, Zhai C (2006) Enhancing text categorization with semantic-enriched representation and training data augmentation. J Am Med Inform Assoc13(5): 526–535.
Wu J, Dang Y, Pan D, Xuan Z, Liu Q (2010) Textual knowledge representation through the semantic-based graph structure in clustering applications In: HICSS 2010: Proceedings of the 43rd Hawaii International Conference on System Sciences, 1–8. IEEE, Washington,
Princeton University - WordNet. http://wordnet.princeton.edu/ . Accessed 8 June 2016.
Fellbaum C (1998) WordNet: an electronic lexical database. MIT Press, Cambridge.
MATH Google Scholar
Weller K (2010) Knowledge representation in the social semantic web. Walter de Gruyter.
Weller K, et al (2007) Folksonomies and ontologies: two new players in indexing and knowledge representation In: Proceedings of the Online Information Conference, 108–115.
Wei TA, Lu YC, Chang HB, Zhou QA, Bao XD (2015) A semantic approach for text clustering using WordNet and lexical chains. Expert Syst Appl42(4): 2264–2275.
Li J, Zhao Y, Liu B (2009) Fully automatic text categorization by exploiting wordnet In: Information Retrieval Technology, 1–12. Springer, Berlin,
Mansuy TN, Hilderman RJ (2006) Evaluating WordNet features in text classification models In: FLAIRS Conference 2006: Proceedings of the Nineteenth International Florida Artificial Intelligence Research Society Conference, 568–573. AAAI PRESS, Florida,
Shin Y, Ahn Y, Kim H, Lee SG (2015) Exploiting synonymy to measure semantic similarity of sentences In: IMCOM ’15: Proceedings of the 9th International Conference on Ubiquitous Information Management and Communication, 40:1–40:4. ACM, New York,
Batet M, Valls A, Gibert K (2010) Performance of ontology-based semantic similarities in clustering In: Artificial Intelligence and Soft Computing, 281–288. Springer, Berlin,
Basu S, Mooney RJ, Pasupuleti KV, Ghosh J (2001) Evaluating the novelty of text-mined rules using lexical knowledge In: KDD’01: Proceedings of the seventh ACM SIGKDD international conference on Knowledge discovery and data mining, 233–238. ACM, San Francisco,
Wikipedia. https://www.wikipedia.org/ . Accessed 8 June 2016.
Kim HJA, Hong KJA, Chang JYb (2015) Semantically enriching text representation model for document clustering In: Proceedings of the ACM Symposium on Applied Computing,922–925. ACM, New York, http://dl.acm.org.ez67.periodicos.capes.gov.br/citation.cfm?id=2696055 .
Yun J, Jing L, Yu J, Huang H (2011) Unsupervised feature weighting based on local feature relatedness In: Advances in Knowledge Discovery and Data Mining, 38–49. Springer, Berlin,
Gabrilovich E, Markovitch S (2009) Wikipedia-based semantic interpretation for natural language processing. J Artif Intell Res34: 443–498.
Hu X, Zhang X, Lu C, Park EK, Zhou X (2009) Exploiting Wikipedia as external knowledge for document clustering In: KDD’09: Proceedings of the 15th ACM SIGKDD international conference on Knowledge discovery and data mining, 389–396. ACM, New York,
Mizzaro S, Pavan M, Scagnetto I, Valenti M (2014) Short text categorization exploiting contextual enrichment and external knowledge In: Proceedings of the First International Workshop on Social Media Retrieval and Analysis, 57–62. ACM, New York,
Janik M, Kochut KJ (2008) Wikipedia in action: ontological knowledge in text categorization In: ICSC 2008: Proceedings of the International Conference on Semantic Computing, 268–275. IEEE, Santa Monica,
Chang MW, Ratinov LA, Roth D, Srikumar V (2008) Importance of semantic representation: dataless classification In: AAAI-08: Proceedings of the Twenty-Third AAAI Conference on Artificial Intelligence, 830–835.
Medelyan O, Milne D, Legg C, Witten IH (2009) Mining meaning from Wikipedia. Int J Human-Computer Stud67(9): 716–754.
HowNet Knowledge Database. http://www.keenage.com/ . Accessed 8 June 2016.
Jin CX, Zhou HY, Bai QC (2012) Short text clustering algorithm with feature keyword expansion. Adv Mater Res532: 1716–1720.
Liu Z, Yu W, Chen W, Wang S, Wu F (2010) Short text feature selection for micro-blog mining In: CiSE 2010: Proceedings of the International Conference on Computational Intelligence and Software Engineering, 1–4. IEEE, Wuhan,
Hu P, He T, Ji D, Wang M (2004) A study of Chinese text summarization using adaptive clustering of paragraphs In: CIT’04: Proceedings of the Fourth International Conference on Computer and Information Technology, 1159–1164. IEEE, Wuhan,
Zhu ZY, Dong SJ, Yu CL, He J (2011) A text hybrid clustering algorithm based on HowNet semantics. Key Eng Mater474: 2071–2078.
Zheng D, Liu H, Zhao T (2011) Search results clustering based on a linear weighting method of similarity In: IALP 2011: Proceedings of the International Conference on Asian Language Processing, 123–126. IEEE, Penang,
Wang R (2010) Cognitive-based emotion classifier of Chinese vocabulary design In: ISISE 2010: Proceedings of the International Symposium on Information Science and Engineering, 582–585. IEEE.
Thorleuchter D, Van den Poel D (2014) Semantic compared cross impact analysis. Expert Syst Appl41(7): 3477–3483.
Roussinov D, Turetken O (2009) Exploring models for semantic category verification. Inf Syst34(8): 753–765.
Zelikovitz S, Kogan M (2006) Using Web searches on important words to create background sets for LSI classification In: FLAIRS Conference 2006: Proceedings of the Nineteenth International Florida Artificial Intelligence Research Society Conference, 298–603.
SentiWordNet. http://sentiwordnet.isti.cnr.it/ . Accessed 8 June 2016.
Al Nasseri A, Tucker A, de Cesare S (2015) Quantifying StockTwits semantic terms’ trading behavior in financial markets: an effective application of decision tree algorithms. Expert Syst Appl42(23): 9192–9210.
Kumar V, Minz S (2013) Mood classifiaction of lyrics using SentiWordNet In: ICCCI 2013: Proceedings of the International Conference on Computer Communication and Informatics, 1–5. IEEE, Coimbatore,
Unified Medical Language System (UMLS) Metathesaurus. https://www.nlm.nih.gov/research/umls/knowledge_sources/metathesaurus/ . Accessed 8 June 2016.
Garla VN, Brandt C (2012) Ontology-guided feature engineering for clinical text classification. J Biomed Inform45(5): 992–998.
Plaza L, Díaz A, Gervás P (2011) A semantic graph-based approach to biomedical summarisation. Artif Intell Med53(1): 1–14.
Aljaber B, Martinez D, Stokes N, Bailey J (2011) Improving MeSH classification of biomedical articles using citation contexts. J Biomed Inform44(5): 881–896.
Medical Subject Headings (MeSH). https://www.nlm.nih.gov/mesh/ . Accessed 8 June 2016.
Logeswari S, Premalatha K (2013) Biomedical document clustering using ontology based concept weight In: ICCCI 2013: Proceedings of the International Conference on Computer Communication and Informatics, 1–4. IEEE, Coimbatore,
Nguyen SH, Jaśkiewicz G, Świeboda W, Nguyen HS (2012) Enhancing search result clustering with semantic indexing In: SoICT’12: Proceedings of the Third Symposium on Information and Communication Technology, 71–80. ACM, New York,
Ginter F, Pyysalo S, Boberg J, Järvinen J, Salakoski T (2004) Ontology-based feature transformations: a data-driven approach In: Advances in Natural Language Processing, 279–290. Springer, Berlin,
Kanavos A, Makris C, Theodoridis E (2012) On topic categorization of PubMed query results In: Artificial Intelligence Applications and Innovations, 556–565. Springer.
Zheng HT, Borchert C, Kim HG (2008) Exploiting gene ontology to conceptualize biomedical document collections In: The Semantic Web, 375–389. Springer, Berlin,
Jin B, Muller B, Zhai C, Lu X (2008) Multi-label literature classification based on the Gene Ontology graph. BMC Bioinforma9(1): 525.
Mannai M, Ben Abdessalem Karaa W (2013) Bayesian information extraction network for Medline abstract. In: 2013 World Congress on Computer and Information Technology (WCCIT), 1–3. IEEE, Sousse,
Jiana B, Tingyu L, Tianfang Y (2012) Event information extraction approach based on complex Chinese texts In: IALP 2012: Proceedings of the International Conference on Asian Language Processing, 61–64.
Hengliang W, Weiwei Z (2012) A web information extraction method based on ontology. Adv Inf Sci Serv Sci4(8): 199–206.
Aghassi H, Sheykhlar Z (2012) Extending information retrieval by adjusting text feature vectors. Commun Comput Inform Sci295 CCIS: 133–142.
Bharathi G, Venkatesan D (2012) Improving information retrieval using document clusters and semantic synonym extraction. J Theor Appl Inf Technol36(2): 167–173.
Egozi O, Markovitch S, Gabrilovich E (2011) Concept-based information retrieval using explicit semantic analysis. ACM Trans Inf Syst29(2): 8:1–8:34.
Nassirtoussi AK, Aghabozorgi S, Wah TY, Ngo DCL (2015) Text mining of news-headlines for FOREX market prediction: a multi-layer dimension reduction algorithm with semantics and sentiment. Expert Syst Appl42(1): 306–324.
Batool R, Khattak AM, Maqbool J, Lee S (2013) Precise tweet classification and sentiment analysis In: 2013 IEEE/ACIS 12th International Conference on Computer and Information Science (ICIS), 461–466. IEEE, Niigata,
Veselovská K (2012) Sentence-level sentiment analysis in Czech In: WIMS’12:Proceedings of the 2Nd International Conference on Web Intelligence, Mining and Semantics, 65:1–65:4. ACM, New York,
Petersen MK, Hansen LK (2012) On an emotional node: modeling sentiment in graphs of action verbs In: 2012 International Conference on Audio, Language and Image Processing, 308–313. IEEE, Shanghai,
Domínguez García R, Schmidt S, Rensing C, Steinmetz R (2012) Automatic taxonomy extraction in different languages using wikipedia and minimal language-specific information. Lect Notes Comp Sci (Incl Subseries Lect Notes Artif Intell Lect Notes Bioinforma)7181 LNCS(PART 1): 42–53.
Punuru J, Chen J (2012) Learning non-taxonomical semantic relations from domain texts. J Intell Inf Syst38(1): 191–207.
Stenetorp P, Soyer H, Pyysalo S, Ananiadou S, Chikayama T (2012) Size (and domain) matters: evaluating semantic word space representations for biomedical text In: SMBM 2012: Proceedings of the 5th International Symposium on Semantic Mining in Biomedicine,42–49.
Froud H, Lachkar A, Ouatik SA (2012) Stemming versus light stemming for measuring the simitilarity between Arabic words with latent semantic analysis model In: 2012 Colloquium in Information Science and Technology, 69–73. IEEE, Fez,
Kuhn A, Ducasse S, Gírba T (2007) Semantic clustering: identifying topics in source code. Inf Softw Technol49: 230–243.
Blei DM, Ng AY, Jordan MI (2003) Latent Dirichlet allocation. J Mach Learn Res3(Jan): 993–1022.
Zrigui M, Ayadi R, Mars M, Maraoui M (2012) Arabic text classification framework based on latent dirichlet allocation. J Comput Inf Technol20(2): 125–140.
Liu Z, Li M, Liu Y, Ponraj M (2011) Performance evaluation of latent Dirichlet allocation in text mining In: FSKD 2011: Proceedings of the Eighth International Conference on Fuzzy Systems and Knowledge Discovery, 2695–2698. IEEE, Shanghai.
Xiang W, Yan J, Ruhua C, Hua F (2013) Improving text categorization with semantic knowledge in Wikipedia. IEICE Trans Inf Syst96(12): 2786–2794.
Spanakis G, Siolas G, Stafylopatis A (2012) Exploiting Wikipedia knowledge for conceptual hierarchical clustering of documents. Comput J55(3): 299–312.
Article MATH Google Scholar
Andreasen T, Bulskov H, Jensen PA, Lassen T (2011) Extracting conceptual feature structures from text In: ISMIS 2011: Proceedings 19th International Symposium on Methodologies for Intelligent Systems, 396–406. Springer, Berlin,
Goossen F, IJntema W, Frasincar F, Hogenboom F, Kaymak U (2011) News personalization using the CF-IDF semantic recommender In: WIMS’11: Proceedings of the International Conference on Web Intelligence, Mining and Semantics, 10. ACM, New York,
Huang A, Milne D, Frank E, Witten IH (2008) Clustering documents with active learning using Wikipedia In: ICDM’08: Eighth IEEE International Conference on Data Mining, 839–844. IEEE, Pisa,
Gabrilovich E, Markovitch S (2007) Computing semantic relatedness using Wikipedia-based explicit semantic analysis In: IJCAI-07: Proceedings of the 20th International Joint Conference on Artifical Intelligence, 1606–1611. Morgan Kaufmann Publishers Inc, San Francisco, http://dl.acm.org.ez67.periodicos.capes.gov.br/citation.cfm?id=1625535 .
Navigli R, Faralli S, Soroa A, de Lacalle O, Agirre E (2011) Two birds with one stone: learning semantic models for text Categorization and word sense disambiguation In: CIKM’11: Proceedings of the 20th ACM International Conference on Information and Knowledge Management, 2317–2320. ACM, Glasgow,
Mostafa MS, Haggag MH, Gomaa WH (2008) Document clustering using word sense disambiguation In: SEDE 2008: Proceedings of 17th International Conference on Software Engineering and Data Engineering, 19–24.
Andreopoulos B, Alexopoulou D, Schroeder M (2008) Word sense disambiguation in biomedical ontologies with term co-occurrence analysis and document clustering. Int J Data Min Bioinforma2(3): 193–215.
Koeling R, McCarthy D, Carroll J (2007) Text categorization for improved priors of word meaning In: Computational Linguistics and Intelligent Text Processing, 241–252. Springer, Berlin,
Sharma A, Swaminathan R, Yang H (2010) A verb-centric approach for relationship extraction in biomedical text In: ICSC 2010: Proceedings of the IEEE Fourth International Conference on Semantic Computing, 377–385. IEEE, Pittsburgh,
Wang W, Zhao D, Zou L, Wang D, Zheng W (2010) Extracting 5W1H event semantic elements from Chinese online news In: WAIM 2010: Proceedings of the Workshops of the 11th International Conference on Web-Age Information Management, 644–655. Springer, Berlin,
Rebholz-Schuhmann D, Jimeno-Yepes A, Arregui M, Kirsch H (2010) Measuring prediction capacity of individual verbs for the identification of protein interactions. J Biomed Inform43(2): 200–207.
Van Der Horn P, Bakker B, Geleijnse G, Korst J, Kurkin S (2008) Classifying verbs in biomedical text using subject-verb-object relationships In: SMBM 2008: Proceedings of the 3rd International Symposium on Semantic Mining in Biomedicine, 137–140.
Kontos J, Malagardi I, Alexandris C, Bouligaraki M (2000) Greek verb semantic processing for stock market text mining In: NLP’00: Proceedings of the Second International Conference on Natural Language Processing, 395–405. Springer-Verlag, London.
Stankov I, Todorov D, Setchi R (2013) Enhanced cross-domain document clustering with a semantically enhanced text stemmer (SETS). Int J Knowl-Based Intell Eng Syst17(2): 113–126.
Huang CH, Yin J, Hou F (2011) A text similarity measurement combining word semantic information with TF-IDF method. Jisuanji Xuebao(Chin J Comput)34(5): 856–864.
Doan S, Kawazoe A, Conway M, Collier N (2009) Towards role-based filtering of disease outbreak reports. J Biomed Inform42(5): 773–780.
Meng X, Chen Q, Wang X (2008) Semantic feature reduction in chinese document clustering In: SMC 2008: Proceedings of the IEEE International Conference on Systems, Man and Cybernetics, 3721–3726. IEEE, Singapore,
Freitas A, O’Riain S, Curry E, da Silva JCP, Carvalho DS (2013) Representing texts as contextualized entity-centric linked data graphs In: DEXA 2013: Proceedings of the 24th International Workshop on Database and Expert Systems Applications, 133–137. IEEE, Los Alamitos,
Fathy I, Fadl D, Aref M (2012) Rich semantic representation based approach for text generation In: INFOS 2012: Proceedings of the 8th International Conference on Informatics and Systems, NLP–20. IEEE, Cairo,
Wu J, Xuan Z, Pan D (2011) Enhancing text representation for classification tasks with semantic graph structures. Int J Innov Comput Inf Control (ICIC)7(5): 2689–2698.
Alencar ROD, Davis Jr CA, Gonçalves MA (2010) Geographical classification of documents using evidence from Wikipedia In: GIR ’10: Proceedings of the 6th Workshop on Geographic Information Retrieval, 12. ACM, New York,
Smirnov I, Tikhomirov I (2009) Heterogeneous semantic networks for text representation in intelligent search engine EXACTUS In: SENSE’09: Proceedings of the Workshop on Conceptual Structures for Extracting Natural Language Semantics, 1–9.
Chau R, Tsoi AC, Hagenbuchner M, Lee V (2009) A conceptlink graph for text structure mining In: ACSC’09: Proceedings of the Thirty-Second Australasian Conference on Computer Science - Volume 91, 141–150. Australian Computer Society, Inc., Darlinghurst,
Schmidhuber J (2015) Deep learning in neural networks: an overview. Neural Netw61: 85–117.
Lebret R, Collobert R (2015) Rehabilitation of count-based models for word vector representations. Lect Notes Comput Sci (Incl Subseries Lect Notes Artif Intell Lect Notes Bioinforma)9041: 417–429.
Li R, Shindo H (2015) Distributed document representation for document classification. Lect Notes Comput Sci (Incl Subseries Lect Notes Artif Intell Lect Notes Bioinforma)9077: 212–225.
Sohrab MG, Miwa M, Sasaki Y (2015) Centroid-means-embedding: an approach to infusing word embeddings into features for text classification. Lect Notes Comput Sci (Incl Subseries Lect Notes Artif Intell Lect Notes Bioinforma)9077: 289–300.
Wang P, Xu B, Xu J, Tian G, Liu CL, Hao H (2016) Semantic expansion using word embedding clustering and convolutional neural network for improving short text classification. Neurocomputing174: 806–814.
Zhang C, Zhang L, Wang CJ, Xie JY (2014) Text summarization based on sentence selection with semantic representation In: Proceedings of the International Conference on Tools with Artificial Intelligence,Vol. 2014-December. IEEE, Limassol. 584–590.
Vulić I, Moens MF (2015) Monolingual and cross-lingual information retrieval models based on (Bilingual) word embeddings In: SIGIR’15: Proceedings of the 38th International ACM SIGIR Conference on Research and Development in Information Retrieval, 363–372. ACM, New York,
Kamal A, Abulaish M, Anwar T (2012) Mining feature-opinion pairs and their reliability scores from web opinion sources In: WIMS’12: Proceedings of the 2nd International Conference on Web Intelligence, Mining and Semantics, 15. ACM, New York,
Kong L, Yan R, He Y, Zhang Y, Zhang Z, Fu L (2011) DVD: a model for event diversified versions discovery In: Web Technologies and Applications, 168–180. Springer, Berlin,
Jing L, Yun J, Yu J, Huang J (2011) High-order co-clustering text data on semantics-based representation model In: Advances in Knowledge Discovery and Data Mining, 171–182. Springer, Berlin,
Krajewski R, Rybinski H, Kozlowski M (2016) A novel method for dictionary translation. J Intell Inf Syst47(3): 491–514.
Luo Z, Miotto R, Weng C (2013) A human–computer collaborative approach to identifying common data elements in clinical trial eligibility criteria. J Biomed Inform46(1): 33–39.
Kayed A (2005) Building e-laws ontology: new approach In: Proceedings of the On the Move to Meaningful Internet Systems 2005: OTM 2005 Workshops, 826–835. Springer, Berlin,
Sevenster M, van Ommering R, Qian Y (2012) Automatically correlating clinical findings and body locations in radiology reports using MedLEE. J Digit Imaging25(2): 240–249.
Volkova S, Caragea D, Hsu WH, Drouhard J, Fowles L (2010) Boosting biomedical entity extraction by using syntactic patterns for semantic relation discovery In: WI-IAT 2010: Proceedings of the IEEE/WIC/ACM International Conference on Web Intelligence and Intelligent Agent Technology, 272–278. IEEE, Toronto.
Waltinger U, Mehler A (2009) Social semantics and its evaluation by means of semantic relatedness and open topic models In: WI-IAT’09: Proceedings of the 2009 IEEE/WIC/ACM International Joint Conference on Web Intelligence and Intelligent Agent Technology-Volume 01, 42–49. IEEE Computer Society, Milan,
Kass A, Cowell-Shah C (2006) Using lightweight NLP and semantic modeling to realize the internet’s potential as a corporate radar In: AAAI Fall Symposium. AAAI PRESS.
Blake C (2010) Beyond genes, proteins, and abstracts: identifying scientific claims from full-text biomedical articles. J Biomed Inform43(2): 173–189.
Hu J, Fang L, Cao Y, Zeng HJ, Li H, Yang Q, et al (2008) Enhancing text clustering by leveraging Wikipedia semantics In: SIGIR’08: Proceedings of the 31st annual international ACM SIGIR conference on Research and development in information retrieval, 179–186. ACM, New York,
Lu CY, Lin SH, Liu JC, Cruz-Lara S, Hong JS (2010) Automatic event-level textual emotion sensing using mutual action histogram between entities. Expert Syst Appl37(2): 1643–1653.
Ahmed ST, Nair R, Patel C, Davulcu H (2009) BioEve: bio-molecular event extraction from text using semantic classification and dependency parsing In: BioNLP’09: Proceedings of the Workshop on Current Trends in Biomedical Natural Language Processing: Shared Task, 99–102. Association for Computational Linguistics.
Jain AK (2010) Data clustering: 50 years beyond k-means. Pattern Recogn Lett31(8): 651–666.
Wordle. http://www.wordle.net/ . Accessed 15 June 2016.
Download references
Acknowledgements
The authors would like to thank the financial support of grant #132666/2016-2, National Council for Scientific and Technological Development (CNPq); grants #2013/14757-6, #2014/08996-0, and #2016/07620-2, São Paulo Research Foundation (FAPESP); and Coordination for the Improvement of Higher Education Personnel (CAPES).
Authors’ contributions
RAS and SOR planned this systematic mapping study. RAS conducted its first cycle (searches performed in January 2014). JA and RAS conducted its second cycle (searches performed in February 2016). RAS and SOR analyzed the results and drafted the manuscript after the first cycle and updated it after the second cycle. JA was involved in updating the manuscript with the second cycle results. All authors revised and approved the final manuscript.
Competing interests
The authors declare that they have no competing interests.
Publisher’s Note
Springer Nature remains neutral with regard to jurisdictional claims in published maps and institutional affiliations.
Author information
Authors and affiliations.
Laboratório de Inteligência Computacional (LABIC), Instituto de Ciências Matemáticas e de Computação (ICMC), Universidade de São Paulo (USP), São Carlos, P.O. Box 668, 13561-970, SP, Brazil
Roberta Akemi Sinoara, João Antunes & Solange Oliveira Rezende
You can also search for this author in PubMed Google Scholar
Corresponding author
Correspondence to Roberta Akemi Sinoara .
Rights and permissions
Open Access This article is distributed under the terms of the Creative Commons Attribution 4.0 International License ( http://creativecommons.org/licenses/by/4.0/ ), which permits unrestricted use, distribution, and reproduction in any medium, provided you give appropriate credit to the original author(s) and the source, provide a link to the Creative Commons license, and indicate if changes were made.
Reprints and permissions
About this article
Cite this article.
Sinoara, R., Antunes, J. & Rezende, S. Text mining and semantics: a systematic mapping study. J Braz Comput Soc 23 , 9 (2017). https://doi.org/10.1186/s13173-017-0058-7
Download citation
Received : 24 March 2017
Accepted : 01 June 2017
Published : 29 June 2017
DOI : https://doi.org/10.1186/s13173-017-0058-7
Share this article
Anyone you share the following link with will be able to read this content:
Sorry, a shareable link is not currently available for this article.
Provided by the Springer Nature SharedIt content-sharing initiative
- Systematic review
- Text mining
- Text semantics
Help | Advanced Search
Computer Science > Cryptography and Security
Title: a survey of relevant text mining technology.
Abstract: Recent advances in text mining and natural language processing technology have enabled researchers to detect an authors identity or demographic characteristics, such as age and gender, in several text genres by automatically analysing the variation of linguistic characteristics. However, applying such techniques in the wild, i.e., in both cybercriminal and regular online social media, differs from more general applications in that its defining characteristics are both domain and process dependent. This gives rise to a number of challenges of which contemporary research has only scratched the surface. More specifically, a text mining approach applied on social media communications typically has no control over the dataset size, the number of available communications will vary across users. Hence, the system has to be robust towards limited data availability. Additionally, the quality of the data cannot be guaranteed. As a result, the approach needs to be tolerant to a certain degree of linguistic noise (for example, abbreviations, non-standard language use, spelling variations and errors). Finally, in the context of cybercriminal fora, it has to be robust towards deceptive or adversarial behaviour, i.e. offenders who attempt to hide their criminal intentions (obfuscation) or who assume a false digital persona (imitation), potentially using coded language. In this work we present a comprehensive survey that discusses the problems that have already been addressed in current literature and review potential solutions. Additionally, we highlight which areas need to be given more attention.
Subjects: | Cryptography and Security (cs.CR) |
Cite as: | [cs.CR] |
(or [cs.CR] for this version) | |
Focus to learn more arXiv-issued DOI via DataCite |
Submission history
Access paper:.
- Other Formats

References & Citations
- Google Scholar
- Semantic Scholar
BibTeX formatted citation

Bibliographic and Citation Tools
Code, data and media associated with this article, recommenders and search tools.
- Institution
arXivLabs: experimental projects with community collaborators
arXivLabs is a framework that allows collaborators to develop and share new arXiv features directly on our website.
Both individuals and organizations that work with arXivLabs have embraced and accepted our values of openness, community, excellence, and user data privacy. arXiv is committed to these values and only works with partners that adhere to them.
Have an idea for a project that will add value for arXiv's community? Learn more about arXivLabs .
Advertisement
A survey of the literature: how scholars use text mining in Educational Studies?
- Published: 12 August 2022
- Volume 28 , pages 2071–2090, ( 2023 )
Cite this article
- Junhe Yang 1 , 2 ,
- Kinshuk 1 &
- Yunjo An 1
1128 Accesses
3 Citations
1 Altmetric
Explore all metrics
The massive amount of text related to education provides rich information to support education in many aspects. In the meantime, the vast yet increasing volume of text makes it impossible to analyze manually. Text mining is a powerful tool to automatically analyze large-scaled texts and generate insights from the texts. However, many educational scholars are not fully aware of whether text mining is useful and how to use it in their studies. To address this problem, we reviewed the literature to examine the educational research that used text mining techniques. Specifically, we proposed an educational text mining workflow and focused on identifying the articles’ bibliographic information, research methodologies, and applications in alignment with the workflow. We selected 161 articles published in educational journals from 2015 to 2020. We find that text mining is becoming more popular and essential in educational research. The conclusion is that we can employ three steps (text source selection, text mining techniques application, and educational information discovery) to use text mining in educational studies. We also summarize different options in each step in this paper. Our work should help educational scholars better understand educational text mining and provide support information for future research in text mining for educational contexts.
This is a preview of subscription content, log in via an institution to check access.
Access this article
Price includes VAT (Russian Federation)
Instant access to the full article PDF.
Rent this article via DeepDyve
Institutional subscriptions
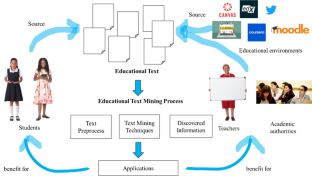
Similar content being viewed by others
Using Text Mining Techniques for Extracting Information from Research Articles
A Text Mining Based Literature Analysis for Learning Theories and Computer Science Education
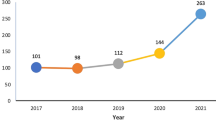
Text mining applied to distance higher education: A systematic literature review
Data availability.
We make sure that all data and materials support our published claims and comply with field standards.
Akçapınar, G. (2015). How automated feedback through text mining changes plagiaristic behavior in online assignments. Computers & Education , 87 , 123–130. https://doi.org/10.1016/j.compedu.2015.04.007
Article Google Scholar
Arispe, M., Capucao, J., Relucio, F., & Maligat, D. E. Jr. (2019). Teachers’ sentiments to Bikol MTB-MLE: Using sentiment analysis and text mining techniques. International Journal of Research Studies in Education , 8 (4), 21–26. https://doi.org/10.5861/ijrse.2019.4906
Abuzir, Y. (2018). Innovative Model for Student Project Evaluation Based on Text Mining. International Journal of Research in Education and Science , 4 (2), 409–419. https://doi.org/10.21890/ijres.409481
Bayrak, T. (2020). A content analysis of top-ranked universities’ mission statements from five global regions. International Journal of Educational Development , 72 , 102130. https://doi.org/10.1016/j.ijedudev.2019.102130
Buenaño-Fernandez, D., González, M., Gil, D., & Luján-Mora, S. (2020). Text mining of open-ended questions in self-assessment of university teachers: An LDA topic modeling approach. Ieee Access : Practical Innovations, Open Solutions , 8 , 35318–35330
Bedenlier, S., Kondakci, Y., & Zawacki-Richter, O. (2018). Two decades of research into the internationalization of higher education: Major themes in the Journal of Studies in International Education (1997–2016). Journal of Studies in International Education , 22 (2), 108–135. https://doi.org/10.1177/1028315317710093
Baddam, S., Bingi, P., & Shuva, S. (2019). Student Evaluation of Teaching in Business Education: Discovering Student Sentiments Using Text Mining Techniques. e-Journal of Business Education and Scholarship of Teaching , 13 (3), 1–13
Google Scholar
Bozkurt, A., Koseoglu, S., & Singh, L. (2019). An analysis of peer reviewed publications on openness in education in half a century: Trends and patterns in the open hemisphere. Australasian Journal of Educational Technology , 35 (4), https://doi.org/10.14742/ajet.4252
Chong, S. W. (2019). A systematic review of written corrective feedback research in ESL/EFL contexts. Language Education & Assessment , 2 (2), 70–95. https://doi.org/10.29140/lea.v2n2.138
Chen, Z., Zhang, R., Xu, T., Yang, Y., Wang, J., & Feng, T. (2020). Emotional attitudes towards procrastination in people: A large-scale sentiment-focused crawling analysis. Computers in Human Behavior , 110 , 106391. https://doi.org/10.1016/j.chb.2020.106391
Chen, X., Zou, D., Cheng, G., & Xie, H. (2020). Detecting latent topics and trends in educational technologies over four decades using structural topic modeling: A retrospective of all volumes of Computers & Education. Computers & Education , 151 , 103855. https://doi.org/10.1016/j.compedu.2020.103855
Cronin, A., Intepe, G., Shearman, D., & Sneyd, A. (2019). Analysis using natural language processing of feedback data from two mathematics support centres. International Journal of Mathematical Education in Science and Technology , 50 (7), 1087–1103. https://doi.org/10.1080/0020739X.2019.1656831
Chee, K. N., Yahaya, N., Ibrahim, N. H., & Hasan, M. N. (2017). Review of mobile learning trends 2010- 2015: A meta-analysis. Journal of Educational Technology & Society , 20 (2), 113–126
Chung, K. S. K., & Paredes, W. C. (2015). Towards a social networks model for online learning & performance. Journal of Educational Technology & Society , 18 (3), 240–253
Çepni, S. B., & Demirel, E. T. (2016). Impact of Text-Mining and Imitating Strategies on Lexical Richness, Lexical Diversity and General Success in Second Language Writing. Turkish Online Journal of Educational Technology-TOJET , 15 (4), 61–68
Doleck, T., Basnet, R. B., Poitras, E. G., & Lajoie, S. P. (2015). Mining learner–system interaction data: implications for modeling learner behaviors and improving overlay models. Journal of Computers in Education , 2 (4), 421–447. https://doi.org/10.1007/s40692-015-0040-3
Elia, G., Solazzo, G., Lorenzo, G., & Passiante, G. (2019). Assessing learners’ satisfaction in collaborative online courses through a big data approach. Computers in Human Behavior , 92 , 589–599. https://doi.org/10.1016/j.chb.2018.04.033
Elena Gallagher, S., O’Dulain, M., O’Mahony, N., Kehoe, C., McCarthy, F., & Morgan, G. (2017). Instructor-provided summary infographics to support online learning. Educational Media International , 54 (2), 129–147. https://doi.org/10.1080/09523987.2017.1362795
Erkens, M., Bodemer, D., & Hoppe, H. U. (2016). Improving collaborative learning in the classroom: Text mining based grouping and representing. International Journal of Computer-Supported Collaborative Learning , 11 (4), 387–415. https://doi.org/10.1007/s11412-016-9243-5
Freak, A., & Miller, J. (2017). Magnifying pre-service generalist teachers’ perceptions of preparedness to teach primary school physical education. Physical Education and Sport Pedagogy , 22 (1), 51–70. https://doi.org/10.1080/17408989.2015.1112775
Fan, W., Wallace, L., Rich, S., & Zhang, Z. (2006). Tapping the power of text mining. Communications of the ACM , 49 (9), 76–82
Gašević, D., Joksimović, S., Eagan, B. R., & Shaffer, D. W. (2019). SENS: Network analytics to combine social and cognitive perspectives of collaborative learning. Computers in Human Behavior , 92 , 562–577. https://doi.org/10.1016/j.chb.2018.07.003
Geng, Z., Chen, G., Han, Y., Lu, G., & Li, F. (2020). Semantic relation extraction using sequential and tree-structured LSTM with attention. Information Sciences , 509 , 183–192
Gottipati, S., Shankararaman, V., & Lin, J. R. (2018). Text analytics approach to extract course improvement suggestions from students’ feedback. Research and Practice in Technology Enhanced Learning , 13 (1), 1–19
Gupta, V., & Lehal, G. S. (2009). A survey of text mining techniques and applications. Journal of emerging technologies in web intelligence , 1 (1), 60–76
Hotho, A., Nürnberger, A., & Paaß, G. (2005, May). A brief survey of text mining. In Ldv Forum (Vol. 20, No. 1, pp. 19–62)
Hyndman, B., Suesee, B., McMaster, N., Harvey, S., Jefferson-Buchanan, R., Cruickshank, V. … Pill, S. (2019). Physical education across the international media: A five-year analysis. Sport Education and Society . https://doi.org/10.1080/13573322.2019.1583640
Harvey, S., & Atkinson, O. (2017). One youth soccer coach’s maiden implementation of the tactical games model. Ágora para la Educación Física y el Deporte , 19 (2–3), 135–157
Howard, S. K., Yang, J., Ma, J., Maton, K., & Rennie, E. (2018). App clusters: Exploring patterns of multiple app use in primary learning contexts. Computers & Education , 127 , 154–164. https://doi.org/10.1016/j.compedu.2018.08.021
Hujala, M., Knutas, A., Hynninen, T., & Arminen, H. (2020). Improving the quality of teaching by utilizing written student feedback: A streamlined process. Computers & Education , 157 , 103965. https://doi.org/10.1016/j.compedu.2020.103965
Haynes, J. E., Miller, J. A., & Varea, V. (2016). Preservice generalist teachers enlightened approach to teaching physical education through teacher biography. Australian Journal of Teacher Education (Online) , 41 (3), 21–38. https://doi.org/10.14221/ajte.2016v41n3.2
Harvey, S., Curtner-Smith, M., & Kuklick, C. (2018). Influence of a models-based physical education teacher education program on the perspectives and practices of preservice teachers. Curriculum Studies in Health and Physical Education , 9 (3), 220–236. https://doi.org/10.1080/25742981.2018.1475246
Harvey, S., & Hyndman, B. (2018). An investigation into the reasons physical education professionals use Twitter. Journal of Teaching in Physical Education , 37 (4), 383–396. https://doi.org/10.1123/jtpe.2017-0188
Harvey, S., Pill, S., Hastie, P., & Wallhead, T. (2020). Physical education teachers’ perceptions of the successes, constraints, and possibilities associated with implementing the sport education model. Physical Education and Sport Pedagogy , 25 (5), 555–566. https://doi.org/10.1080/17408989.2020.1752650
Harvey, S., Carpenter, J. P., & Hyndman, B. P. (2020). Introduction to social media for professional development and learning in physical education and sport pedagogy. Journal of Teaching in Physical Education , 39 (4), 425–433
Intepe, G., & Shearman, D. (2020). Developing Statistical Understanding and Overcoming Anxiety via Drop-In Consultations.Statistics Education Research Journal, 19 (1)
Jo, T. (2019). Text mining. Studies in Big Data. Cham :. Springer International Publishing
Joo, S., & Cahill, M. (2018). Exploring research topics in the field of school librarianship based on text mining. School Libraries Worldwide , 24 (1), 15–28
Kim, D. H., & Pior, M. Y. (2018). A Study on the Mainstream of Real Estate Education with Core Term Analysis. Education Sciences , 8 (4), 182. https://doi.org/10.3390/educsci8040182
Koseoglu, S., & Bozkurt, A. (2018). An exploratory literature review on open educational practices. Distance education , 39 (4), 441–461. https://doi.org/10.1080/01587919.2018.1520042
Kagklis, V., Karatrantou, A., Tantoula, M., Panagiotakopoulos, C. T., & Verykios, V. S. (2015). A learning analytics methodology for detecting sentiment in student fora: A Case Study in Distance Education. European Journal of Open Distance and E-learning , 18 (2), 74–94
Liu, Q., Zhang, S., Wang, Q., & Chen, W. (2017). Mining online discussion data for understanding teachers reflective thinking. IEEE Transactions on Learning Technologies , 11 (2), 243–254
Martí-Parreño, J., Méndez‐Ibáñez, E., & Alonso‐Arroyo, A. (2016). The use of gamification in education: a bibliometric and text mining analysis. Journal of computer assisted learning , 32 (6), 663–676. https://doi.org/10.1111/jcal.12161
Machado, C. J. R., Maciel, A. M. A., Rodrigues, R. L., & Menezes, R. (2019). An approach for thematic relevance analysis applied to textual contributions in discussion forums. International Journal of Distance Education Technologies (IJDET) , 17 (3), 37–51
Ming, N. C., & Ming, V. L. (2015). Visualizing and Assessing Knowledge from Unstructured Student Writing.Technology, Instruction, Cognition & Learning, 10 (1)
Magnier-Watanabe, R., Watanabe, Y., Aba, O., & Herrig, H. (2017). Global virtual teams’ education: experiential learning in the classroom. On the Horizon , 25 (4), 267–285. https://doi.org/10.1108/OTH-02-2017-0007
Nuankaew, W., & Nuankaew, P. (2019). The study of the factors and development of educational model: The relationship between the learner context and the curriculum context in higher education. International Journal of Emerging Technologies in Learning (iJET) , 14 (21), 205–226
Okoye, K., Arrona-Palacios, A., Camacho-Zuñiga, C., Hammout, N., Nakamura, E. L., Escamilla, J., & Hosseini, S. (2020). Impact of students evaluation of teaching: a text analysis of the teachers qualities by gender. International Journal of Educational Technology in Higher Education , 17 (1), 1–27. https://doi.org/10.1186/s41239-020-00224-z
Okada, Y., Sawaumi, T., & Ito, T. (2017). Effects of Observing Model Video Presentations on Japanese EFL Learners’ Oral Performance.Electronic Journal of Foreign Language Teaching, 14 (2)
Poblete, C., Leguina, A., Masquiarán, N., & Carreño, B. (2019). Informal and non formal music experience: power, knowledge and learning in music teacher education in Chile. International Journal of Music Education , 37 (2), 272–285. https://doi.org/10.1177/0255761419836015
Park, A., Conway, M., & Chen, A. T. (2018). Examining thematic similarity, difference, and membership in three online mental health communities from Reddit: a text mining and visualization approach. Computers in human behavior , 78 , 98–112. https://doi.org/10.1016/j.chb.2017.09.001
Pei, B., Xing, W., & Lee, H. S. (2019). Using automatic image processing to analyze visual artifacts created by students in scientific argumentation. British Journal of Educational Technology , 50 (6), 3391–3404. https://doi.org/10.1111/bjet.12741
Peng, X., & Xu, Q. (2020). Investigating learners’ behaviors and discourse content in MOOC course reviews. Computers & Education , 143 , 103673. https://doi.org/10.1016/j.compedu.2019.103673
Pillutla, V. S., Tawfik, A. A., & Giabbanelli, P. J. (2020). Detecting the depth and progression of learning in massive open online courses by mining discussion data. Technology Knowledge and Learning , 25 (4), 881–898. https://doi.org/10.1007/s10758-020-09434-w
Poole, F., Clarke-Midura, J., Sun, C., & Lam, K. (2019). Exploring the pedagogical affordances of a collaborative board game in a dual language immersion classroom. Foreign Language Annals , 52 (4), 753–775
Romero, C., & Ventura, S. (2007). Educational data mining: A survey from 1995 to 2005. Expert systems with applications , 33 (1), 135–146. https://doi.org/10.1016/j.eswa.2006.04.005
Rodriguez-Andara, A., Río-Belver, R. M., Rodríguez-Salvador, M., & Lezama-Nicolás, R. (2018). Roadmapping towards sustainability proficiency in engineering education. International Journal of Sustainability in Higher Education , 19 (2), 413–438. https://doi.org/10.1108/IJSHE-06-2017-0079
Sukanya, M., & Biruntha, S. (2012, August). Techniques on text mining. In 2012 IEEE International Conference on Advanced Communication Control and Computing Technologies (ICACCCT) (pp. 269–271). IEEE. https://doi.org/10.1109/ICACCCT.2012.6320784
Song, D., Lin, H., & Yang, Z. (2007). Opinion mining in e-learning system. In 2007 IFIP international conference on network and parallel computing workshops (NPC 2007) (pp. 788-792). IEEE. https://doi.org/10.1109/NPC.2007.51
Sumathy, K. L., & Chidambaram, M. (2013). Text mining: concepts, applications, tools and issues-an overview.International Journal of Computer Applications, 80 (4)
Stupans, I., McGuren, T., & Babey, A. M. (2016). Student evaluation of teaching: A study exploring student rating instrument free-form text comments. Innovative Higher Education , 41 (1), 33–42. https://doi.org/10.1007/s10755-015-9328-5
Shen, W., & Zhang, S. (2018). Emotional Tendency Dictionary Construction for College Teaching Evaluation. International Journal of Emerging Technologies in Learning , 13 (11), https://doi.org/10.3991/ijet.v13i11.9605
Schiller, S. Z. (2016). CHAT for chat: Mediated learning in online chat virtual reference service. Computers in Human Behavior , 65 , 651–665. https://doi.org/10.1016/j.chb.2016.06.053
Tan, A. H. (1999, April). Text mining: The state of the art and the challenges. In Proceedings of the pakdd 1999 workshop on knowledge disocovery from advanced databases (Vol. 8, pp. 65–70). sn
Tseng, W. T. (2020). Mining Text in Online News Reports of COVID-19 Virus: Key Phrase Extractions and Graphic Modeling. English Teaching & Learning , 1–11. https://doi.org/10.1007/s42321-020-00070?2
Tawfik, A. A., Law, V., Ge, X., Xing, W., & Kim, K. (2018). The effect of sustained vs. faded scaffolding on students’ argumentation in ill-structured problem solving. Computers in Human Behavior , 87 , 436–449. https://doi.org/10.1016/j.chb.2018.01.035
Takagi, D., Hayashi, M., Iida, T., Tanaka, Y., Sugiyama, S., Nishizaki, H., & Morimoto, Y. (2019). Effects of dental students’ training using immersive virtual reality technology for home dental practice. Educational Gerontology , 45 (11), 670–680. https://doi.org/10.1080/03601277.2019.1686284
Tao, Y., & Xie, M. (2019). Technical Writing as a Supplement. In Restructuring Translation Education (pp. 145–156) . Springer, Singapore
Wang, Y., & Fikis, D. J. (2019). Common core state standards on Twitter: Public sentiment and opinion leaders. Educational Policy , 33 (4), 650–683. https://doi.org/10.1177/0895904817723739
Wang, S. (2017). Determinants of mobile apps downloads: A systematic literature review. In The European Conference on Information Systems Management (pp. 353–360). Academic Conferences International Limited
Wu, J. Y., Hsiao, Y. C., & Nian, M. W. (2020). Using supervised machine learning on large-scale online Forums to classify course-related Facebook messages in predicting learning achievement within the personal learning environment. Interactive Learning Environments , 28 (1), 65–80. https://doi.org/10.1080/10494820.2018.1515085
Wu, P., Yu, S., & Wang, D. (2018). Using a Learner-Topic Model for Mining Learner Interests in Open Learning. Educational Technology & Society , 21 (2), 192–204
Wu, F., & Lai, S. (2019). Linking prediction with personality traits: a learning analytics approach. Distance Education , 40 (3), 330–349. https://doi.org/10.1080/01587919.2019.1632170
Wook, M., Razali, N. A. M., Ramli, S., Wahab, N. A., Hasbullah, N. A., Zainudin, N. M., & Talib, M. L. (2019). Opinion mining technique for developing student feedback analysis system using lexicon- based approach (OMFeedback). Education and Information Technologies , 1–12. https://doi.org/10.1007/s10639-019-10073-7
Wook, M., Razali, N. A. M., Ramli, S., Wahab, N. A., Hasbullah, N. A., Zainudin, N. M., & Talib, M. L. (2020). Opinion mining technique for developing student feedback analysis system using lexicon-based approach (OMFeedback). Education and Information Technologies , 25 (4), 2549–2560
Xing, W., & Gao, F. (2018). Exploring the relationship between online discourse and commitment in Twitter professional learning communities. Computers & Education , 126 , 388–398. https://doi.org/10.1016/j.compedu.2018.08.010
Xie, K., Di Tosto, G., Lu, L., & Cho, Y. S. (2018). Detecting leadership in peer-moderated online collaborative learning through text mining and social network analysis. The Internet and Higher Education , 38 , 9–17. https://doi.org/10.1016/j.iheduc.2018.04.002
Yim, S., & Warschauer, M. (2017). Web-based collaborative writing in L2 contexts: Methodological insights from text mining. Language Learning & Technology , 21 (1), 146–165
Zanini, N., & Dhawan, V. (2015). Text Mining: An introduction to theory and some applications. Research Matters , 19 , 38–45
Zawacki-Richter, O., & Latchem, C. (2018). Exploring four decades of research in Computers & Education. Computers & Education , 122 , 136–152. https://doi.org/10.1016/j.compedu.2018.04.001
Zheng, J., Xing, W., & Zhu, G. (2019). Examining sequential patterns of self-and socially shared regulation of STEM learning in a CSCL environment. Computers & Education , 136 , 34–48. https://doi.org/10.1016/j.compedu.2019.03.005
Zuo, Z., Zhao, K., & Eichmann, D. (2017). The state and evolution of US iSchools: From talent acquisitions to research outcome. Journal of the Association for Information Science and Technology , 68 (5), 1266–1277. https://doi.org/10.1002/asi.23751
Zhang, K. (2015). Mining data from Weibo to WeChat: A comparative case study of MOOC communities on social media in China. International Journal on E-Learning , 14 (3), 305–329
Download references
Not applicable.
Author information
Authors and affiliations.
Department of Learning Technologies, University of North Texas, Denton, TX, USA
Junhe Yang, Kinshuk & Yunjo An
Department of Learning Technologies, University of North Texas, 3940 N. Elm St., Suite G150, UNT College of Information, Denton, TX, 76207, USA
You can also search for this author in PubMed Google Scholar
Corresponding author
Correspondence to Junhe Yang .
Ethics declarations
Conflict of interest.
The author declares no competing interests.
Additional information
Publisher’s note.
Springer Nature remains neutral with regard to jurisdictional claims in published maps and institutional affiliations.
Rights and permissions
Reprints and permissions
About this article
Yang, J., Kinshuk & An, Y. A survey of the literature: how scholars use text mining in Educational Studies?. Educ Inf Technol 28 , 2071–2090 (2023). https://doi.org/10.1007/s10639-022-11193-3
Download citation
Received : 05 September 2021
Accepted : 28 June 2022
Published : 12 August 2022
Issue Date : February 2023
DOI : https://doi.org/10.1007/s10639-022-11193-3
Share this article
Anyone you share the following link with will be able to read this content:
Sorry, a shareable link is not currently available for this article.
Provided by the Springer Nature SharedIt content-sharing initiative
- Educational text mining
- Literature review
- Educational technology
- Find a journal
- Publish with us
- Track your research
Subscribe to the PwC Newsletter
Join the community, add a new evaluation result row, topic models.
212 papers with code • 6 benchmarks • 12 datasets
A topic model is a type of statistical model for discovering the abstract "topics" that occur in a collection of documents. Topic modeling is a frequently used text-mining tool for the discovery of hidden semantic structures in a text body.

Benchmarks Add a Result
Trend | Dataset | Best Model | -->Paper | Code | Compare |
---|---|---|---|---|---|
DeTiME | -->|||||
vONTSS | -->|||||
Bayesian SMM | -->|||||
JoSH | -->|||||
JoSH | -->|||||
vONTSS | -->

Latest papers
Modeling dynamic topics in chain-free fashion by evolution-tracking contrastive learning and unassociated word exclusion.

However, existing models suffer from repetitive topic and unassociated topic issues, failing to reveal the evolution and hindering further applications.
FASTopic: A Fast, Adaptive, Stable, and Transferable Topic Modeling Paradigm
This brings about a neat and efficient topic modeling framework.
Concept Induction: Analyzing Unstructured Text with High-Level Concepts Using LLooM
michelle123lam/lloom • 18 Apr 2024
Data analysts have long sought to turn unstructured text data into meaningful concepts.
GINopic: Topic Modeling with Graph Isomorphism Network
Topic modeling is a widely used approach for analyzing and exploring large document collections.
Enhanced Short Text Modeling: Leveraging Large Language Models for Topic Refinement
Crafting effective topic models for brief texts, like tweets and news headlines, is essential for capturing the swift shifts in social dynamics.
Neural Multimodal Topic Modeling: A Comprehensive Evaluation
gonzalezf/multimodal_neural_topic_modeling • 26 Mar 2024
This paper presents the first systematic and comprehensive evaluation of multimodal topic modeling of documents containing both text and images.
Automating the Information Extraction from Semi-Structured Interview Transcripts
This paper explores the development and application of an automated system designed to extract information from semi-structured interview transcripts.
Membership Inference Attacks and Privacy in Topic Modeling
nicomanzonelli/topic_model_attacks • 7 Mar 2024
Recent research shows that large language models are susceptible to privacy attacks that infer aspects of the training data.
Network-based Topic Structure Visualization
jeon9677/gviz • 31 Jan 2024
In the real world, many topics are inter-correlated, making it challenging to investigate their structure and relationships.
Improving the TENOR of Labeling: Re-evaluating Topic Models for Content Analysis
zli12321/tenor • 29 Jan 2024
Topic models are a popular tool for understanding text collections, but their evaluation has been a point of contention.
Information
- Author Services
Initiatives
You are accessing a machine-readable page. In order to be human-readable, please install an RSS reader.
All articles published by MDPI are made immediately available worldwide under an open access license. No special permission is required to reuse all or part of the article published by MDPI, including figures and tables. For articles published under an open access Creative Common CC BY license, any part of the article may be reused without permission provided that the original article is clearly cited. For more information, please refer to https://www.mdpi.com/openaccess .
Feature papers represent the most advanced research with significant potential for high impact in the field. A Feature Paper should be a substantial original Article that involves several techniques or approaches, provides an outlook for future research directions and describes possible research applications.
Feature papers are submitted upon individual invitation or recommendation by the scientific editors and must receive positive feedback from the reviewers.
Editor’s Choice articles are based on recommendations by the scientific editors of MDPI journals from around the world. Editors select a small number of articles recently published in the journal that they believe will be particularly interesting to readers, or important in the respective research area. The aim is to provide a snapshot of some of the most exciting work published in the various research areas of the journal.
Original Submission Date Received: .
- Active Journals
- Find a Journal
- Proceedings Series
- For Authors
- For Reviewers
- For Editors
- For Librarians
- For Publishers
- For Societies
- For Conference Organizers
- Open Access Policy
- Institutional Open Access Program
- Special Issues Guidelines
- Editorial Process
- Research and Publication Ethics
- Article Processing Charges
- Testimonials
- Preprints.org
- SciProfiles
- Encyclopedia
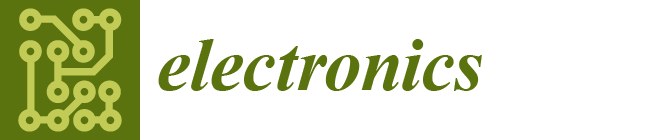
Article Menu

- Subscribe SciFeed
- Recommended Articles
- Google Scholar
- on Google Scholar
- Table of Contents
Find support for a specific problem in the support section of our website.
Please let us know what you think of our products and services.
Visit our dedicated information section to learn more about MDPI.
JSmol Viewer
Design research insights on text mining analysis: establishing the most used and trends in keywords of design research journals.
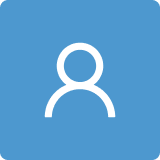
1. Introduction
2. design research, 2.1. design research dimensions, 2.2. research methods conducted in the design, 3. research methodology.
- RQ1: What are the major design research topics observed in the dataset?
- RQ2: What changes in design studies were observed during the sample period from January 2007 to March 2019?
- RQ3: What are the vital design research topics that determine the direction of future research?
4. Data Analysis and Discussion
4.1. descriptive analysis, 4.2. text mining analysis (clustering), 4.3. clustering results.
- Co-creation: for instance, that work is related to design thinking, innovation, creativity, design process, and design.
- Co-innovation: increasing the number in design research fields is a hot topic, and it seems very motivating as it appeared in three periods with a greater concern on collaborative design.
- Ethical design: offer new insights/knowledge about the design process within design research.
- Social practice design: it is essential to associate terms that focus on its adoption and other elements affecting the area—research related to participatory design, collaboration, sustainability, design innovation, and articulating design.
4.4. Word Frequency Distribution
5. conclusions, 5.1. contributions, 5.2. limitations, supplementary materials, author contributions, data availability statement, conflicts of interest, appendix a. the most popular terms.
creativity | 47 | 48 | 68 |
product design | 35 | 39 | 48 |
design process | 31 | 35 | 49 |
design education | 30 | 52 | 97 |
collaborative design | 29 | 23 | 27 |
design | 24 | 40 | 117 |
conceptual design | 26 | 32 | 20 |
collaborative design | 23 | 25 | 25 |
technology education | 26 | 25 | 15 |
engineering design | 29 | 30 | 48 |
innovation | 17 | 19 | 26 |
design cognition | 19 | 23 | 28 |
design theory | 16 | 32 | 12 |
industrial design | 16 | 18 | 12 |
communication | 14 | 10 | 30 |
design practice | 0 | 17 | 18 |
problem solving | 13 | 0 | 0 |
aesthetics | 13 | 10 | 21 |
interaction design | 13 | 13 | 21 |
product development | 12 | 10 | 0 |
case study | 12 | 18 | 0 |
design methods | 11 | 15 | 23 |
architectural design | 11 | 15 | 0 |
evaluation | 11 | 12 | 16 |
design activity | 11 | 17 | 0 |
creative design | 10 | 0 | 0 |
teamwork | 10 | 3 | 0 |
service design | 9 | 9 | 43 |
technological literacy | 9 | 0 | 0 |
research methods | 9 | 10 | 10 |
design tools | 9 | 11 | 24 |
design research | 9 | 16 | 54 |
participatory design | 9 | 18 | 59 |
design methodology | 8 | 8 | 0 |
collaboration | 8 | 14 | 32 |
curriculum | 8 | 9 | 0 |
sustainability design | 8 | 10 | 51 |
interface design | 8 | 0 | 0 |
learning | 7 | 0 | 18 |
technology | 7 | 0 | 19 |
architecture | 7 | 0 | 0 |
culture | 7 | 0 | 0 |
design strategy | 7 | 7 | 0 |
graphic design | 7 | 0 | 0 |
emotion | 7 | 0 | 0 |
perception | 7 | 8 | 0 |
philosophy of design | 7 | 8 | 0 |
user participation | 7 | 0 | 0 |
product experience | 7 | 0 | 0 |
healthcare | 0 | 0 | 25 |
interdisciplinary | 0 | 0 | 21 |
craft | 0 | 0 | 22 |
design fiction | 0 | 0 | 21 |
circular economy | 0 | 0 | 20 |
design management | 0 | 17 | 18 |
usability design | 0 | 0 | 17 |
user-centered design | 0 | 0 | 17 |
speculative design | 0 | 0 | 17 |
participation | 0 | 0 | 17 |
social design | 0 | 0 | 16 |
decision making | 0 | 0 | 16 |
product development | 0 | 0 | 10 |
inclusive design | 0 | 0 | 8 |
epistemology | 0 | 0 | 8 |
art education | 0 | 11 | 16 |
empathy | 0 | 0 | 16 |
pedagogy | 0 | 0 | 16 |
epistemology | 0 | 0 | 8 |
co-innovation | 0 | 3 | 23 |
ethical design | 0 | 6 | 19 |
social practice design | 0 | 2 | 21 |
Appendix B. List of Abbreviations
CAD | computer-aided design |
ICT | Information and Communication Technology |
IT | Information technology |
MDO | Multidisciplinary Design Optimization |
AM | Additive manufacturing |
ABSA | The aspect-based sentiment analysis |
MTMVN | multitask Multiview network |
SOTA | Most State-Of-The-Art |
NMT | Neural Machine Translation |
LRLs | Low-Resource Languages |
TL | Transfer Learning |
CDCAT | Cross-Document Coreference Annotation Tool |
ESCI | Emerging Source Citation Index |
CSV | excel sheet |
HTML | Hypertext Markup Language |
XML | extensible markup language |
TF-IDF | term frequency-inverse document frequency |
- Gemser, G.; de Bont, C. Design-Related and Design-Focused Research: A Study of Publication Patterns in Design Journals. She Ji 2016 , 2 , 46–58. [ Google Scholar ] [ CrossRef ] [ Green Version ]
- Cooper, R. Editorial: Moving design forward. Des. J. 2008 , 11 , 5–7. [ Google Scholar ] [ CrossRef ]
- Atkinson, P. The Design Journal and the Meaning of Design. Des. J. 2017 , 20 , 1–4. [ Google Scholar ] [ CrossRef ]
- Cooper, R. Design Research: Past, Present and Future. Des. J. 2017 , 20 , 5–11. [ Google Scholar ] [ CrossRef ]
- Giacomin, J. What is Design for Meaning? J. Des. Bus. Soc. 2017 , 3 , 167–190. [ Google Scholar ] [ CrossRef ] [ PubMed ]
- Cooper, R. Design Research Comes of Age. Des. J. 1997 , 1 (Suppl. 1), 1. [ Google Scholar ] [ CrossRef ]
- Ralph, P.; Wand, Y. A proposal for a formal definition of the design concept. In Design Requirements Engineering: A Ten-Year Perspective ; Lecture Notes in Business Information Processing; Springer: Berlin/Heidelberg, Germany, 2009; Volume 14, pp. 103–136. [ Google Scholar ] [ CrossRef ]
- Cross, N. Developing design as a discipline. J. Eng. Des. 2018 , 29 , 691–708. [ Google Scholar ] [ CrossRef ]
- Overkamp, T.; Blomkvist, J.; Rodrigues, V.; Arvola, M.; Holmlid, S. Resource Integration as a Perspective on Value in Interaction Design. In Proceedings of the 32nd International BCS Human Computer Interaction Conference, Belfast, UK, 4–6 July 2018. [ Google Scholar ]
- Jain, A.K. Data clustering: 50 years beyond K-means. Pattern Recognit. Lett. 2010 , 31 , 651–666. [ Google Scholar ] [ CrossRef ]
- Cross, N. Designerly ways of knowing Journal Item. Des. Stud. 1982 , 3 , 221–227. [ Google Scholar ] [ CrossRef ]
- Christensen, B.T.; Ball, L.J. Building a discipline: Indicators of expansion, integration and consolidation in design research across four decades. Des. Stud. 2019 , 65 , 18–34. [ Google Scholar ] [ CrossRef ]
- Liedtka, J. Perspective: Linking Design Thinking with Innovation Outcomes through Cognitive Bias Reduction. J. Prod. Innov. Manag. 2015 , 32 , 925–938. [ Google Scholar ] [ CrossRef ]
- Bremner, C.; Rodgers, P. Design Without Discipline. Des. Issues 2013 , 29 , 4–13. [ Google Scholar ] [ CrossRef ] [ Green Version ]
- Cash, P.J. Developing theory-driven design research. Des. Stud. 2018 , 56 , 84–119. [ Google Scholar ] [ CrossRef ] [ Green Version ]
- Dorst, K. The core of design thinking and its application. Des. Stud. 2011 , 32 , 521–532. [ Google Scholar ] [ CrossRef ]
- Brown, T. Change by Design: How Design Thinking Transforms Organizations and Inspires Innovation ; HarperCollins Publishers: New York, NY, USA, 2009. [ Google Scholar ]
- Chamberlain, P.; Bonsiepe, G.; Cross, N.; Keller, I.; Frens, J.; Buchanan, R.; Schneider, B. Design Research Now: Essays and Selected Projects ; Walter de Gruyter: Berlin, Germany, 2012. [ Google Scholar ]
- Grudin, J. Interface: An evolving concept. Commun. ACM 1993 , 36 , 110–119. [ Google Scholar ] [ CrossRef ]
- Hirtz, J.; Stone, R.B.; McAdams, D.A.; Szykman, S.; Wood, K.L. A functional basis for engineering design: Reconciling and evolving previous efforts. Res. Eng. Des. 2002 , 13 , 65–82. [ Google Scholar ] [ CrossRef ]
- Johnson, C. Strategic planning for post-disaster temporary housing. Disasters 2007 , 31 , 435–458. [ Google Scholar ] [ CrossRef ]
- Chai, K.H.; Xiao, X. Understanding design research: A bibliometric analysis of Design Studies (1996–2010). Des. Stud. 2012 , 33 , 24–43. [ Google Scholar ] [ CrossRef ]
- Riaz, M.; Breaux, T.; Williams, L. How have we evaluated software pattern application? A systematic mapping study of research design practices. Inf. Softw. Technol. 2015 , 65 , 14–38. [ Google Scholar ] [ CrossRef ]
- Chakrabarti, A. Towards a taxonomy of design research areas. In The future of Design Methodology ; Springer: London, UK, 2011; pp. 249–259. [ Google Scholar ] [ CrossRef ]
- Caussade, S.; de Dios Ortúzar, J.; Rizzi, L.I.; Hensher, D.A. Assessing the influence of design dimensions on stated choice experiment estimates. Transp. Res. Part B Methodol. 2005 , 39 , 621–640. [ Google Scholar ] [ CrossRef ]
- Fan, Z.; Ge, Y. The Influence of Techno ethics on Industrial Design. MATEC Web Conf. 2018 , 167 , 01008. [ Google Scholar ] [ CrossRef ] [ Green Version ]
- Hernández, R.J.; Cooper, R.; Tether, B.; Murphy, E. Design, the language of innovation: A review of the design studies literature. She Ji J. Des. Econ. Innov. 2018 , 4 , 249–274. [ Google Scholar ] [ CrossRef ]
- Lee, D.; Lee, H. Mapping the Characteristics of Design Research in Social Sciences. Arch. Des. Res. 2019 , 32 , 39–51. [ Google Scholar ] [ CrossRef ]
- Nusir, M.; Tariq, U.; Ahanger, T.A. Engaging Diverse Stakeholders in Interdisciplinary Co-Design Project for Better Service Design. J. Cases Inf. Technol. (JCIT) 2021 , 23 , 1–29. [ Google Scholar ] [ CrossRef ]
- Bentley, R.A. Random Drift Versus Selection in Academic Vocabulary: An Evolutionary Analysis of Published Keywords. 2008. Available online: https://www.ncbi.nlm.nih.gov/pmc/articles/PMC2518107/ (accessed on 13 March 2019).
- Evans, M. Design Thinking: Understanding How Designers Think and Work by Nigel Cross. Des. J. 2012 , 15 , 141–143. [ Google Scholar ] [ CrossRef ]
- Wang, L.H.; Wang, Q.; Zhang, X.; Cai, W.; Sun, X. A bibliometric analysis of anaerobic digestion for methane research during the period 1994–2011. J. Mater. Cycles Waste Manag. 2013 , 15 , 1–8. [ Google Scholar ] [ CrossRef ]
- Yao, X.; Moon, S.K.; Bi, G. Multidisciplinary design optimization to identify additive manufacturing resources in customized product development. J. Comput. Des. Eng. 2017 , 4 , 131–142. [ Google Scholar ] [ CrossRef ] [ Green Version ]
- Nie, B.; Sun, S. Using text mining techniques to identify research trends: A case study of design research. Appl. Sci. 2017 , 7 , 401. [ Google Scholar ] [ CrossRef ]
- Andreasen, M.M. 45 Years with design methodology. J. Eng. Des. 2011 , 22 , 293–332. [ Google Scholar ] [ CrossRef ]
- Johnson, J.; Cook, M. Policy Design: A New Area of Design Research and Practice. In Complex Systems Design and Management ; Springer: Cham, Switzerland, 2014; pp. 51–62. [ Google Scholar ] [ CrossRef ]
- Kavousi, S.; Miller, P.A.; Alexander, P.A. Modeling metacognition in design thinking and design making. Int. J. Technol. Des. Educ. 2020 , 30 , 709–735. [ Google Scholar ] [ CrossRef ]
- Lloyd, P. From Design Methods to Future-Focused Thinking: 50 years of design research. Des. Stud. 2017 , 48 , A1–A8. [ Google Scholar ] [ CrossRef ]
- Yong, B.; Yang, Y. A multitask multiview neural network for end-to-end aspect-based sentiment analysis. Big Data Min. Anal. 2021 , 4 , 195–207. [ Google Scholar ] [ CrossRef ]
- Maimaiti, M.; Liu, Y.; Luan, H.; Sun, M. Enriching the transfer learning with pre-trained lexicon embedding for low-resource neural machine translation. Tsinghua Sci. Technol. 2022 , 27 , 150–163. [ Google Scholar ] [ CrossRef ]
- Xu, Y.; Xia, B.; Wan, Y.; Zhang, F.; Xu, J.; Ning, H. CDCAT: A multi-language cross-document entity and event coreference annotation tool. Tsinghua Sci. Technol. 2022 , 27 , 589–598. [ Google Scholar ] [ CrossRef ]
- Shen, L.; Liu, Q.; Chen, G.; Ji, S. Text-based price recommendation system for online rental houses. Big Data Min. Anal. 2020 , 3 , 143–152. [ Google Scholar ] [ CrossRef ]
- Sawsan, A.; Jaradat, R. Clarification of research design, research methods, and research methodology: A guide for public administration researchers and practitioners. Teach. Public Adm. 2018 , 36 , 237–258.–258. [ Google Scholar ] [ CrossRef ]
- Hicks, B. The language of collaborative engineering projects. In Proceedings of the 19th International Conference on Engineering Design (ICED13), Seoul, Korea, 19–22 August 2013; pp. 321–330. [ Google Scholar ] [ CrossRef ]
- Aggarwal, C.C.; Zhai, C.X. Aggarwal, C.C.; Zhai, C.X. A survey of text clustering algorithms. In Mining Text Data ; Springer US.: Boston, MA, USA, 2012; pp. 77–128. [ Google Scholar ] [ CrossRef ] [ Green Version ]
- Bekkerman, R.; El-Yaniv, R.; Winter, Y.; Tishby, N. On feature distributional clustering for text categorization. In Proceedings of the 24th Annual International ACM SIGIR Conference on Research and Development in Information Retrieval, New Orleans, LA, USA, 9–13 September 2001; pp. 146–153. [ Google Scholar ] [ CrossRef ]
- Abu-Shanab, E.; Harb, Y. E-government research insights: Text mining analysis. Electron. Commer. Res. Appl. 2019 , 38 , 100892. [ Google Scholar ] [ CrossRef ]
- Bird, S.; Klein, E.; Loper, E. Natural Language Processing with Python. 2009. Available online: https://books.google.com.au/books/about/Natural_Language_Processing_with_Python.html?id=KGIbfiiP1i4C&source=kp_book_description&redir_esc=y (accessed on 19 March 2020).
- Cielen, D.; Meysman, A.; Ali, M. Introducing Data Science: Big Data, Machine Learning, and More, Using Python Tools. 2016. Available online: https://dl.acm.org/citation.cfm?id=3051941 (accessed on 19 March 2020).
- Haddi, E.; Liu, X.; Shi, Y. The role of text pre-processing in sentiment analysis. Procedia Comput. Sci. 2013 , 17 , 26–32. [ Google Scholar ] [ CrossRef ] [ Green Version ]
- Pham, D.T.; Dimov, S.S.; Nguyen, C.D. Selection of K in K-means clustering. Proc. Inst. Mech. Eng. Part C J. Mech. Eng. Sci. 2005 , 219 , 103–119. [ Google Scholar ] [ CrossRef ] [ Green Version ]
- Abu-Shanab, E.A.; Abu-Baker, A.N. Using and buying mobile phones in Jordan: Implications for future research and the Development of New Methodology. Technol. Soc. 2014 , 38 , 103–110. [ Google Scholar ] [ CrossRef ]
- Salton, G.; Wong, A.; Yang, C.S. A Vector Space Model for Automatic Indexing. Commun. ACM 1975 , 18 , 613–620. [ Google Scholar ] [ CrossRef ] [ Green Version ]
- Haug, A. Four dimensions of product designs. J. Des. Res. 2015 , 13 , 20–35. [ Google Scholar ] [ CrossRef ]
Code | Journal Name | Total Articles | Total Keywords |
---|---|---|---|
1 | Research in engineering design | 271 | 1315 |
2 | International Journal of technology and design education | 441 | 2185 |
3 | Design studies | 390 | 1798 |
4 | Design journal | 1152 | 5271 |
5 | Co-design-International journal of co-creation in design and the arts | 186 | 955 |
6 | Journal of Engineering design | 275 | 1288 |
7 | International journal of design | 252 | 1218 |
8 | Journal of Engineering design and technology | 231 | 1255 |
9 | Ergonomics in design | 81 | 677 |
10 | International journal of art and design education | 274 | 1524 |
Keyword | Keyword | Keyword Count |
---|---|---|
0 | Designeducation | 185 |
1 | design | 181 |
2 | Creativity | 163 |
3 | product design | 122 |
4 | co-design | 117 |
5 | Designprocess | 115 |
6 | Participtorydesign | 108 |
7 | Innovation | 107 |
8 | Designthinking | 97 |
9 | Technologyeducation | 87 |
10 | Designresearch | 86 |
11 | Collaborativeresearch | 79 |
12 | Conceptualdesign | 78 |
13 | Sustainability | 77 |
14 | Designcognition | 74 |
15 | Designtheory | 70 |
16 | Servicedesign | 69 |
17 | Engineeringdesign | 66 |
18 | Industrialdesign | 66 |
19 | Designpractice | 64 |
20 | Interactiondesign | 62 |
21 | Collaboration | 58 |
22 | Designmethods | 54 |
23 | Designtools | 49 |
24 | Casestudy | 48 |
25 | Education | 45 |
26 | Aesthetics | 44 |
27 | Communication | 44 |
28 | Designactivity | 42 |
29 | Evaluation | 42 |
30 | Architecturaldesign | 42 |
31 | Socialinnovation | 42 |
32 | Productdevelopment | 39 |
33 | Designknowledge | 37 |
34 | Learning | 36 |
35 | Pedagogy | 35 |
36 | Technology | 35 |
37 | Arteducation | 35 |
38 | Protocolanalysis | 34 |
39 | Designmanegment | 33 |
40 | Researchmethods | 33 |
41 | Architecture | 32 |
42 | Usability | 31 |
43 | Participation | 31 |
44 | Healthcare | 31 |
45 | Designmethodology | 30 |
46 | user-centereddesign | 30 |
47 | Problemsolving | 29 |
48 | Simulation | 28 |
49 | Sustainabledesign | 28 |
Term | 2007–2011 | 2012–2015 | 2016–2019 |
---|---|---|---|
0 creativity | 40 | 48 | 68 |
1 product design | 30 | 31 | 48 |
2 design process | 27 | 31 | 49 |
3 design education | 25 | 49 | 97 |
4 collaborative design | 24 | 18 | 27 |
5 design | 23 | 40 | 117 |
6 conceptual design | 23 | 27 | 20 |
7 technology education | 23 | 25 | 25 |
8 engineering design | 21 | 19 | 15 |
9 innovation | 18 | 24 | 30 |
10 design cognition | 17 | 19 | 26 |
11 design theory | 16 | 20 | 28 |
12 industrial design | 16 | 32 | 12 |
13 communication | 14 | 18 | 10 |
14 design practice | 14 | 10 | 30 |
15 design management | 0 | 17 | 18 |
16 problem solving | 13 | 0 | 0 |
17 aesthetics | 13 | 10 | 21 |
Terms | 2007–2011 | 2012–2015 | 2016–2019 | Trend Lines | General Term |
---|---|---|---|---|---|
Service design | 9 | 9 | 43 | Co-design method and approach | |
Design tools | 9 | 11 | 24 | ||
Design research | 9 | 16 | 54 | ||
Participatory design | 9 | 18 | 59 | ||
Design methodology | 8 | 8 | 0 | ||
Collaboration | 8 | 14 | 32 | ||
Participation | 0 | 0 | 17 | ||
Design graphic | 7 | 0 | 0 | Anthropomorphic design | |
Usability design | 0 | 0 | 17 | ||
Emotion | 7 | 0 | 0 | ||
Perception | 7 | 8 | 0 | ||
Interface design | 8 | 0 | 0 | ||
Philosophy of design | 7 | 8 | 0 | Assumptions, foundations and implications of design | |
Design fiction | 0 | 0 | 21 | ||
Technology design | 7 | 0 | 19 | ||
Design strategy | 7 | 7 | 0 | Eco-design strategy | |
Sustainability design | 8 | 10 | 51 | ||
User participation | 7 | 0 | 0 | Co-innovation | |
User-centered design | 0 | 0 | 17 | ||
Co-innovation | 0 | 3 | 23 | ||
Product experience | 7 | 0 | 0 | Co-production | |
Product development | 0 | 0 | 10 | ||
Design management | 0 | 17 | 18 | ||
Ethical design | 0 | 6 | 19 | Social-practice design | |
Inclusive design | 0 | 0 | 8 | ||
Speculative design | 0 | 0 | 17 | ||
Social design | 0 | 0 | 16 | ||
Social practice design | 0 | 2 | 21 |
MDPI stays neutral with regard to jurisdictional claims in published maps and institutional affiliations. |
Share and Cite
Nusir, M.; Louati, A.; Louati, H.; Tariq, U.; Zitar, R.A.; Abualigah, L.; Gandomi, A.H. Design Research Insights on Text Mining Analysis: Establishing the Most Used and Trends in Keywords of Design Research Journals. Electronics 2022 , 11 , 3930. https://doi.org/10.3390/electronics11233930
Nusir M, Louati A, Louati H, Tariq U, Zitar RA, Abualigah L, Gandomi AH. Design Research Insights on Text Mining Analysis: Establishing the Most Used and Trends in Keywords of Design Research Journals. Electronics . 2022; 11(23):3930. https://doi.org/10.3390/electronics11233930
Nusir, Muneer, Ali Louati, Hassen Louati, Usman Tariq, Raed Abu Zitar, Laith Abualigah, and Amir H. Gandomi. 2022. "Design Research Insights on Text Mining Analysis: Establishing the Most Used and Trends in Keywords of Design Research Journals" Electronics 11, no. 23: 3930. https://doi.org/10.3390/electronics11233930
Article Metrics
Article access statistics, supplementary material.
ZIP-Document (ZIP, 386 KiB)
Further Information
Mdpi initiatives, follow mdpi.
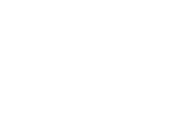
Subscribe to receive issue release notifications and newsletters from MDPI journals
Academia.edu no longer supports Internet Explorer.
To browse Academia.edu and the wider internet faster and more securely, please take a few seconds to upgrade your browser .
- We're Hiring!
- Help Center
Text-mining
- Most Cited Papers
- Most Downloaded Papers
- Newest Papers
- Save to Library
- Themes Follow Following
- Sampling Follow Following
- Bioinformatics Follow Following
- Social Media Follow Following
- Data mining and Text mining Follow Following
- Giovani Follow Following
- Text Mining, Document clustering, Data Mining Follow Following
- Analisi Testuale Follow Following
- Natural Language Processing Text Mining Follow Following
- Natural Language Processing Follow Following
Enter the email address you signed up with and we'll email you a reset link.
- Academia.edu Publishing
- We're Hiring!
- Help Center
- Find new research papers in:
- Health Sciences
- Earth Sciences
- Cognitive Science
- Mathematics
- Computer Science
- Academia ©2024
Thank you for visiting nature.com. You are using a browser version with limited support for CSS. To obtain the best experience, we recommend you use a more up to date browser (or turn off compatibility mode in Internet Explorer). In the meantime, to ensure continued support, we are displaying the site without styles and JavaScript.
- View all journals
- Explore content
- About the journal
- Publish with us
- Sign up for alerts
- Open access
- Published: 05 June 2024
Scaling neural machine translation to 200 languages
Nature ( 2024 ) Cite this article
26k Accesses
1 Citations
521 Altmetric
Metrics details
- Communication
- Computer science
The development of neural techniques has opened up new avenues for research in machine translation. Today, neural machine translation (NMT) systems can leverage highly multilingual capacities and even perform zero-shot translation, delivering promising results in terms of language coverage and quality. However, scaling quality NMT requires large volumes of parallel bilingual data, which are not equally available for the 7,000+ languages in the world 1 . Focusing on improving the translation qualities of a relatively small group of high-resource languages comes at the expense of directing research attention to low-resource languages, exacerbating digital inequities in the long run. To break this pattern, here we introduce No Language Left Behind—a single massively multilingual model that leverages transfer learning across languages. We developed a conditional computational model based on the Sparsely Gated Mixture of Experts architecture 2 , 3 , 4 , 5 , 6 , 7 , which we trained on data obtained with new mining techniques tailored for low-resource languages. Furthermore, we devised multiple architectural and training improvements to counteract overfitting while training on thousands of tasks. We evaluated the performance of our model over 40,000 translation directions using tools created specifically for this purpose—an automatic benchmark (FLORES-200), a human evaluation metric (XSTS) and a toxicity detector that covers every language in our model. Compared with the previous state-of-the-art models, our model achieves an average of 44% improvement in translation quality as measured by BLEU. By demonstrating how to scale NMT to 200 languages and making all contributions in this effort freely available for non-commercial use, our work lays important groundwork for the development of a universal translation system.
Similar content being viewed by others
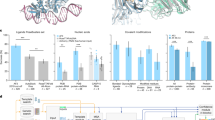
Accurate structure prediction of biomolecular interactions with AlphaFold 3
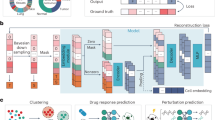
Large-scale foundation model on single-cell transcriptomics
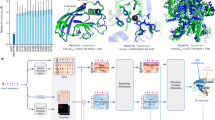
Highly accurate protein structure prediction with AlphaFold
The recent advent of neural machine translation (NMT) has pushed translation technologies to new frontiers, but its benefits are unevenly distributed 1 . The vast majority of improvements made have mainly benefited high-resource languages, leaving many low-resource languages behind. (For the purpose of our research, we define a high-resource language as a language for which we have at least 1 million sentences of aligned textual data (or bitext) with another language). This disparity could largely be attributed to a data gap: NMT models typically require large volumes of data to produce quality translations and, by definition, these volumes are not available for lower-resource languages. The No Language Left Behind (NLLB-200) project seeks to overcome this limitation by leveraging previously unknown approaches for building massively multilingual models with cross-lingual transfer abilities 8 , 9 , thereby enabling related languages to learn from each other 1 , 10 , 11 .
It has now been widely acknowledged that multilingual models have demonstrated promising performance improvement over bilingual models 12 . However, the question remains whether massively multilingual models can enable the representation of hundreds of languages without compromising quality. Our results demonstrate that doubling the number of supported languages in machine translation and maintaining output quality are not mutually exclusive endeavours. Our final model—which includes 200 languages and three times as many low-resource languages as high-resource ones—performs, as a mean, 44% better than the previous state-of-the-art systems. This paper presents some of the most important data-gathering, modelling and evaluation techniques used to achieve this goal.
First, compared with their high-resource counterparts, training data for low-resource languages are expensive and logistically challenging to procure 13 , 14 , 15 . Publicly available digital resources are either limited in volume or difficult for automated systems to detect (particularly in large public web datasets such as CommonCrawl). Regardless of whether collecting a critical mass of human-translated seed data is necessary, sufficient data acquisition relies on large-scale data mining and monolingual data pipelines 16 , 17 , 18 , 19 . The latter techniques are often affected by noise and biases, thereby making validating the quality of the datasets they generate tedious 20 . In NLLB-200, we show that a distillation-based sentence encoding technique, LASER3 (ref. 21 ), facilitates the effective mining of parallel data for low-resource languages.
Second, on the modelling side, we use an assemblage of seed, mined, open-source and back-translated datasets to train multilingual conditional computational models (more specifically, Sparsely Gated Mixtures-of-Experts models 2 , 3 , 4 , 5 , 6 , 7 that enable cross-lingual transfer between related languages without increasing interference between unrelated languages). We show how we can achieve state-of-the-art performance with a more optimal trade-off between cross-lingual transfer and interference, and improve performance for low-resource languages.
Finally, for the purpose of quality evaluation, we created FLORES-200—a massive multilingual benchmark that enables the measurement of translation quality across any of the approximately 40,000 translation directions covered by the NLLB-200 models. Apart from automatic metrics, we also created Cross-lingual Semantic Text Similarity (XSTS) and Evaluation of Toxicity (ETOX). XSTS is a human evaluation protocol that provides consistency across languages; ETOX is a tool to detect added toxicity in translations using toxicity word lists.
Beyond creating these models, we also reflect on the potential societal impact of NLLB. To amplify the practical applicability of our work in service of low-resource-speaking communities, we provide all the benchmarks, data, code and models described in this effort as resources freely available for non-commercial use ( https://github.com/facebookresearch/fairseq/tree/nllb ) (see Data and Code availability statements for details).
Automatically creating translation training data
The current techniques used for training translation models are difficult to extend to low-resource settings, in which aligned bilingual textual data (or bitext data) are relatively scarce 22 . Many low-resource languages are supported only by small targeted bitext data consisting primarily of translations of the Christian Bible 23 , which provide limited domain diversity.
To build a large-scale parallel training dataset that covers hundreds of languages, our approach centres around extending existing datasets by first collecting non-aligned monolingual data. Then, we used a semantic sentence similarity metric to guide a large-scale data mining effort aiming to identify sentences that have a high probability of being semantically equivalent in different languages 18 .
Language identification for monolingual data collection
Collecting monolingual data at scale requires a language identification (LID) system that accurately classifies textual resources for all NLLB-200 languages. Although LID could be seen as a solved problem in some domains 24 , it remains an open challenge for web data 25 , 26 . Specifically, issues coalesce around domain mismatch 26 , similar language disambiguation 27 and successful massively multilingual scaling 28 .
Devoted attention to advancing LID techniques led to a noticeable increase in both language coverage and accuracy over time. CLD3 ( https://github.com/google/cld3 ) and fasttext 29 are two readily available models offering high detection performance for 107 and 187 languages, respectively. By using numerous public datasets, previous studies 30 , 31 report even higher coverage—464 and 1,366 languages, respectively. Another study 32 scales LID performance up to 1,629 languages using word lists and self-supervision to bootstrap training data found on the web. However, these approaches using found data suffer from domain imbalance. That is, because the available text domains vary by language, classifiers conflate different domains with different languages.
In our work, we curated FLORES-200 to use as a development set so that our LID system performance 33 is tuned over a uniform domain mix. Our approach combines a data-driven fasttext model trained on FLORES-200 with a small set of handwritten rules to address human feedback on classification errors. These rules are specifically mentioned in section 5.1.3 of ref. 34 and include linguistic filters to mitigate the learning of spurious correlations due to noisy training samples while modelling hundreds of languages.
We compare our LID model with three publicly available models: CLD3, LangId ( https://github.com/saffsd/langid.py ) and LangDetect ( https://pypi.org/project/langdetect/ ). Table 1 reports the performance on three cascading sets of languages intersecting with NLLB-200: (1) 51 languages also supported by LangId, LangDetect and CLD3; (2) 78 languages also supported by LangId and CLD3; (3) 95 languages also supported by CLD3. We also report false-positive rates (FPR) to reflect the impact of false positives on unseen languages. Our results show that our model is equipped to handle all 200 languages found in FLORES-200 while achieving notably higher performance than LangId, LangDetect and CLD3. Furthermore, the gain in F1 score is accompanied by a notable improvement in FPR, suggesting a much stronger fit for extracting low-resource languages from web corpora 32 .
Mining for bitext
Previous work 35 notes that translation quality generally increases with the amount of high-quality training data, which is difficult to procure when working with low-resource languages. Existing parallel corpora for low-resource languages are often conveniently drawn from known multilingual collections, such as the Christian Bible or the publications of multinational organizations, which are limited in quantity and domain. To overcome this problem, we created training datasets through global bitext mining in publicly available web content (drawn from repositories such as CommonCrawl). The underlying idea of our bitext mining approach is first to learn a multilingual sentence embedding space and use a similarity measure in that space to decide whether two sentences are parallel. This comparison can be done for all possible pairs in two collections of monolingual texts.
As our mining approach requires a multilingual embedding space, there are several challenges when scaling this representation to all NLLB-200 languages. First, we had to ensure that all languages were well learnt and that we accounted for large imbalances in available training data. Second, training a massively multilingual sentence encoder from scratch each time a new set of languages is introduced is computationally expensive. Furthermore, the main drawback of this approach is that the learnt embedding spaces from each new model are not necessarily mutually compatible. This can make mining intractable as for each new encoder, the entirety of available monolingual data needs to be re-embedded (for example, for English alone, this means thousands of millions of sentences and considerable computational resources). We solved this problem using a teacher–student approach 21 that extends the LASER embedding space 36 to all NLLB-200 languages. Languages are trained either as individual students or together with languages from the same family. The training of students follows the approach described in ref. 21 .
Our approach enables us to focus on the specifics of each language while taking advantage of related languages, which is crucial for dealing with very low-resource languages. (A language is defined as very low-resource if it has fewer than 100,000 samples across all pairings with any other language in our dataset). Using this method, we generated more than 1,100 million new sentence pairs of training data for 148 languages. This additional training data, paired with back translation (a conventional technique for data augmentation in NMT; ref. 37 ), ushered notable improvements in translation quality—specifically, +12.5 chrF++ (ref. 38 ) for translating very low-resource languages into English. For more details, see Supplementary Information D .
Even with marked data volume increases, the main challenge of low-resource translation is for training models to adequately represent 200 languages while adjusting to variable data capacity per language pair. Apart from techniques such as data augmentation (for example, with back translation) and self-supervision strategies on monolingual data, we used conditional computational models—more specifically, Sparsely Gated Mixture of Experts (henceforth MoE)—to minimize interference between unrelated language directions.
MoE transformer models differ from dense transformer models in that some of the feed-forward network layers are replaced with MoE layers in both the encoder and the decoder. An MoE layer consists of E experts (each is a feed-forward network) and a gating network to decide how to route input tokens to experts. The transformer encoder–decoder model, supplemented with MoE layers and their respective gating networks, learns to route input tokens to the corresponding top two experts by optimizing a linearly weighted combination of label-smoothed cross entropy 39 and an auxiliary load balancing loss 6 .
We find that vanilla MoE models with overall dropout are suboptimal for low-resource languages and significantly overfit on low-resource pairs. To remedy this issue, we designed Expert Output Masking (EOM), a regularization strategy specific to MoE architectures, and compared it with existing regularization strategies, such as Gating Dropout 40 . We find that Gating Dropout performs better than vanilla MoE with overall dropout but is outperformed by EOM.
To further reduce overfitting on low-resource language pairs, we devised a curriculum learning that introduces language pairs in phases during model training. Pairs that empirically overfit within K updates are introduced with K updates before the end of training. This reduces overfitting while allowing pairs that benefit from additional training to continue their learning. Table 2 shows that combining curriculum learning and EOM improves performance, especially on low and very low-resource language pairs (see section ‘Modelling’ for more details).
To understand how MoE models are helpful for multilingual machine translation, we visualize similarities of experts in the MoE layers using heat maps (Fig. 1a–d ). These heat maps demonstrate that in late decoder layers (Fig. 1d ), languages are being separated (that is, dispatched to different sets of experts). Moreover, we observe that languages within the same family are highly similar in their choice of experts (that is, the late decoder MoE layers are language-specific). This is particularly the case for the Arabic dialects (the six rows and columns in the top-left corner), languages in the Benue–Congo subgrouping, as well as languages in the Devanagari script. By contrast, the early decoder MoE layers (Fig. 1c ) seem to be less language-specific. The late encoder MoE layers are particularly language-agnostic in how they route tokens as can be attested by the uniform heat map in Fig. 1b .
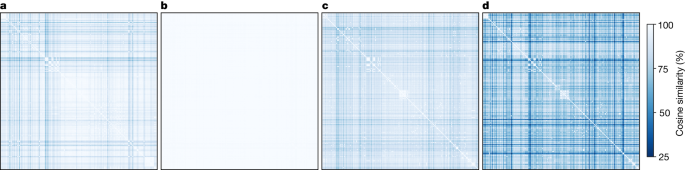
a – d , The first ( a ) and last ( b ) encoder layers and then the first ( c ) and last ( d ) decoder layers. The similarity is measured with respect to the gating decisions (expert choice) per language (source side in the encoder and target side in the decoder). Lighter colours represent higher experts similarity, hence, a language-agnostic processing.
Combining data (see section ‘ Automatically creating translation training data ’) and modelling contributions, Table 3 shows that NLLB-200 outperforms the nearest state-of-the-art system by almost +7.3 spBLEU (ref. 41 ) on average, constituting a 44% improvement. We then compared NLLB-200 with a few other state-of-the-art models, such as Deepnet 42 and M2M-100 (ref. 1 ), to report scores for 87 languages against FLORES-101. On this smaller subset, NLLB-200 again outperforms by +7.0 spBLEU on average. Overall, the results show that NLLB-200 improves on state-of-the-art systems by a notable margin despite supporting 200 languages, or twice as many languages (and more than 30,000 additional directions) compared with any previous work. We also show in additional experiments that NLLB-200 is a general-purpose NMT model, transferable to other domains by fine-tuning on small quantities of high-quality bitexts (see Supplementary Information E.3 ).
Evaluations
Among the many aspects of model performance that can be evaluated 43 , this section emphasizes three aspects that have a marked impact on the overall quality assessment: benchmarks for automatic evaluation, human evaluation protocols and toxicity evaluation.
A benchmark for automatic evaluation using FLORES-200
The quality of NMT outputs is typically evaluated by automatic metrics such as BLEU 44 or spBLEU 41 . The computation of automatic quality scores using these metrics requires benchmark datasets that provide gold-standard human translations as references. In turn, the apples-to-apples evaluation of different approaches made possible by these benchmark datasets gives us a better understanding of what requires further research and development. For example, creating benchmark data sets at the Workshop on Machine Translation (WMT) 45 led to rapid progress in translation directions such as English to German and English to French.
For massively multilingual NMT, the largest benchmark dataset available was FLORES-101, which supports roughly half the number of languages in NLLB-200. The necessary expansion of FLORES-101 to FLORES-200 constitutes a further challenge in terms of quality assurance, in part because of differences in standardization practices and limited access to professional translators for all languages involved. To overcome this challenge, we adapted our workflow to pay particular attention to quality assurance mechanisms. The FLORES-200 workflow consists of four phases: (1) alignment; (2) translation, initial quality assurance and iteration(s); (3) final quality assurance; and (4) completion. A language FLORES-200 set is considered ready after passing a final human quality test with a 90 out of 100 quality score (that is, independent raters agreed with 90% of the FLORES-200 reference translations in that direction).
As a result of this redesigned workflow, we produced a three-split (dev, devtest, test) data set of parallel human reference translations for all NLLB-200 languages meeting the 90% quality threshold in a maximum turnaround time of 287 days (119 days on average, 70 days minimum). (Note that to avoid leakage with our models, we filtered data from FLORES and other evaluation benchmarks used (such as WMT and IWSLT) from our training data. This was done by comparing the hashes of training sentences against those of evaluation sentences, using the xxHash algorithm). Please refer to Supplementary Information C for more details on the evaluation process. Figure 2 shows the quality scores for all languages, some of which are labelled as examples.
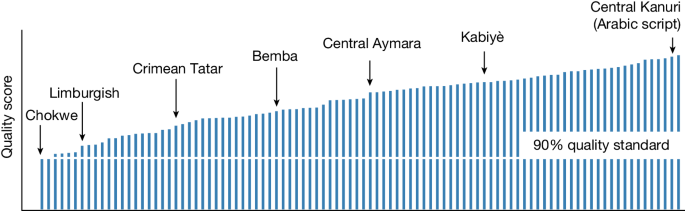
Quality assurance scores for the languages in FLORES-200. The minimum acceptable standard is 90%.
Reliable human evaluation
State-of-the-art automatic metrics often fail to capture aspects of language that, while subtle, can have a notable bearing on translation quality. Human evaluations are, therefore, essential to ensuring meaningful quality assessments 46 . That said, relying on them comes with two challenges: (1) any large-scale human evaluation of NMT quality, regardless of the number of translation directions involved, contends with potentially low inter-evaluator agreement (in the vicinity of 0.5 kappa); and (2) massively multilingual NMT introduces another complexity—that of quality evaluation consistency across language directions. We address these two issues by developing XSTS 47 , a new scoring metric focused on meaning, and by using a protocol that allows for the calibration of scores across evaluators and language pairs.
XSTS is a human evaluation protocol inspired by STS 48 , emphasizing meaning preservation over fluency. XSTS uses a five-point scale, in which 1 is the lowest score, and 3 represents the acceptability threshold. To ensure consistency not only across languages but also among different evaluators of any given language, we included the same subset of sentence pairs in the full set of sentence pairs given to each evaluator, making it possible to calibrate results.
We find that automated metrics such as spBLEU and chrF++ correlate reasonably well with calibrated human evaluations of translation quality, as shown in Fig. 3 . Spearman’s R correlation coefficients between aggregated XSTS and spBLEU, chrF++ (corpus) and chrF++ (average sentence-level) are 0.710, 0.687 and 0.694, respectively. Other correlation coefficients (Kendall’s τ and Pearson’s R ) have the same ordering. Corpus spBLEU provides the best nominal correlation, followed by average sentence-level chrF++.
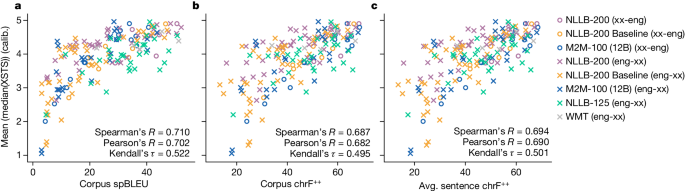
a , The relationship between spBLEU and XSTS. b , The relationship between chrF++ and XSTS. c , The relationship between average sentence-level chrF++ and XSTS. All automated scores were computed only on the sentences evaluated for a given model and translation direction (either the full FLORES-200 dataset or a subset). NLLB-200 refers to a 55B parameter MoE model, and NLLB-200 Baseline refers to a dense 3.3B parameter model.
We also find that calibrated human evaluation scores correlate more strongly with automated scores than uncalibrated human evaluation scores across all automated metrics and choices of correlation coefficient. In particular, uncalibrated human evaluation scores have a Spearman’s R correlation coefficient of 0.625, 0.607 and 0.611 for spBLEU, chrF++ (corpus) and chrF++ (average sentence-level), respectively.
Overall, a sample of 55 language directions were evaluated, including 8 into English, 27 out of English, and 20 other direct language directions. The overall mean of calibrated XSTS scores was 4.26, with 38/55 directions scoring over 4.0 (that is, high quality) and 52/56 directions scoring over 3.0.
We hypothesize that added toxicity may be because of the presence of toxicity in the training data and used our detectors to estimate, more specifically, unbalanced toxicity in the bitext data. We find that estimated levels of unbalanced toxicity vary from one corpus of bitext to the next and that unbalanced toxicity can be greatly attributed to misaligned bitext. In other words, training with this misaligned bitext could encourage mistranslations with added toxicity.
To mitigate this issue, we designed a bitext filtering procedure based on the detection of multiple instances of added toxicity (that is, cases in which one sentence in the bitext pair contains at least two more toxic items than the other sentence in the pair). (A previous detector quality analysis showed that a higher precision was reached in this situation). We added this toxicity filtering procedure as an option to the filtering process and experimented with or without it for comparison.
The experimental results on the FLORES-200 dev set for 10 translation directions (from and into English for Somali, Southern Sotho, Twi, Umbundu and Venetian) show that after filtering an average amount of around 30% parallel sentences, the translation quality (chrF++) improves by 5% and added toxicity (ETOX) reduces by the same amount. Therefore, the filtering pipeline that includes toxicity filtering not only reduces the number of toxic items in the translation output but also improves the overall translation performance.
In 2016, the United Nations declared internet access a basic human right. Although the intent of this declaration was to limit censorship and allow for information and ideas to flow without interference, much of the internet today remains inaccessible to many due to language barriers. Our effort was designed to contribute one solution to help alter this status quo.
For many low-resource language communities, NLLB-200 is one of the first models designed to support translation into or out of their languages. Although applications of these new translation capabilities could be found in several domains of everyday life, we believe their impact would be most significant in a domain such as education. In formal educational settings, for instance, students and educators belonging to low-resource language groups could, with the help of NLLB-200, tap into more books, research articles and archives than before. Within the realms of informal learning, low-resource language speakers could experience greater access to information from global news outlets and social media platforms, as well as online encyclopaedias such as Wikipedia. Access to machine translation motivates more low-resource language writers or content creators to share localized knowledge or various aspects of their culture. Giving individuals access to new translation tools could thus open up opportunities for bidirectional learning, thereby also challenging Western-centric modes of knowledge production and dissemination, ultimately aiding in revitalizing certain minority cultures and languages.
Since launching NLLB-200, we can already see the impact of the model across many directions. Four months after the launch of NLLB-200, Wikimedia reported that our model was the third most used machine translation engine used by Wikipedia editors (accounting for 3.8% of all published translations) ( https://web.archive.org/web/20221107181300/https://nbviewer.org/github/wikimedia-research/machine-translation-service-analysis-2022/blob/main/mt_service_comparison_Sept2022_update.ipynb ). Compared with other machine translation services and across all languages, articles translated with NLLB-200 has the lowest percentage of deletion (0.13%) and highest percentage of translation modification kept under 10%.
In many ways, the composition of the NLLB-200 effort speaks to the centrality of interdisciplinarity in shaping our vision. Machine translation and AI advancements lie at the intersection of technological, cultural and societal development, and thus require scholars with diverse training and standpoints to fully comprehend every angle 49 , 50 . It is our hope that in future iterations, NLLB-200 continues to include scholars from fields underrepresented in the world of machine translation and AI, particularly those from humanities and social sciences backgrounds. More importantly, we hope that teams developing these initiatives would come from a wide range of race, gender and cultural identities, much like the communities whose lives we seek to improve.
Finally, we want to emphasize that overcoming the challenges that prevent the web from being accessible to speakers of all languages requires a multifaceted approach. At the technical level, NLLB-200 overcomes many data, modelling and evaluation challenges in NMT research, but it still has its limitations, some of which are documented in Supplementary Information G . As a single technological intervention, NLLB-200 is all but one piece of a massive puzzle; policy interventions aimed at more fundamental issues surrounding education, internet access and digital literacy are imperative to eradicate the structural problem of language disparities.
This section describes the steps taken to design our language identification system and bitext mining protocol.
Language identification
To train language identification models, we used fasttext 33 , 51 , which has been widely used for text classification tasks because of its simplicity and speed. We embedded character-level n -grams from the input text and leveraged a multiclass linear classifier on top. The lightweight nature of fasttext enables our LID models to handle web-scale data. Furthermore, a linear model has the benefit of being easily explainable, allowing us to trace any classification error back to its root cause. This is instrumental in addressing common pitfalls that arise when detecting language on web corpora 32 .
Classifier design
We experimented with two different designs. First, we used a combination of multiple binary classifiers in which the final decision was obtained by selecting the language with the highest score after applying a threshold. We applied threshold optimization so that when the confidence of a classifier is low, the corresponding language is not considered for the final decision. A sentence was filtered out if none of the classifiers surpassed its threshold. Second, we built a multiclass classifier using softmax over all possible languages. In this case, the threshold optimization is done after the softmax.
Our results directed us to focus on the second approach, which offers several advantages. First, changing the threshold for one language did not affect the performance of the other (which is not true in the first setting). Second, this approach generalizes better to out-of-domain data, which is our primary use case (Wikipedia → web data). Finally, a single classifier has the added benefit of being computationally simpler, thus streamlining the language identification process.
Training data and handling massive class imbalance
We used publicly available datasets to train our LID system, partially covering our languages of interest. The public datasets deployed were mostly built from web pages such as CommonCrawl. We then supplemented these with NLLB-Seed data (Supplementary Information B ) for any missing languages. However, this supplementation is insufficient in ensuring balance in the raw training data 32 , 30 . For example, English alone represents 10.1% of our training data, whereas Minangkabau (Latin script) represents only 0.06%. Following ref. 10 , we experimented with multiple settings of temperature upsampling for underrepresented languages, in which sentences from a language l representing p l per cent of the data set are sampled proportionally to \({p}_{l}^{1/T}\) . Optimal performance was obtained at 1/ T = 0.3 (for more details, see section 5.1 of ref. 34 ).
Training parameters
Our best-performing model was trained with softmax loss over two epochs with a learning rate of 0.8 and embeddings with 256 dimensions. We discarded words with less than a thousand occurrences after upsampling and selecting a minimum and maximum character n -gram length of two and five, respectively (which were assigned a slot in buckets of size 1,000,000). (In fasttext, we refer to ‘word’ when it is separated by spaces. When it is a non-segmenting language, there is only one ‘word’ for the whole sentence (and we take character n -grams)). All hyperparameters were tuned on FLORES-200 dev (see section 5.1.2 of ref. 34 ).
Improving LID with linguistic analysis
Language identification is a challenging task in which numerous failure modes exist, often exacerbated by the gaps between the clean data on which LID models are trained and noisy data on which LID models are applied. In other words, LID models trained in a supervised manner on fluently written sentences may have difficulty identifying grammatically incorrect and incomplete strings extracted from the web. Furthermore, models can easily learn spurious correlations that are not meaningful for the task itself. Given these challenges, we collaborated closely with a team of linguists throughout different stages of LID development to identify proper focus areas, mitigate issues and explore solutions (see section 5.1.3 of ref. 34 ).
Bitext mining
The overall approach for bitext mining focused on starting with a massively multilingual sentence encoder teacher model and adapting it to several different low-resource student models. This approach enabled us to add low-resource languages without competing with high-resource languages for capacity. Doing so circumvents the need to retrain the entire model from scratch while maintaining compatibility with the multilingual embedding spaces for subsequent mining. Extended data Fig. 1 summarizes the overall architecture of the teacher–student approach. The teacher, LASER2, is an improved version of the open-source LASER encoder ( https://github.com/facebookresearch/LASER ). The original training procedure 36 was adapted to include SentencePiece tokenization (including a vocabulary of 7,000 tokens) and the upsampling of low-resource languages.
The architecture of the five-layer BiLSTM encoder and the max pooling method to obtain sentence embeddings were left unchanged. The training was then performed on the same 93 languages with public resources obtained from OPUS 52 . See ref. 36 for details on the original LASER training procedure. Training of the students followed the approach described in greater detail in ref. 21 , summarized below:
students specialized in one language or several similar languages;
students were randomly initialized because we wanted to handle low-resource language for which we did not have a pre-trained language model;
students may have a dedicated SentencePiece vocabulary different from the teacher to better accommodate scripts and tokens in the student languages;
as we used cosine distance for bitext mining (Fig. 1 ), students learnt to minimize the cosine loss with the teacher;
students can have an MLM loss to leverage student language monolingual data (Fig. 1 ).
Our student encoders used a 12-layer transformer with a hidden size of 1,024, four attention heads, and around 250 million parameters. All students were trained with available bitexts in their respective language, complemented by 2 million sentences of English/English and English/Spanish. The motivation behind this approach is to anchor the students to the English embedding space, increasing robustness by including English/Spanish bitexts from CCMatrix and allowing for the joint learning of new languages. This technique is particularly useful when only limited amounts of bitexts are available to train the students. Teacher–student training was performed on 16 GPUs, the ADAM optimizer, a learning rate of 0.0005 and a batch size of 10,000. We trained student encoders for 148 languages and named these models LASER3.
Proxy metric for new encoders
Mined bitexts were subsequently used to improve translation quality for the languages of NLLB-200. However, mining and NMT training are computationally expensive, and it is intractable to perform this evaluation systematically for many different sentence encoder variants. As an evaluation proxy, we used a mining-based multilingual similarity search error rate, referred to here as xsim. In contrast to cosine accuracy, which aligns embeddings based on the highest cosine score, xsim aligns source and target embeddings based on the highest margin score, which has been shown to be beneficial in mining 53 . The margin-based score is defined as
where x and y are the source and target sentences, and N N k ( x ) denotes the k nearest neighbours of x in the other language. We set k to 4. All xsim results are calculated on FLORES-200 devtest, using the ratio margin, where margin( a , b ) = a / b . Moreover, all scores are calculated for translations into English (that is, xxx → eng). English is encoded by the teacher, and the other language is encoded by the LASER3 student. To facilitate further research using xsim, we also provide this evaluation method as an open-source resource ( https://github.com/facebookresearch/LASER/ ).
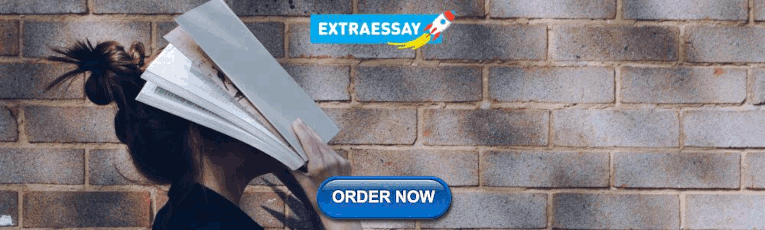
End-to-end encoder evaluation
Once we had identified the best sentence encoder for each language using the xsim scores, we performed mining, added the mined data to the existing bitexts and trained a bilingual NMT system. Initial experiments indicated that a threshold on the margin of 1.06 seems to be the best compromise between precision and recall for most languages. For these NMT baselines, we do not apply extra filtering on the bitexts and leave this to the training procedure of our massively multilingual NMT system.
We did not attempt to optimize the architecture and parameters of the bilingual NMT systems to the characteristics of each language pair but used the same architecture for all. Therefore, the reported results should not be interpreted as the best possible ones given the available resources—they are mainly provided to validate the mined bitexts. We used a 12-layer encoder and decoder and trained for 100 epochs. Moreover, we looked for the best performance on the FLORES-200 development set and report detokenized BLEU on the FLORES-200 devtest.
In this section, we first describe the multilingual machine translation task setup, which includes tokenization and base model architecture. Then, we outline how we leveraged conditional computation for massively multilingual machine translation with EOM regulation and our Curriculum Learning (CL) strategy for low-resource languages.
We modelled multilingual NMT as a sequence-to-sequence task, in which we conditioned on an input sequence in the source language with an encoder and generated the output sequence in the expected target language with a decoder 54 . With the source sentence S , source language ℓ s , and target language ℓ t in hand, we trained to maximize the probability of the translation in the target language T —that is, P ( T ∣ S , ℓ s , ℓ t ). Below, we discuss details of the (1) tokenization of the text sequences in the source and target languages; and (2) model architecture with the input and output designed specifically for multilingual machine translation. For further details on the task setup, such as the amount of training data per language pair, please refer to Supplementary Information F or section 8 of ref. 34 .
Segmentation with SentencePiece
To tokenize our text sequences, we trained a single SentencePiece model (SPM) 55 for all languages. We sampled a total of 100 million sentences from primary bitext data. To ensure low-resource languages are well-represented in the vocabulary, we downsampled high-resource and upsampled low-resource languages with a sampling temperature of five (ref. 10 ). Notably, vocabulary size is an important hyperparameter in multilingual translation models involving low-resource languages 56 , 57 , 58 . The vocabulary size of our trained SPM model is 256,000. Such a large vocabulary ensures adequate representation across the wide spectrum of languages we support.
Model architecture
Our sequence-to-sequence multilingual machine translation model is based on the transformer encoder–decoder architecture 59 . The encoder transforms the source token sequence into a sequence of token embeddings. Then, the decoder attends to the encoder output and autoregressively generates the target sentence token by token. More precisely, the encoder takes the sequence of tokens W = ( w 1 , …, w S ) and the source language ℓ s , and produces a sequence of embeddings H = ( h 1 , …, h S ), which are then provided to the decoder with the target language ℓ t to produce the target tokens V = ( v 1 , …, v T ) sequentially. In sum,
Note that we prefixed the source sequence with the source language, as opposed to the target language, as done in previous work 10 , 60 . We did so because we prioritized optimizing the zero-shot performance of our model on any pair of 200 languages at a minor cost to supervised performance. Empirically, we find zero-shot performance to be negatively affected when conditioning the encoder on the target language. When the source is conditioned on only the source language, the encoder generalizes better to pairs of source and target languages not encountered during training 1 .
Conditional computation for multilingual machine translation
A massively multilingual translation (MMT) model uses the same shared model capacity to train on several translation directions simultaneously. While doing so can lead to beneficial cross-lingual transfer between related languages, it can also add to the risk of interference between unrelated languages 1 , 61 . MoE models are a type of conditional computational models 62 , 63 that activate a subset of model parameters per input, as opposed to dense models that activate all model parameters per input. MoE models unlock marked representational capacity while maintaining the same inference and training efficiencies in terms of FLOPs compared with the core dense architecture.
However, as we increase the model capacity and the computational cost per update, the propensity for low or very low-resource languages to overfit increases, thus causing performance to deteriorate. In this section, we examine how we can use Sparsely Gated Mixture of Experts models 2 , 3 , 4 , 5 , 6 , 7 to achieve a more optimal trade-off between cross-lingual transfer and interference and improve performance for low-resource languages.
Sparsely gated mixture of experts
To build our MoE models, we substitute a quarter of the encoder and decoder feed-forward network layers with MoE layers, each with E distinct experts. We followed the Top- k -Gating algorithm in ref. 4 and dispatched each token to at most k = 2 experts. For more details on the training of MoE models, see Supplementary Information E .
Expert output masking
In this proposed regularization strategy, we masked the expert output for a random fraction ( p eom ) of the input tokens. For input tokens with dropped expert outputs, the first and/or second expert is effectively skipped. As shown in the second panel of Extended data Fig. 2 , we masked both experts for the first token ( x 1 in red), chose not to mask any of the expert outputs for the second token ( x 2 in blue) and in the final scenario, masked only one expert for the last token ( x 3 in green).
Curriculum learning for MMT
Orthogonal to model-side regularization methods such as dropout, we explored regularizing MMT models by means of CL. We proposed starting training with high-resource pairs first, then introducing low-resource pairs—prone to overfitting—in later phases. To derive the phases of the curriculum, we first trained a vanilla MoE model (without CL), followed by partitioning the translation directions into n bins { b 1 , …, b n }. If T is the total number of training updates, we introduced each bin b i after T − k i updates. We based when \({({k}_{i})}_{i}\) and what \({({b}_{i})}_{i}\) directions to add at every phase of the step when we observed a language pair starting to overfit. Review the step-based CL algorithm in ref. 64 for more on how the directions are partitioned. See Supplementary Information E.2 for the list of directions added at each stage.
Automatic evaluation
Many automatic translation quality assessment metrics exist, including model-based ones such as COMET 65 and BLEURT 66 . Although model-based metrics have shown better correlation with human judgement in recent metrics shared tasks of the WMT 43 , they require training and are not easily extendable to a large set of low-resource languages. In this work, we rely on BLEU (and a variant of it) and chrF++. Both measures draw on the idea that translation quality can be quantified based on how similar a machine translation output is compared with that produced by a human translator.
BLEU and spBLEU
The BLEU score 44 has been the standard metric for machine translation evaluation since its inception two decades ago. It measures the overlap between machine and human translations by combining the precision of 1-grams to 4-grams with a brevity penalty. The main disadvantage of BLEU is that it is tokenization-dependent. Efforts such as sacrebleu 67 have taken strides towards standardization, supporting the use of community-standard tokenizers under the hood. However, these tokenizers do not extend to many languages. Reference 41 proposes spBLEU, a BLEU metric based on a standardized SentencePiece model (SPM) covering 101 languages, released alongside FLORES-101. In this work, we provide SPM-200 along with FLORES-200 to enable the measurement of spBLEU. (Our analyses demonstrate that there are minor differences between SPM-200 from FLORES-200 and SPM-100 from FLORES-101 when measuring on the FLORES-101 languages. The major advantage of SPM-200 is that it covers 200 languages. More details on SPM-200 are reported in section 8.1.1 of ref. 34 ).
The chrF++ score 38 overcomes the limitation of the BLEU score, which requires that a sentence can be broken up into word tokens. However, some languages, such as Chinese or Thai, do not use spaces to separate words, and word segmentation tools may not be readily available. There is also a concern about highly agglutinative languages in which BLEU fails to assign any credit to morphological variants. chrF++ overcomes these weaknesses by basing the overlap calculation on character-level n -grams F -score ( n ranging from 1 to 6) and complementing with word unigrams and bi-grams. In this work, we primarily evaluated using chrF++ using the settings from sacrebleu. However, when comparing with other published work, we used BLEU and spBLEU where appropriate.
Human evaluation methodology
When building machine translation systems for thousands of different language pairs, a core question is which pairs reach certain levels of quality. Therefore, we needed meaningful scores that are comparable across language pairs.
XSTS evaluation protocol
We adapted the recently proposed XSTS methodology 48 . In short, XSTS is a human evaluation protocol focusing on meaning preservation above fluency. See details on this protocol in Supplementary Information F . For low-resource languages, translations are usually of poorer quality, and so we focused more on usable (that is, meaning-preserving) translations, even if they are not fully fluent. Compared with Direct Assessment 68 with a 5-point scale (the original direct assessment uses a 100-point scale), it is found that XSTS yields higher inter-annotator agreement 47 . XSTS rates each source sentence and its machine translation on a 5-point scale, in which 1 is the lowest and 5 is the highest.
Calibration set
To enable meaningful scores comparable across language pairs, we asked each evaluator to provide assessments using the XSTS scale on precisely the same set of sentence pairs. This aims to identify annotators who have a systematic tendency to be more harsh or generous in their scoring and correct for this effect. The calibration set consists of the machine translation output paired with the reference translation only in English. Based on how evaluators used the XSTS scale on this calibration set, we adjusted their raw scores on the actual evaluation task to ensure consistency across evaluators. Although this monolingual calibration task does not precisely mimic the bilingual XSTS task, it is a reasonable first approximation and has been shown to increase the correlation between human and automatic metrics primarily by reducing one source of ‘noise’ in the human evaluations—the lack of score calibration between annotators.
Obtaining aggregated human quality metrics from multiple studies
To obtain an aggregate human quality metric for each language direction in an evaluation study, we take the majority XSTS score (that is, mean–median score) for each sentence and average these majority scores over all evaluated sentences. In a given study, the aggregate human evaluation score for any translation direction l s → l t is
where l s and l t denote the source language and the target language, respectively; \({X}_{{l}_{{\rm{s}}}\to {l}_{{\rm{t}}},i}(S,T)\) denotes the XSTS score of the i th evaluator who evaluates sentences in a given translation direction l s → l t for a source sentence S and a target sentence T ; \({M}_{{l}_{{\rm{s}}}\to {l}_{{\rm{t}}}}\) denotes the total number of evaluators who evaluate the (source, translation) sentence pair ( S , T ) for translation direction l s → l t ; \({{\mathcal{T}}}_{{l}_{{\rm{s}}}\to {l}_{{\rm{t}}}}=\{({S}_{{l}_{{\rm{s}}}\to {l}_{{\rm{t}}},k},{T}_{{l}_{{\rm{s}}}\to {l}_{{\rm{t}}},k})| 1\le k\le {N}_{{l}_{{\rm{s}}}\to {l}_{{\rm{t}}}}\}\) is the set of \({N}_{{l}_{{\rm{s}}}\to {l}_{{\rm{t}}}}\) (source, translation) sentence pairs being evaluated for translation direction l s → l t .
Every evaluator in a given study s is also asked to provide ratings for all or parts of a calibration set— \({{\mathcal{C}}}_{s}=\{({S}_{s,k},{T}_{s,k})| 1\le k\le {K}_{s}\}\) . S s , k denotes the k th source sentence in the calibration set for an evaluation study; s , T s , k denotes the translated sentence corresponding to S s , k ; and \({K}_{s}=| {{\mathcal{C}}}_{s}| \) is the number of sentence pairs in the calibration set for an evaluation study.
For each language direction evaluated in a study, we obtained the majority score on the calibration set as follows:
where \({X}_{l,i}^{(s)}(S,T)\) denotes the XSTS score provided by the i th evaluator, for the language direction l s → l t , in study s , for a given source sentence S and a translated sentence T , in the calibration set \({{\mathcal{C}}}_{s}\) of the study.
To obtain aggregated calibrated XSTS scores on the language direction level, we explored several different calibration methodologies. None of the calibration methods we investigated showed a marked difference in correlation with automated scores, and all calibration methodologies we explored provided superior correlation compared with uncalibrated XSTS scores. For more details on these calibration methodologies, see section 7.2 of ref. 34 .
Added toxicity detection for 200 languages
To enable toxicity detection at scale, we used a detector based on word lists. In this section, we provide more details about our toxicity definition and describe the detector (ETOX) and associated word lists.
Toxic content
Owing to the subjective nature of toxicity, definitions of toxic language can vary. We included items that are commonly referred to as vulgar or profane language. (Note that vulgar or profane language is not always necessarily toxic. Some common slang, for instance, may be considered vulgar but is not necessarily toxic). Moreover, we also included items associated with depictions of pornographic content or sexual acts, some frequently used hate speech expressions and some expressions tied to bullying. We also included items, vulgar or not, referring to body parts that are commonly associated with sexual practices.
The ETOX detector
We started with the assumption that general-purpose machine translation systems should remain faithful to the source content and not add any toxic elements during the translation process. We define toxic elements as word tokens or short phrases present in our lists. ETOX identifies added toxicity using the following two criteria: number of toxic items and matched or non-matched toxicity. A toxic item is considered detected if it is present in a line and surrounded by spaces or the start or end of a line. ETOX tracks the number of unique toxic items found in a line but does not count a phrase again if it has multiple occurrences. Matched toxicity indicates that the number of toxic items is the same in both the source and the translated content (that is, no added toxicity). Added toxicity is an instance of non-matched toxicity in which more toxic items are found in the translation output than in the source. For non-segmenting languages or some languages that use complex diacritics, space tokenization is insufficient to distinguish words from one another. In those cases, we used SentencePiece tokenization of both the sentence and toxicity word list.
Toxicity-200 lists
Lists are based on professional translations from English, which were then heuristically adapted by linguists to better serve the target language. As toxicity is culturally sensitive, attempting to find equivalents in a largely multilingual setting constitutes a challenge when starting from one source language. To address this issue, translators were allowed to forgo translating some of the source items and add more culturally relevant items.
In the initial release of the Toxicity-200 lists, the average number of items in a toxicity detection list was 271 entries, whereas the median number of entries was 143. The latter may be a better measure of central tendency than the mean average, given that languages with a rich inflectional morphology constitute extreme outliers (for example, the Czech list had 2,534 entries and the Polish list 2,004). The shortest list had 36 entries, and the longest 6,078.
Data availability
All data generated and described in the Article and its Supplementary Information are available at GitHub ( https://github.com/facebookresearch/fairseq/tree/nllb ) 69 as follows. The FLORES-200 dataset contains human-translated evaluation data in 204 languages. The NLLB-Seed database contains human-translation seed training data in 39 languages (Supplementary Information I ). The NLLB-MD database contains human-translated seed data in different domains in six languages to assess generalization (Supplementary Information J ). The Toxicity-200 database contains wordlists to detect toxicity in 200 languages. Mined bitext database contains publicly available web data for 148 English-centric and 1,465 non-English-centric language pairs. Publicly available data used to train NLLB models with references to download the data are listed in Supplementary Table 2 .
Code availability
To make our work available to the community, we provide the following models and supporting code as resources freely available for non-commercial use, available at GitHub ( https://github.com/facebookresearch/fairseq/tree/nllb ) 69 as follows. The translation models cover 200 languages; the NLLB models come in multiple sizes (54.5B MoE, 3.3B and 1.3B Dense, and 1.3B and 600M distilled). The language identification models contain more than 200 languages. LASER3 comprises sentence encoders for identifying aligned bitext for 148 languages. Stopes consists of a data-mining library that can be used to process and clean monolingual data, followed by the creation of aligned bitext. Scripts to recreate our training data and training and generation scripts to reproduce our models are also included.
Fan, A. et al. Beyond English-centric multilingual machine translation. J. Mach. Learn. Res 22 , 1–48 (2021).
MathSciNet Google Scholar
Du, N. et al. GlaM: efficient scaling of language models with mixture-of-experts. In Proceedings of the 39th International Conference on Machine Learning Vol. 162, 5547–5569 (PMLR, 2022).
Hwang, C. et al. Tutel: adaptive mixture-of-experts at scale. In 6th Conference on Machine Learning and Systems (MLSys, 2023).
Lepikhin, D. et al. GShard: scaling giant models with conditional computation and automatic sharding. In International Conference on Learning Representations (ICLR, 2021).
Lewis, M., Bhosale, S., Dettmers, T., Goyal, N. & Zettlemoyer, L. BASE layers: simplifying training of large, sparse models. In Proc. 38th International Conference on Machine Learning Vol. 139, 6265–6274 (PMLR, 2021).
Shazeer, N. et al. Outrageously large neural networks: the sparsely-gated mixture-of-experts layer. In Proc. 2017 International Conference on Learning Representations (ICLR) 1–19 (ICLR, 2017).
Zoph, B. et al. ST-MoE: designing stable and transferable sparse expert models. Preprint at https://arxiv.org/abs/2202.08906 (2022).
Zoph, B., Yuret, D., May, J. & Knight, K. Transfer learning for low-resource neural machine translation. In Proc. 2016 Conference on Empirical Methods in Natural Language Processing (eds Su, J. et al.) 1568–1575 (Association for Computational Linguistics, 2016).
Nguyen, T. Q. & Chiang, D. Transfer learning across low-resource, related languages for neural machine translation. In Proc. Eighth International Joint Conference on Natural Language Processing Vol. 2 (eds Kondrak, G. & Watanabe, T.) 296–301 (Asian Federation of Natural Language Processing, 2017).
Arivazhagan, N. et al. Massively multilingual neural machine translation in the wild: findings and challenges. In Proceedings of the 2019 Conference of the North American Chapter of the Association for Computational Linguistics: Human Language Technologies Vol. 1, 3874–3884 (Association for Computational Linguistics, 2019).
Zhang, B., Williams, P., Titov, I. & Sennrich, R. Improving massively multilingual neural machine translation and zero-shot translation. In Proc. 58th Annual Meeting of the Association for Computational Linguistics (eds Jurafsky, D. et al.) 1628–1639 (ACL, 2020).
Tran, C. et al. Facebook AI’s WMT21 news translation task submission. In Proc. Sixth Conference on Machine Translation (eds Barrault, L.) 205–215 (ACL, 2021); https://aclanthology.org/2021.wmt-1.19 .
Orife, I. et al. Masakhane – machine translation for Africa. Preprint at https://arxiv.org/abs/2003.11529 (2020).
Kuwanto, G. et al. Low-resource machine translation training curriculum fit for low-resource languages. Preprint at https://arxiv.org/abs/2103.13272 (2021).
Nekoto, W. et al. Participatory research for low-resourced machine translation: a case study in African languages. In Findings of the Association for Computational Linguistics: EMNLP 2020 (eds Cohn, T. et al.) 2144–2160 (ACL, 2020).
Karakanta, A., Dehdari, J. & van Genabith, J. Neural machine translation for low-resource languages without parallel corpora. Mach. Transl. 32 , 167–189 (2018).
Article Google Scholar
Bañón, M. et al. ParaCrawl: web-scale acquisition of parallel corpora. In Proc. 58th Annual Meeting of the Association for Computational Linguistics (eds Jurafsky, D. et al.) 4555–4567 (ACL, 2020).
Schwenk, H. et al. CCMatrix: mining billions of high-quality parallel sentences on the web. In Proc. 59th Annual Meeting of the Association for Computational Linguistics and the 11th International Joint Conference on Natural Language Processing Vol. 1 (eds Zong, C. et al.) 6490–6500 (ACL, 2021).
Ramesh, G. et al. Samanantar : the largest publicly available parallel corpora collection for 11 Indic languages. Trans. Assoc. Comput. Linguist. 10 , 145–162 (2022).
Kreutzer, J. et al. Quality at a glance: an audit of web-crawled multilingual datasets. Trans. Assoc. Comput. Linguist. 10 , 50–72 (2022).
Heffernan, K., Çelebi, O. & Schwenk, H. Bitext mining using distilled sentence representations for low-resource languages. Preprint at https://arxiv.org/abs/2205.12654 (2022).
Gowda, T., Zhang, Z., Mattmann, C. & May, J. Many-to-English machine translation tools, data, and pretrained models. In Proc. 59th Annual Meeting of the Association for Computational Linguistics and the 11th International Joint Conference on Natural Language Processing: System Demonstrations (eds Ji, H. et al.) 306–316 (ACL, 2021).
McCarthy, A. D. et al. The Johns Hopkins University Bible corpus: 1600+ tongues for typological exploration. In Proc. 12th Language Resources and Evaluation Conference (eds Calzolari, N. et al.) 2884–2892 (European Language Resources Association, 2020); https://aclanthology.org/2020.lrec-1.352 .
McNamee, P. Language identification: a solved problem suitable for undergraduate instruction. J. Comput. Sci. Coll. 20 , 94–101 (2005).
Google Scholar
Abadji, J., Suárez, P. J. O., Romary, L. & Sagot, B. Towards a cleaner document-oriented multilingual crawled corpus. Preprint at https://arxiv.org/abs/2201.06642 (2022).
Widdows, D. & Brew, C. Language identification with a reciprocal rank classifier. Preprint at https://arxiv.org/abs/2109.09862 (2021).
Goutte, C., Léger, S., Malmasi, S. & Zampieri, M. Discriminating similar languages: evaluations and explorations. Preprint at http://arxiv.org/abs/1610.00031 (2016).
Jauhiainen, T., Lindén, K. & Jauhiainen, H. Evaluation of language identification methods using 285 languages. In Proc. 21st Nordic Conference on Computational Linguistics (eds. Tiedemann, J. & Tahmasebi, N.) 183–191 (2017).
Grave, É., Bojanowski, P., Gupta, P., Joulin, A. & Mikolov, T. Learning word vectors for 157 languages. In Proc. 11th International Conference on Language Resources and Evaluation (LREC 2018) (eds Calzolari, N. et al.) (ELRA, 2018).
Dunn, J. Mapping languages: the corpus of global language use. Lang. Resour. Eval. 54 , 999–1018 (2020).
Brown, R. D. Non-linear mapping for improved identification of 1300+ languages. In Proc. 2014 Conference on Empirical Methods in Natural Language Processing (EMNLP) (eds Moschitti, A. et al.) 627–632 (ACL, 2014).
Caswell, I., Breiner, T., van Esch, D. & Bapna, A. Language ID in the wild: unexpected challenges on the path to a thousand-language web text corpus. In Proc. 28th International Conference on Computational Linguistics (eds Scott, D. et al.) 6588–6608 (International Committee on Computational Linguistics, 2020); https://aclanthology.org/2020.coling-main.579 .
Joulin, A., Grave, E., Bojanowski, P. & Mikolov, T. Bag of tricks for efficient text classification. In Proc. 15th Conference of the European Chapter of the Association for Computational Linguistics Vol. 2 (eds Lapata, M. et al.) 427–431 (ACL, 2017).
NLLB Team et al. No language left behind: scaling human-centered machine translation. Preprint at https://arxiv.org/abs/2207.04672 (2022).
Koehn, P. & Knowles, R. Six challenges for neural machine translation. In Proc. First Workshop on Neural Machine Translation (eds Luong, T. et al.) 28–39 (ACL, 2017).
Artetxe, M. & Schwenk, H. Massively multilingual sentence embeddings for zero-shot cross-lingual transfer and beyond. Trans. Assoc. Comput. Linguist. 7 , 597–610 (2019).
Sennrich, R., Haddow, B. & Birch, A. Improving neural machine translation models with monolingual data. In Proc. 54th Annual Meeting of the Association for Computational Linguistics (ACL) Vol. 1 (eds Erk, K. & Smith, N. A.) 86–96 (ACL, 2016).
Popović, M. chrf++: words helping character n-grams. In Proc. Second Conference on Machine Translation Vol. 2 (eds Bojar, O. et al.) 612–618 (ACL, 2017).
Szegedy, C., Vanhoucke, V., Ioffe, S., Shlens, J. & Wojna, Z. Rethinking the inception architecture for computer vision. In IEEE Conference on Computer Vision and Pattern Recognition (CVPR) 2818–2826 (IEEE, 2016).
Liu, R., Kim, Y. J., Muzio, A., Mozafari, B. & Awadalla, H. H. Gating dropout: communication-efficient regularization for sparsely activated transformers. In Proceedings of the 39th International Conference on Machine Learning (PMLR, 2022).
Goyal, N. et al. The Flores-101 evaluation benchmark for low-resource and multilingual machine translation. Trans. Assoc. Comput. Linguist. 10 , 522–538 (2022).
Wang, H. et al. DeepNet: scaling transformers to 1,000 layers. In IEEE Transactions on Pattern Analysis and Machine Intelligence https://doi.org/10.1109/TPAMI.2024.3386927 (IEEE, 2024)
Freitag, M. et al. Results of the WMT21 metrics shared task: evaluating metrics with expert-based human evaluations on TED and news domain. In Proc. Sixth Conference on Machine Translation (eds Barrault, L. et al.) 733–774 (ACL, 2021); https://aclanthology.org/2021.wmt-1.73 .
Papineni, K., Roukos, S., Ward, T. & Zhu, W.-J. Bleu: a method for automatic evaluation of machine translation. In Proc. 40th annual meeting of the Association for Computational Linguistics (eds Isabelle, P. et al.) 311–318 (ACL, 2002).
Akhbardeh, F. et al. Findings of the 2021 conference on machine translation (WMT21). In Proc. Sixth Conference on Machine Translation (eds Barrault, L. et al.) 1–88 (ACL, 2021); https://aclanthology.org/2021.wmt-1.1 .
Kocmi, T. et al. To ship or not to ship: an extensive evaluation of automatic metrics for machine translation. In Proc. Sixth Conference on Machine Translation (eds Barrault, L. et al.) 478–494 (ACL, 2021).
Licht, D. et al. Consistent human evaluation of machine translation across language pairs. In Proceedings of the 15th Biennial Conference of the Association for Machine Translation in the Americas Vol. 1, 309–321 (Association for Machine Translation in the Americas, 2022).
Agirre, E. et al. SemEval-2012 task 6: a pilot on semantic textual similarity. In Proc. *SEM 2012: The First Joint Conference on Lexical and Computational Semantics Vols 1–2 (eds Aggire, E. et al.) 385–393 (ACL, 2012).
Kusters, R. et al. Interdisciplinary research in artificial intelligence: Challenges and opportunities. Front. Big Data 3 , 577974 (2020).
Article PubMed PubMed Central Google Scholar
Wang, S., Cooper, N., Eby, M. & Jo, E. S. From human-centered to social-centered artificial intelligence: assessing ChatGPT’s impact through disruptive events. Preprint at https://arxiv.org/abs/2306.00227 (2023).
Bojanowski, P., Grave, E., Joulin, A. & Mikolov, T. Enriching word vectors with subword onformation. Trans. Assoc. Comput. Linguist. 5 , 135–146 (2017).
Tiedemann, J. Parallel data, tools and interfaces in OPUS. In Proc. Eighth International Conference on Language Resources and Evaluation (eds Calzolari, N. et al.) 2214–2218 (ACL, 2012).
Artetxe, M. & Schwenk, H. Margin-based parallel corpus mining with multilingual sentence embeddings. In Proc. 57th Annual Meeting of the Association for Computational Linguistics (eds Korhonen, A.) 3197–3203 (ACL, 2019).
Bahdanau, D., Cho, K. H. & Bengio, Y. Neural machine translation by jointly learning to align and translate. In Proc. of the 3rd International Conference on Learning Representations (ICLR, 2015).
Kudo, T. & Richardson, J. SentencePiece: A simple and language independent subword tokenizer and detokenizer for Neural Text Processing. In Proc. 2018 Conference on Empirical Methods in Natural Language Processing, EMNLP 2018 (eds Blanco, E. & Lu, W.) 66–71 (ACL, 2018); https://doi.org/10.18653/v1/d18-2012 .
Gu, J., Hassan, H., Devlin, J. & Li, V. O. Universal Neural Machine Translation for Extremely Low Resource Languages. In Proc. 2018 Conference of the North American Chapter of the Association for Computational Linguistics: Human Language Technologies Vol. 1 (eds Walker, M. et al.) 344–354 (ACL, 2018); https://aclanthology.org/N18-1032 .
Wang, X., Pham, H., Arthur, P. & Neubig, G. Multilingual neural machine translation with soft decoupled encoding. Preprint at https://arxiv.org/abs/1902.03499 (2019).
Rajab, J. Effect of tokenisation strategies for low-resourced Southern African languages. In 3rd Workshop on African Natural Language Processing (ICLR, 2022).
Vaswani, A. et al. Attention is all you need. In Proc. 31st Conference on Neural Information Processing Systems 5998–6008 (NIPS, 2017).
Johnson, M. et al. Google’s multilingual neural machine translation system: enabling zero-shot translation. Trans. Assoc. Comput. Linguist. 5 , 339–351 (2017).
Conneau, A. et al. Unsupervised cross-lingual representation learning at scale. In Proc. 58th Annual Meeting of the Association for Computational Linguistics (eds Jurafsky, D. et al.) 8440–8451 (ACL, 2020).
Bengio, Y., Léonard, N. & Courville, A. C. Estimating or propagating gradients through stochastic neurons for conditional computation. Preprint at http://arxiv.org/abs/1308.3432 (2013).
Almahairi, A. et al. Dynamic capacity networks. In Proc. 33rd International Conference on International Conference on Machine Learning Vol. 48, 2091–2100 (PMLR, 2016).
Elbayad, M., Sun, A. & Bhosale, S. Fixing MoE over-fitting on low-resource languages in multilingual machine translation. In Findings of the Association for Computational Linguistics: ACL 2023 (eds Rogers, A. et al.) 14237–14253 (ACL, 2023); https://aclanthology.org/2023.findings-acl.897 .
Rei, R., Stewart, C., Farinha, A. C. & Lavie, A. COMET: a neural framework for MT evaluation. In Proc. 2020 Conference on Empirical Methods in Natural Language Processing (EMNLP) (eds Webber, B. et al.) 2685–2702 (ACL, 2020).
Sellam, T., Das, D. & Parikh, A. BLEURT: learning robust metrics for text generation. In Proc. 58th Annual Meeting of the Association for Computational Linguistics (eds Jurafsky, D. et al.) 7881–7892 (ACL, 2020).
Post, M. A Call for Clarity in Reporting BLEU Scores. In Proc. Third Conference on Machine Translation: Research Papers (eds Bojar, O. et al.) 186–191 (ACL, 2018); https://aclanthology.org/W18-6319 .
Graham, Y., Baldwin, T., Moffat, A. & Zobel, J. Continuous measurement scales in human evaluation of machine translation. In Proc. 7th Linguistic Annotation Workshop and Interoperability with Discourse 33–41 (eds Graham, Y. et al.) (ACL, 2013).
NLLB Team et al. No Language Left Behind: scaling human-centered machine translation. GitHub https://github.com/facebookresearch/fairseq/tree/nllb (2022).
Download references
Acknowledgements
We thank the following interns for their contributions to the project: C. Baziotis, D. Dua, A. Guo, O. Ignat, A. Kamran, T. Mohiuddin, A. N. Rubungo, S. Sun, S. Tan, H. Xu, S. Wu and Y. Zhang. We are grateful to all the Wikimedia Foundation staff and volunteers who worked with us and provided helpful feedback on our project. We thank V. Chaudhary for help with the data pipeline; E. Grave for his help in scaling fasttext to all FLORES-200 languages; M. Diab for her work on XSTS; L. Specia for her feedback on toxicity and XSTS; J. Ferrando and C. Escolano for their help in using the ALTI+ method; G. Chang, C.-J. Wu and R. Raghavendra for helping us to compute the CO 2 cost of training our models; A. Sridhar for helping with FSDP; S. Jeschonek, G. Anantharaman, D. Sarina, J. Colombo, S. Krishnan, D. Kannappan, K. Saladi, V. Pai, A. Yajurvedi and S. Sengupta for their assistance with training infrastructure; K. Johnson for his help with UXR studies and model evaluation; B. O’Horo and J. Kao for their generative insights and guidance; P. Fung, N. Usunier, S. Riedel, S. Sengupta and E. Dinan for their helpful feedback on the paper. We would also like to thank A. Bordes, M. Zannoli and C. Moghbel for their overall support of this project. Finally, we are indebted to the translators, reviewers, human evaluators, linguists, as well as the translation and quality assurance agencies we partnered with, for helping to create FLORES-200, NLLB-Seed, NLLB-MD and Toxicity-200; performing human evaluations; and teaching us about their native languages.
Author information
Authors and affiliations.
Foundational AI Research (FAIR), Meta, Paris, France
Marta R. Costa-jussà, Onur Çelebi, Guillaume Wenzek, Loic Barrault, Shannon Spruit, Pierre Andrews, Alexandre Mourachko & Holger Schwenk
Foundational AI Research (FAIR), Meta, New York, NY, USA
James Cross, Angela Fan, Philipp Koehn & Safiyyah Saleem
Foundational AI Research (FAIR), Meta, Menlo Park, CA, USA
Maha Elbayad, Elahe Kalbassi, Janice Lam, Daniel Licht, Jean Maillard, Anna Sun, Skyler Wang, Al Youngblood, Bapi Akula, Gabriel Mejia Gonzalez, Prangthip Hansanti, John Hoffman, Semarley Jarrett, Kaushik Ram Sadagopan, Dirk Rowe, Chau Tran, Necip Fazil Ayan, Shruti Bhosale, Sergey Edunov, Cynthia Gao, Vedanuj Goswami, Francisco Guzmán, Christophe Ropers & Jeff Wang
Foundational AI Research (FAIR), Meta, London, UK
Kenneth Heafield & Kevin Heffernan
University of California, Berkeley, CA, USA
Skyler Wang
Johns Hopkins University, Baltimore, MD, USA
Philipp Koehn
- Marta R. Costa-jussà
- , James Cross
- , Onur Çelebi
- , Maha Elbayad
- , Kenneth Heafield
- , Kevin Heffernan
- , Elahe Kalbassi
- , Janice Lam
- , Daniel Licht
- , Jean Maillard
- , Skyler Wang
- , Guillaume Wenzek
- , Al Youngblood
- , Bapi Akula
- , Loic Barrault
- , Gabriel Mejia Gonzalez
- , Prangthip Hansanti
- , John Hoffman
- , Semarley Jarrett
- , Kaushik Ram Sadagopan
- , Dirk Rowe
- , Shannon Spruit
- , Chau Tran
- , Pierre Andrews
- , Necip Fazil Ayan
- , Shruti Bhosale
- , Sergey Edunov
- , Angela Fan
- , Cynthia Gao
- , Vedanuj Goswami
- , Francisco Guzmán
- , Philipp Koehn
- , Alexandre Mourachko
- , Christophe Ropers
- , Safiyyah Saleem
- , Holger Schwenk
- & Jeff Wang
Contributions
B.A., P.A., O.Ç., K. Heafield, K. Heffernan, S.J., H.S. and G.W. contributed to the data workstream of the project, which includes developing tools to facilitate data mining, cleaning and consolidation. L.B., S.B., J.C., M.E., V.G., J.M., K.R.S., A.S. and C.T. conducted research and experiments that gave rise to the models in this work. M.R.C., C.G., J.H., E.K., P.K., D.L., D.R., S.Spruit., S.W. and A.Y. implemented automatic and human evaluations of NLLB, including but not limited to quality, bias and toxicity. G.M.G., P.H., J.L. and C.R. performed all linguistics work in this project. N.F.A., S.E., A.F., F.G., A.M., S.S. and J.W. provided crucial technical and organizational leadership to help materialize this overall project. M.R.C., C.R., M.E. and S.W. prepared the paper for publication.
Corresponding author
Correspondence to Marta R. Costa-jussà .
Ethics declarations
Competing interests.
The authors declare no competing interests.
Peer review
Peer review information.
Nature thanks David Adelani, Sunipa Dev and the other, anonymous, reviewer(s) for their contribution to the peer review of this work. Peer reviewer reports are available.
Additional information
Publisher’s note Springer Nature remains neutral with regard to jurisdictional claims in published maps and institutional affiliations.
Extended data figures and tables
Extended data fig. 1 architecture of the laser3 teacher-student approach..
See 21 for more details.
Extended Data Fig. 2 Illustration of EOM (panel c) in contrast to overall dropout (panel b) for MoE layers.
A color represents a token, and each token is dispatched to two experts (Top-2-Gating) depending on the gating decision (panel a). Faded colors correspond to dropped units or masked outputs.
Supplementary information
Supplementary information.
This file contains Supplementary Information Sections A–K and Supplementary References – see Supplementary Contents page for details.
Peer Review File
Rights and permissions.
Open Access This article is licensed under a Creative Commons Attribution 4.0 International License, which permits use, sharing, adaptation, distribution and reproduction in any medium or format, as long as you give appropriate credit to the original author(s) and the source, provide a link to the Creative Commons licence, and indicate if changes were made. The images or other third party material in this article are included in the article’s Creative Commons licence, unless indicated otherwise in a credit line to the material. If material is not included in the article’s Creative Commons licence and your intended use is not permitted by statutory regulation or exceeds the permitted use, you will need to obtain permission directly from the copyright holder. To view a copy of this licence, visit http://creativecommons.org/licenses/by/4.0/ .
Reprints and permissions
About this article
Cite this article.
NLLB Team. Scaling neural machine translation to 200 languages. Nature (2024). https://doi.org/10.1038/s41586-024-07335-x
Download citation
Received : 08 May 2023
Accepted : 19 March 2024
Published : 05 June 2024
DOI : https://doi.org/10.1038/s41586-024-07335-x
Share this article
Anyone you share the following link with will be able to read this content:
Sorry, a shareable link is not currently available for this article.
Provided by the Springer Nature SharedIt content-sharing initiative
This article is cited by
Meta’s ai system is a boost to endangered languages — as long as humans aren’t forgotten.
Nature (2024)
By submitting a comment you agree to abide by our Terms and Community Guidelines . If you find something abusive or that does not comply with our terms or guidelines please flag it as inappropriate.
Quick links
- Explore articles by subject
- Guide to authors
- Editorial policies
Sign up for the Nature Briefing: AI and Robotics newsletter — what matters in AI and robotics research, free to your inbox weekly.


An official website of the United States government
The .gov means it’s official. Federal government websites often end in .gov or .mil. Before sharing sensitive information, make sure you’re on a federal government site.
The site is secure. The https:// ensures that you are connecting to the official website and that any information you provide is encrypted and transmitted securely.
- Publications
- Account settings
Preview improvements coming to the PMC website in October 2024. Learn More or Try it out now .
- Advanced Search
- Journal List
- v.24(3); 2021 Mar 19
Opportunities and challenges of text mining in aterials research
Olga kononova.
1 Department of Materials Science & Engineering, University of California, Berkeley, CA 94720, USA
2 Materials Sciences Division, Lawrence Berkeley National Laboratory, Berkeley, CA 94720, USA
Amalie Trewartha
Elsa a. olivetti.
3 Department of Materials Science & Engineering, MIT, Cambridge, MA 02139, USA
Gerbrand Ceder
Research publications are the major repository of scientific knowledge. However, their unstructured and highly heterogenous format creates a significant obstacle to large-scale analysis of the information contained within. Recent progress in natural language processing (NLP) has provided a variety of tools for high-quality information extraction from unstructured text. These tools are primarily trained on non-technical text and struggle to produce accurate results when applied to scientific text, involving specific technical terminology. During the last years, significant efforts in information retrieval have been made for biomedical and biochemical publications. For materials science, text mining (TM) methodology is still at the dawn of its development. In this review, we survey the recent progress in creating and applying TM and NLP approaches to materials science field. This review is directed at the broad class of researchers aiming to learn the fundamentals of TM as applied to the materials science publications.
Graphical Abstract

Data Analysis; Computing Methodology; Computational Materials Science; Materials Design
Introduction and background
The first example of statistical analysis of publications dates back to 1887 when Thomas C. Mendenhall suggested a quantitative metric to characterize authors' writing styles ( Mendenhall, 1887 ). At that time, the analysis of the literature was widely used to resolve authorship disputes, and, of course, was entirely manual. In the 1940-1960s, the development of computers gave a significant boost to the growth of linguistic analysis. The work of Stephen C. Kleene on regular expressions and finite automata ( Kleene, 1956 ), subsequent formal language theory described by Noam Chomsky (1956) , and the important fundamental work on information theory by Claude Shannon (1951) became the foundation for what is now known as natural language processing (NLP). The following decades brought diverse research results along different aspects of text mining (TM) and NLP: automated generation of article abstracts ( Luhn, 1958 ), regular expressions compilers ( Thompson, 1968 ), automated dialog assistant ( Weizenbaum, 1983 ), the first structured text collection – the Brown University Standard Corpus of American English ( www.korpus.uib.no/icame/manuals ), and many others ( Miner et al., 2012 ).
In the 1990s, technological progress permitted storage and access to large amounts of data. This shifted NLP and machine learning (ML) from a knowledge-based methodology toward data-driven approaches ( Kurgan and Musilek, 2006 ). The accelerated development of the Internet and the Web during this decade facilitated information sharing and exchange. This is also reflected in the rapid growth of scientific publications ( Bornmann and Mutz, 2015 ) over this period. Our analysis of the papers indexed in the Web of Science repository shows that since the beginning of 2000s, the number of publications in different fields of materials science has increased exponentially ( Figure 1 ).

Publication trend over the past 14 years
Top panel: Number of publications appearing every year in different fields of materials science. All data were obtained by manually querying Web of Science publications resource. The analysis includes only research articles, communications, letters, and conference proceedings. The number of publications is on the order of 10 3 . Bottom panel: Relative comparison of the fraction of scientific papers available on-line as image PDF or embedded PDF versus articles in HTML/XML format. The gray arrow marks time intervals for both top and bottom panels.
There are significant opportunities in leveraging data to guide materials research, which is driven by such aspects as property prediction, the search for novel materials, identifying synthesis routes, or determining device parameters. Data are central to the materials informatics enterprise as the availability of large quantities of machine-readable data is a prerequisite to leverage statistical approaches to accelerate materials research ( Ramprasad et al., 2017 ). Not surprisingly, early work on data-driven learning approaches therefore focused on the few highly curated datasets in the materials field, such as crystal structure data ( Fischer et al., 2006 ; Hautier et al., 2011 ) or on computed property data which can be generated homogeneously and at high rate ( Jain et al., 2013 ; de Jong et al., 2015 ; Ricci et al., 2017 ).
However, knowledge acquisition in materials science must generally be performed across insufficient, diverse, and heterogeneous data. These data range across disparate materials systems and a multitude of characterization approaches to comprehend thermomechanical, electromagnetic and chemical properties ( Morgan and Jacobs, 2020 ). Publications are still the primary way to communicate within the scientific discipline. Therefore, there is substantial potential in capturing unstructured information from the vast and ever-growing number of scientific literature.
Textual information exists in an unstructured or highly heterogeneous format. Manual data extraction is expensive, labor-intensive, and error-prone (although some powerful examples exist in the materials community ( Blokhin and Villars, 2020 ; Gallego et al., 2016b ; Gallego et al., 2016a )). As a result, there are tremendous opportunities for large-scale automated data extraction to transform materials science into a more quantitative and data-rich field.
This review discusses recent advances in automated text processing and information extraction from a large corpus of chemical, physical and materials science publications. We first discuss the methods and approaches widely used in TM and NLP ( Section 2 ). Then we survey some prominent case studies that are focused on data collection and data mining ( Section 3 ). We highlight some major challenges and obstacles in scientific TM ( Section 4 ). Lastly, we discuss potential future research developments for NLP in its application to materials science ( Section 5 ).
Text mining of scientific literature
Modern computers encode text as a monotonic sequence of bits representing each character but without reflecting its internal structure or other high-order organization (e.g. words, sentences, paragraphs). Building algorithms to interpret the sequences of characters and to derive logical information from them is the primary purpose of TM and NLP. Unlike standard texts on general topics, such as newswire or popular press, scientific documents are written in specific language requiring sufficient domain knowledge to follow the ideas. Application of general-purpose TM and NLP approaches to the chemical or materials science domain requires adaptation of both methods and models, including development of an adequate training sets that comply with the goals of the TM project.
Generally, a scientific TM pipeline breaks down into the following steps ( Figure 2 ): (i) retrieval of documents and conversion from markup languages or PDF into plain text; (ii) text pre-processing, i.e. segmentation into sentences and tokens, text normalization, and morphological parsing; (iii) text analysis and information extraction; (iv) data normalization and database structuring. The resulting collection either serves as a final product of the TM or provides a source of data for further mining and analysis.

Schematic representation of the standard text mining pipeline for information extraction from the scientific publications
While a comprehensive discussion of the algorithms and methods used to accomplish each task of the pipeline is beyond the scope of this review, we cover in this Section those methods that are widely applied in scientific TM. We also revise state-of-the-art NLP parsing tools needed to handle chemical and materials science texts. We emphasize the challenges arising along the way and discuss possible solutions. For details and theoretical background on TM and NLP models in general, we refer the reader to the following books ( Miner et al., 2012 ): and ( Jurafsky and Martin, 2009 ).
Obtaining the text corpus
In computational linguistics, a large organized set of human-created documents is referred to as a text corpus . Scientific discourse generally occurs across a wide variety of document formats and types: abstracts in proceedings, research articles, technical reports, and pre-prints, patents, e-encyclopedias, and many more. There are two primary ways to obtain the text corpus: (i) by using existing indexed repositories with the available text-mining application programming interfaces (APIs) and search tools; or (ii) by having access to an individual publisher's content.
Text databases
A comprehensive overview of scientific text resources can be found in review of Kolářik et al. (2008) . Table 1 lists some common repositories for scientific texts in the domain of chemistry and material science, their document types, and access options. The main advantage of using established databases for TM is the uniform format of their metadata, a convenient API, and sometimes analysis tools. However, the majority of the publications in these repositories are heavily biased toward biomedical and biochemical subjects with a smaller fraction belonging to physics, (in)organic chemistry, and materials science. Moreover, the access to the content is limited: it either requires having a subscription or provides a search over open-access publications only.
List of some common text repositories in chemistry and material science subjects that provide an API for querying
Data repository | Documents types | Access | Reference |
---|---|---|---|
CAplus | Research articles, patents, reports | Subscription | |
DOAJ | Research articles (open-access only) | Public | |
PubMed Central | Research articles | Public | |
Science Direct (Elsevier) | Research articles | Subscription | |
Scopus (Elsevier) | Abstracts | Public | |
Springer Nature | Research articles, books chapters | Subscription |
Note 1 : Elsevier provides API for both Science Direct (collection of Elsevier published full-text) and Scopus (collection of abstracts from various publishers). Note 2 : Springer Nature provides access only to its own published full texts.
Individual publisher access
Implementation of a customized scraping routine to screen the publisher's web-pages and download the content requires more effort. However, this approach allows for accessing content from those resources that are not providing an API, for example, e-print repositories. In most cases, downloading and accessing significant publisher content require text and data mining (TDM) agreements. We note that this TDM agreement differs from a standard academic subscription granted to the libraries of the institutions because scraping and downloading large volumes, affect the operation of the publishers' server.
Web-scraping not only requires a substantial amount of work, but it also has to respond to dynamic web pages in which content is generated by a client browser. In our recent work, we implemented such a solution for Elsevier, RSC, ECS, and AIP publishers ( Kononova et al., 2019 ). Similarly, ChemDataExtractor ( Swain and Cole, 2016 ) provides the web-scrapers for Elsevier, RSC, and Springer. In the research fields where most of the literature has an open access repository, e.g. physics, mathematics or the rapidly growing literature collection on COVID-19 ( Trewartha et al., 2020 ), the corpus acquisition step will be considerably easier.
Conversion into raw text
In general, the retrieved content includes the targeted text and other metadata, such as journal name, title, authors, keywords, and others. Querying text databases, as those in Table 1 , provide a structured output with raw text ready for processing and analysis. In contrast, web-scraped content usually consists of a complete paper files requiring the additional step to convert it into a raw text. Nowadays, most of the text sources provide as HTML/XML/JSON documents, whereas older papers are usually available as embedded or image PDFs ( Figure 1 ).
While parsing of HTML/XML markups can be performed with various programming tools, extraction of the plain text from PDF files is more laborious. Embedded PDFs usually have a block structure with the text arranged in columns and intermixed with tables, figures, and equations. This affects the accuracy of conversion and text sequence. Some work has been done attempting to recover a logical text structure from PDF-formatted scientific articles by utilizing rule-based ( Constantin et al., 2013 ) and ML ( Tkaczyk et al., 2015 ; Luong et al., 2010 ) approaches. However, the accuracy of these models measured as F1-score is still below ∼80%. The authors' experience demonstrates that this can dramatically impact the final output of the extraction pipeline ( Figure 2 ). Hence, the decision on whether to include PDF text strongly depends on the tasks that are being solved.
A great number of documents, in particular, those published before the 1990s, are only available as an image PDF ( Figure 1 ). Conversion of these files into a raw text requires advanced optical character recognition (OCR), and, to the best of our knowledge, the currently available solutions still fail to provide high enough accuracy to reliably extract chemistry ( Mouchère et al., 2016 ; Mahdavi et al., 2019 ). Often, interpretation errors in PDFs originate from subscripts in chemical formulas and equations, and from confusion between symbols and digits. Creating a rigorous parser for PDF articles, and especially an OCR for scientific text is an area of active research in the computer science and TM community ( Memon et al., 2020 ; Ramakrishnan et al., 2012 ).
Text pre-processing, grammatical, and morphological parsing
The raw documents proceed through normalization, segmentation, and grammar parsing. During this step, the text is split into logical constitutes (e.g. sentences) and tokens (e.g. words and phrases), that are used to build a grammatical structure of the text. Depending on the final text and data mining goal, the text tokens may be normalized by stemming or lemmatization and processed through the part of speech tagging (POS tagging), and dependencies parsing to build the sentences structure. These are explained below.
Paragraph segmentation and sentence tokenization identify, respectively, the boundaries of the sentences and word phrases (tokens) in a text. In general, finding the start/end of a sentence segment requires recognition of certain symbolic markers, such as period (“.”), question mark (“?”), and exclamation mark (“!”), which is usually performed with (un)supervised ML models ( Read et al., 2012 ). State-of-the-art implementations attain ∼95-98% accuracy (measured as F1-score). However, applying these models to scientific text requires modification. Commonly used expressions such as “Fig. X”, “et al.” and a period in chemical formulas often result in over-segmentation of a paragraph. Conversely, citation numbers at the end of a sentence promote the merging of two sentences together. There is no generally accepted solution to this problem, and it is usually approached by hard-coding a set of rules that capture particular cases ( Leaman et al., 2015 ).
Sentence tokenization, i.e. splitting a sentence into logical constituents, is a crucial step on the way to information extraction, because the errors produced in this step tend to propagate down the pipeline ( Figure 2 ) and affect the accuracy of the final results. Tokenization requires both unambiguous definition of grammatical tokens and robust algorithms for identification of the token boundaries. For general-purpose text, tokenization has been the subject of extensive research resulting in the development of various advanced methods and techniques ( Jurafsky and Martin, 2009 ). However, for chemical and materials science text, accurate tokenization still requires substantial workarounds and revision of the standard approaches. Table 2 displays some typical examples of sentence tokenization produced by general-purpose tokenizers such as NLTK ( Bird et al., 2009 ) and SpaCy ( Honnibal and Johnson, 2015 ). As in the case of sentence segmentation, the major source of errors is the arbitrary usage of punctuation symbols within chemical formulas and other domain-specific terms. The chemical NLP toolkits such as OSCAR4 ( Jessop et al., 2011 ), ChemicalTagger ( Hawizy et al., 2011 ), and ChemDataExtractor ( Swain and Cole, 2016 ) implement their own rules- and dictionaries-based approaches to solve the over-tokenization problem. The advantage of chemical NLP toolkits is that they provide good performance on chemical terms, even if the rest of the text may have lower tokenization accuracy.
Examples of how different tokenizers split sentences into tokens
Reagents | | | | | and | Sm2O3 | were | mixed | |
Reagents | | | and | Sm2O3 | were | mixed | |
Reagents | | and | Sm2O3 | were | mixed | |
Reagents | | and | Sm2O3 | were | mixed | |
Reagents | | and | Sm2O3 | were | mixed | |
We | made | | | | | | | at | 1200 | | for | 2 |h | |
We | made | | | | | at | 1200 | | | for | 2 |h | |
We | made | | | | | at | 1200 | | for | 2 |h | |
We | made | | | at | 1200 | | for | 2 |h | |
We | made | | | | | at | 1200 | | | for | 2 |h | |
| | | | | | | | | | ceramics | was | investigated | |
| | | | ceramics | was | investigated | |
| | | | ceramics | was | investigated | |
| | ceramics | was | investigated | |
| | ceramics | was | investigated |
NLTK ( Bird et al., 2009 ) and SpaCy ( Honnibal and Johnson, 2015 ) are general-purpose tokenizing tools, whereas ChemDataExtractor ( Swain and Cole, 2016 ), OSCAR4 ( Jessop et al., 2011 ), ChemicalTagger ( Hawizy et al., 2011 ) are the tools trained for a scientific corpus. Tokens are bound by “ | ” symbol.
However, another prominent reason for tokenization errors is the lack of generally accepted rules regarding tokenization of chemical terms consisting of multiple words. For instance, complex terms such as “lithium battery” or “yttria-doped zirconium oxide” or “(Na 0.5 K 0.5 )NbO 3 + x wt% CuF 2 ” often become split into separate tokens “lithium” and “battery”, “yttria-doped” and “zirconium” and “oxide”, “(Na 0.5 K 0.5 )NbO 3 ” and “+” and “x wt% CuF 2 ”. This significantly modifies the meaning of the tokens and usually results in lowered accuracy of the named entity recognition (see below). Currently, this problem is solved case-by-case by creating task-specific wrappers for existing tokenizers and named entity recognition models ( Huang and Ling, 2019 ; Alperin et al., 2016 ; He et al., 2020 ). Building a robust approach for chemistry-specific sentence tokenization and data extraction requires a thorough development of standard nomenclature for complex chemical terms and materials names. We discuss this challenge in detail in Section 4 below.
Text normalization, part-of-speech tagging, and dependency parsing are often used to reduce the overall document lexicon and to design words' morphological and grammatical features used as an input for entity extraction and other TM tasks ( Leaman et al., 2015 ). Text normalization usually consists of lemmatization and/or its simpler version – stemming. While during the stemming the inflected word is cut to its stem (e.g. “changed” becomes “chang”), lemmatization aims to identify a word's lemma, i.e. a word's dictionary (canonical) form (e.g. “changed” becomes “change”) ( Jurafsky and Martin, 2009 ). Stemming and/or lemmatization help to reduce the variability of the language, but the decision whether to apply it or not, depends on the task and expected outcome. For instance, recognition of chemical terms will benefit less from stemming or lemmatization ( Corbett and Copestake, 2008 ) as it may truncate a word's ending resulting in a change of meaning (compare “methylation” vs. “methyl”). But when a word identifies, for example, a synthesis action, lemmatization helps to obtain the infinitive form of the verb and avoids redundancy in the document vocabulary ( Kononova et al., 2019 ).
Part-of-speech (POS) tagging identifies grammatical properties of the words and labels them with the corresponding tags, i.e. noun, verb, article, adjective, and others. This procedure does not modify the text corpus but rather provides linguistic and grammar-based features of the words that are used as input for ML models. A challenge in identifying the POS tags in scientific text often arises due to the ambiguity introduced by the word's context. As an example, compare two phrases: “the chemical tube is on the ground” and “the chemical was finely ground”. In the first case, the general-purpose POS tagger will work correctly, while in the second example, it will likely misidentify “chemical” and “ground” as adjective and noun, respectively. Therefore, using a standard POS tagger often requires re-training of the underlying NLP model, or post-processing and correction of the obtained results.
Dependency parsing creates a mapping of a linear sequence of sentence tokens into a hierarchical structure by resolving the internal grammatical dependencies between the words. This hierarchy is usually represented as a dependency tree , starting from the root token and going down to the terminal nodes. Parsing grammatical dependencies helps to deal with the arbitrary order of the words in the sentence and establishes semantic relationships between words and parts of the sentence ( Jurafsky and Martin, 2009 ). Grammatical dependency parsing is a rapidly developing area of NLP research providing a wealth of algorithms and models for general-purpose corpus (see www.nlpprogress.com for specific examples and evaluation).
Application of the currently existing dependency parsing models to scientific text comes with some challenges. First, sentences in science are often depersonalized, with excessive usage of passive and past verbs tense, and limited usage of pronouns. These features of the sentence are not well captured by general-purpose models. Secondly, the accuracy of the dependency tree construction is highly sensitive to punctuation and correct word forms, particularly verb tenses. As the scientific articles do not always exhibit perfect language grammar, the standard dependency parsing models can produce highly unpredictable results. To the best of our knowledge, these specific challenges of dependency parsing for scientific text have not yet been addressed or explored in detail.
Text representation modeling
The application of ML models requires mapping the document into a linear (vector) space. A common approach is to represent a text as a collection of multidimensional (and finite) numerical vectors that preserve the text features, e.g. synonymous words and phrases should have a similar vector representation, and phrases having an opposite meaning should be mapped into dissimilar vectors ( Harris, 1954 ). Modeling of the vectorized text representation is a broad and rapidly developing area of research ( Liu et al., 2020 ). In this section, we highlight only some of the approaches applied to scientific TM, whereas a more detailed discussion of the methods can be found elsewhere ( Jurafsky and Martin, 2009 ).
The bag-of-words model is one of the simplest models of text representation. It maps a document into a vector by counting how many times every word from a pre-defined vocabulary occurs in that document. While this model works well for recognizing specific topics defined by keywords, it does not reflect word context and cannot identify the importance of a particular word in the text. The latter can be solved by introducing a normalization factor and applying it to every word count. An example of such normalization is the tf-idf model ( term frequency-inverse document frequency ) which combines two metrics: the frequency of a word in a document and the fraction of the documents containing the word. The method can thereby identify the terms specific to a particular document. Bag-of-words and tf-idf are the most commonly used models to classify scientific documents or to identify parts of text with relevant information ( Court and Cole, 2018 ; Kim et al., 2017c ; Hiszpanski et al., 2020 ).
While bag-of-words and tf-idf are relatively versatile, they do not identify similarity between words across documents. This can be done through topic modeling approaches ( Blei, 2012 ). Topic modeling is a statistical model that examines the documents corpus and produces a set of abstract topics – clusters of the keywords that characterize a particular text. Then, every document is assigned with a probability distribution over topical clusters. Latent Dirichlet Allocation, a specific topic modeling approach ( Blei et al., 2003 ), has been applied to analyze the topic distribution over materials science papers on oxide synthesis ( Kim et al., 2017c ) and to classify these papers based by synthesis method used in the paper ( Huo et al., 2019 ).
Significant progress in TM and NLP has been achieved with the introduction of word embedding models which construct a vectorized representation of a single word rather than of the entire document. These approaches use the distributional hypothesis ( Harris, 1954 ) and are based on neural networks trained to predict word context in a self-supervised fashion. Multiple variations of word embeddings models include GloVe ( Pennington et al., 2014 ), ELMo ( Peters et al., 2018 ), word2vec ( Mikolov et al., 2013 ), and FastText ( Bojanowski et al., 2017 ). Besides being intuitively simple, the main advantage of word embedding models is their ability to capture similarity and relations between words based on mutual associations. Word embeddings are applied ubiquitously in materials science TM and NLP to engineer words features that are used as an input in various named entity recognition tasks ( Kononova et al., 2019 ; Kim et al., 2020a ; Huang and Ling, 2019 ; Weston et al., 2019 ). Moreover, they also seem to be a promising tool to discover properties of materials through words association ( Tshitoyan et al., 2019 ).
Recently, research on text representation has shifted toward context-aware models. A breakthrough was achieved with the development of sequence-to-sequence models ( Bahdanau et al., 2016 ) and, later, an attention mechanism ( Vaswani et al., 2017 ) for the purpose of neural machine translation (NMT). The most recent models such as Bidirectional Encoder Representations from Transformers (BERT) ( Devlin et al., 2019 ) and Generative Pre-trained Transformer (GPT) ( Radford et al., 2019 ; Brown et al., 2020 ) are multi-layered deep neural networks trained on very large unlabeled text corpora and demonstrate state-of-the-art NLP performance. These models offer fascinating opportunities for the future NLP development in domain of materials science ( Kuniyoshi et al., 2020 ; Vaucher et al., 2020 ). We discuss them in greater details in the Section 5.
Retrieval of information from the text
Information retrieval (IR) represents a broad spectrum of NLP tasks that extract various types of data from the pre-processed corpus ( Figure 3 ). The most ubiquitous IR task is named entities recognition (NER) which classifies text tokens in a specific category. In general-purpose text, these categories are usually names of locations, persons, etc., but in scientific literature the named entities can include chemical terms as well as physical parameters and properties. Extraction of action graphs of chemical synthesis and materials fabrication is another class of IR task that is closely related to NER. This task requires identification of action keywords, linking of them into a graph structure, and, if necessary, augmenting with the corresponding attributes characterizing the action (e.g. the action “material mixing” can be augmented with the attribute “mixing media” or “mixing time”). Lastly, data extraction from figures and tables represents another class of information that can be retrieved from scientific literature. This requires not only TM methods but also image recognition approaches. In this section we will mainly review the recent progress for chemical and materials NER and action graphs extraction and will provide a brief survey of the efforts spent on mining of scientific tables and figures.

Schematic representation of various information types that can be extracted from a typical materials science paper
Chemical NER is a broadly defined IR task. It usually includes identification of chemical and materials terms in the text but can also involve extraction of properties, physical characteristics, and synthesis actions. The early applications of chemical NER were mainly focused on extraction of drugs and biochemical information to perform more effective document searches ( Corbett and Copestake, 2008 ; Jessop et al., 2011 ; Rocktäschel et al., 2012 ; García-Remesal et al., 2013 ). Recently, chemical NER has shifted toward (in)organic materials and their characteristics ( Swain and Cole, 2016 ; He et al., 2020 ; Weston et al., 2019 ; Shah et al., 2018 ), polymers ( Tchoua et al., 2019 ), nanoparticles ( Hiszpanski et al., 2020 ), synthesis actions and conditions ( Vaucher et al., 2020 ; Hawizy et al., 2011 ; Kim et al., 2017c ; Kononova et al., 2019 ). The methods used for NER vary from traditional rule-based and dictionary look-up approaches to modern methodology built around advanced ML and NLP techniques, including conditional random field (CRF) ( Lafferty et al., 2001 ), long short-term memory (LSTM) neural networks ( Hochreiter and Schmidhuber, 1997 ), and others. A detailed survey on the chemical NER and its methods can be found in recent reviews ( Krallinger et al., 2017 ; Gurulingappa et al., 2013 ; Olivetti et al., 2020 ).
Extraction of chemical and materials terms has been a direction of intensive development in the past decade ( Krallinger et al., 2017 ; Eltyeb and Salim, 2014 ). The publicly available toolkits use rules- and dictionaries-based approaches (e.g LeadMine ( Lowe and Sayle, 2015 )), statistical models (e.g OSCAR4 ( Jessop et al., 2011 )), and, predominantly, the CRF model (e.g. ChemDataExtractor ( Swain and Cole, 2016 ), ChemSpot ( Rocktäschel et al., 2012 ), tmChem ( Leaman et al., 2015 )) to assign labels to chemical terms. Some recent works implemented advanced ML models such as bidirectional LSTM models ( He et al., 2020 ; Weston et al., 2019 ; Kuniyoshi et al., 2020 ) as well as a combination of deep convolutional and recurrent neural networks ( Korvigo et al., 2018 ) to identify chemical and material terms in the text and use context information to assign their roles. Table 3 shows a few examples of the NER output obtained using some of these tools and compares it to non-scientific NER models implemented in NLTK ( Bird et al., 2009 ) and SpaCy ( Honnibal and Johnson, 2015 ) libraries.
Examples of chemical NER extraction
– | |
‘Manganese’ ( ) | |
‘Aqueous’, ‘lithium’, ‘cobalt’, ‘manganese’, ‘nitrates’, ‘water’ | |
‘Lithium’, ‘cobalt’, ‘manganese nitrates’ | |
‘Lithium’, ‘cobalt’, ‘manganese nitrates’ | |
‘Lithium’, ‘cobalt’, ‘manganese nitrates’, ‘water’ | |
‘Lithium, cobalt, and manganese nitrates’, ‘water’ | |
– | |
– | |
‘Ce3+’, ‘Eu2+’, ‘Ca2Si5N8’ | |
‘Ce3+-Eu2+’, ‘Ca2Si5N8’ | |
‘Ce3+-Eu2+’, ‘Ca2Si5N8’ | |
‘Ce3+-Eu2’, ‘co’, ‘Ca2Si5N8’ | |
‘Ce3+-Eu2+ co-doped Ca2Si5N8’ | |
‘NO3’, ‘NO3’, ‘CH3COO’ ( ); ‘Ni’, ‘Cu’ ( ) | |
‘Bi2Cu1-xNixO4’ ( ) | |
‘Bi(NO3)3·5H2O’, ‘Ni(NO3)2·6H2O’, ‘Cu(CH3COO)2·H2O’ | |
‘Bi(NO3)3·5H2O’, ‘Ni(NO3)2·6H2O’, ‘Cu(CH3COO)2·H2O’, ‘Bi2Cu1-xNixO4’ | |
‘Bi(NO3)3·5H2O’, ‘Ni(NO3)2·6H2O’, ‘Cu(CH3COO)2·H2O’, ‘Bi2Cu1-xNixO4’ | |
‘Bi(NO3)3·5H2O’, ‘Ni(NO3)2·6H2O’, ‘Cu(CH3COO)2·H2O’, ‘Bi2Cu1-xNixO4’ | |
‘Bi(NO3)3·5H2O’, ‘Ni(NO3)2·6H2O’, ‘Cu(CH3COO)2·H2O’, ‘Bi2Cu1-xNixO4’ |
Examples of the chemical named entities extracted by the general-purpose NER tools NLTK ( Bird et al., 2009 ) and SpaCy ( Honnibal and Johnson, 2015 ), and the tools trained on chemical corpus OSCAR4 ( Jessop et al., 2011 ), tmChem ( Leaman et al., 2015 ), ChemSpot ( Rocktäschel et al., 2012 ), ChemDataExtractor ( Swain and Cole, 2016 ), BiLSTM chemical NER ( He et al., 2020 ). For the general-purpose tools, the assigned labels are given in parenthesis. For the chemical NERs, only entities labeled as chemical compounds are shown.
Often, the objective of scientific NER task is not limited to the identification of chemicals and materials, but also includes recognition of their associated attributes: structure and properties, amounts, roles, and actions performed on them. Assigning attributes to the entities is usually accomplished by constructing a graph-like structure that links together all the entities and build relations between them. A commonly used graph structure is the grammatical dependency tree for a sentence (see Section 2.3 ). Traversing the sentence trees allows for resolving relations between tokens, hence, link the entities with attributes. ChemicalTagger ( Hawizy et al., 2011 ) is one of the most robust frameworks that extends the OSCAR4 ( Jessop et al., 2011 ) functionality and provides tools for grammatical parsing of chemical text to find the relation between entities and the corresponding action verbs. Similarly, ChemDataExtractor ( Swain and Cole, 2016 ) can identify the chemical and physical characteristics (e.g. melting temperature) in the text and assign it to a material entity. A rules- and dictionaries-based relation-aware chemical NER model has been proposed by Shah et al. (2018) to build a search engine for publications. Weston et al. (2019) used the random forest decision model to resolve synonyms between chemical entities and materials-related terms. He et al. (2020) applied a two-step LSTM model to resolve the role of materials in a synthesis procedure. Onishi et al. (2018) used convolutional neural network model to build relations between materials, their mechanical properties and processing conditions which were extracted from publications by keywords search. Lastly, a combination of advanced NLP models has been recently used to extract the materials synthesis steps and link them into an action graph of synthesis procedures for solid-state battery materials ( Kuniyoshi et al., 2020 ) and inorganic materials in general ( Mysore et al., 2017 ).
Despite significant effort, the accuracy of the NER for chemical names and formulas is still relatively low compared to the general state-of-the-art NER models ( Baevski et al., 2019 ; Li et al., 2020 ). Figure 4 A displays the overall precision and recall for different chemical NER models reported in the corresponding publications. Both, precision and recall of the models vary from 60% to 98% ( Figure 4 A), whereas for the general-purpose NER, these values are >91% (see www.nlpprogress.com ). There are two major challenges that obstruct training of high-accuracy chemical NER models: (i) the lack of unambiguous definitions of the chemical tokens and their boundaries, and (ii) the lack of the robust annotation schema as well as comprehensive labeled training sets for the supervised ML algorithms. Oftentimes, researchers manually create their own training set for specific tasks but with limited use for more general goals. Therefore, the success of chemical NER becomes a trade-off between the size of the annotated set and model complexity: either using simple model with limited capabilities on a small set of labeled data, or investing effort into annotation of a large dataset and using it with advanced models providing a higher accuracy of data extraction.

Accuracy of chemical NER extraction
(A) Precision and recall of the published models for chemical NER manually extracted from the reports
Color denotes the primary algorithm underlying the model.
(B) Accuracy of the data extracted from materials synthesis paragraphs plotted against the complexity of the paragraphs. The accuracy is computed using chemical NER models developed by our team ( Kononova et al., 2019 ; He et al., 2020 ) to the manually annotated paragraphs. The text complexity is calculated as a Flesch-Kincaid grade level (FKGL) score indicating the education level required to understand the paragraph ( Kincaid et al., 1975 ). ρ is a Pearson correlation coefficient between the accuracy of NER model and the FKGL score.
An early attempt in creating a labeled data set for the chemical NER task was done by Kim et al. (2003) and Krallinger et al., 2015 . The GENIA and CHEMDNER sets provide annotation schema and labeled data of chemicals and drugs extracted from MEDLINE and PubMed abstracts, respectively. However, these corpora are heavily biased toward biomedicine and biochemical terms with only a small fraction of organic materials names present. The progress of the past few years brought a variety of annotated corpora to the materials science domain. Among the publicly available labeled dataset, there is the NaDev corpus consisting of 392 sentences and 2,870 terms on nanocrystal device development ( Dieb et al., 2015 ), the data set of 622 wet lab protocols of biochemical experiments and solution syntheses ( Kulkarni et al., 2018 ), a set of 9,499 labeled sentences on solid oxide fuel cells ( Friedrich et al., 2020 ), and an annotated set of 230 materials synthesis procedures ( Mysore et al., 2019 ).
Extraction of information from tables and figures is another branch of scientific IR that has been rapidly developing in the past few years. The specific format of the figures and tables in scientific papers imposes substantial challenges for the data retrieval process. First, it is common that images (and sometimes the tables) are not directly embedded in the HTML/XML text but instead contain a link to an external resource. Second, connecting tables/images to the specific part of the paper text is an advanced task that does not have a robust solution to date. Third, both tables and images can be very complex: images can include multiple panels and inserts that require segmentation, while tables may have combined several rows and columns imposing additional dependencies on the data. To the best of our knowledge, only a few publications have attempted to parse tables from the scientific literature using heuristics and machine learning approaches ( Jensen et al., 2019 ; Milosevic et al., 2019 ).
Image recognition methods have been broadly used in materials science but have so far been primarily focused on extracting information about the size, morphology, and the structure of materials from microscopy images. To date, the existing solutions for interpretation of microscopy images use variations of convolutional neural networks, and address diverse spectra of materials science problems ( Azimi et al., 2018 ; Matson et al., 2019 ; Maksov et al., 2019 ; Roberts et al., 2019 ). While these models demonstrate a remarkable accuracy when applied directly to microscopy output, they are not intended to separate and process the images embedded in scientific articles. Steps toward parsing of article's images were reported recently. Mukaddem et al. (2020) developed the ImageDataExtractor tool that uses a combination of OCR and CNN to extract the size and shape of the particles from microscopy images. Kim et al. (2020b) used Google Inception-V3 network ( Szegedy et al., 2016 ) to create the Livermore SEM Image Tools for electron microscopy images. This tool was later applied by Hiszpanski et al. (2020) to ∼35,000 publications to obtain information about the variability of nanoparticles sizes and morphologies.
Using text mining in materials science: case studies
Data-driven materials discovery usually relies either on computational methods to calculate the structure and properties of materials and collect them in databases ( Jain et al., 2013 ), or on experimental datasets that have been painstakingly collected and curated. Development of advanced approaches for scientific TM creates broad opportunities to augment such data with a large amount of reported but uncollected experimental results. A few large-scale data sets extracted from the scientific publications have become available over the last few years ( Court and Cole, 2018 ; Huang and Cole, 2020 ; Kim et al., 2017c ; Jensen et al., 2019 ; Kononova et al., 2019 ). In this Section, we survey the publicly available data sets created by retrieval of information from chemistry, physics, and materials science publications and discuss the most interesting results obtained from them.
Publicly available collections of text-mined data
While recently several data collections have been obtained by automated TM and NLP-based pipelines, there are a few large-scale data sets that have been manually extracted from scientific publications and are worth mentioning here.
The Pauling File Project ( Blokhin and Villars, 2020 ) is one of the biggest manually curated collections of data for inorganic crystalline substances, covering crystallographic data, physical properties, and phase diagrams. The Pauling File Project provides data for the Materials Platform for Data Science ( www.mpds.io ), Pearson’s Crystal Data ( www.crystalimpact.com ), and Springer Materials ( www.materials.springer.com ). Together, it contains more than 350,000 crystalline structures, 150,000 physical properties, and 50,000 phase diagrams extracted from the scientific literature in materials science, engineering, physics, and inorganic chemistry from 1891 to present. The quality and accuracy of the extracted records are high, and they include expert interpretation and a summary of the original text. Nonetheless, significant human labor is required to maintain and update this database. Moreover, due to the human interpretation of the data, the records are highly heterogeneous and may require additional processing and normalization.
The Dark Reactions Project ( www.darkreactions.haverford.edu ) is another prominent dataset extracted manually from laboratory journals containing 3,955 parameters of failed hydrothermal synthesis experiments ( Raccuglia et al., 2016 ). So-called “negative” sampling data are critical for ML applications that need to predict a “yes/no” answer. Unfortunately, the “no” results, i.e. unsuccessful experimental outcomes, are rarely published or made available to the broad research community. The Dark Reaction Project represents the first attempt to demonstrate the importance of sharing negative-result data within the chemistry and materials science domain.
A substantial effort in the automated extraction of materials properties from scientific publications has been done by the research group of J. Cole (University of Cambridge, UK). They developed ChemDataExtractor ( Swain and Cole, 2016 ), an NLP toolkit for chemical text and used it to build a large collection of phase transition temperatures of magnetic materials ( Court and Cole, 2018 ), and a dataset of electrochemical properties of battery materials ( Huang and Cole, 2020 ). The first set contains 39,822 records of Curie and Néel temperatures for various chemical compounds retrieved from 68,078 research articles ( Court and Cole, 2018 ). These data augment the MAGNDATA database – a collection of ∼1,000 magnetic structures manually extracted from publications by Gallego et al. ( Gallego et al., 2016a , 2016b ). The battery data set includes 292,313 records collected from 229,061 papers covering electrochemical properties of battery materials such as capacity, voltage, conductivity, Coulombic efficiency, and energy density. It enhances by more than an order of magnitude the manually constructed data set of Ghadbeigi et al. (2015) containing 16,000 property entries for Li-ion battery materials extracted from 200 publications.
A large-scale text-mined data collection of materials synthesis parameters has been developed by our team during the past few years. Kim et al. (2017c) generated a data set of synthesis operations and temperatures for 30 different oxide systems mined from 640,000 full-text publications. Later on, this set was extended by 1,214 sol-gel-synthesis conditions for germanium-based zeolites ( Jensen et al., 2019 ). A collection of 19,488 solid-state ceramics synthesis reactions containing precursors chemicals, synthesis steps and their attributes was generated from 53,538 materials science papers by Kononova et al. (2019) .
It is important to highlight that although the TM and NLP methods help to generate large-scale data sets, the output can suffer from lower accuracy of extraction as compared to any manually curated data set. For instance, the extraction precision of the Curie and Néel temperatures are ∼82% ( Court and Cole, 2018 ), and that of the electrochemical properties – ∼80% ( Huang and Cole, 2020 ), meaning that up to ∼20% of the obtained records have one or more attributes incorrectly extracted. The dataset of oxides synthesis parameters shows categorical accuracy (i.e. the fraction of the predicted labels of the text tokens that match the true labels) for the chemical NER task of ∼81% ( Kim et al., 2017c ). For the data set of solid-state synthesis reactions, precision (i.e. fraction of correctly extracted entities) of extracted synthesis parameters varies from ∼62% for fully accurate retrieval of synthesis conditions, to ∼97–99% for extraction of precursor materials and final products ( Kononova et al., 2019 ).
Text-mining-driven materials discoveries
Research exploring TM-based data-driven approaches to provide insights on materials emerged well before any progress in the development of robust NLP tools had been made. Several groups have attempted manual information extraction from a narrow set of publications with a specific scope.
The group of T. Sparks (University of Utah, US) explored the correlation between materials performance and the elemental availability for high-temperature thermoelectric materials ( Gaultois et al., 2013 ) and Li-ion battery materials ( Ghadbeigi et al., 2015 ). In both of these publications, the sets of physical parameters for materials classes were manually retrieved from queried materials science literature, and augmented with data on market concentration and Earth abundance for chemical elements. Based on this data the importance of considering global market state and geopolitical factors when designing materials was discussed.
An analysis of cellular toxicity of cadmium-containing semiconductor quantum dots was performed by applying random forest models to the 1,741 data samples manually collected from 307 relevant publications ( Oh et al., 2016 ). The authors found that the toxicity induced by quantum dots strongly correlates with their intrinsic properties, such as diameter, surface ligand, shell, and surface modification.
The data set of failed hydrothermal synthesis reactions collected in the course of the Dark Reactions Project (see above) was used to explore synthesis routes for organically templated vanadium selenites and molybdates ( Raccuglia et al., 2016 ). In particular, the authors applied support vector machine and decision tree models to define the upper/lower boundaries of the synthesis parameters that lead to formation of crystals from solution. The suggested synthesis routes were tested against real experiments and showed 89% success rate exceeding human intuition by 11%.
Although the manual approach to abstract a large text corpus is very laborious, it allows for obtaining high-quality data from the tables and figures as well as from the text, thus justifying the small size of these data sets. Nonetheless, a growing amount of research uses the automated TM pipelines to obtain a collection from which to initiate data-driven materials discoveries.
Young et al. (2018) developed a semi-automated TM pipeline to extract and analyze the growth conditions for four different oxide materials synthesized with pulsed laser deposition technique. They were able to obtain the range of growth temperatures and pressures and predict the relative values of critical temperatures by applying a decision tree classifier.
Cooper et al., 2019 applied a TM pipeline to effectively screen and sort organic dyes for panchromatic solar cells. Their approach identified 9,431 dye candidates which were then narrowed down to five prospective molecules for experimental validation. This work is an important step toward a so-called “design-to-device” approach to fabrication of advanced materials ( Cole, 2020 ). The approach consists of the four steps of (i) data extraction from literature, (ii) data augmentation with computations, (iii) AI-guided materials design, and (iv) experimental validation.
In other work, Court and Cole (2020) used the records of Curie and Néel temperatures text-mined from the scientific literature ( Court and Cole, 2018 ) (see previous section) to reconstruct the phase diagrams of magnetic and superconducting materials. They used the materials bulk and structural properties as descriptors in ML models to predict the critical temperature for a magnetic phase transition. The trained models are formulated into a web application that provides multiple options for predicting and exploring magnetic and superconducting properties of arbitrary materials ( www.magneticmaterials.org ).
Our team has extensively used TM aiming to uncover insights about materials synthesis from scientific publications. Kim et al. (2017b) explored the parameters of hydrothermal and calcination reactions for metal oxides by analyzing the data extracted from 22,065 scientific publications. They found a strong correlation between the complexity of the target material and the choice of reaction temperature. A decision tree model applied to predict synthesis routes for titania nanotubes identified the concentration of NaOH and synthesis temperature as the most important factors that lead to nanotube formation. A similar approach was used to predict the density of germanium-containing zeolite frameworks and to uncover their synthesis parameters ( Jensen et al., 2019 ).
In other work, Kim et al. (2017a) applied a variational autoencoder to learn the latent representation of synthesis parameters and to explore the conditions for the synthesis of TiO 2 brookite and for polymorph selection in the synthesis of MnO 2 . Their results showed that the use of ethanol as a reaction medium is a sufficient but not necessary condition to form the brookite phase of TiO 2 . Their latent representation of synthesis parameters also captures the requirement of alkalai ions for the generation of certain MnO 2 polymorph, consistent with ab initio findings ( Kitchaev et al., 2017 ). A conditional variational autoencoder was also used to generate a precursors list for some perovskite materials ( Kim et al., 2020a ).
Building relations between materials, their properties and applications and combining them into a so-called knowledge graph structure is an emerging area of research in materials science that became enabled by the development of scientific TM. Onishi et al. (2018) implemented the Computer-Aided Material Design (CAMaD) system which is an elegant TM framework that reconstructs and visualizes a knowledge graph in the form of a process-structure-property-performance chart for desired materials. While the presented performance of the CAMaD system is still limited, it demonstrates the capabilities of TM to create a comprehensive knowledge-based structure that can be used for optimization of materials design.
The relation between materials reported in the different application areas of materials science was explored by Tshitoyan et al. (2019) . They applied the word2vec model ( Mikolov et al., 2013 ) to 3 million abstracts to learn a vectorized representation of words and materials specifically. Interestingly, the model was able to not only learn some aspects of the chemistry underlying the relations between materials but also to draw a similarity between materials for different applications. In particular, it was demonstrated that such a cross-field correlation between the material properties required in different application could be used to predict novel thermoelectric materials. This work highlights an important aspect of scientific TM and NLP: its capability to uncover latent knowledge about a subject by comprehending a large amount of unstructured data – a task that is not possible for a human.
The question of materials similarity was also studied by He et al. (2020) . In their work, a measure of similarity for synthesis precursors was defined by two parameters: (i) the probability to substitute one precursor with another in the synthesis reaction for a common target material, and (ii) the area of overlap of synthesis temperature distributions for two precursors. The results demonstrate that some of the empirical rules widely used by researchers when choosing the precursors for materials synthesis can be learned from text data.
Challenges and Caveats of the Text-Mining-Driven Research
While TM and NLP are tremendously promising tools to extract the enormous amount of information locked up in published research, several challenges for the approach remain. We categorize these below.
Lack of annotated data
The lack of a large dataset corresponding to a “gold standard” of annotated data significantly slows down the development of robust high-precision methods for chemical NER. The majority of the existing annotated sets have been created to serve a specific purpose or subfield of materials science and their broad application is not straightforward. Current attempts to create standardization for annotated data in materials science are limited to chemical named entities with emphasis on organic chemistry ( Corbett et al., 2007 ; Krallinger et al., 2015 ; Kim et al., 2003 ). Building more structured databases of experimental data that can be related to the papers from which the data are sourced, could potentially help to test the performance of NLP methods. One can even conceive creating machine-annotated data based on an existing relation between data and publications. We are, however, not hopeful that the scientific community can come together around central data deposition without an incentive structure from publishers or government agencies, which further stresses the important role that TM will have in generating large amounts of materials data.
Ambiguity and lack of standard nomenclature to describe and categorize complex materials
An engineering material is not merely a compound that requires a chemical description. It can be a doped system, inhomogeneous, a multi-phase system, or a composite. Each of these complexities comes with its morphology and length scale. While for common chemical terms, IUPAC provides nomenclature recommendations, writers usually prefer to simplify them or use arbitrary notations for materials names if no standard terminology is established. For instance, even for a basic concept such as a doped material, various nomenclatures are used e.g. “Sc 2 (MoO 4 ) 3 :Eu 3+ ”, “Sc 2 (MoO 4 ) 3 + x% Eu 3+ ” or “Eu 3+ -doped Sc 2 (MoO 4 )”. Composites and mixtures can be written in various ways (e.g. (1-x)Pb(Zr 0.52 Ti 0.48 )O 3 -xBaTiO 3 or Pb(Zr 0.52 Ti 0.48 )O 3 + x wt% BaTiO 3 ). The abbreviated names of chemicals and materials (e.g. EDTA, BNT-BT-KNN, LMO) are also ubiquitous. Even within one journal or publisher no standards are applied. This complicates comparison and aggregation of extracted data across papers and requires substantial data post-processing in order to normalize and unify the results. In some cases it creates ambiguity that cannot be resolved, or whose resolution leads to errors.
Positive bias
Authors often “cherry-pick” data in the main body of a paper, either leaving out less successful data or moving it to supplementary information (which is often only available as PDF and with too low information content to do meaningful automated data extraction). This positive bias introduces substantial problems for ML models trained on these data, and requires caution when choosing the questions which one asks from ML models. In recent work, Jia et al. (2019) explored the effect of human bias in the choice of starting materials for the synthesis of metal organic crystals. They found a strong preference in the literature for selecting some reagents over others which was attributed to historically established rule-of-thumbs. In their explicit experimental testing they found no value of the implied precursor selection bias, something that an ML based on the published data would not have been able to resolve without additional data. In our own work on the prediction of novel compounds ( Fischer et al., 2006 ; Hautier et al., 2011 ) or their synthesis methods ( Kim et al., 2017b ), the lack of negative information is severely limiting. For example, the lack of a known compound at a given composition in a complex phase diagram may mean that no compound exists at that composition, or, that nobody has looked carefully for it. These are very different pieces of input information for an ML model that tries to predict which compositions are compound forming or not. One can imagine that some researchers may have investigated the specific composition, but because they did not find anything, the investigation was not reported. In a similar problem, failed synthesis experiments are rarely reported. This lack of negative data prevents one from capturing the boundaries on the space of possible ML outcomes. The effect of human bias on the quality of ML model predictions has not been investigated in detail and remains a challenging aspect of NLP-based data collections.
Language complexity and error accumulation
The narrative of a research paper is known to have a very specific style and language. It was shown for the corpus of newspapers of various subjects that the texts covering a scientific topic have the lowest readability score as compared to other topics, such as sports or weather ( Flaounas et al., 2013 ). To explore the dependence between complexity of a scientific paragraph and the quality of the data extraction, we computed the categorical accuracy (fraction of predicted values that match with actual values) of data extraction for ∼100 manually annotated paragraphs on materials synthesis and their corresponding Flesch-Kincaid grade level (FKGL) score ( Kincaid et al., 1975 ). Figure 4 B shows the extraction accuracy of synthesis steps and material entities per each paragraph obtained using the NLP models developed by our team previously ( Kononova et al., 2019 ; He et al., 2020 ), plotted against the corresponding FKGL score. Although the data are highly scattered, the negative correlation trend between the extraction accuracy and the FKGL score can be noticed. The computed Pearson correlation coefficients between the value of the FKGL score and the extraction accuracy of synthesis steps and materials entities are −0.42 and −0.38, respectively. It is worth noting that the correlation is stronger when the NLP model is applied to extract synthesis steps rather than materials entities. This can be explained with the fact that the context of a sentence defining a synthesis action is more ambiguous than that for materials terms ( Kim et al., 2019 ). This complexity stresses the need to improve the general NLP tools to deal with scientific text. The accuracy of the text processing along the TM pipeline is crucial as errors usually accumulate from step to step, leading to a strong reduction in quality and size of the output ( Kononova et al., 2019 ). As was noted before, the problem with sentence tokenization significantly affect the outcome of information extraction, in particular, chemical NER. Overcoming this problem may be possible by developing a hybrid NLP methods that introduces domain knowledge.
The accuracy of scientific NLP imposes constraints on the potential range of questions that the extracted data can address. Kauwe et al. (2019) have investigated the viability and fidelity of ML modeling based on a text-mined dataset. They used various ML algorithms and material structure models to predict the discharge capacity of battery materials after 25 cycles based on a dataset extracted from the literature and found inconclusive results. While one can speculate on the origin of this outcome, it is clear that the high level of uncertainty of the predictions can arise from invalid descriptors or models, as well as from the human bias and imperfectness of the experimental measurements ( Kauwe et al., 2019 ). As the “no-free-lunch” theorem states, there is no any particular ML model that will work best for a given task. Therefore, interpretation of results obtained by application of ML algorithms to text mined data should always be treated with caution and keeping the limitations of the input data in mind. In general, limitations of ML predictions are much more likely to be caused by limitations of input data than by problem with the ML method.
Future directions
Data are considered the fourth paradigm of science ( Tolle et al., 2011 ). Access to a large amount of data allows the quantification and more accurate testing of hypothesis, and even potentially the machine learning of the relation between composition, structure, processing and properties of materials. The Materials Genome Initiative (MGI) ( Holden, 2011 ) led to some highly successful data-driven research projects (e.g. www.mgi.gov , www.nsf.gov/ funding/pgm_summ.jsp?pims_id = 505073 and Jain et al., 2013 and Jain et al. (2016) ). But the personal experience of one of the authors in helping launch MGI is that experimental data is unlikely to be collected one piece at a time, by having scientists enter it in databases, the way it was envisioned by some when MGI started. While ML is an exciting new direction for materials research, it is telling that much of published ML work is either on computed data sets (which can be generated with high-throughput computing) ( Jain et al., 2011 ), or on very small experimental datasets, often containing no more than 50–100 data items. Because of this failure to collect experimental data in more organized ways, TM and NLP are likely to play a critical role in enabling more data-driven materials research. The willingness of publishers to share access to their large corpus for TM and several new developments in the NLP field are likely to lead to increased volume and quality of extracted information from scientific text.
The most notable advance in NLP in recent years has been the advent of transformer models , which have dramatically improved state-of-the-art performance on almost all benchmark tasks. The transformer uses an idea of sequence encoding-decoding ( Bahdanau et al., 2016 ) and creates a latent vectorized representation of a text. The advantage of the model is its attention functionality ( Vaswani et al., 2017 ) that allows for the model to recognize the key parts of a sequence that are crucial for understanding the meaning of text. The transformers have ushered in a new paradigm in NLP, whereby very large general-purpose models (with typically hundreds of millions of parameters) are pre-trained on publicly available corpora with unsupervised objective, before being fine-tuned to individual tasks. This so-called transfer learning approach allows the transformer to have high performance on supervised-training tasks with only a small number of training examples, significantly reducing the burden on human annotation.
From a materials science perspective, the transfer learning still meets some difficulties. The publicly available transformer models are pre-trained on general-purpose corpora, thus performing poorly on tasks involving scientific language. Moreover, the computational cost to train them “from scratch” is also significant: training BERTLarge on a corpus of 3.3 billion words with 64 TPU cores took 4 days ( Devlin et al., 2019 ). There have been a number of recent efforts to pre-train domain-specific transformer models on scientific text, including SciBERT ( Beltagy et al., 2019 ), BioBERT ( Lee et al., 2019 ), and MedBERT ( Rasmy et al., 2020 ). Although the corpus of available materials science publications ( Figure 1 ) is of comparable size to the corpora used to train the original BERT models, no materials science-specific pre-trained BERT-style model is publicly available to date. Training and release of such a model would be of tremendous impact for the materials science community.
Prominent progress has been also achieved for Neural Machine Translation (NMT), providing an opportunity to apply TM on scientific literature written in non-English languages. While NMT has reached parity with human translation in a number of languages ( Hassan, 2018 ), the dominant methodology relies on supervised training on a large bilingual corpus with parallel texts in source and target languages. However, there are significant difficulties in implementing the parallel-translation approach tailored specifically to the peculiarities of the scientific text. The domain-specific vocabulary of scientific texts requires a significant bilingual corpora for training the parallel-translation model ( Tehseen et al., 2018 ). The latest development in unsupervised NMT models ( Lample et al., 2017 , 2018 ; Artetxe et al., 2017 ) utilizes monolingual corpora, escaping the need for parallel texts. This opens possibilities for domain-specific training of the NMT and its application to the non-English scientific text.
As mentioned previously, the lack of large-scale annotated datasets often obstructs application of advanced NLP techniques for scientific TM. Crowd-sourcing for data collection may be a solution to this problem. Diverse approaches to collaborative data management have been widely used in projects such as OpenEI ( www.openei.org ), Folding@home ( www.foldingathome.org ) and others ( Zhai et al., 2013 ; Doan et al., 2011 ), as well as have proven to be highly efficient for gathering a large amount of data. To date, only a few projects have utilized crowd-sourcing in materials science TM research ( Young et al., 2018 ; Tchoua et al., 2016 ). But development of a collaborative data collection platform for application of NLP in materials science meets several challenges. First, building and maintenance of the software part requires a substantial labor investment one for which government science agencies do not seem quite ready for. Second, efficient data collection and annotation requires well established standards for labeling of scientific texts that can be unambiguously applied to a wide variety of research tasks.
The accelerated development of high-throughput computations and emergence of “big data” in materials science in the past few years has shifted focus toward data management and curation. This has resulted in engineering and production of high-quality databases with flexible graphical interfaces and programming APIs that provide facile and convenient access to the data for their mining and analysis ( Alberi et al., 2018 ). Rapidly growing sets of the data extracted from scientific publications call for development of a similar advanced infrastructure for representations, maintenance and distribution of these data.
Prevalent, broad and accurate data are a pillar of science. It inspires, negates, or validates theories. In society and business, data has become a highly valued commodity from which to take strategic decision, construct more effective marketing campaigns, or to improve products. For materials science to fully benefit from the new data paradigm significantly more effort will need to be directed toward data collection. TM and NLP are clearly a tool to make the results of hundred years of materials research available toward the realization of this paradigm.
Acknowledgment
Funding to support this work was provided by the National Science Foundation under grant numbers 1922311, 1922372, and 1922090, the Office of Naval Research (ONR) Award #N00014-16-1-2432, the U.S. Department of Energy, Office of Science, Office of Basic Energy Sciences, Materials Sciences and Engineering Division under contract no. DE-AC02-05-CH11231 (D2S2 program KCD2S2), the Assistant Secretary of Energy Efficiency and Renewable Energy, Vehicle Technologies Office, U.S. Department of Energy under contract no. DE-AC02-05CH11231, and the Energy Biosciences Institute through the EBI-Shell program (award nos. PT74140 and PT78473).
Author contributions
Conceptualization, O.K., T.H., and H.H.; Investigation, O.K., T.H., H.H., and A.T.; Writing – Original Draft, O.K.; Writing – Review & Editing, O.K., A.T., and E.A.O; Funding Acquisition, G.C. and E.A.O; Supervision, G.C. All authors participated in the discussion and modification of the manuscript structure and text.
Declaration of interests
The authors declare no competing interests.
Inclusion and diversity
One or more of the authors of this paper self-identifies as a member of the LGBTQ + community.
- Alberi K., Nardelli M., Zakutayev A., Mitas L., Curtarolo S., Jain A., Fornari M., Marzari N., Takeuchi I., Green M. The 2019 materials by design roadmap. J. Phys. D: Appl. Phys. 2018; 52.1 :013001. doi: 10.1088/1361-6463/aad926. [ CrossRef ] [ Google Scholar ]
- Alperin B.L., Kuzmin A.O., Ilina L.Y., Gusev V.D., Salomatina N.V., Parmon V.N. Terminology spectrum analysis of natural-language chemical documents: term-like phrases retrieval routine. J. Cheminform. 2016; 8 :22. doi: 10.1186/s13321-016-0136-4. [ PMC free article ] [ PubMed ] [ CrossRef ] [ Google Scholar ]
- Artetxe M., Labaka G., Agirre E. Proceedings of the 55th Annual Meeting of the Association for Computational Linguistics (Volume 1: Long Papers) Association for Computational Linguistics; 2017. Learning bilingual word embeddings with (almost) no bilingual data; pp. 451–462. [ CrossRef ] [ Google Scholar ]
- Azimi S.M., Britz D., Engstler M., Fritz M., Mücklich F. Advanced steel microstructural classification by deep learning methods. Sci. Rep. 2018; 8 :2128. doi: 10.1038/s41598-018-20037-5. [ PMC free article ] [ PubMed ] [ CrossRef ] [ Google Scholar ]
- Baevski A., Edunov S., Liu Y., Zettlemoyer L., Auli M. Proceedings of the 2019 Conference on Empirical Methods in Natural Language Processing and the 9th International Joint Conference on Natural Language Processing (EMNLP-IJCNLP) Association for Computational Linguistics; 2019. Cloze-driven pretraining of selfattention networks; pp. 5360–5369. [ CrossRef ] [ Google Scholar ]
- Bahdanau D., Cho K., Bengio Y. Neural machine translation by jointly learning to align and translate. arXiv. 2016 [ Google Scholar ]
- Beltagy I., Lo K., Cohan A. Proceedings of the 2019 Conference on Empirical Methods in Natural Language Processing and the 9th International Joint Conference on Natural Language Processing (EMNLP-IJCNLP) Association for Computational Linguistics; 2019. SciBERT: a pretrained language model for scientific text; pp. 3615–3620. [ CrossRef ] [ Google Scholar ]
- Bird S., Loper E., Klein E. O’Reilly Media Inc.; 2009. Natural Language Processing with Python. [ Google Scholar ]
- Blei D.M. Probabilistic topic models. Commun. ACM. 2012; 55 :77–84. [ Google Scholar ]
- Blei D.M., Ng A.Y., Jordan M.I. Latent dirichlet allocation. J. Mach. Learn. Res. 2003; 3 :993–1022. [ Google Scholar ]
- Blokhin E., Villars P. Handbook of Materials Modeling: Methods: Theory and Modeling. 2020. The PAULING FILE project and materials platform for data science: from big data toward materials genome; pp. 1837–1861. [ CrossRef ] [ Google Scholar ]
- Bojanowski P., Grave E., Joulin A., Mikolov T. Enriching word vectors with subword information. Trans. Assoc. Comput. Linguistics. 2017; 5 :135–146. doi: 10.1162/tacl_a_00051. [ CrossRef ] [ Google Scholar ]
- Bornmann L., Mutz R. Growth rates of modern science: a bibliometric analysis based on the number of publications and cited references. J. Assn. Inf. Sci. Tec. 2015; 66 :2215. [ Google Scholar ]
- Brown T.B., Mann B., Ryder N., Subbiah M., Kaplan J., Dhariwal P., Neelakantan A., Shyam P., Sastry G., Askell A. Language models are few-shot learners. arXiv. 2020 [ Google Scholar ]
- Chomsky N. Three models for the description of language. IRE Trans. Inf. Theor. 1956; 2 :113–124. doi: 10.1109/TIT.1956.1056813. [ CrossRef ] [ Google Scholar ]
- Cole J.M. A design-to-device pipeline for data-driven materials discovery. Acc. Chem. Res. 2020; 53 :599–610. doi: 10.1021/acs.accounts.9b00470. [ PubMed ] [ CrossRef ] [ Google Scholar ]
- Constantin A., Pettifer S., Voronkov A. Proceedings of the 2013 ACM Symposium on Document Engineering. DocEng ’13. Association for Computing Machinery; 2013. PDFX: fully-automated PDF-to-XML conversion of scientific literature; pp. 177–180. [ CrossRef ] [ Google Scholar ]
- Cooper C., Beard E., Vazquez-Mayagoitia A., Stan L., Stenning G., Nye D., Vigil J., Tomar T., Jia J., Bodedla G. Design-to-Device approach affords panchromatic Co-sensitized solar cells. Adv. Energy Mater. 2019; 9 :1802820. doi: 10.1002/aenm.201802820. [ CrossRef ] [ Google Scholar ]
- Corbett P., Batchelor C., Teufel S. Annotation of chemical named entities. Tech. Rep. 2007:57–64. [ Google Scholar ]
- Corbett P., Copestake A. Cascaded classifiers for confidence-based chemical named entity recognition. BMC Bioinformatics. 2008; 9 (Suppl 11):S4. doi: 10.1186/1471-2105-9-S11-S4. [ PMC free article ] [ PubMed ] [ CrossRef ] [ Google Scholar ]
- Court C., Cole J.M. Auto-generated materials database of Curie and Néel temperatures via semi-supervised relationship extraction. Sci. Data. 2018; 5 :180111. doi: 10.1038/sdata.2018.111. [ PMC free article ] [ PubMed ] [ CrossRef ] [ Google Scholar ]
- Court C.J., Cole J.M. Magnetic and superconducting phase diagrams and transition temperatures predicted using text mining and machine learning. Npj Comput. Mater. 2020; 6 :1–9. doi: 10.1038/s41524-020-0287-8. [ CrossRef ] [ Google Scholar ]
- de Jong M., Chen W., Angsten T., Jain A., Notestine R., Gamst A., Sluiter M., Ande C., van der Zwaag S., Plata J. Charting the complete elastic properties of inorganic crystalline compounds. Sci. Data. 2015; 2 :150009. doi: 10.1038/sdata.2015.9. [ PMC free article ] [ PubMed ] [ CrossRef ] [ Google Scholar ]
- Devlin J., Chang M.-W., Lee K., Toutanova K. Bert: pre-training of deep bidirectional transformers for language understanding. arXiv. 2019 [ Google Scholar ]
- Dieb T.M., Yoshioka M., Hara S., Newton M.C. Framework for automatic information extraction from research papers on nanocrystal devices. Beilstein J. Nanotechnol. 2015; 6 :1872–1882. doi: 10.3762/bjnano.6.190. [ PMC free article ] [ PubMed ] [ CrossRef ] [ Google Scholar ]
- Doan A., Ramakrishnan R., Halevy A.Y. Crowdsourcing systems on the world-wide web. Commun. ACM. 2011; 54 :86–96. doi: 10.1145/1924421.1924442. [ CrossRef ] [ Google Scholar ]
- Eltyeb S., Salim N. Chemical named entities recognition: a review on approaches and applications. J. Cheminform. 2014; 6 :1–12. doi: 10.1186/1758-2946-6-17. [ PMC free article ] [ PubMed ] [ CrossRef ] [ Google Scholar ]
- Fischer C.C., Tibbetts K.J., Morgan D., Ceder G. Predicting crystal structure by merging data mining with quantum mechanics. Nat. Mater. 2006; 5 :641–646. doi: 10.1038/nmat1691. [ PubMed ] [ CrossRef ] [ Google Scholar ]
- Flaounas I., Ali O., Lansdall-Welfare T., De Bie T., Mosdell N., Lewis J., Cristianini N. Research methods in the age of digital journalism. Digital Journalism. 2013; 1 :102–116. doi: 10.1080/21670811.2012.714928. [ CrossRef ] [ Google Scholar ]
- Friedrich A., Adel H., Tomazic F., Hingerl J., Benteau R., Marusczyk A., Lange L. Proceedings of the 58th Annual Meeting of the Association for Computational Linguistics. Association for Computational Linguistics; 2020. The SOFCExp corpus and neural approaches to information extraction in the materials science domain; pp. 1255–1268. [ CrossRef ] [ Google Scholar ]
- Gallego S.V., Perez-Mato J.M., Elcoro L., Tasci E.S., Hanson R.M., Aroyo M.I., Madariaga G. MAGNDATA: towards a database of magnetic structures. II. The incommensurate case. J. Appl. Cryst. 2016; 49 :1941–1956. doi: 10.1107/S1600576716015491. [ CrossRef ] [ Google Scholar ]
- Gallego S.V., Perez-Mato J.M., Elcoro L., Tasci E.S., Hanson R.M., Momma K., Aroyo M.I., Madariaga G. MAGNDATA: towards a database of magnetic structures. I. The commensurate case. J. Appl. Cryst. 2016; 49 :1750–1776. doi: 10.1107/S1600576716012863. [ CrossRef ] [ Google Scholar ]
- García-Remesal M., García-Ruiz A., Pérez-Rey D., De La Iglesia D., Maojo V. Using nanoinformatics methods for automatically identifying relevant nanotoxicology entities from the literature. Biomed. Res. Int. 2013; 2013 doi: 10.1155/2013/410294. [ PMC free article ] [ PubMed ] [ CrossRef ] [ Google Scholar ]
- Gaultois M.W., Sparks T.D., Borg C.K.H., Seshadri R., Bonificio W.D., Clarke D.R. Data- driven review of thermoelectric materials: performance and resource considerations. Chem. Mater. 2013; 25 :2911–2920. doi: 10.1021/cm400893e. [ CrossRef ] [ Google Scholar ]
- Ghadbeigi L., Harada J.K., Lettiere B.R., Sparks T.D. Performance and resource considerations of Li-ion battery electrode materials. Energy Environ. Sci. 2015; 8 :1640–1650. doi: 10.1039/C5EE00685F. [ CrossRef ] [ Google Scholar ]
- Gurulingappa H., Mudi A., Toldo L., Hofmann-Apitius M., Bhate J. Challenges in mining the literature for chemical information. RSC Adv. 2013; 3 :16194. doi: 10.1039/c3ra40787j. [ CrossRef ] [ Google Scholar ]
- Harris Z.S. Distributional structure. Word. 1954; 10 :146–162. [ Google Scholar ]
- Hassan H. Achieving human parity on automatic Chinese to English news translation. arXiv. 2018 [ Google Scholar ]
- Hautier G., Fischer C., Ehrlacher V., Jain A., Ceder G. Data mined ionic substitutions for the discovery of new compounds. Inorg. Chem. 2011; 50 :656–663. doi: 10.1021/ic102031h. [ PubMed ] [ CrossRef ] [ Google Scholar ]
- Hawizy L., Jessop D.M., Adams N., Murray-Rust P. ChemicalTagger: a tool for semantic text-mining in chemistry. J. Cheminform. 2011; 3 :1–13. doi: 10.1186/1758-2946-3-17. [ PMC free article ] [ PubMed ] [ CrossRef ] [ Google Scholar ]
- He T., Sun W., Huo H., Kononova O., Rong Z., Tshitoyan V., Botari T., Ceder G. Similarity of precursors in solid-state synthesis as text-mined from scientific literature. Chem. Mater. 2020; 32 :7861–7873. doi: 10.1021/acs.chemmater.0c02553. [ CrossRef ] [ Google Scholar ]
- Hiszpanski A.M., Gallagher B., Chellappan K., Li P., Liu S., Kim H., Kailkhura B., Han J., Buttler D., Han T.Y.-J. Nanomaterials synthesis insights from machine learning of scientific articles by extracting, structuring, and visualizing knowledge. J. Chem. Inf. Model. 2020; 60 :2876–2887. doi: 10.1021/acs.jcim.0c00199. [ PubMed ] [ CrossRef ] [ Google Scholar ]
- Hochreiter S., Schmidhuber J. Long short-term memory. Neural Comput. 1997; 9 :1735–1780. doi: 10.1162/neco.1997.9.8.1735. [ PubMed ] [ CrossRef ] [ Google Scholar ]
- Holden J. Tech. rep. National Science and Technology Council; 2011. Materials Genome Initiative for Global Competitiveness. [ Google Scholar ]
- Honnibal M., Johnson M. Proceedings of the 2015 Conference on Empirical Methods in Natural Language Processing. Association for Computational Linguistics; 2015. An improved non-monotonic transition system for dependency parsing; pp. 1373–1378. [ CrossRef ] [ Google Scholar ]
- Huang L., Ling C. Representing multiword chemical terms through phrase-level preprocessing and word embedding. ACS Omega. 2019; 4 :18510–18519. doi: 10.1021/acsomega.9b02060. [ PMC free article ] [ PubMed ] [ CrossRef ] [ Google Scholar ]
- Huang S., Cole J.M. A database of battery materials auto-generated using ChemDataExtractor. Sci. Data. 2020; 7 :1–13. doi: 10.1038/s41597-020-00602-2. [ PMC free article ] [ PubMed ] [ CrossRef ] [ Google Scholar ]
- Huo H., Rong Z., Kononova O., Sun W., Botari T., He T., Tshitoyan V., Ceder G. Semisupervised machine-learning classification of materials synthesis procedures. Npj Comput. Mater. 2019; 5 :1–7. doi: 10.1038/s41524-019-0204-1. [ CrossRef ] [ Google Scholar ]
- Jain A., Hautier G., Moore C.J., Ong S.-P., Fischer C.C., Mueller T., Persson K.A., Ceder G. A high-throughput infrastructure for density functional theory calculations. Comput. Mater. Sci. 2011; 50 :2295–2310. doi: 10.1016/j.commatsci.2011.02.023. [ CrossRef ] [ Google Scholar ]
- Jain A., Ong S.P., Hautier G., Chen W., Richards W., Dacek S., Cholia S., Gunter D., Skinner D., Ceder G., Persson K. Commentary: the Materials Project: a materials genome approach to accelerating materials innovation. APL Mater. 2013; 1 :011002. doi: 10.1063/1.4812323. [ CrossRef ] [ Google Scholar ]
- Jain A., Persson K.A., Ceder G. Research Update: the materials genome initiative: data sharing and the impact of collaborative ab initio databases. APL Mater. 2016; 4 :053102. doi: 10.1063/1.4944683. [ CrossRef ] [ Google Scholar ]
- Jensen Z., Kim E., Kwon S., Gani T.Z.H., Roman-Leshkov Y., Moliner M., Corma A., Olivetti E. A machine learning approach to zeolite synthesis enabled by automatic literature data extraction. ACS Cent. Sci. 2019; 5 :892–899. doi: 10.1021/acscentsci.9b00193. [ PMC free article ] [ PubMed ] [ CrossRef ] [ Google Scholar ]
- Jessop D.M., Adams S.E., Willighagen E.L., Hawizy L., Murray-Rust P. OSCAR4: a flexible architecture for chemical text-mining. J. Cheminform. 2011; 3 :41. doi: 10.1186/1758-2946-3-41. [ PMC free article ] [ PubMed ] [ CrossRef ] [ Google Scholar ]
- Jia X., Lynch A., Huang Y., Danielson M., Lang’at I., Milder A., Ruby A.E., Wang H., Friedler S.A., Norquist A.J. Anthropogenic biases in chemical reaction data hinder exploratory inorganic synthesis. Nature. 2019; 573 :251–255. doi: 10.1038/s41586-019-1540-5. [ PubMed ] [ CrossRef ] [ Google Scholar ]
- Jurafsky D., Martin J.H. Pearson Prentice Hall; 2009. Speech and Language Processing: An Introduction to Natural Language Processing, Computational Linguistics, and Speech Recognition. Prentice Hall Series in Artificial Intelligence. [ Google Scholar ]
- Kauwe S., Rhone T., Sparks T. Data-driven studies of Li-Ion-Battery materials. Crystals. 2019; 9 :54. doi: 10.3390/cryst9010054. [ CrossRef ] [ Google Scholar ]
- Kim E., Huang K., Jegelka S., Olivetti E. Virtual screening of inorganic materials synthesis parameters with deep learning. Npj Comput. Mater. 2017; 3 :53. doi: 10.1038/s41524-017-0055-6. [ CrossRef ] [ Google Scholar ]
- Kim E., Huang K., Kononova O., Ceder G., Olivetti E. Distilling a materials synthesis Ontology. Matter. 2019; 1 :8–12. doi: 10.1016/j.matt.2019.05.011. [ CrossRef ] [ Google Scholar ]
- Kim E., Huang K., Saunders A., McCallum A., Ceder G., Olivetti E. Materials synthesis insights from scientific literature via text extraction and machine learning. Chem. Mater. 2017; 29 :9436–9444. doi: 10.1021/acs.chemmater.7b03500. [ CrossRef ] [ Google Scholar ]
- Kim E., Huang K., Tomala A., Matthews S., Strubell E., Saunders A., McCallum A., Olivetti E. Machine-learned and codified synthesis parameters of oxide materials. Sci. Data. 2017; 4 :170127. doi: 10.1038/sdata.2017.127. [ PMC free article ] [ PubMed ] [ CrossRef ] [ Google Scholar ]
- Kim E., Jensen Z., van Grootel A., Huang K., Staib M., Mysore S., Chang H.S., Strubell E., McCallum A., Jegelka S., Olivetti E. Inorganic materials synthesis planning with literature-trained neural networks. J. Chem. Inf. Model. 2020; 60 :1194–1201. doi: 10.1021/acs.jcim.9b00995. [ PubMed ] [ CrossRef ] [ Google Scholar ]
- Kim H., Han J., Han T.Y.-J. Machine vision-driven automatic recognition of particle size and morphology in SEM images. Nanoscale. 2020; 12 :19461–19469. doi: 10.1039/D0NR04140H. [ PubMed ] [ CrossRef ] [ Google Scholar ]
- Kim J.-D., Ohta T., Tateisi Y., Tsujii J. GENIA corpus – a semantically annotated corpus for bio-textmining. Bioinformatics. 2003; 19 (suppl_1):i180–i182. doi: 10.1093/bioinformatics/btg1023. [ PubMed ] [ CrossRef ] [ Google Scholar ]
- Kincaid J.P., Fishburne R.P., Jr., Rogers R.L., Chissom B.S. Tech. rep. Institute for Simulation and Training, University of Central Florida; 1975. Derivation of New Readability Formulas (Automated Readability Index, Fog Count and Flesch Reading Ease Formula) for Navy Enlisted Personnel. [ Google Scholar ]
- Kitchaev Daniil A., Dacek Stephen T., Sun Wenhao, Ceder Gerbrand. Thermodynamics of phase selection in MnO2 framework structures through alkali intercalation and hydration. J. Am. Chem. Soc. 2017; 139 :2672–2681. doi: 10.1021/jacs.6b11301. [ PubMed ] [ CrossRef ] [ Google Scholar ]
- Kleene S.C. Princeton. Princeton University Press; 1956. Representation of events in nerve nets and finite automata; pp. 3–42. [ CrossRef ] [ Google Scholar ]
- Kolářik C., Klinger R., Friedrich C.M., Hofmann-Apitius M., Fluck J. Workshop on Building and Evaluating Resources for Biomedical Text Mining. 2008. Chemical names: terminological resources and corpora annotation; pp. 51–58. [ Google Scholar ]
- Kononova O., Huo H., He T., Rong Z., Botari T., Sun W., Tshitoyan V., Ceder G. Text-mined dataset of inorganic materials synthesis recipes. Sci. Data. 2019; 6 :1–11. doi: 10.1038/s41597-019-0224-1. [ PMC free article ] [ PubMed ] [ CrossRef ] [ Google Scholar ]
- Korvigo I., Holmatov M., Zaikovskii A., Skoblov M. Putting hands to rest: efficient deep CNNRNN architecture for chemical named entity recognition with no hand-crafted rules. J. Cheminform. 2018; 10 :28. doi: 10.1186/s13321-018-0280-0. [ PMC free article ] [ PubMed ] [ CrossRef ] [ Google Scholar ]
- Krallinger M., Rabal O., Leitner F., Vazquez M., Salgado D., Lu Z., Leaman R., Lu Y., Ji D., Lowe D. The CHEMDNER corpus of chemicals and drugs and its annotation principles. J. Cheminform. 2015; 7 :S2. doi: 10.1186/1758-2946-7-S1-S2. [ PMC free article ] [ PubMed ] [ CrossRef ] [ Google Scholar ]
- Krallinger M., Rabal O., Lourenço A., Oyarzabal J., Valencia A. Information retrieval and text mining Technologies for chemistry. Chem. Rev. 2017; 117 :7673–7761. doi: 10.1021/acs.chemrev.6b00851. [ PubMed ] [ CrossRef ] [ Google Scholar ]
- Kulkarni C., Xu W., Ritter A., Machiraju R. Volume 2. Association for Computational Linguistics; 2018. An annotated corpus for machine reading of instructions in wet lab protocols; pp. 97–106. (Proceedings of the 2018 Conference of the North American Chapter of the Association for Computational Linguistics: Human Language Technologies). (Short Papers) [ CrossRef ] [ Google Scholar ]
- Kuniyoshi Fusataka, Makino Kohei, Ozawa Jun, Miwa Makoto. Annotating and extracting synthesis process of all-solid-state batteries from scientific literature. arXiv. 2020 [ Google Scholar ]
- Kurgan L.A., Musilek P. A survey of knowledge discovery and data mining process models. Knowledge Eng. Rev. 2006; 21 :1–24. doi: 10.1017/S0269888906000737. [ CrossRef ] [ Google Scholar ]
- Lafferty, J., A. McCallum, and F. Pereira (2001). Conditional Random Fields: Probabilistic Models for Segmenting and Labeling Sequence Data. In: Proceedings of the Eighteenth International Conference on Machine Learning. ICML ’01. San Francisco, CA, USA: Morgan Kaufmann Publishers Inc., pp. 282–289. isbn: 1558607781.
- Lample G., Conneau A., Denoyer L., Ranzato M.A. Unsupervised machine translation using monolingual corpora only. arXiv. 2017 [ Google Scholar ]
- Lample G., Ott M., Conneau A., Denoyer L., Ranzato M. Proceedings of the 2018 Conference on Empirical Methods in Natural Language Processing. Association for Computational Linguistics; 2018. Phrase-based & neural unsupervised machine translation; pp. 5039–5049. [ CrossRef ] [ Google Scholar ]
- Leaman R., Wei C.-H., Lu Z. tmChem: a high performance approach for chemical named entity recognition and normalization. J. Cheminform. 2015; 7 :S3. doi: 10.1186/1758-2946-7-S1-S3. [ PMC free article ] [ PubMed ] [ CrossRef ] [ Google Scholar ]
- Lee J., Yoon W., Kim S., Kim D., Kim S., So C.-H., Kang J. BioBERT: a pre-trained biomedical language representation model for biomedical text mining. Bioinformatics. 2019; 36 :1234–1240. doi: 10.1093/bioinformatics/btz682. [ PMC free article ] [ PubMed ] [ CrossRef ] [ Google Scholar ]
- Li X., Sun X., Meng Y., Liang J., Wu F., Li J. Proceedings of the 58th Annual Meeting of the Association for Computational Linguistics. Association for Computational Linguistics; 2020. Dice loss for data-imbalanced NLP tasks; pp. 465–476. [ CrossRef ] [ Google Scholar ]
- Liu Z., Lin Y., Sun M. 1st ed. Springer; 2020. Representation Learning for Natural Language Processing. [ CrossRef ] [ Google Scholar ]
- Lowe D.M., Sayle R.A. LeadMine: a grammar and dictionary driven approach to entity recognition. J. Cheminform. 2015; 7 :S5. doi: 10.1186/1758-2946-7-S1-S5. [ PMC free article ] [ PubMed ] [ CrossRef ] [ Google Scholar ]
- Luhn H.P. The automatic creation of literature abstracts. IBM J. Res. Dev. 1958; 2 :159–165. doi: 10.1147/rd.22.0159. [ CrossRef ] [ Google Scholar ]
- Luong M.-T., Nguyen T.D., Kan M.-Y. Logical structure recovery in scholarly articles with rich document features. Int. J. Digit. Libr. Syst. 2010; 1 :1–23. doi: 10.4018/jdls.2010100101. [ CrossRef ] [ Google Scholar ]
- Mahdavi M., Zanibbi R., Mouchère H., Viard-Gaudin C., Garain U. 2019 International Conference on Document Analysis and Recognition (ICDAR) IEEE; 2019. ICDAR 2019 CROHME+ TFD: competition on recognition of handwritten mathematical expressions and typeset formula detection; pp. 1533–1538. [ CrossRef ] [ Google Scholar ]
- Maksov A., Dyck O., Wang K., Xiao K., Geohegan D.B., Sumpter B.G., Vasudevan R.K., Jesse S., Kalinin S.V., Ziatdinov M. Deep learning analysis of defect and phase evolution during electron beam-induced transformations in WS2. Npj Comput. Mater. 2019; 5 :12. doi: 10.1038/s41524-019-0152-9. [ CrossRef ] [ Google Scholar ]
- Matson T., Farfel M., Levin N., Holm E., Wang C. Machine learning and computer vision for the classification of carbon nanotube and nanofiber structures from transmission electron microscopy data. Microsc. Microanalysis. 2019; 25 :198–199. doi: 10.1017/S1431927619001727. [ CrossRef ] [ Google Scholar ]
- Memon J., Sami M., Khan R.A., Uddin M. Handwritten optical character recognition (OCR): a comprehensive systematic literature review (SLR) IEEE Access. 2020; 8 :142642–142668. doi: 10.1109/ACCESS.2020.3012542. [ CrossRef ] [ Google Scholar ]
- Mendenhall T.C. The characteristic curves of composition. Science. 1887:237–246. doi: 10.1126/science.ns-9.214S.237. [ PubMed ] [ CrossRef ] [ Google Scholar ]
- Mikolov T., Sutskever I., Chen K., Corrado G.S., Dean J. Distributed representations of words and phrases and their compositionality. arXiv. 2013 [ Google Scholar ]
- Milosevic N., Gregson C., Hernandez R., Nenadic G. A framework for information extraction from tables in biomedical literature. IJDAR. 2019; 22 :55–78. doi: 10.1007/s10032-019-00317-0. [ CrossRef ] [ Google Scholar ]
- Miner G., Elder J., Fast A., Hill T., Nisbet R., Delen D. Elsevier Sci.; 2012. Practical text mining and statistical analysis for non-structured text data applications. [ Google Scholar ]
- Morgan D., Jacobs R. Opportunities and challenges for machine learning in materials science. Annu. Rev. Mater. Res. 2020; 50 doi: 10.1146/annurev-matsci-070218-010015. [ CrossRef ] [ Google Scholar ]
- Mouchère H., Zanibbi R., Garain U., Viard-Gaudin C. Advancing the state of the art for handwritten math recognition: the CROHME competitions, 2011–2014. IJDAR. 2016; 19 :173–189. doi: 10.1007/s10032-016-0263-5. [ CrossRef ] [ Google Scholar ]
- Mukaddem K.T., Beard E.J., Yildirim B., Cole J.M. ImageDataExtractor: a tool to extract and quantify data from microscopy images. J. Chem. Inf. Model. 2020; 60 :2492–2509. doi: 10.1021/acs.jcim.9b00734. [ PubMed ] [ CrossRef ] [ Google Scholar ]
- Mysore, S., Z. Jensen, E. Kim, K. Huang, H.-S. Chang, E. Strubell, J. Flanigan, A. McCallum, and E. Olivetti (2019). The Materials Science Procedural Text Corpus: Annotating Materials Synthesis Procedures with Shallow Semantic Structures. In: LAW 2019-13th Linguistic Annotation Workshop, Proceedings of the Workshop, pp. 56–64. arXiv: 1905.06939.
- Mysore S., Kim E., Strubell E., Liu A., Chang H.-S., Kompella S., Huang K., McCallum A., Olivetti E. Automatically extracting action graphs from materials science synthesis procedures. arXiv: 2017 [ Google Scholar ]
- Oh E., Liu R., Nel A., Gemill K.B., Bilal M., Cohen Y., Medintz I.L. Meta-analysis of cellular toxicity for cadmium-containing quantum dots. Nat. Nanotech. 2016; 11 :479. [ PubMed ] [ Google Scholar ]
- Olivetti E., Cole J., Kim E., Kononova O., Ceder G., Han T., Hiszpanksi A. Data-driven materials research enabled by natural language processing. Appl. Phys. Rev. 2020; 7 :041317. doi: 10.1063/5.0021106. [ CrossRef ] [ Google Scholar ]
- Onishi T., Kadohira T., Watanabe I. Relation extraction with weakly supervised learning based on process-structure-property-performance reciprocity. Sci. Technol. Adv. Mater. 2018; 19 :649–659. doi: 10.1080/14686996.2018.1500852. [ PMC free article ] [ PubMed ] [ CrossRef ] [ Google Scholar ]
- Pennington J., Socher R., Manning C. Proceedings of the 2014 Conference on Empirical Methods in Natural Language Processing (EMNLP) Association for Computational Linguistics; 2014. GloVe: global vectors for word representation; pp. 1532–1543. [ CrossRef ] [ Google Scholar ]
- Peters M., Neumann M., Iyyer M., Gardner M., Clark C., Lee K., Zettlemoyer L. Proceedings of the 2018 Conference of the North American Chapter of the Association for Computational Linguistics: Human Language Technologies, Volume 1 (Long Papers) Association for Computational Linguistics; 2018. Deep contextualized word representations; pp. 2227–2237. [ CrossRef ] [ Google Scholar ]
- Raccuglia P., Elbert K.C., Adler P.D.F., Falk C., Wenny M.B., Mollo A., Zeller M., Friedler S.A., Schrier J., Norquist A.J. Machine-learning-assisted materials discovery using failed experiments. Nature. 2016; 533 :73–76. doi: 10.1038/nature17439. [ PubMed ] [ CrossRef ] [ Google Scholar ]
- Radford A., Wu J., Child R., Luan D., Amodei D., Sutskever I. Language models are unsupervised multitask learners. OpenAI Blog. 2019; 1 :9. [ Google Scholar ]
- Ramakrishnan C., Patnia A., Hovy E., Burns G. Layout-aware text extraction from full-text PDF of scientific articles. Source Code Biol. Med. 2012; 7 :7. doi: 10.1186/1751-0473-7-7. [ PMC free article ] [ PubMed ] [ CrossRef ] [ Google Scholar ]
- Ramprasad R., Batra R., Pilania G., Mannodi-Kanakkithodi A., Kim C. Machine learning in materials informatics: recent applications and prospects. Npj Comput. Mater. 2017; 3 :1–13. doi: 10.1038/s41524-017-0056-5. [ CrossRef ] [ Google Scholar ]
- Rasmy L., Xiang Y., Xie Z., Tao C., Zhi D. Med-BERT: pre-trained contextualized embeddings on large-scale structured electronic health records for disease prediction. arXiv. 2020 [ PMC free article ] [ PubMed ] [ Google Scholar ]
- Read J., Dridan R., Oepen S., Solberg L.J. Proceedings of COLING 2012: Posters. 2012. Sentence boundary detection: a long solved problem? pp. 985–994. [ Google Scholar ]
- Ricci F., Chen W., Aydemir U., Snyder G.J., Rignanese G.-M., Jain A., Hautier G. An ab initio electronic transport database for inorganic materials. Sci. Data. 2017; 4 :170085. doi: 10.1038/sdata.2017.85. [ PMC free article ] [ PubMed ] [ CrossRef ] [ Google Scholar ]
- Roberts G., Haile S.Y., Sainju R., Edwards D.J., Hutchinson B., Zhu Y. Deep learning for semantic segmentation of defects in advanced STEM images of steels. Sci. Rep. 2019; 9 :12744. doi: 10.1038/s41598-019-49105-0. [ PMC free article ] [ PubMed ] [ CrossRef ] [ Google Scholar ]
- Rocktäschel T., Weidlich M., Leser U. Chemspot: a hybrid system for chemical named entity recognition. Bioinformatics. 2012; 28 :1633–1640. doi: 10.1093/bioinformatics/bts183. [ PubMed ] [ CrossRef ] [ Google Scholar ]
- Shah S., Vora D., Gautham B.P., Reddy S. A relation aware search engine for materials science. Integr. Mater. Manuf. Innov. 2018; 7 :1–11. doi: 10.1007/s40192-017-0105-4. [ CrossRef ] [ Google Scholar ]
- Shannon C.E. Prediction and entropy of printed English. Bell Syst. Tech. J. 1951; 30 :50–64. doi: 10.1002/j.1538-7305.1951.tb01366.x. [ CrossRef ] [ Google Scholar ]
- Swain M.C., Cole J.M. ChemDataExtractor: a toolkit for automated extraction of chemical information from the scientific literature. J. Chem. Inf. Model. 2016; 56 :1894–1904. doi: 10.1021/acs.jcim.6b00207. [ PubMed ] [ CrossRef ] [ Google Scholar ]
- Szegedy C., Vanhoucke V., Ioffe S., Shlens J., Wojna Z. 2016 IEEE Conference on Computer Vision and Pattern Recognition (CVPR) IEEE Computer Society; 2016. Rethinking the inception architecture for computer vision; pp. 2818–2826. [ CrossRef ] [ Google Scholar ]
- Tchoua R.B., Qin J., Audus D.J., Chard K., Foster I.T., de Pablo J. Blending education and polymer science: semiautomated creation of a thermodynamic property database. J. Chem. Educ. 2016; 93 :1561–1568. doi: 10.1021/acs.jchemed.5b01032. [ PMC free article ] [ PubMed ] [ CrossRef ] [ Google Scholar ]
- Tchoua R.B., Ajith A., Hong Z., Ward L.T., Chard K., Belikov A., Audus D.J., Patel S., de Pablo J.J., Foster I.T. Creating training data for scientific named entity recognition with minimal human effort. In: Rodrigues J.M.F., Cardoso P.J.S., Monteiro J., Lam R., Krzhizhanovskaya V.V., Lees M.H., Dongarra J.J., Sloot P.M.A., editors. LNCS. Vol. 11536. Springer International Publishing; 2019. pp. 398–411. [ CrossRef ] [ Google Scholar ]
- Tehseen I., Tahir G.R., Shakeel K., Ali M. Corpus based machine translation for scientific text. In: Iliadis L., Maglogiannis I., Plagianakos V., editors. Artificial Intelligence Applications and Innovations. Springer International Publishing; 2018. pp. 196–206. [ CrossRef ] [ Google Scholar ]
- Thompson K. Programming Techniques: regular expression search algorithm. Commun. ACM. 1968; 11 :419–422. doi: 10.1145/363347.363387. [ CrossRef ] [ Google Scholar ]
- Tkaczyk D., Szostek P., Fedoryszak M., Dendek P.J., Bolikowski Ł. CERMINE: automatic extraction of structured metadata from scientific literature. Int. J. Document Anal. Recognition (Ijdar) 2015; 18 :317–335. doi: 10.1007/s10032-015-0249-8. [ CrossRef ] [ Google Scholar ]
- Tolle K.M., Tansley D.S.W., Hey A.J.G. Proceedings of the IEEE 99. 2011. The fourth paradigm: data-intensive scientific discovery [point of view] pp. 1334–1337. [ Google Scholar ]
- Trewartha A., Dagdelen J., Huo H., Cruse K., Wang Z., He T., Subramanian A., Fei Y., Justus B., Persson K., Ceder G. COVIDScholar: an automated COVID-19 research aggregation and analysis platform. arXiv. 2020 [ PMC free article ] [ PubMed ] [ Google Scholar ]
- Tshitoyan V., Dagdelen J., Weston L., Dunn A., Rong Z., Kononova O., A Persson K., Ceder G., Jain A. Unsupervised word embeddings capture latent knowledge from materials science literature. Nature. 2019; 571 :95–98. doi: 10.1038/s41586-019-1335-8. [ PubMed ] [ CrossRef ] [ Google Scholar ]
- Vaswani A., Shazeer N., Parmar N., Uszkoreit J., Jones L., Gomez A.N., Kaiser L., Polosukhin I. Attention is all you need. arXiv. 2017 [ Google Scholar ]
- Vaucher A.C., Zipoli F., Geluykens J., Nair V.H., Schwaller P., Laino T. Automated extraction of chemical synthesis actions from experimental procedures. Nat. Commun. 2020; 11 :3601. doi: 10.1038/s41467-020-17266-6. [ PMC free article ] [ PubMed ] [ CrossRef ] [ Google Scholar ]
- Weizenbaum J. Eliza – a computer program for the study of natural language communication between man and machine. Commun. ACM. 1983; 26 :23–28. doi: 10.1145/357980.357991. [ CrossRef ] [ Google Scholar ]
- Weston L., Tshitoyan V., Dagdelen J., Kononova O., Trewartha A., Persson K.A., Ceder G., Jain A. Named entity recognition and normalization applied to large-scale information extraction from the materials science literature. J. Chem. Inf. Model. 2019; 59 :3692–3702. [ PubMed ] [ Google Scholar ]
- Young S.R., Maksov A., Ziatdinov M., Cao Y., Burch M., Balachandran J., Li L., Somnath S., Patton R.M., Kalinin S.V. Data mining for better material synthesis: the case of pulsed laser deposition of complex oxides. J. Appl. Phys. 2018; 123 :115303. doi: 10.1063/1.5009942. [ CrossRef ] [ Google Scholar ]
- Zhai H., Lingren T., Deleger L., Li Q., Kaiser M., Stoutenborough L., Solti I. Web 2.0-based crowdsourcing for high-quality gold standard development in clinical natural language processing. J. Med. Internet Res. 2013; 15 :e73. doi: 10.2196/jmir.2426. [ PMC free article ] [ PubMed ] [ CrossRef ] [ Google Scholar ]
- Open access
- Published: 03 December 2022
Negative emotions experienced by healthcare staff following medication administration errors: a descriptive study using text-mining and content analysis of incident data
- Sanu Mahat 1 ,
- Anne Marie Rafferty 2 ,
- Katri Vehviläinen-Julkunen 3 , 4 &
- Marja Härkänen 1
BMC Health Services Research volume 22 , Article number: 1474 ( 2022 ) Cite this article
4482 Accesses
4 Citations
73 Altmetric
Metrics details
Medication errors regardless of the degree of patient harm can have a negative emotional impact on the healthcare staff involved. The potential for self-victimization of healthcare staff following medication errors can add to the moral distress of healthcare staff. The stigma associated with errors and their disclosure often haunts healthcare professionals, leading them to question their own professional competence. This paper investigates the negative emotions expressed by healthcare staff in their reported medication administration error incidents along with the immediate responses they received from their seniors and colleagues after the incident.
This is a retrospective study using a qualitative descriptive design and text mining. This study includes free-text descriptions of medication administration error incidents ( n = 72,390) reported to National Reporting & Learning System in 2016 from England and Wales. Text-mining by SAS text miner and content analysis was used to analyse the data.
Analysis of data led to the extraction of 93 initial codes and two categories i.e., 1) negative emotions expressed by healthcare staff which included 4 sub-categories of feelings: (i) fear; (ii) disturbed; (iii) sadness; (iv) guilt and 2) Immediate response from seniors and colleagues which included 2 sub-categories: (i) Reassurance and support and (ii) Guidance on what to do after an error.
Negative emotions expressed by healthcare staff when reporting medication errors could be a catalyst for learning and system change. However, negative emotions when internalized as fear, guilt, or self-blame, could have a negative impact on the mental health of individuals concerned, reporting culture, and opportunities for learning from the error. Findings from this study, hence, call for future research to investigate the impact of negative emotions on healthcare staff well-being and identify ways to mitigate these in practice.
Peer Review reports
Medication Errors (MEs) are recognized by the World Health Organization as the leading cause of injury and avoidable harm in healthcare, costing approximately 42 billion dollars annually, which is nearly 1% of total global health expenditure [ 1 ]. The safety of patients is at the forefront of the healthcare system; however, healthcare staff can also be traumatized by the aftermath of MEs. Although the healthcare mantra is “first do no harm”, healthcare professionals involved in adverse events can feel guilt, shame, anger, fear, and anxiety [ 2 ]. They are often neglected with only a few coping strategies and support systems available to help them [ 3 ]. Negative consequences of an adverse event can reach far beyond the “first victim” i.e., the patient. Thus, affecting healthcare staff psychologically making them “second victims” [ 4 ]. The term “second victim” was first coined by Dr. Albert Wu to explain the emotions of a young resident who committed an error and had experienced ridicule, shame, and lack of support, from his peers [ 2 ]. Although this concept was first applied to physicians, other healthcare staff, including nurses, also experience similar emotions. Scott et al. [ 5 ] described the term second victim as “a healthcare provider involved in an unanticipated adverse patient event, medical error and/or a patient-related injury who has become victimized in the sense that the provider is traumatized by the event. Frequently, second victims feel personally responsible for the unexpected patient outcomes and experience as though they have failed their patient, feeling doubts about their clinical skills and knowledge base”[ 5 ].
The use of the term second victim has been criticized recently [ 6 , 7 ] arguing that it might act as a way in which healthcare providers can evade responsibility and accountability and it might be offensive to affected patients and families [ 6 ]. Laying accountability at the door of an individual, ignoring the wider organizational ramifications of accountability in terms of the conditions which trigger errors in the first place, can let the organization off the hook. Even though the use of the term “victim” may sound spurious and uncomfortable to many healthcare professionals, patients, and families, it is indubitably an advantage in reinforcing the seriousness and urgency of the problem among policymakers and healthcare managers [ 8 ]. Wu et al.[ 8 ] have suggested the importance of the use of the term second victim as it is notable and denotes urgency. These assumptions regarding the use of the term second victim are inherent in both positions. Therefore, our research is designed to take this debate one step further by analyzing the consequences of errors in terms of emotional response and lived experiences of healthcare staff.
Regardless of the degree of patient harm, the mere thought of potential patient injury caused by ME is sufficient to induce the feelings of fear, distress, anger, anxiety, guilt and remorse in healthcare staff [ 9 , 10 , 11 ]. Although evidence suggests multiple system-based causes of MEs, the error-maker still tends to blame themselves i.e., they should have functioned proficiently [ 11 ]. If the seriousness of these issues remains unaddressed, it can negatively affect healthcare workers’ personal and professional well-being causing depression, burnout, Post Traumatic Stress Disorder (PTSD), and even suicidal thoughts [ 4 , 12 , 13 ]. Error prevention has therefore been a focus of major attention for healthcare organizations for years but the impact of MEs on the healthcare professional involved has received less attention. A more nuanced and textured exploration of the impact of the problem upon healthcare workers is required if preventative strategies are to be effective [ 11 ].
Previous studies have shown that often MEs causing harm are reported whereas near misses are often under-reported [ 14 ]. This underestimates the number of healthcare staff going through negative experiences [ 15 ]. Fear of legal consequences, blame, losing patients’ trust, and punishment have been recognized as barriers to ME reporting[ 16 ] leading healthcare staff to suffer in silence, sometimes struggling alone in isolation and burdened with a sense of shame [ 9 ]. Therefore, a system is needed to mitigate these barriers and create a “just culture guide” which helps healthcare managers to treat staff involved in adverse events fairly, support open and fair culture and maximize learning from errors [ 17 ]. However, it is apparent that irrespective of organizational effort in promoting a just and no-blame culture, the stigma persists with respect to speaking up about errors [ 18 ].
Patient safety incident reporting has become a common practice, but little is known about the feelings of those who commit or witness incidents. Despite the recent debate regarding the use of the term second victim, we are adopting this terminology throughout our research to analyse the consequences of MEs in terms of psychological responses from healthcare staff. Previous research into second victims has mainly been carried out in a single setting, but this study uses reported incidents at a national level drawing from a range of settings. Also, no previous studies, as far as we are aware, have focused only on Medication Administration Errors (MAEs). To our knowledge, none of these studies have used free-text descriptions of reported medication incidents to review the feelings and emotional responses associated with reporting nor text mining as an innovative method for such analysis.
The aim of this study was to investigate negative emotions expressed by healthcare staff in their reported MAE incidents along with the immediate responses they received from their seniors and colleagues after the incident.
Study design and setting
A retrospective study using qualitative descriptive method and text-mining with an inductive content analysis of the incident data related to Medication Administration (MA) reported in England and Wales was done.
Description of the data
The data consists of MA incidents ( n = 72,390) retrieved from the National reporting and Learning System (NRLS) database based on inclusion criteria: (1) incidents reported to have occurred in England and Wales between 1 January and 31 December 2016, (2) medication incident, (3) administration/supply of medicine from a clinical area, and (4) acute National Health Services (NHS) trust (either specialist or non-specialist). The data included incident reports from all levels of healthcare staff ranging from student nurses to senior-level health professionals who were involved in and who have witnessed the MAE incidents.
Data were acquired from NHS England and NHS Improvement. NRLS is largely voluntary and is the only database that includes all types of patient safety incidents. This study used free-text descriptions of the incidents i.e., healthcare staffs’ descriptions of “what has happened?” or “when the incident occurred?” during the medication process.
Data analysis
First, negative emotional expressions associated with MAEs were defined using the literature and dictionaries (Oxford Learners’ Dictionary, Merriam-Websters’ Dictionary, and Cambridge Dictionary) to define synonyms of the negative emotional expressions (Table 1 ). Second, those expressions were searched from the free-text descriptions of the incidents which were specifically related to MA. For that, The SAS® Enterprise Miner 13.2 and its Text Miner tool were used. Multiple steps were followed for data analysis as described in Fig. 1 . SAS® Text Miner automatically processes the data using ‘text parsing’ i.e., converting unstructured text into a structured form. Text parsing includes tokenization (breaking text into words/terms), stemming (which chops off the end of words reducing words to their stem or root forms), and part-of text tagging (for each word, the algorithm decides whether it is a noun, verb, adjective, adverb, preposition and so on). ‘Text filtering’ was then used to reduce the total number of parsed terms and check the spellings. The English language was chosen for parsing and filtering the text. Using an interactive filter viewer, negative emotional expressions described in the free text were identified and the number of each expression was collected (See Supplementary file 1 ). For the next phase of the analysis, the most common expressions were chosen which are bolded in online-only material 2 (See Supplementary file 2 ).
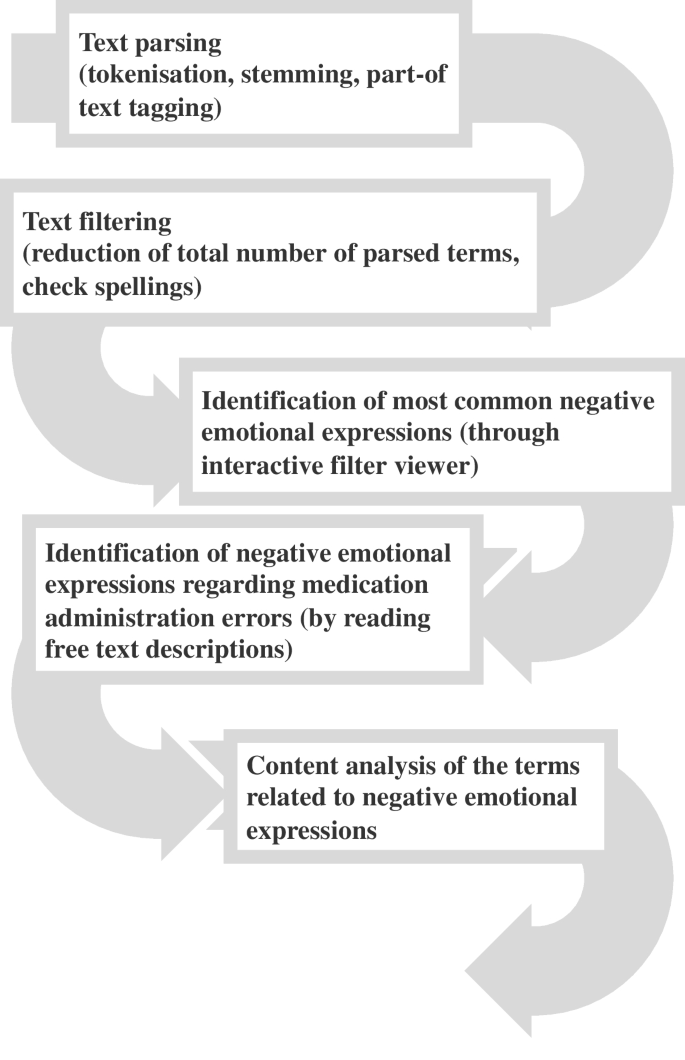
Analysis process of medication administration incident reports’ free text descriptions
Expressions chosen for analysis were used as a search term in an interactive filter viewer. All the descriptions of the incidents that included those expressions (a total of 1861 incident reports) were collected and read through repeatedly. In the first phase of this analysis, the aim was to define who had experienced the emotional feeling. Most of negative emotions were expressed by patients or relatives (See Supplementary file 1 ). Those descriptions of incidents that included negative emotions expressed by healthcare staff and which were expressed in relation to MAEs ( n = 93) were then selected for further analysis.
Content analysis was used to analyze the data. The lead author followed an inductive content analysis where the researchers carefully read, organized, and integrated and formed categories, concepts, and themes by comparing the similarities and differences between the coded data [ 19 ]. The lead author read through the data repeatedly and during this process, identified the main theme which is: Emotional expressions of healthcare staff after MAEs. The data were organized into main themes and sub-themes. After the preliminary classification, a co-coder [the last author of this paper] participated in the analysis and read the classification structure and the related data independently. Once thematic saturation was achieved, both researchers analyzed the entire data corpus according to standard thematic analysis techniques [ 20 ]. All authors contributed to the final form of the analysis. Finally, direct quotes were used to support the findings.
Negative emotional expressions of healthcare staff after MAEs
We found 15 different types of negative emotional expressions used including worry, anxiety, annoyance, agitation, stress, unhappiness, distress, concern, anger, upset, shock, sorry, fault, depression, and frustration. These 15 different types of emotions were expressed 1,861 times in the incident reports (See Supplementary file 1 ).
Among those emotional expressions, 12 were exhibited by the healthcare staff and were mentioned 154 times. Only eight of those 12 expressions: worry, upset, agitation, faulty, sorry, concerned, stressed, and distress were expressed by healthcare staff in direct relation to MAEs, the frequency of expression here was 93 times. The data extraction process in presented as a flowchart in Fig. 2 .
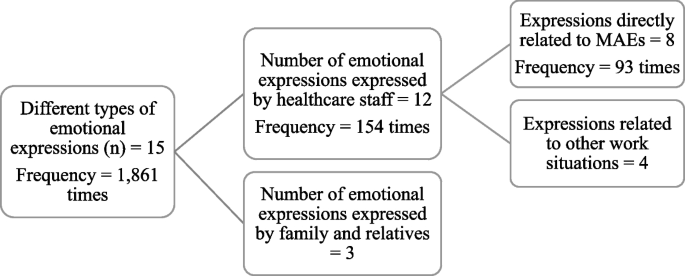
Typology and frequency of emotional expressions
The key emotions revealed were further classified into four categories: (1) feeling of fear, (2) feeling of upset, (3) feeling of sadness, and (4) feeling of guilt (Table 2 ).
Feeling of fear
Healthcare staff described their feeling of fear regarding MAEs using four different synonyms i.e., distressed, concerned, stressed, and worried. Staff mentioned how fearful they were when they discovered their mistakes. Distress was revealed in three of the incident reports as expressions of fear of healthcare staff. Usually, MAE incidents were reported either by the error-makers themselves or by those witnessing their errors. One of the staff described the fear felt by her colleague (staff nurse) by reporting how distressed he was after he administered a medication through wrong route (intravenous instead of oral):
“ I was assessing a patient on Ward X when a staff nurse approached me extremely distressed and agitated. He then ran into the utility without explaining what the problem was. I followed him…nurses were present who proceeded to explain that the nurse who approached me had given a patient 2mls of Oramorph [liquid morphine that has to be given orally] intravenously …"
Healthcare staff also expressed the extreme pressure which acted as an important contextual trigger, driving intense the feelings of fear. Another emotion linked to fear was “concerned” which was expressed in 23 cases by healthcare staff after making an error. One of the healthcare staff reported an error (prescribed wrong strength), which the staff realized two hours later and became concerned about it:
" Prescribed TTA (to take away) of ‘Augmentin [Amoxicillin Clavulanate] Duo’125/31 8 ml TDS [three times a day]. As written, this would be a drug error-there is no 125/31 strength of …This was my error, which I realized and became concerned about 2 hours later …"
Stress was expressed in three cases by healthcare staff while reporting the incident; however, this emotion was expressed by staff not as their feelings after MAEs, but as the reason underlying MAEs. These kinds of explanations were found in many incident reports where healthcare staff accepted the error but eventually pointed towards other hidden causes behind the error:
" Gave Clexane [Enoxaparin] 60 mg to wrong patient. Ward extremely busy- heavy workload and was very stressed due to workload …"
Being “worried” was another expression of fear reported in 11 incident reports by healthcare staff. They were found to be worried about several situations such as the health of patient, degree of harm caused by error, associated legal procedures, and their professional career. One staff nurse was worried about the patients’ condition as he did not administer insulin dosage to one of his patients:
" Staff nurse came to me at the end of the shift and stated that he thought that the patients’ insulin was prescribed prn [whenever necessary] and had not given any…I explained he needed to inform the nurse in charge…he was very sincere and worried that he had not given this insulin …"
Feeling disturbed
The feeling of being disturbed was expressed using two synonyms: upset and agitated. They addressed themselves as being upset in 24 incident reports following MAEs committed either by themselves or by their fellow staff. Healthcare staff reported the error made by fellow staff member and described the emotion of his/her colleague as:
" Nurse called me was very upset to explain that she had given wrong treatment to patient …"
Even near miss situations have caused healthcare staff to get emotionally disturbed. Even after apologizing with patient and family, healthcare staff felt upset thinking that if they were not aware of the near miss situation in time, patients’ condition would have been severe:
" SN asked me to do a syringe driver with her for a palliative patient…on drawing up the ketamine driver, myself and SN made a drug error in which we drew 5 times more ketamine than the required dose…The family and patient have been informed of the drug error we made and we gave our sincere apology for our faults…both myself and SN are very upset with the near miss situation and aware that things could have gone very differently …"
Healthcare staff expressed being agitated in two reports after discovering that they had committed MAEs, except in some situations, where staff though agitated denied their mistake by underestimating the severity of the error they made:
" Patient was discharged off the system by the nurse without confirming with medical team/pharmacy that patient was ready to go… Patient left without anti-sickness medication which the team had told her she could have…Nurse was evidently agitated that the incident was being reported and did not understand that she should check with the team before authorizing …"
Some reports revealed extreme negative emotions associated with feelings of upset such as being devastated and questioning one’s own professional competence. The use of such intense and traumatic language can reflect how much the healthcare staff were impacted and even emotionally wrecked after MAE. One healthcare staff after accidentally administering wrong dosage to the patient, reported that the error was entirely his/her own fault:
" Pt px 120 mg on gentamicin on EOMA, I accidentally gave 210 mg in error. This was entirely my fault …The checker confirmed what I had done. I am so devastated about this and really upset I’d made such a mistake…today was just hectic and I lost concentration ..."
Feeling of sadness
Healthcare staff expressed their feeling of sadness at being sorry for the mistake they had made; it was one of the most common negative emotional expressions expressed in 13 cases. Most staff used this to express a sense of remorse after the error. After missing a dose of insulin for a patient, one healthcare staff expressed his/her sadness by stating that he/she is sorry about the incident:
" I am sorry to say that I missed one dose of insulin (at 22.30…) for one of my patients …"
Along with the feeling of sadness, one healthcare staff also mentioned about learning from the error and how he/she have accepted that she was wrong to assume things:
" I was sitting at the desk, staff nurse handed me a tray with intravenous antibiotics and said, here is one because I had given her patient drug chart, I assume it was patients’ medication. I did not take the drug chart with me to the patient and afterwards when staff nurse came with patients’ drug, I realized I have given the wrong drug. I was very upset as I have never done anything in this form before. I always take the drug chart with me to the patient. I am deeply sorry, and this is a massive learning curve for me, I hold my hand up it was wrong to assume this ."
Healthcare staff who had mentioned learning from the error was quite common in many incident reports. However, there were few cases where the staff did not understand the seriousness of the error she has caused:
"… I spoke to the student nurse about the seriousness of her actions, she said sorry; however, I did not feel she understood the seriousness of what she did …"
Feeling of guilt
In 14 incident reporting cases, healthcare staff were aware of their mistakes and the consequences they might have. They expressed their guilt and identified themselves as being at fault and blaming themselves.
" IV flucloxacillin drawn up and checked by myself and staff nurse…administered drug however in error name band/ allergy band not checked. Realized immediately after administration that I had gone to the wrong patient and given the incorrect medication…conversation with senior staff nurse about error. Explained that the error was my fault completely…patient does not appear to have come to any harm …"
However, this emotion was not just expressed following the error, but also as another reason for error attribution. For example, in the report below, a staff member made an error, and blamed herself and phone reception for being muffled:
" I had to hand over two diabetic patients to the 5–8 pm. I rang Ward sister and confirmed this again later. However, patient was not reallocated, and insulin omitted…Ward sister apologized for yesterday missed patient…she said the reception to her phone was muffled and that it was her fault …"
Immediate response from seniors and colleagues
Some of the healthcare staff while reporting their feelings behind MAE incidents also discussed regarding the immediate responses they received from their seniors and colleagues. Healthcare staff explained how their seniors and colleagues responded after they were informed about MAEs. These responses are categorized into two sub-categories: (1) Reassurance and support and (2) Guidance on what to do after an error.
Reassurance and support
In three incident reports, healthcare staff mentioned about the reassurance and positive support they received from their seniors and colleagues after the disclosure of MAEs, about how they tried to handle the situation very calmly without getting angry. This helped them to cope effectively without undue stress and burden. A nurse mentioned that she reassured one of her colleagues who was very disturbed after she gave the wrong medication to her patient:
" Staff nurse by mistake gave the patient wrong medication…. misread the information by being interrupted by a patient and member of staff…. I reassured the staff nurse as she was very upset …"
Even a little support and reassurance and few kind words during the time of MAEs can help the healthcare staff to cope up with the situation effectively. As one member remarked:
" Medication error – digoxin prescribed in two doses (125mcg and 62.5mcg) did not realize and administered…Immediately alerted sister in-charge of ward and contacted doctor. Doctor did not come to the ward but was happy that observations had been recorded…and told us not to worry …"
Guidance on what to do after error
In 11 incident reports, healthcare staff mentioned about receiving advice from their seniors and colleagues regarding the right thing to do after making an error. They have been guided to observe the situation of the patient to ensure that no serious harm would be caused to them:
"… Administered the oramorph in an unlabeled syringe which was in the same tray as a 10ml flush…I discussed the situation with the medical registrar on call who advised me to monitor observations regularly …" "… I spoke to the nurse in charge after the error from the following shift who said that I should speak to the ward manager at the earliest opportunity which I did …"
Furthermore, in cases where healthcare staff neglected to document the incident, a colleague intervened to guide the staff member to follow the protocol. As one staff member described:
"… I discussed the incident with a colleague shortly afterwards. However, I neglected to escalate and correctly document the incident…The aforementioned colleague has since approached me to discuss the incident, further to this I approached and discussed the incident with my ward manager …"
Our study identified four categories of negative emotions expressed in incident reports: feelings of fear, disturbed, sadness, and guilt with various sub-categories. In addition, this study also captured the immediate responses received by healthcare staff after they informed their seniors and colleagues about MAEs including the reassurance, support, and guidance on what to do after an error. Incident reporting by healthcare staff in this study indicated that unintentional harm caused due to MAEs and even near misses can affect the healthcare staff involved in error emotionally, increasing their risk of becoming the second victim of MAEs, confirming previous research [ 9 , 21 ].
A major finding of this study was the negative emotions experienced by healthcare staff after MAEs. Healthcare staff in this study expressed their fear while reporting incidents by using negative emotions such as stressed, distressed, concerned, and worried. They not only blamed themselves for these mistakes, but also considered other additional explanations which, they perceived as causing the error. These kinds of emotions can be related to staff members’ narration of fear and anxiety for patients’ well-being and for their own professional careers [ 22 ]. Similarly, feelings of being disturbed expressed as being upset and agitated were widely mentioned in incident reports. Identical reasons such as realization of the error and thoughts of the possible seriousness of the error and associated issues lay behind emotions. Further, feeling of sadness expressed as being sorry for the mistake made was another most common emotional expression. Also, healthcare staff felt a deep burden of responsibility for their actions. Feelings of being guilty or at fault is one of the risk factors for healthcare staff for becoming the second victim of MEs. It can also cause loss of self-esteem and inculcate a sense of failure and hopelessness. In a similar study by Treiber & Jones [ 22 ], nurses, upon committing even minor errors, expressed raw and painful emotions, regardless of the degree of harm. Nurses can often recall the details of the error and what they felt at that time [ 22 ]. While the lack of any apparent linkage between emotional response and degree of patient harm might appear counter intuitive, one possible explanation might be that healthcare professionals are not well enough supported by their organizations to cope with any form of negative experience. Thus, those affected might develop strong negative emotion [ 23 ].
Making an error might also have serious consequences for disrupting the personal and professional lives of staff, causing personal and moral distress, and affecting the quality and safety of patient care [ 23 ]. It is crucial to pay attention to these emotional expressions as incidents that are sensitive and make an impact, are often remembered, and reflected in the attempt to prevent recurrence. On the other hand, these incidents can unintentionally impose a mental burden on healthcare staff making them second victim [ 2 ]. Our findings confirms that MAEs can generate negative feelings in healthcare staff associated with it, which can endure long beyond the immediate effect.
Research has confirmed a direct relationship between nurse staffing and missed patient care [ 24 , 25 ], revealing poor nurse staffing as a risk factor for MEs along with other organizational factors such as poor working conditions, distractions, and high workload [ 26 ]. Similarly, in this study, reporters mentioned their own actions as a trigger for MAEs along with the above-mentioned factors whereas some reporters explained organizational and environmental conditions and context surrounding the error as reasons to reduce blame. In the absence of support, self-blame seems to assume greater prominence. This can have long-term repercussions for maintaining emotional health and well-being, a major failure of workforce strategy, especially during the pandemic situations.
The current study also found other healthcare authorities responding in several ways after being informed about MAEs. Sometimes, staff may not know what to do after MEs, they might panic and lose control. Thus, adequate support from colleagues and seniors sensitive to these issues may prevent the error-makers from translating further into second victimhood of MEs. How the organization and related individuals responds is clearly linked to the emotional impact the error can have on the healthcare staff who made the error. Appropriate support and guidance from seniors and colleagues have been found to alleviate the suffering, while lack of support has increased their psychological burden [ 27 ]. Some of the healthcare professionals in our study also opted for consulting with their seniors: doctors, colleagues, and mentors after MAEs and reported about how they have received guidance and suggestions, which helped them to cope effectively. Emotional support plays a vital role in restoring faith and confidence among healthcare professionals in patient safety. Support from co-workers and healthcare institution helps the error-makers to retain a sense of control [ 2 ]. Reassurance from seniors and colleagues can also strengthen healthcare staff’s self-esteem and facilitate the correct reporting of MAEs. As is well known, only a fraction of incidents are reported thus deterring the improvement of patient safety with barriers identified as time pressure, fear of the consequences [ 28 ], poor institutional support, lack of feedback, a blame culture, and inadequate training [ 15 ]. Yet, we can still improve patient safety by identifying these barriers. Moreover, while some staff members perhaps too readily assumed responsibility for errors, as reflected in the prominence of self-blame, others demonstrated reluctance, which could be linked to fear of the consequences of MAEs. Furthermore, little is known about the dynamics and consequences of reporting-what prompts some to report and others not to do so. We demonstrate that the emotional expression of staff can be extremely distressing and negatively impact health and well-being of healthcare staff.
Implications for practice
Our findings indicate that immediate negative feelings experienced by healthcare staff after making MAEs can have long-lasting impacts that stretch far beyond the event itself thus potentially traumatizing them and inducing ruminative thoughts, which trigger the memory. The short, medium, and long-term consequences of errors are unknown as yet but could contribute to burnout and other factors associated with intention to leave the profession. Indeed, a negative memory that will stay with them forever, if not handled accurately. They could potentially become second victims of an error, if unable to confront and deal with negative feelings associated with the error. One source of challenge could be stigma related to this making it difficult to continue to work after MAE. Our findings suggest appropriate guidance and support from fellow staff members could help healthcare staff to handle the situation effectively. Therefore, it should be paramount to tailor appropriate support from persons in-charge and colleagues and to promote an open culture where it is understood. Errors can impair mental health of those who are involved, hence, the system triggers surrounding such errors need to be understood and prevented. In addition, more detailed information about these emotions after incidents and their long-term consequences on emotional well-being should be studied in future.
Implications for research
The negative feelings expressed by healthcare staff after MAEs identified in this study could provide the basis for designing an intervention study to support emotionally affected staff in healthcare institutions. It could be helpful to design a support program which recognizes the importance of expressed emotion and its consequences for internationalizing a sense of self blame and victimhood and the long-term repercussions this might have for the mental health and well-being of the health workforce.
Strengths and Limitations
As far as we are aware, this is the first-time text-mining and content analysis have been used to identify negative emotions reported by healthcare staffs’ MAEs, derived from free text in a large national database. A text-mining approach was used for identifying reports that included emotional expressions, as manual data analysis would have been almost impossible for such a big data set and this approach has been recognized to be time-effective in analysing big-data regarding medication incidents (Härkänen et al., 2019). Further, the emotional expressions identified in this study are relatively rare. These descriptive data of emotional expressions nevertheless cast light on the issues related to MAEs. Furthermore, the researchers adhered to the Standards for Reporting Qualitative Research (SRQR) checklist (see the list in Supplementary file 3 ).
However, while analyzing the free-text descriptions, we may have missed some important expressions as this was a pilot methodology we were testing, subjective decisions were made. Similarly, it was very difficult to combine the synonyms of the word used to express the negative emotions which can give rise to ambiguities. For example, in many cases, one single word could either be a verb, or noun or an adjective i.e., words can have different implication [ 29 ]. On the contrary, this study sheds some light upon how important it is to write incident report and to identify the negative emotions of staff, to prevent further consequences from occurring, encourage reporting and put support mechanisms in place. Patient safety incident data is likely to contain some limitations, more specifically, reporting error and bias which will affect the number, type and temporality of reported incidents and data interpretation [ 30 ]. Since reporting is largely voluntary, there are some potential limitations of NRLS being a reliable indicator of exact number of incidents. Nevertheless, increasing number of incidents may reflect an improved reporting culture. Further, the methodology did not allow for the identification of any positive emotions that might have been expressed by healthcare staff when reporting MAE incidents, as only free-text descriptions which included negative emotions were analyzed .From the free text-descriptions, most of the reports were found to be from nurses, however, staff-specific generalizability and scope is limited due to lack of staff type identification in NRLS data i.e., ST01 [ 31 ]. This makes it difficult to precisely quantify the impact and potential benefits of this research.
A wide range of negative emotions was expressed by healthcare staff after reported MA incidents. However, the associated psychological trauma and low mood expressed by healthcare staff represent significant negative impacts underlying reported negative emotions. It is more likely that MAE incidents are under-reported, therefore problems could be much higher in terms of prevalence and magnitude. There was tremendous variation in reports of healthcare staff encountering with MAEs; some reacted in extremely negative ways, whereas the majority expressed little about their feelings. Although many of the incident reporters did not express their feelings in their reports, there is also the possibility of them being affected by the aftermath of MAEs. Several actions were taken by healthcare staff to help cope with the error: which included, seeking guidance, reassuring, and supporting each other. This calls for further efforts from healthcare organizations to support healthcare staff as a matter of routine when encouraging reporting. Though we do know little about the long-term consequences, from what we see in our data, the scarring effect could potentially be considerable. Therefore, support programs need to be co-designed but incentivize to reward reporting without imposing an emotional burden on already overburdened staff. This is vital for error reporting, safety, and ultimately prevention to flourish in the long run. First and foremost, the system needs to promote psychological safety for its users, which our research currently demonstrates.
Availability of data and materials
Data supporting the findings of this study are made available from NRLS/NHS Improvement. However, restrictions apply to the availability of these data. For this current study, these data were used under license, therefore, are not publicly available. Data are however available if contacted to authors (MH, AMR, SM) upon reasonable request and with permission from NRLS/NHS Improvement.
Abbreviations
Medication Error
Medication Administration Error
Medication Administration
National Reporting and Learning System
National Health Services
Standards for Reporting Qualitative Research
World Health Organization. WHO launches global effort to halve medication-related errors in 5 years [Internet]. 2017 [Cited 2021 Jun 21]. Available from: https://www.who.int/news/item/29-03-2017-who-launches-global-effort-to-halve-medication-related-errors-in-5-years .
Wu AW. Medical error: The second victim. BMJ. 2000;18(7237):726–7. 320.
Article Google Scholar
Wu AW, Steckelberg RC. Medical error, incident investigation and the second victim: Doing better but feeling worse? BMJ Qual Saf. 2012;21(4):267–70.
Article PubMed Google Scholar
Busch IM, Moretti F, Purgato M, Barbui C, Wu AW, Rimondini M. Dealing with Adverse Events: A Meta-analysis on Second Victims’ Coping Strategies. J Patient Saf. 2020;16(2):E51–60.
Scott SD, Hirschinger LE, Cox KR, McCoig M, Hahn-Cover K, Epperly KM, et al. Caring for our own: Deploying a systemwide second victim rapid response team. Jt Comm J Qual Patient Saf. 2010 May;36(5)(1):233–40.
PubMed Google Scholar
Clarkson MD, Haskell H, Hemmelgarn C, Skolnik PJ. Abandon the term “second victim.” BMJ. 2019;27(364):l1233.
Tumelty ME. The second victim: A contested term? J Patient Saf. 2021;17(8):E1488–93.
Wu AW, Shapiro J, Harrison R, Scott SD, Connors C, Kenney L, et al. The Impact of Adverse Events on Clinicians: What’s in a Name? J Patient Saf. 2020;16(1):65–72.
Article PubMed CAS Google Scholar
Seys D, Wu AW, Gerven E, Van, Vleugels A, Euwema M, Panella M, et al. Health Care Professionals as Second Victims after Adverse Events: A Systematic Review. Eval Heal Prof. 2013;36(2):135–62.
Helo S, Moulton CE. Complications: acknowledging, managing, and coping with human error. Transl Androl Urol. 2017;6(6):773–82.
Article PubMed PubMed Central Google Scholar
Jones JH, Treiber LA. More Than 1 Million Potential Second Victims: How Many Could Nursing Education Prevent? Nurse Educ. 2018;43(3):154–7.
Headley M. Are second victims getting the help they need [Internet]. Vol. 15, Patient Safety & Quality Healthcare. 2018 [Cited 2022 May 17]. p. 12–6. Available from: https://www.psqh.com/analysis/are-second-victims-getting-the-help-they-need/ .
Stehman CR, Testo Z. Burnout DO. Suicide : Physician Loss in Emergency Medicine, Part I. West J Emerg Med. 2019;20:485–94.
Cottell M, Wätterbjörk I, Hälleberg Nyman M. Medication-related incidents at 19 hospitals: A retrospective register study using incident reports. Nurs Open. 2020;7(5):1526–35.
Hartnell N, MacKinnon N, Sketris I, Fleming M. Identifying, understanding and overcoming barriers to medication error reporting in hospitals: A focus group study. BMJ Qual Saf. 2012 May;21(5):361–8.
Mahdaviazad H, Askarian M, Kardeh B. Medical Error Reporting: Status Quo and Perceived Barriers in an Orthopedic Center in Iran. Int J Prev Med. 2020;11:14. https://doi.org/10.4103/ijpvm.IJPVM_235_18 .
NHS England. NHS Just Culture Guide [Internet]. 2018 [Cited 2022 May 17]. Available from: https://www.england.nhs.uk/patient-safety/a-just-culture-guide/ .
Edrees HH, Wu AW. Does One Size Fit All? Assessing the Need for Organizational Second Victim Support Programs. J Patient Saf. 2021;17(3):e247–54.
Kyngäs H. Inductive content analysis. In: The application of content analysis in nursing science research. New York (NY): Springer, Cham; 2020. p. 13–21. https://doi.org/10.1007/978-3-030-30199-6_2 .
Saunders B, Sim J, Tom K, Baker S, Waterfield J, Bartlam B, et al. Saturation in qualitative research: exploring its conceptualization and operationalization. Qual Quant. 2018;52(4):1893–907.
Ullström S, Sachs MA, Hansson J, Øvretveit J, Brommels M. Suffering in silence: A qualitative study of second victims of adverse events. BMJ Qual Saf. 2014;23(4):325–31.
Treiber LA, Jones JH. Devastatingly human: An analysis of registered nurses’ medication error accounts. Qual Health Res. 2010;20(10):1327–42.
Harrison R, Lawton R, Perlo J, Gardner P, Armitage G, Shapiro J. Emotion and Coping in the Aftermath of Medical Error: A Cross-Country Exploration. J Patient Saf. 2015;11(1):28–35.
Ball JE, Griffiths P, Rafferty AM, Lindqvist R, Murrells T, Tishelman C. A cross-sectional study of ‘care left undone’ on nursing shifts in hospitals. J Adv Nurs. 2016;72(9):2086–97.
Griffiths P, Recio-Saucedo A, Dall’Ora C, Briggs J, Maruotti A, Meredith P, et al. The association between nurse staffing and omissions in nursing care: A systematic review. J Adv Nurs. 2018;74:1474–87.
Sessions LC, Nemeth LS, Catchpole K, Kelechi TJ. Nurses’ perceptions of high-alert medication administration safety: A qualitative descriptive study. J Adv Nurs. 2019;75(12):3654–67.
Lee W, Pyo J, Jang SG, Choi JE, Ock M. Experiences and responses of second victims of patient safety incidents in Korea: A qualitative study. BMC Health Serv Res. 2019;19(1):1–12.
Mahajan RP. Critical incident reporting and learning. Br J Anaesth [Internet]. 2010;105(1):69–75. Available from: https://doi.org/10.1093/bja/aeq133 .
Härkänen M, Paananen J, Murrells T, Rafferty AM, Franklin BD. Identifying risks areas related to medication administrations - Text mining analysis using free-text descriptions of incident reports. BMC Health Serv Res. 2019;19(1):1–9.
NHS Improvement. NRLS official statistics publications: data quality statement [Internet]. 2018. Available from: https://improvement.nhs.uk/documents/2549/NRLS_Guidance_notes_March_2018.pdf .
NHS England. Patient Safety Alert: improving medication error incident reporting and learning (supporting information) [Internet]. Patient Safety Alert: Stage 3 (directive). 2014. Available from: https://www.england.nhs.uk/2014/03/improving-medication-error-incident-reporting-and-learning/ .
Download references
Acknowledgements
The authors want to thank the NHS England and the NHS Improvement Patient safety team for helping the authors through the data acquisition process and refining the data extraction.
This study has been partially supported from the grant received from Sairaanhoitajien Koulultussäätiö and from early-stage researcher position from the University of Eastern Finland for the first author.
Author information
Authors and affiliations.
Department of Nursing Science, University of Eastern Finland, Yliopistonranta 1c, Kuopio, Finland
Sanu Mahat & Marja Härkänen
King’s College London: Florence Nightingale Faculty of Nursing, Midwifery and Palliative Care, James Clerk Maxwell Building, 57 Waterloo Road, SE1 8WA, London, UK
Anne Marie Rafferty
Department of Nursing Science, University of Eastern Finland, Kuopio, Yliopistonranta 1, 70210, Finland
Katri Vehviläinen-Julkunen
Kuopio University Hospital, Puijonlaaksontie 2, 70210, Kuopio, Finland
You can also search for this author in PubMed Google Scholar
Contributions
SM conducted the analysis, but all authors (SM, AMR, KV-J, and MH) participated in interpretation of data and in drafting and revising the manuscript critically and gave final approval of the version to be submitted.
Corresponding author
Correspondence to Sanu Mahat .
Ethics declarations
Ethics approval and consent to participate.
Data sharing agreement (Ref: 063.DSA.17) between NHS Improvement and King’s College London dated 22.08.2019 allowed us to use this data. As the data used for this study were voluntarily and anonymously submitted incident reports data (a register study), the need for seeking informed consent from the incident reporters was waived from the ethics committee. The King’s College London ethics committee (LRS-17/18-5150) gave ethical approval for this study in October 2017. Incident data used for this study did not comprise any personal or professional identifiers. Therefore, the anonymity and confidentiality of the data and the persons involved were fully ensured. Further, data handling was made confidential and ethical guidelines were followed.
Consent for publication
Not applicable.
Competing interests
No competing interests have been declared by authors.
Additional information
Publisher’s note.
Springer Nature remains neutral with regard to jurisdictional claims in published maps and institutional affiliations.
Supplementary Information
Additional file 1: supplementary file 1..
Number of incident reports with negative emotional expressions and description about the healthcare staffs’ feeling. Supplementary file 2. Number of negative emotional expressions related specifically to medication administration incident reports ( n =72,390). Supplementary file 3. SRQR checklist for reporting qualitative studies.
Rights and permissions
Open Access This article is licensed under a Creative Commons Attribution 4.0 International License, which permits use, sharing, adaptation, distribution and reproduction in any medium or format, as long as you give appropriate credit to the original author(s) and the source, provide a link to the Creative Commons licence, and indicate if changes were made. The images or other third party material in this article are included in the article's Creative Commons licence, unless indicated otherwise in a credit line to the material. If material is not included in the article's Creative Commons licence and your intended use is not permitted by statutory regulation or exceeds the permitted use, you will need to obtain permission directly from the copyright holder. To view a copy of this licence, visit http://creativecommons.org/licenses/by/4.0/ . The Creative Commons Public Domain Dedication waiver ( http://creativecommons.org/publicdomain/zero/1.0/ ) applies to the data made available in this article, unless otherwise stated in a credit line to the data.
Reprints and permissions
About this article
Cite this article.
Mahat, S., Rafferty, A.M., Vehviläinen-Julkunen, K. et al. Negative emotions experienced by healthcare staff following medication administration errors: a descriptive study using text-mining and content analysis of incident data. BMC Health Serv Res 22 , 1474 (2022). https://doi.org/10.1186/s12913-022-08818-1
Download citation
Received : 29 June 2022
Accepted : 09 November 2022
Published : 03 December 2022
DOI : https://doi.org/10.1186/s12913-022-08818-1
Share this article
Anyone you share the following link with will be able to read this content:
Sorry, a shareable link is not currently available for this article.
Provided by the Springer Nature SharedIt content-sharing initiative
- Incident report
- Medication error
- Negative emotions
- Second victim
- Healthcare staff
- Text-mining
- Content analysis
BMC Health Services Research
ISSN: 1472-6963
- General enquiries: [email protected]
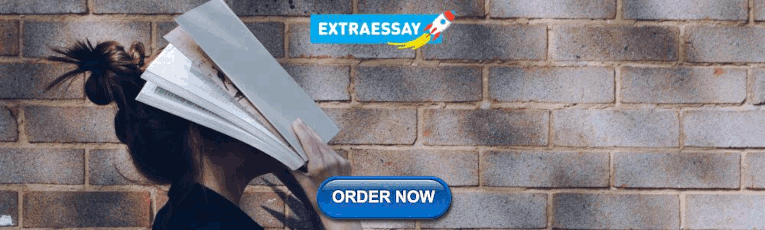
COMMENTS
Our findings indicate that research papers on text mining have been published in 45 academic disciplines in the 1980s and 1990s, 105 disciplines in the 2000s, and 171 disciplines in the 2010s. The results show that using text mining as a research topic and method has rapidly increased. We also demonstrate that the main theme of text mining ...
As it is almost impossible to craft an all-embracing and coherent picture of research questions that can be answered by using text mining to analyze such texts, we outline fields of research that are based both on our experience of using text mining in innovation research and are particularly salient or trending in the innovation research ...
The aim of the Special Issue is to offer an opportunity to publish original research: cutting-edge theories, innovative algorithms, and novel applications. In particular, we welcome manuscripts from text summarization which has been commonly regarded as the most challenging area of text mining. Survey articles describing the state of the art ...
Text mining is increasingly being used in organizational research and practice because up to 80% of organizational data are stored as unstructured, natural language text (Grimes, 2008).Researchers have used closed vocabulary text mining over the past three decades to summarize text data by counting conceptually related words and phrases to score constructs (e.g., entrepreneurial orientation ...
The government makes an effort to provide a means of public complaints through an online aspiration and complaint service called "LaporGub..!". To group incoming reports easier, the topic of the report is searched by using clustering. Text Mining is used to convert text data into numeric data so that it can be processed further.
To address this, we conduct two complementary reviews of computational linguistics and organizational text mining research to provide empirically grounded text preprocessing decision-making recommendations that account for the type of text mining conducted (i.e., open or closed vocabulary), the research question under investigation, and the ...
We discuss this challenge in detail in Section 4 below. Text normalization, part-of-speech tagging, and dependency parsing are often used to reduce the overall document lexicon and to design words' morphological and grammatical features used as an input for entity extraction and other TM tasks (Leaman et al., 2015).
As text semantics has an important role in text meaning, the term semantics has been seen in a vast sort of text mining studies. However, there is a lack of studies that integrate the different research branches and summarize the developed works. This paper reports a systematic mapping about semantics-concerned text mining studies. This systematic mapping study followed a well-defined protocol ...
researchers and research institutions. In this paper, we review several existing methodologies for analyzing texts and introduce a formal pro-cess of applying text mining techniques using the open-source software R. In addition, we discuss potential empirical applications.
During the last years, significant efforts in information retrieval have been made for biomedical and biochemical publications. For materials science, text mining (TM) methodology is still at the dawn of its development. In this review, we survey the recent progress in creating and applying TM and NLP approaches to materials science field.
Recent advances in text mining and natural language processing technology have enabled researchers to detect an authors identity or demographic characteristics, such as age and gender, in several text genres by automatically analysing the variation of linguistic characteristics. However, applying such techniques in the wild, i.e., in both cybercriminal and regular online social media, differs ...
4. DISCUSSION. In this study, we aimed to identify the most common research topics studied by researchers in the nursing field and analyse their trends between 1998 and 2021. First, we identified these topics using text mining strategies and grouped them according to their similarities.
The massive amount of text related to education provides rich information to support education in many aspects. In the meantime, the vast yet increasing volume of text makes it impossible to analyze manually. Text mining is a powerful tool to automatically analyze large-scaled texts and generate insights from the texts. However, many educational scholars are not fully aware of whether text ...
Topic Models. 212 papers with code • 6 benchmarks • 12 datasets. A topic model is a type of statistical model for discovering the abstract "topics" that occur in a collection of documents. Topic modeling is a frequently used text-mining tool for the discovery of hidden semantic structures in a text body. Benchmarks.
A comprehensive overview of scientific text resources can be found in review of Kolářik et al. (2008). Table 1 lists some common repositories for scientific texts in the domain of chemistry and material science, their document types, and access options. The main advantage of using established databases for TM is the uniform format of their metadata, a convenient API, and sometimes analysis ...
This paper reports a work in progress with contributions including: the development of a framework for gathering and analyzing the views and experiences of users of drug and cosmetic products using machine learning, text mining and sentiment analysis; the application of the proposed framework on Facebook comments and data from Twitter for brand ...
Design research topics attract exponentially more attention and consideration among researchers. This study is the first research article that endeavors to analyze selected design research publications using an advanced approach called "text mining". This approach speculates its results depending on the existence of a research term (i.e., keywords), which can be more robust than other ...
Text mining is the multidisciplinary field which draws on data mining, machine learning, information retrieval, computational linguistics and statistics. Important text mining processes are information extraction, information retrieval, natural language processing, text classification, content analysis and text clustering.
The translation models cover 200 languages; the NLLB models come in multiple sizes (54.5B MoE, 3.3B and 1.3B Dense, and 1.3B and 600M distilled). The language identification models contain more ...
Text-mining-driven materials discoveries. Research exploring TM-based data-driven approaches to provide insights on materials emerged well before any progress in the development of robust NLP tools had been made. Several groups have attempted manual information extraction from a narrow set of publications with a specific scope.
Background Medication errors regardless of the degree of patient harm can have a negative emotional impact on the healthcare staff involved. The potential for self-victimization of healthcare staff following medication errors can add to the moral distress of healthcare staff. The stigma associated with errors and their disclosure often haunts healthcare professionals, leading them to question ...
This paper reviews the most common text mining methodologies with R. •. We offer a detailed step-by-step tutorial to analyze central bank texts. •. Comprehensive code excerpts and examples of output are provided. •. Examples include text cleaning, sentiment analysis, and topic modeling.
for scientic text is an area of active research in the computer science and text mining community (Memon et al., 2020; Ramakrishnan et al., 2012). 2.3 Text pre-processing, grammatical and morphological parsing The raw documents proceed through normalization, segmentation, and grammar parsing. During this step,
In association with the International Water Association Water Research has an open access companion journal Water Research X, sharing the same aims and scope, editorial team, submission system and rigorous peer review. Water Research publishes refereed, original research papers on all aspects of the science and technology of the anthropogenic water cycle, water quality, and its management ...
Food Research International provides a forum for the rapid dissemination of significant novel and high impact research in food science, technology, engineering and nutrition. The journal only publishes novel, high quality and high impact review papers, original research papers and letters to the …. View full aims & scope.
Part E's aims and scope are complementary to Transportation Research Part A: Policy and Practice, Part B: Methodological, Part C: Emerging Technologies, Part D: Transport and Environment and Part F: Traffic Psychology and Behaviour. The complete set forms the most cohesive and comprehensive reference of current research in transportation science.