Have a language expert improve your writing
Run a free plagiarism check in 10 minutes, generate accurate citations for free.
- Knowledge Base
Methodology
- Population vs. Sample | Definitions, Differences & Examples
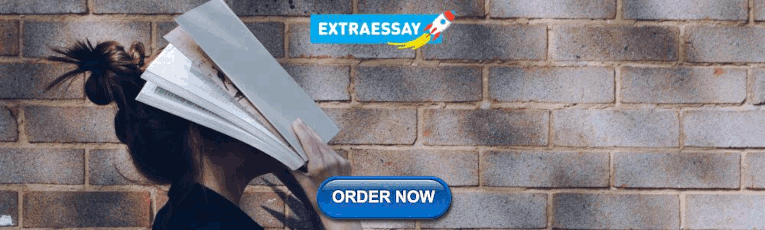
Population vs. Sample | Definitions, Differences & Examples
Published on May 14, 2020 by Pritha Bhandari . Revised on June 21, 2023.
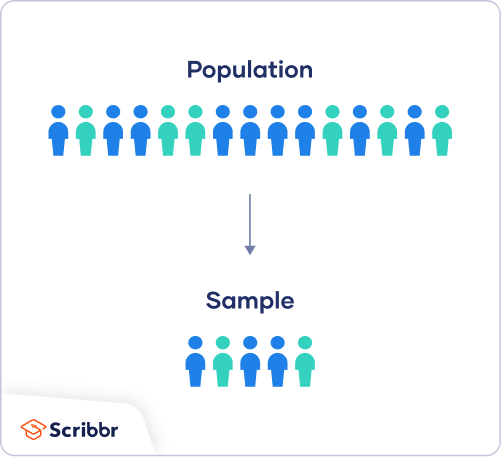
A population is the entire group that you want to draw conclusions about.
A sample is the specific group that you will collect data from. The size of the sample is always less than the total size of the population.
In research, a population doesn’t always refer to people. It can mean a group containing elements of anything you want to study, such as objects, events, organizations, countries, species, organisms, etc.
Table of contents
Collecting data from a population, collecting data from a sample, population parameter vs. sample statistic, practice questions : populations vs. samples, other interesting articles, frequently asked questions about samples and populations.
Populations are used when your research question requires, or when you have access to, data from every member of the population.
Usually, it is only straightforward to collect data from a whole population when it is small, accessible and cooperative.
For larger and more dispersed populations, it is often difficult or impossible to collect data from every individual. For example, every 10 years, the federal US government aims to count every person living in the country using the US Census. This data is used to distribute funding across the nation.
However, historically, marginalized and low-income groups have been difficult to contact, locate and encourage participation from. Because of non-responses, the population count is incomplete and biased towards some groups, which results in disproportionate funding across the country.
In cases like this, sampling can be used to make more precise inferences about the population.
Receive feedback on language, structure, and formatting
Professional editors proofread and edit your paper by focusing on:
- Academic style
- Vague sentences
- Style consistency
See an example
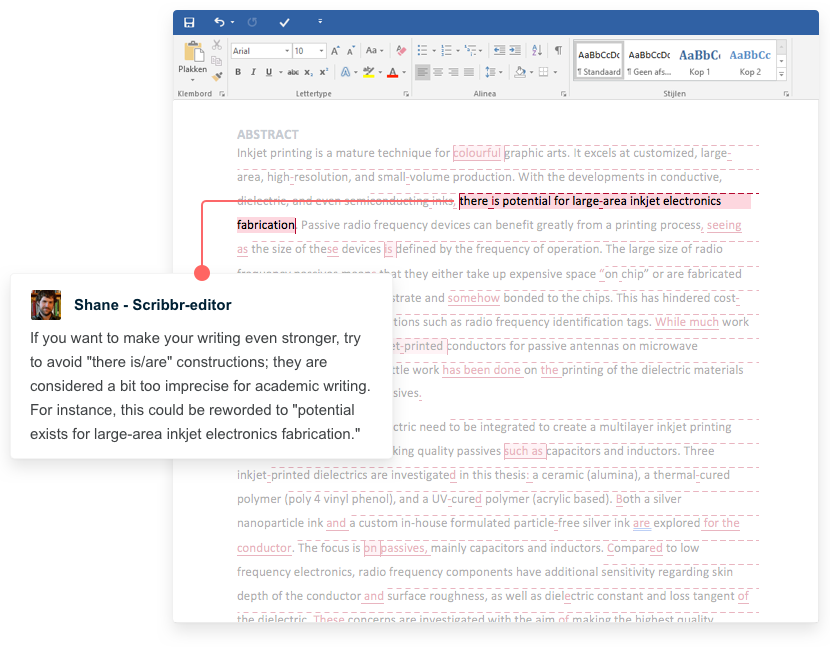
When your population is large in size, geographically dispersed, or difficult to contact, it’s necessary to use a sample. With statistical analysis , you can use sample data to make estimates or test hypotheses about population data.
Ideally, a sample should be randomly selected and representative of the population. Using probability sampling methods (such as simple random sampling or stratified sampling ) reduces the risk of sampling bias and enhances both internal and external validity .
For practical reasons, researchers often use non-probability sampling methods. Non-probability samples are chosen for specific criteria; they may be more convenient or cheaper to access. Because of non-random selection methods, any statistical inferences about the broader population will be weaker than with a probability sample.
Reasons for sampling
- Necessity : Sometimes it’s simply not possible to study the whole population due to its size or inaccessibility.
- Practicality : It’s easier and more efficient to collect data from a sample.
- Cost-effectiveness : There are fewer participant, laboratory, equipment, and researcher costs involved.
- Manageability : Storing and running statistical analyses on smaller datasets is easier and reliable.
When you collect data from a population or a sample, there are various measurements and numbers you can calculate from the data. A parameter is a measure that describes the whole population. A statistic is a measure that describes the sample.
You can use estimation or hypothesis testing to estimate how likely it is that a sample statistic differs from the population parameter.
Sampling error
A sampling error is the difference between a population parameter and a sample statistic. In your study, the sampling error is the difference between the mean political attitude rating of your sample and the true mean political attitude rating of all undergraduate students in the Netherlands.
Sampling errors happen even when you use a randomly selected sample. This is because random samples are not identical to the population in terms of numerical measures like means and standard deviations .
Because the aim of scientific research is to generalize findings from the sample to the population, you want the sampling error to be low. You can reduce sampling error by increasing the sample size.
Here's why students love Scribbr's proofreading services
Discover proofreading & editing
If you want to know more about statistics , methodology , or research bias , make sure to check out some of our other articles with explanations and examples.
- Student’s t -distribution
- Normal distribution
- Null and Alternative Hypotheses
- Chi square tests
- Confidence interval
- Cluster sampling
- Stratified sampling
- Data cleansing
- Reproducibility vs Replicability
- Peer review
- Likert scale
Research bias
- Implicit bias
- Framing effect
- Cognitive bias
- Placebo effect
- Hawthorne effect
- Hindsight bias
- Affect heuristic
Samples are used to make inferences about populations . Samples are easier to collect data from because they are practical, cost-effective, convenient, and manageable.
Populations are used when a research question requires data from every member of the population. This is usually only feasible when the population is small and easily accessible.
A statistic refers to measures about the sample , while a parameter refers to measures about the population .
A sampling error is the difference between a population parameter and a sample statistic .
Cite this Scribbr article
If you want to cite this source, you can copy and paste the citation or click the “Cite this Scribbr article” button to automatically add the citation to our free Citation Generator.
Bhandari, P. (2023, June 21). Population vs. Sample | Definitions, Differences & Examples. Scribbr. Retrieved March 23, 2024, from https://www.scribbr.com/methodology/population-vs-sample/
Is this article helpful?
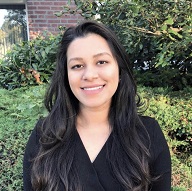
Pritha Bhandari
Other students also liked, simple random sampling | definition, steps & examples, sampling bias and how to avoid it | types & examples, parameter vs statistic | definitions, differences & examples, unlimited academic ai-proofreading.
✔ Document error-free in 5minutes ✔ Unlimited document corrections ✔ Specialized in correcting academic texts
Join thousands of product people at Insight Out Conf on April 11. Register free.
Insights hub solutions
Analyze data
Uncover deep customer insights with fast, powerful features, store insights, curate and manage insights in one searchable platform, scale research, unlock the potential of customer insights at enterprise scale.
Featured reads
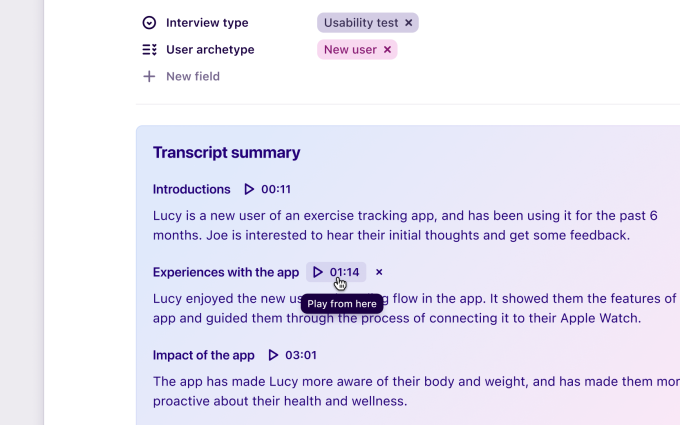
Tips and tricks
Make magic with your customer data in Dovetail
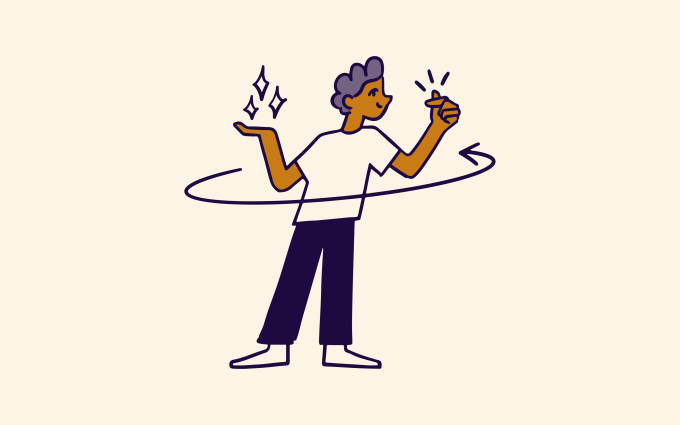
Four ways Dovetail helps Product Managers master continuous product discovery
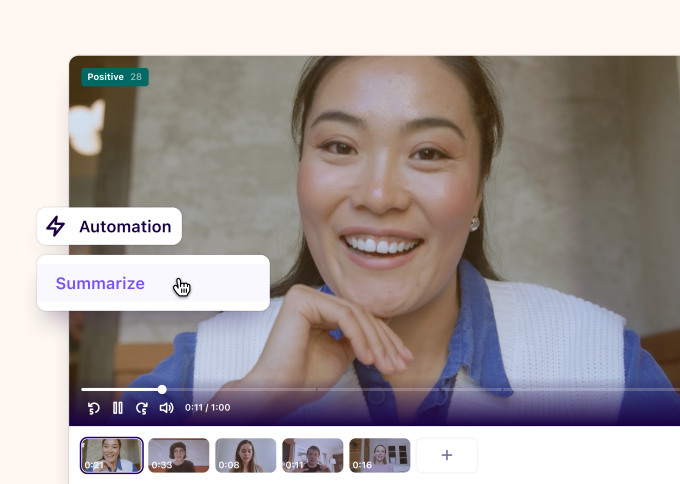
Product updates
Dovetail retro: our biggest releases from the past year
Events and videos
© Dovetail Research Pty. Ltd.
Guide to population vs. sample in research
Last updated
29 May 2023
Reviewed by
Miroslav Damyanov
Population data consists of information collected from every individual in a particular population. Meanwhile, sample data consists of information taken from a subset—or sample —of the population.
In this guide, we’ll discuss the differences between population and sample data, the advantages and disadvantages of each, how to collect data from a sample and a population, and common sampling techniques . By the end, you'll have a better understanding of the differences between population and sample data and when to use them.
Make research less tedious
Dovetail streamlines research to help you uncover and share actionable insights
- What is "population" in research?
Population data is the total number of measurements taken from every individual within a group. For example, if you were measuring the heights of all humans on Earth, you’d include all 7 billion people in your population data set.
When analyzing population data, researchers use statistics such as the population mean, median, and standard deviation.
Types of populations
Finite population.
A finite population is a population in which all the members are known and can be counted. Examples of this type of population include all the employees of a company, all the students in a school, or the entire population of a city. When working with a finite population, you can calculate the exact population mean, median, and standard deviation.
Infinite population
An infinite population is a population that is too large to be measured or counted. This could be the entire human population on Earth or the number of stars in the sky. Because it’s impossible to measure or count these populations, it isn’t possible to calculate their exact mean, median, and standard deviation.
Closed population
A closed population is one in which you allow no new members to join. An example of a closed population would be a country's citizens over the age of 18 who have been living there for more than 10 years. As no new members can join, the population remains constant and can easily be measured and analyzed.
Open population
An open population is one in which new members can join. For example, all people living in a certain city are considered an open population because new members can move into the city and become part of the population. This type of population is constantly changing, so it isn’t possible to measure and analyze its exact characteristics.
Advantages of population data
Representative.
It offers a complete representation of all elements in the population, which can increase the generalizability of findings.
High quality
Population data is usually very accurate and detailed because standardized data collection methods and quality control measures are in place to provide data from every element in the population.
Large sample size
The sample size is large, which can increase the statistical power of a study and help detect small but meaningful differences.
Can address rare events
You can use population data to study rare events or diseases that wouldn’t be feasible to study through other methods.
Allows for subgroup analysis
You can use population data to examine subgroups of the population, which can help identify disparities and inform interventions.
Disadvantages of population data
Time and cost constraints.
Collecting data from a large population is expensive and time-consuming, especially when it comes to data cleaning and preparation before using it for analysis.
Limited access
Depending on the source of population data, it can be difficult to get access to the population or convince people to participate, especially when there are privacy concerns or restrictions on the use of data.
Limited variables
Population data may have limited variables or lack information on important factors, which may not allow one to answer a particular research question if the data wasn’t originally collected for that purpose.
Difficult to analyze
Population data can be large, complex, and contain a wide variety of data or even missing data which demands advanced analytical skills and high computational requirements.
Outdated information
Population data may become outdated, especially if it was collected some time ago, which can limit its relevance to current research questions.
- What is a sample in research?
Sampling is the process of selecting individuals from a larger population and is used to generate representative information about the population of interest. There are two forms of sampling: non-probability.
Probability sampling is from a randomly selected small subset and provides statistical inferences about the whole population without bias. Non-probability sampling collects data from a selected subset chosen for its convenience or, sometimes, to control and manipulate the data collected.
Types of probability sampling
Random sampling.
This type of sampling is completely by chance. Each member of the population has an equal chance of being selected for the sample, and the results of a random sample will be statistically representative of the whole population.
For example, if you wanted to know how people felt about a new product, you could use a random number generator to select members from a population for the study.
Stratified sampling
Stratified sampling is when the population is split into different subgroups, or strata, based on one or more characteristics. The researcher then randomly selects members from each stratum to represent the population. This allows the researcher to accurately compare data between different groups because it ensures that all subgroups are represented in the sample.
For example, if you wanted to measure the opinion of people in different age groups, you could divide your population into groups based on age and then take random samples from each stratum.
Cluster sampling
This type of sampling divides the population into clusters or groups and then further takes a sample from each cluster. This method is often used when it isn’t possible to access the entire population.
For example, if you wanted to measure public opinion on an issue in a large city, it wouldn’t be feasible to survey every single person. Instead, you could divide the city into neighborhoods and take random samples from each one.
Systematic sample
Systematic sampling involves selecting items from a population based on a set pattern or system. This type of sampling is useful when it’s impossible or impractical to create a list of all items in a population. It’s similar to random sampling in that it helps eliminate any bias from the selection process, but it’s more efficient because it requires fewer samples to be taken.
If a researcher can only select 10 members from a population of 200 people, they could use systematic sampling by selecting every 20th person in the list to eliminate bias.
Types of non-probability sampling
Convenience sampling.
This form of sampling involves selecting participants based on availability and willingness to take part. This can lead to volunteer bias, meaning that individuals who are more motivated or have more time may be more likely to participate.
Quota sampling
A method of selecting participants from a larger population to match certain criteria is referred to as quota sampling. For example, market researchers might use quota sampling to select a certain number of individuals within specific age groups.
Judgemental sampling
This technique is also referred to as purposive sampling or authoritative sampling. You can use it to target specific individuals who possess a certain set of qualities like age, ethnicity, or religious beliefs. It can help researchers access important information from people with specific knowledge or experience.
However, this kind of sampling can also lead to selection bias, which is the distortion of results due to the non-random selection of participants.
Snowball sampling
Snowball sampling is often used to reach individuals who may be difficult to access through traditional means. This type of sampling involves asking participants to refer others who fit the same criteria. It’s often used in social sciences research to identify people within a certain community or social group. For example, researchers may conduct a survey offering a reward to participants who refer their close friends or family and get them to participate.
While this technique can be useful in reaching underserved or underrepresented populations, it also carries the risk of selection bias.
Advantages of sample data
Cost-effective.
Collecting data from a sample is typically less expensive and time-consuming than collecting data from an entire population.
Higher quality
Collecting data from a smaller subset of a population can often result in higher-quality data when more resources are dedicated to ensuring the accuracy and completeness of the data.
Feasibility
In some cases, it may be impossible or impractical to collect data from an entire population, making sample data a more feasible option.
Sample data is usually smaller and more manageable than population data, which makes it easier to analyze.
Reduced sampling bias
With appropriate sampling methods, sample data can be representative of the large population and provide valuable insights for research.
Disadvantages of using sample data
Generalizability.
The quality of the data depends on the quality of the sample selection process. If the sample isn’t representative of the population, it leads to skewed results.
Sampling bias
A sample may not provide a complete picture of an entire population when certain groups are overrepresented or underrepresented in the sample.
Sampling error
Because sample data is drawn from a subset of a larger population, there is always a risk of sampling error . It occurs when the sample doesn’t accurately represent the larger population, which can lead to inaccurate results.
Statistical power
A small sample size can limit the statistical power of the data analysis, making it more difficult to detect meaningful differences or relationships between studied variables.
Limited score
Sample data may be limited in scope and may not capture the full range of variables present in an entire population. This can limit the depth and breadth of the findings.
- Differences between population and sample
When discussing research and data analysis, it’s important to understand the differences between population and sample data. Here are some key points to consider when distinguishing between the two:
Population vs. sample
A population is a set of all individuals or objects that share a common characteristic, while a sample is a subset of that population used to draw conclusions about the entire population.
For example, if you wanted to research the opinions of all people living in the United States, the population would be all citizens in the US, while the sample would be a smaller subset of people surveyed to represent the opinion of the entire population.
Sample vs. population mean
The sample mean is an average of a sample's values, while the population mean is an average of all values in a population. For example, if you’re researching the average income of households in America, the sample mean would be an average of incomes from a smaller group of households selected from the population of all households in the US.
Sample vs. population standard deviation
Standard deviation measures the variation of a set of values from their mean. The sample standard deviation is based on the variation within a sample, while the population standard deviation is based on the variation within a population.
For example, if you were researching the variation in test scores for students at a particular school, the sample standard deviation would be based on the scores of a smaller subset of students from the school, while the population standard deviation would be based on all scores from every student at the school.
- How to collect and use data from a sample
1. Choose the right sampling technique
The most common sampling techniques include random, stratified, convenience, and cluster sampling . Selecting the right technique for your research will depend on your specific needs, resources, goals, and objectives.
2. Decide the sample size
Determining the sample size will vary depending on the goal of your research. Generally speaking, the larger the sample size, the more reliable your results will be. However, there are tradeoffs, such as the cost and resources required to collect data from larger samples.
3. Design an instrument for collecting data
Once you've chosen your sampling technique and decided on the sample size, you'll need to design an instrument for collecting data. This could include surveys , interviews, or experiments. Make sure that the instrument is valid and reliable so that it provides accurate results.
4. Determine a sample frame
Decide who you’ll include in the sample by selecting the population or subpopulation you want to study. Consider factors like location, age, gender, behavior, and so on when choosing your sample frame.
5. Execute the sample selection process
In this step, you'll select individuals to form your sample. To ensure accuracy, it’s best to use random sampling techniques to guarantee a representative sample.
6. Collect data from a sample
Once you’ve selected the sample, you can begin collecting data. Depending on the method you chose (e.g., survey, interview, experiment), you may need to do some additional steps before you can begin collecting data:
For example, if you’re collecting data through a survey, you may need to obtain permission to conduct the survey from relevant authorities, such as a workplace or community group.
If you plan to conduct interviews as your data collection method, ensure your questions are well-formed and that your interviewees are comfortable answering them. Before the interview, you may also want to send a pre-interview questionnaire to participants to collect basic information to make the interview process more efficient.
Most experiments require a significant amount of planning and preparation to ensure that data is collected in a controlled and systematic manner. Additionally, you may need to consider the ethical implications of conducting the experiment, such as obtaining informed consent from participants and ensuring their safety throughout the experiment.
7. Analyze the data
After you've collected data from the sample, analyze it to find meaningful patterns and trends that you can use to draw conclusions about the population. Remember, since you're working with a sample, your conclusions may not apply to the entire population.
By following these steps, you can easily collect data from a sample to gain insights about a population without having to analyze all of the data from the population itself. When used correctly, sample data can provide valuable insights that can help shape your research conclusions.
- How to collect and use data from a population
1. Define the population
Before collecting data from a population, it’s important to first clearly define what population you’re looking to collect data from. This definition should be as specific as possible and include any relevant behavioral characteristics (e.g., shopping frequency, product use, or commute options) or demographic characteristics (e.g., age, gender, and geography).
2. Create a comprehensive list
After identifying the population in terms of traits, past experiences, outlooks, or other components, create a comprehensive list of the population you’ll be studying. Depending on the purpose of the study, this could include both people and organizations.
3. Contact population and collect data
Once you’ve defined the population and chosen your sampling method, it’s time to collect data. You can obtain this data by conducting experiments, surveys, or interviews. Make sure to collect feedback from every person or entity on the population list to generate an exhaustive population sample.
4. Analyze the data
After collecting the data, it’s important to analyze it to draw meaningful conclusions about the population. This analysis should include calculating the sample mean and sample standard deviation for the data set, as well as comparing these values to the population mean and population standard deviation.
5. Draw conclusions
Once you’ve analyzed the data, use the results to draw conclusions about the population. Make sure to be as accurate and objective as possible when making claims about the population.
- Choosing high-quality samples
High-quality samples are essential when it comes to research. A high-quality sample will produce accurate and reliable study results. A poor-quality sample can result in incorrect or inexact data. These results can be costly and time-consuming to fix.
A good-quality sample is representative of the population. That means the sample has similar characteristics as the population in terms of age, gender, race, and other factors. The sample should also be randomly selected so as not to bias the results. In addition, the sample should be of a large enough size to be statistically significant .
How to select a high-quality sample
Choose a probability sampling method.
Random selection is the most important part of choosing a high-quality sample. You want to ensure that the sample truly represents the population and that no bias has been introduced. You can do this through methods such as random sampling, stratified sampling, cluster sampling, and systematic sampling.
Monitor selection process
You should monitor the selection process to ensure that no bias has been introduced during the selection process. You should also make sure that the sample size is large enough to be statistically significant.
Test for accuracy
You should test the accuracy of your sample by comparing it to the population data. Compare the sample mean vs. population mean, sample vs. population standard deviation, and other factors. If there are any discrepancies between the two, then the sample may not be representative of the population and should be re-evaluated.
By following these steps, you can ensure that your sample is quality and that it correctly reflects the population and produces precise and accurate results.
Using sample and population data can be beneficial in many ways. For example, using sample data allows researchers to make more efficient use of resources while still being able to conclude the population. Additionally, sample data is useful in making statistical inferences about a population, such as the mean or standard deviation.
On the other hand, population data provides an accurate representation of the whole population, which can be beneficial when researchers need detailed information.
To ensure accurate and representative data, researchers must understand the differences between populations and weigh the advantages and risks of each sampling technique. By understanding the difference between population and sample data, researchers can gain valuable insights about their target group and use these insights to make informed decisions.
Get started today
Go from raw data to valuable insights with a flexible research platform
Editor’s picks
Last updated: 21 December 2023
Last updated: 16 December 2023
Last updated: 17 February 2024
Last updated: 19 November 2023
Last updated: 5 March 2024
Last updated: 15 February 2024
Last updated: 11 March 2024
Last updated: 12 December 2023
Last updated: 6 March 2024
Last updated: 10 April 2023
Last updated: 20 December 2023
Latest articles
Related topics, log in or sign up.
Get started for free

Unraveling Research Population and Sample: Understanding their role in statistical inference
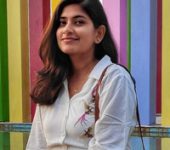
Research population and sample serve as the cornerstones of any scientific inquiry. They hold the power to unlock the mysteries hidden within data. Understanding the dynamics between the research population and sample is crucial for researchers. It ensures the validity, reliability, and generalizability of their findings. In this article, we uncover the profound role of the research population and sample, unveiling their differences and importance that reshapes our understanding of complex phenomena. Ultimately, this empowers researchers to make informed conclusions and drive meaningful advancements in our respective fields.
Table of Contents
What Is Population?
The research population, also known as the target population, refers to the entire group or set of individuals, objects, or events that possess specific characteristics and are of interest to the researcher. It represents the larger population from which a sample is drawn. The research population is defined based on the research objectives and the specific parameters or attributes under investigation. For example, in a study on the effects of a new drug, the research population would encompass all individuals who could potentially benefit from or be affected by the medication.
When Is Data Collection From a Population Preferred?
In certain scenarios where a comprehensive understanding of the entire group is required, it becomes necessary to collect data from a population. Here are a few situations when one prefers to collect data from a population:
1. Small or Accessible Population
When the research population is small or easily accessible, it may be feasible to collect data from the entire population. This is often the case in studies conducted within specific organizations, small communities, or well-defined groups where the population size is manageable.
2. Census or Complete Enumeration
In some cases, such as government surveys or official statistics, a census or complete enumeration of the population is necessary. This approach aims to gather data from every individual or entity within the population. This is typically done to ensure accurate representation and eliminate sampling errors.
3. Unique or Critical Characteristics
If the research focuses on a specific characteristic or trait that is rare and critical to the study, collecting data from the entire population may be necessary. This could be the case in studies related to rare diseases, endangered species, or specific genetic markers.
4. Legal or Regulatory Requirements
Certain legal or regulatory frameworks may require data collection from the entire population. For instance, government agencies might need comprehensive data on income levels, demographic characteristics, or healthcare utilization for policy-making or resource allocation purposes.
5. Precision or Accuracy Requirements
In situations where a high level of precision or accuracy is necessary, researchers may opt for population-level data collection. By doing so, they mitigate the potential for sampling error and obtain more reliable estimates of population parameters.
What Is a Sample?
A sample is a subset of the research population that is carefully selected to represent its characteristics. Researchers study this smaller, manageable group to draw inferences that they can generalize to the larger population. The selection of the sample must be conducted in a manner that ensures it accurately reflects the diversity and pertinent attributes of the research population. By studying a sample, researchers can gather data more efficiently and cost-effectively compared to studying the entire population. The findings from the sample are then extrapolated to make conclusions about the larger research population.
What Is Sampling and Why Is It Important?
Sampling refers to the process of selecting a sample from a larger group or population of interest in order to gather data and make inferences. The goal of sampling is to obtain a sample that is representative of the population, meaning that the sample accurately reflects the key attributes, variations, and proportions present in the population. By studying the sample, researchers can draw conclusions or make predictions about the larger population with a certain level of confidence.
Collecting data from a sample, rather than the entire population, offers several advantages and is often necessary due to practical constraints. Here are some reasons to collect data from a sample:
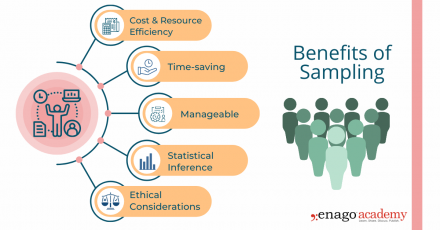
1. Cost and Resource Efficiency
Collecting data from an entire population can be expensive and time-consuming. Sampling allows researchers to gather information from a smaller subset of the population, reducing costs and resource requirements. It is often more practical and feasible to collect data from a sample, especially when the population size is large or geographically dispersed.
2. Time Constraints
Conducting research with a sample allows for quicker data collection and analysis compared to studying the entire population. It saves time by focusing efforts on a smaller group, enabling researchers to obtain results more efficiently. This is particularly beneficial in time-sensitive research projects or situations that necessitate prompt decision-making.
3. Manageable Data Collection
Working with a sample makes data collection more manageable . Researchers can concentrate their efforts on a smaller group, allowing for more detailed and thorough data collection methods. Furthermore, it is more convenient and reliable to store and conduct statistical analyses on smaller datasets. This also facilitates in-depth insights and a more comprehensive understanding of the research topic.
4. Statistical Inference
Collecting data from a well-selected and representative sample enables valid statistical inference. By using appropriate statistical techniques, researchers can generalize the findings from the sample to the larger population. This allows for meaningful inferences, predictions, and estimation of population parameters, thus providing insights beyond the specific individuals or elements in the sample.
5. Ethical Considerations
In certain cases, collecting data from an entire population may pose ethical challenges, such as invasion of privacy or burdening participants. Sampling helps protect the privacy and well-being of individuals by reducing the burden of data collection. It allows researchers to obtain valuable information while ensuring ethical standards are maintained .
Key Steps Involved in the Sampling Process
Sampling is a valuable tool in research; however, it is important to carefully consider the sampling method, sample size, and potential biases to ensure that the findings accurately represent the larger population and are valid for making conclusions and generalizations. While the specific steps may vary depending on the research context, here is a general outline of the sampling process:
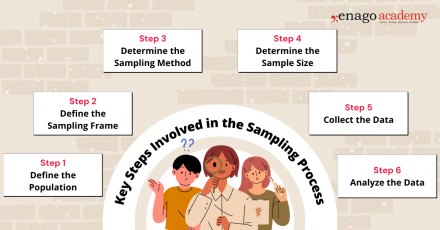
1. Define the Population
Clearly define the target population for your research study. The population should encompass the group of individuals, elements, or units that you want to draw conclusions about.
2. Define the Sampling Frame
Create a sampling frame, which is a list or representation of the individuals or elements in the target population. The sampling frame should be comprehensive and accurately reflect the population you want to study.
3. Determine the Sampling Method
Select an appropriate sampling method based on your research objectives, available resources, and the characteristics of the population. You can perform sampling by either utilizing probability-based or non-probability-based techniques. Common sampling methods include random sampling, stratified sampling, cluster sampling, and convenience sampling.
4. Determine Sample Size
Determine the desired sample size based on statistical considerations, such as the level of precision required, desired confidence level, and expected variability within the population. Larger sample sizes generally reduce sampling error but may be constrained by practical limitations.
5. Collect Data
Once the sample is selected using the appropriate technique, collect the necessary data according to the research design and data collection methods . Ensure that you use standardized and consistent data collection process that is also appropriate for your research objectives.
6. Analyze the Data
Perform the necessary statistical analyses on the collected data to derive meaningful insights. Use appropriate statistical techniques to make inferences, estimate population parameters, test hypotheses, or identify patterns and relationships within the data.
Population vs Sample — Differences and examples
While the population provides a comprehensive overview of the entire group under study, the sample, on the other hand, allows researchers to draw inferences and make generalizations about the population. Researchers should employ careful sampling techniques to ensure that the sample is representative and accurately reflects the characteristics and variability of the population.
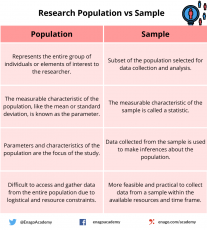
Research Study: Investigating the prevalence of stress among high school students in a specific city and its impact on academic performance.
Population: All high school students in a particular city
Sampling Frame: The sampling frame would involve obtaining a comprehensive list of all high schools in the specific city. A random selection of schools would be made from this list to ensure representation from different areas and demographics of the city.
Sample: Randomly selected 500 high school students from different schools in the city
The sample represents a subset of the entire population of high school students in the city.
Research Study: Assessing the effectiveness of a new medication in managing symptoms and improving quality of life in patients with the specific medical condition.
Population: Patients diagnosed with a specific medical condition
Sampling Frame: The sampling frame for this study would involve accessing medical records or databases that include information on patients diagnosed with the specific medical condition. Researchers would select a convenient sample of patients who meet the inclusion criteria from the sampling frame.
Sample: Convenient sample of 100 patients from a local clinic who meet the inclusion criteria for the study
The sample consists of patients from the larger population of individuals diagnosed with the medical condition.
Research Study: Investigating community perceptions of safety and satisfaction with local amenities in the neighborhood.
Population: Residents of a specific neighborhood
Sampling Frame: The sampling frame for this study would involve obtaining a list of residential addresses within the specific neighborhood. Various sources such as census data, voter registration records, or community databases offer the means to obtain this information. From the sampling frame, researchers would randomly select a cluster sample of households to ensure representation from different areas within the neighborhood.
Sample: Cluster sample of 50 households randomly selected from different blocks within the neighborhood
The sample represents a subset of the entire population of residents living in the neighborhood.
To summarize, sampling allows for cost-effective data collection, easier statistical analysis, and increased practicality compared to studying the entire population. However, despite these advantages, sampling is subject to various challenges. These challenges include sampling bias, non-response bias, and the potential for sampling errors.
To minimize bias and enhance the validity of research findings , researchers should employ appropriate sampling techniques, clearly define the population, establish a comprehensive sampling frame, and monitor the sampling process for potential biases. Validating findings by comparing them to known population characteristics can also help evaluate the generalizability of the results. Properly understanding and implementing sampling techniques ensure that research findings are accurate, reliable, and representative of the larger population. By carefully considering the choice of population and sample, researchers can draw meaningful conclusions and, consequently, make valuable contributions to their respective fields of study.
Now, it’s your turn! Take a moment to think about a research question that interests you. Consider the population that would be relevant to your inquiry. Who would you include in your sample? How would you go about selecting them? Reflecting on these aspects will help you appreciate the intricacies involved in designing a research study. Let us know about it in the comment section below or reach out to us using #AskEnago and tag @EnagoAcademy on Twitter , Facebook , and Quora .

Thank you very much, this is helpful
Very impressive and helpful and also easy to understand….. Thanks to the Author and Publisher….
Rate this article Cancel Reply
Your email address will not be published.
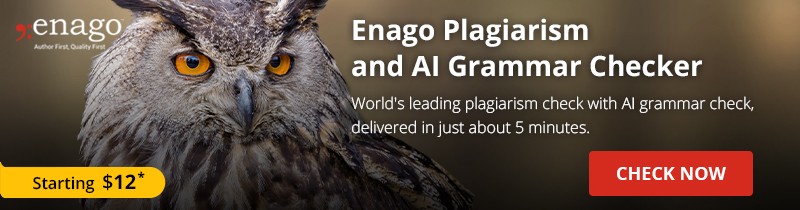
Enago Academy's Most Popular Articles
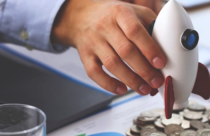
- Diversity and Inclusion
- Trending Now
The Silent Struggle: Confronting gender bias in science funding
In the 1990s, Dr. Katalin Kariko’s pioneering mRNA research seemed destined for obscurity, doomed by…
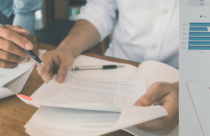
- Reporting Research
Choosing the Right Analytical Approach: Thematic analysis vs. content analysis for data interpretation
In research, choosing the right approach to understand data is crucial for deriving meaningful insights.…
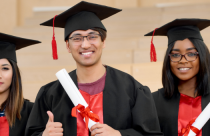
Addressing Barriers in Academia: Navigating unconscious biases in the Ph.D. journey
In the journey of academia, a Ph.D. marks a transitional phase, like that of a…
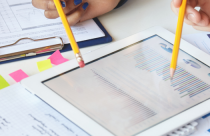
Comparing Cross Sectional and Longitudinal Studies: 5 steps for choosing the right approach
The process of choosing the right research design can put ourselves at the crossroads of…
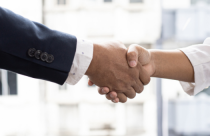
- Career Corner
Unlocking the Power of Networking in Academic Conferences
Embarking on your first academic conference experience? Fear not, we got you covered! Academic conferences…
Choosing the Right Analytical Approach: Thematic analysis vs. content analysis for…
Comparing Cross Sectional and Longitudinal Studies: 5 steps for choosing the right…
Research Recommendations – Guiding policy-makers for evidence-based decision making

Sign-up to read more
Subscribe for free to get unrestricted access to all our resources on research writing and academic publishing including:
- 2000+ blog articles
- 50+ Webinars
- 10+ Expert podcasts
- 50+ Infographics
- 10+ Checklists
- Research Guides
We hate spam too. We promise to protect your privacy and never spam you.
I am looking for Editing/ Proofreading services for my manuscript Tentative date of next journal submission:
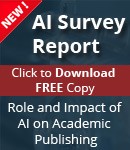
What should universities' stance be on AI tools in research and academic writing?
- How it works
Population vs Sample – Definitions, Types & Examples
Published by Alvin Nicolas at September 20th, 2021 , Revised On July 19, 2023
Wondering who wins in the Population vs. Sample battle? Don’t know which one to choose for your survey?
If you are hunting similar questions, congratulations, you have come to the right place.
The Sample and Population sections tend to be a stumbling block for most students, if not all. And if you are one of those people, now is the perfect time to seize an opportunity. This guide contains all the information in the world to sweep through the methodology section of your dissertation proficiently.
Sounds interesting? Let’s get started then!
What is Population in Research?
Population in the research market comprises all the members of a defined group that you generalize to find the results of your study. This means the exact population will always depend on the scope of your respected study. Population in research is not limited to assessing humans; it can be any data parameter, including events, objects, histories, and more possessing a common trait. The measurable quality of the population is called a parameter .
For instance…
If you are to evaluate findings for Health Concerns of Women , you might have to consider all the women in the world that are dead, alive, and will live in the future.
Types of Population
Though there are different types and sub-categories of population, below are the four most common yet important ones to consider.
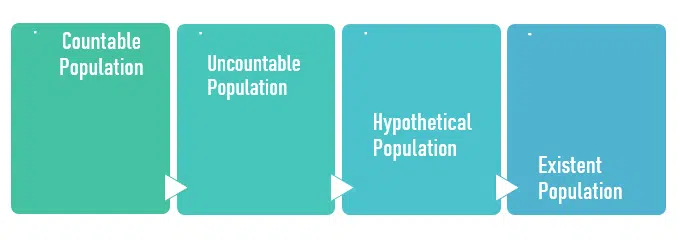
Countable Population
As the term itself explains, this type of population is one that can be numbered and calculated. It is also known as finite population . An example of a finite or countable population would be all the students in a college or potential buyers of a brand. A countable population in statistical analysis is thought to be of more benefit than other types.
Uncountable Population
The uncountable population, primarily known as an infinite population, is where the counting units are beyond one’s consideration and capabilities. For instance, the number of rice grains in the field. Or the total number of protons and electrons on a blank page. The fact that this type of population cannot be calculated often leaves room for error and uncertainty.
Hypothetical Population
This is the population whose unit is not available in a tangible form. Although the population in research analysis includes all sets of possible observations, events, and objects, there still are situations that can only be hypothetical. The perfect example to explain this would be the population of the world. You can give an estimated and hypothetical value gathered by different governments, but can you count all humans existing on the planet? Certainly, no! Another example would be the outcome of rolling dice.
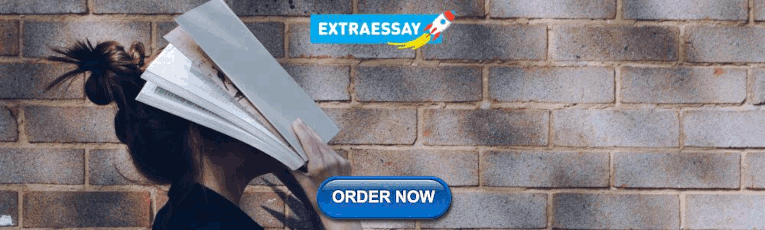
Existent Population
The existent population is the opposite of a hypothetical population, i.e., everything is countable in a concrete form. All the notebooks and pens of students of a particular class could be an example of an existent population.
Is all clear?
Let us move on to the next important term of this guide.
What is Sample in Research?
In quantitative research methodology , the sample is a set of collected data from a defined procedure. It is basically a much smaller part of the whole, i.e., population. The sample depicts all the members of the population that are under observation when conducting research surveys . It can be further assessed to find out about the behavior of the entire population data. The measurable quality of the sample is called a statistic .
Say you send a research questionnaire to all the 200 contacts on your phone, and 42 of them end up filling up the forms. Your sample here is the 42 contacts that participated in the study. The rest of the people who did not participate but were sent invitations become part of your sampling frame . The sampling frame is the group of people who could possibly be in your research or can be a good fit, which here are the 158 people on your phone.
Can you think of more examples?
Before we start with the sampling types, here are a few other terminologies related to sampling for a better understanding.
Sample Size : the total number of people selected for the survey/study
Sample Technique : The technique you use in order to get your desired sample size.
Pro Tip: Use a sample for your research when you have a larger population, and you want to generalize your findings for the entire population from this sample.
What data collection best suits your research?
- Find out by hiring an expert from ResearchProspect today!
- Despite how challenging the subject may be, we are here to help you.
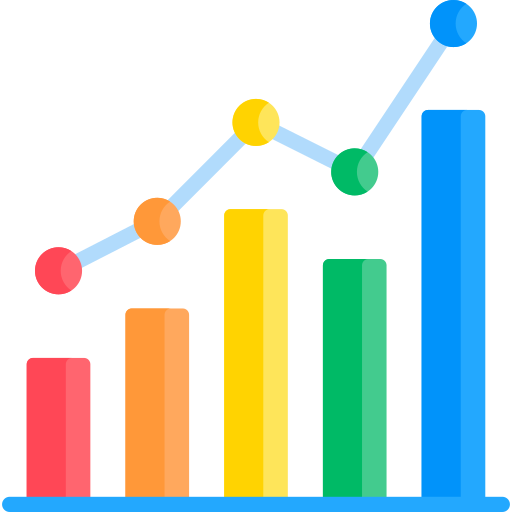
Types of Sampling Methods
There are two major types of sampling; Probability Sampling and Non-probability Sampling.
Probability Sampling
In this type of sampling, the researcher tends to set a selection of a few criteria and selects members of a population randomly. This means all the members have an equal chance to be a part of the study.
For example, you are to examine a bag containing rice or some other food item. Now any small portion or part you take for observation will be a true representative of the whole food bag.
It is further divided into the following five types:
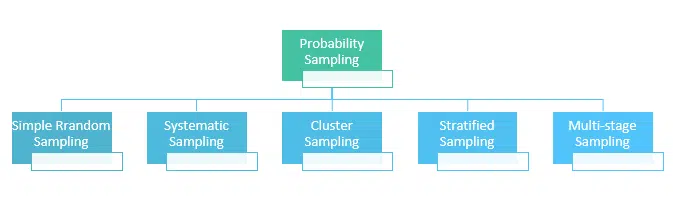
- Simple Random Sampling
In this type of probability sampling, the members of the study are chosen by chance or randomly. Wondering if this affects the overall quality of your research? Well, it does not. The fact that every member has an equal chance of being selected, this random selection will do just as fine and speak well for the whole group. The only thing you need to make sure of is that the population is homogenous , like the bag of rice.
- Systematic Sampling
In systematic sampling, the researcher will select a member after a fixed interval of time. The member selected for the study after this fixed interval is known as the Kth element.
For example, if the researcher decides to select a member occurring after every 30 members, the Kth element here would be the 30th element.
- Stratified Random Sampling
If you know the meaning of strata, you might have guessed by now what stratified random sampling is. So, in this type of sampling, the population is first divided into sub-categories. There is no hard and fast rule for it; it is all done randomly.
So, when do we need this kind of sampling?
Stratified random sampling is adopted when the population is not homogenous. It is first divided into groups and categories based on similarities, and later members from each group are randomly selected. The idea is to address the problem of less homogeneity of the population to get a truly representative sample.
- Cluster Sampling
This is where researchers divide the population into clusters that tend to represent the whole population. They are usually divided based on demographic parameters , such as location, age, and sex. It can be a little difficult than the ones earlier mentioned, but cluster sampling is one of the most effective ways to derive interface from the feedback.
For example, suppose the United States government wishes to evaluate the number of people taking the first dose of the COVID-19 vaccine. In that case, they can divide it into groups based on various country estates. Not only will the results be accurate using this sampling method, but it will also be easier for future diagnoses.
- Multi-stage Sampling
Multi-stage sampling is similar to cluster sampling, but let’s say, a complex form of it. In this type of cluster sampling, all the clusters are further divided into sub-clusters. It involves multiple stages, thus the name. Initially, the naturally occurring categories in a population are chosen as clusters, then each cluster is categorized into smaller clusters, and lastly, members are selected from each smaller cluster.
How many stages are enough?
Well, that depends on the nature of your study/research. For some, two to three would be more than enough, while others can take up to 10 rounds or more.
Non-Probability Sampling
Non-probability sampling is the other sampling type where you cannot calculate the probability or chances of any members selected for research. In other words, it is everything the probability sampling is NOT. We just figured out that probability sampling includes selection by chance; this one depends on the subjective judgment of the researcher.
For example, one member might have a 20 percent chance of getting selected in non-probability sampling, while another could have a 60 percent chance.
Get statistical analysis help at an affordable price
- An expert statistician will complete your work
- Rigorous quality checks
- Confidentiality and reliability
- Any statistical software of your choice
- Free Plagiarism Report

Which type of sampling do you think is better?
The debate on this might prevail forever because there is no correct answer for this. Both have their advantages and disadvantages. While non-probability sampling cannot be reliable, it does save your time and costs. Similarly, if probability sampling yields accurate results, it also is not easy to use and sometimes impossible to be conducted, especially when you have a small population at hand.
Types of Non-Probability Sampling
The Four types of non-probability sampling are:
- Convenience Sampling
Convenience sampling relies on the ease of access to specific subjects such as students in the college café or pedestrians on the road. If the researcher can conveniently get the sample for their study, it will fall under this type of sampling. This type of sampling is usually effective when researchers lack time, resources, and money. They have almost zero authority to choose the sample elements and are purely done on immediacy. You send your questionnaire to random contacts on your phone would be convenience sampling as you did not walk extra miles to get the job done.
- Purposive Sampling
Purposive sampling is also known as judgmental sampling because researchers here would effectively consider the study’s purpose and some understanding of what to expect from the target audience. In other words, the target audience is defined here. For instance, if a study is conducted exclusively for Coronavirus patients, all others not affected by the virus will automatically be rejected or excluded from the study.
- Quota Sampling
For quota sampling, you need to have a pre-set standard of sample selection. What happens in quota sampling is that the sample is formed on the basis of specific attributes so that the qualities of this sample can be found in the total population. Slightly complex but worth the hassle.
- Snowball Sampling
Lastly, this type of non-probability sampling is applied when the subjects are rare and difficult to get. For example, if you are to trace and research drug dealers, it would be almost impossible to get them interviewed for the study. This is where snowball sampling comes into play. Similarly, writing a paper on the mental health of rape victims would also be a hard row to hoe. In such a situation, you will only tract a few sources/members and base the rest of your research on it.
To put it briefly, your sample is the group of people participating in the study, while the population is the total number of people to whom the results will apply. As an analogy, if the sample is the garden in your house, the population will be the forests out there.
Now that you have all the details on these two, can you spot three differences between population and sample ?
Well, we are sure you can give more than just three.
Here are a few differences in case you need a quick revision.
Differences between Population and Sample
This brings us to the end of this guide. We hope you are now clear on these topics and have made up your mind to use a sample for your research or population. The final choice is yours; however, make sure to keep all the above-mentioned facts and particulars in mind and see what works best for you.
Meanwhile, if you have questions and queries or wish to add to this guide, please drop a comment in the comments section below.
FAQs About Population vs. Sample
How can you identify a sample and population.
Sample is the specific group you collect data from, and the population is the entire group you deduce conclusions about. The population is the bigger sample size.
What is a population parameter?
Parameter is some characteristic of the population that cannot be studied directly. It is usually estimated by numbers and figures calculated from the sample data.
Is it better to use a sample instead of a population?
Yes, if you looking for a cost-effective and easier way, a sample is the better option.
What is an example of statistics?
If one office is the sample of the population of all offices in a building, then the average of salaries earned by all employees in the sample office annually would be an example of a statistic .
Does a sample represent the entire population?
Not always. Only a representative sample reflects the entire population of your study. It is an unbiased reflection of what the population is actually like. For instance, you can evaluate the effectiveness by dividing your population on the basis of gender, education, profession, and so on. It depends on how much information is available about your population and the scope of your study. Not to mention how detailed you want your study to be.
You May Also Like
Standard error, abbreviated as SE, is a mathematical tool used to assess the variability in statistics.
Descriptive statistics is the summarising and organising of the characteristics of a dataset. Here is a definitive guide to descriptive statistics.
Level of measurement in statistics is a classification that describes the values assigned to different variables and the relationship of these variables with each other.
USEFUL LINKS
LEARNING RESOURCES
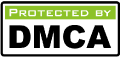
COMPANY DETAILS
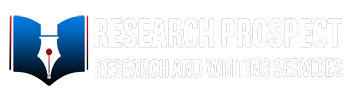
- How It Works
3. Populations and samples
Populations, unbiasedness and precision, randomisation, variation between samples, standard error of the mean.

Studying every person in a target population is more or less impossible. Hence, psychologists select a sample or sub-group of the population that is likely to be representative of the target population we are interested in.
This is important because we want to generalize from the sample to the target population. The more representative the sample, the more confident the researcher can be that the results can be generalized to the target population.
One of the problems that can occur when selecting a sample from a target population is sampling bias. Sampling bias refers to situations where the sample does not reflect the characteristics of the target population.
Many psychology studies have a biased sample because they have used an opportunity sample that comprises university students as their participants (e.g., Asch ).
OK, so you’ve thought up this brilliant psychological study and designed it perfectly. But who will you try it out on, and how will you select your participants?
There are various sampling methods. The one chosen will depend on a number of factors (such as time, money, etc.).
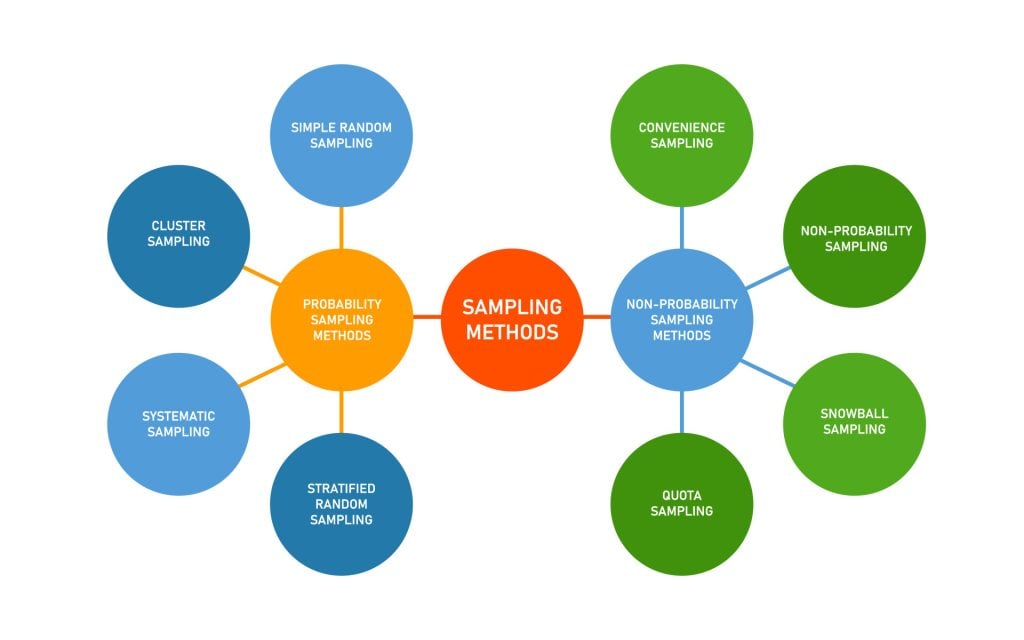
Random Sampling
Random sampling is a type of probability sampling where everyone in the entire target population has an equal chance of being selected.
This is similar to the national lottery. If the “population” is everyone who bought a lottery ticket, then everyone has an equal chance of winning the lottery (assuming they all have one ticket each).
Random samples require naming or numbering the target population and then using some raffle method to choose those to make up the sample. Random samples are the best method of selecting your sample from the population of interest.
- The advantages are that your sample should represent the target population and eliminate sampling bias.
- The disadvantage is that it is very difficult to achieve (i.e., time, effort, and money).
Stratified Sampling
During stratified sampling , the researcher identifies the different types of people that make up the target population and works out the proportions needed for the sample to be representative.
A list is made of each variable (e.g., IQ, gender, etc.) that might have an effect on the research. For example, if we are interested in the money spent on books by undergraduates, then the main subject studied may be an important variable.
For example, students studying English Literature may spend more money on books than engineering students, so if we use a large percentage of English students or engineering students, our results will not be accurate.
We have to determine the relative percentage of each group at a university, e.g., Engineering 10%, Social Sciences 15%, English 20%, Sciences 25%, Languages 10%, Law 5%, and Medicine 15%. The sample must then contain all these groups in the same proportion as the target population (university students).
- The disadvantage of stratified sampling is that gathering such a sample would be extremely time-consuming and difficult to do. This method is rarely used in Psychology.
- However, the advantage is that the sample should be highly representative of the target population, and therefore we can generalize from the results obtained.
Opportunity Sampling
Opportunity sampling is a method in which participants are chosen based on their ease of availability and proximity to the researcher, rather than using random or systematic criteria. It’s a type of convenience sampling .
An opportunity sample is obtained by asking members of the population of interest if they would participate in your research. An example would be selecting a sample of students from those coming out of the library.
- This is a quick and easy way of choosing participants (advantage)
- It may not provide a representative sample and could be biased (disadvantage).
Systematic Sampling
Systematic sampling is a method where every nth individual is selected from a list or sequence to form a sample, ensuring even and regular intervals between chosen subjects.
Participants are systematically selected (i.e., orderly/logical) from the target population, like every nth participant on a list of names.
To take a systematic sample, you list all the population members and then decide upon a sample you would like. By dividing the number of people in the population by the number of people you want in your sample, you get a number we will call n.
If you take every nth name, you will get a systematic sample of the correct size. If, for example, you wanted to sample 150 children from a school of 1,500, you would take every 10th name.
- The advantage of this method is that it should provide a representative sample.
Sample size
The sample size is a critical factor in determining the reliability and validity of a study’s findings. While increasing the sample size can enhance the generalizability of results, it’s also essential to balance practical considerations, such as resource constraints and diminishing returns from ever-larger samples.
Reliability and Validity
Reliability refers to the consistency and reproducibility of research findings across different occasions, researchers, or instruments. A small sample size may lead to inconsistent results due to increased susceptibility to random error or the influence of outliers. In contrast, a larger sample minimizes these errors, promoting more reliable results.
Validity pertains to the accuracy and truthfulness of research findings. For a study to be valid, it should accurately measure what it intends to do. A small, unrepresentative sample can compromise external validity, meaning the results don’t generalize well to the larger population. A larger sample captures more variability, ensuring that specific subgroups or anomalies don’t overly influence results.
Practical Considerations
Resource Constraints : Larger samples demand more time, money, and resources. Data collection becomes more extensive, data analysis more complex, and logistics more challenging.
Diminishing Returns : While increasing the sample size generally leads to improved accuracy and precision, there’s a point where adding more participants yields only marginal benefits. For instance, going from 50 to 500 participants might significantly boost a study’s robustness, but jumping from 10,000 to 10,500 might not offer a comparable advantage, especially considering the added costs.
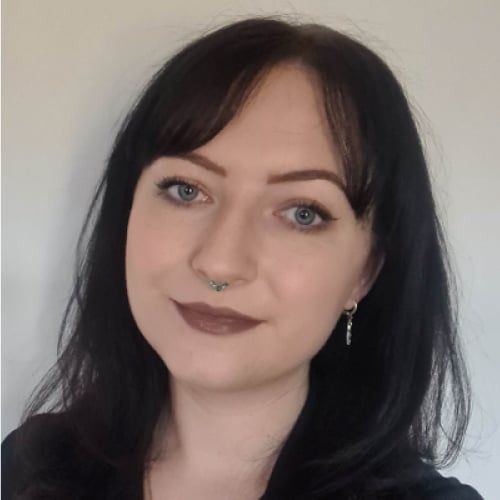
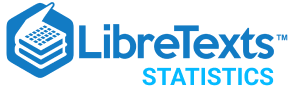
- school Campus Bookshelves
- menu_book Bookshelves
- perm_media Learning Objects
- login Login
- how_to_reg Request Instructor Account
- hub Instructor Commons
- Download Page (PDF)
- Download Full Book (PDF)
- Periodic Table
- Physics Constants
- Scientific Calculator
- Reference & Cite
- Tools expand_more
- Readability
selected template will load here
This action is not available.
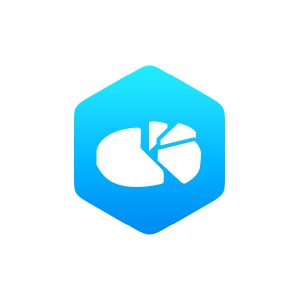
8.1: Samples, Populations and Sampling
- Last updated
- Save as PDF
- Page ID 36115
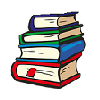
- Danielle Navarro
- University of New South Wales
In the prelude to Part I discussed the riddle of induction, and highlighted the fact that all learning requires you to make assumptions. Accepting that this is true, our first task to come up with some fairly general assumptions about data that make sense. This is where sampling theory comes in. If probability theory is the foundations upon which all statistical theory builds, sampling theory is the frame around which you can build the rest of the house. Sampling theory plays a huge role in specifying the assumptions upon which your statistical inferences rely. And in order to talk about “making inferences” the way statisticians think about it, we need to be a bit more explicit about what it is that we’re drawing inferences from (the sample) and what it is that we’re drawing inferences about (the population).
In almost every situation of interest, what we have available to us as researchers is a sample of data. We might have run experiment with some number of participants; a polling company might have phoned some number of people to ask questions about voting intentions; etc. Regardless: the data set available to us is finite, and incomplete. We can’t possibly get every person in the world to do our experiment; a polling company doesn’t have the time or the money to ring up every voter in the country etc. In our earlier discussion of descriptive statistics (Chapter 5, this sample was the only thing we were interested in. Our only goal was to find ways of describing, summarising and graphing that sample. This is about to change.
Defining a population
A sample is a concrete thing. You can open up a data file, and there’s the data from your sample. A population , on the other hand, is a more abstract idea. It refers to the set of all possible people, or all possible observations, that you want to draw conclusions about, and is generally much bigger than the sample. In an ideal world, the researcher would begin the study with a clear idea of what the population of interest is, since the process of designing a study and testing hypotheses about the data that it produces does depend on the population about which you want to make statements. However, that doesn’t always happen in practice: usually the researcher has a fairly vague idea of what the population is and designs the study as best he/she can on that basis.
Sometimes it’s easy to state the population of interest. For instance, in the “polling company” example that opened the chapter, the population consisted of all voters enrolled at the a time of the study – millions of people. The sample was a set of 1000 people who all belong to that population. In most situations the situation is much less simple. In a typical a psychological experiment, determining the population of interest is a bit more complicated. Suppose I run an experiment using 100 undergraduate students as my participants. My goal, as a cognitive scientist, is to try to learn something about how the mind works. So, which of the following would count as “the population”:
- All of the undergraduate psychology students at the University of Adelaide?
- Undergraduate psychology students in general, anywhere in the world?
- Australians currently living?
- Australians of similar ages to my sample?
- Anyone currently alive?
- Any human being, past, present or future?
- Any biological organism with a sufficient degree of intelligence operating in a terrestrial environment?
- Any intelligent being?
Each of these defines a real group of mind-possessing entities, all of which might be of interest to me as a cognitive scientist, and it’s not at all clear which one ought to be the true population of interest. As another example, consider the Wellesley-Croker game that we discussed in the prelude. The sample here is a specific sequence of 12 wins and 0 losses for Wellesley. What is the population?
- All outcomes until Wellesley and Croker arrived at their destination?
- All outcomes if Wellesley and Croker had played the game for the rest of their lives?
- All outcomes if Wellseley and Croker lived forever and played the game until the world ran out of hills?
- All outcomes if we created an infinite set of parallel universes and the Wellesely/Croker pair made guesses about the same 12 hills in each universe?
Again, it’s not obvious what the population is.
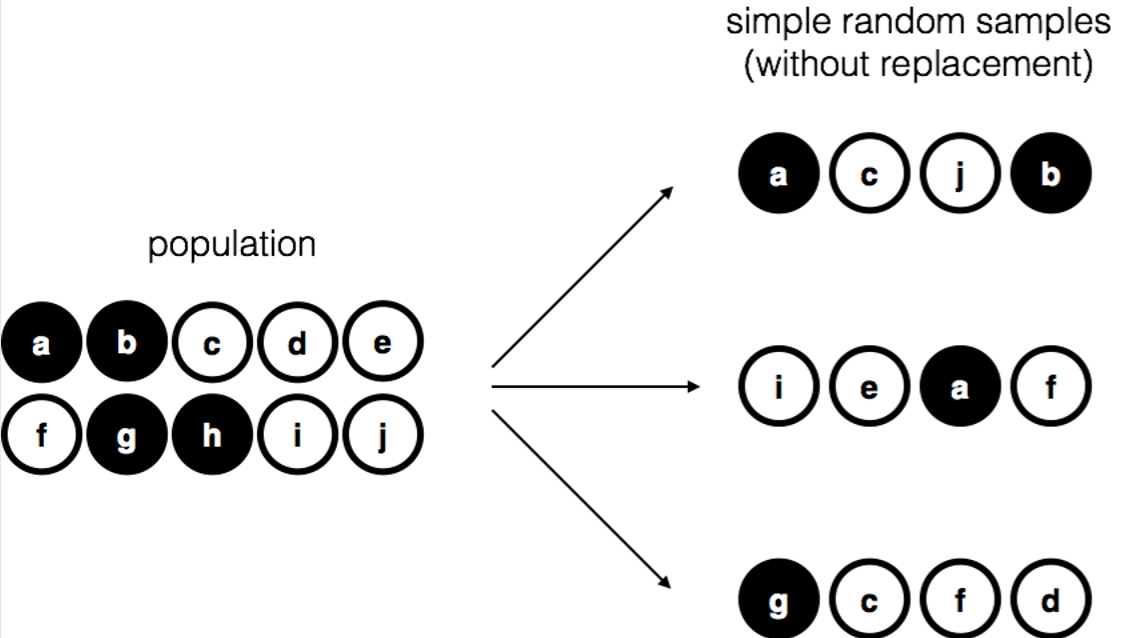
Irrespective of how I define the population, the critical point is that the sample is a subset of the population, and our goal is to use our knowledge of the sample to draw inferences about the properties of the population. The relationship between the two depends on the procedure by which the sample was selected. This procedure is referred to as a sampling method , and it is important to understand why it matters.
To keep things simple, let’s imagine that we have a bag containing 10 chips. Each chip has a unique letter printed on it, so we can distinguish between the 10 chips. The chips come in two colours, black and white. This set of chips is the population of interest, and it is depicted graphically on the left of Figure 10.1. As you can see from looking at the picture, there are 4 black chips and 6 white chips, but of course in real life we wouldn’t know that unless we looked in the bag. Now imagine you run the following “experiment”: you shake up the bag, close your eyes, and pull out 4 chips without putting any of them back into the bag. First out comes the a chip (black), then the c chip (white), then j (white) and then finally b (black). If you wanted, you could then put all the chips back in the bag and repeat the experiment, as depicted on the right hand side of Figure 10.1. Each time you get different results, but the procedure is identical in each case. The fact that the same procedure can lead to different results each time, we refer to it as a random process. 147 However, because we shook the bag before pulling any chips out, it seems reasonable to think that every chip has the same chance of being selected. A procedure in which every member of the population has the same chance of being selected is called a simple random sample . The fact that we did not put the chips back in the bag after pulling them out means that you can’t observe the same thing twice, and in such cases the observations are said to have been sampled without replacement .
To help make sure you understand the importance of the sampling procedure, consider an alternative way in which the experiment could have been run. Suppose that my 5-year old son had opened the bag, and decided to pull out four black chips without putting any of them back in the bag. This biased sampling scheme is depicted in Figure 10.2. Now consider the evidentiary value of seeing 4 black chips and 0 white chips. Clearly, it depends on the sampling scheme, does it not? If you know that the sampling scheme is biased to select only black chips, then a sample that consists of only black chips doesn’t tell you very much about the population! For this reason, statisticians really like it when a data set can be considered a simple random sample, because it makes the data analysis much easier.
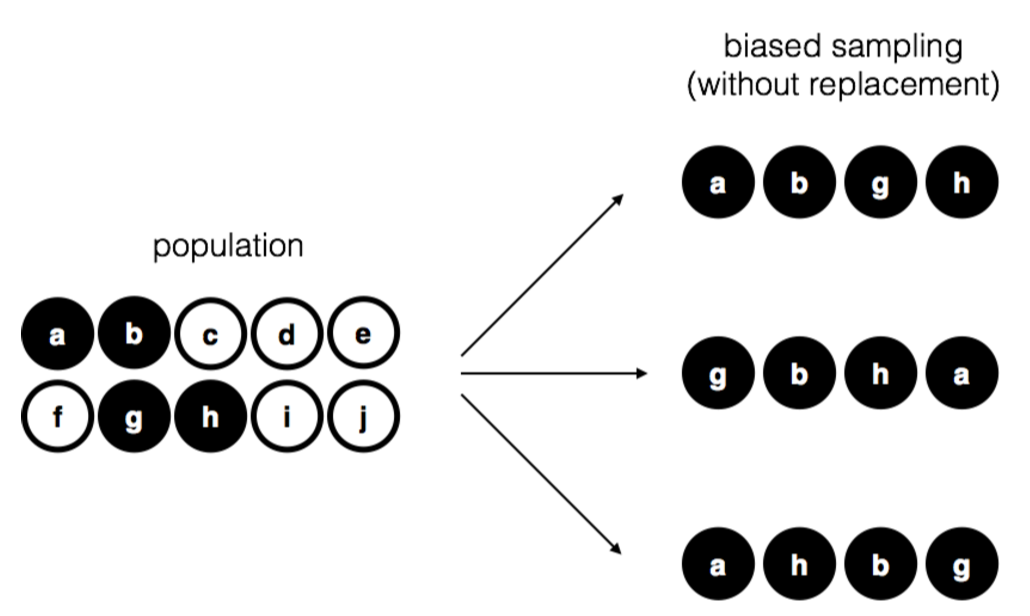
A third procedure is worth mentioning. This time around we close our eyes, shake the bag, and pull out a chip. This time, however, we record the observation and then put the chip back in the bag. Again we close our eyes, shake the bag, and pull out a chip. We then repeat this procedure until we have 4 chips. Data sets generated in this way are still simple random samples, but because we put the chips back in the bag immediately after drawing them it is referred to as a sample with replacement . The difference between this situation and the first one is that it is possible to observe the same population member multiple times, as illustrated in Figure 10.3.
In my experience, most psychology experiments tend to be sampling without replacement, because the same person is not allowed to participate in the experiment twice. However, most statistical theory is based on the assumption that the data arise from a simple random sample with replacement. In real life, this very rarely matters. If the population of interest is large (e.g., has more than 10 entities!) the difference between sampling with- and without- replacement is too small to be concerned with. The difference between simple random samples and biased samples, on the other hand, is not such an easy thing to dismiss.
Most samples are not simple random samples
As you can see from looking at the list of possible populations that I showed above, it is almost impossible to obtain a simple random sample from most populations of interest. When I run experiments, I’d consider it a minor miracle if my participants turned out to be a random sampling of the undergraduate psychology students at Adelaide university, even though this is by far the narrowest population that I might want to generalise to. A thorough discussion of other types of sampling schemes is beyond the scope of this book, but to give you a sense of what’s out there I’ll list a few of the more important ones:
- Stratified sampling . Suppose your population is (or can be) divided into several different subpopulations, or strata . Perhaps you’re running a study at several different sites, for example. Instead of trying to sample randomly from the population as a whole, you instead try to collect a separate random sample from each of the strata. Stratified sampling is sometimes easier to do than simple random sampling, especially when the population is already divided into the distinct strata. It can also be more efficient that simple random sampling, especially when some of the subpopulations are rare. For instance, when studying schizophrenia it would be much better to divide the population into two 148 strata (schizophrenic and not-schizophrenic), and then sample an equal number of people from each group. If you selected people randomly, you would get so few schizophrenic people in the sample that your study would be useless. This specific kind of of stratified sampling is referred to as oversampling because it makes a deliberate attempt to over-represent rare groups.
- Snowball sampling is a technique that is especially useful when sampling from a “hidden” or hard to access population, and is especially common in social sciences. For instance, suppose the researchers want to conduct an opinion poll among transgender people. The research team might only have contact details for a few trans folks, so the survey starts by asking them to participate (stage 1). At the end of the survey, the participants are asked to provide contact details for other people who might want to participate. In stage 2, those new contacts are surveyed. The process continues until the researchers have sufficient data. The big advantage to snowball sampling is that it gets you data in situations that might otherwise be impossible to get any. On the statistical side, the main disadvantage is that the sample is highly non-random, and non-random in ways that are difficult to address. On the real life side, the disadvantage is that the procedure can be unethical if not handled well, because hidden populations are often hidden for a reason. I chose transgender people as an example here to highlight this: if you weren’t careful you might end up outing people who don’t want to be outed (very, very bad form), and even if you don’t make that mistake it can still be intrusive to use people’s social networks to study them. It’s certainly very hard to get people’s informed consent before contacting them, yet in many cases the simple act of contacting them and saying “hey we want to study you” can be hurtful. Social networks are complex things, and just because you can use them to get data doesn’t always mean you should.
- Convenience sampling is more or less what it sounds like. The samples are chosen in a way that is convenient to the researcher, and not selected at random from the population of interest. Snowball sampling is one type of convenience sampling, but there are many others. A common example in psychology are studies that rely on undergraduate psychology students. These samples are generally non-random in two respects: firstly, reliance on undergraduate psychology students automatically means that your data are restricted to a single subpopulation. Secondly, the students usually get to pick which studies they participate in, so the sample is a self selected subset of psychology students not a randomly selected subset. In real life, most studies are convenience samples of one form or another. This is sometimes a severe limitation, but not always.
much does it matter if you don’t have a simple random sample?
Okay, so real world data collection tends not to involve nice simple random samples. Does that matter? A little thought should make it clear to you that it can matter if your data are not a simple random sample: just think about the difference between Figures 10.1 and 10.2. However, it’s not quite as bad as it sounds. Some types of biased samples are entirely unproblematic. For instance, when using a stratified sampling technique you actually know what the bias is because you created it deliberately, often to increase the effectiveness of your study, and there are statistical techniques that you can use to adjust for the biases you’ve introduced (not covered in this book!). So in those situations it’s not a problem.
More generally though, it’s important to remember that random sampling is a means to an end, not the end in itself. Let’s assume you’ve relied on a convenience sample, and as such you can assume it’s biased. A bias in your sampling method is only a problem if it causes you to draw the wrong conclusions. When viewed from that perspective, I’d argue that we don’t need the sample to be randomly generated in every respect: we only need it to be random with respect to the psychologically-relevant phenomenon of interest. Suppose I’m doing a study looking at working memory capacity. In study 1, I actually have the ability to sample randomly from all human beings currently alive, with one exception: I can only sample people born on a Monday. In study 2, I am able to sample randomly from the Australian population. I want to generalise my results to the population of all living humans. Which study is better? The answer, obviously, is study 1. Why? Because we have no reason to think that being “born on a Monday” has any interesting relationship to working memory capacity. In contrast, I can think of several reasons why “being Australian” might matter. Australia is a wealthy, industrialised country with a very well-developed education system. People growing up in that system will have had life experiences much more similar to the experiences of the people who designed the tests for working memory capacity. This shared experience might easily translate into similar beliefs about how to “take a test”, a shared assumption about how psychological experimentation works, and so on. These things might actually matter. For instance, “test taking” style might have taught the Australian participants how to direct their attention exclusively on fairly abstract test materials relative to people that haven’t grown up in a similar environment; leading to a misleading picture of what working memory capacity is.
There are two points hidden in this discussion. Firstly, when designing your own studies, it’s important to think about what population you care about, and try hard to sample in a way that is appropriate to that population. In practice, you’re usually forced to put up with a “sample of convenience” (e.g., psychology lecturers sample psychology students because that’s the least expensive way to collect data, and our coffers aren’t exactly overflowing with gold), but if so you should at least spend some time thinking about what the dangers of this practice might be.
Secondly, if you’re going to criticise someone else’s study because they’ve used a sample of convenience rather than laboriously sampling randomly from the entire human population, at least have the courtesy to offer a specific theory as to how this might have distorted the results. Remember, everyone in science is aware of this issue, and does what they can to alleviate it. Merely pointing out that “the study only included people from group BLAH” is entirely unhelpful, and borders on being insulting to the researchers, who are of course aware of the issue. They just don’t happen to be in possession of the infinite supply of time and money required to construct the perfect sample. In short, if you want to offer a responsible critique of the sampling process, then be helpful . Rehashing the blindingly obvious truisms that I’ve been rambling on about in this section isn’t helpful.
Population parameters and sample statistics
Okay. Setting aside the thorny methodological issues associated with obtaining a random sample and my rather unfortunate tendency to rant about lazy methodological criticism, let’s consider a slightly different issue. Up to this point we have been talking about populations the way a scientist might. To a psychologist, a population might be a group of people. To an ecologist, a population might be a group of bears. In most cases the populations that scientists care about are concrete things that actually exist in the real world. Statisticians, however, are a funny lot. On the one hand, they are interested in real world data and real science in the same way that scientists are. On the other hand, they also operate in the realm of pure abstraction in the way that mathematicians do. As a consequence, statistical theory tends to be a bit abstract in how a population is defined. In much the same way that psychological researchers operationalise our abstract theoretical ideas in terms of concrete measurements (Section 2.1, statisticians operationalise the concept of a “population” in terms of mathematical objects that they know how to work with. You’ve already come across these objects in Chapter 9: they’re called probability distributions.
The idea is quite simple. Let’s say we’re talking about IQ scores. To a psychologist, the population of interest is a group of actual humans who have IQ scores. A statistician “simplifies” this by operationally defining the population as the probability distribution depicted in Figure ?? . IQ tests are designed so that the average IQ is 100, the standard deviation of IQ scores is 15, and the distribution of IQ scores is normal. These values are referred to as the population parameters because they are characteristics of the entire population. That is, we say that the population mean μ is 100, and the population standard deviation σ is 15.

Now suppose I run an experiment. I select 100 people at random and administer an IQ test, giving me a simple random sample from the population. My sample would consist of a collection of numbers like this:
Each of these IQ scores is sampled from a normal distribution with mean 100 and standard deviation 15. So if I plot a histogram of the sample, I get something like the one shown in Figure 10.4b. As you can see, the histogram is roughly the right shape, but it’s a very crude approximation to the true population distribution shown in Figure 10.4a. When I calculate the mean of my sample, I get a number that is fairly close to the population mean 100 but not identical. In this case, it turns out that the people in my sample have a mean IQ of 98.5, and the standard deviation of their IQ scores is 15.9. These sample statistics are properties of my data set, and although they are fairly similar to the true population values, they are not the same. In general, sample statistics are the things you can calculate from your data set, and the population parameters are the things you want to learn about. Later on in this chapter I’ll talk about how you can estimate population parameters using your sample statistics (Section 10.4 and how to work out how confident you are in your estimates (Section 10.5 but before we get to that there’s a few more ideas in sampling theory that you need to know about.
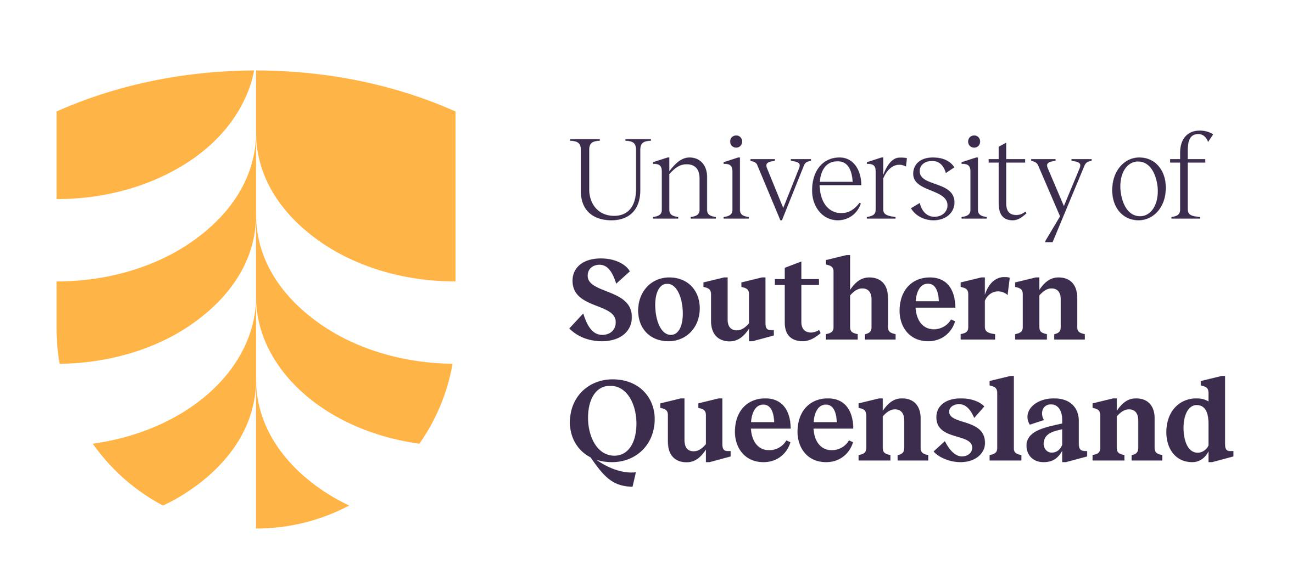
Want to create or adapt books like this? Learn more about how Pressbooks supports open publishing practices.
Sampling is the statistical process of selecting a subset—called a ‘sample’—of a population of interest for the purpose of making observations and statistical inferences about that population. Social science research is generally about inferring patterns of behaviours within specific populations. We cannot study entire populations because of feasibility and cost constraints, and hence, we must select a representative sample from the population of interest for observation and analysis. It is extremely important to choose a sample that is truly representative of the population so that the inferences derived from the sample can be generalised back to the population of interest. Improper and biased sampling is the primary reason for the often divergent and erroneous inferences reported in opinion polls and exit polls conducted by different polling groups such as CNN/Gallup Poll, ABC, and CBS, prior to every US Presidential election.
The sampling process
As Figure 8.1 shows, the sampling process comprises of several stages. The first stage is defining the target population. A population can be defined as all people or items ( unit of analysis ) with the characteristics that one wishes to study. The unit of analysis may be a person, group, organisation, country, object, or any other entity that you wish to draw scientific inferences about. Sometimes the population is obvious. For example, if a manufacturer wants to determine whether finished goods manufactured at a production line meet certain quality requirements or must be scrapped and reworked, then the population consists of the entire set of finished goods manufactured at that production facility. At other times, the target population may be a little harder to understand. If you wish to identify the primary drivers of academic learning among high school students, then what is your target population: high school students, their teachers, school principals, or parents? The right answer in this case is high school students, because you are interested in their performance, not the performance of their teachers, parents, or schools. Likewise, if you wish to analyse the behaviour of roulette wheels to identify biased wheels, your population of interest is not different observations from a single roulette wheel, but different roulette wheels (i.e., their behaviour over an infinite set of wheels).
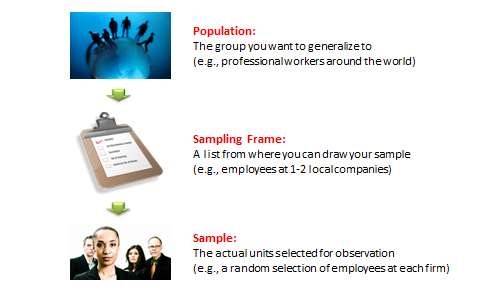
The second step in the sampling process is to choose a sampling frame . This is an accessible section of the target population—usually a list with contact information—from where a sample can be drawn. If your target population is professional employees at work, because you cannot access all professional employees around the world, a more realistic sampling frame will be employee lists of one or two local companies that are willing to participate in your study. If your target population is organisations, then the Fortune 500 list of firms or the Standard & Poor’s (S&P) list of firms registered with the New York Stock exchange may be acceptable sampling frames.
Note that sampling frames may not entirely be representative of the population at large, and if so, inferences derived by such a sample may not be generalisable to the population. For instance, if your target population is organisational employees at large (e.g., you wish to study employee self-esteem in this population) and your sampling frame is employees at automotive companies in the American Midwest, findings from such groups may not even be generalisable to the American workforce at large, let alone the global workplace. This is because the American auto industry has been under severe competitive pressures for the last 50 years and has seen numerous episodes of reorganisation and downsizing, possibly resulting in low employee morale and self-esteem. Furthermore, the majority of the American workforce is employed in service industries or in small businesses, and not in automotive industry. Hence, a sample of American auto industry employees is not particularly representative of the American workforce. Likewise, the Fortune 500 list includes the 500 largest American enterprises, which is not representative of all American firms, most of which are medium or small sized firms rather than large firms, and is therefore, a biased sampling frame. In contrast, the S&P list will allow you to select large, medium, and/or small companies, depending on whether you use the S&P LargeCap, MidCap, or SmallCap lists, but includes publicly traded firms (and not private firms) and is hence still biased. Also note that the population from which a sample is drawn may not necessarily be the same as the population about which we actually want information. For example, if a researcher wants to examine the success rate of a new ‘quit smoking’ program, then the target population is the universe of smokers who had access to this program, which may be an unknown population. Hence, the researcher may sample patients arriving at a local medical facility for smoking cessation treatment, some of whom may not have had exposure to this particular ‘quit smoking’ program, in which case, the sampling frame does not correspond to the population of interest.
The last step in sampling is choosing a sample from the sampling frame using a well-defined sampling technique. Sampling techniques can be grouped into two broad categories: probability (random) sampling and non-probability sampling. Probability sampling is ideal if generalisability of results is important for your study, but there may be unique circumstances where non-probability sampling can also be justified. These techniques are discussed in the next two sections.
Probability sampling
Probability sampling is a technique in which every unit in the population has a chance (non-zero probability) of being selected in the sample, and this chance can be accurately determined. Sample statistics thus produced, such as sample mean or standard deviation, are unbiased estimates of population parameters, as long as the sampled units are weighted according to their probability of selection. All probability sampling have two attributes in common: every unit in the population has a known non-zero probability of being sampled, and the sampling procedure involves random selection at some point. The different types of probability sampling techniques include:

Stratified sampling. In stratified sampling, the sampling frame is divided into homogeneous and non-overlapping subgroups (called ‘strata’), and a simple random sample is drawn within each subgroup. In the previous example of selecting 200 firms from a list of 1,000 firms, you can start by categorising the firms based on their size as large (more than 500 employees), medium (between 50 and 500 employees), and small (less than 50 employees). You can then randomly select 67 firms from each subgroup to make up your sample of 200 firms. However, since there are many more small firms in a sampling frame than large firms, having an equal number of small, medium, and large firms will make the sample less representative of the population (i.e., biased in favour of large firms that are fewer in number in the target population). This is called non-proportional stratified sampling because the proportion of the sample within each subgroup does not reflect the proportions in the sampling frame—or the population of interest—and the smaller subgroup (large-sized firms) is oversampled . An alternative technique will be to select subgroup samples in proportion to their size in the population. For instance, if there are 100 large firms, 300 mid-sized firms, and 600 small firms, you can sample 20 firms from the ‘large’ group, 60 from the ‘medium’ group and 120 from the ‘small’ group. In this case, the proportional distribution of firms in the population is retained in the sample, and hence this technique is called proportional stratified sampling. Note that the non-proportional approach is particularly effective in representing small subgroups, such as large-sized firms, and is not necessarily less representative of the population compared to the proportional approach, as long as the findings of the non-proportional approach are weighted in accordance to a subgroup’s proportion in the overall population.
Cluster sampling. If you have a population dispersed over a wide geographic region, it may not be feasible to conduct a simple random sampling of the entire population. In such case, it may be reasonable to divide the population into ‘clusters’—usually along geographic boundaries—randomly sample a few clusters, and measure all units within that cluster. For instance, if you wish to sample city governments in the state of New York, rather than travel all over the state to interview key city officials (as you may have to do with a simple random sample), you can cluster these governments based on their counties, randomly select a set of three counties, and then interview officials from every office in those counties. However, depending on between-cluster differences, the variability of sample estimates in a cluster sample will generally be higher than that of a simple random sample, and hence the results are less generalisable to the population than those obtained from simple random samples.
Matched-pairs sampling. Sometimes, researchers may want to compare two subgroups within one population based on a specific criterion. For instance, why are some firms consistently more profitable than other firms? To conduct such a study, you would have to categorise a sampling frame of firms into ‘high profitable’ firms and ‘low profitable firms’ based on gross margins, earnings per share, or some other measure of profitability. You would then select a simple random sample of firms in one subgroup, and match each firm in this group with a firm in the second subgroup, based on its size, industry segment, and/or other matching criteria. Now, you have two matched samples of high-profitability and low-profitability firms that you can study in greater detail. Matched-pairs sampling techniques are often an ideal way of understanding bipolar differences between different subgroups within a given population.
Multi-stage sampling. The probability sampling techniques described previously are all examples of single-stage sampling techniques. Depending on your sampling needs, you may combine these single-stage techniques to conduct multi-stage sampling. For instance, you can stratify a list of businesses based on firm size, and then conduct systematic sampling within each stratum. This is a two-stage combination of stratified and systematic sampling. Likewise, you can start with a cluster of school districts in the state of New York, and within each cluster, select a simple random sample of schools. Within each school, you can select a simple random sample of grade levels, and within each grade level, you can select a simple random sample of students for study. In this case, you have a four-stage sampling process consisting of cluster and simple random sampling.
Non-probability sampling
Non-probability sampling is a sampling technique in which some units of the population have zero chance of selection or where the probability of selection cannot be accurately determined. Typically, units are selected based on certain non-random criteria, such as quota or convenience. Because selection is non-random, non-probability sampling does not allow the estimation of sampling errors, and may be subjected to a sampling bias. Therefore, information from a sample cannot be generalised back to the population. Types of non-probability sampling techniques include:
Convenience sampling. Also called accidental or opportunity sampling, this is a technique in which a sample is drawn from that part of the population that is close to hand, readily available, or convenient. For instance, if you stand outside a shopping centre and hand out questionnaire surveys to people or interview them as they walk in, the sample of respondents you will obtain will be a convenience sample. This is a non-probability sample because you are systematically excluding all people who shop at other shopping centres. The opinions that you would get from your chosen sample may reflect the unique characteristics of this shopping centre such as the nature of its stores (e.g., high end-stores will attract a more affluent demographic), the demographic profile of its patrons, or its location (e.g., a shopping centre close to a university will attract primarily university students with unique purchasing habits), and therefore may not be representative of the opinions of the shopper population at large. Hence, the scientific generalisability of such observations will be very limited. Other examples of convenience sampling are sampling students registered in a certain class or sampling patients arriving at a certain medical clinic. This type of sampling is most useful for pilot testing, where the goal is instrument testing or measurement validation rather than obtaining generalisable inferences.
Quota sampling. In this technique, the population is segmented into mutually exclusive subgroups (just as in stratified sampling), and then a non-random set of observations is chosen from each subgroup to meet a predefined quota. In proportional quota sampling , the proportion of respondents in each subgroup should match that of the population. For instance, if the American population consists of 70 per cent Caucasians, 15 per cent Hispanic-Americans, and 13 per cent African-Americans, and you wish to understand their voting preferences in an sample of 98 people, you can stand outside a shopping centre and ask people their voting preferences. But you will have to stop asking Hispanic-looking people when you have 15 responses from that subgroup (or African-Americans when you have 13 responses) even as you continue sampling other ethnic groups, so that the ethnic composition of your sample matches that of the general American population.
Non-proportional quota sampling is less restrictive in that you do not have to achieve a proportional representation, but perhaps meet a minimum size in each subgroup. In this case, you may decide to have 50 respondents from each of the three ethnic subgroups (Caucasians, Hispanic-Americans, and African-Americans), and stop when your quota for each subgroup is reached. Neither type of quota sampling will be representative of the American population, since depending on whether your study was conducted in a shopping centre in New York or Kansas, your results may be entirely different. The non-proportional technique is even less representative of the population, but may be useful in that it allows capturing the opinions of small and under-represented groups through oversampling.
Expert sampling. This is a technique where respondents are chosen in a non-random manner based on their expertise on the phenomenon being studied. For instance, in order to understand the impacts of a new governmental policy such as the Sarbanes-Oxley Act, you can sample a group of corporate accountants who are familiar with this Act. The advantage of this approach is that since experts tend to be more familiar with the subject matter than non-experts, opinions from a sample of experts are more credible than a sample that includes both experts and non-experts, although the findings are still not generalisable to the overall population at large.
Snowball sampling. In snowball sampling, you start by identifying a few respondents that match the criteria for inclusion in your study, and then ask them to recommend others they know who also meet your selection criteria. For instance, if you wish to survey computer network administrators and you know of only one or two such people, you can start with them and ask them to recommend others who also work in network administration. Although this method hardly leads to representative samples, it may sometimes be the only way to reach hard-to-reach populations or when no sampling frame is available.
Statistics of sampling
In the preceding sections, we introduced terms such as population parameter, sample statistic, and sampling bias. In this section, we will try to understand what these terms mean and how they are related to each other.
When you measure a certain observation from a given unit, such as a person’s response to a Likert-scaled item, that observation is called a response (see Figure 8.2). In other words, a response is a measurement value provided by a sampled unit. Each respondent will give you different responses to different items in an instrument. Responses from different respondents to the same item or observation can be graphed into a frequency distribution based on their frequency of occurrences. For a large number of responses in a sample, this frequency distribution tends to resemble a bell-shaped curve called a normal distribution , which can be used to estimate overall characteristics of the entire sample, such as sample mean (average of all observations in a sample) or standard deviation (variability or spread of observations in a sample). These sample estimates are called sample statistics (a ‘statistic’ is a value that is estimated from observed data). Populations also have means and standard deviations that could be obtained if we could sample the entire population. However, since the entire population can never be sampled, population characteristics are always unknown, and are called population parameters (and not ‘statistic’ because they are not statistically estimated from data). Sample statistics may differ from population parameters if the sample is not perfectly representative of the population. The difference between the two is called sampling error . Theoretically, if we could gradually increase the sample size so that the sample approaches closer and closer to the population, then sampling error will decrease and a sample statistic will increasingly approximate the corresponding population parameter.
If a sample is truly representative of the population, then the estimated sample statistics should be identical to the corresponding theoretical population parameters. How do we know if the sample statistics are at least reasonably close to the population parameters? Here, we need to understand the concept of a sampling distribution . Imagine that you took three different random samples from a given population, as shown in Figure 8.3, and for each sample, you derived sample statistics such as sample mean and standard deviation. If each random sample was truly representative of the population, then your three sample means from the three random samples will be identical—and equal to the population parameter—and the variability in sample means will be zero. But this is extremely unlikely, given that each random sample will likely constitute a different subset of the population, and hence, their means may be slightly different from each other. However, you can take these three sample means and plot a frequency histogram of sample means. If the number of such samples increases from three to 10 to 100, the frequency histogram becomes a sampling distribution. Hence, a sampling distribution is a frequency distribution of a sample statistic (like sample mean) from a set of samples , while the commonly referenced frequency distribution is the distribution of a response (observation) from a single sample . Just like a frequency distribution, the sampling distribution will also tend to have more sample statistics clustered around the mean (which presumably is an estimate of a population parameter), with fewer values scattered around the mean. With an infinitely large number of samples, this distribution will approach a normal distribution. The variability or spread of a sample statistic in a sampling distribution (i.e., the standard deviation of a sampling statistic) is called its standard error . In contrast, the term standard deviation is reserved for variability of an observed response from a single sample.
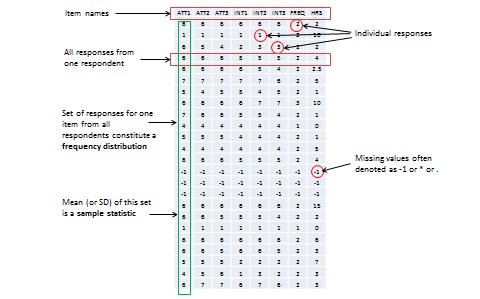
Social Science Research: Principles, Methods and Practices (Revised edition) Copyright © 2019 by Anol Bhattacherjee is licensed under a Creative Commons Attribution-NonCommercial-ShareAlike 4.0 International License , except where otherwise noted.
Share This Book
Have a language expert improve your writing
Run a free plagiarism check in 10 minutes, automatically generate references for free.
- Knowledge Base
- Methodology
- Population vs Sample | Definitions, Differences & Examples
Population vs Sample | Definitions, Differences & Examples
Published on 3 May 2022 by Pritha Bhandari . Revised on 5 December 2022.
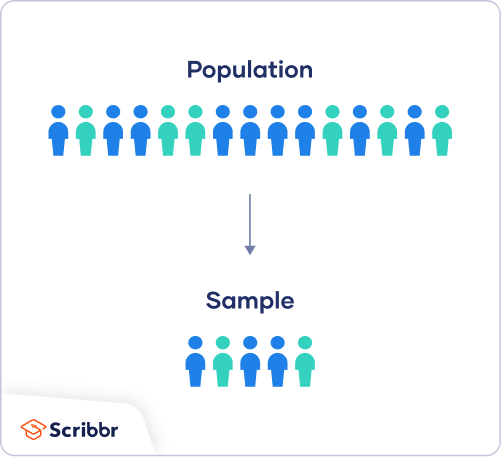
A population is the entire group that you want to draw conclusions about.
A sample is the specific group that you will collect data from. The size of the sample is always less than the total size of the population.
In research, a population doesn’t always refer to people. It can mean a group containing elements of anything you want to study, such as objects, events, organisations, countries, species, or organisms.
Table of contents
Collecting data from a population, collecting data from a sample, population parameter vs sample statistic, practice questions: populations vs samples, frequently asked questions about samples and populations.
Populations are used when your research question requires, or when you have access to, data from every member of the population.
Usually, it is only straightforward to collect data from a whole population when it is small, accessible and cooperative.
For larger and more dispersed populations, it is often difficult or impossible to collect data from every individual. For example, every 10 years, the federal US government aims to count every person living in the country using the US Census. This data is used to distribute funding across the nation.
However, historically, marginalised and low-income groups have been difficult to contact, locate, and encourage participation from. Because of non-responses, the population count is incomplete and biased towards some groups, which results in disproportionate funding across the country.
In cases like this, sampling can be used to make more precise inferences about the population.
Prevent plagiarism, run a free check.
When your population is large in size, geographically dispersed, or difficult to contact, it’s necessary to use a sample. With statistical analysis , you can use sample data to make estimates or test hypotheses about population data.
Ideally, a sample should be randomly selected and representative of the population. Using probability sampling methods (such as simple random sampling or stratified sampling ) reduces the risk of sampling bias and enhances both internal and external validity .
For practical reasons, researchers often use non-probability sampling methods . Non-probability samples are chosen for specific criteria; they may be more convenient or cheaper to access. Because of non-random selection methods, any statistical inferences about the broader population will be weaker than with a probability sample.
Reasons for sampling
- Necessity : Sometimes it’s simply not possible to study the whole population due to its size or inaccessibility.
- Practicality : It’s easier and more efficient to collect data from a sample.
- Cost-effectiveness : There are fewer participant, laboratory, equipment, and researcher costs involved.
- Manageability : Storing and running statistical analyses on smaller datasets is easier and reliable.
When you collect data from a population or a sample, there are various measurements and numbers you can calculate from the data. A parameter is a measure that describes the whole population. A statistic is a measure that describes the sample.
You can use estimation or hypothesis testing to estimate how likely it is that a sample statistic differs from the population parameter.
Sampling error
A sampling error is the difference between a population parameter and a sample statistic. In your study, the sampling error is the difference between the mean political attitude rating of your sample and the true mean political attitude rating of all undergraduate students in the Netherlands.
Sampling errors happen even when you use a randomly selected sample. This is because random samples are not identical to the population in terms of numerical measures like means and standard deviations .
Because the aim of scientific research is to generalise findings from the sample to the population, you want the sampling error to be low. You can reduce sampling error by increasing the sample size.
Samples are used to make inferences about populations . Samples are easier to collect data from because they are practical, cost-effective, convenient, and manageable.
Populations are used when a research question requires data from every member of the population. This is usually only feasible when the population is small and easily accessible.
A statistic refers to measures about the sample , while a parameter refers to measures about the population .
A sampling error is the difference between a population parameter and a sample statistic .
Cite this Scribbr article
If you want to cite this source, you can copy and paste the citation or click the ‘Cite this Scribbr article’ button to automatically add the citation to our free Reference Generator.
Bhandari, P. (2022, December 05). Population vs Sample | Definitions, Differences & Examples. Scribbr. Retrieved 20 March 2024, from https://www.scribbr.co.uk/research-methods/population-versus-sample/
Is this article helpful?
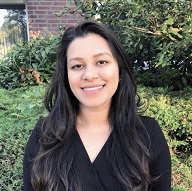
Pritha Bhandari
Other students also liked, sampling methods | types, techniques, & examples, a quick guide to experimental design | 5 steps & examples, what is quantitative research | definition & methods.
Introduction to Research Methods
7 samples and populations.
So you’ve developed your research question, figured out how you’re going to measure whatever you want to study, and have your survey or interviews ready to go. Now all your need is other people to become your data.
You might say ‘easy!’, there’s people all around you. You have a big family tree and surely them and their friends would have happy to take your survey. And then there’s your friends and people you’re in class with. Finding people is way easier than writing the interview questions or developing the survey. That reaction might be a strawman, maybe you’ve come to the conclusion none of this is easy. For your data to be valuable, you not only have to ask the right questions, you have to ask the right people. The “right people” aren’t the best or the smartest people, the right people are driven by what your study is trying to answer and the method you’re using to answer it.
Remember way back in chapter 2 when we looked at this chart and discussed the differences between qualitative and quantitative data.
One of the biggest differences between quantitative and qualitative data was whether we wanted to be able to explain something for a lot of people (what percentage of residents in Oklahoma support legalizing marijuana?) versus explaining the reasons for those opinions (why do some people support legalizing marijuana and others not?). The underlying differences there is whether our goal is explain something about everyone, or whether we’re content to explain it about just our respondents.
‘Everyone’ is called the population . The population in research is whatever group the research is trying to answer questions about. The population could be everyone on planet Earth, everyone in the United States, everyone in rural counties of Iowa, everyone at your university, and on and on. It is simply everyone within the unit you are intending to study.
In order to study the population, we typically take a sample or a subset. A sample is simply a smaller number of people from the population that are studied, which we can use to then understand the characteristics of the population based on that subset. That’s why a poll of 1300 likely voters can be used to guess at who will win your states Governor race. It isn’t perfect, and we’ll talk about the math behind all of it in a later chapter, but for now we’ll just focus on the different types of samples you might use to study a population with a survey.
If correctly sampled, we can use the sample to generalize information we get to the population. Generalizability , which we defined earlier, means we can assume the responses of people to our study match the responses everyone would have given us. We can only do that if the sample is representative of the population, meaning that they are alike on important characteristics such as race, gender, age, education. If something makes a large difference in people’s views on a topic in your research and your sample is not balanced, you’ll get inaccurate results.
Generalizability is more of a concern with surveys than with interviews. The goal of a survey is to explain something about people beyond the sample you get responses from. You’ll never see a news headline saying that “53% of 1250 Americans that responded to a poll approve of the President”. It’s only worth asking those 1250 people if we can assume the rest of the United States feels the same way overall. With interviews though we’re looking for depth from their responses, and so we are less hopefully that the 15 people we talk to will exactly match the American population. That doesn’t mean the data we collect from interviews doesn’t have value, it just has different uses.
There are two broad types of samples, with several different techniques clustered below those. Probability sampling is associated with surveys, and non-probability sampling is often used when conducting interviews. We’ll first describe probability samples, before discussing the non-probability options.
The type of sampling you’ll use will be based on the type of research you’re intending to do. There’s no sample that’s right or wrong, they can just be more or less appropriate for the question you’re trying to answer. And if you use a less appropriate sampling strategy, the answer you get through your research is less likely to be accurate.
7.1 Types of Probability Samples
So we just hinted at the idea that depending on the sample you use, you can generalize the data you collect from the sample to the population. That will depend though on whether your sample represents the population. To ensure that your sample is representative of the population, you will want to use a probability sample. A representative sample refers to whether the characteristics (race, age, income, education, etc) of the sample are the same as the population. Probability sampling is a sampling technique in which every individual in the population has an equal chance of being selected as a subject for the research.
There are several different types of probability samples you can use, depending on the resources you have available.
Let’s start with a simple random sample . In order to use a simple random sample all you have to do is take everyone in your population, throw them in a hat (not literally, you can just throw their names in a hat), and choose the number of names you want to use for your sample. By drawing blindly, you can eliminate human bias in constructing the sample and your sample should represent the population from which it is being taken.
However, a simple random sample isn’t quite that easy to build. The biggest issue is that you have to know who everyone is in order to randomly select them. What that requires is a sampling frame , a list of all residents in the population. But we don’t always have that. There is no list of residents of New York City (or any other city). Organizations that do have such a list wont just give it away. Try to ask your university for a list and contact information of everyone at your school so you can do a survey? They wont give it to you, for privacy reasons. It’s actually harder to think of popultions you could easily develop a sample frame for than those you can’t. If you can get or build a sampling frame, the work of a simple random sample is fairly simple, but that’s the biggest challenge.
Most of the time a true sampling frame is impossible to acquire, so researcher have to settle for something approximating a complete list. Earlier generations of researchers could use the random dial method to contact a random sample of Americans, because every household had a single phone. To use it you just pick up the phone and dial random numbers. Assuming the numbers are actually random, anyone might be called. That method actually worked somewhat well, until people stopped having home phone numbers and eventually stopped answering the phone. It’s a fun mental exercise to think about how you would go about creating a sampling frame for different groups though; think through where you would look to find a list of everyone in these groups:
Plumbers Recent first-time fathers Members of gyms
The best way to get an actual sampling frame is likely to purchase one from a private company that buys data on people from all the different websites we use.
Let’s say you do have a sampling frame though. For instance, you might be hired to do a survey of members of the Republican Party in the state of Utah to understand their political priorities this year, and the organization could give you a list of their members because they’ve hired you to do the reserach. One method of constructing a simple random sample would be to assign each name on the list a number, and then produce a list of random numbers. Once you’ve matched the random numbers to the list, you’ve got your sample. See the example using the list of 20 names below

and the list of 5 random numbers.

Systematic sampling is similar to simple random sampling in that it begins with a list of the population, but instead of choosing random numbers one would select every kth name on the list. What the heck is a kth? K just refers to how far apart the names are on the list you’re selecting. So if you want to sample one-tenth of the population, you’d select every tenth name. In order to know the k for your study you need to know your sample size (say 1000) and the size of the population (75000). You can divide the size of the population by the sample (75000/1000), which will produce your k (750). As long as the list does not contain any hidden order, this sampling method is as good as the random sampling method, but its only advantage over the random sampling technique is simplicity. If we used the same list as above and wanted to survey 1/5th of the population, we’d include 4 of the names on the list. It’s important with systematic samples to randomize the starting point in the list, otherwise people with A names will be oversampled. If we started with the 3rd name, we’d select Annabelle Frye, Cristobal Padilla, Jennie Vang, and Virginia Guzman, as shown below. So in order to use a systematic sample, we need three things, the population size (denoted as N ), the sample size we want ( n ) and k , which we calculate by dividing the population by the sample).
N= 20 (Population Size) n= 4 (Sample Size) k= 5 {20/4 (kth element) selection interval}

We can also use a stratified sample , but that requires knowing more about the population than just their names. A stratified sample divides the study population into relevant subgroups, and then draws a sample from each subgroup. Stratified sampling can be used if you’re very concerned about ensuring balance in the sample or there may be a problem of underrepresentation among certain groups when responses are received. Not everyone in your sample is equally likely to answer a survey. Say for instance we’re trying to predict who will win an election in a county with three cities. In city A there are 1 million college students, in city B there are 2 million families, and in City C there are 3 million retirees. You know that retirees are more likely than busy college students or parents to respond to a poll. So you break the sample into three parts, ensuring that you get 100 responses from City A, 200 from City B, and 300 from City C, so the three cities would match the population. A stratified sample provides the researcher control over the subgroups that are included in the sample, whereas simple random sampling does not guarantee that any one type of person will be included in the final sample. A disadvantage is that it is more complex to organize and analyze the results compared to simple random sampling.
Cluster sampling is an approach that begins by sampling groups (or clusters) of population elements and then selects elements from within those groups. A researcher would use cluster sampling if getting access to elements in an entrie population is too challenging. For instance, a study on students in schools would probably benefit from randomly selecting from all students at the 36 elementary schools in a fictional city. But getting contact information for all students would be very difficult. So the researcher might work with principals at several schools and survey those students. The researcher would need to ensure that the students surveyed at the schools are similar to students throughout the entire city, and greater access and participation within each cluster may make that possible.
The image below shows how this can work, although the example is oversimplified. Say we have 12 students that are in 6 classrooms. The school is in total 1/4th green (3/12), 1/4th yellow (3/12), and half blue (6/12). By selecting the right clusters from within the school our sample can be representative of the entire school, assuming these colors are the only significant difference between the students. In the real world, you’d want to match the clusters and population based on race, gender, age, income, etc. And I should point out that this is an overly simplified example. What if 5/12s of the school was yellow and 1/12th was green, how would I get the right proportions? I couldn’t, but you’d do the best you could. You still wouldn’t want 4 yellows in the sample, you’d just try to approximiate the population characteristics as best you can.

7.2 Actually Doing a Survey
All of that probably sounds pretty complicated. Identifying your population shouldn’t be too difficult, but how would you ever get a sampling frame? And then actually identifying who to include… It’s probably a bit overwhelming and makes doing a good survey sound impossible.
Researchers using surveys aren’t superhuman though. Often times, they use a little help. Because surveys are really valuable, and because researchers rely on them pretty often, there has been substantial growth in companies that can help to get one’s survey to its intended audience.
One popular resource is Amazon’s Mechanical Turk (more commonly known as MTurk). MTurk is at its most basic a website where workers look for jobs (called hits) to be listed by employers, and choose whether to do the task or not for a set reward. MTurk has grown over the last decade to be a common source of survey participants in the social sciences, in part because hiring workers costs very little (you can get some surveys completed for penny’s). That means you can get your survey completed with a small grant ($1-2k at the low end) and get the data back in a few hours. Really, it’s a quick and easy way to run a survey.
However, the workers aren’t perfectly representative of the average American. For instance, researchers have found that MTurk respondents are younger, better educated, and earn less than the average American.
One way to get around that issue, which can be used with MTurk or any survey, is to weight the responses. Because with MTurk you’ll get fewer responses from older, less educated, and richer Americans, those responses you do give you want to count for more to make your sample more representative of the population. Oversimplified example incoming!
Imagine you’re setting up a pizza party for your class. There are 9 people in your class, 4 men and 5 women. You only got 4 responses from the men, and 3 from the women. All 4 men wanted peperoni pizza, while the 3 women want a combination. Pepperoni wins right, 4 to 3? Not if you assume that the people that didn’t respond are the same as the ones that did. If you weight the responses to match the population (the full class of 9), a combination pizza is the winner.

Because you know the population of women is 5, you can weight the 3 responses from women by 5/3 = 1.6667. If we weight (or multiply) each vote we did receive from a woman by 1.6667, each vote for a combination now equals 1.6667, meaning that the 3 votes for combination total 5. Because we received a vote from every man in the class, we just weight their votes by 1. The big assumption we have to make is that the people we didn’t hear from (the 2 women that didn’t vote) are similar to the ones we did hear from. And if we don’t get any responses from a group we don’t have anything to infer their preferences or views from.
Let’s go through a slightly more complex example, still just considering one quality about people in the class. Let’s say your class actually has 100 students, but you only received votes from 50. And, what type of pizza people voted for is mixed, but men still prefer peperoni overall, and women still prefer combination. The class is 60% female and 40% male.
We received 21 votes from women out of the 60, so we can weight their responses by 60/21 to represent the population. We got 29 votes out of the 40 for men, so their responses can be weighted by 40/29. See the math below.

53.8 votes for combination? That might seem a little odd, but weighting isn’t a perfect science. We can’t identify what a non-respondent would have said exactly, all we can do is use the responses of other similar people to make a good guess. That issue often comes up in polling, where pollsters have to guess who is going to vote in a given election in order to project who will win. And we can weight on any characteristic of a person we think will be important, alone or in combination. Modern polls weight on age, gender, voting habits, education, and more to make the results as generalizable as possible.
There’s an appendix later in this book where I walk through the actual steps of creating weights for a sample in R, if anyone actually does a survey. I intended this section to show that doing a good survey might be simpler than it seemed, but now it might sound even more difficult. A good lesson to take though is that there’s always another door to go through, another hurdle to improve your methods. Being good at research just means being constantly prepared to be given a new challenge, and being able to find another solution.
7.3 Non-Probability Sampling
Qualitative researchers’ main objective is to gain an in-depth understanding on the subject matter they are studying, rather than attempting to generalize results to the population. As such, non-probability sampling is more common because of the researchers desire to gain information not from random elements of the population, but rather from specific individuals.
Random selection is not used in nonprobability sampling. Instead, the personal judgment of the researcher determines who will be included in the sample. Typically, researchers may base their selection on availability, quotas, or other criteria. However, not all members of the population are given an equal chance to be included in the sample. This nonrandom approach results in not knowing whether the sample represents the entire population. Consequently, researchers are not able to make valid generalizations about the population.
As with probability sampling, there are several types of non-probability samples. Convenience sampling , also known as accidental or opportunity sampling, is a process of choosing a sample that is easily accessible and readily available to the researcher. Researchers tend to collect samples from convenient locations such as their place of employment, a location, school, or other close affiliation. Although this technique allows for quick and easy access to available participants, a large part of the population is excluded from the sample.
For example, researchers (particularly in psychology) often rely on research subjects that are at their universities. That is highly convenient, students are cheap to hire and readily available on campuses. However, it means the results of the study may have limited ability to predict motivations or behaviors of people that aren’t included in the sample, i.e., people outside the age of 18-22 that are going to college.
If I ask you to get find out whether people approve of the mayor or not, and tell you I want 500 people’s opinions, should you go stand in front of the local grocery store? That would be convinient, and the people coming will be random, right? Not really. If you stand outside a rural Piggly Wiggly or an urban Whole Foods, do you think you’ll see the same people? Probably not, people’s chracteristics make the more or less likely to be in those locations. This technique runs the high risk of over- or under-representation, biased results, as well as an inability to make generalizations about the larger population. As the name implies though, it is convenient.
Purposive sampling , also known as judgmental or selective sampling, refers to a method in which the researcher decides who will be selected for the sample based on who or what is relevant to the study’s purpose. The researcher must first identify a specific characteristic of the population that can best help answer the research question. Then, they can deliberately select a sample that meets that particular criterion. Typically, the sample is small with very specific experiences and perspectives. For instance, if I wanted to understand the experiences of prominent foreign-born politicians in the United States, I would purposefully build a sample of… prominent foreign-born politicians in the United States. That would exclude anyone that was born in the United States or and that wasn’t a politician, and I’d have to define what I meant by prominent. Purposive sampling is susceptible to errors in judgment by the researcher and selection bias due to a lack of random sampling, but when attempting to research small communities it can be effective.
When dealing with small and difficult to reach communities researchers sometimes use snowball samples , also known as chain referral sampling. Snowball sampling is a process in which the researcher selects an initial participant for the sample, then asks that participant to recruit or refer additional participants who have similar traits as them. The cycle continues until the needed sample size is obtained.
This technique is used when the study calls for participants who are hard to find because of a unique or rare quality or when a participant does not want to be found because they are part of a stigmatized group or behavior. Examples may include people with rare diseases, sex workers, or a child sex offenders. It would be impossible to find an accurate list of sex workers anywhere, and surveying the general population about whether that is their job will produce false responses as people will be unwilling to identify themselves. As such, a common method is to gain the trust of one individual within the community, who can then introduce you to others. It is important that the researcher builds rapport and gains trust so that participants can be comfortable contributing to the study, but that must also be balanced by mainting objectivity in the research.
Snowball sampling is a useful method for locating hard to reach populations but cannot guarantee a representative sample because each contact will be based upon your last. For instance, let’s say you’re studying illegal fight clubs in your state. Some fight clubs allow weapons in the fights, while others completely ban them; those two types of clubs never interreact because of their disagreement about whether weapons should be allowed, and there’s no overlap between them (no members in both type of club). If your initial contact is with a club that uses weapons, all of your subsequent contacts will be within that community and so you’ll never understand the differences. If you didn’t know there were two types of clubs when you started, you’ll never even know you’re only researching half of the community. As such, snowball sampling can be a necessary technique when there are no other options, but it does have limitations.
Quota Sampling is a process in which the researcher must first divide a population into mutually exclusive subgroups, similar to stratified sampling. Depending on what is relevant to the study, subgroups can be based on a known characteristic such as age, race, gender, etc. Secondly, the researcher must select a sample from each subgroup to fit their predefined quotas. Quota sampling is used for the same reason as stratified sampling, to ensure that your sample has representation of certain groups. For instance, let’s say that you’re studying sexual harassment in the workplace, and men are much more willing to discuss their experiences than women. You might choose to decide that half of your final sample will be women, and stop requesting interviews with men once you fill your quota. The core difference is that while stratified sampling chooses randomly from within the different groups, quota sampling does not. A quota sample can either be proportional or non-proportional . Proportional quota sampling refers to ensuring that the quotas in the sample match the population (if 35% of the company is female, 35% of the sample should be female). Non-proportional sampling allows you to select your own quota sizes. If you think the experiences of females with sexual harassment are more important to your research, you can include whatever percentage of females you desire.
7.4 Dangers in sampling
Now that we’ve described all the different ways that one could create a sample, we can talk more about the pitfalls of sampling. Ensuring a quality sample means asking yourself some basic questions:
- Who is in the sample?
- How were they sampled?
- Why were they sampled?
A meal is often only as good as the ingredients you use, and your data will only be as good as the sample. If you collect data from the wrong people, you’ll get the wrong answer. You’ll still get an answer, it’ll just be inaccurate. And I want to reemphasize here wrong people just refers to inappropriate for your study. If I want to study bullying in middle schools, but I only talk to people that live in a retirement home, how accurate or relevant will the information I gather be? Sure, they might have grandchildren in middle school, and they may remember their experiences. But wouldn’t my information be more relevant if I talked to students in middle school, or perhaps a mix of teachers, parents, and students? I’ll get an answer from retirees, but it wont be the one I need. The sample has to be appropriate to the research question.
Is a bigger sample always better? Not necessarily. A larger sample can be useful, but a more representative one of the population is better. That was made painfully clear when the magazine Literary Digest ran a poll to predict who would win the 1936 presidential election between Alf Landon and incumbent Franklin Roosevelt. Literary Digest had run the poll since 1916, and had been correct in predicting the outcome every time. It was the largest poll ever, and they received responses for 2.27 million people. They essentially received responses from 1 percent of the American population, while many modern polls use only 1000 responses for a much more populous country. What did they predict? They showed that Alf Landon would be the overwhelming winner, yet when the election was held Roosevelt won every state except Maine and Vermont. It was one of the most decisive victories in Presidential history.
So what went wrong for the Literary Digest? Their poll was large (gigantic!), but it wasn’t representative of likely voters. They polled their own readership, which tended to be more educated and wealthy on average, along with people on a list of those with registered automobiles and telephone users (both of which tended to be owned by the wealthy at that time). Thus, the poll largely ignored the majority of Americans, who ended up voting for Roosevelt. The Literary Digest poll is famous for being wrong, but led to significant improvements in the science of polling to avoid similar mistakes in the future. Researchers have learned a lot in the century since that mistake, even if polling and surveys still aren’t (and can’t be) perfect.
What kind of sampling strategy did Literary Digest use? Convenience, they relied on lists they had available, rather than try to ensure every American was included on their list. A representative poll of 2 million people will give you more accurate results than a representative poll of 2 thousand, but I’ll take the smaller more representative poll than a larger one that uses convenience sampling any day.
7.5 Summary
Picking the right type of sample is critical to getting an accurate answer to your reserach question. There are a lot of differnet options in how you can select the people to participate in your research, but typically only one that is both correct and possible depending on the research you’re doing. In the next chapter we’ll talk about a few other methods for conducting reseach, some that don’t include any sampling by you.
- Privacy Policy
Buy Me a Coffee

Home » Sampling Methods – Types, Techniques and Examples
Sampling Methods – Types, Techniques and Examples
Table of Contents

Sampling refers to the process of selecting a subset of data from a larger population or dataset in order to analyze or make inferences about the whole population.
In other words, sampling involves taking a representative sample of data from a larger group or dataset in order to gain insights or draw conclusions about the entire group.
Sampling Methods
Sampling methods refer to the techniques used to select a subset of individuals or units from a larger population for the purpose of conducting statistical analysis or research.
Sampling is an essential part of the Research because it allows researchers to draw conclusions about a population without having to collect data from every member of that population, which can be time-consuming, expensive, or even impossible.
Types of Sampling Methods
Sampling can be broadly categorized into two main categories:
Probability Sampling
This type of sampling is based on the principles of random selection, and it involves selecting samples in a way that every member of the population has an equal chance of being included in the sample.. Probability sampling is commonly used in scientific research and statistical analysis, as it provides a representative sample that can be generalized to the larger population.
Type of Probability Sampling :
- Simple Random Sampling: In this method, every member of the population has an equal chance of being selected for the sample. This can be done using a random number generator or by drawing names out of a hat, for example.
- Systematic Sampling: In this method, the population is first divided into a list or sequence, and then every nth member is selected for the sample. For example, if every 10th person is selected from a list of 100 people, the sample would include 10 people.
- Stratified Sampling: In this method, the population is divided into subgroups or strata based on certain characteristics, and then a random sample is taken from each stratum. This is often used to ensure that the sample is representative of the population as a whole.
- Cluster Sampling: In this method, the population is divided into clusters or groups, and then a random sample of clusters is selected. Then, all members of the selected clusters are included in the sample.
- Multi-Stage Sampling : This method combines two or more sampling techniques. For example, a researcher may use stratified sampling to select clusters, and then use simple random sampling to select members within each cluster.
Non-probability Sampling
This type of sampling does not rely on random selection, and it involves selecting samples in a way that does not give every member of the population an equal chance of being included in the sample. Non-probability sampling is often used in qualitative research, where the aim is not to generalize findings to a larger population, but to gain an in-depth understanding of a particular phenomenon or group. Non-probability sampling methods can be quicker and more cost-effective than probability sampling methods, but they may also be subject to bias and may not be representative of the larger population.
Types of Non-probability Sampling :
- Convenience Sampling: In this method, participants are chosen based on their availability or willingness to participate. This method is easy and convenient but may not be representative of the population.
- Purposive Sampling: In this method, participants are selected based on specific criteria, such as their expertise or knowledge on a particular topic. This method is often used in qualitative research, but may not be representative of the population.
- Snowball Sampling: In this method, participants are recruited through referrals from other participants. This method is often used when the population is hard to reach, but may not be representative of the population.
- Quota Sampling: In this method, a predetermined number of participants are selected based on specific criteria, such as age or gender. This method is often used in market research, but may not be representative of the population.
- Volunteer Sampling: In this method, participants volunteer to participate in the study. This method is often used in research where participants are motivated by personal interest or altruism, but may not be representative of the population.
Applications of Sampling Methods
Applications of Sampling Methods from different fields:
- Psychology : Sampling methods are used in psychology research to study various aspects of human behavior and mental processes. For example, researchers may use stratified sampling to select a sample of participants that is representative of the population based on factors such as age, gender, and ethnicity. Random sampling may also be used to select participants for experimental studies.
- Sociology : Sampling methods are commonly used in sociological research to study social phenomena and relationships between individuals and groups. For example, researchers may use cluster sampling to select a sample of neighborhoods to study the effects of economic inequality on health outcomes. Stratified sampling may also be used to select a sample of participants that is representative of the population based on factors such as income, education, and occupation.
- Social sciences: Sampling methods are commonly used in social sciences to study human behavior and attitudes. For example, researchers may use stratified sampling to select a sample of participants that is representative of the population based on factors such as age, gender, and income.
- Marketing : Sampling methods are used in marketing research to collect data on consumer preferences, behavior, and attitudes. For example, researchers may use random sampling to select a sample of consumers to participate in a survey about a new product.
- Healthcare : Sampling methods are used in healthcare research to study the prevalence of diseases and risk factors, and to evaluate interventions. For example, researchers may use cluster sampling to select a sample of health clinics to participate in a study of the effectiveness of a new treatment.
- Environmental science: Sampling methods are used in environmental science to collect data on environmental variables such as water quality, air pollution, and soil composition. For example, researchers may use systematic sampling to collect soil samples at regular intervals across a field.
- Education : Sampling methods are used in education research to study student learning and achievement. For example, researchers may use stratified sampling to select a sample of schools that is representative of the population based on factors such as demographics and academic performance.
Examples of Sampling Methods
Probability Sampling Methods Examples:
- Simple random sampling Example : A researcher randomly selects participants from the population using a random number generator or drawing names from a hat.
- Stratified random sampling Example : A researcher divides the population into subgroups (strata) based on a characteristic of interest (e.g. age or income) and then randomly selects participants from each subgroup.
- Systematic sampling Example : A researcher selects participants at regular intervals from a list of the population.
Non-probability Sampling Methods Examples:
- Convenience sampling Example: A researcher selects participants who are conveniently available, such as students in a particular class or visitors to a shopping mall.
- Purposive sampling Example : A researcher selects participants who meet specific criteria, such as individuals who have been diagnosed with a particular medical condition.
- Snowball sampling Example : A researcher selects participants who are referred to them by other participants, such as friends or acquaintances.
How to Conduct Sampling Methods
some general steps to conduct sampling methods:
- Define the population: Identify the population of interest and clearly define its boundaries.
- Choose the sampling method: Select an appropriate sampling method based on the research question, characteristics of the population, and available resources.
- Determine the sample size: Determine the desired sample size based on statistical considerations such as margin of error, confidence level, or power analysis.
- Create a sampling frame: Develop a list of all individuals or elements in the population from which the sample will be drawn. The sampling frame should be comprehensive, accurate, and up-to-date.
- Select the sample: Use the chosen sampling method to select the sample from the sampling frame. The sample should be selected randomly, or if using a non-random method, every effort should be made to minimize bias and ensure that the sample is representative of the population.
- Collect data: Once the sample has been selected, collect data from each member of the sample using appropriate research methods (e.g., surveys, interviews, observations).
- Analyze the data: Analyze the data collected from the sample to draw conclusions about the population of interest.
When to use Sampling Methods
Sampling methods are used in research when it is not feasible or practical to study the entire population of interest. Sampling allows researchers to study a smaller group of individuals, known as a sample, and use the findings from the sample to make inferences about the larger population.
Sampling methods are particularly useful when:
- The population of interest is too large to study in its entirety.
- The cost and time required to study the entire population are prohibitive.
- The population is geographically dispersed or difficult to access.
- The research question requires specialized or hard-to-find individuals.
- The data collected is quantitative and statistical analyses are used to draw conclusions.
Purpose of Sampling Methods
The main purpose of sampling methods in research is to obtain a representative sample of individuals or elements from a larger population of interest, in order to make inferences about the population as a whole. By studying a smaller group of individuals, known as a sample, researchers can gather information about the population that would be difficult or impossible to obtain from studying the entire population.
Sampling methods allow researchers to:
- Study a smaller, more manageable group of individuals, which is typically less time-consuming and less expensive than studying the entire population.
- Reduce the potential for data collection errors and improve the accuracy of the results by minimizing sampling bias.
- Make inferences about the larger population with a certain degree of confidence, using statistical analyses of the data collected from the sample.
- Improve the generalizability and external validity of the findings by ensuring that the sample is representative of the population of interest.
Characteristics of Sampling Methods
Here are some characteristics of sampling methods:
- Randomness : Probability sampling methods are based on random selection, meaning that every member of the population has an equal chance of being selected. This helps to minimize bias and ensure that the sample is representative of the population.
- Representativeness : The goal of sampling is to obtain a sample that is representative of the larger population of interest. This means that the sample should reflect the characteristics of the population in terms of key demographic, behavioral, or other relevant variables.
- Size : The size of the sample should be large enough to provide sufficient statistical power for the research question at hand. The sample size should also be appropriate for the chosen sampling method and the level of precision desired.
- Efficiency : Sampling methods should be efficient in terms of time, cost, and resources required. The method chosen should be feasible given the available resources and time constraints.
- Bias : Sampling methods should aim to minimize bias and ensure that the sample is representative of the population of interest. Bias can be introduced through non-random selection or non-response, and can affect the validity and generalizability of the findings.
- Precision : Sampling methods should be precise in terms of providing estimates of the population parameters of interest. Precision is influenced by sample size, sampling method, and level of variability in the population.
- Validity : The validity of the sampling method is important for ensuring that the results obtained from the sample are accurate and can be generalized to the population of interest. Validity can be affected by sampling method, sample size, and the representativeness of the sample.
Advantages of Sampling Methods
Sampling methods have several advantages, including:
- Cost-Effective : Sampling methods are often much cheaper and less time-consuming than studying an entire population. By studying only a small subset of the population, researchers can gather valuable data without incurring the costs associated with studying the entire population.
- Convenience : Sampling methods are often more convenient than studying an entire population. For example, if a researcher wants to study the eating habits of people in a city, it would be very difficult and time-consuming to study every single person in the city. By using sampling methods, the researcher can obtain data from a smaller subset of people, making the study more feasible.
- Accuracy: When done correctly, sampling methods can be very accurate. By using appropriate sampling techniques, researchers can obtain a sample that is representative of the entire population. This allows them to make accurate generalizations about the population as a whole based on the data collected from the sample.
- Time-Saving: Sampling methods can save a lot of time compared to studying the entire population. By studying a smaller sample, researchers can collect data much more quickly than they could if they studied every single person in the population.
- Less Bias : Sampling methods can reduce bias in a study. If a researcher were to study the entire population, it would be very difficult to eliminate all sources of bias. However, by using appropriate sampling techniques, researchers can reduce bias and obtain a sample that is more representative of the entire population.
Limitations of Sampling Methods
- Sampling Error : Sampling error is the difference between the sample statistic and the population parameter. It is the result of selecting a sample rather than the entire population. The larger the sample, the lower the sampling error. However, no matter how large the sample size, there will always be some degree of sampling error.
- Selection Bias: Selection bias occurs when the sample is not representative of the population. This can happen if the sample is not selected randomly or if some groups are underrepresented in the sample. Selection bias can lead to inaccurate conclusions about the population.
- Non-response Bias : Non-response bias occurs when some members of the sample do not respond to the survey or study. This can result in a biased sample if the non-respondents differ from the respondents in important ways.
- Time and Cost : While sampling can be cost-effective, it can still be expensive and time-consuming to select a sample that is representative of the population. Depending on the sampling method used, it may take a long time to obtain a sample that is large enough and representative enough to be useful.
- Limited Information : Sampling can only provide information about the variables that are measured. It may not provide information about other variables that are relevant to the research question but were not measured.
- Generalization : The extent to which the findings from a sample can be generalized to the population depends on the representativeness of the sample. If the sample is not representative of the population, it may not be possible to generalize the findings to the population as a whole.
About the author
Muhammad Hassan
Researcher, Academic Writer, Web developer
You may also like

Stratified Random Sampling – Definition, Method...

Purposive Sampling – Methods, Types and Examples

Non-probability Sampling – Types, Methods and...

Cluster Sampling – Types, Method and Examples

Systematic Sampling – Types, Method and Examples

Snowball Sampling – Method, Types and Examples
Thank you for visiting nature.com. You are using a browser version with limited support for CSS. To obtain the best experience, we recommend you use a more up to date browser (or turn off compatibility mode in Internet Explorer). In the meantime, to ensure continued support, we are displaying the site without styles and JavaScript.
- View all journals
- Explore content
- About the journal
- Publish with us
- Sign up for alerts
- Perspective
- Published: 22 March 2024
Recommendations for the responsible use and communication of race and ethnicity in neuroimaging research
- Carlos Cardenas-Iniguez ORCID: orcid.org/0000-0002-6736-3020 1 &
- Marybel Robledo Gonzalez 2
Nature Neuroscience ( 2024 ) Cite this article
Metrics details
- Cognitive neuroscience
- Research data
The growing availability of large-population human biomedical datasets provides researchers with unique opportunities to conduct rigorous and impactful studies on brain and behavioral development, allowing for a more comprehensive understanding of neurodevelopment in diverse populations. However, the patterns observed in these datasets are more likely to be influenced by upstream structural inequities (that is, structural racism), which can lead to health disparities based on race, ethnicity and social class. This paper addresses the need for guidance and self-reflection in biomedical research on conceptualizing, contextualizing and communicating issues related to race and ethnicity. We provide recommendations as a starting point for researchers to rethink race and ethnicity choices in study design, model specification, statistical analysis and communication of results, implement practices to avoid the further stigmatization of historically minoritized groups, and engage in research practices that counteract existing harmful biases.
This is a preview of subscription content, access via your institution
Access options
Access Nature and 54 other Nature Portfolio journals
Get Nature+, our best-value online-access subscription
24,99 € / 30 days
cancel any time
Subscribe to this journal
Receive 12 print issues and online access
195,33 € per year
only 16,28 € per issue
Rent or buy this article
Prices vary by article type
Prices may be subject to local taxes which are calculated during checkout
Laird, A. R. Large, open datasets for human connectomics research: considerations for reproducible and responsible data use. NeuroImage 244 , 118579 (2021).
Article PubMed Google Scholar
Bailey, Z. D. et al. Structural racism and health inequities in the USA: evidence and interventions. Lancet 389 , 1453–1463 (2017).
Graves, J. L. Jr & Goodman, A. H. Racism, Not Race: Answers to Frequently Asked Questions (Columbia Univ. Press, 2021).
Müller, R. et al. Next steps for global collaboration to minimize racial and ethnic bias in neuroscience. Nat. Neurosci . https://doi.org/10.1038/s41593-023-01369-6 (2023).
Nature. Why Nature is updating its advice to authors on reporting race or ethnicity. Nature 616 , 219–219 (2023).
Abiodun, S. J. ‘Seeing color,’ a discussion of the implications and applications of race in the field of neuroscience. Front. Hum. Neurosci. 13 , 280 (2019).
Article PubMed PubMed Central Google Scholar
Girolamo, T., Parker, T. C. & Eigsti, I. -M. Incorporating dis/ability studies and critical race theory to combat systematic exclusion of black, indigenous, and people of color in clinical neuroscience. Front. Neurosci. 16 , 988092 (2022).
Green, K. H. et al. A perspective on enhancing representative samples in developmental human neuroscience: connecting science to society. Front. Integr. Neurosci. 16 , 981657 (2022).
Rebello, V. & Uban, K. A. A call to leverage a health equity lens to accelerate human neuroscience research. Front. Integr. Neurosci. 17 , 1035597 (2023).
Ricard, J. A. et al. Confronting racially exclusionary practices in the acquisition and analyses of neuroimaging data. Nat. Neurosci. 26 , 4–11 (2023).
Article CAS PubMed Google Scholar
Rollins, O. Towards an antiracist (neuro)science. Nat. Hum. Behav. 5 , 540–541 (2021).
Webb, E. K., Cardenas-Iniguez, C. & Douglas, R. Radically reframing studies on neurobiology and socioeconomic circumstances: a call for social justice-oriented neuroscience. Front. Integr. Neurosci. 16 , 958545 (2022).
Okun, T. White Supremacy Culture—Still Here https://www.dismantlingracism.org/uploads/4/3/5/7/43579015/okun_-_white_sup_culture.pdf (2021).
Valles, S. Philosophy of Population Health: Philosophy for a New Public Health Era (Routledge, 2018).
Morning, A. Ethnic classification in global perspective: a cross-national survey of the 2000 Census round. Popul. Res. Policy Rev. 27 , 239–272 (2008).
Article Google Scholar
Roberts, D. Fatal Invention: How Science, Politics, and Big Business Re-Create Race in the Twenty-First Century (New Press/ORIM, 2011). This book provides a rich account of how racial categories have been created and maintained in the United States, and how they have been used in biomedical research .
Pitts-Taylor, V. Neurobiologically poor? Brain phenotypes, inequality, and biosocial determinism. Sci. Technol. Hum. Values 44 , 660–685 (2019).
Rogers, L. O., Niwa, E. Y., Chung, K., Yip, T. & Chae, D. M(ai)cro: centering the macrosystem in human development. Hum. Dev. 65 , 270–292 (2021).
Dennis, A. C., Chung, E. O., Lodge, E. K., Martinez, R. A. & Wilbur, R. E. Looking back to leap forward: a framework for operationalizing the structural racism construct in minority and immigrant health research. Ethn. Dis. 31 , 301–310 (2021).
Goldfarb, M. G. & Brown, D. R. Diversifying participation: the rarity of reporting racial demographics in neuroimaging research. NeuroImage 254 , 119122 (2022).
Sterling, E., Pearl, H., Liu, Z., Allen, J. W. & Fleischer, C. C. Demographic reporting across a decade of neuroimaging: a systematic review. Brain Imaging Behav. 16 , 2785–2796 (2022).
Taylor, L. & Rommelfanger, K. S. Mitigating white Western individualistic bias and creating more inclusive neuroscience. Nat. Rev. Neurosci. 23 , 389–390 (2022).
Atkin, A. L. et al. Race terminology in the field of psychology: acknowledging the growing multiracial population in the US. Am. Psychol. 77 , 381–393 (2022).
Balestra, C. & Fleischer, L. Diversity statistics in the OECD: how do OECD countries collect data on ethnic, racial and indigenous identity? OECD https://doi.org/10.1787/89bae654-en (2018).
American Psychological Association. APA Guidelines on Race and Ethnicity in Psychology: Promoting Responsiveness and Equity https://www.apa.org/about/policy/guidelines-race-ethnicity.pdf (2019).
Flanagin, A., Frey, T. & Christiansen, S. L., AMA Manual of Style Committee. Updated guidance on the reporting of race and ethnicity in medical and science journals. JAMA 326 , 621–627 (2021).
Duggan, C. P., Kurpad, A., Stanford, F. C., Sunguya, B. & Wells, J. C. Race, ethnicity, and racism in the nutrition literature: an update for 2020. Am. J. Clin. Nutr. 112 , 1409–1414 (2020).
Krieger, N. Structural racism, health inequities, and the two-edged sword of data: structural problems require structural solutions. Front. Public Health 9 , 655447 (2021).
Saini, A. Superior: the Return of Race Science (Press, 2019).
Zuberi, T. & Bonilla-Silva, E. White Logic, White Methods: Racism and Methodology (Rowman & Littlefield, 2008).
Fan, C. C. et al. Adolescent Brain Cognitive Development (ABCD) study Linked External Data (LED): Protocol and practices for geocoding and assignment of environmental data. Dev. Cogn. Neurosci. 52 , 101030 (2021).
Cardenas-Iniguez, C. et al. Building towards an adolescent neural urbanome: expanding environmental measures using linked external data (LED) in the ABCD Study. Dev. Cogn. Neurosci . https://doi.org/10.1016/j.dcn.2023.101338 (2024). This article provides a comprehensive review of many variables that can be used to directly measure social and physical environments, instead of using race and ethnicity as proxies .
Gonzalez, M. R. et al. Positive economic, psychosocial, and physiological ecologies predict brain structure and cognitive performance in 9-10-year-old children. Front. Hum. Neurosci. 14 , 578822 (2020).
Gonzalez, R. et al. An update on the assessment of culture and environment in the ABCD Study: emerging literature and protocol updates over three measurement waves. Dev. Cogn. Neurosci. 52 , 101021 (2021).
Hoffman, E. A. et al. Stress exposures, neurodevelopment and health measures in the ABCD study. Neurobiol. Stress 10 , 100157 (2019).
Meredith, W. J., Cardenas-Iniguez, C., Berman, M. G. & Rosenberg, M. D. Effects of the physical and social environment on youth cognitive performance. Dev. Psychobiol. 64 , e22258 (2022).
Zucker, R. A. et al. Assessment of culture and environment in the Adolescent Brain and Cognitive Development Study: rationale, description of measures, and early data. Dev. Cogn. Neurosci. 32 , 107–120 (2018).
Benmarhnia, T., Hajat, A. & Kaufman, J. S. Inferential challenges when assessing racial/ethnic health disparities in environmental research. Environ. Health 20 , 7 (2021).
Johfre, S. S. & Freese, J. Reconsidering the reference category. Sociol. Methodol. 51 , 253–269 (2021).
Roberts, D. E. & Rollins, O. Why sociology matters to race and biosocial science. Annu. Rev. Socio. 46 , 195–214 (2020).
National Academies of Sciences, Engineering, and Medicine. Using Population Descriptors in Genetics and Genomics Research: a New Framework for an Evolving Field https://doi.org/10.17226/26902 (National Academies Press, 2023). This report summarizes important issues relevant to genetics research and provides concrete recommendations for researchers, including flowcharts, checklists and additional tools for implementation .
Cosgrove, K. T. et al. Limits to the generalizability of resting-state functional magnetic resonance imaging studies of youth: an examination of ABCD Study baseline data. Brain Imaging Behav . https://doi.org/10.1007/s11682-022-00665-2 (2022).
White, E. J. et al. Five recommendations for using large-scale publicly available data to advance health among American Indian peoples: the Adolescent Brain and Cognitive Development (ABCD) StudySM as an illustrative case. Neuropsychopharmacology 48 , 263–269 (2023).
Gard, A. M., Hyde, L. W., Heeringa, S. G., West, B. T. & Mitchell, C. Why weight? Analytic approaches for large-scale population neuroscience data. Dev. Cogn. Neurosci. 59 , 101196 (2023).
Lett, E. et al. Health equity tourism: ravaging the justice landscape. J. Med. Syst. 46 , 17 (2022).
Krieger, N., Boyd, R. W., Maio, F. D. & Maybank, A. Medicine’s privileged gatekeepers: producing harmful ignorance about racism and health. Health Affairs Forefront https://www.healthaffairs.org/do/10.1377/forefront.20210415.305480 (2021).
Braveman, P. Health disparities and health equity: concepts and measurement. Annu. Rev. Public Health 27 , 167–194 (2006).
Braveman, P., Arkin, E., Orleans, T., Proctor, D. & Plough, A. What is health equity? And what difference does a definition make? The Equity Initiative https://resources.equityinitiative.org/handle/ei/418 (2017).
Ford, C. L. & Airhihenbuwa, C. O. Critical race theory, race equity, and public health: toward antiracism praxis. Am. J. Public Health 100 , S30–S35 (2010). This article provides a framework for applying concepts of CRT to empirical studies in public health, and other fields focusing on human research .
Ford, C. L. & Airhihenbuwa, C. O. The public health critical race methodology: praxis for antiracism research. Soc. Sci. Med. 71 , 1390–1398 (2010).
Ford, C. L. & Airhihenbuwa, C. O. Commentary: just what is critical race theory and what’s it doing in a progressive field like public health? Ethn. Dis. 28 , 223–230 (2018).
Katikireddi, S. V. & Valles, S. A. Coupled ethical–epistemic analysis of public health research and practice: categorizing variables to improve population health and equity. Am. J. Public Health 105 , e36–e42 (2015).
Tuana, N. in Scientific Integrity and Ethics in the Geosciences (ed. Gunderson, L. C.) 155–173 (American Geophysical Union, 2017).
Valles, S. A., Piso, Z. & O’Rourke, M. Coupled ethical-epistemic analysis as a tool for environmental science. Ethics Policy Environ. 22 , 267–286 (2019).
Causadias, J. M., Vitriol, J. A. & Atkin, A. L. Do we overemphasize the role of culture in the behavior of racial/ethnic minorities? Evidence of a cultural (mis)attribution bias in American psychology. Am. Psychol. 73 , 243–255 (2018).
Szanton, S. L., LaFave, S. E. & Thorpe, R. J. Jr. Structural racial discrimination and structural resilience: measurement precedes change. J. Gerontol. Ser. A 77 , 402–404 (2022).
La Scala, S., Mullins, J. L., Firat, R. B.; Emotional Learning Research Community Advisory Board & Michalska, K. J. Equity, diversity, and inclusion in developmental neuroscience: practical lessons from community-based participatory research. Front. Integr. Neurosci. 16, 1007249 (2023).
Randolph, A. C. et al. Creating a sustainable action-oriented engagement infrastructure—a UMN-MIDB perspective. Front. Integr. Neurosci. 16 , 1060896 (2022).
Shalowitz, M. U. et al. Community-based participatory research: a review of the literature with strategies for community engagement. J. Dev. Behav. Pediatr. 30 , 350–361 (2009).
Buchanan, N. T. & Wiklund, L. O. Why clinical science must change or die: integrating intersectionality and social justice. Women Ther. 43 , 309–329 (2020).
Settles, I. H., Warner, L. R., Buchanan, N. T. & Jones, M. K. Understanding psychology’s resistance to intersectionality theory using a framework of epistemic exclusion and invisibility. J. Soc. Issues 76 , 796–813 (2020).
Bodison, S. C., Nagel, B., Lopez, D. A., Huber, R. & Members of ABCD JEDI WG3. Equity-focused questions for researchers using the ABCD Study. OSF Home https://doi.org/10.17605/OSF.IO/PM7SY (2023).
Carrero Pinedo, A., Caso, T. J., Rivera, R. M., Carballea, D. & Louis, E. F. Black, indigenous, and trainees of color stress and resilience: the role of training and education in decolonizing psychology. Psychol. Trauma Theory Res. Pract. Policy 14 , S140–S147 (2022).
Rodriguez-Seijas, C. A. et al. The next generation of clinical psychological science: moving toward antiracism. Clin. Psychol. Sci. https://doi.org/10.1177/21677026231156545 (2023).
Buchanan, N. T., Perez, M., Prinstein, M. J. & Thurston, I. B. Upending racism in psychological science: strategies to change how science is conducted, reported, reviewed, and disseminated. Am. Psychol. 76 , 1097–1112 (2021).
Garcini, L. M. et al. Increasing diversity in developmental cognitive neuroscience: a roadmap for increasing representation in pediatric neuroimaging research. Dev. Cogn. Neurosci. 58 , 101167 (2022).
Roberts, S. O., Bareket-Shavit, C., Dollins, F. A., Goldie, P. D. & Mortenson, E. Racial inequality in psychological research: trends of the past and recommendations for the future. Perspect. Psychol. Sci. 15 , 1295–1309 (2020).
Bryant, B. E., Jordan, A. & Clark, U. S. Race as a social construct in psychiatry research and practice. JAMA Psychiatry 79 , 93–94 (2022).
Roth, W. D. The multiple dimensions of race. Ethn. Racial Stud. 39 , 1310–1338 (2016).
Ford, C. L. & Harawa, N. T. A new conceptualization of ethnicity for social epidemiologic and health equity research. Soc. Sci. Med. 71 , 251–258 (2010).
Delgado, R. & Stefancic, J. Critical Race Theory: an Introduction (NYU Press, 2012).
Ray, V. On Critical Race Theory: Why it Matters & Why You Should Care (Random House, 2023).
Kaplan, J. B. & Bennett, T. Use of race and ethnicity in biomedical publication. JAMA 289 , 2709–2716 (2003).
Mir, G. et al. Principles for research on ethnicity and health: the Leeds Consensus Statement. Eur. J. Public Health 23 , 504–510 (2013). This paper outlines the process through which the Leeds Consensus Statement, an international set of recommendations for ethnicity in research, was developed .
Bowleg, L. ‘The master’s tools will never dismantle the master’s house’: ten critical lessons for black and other health equity researchers of color. Health Educ. Behav. 48 , 237–249 (2021).
Hardeman, R. R., Homan, P. A., Chantarat, T., Davis, B. A. & Brown, T. H. Improving the measurement of structural racism to achieve antiracist health policy. Health Aff. 41 , 179–186 (2022).
Lett, E., Asabor, E., Beltrán, S., Cannon, A. M. & Arah, O. A. Conceptualizing, contextualizing, and operationalizing race in quantitative health sciences research. Ann. Fam. Med. 20 , 157–163 (2022).
López, N., Erwin, C., Binder, M. & Chavez, M. J. Making the invisible visible: advancing quantitative methods in higher education using critical race theory and intersectionality. Race Ethn. Educ. 21 , 180–207 (2018).
Nketia, J., Amso, D. & Brito, N. H. Towards a more inclusive and equitable developmental cognitive neuroscience. Dev. Cogn. Neurosci. 52 , 101014 (2021).
Gillborn, D., Warmington, P. & Demack, S. QuantCrit: education, policy, ‘big data’ and principles for a critical race theory of statistics. Race Ethn. Educ. 21 , 158–179 (2018).
Crossing, A. E., Gumudavelly, D., Watkins, N., Logue, C. & Anderson, R. E. A critical race theory of psychology as praxis: proposing and utilizing principles of PsyCrit. J. Adolesc. Res . https://doi.org/10.1177/07435584221101930 (2022).
Martín-Baró, I. Writings for a Liberation Psychology (Harvard Univ. Press, 1994).
Omi, M. & Winant, H. Racial Formation in the United States: From the 1960s to the 1980s (Routledge & Kegan Paul, 1986).
Feagin, J. R. & Ducey, K. Racist America: Roots, Current Realities, and Future Reparations (Routledge, 2018).
Williams, D. R. & Sternthal, M. Understanding racial-ethnic disparities in health: sociological contributions. J. Health Soc. Behav. 51 , S15–S27 (2010).
Haeny, A. M., Holmes, S. C. & Williams, M. T. The need for shared nomenclature on racism and related terminology in psychology. Perspect. Psychol. Sci. 16 , 886–892 (2021).
Bonilla-Silva, E. Rethinking racism: toward a structural interpretation. Am. Sociol. Rev. 62 , 465–480 (1997).
Chen, J. & Courtwright, A. in Encyclopedia of Global Bioethics (ed. ten Have, H.) 2706–2712 (Springer International Publishing, Cham, 2016).
Garavan, H. et al. Recruiting the ABCD sample: design considerations and procedures. Dev. Cogn. Neurosci. 32 , 16–22 (2018).
Article CAS PubMed PubMed Central Google Scholar
Jernigan, T. L., Brown, S. A. & Dowling, G. J. The Adolescent Brain Cognitive Development Study. J. Res. Adolesc. 28 , 154–156 (2018).
Compton, W. M., Dowling, G. J. & Garavan, H. Ensuring the best use of data: the Adolescent Brain Cognitive Development Study. JAMA Pediatr. 173 , 809–810 (2019).
Dick, A. S. et al. Meaningful associations in the adolescent brain cognitive development study. NeuroImage 239 , 118262 (2021).
Hoffman, E. A., LeBlanc, K., Weiss, S. R. B. & Dowling, G. J. Transforming the future of adolescent health: opportunities from the Adolescent Brain Cognitive Development Study. J. Adolesc. Health 70 , 186–188 (2022).
Simmons, C. et al. Responsible use of open-access developmental data: The Adolescent Brain Cognitive Development (ABCD) Study. Psychol. Sci . https://doi.org/10.1177/09567976211003564 (2021).
Telles, E. Latinos, race, and the U.S. census. Ann. Am. Acad. Pol. Soc. Sci. 677 , 153–164 (2018).
Garcia, N. M., López, N. & Vélez, V. N. QuantCrit: rectifying quantitative methods through critical race theory. Race Ethn. Educ. 21 , 149–157 (2018).
Tabron, L. A. & Thomas, A. K. Deeper than wordplay: a systematic review of critical quantitative approaches in education research (2007–2021). Rev. Educ. Res . https://doi.org/10.3102/00346543221130017 (2023).
Suzuki, S., Morris, S. L. & Johnson, S. K. Using QuantCrit to advance an anti-racist developmental science: applications to mixture modeling. J. Adolesc. Res. 36 , 535–560 (2021). This article provides a review of the QuantCrit literature and provides recommendations that researchers can use when conducting antiracism-focused quantitative research .
Castillo, W. & Gillborn, D. How to ‘QuantCrit:’ practices and questions for education data researchers and users. EdWorkingPapers https://www.edworkingpapers.com/ai22-546 (2022).
Roberts, S. O. & Mortenson, E. Challenging the white = neutral framework in psychology. Perspect. Psychol. Sci . https://doi.org/10.1177/17456916221077117 (2022).
Download references
Acknowledgements
We thank the large number of people who provided feedback, comments and critiques over the development of this paper. In particular, we thank the members of the ABCD Study JEDI Working Groups, who provided many of the initial discussions that led to the development of this paper. We particularly acknowledge the following people for providing numerous comments on drafts of this manuscript: M. Herting, K. Bagot, L. Uddin, S. Bodison, R. Huber, D. Lopez, E. Hoffman, S. Adise, A. Potter and K. Uban. C.C.-I. acknowledges fellow NSP (R25NS089462), BRAINS (R25NS094094) and Diversifying CNS (R25NS117356) scholars, who have provided invaluable support and inspiration for addressing structural barriers in neuroscience for BIPOC scholars, as well as T32ES013678, R25DA059073, and R25MH125545. C.C.-I. is supported by National Institute of Environmental Health Science grants T32ES013678, R01ES031074 and P30ES007048, and National Institute on Minority Health and Health Disparities grant P50MD015705. M.R.G. is supported by National Institute on Alcohol Abuse and Alcoholism grant K01AA030325 and National Institute on Drug Abuse grants R61DA058976, R25DA050724, and R25DA050687.
Author information
Authors and affiliations.
Department of Population and Public Health Sciences, University of Southern California, Los Angeles, CA, USA
Carlos Cardenas-Iniguez
Department of Psychiatry and Behavioral Health, The Ohio State University, Columbus, OH, USA
Marybel Robledo Gonzalez
You can also search for this author in PubMed Google Scholar
Contributions
C.C.-I. wrote the first draft of the manuscript. M.R.G. wrote sections of the manuscript. All authors contributed to the revision of the paper and approved the submitted version.
Corresponding author
Correspondence to Carlos Cardenas-Iniguez .
Ethics declarations
Competing interests.
The authors declare no competing interests.
Peer review
Peer review information.
Nature Neuroscience thanks Elvisha Dhamala and Bradley Voytek for their contribution to the peer review of this work.
Additional information
Publisher’s note Springer Nature remains neutral with regard to jurisdictional claims in published maps and institutional affiliations.
Rights and permissions
Springer Nature or its licensor (e.g. a society or other partner) holds exclusive rights to this article under a publishing agreement with the author(s) or other rightsholder(s); author self-archiving of the accepted manuscript version of this article is solely governed by the terms of such publishing agreement and applicable law.
Reprints and permissions
About this article
Cite this article.
Cardenas-Iniguez, C., Gonzalez, M.R. Recommendations for the responsible use and communication of race and ethnicity in neuroimaging research. Nat Neurosci (2024). https://doi.org/10.1038/s41593-024-01608-4
Download citation
Received : 05 July 2023
Accepted : 16 February 2024
Published : 22 March 2024
DOI : https://doi.org/10.1038/s41593-024-01608-4
Share this article
Anyone you share the following link with will be able to read this content:
Sorry, a shareable link is not currently available for this article.
Provided by the Springer Nature SharedIt content-sharing initiative
Quick links
- Explore articles by subject
- Guide to authors
- Editorial policies
Sign up for the Nature Briefing newsletter — what matters in science, free to your inbox daily.

Advertisement
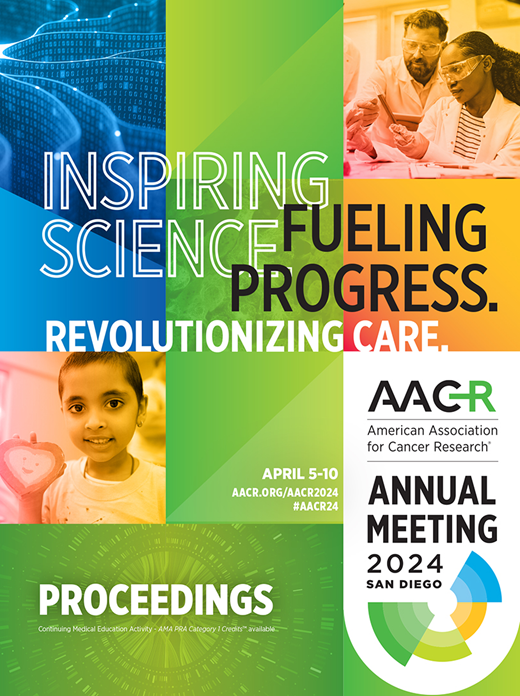
Abstract 4443: Trans-population two-sample Mendelian randomization study of circulating metabolites and prostate cancer risk
- Split-Screen
- Article contents
- Figures & tables
- Supplementary Data
- Peer Review
- Get Permissions
- Cite Icon Cite
- Search Site
- Version of Record March 22 2024
Harriett Fuller , Rebecca Rohde , Heather Highland , Jiayi Shen , Bing Yu , Eric Boerwinkle , Megan Grove , Kari E. North , David V. Conti , Christopher A. Haiman , Kristin Young , Burcu Darst; Abstract 4443: Trans-population two-sample Mendelian randomization study of circulating metabolites and prostate cancer risk. Cancer Res 15 March 2024; 84 (6_Supplement): 4443. https://doi.org/10.1158/1538-7445.AM2024-4443
Download citation file:
- Ris (Zotero)
- Reference Manager
While prostate cancer (PCa) is highly heritable, mechanisms underlying disease risk and PCa disparities are not well understood. Here, we conducted a two-sample Mendelian randomization (MR) to assess whether serum metabolites are causally associated with PCa risk in European, African, and Hispanic populations.
MR analyses were performed on metabolite quantitative trait loci (mQTLs) for 250 metabolites quantified by untargeted mass spectroscopy via the Metabolon platform for 1,498 European and 1,740 African ancestry individuals from the Atherosclerosis Risk in Communities (ARIC) cohort and for 711 metabolites measured in 3,166 Hispanic individuals from the Hispanic Community Health Study/Study of Latinos (SOL). PCa GWAS summary statistics were obtained for men from European (122,188 cases, 604,640 controls), African (19,391 cases, 61,608 controls) and Hispanic (3,931 cases, 26,405 controls) populations from the PRACTICAL Consortium. Within each population, QTLs associated with metabolites at the genome-wide significance level (P<5x10 -8 ) were included in instruments upon removing rare (minor allele frequency≤0.01) or correlated (R 2 ≥0.2) SNPs calculated in ancestry-matched TOPMed populations. Inverse variance weighted (IVW) random effect models are presented as primary results. Sensitivity analyses were utilized to assess assumption violations (weighted mode, weighted median and MR egger). MR were conducted separately in each population, and fixed effect meta-analyses were conducted across population-specific MR results to identify trans-population associations. A false discovery rate was implemented to account for multiple testing.
In total, 22, 4 and 1 metabolites significantly associated with PCa risk in Hispanic, European and African populations, respectively. Of these, 13 metabolites had a MR instrument in ≥1 population, 12 of which were significant following a trans-population meta-analysis, including 5 fatty acids, 3 lysophospholipids, 1 amino acid, 1 carbohydrate, 1 nucleotide and 1 xenobiotic. All fatty acids were associated with decreased PCa odds (2%-10%), as were 4 other metabolites (amino acid 3-methyoxytyrosine, nucleotide 5-methyluridine and lysophospholipids 1-archidonoyl-GPC (20:4n6) and 1-archidonoyl-GPE (20:4n6), 4%-9%). The 3 remaining metabolites, erythritol, 1-linoleoyl-GPE (18:2) and mannose, were found to increase PCa odds by 10%, 6% and 4%, respectively. Additional metabolite harmonization efforts are underway to conduct trans-population analyses metabolome-wide.
This study provides evidence of associations between metabolites, such as fatty acids and lysophysopholipids, and PCa across diverse populations. These findings point to mechanisms that could inform preventive or therapeutic strategies pending functional investigations. Multivariable and bidirectional MR are ongoing to further assess findings.
Citation Format: Harriett Fuller, Rebecca Rohde, Heather Highland, Jiayi Shen, Bing Yu, Eric Boerwinkle, Megan Grove, Kari E. North, David V. Conti, Christopher A. Haiman, Kristin Young, Burcu Darst. Trans-population two-sample Mendelian randomization study of circulating metabolites and prostate cancer risk [abstract]. In: Proceedings of the American Association for Cancer Research Annual Meeting 2024; Part 1 (Regular Abstracts); 2024 Apr 5-10; San Diego, CA. Philadelphia (PA): AACR; Cancer Res 2024;84(6_Suppl):Abstract nr 4443.
Citing articles via
Email alerts.
- Online First
- Collections
- Online ISSN 1538-7445
- Print ISSN 0008-5472
AACR Journals
- Blood Cancer Discovery
- Cancer Discovery
- Cancer Epidemiology, Biomarkers & Prevention
- Cancer Immunology Research
- Cancer Prevention Research
- Cancer Research
- Cancer Research Communications
- Clinical Cancer Research
- Molecular Cancer Research
- Molecular Cancer Therapeutics
- Info for Advertisers
- Information for Institutions/Librarians

- Privacy Policy
- Copyright © 2023 by the American Association for Cancer Research.
This Feature Is Available To Subscribers Only
Sign In or Create an Account

An official website of the United States government
The .gov means it’s official. Federal government websites often end in .gov or .mil. Before sharing sensitive information, make sure you’re on a federal government site.
The site is secure. The https:// ensures that you are connecting to the official website and that any information you provide is encrypted and transmitted securely.
- Publications
- Account settings
Preview improvements coming to the PMC website in October 2024. Learn More or Try it out now .
- Advanced Search
- Journal List
- Indian J Psychol Med
- v.42(1); Jan-Feb 2020
Sample Size and its Importance in Research
Chittaranjan andrade.
Clinical Psychopharmacology Unit, Department of Clinical Psychopharmacology and Neurotoxicology, National Institute of Mental Health and Neurosciences, Bengaluru, Karnataka, India
The sample size for a study needs to be estimated at the time the study is proposed; too large a sample is unnecessary and unethical, and too small a sample is unscientific and also unethical. The necessary sample size can be calculated, using statistical software, based on certain assumptions. If no assumptions can be made, then an arbitrary sample size is set for a pilot study. This article discusses sample size and how it relates to matters such as ethics, statistical power, the primary and secondary hypotheses in a study, and findings from larger vs. smaller samples.
Studies are conducted on samples because it is usually impossible to study the entire population. Conclusions drawn from samples are intended to be generalized to the population, and sometimes to the future as well. The sample must therefore be representative of the population. This is best ensured by the use of proper methods of sampling. The sample must also be adequate in size – in fact, no more and no less.
SAMPLE SIZE AND ETHICS
A sample that is larger than necessary will be better representative of the population and will hence provide more accurate results. However, beyond a certain point, the increase in accuracy will be small and hence not worth the effort and expense involved in recruiting the extra patients. Furthermore, an overly large sample would inconvenience more patients than might be necessary for the study objectives; this is unethical. In contrast, a sample that is smaller than necessary would have insufficient statistical power to answer the primary research question, and a statistically nonsignificant result could merely be because of inadequate sample size (Type 2 or false negative error). Thus, a small sample could result in the patients in the study being inconvenienced with no benefit to future patients or to science. This is also unethical.
In this regard, inconvenience to patients refers to the time that they spend in clinical assessments and to the psychological and physical discomfort that they experience in assessments such as interviews, blood sampling, and other procedures.
ESTIMATING SAMPLE SIZE
So how large should a sample be? In hypothesis testing studies, this is mathematically calculated, conventionally, as the sample size necessary to be 80% certain of identifying a statistically significant outcome should the hypothesis be true for the population, with P for statistical significance set at 0.05. Some investigators power their studies for 90% instead of 80%, and some set the threshold for significance at 0.01 rather than 0.05. Both choices are uncommon because the necessary sample size becomes large, and the study becomes more expensive and more difficult to conduct. Many investigators increase the sample size by 10%, or by whatever proportion they can justify, to compensate for expected dropout, incomplete records, biological specimens that do not meet laboratory requirements for testing, and other study-related problems.
Sample size calculations require assumptions about expected means and standard deviations, or event risks, in different groups; or, upon expected effect sizes. For example, a study may be powered to detect an effect size of 0.5; or a response rate of 60% with drug vs. 40% with placebo.[ 1 ] When no guesstimates or expectations are possible, pilot studies are conducted on a sample that is arbitrary in size but what might be considered reasonable for the field.
The sample size may need to be larger in multicenter studies because of statistical noise (due to variations in patient characteristics, nonspecific treatment characteristics, rating practices, environments, etc. between study centers).[ 2 ] Sample size calculations can be performed manually or using statistical software; online calculators that provide free service can easily be identified by search engines. G*Power is an example of a free, downloadable program for sample size estimation. The manual and tutorial for G*Power can also be downloaded.
PRIMARY AND SECONDARY ANALYSES
The sample size is calculated for the primary hypothesis of the study. What is the difference between the primary hypothesis, primary outcome and primary outcome measure? As an example, the primary outcome may be a reduction in the severity of depression, the primary outcome measure may be the Montgomery-Asberg Depression Rating Scale (MADRS) and the primary hypothesis may be that reduction in MADRS scores is greater with the drug than with placebo. The primary hypothesis is tested in the primary analysis.
Studies almost always have many hypotheses; for example, that the study drug will outperform placebo on measures of depression, suicidality, anxiety, disability and quality of life. The sample size necessary for adequate statistical power to test each of these hypotheses will be different. Because a study can have only one sample size, it can be powered for only one outcome, the primary outcome. Therefore, the study would be either overpowered or underpowered for the other outcomes. These outcomes are therefore called secondary outcomes, and are associated with secondary hypotheses, and are tested in secondary analyses. Secondary analyses are generally considered exploratory because when many hypotheses in a study are each tested at a P < 0.05 level for significance, some may emerge statistically significant by chance (Type 1 or false positive errors).[ 3 ]
INTERPRETING RESULTS
Here is an interesting question. A test of the primary hypothesis yielded a P value of 0.07. Might we conclude that our sample was underpowered for the study and that, had our sample been larger, we would have identified a significant result? No! The reason is that larger samples will more accurately represent the population value, whereas smaller samples could be off the mark in either direction – towards or away from the population value. In this context, readers should also note that no matter how small the P value for an estimate is, the population value of that estimate remains the same.[ 4 ]
On a parting note, it is unlikely that population values will be null. That is, for example, that the response rate to the drug will be exactly the same as that to placebo, or that the correlation between height and age at onset of schizophrenia will be zero. If the sample size is large enough, even such small differences between groups, or trivial correlations, would be detected as being statistically significant. This does not mean that the findings are clinically significant.
Financial support and sponsorship
Conflicts of interest.
There are no conflicts of interest.
New World Bank country classifications by income level: 2022-2023
Nada hamadeh, catherine van rompaey, eric metreau, shwetha grace eapen.
Updated country income classifications are available here.
The World Bank assigns the world’s economies [1] to four income groups—low, lower-middle, upper-middle, and high income. The classifications are updated each year on July 1 and are based on the GNI per capita of the previous year (2021). GNI measures are expressed in United States dollars (USD), and are determined using conversion factors derived according to the Atlas method .
Classifications can change for two reasons:
- Changes to Atlas GNI per capita: In each country, factors such as economic growth, inflation, exchange rates, and population growth influence the level of Atlas GNI per capita. Revisions to improve national accounts estimates and methods can also have an impact. Updated data on Atlas GNI per capita for 2021 can be accessed here .
- Changes to classification thresholds: To keep income classification thresholds fixed in real terms, they are adjusted annually for inflation using the Special Drawing Rights (SDR) deflator , a weighted average of the GDP deflators of China, Japan, the United Kingdom, the United States, and the Euro Area. The new thresholds for Atlas GNI per capita are as follows:
Changes in classifications
The tables below list the economies moving to a different classification group this year.
Economies moving to a higher income group
The economy of Belize was severely affected by the COVID-19 pandemic in 2020 and moved to the lower-middle-income group. In 2021, economic growth rebounded, led by tourist-related activities and investments, bringing Belize back to its prior classification as an upper-middle-income country.
The economies of Panama and Romania were each also impacted by the COVID-19 pandemic in 2020 and moved to the upper-middle-income group. In 2021, both experienced a strong rebound, bringing them back to the high-income group.
Economies moving to a lower income group
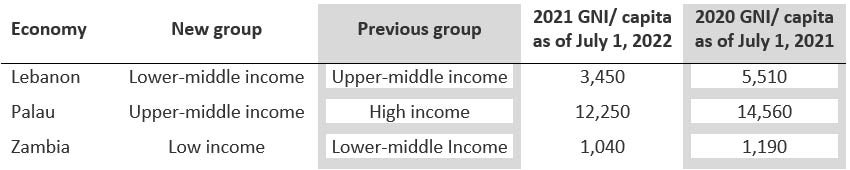
For the eleventh consecutive year, Lebanon’s real GDP per capita fell in 2021, and the country also experienced sharp exchange rate depreciation. Therefore, Lebanon, an upper-middle-income country for almost 25 years, now moves to the lower-middle income group.
Palau’s economy has experienced a downward trend since 2016. Tourism and related industries have been severely impacted by the pandemic, and trade flows were disrupted. While Palau has been a high-income country since FY18, it will now move to the upper-middle-income group. [2]
While a rebound in the price of copper boosted Zambia’s GDP in 2021, a sharp deterioration in exchange rates led to a large decrease in Atlas GNI per capita expressed in US dollars, reclassifying the country to the low-income group.
It is to be noted that Venezuela, classified as an upper-middle income country until FY21, has been unclassified since then due to the unavailability of data.
More information
Detailed information on how the World Bank classifies countries is available here . The country and lending groups page provides a complete list of economies classified by income, region, and World Bank lending status and includes links to prior years’ classifications. The classification tables include World Bank member countries, along with all other economies with populations greater than 30,000.
These classifications reflect the best available GNI figures for 2021, which may be revised as countries publish improved final estimates.
In countries where dual or multiple exchange rates are in use, the exchange rate used to convert local currency units to US$ is an average of these exchange rates, provided necessary data are available.
Data for GNI , GNI per capita , GDP , GDP PPP , and Population for 2021 are now available on the World Bank's Open Data Catalog. Note that these are preliminary estimates and may be revised. For more information, please contact us at [email protected] .
[1] The term country, used interchangeably with economy, does not imply political independence but refers to any territory for which authorities report separate social or economic statistics.
[2] Based on internal Bank estimates, pending publication of official data.
- The World Region
Manager, Development Data Group, World Bank
Senior Economist, Development Data Group, World Bank
Senior Economist
Consultant, Development Data Group, World Bank
Join the Conversation
- Share on mail
- comments added
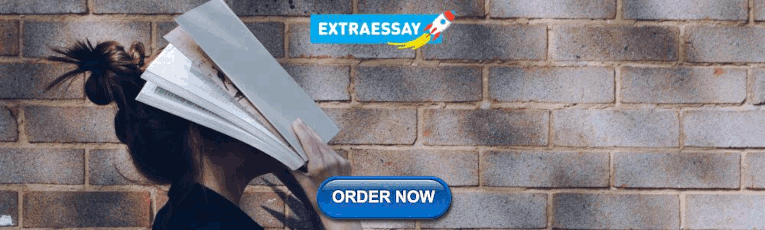
IMAGES
VIDEO
COMMENTS
A population is the entire group that you want to draw conclusions about. A sample is the specific group that you will collect data from. The size of the sample is always less than the total size of the population. In research, a population doesn't always refer to people. It can mean a group containing elements of anything you want to study ...
Population data consists of information collected from every individual in a particular population. Meanwhile, sample data consists of information taken from a subset—or sample —of the population. In this guide, we'll discuss the differences between population and sample data, the advantages and disadvantages of each, how to collect data ...
Example 1: Research Study: Investigating the prevalence of stress among high school students in a specific city and its impact on academic performance. Population: All high school students in a particular city Sampling Frame: The sampling frame would involve obtaining a comprehensive list of all high schools in the specific city. A random selection of schools would be made from this list to ...
Population vs sample is a crucial distinction in statistics. Typically, researchers use samples to learn about populations. Let's explore the differences between these concepts! Population: The whole group of people, items, or element of interest. Sample: A subset of the population that researchers select and include in their study.
Definition. In quantitative research methodology, the sample is a set of collected data from a defined procedure. It is basically a much smaller part of the whole, i.e., population. The sample depicts all the members of the population that are under observation when conducting research surveys.
Answers Chapter 3 Q3.pdf. Populations In statistics the term "population" has a slightly different meaning from the one given to it in ordinary speech. It need not refer only to people or to animate creatures - the population of Britain, for instance or the dog population of London. Statisticians also speak of a population.
A population is a complete set of people with specified characteristics, while a sample is a subset of the population. 1 In general, most people think of the defining characteristic of a population in terms of geographic location. However, in research, other characteristics will define a population.
A sample is a subset of individuals from a larger population. Sampling means selecting the group that you will actually collect data from in your research. For example, if you are researching the opinions of students in your university, you could survey a sample of 100 students.
Sampling methods in psychology refer to strategies used to select a subset of individuals (a sample) from a larger population, to study and draw inferences about the entire population. Common methods include random sampling, stratified sampling, cluster sampling, and convenience sampling. Proper sampling ensures representative, generalizable, and valid research results.
In selecting a population for study, the research question or purpose of the study will suggest a suitable definition of the population to be studied, in terms of location and restriction to a particular age group, sex or occupation. ... We do not know to what extent the study sample and population of Delhi is typical of the larger population ...
A sample is a concrete thing. You can open up a data file, and there's the data from your sample. A population, on the other hand, is a more abstract idea. It refers to the set of all possible people, or all possible observations, that you want to draw conclusions about, and is generally much bigger than the sample.
Sampling is the statistical process of selecting a subset—called a 'sample'—of a population of interest for the purpose of making observations and statistical inferences about that population. Social science research is generally about inferring patterns of behaviours within specific populations. We cannot study entire populations because of feasibility and cost constraints, and hence ...
A population is the entire group that you want to draw conclusions about. A sample is the specific group that you will collect data from. The size of the sample is always less than the total size of the population. In research, a population doesn't always refer to people. It can mean a group containing elements of anything you want to study ...
To ensure that your sample is representative of the population, you will want to use a probability sample. A representative sample refers to whether the characteristics (race, age, income, education, etc) of the sample are the same as the population. Probability sampling is a sampling technique in which every individual in the population has an ...
The population refers to an entire set of units that exhibit a variable characteristic under investigation and for which research findings can be generalized (Shukla, 2020). Meanwhile, a sample is ...
Sampling types. There are two major categories of sampling methods ( figure 1 ): 1; probability sampling methods where all subjects in the target population have equal chances to be selected in the sample [ 1, 2] and 2; non-probability sampling methods where the sample population is selected in a non-systematic process that does not guarantee ...
Sampling methods are used in research when it is not feasible or practical to study the entire population of interest. Sampling allows researchers to study a smaller group of individuals, known as a sample, and use the findings from the sample to make inferences about the larger population. Sampling methods are particularly useful when:
A sample is the subset of the population that you actually measure, test, or evaluate and base your results. Sampling methods are how you obtain your sample. Before beginning your study, carefully define the population because your results apply to the target population. You can define your population as narrowly as necessary to meet the needs ...
Population and sample in research are often confused with one another, so it is important to understand the differences between the terms population and sample. A population is an entire group of ...
Sampling is the process of selecting a statistically representative sample of individuals from the target population. The sample should be large enough to answer the research question (Majid, 2017 ...
The essential topics related to the selection of participants for a health research are: 1) whether to work with samples or include the whole reference population in the study (census); 2) the sample basis; 3) the sampling process and 4) the potential effects nonrespondents might have on study results. We will refer to each of these aspects ...
While this Perspective provides recommendations, in the end, each research project will require a tailored plan, depending on the measures used, the subset of the sample population and the ...
Abstract. While prostate cancer (PCa) is highly heritable, mechanisms underlying disease risk and PCa disparities are not well understood. Here, we conducted a two-sample Mendelian randomization (MR) to assess whether serum metabolites are causally associated with PCa risk in European, African, and Hispanic populations.MR analyses were performed on metabolite quantitative trait loci (mQTLs ...
The sample size for a study needs to be estimated at the time the study is proposed; too large a sample is unnecessary and unethical, and too small a sample is unscientific and also unethical. The necessary sample size can be calculated, using statistical software, based on certain assumptions. If no assumptions can be made, then an arbitrary ...
Changes to Atlas GNI per capita: In each country, factors such as economic growth, inflation, exchange rates, and population growth influence the level of Atlas GNI per capita. Revisions to improve national accounts estimates and methods can also have an impact. Updated data on Atlas GNI per capita for 2021 can be accessed here.