
- Ethics of AI
- AI in Education
- Digital Inclusion
- Digital Policy, Capacities and Inclusion
- Women’s access to and participation in technological developments
- Internet Universality Indicators
- All publications
- Recommendation on the Ethics of AI
- Report on ethics in robotics
- Map of emerging AI areas in the Global South
- 7 minutes to understand AI
- On the ethics of AI
- On a possible normative instrument for the ethics of AI
- On technical and legal aspects of the desirability of a standard-setting instrument for AI ethics

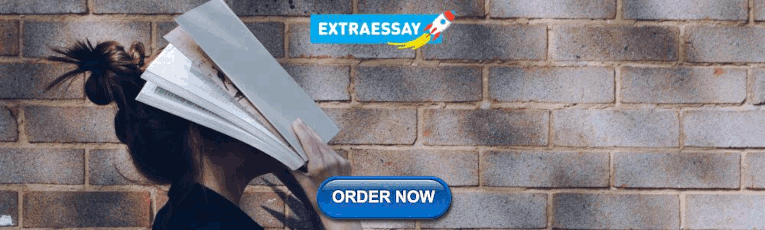
Artificial intelligence in education
Artificial Intelligence (AI) has the potential to address some of the biggest challenges in education today, innovate teaching and learning practices, and accelerate progress towards SDG 4. However, rapid technological developments inevitably bring multiple risks and challenges, which have so far outpaced policy debates and regulatory frameworks. UNESCO is committed to supporting Member States to harness the potential of AI technologies for achieving the Education 2030 Agenda, while ensuring that its application in educational contexts is guided by the core principles of inclusion and equity. UNESCO’s mandate calls inherently for a human-centred approach to AI . It aims to shift the conversation to include AI’s role in addressing current inequalities regarding access to knowledge, research and the diversity of cultural expressions and to ensure AI does not widen the technological divides within and between countries. The promise of “AI for all” must be that everyone can take advantage of the technological revolution under way and access its fruits, notably in terms of innovation and knowledge.
Furthermore, UNESCO has developed within the framework of the Beijing Consensus a publication aimed at fostering the readiness of education policy-makers in artificial intelligence. This publication, Artificial Intelligence and Education: Guidance for Policy-makers , will be of interest to practitioners and professionals in the policy-making and education communities. It aims to generate a shared understanding of the opportunities and challenges that AI offers for education, as well as its implications for the core competencies needed in the AI era
- Greek (modern)

The UNESCO Courier, October-December 2023

- Plurilingual
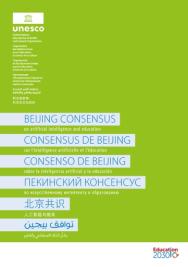
by Stefania Giannini, UNESCO Assistant Director-General for Education
International Forum on artificial intelligence and education
- More information
- Analytical report

Through its projects, UNESCO affirms that the deployment of AI technologies in education should be purposed to enhance human capacities and to protect human rights for effective human-machine collaboration in life, learning and work, and for sustainable development. Together with partners, international organizations, and the key values that UNESCO holds as pillars of their mandate, UNESCO hopes to strengthen their leading role in AI in education, as a global laboratory of ideas, standard setter, policy advisor and capacity builder. If you are interested in leveraging emerging technologies like AI to bolster the education sector, we look forward to partnering with you through financial, in-kind or technical advice contributions. 'We need to renew this commitment as we move towards an era in which artificial intelligence – a convergence of emerging technologies – is transforming every aspect of our lives (…),' said Ms Stefania Giannini, UNESCO Assistant Director-General for Education at the International Conference on Artificial Intelligence and Education held in Beijing in May 2019. 'We need to steer this revolution in the right direction, to improve livelihoods, to reduce inequalities and promote a fair and inclusive globalization.’'

Related items
- Artificial intelligence
Artificial Intelligence and Education: A Reading List
A bibliography to help educators prepare students and themselves for a future shaped by AI—with all its opportunities and drawbacks.
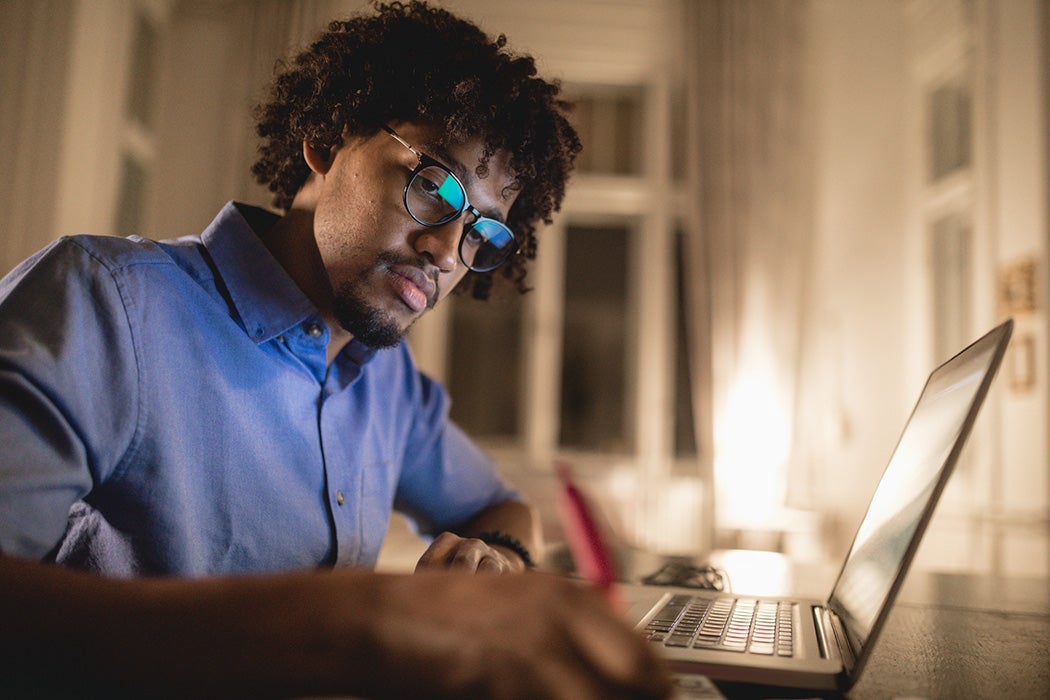
How should education change to address, incorporate, or challenge today’s AI systems, especially powerful large language models? What role should educators and scholars play in shaping the future of generative AI? The release of ChatGPT in November 2022 triggered an explosion of news, opinion pieces, and social media posts addressing these questions. Yet many are not aware of the current and historical body of academic work that offers clarity, substance, and nuance to enrich the discourse.
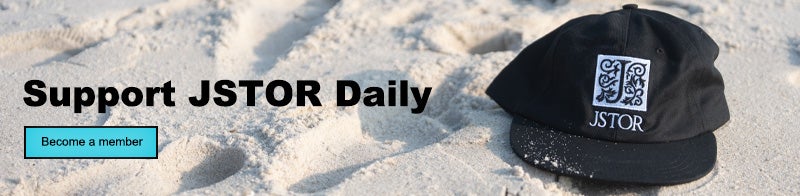
Linking the terms “AI” and “education” invites a constellation of discussions. This selection of articles is hardly comprehensive, but it includes explanations of AI concepts and provides historical context for today’s systems. It describes a range of possible educational applications as well as adverse impacts, such as learning loss and increased inequity. Some articles touch on philosophical questions about AI in relation to learning, thinking, and human communication. Others will help educators prepare students for civic participation around concerns including information integrity, impacts on jobs, and energy consumption. Yet others outline educator and student rights in relation to AI and exhort educators to share their expertise in societal and industry discussions on the future of AI.
Nabeel Gillani, Rebecca Eynon, Catherine Chiabaut, and Kelsey Finkel, “ Unpacking the ‘Black Box’ of AI in Education ,” Educational Technology & Society 26, no. 1 (2023): 99–111.
Whether we’re aware of it or not, AI was already widespread in education before ChatGPT. Nabeel Gillani et al. describe AI applications such as learning analytics and adaptive learning systems, automated communications with students, early warning systems, and automated writing assessment. They seek to help educators develop literacy around the capacities and risks of these systems by providing an accessible introduction to machine learning and deep learning as well as rule-based AI. They present a cautious view, calling for scrutiny of bias in such systems and inequitable distribution of risks and benefits. They hope that engineers will collaborate deeply with educators on the development of such systems.
Jürgen Rudolph, Samson Tan, and Shannon Tan, “ ChatGPT: Bullshit Spewer or the End of Traditional Assessments in Higher Education? ” The Journal of Applied Learning and Teaching 6, no. 1 (January 24, 2023).
Jürgen Rudolph et al. give a practically oriented overview of ChatGPT’s implications for higher education. They explain the statistical nature of large language models as they tell the history of OpenAI and its attempts to mitigate bias and risk in the development of ChatGPT. They illustrate ways ChatGPT can be used with examples and screenshots. Their literature review shows the state of artificial intelligence in education (AIEd) as of January 2023. An extensive list of challenges and opportunities culminates in a set of recommendations that emphasizes explicit policy as well as expanding digital literacy education to include AI.
Emily M. Bender, Timnit Gebru, Angela McMillan-Major, and Shmargaret Shmitchell, “ On the Dangers of Stochastic Parrots: Can Language Models Be Too Big? 🦜 ,” FAccT ’21: Proceedings of the 2021 ACM Conference on Fairness, Accountability, and Transparency (March 2021): 610–623.
Student and faculty understanding of the risks and impacts of large language models is central to AI literacy and civic participation around AI policy. This hugely influential paper details documented and likely adverse impacts of the current data-and-resource-intensive, non-transparent mode of development of these models. Bender et al. emphasize the ways in which these costs will likely be borne disproportionately by marginalized groups. They call for transparency around the energy use and cost of these models as well as transparency around the data used to train them. They warn that models perpetuate and even amplify human biases and that the seeming coherence of these systems’ outputs can be used for malicious purposes even though it doesn’t reflect real understanding.
The authors argue that inclusive participation in development can encourage alternate development paths that are less resource intensive. They further argue that beneficial applications for marginalized groups, such as improved automatic speech recognition systems, must be accompanied by plans to mitigate harm.
Erik Brynjolfsson, “ The Turing Trap: The Promise & Peril of Human-Like Artificial Intelligence ,” Daedalus 151, no. 2 (2022): 272–87.
Erik Brynjolfsson argues that when we think of artificial intelligence as aiming to substitute for human intelligence, we miss the opportunity to focus on how it can complement and extend human capabilities. Brynjolfsson calls for policy that shifts AI development incentives away from automation toward augmentation. Automation is more likely to result in the elimination of lower-level jobs and in growing inequality. He points educators toward augmentation as a framework for thinking about AI applications that assist learning and teaching. How can we create incentives for AI to support and extend what teachers do rather than substituting for teachers? And how can we encourage students to use AI to extend their thinking and learning rather than using AI to skip learning?
Kevin Scott, “ I Do Not Think It Means What You Think It Means: Artificial Intelligence, Cognitive Work & Scale ,” Daedalus 151, no. 2 (2022): 75–84.
Brynjolfsson’s focus on AI as “augmentation” converges with Microsoft computer scientist Kevin Scott’s focus on “cognitive assistance.” Steering discussion of AI away from visions of autonomous systems with their own goals, Scott argues that near-term AI will serve to help humans with cognitive work. Scott situates this assistance in relation to evolving historical definitions of work and the way in which tools for work embody generalized knowledge about specific domains. He’s intrigued by the way deep neural networks can represent domain knowledge in new ways, as seen in the unexpected coding capabilities offered by OpenAI’s GPT-3 language model, which have enabled people with less technical knowledge to code. His article can help educators frame discussions of how students should build knowledge and what knowledge is still relevant in contexts where AI assistance is nearly ubiquitous.
Laura D. Tyson and John Zysman, “ Automation, AI & Work ,” Daedalus 151, no. 2 (2022): 256–71.
How can educators prepare students for future work environments integrated with AI and advise students on how majors and career paths may be affected by AI automation? And how can educators prepare students to participate in discussions of government policy around AI and work? Laura Tyson and John Zysman emphasize the importance of policy in determining how economic gains due to AI are distributed and how well workers weather disruptions due to AI. They observe that recent trends in automation and gig work have exacerbated inequality and reduced the supply of “good” jobs for low- and middle-income workers. They predict that AI will intensify these effects, but they point to the way collective bargaining, social insurance, and protections for gig workers have mitigated such impacts in countries like Germany. They argue that such interventions can serve as models to help frame discussions of intelligent labor policies for “an inclusive AI era.”
Todd C. Helmus, Artificial Intelligence, Deepfakes, and Disinformation: A Primer (RAND Corporation, 2022).
Educators’ considerations of academic integrity and AI text can draw on parallel discussions of authenticity and labeling of AI content in other societal contexts. Artificial intelligence has made deepfake audio, video, and images as well as generated text much more difficult to detect as such. Here, Todd Helmus considers the consequences to political systems and individuals as he offers a review of the ways in which these can and have been used to promote disinformation. He considers ways to identify deepfakes and ways to authenticate provenance of videos and images. Helmus advocates for regulatory action, tools for journalistic scrutiny, and widespread efforts to promote media literacy. As well as informing discussions of authenticity in educational contexts, this report might help us shape curricula to teach students about the risks of deepfakes and unlabeled AI.
William Hasselberger, “ Can Machines Have Common Sense? ” The New Atlantis 65 (2021): 94–109.
Students, by definition, are engaged in developing their cognitive capacities; their understanding of their own intelligence is in flux and may be influenced by their interactions with AI systems and by AI hype. In his review of The Myth of Artificial Intelligence: Why Computers Can’t Think the Way We Do by Erik J. Larson, William Hasselberger warns that in overestimating AI’s ability to mimic human intelligence we devalue the human and overlook human capacities that are integral to everyday life decision making, understanding, and reasoning. Hasselberger provides examples of both academic and everyday common-sense reasoning that continue to be out of reach for AI. He provides a historical overview of debates around the limits of artificial intelligence and its implications for our understanding of human intelligence, citing the likes of Alan Turing and Marvin Minsky as well as contemporary discussions of data-driven language models.
Gwo-Jen Hwang and Nian-Shing Chen, “ Exploring the Potential of Generative Artificial Intelligence in Education: Applications, Challenges, and Future Research Directions ,” Educational Technology & Society 26, no. 2 (2023).
Gwo-Jen Hwang and Nian-Shing Chen are enthusiastic about the potential benefits of incorporating generative AI into education. They outline a variety of roles a large language model like ChatGPT might play, from student to tutor to peer to domain expert to administrator. For example, educators might assign students to “teach” ChatGPT on a subject. Hwang and Chen provide sample ChatGPT session transcripts to illustrate their suggestions. They share prompting techniques to help educators better design AI-based teaching strategies. At the same time, they are concerned about student overreliance on generative AI. They urge educators to guide students to use it critically and to reflect on their interactions with AI. Hwang and Chen don’t touch on concerns about bias, inaccuracy, or fabrication, but they call for further research into the impact of integrating generative AI on learning outcomes.
Weekly Newsletter
Get your fix of JSTOR Daily’s best stories in your inbox each Thursday.
Privacy Policy Contact Us You may unsubscribe at any time by clicking on the provided link on any marketing message.
Lauren Goodlad and Samuel Baker, “ Now the Humanities Can Disrupt ‘AI’ ,” Public Books (February 20, 2023).
Lauren Goodlad and Samuel Baker situate both academic integrity concerns and the pressures on educators to “embrace” AI in the context of market forces. They ground their discussion of AI risks in a deep technical understanding of the limits of predictive models at mimicking human intelligence. Goodlad and Baker urge educators to communicate the purpose and value of teaching with writing to help students engage with the plurality of the world and communicate with others. Beyond the classroom, they argue, educators should question tech industry narratives and participate in public discussion on regulation and the future of AI. They see higher education as resilient: academic skepticism about former waves of hype around MOOCs, for example, suggests that educators will not likely be dazzled or terrified into submission to AI. Goodlad and Baker hope we will instead take up our place as experts who should help shape the future of the role of machines in human thought and communication.
Kathryn Conrad, “ Sneak Preview: A Blueprint for an AI Bill of Rights for Education ,” Critical AI 2.1 (July 17, 2023).
How can the field of education put the needs of students and scholars first as we shape our response to AI, the way we teach about it, and the way we might incorporate it into pedagogy? Kathryn Conrad’s manifesto builds on and extends the Biden administration’s Office of Science and Technology Policy 2022 “Blueprint for an AI Bill of Rights.” Conrad argues that educators should have input into institutional policies on AI and access to professional development around AI. Instructors should be able to decide whether and how to incorporate AI into pedagogy, basing their decisions on expert recommendations and peer-reviewed research. Conrad outlines student rights around AI systems, including the right to know when AI is being used to evaluate them and the right to request alternate human evaluation. They deserve detailed instructor guidance on policies around AI use without fear of reprisals. Conrad maintains that students should be able to appeal any charges of academic misconduct involving AI, and they should be offered alternatives to any AI-based assignments that might put their creative work at risk of exposure or use without compensation. Both students’ and educators’ legal rights must be respected in any educational application of automated generative systems.
Support JSTOR Daily! Join our new membership program on Patreon today.
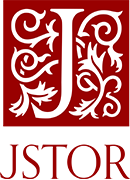
JSTOR is a digital library for scholars, researchers, and students. JSTOR Daily readers can access the original research behind our articles for free on JSTOR.
Get Our Newsletter
More stories.
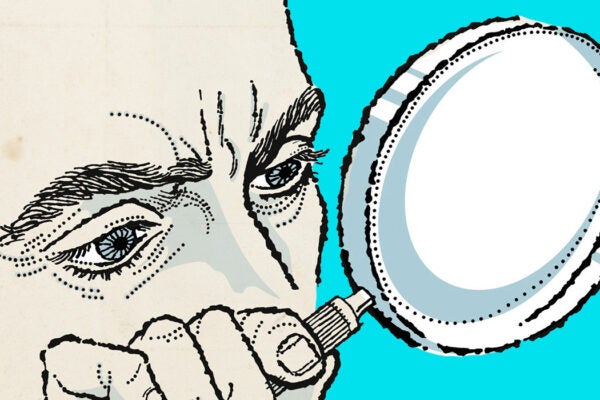
From Gamification to Game-Based Learning
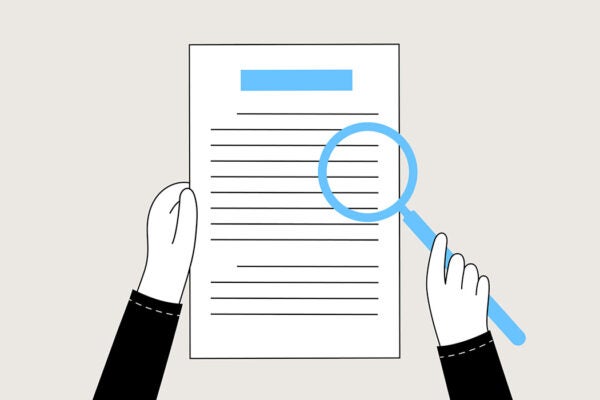
The History of Peer Review Is More Interesting Than You Think
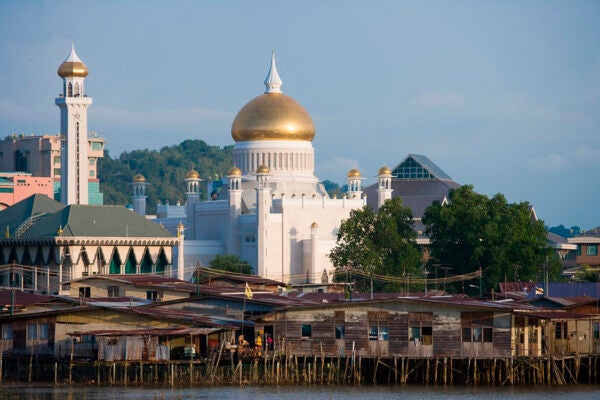
Brunei: A Tale of Soil and Oil
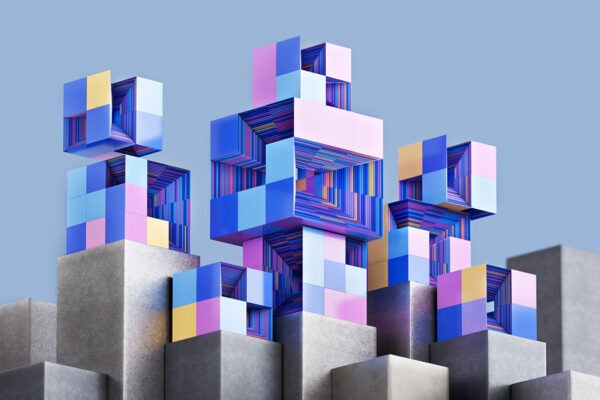
Why Architects Need Philosophy to Guide the AI Design Revolution
Recent posts.
- From Folkway to Art: The Transformation of Quilts
- The “Soundscape” Heard ’Round the World
- Olympic Tech, Emotional Dogs, and Atlantic Currents
- The Spiritual Side of Calligraphy
- All The Way With LBJ?
Support JSTOR Daily
Sign up for our weekly newsletter.
IEEE Account
- Change Username/Password
- Update Address
Purchase Details
- Payment Options
- Order History
- View Purchased Documents
Profile Information
- Communications Preferences
- Profession and Education
- Technical Interests
- US & Canada: +1 800 678 4333
- Worldwide: +1 732 981 0060
- Contact & Support
- About IEEE Xplore
- Accessibility
- Terms of Use
- Nondiscrimination Policy
- Privacy & Opting Out of Cookies
A not-for-profit organization, IEEE is the world's largest technical professional organization dedicated to advancing technology for the benefit of humanity. © Copyright 2024 IEEE - All rights reserved. Use of this web site signifies your agreement to the terms and conditions.
- Review article
- Open access
- Published: 28 October 2019
Systematic review of research on artificial intelligence applications in higher education – where are the educators?
- Olaf Zawacki-Richter ORCID: orcid.org/0000-0003-1482-8303 1 ,
- Victoria I. Marín ORCID: orcid.org/0000-0002-4673-6190 1 ,
- Melissa Bond ORCID: orcid.org/0000-0002-8267-031X 1 &
- Franziska Gouverneur 1
International Journal of Educational Technology in Higher Education volume 16 , Article number: 39 ( 2019 ) Cite this article
320k Accesses
903 Citations
244 Altmetric
Metrics details
According to various international reports, Artificial Intelligence in Education (AIEd) is one of the currently emerging fields in educational technology. Whilst it has been around for about 30 years, it is still unclear for educators how to make pedagogical advantage of it on a broader scale, and how it can actually impact meaningfully on teaching and learning in higher education. This paper seeks to provide an overview of research on AI applications in higher education through a systematic review. Out of 2656 initially identified publications for the period between 2007 and 2018, 146 articles were included for final synthesis, according to explicit inclusion and exclusion criteria. The descriptive results show that most of the disciplines involved in AIEd papers come from Computer Science and STEM, and that quantitative methods were the most frequently used in empirical studies. The synthesis of results presents four areas of AIEd applications in academic support services, and institutional and administrative services: 1. profiling and prediction, 2. assessment and evaluation, 3. adaptive systems and personalisation, and 4. intelligent tutoring systems. The conclusions reflect on the almost lack of critical reflection of challenges and risks of AIEd, the weak connection to theoretical pedagogical perspectives, and the need for further exploration of ethical and educational approaches in the application of AIEd in higher education.
Introduction
Artificial intelligence (AI) applications in education are on the rise and have received a lot of attention in the last couple of years. AI and adaptive learning technologies are prominently featured as important developments in educational technology in the 2018 Horizon report (Educause, 2018 ), with a time to adoption of 2 or 3 years. According to the report, experts anticipate AI in education to grow by 43% in the period 2018–2022, although the Horizon Report 2019 Higher Education Edition (Educause, 2019 ) predicts that AI applications related to teaching and learning are projected to grow even more significantly than this. Contact North, a major Canadian non-profit online learning society, concludes that “there is little doubt that the [AI] technology is inexorably linked to the future of higher education” (Contact North, 2018 , p. 5). With heavy investments by private companies such as Google, which acquired European AI start-up Deep Mind for $400 million, and also non-profit public-private partnerships such as the German Research Centre for Artificial Intelligence Footnote 1 (DFKI), it is very likely that this wave of interest will soon have a significant impact on higher education institutions (Popenici & Kerr, 2017 ). The Technical University of Eindhoven in the Netherlands, for example, recently announced that they will launch an Artificial Intelligence Systems Institute with 50 new professorships for education and research in AI. Footnote 2
The application of AI in education (AIEd) has been the subject of research for about 30 years. The International AIEd Society (IAIED) was launched in 1997, and publishes the International Journal of AI in Education (IJAIED), with the 20th annual AIEd conference being organised this year. However, on a broader scale, educators have just started to explore the potential pedagogical opportunities that AI applications afford for supporting learners during the student life cycle.
Despite the enormous opportunities that AI might afford to support teaching and learning, new ethical implications and risks come in with the development of AI applications in higher education. For example, in times of budget cuts, it might be tempting for administrators to replace teaching by profitable automated AI solutions. Faculty members, teaching assistants, student counsellors, and administrative staff may fear that intelligent tutors, expert systems and chat bots will take their jobs. AI has the potential to advance the capabilities of learning analytics, but on the other hand, such systems require huge amounts of data, including confidential information about students and faculty, which raises serious issues of privacy and data protection. Some institutions have recently been established, such as the Institute for Ethical AI in Education Footnote 3 in the UK, to produce a framework for ethical governance for AI in education, and the Analysis & Policy Observatory published a discussion paper in April 2019 to develop an AI ethics framework for Australia. Footnote 4
Russel and Norvig ( 2010 ) remind us in their leading textbook on artificial intelligence, “All AI researchers should be concerned with the ethical implications of their work” (p. 1020). Thus, we would like to explore what kind of fresh ethical implications and risks are reflected by the authors in the field of AI enhanced education. The aim of this article is to provide an overview for educators of research on AI applications in higher education. Given the dynamic development in recent years, and the growing interest of educators in this field, a review of the literature on AI in higher education is warranted.
Specifically, this paper addresses the following research questions in three areas, by means of a systematic review (see Gough, Oliver, & Thomas, 2017 ; Petticrew & Roberts, 2006 ):
How have publications on AI in higher education developed over time, in which journals are they published, and where are they coming from in terms of geographical distribution and the author’s disciplinary affiliations?
How is AI in education conceptualised and what kind of ethical implications, challenges and risks are considered?
What is the nature and scope of AI applications in the context of higher education?
The field AI originates from computer science and engineering, but it is strongly influenced by other disciplines such as philosophy, cognitive science, neuroscience, and economics. Given the interdisciplinary nature of the field, there is little agreement among AI researchers on a common definition and understanding of AI – and intelligence in general (see Tegmark, 2018 ). With regard to the introduction of AI-based tools and services in higher education, Hinojo-Lucena, Aznar-Díaz, Cáceres-Reche, and Romero-Rodríguez ( 2019 ) note that “this technology [AI] is already being introduced in the field of higher education, although many teachers are unaware of its scope and, above all, of what it consists of” (p. 1). For the purpose of our analysis of artificial intelligence in higher education, it is desirable to clarify terminology. Thus, in the next section, we explore definitions of AI in education, and the elements and methods that AI applications might entail in higher education, before we proceed with the systematic review of the literature.
AI in education (AIEd)
The birth of AI goes back to the 1950s when John McCarthy organised a two-month workshop at Dartmouth College in the USA. In the workshop proposal, McCarthy used the term artificial intelligence for the first time in 1956 (Russel & Norvig, 2010 , p. 17):
The study [of artificial intelligence] is to proceed on the basis of the conjecture that every aspect of learning or any other feature of intelligence can in principle be so precisely described that a machine can be made to simulate it. An attempt will be made to find how to make machines use language, form abstractions and concepts, solve kinds of problems now reserved for humans, and improve themselves.
Baker and Smith ( 2019 ) provide a broad definition of AI: “Computers which perform cognitive tasks, usually associated with human minds, particularly learning and problem-solving” (p. 10). They explain that AI does not describe a single technology. It is an umbrella term to describe a range of technologies and methods, such as machine learning, natural language processing, data mining, neural networks or an algorithm.
AI and machine learning are often mentioned in the same breath. Machine learning is a method of AI for supervised and unsupervised classification and profiling, for example to predict the likelihood of a student to drop out from a course or being admitted to a program, or to identify topics in written assignments. Popenici and Kerr ( 2017 ) define machine learning “as a subfield of artificial intelligence that includes software able to recognise patterns, make predictions, and apply newly discovered patterns to situations that were not included or covered by their initial design” (p. 2).
The concept of rational agents is central to AI: “An agent is anything that can be viewed as perceiving its environment through sensors and acting upon that environment through actuators” (Russel & Norvig, 2010 , p. 34). The vacuum-cleaner robot is a very simple form of an intelligent agent, but things become very complex and open-ended when we think about an automated taxi.
Experts in the field distinguish between weak and strong AI (see Russel & Norvig, 2010 , p. 1020) or narrow and general AI (see Baker & Smith, 2019 , p. 10). A philosophical question remains whether machines will be able to actually think or even develop consciousness in the future, rather than just simulating thinking and showing rational behaviour. It is unlikely that such strong or general AI will exist in the near future. We are therefore dealing here with GOFAI (“ good old-fashioned AI ”, a term coined by the philosopher John Haugeland, 1985 ) in higher education – in the sense of agents and information systems that act as if they were intelligent.
Given this understanding of AI, what are potential areas of AI applications in education, and higher education in particular? Luckin, Holmes, Griffiths, and Forcier ( 2016 ) describe three categories of AI software applications in education that are available today: a) personal tutors, b) intelligent support for collaborative learning, and c) intelligent virtual reality.
Intelligent tutoring systems (ITS) can be used to simulate one-to-one personal tutoring. Based on learner models, algorithms and neural networks, they can make decisions about the learning path of an individual student and the content to select, provide cognitive scaffolding and help, to engage the student in dialogue. ITS have enormous potential, especially in large-scale distance teaching institutions, which run modules with thousands of students, where human one-to-one tutoring is impossible. A vast array of research shows that learning is a social exercise; interaction and collaboration are at the heart of the learning process (see for example Jonassen, Davidson, Collins, Campbell, & Haag, 1995 ). However, online collaboration has to be facilitated and moderated (Salmon, 2000 ). AIEd can contribute to collaborative learning by supporting adaptive group formation based on learner models, by facilitating online group interaction or by summarising discussions that can be used by a human tutor to guide students towards the aims and objectives of a course. Finally, also drawing on ITS, intelligent virtual reality (IVR) is used to engage and guide students in authentic virtual reality and game-based learning environments. Virtual agents can act as teachers, facilitators or students’ peers, for example, in virtual or remote labs (Perez et al., 2017 ).
With the advancement of AIEd and the availability of (big) student data and learning analytics, Luckin et al. ( 2016 ) claim a “[r] enaissance in assessment” (p. 35). AI can provide just-in-time feedback and assessment. Rather than stop-and-test, AIEd can be built into learning activities for an ongoing analysis of student achievement. Algorithms have been used to predict the probability of a student failing an assignment or dropping out of a course with high levels of accuracy (e.g. Bahadır, 2016 ).
In their recent report, Baker and Smith ( 2019 ) approach educational AI tools from three different perspectives; a) learner-facing, b) teacher-facing, and c) system-facing AIEd. Learner-facing AI tools are software that students use to learn a subject matter, i.e. adaptive or personalised learning management systems or ITS. Teacher-facing systems are used to support the teacher and reduce his or her workload by automating tasks such as administration, assessment, feedback and plagiarism detection. AIEd tools also provide insight into the learning progress of students so that the teacher can proactively offer support and guidance where needed. System-facing AIEd are tools that provide information for administrators and managers on the institutional level, for example to monitor attrition patterns across faculties or colleges.
In the context of higher education, we use the concept of the student life-cycle (see Reid, 1995 ) as a framework to describe the various AI based services on the broader institutional and administrative level, as well as for supporting the academic teaching and learning process in the narrower sense.
The purpose of a systematic review is to answer specific questions, based on an explicit, systematic and replicable search strategy, with inclusion and exclusion criteria identifying studies to be included or excluded (Gough, Oliver & Thomas, 2017 ). Data is then coded and extracted from included studies, in order to synthesise findings and to shine light on their application in practice, as well as on gaps or contradictions. This contribution maps 146 articles on the topic of artificial intelligence in higher education.
Search strategy
The initial search string (see Table 1 ) and criteria (see Table 2 ) for this systematic review included peer-reviewed articles in English, reporting on artificial intelligence within education at any level, and indexed in three international databases; EBSCO Education Source, Web of Science and Scopus (covering titles, abstracts, and keywords). Whilst there are concerns about peer-review processes within the scientific community (e.g., Smith, 2006 ), articles in this review were limited to those published in peer-reviewed journals, due to their general trustworthiness in academia and the rigorous review processes undertaken (Nicholas et al., 2015 ). The search was undertaken in November 2018, with an initial 2656 records identified.
After duplicates were removed, it was decided to limit articles to those published during or after 2007, as this was the year that iPhone’s Siri was introduced; an algorithm-based personal assistant, started as an artificial intelligence project funded by the US Defense Advanced Research Projects Agency (DARPA) in 2001, turned into a company that was acquired by Apple Inc. It was also decided that the corpus would be limited to articles discussing applications of artificial intelligence in higher education only.
Screening and inter-rater reliability
The screening of 1549 titles and abstracts was carried out by a team of three coders and at this first screening stage, there was a requirement of sensitivity rather than specificity, i.e. papers were included rather than excluded. In order to reach consensus, the reasons for inclusion and exclusion for the first 80 articles were discussed at regular meetings. Twenty articles were randomly selected to evaluate the coding decisions of the three coders (A, B and C) to determine inter-rater reliability using Cohen’s kappa (κ) (Cohen, 1960 ), which is a coefficient for the degree of consistency among raters, based on the number of codes in the coding scheme (Neumann, 2007 , p. 326). Kappa values of .40–.60 are characterised as fair, .60 to .75 as good, and over .75 as excellent (Bakeman & Gottman, 1997 ; Fleiss, 1981 ). Coding consistency for inclusion or exclusion of articles between rater A and B was κ = .79, between rater A and C it was κ = .89, and between rater B and C it was κ = .69 (median = .79). Therefore, inter-rater reliability can be considered as excellent for the coding of inclusion and exclusion criteria.
After initial screening, 332 potential articles remained for screening on full text (see Fig. 1 ). However, 41 articles could not be retrieved, either through the library order scheme or by contacting authors. Therefore, 291 articles were retrieved, screened and coded, and following the exclusion of 149 papers, 146 articles remained for synthesis. Footnote 5
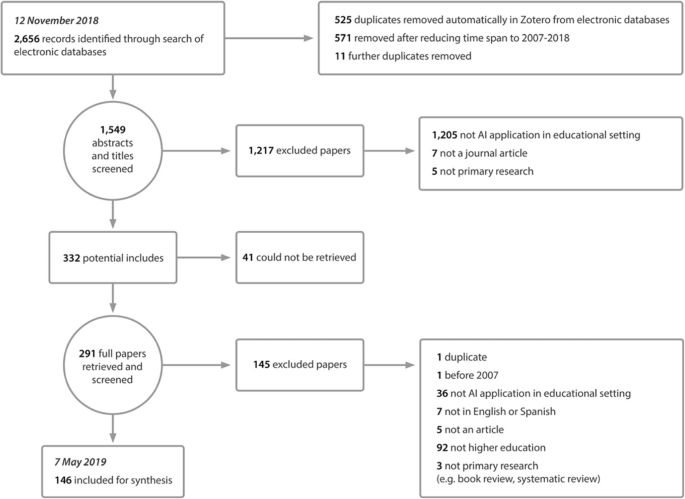
PRISMA diagram (slightly modified after Brunton & Thomas, 2012 , p. 86; Moher, Liberati, Tetzlaff, & Altman, 2009 , p. 8)
Coding, data extraction and analysis
In order to extract the data, all articles were uploaded into systematic review software EPPI Reviewer Footnote 6 and a coding system was developed. Codes included article information (year of publication, journal name, countries of authorship, discipline of first author), study design and execution (empirical or descriptive, educational setting) and how artificial intelligence was used (applications in the student life cycle, specific applications and methods). Articles were also coded on whether challenges and benefits of AI were present, and whether AI was defined. Descriptive data analysis was carried out with the statistics software R using the tidyr package (Wickham & Grolemund, 2016 ).
Limitations
Whilst this systematic review was undertaken as rigorously as possible, each review is limited by its search strategy. Although the three educational research databases chosen are large and international in scope, by applying the criteria of peer-reviewed articles published only in English or Spanish, research published on AI in other languages were not included in this review. This also applies to research in conference proceedings, book chapters or grey literature, or those articles not published in journals that are indexed in the three databases searched. In addition, although Spanish peer-reviewed articles were added according to inclusion criteria, no specific search string in the language was included, which narrows down the possibility of including Spanish papers that were not indexed with the chosen keywords. Future research could consider using a larger number of databases, publication types and publication languages, in order to widen the scope of the review. However, serious consideration would then need to be given to project resources and the manageability of the review (see Authors, in press).
Journals, authorship patterns and methods
Articles per year.
There was a noticeable increase in the papers published from 2007 onwards. The number of included articles grew from six in 2007 to 23 in 2018 (see Fig. 2 ).
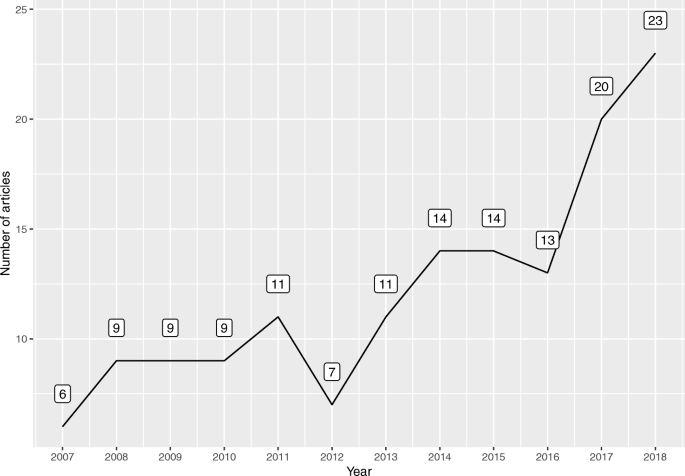
Number of included articles per year ( n = 146)
The papers included in the sample were published in 104 different journals. The greatest number of articles were published in the International Journal of Artificial Intelligence in Education ( n = 11) , followed by Computers & Education ( n = 8) , and the International Journal of Emerging Technologies in Learning ( n = 5) . Table 3 lists 19 journals that published at least two articles on AI in higher education from 2007 to 2018.
For the geographical distribution analysis of articles, the country of origin of the first author was taken into consideration ( n = 38 countries). Table 4 shows 19 countries that contributed at least two papers, and it reveals that 50% of all articles come from only four countries: USA, China, Taiwan, and Turkey.
Author affiliations
Again, the affiliation of the first author was taken into consideration (see Table 5 ). Researchers working in departments of Computer Science contributed by far the greatest number of papers ( n = 61) followed by Science, Technology, Engineering and Mathematics (STEM) departments ( n = 29). Only nine first authors came from an Education department, some reported dual affiliation with Education and Computer Science ( n = 2), Education and Psychology ( n = 1), or Education and STEM ( n = 1).
Thus, 13 papers (8.9%) were written by first authors with an Education background. It is noticeable that three of them were contributed by researchers from the Teachers College at Columbia University, New York, USA (Baker, 2016 ; Paquette, Lebeau, Beaulieu, & Mayers, 2015 ; Perin & Lauterbach, 2018 ) – and they were all published in the same journal, i.e. the International Journal of Artificial Intelligence in Education .
Thirty studies (20.5%) were coded as being theoretical or descriptive in nature. The vast majority of studies (73.3%) applied quantitative methods, whilst only one (0.7%) was qualitative in nature and eight (5.5%) followed a mixed-methods approach. The purpose of the qualitative study, involving interviews with ESL students, was to explore the nature of written feedback coming from an automated essay scoring system compared to a human teacher (Dikli, 2010 ). In many cases, authors employed quasi-experimental methods, being an intentional sample divided into the experimental group, where an AI application (e.g. an intelligent tutoring system) was applied, and the control group without the intervention, followed by pre- and posttest (e.g. Adamson, Dyke, Jang, & Rosé, 2014 ).
Understanding of AI and critical reflection of challenges and risks
There are many different types and levels of AI mentioned in the articles, however only five out of 146 included articles (3.4%) provide an explicit definition of the term “Artificial Intelligence”. The main characteristics of AI, described in all five studies, are the parallels between the human brain and artificial intelligence. The authors conceptualise AI as intelligent computer systems or intelligent agents with human features, such as the ability to memorise knowledge, to perceive and manipulate their environment in a similar way as humans, and to understand human natural language (see Huang, 2018 ; Lodhi, Mishra, Jain, & Bajaj, 2018 ; Welham, 2008 ). Dodigovic ( 2007 ) defines AI in her article as follows (p. 100):
Artificial intelligence (AI) is a term referring to machines which emulate the behaviour of intelligent beings [ … ] AI is an interdisciplinary area of knowledge and research, whose aim is to understand how the human mind works and how to apply the same principles in technology design. In language learning and teaching tasks, AI can be used to emulate the behaviour of a teacher or a learner [ … ] . (p. 100)
Dodigovic is the only author who gives a definition of AI, and comes from an Arts, Humanities and Social Science department, taking into account aspects of AI and intelligent tutors in second language learning.
A stunningly low number of authors, only two out of 146 articles (1.4%), critically reflect upon ethical implications, challenges and risks of applying AI in education. Li ( 2007 ) deals with privacy concerns in his article about intelligent agent supported online learning:
Privacy is also an important concern in applying agent-based personalised education. As discussed above, agents can autonomously learn many of students’ personal information, like learning style and learning capability. In fact, personal information is private. Many students do not want others to know their private information, such as learning styles and/or capabilities. Students might show concern over possible discrimination from instructors in reference to learning performance due to special learning needs. Therefore, the privacy issue must be resolved before applying agent-based personalised teaching and learning technologies. (p. 327)
Another challenge of applying AI is mentioned by Welham ( 2008 , p. 295) concerning the costs and time involved in developing and introducing AI-based methods that many public educational institutions cannot afford.
AI applications in higher education
As mentioned before, we used the concept of the student life-cycle (see Reid, 1995 ) as a framework to describe the various AI based services at the institutional and administrative level (e.g. admission, counselling, library services), as well as at the academic support level for teaching and learning (e.g. assessment, feedback, tutoring). Ninety-two studies (63.0%) were coded as relating to academic support services and 48 (32.8%) as administrative and institutional services; six studies (4.1%) covered both levels. The majority of studies addressed undergraduate students ( n = 91, 62.3%) compared to 11 (7.5%) focussing on postgraduate students, and another 44 (30.1%) that did not specify the study level.
The iterative coding process led to the following four areas of AI applications with 17 sub-categories, covered in the publications: a) adaptive systems and personalisation, b) assessment and evaluation, c) profiling and prediction, and d) intelligent tutoring systems. Some studies addressed AI applications in more than one area (see Table 6 ).
The nature and scope of the various AI applications in higher education will be described along the lines of these four application categories in the following synthesis.
Profiling and prediction
The basis for many AI applications are learner models or profiles that allow prediction, for example of the likelihood of a student dropping out of a course or being admitted to a programme, in order to offer timely support or to provide feedback and guidance in content related matters throughout the learning process. Classification, modelling and prediction are an essential part of educational data mining (Phani Krishna, Mani Kumar, & Aruna Sri, 2018 ).
Most of the articles (55.2%, n = 32) address issues related to the institutional and administrative level, many (36.2%, n = 21) are related to academic teaching and learning at the course level, and five (8.6%) are concerned with both levels. Articles dealing with profiling and prediction were classified into three sub-categories; admission decisions and course scheduling ( n = 7), drop-out and retention ( n = 23), and student models and academic achievement ( n = 27). One study that does not fall into any of these categories is the study by Ge and Xie ( 2015 ), which is concerned with forecasting the costs of a Chinese university to support management decisions based on an artificial neural network.
All of the 58 studies in this area applied machine learning methods, to recognise and classify patterns, and to model student profiles to make predictions. Thus, they are all quantitative in nature. Many studies applied several machine learning algorithms (e.g. ANN, SVM, RF, NB; see Table 7 ) Footnote 7 and compared their overall prediction accuracy with conventional logistic regression. Table 7 shows that machine learning methods outperformed logistic regression in all studies in terms of their classification accuracy in percent. To evaluate the performance of classifiers, the F1-score can also be used, which takes into account the number of positive instances correctly classified as positive, the number of negative instances incorrectly classified as positive, and the number of positive instances incorrectly classified as negative (Umer et al., 2017 ; for a brief overview of measures of diagnostic accuracy, see Šimundić, 2009 ). The F1-score ranges between 0 and 1 with its best value at 1 (perfect precision and recall). Yoo and Kim ( 2014 ) reported high F1-scores of 0.848, 0.911, and 0.914 for J48, NB, and SVM, in a study to predict student’s group project performance from online discussion participation.
Admission decisions and course scheduling
Chen and Do ( 2014 ) point out that “the accurate prediction of students’ academic performance is of importance for making admission decisions as well as providing better educational services” (p. 18). Four studies aimed to predict whether or not a prospective student would be admitted to university. For example, Acikkar and Akay ( 2009 ) selected candidates for a School of Physical Education and Sports in Turkey based on a physical ability test, their scores in the National Selection and Placement Examination, and their graduation grade point average (GPA). They used the support vector machine (SVM) technique to classify the students and where able to predict admission decisions on a level of accuracy of 97.17% in 2006 and 90.51% in 2007. SVM was also applied by Andris, Cowen, and Wittenbach ( 2013 ) to find spatial patterns that might favour prospective college students from certain geographic regions in the USA. Feng, Zhou, and Liu ( 2011 ) analysed enrolment data from 25 Chinese provinces as the training data to predict registration rates in other provinces using an artificial neural network (ANN) model. Machine learning methods and ANN are also used to predict student course selection behaviour to support course planning. Kardan, Sadeghi, Ghidary, and Sani ( 2013 ) investigated factors influencing student course selection, such as course and instructor characteristics, workload, mode of delivery and examination time, to develop a model to predict course selection with an ANN in two Computer Engineering and Information Technology Masters programs. In another paper from the same author team, a decision support system for course offerings was proposed (Kardan & Sadeghi, 2013 ). Overall, the research shows that admission decisions can be predicted at high levels of accuracy, so that an AI solution could relieves the administrative staff and allows them to focus on the more difficult cases.
Drop-out and retention
Studies pertaining to drop-out and retention are intended to develop early warning systems to detect at-risk students in their first year (e.g., Alkhasawneh & Hargraves, 2014 ; Aluko, Adenuga, Kukoyi, Soyingbe, & Oyedeji, 2016 ; Hoffait & Schyns, 2017 ; Howard, Meehan, & Parnell, 2018 ) or to predict the attrition of undergraduate students in general (e.g., Oztekin, 2016 ; Raju & Schumacker, 2015 ). Delen ( 2011 ) used institutional data from 25,224 students enrolled as Freshmen in an American university over 8 years. In this study, three classification techniques were used to predict dropout: ANN, decision trees (DT) and logistic regression. The data contained variables related to students’ demographic, academic, and financial characteristics (e.g. age, sex, ethnicity, GPA, TOEFL score, financial aid, student loan, etc.). Based on a 10-fold cross validation, Delen ( 2011 ) found that the ANN model worked best with an accuracy rate of 81.19% (see Table 7 ) and he concluded that the most important predictors of student drop-out are related to the student’s past and present academic achievement, and whether they receive financial support. Sultana, Khan, and Abbas ( 2017 , p. 107) discussed the impact of cognitive and non-cognitive features of students for predicting academic performance of undergraduate engineering students. In contrast to many other studies, they focused on non-cognitive variables to improve prediction accuracy, i.e. time management, self-concept, self-appraisal, leadership, and community support.
Student models and academic achievement
Many more studies are concerned with profiling students and modelling learning behaviour to predict their academic achievements at the course level. Hussain et al. ( 2018 ) applied several machine learning algorithms to analyse student behavioural data from the virtual learning environment at the Open University UK, in order to predict student engagement, which is of particular importance at a large scale distance teaching university, where it is not possible to engage the majority of students in face-to-face sessions. The authors aim to develop an intelligent predictive system that enables instructors to automatically identify low-engaged students and then to make an intervention. Spikol, Ruffaldi, Dabisias, and Cukurova ( 2018 ) used face and hand tracking in workshops with engineering students to estimate success in project-based learning. They concluded that results generated from multimodal data can be used to inform teachers about key features of project-based learning activities. Blikstein et al. ( 2014 ) investigated patterns of how undergraduate students learn computer programming, based on over 150,000 code transcripts that the students created in software development projects. They found that their model, based on the process of programming, had better predictive power than the midterm grades. Another example is the study of Babić ( 2017 ), who developed a model to predict student academic motivation based on their behaviour in an online learning environment.
The research on student models is an important foundation for the design of intelligent tutoring systems and adaptive learning environments.
- Intelligent tutoring systems
All of the studies investigating intelligent tutoring systems (ITS) ( n = 29) are only concerned with the teaching and learning level, except for one that is contextualised at the institutional and administrative level. The latter presents StuA , an interactive and intelligent student assistant that helps newcomers in a college by answering queries related to faculty members, examinations, extra curriculum activities, library services, etc. (Lodhi et al., 2018 ).
The most common terms for referring to ITS described in the studies are intelligent (online) tutors or intelligent tutoring systems (e.g., in Dodigovic, 2007 ; Miwa, Terai, Kanzaki, & Nakaike, 2014 ), although they are also identified often as intelligent (software) agents (e.g., Schiaffino, Garcia, & Amandi, 2008 ), or intelligent assistants (e.g., in Casamayor, Amandi, & Campo, 2009 ; Jeschike, Jeschke, Pfeiffer, Reinhard, & Richter, 2007 ). According to Welham ( 2008 ), the first ITS reported was the SCHOLAR system, launched in 1970, which allowed the reciprocal exchange of questions between teacher and student, but not holding a continuous conversation.
Huang and Chen ( 2016 , p. 341) describe the different models that are usually integrated in ITS: the student model (e.g. information about the student’s knowledge level, cognitive ability, learning motivation, learning styles), the teacher model (e.g. analysis of the current state of students, select teaching strategies and methods, provide help and guidance), the domain model (knowledge representation of both students and teachers) and the diagnosis model (evaluation of errors and defects based on domain model).
The implementation and validation of the ITS presented in the studies usually took place over short-term periods (a course or a semester) and no longitudinal studies were identified, except for the study by Jackson and Cossitt ( 2015 ). On the other hand, most of the studies showed (sometimes slightly) positive / satisfactory preliminary results regarding the performance of the ITS, but they did not take into account the novelty effect that a new technological development could have in an educational context. One study presented negative results regarding the type of support that the ITS provided (Adamson et al., 2014 ), which could have been more useful if it was more adjusted to the type of (in this case, more advanced) learners.
Overall, more research is needed on the effectiveness of ITS. The last meta-analysis of 39 ITS studies was published over 5 years ago: Steenbergen-Hu and Cooper ( 2014 ) found that ITS had a moderate effect of students’ learning, and that ITS were less effective that human tutoring, but ITS outperformed all other instruction methods (such as traditional classroom instruction, reading printed or digital text, or homework assignments).
The studies addressing various ITS functions were classified as follows: teaching course content ( n = 12), diagnosing strengths or gaps in students’ knowledge and providing automated feedback ( n = 7), curating learning materials based on students’ needs ( n = 3), and facilitating collaboration between learners ( n = 2).
Teaching course content
Most of the studies ( n = 4) within this group focused on teaching Computer Science content (Dobre, 2014 ; Hooshyar, Ahmad, Yousefi, Yusop, & Horng, 2015 ; Howard, Jordan, di Eugenio, & Katz, 2017 ; Shen & Yang, 2011 ). Other studies included ITS teaching content for Mathematics (Miwa et al., 2014 ), Business Statistics and Accounting (Jackson & Cossitt, 2015 ; Palocsay & Stevens, 2008 ), Medicine (Payne et al., 2009 ) and writing and reading comprehension strategies for undergraduate Psychology students (Ray & Belden, 2007 ; Weston-Sementelli, Allen, & McNamara, 2018 ). Overall, these ITS focused on providing teaching content to students and, at the same time, supporting them by giving adaptive feedback and hints to solve questions related to the content, as well as detecting students’ difficulties/errors when working with the content or the exercises. This is made possible by monitoring students’ actions with the ITS.
In the study by Crown, Fuentes, Jones, Nambiar, and Crown ( 2011 ), a combination of teaching content through dialogue with a chatbot, that at the same time learns from this conversation - defined as a text-based conversational agent -, is described, which moves towards a more active, reflective and thinking student-centred learning approach. Duffy and Azevedo ( 2015 ) present an ITS called MetaTutor, which is designed to teach students about the human circulatory system, but it also puts emphasis on supporting students’ self-regulatory processes assisted by the features included in the MetaTutor system (a timer, a toolbar to interact with different learning strategies, and learning goals, amongst others).
Diagnosing strengths or gaps in student knowledge, and providing automated feedback
In most of the studies ( n = 4) of this group, ITS are presented as a rather one-way communication from computer to student, concerning the gaps in students’ knowledge and the provision of feedback. Three examples in the field of STEM have been found: two of them where the virtual assistance is presented as a feature in virtual laboratories by tutoring feedback and supervising student behaviour (Duarte, Butz, Miller, & Mahalingam, 2008 ; Ramírez, Rico, Riofrío-Luzcando, Berrocal-Lobo, & Antonio, 2018 ), and the third one is a stand-alone ITS in the field of Computer Science (Paquette et al., 2015 ). One study presents an ITS of this kind in the field of second language learning (Dodigovic, 2007 ).
In two studies, the function of diagnosing mistakes and the provision of feedback is accomplished by a dialogue between the student and the computer. For example, with an interactive ubiquitous teaching robot that bases its speech on question recognition (Umarani, Raviram, & Wahidabanu, 2011 ), or with the tutoring system, based on a tutorial dialogue toolkit for introductory college Physics (Chi, VanLehn, Litman, & Jordan, 2011 ). The same tutorial dialogue toolkit (TuTalk) is the core of the peer dialogue agent presented by Howard et al. ( 2017 ), where the ITS engages in a one-on-one problem-solving peer interaction with a student and can interact verbally, graphically and in a process-oriented way, and engage in collaborative problem solving instead of tutoring. This last study could be considered as part of a new category regarding peer-agent collaboration.
Curating learning materials based on student needs
Two studies focused on this kind of ITS function (Jeschike et al., 2007 ; Schiaffino et al., 2008 ), and a third one mentions it in a more descriptive way as a feature of the detection system presented (Hall Jr & Ko, 2008 ). Schiaffino et al. ( 2008 ) present eTeacher as a system for personalised assistance to e-learning students by observing their behaviour in the course and generating a student’s profile. This enables the system to provide specific recommendations regarding the type of reading material and exercises done, as well as personalised courses of action. Jeschike et al. ( 2007 ) refers to an intelligent assistant contextualised in a virtual laboratory of statistical mechanics, where it presents exercises and the evaluation of the learners’ input to content, and interactive course material that adapts to the learner.
Facilitating collaboration between learners
Within this group we can identify only two studies: one focusing on supporting online collaborative learning discussions by using academically productive talk moves (Adamson et al., 2014 ); and the second one, on facilitating collaborative writing by providing automated feedback, generated automatic questions, and the analysis of the process (Calvo, O’Rourke, Jones, Yacef, & Reimann, 2011 ). Given the opportunities that the applications described in these studies afford for supporting collaboration among students, more research in this area would be desireable.
The teachers’ perspective
As mentioned above, Baker and Smith ( 2019 , p.12) distinguish between student and teacher-facing AI. However, only two included articles in ITS focus on the teacher’s perspective. Casamayor et al. ( 2009 ) focus on assisting teachers with the supervision and detection of conflictive cases in collaborative learning. In this study, the intelligent assistant provides the teachers with a summary of the individual progress of each group member and the type of participation each of them have had in their work groups, notification alerts derived from the detection of conflict situations, and information about the learning style of each student-logging interactions, so that the teachers can intervene when they consider it convenient. The other study put the emphasis on the ITS sharing teachers’ tutoring tasks by providing immediate feedback (automating tasks), and leaving the teachers the role of providing new hints and the correct solution to the tasks (Chou, Huang, & Lin, 2011 ). The study of Chi et al. ( 2011 ) also mentions the ITS purpose to share teacher’s tutoring tasks. The main aim in any of these cases is to reduce teacher’s workload. Furthermore, many of the learner-facing studies deal with the teacher-facing functions too, although they do not put emphasis on the teacher’s perspective.
Assessment and evaluation
Assessment and evaluation studies also largely focused on the level of teaching and learning (86%, n = 31), although five studies described applications at the institutional level. In order to gain an overview of student opinion about online and distance learning at their institution, academics at Anadolu University (Ozturk, Cicek, & Ergul, 2017 ) used sentiment analysis to analyse mentions by students on Twitter, using Twitter API Twython and terms relating to the system. This analysis of publicly accessible data, allowed researchers insight into student opinion, which otherwise may not have been accessible through their institutional LMS, and which can inform improvements to the system. Two studies used AI to evaluate student Prior Learning and Recognition (PLAR); Kalz et al. ( 2008 ) used Latent Semantic Analysis and ePortfolios to inform personalised learning pathways for students, and Biletska, Biletskiy, Li, and Vovk ( 2010 ) used semantic web technologies to convert student credentials from different institutions, which could also provide information from course descriptions and topics, to allow for easier granting of credit. The final article at the institutional level (Sanchez et al., 2016 ) used an algorithm to match students to professional competencies and capabilities required by companies, in order to ensure alignment between courses and industry needs.
Overall, the studies show that AI applications can perform assessment and evaluation tasks at very high accuracy and efficiency levels. However, due to the need to calibrate and train the systems (supervised machine learning), they are more applicable to courses or programs with large student numbers.
Articles focusing on assessment and evaluation applications of AI at the teaching and learning level, were classified into four sub-categories; automated grading ( n = 13), feedback ( n = 8), evaluation of student understanding, engagement and academic integrity ( n = 5), and evaluation of teaching ( n = 5).
Automated grading
Articles that utilised automated grading, or Automated Essay Scoring (AES) systems, came from a range of disciplines (e.g. Biology, Medicine, Business Studies, English as a Second Language), but were mostly focused on its use in undergraduate courses ( n = 10), including those with low reading and writing ability (Perin & Lauterbach, 2018 ). Gierl, Latifi, Lai, Boulais, and Champlain’s ( 2014 ) use of open source Java software LightSIDE to grade postgraduate medical student essays resulted in an agreement between the computer classification and human raters between 94.6% and 98.2%, which could enable reducing cost and the time associated with employing multiple human assessors for large-scale assessments (Barker, 2011 ; McNamara, Crossley, Roscoe, Allen, & Dai, 2015 ). However, they stressed that not all writing genres may be appropriate for AES and that it would be impractical to use in most small classrooms, due to the need to calibrate the system with a large number of pre-scored assessments. The benefits of using algorithms that find patterns in text responses, however, has been found to lead to encouraging more revisions by students (Ma & Slater, 2015 ) and to move away from merely measuring student knowledge and abilities by multiple choice tests (Nehm, Ha, & Mayfield, 2012 ). Continuing issues persist, however, in the quality of feedback provided by AES (Dikli, 2010 ), with Barker ( 2011 ) finding that the more detailed the feedback provided was, the more likely students were to question their grades, and a question was raised over the benefits of this feedback for beginning language students (Aluthman, 2016 ).
Articles concerned with feedback included a range of student-facing tools, including intelligent agents that provide students with prompts or guidance when they are confused or stalled in their work (Huang, Chen, Luo, Chen, & Chuang, 2008 ), software to alert trainee pilots when they are losing situation awareness whilst flying (Thatcher, 2014 ), and machine learning techniques with lexical features to generate automatic feedback and assist in improving student writing (Chodorow, Gamon, & Tetreault, 2010 ; Garcia-Gorrostieta, Lopez-Lopez, & Gonzalez-Lopez, 2018 ; Quixal & Meurers, 2016 ), which can help reduce students cognitive overload (Yang, Wong, & Yeh, 2009 ). The automated feedback system based on adaptive testing reported by Barker ( 2010 ), for example, not only determines the most appropriate individual answers according to Bloom’s cognitive levels, but also recommends additional materials and challenges.
Evaluation of student understanding, engagement and academic integrity
Three articles reported on student-facing tools that evaluate student understanding of concepts (Jain, Gurupur, Schroeder, & Faulkenberry, 2014 ; Zhu, Marquez, & Yoo, 2015 ) and provide personalised assistance (Samarakou, Fylladitakis, Früh, Hatziapostolou, & Gelegenis, 2015 ). Hussain et al. ( 2018 ) used machine learning algorithms to evaluate student engagement in a social science course at the Open University, including final results, assessment scores and the number of clicks that students make in the VLE, which can alert instructors to the need for intervention, and Amigud, Arnedo-Moreno, Daradoumis, and Guerrero-Roldan ( 2017 ) used machine learning algorithms to check academic integrity, by assessing the likelihood of student work being similar to their other work. With a mean accuracy of 93%, this opens up possibilities of reducing the need for invigilators or to access student accounts, thereby reducing concerns surrounding privacy.
Evaluation of teaching
Four studies used data mining algorithms to evaluate lecturer performance through course evaluations (Agaoglu, 2016 ; Ahmad & Rashid, 2016 ; DeCarlo & Rizk, 2010 ; Gutierrez, Canul-Reich, Ochoa Zezzatti, Margain, & Ponce, 2018 ), with Agaoglu ( 2016 ) finding, through using four different classification techniques, that many questions in the evaluation questionnaire were irrelevant. The application of an algorithm to evaluate the impact of teaching methods in a differential equations class, found that online homework with immediate feedback was more effective than clickers (Duzhin & Gustafsson, 2018 ). The study also found that, whilst previous exam results are generally good predictors for future exam results, they say very little about students’ expected performance in project-based tasks.
Adaptive systems and personalisation
Most of the studies on adaptive systems (85%, n = 23) are situated at the teaching and learning level, with four cases considering the institutional and administrative level. Two studies explored undergraduate students’ academic advising (Alfarsi, Omar, & Alsinani, 2017 ; Feghali, Zbib, & Hallal, 2011 ), and Nguyen et al. ( 2018 ) focused on AI to support university career services. Ng, Wong, Lee, and Lee ( 2011 ) reported on the development of an agent-based distance LMS, designed to manage resources, support decision making and institutional policy, and assist with managing undergraduate student study flow (e.g. intake, exam and course management), by giving users access to data across disciplines, rather than just individual faculty areas.
There does not seem to be agreement within the studies on a common term for adaptive systems, and that is probably due to the diverse functions they carry out, which also supports the classification of studies. Some of those terms coincide in part with the ones used for ITS, e.g. intelligent agents (Li, 2007 ; Ng et al., 2011 ). The most general terms used are intelligent e-learning system (Kose & Arslan, 2016 ), adaptive web-based learning system (Lo, Chan, & Yeh, 2012 ), or intelligent teaching system (Yuanyuan & Yajuan, 2014 ). As in ITS, most of the studies either describe the system or include a pilot study but no longer-term results are reported. Results from these pilot studies are usually reported as positive, except in Vlugter, Knott, McDonald, and Hall ( 2009 ), where the experimental group that used the dialogue-based computer assisted language-system scored lower than the control group in the delayed post-tests.
The 23 studies focused on teaching and learning can be classified into five sub-categories; teaching course content ( n = 7), recommending/providing personalised content ( n = 5), supporting teachers in learning and teaching design ( n = 3), using academic data to monitor and guide students ( n = 2), and supporting representation of knowledge using concept maps ( n = 2). However, some studies were difficult to classify, due to their specific and unique functions; helping to organise online learning groups with similar interests (Yang, Wang, Shen, & Han, 2007 ), supporting business decisions through simulation (Ben-Zvi, 2012 ), or supporting changes in attitude and behaviour for patients with Anorexia Nervosa, through embodied conversational agents (Sebastian & Richards, 2017 ). Aparicio et al. ( 2018 ) present a study where no adaptive system application was analysed, rather students’ perceptions of the use of information systems in education in general - and biomedical education in particular - were analysed, including intelligent information access systems .
The disciplines that are taught through adaptive systems are diverse, including environmental education (Huang, 2018 ), animation design (Yuanyuan & Yajuan, 2014 ), language learning (Jia, 2009 ; Vlugter et al., 2009 ), Computer Science (Iglesias, Martinez, Aler, & Fernandez, 2009 ) and Biology (Chaudhri et al., 2013 ). Walsh, Tamjidul, and Williams ( 2017 ), however, present an adaptive system based on machine learning-human machine learning symbiosis from a descriptive perspective, without specifying any discipline.
Recommending/providing personalised content
This group refers to adaptive systems that deliver customised content, materials and exercises according to students’ behaviour profiling in Business and Administration studies (Hall Jr & Ko, 2008 ) and Computer Science (Kose & Arslan, 2016 ; Lo et al., 2012 ). On the other hand, Tai, Wu, and Li ( 2008 ) present an e-learning recommendation system for online students to help them choose among courses, and Torres-Díaz, Infante Moro, and Valdiviezo Díaz ( 2014 ) emphasise the usefulness of (adaptive) recommendation systems in MOOCs to suggest actions, new items and users, according to students’ personal preferences.
Supporting teachers in learning and teaching design
In this group, three studies were identified. One study puts the emphasis on a hybrid recommender system of pedagogical patterns, to help teachers define their teaching strategies, according to the context of a specific class (Cobos et al., 2013 ), and another study presents a description of a metadata-based model to implement automatic learning designs that can solve detected problems (Camacho & Moreno, 2007 ). Li’s ( 2007 ) descriptive study argues that intelligent agents save time for online instructors, by leaving the most repetitive tasks to the systems, so that they can focus more on creative work.
Using academic data to monitor and guide students
The adaptive systems within this category focus on the extraction of student academic information to perform diagnostic tasks, and help tutors to offer a more proactive personal guidance (Rovira, Puertas, & Igual, 2017 ); or, in addition to that task, include performance evaluation and personalised assistance and feedback, such as the Learner Diagnosis, Assistance, and Evaluation System based on AI (StuDiAsE) for engineering learners (Samarakou et al., 2015 ).
Supporting representation of knowledge in concept maps
To help build students’ self-awareness of conceptual structures, concept maps can be quite useful. In the two studies of this group, an expert system was included, e.g. in order to accommodate selected peer ideas in the integrated concept maps and allow teachers to flexibly determine in which ways the selected concept maps are to be merged ( ICMSys ) (Kao, Chen, & Sun, 2010 ), or to help English as a Foreign Language college students to develop their reading comprehension through mental maps of referential identification (Yang et al., 2009 ). This latter system also includes system-guided instruction, practice and feedback.
Conclusions and implications for further educational research
In this paper, we have explored the field of AIEd research in terms of authorship and publication patterns. It is evident that US-American, Chinese, Taiwanese and Turkish colleagues (accounting for 50% of the publications as first authors) from Computer Science and STEM departments (62%) dominate the field. The leading journals are the International Journal of Artificial Intelligence in Education , Computers & Education , and the International Journal of Emerging Technologies in Learning .
More importantly, this study has provided an overview of the vast array of potential AI applications in higher education to support students, faculty members, and administrators. They were described in four broad areas (profiling and prediction, intelligent tutoring systems, assessment and evaluation, and adaptive systems and personalisation) with 17 sub-categories. This structure, which was derived from the systematic review, contributes to the understanding and conceptualisation of AIEd practice and research.
On the other hand, the lack of longitudinal studies and the substantial presence of descriptive and pilot studies from the technological perspective, as well as the prevalence of quantitative methods - especially quasi-experimental methods - in empirical studies, shows that there is still substantial room for educators to aim at innovative and meaningful research and practice with AIEd that could have learning impact within higher education, e.g. adopting design-based approaches (Easterday, Rees Lewis, & Gerber, 2018 ). A recent systematic literature review on personalisation in educational technology coincided with the predominance of experiences in technological developments, which also often used quantitative methods (Bartolomé, Castañeda, & Adell, 2018 ). Misiejuk and Wasson ( 2017 , p. 61) noted in their systematic review on Learning Analytics that “there are very few implementation studies and impact studies” (p. 61), which is also similar to the findings in the present article.
The full consequences of AI development cannot yet be foreseen today, but it seems likely that AI applications will be a top educational technology issue for the next 20 years. AI-based tools and services have a high potential to support students, faculty members and administrators throughout the student lifecycle. The applications that are described in this article provide enormous pedagogical opportunities for the design of intelligent student support systems, and for scaffolding student learning in adaptive and personalized learning environments. This applies in particular to large higher education institutions (such as open and distance teaching universities), where AIEd might help to overcome the dilemma of providing access to higher education for very large numbers of students (mass higher education). On the other hand, it might also help them to offer flexible, but also interactive and personalized learning opportunities, for example by relieving teachers from burdens, such as grading hundreds or even thousands of assignments, so that they can focus on their real task: empathic human teaching.
It is crucial to emphasise that educational technology is not (only) about technology – it is the pedagogical, ethical, social, cultural and economic dimensions of AIEd we should be concerned about. Selwyn ( 2016 , p. 106) writes:
The danger, of course, lies in seeing data and coding as an absolute rather than relative source of guidance and support. Education is far too complex to be reduced solely to data analysis and algorithms. As with digital technologies in general, digital data do not offer a neat technical fix to education dilemmas – no matter how compelling the output might be.
We should not strive for what is technically possible, but always ask ourselves what makes pedagogical sense. In China, systems are already being used to monitor student participation and expressions via face recognition in classrooms (so called Intelligent Classroom Behavior Management System, Smart Campus Footnote 8 ) and display them to the teacher on a dashboard. This is an example of educational surveillance, and it is highly questionable whether such systems provide real added value for a good teacher who should be able to capture the dynamics in a learning group (online and in an on-campus setting) and respond empathically and in a pedagogically meaningful way. In this sense, it is crucial to adopt an ethics of care (Prinsloo, 2017 ) to start thinking on how we are exploring the potential of algorithmic decision-making systems that are embedded in AIEd applications. Furthermore, we should also always remember that AI systems “first and foremost, require control by humans. Even the smartest AI systems can make very stupid mistakes. […] AI Systems are only as smart as the date used to train them” (Kaplan & Haenlein, 2019 , p. 25). Some critical voices in educational technology remind us that we should go beyond the tools, and talk again about learning and pedagogy, as well as acknowledging the human aspects of digital technology use in education (Castañeda & Selwyn, 2018 ). The new UNESCO report on challenges and opportunities of AIEd for sustainable development deals with various areas, all of which have an important pedagogical, social and ethical dimension, e.g. ensuring inclusion and equity in AIEd, preparing teachers for AI-powered education, developing quality and inclusive data systems, or ethics and transparency in data collection, use and dissemination (Pedró, Subosa, Rivas, & Valverde, 2019 ).
That being said, a stunning result of this review is the dramatic lack of critical reflection of the pedagogical and ethical implications as well as risks of implementing AI applications in higher education. Concerning ethical implications, privacy issues were also noted to be rarely addressed in empirical studies in a recent systematic review on Learning Analytics (Misiejuk & Wasson, 2017 ). More research is needed from educators and learning designers on how to integrate AI applications throughout the student lifecycle, to harness the enormous opportunities that they afford for creating intelligent learning and teaching systems. The low presence of authors affiliated with Education departments identified in our systematic review is evidence of the need for educational perspectives on these technological developments.
The lack of theory might be a syndrome within the field of educational technology in general. In a recent study, Hew, Lan, Tang, Jia, and Lo ( 2019 ) found that more than 40% of articles in three top educational technology journals were wholly a-theoretical. The systematic review by Bartolomé et al. ( 2018 ) also revealed this lack of explicit pedagogical perspectives in the studies analysed. The majority of research included in this systematic review is merely focused on analysing and finding patterns in data to develop models, and to make predictions that inform student and teacher facing applications, or to support administrative decisions using mathematical theories and machine learning methods that were developed decades ago (see Russel & Norvig, 2010 ). This kind of research is now possible through the growth of computing power and the vast availability of big digital student data. However, at this stage, there is very little evidence for the advancement of pedagogical and psychological learning theories related to AI driven educational technology. It is an important implication of this systematic review, that researchers are encouraged to be explicit about the theories that underpin empirical studies about the development and implementation of AIEd projects, in order to expand research to a broader level, helping us to understand the reasons and mechanisms behind this dynamic development that will have an enormous impact on higher education institutions in the various areas we have covered in this review.
Availability of data and materials
The datasets used and/or analysed during the current study (the bibliography of included studies) are available from the corresponding author upon request.
https://www.dfki.de/en/web/ (accessed 22 July, 2019)
https://www.tue.nl/en/news/news-overview/11-07-2019-tue-announces-eaisi-new-institute-for-intelligent-machines/ (accessed 22 July, 2019)
http://instituteforethicalaiineducation.org (accessed 22 July, 2019)
https://apo.org.au/node/229596 (accessed 22 July, 2019)
A file with all included references is available at: https://www.researchgate.net/publication/ 335911716_AIED-Ref (CC-0; DOI: https://doi.org/10.13140/RG.2.2.13000.88321 )
https://eppi.ioe.ac.uk/cms/er4/ (accessed July 22, 2019)
It is beyond the scope of this article to discuss the various machine learning methods for classification and prediction. Readers are therefore encouraged to refer to the literature referenced in the articles that are included in this review (e.g. Delen, 2010 and Umer, Susnjak, Mathrani, & Suriadi, 2017 ).
https://www.businessinsider.de/china-school-facial-recognition-technology-2018-5?r=US&IR=T (accessed July 5, 2019)
Acikkar, M., & Akay, M. F. (2009). Support vector machines for predicting the admission decision of a candidate to the School of Physical Education and Sports at Cukurova University. Expert Systems with Applications , 36 (3 PART 2), 7228–7233. https://doi.org/10.1016/j.eswa.2008.09.007 .
Article Google Scholar
Adamson, D., Dyke, G., Jang, H., & Rosé, C. P. (2014). Towards an agile approach to adapting dynamic collaboration support to student needs. International Journal of Artificial Intelligence in Education , 24 (1), 92–124. https://doi.org/10.1007/s40593-013-0012-6 .
Agaoglu, M. (2016). Predicting instructor performance using data mining techniques in higher education. IEEE Access , 4 , 2379–2387. https://doi.org/10.1109/ACCESS.2016.2568756 .
Ahmad, H., & Rashid, T. (2016). Lecturer performance analysis using multiple classifiers. Journal of Computer Science , 12 (5), 255–264. https://doi.org/10.3844/fjcssp.2016.255.264 .
Alfarsi, G. M. S., Omar, K. A. M., & Alsinani, M. J. (2017). A rule-based system for advising undergraduate students. Journal of Theoretical and Applied Information Technology , 95 (11) Retrieved from http://www.jatit.org .
Alkhasawneh, R., & Hargraves, R. H. (2014). Developing a hybrid model to predict student first year retention in STEM disciplines using machine learning techniques. Journal of STEM Education: Innovations & Research , 15 (3), 35–42 https://core.ac.uk/download/pdf/51289621.pdf .
Google Scholar
Aluko, R. O., Adenuga, O. A., Kukoyi, P. O., Soyingbe, A. A., & Oyedeji, J. O. (2016). Predicting the academic success of architecture students by pre-enrolment requirement: Using machine-learning techniques. Construction Economics and Building , 16 (4), 86–98. https://doi.org/10.5130/AJCEB.v16i4.5184 .
Aluthman, E. S. (2016). The effect of using automated essay evaluation on ESL undergraduate students’ writing skill. International Journal of English Linguistics , 6 (5), 54–67. https://doi.org/10.5539/ijel.v6n5p54 .
Amigud, A., Arnedo-Moreno, J., Daradoumis, T., & Guerrero-Roldan, A.-E. (2017). Using learning analytics for preserving academic integrity. International Review of Research in Open and Distance Learning , 18 (5), 192–210. https://doi.org/10.19173/irrodl.v18i5.3103 .
Andris, C., Cowen, D., & Wittenbach, J. (2013). Support vector machine for spatial variation. Transactions in GIS , 17 (1), 41–61. https://doi.org/10.1111/j.1467-9671.2012.01354.x .
Aparicio, F., Morales-Botello, M. L., Rubio, M., Hernando, A., Muñoz, R., López-Fernández, H., … de Buenaga, M. (2018). Perceptions of the use of intelligent information access systems in university level active learning activities among teachers of biomedical subjects. International Journal of Medical Informatics , 112 (December 2017), 21–33. https://doi.org/10.1016/j.ijmedinf.2017.12.016 .
Babić, I. D. (2017). Machine learning methods in predicting the student academic motivation. Croatian Operational Research Review , 8 (2), 443–461. https://doi.org/10.17535/crorr.2017.0028 .
Article MathSciNet Google Scholar
Bahadır, E. (2016). Using neural network and logistic regression analysis to predict prospective mathematics teachers’ academic success upon entering graduate education. Kuram ve Uygulamada Egitim Bilimleri , 16 (3), 943–964. https://doi.org/10.12738/estp.2016.3.0214 .
Bakeman, R., & Gottman, J. M. (1997). Observing interaction - an introduction to sequential analysis . Cambridge: Cambridge University Press.
Book Google Scholar
Baker, R. S. (2016). Stupid Tutoring Systems, Intelligent Humans. International Journal of Artificial Intelligence in Education , 26 (2), 600–614. https://doi.org/10.1007/s40593-016-0105-0 .
Baker, T., & Smith, L. (2019). Educ-AI-tion rebooted? Exploring the future of artificial intelligence in schools and colleges. Retrieved from Nesta Foundation website: https://media.nesta.org.uk/documents/Future_of_AI_and_education_v5_WEB.pdf
Barker, T. (2010). An automated feedback system based on adaptive testing: Extending the model. International Journal of Emerging Technologies in Learning , 5 (2), 11–14. https://doi.org/10.3991/ijet.v5i2.1235 .
Barker, T. (2011). An automated individual feedback and marking system: An empirical study. Electronic Journal of E-Learning , 9 (1), 1–14 https://www.learntechlib.org/p/52053/ .
Bartolomé, A., Castañeda, L., & Adell, J. (2018). Personalisation in educational technology: The absence of underlying pedagogies. International Journal of Educational Technology in Higher Education , 15 (14). https://doi.org/10.1186/s41239-018-0095-0 .
Ben-Zvi, T. (2012). Measuring the perceived effectiveness of decision support systems and their impact on performance. Decision Support Systems , 54 (1), 248–256. https://doi.org/10.1016/j.dss.2012.05.033 .
Biletska, O., Biletskiy, Y., Li, H., & Vovk, R. (2010). A semantic approach to expert system for e-assessment of credentials and competencies. Expert Systems with Applications , 37 (10), 7003–7014. https://doi.org/10.1016/j.eswa.2010.03.018 .
Blikstein, P., Worsley, M., Piech, C., Sahami, M., Cooper, S., & Koller, D. (2014). Programming pluralism: Using learning analytics to detect patterns in the learning of computer programming. Journal of the Learning Sciences , 23 (4), 561–599. https://doi.org/10.1080/10508406.2014.954750 .
Brunton, J., & Thomas, J. (2012). Information management in systematic reviews. In D. Gough, S. Oliver, & J. Thomas (Eds.), An introduction to systematic reviews , (pp. 83–106). London: SAGE.
Calvo, R. A., O’Rourke, S. T., Jones, J., Yacef, K., & Reimann, P. (2011). Collaborative writing support tools on the cloud. IEEE Transactions on Learning Technologies , 4 (1), 88–97 https://www.learntechlib.org/p/73461/ .
Camacho, D., & Moreno, M. D. R. (2007). Towards an automatic monitoring for higher education learning design. International Journal of Metadata, Semantics and Ontologies , 2 (1), 1. https://doi.org/10.1504/ijmso.2007.015071 .
Casamayor, A., Amandi, A., & Campo, M. (2009). Intelligent assistance for teachers in collaborative e-learning environments. Computers & Education , 53 (4), 1147–1154. https://doi.org/10.1016/j.compedu.2009.05.025 .
Castañeda, L., & Selwyn, N. (2018). More than tools? Making sense of he ongoing digitizations of higher education. International Journal of Educational Technology in Higher Education , 15 (22). https://doi.org/10.1186/s41239-018-0109-y .
Chaudhri, V. K., Cheng, B., Overtholtzer, A., Roschelle, J., Spaulding, A., Clark, P., … Gunning, D. (2013). Inquire biology: A textbook that answers questions. AI Magazine , 34 (3), 55–55. https://doi.org/10.1609/aimag.v34i3.2486 .
Chen, J.-F., & Do, Q. H. (2014). Training neural networks to predict student academic performance: A comparison of cuckoo search and gravitational search algorithms. International Journal of Computational Intelligence and Applications , 13 (1). https://doi.org/10.1142/S1469026814500059 .
Chi, M., VanLehn, K., Litman, D., & Jordan, P. (2011). Empirically evaluating the application of reinforcement learning to the induction of effective and adaptive pedagogical strategies. User Modeling and User-Adapted Interaction , 21 (1), 137–180. https://doi.org/10.1007/s11257-010-9093-1 .
Chodorow, M., Gamon, M., & Tetreault, J. (2010). The utility of article and preposition error correction systems for English language learners: Feedback and assessment. Language Testing , 27 (3), 419–436. https://doi.org/10.1177/0265532210364391 .
Chou, C.-Y., Huang, B.-H., & Lin, C.-J. (2011). Complementary machine intelligence and human intelligence in virtual teaching assistant for tutoring program tracing. Computers & Education , 57 (4), 2303–2312 https://www.learntechlib.org/p/167322/ .
Cobos, C., Rodriguez, O., Rivera, J., Betancourt, J., Mendoza, M., León, E., & Herrera-Viedma, E. (2013). A hybrid system of pedagogical pattern recommendations based on singular value decomposition and variable data attributes. Information Processing and Management , 49 (3), 607–625. https://doi.org/10.1016/j.ipm.2012.12.002 .
Cohen, J. (1960). A coefficient of agreement for nominal scales. Educational and Psychological Measurement , 20 , 37–46. https://doi.org/10.1177/001316446002000104 .
Contact North. (2018). Ten facts about artificial intelligence in teaching and learning. Retrieved from https://teachonline.ca/sites/default/files/tools-trends/downloads/ten_facts_about_artificial_intelligence.pdf
Crown, S., Fuentes, A., Jones, R., Nambiar, R., & Crown, D. (2011). Anne G. Neering: Interactive chatbot to engage and motivate engineering students. Computers in Education Journal , 21 (2), 24–34.
DeCarlo, P., & Rizk, N. (2010). The design and development of an expert system prototype for enhancing exam quality. International Journal of Advanced Corporate Learning , 3 (3), 10–13. https://doi.org/10.3991/ijac.v3i3.1356 .
Delen, D. (2010). A comparative analysis of machine learning techniques for student retention management. Decision Support Systems , 49 (4), 498–506. https://doi.org/10.1016/j.dss.2010.06.003 .
Delen, D. (2011). Predicting student attrition with data mining methods. Journal of College Student Retention: Research, Theory and Practice , 13 (1), 17–35. https://doi.org/10.2190/CS.13.1.b .
Dikli, S. (2010). The nature of automated essay scoring feedback. CALICO Journal , 28 (1), 99–134. https://doi.org/10.11139/cj.28.1.99-134 .
Dobre, I. (2014). Assessing the student′s knowledge in informatics discipline using the METEOR metric. Mediterranean Journal of Social Sciences , 5 (19), 84–92. https://doi.org/10.5901/mjss.2014.v5n19p84 .
Dodigovic, M. (2007). Artificial intelligence and second language learning: An efficient approach to error remediation. Language Awareness , 16 (2), 99–113. https://doi.org/10.2167/la416.0 .
Duarte, M., Butz, B., Miller, S., & Mahalingam, A. (2008). An intelligent universal virtual laboratory (UVL). IEEE Transactions on Education , 51 (1), 2–9. https://doi.org/10.1109/SSST.2002.1027009 .
Duffy, M. C., & Azevedo, R. (2015). Motivation matters: Interactions between achievement goals and agent scaffolding for self-regulated learning within an intelligent tutoring system. Computers in Human Behavior , 52 , 338–348. https://doi.org/10.1016/j.chb.2015.05.041 .
Duzhin, F., & Gustafsson, A. (2018). Machine learning-based app for self-evaluation of teacher-specific instructional style and tools. Education Sciences , 8 (1). https://doi.org/10.3390/educsci8010007 .
Easterday, M. W., Rees Lewis, D. G., & Gerber, E. M. (2018). The logic of design research. Learning: Research and Practice , 4 (2), 131–160. https://doi.org/10.1080/23735082.2017.1286367 .
EDUCAUSE. (2018). Horizon report: 2018 higher education edition. Retrieved from EDUCAUSE Learning Initiative and The New Media Consortium website: https://library.educause.edu/~/media/files/library/2018/8/2018horizonreport.pdf
EDUCAUSE. (2019). Horizon report: 2019 higher education edition. Retrieved from EDUCAUSE Learning Initiative and The New Media Consortium website: https://library.educause.edu/-/media/files/library/2019/4/2019horizonreport.pdf
Feghali, T., Zbib, I., & Hallal, S. (2011). A web-based decision support tool for academic advising. Educational Technology and Society , 14 (1), 82–94 https://www.learntechlib.org/p/52325/ .
Feng, S., Zhou, S., & Liu, Y. (2011). Research on data mining in university admissions decision-making. International Journal of Advancements in Computing Technology , 3 (6), 176–186. https://doi.org/10.4156/ijact.vol3.issue6.21 .
Fleiss, J. L. (1981). Statistical methods for rates and proportions . New York: Wiley.
MATH Google Scholar
Garcia-Gorrostieta, J. M., Lopez-Lopez, A., & Gonzalez-Lopez, S. (2018). Automatic argument assessment of final project reports of computer engineering students. Computer Applications in Engineering Education, 26(5), 1217–1226. https://doi.org/10.1002/cae.21996
Ge, C., & Xie, J. (2015). Application of grey forecasting model based on improved residual correction in the cost estimation of university education. International Journal of Emerging Technologies in Learning , 10 (8), 30–33. https://doi.org/10.3991/ijet.v10i8.5215 .
Gierl, M., Latifi, S., Lai, H., Boulais, A., & Champlain, A. (2014). Automated essay scoring and the future of educational assessment in medical education. Medical Education , 48 (10), 950–962. https://doi.org/10.1111/medu.12517 .
Gough, D., Oliver, S., & Thomas, J. (2017). An introduction to systematic reviews , (2nd ed., ). Los Angeles: SAGE.
Gutierrez, G., Canul-Reich, J., Ochoa Zezzatti, A., Margain, L., & Ponce, J. (2018). Mining: Students comments about teacher performance assessment using machine learning algorithms. International Journal of Combinatorial Optimization Problems and Informatics , 9 (3), 26–40 https://ijcopi.org/index.php/ojs/article/view/99 .
Hall Jr., O. P., & Ko, K. (2008). Customized content delivery for graduate management education: Application to business statistics. Journal of Statistics Education , 16 (3). https://doi.org/10.1080/10691898.2008.11889571 .
Haugeland, J. (1985). Artificial intelligence: The very idea. Cambridge, Mass.: MIT Press
Hew, K. F., Lan, M., Tang, Y., Jia, C., & Lo, C. K. (2019). Where is the “theory” within the field of educational technology research? British Journal of Educational Technology , 50 (3), 956–971. https://doi.org/10.1111/bjet.12770 .
Hinojo-Lucena, F.-J., Aznar-Díaz, I., Cáceres-Reche, M.-P., & Romero-Rodríguez, J.-M. (2019). Artificial intelligence in higher education: A bibliometric study on its impact in the scientific literature. Education Sciences , 9 (1), 51. https://doi.org/10.3390/educsci9010051 .
Hoffait, A.-S., & Schyns, M. (2017). Early detection of university students with potential difficulties. Decision Support Systems , 101 , 1–11. https://doi.org/10.1016/j.dss.2017.05.003 .
Hooshyar, D., Ahmad, R., Yousefi, M., Yusop, F., & Horng, S. (2015). A flowchart-based intelligent tutoring system for improving problem-solving skills of novice programmers. Journal of Computer Assisted Learning , 31 (4), 345–361. https://doi.org/10.1111/jcal.12099 .
Howard, C., Jordan, P., di Eugenio, B., & Katz, S. (2017). Shifting the load: A peer dialogue agent that encourages its human collaborator to contribute more to problem solving. International Journal of Artificial Intelligence in Education , 27 (1), 101–129. https://doi.org/10.1007/s40593-015-0071-y .
Howard, E., Meehan, M., & Parnell, A. (2018). Contrasting prediction methods for early warning systems at undergraduate level. Internet and Higher Education , 37 , 66–75. https://doi.org/10.1016/j.iheduc.2018.02.001 .
Huang, C.-J., Chen, C.-H., Luo, Y.-C., Chen, H.-X., & Chuang, Y.-T. (2008). Developing an intelligent diagnosis and assessment e-Learning tool for introductory programming. Educational Technology & Society , 11 (4), 139–157 https://www.jstor.org/stable/jeductechsoci.11.4.139 .
Huang, J., & Chen, Z. (2016). The research and design of web-based intelligent tutoring system. International Journal of Multimedia and Ubiquitous Engineering , 11 (6), 337–348. https://doi.org/10.14257/ijmue.2016.11.6.30 .
Huang, S. P. (2018). Effects of using artificial intelligence teaching system for environmental education on environmental knowledge and attitude. Eurasia Journal of Mathematics, Science and Technology Education , 14 (7), 3277–3284. https://doi.org/10.29333/ejmste/91248 .
Hussain, M., Zhu, W., Zhang, W., & Abidi, S. M. R. (2018). Student engagement predictions in an e-Learning system and their impact on student course assessment scores. Computational Intelligence and Neuroscience . https://doi.org/10.1155/2018/6347186 .
Iglesias, A., Martinez, P., Aler, R., & Fernandez, F. (2009). Reinforcement learning of pedagogical policies in adaptive and intelligent educational systems. Knowledge-Based Systems , 22 (4), 266–270 https://e-archivo.uc3m.es/bitstream/handle/10016/6502/reinforcement_aler_KBS_2009_ps.pdf?sequence=1&isAllowed=y .
Jackson, M., & Cossitt, B. (2015). Is intelligent online tutoring software useful in refreshing financial accounting knowledge? Advances in Accounting Education: Teaching and Curriculum Innovations , 16 , 1–19. https://doi.org/10.1108/S1085-462220150000016001 .
Jain, G. P., Gurupur, V. P., Schroeder, J. L., & Faulkenberry, E. D. (2014). Artificial intelligence-based student learning evaluation: A concept map-based approach for analyzing a student’s understanding of a topic. IEEE Transactions on Learning Technologies , 7 (3), 267–279. https://doi.org/10.1109/TLT.2014.2330297 .
Jeschike, M., Jeschke, S., Pfeiffer, O., Reinhard, R., & Richter, T. (2007). Equipping virtual laboratories with intelligent training scenarios. AACE Journal , 15 (4), 413–436 h ttps://www.learntechlib.org/primary/p/23636/ .
Jia, J. (2009). An AI framework to teach English as a foreign language: CSIEC. AI Magazine , 30 (2), 59–59. https://doi.org/10.1609/aimag.v30i2.2232 .
Jonassen, D., Davidson, M., Collins, M., Campbell, J., & Haag, B. B. (1995). Constructivism and computer-mediated communication in distance education. American Journal of Distance Education , 9 (2), 7–25. https://doi.org/10.1080/08923649509526885 .
Kalz, M., van Bruggen, J., Giesbers, B., Waterink, W., Eshuis, J., & Koper, R. (2008). A model for new linkages for prior learning assessment. Campus-Wide Information Systems , 25 (4), 233–243. https://doi.org/10.1108/10650740810900676 .
Kao, Chen, & Sun (2010). Using an e-Learning system with integrated concept maps to improve conceptual understanding. International Journal of Instructional Media , 37 (2), 151–151.
Kaplan, A., & Haenlein, M. (2019). Siri, Siri, in my hand: Who’s the fairest in the land? On the interpretations, illustrations, and implications of artificial intelligence. Business Horizons , 62 (1), 15–25. https://doi.org/10.1016/j.bushor.2018.08.004 .
Kardan, A. A., & Sadeghi, H. (2013). A decision support system for course offering in online higher education institutes. International Journal of Computational Intelligence Systems , 6 (5), 928–942. https://doi.org/10.1080/18756891.2013.808428 .
Kardan, A. A., Sadeghi, H., Ghidary, S. S., & Sani, M. R. F. (2013). Prediction of student course selection in online higher education institutes using neural network. Computers and Education , 65 , 1–11. https://doi.org/10.1016/j.compedu.2013.01.015 .
Kose, U., & Arslan, A. (2016). Intelligent e-Learning system for improving students’ academic achievements in computer programming courses. International Journal of Engineering Education , 32 (1, A), 185–198.
Li, X. (2007). Intelligent agent-supported online education. Decision Sciences Journal of Innovative Education , 5 (2), 311–331. https://doi.org/10.1111/j.1540-4609.2007.00143.x .
Lo, J. J., Chan, Y. C., & Yeh, S. W. (2012). Designing an adaptive web-based learning system based on students’ cognitive styles identified online. Computers and Education , 58 (1), 209–222. https://doi.org/10.1016/j.compedu.2011.08.018 .
Lodhi, P., Mishra, O., Jain, S., & Bajaj, V. (2018). StuA: An intelligent student assistant. International Journal of Interactive Multimedia and Artificial Intelligence , 5 (2), 17–25. https://doi.org/10.9781/ijimai.2018.02.008 .
Luckin, R., Holmes, W., Griffiths, M., & Forcier, L. B. (2016). Intelligence unleashed - an argument for AI in education. Retrieved from http://discovery.ucl.ac.uk/1475756/
Ma, H., & Slater, T. (2015). Using the developmental path of cause to bridge the gap between AWE scores and writing teachers’ evaluations. Writing & Pedagogy , 7 (2), 395–422. https://doi.org/10.1558/wap.v7i2-3.26376 .
McNamara, D. S., Crossley, S. A., Roscoe, R. D., Allen, L. K., & Dai, J. (2015). A hierarchical classification approach to automated essay scoring. Assessing Writing , 23 , 35–59. https://doi.org/10.1016/j.asw.2014.09.002 .
Misiejuk, K., & Wasson, B. (2017). State of the field report on learning analytics. SLATE report 2017–2 . Bergen: Centre for the Science of Learning & Technology (SLATE) Retrieved from http://bora.uib.no/handle/1956/17740 .
Miwa, K., Terai, H., Kanzaki, N., & Nakaike, R. (2014). An intelligent tutoring system with variable levels of instructional support for instructing natural deduction. Transactions of the Japanese Society for Artificial Intelligence , 29 (1), 148–156. https://doi.org/10.1527/tjsai.29.148 .
Moher, D., Liberati, A., Tetzlaff, J., & Altman, D. G. (2009). Preferred reporting items for systematic reviews and meta-analyses: The PRISMA statement. BMJ , 339 , b2535. https://doi.org/10.1136/bmj.b2535 Clinical Research Ed.
Nehm, R. H., Ha, M., & Mayfield, E. (2012). Transforming biology assessment with machine learning: Automated scoring of written evolutionary explanations. Journal of Science Education and Technology , 21 (1), 183–196. https://doi.org/10.1007/s10956-011-9300-9 .
Neumann, W. L. (2007). Social research methods: Qualitative and quantitative approaches . Boston: Pearson.
Ng, S. C., Wong, C. K., Lee, T. S., & Lee, F. Y. (2011). Design of an agent-based academic information system for effective education management. Information Technology Journal , 10 (9), 1784–1788. https://doi.org/10.3923/itj.2011.1784.1788 .
Nguyen, J., Sánchez-Hernández, G., Armisen, A., Agell, N., Rovira, X., & Angulo, C. (2018). A linguistic multi-criteria decision-aiding system to support university career services. Applied Soft Computing Journal , 67 , 933–940. https://doi.org/10.1016/j.asoc.2017.06.052 .
Nicholas, D., Watkinson, A., Jamali, H. R., Herman, E., Tenopir, C., Volentine, R., … Levine, K. (2015). Peer review: still king in the digital age. Learned Publishing , 28 (1), 15–21. https://doi.org/10.1087/20150104 .
Oztekin, A. (2016). A hybrid data analytic approach to predict college graduation status and its determinative factors. Industrial Management and Data Systems , 116 (8), 1678–1699. https://doi.org/10.1108/IMDS-09-2015-0363 .
Ozturk, Z. K., Cicek, Z. I. E., & Ergul, Z. (2017). Sentiment analysis: An application to Anadolu University. Acta Physica Polonica A , 132 (3), 753–755. https://doi.org/10.12693/APhysPolA.132.753 .
Palocsay, S. W., & Stevens, S. P. (2008). A study of the effectiveness of web-based homework in teaching undergraduate business statistics. Decision Sciences Journal of Innovative Education , 6 (2), 213–232. https://doi.org/10.1111/j.1540-4609.2008.00167.x .
Paquette, L., Lebeau, J. F., Beaulieu, G., & Mayers, A. (2015). Designing a knowledge representation approach for the generation of pedagogical interventions by MTTs. International Journal of Artificial Intelligence in Education , 25 (1), 118–156 https://www.learntechlib.org/p/168275/ .
Payne, V. L., Medvedeva, O., Legowski, E., Castine, M., Tseytlin, E., Jukic, D., & Crowley, R. S. (2009). Effect of a limited-enforcement intelligent tutoring system in dermatopathology on student errors, goals and solution paths. Artificial Intelligence in Medicine , 47 (3), 175–197. https://doi.org/10.1016/j.artmed.2009.07.002 .
Pedró, F., Subosa, M., Rivas, A., & Valverde, P. (2019). Artificial intelligence in education: Challenges and opportunities for sustainable development . Paris: UNESCO.
Perez, S., Massey-Allard, J., Butler, D., Ives, J., Bonn, D., Yee, N., & Roll, I. (2017). Identifying productive inquiry in virtual labs using sequence mining. In E. André, R. Baker, X. Hu, M. M. T. Rodrigo, & B. du Boulay (Eds.), Artificial intelligence in education , (vol. 10,331, pp. 287–298). https://doi.org/10.1007/978-3-319-61425-0_24 .
Chapter Google Scholar
Perin, D., & Lauterbach, M. (2018). Assessing text-based writing of low-skilled college students. International Journal of Artificial Intelligence in Education , 28 (1), 56–78. https://doi.org/10.1007/s40593-016-0122-z .
Petticrew, M., & Roberts, H. (2006). Systematic reviews in the social sciences: A practical guide . Malden; Oxford: Blackwell Pub.
Phani Krishna, K. V., Mani Kumar, M., & Aruna Sri, P. S. G. (2018). Student information system and performance retrieval through dashboard. International Journal of Engineering and Technology (UAE) , 7 , 682–685. https://doi.org/10.14419/ijet.v7i2.7.10922 .
Popenici, S., & Kerr, S. (2017). Exploring the impact of artificial intelligence on teaching and learning in higher education. Research and Practice in Technology Enhanced Learning . https://doi.org/10.1186/s41039-017-0062-8 .
Prinsloo, P. (2017). Fleeing from Frankenstein’s monster and meeting Kafka on the way: Algorithmic decision-making in higher education. E-Learning and Digital Media , 14 (3), 138–163. https://doi.org/10.1177/2042753017731355 .
Quixal, M., & Meurers, D. (2016). How can writing tasks be characterized in a way serving pedagogical goals and automatic analysis needs? Calico Journal , 33 (1), 19–48. https://doi.org/10.1558/cj.v33i1.26543 .
Raju, D., & Schumacker, R. (2015). Exploring student characteristics of retention that lead to graduation in higher education using data mining models. Journal of College Student Retention: Research, Theory and Practice , 16 (4), 563–591. https://doi.org/10.2190/CS.16.4.e .
Ramírez, J., Rico, M., Riofrío-Luzcando, D., Berrocal-Lobo, M., & Antonio, A. (2018). Students’ evaluation of a virtual world for procedural training in a tertiary-education course. Journal of Educational Computing Research , 56 (1), 23–47. https://doi.org/10.1177/0735633117706047 .
Ray, R. D., & Belden, N. (2007). Teaching college level content and reading comprehension skills simultaneously via an artificially intelligent adaptive computerized instructional system. Psychological Record , 57 (2), 201–218 https://opensiuc.lib.siu.edu/cgi/viewcontent.cgi?referer=https://www.google.com/&httpsredir=1&article=1103&context=tpr .
Reid, J. (1995). Managing learner support. In F. Lockwood (Ed.), Open and distance learning today , (pp. 265–275). London: Routledge.
Rovira, S., Puertas, E., & Igual, L. (2017). Data-driven system to predict academic grades and dropout. PLoS One , 12 (2), 1–21. https://doi.org/10.1371/journal.pone.0171207 .
Russel, S., & Norvig, P. (2010). Artificial intelligence - a modern approach . New Jersey: Pearson Education.
Salmon, G. (2000). E-moderating - the key to teaching and learning online , (1st ed., ). London: Routledge.
Samarakou, M., Fylladitakis, E. D., Früh, W. G., Hatziapostolou, A., & Gelegenis, J. J. (2015). An advanced eLearning environment developed for engineering learners. International Journal of Emerging Technologies in Learning , 10 (3), 22–33. https://doi.org/10.3991/ijet.v10i3.4484 .
Sanchez, E. L., Santos-Olmo, A., Alvarez, E., Huerta, M., Camacho, S., & Fernandez-Medina, E. (2016). Development of an expert system for the evaluation of students’ curricula on the basis of competencies. Future Internet , 8 (2). https://doi.org/10.3390/fi8020022 .
Schiaffino, S., Garcia, P., & Amandi, A. (2008). eTeacher: Providing personalized assistance to e-learning students. Computers & Education , 51 (4), 1744–1754. https://doi.org/10.1016/j.compedu.2008.05.008 .
Sebastian, J., & Richards, D. (2017). Changing stigmatizing attitudes to mental health via education and contact with embodied conversational agents. Computers in Human Behavior , 73 , 479–488. https://doi.org/10.1016/j.chb.2017.03.071 .
Selwyn, N. (2016). Is technology good for education? Cambridge, UK: Malden, MA : Polity Press.
Shen, V. R. L., & Yang, C.-Y. (2011). Intelligent multiagent tutoring system in artificial intelligence. International Journal of Engineering Education , 27 (2), 248–256.
Šimundić, A.-M. (2009). Measures of diagnostic accuracy: Basic definitions. Journal of the International Federation of Clinical Chemistry and Laboratory Medicine , 19 (4), 203–2011 https://www.ncbi.nlm.nih.gov/pubmed/27683318 .
Smith, R. (2006). Peer review: a flawed process at the heart of science and journals. Journal of the Royal Society of Medicine , 99 , 178–182. https://doi.org/10.1258/jrsm.99.4.178 .
Spikol, D., Ruffaldi, E., Dabisias, G., & Cukurova, M. (2018). Supervised machine learning in multimodal learning analytics for estimating success in project-based learning. Journal of Computer Assisted Learning , 34 (4), 366–377. https://doi.org/10.1111/jcal.12263 .
Sreenivasa Rao, K., Swapna, N., & Praveen Kumar, P. (2018). Educational data mining for student placement prediction using machine learning algorithms. International Journal of Engineering and Technology (UAE) , 7 (1.2), 43–46. https://doi.org/10.14419/ijet.v7i1.2.8988 .
Steenbergen-Hu, S., & Cooper, H. (2014). A meta-analysis of the effectiveness of intelligent tutoring systems on college students’ academic learning. Journal of Educational Psychology , 106 (2), 331–347. https://doi.org/10.1037/a0034752 .
Sultana, S., Khan, S., & Abbas, M. (2017). Predicting performance of electrical engineering students using cognitive and non-cognitive features for identification of potential dropouts. International Journal of Electrical Engineering Education , 54 (2), 105–118. https://doi.org/10.1177/0020720916688484 .
Tai, D. W. S., Wu, H. J., & Li, P. H. (2008). Effective e-learning recommendation system based on self-organizing maps and association mining. Electronic Library , 26 (3), 329–344. https://doi.org/10.1108/02640470810879482 .
Tegmark, M. (2018). Life 3.0: Being human in the age of artificial intelligence . London: Penguin Books.
Teshnizi, S. H., & Ayatollahi, S. M. T. (2015). A comparison of logistic regression model and artificial neural networks in predicting of student’s academic failure. Acta Informatica Medica, 23(5), 296-300. https://doi.org/10.5455/aim.2015.23.296-300
Thatcher, S. J. (2014). The use of artificial intelligence in the learning of flight crew situation awareness in an undergraduate aviation programme. World Transactions on Engineering and Technology Education , 12 (4), 764–768 https://www.semanticscholar.org/paper/The-use-of-artificial-intelligence-in-the-learning-Thatcher/758d3053051511cde2f28fc6b2181b8e227f8ea2 .
Torres-Díaz, J. C., Infante Moro, A., & Valdiviezo Díaz, P. (2014). Los MOOC y la masificación personalizada. Profesorado , 18 (1), 63–72 http://www.redalyc.org/articulo.oa?id=56730662005 .
Umarani, S. D., Raviram, P., & Wahidabanu, R. S. D. (2011). Speech based question recognition of interactive ubiquitous teaching robot using supervised classifier. International Journal of Engineering and Technology , 3 (3), 239–243 http://www.enggjournals.com/ijet/docs/IJET11-03-03-35.pdf .
Umer, R., Susnjak, T., Mathrani, A., & Suriadi, S. (2017). On predicting academic performance with process mining in learning analytics. Journal of Research in Innovative Teaching , 10 (2), 160–176. https://doi.org/10.1108/JRIT-09-2017-0022 .
Vlugter, P., Knott, A., McDonald, J., & Hall, C. (2009). Dialogue-based CALL: A case study on teaching pronouns. Computer Assisted Language Learning , 22 (2), 115–131. https://doi.org/10.1080/09588220902778260 .
Walsh, K., Tamjidul, H., & Williams, K. (2017). Human machine learning symbiosis. Journal of Learning in Higher Education , 13 (1), 55–62 http://cs.uno.edu/~tamjid/pub/2017/JLHE.pdf .
Welham, D. (2008). AI in training (1980–2000): Foundation for the future or misplaced optimism? British Journal of Educational Technology , 39 (2), 287–303. https://doi.org/10.1111/j.1467-8535.2008.00818.x .
Weston-Sementelli, J. L., Allen, L. K., & McNamara, D. S. (2018). Comprehension and writing strategy training improves performance on content-specific source-based writing tasks. International Journal of Artificial Intelligence in Education , 28 (1), 106–137. https://doi.org/10.1007/s40593-016-0127-7 .
Wickham, H., & Grolemund, G. (2016). R for data science: Import, tidy, transform, visualize, and model data , (1st ed., ). Sebastopol: O’Reilly.
Yang, F., Wang, M., Shen, R., & Han, P. (2007). Community-organizing agent: An artificial intelligent system for building learning communities among large numbers of learners. Computers & Education , 49 (2), 131–147. https://doi.org/10.1016/j.compedu.2005.04.019 .
Yang, Y. F., Wong, W. K., & Yeh, H. C. (2009). Investigating readers’ mental maps of references in an online system. Computers and Education , 53 (3), 799–808. https://doi.org/10.1016/j.compedu.2009.04.016 .
Yoo, J., & Kim, J. (2014). Can online discussion participation predict group project performance? Investigating the roles of linguistic features and participation patterns. International Journal of Artificial Intelligence in Education , 24 (1), 8–32 https://www.learntechlib.org/p/155243/ .
Yuanyuan, J., & Yajuan, L. (2014). Development of an intelligent teaching system based on 3D technology in the course of digital animation production. International Journal of Emerging Technologies in Learning , 9 (9), 81–86. https://doi.org/10.3991/ijet.v11i09.6116 .
Zhu, W., Marquez, A., & Yoo, J. (2015). “Engineering economics jeopardy!” Mobile app for university students. Engineering Economist , 60 (4), 291–306. https://doi.org/10.1080/0013791X.2015.1067343 .
Download references
Acknowledgements
Not applicable.
This study received no external funding.
Author information
Authors and affiliations.
Faculty of Education and Social Sciences, University of Oldenburg, Ammerländer Heerstr. 138, 26129, Oldenburg, Germany
Olaf Zawacki-Richter, Victoria I. Marín, Melissa Bond & Franziska Gouverneur
You can also search for this author in PubMed Google Scholar
Contributions
The authors declare that each author has made a substantial contribution to this article, has approved the submitted version of this article and hast agreed to be personally accountable for the author’s own contributions. In particular, OZR as the leading author, has made a major contribution to the conception and design of the research; the data collection, screening of abstracts and full papers, the analysis, synthesis and interpretation of data; VIM has made a major contribution to the data collection, screening of abstracts and full papers, the analysis, synthesis and interpretation of data; MB has made a major contribution to the data collection, screening of full papers, the analysis, synthesis and interpretation of data; as a native speaker of English she was also responsible for language editing; FG has made a major contribution to the data collection, and the screening of abstracts and full papers. She calculated Cohen’s kappa values of interrater reliability.
Authors’ information
Dr. Olaf Zawacki-Richter is a Professor of Educational Technology in the Faculty of Education and Social Sciences at the University of Oldenburg in Germany. He is the Director of the Center for Open Education Research (COER) and the Center for Lifelong Learning (C3L).
Dr. Victoria I. Marín is a Post-doctoral Researcher in the Faculty of Education and Social Sciences / Center for Open Education Research (COER) at the University of Oldenburg in Germany.
Melissa Bond is a PhD candidate and Research Associate in the Faculty of Education and Social Sciences / Center for Open Education Research (COER) at the University of Oldenburg in Germany.
Franziska Gouverneur is a Masters student and Research Assistant in the Faculty of Education and Social Sciences / Center for Open Education Research (COER) at the University of Oldenburg in Germany.
Corresponding author
Correspondence to Olaf Zawacki-Richter .
Ethics declarations
Competing interests.
The authors declare that they have no competing interests.
Additional information
Publisher’s note.
Springer Nature remains neutral with regard to jurisdictional claims in published maps and institutional affiliations.
Rights and permissions
Open Access This article is distributed under the terms of the Creative Commons Attribution 4.0 International License ( http://creativecommons.org/licenses/by/4.0/ ), which permits unrestricted use, distribution, and reproduction in any medium, provided you give appropriate credit to the original author(s) and the source, provide a link to the Creative Commons license, and indicate if changes were made.
Reprints and permissions
About this article
Cite this article.
Zawacki-Richter, O., Marín, V.I., Bond, M. et al. Systematic review of research on artificial intelligence applications in higher education – where are the educators?. Int J Educ Technol High Educ 16 , 39 (2019). https://doi.org/10.1186/s41239-019-0171-0
Download citation
Received : 26 July 2019
Accepted : 01 October 2019
Published : 28 October 2019
DOI : https://doi.org/10.1186/s41239-019-0171-0
Share this article
Anyone you share the following link with will be able to read this content:
Sorry, a shareable link is not currently available for this article.
Provided by the Springer Nature SharedIt content-sharing initiative
- Artificial intelligence
- Higher education
- Machine learning
- Systematic review
AI Will Transform Teaching and Learning. Let’s Get it Right.
At the recent AI+Education Summit, Stanford researchers, students, and industry leaders discussed both the potential of AI to transform education for the better and the risks at play.
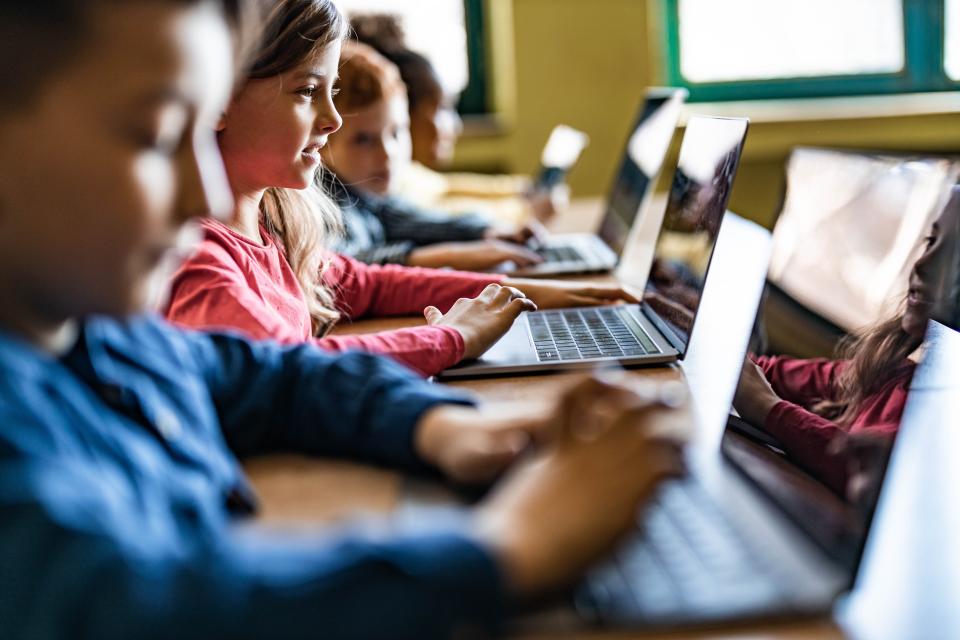
When the Stanford Accelerator for Learning and the Stanford Institute for Human-Centered AI began planning the inaugural AI+Education Summit last year, the public furor around AI had not reached its current level. This was the time before ChatGPT. Even so, intensive research was already underway across Stanford University to understand the vast potential of AI, including generative AI, to transform education as we know it.
By the time the summit was held on Feb. 15, ChatGPT had reached more than 100 million unique users , and 30% of all college students had used it for assignments, making it one of the fastest-ever applications ever adopted overall – and certainly in education settings. Within the education world, teachers and school districts have been wrestling with how to respond to this emerging technology.
The AI+Education Summit explored a central question: How can AI like this and other applications be best used to advance human learning?
“Technology offers the prospect of universal access to increase fundamentally new ways of teaching,” said Graduate School of Education Dean Daniel Schwartz in his opening remarks. “I want to emphasize that a lot of AI is also going to automate really bad ways of teaching. So [we need to] think about it as a way of creating new types of teaching.”
Researchers across Stanford – from education, technology, psychology, business, law, and political science – joined industry leaders like Sal Khan, founder and CEO of Khan Academy, in sharing cutting-edge research and brainstorming ways to unlock the potential of AI in education in an ethical, equitable, and safe manner.
Participants also spent a major portion of the day engaged in small discussion groups in which faculty, students, researchers, staff, and other guests shared their ideas about AI in education. Discussion topics included natural language processing applied to education; developing students’ AI literacy; assisting students with learning differences; informal learning outside of school; fostering creativity; equity and closing achievement gaps; workforce development; and avoiding potential misuses of AI with students and teachers.
Several themes emerged over the course of the day on AI’s potential, as well as its significant risks.
First, a look at AI’s potential:
1. Enhancing personalized support for teachers at scale
Great teachers remain the cornerstone of effective learning. Yet teachers receive limited actionable feedback to improve their practice. AI presents an opportunity to support teachers as they refine their craft at scale through applications such as:
- Simulating students: AI language models can serve as practice students for new teachers. Percy Liang , director of the Stanford HAI Center for Research on Foundation Models , said that they are increasingly effective and are now capable of demonstrating confusion and asking adaptive follow-up questions.
- Real-time feedback and suggestions: Dora Demszky , assistant professor of education data science, highlighted the ability for AI to provide real-time feedback and suggestions to teachers (e.g., questions to ask the class), creating a bank of live advice based on expert pedagogy.
- Post-teaching feedback: Demszky added that AI can produce post-lesson reports that summarize the classroom dynamics. Potential metrics include student speaking time or identification of the questions that triggered the most engagement. Research finds that when students talk more, learning is improved.
- Refreshing expertise: Sal Khan, founder of online learning environment Khan Academy, suggested that AI could help teachers stay up-to-date with the latest advancements in their field. For example, a biology teacher would have AI update them on the latest breakthroughs in cancer research, or leverage AI to update their curriculum.
2. Changing what is important for learners
Stanford political science Professor Rob Reich proposed a compelling question: Is generative AI comparable to the calculator in the classroom, or will it be a more detrimental tool? Today, the calculator is ubiquitous in middle and high schools, enabling students to quickly solve complex computations, graph equations, and solve problems. However, it has not resulted in the removal of basic mathematical computation from the curriculum: Students still know how to do long division and calculate exponents without technological assistance. On the other hand, Reich noted, writing is a way of learning how to think. Could outsourcing much of that work to AI harm students’ critical thinking development?
Liang suggested that students must learn about how the world works from first principles – this could be basic addition or sentence structure. However, they no longer need to be fully proficient – in other words, doing all computation by hand or writing all essays without AI support.
In fact, by no longer requiring mastery of proficiency, Demszky argued that AI may actually raise the bar. The models won’t be doing the thinking for the students; rather, students will now have to edit and curate, forcing them to engage deeper than they have previously. In Khan’s view, this allows learners to become architects who are able to pursue something more creative and ambitious.
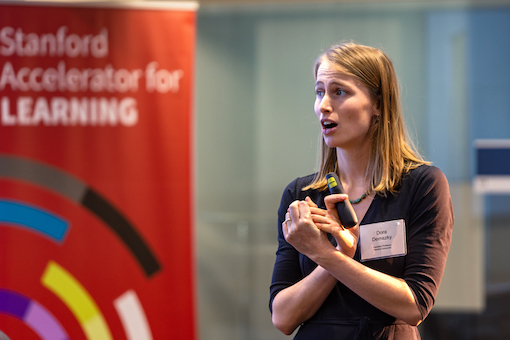
And Noah Goodman , associate professor of psychology and of computer science, questioned the analogy, saying this tool may be more like the printing press, which led to democratization of knowledge and did not eliminate the need for human writing skills.
3. Enabling learning without fear of judgment
Ran Liu, chief AI scientist at Amira Learning, said that AI has the potential to support learners’ self-confidence. Teachers commonly encourage class participation by insisting that there is no such thing as a stupid question. However, for most students, fear of judgment from their peers holds them back from fully engaging in many contexts. As Liu explained, children who believe themselves to be behind are the least likely to engage in these settings.
Interfaces that leverage AI can offer constructive feedback that does not carry the same stakes or cause the same self-consciousness as a human’s response. Learners are therefore more willing to engage, take risks, and be vulnerable.
One area in which this can be extremely valuable is soft skills. Emma Brunskill , associate professor of computer science, noted that there are an enormous number of soft skills that are really hard to teach effectively, like communication, critical thinking, and problem-solving. With AI, a real-time agent can provide support and feedback, and learners are able to try different tactics as they seek to improve.
4. Improving learning and assessment quality
Bryan Brown , professor of education, said that “what we know about learning is not reflected in how we teach.” For example, teachers know that learning happens through powerful classroom discussions. However, only one student can speak up at a time. AI has the potential to support a single teacher who is trying to generate 35 unique conversations with each student.
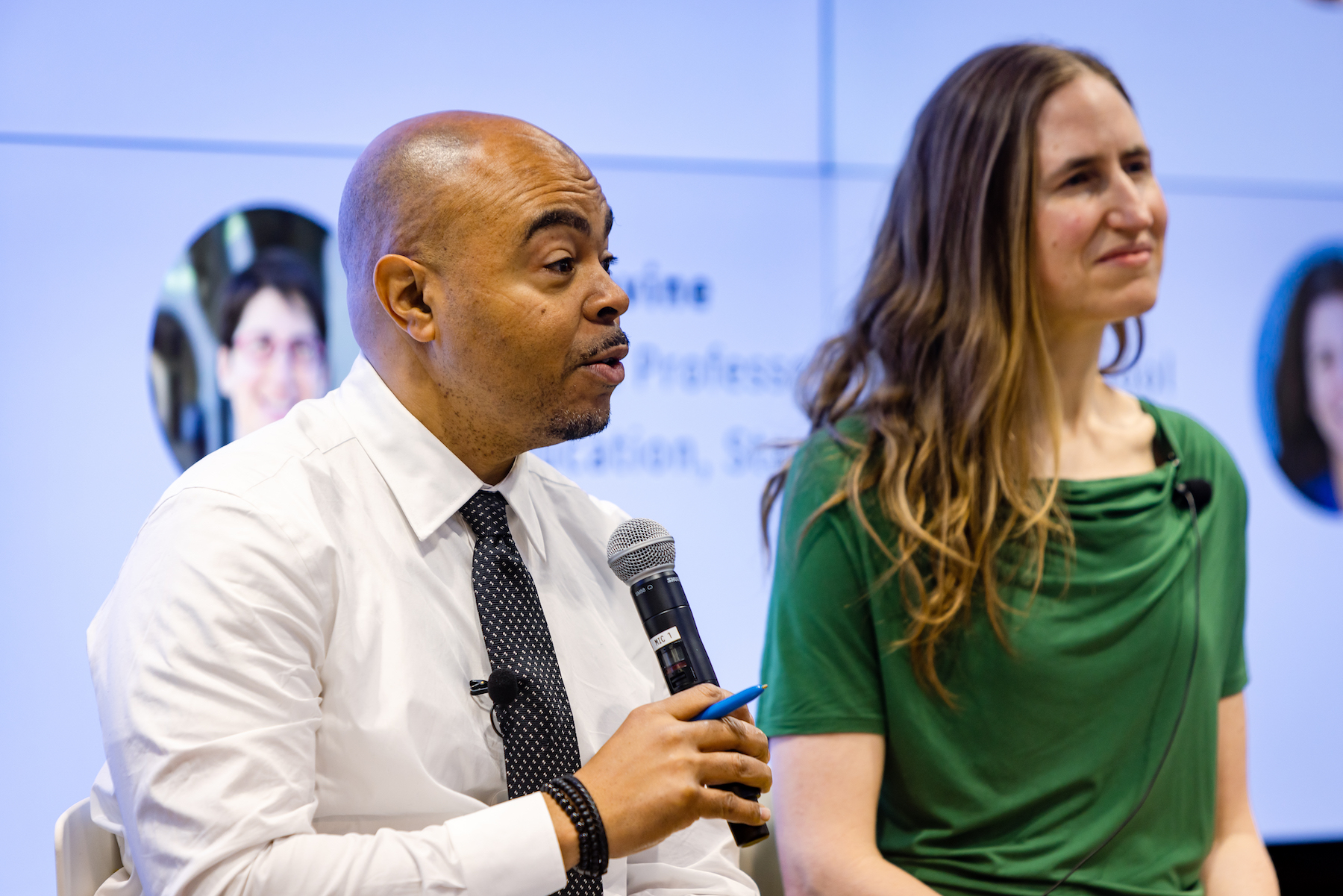
This also applies to the workforce. During a roundtable discussion facilitated by Stanford Digital Economy Lab Director Erik Brynjolfsson and Candace Thille , associate professor of education and faculty lead on adult learning at the Stanford Accelerator for Learning, attendees noted that the inability to judge a learner’s skill profile is a leading industry challenge. AI has the potential to quickly determine a learner’s skills, recommend solutions to fill the gaps, and match them with roles that actually require those skills.
Of course, AI is never a panacea. Now a look at AI’s significant risks:
1. Model output does not reflect true cultural diversity
At present, ChatGPT and AI more broadly generates text in language that fails to reflect the diversity of students served by the education system or capture the authentic voice of diverse populations. When the bot was asked to speak in the cadence of the author of The Hate U Give , which features an African American protagonist, ChatGPT simply added “yo” in front of random sentences. As Sarah Levine , assistant professor of education, explained, this overwhelming gap fails to foster an equitable environment of connection and safety for some of America’s most underserved learners.
2. Models do not optimize for student learning
While ChatGPT spits out answers to queries, these responses are not designed to optimize for student learning. As Liang noted, the models are trained to deliver answers as fast as possible, but that is often in conflict with what would be pedagogically sound, whether that’s a more in-depth explanation of key concepts or a framing that is more likely to spark curiosity to learn more.
3. Incorrect responses come in pretty packages
Goodman demonstrated that AI can produce coherent text that is completely erroneous. His lab trained a virtual tutor that was tasked with solving and explaining algebra equations in a chatbot format. The chatbot would produce perfect sentences that exhibited top-quality teaching techniques, such as positive reinforcement, but fail to get to the right mathematical answer.
4. Advances exacerbate a motivation crisis
Chris Piech , assistant professor of computer science, told a story about a student who recently came into his office crying. The student was concerned about the rapid progress of ChatGPT and how this would deter future job prospects after many years of learning how to code. Piech connected the incident to a broader existential motivation crisis, where many students may no longer know what they should be focusing on or don’t see the value of their hard-earned skills.
The full impact of AI in education remains unclear at this juncture, but as all speakers agreed, things are changing, and now is the time to get it right.
Watch the full conference:
Stanford HAI’s mission is to advance AI research, education, policy and practice to improve the human condition. Learn more
More News Topics
Advertisement
Generative Artificial Intelligence in Education and Its Implications for Assessment
- Original Paper
- Published: 11 November 2023
- Volume 68 , pages 58–66, ( 2024 )
Cite this article
- Jin Mao ORCID: orcid.org/0000-0001-8498-3523 1 ,
- Baiyun Chen ORCID: orcid.org/0000-0002-4010-9890 2 &
- Juhong Christie Liu ORCID: orcid.org/0000-0002-3384-4379 3
4959 Accesses
10 Citations
Explore all metrics
The abrupt emergence and rapid advancement of generative artificial intelligence (AI) technologies, transitioning from research labs to potentially all aspects of social life, has brought a profound impact on education, science, arts, journalism, and every facet of human life and communication. The purpose of this paper is to recapitulate the use of AI in education and examine potential opportunities and challenges of employing generative AI for educational assessment, with systems thinking in mind. Following a review of the opportunities and challenges, we discuss key issues and dilemmas associated with using generative AI for assessment and for education in general. We hope that the opportunities, challenges, and issues discussed in this paper could serve as a foundation for educators to harness the power of AI within the digital learning ecosystem.
This is a preview of subscription content, log in via an institution to check access.
Access this article
Subscribe and save.
- Get 10 units per month
- Download Article/Chapter or eBook
- 1 Unit = 1 Article or 1 Chapter
- Cancel anytime
Price includes VAT (Russian Federation)
Instant access to the full article PDF.
Rent this article via DeepDyve
Institutional subscriptions
Similar content being viewed by others
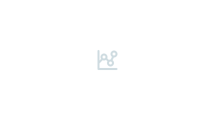
Generative Artificial Intelligence in Education, Part Two: International Perspectives
The Metaverse: A New Frontier for Learning and Teaching from the Perspective of AI
AI-Enhanced Education: Teaching and Learning Reimagined
Archibald, A., Hudson, C., Heap, T., Thompson, R. R., Lin, L., DeMeritt, J., & Lucke, H. (2023). A validation of ai-enabled discussion platform metrics and relationships to student efforts. TechTrends, 67 (2), 285–293. https://doi.org/10.1007/s11528-022-00825-7
Article Google Scholar
Armstrong, K. (2023). ChatGPT: US lawyer admits using AI for case research . BBC News. https://www.bbc.com/news/world-us-canada-65735769?via=aitoolzs . Accessed 10 June 2023
Baker, M. (2000). The roles of models in Artificial Intelligence and education research: A prospective view. International Journal of Artificial Intelligence in Education, 11 , 122–143.
Google Scholar
Bowen, J. A. (2012). Teaching naked: How moving technology out of your college classroom will improve student learning . John Wiley & Sons.
Cardon, P., Fleischmann, C., Aritz, J., Logemann, M., & Heidewald, J. (2023). The challenges and opportunities of AI-assisted writing: Developing AI literacy for the AI age. Business and Professional Communication Quarterly, 86 (3), 257–295. https://doi.org/10.1177/23294906231176517
Celik, I., Dindar, M., Muukkonen, H., & Järvelä, S. (2022). The promises and challenges of artificial intelligence for teachers: A systematic review of research. TechTrends, 66 (4), 616–630. https://doi.org/10.1007/s11528-022-00715-y
Chrisinger, B. (2023). It’s not just our students — ChatGPT is coming for faculty writing and there’s little agreement on the rules that should govern it . The Chronicle of Higher Education. https://www.chronicle.com/article/its-not-just-our-students-ai-is-coming-for-faculty-writing?cid=gen_sign_in . Accessed 20 Mar 2023
Cooper, G. (2023). Examining science education in ChatGPT: An exploratory study of generative artificial intelligence. Journal of Science Education and Technology, 32 (3), 444–452. https://doi.org/10.1007/s10956-023-10039-y
Cope, B., Kalantzis, M., & Searsmith, D. (2021). Artificial intelligence for education: Knowledge and its assessment in AI-enabled learning ecologies. Educational Philosophy and Theory, 53 (12), 1229–1245.
Darling-Hammond, L. (2017). Developing and measuring higher order skills: Models for state performance assessment systems . Learning Policy Institute and Council of Chief State School Officers.
von Davier, M. (2019). Training Optimus Prime, M.D.: Generating medical certification items by fine-tuning OpenAI’s GPT2 transformer model. arXiv Computer Science: Computation and Language . https://doi.org/10.48550/arXiv.1908.08594
Dhirani, L. L., Mukhtiar, N., Chowdhry, B. S., & Newe, T. (2023). Ethical dilemmas and privacy issues in emerging technologies: A review. Sensors, 23 (3), 1151. https://doi.org/10.3390/s23031151
Dwivedi, Y. K., Kshetri, N., Hughes, L., Slade, E. L., Jeyaraj, A., Kar, A. K., Baabdullah, A. M., Koohang, A., Raghavan, V., Ahuja, M., Hanaa Albanna, H., Albashrawi, M. A., Al-Busaidi, A. S., Janarthanan Balakrishnan, J., Barlette, Y., Basu, S., Bose, I., Brooks, L., Buhalis, D.,… Wright, R. (2023). “So what if ChatGPT wrote it?” Multidisciplinary perspectives on opportunities, challenges and implications of generative conversational AI for research, practice and policy. International Journal of Information Management , 71 , 102642 https://doi.org/10.1016/j.ijinfomgt.2023.102642
Floridi, L. (2023). AI as agency without intelligence: On ChatGPT, large language models, and other generative models. Philosophy and Technology . https://doi.org/10.2139/ssrn.4358789
Gamage, K. A., Silva, E. K. D., & Gunawardhana, N. (2020). Online delivery and assessment during COVID-19: Safeguarding academic integrity. Education Sciences, 10 (11), 301. https://doi.org/10.3390/educsci10110301
Gewirtz, D. (2023). Can AI detectors save us from ChatGPT? I tried 3 online tools to find out . ZDNET. https://www.zdnet.com/article/can-ai-detectors-save-us-from-chatgpt-i-tried-3-online-tools-to-find-out/ . Accessed 4 Nov 2023
Hu, K. (2023). ChatGPT sets record for fastest-growing user base - analyst note . REUTERS. https://www.reuters.com/technology/chatgpt-sets-record-fastest-growing-user-base-analyst-note-2023-02-01/ . Accessed 15 Feb 2023
Humble, N., & Mozelius, P. (2022). The threat, hype, and promise of artificial intelligence in education. Discover Artificial Intelligence, 2 (22). https://doi.org/10.1007/s44163-022-00039-z
Ifenthaler, D., & Schumacher, C. (2016). Student perceptions of privacy principles for learning analytics. Educational Technology Research and Development, 64 , 923–938. https://doi.org/10.1007/s11423-016-9477-y
Ivarsson, J., & Lindwall, O. (2023). Suspicious minds: The problem of trust and conversational agents. Computer Supported Cooperative Work (CSCW) https://doi.org/10.1007/s10606-023-09465-8
Jones-Rooy, A. (2019). What does the data say? I’m a data scientist who is skeptical about data . Quartz. https://qz.com/1664575/is-data-science-legit . Accessed Mar 5 2023
Kaipa, R. M. (2021). Multiple choice questions and essay questions in curriculum. Journal of Applied Research in Higher Education, 13 (1), 16–32. https://doi.org/10.1108/jarhe-01-2020-0011
Kan, M. (2023). ChatGPT may be the fastest growing app of all time, beating TikTok . PCMag . https://www.pcmag.com/news/chatgpt-may-be-the-fastest-growing-app-of-all-time-beating-tiktok . Accessed March 5, 2023
Kaplan-Rakowski, R., Grotewold, K., Hartwick, P., & Papin, K. (2023). Generative AI and teachers’ perspectives on its implementation in education. Journal of Interactive Learning Research, 34 (2), 313–338.
Kelley, K. J. (2023). Teaching actual student writing in an AI world . Inside Higher Ed. https://www.insidehighered.com/advice/2023/01/19/ways-prevent-students-using-ai-tools-their-classes-opinion . Accessed June 10, 2023
Kennedy, B., Tyson, A., & Saks, E. (2023). Public awareness of artificial intelligence in everyday activities: Limited enthusiasm in U.S. over AI’s growing influence in daily life . Pew Research Center. https://www.pewresearch.org/science/2023/02/15/public-awareness-of-artificial-intelligence-in-everyday-activities/ . Accessed 1 Mar 2023
Kowch, E. G., & Liu, J. C. (2018). Principles for teaching, leading, and participatory learning with a new participant: AI. In 2018 International Joint Conference on Information, Media and Engineering (ICIME) (pp. 320-325). IEEE
Book Google Scholar
Lamb, R., Neumann, K., & Linder, K. A. (2022). Real-time prediction of science student learning outcomes using machine learning classification of hemodynamics during virtual reality and online learning sessions. Computers and Education: Artificial Intelligence, 3 . https://doi.org/10.1016/j.caeai.2022.100078
Lee, V. R. (2023). Generative AI is forcing people to rethink what it means to be authentic . The Conversation. https://theconversation.com/generative-ai-is-forcing-people-to-rethink-what-it-means-to-be-authentic-204347?fbclid=IwAR2oio90vOzTQbXyPjaCrihKj7S5SYJ9lBsBHHnrN4PayQucLP7T1QCUzw4&mibextid=Zxz2cZ . Accessed 11 June 2023
Markauskaite, L., Marrone, R., Poquet, O., Knight, S., Martinez-Maldonado, R., Howard, S., Tondeur, J., De Laat, M., Shum, S. B., Gašević, D., & Siemens, G. (2022). Rethinking the entwinement between artificial intelligence and human learning: What capabilities do learners need for a world with AI?, Computers and Education: Artificial Intelligence, 3 , https://doi.org/10.1016/j.caeai.2022.100056
Martinez, D., Malyska, N., Streilein, B., Caceres, R., Campbell, W., Dagli, C., Gadepally, V., Greenfield, K., Hall, R., King, A., Lippmann, R., Miller, B., Reynolds, D., Richardson, F., Sahin, C., Tran, A., Trepagnier, P., & Zipkin, J. (2019). Artificial intelligence: Short history, present developments, and future outlook . Massachusetts Institute of Technology.
McAdoo, T., (2023). How to cite ChatGPT? APA Style. https://apastyle.apa.org/blog/how-to-cite-chatgpt . Accessed 10 June 2023
McKinsey & Company (2023, January 19). What is generative AI? https://www.mckinsey.com/featured-insights/mckinsey-explainers/what-is-generative-ai . Accessed 11 June 2023
Metz, C. (2023). What makes A.I. chatbots go wrong? The curious case of the hallucinating software . The New York Times. https://www.nytimes.com/2023/03/29/technology/ai-chatbots-hallucinations.html . Accessed 10 June 2023
Mislevy, R. J., Behrens, J. T., Dicerbo, K. E., & Levy, R. (2012). Design and discovery in educational assessment: Evidence-centered design, psychometrics, and educational data mining. Journal of Educational Data Mining, 4 (1), 11–48.
Mutimukwe, C., Viberg, O., Oberg, O. M., & Cerratto- Pargman, T. (2022). Students’ privacy concerns in learning analytics: Model development. British Journal of Educational Technology, 53 , 932–951. https://doi.org/10.1111/bjet.13234
Nelson, J. (2023). Italy welcomes ChatGPT back after ban over AI privacy concerns . Decrypt. https://decrypt.co/138362/italy-welcomes-chatgpt-back-after-ban-over-ai-privacy-concerns . Accessed 3 May 2023
Ntoutsi, E., Fafalios, P., Gadiraju, U., Iosifidis, V., Nejdl, W., Vidal, M. E., Ruggieri, S., Turini, F., Papadopoulos, S., Krasanakis, E., Kompatsiaris, I., Kinder-Kurlanda, K., Wagner, C., Karimi, F., Fernandez, M., Alani, H., Berendt, B., Kruegel, T., Heinze, C., … Staab, S. (2019). Bias in data-driven artificial intelligence systems—An introductory survey. WIREs Data Mining and Knowledge Discovery, 10 (3). https://doi.org/10.1002/widm.1356
OpenAI. (2022). Introducing ChatGPT . https://openai.com/blog/chatgpt/ . Accessed 1 June 2023
Oppy, G., & Dowe, D. (2021). The turing test, In: E. N. Zalta (Ed.), The Stanford Encyclopedia of Philosophy . https://plato.stanford.edu/archives/win2021/entries/turing-test/ . Accessed 5 May 2023
Ouyang, F., Zheng, L., & Jiao, P. (2022). Artificial intelligence in online higher education: A systematic review of empirical research from 2011 to 2020. Education and Information Technologies, 27 (6), 7893–7925. https://doi.org/10.1007/s10639-022-10925-9
Pavlik, J. V. (2023). Collaborating with ChatGPT: Considering the implications of generative artificial intelligence for journalism and media education. Journalism & Mass Communication Educator . https://doi.org/10.1177/10776958221149577
Pham, Y. K., Murray, C., & Gau, J. (2021). The inventory of teacher-student relationships: Factor structure and associations with school engagement among high-risk youth. Psychology in the Schools, 59 (2), 413–429. https://doi.org/10.1002/pits.22617
Popenici, S. A., & Kerr, S. (2017). Exploring the impact of artificial intelligence on teaching and learning in higher education. Research and Practice in Technology Enhanced Learning, 12 (1), 1–13. https://doi.org/10.1186/s41039-017-0062-8
Pressey, S. L. (1950). Development and appraisal of devices providing immediate automatic scoring of objective tests and concomitant self-instruction. The Journal of Psychology, 29 (2), 417–447.
Price, W. N., & Cohen, I. G. (2019). Privacy in the age of medical big data. Nature Medicine, 25 , 37–43. https://doi.org/10.1038/s41591-018-0272-7
Satariano, A. (2023). ChatGPT is banned in Italy over privacy concerns . The New York Times. https://www.nytimes.com/2023/03/31/technology/chatgpt-italy-ban.html . Accessed 1 April 2023
Selwyn, N. (2022). The future of AI and education: Some cautionary notes. European Journal of Education Research, Development, and Policy, 57 (4), 620–631. https://doi.org/10.1111/ejed.12532
Senge, P. M. (2006). The fifth discipline: The art & practice of the learning organization . Doubleday.
Shepard, L. A. (2000). The role of assessment in a learning culture. Educational Researcher, 29 (7), 4–14.
Stokel-Walker, C. (2023). January 18). ChatGPT listed as author on research papers: Many scientists disapprove. Nature, 613 , 620–621. https://doi.org/10.1038/d41586-023-00107-z
Surden, H. (2019). Artificial intelligence and law: An overview. Georgia State University Law Review 35 (4). https://readingroom.law.gsu.edu/gsulr/vol35/iss4/8 . Accessed 2 June 2023
Swiecki, Z., Khosravi, H., Chen, G.; Martinez-Maldonado, R., Lodge, J. M., Milligan, S., Selwyn, N., & Gašević, D. (2022). Assessment in the age of artificial intelligence. Computers and Education: Artificial Intelligence, 3 . https://doi.org/10.1016/j.caeai.2022.100075
Terry, O. K. (2023). I’m a student. You have no idea how much we’re using ChatGPT. No professor or software could ever pick up on it. The Chronicle of Higher Education . https://www.chronicle.com/article/im-a-student-you-have-no-idea-how-much-were-using-chatgpt . Accessed 9 June 2023
The National Assessment of Educational Progress (NAEP) (2021). Technology based assessment project . https://nces.ed.gov/nationsreportcard/studies/tba/ . Accessed 1 May 2023
Thorp, H. H. (2023). ChatGPT is fun, but not an author. Science, 379 (6630), 313. https://doi.org/10.1126/science.adg78
U.S. DOE Office of Educational Technology (2017). Reimagining the role of technology in education: 2017 National Education Technology Plan Update . Washington, D.C. https://tech.ed.gov/netp/ . Accessed 3 Jan 2023
U.S. DOE Office of Educational Technology. (2023). Artificial intelligence and future of teaching and learning: Insights and recommendations , Washington, DC. https://tech.ed.gov/ai-future-of-teaching-and-learning/ . Accessed 1 June 2023
Waller, C. (2023). The state of global digital accessibility: Current challenges and opportunities . Accessibility.com. https://www.accessibility.com/blog/the-state-of-global-digital-accessibility-current-challenges-and-opportunities . Accessed 13 June 2023
Wang, P. (2020). On defining artificial intelligence. Journal of Artificial General Intelligence, 11 (2), 73–86.
Wiggins, G. (1998). Educative assessment: Designing assessments to inform and improve student performance . Jossey-Bass Publishers.
Xu, W., & Ouyang, F. (2022). A systematic review of AI role in the educational system based on a proposed conceptual framework. Education and Information Technologies , 1–29. https://doi.org/10.1007/s10639-021-10774-y
Yu, E. (2023). Intelligent enough? Artificial intelligence for online learners. Journal of Educators Online, 20 (1). https://doi.org/10.9743/JEO.2023.20.1.16
Zhou, H., & Hunter, S. I. (2023). Guest post — Accessibility powered by AI: How artificial intelligence can help universalize access to digital content. The Scholarly Kitchen. https://scholarlykitchen.sspnet.org/2023/06/05/guest-post-accessibility-powered-by-ai-how-artificial-intelligence-can-help-universalize-access-to-digital-content/ . Accessed 13 June 2023
Download references
Author information
Authors and affiliations.
Wilkes University, Wilkes Barre, PA, USA
University of Central Florida, Orlando, FL, USA
Baiyun Chen
James Madison University, Harrisonburg, VA, USA
Juhong Christie Liu
You can also search for this author in PubMed Google Scholar
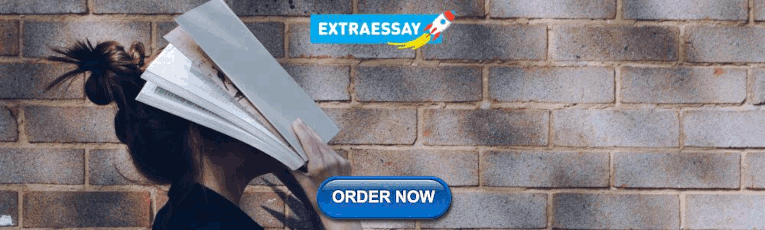
Corresponding author
Correspondence to Jin Mao .
Ethics declarations
Ethical approval.
This article does not contain any studies with human participants or animals performed by any of the authors.
Conflict of Interest
The authors declare that they have no conflict of interest. The first author is serving as co-editor of the DELT-STC special issue but will recuse herself from the review process for this manuscript.
Additional information
Publisher's note.
Springer Nature remains neutral with regard to jurisdictional claims in published maps and institutional affiliations.
Rights and permissions
Springer Nature or its licensor (e.g. a society or other partner) holds exclusive rights to this article under a publishing agreement with the author(s) or other rightsholder(s); author self-archiving of the accepted manuscript version of this article is solely governed by the terms of such publishing agreement and applicable law.
Reprints and permissions
About this article
Mao, J., Chen, B. & Liu, J.C. Generative Artificial Intelligence in Education and Its Implications for Assessment. TechTrends 68 , 58–66 (2024). https://doi.org/10.1007/s11528-023-00911-4
Download citation
Accepted : 10 October 2023
Published : 11 November 2023
Issue Date : January 2024
DOI : https://doi.org/10.1007/s11528-023-00911-4
Share this article
Anyone you share the following link with will be able to read this content:
Sorry, a shareable link is not currently available for this article.
Provided by the Springer Nature SharedIt content-sharing initiative
- Generative Artificial Intelligence
- Systems Thinking
- Find a journal
- Publish with us
- Track your research
An official website of the United States government
Here’s how you know
Official websites use .gov A .gov website belongs to an official government organization in the United States.
Secure .gov websites use HTTPS A lock ( Lock Locked padlock icon ) or https:// means you’ve safely connected to the .gov website. Share sensitive information only on official, secure websites.
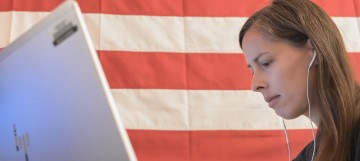
Research and Teach AI
American researchers and educators are foundational to ensuring our nation’s leadership in AI. The Biden-Harris Administration is investing in helping U.S. researchers and entrepreneurs build the next generation of safe, secure, and trustworthy AI, as well as supporting educators and institutions developing the future AI workforce.
National AI Research Resource Pilot
The National AI Research Resource (NAIRR) pilot, launched by the U.S. National Science Foundation (NSF) in January 2024, aims to expand access to critical AI research resources by connecting U.S. researchers and students to compute, data, software, model, and training resources they need to engage in AI research.
National AI Research Institutes
Call for proposals.
Submit proposals to expand the NAIRR Pilot community to new and emerging researchers and to educators bringing inclusive AI educational experiences to classrooms nationwide.
Advice for renewal of existing AI Institute Awards
Resources for researchers and entrepreneurs, supercharging america’s ai workforce.
An AI-ready workforce is essential for the United States to fully realize AI’s potential to advance scientific discovery, economic prosperity, and national security. By 2025, a pilot program led by the U.S. Department of Energy, in coordination with the U.S. National Science Foundation, will have leveraged a suite of existing training programs to augment the national AI workforce at national laboratories, institutions of higher education, and other pathways. The pilot program will train more than 500 new researchers at all academic levels and career stages in a variety of critical basic research and enabling technology development areas.
AI Test Beds
Submit proposals for new approaches to develop and evaluate AI systems in real-world settings. NSF is encouraging the community to submit proposals for planning grants to expand existing test beds and infrastructure to evaluate AI systems.
Entrepreneurial Fellowships Program
Through the National Science Foundation’s partnership with Activate, this program supports budding entrepreneurs for two years, providing them mentorship, stipends, and access to vital research tools, equipment, facilities, and expertise through collaboration with host laboratories.
National Defense Science and Engineering Graduate (NDSEG) Fellowship
The Department of Defense (DoD)’s NDSEG program offers graduate fellowships in 19 research disciplines, including AI, of strategic interest to the DoD. The program provides 3-year fellowships for students at or near the beginning of graduate study.
Privacy-Preserving Data Sharing in Practice (PDaSP)
This funding opportunity aims to enable and promote data sharing in a privacy-preserving and responsible manner to harness the power and insights of data for public good, such as for training powerful AI models. Led by the U.S. National Science Foundation in partnership with the U.S. Department of Transportation, the National Institute of Standards and Technology, and two technology companies, PDaSP will specifically prioritize use-inspired and translational research that empowers federal agencies and the private sector to adopt leading-edge privacy enhancing technologies in their work.
Responsible Design, Development, and Deployment of Technologies (ReDDDoT)
Submit proposals for research, implementation, and education projects involving multi-sector teams that focus on the responsible design, development, or deployment of technologies, including AI.
Resources for Educators and Institutions
Artificial intelligence and the future of teaching and learning.
The Department of Education (ED) has released a report to guide educators in understanding what AI can do to advance educational goals, while evaluating and limiting key risks.
Computer Science for All (CSforAll)
NSF’s CSforAll program supports partnerships and research that equip high school teachers to teach computer science, K-8 teachers to incorporate computer science and computational thinking in their classes, and school districts to create computing pathways across all grades.
NSF’s EducateAI initiative invites schools, school districts, community colleges, universities, and partner institutions to submit proposals to support educators advancing inclusive computing education, integrate AI-focused curricula for high school and undergraduate classrooms, and help create engaging and comprehensive educational materials that align with the latest advancements in AI.
Make Your Voice Heard
AI only works when it works for all of us. Let us know how AI can work better for you.

An official website of the United States government
The .gov means it’s official. Federal government websites often end in .gov or .mil. Before sharing sensitive information, make sure you’re on a federal government site.
The site is secure. The https:// ensures that you are connecting to the official website and that any information you provide is encrypted and transmitted securely.
- Publications
- Account settings
Preview improvements coming to the PMC website in October 2024. Learn More or Try it out now .
- Advanced Search
- Journal List
- Springer Nature - PMC COVID-19 Collection

Artificial intelligence in education: Addressing ethical challenges in K-12 settings
Selin akgun.
Michigan State University, East Lansing, MI USA
Christine Greenhow
Associated data.
Not applicable.
Artificial intelligence (AI) is a field of study that combines the applications of machine learning, algorithm productions, and natural language processing. Applications of AI transform the tools of education. AI has a variety of educational applications, such as personalized learning platforms to promote students’ learning, automated assessment systems to aid teachers, and facial recognition systems to generate insights about learners’ behaviors. Despite the potential benefits of AI to support students’ learning experiences and teachers’ practices, the ethical and societal drawbacks of these systems are rarely fully considered in K-12 educational contexts. The ethical challenges of AI in education must be identified and introduced to teachers and students. To address these issues, this paper (1) briefly defines AI through the concepts of machine learning and algorithms; (2) introduces applications of AI in educational settings and benefits of AI systems to support students’ learning processes; (3) describes ethical challenges and dilemmas of using AI in education; and (4) addresses the teaching and understanding of AI by providing recommended instructional resources from two providers—i.e., the Massachusetts Institute of Technology’s (MIT) Media Lab and Code.org. The article aims to help practitioners reap the benefits and navigate ethical challenges of integrating AI in K-12 classrooms, while also introducing instructional resources that teachers can use to advance K-12 students’ understanding of AI and ethics.
Introduction
“Success in creating AI would be the biggest event in human history. Unfortunately, it might also be the last, unless we learn how to avoid the risks.” — Stephen Hawking.
We may not think about artificial intelligence (AI) on a daily basis, but it is all around us, and we have been using it for years. When we are doing a Google search, reading our emails, getting a doctor’s appointment, asking for driving directions, or getting movie and music recommendations, we are constantly using the applications of AI and its assistance in our lives. This need for assistance and our dependence on AI systems has become even more apparent during the COVID-19 pandemic. The growing impact and dominance of AI systems reveals itself in healthcare, education, communications, transportation, agriculture, and more. It is almost impossible to live in a modern society without encountering applications powered by AI [ 10 , 32 ].
Artificial intelligence (AI) can be defined briefly as the branch of computer science that deals with the simulation of intelligent behavior in computers and their capacity to mimic, and ideally improve, human behavior [ 43 ]. AI dominates the fields of science, engineering, and technology, but also is present in education through machine-learning systems and algorithm productions [ 43 ]. For instance, AI has a variety of algorithmic applications in education, such as personalized learning systems to promote students’ learning, automated assessment systems to support teachers in evaluating what students know, and facial recognition systems to provide insights about learners’ behaviors [ 49 ]. Besides these platforms, algorithm systems are prominent in education through different social media outlets, such as social network sites, microblogging systems, and mobile applications. Social media are increasingly integrated into K-12 education [ 7 ] and subordinate learners’ activities to intelligent algorithm systems [ 17 ]. Here, we use the American term “K–12 education” to refer to students’ education in kindergarten (K) (ages 5–6) through 12th grade (ages 17–18) in the United States, which is similar to primary and secondary education or pre-college level schooling in other countries. These AI systems can increase the capacity of K-12 educational systems and support the social and cognitive development of students and teachers [ 55 , 8 ]. More specifically, applications of AI can support instruction in mixed-ability classrooms; while personalized learning systems provide students with detailed and timely feedback about their writing products, automated assessment systems support teachers by freeing them from excessive workloads [ 26 , 42 ].
Despite the benefits of AI applications for education, they pose societal and ethical drawbacks. As the famous scientist, Stephen Hawking, pointed out that weighing these risks is vital for the future of humanity. Therefore, it is critical to take action toward addressing them. The biggest risks of integrating these algorithms in K-12 contexts are: (a) perpetuating existing systemic bias and discrimination, (b) perpetuating unfairness for students from mostly disadvantaged and marginalized groups, and (c) amplifying racism, sexism, xenophobia, and other forms of injustice and inequity [ 40 ]. These algorithms do not occur in a vacuum; rather, they shape and are shaped by ever-evolving cultural, social, institutional and political forces and structures [ 33 , 34 ]. As academics, scientists, and citizens, we have a responsibility to educate teachers and students to recognize the ethical challenges and implications of algorithm use. To create a future generation where an inclusive and diverse citizenry can participate in the development of the future of AI, we need to develop opportunities for K-12 students and teachers to learn about AI via AI- and ethics-based curricula and professional development [ 2 , 58 ]
Toward this end, the existing literature provides little guidance and contains a limited number of studies that focus on supporting K-12 students and teachers’ understanding of social, cultural, and ethical implications of AI [ 2 ]. Most studies reflect university students’ engagement with ethical ideas about algorithmic bias, but few addresses how to promote students’ understanding of AI and ethics in K-12 settings. Therefore, this article: (a) synthesizes ethical issues surrounding AI in education as identified in the educational literature, (b) reflects on different approaches and curriculum materials available for teaching students about AI and ethics (i.e., featuring materials from the MIT Media Lab and Code.org), and (c) articulates future directions for research and recommendations for practitioners seeking to navigate AI and ethics in K-12 settings.
Next, we briefly define the notion of artificial intelligence (AI) and its applications through machine-learning and algorithm systems. As educational and educational technology scholars working in the United States, and at the risk of oversimplifying, we provide only a brief definition of AI below, and recognize that definitions of AI are complex, multidimensional, and contested in the literature [ 9 , 16 , 38 ]; an in-depth discussion of these complexities, however, is beyond the scope of this paper. Second, we describe in more detail five applications of AI in education, outlining their potential benefits for educators and students. Third, we describe the ethical challenges they raise by posing the question: “how and in what ways do algorithms manipulate us?” Fourth, we explain how to support students’ learning about AI and ethics through different curriculum materials and teaching practices in K-12 settings. Our goal here is to provide strategies for practitioners to reap the benefits while navigating the ethical challenges. We acknowledge that in centering this work within U.S. education, we highlight certain ethical issues that educators in other parts of the world may see as less prominent. For example, the European Union (EU) has highlighted ethical concerns and implications of AI, emphasized privacy protection, surveillance, and non-discrimination as primary areas of interest, and provided guidelines on how trustworthy AI should be [ 3 , 15 , 23 ]. Finally, we reflect on future directions for educational and other research that could support K-12 teachers and students in reaping the benefits while mitigating the drawbacks of AI in education.
Definition and applications of artificial intelligence
The pursuit of creating intelligent machines that replicate human behavior has accelerated with the realization of artificial intelligence. With the latest advancements in computer science, a proliferation of definitions and explanations of what counts as AI systems has emerged. For instance, AI has been defined as “the ability of a digital computer or computer-controlled robot to perform tasks commonly associated with intelligent beings” [ 49 ]. This particular definition highlights the mimicry of human behavior and consciousness. Furthermore, AI has been defined as “the combination of cognitive automation, machine learning, reasoning, hypothesis generation and analysis, natural language processing, and intentional algorithm mutation producing insights and analytics at or above human capability” [ 31 ]. This definition incorporates the different sub-fields of AI together and underlines their function while reaching at or above human capability.
Combining these definitions, artificial intelligence can be described as the technology that builds systems to think and act like humans with the ability of achieving goals . AI is mainly known through different applications and advanced computer programs, such as recommender systems (e.g., YouTube, Netflix), personal assistants (e.g., Apple’s Siri), facial recognition systems (e.g., Facebook’s face detection in photographs), and learning apps (e.g., Duolingo) [ 32 ]. To build on these programs, different sub-fields of AI have been used in a diverse range of applications. Evolutionary algorithms and machine learning are most relevant to AI in K-12 education.
Algorithms are the core elements of AI. The history of AI is closely connected to the development of sophisticated and evolutionary algorithms. An algorithm is a set of rules or instructions that is to be followed by computers in problem-solving operations to achieve an intended end goal. In essence, all computer programs are algorithms. They involve thousands of lines of codes which represent mathematical instructions that the computer follows to solve the intended problems (e.g., as computing numerical calculation, processing an image, and grammar-checking in an essay). AI algorithms are applied to fields that we might think of as essentially human behavior—such as speech and face recognition, visual perception, learning, and decision-making and learning. In that way, algorithms can provide instructions for almost any AI system and application we can conceive [ 27 ].
Machine learning
Machine learning is derived from statistical learning methods and uses data and algorithms to perform tasks which are typically performed by humans [ 43 ]. Machine learning is about making computers act or perform without being given any line-by-line step [ 29 ]. The working mechanism of machine learning is the learning model’s exposure to ample amounts of quality data [ 41 ]. Machine-learning algorithms first analyze the data to determine patterns and to build a model and then predict future values through these models. In other words, machine learning can be considered a three-step process. First, it analyzes and gathers the data, and then, it builds a model to excel for different tasks, and finally, it undertakes the action and produces the desired results successfully without human intervention [ 29 , 56 ]. The widely known AI applications such as recommender or facial recognition systems have all been made possible through the working principles of machine learning.
Benefits of AI applications in education
Personalized learning systems, automated assessments, facial recognition systems, chatbots (social media sites), and predictive analytics tools are being deployed increasingly in K-12 educational settings; they are powered by machine-learning systems and algorithms [ 29 ]. These applications of AI have shown promise to support teachers and students in various ways: (a) providing instruction in mixed-ability classrooms, (b) providing students with detailed and timely feedback on their writing products, (c) freeing teachers from the burden of possessing all knowledge and giving them more room to support their students while they are observing, discussing, and gathering information in their collaborative knowledge-building processes [ 26 , 50 ]. Below, we outline benefits of each of these educational applications in the K-12 setting before turning to a synthesis of their ethical challenges and drawbacks.
Personalized learning systems
Personalized learning systems, also known as adaptive learning platforms or intelligent tutoring systems, are one of the most common and valuable applications of AI to support students and teachers. They provide students access to different learning materials based on their individual learning needs and subjects [ 55 ]. For example, rather than practicing chemistry on a worksheet or reading a textbook, students may use an adaptive and interactive multimedia version of the course content [ 39 ]. Comparing students’ scores on researcher-developed or standardized tests, research shows that the instruction based on personalized learning systems resulted in higher test scores than traditional teacher-led instruction [ 36 ]. Microsoft’s recent report (2018) of over 2000 students and teachers from Singapore, the U.S., the UK, and Canada shows that AI supports students’ learning progressions. These platforms promise to identify gaps in students’ prior knowledge by accommodating learning tools and materials to support students’ growth. These systems generate models of learners using their knowledge and cognition; however, the existing platforms do not yet provide models for learners’ social, emotional, and motivational states [ 28 ]. Considering the shift to remote K-12 education during the COVID-19 pandemic, personalized learning systems offer a promising form of distance learning that could reshape K-12 instruction for the future [ 35 ].
Automated assessment systems
Automated assessment systems are becoming one of the most prominent and promising applications of machine learning in K-12 education [ 42 ]. These scoring algorithm systems are being developed to meet the need for scoring students’ writing, exams and assignments, and tasks usually performed by the teacher. Assessment algorithms can provide course support and management tools to lessen teachers’ workload, as well as extend their capacity and productivity. Ideally, these systems can provide levels of support to students, as their essays can be graded quickly [ 55 ]. Providers of the biggest open online courses such as Coursera and EdX have integrated automated scoring engines into their learning platforms to assess the writings of hundreds of students [ 42 ]. On the other hand, a tool called “Gradescope” has been used by over 500 universities to develop and streamline scoring and assessment [ 12 ]. By flagging the wrong answers and marking the correct ones, the tool supports instructors by eliminating their manual grading time and effort. Thus, automated assessment systems deal very differently with marking and giving feedback to essays compared to numeric assessments which analyze right or wrong answers on the test. Overall, these scoring systems have the potential to deal with the complexities of the teaching context and support students’ learning process by providing them with feedback and guidance to improve and revise their writing.
Facial recognition systems and predictive analytics
Facial recognition software is used to capture and monitor students’ facial expressions. These systems provide insights about students’ behaviors during learning processes and allow teachers to take action or intervene, which, in turn, helps teachers develop learner-centered practices and increase student’s engagement [ 55 ]. Predictive analytics algorithm systems are mainly used to identify and detect patterns about learners based on statistical analysis. For example, these analytics can be used to detect university students who are at risk of failing or not completing a course. Through these identifications, instructors can intervene and get students the help they need [ 55 ].
Social networking sites and chatbots
Social networking sites (SNSs) connect students and teachers through social media outlets. Researchers have emphasized the importance of using SNSs (such as Facebook) to expand learning opportunities beyond the classroom, monitor students’ well-being, and deepen student–teacher relations [ 5 ]. Different scholars have examined the role of social media in education, describing its impact on student and teacher learning and scholarly communication [ 6 ]. They point out that the integration of social media can foster students’ active learning, collaboration skills, and connections with communities beyond the classroom [ 6 ]. Chatbots also take place in social media outlets through different AI systems [ 21 ]. They are also known as dialogue systems or conversational agents [ 26 , 52 ]. Chatbots are helpful in terms of their ability to respond naturally with a conversational tone. For instance, a text-based chatbot system called “Pounce” was used at Georgia State University to help students through the registration and admission process, as well as financial aid and other administrative tasks [ 7 ].
In summary, applications of AI can positively impact students’ and teachers’ educational experiences and help them address instructional challenges and concerns. On the other hand, AI cannot be a substitute for human interaction [ 22 , 47 ]. Students have a wide range of learning styles and needs. Although AI can be a time-saving and cognitive aide for teachers, it is but one tool in the teachers’ toolkit. Therefore, it is critical for teachers and students to understand the limits, potential risks, and ethical drawbacks of AI applications in education if they are to reap the benefits of AI and minimize the costs [ 11 ].
Ethical concerns and potential risks of AI applications in education
The ethical challenges and risks posed by AI systems seemingly run counter to marketing efforts that present algorithms to the public as if they are objective and value-neutral tools. In essence, algorithms reflect the values of their builders who hold positions of power [ 26 ]. Whenever people create algorithms, they also create a set of data that represent society’s historical and systemic biases, which ultimately transform into algorithmic bias. Even though the bias is embedded into the algorithmic model with no explicit intention, we can see various gender and racial biases in different AI-based platforms [ 54 ].
Considering the different forms of bias and ethical challenges of AI applications in K-12 settings, we will focus on problems of privacy, surveillance, autonomy, bias, and discrimination (see Fig. 1 ). However, it is important to acknowledge that educators will have different ethical concerns and challenges depending on their students’ grade and age of development. Where strategies and resources are recommended, we indicate the age and/or grade level of student(s) they are targeting (Fig. (Fig.2 2 ).

Potential ethical and societal risks of AI applications in education

Student work from the activity of “Youtube Redesign” (MIT Media Lab, AI and Ethics Curriculum, p.1, [ 45 ])
One of the biggest ethical issues surrounding the use of AI in K-12 education relates to the privacy concerns of students and teachers [ 47 , 49 , 54 ]. Privacy violations mainly occur as people expose an excessive amount of personal information in online platforms. Although existing legislation and standards exist to protect sensitive personal data, AI-based tech companies’ violations with respect to data access and security increase people’s privacy concerns [ 42 , 54 ]. To address these concerns, AI systems ask for users’ consent to access their personal data. Although consent requests are designed to be protective measures and to help alleviate privacy concerns, many individuals give their consent without knowing or considering the extent of the information (metadata) they are sharing, such as the language spoken, racial identity, biographical data, and location [ 49 ]. Such uninformed sharing in effect undermines human agency and privacy. In other words, people’s agency diminishes as AI systems reduce introspective and independent thought [ 55 ]. Relatedly, scholars have raised the ethical issue of forcing students and parents to use these algorithms as part of their education even if they explicitly agree to give up privacy [ 14 , 48 ]. They really have no choice if these systems are required by public schools.
Another ethical concern surrounding the use of AI in K-12 education is surveillance or tracking systems which gather detailed information about the actions and preferences of students and teachers. Through algorithms and machine-learning models, AI tracking systems not only necessitate monitoring of activities but also determine the future preferences and actions of their users [ 47 ]. Surveillance mechanisms can be embedded into AI’s predictive systems to foresee students’ learning performances, strengths, weaknesses, and learning patterns . For instance, research suggests that teachers who use social networking sites (SNSs) for pedagogical purposes encounter a number of problems, such as concerns in relation to boundaries of privacy, friendship authority, as well as responsibility and availability [ 5 ]. While monitoring and patrolling students’ actions might be considered part of a teacher’s responsibility and a pedagogical tool to intervene in dangerous online cases (such as cyber-bullying or exposure to sexual content), such actions can also be seen as surveillance systems which are problematic in terms of threatening students’ privacy. Monitoring and tracking students’ online conversations and actions also may limit their participation in the learning event and make them feel unsafe to take ownership for their ideas. How can students feel secure and safe, if they know that AI systems are used for surveilling and policing their thoughts and actions? [ 49 ].
Problems also emerge when surveillance systems trigger issues related to autonomy, more specifically, the person’s ability to act on her or his own interest and values. Predictive systems which are powered by algorithms jeopardize students and teachers’ autonomy and their ability to govern their own life [ 46 , 47 ]. Use of algorithms to make predictions about individuals’ actions based on their information raise questions about fairness and self-freedom [ 19 ]. Therefore, the risks of predictive analysis also include the perpetuation of existing bias and prejudices of social discrimination and stratification [ 42 ].
Finally, bias and discrimination are critical concerns in debates of AI ethics in K-12 education [ 6 ]. In AI platforms, the existing power structures and biases are embedded into machine-learning models [ 6 ]. Gender bias is one of the most apparent forms of this problem, as the bias is revealed when students in language learning courses use AI to translate between a gender-specific language and one that is less-so. For example, while Google Translate translated the Turkish equivalent of “S he/he is a nurse ” into the feminine form, it also translated the Turkish equivalent of “ She/he is a doctor ” into the masculine form [ 33 ]. This shows how AI models in language translation carry the societal biases and gender-specific stereotypes in the data [ 40 ]. Similarly, a number of problematic cases of racial bias are also associated with AI’s facial recognition systems. Research shows that facial recognition software has improperly misidentified a number of African American and Latino American people as convicted felons [ 42 ].
Additionally, biased decision-making algorithms reveal themselves throughout AI applications in K-12 education: personalized learning, automated assessment, SNSs, and predictive systems in education. Although the main promise of machine-learning models is increased accuracy and objectivity, current incidents have revealed the contrary. For instance, England’s A-level and GCSE secondary level examinations were cancelled due to the pandemic in the summer of 2020 [ 1 , 57 ]. An alternative assessment method was implemented to determine the qualification grades of students. The grade standardization algorithm was produced by the regulator Ofqual. With the assessment of Ofqual’s algorithm based on schools' previous examination results, thousands of students were shocked to receive unexpectedly low grades. Although a full discussion of the incident is beyond the scope of this article [ 51 ] it revealed how the score distribution favored students who attended private or independent schools, while students from underrepresented groups were hit hardest. Unfortunately, automated assessment algorithms have the potential to reconstruct unfair and inconsistent results by disrupting student’s final scores and future careers [ 53 ].
Teaching and understanding AI and ethics in educational settings
These ethical concerns suggest an urgent need to introduce students and teachers to the ethical challenges surrounding AI applications in K-12 education and how to navigate them. To meet this need, different research groups and nonprofit organizations offer a number of open-access resources based on AI and ethics. They provide instructional materials for students and teachers, such as lesson plans and hands-on activities, and professional learning materials for educators, such as open virtual learning sessions. Below, we describe and evaluate three resources: “AI and Ethics” curriculum and “AI and Data Privacy” workshop from the Massachusetts Institute of Technology (MIT) Media Lab as well as Code.org’s “AI and Oceans” activity. For readers who seek to investigate additional approaches and resources for K-12 level AI and ethics interaction, see: (a) The Chinese University of Hong Kong (CUHK)’s AI for the Future Project (AI4Future) [ 18 ]; (b) IBM’s Educator’s AI Classroom Kit [ 30 ], Google’s Teachable Machine [ 25 ], UK-based nonprofit organization Apps for Good [ 4 ], and Machine Learning for Kids [ 37 ].
"AI and Ethics Curriulum" for middle school students by MIT Media Lab
The MIT Media Lab team offers an open-access curriculum on AI and ethics for middle school students and teachers. Through a series of lesson plans and hand-on activities, teachers are guided to support students’ learning of the technical terminology of AI systems as well as the ethical and societal implications of AI [ 2 ]. The curriculum includes various lessons tied to learning objectives. One of the main learning goals is to introduce students to basic components of AI through algorithms, datasets, and supervised machine-learning systems all while underlining the problem of algorithmic bias [ 45 ]. For instance, in the activity “ AI Bingo” , students are given bingo cards with various AI systems, such as online search engine, customer service bot, and weather app. Students work with their partners collaboratively on these AI systems. In their AI Bingo chart, students try to identify what prediction the selected AI system makes and what dataset it uses. In that way, they become more familiar with the notions of dataset and prediction in the context of AI systems [ 45 ].
In the second investigation, “Algorithms as Opinions” , students think about algorithms as recipes, which are created by set of instructions that modify an input to produce an output [ 45 ]. Initially, students are asked to write an algorithm to make the “ best” jelly sandwich and peanut butter. They explore what it means to be “ best” and see how their opinions of best in their recipes are reflected in their algorithms. In this way, students are able to figure out that algorithms can have various motives and goals. Following this activity, students work on the “Ethical Matrix” , building on the idea of the algorithms as opinions [ 45 ]. During this investigation, students first refer back to their developed algorithms through their “best” jelly sandwich and peanut butter. They discuss what counts as the “best” sandwich for themselves (most healthy, practical, delicious, etc.). Then, through their ethical matrix (chart), students identify different stakeholders (such as their parents, teacher, or doctor) who care about their peanut butter and jelly sandwich algorithm. In this way, the values and opinions of those stakeholders also are embedded in the algorithm. Students fill out an ethical matrix and look for where those values conflict or overlap with each other. This matrix is a great tool for students to recognize different stakeholders in a system or society and how they are able to build and utilize the values of the algorithms in an ethical matrix.
The final investigation which teaches about the biased nature of algorithms is “Learning and Algorithmic Bias” [ 45 ]. During the investigation, students think further about the concept of classification. Using Google’s Teachable Machine tool [ 2 ], students explore the supervised machine-learning systems. Students train a cat–dog classifier using two different datasets. While the first dataset reflects the cats as the over-represented group, the second dataset indicates the equal and diverse representation between dogs and cats [ 2 ]. Using these datasets, students compare the accuracy between the classifiers and then discuss which dataset and outcome are fairer. This activity leads students into a discussion about the occurrence of bias in facial recognition algorithms and systems [ 2 ].
In the rest of the curriculum, similar to the AI Bingo investigation, students work with their partners to determine the various forms of AI systems in the YouTube platform (such as its recommender algorithm and advertisement matching algorithm). Through the investigation of “ YouTube Redesign”, students redesign YouTube’s recommender system. They first identify stakeholders and their values in the system, and then use an ethical matrix to reflect on the goals of their YouTube’s recommendation algorithm [ 45 ]. Finally, through the activity of “YouTube Socratic Seminar” , students read an abridged version of Wall Street Journal article by participating in a Socratic seminar. The article was edited to shorten the text and to provide more accessible language for middle school students. They discuss which stakeholders were most influential or significant in proposing changes in the YouTube Kids app and whether or not technologies like auto play should ever exist. During their discussion, students engage with the questions of: “Which stakeholder is making the most change or has the most power?”, “Have you ever seen an inappropriate piece of content on YouTube? What did you do?” [ 45 ].
Overall, the MIT Media Lab’s AI and Ethics curriculum is a high quality, open-access resource with which teachers can introduce middle school students to the risks and ethical implications of AI systems. The investigations described above involve students in collaborative, critical thinking activities that force them to wrestle with issues of bias and discrimination in AI, as well as surveillance and autonomy through the predictive systems and algorithmic bias.
“AI and Data Privacy” workshop series for K-9 students by MIT Media Lab
Another quality resource from the MIT Media Lab’s Personal Robots Group is a workshop series designed to teach students (between the ages 7 and 14) about data privacy and introduce them to designing and prototyping data privacy features. The group has made the content, materials, worksheets, and activities of the workshop series into an open-access online document, freely available to teachers [ 44 ].
The first workshop in the series is “ Mystery YouTube Viewer: A lesson on Data Privacy” . During the workshop, students engage with the question of what privacy and data mean [ 44 ]. They observe YouTube’s home page from the perspective of a mystery user. Using the clues from the videos, students make predictions about what the characters in the videos might look like or where they might live. In a way, students imitate YouTube algorithms’ prediction mode about the characters. Engaging with these questions and observations, students think further about why privacy and boundaries are important and how each algorithm will interpret us differently based on who creates the algorithm itself.
The second workshop in the series is “ Designing ads with transparency: A creative workshop” . Through this workshop, students are able to think further about the meaning, aim, and impact of advertising and the role of advertisements in our lives [ 44 ]. Students collaboratively create an advertisement using an everyday object. The objective is to make the advertisement as “transparent” as possible. To do that, students learn about notions of malware and adware, as well as the components of YouTube advertisements (such as sponsored labels, logos, news sections, etc.). By the end of the workshop, students design their ads as a poster, and they share with their peers.
The final workshop in MIT’s AI and data privacy series is “ Designing Privacy in Social Media Platforms”. This workshop is designed to teach students about YouTube, design, civics, and data privacy [ 44 ]. During the workshop, students create their own designs to solve one of the biggest challenges of the digital era: problems associated with online consent. The workshop allows students to learn more about the privacy laws and how they impact youth in terms of media consumption. Students consider YouTube within the lenses of the Children’s Online Privacy Protections Rule (COPPA). In this way, students reflect on one of the components of the legislation: how might students get parental permission (or verifiable consent)?
Such workshop resources seem promising in helping educate students and teachers about the ethical challenges of AI in education. Specifically, social media such as YouTube are widely used as a teaching and learning tool within K-12 classrooms and beyond them, in students’ everyday lives. These workshop resources may facilitate teachers’ and students’ knowledge of data privacy issues and support them in thinking further about how to protect privacy online. Moreover, educators seeking to implement such resources should consider engaging students in the larger question: who should own one’s data? Teaching students the underlying reasons for laws and facilitating debate on the extent to which they are just or not could help get at this question.
Investigation of “AI for Oceans” by Code.org
A third recommended resource for K-12 educators trying to navigate the ethical challenges of AI with their students comes from Code.org, a nonprofit organization focused on expanding students’ participation in computer science. Sponsored by Microsoft, Facebook, Amazon, Google, and other tech companies, Code.org aims to provide opportunities for K-12 students to learn about AI and machine-learning systems [ 20 ]. To support students (grades 3–12) in learning about AI, algorithms, machine learning, and bias, the organization offers an activity called “ AI for Oceans ”, where students are able to train their machine-learning models.
The activity is provided as an open-access tutorial for teachers to help their students explore how to train, model and classify data , as well as to understand how human bias plays a role in machine-learning systems. During the activity, students first classify the objects as either “fish” or “not fish” in an attempt to remove trash from the ocean. Then, they expand their training data set by including other sea creatures that belong underwater. Throughout the activity, students are also able to watch and interact with a number of visuals and video tutorials. With the support of their teachers, they discuss machine learning, steps and influences of training data, as well as the formation and risks of biased data [ 20 ].
Future directions for research and teaching on AI and ethics
In this paper, we provided an overview of the possibilities and potential ethical and societal risks of AI integration in education. To help address these risks, we highlighted several instructional strategies and resources for practitioners seeking to integrate AI applications in K-12 education and/or instruct students about the ethical issues they pose. These instructional materials have the potential to help students and teachers reap the powerful benefits of AI while navigating ethical challenges especially related to privacy concerns and bias. Existing research on AI in education provides insight on supporting students’ understanding and use of AI [ 2 , 13 ]; however, research on how to develop K-12 teachers’ instructional practices regarding AI and ethics is still in its infancy.
Moreover, current resources, as demonstrated above, mainly address privacy and bias-related ethical and societal concerns of AI. Conducting more exploratory and critical research on teachers’ and students’ surveillance and autonomy concerns will be important to designing future resources. In addition, curriculum developers and workshop designers might consider centering culturally relevant and responsive pedagogies (by focusing on students’ funds of knowledge, family background, and cultural experiences) while creating instructional materials that address surveillance, privacy, autonomy, and bias. In such student-centered learning environments, students voice their own cultural and contextual experiences while trying to critique and disrupt existing power structures and cultivate their social awareness [ 24 , 36 ].
Finally, as scholars in teacher education and educational technology, we believe that educating future generations of diverse citizens to participate in the ethical use and development of AI will require more professional development for K-12 teachers (both pre-service and in-service). For instance, through sustained professional learning sessions, teachers could engage with suggested curriculum resources and teaching strategies as well as build a community of practice where they can share and critically reflect on their experiences with other teachers. Further research on such reflective teaching practices and students’ sense-making processes in relation to AI and ethics lessons will be essential to developing curriculum materials and pedagogies relevant to a broad base of educators and students.
This work was supported by the Graduate School at Michigan State University, College of Education Summer Research Fellowship.
Data availability
Code availability, declarations.
The authors declare that they have no conflict of interest.
Publisher's Note
Springer Nature remains neutral with regard to jurisdictional claims in published maps and institutional affiliations.
Contributor Information
Selin Akgun, Email: ude.usm@lesnugka .
Christine Greenhow, Email: ude.usm@wohneerg .
- Case studies, insights and research
Artificial intelligence and English language teaching: Preparing for the future
How is artificial intelligence (AI) being used for English language teaching and learning (ELT/L) worldwide? What are the opportunities, issues, and challenges? Educational technology experts working with the British Council looked at the current literature and consulted a range of people to understand their views on this subject.

This page includes a summary of our findings.
AI can be described as computer systems that mimic human intelligence and can understand human language. However, AI means different things to different people and clear definitions are needed. AI technologies can be: 1) used by pupils to learn, 2) used by teachers to help in teaching activities e.g., grading, and 3) used by admin staff to manage learner data 1 .
What the literature says
Experts at the British Council and Dr Helen Crompton from the Research Institute of Digital Innovation in Learning at ODUGlobal examined 43 research studies about this topic. They found:
- AI tools are being used to improve speaking, writing, and reading skills. It can provide new ways of teaching and supports students with goal setting and managing their own learning. AI tools don’t seem to be used much for improving listening skills.
- AI tools can help learners practise English outside class. They can also lessen learners’ fear of speaking in English. But we need more research to see if these benefits last without the continued use of AI.
- Even with rapid changes in technology, traditional lecture-style teaching is common.
- sometimes, the technologies don’t work as they should
- AI has limited capabilities
- some learners fear using AI
- use of AI may reflect biases about ‘appropriate’ language use.
As ELT is the most common discipline for AI use in education 2 , English language teachers must develop their AI literacy skills. Teachers should also develop learners’ AI literacy so that they can understand its limitations and risks. Experts should think carefully about which AI models to use, as models may not include all varieties of English. Clear rules on data privacy and ethics statements for AI in ELT are needed. Future research should include more geographies and learner groups, particularly K–12 (school-level education) and adult learning. We need more research on how AI can help with developing receptive skills, particularly listening. We also need to know more about the specific challenges around AI use in ELT. Finally, we need more studies on how AI can be used for assessment.
What teachers say
The British Council surveyed 1,348 English language teachers from 118 countries and territories to understand their views on AI in their teaching.
Which AI-powered tools do teachers use?
The survey found that the most-used AI tools by teachers were as follows. These are listed in descending order:
- language learning apps
- language generation AI
- chatbots
- automated grading
- speech recognition software
- text-to-speech tools
- data and learning analytics tools
- virtual and augmented reality tools
Interestingly, 24 per cent of teachers said they did not use any AI tools.
What tasks do teachers use AI tools for?
The survey found that teachers used AI powered tools for the following tasks. These are listed in descending order:
- creating materials
- helping students practice English
- creating lesson plans
- correcting students’ English
- grading or assessing students
- administrative tasks
However, 18 per cent of teachers said they didn’t use AI for any of these tasks. 1,112 teachers rated a number of statements about AI in English language teaching. Some teachers also wrote comments to explain their ratings. Here are the key findings:
- Teachers are using AI-powered tools for a range of tasks in English language teaching.
- Teachers feel AI benefits the development of all four English language skills fairly equally.
- Teachers have mixed feelings about how AI affects learning. Some think it impacts their learners’ English development negatively, others see it more positively.
- Many teachers feel they haven’t had enough training on how to use AI. Only some felt they had received enough training on AI.
- Teachers are split on whether AI will change their role. Some are worried about the effect of AI, others are not worried.
- Many believe that English language teaching will continue to be done by humans, not AI.
However, many teachers gave neutral responses to the statements, showing that they are unsure about how AI will affect ELT. As one teacher noted: ‘ Any teaching tool can have a negative impact if not used correctly. ’
What our key witnesses say
The British Council also interviewed 19 participants from 12 countries and territories to get their views. They included academics, ministry of education representatives, CEOs of EdTech companies, training institute directors, and teacher educators. Eleven themes developed from these discussions. These are:
- Definitions: There is a clear need for a set of agreed definitions so that when we discuss Al in ELT, we are talking about the same type of technology.
- Pedagogy: AI may have the potential to be transformative, but the question remains if it will it be held back with outdated learning theory.
- Big Tech and neoliberalism: While some expressed concern about how large, mostly US-based tech companies could influence ELT classrooms, it is not all Big Tech. There is both a place and a need for local, grassroots and more context-sensitive AI.
- Replacing humans: The majority view is that AI will not replace the need for human teachers any time soon and may never.
- Relevance for ELT: There is some evidence that AI will be more usefully deployed in ELT than in other disciplines, but not all are convinced by this idea.
- Bias: Bias is evident in Al and needs to be addressed. Regulatory frameworks can help to manage bias from the top down, but these may be difficult to enforce universally.
- Teacher readiness: There is already a huge knowledge gap around digital literacies. Addressing Al literacy will be a massive challenge.
- Motivation: Motivation remains a barrier or enabler to learning. AI does not appear to be changing that, yet.
- Inclusion: The digital divide is likely to worsen if AI has significant, positive impact on learning outcomes.
- Assessment: More research is needed into AI and assessment in ELT. Preventing cheating with AI may mean use of new and better assessment tasks.
- Ethics frameworks and regulation: There is a need to review all international, regional, and national AI ethics guidelines.
Our findings point us towards future activity. First, we need to agree on definitions of AI so we can be sure we are referring to the same technology type. Then, principles for ethical use of AI in ELT/L can be drawn up. It would also be helpful to list how AI may or may not be used for specific teacher tasks. In the future, AI will likely transform many aspects of how we live. Education tends to lag behind other sectors for many reasons, good (safeguarding, protecting the learning process) and bad (resistance to change, power structures, revenue concerns). Whether or not these new technologies will bring the widespread change in education systems is an ongoing debate.
[1] Pokrivčáková, S. (2019). Preparing teachers for the application of AI-powered technologies in foreign language education. Journal of Language and Cultural Education, 7(3), 135–153. https://doi.org/10.2478/jolace-2019-0025
[2] Crompton, H. & Burke, D. (2023). Artificial intelligence in higher education: The state of the field. International Journal of Educational Technology in Higher Education, 20, 22 (2023). https://doi.org/10.1186/s41239-023-00392-8
I would like to attend this
- Log in or register to post comments
This is not an event, it is a report about AI and the future of English language teaching. You can download the report by clicking / tapping above.
You might be interested in this recording of the Artificial Intelligence and ELT webinar.
Hope you find it useful.
TeachingEnglish team
Research and insight
Browse fascinating case studies, research papers, publications and books by researchers and ELT experts from around the world.
See our publications, research and insight
- DOI: 10.51985/jbumdc2024224
- Corpus ID: 271435868
Artificial intelligence (AI) is an Academic Handicap for the Learners (challenge of a new era)
- Fareeha Shahid
- Published in Journal of Bahria University… 23 July 2024
- Computer Science, Medicine, Education
- Journal of Bahria University Medical and Dental College
9 References
The impact of artificial intelligence on human society and bioethics, artificial intelligence in education: addressing ethical challenges in k-12 settings, the future of chatgpt in academic research and publishing: a commentary for clinical and translational medicine, machine learning: algorithms, real-world applications and research directions, impact of chatgpt on medical chatbots as a disruptive technology, chatgpt for healthcare services: an emerging stage for an innovative perspective, related papers.
Showing 1 through 3 of 0 Related Papers
America's Education News Source
Copyright 2024 The 74 Media, Inc
- EDlection 2024
- Hope Rises in Pine Bluff
- Artificial Intelligence
- science of reading
From COVID Learning Loss to Artificial Intelligence, Education R&D Can’t Wait
Schwinn & wright: in our states, we used the latest research to help students learn. congress, state leaders must make education innovation a priority.
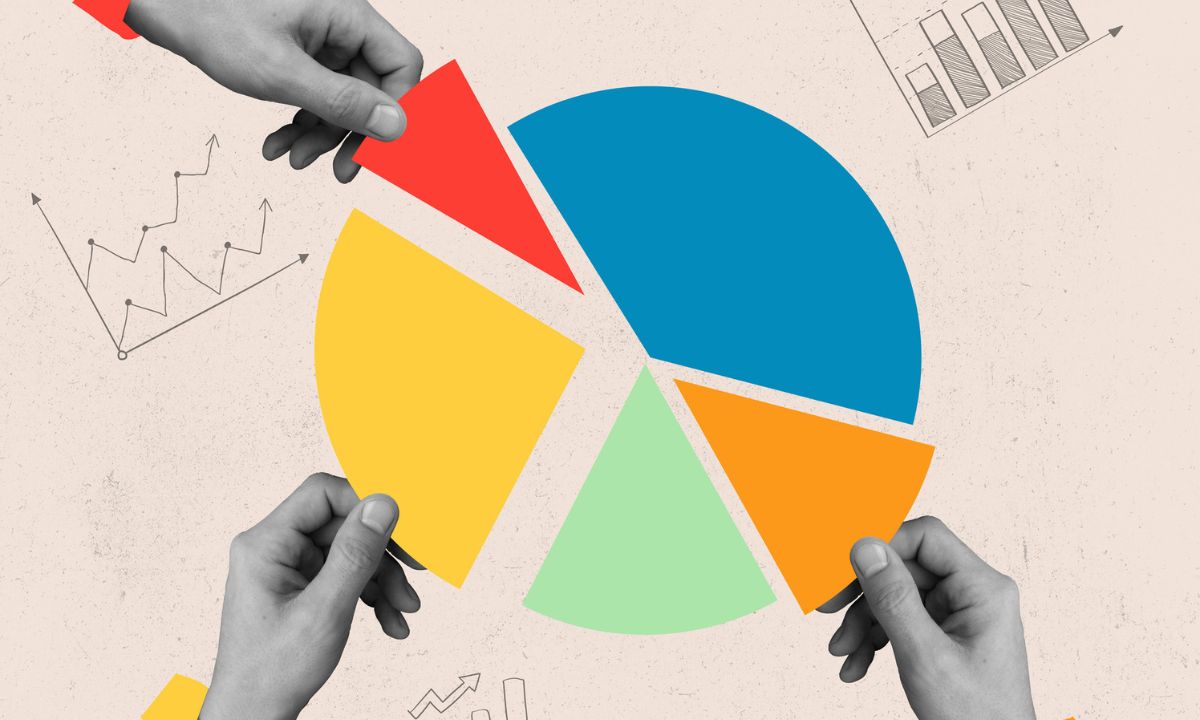
Sharpen Up!
Sign up for our free newsletter and start your day with in-depth reporting on the latest topics in education.
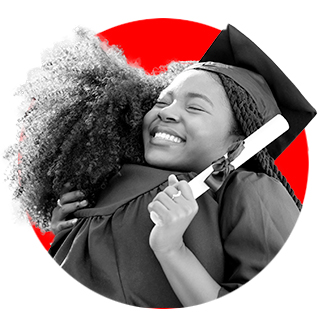
74 Million Reasons to Give
Support The 74’s year-end campaign with a tax-exempt donation and invest in our future.
Most Popular
Students speak out: how to make high schools places where they want to learn, project 2025 would cut ed. dept., fulfill gop k-12 wish list under trump, students headed to high school are academically a year behind, covid study finds, distracted kids: 75% of schools say ‘lack of focus’ hurting student performance, no textbooks, times tables or spelling tests: things my 6th grader didn’t learn.
Get stories like these delivered straight to your inbox. Sign up for The 74 Newsletter
When COVID struck, scientists rushed to stem the pandemic in a coordinated effort that led to the creation of new vaccines in record time, saving millions of lives. These vaccines resulted from decades of investment by the federal government in mRNA research. Investing in research and development is a time-tested and effective way to solve big, complex problems. After all, R&D drives innovation in fields like health care, tech, energy and agriculture.
Unfortunately, the same cannot be said about education. The U.S. has never adequately invested in R&D related to education, so persistent problems remain unsolved and the system is largely unable to handle unexpected emergencies, like COVID. Although strong research does exist, few education leaders use it to guide their decisions on behalf of kids.
As former state education commissioners in Tennessee and Mississippi, we know that education research, when consulted and applied in classrooms, can yield huge academic gains for students.
Take literacy, for example.
For generations, Mississippi students ranked at or near the bottom in national reading scores, and Tennessee didn’t fare much better . In the late 1990s, the federal government poured millions of dollars into researching the most effective ways to teach young people how to read. But like a lot of good education research, those findings did little to change what was happening in classrooms and teachers colleges.
As education leaders, we knew we had to act on the findings, which supported systematic and explicit phonics-based instruction. It’s malpractice to look at stagnant achievement year after year and say, “Let’s keep doing the same thing.”
So we aligned our states’ approaches to what the research said was most effective. In Mississippi, that meant training teachers on the science of reading . In eight years, Mississippi’s national literacy ranking for fourth graders improved 29 places, from 49th to 21st.
For Tennessee, it meant a revised program based on the science of reading and high-quality instructional materials, as well as new tutoring and summer school programs. This led to a nearly 8 percentage point jump in the third-grade reading proficiency rate – to 40% – in just two years.
There is still a long road ahead to get children in Mississippi and Tennessee where they need to be, but the key to each state’s progress was a desire to learn from researchers and implement evidence-based solutions — even if that meant admitting that current strategies weren’t working.
That’s not an easy admission, but from our experience leading education efforts in states both red and blue, we believe that education R&D should be the foundation for every decision that affects student learning. That’s why we are calling on leaders in states and in Congress to make it a top priority.
Why now?
First, students lost significant learning due to COVID, creating an academic gap that may take years to close. Solving this problem requires innovative programs, new platforms and evidence-based approaches. The status quo isn’t sufficient. Education leaders and policymakers need to move with urgency.
Second, America is on the cusp of a new age of technological opportunity. With AI-powered tools like ChatGPT and advances in learning analytics , researchers and developers are just beginning to tap the vast potential these technologies hold for implementing personalized learning, reducing teachers’ administrative responsibilities and improving feedback on student writing . They can even help teachers make sense of education research . Without adequate R&D, however, these technologies may fall short of their potential to help students or – worse – could interfere with learning by perpetuating bias or giving students incorrect information .
But in order to tap this vast potential, the R&D process must be structured around the pressing needs facing schools. Educators, researchers and developers must collaborate to solve real-world classroom problems. Too often, tech tools are conceived by companies with sales in mind, while research agendas are set by academics whose goals and interests do not always align with what schools truly need. Both situations leave educators disconnected from the R&D process, so it’s no wonder they are often unenthusiastic when asked to implement yet another new strategy or tool.
The field needs educators, researchers and companies working together to prioritize which problems to solve, what gets studied, what interventions get developed and where the field goes next. Instead of education leaders selecting from an existing menu of tools and approaches, they should be driving the demand for better options that reflect their students’ needs.
Leaders at both the state and federal levels have an important role to play in making this standard operating procedure.
At the state level, superintendents and other leaders must be deliberate in using research to make evidence-based decisions for the benefit of students. Every state and school district has access to a federally funded Regional Education Laboratory , which stands ready to generate and apply evidence to improve student outcomes. But too few leaders take advantage of this resource. Local universities offer opportunities for partnerships that can benefit K-12 students. For example, the Tennessee Department of Education and the University of Tennessee established a reading research center to study the state’s literacy efforts. Programs like Harvard University’s Strategic Data Project Fellowship and the Invest in What Works State Education Fellowship can provide states and districts with talented and affordable experts who can help build their in-house research capabilities. If you’re a state or district education leader who hasn’t yet tapped into your Regional Lab, forged partnerships with universities or hired an R&D fellow, these are three easy ways to start becoming an evidence-driven leader.
At the federal level, Congress can do much more to engender a bolder approach to education
R&D. A great first step would be to create a National Center for Advanced Development in Education , at the Institute of Education Sciences, the research arm of the U.S. Department of Education.
This center would tackle ambitious projects not otherwise addressed by basic research or the market — and support interdisciplinary teams to conduct outside-the-box R&D. The idea is to create a nimble, flexible research center modeled after agencies like DARPA , whose research produced game-changing inventions like GPS and the Internet. Rather than just making incremental changes, the center would strive to solve the biggest, most complex challenges in education and develop innovations that could fundamentally transform teaching and learning.
Congress can make this possible by passing the bipartisan New Essential Education Discoveries (NEED) Act (H.R. 6691), soon to be introduced in the Senate. Or, the center could be included in a reauthorization of the Education Sciences Reform Act, legislation that shapes the activities of the Institute of Education Sciences and is long overdue for an update.
This is the leadership students need from Congress and state officials, now. Education innovation won’t happen if school systems continue to rely on old ways of thinking and operating. Education needs a bold, “what if” mentality – embracing ambitious goals, smart risks, and game-changing solutions – all guided by the north star of evidence. Only when educators, researchers, companies and policymakers champion a new model for education R&D, will schools pioneer a future where every student receives a truly transformative education.
Dr. Penny Schwinn is a former education commissioner of Tennessee. She is a vice president at the University of Florida.
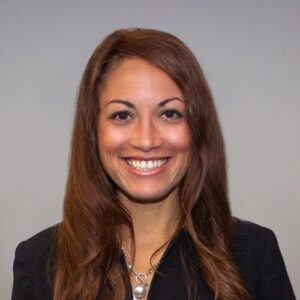
Dr. Carey Wright is the state superintendent of schools in Maryland and former Mississippi state superintendent of education.
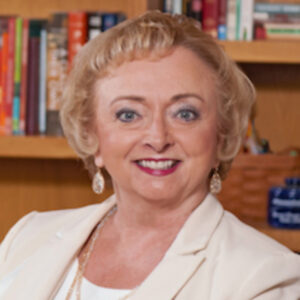
- education research
- mississippi
We want our stories to be shared as widely as possible — for free.
Please view The 74's republishing terms.
By Penny Schwinn & Carey Wright
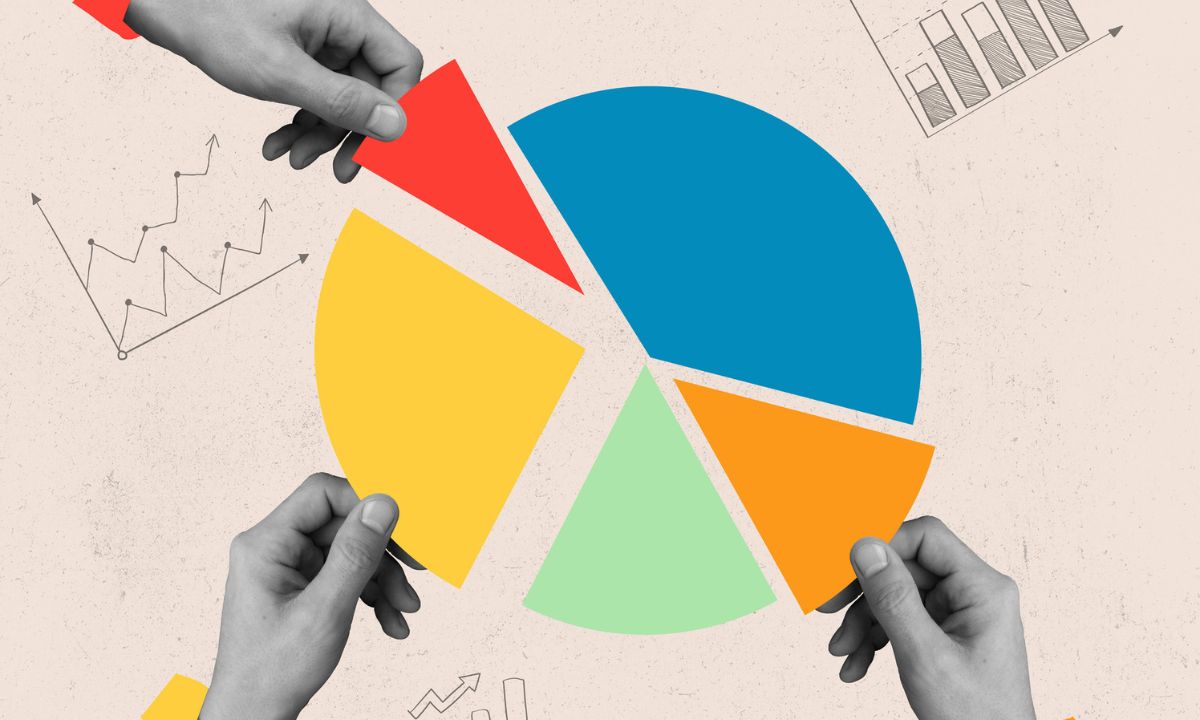
This story first appeared at The 74 , a nonprofit news site covering education. Sign up for free newsletters from The 74 to get more like this in your inbox.
On The 74 Today
- Open access
- Published: 27 July 2024
Artificial intelligence in medical education - perception among medical students
- Preetha Jackson 1 ,
- Gayathri Ponath Sukumaran 1 ,
- Chikku Babu 1 ,
- M. Christa Tony 1 ,
- Deen Stephano Jack 1 ,
- V. R. Reshma 1 ,
- Dency Davis 1 ,
- Nisha Kurian 1 &
- Anjum John 1
BMC Medical Education volume 24 , Article number: 804 ( 2024 ) Cite this article
307 Accesses
10 Altmetric
Metrics details
As Artificial Intelligence (AI) becomes pervasive in healthcare, including applications like robotic surgery and image analysis, the World Medical Association emphasises integrating AI education into medical curricula. This study evaluates medical students’ perceptions of ‘AI in medicine’, their preferences for AI training in education, and their grasp of AI’s ethical implications in healthcare.
Materials & methods
A cross-sectional study was conducted among 325 medical students in Kerala using a pre-validated, semi structured questionnaire. The survey collected demographic data, any past educational experience about AI, participants’ self-evaluation of their knowledge and evaluated self-perceived understanding of applications of AI in medicine. Participants responded to twelve Likert-scale questions targeting perceptions and ethical aspects and their opinions on suggested topics on AI to be included in their curriculum.
Results & discussion
AI was viewed as an assistive technology for reducing medical errors by 57.2% students and 54.2% believed AI could enhance medical decision accuracy. About 49% agreed that AI could potentially improve accessibility to healthcare. Concerns about AI replacing physicians were reported by 37.6% and 69.2% feared a reduction in the humanistic aspect of medicine. Students were worried about challenges to trust (52.9%), patient-physician relationships (54.5%) and breach of professional confidentiality (53.5%). Only 3.7% felttotally competent in informing patients about features and risks associated with AI applications. Strong demand for structured AI training was expressed, particularly on reducing medical errors (76.9%) and ethical issues (79.4%).
This study highlights medical students’ demand for structured AI training in undergraduate curricula, emphasising its importance in addressing evolving healthcare needs and ethical considerations. Despite widespread ethical concerns, the majority perceive AI as an assistive technology in healthcare. These findings provide valuable insights for curriculum development and defining learning outcomes in AI education for medical students.
Peer Review reports
Introduction
The concept of Artificial Intelligence (AI) dates back to the 1950s when Alan Turing, often referred to as the father of computer science, proposed the question, “Can machines think”? Interestingly, he designed the now famous ‘Turing Test’ where humans were to identify the responder of a question as human or machine [ 1 ]. Subsequently in 1956 John McCarthy coined the term “Artificial Intelligence” [ 2 ] and the next decade saw the birth of the first ever artificial neural network which was “the first machine which is capable of having an original idea” [ 3 ]. Thus progressed the growth of this once unimaginable phenomenon. In this 21st century, most people are familiar with the term AI because of Siri (Intelligent Virtual Assistant) [ 4 ], Open AI’s ChatGPT (language model based chatbot) [ 5 ], traffic prediction by Google Maps or Uber [ 6 ] or customer service bots (AI powered assistants) [ 4 ] that intelligently provide suggestions.
There is no universally accepted definition for AI, but it can be simply defined as “the ability of machines to mimic intelligent human behaviour, including problem solving and learning” [ 7 ]. Specific applications of AI include expert systems, natural language processing, speech recognition, machine vision, and many more, applying which AI has exhibited qualities similar to or even above those of humans [ 8 ].
The use of AI and related technologies is becoming increasingly prevalent in all aspects of human life and beginning to influence the field of healthcare too [ 9 ]. AI technologies have already developed algorithms to analyse a variety of health data, including clinical, behavioural, environmental, and drug information using data from both patients as well as biomedical literature [ 10 ]. Convoluted Neural Networks, designed to automatically and adaptively learn spatial hierarchies of features, can be successfully used to develop diabetic retinopathy screening [ 11 ], skin lesion classification [ 12 ], lymph node metastasis detection [ 13 ], and detection of an abnormality in a radiograph [ 14 ].
Artificial Intelligence can help patients understand their symptoms, influence health seeking behaviour, and thereby improve their quality of life [ 15 ]. AI assistants have even suggested medicines for cancer patients with equal or better efficiency than human experts [ 16 ]. With a capable AI assistant, it is possible to sift through and analyse multitudes of data in a matter of seconds and make conclusions, thus exponentially increasing its applications in biomedical research. AI promises future influences in healthcare in terms of AI assisted robotic surgery, virtual nursing assistants, and image analysis. Simply put, AI can help patients and healthcare providers in diagnosing a disease, assessing risk of disease, estimating treatment success, managing complications, and supporting patients [ 17 ].
Though AI has limitless potential, it has certain vulnerabilities and weaknesses. The quality and relevance of the input data can affect the accuracy of a deep learning diagnostic AI.The kind of funding that is required to construct the machinery and develop an intelligence is not easily accessible in the field of medicine, not to mention the constraints of machine ethics and confidentiality. However, being familiar with the concepts, applications and advantages of AI is definitely beneficial and therefore advisable, especially in the field of medical education and policy making [ 17 , 18 ].
The World Medical Association advocates for a change in medical curricula and educational opportunities for patients, physicians, medical students, health administrators, and other health care professionals to foster a better understanding of the numerous aspects of the healthcare AI, both positive and negative [ 19 ]. Additionally, in 2019, the Standing Committee of European Doctors stressed the need to use AI systems in basic and continuing medical education [ 20 ]. They recommended the need for AI systems to be integrated into medical education, residency training, and continuing medical education courses to increase awareness of the proper use of AI. In this context, there is an emerging need for developing curricula specifically designed to train future physicians on AI.
To develop an effective AI curriculum, we need to understand how today’s medical students perceive AI in medicine, and their comprehension of AI’s ethical dimension as well. However, the available need assessment studies in an Indian setting are barely enough. Grunhut et al. had recommended in 2021 that national surveys need to be carried out among medical students on the attitude and expectations of learning AI in medical colleges for developing a curriculum [ 21 ]. Similar unbiased probability based, large scale surveys would identify the realistic goals physicians will be asked to meet, the expectations that will be put on them, and the resources and knowledge they would need to meet these goals. Also, current literature falls short of a comprehensive needs assessment which is important for curriculum development and defining learning outcomes. Hence in this study we aimed to assess the perceptions on ‘AI in medicine’ among Indian medical students, to assess the proportion of medical students who are in favour of structured training on AI applications during their undergraduate course, and also to assess their perceptions on AI’s ethical dimensions.
Recruitment: A cross-sectional study was conducted among the undergraduate medical students of Pushpagiri Institute of Medical Sciences and Research Centre during the period of June – August 2023. An introductory discussion on the purpose and importance of this study was conducted with each batch of students from first year to house surgeons following which the link to the Google-form containing the consent and questionnaire was shared in the batch Whatsapp groups.
There were a total of 500 medical students in the Institute from 1st year MBBS to the medical students undergoing their internship. The Google form was open for 3 months, with reminder messages sent at intervals of one month. Participation was voluntary (informed consent was obtained through the first section of the Google form)due to which no randomisation could be ensured, implying that some selection bias can be expected.
Participants who did not consent or submitted incomplete questionnaires were excluded from the study. An online survey using Google forms was conducted using a validated semi structured questionnaire which had 3 sections. The questions were adopted from a Turkish study by Civaner et al. [ 22 ]. Since the questionnaire was originally drafted in English, there was no need for translation into a comprehensible language. The first section dealt with demographic details (age, gender and year of study), any past educational experience about AI (had attended training or seminars) and participants’ self-evaluation of their knowledge of AI. The second section consisted of 12 five point Likert questions on medical students’ perceptions of AI including five questions on ethical aspects as well, which were expressed in the form of agreement or disagreement. The last section was about their opinions on selected topics on AI - whether they should be included in their medical curriculum or not. A pilot study was undertaken by administering the questionnaire to a group of 20 medical students who were then posted in the Department of Community Medicine.
Statistical Analysis: Responses on medical students’ perception on the possible influences of AI were graded using Likert scale ranging from 0 (totally disagree) to 4 (totally agree). Data was entered into Microsoft Excel and analysed using Statistical Package for Social Sciences 25.0. Age of the participants is expressed as mean with standard deviation and categorical variables such as opinions, perceptions and year of study are expressed as frequencies and percentages.
Out of 500 medical students in the institution, 327 students participated in the survey. After excluding the incomplete questionnaires, data of 325 participants were analysed. Therefore the response rate amounts to 65%.
The mean (SD) age of the participants was 21.4 (1.9) years, (ranging from 18 to 25 years) with 76% (248/325) females.
AI in medicine- prior knowledge and self-evaluation
Majority of students (91.4%)(297/325) stated that they had not received any training on AI in their medical curriculum, while the others mentioned that they had attended events like seminars and presentations on AI. Almost 52%(169/325) students have heard about AI but possess no knowledge of it. One third of the participants (106/325) self-reported to have ‘partial knowledge’ on AI while none of them reported to be ‘very knowledgeable.’
Of all the participants, only 37.2% (121/325) did not agree with the opinion that AI could replace physicians; instead, the majority thought that it could be an assistant or a tool that would help them. About 37.6% (122/325) of participants agreed that the use of AI would reduce the need for physicians and thus result in loss of jobs. More than half of the participants (173/325) agreed that they would become better physicians with the widespread use of AI applications. Almost 35% (114/325) stated that their choice of specialization would be influenced by how AI was used in that field. Only 26.8% (87/325) of participants totally or mostly agreed that they felt competent enough to give information on AI to patients. More than half of the participants (166/325) were unsure of protecting patient confidentiality while using AI.
Perceptions on the possible influences of AI in medicine
Regarding student perceptions on the possible influences of AI in medicine (Fig. 1 ), the highest agreement (72.3%) was observed on the item ‘reduces error in medical practice’ (235/325) while the lowest agreement (40.3%) was on ‘devalues the medical profession’ (131/325). Students were mostly in favour of applying AI in medicine because they felt it would enable them to make more accurate decisions (72%, (234/325) and would facilitate patients’ access to healthcare (60.9%, 198/325). There were 59.4% (193/325) of participants who agreed that AI would facilitate patient education and 50.5% (164/325) who agreed that AI would allow the patient to increase their control over their own health.
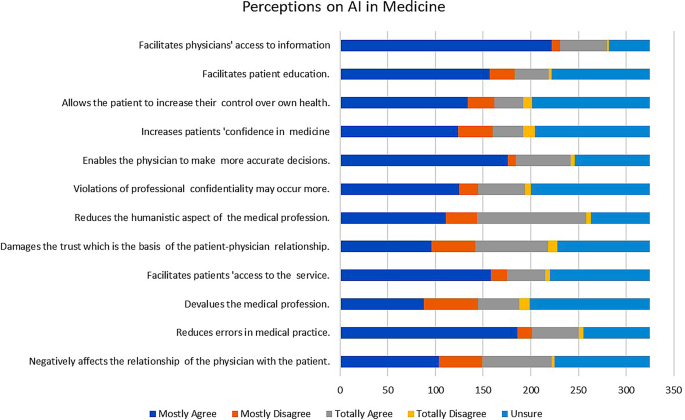
Frequency distribution of perceptions of medical students on AI in medicine
Need for training on AI in medical curriculum
Almost three-fourths of the participants were in favour of structured training on AI applications that should be given during medical education (74.8%, 243/325). The participants thought that it was important to be trained on various topics related to AI in medicine as depicted in Fig. 2 . The most frequent topics that they perceived necessary in this domain were knowledge and skills about AI applications (84.3%274/325), training to prevent and solve ethical problems that may arise with AI applications (79.4%258/325), and AI assisted risk analysis for diseases (78.1%254/325).
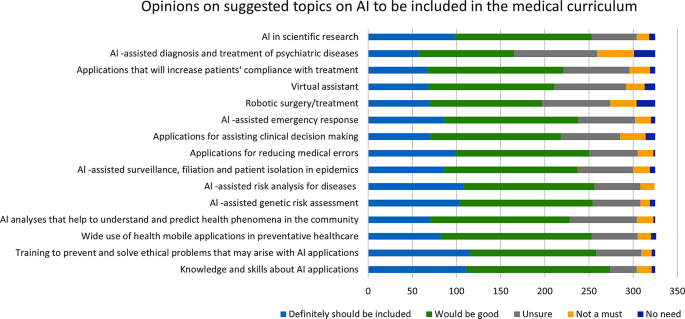
Frequency distribution of opinions of medical students as to whether the suggested topics should be included in their medical curriculum
Ethical concerns regarding AI in medicine (Table 1 )
On the topic of disadvantages and risks of using AI in medicine 69.2% (225/325) agreed that AI would reduce the humanistic aspect of the medical profession, 54.5% (177/325) agreed that it could negatively affect the patient-physician relationship, 52.9% (173/325) were concerned that using AI assisted applications can damage trust in patients while 53.5% (174/325) thought that AI could possibly cause violations of professional confidentiality.
Sub group analysis
Perceptions about being a better doctor with the use of AI applications, being competent enough to inform patients about features & risks of AI applications and the perception about the use of AI in medicine causing a reduction in job opportunities were the ones which showed significant association with the baseline variables like gender, year of study and having prior exposure to course of AI applications as shown in Table 2 .
Although there has been extensive research on the utilisation of AI in medical education the perceptions of medical professionals, and their dilemmas regarding its integration into their daily practice remains relatively underexplored. This research is focused on the perception of medical students about the use of Artificial Intelligence in medicine and its ethical aspects, which reflects their confusions and concerns regarding the situation.
The mean age of the medical students studied was around 21 years and the majority of students were females. Most participants in our study (53.3%) agreed that AI could not replace the presence of a physician but could help them in their work. This is in accordance with the 2021 study conducted by Bisdas S et al. on medical students from 63 countries that AI could work as a “partner” rather than as a “competitor” in their medical practice. A third of our participants (37.6%) felt that the use of AI would reduce the need for physicians and would result in a loss of job opportunities for them. This is a different finding than the study published by D Pinto Dos Santos in European Radiology in 2019 where a majority of participants (83%) felt that human radiologists would not be replaced by robots or computers [ 23 ]. In fact, there are many studies which argue that rather than physicians becoming redundant because of AI, they would change their practice and become “managers” rather than “custodians of information” [ 24 , 25 ].
More than half the respondents in our study (53.3%) agreed that they would become better physicians with the widespread use of AI applications. This is in concurrence with a recently published Western Australian study among medical students which showed about 75% of the participants agreeing that AI would improve their practice [ 26 ]. Respondents from other studies felt that currently available AI systems would actually complement physicians’ decision-making skills by synthesising large amounts of medical literature in order to produce the most up-to-date medical protocols and evidence [ 27 , 28 , 29 , 30 ]. Similarly, studies show that AI systems actually work by complementing the practice of medicine, rather than competing with human minds. After all, human minds have designed artificial intelligence. Furthermore, the study by Paranjape et al. comments that physicians will be able to focus on providing patients with the humanistic care considering the biopsychosocial model of disease as the technicalities can be handled by the AI supported technologies to a greater extent [ 28 ].
A third of the participants (35.1%) in our research stated that their choice of specialisation would be influenced by how AI was used in that field. Much has been written about how AI might replace specialists in the fields of radiology and pathology as perceived by medical doctors and students. These are specialisations that use computers and digital algorithms more when compared to other medical specialties. A Canadian study published in 2019 by Bo Gong et al. found that 67% of the respondents felt that AI would “reduce the demand” for radiologists. Many of the medical students interviewed in this study said that the anxiety they felt about being “displaced” by AI technologies in radiology would discourage them from considering the field for specialisation [ 14 , 31 , 32 , 33 ]. In fact, a paper published by Yurdasik et al. in 2021 had respondents encouraging practitioners to move away from specialisations that used AI [ 34 ]. However, there were other studies that reported results encouraging radiologists to get exposed to AI technologies so as to lower the rates of “imaging related medical errors” and “lessening time spent in reading films,” resulting in more time spent with patients. German medical students have shown a positive attitude towards AI and have reported “not being afraid of being replaced by AI” should they choose radiology as their specialisation [ 23 ]. Attitude towards the choice of specialisation being influenced by AI depended on where the person was viewing the problem from- as a student or as a specialist and also from the degree of familiarity they had with AI applications.
The majority of the students (91.4%) stated that they had not received any training on AI in medicine. The American Medical Association meeting of 2018 on Augmented Intelligence advocated for the training of physicians so that they could understand algorithms and work effectively with AI systems to make the best clinical care decisions for their patients [ 35 ]. Despite this, Paranjape et al. reported that training on the backend of electronic health record systems like, the quality of the data obtained, impact of computer use in front of patients, patient physician relationships etc. have not been addressed through medical education. If used with adequate training and understanding, AI will free up physicians’ time/ optimise a physician’s work hours, so that they can care and communicate with patients in the free time thus obtained. The findings of the research published by Jha et al. in the year 2022 agrees with this observation regarding inadequate coverage of AI and machine learning in medical curricula [ 36 ]. This deficiency leaves medical students underprepared to navigate the integration of AI technologies into their future practice. A significant percentage (37.6%) of respondents expressed concerns about job displacements due to AI, echoing sentiments observed in previous research. The concerns on AI induced loss of jobs particularly in fields like radiology and pathology, accentuate the importance of addressing misconceptions and fostering a meticulous understanding of AI’s role in healthcare. Jha et al’s study also highlights the importance of integrating soft skills, such as compassion and empathy, alongside AI education. Medical students must be equipped not only with technical AI competencies but also with the interpersonal skills necessary for holistic patient care. Collaborative efforts are needed to develop curricula that balance AI education with the cultivation of humanistic values, ensuring that future healthcare professionals can effectively navigate the intersection of technology and patient-centred care.
A major proportion of students in the study conducted by Sharma et al. demonstrated only a limited understanding of AI’s applications in medicine, primarily attributed to a lack of formal education, awareness, and interest. Interestingly, while a substantial portion (26.3%) of respondents demonstrated familiarity with AI, the majority (53.6%) exhibited only a superficial understanding of its applications in medicine [ 37 ]. This gap in knowledge highlights the need for enhanced educational initiatives to provide comprehensive insights into the potential of AI in healthcare delivery and patient outcomes. Concerns about the overreliance (49.2%) on AI and perceived lack of empathy (43.7%) were highlighted by a considerable proportion of students. These concerns underscore the importance of fostering a balanced approach to AI adoption in medical practice and education, ensuring that students are equipped to navigate the ethical challenges associated with AI integration.
Medical curriculum does not address mathematical concepts (to understand algorithms), the fundamentals of AI like data science, or the ethical and legal issues that can come up with the use of AI [ 27 ]. Only 26.8% of participants felt partially or completely competent to give information on AI to patients. Unless physicians have a foundational understanding of AI, or the methods to critically appraise AI, they will be at a loss when called to train medical students on the use of AI tools that assist in medical decision making. Consequently, medical students will be deficient in AI skills. Liaw et al. advocate for Quintuple Competencies for the use of AI in primary health care, one of which is the need to understand how to communicate with patients regarding the why and how of the use of AI tools, privacy and confidentiality questions that patients may raise during patient physician interactions, and understand the emotional, trust or patient satisfaction issues that may arise because of use of AI in health care [ 38 ].
More than half of the participants (51.1%) are unsure of being able to protect professional confidentiality of patients during the use of AI technologies. Direct providers of health care need to be aware of what precautions to take when sharing data with third parties who are not the direct care providers to the patients [ 16 ]. Artificial intelligence algorithms are derived from large data sets from human participants, and they may use data differently at different points in time. In such cases, patients can lose control of information they had consented to share especially where the impact on their privacy have not been adequately addressed [ 39 ]. However much regulations might be made to protect patient confidentiality and privacy of data, they might always fall behind AI advances, which means the human brain has to work consistently to remain ahead of the artificial intelligence it created. Guidelines set forth by reputable organisations such as the European Union’s “Guidelines for Trustworthy AI“ [ 40 ] and the World Health Organization’s “Ethics and Governance of Artificial Intelligence for Health” address critical ethical concerns in AI [ 41 ]. These core principles can be integrated into medical education to cultivate ethical awareness among medical students.
The perceptions of medical students on the possible influences of AI in medicine were evaluated through the questionnaire. The highest agreement was found on the question, whether they thought the use of AI ‘reduces error in medical practice’ (72.3%) while the lowest agreement was on the question AI ‘devalues the medical profession’ (40.3%).Students were mostly in favour of the use of AI in medicine because they felt that it would enable them as physicians to make more accurate decisions (72%) and facilitate patients’ access to healthcare (60.9%). Research by Topol et al. and Sharique et al. have shown that AI technologies can help reduce medical errors by improving data flow patterns and improving diagnostic accuracy [ 39 , 42 ]. The study from Western Australian students mentioned above [ 26 ] showed 74.4% of the participants agreeing that the use of AI would improve practice of medicine in general. It is encouraging to find that medical students in this research showed low agreement when asked if AI would devalue the medical profession and agreed that the use of AI would reduce medical errors caused inadvertently. It should also be noted that some research has shown that the inappropriate use of AI itself can introduce errors in medical practice [ 43 ].
On “disadvantages and risks of AI in medicine”, 69.2% of the students agreed that AI would reduce the humanistic aspect of the medical profession, 54.5% agreed that it can negatively affect the patient-physician relationship, 52.9% were concerned that using AI assisted applications could damage the trust patients placed on physicians, 59.4% agreed that AI would facilitate patient education, and 50.5% agreed that AI would allow the patient to increase their control over their own health. Hadithy et al. (2023) found that students believed AI technology was advantageous for improving overall health by personalising health care through analysing patient information [ 44 ].
Medical education in the 21st century is swiftly transitioning from the conventional approach of observing patients objectively from a distance and holding the belief that compassion is an innate skill to a contemporary paradigm. The new model emphasises the development of competencies such as doctor-patient relationships, communication skills, and professionalism. In modern medicine, AI is being viewed as an additional barrier between a patient and his physician. Machines have many advantages over humans as rightly observed by Wartman especially in view of not being affected by many of the human frailties like fatigue, information overload, inability to retain material beyond a limit etc. [ 24 ]. Scepticism over the use of AI in medical practice often stems from the lack of knowledge in this domain. Medical students, in many studies, opined that classes on artificial intelligence need to be included in syllabus, but only very few medical schools have included these in their medical curricula. Practising with compassion and empathy must be a learnt and cultivated skill along with artificial intelligence. The two should go together, taught in tandem throughout the medical course. Studies such as this have highlighted that students are open to being taught but are deficient in the skills and knowledge. There is a gap here that needs to be addressed. Man, and machine have to work as partners so as to improve the health of the people.
Limitations
Though this research was one of the first conducted in the state of Kerala and covered about 65% of medical students of the institution, which is more than other similar surveys conducted, there are a few limitations that have been identified. As an online survey method using Google Forms was implied for data collection, the voluntary nature of the participation from only those who were interested, might have introduced a self-selection bias and a non-response bias in this research. As this study only includes the responses from the medical students of one institution, it might not have captured a wide variety of responses. Hence the generalizability of the study may be limited. The questionnaire did not delve deep into how AI terms are understood, or how proficient students were with AI and so might have missed more relevant AI terms and concepts that students might be unfamiliar with. Most data collected in this study were quantitative so we might not have captured the depth of the students’ understanding or perceptions about AI. As many of the students had no exposure to computer science or had not attended AI classes, their perceptions might have been influenced by lack of exposure. Thus, the study might not have captured the views of those who had a more informed background on the subject.
Future studies are recommended to replicate and validate the findings in larger and more diverse populations to understand regional variations in knowledge, attitude, and perceptions among medical students. This study tool (questionnaire) was adopted from a parent study by Civaner M M [ 10 ], but the last question on the need for any other topic to be included was not met with enthusiasm.
This exploration into the perceptions of medical students regarding the integration of Artificial Intelligence (AI) into medical education reveals a nuanced landscape. The majority of participants in this study recognize the collaborative potential of AI, viewing it not as a replacement for physicians but as a valuable ally in healthcare. Interestingly, concerns on job displacement coexist with the optimism about improved decision-making and enhanced medical practice. The knowledge deficit in this context can extend an incompetence in communicating AI related information to patients, highlighting the urgent need for a holistic approach to medical education. The findings complement the perceived need of a proactive approach in preparing medical students for a future where AI plays a pivotal role in healthcare, ensuring that they not only embrace technological advancements but also uphold the humanistic values inherent to the practice of medicine.
Data availability
Data is provided as supplementary information files.
Abbreviations
Artificial Intelligence
Indian Institute of Technology
McCarthy J. What is artificial intelligence? [Internet]. Stanford.edu. [cited 2023 Jul 24]. https://www-formal.stanford.edu/jmc/whatisai.pdf .
Turing AM. Computing Machinery and Intelligence. Parsing the Turing Test. Dordrecht: Springer Netherlands; 2009;23–65.
Google Scholar
Rosenblatt F. The Perceptron-a perceiving and recognizing automaton. 1957.
Nobles AL, Leas EC, Caputi TL, Zhu SH, Strathdee SA, Ayers JW. Responses to addiction help-seeking from Alexa, Siri, Google Assistant, Cortana, and Bixby intelligent virtual assistants. NPJ Digit Med. 2020;3:11. https://doi.org/10.1038/s41746-019-0215-9 . PMID: 32025572; PMCID: PMC6989668.
Article Google Scholar
Research [Internet]. Openai.com. [cited 2023 Jul 24]. https://openai.com/research/overview .
Biswal A. Top 18 Artificial Intelligence (AI) applications in 2023 [Internet]. Simplilearn.com. Simplilearn; 2020 [cited 2023 Jul 24]. https://www.simplilearn.com/tutorials/artificial-intelligence-tutorial/artificial-intelligence-applications .
Michalski RS, Carbonell JG, Mitchell TM. Machine learning an artificial intelligence approach (volume I). Morgan Kaufmann; 1984.
Jimma BL. Artificial intelligence in healthcare: A bibliometric analysis. Telematics and Informatics Reports [Internet]. 2023;9(100041):100041. https://www.sciencedirect.com/science/article/pii/S2772503023000014 .
Davenport T, Kalakota R. The potential for artificial intelligence in healthcare. Future Healthc J. 2019;6(2):94.
Civaner MM, Uncu Y, Bulut F, Chalil EG, Tatli A. Artificial intelligence in medical education: a cross-sectional needs assessment. BMC Med Educ. 2022;22(1):772.
Gulshan V, Peng L, Coram M, Stumpe MC, Wu D, Narayanaswamy A et al. Development and validation of a deep learning algorithm for detection of diabetic retinopathy in retinal fundus photographs. JAMA [Internet]. 2016 [cited 2023 Jul 24];316(22):2402–10. https://pubmed.ncbi.nlm.nih.gov/27898976/ .
Esteva A, Kuprel B, Novoa RA, Ko J, Swetter SM, Blau HM et al. Dermatologist-level classification of skin cancer with deep neural networks. Nature [Internet]. 2017 [cited 2023 Jul 24];542(7639):115–8. https://www.nature.com/articles/nature21056 .
Ehteshami Bejnordi B, Veta M, van Johannes P, van Ginneken B, Karssemeijer N, Litjens G et al. Diagnostic assessment of deep learning algorithms for detection of lymph node metastases in women with breast cancer. JAMA [Internet]. 2017 [cited 2023 Jul 24];318(22):2199–210. https://doi.org/10.1001/jama.2017.14585 .
Yamashita R, Nishio M, Do RKG, Togashi K. Convolutional neural networks: an overview and application in radiology. Insights Imaging [Internet]. 2018;9(4):611–29. https://doi.org/10.1007/s13244-018-0639-9 .
Kreps GL, Neuhauser L. Artificial intelligence and immediacy: designing health communication to personally engage consumers and providers. Patient Educ Couns [Internet]. 2013;92(2):205–10. Available from:. https://www.sciencedirect.com/science/article/pii/S0738399113001729 .
Bali J, Garg R, Bali RT. Artificial intelligence (AI) in healthcare and biomedical research: Why a strong computational/AI bioethics framework is required? Indian J Ophthalmol [Internet]. 2019 [cited 2023 Jul 24];67(1):3–6. https://doi.org/10.4103/ijo.IJO_1292_18 .
Guo Y, Hao Z, Zhao S, Gong J, Yang F. Artificial intelligence in health care: Bibliometric analysis. J Med Internet Res [Internet]. 2020 [cited 2023 Jul 24];22(7):e18228. https://www.jmir.org/2020/7/e18228/ .
Becker A. Artificial intelligence in medicine: What is it doing for us today? Health Policy Technol [Internet]. 2019;8(2):198–205. https://www.sciencedirect.com/science/article/pii/S2211883718301758 .
WMA - The World Medical Association-WMA Statement on Augmented Intelligence. in Medical Care [Internet]. [cited 2023 Jul 17]. https://www.wma.net/policies-post/wma-statement-on-augmented-intelligence-in-medical-care/ .
Bisdas S, Topriceanu CC, Zakrzewska Z, Irimia AV, Shakallis L, Subhash J et al. Artificial Intelligence in Medicine: A Multinational Multi-Center Survey on the Medical and Dental Students’ Perception. Front Public Health [Internet]. 2021 [cited 2023 Jul 10];9. https://www.ncbi.nlm.nih.gov/pmc/articles/PMC8739771/ .
Grunhut J, Wyatt AT, Marques O. Educating Future Physicians in Artificial Intelligence (AI): an integrative review and proposed changes. J Med Educ Curric Dev. 2021;8:23821205211036836.
Artificial intelligence in medical applications and ethical problems: knowledge and thoughts of medical students Survey form used in: Civaner MM, Uncu Y, Bulut F, Chalil EG, Tatlı A. Artificial intelligence in medical education: a cross-sectional needs assessment. BMC Medical Education. 2022;22(1):772. [email protected].
Pinto Dos Santos D, Giese D, Brodehl S, Chon SH, Staab W, Kleinert R, Maintz D, Baeßler B. Medical students’ attitude towards artificial intelligence: a multicentre survey. Eur Radiol. 2019;29(4):1640–6. https://doi.org/10.1007/s00330-018-5601-1 . Epub 2018 Jul 6. PMID: 29980928.
Wartman SA, Combs CD. Medical Education Must Move From the Information Age to the Age of Artificial Intelligence. Acad Med. 2018;93(8):1107–1109. https://doi.org/10.1097/ACM.0000000000002044 . PMID: 29095704.
Wartman SA, Medicine, Machines. and Medical Education. Acad Med. 2021;96(7):947–950. https://doi.org/10.1097/ACM.0000000000004113 . PMID: 33788788.
Stewart J, Lu J, Gahungu N, Goudie A, Fegan PG, Bennamoun M, et al. Western Australian medical students’ attitudes towards artificial intelligence in healthcare. PLoS ONE. 2023;18(8):e0290642. https://doi.org/10.1371/journal.pone.0290642 .
Fabien Lareyre Cédric, Adam M, Carrier N, Chakfé. Juliette Raffort.Artificial Intelligence for Education of Vascular Surgeons.European Journal of Vascular and Endovascular Surgery,Volume 59, Issue 6,2020,Pages 870–871, https://doi.org/10.1016/j.ejvs.2020.02.030 .(https://www.sciencedirect.com/science/article/pii/S1078588420301659 ).
Paranjape K, Schinkel M, Nannan Panday R, CAr J, Nanayakkara P. Introducing artificial intelligence training in medical education. JMIR Med Educ. 2019;5:e16048.
Srivastava TK, Waghmere L, Mar. Vol. -14(3): JI01–2.
Park SH, Do KH, Kim S, Park JH, Lim YS. What should medical students know about artificial intelligence in medicine? J Educ Eval Health Prof. 2019;16:18. https://doi.org/10.3352/jeehp.2019.16.18 . Epub 2019 Jul 3. PMID: 31319450; PMCID: PMC6639123.
Hosny A, Parmar C, Quackenbush J, Schwartz LH, Aerts HJWL. Artificial intelligence in radiology. Nat Rev Cancer. 2018;18(8):500.
Sit C, Srinivasan R, Amlani A, Muthuswamy K, Azam A, Monzon L et al. Attitudes and perceptions of UK medical students towards artificial intelligence and radiology: a multicentre survey. Insights Imaging [Internet]. 2020 Dec [cited 2023 Jul 10];11. https://www.ncbi.nlm.nih.gov/pmc/articles/PMC7002761/ .
Gong B, Nugent JP, Guest W, Parker W, Chang PJ, Khosa F, Nicolaou S. Influence of Artificial Intelligence on Canadian Medical Students’ Preference for Radiology Specialty: ANational Survey Study. Acad Radiol. 2019;26(4):566–577. https://doi.org/10.1016/j.acra.2018.10.007 . Epub 2018 Nov 11. PMID: 30424998.
Yurdaisik I, Aksoy SH. Evaluation of knowledge and attitudes of radiology department workers about artificial intelligence. Ann Clin Anal Med. 2021;12:186–90. https://doi.org/10.4328/ACAM.20453 .
Augmented Intelligence in Health Care. AMA AI, Board Report. 2018. https://www.ama-assn.org/system/files/2019-08/ai-2018-board-report.pdf .
Jha N, Shankar PR, Al-Betar MA, Mukhia R, Hada K, Palaian S. Undergraduate medical students’ and interns’ knowledge and perception of artificial intelligence in medicine. Adv Med Educ Pract [Internet]. 2022 [cited 2024 Mar 25];13:927–37. https://doi.org/10.2147/amep.s368519 .
Vipul Sharma U, Saini V, Pareek L, Sharma. Susheel Kumar. Artificial Intelligence (AI) integration in Medical Education: a Pan-india Cross-sectional Observation of Acceptance and understanding among students. Scripta Med. 2023;55:343–52.
Liaw W, Kueper JK, Lin S, Bazemore A, Kakadiaris I. Competencies for the Use of Artificial Intelligence in Primary Care. Ann Fam Med. 2022 Nov-Dec;20(6):559–63. https://doi.org/10.1370/afm.2887 . PMID: 36443071; PMCID: PMC9705044.
Murdoch B. Privacy and artificial intelligence: challenges for protecting health information in a new era. BMC Med Ethics. 2021;22:122. https://doi.org/10.1186/s12910-021-00687-3 .
Cannarsa M. Ethics guidelines for trustworthy AI. The Cambridge Handbook of lawyering in the Digital Age. Cambridge University Press; 2021. pp. 283–97.
Ethics and governance of artificial intelligence for health [Internet]. Who.int. World Health Organization; 2021 [cited 2024 Mar 25]. https://www.who.int/publications/i/item/9789240029200 .
Sharique Ahmad & Saeeda Wasim. Prevent medical errors through Artificial Intelligence: a review. Saudi J Med Pharm Sci. 2023;9(7):419–23.
Choudhury A, Asan O. Role of Artificial Intelligence in Patient Safety outcomes: systematic literature review. JMIR Med Inf. 2020;8(7):e18599. https://doi.org/10.2196/18599 . PMID: 32706688; PMCID: PMC7414411.
Al Hadithy ZA, Al Lawati A, Al-Zadjali R, Al Sinawi H. Knowledge, attitudes, and perceptions of Artificial Intelligence in Healthcare among Medical students at Sultan Qaboos University. Cureus. 2023;15(9):e44887. https://doi.org/10.7759/cureus.44887 . PMID: 37814766; PMCID: PMC10560391.
Download references
Acknowledgements
Dr. Civaner and team - for providing us with the questionnaire that we used for this research and being available to answer any questions we had.Dr. Rosin George Varghese and Dr. Joe Abraham- for reviewing the protocol of the research and suggesting corrections. Dr. Felix Johns- the Head of the department of Community Medicine for his support during the project.
There has been no funding provided for this research or publication so far.
Author information
Authors and affiliations.
Pushpagiri Medical College, Tiruvalla, Kerala, India
Preetha Jackson, Gayathri Ponath Sukumaran, Chikku Babu, M. Christa Tony, Deen Stephano Jack, V. R. Reshma, Dency Davis, Nisha Kurian & Anjum John
You can also search for this author in PubMed Google Scholar
Contributions
P.J. - Design, acquisition, analysis, interpretation of data, drafting of the work and revisions.G.P.S.- Acquisition, analysis, interpretation of data, substantially revising the work.D.D- Acquisition, interpretation of data, substantially revising the work. C.B., C.T.M, & D.S.J.- acquisition, and reading through manuscript during final revision.R.V.R- Analysis, Interpretation of data, reading through manuscript and substantial revisions.N.K- Substantially revising the work.A.J- Conception, design, acquisition, drafting of the work and substantially contributing to revisions. All authors reviewed the final manuscript.
Corresponding author
Correspondence to Anjum John .
Ethics declarations
Ethics approval and consent to participate.
Ethical approval of the study was obtained from the Institutional Ethics Committee of Pushpagiri Institute of Medical Sciences and Research Centre (Dated: 29 June 2023 No. PIMSRC /E1/388A/72/2023).
Informed consent
Informed consent to participate was obtained from each participant through Google Forms after information about the study was provided.
Consent for publication
Not applicable.
Competing interests
The authors declare no competing interests.
Additional information
Publisher’s note.
Springer Nature remains neutral with regard to jurisdictional claims in published maps and institutional affiliations.
Electronic supplementary material
Below is the link to the electronic supplementary material.
Supplementary Material 1
Supplementary material 2, rights and permissions.
Open Access This article is licensed under a Creative Commons Attribution 4.0 International License, which permits use, sharing, adaptation, distribution and reproduction in any medium or format, as long as you give appropriate credit to the original author(s) and the source, provide a link to the Creative Commons licence, and indicate if changes were made. The images or other third party material in this article are included in the article’s Creative Commons licence, unless indicated otherwise in a credit line to the material. If material is not included in the article’s Creative Commons licence and your intended use is not permitted by statutory regulation or exceeds the permitted use, you will need to obtain permission directly from the copyright holder. To view a copy of this licence, visit http://creativecommons.org/licenses/by/4.0/ . The Creative Commons Public Domain Dedication waiver ( http://creativecommons.org/publicdomain/zero/1.0/ ) applies to the data made available in this article, unless otherwise stated in a credit line to the data.
Reprints and permissions
About this article
Cite this article.
Jackson, P., Ponath Sukumaran, G., Babu, C. et al. Artificial intelligence in medical education - perception among medical students . BMC Med Educ 24 , 804 (2024). https://doi.org/10.1186/s12909-024-05760-0
Download citation
Received : 04 January 2024
Accepted : 09 July 2024
Published : 27 July 2024
DOI : https://doi.org/10.1186/s12909-024-05760-0
Share this article
Anyone you share the following link with will be able to read this content:
Sorry, a shareable link is not currently available for this article.
Provided by the Springer Nature SharedIt content-sharing initiative
- Artificial intelligence
- Medical curriculum
- Medical ethics
- Medical education
BMC Medical Education
ISSN: 1472-6920
- Submission enquiries: [email protected]
- General enquiries: [email protected]
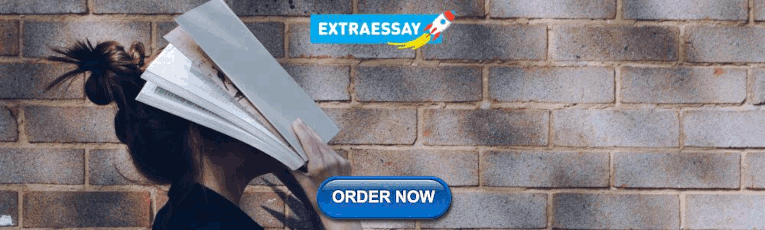
IMAGES
VIDEO
COMMENTS
2.1 Prolific countries. Artificial intelligence in education (AIEd) research has been conducted in many countries around the world. The 40 articles reported AIEd research studies in 16 countries (See Table 1).USA was so far the most prolific, with nine articles meeting all criteria applied in this study, and noticeably seven of them were conducted in K-12.
1. Introduction. Information technologies, particularly artificial intelligence (AI), are revolutionizing modern education. AI algorithms and educational robots are now integral to learning management and training systems, providing support for a wide array of teaching and learning activities (Costa et al., 2017, García et al., 2007).Numerous applications of AI in education (AIED) have emerged.
The use of Artificial Intelligence (AI) in education is transforming various dimensions of the education system, such as instructional practices, assessment strategies, and administrative processes. It also plays an active role in the progression of science education. This systematic review attempts to render an inherent understanding of the evidence-based interaction between AI and science ...
This systematic review provides unique findings with an up-to-date examination of artificial intelligence (AI) in higher education (HE) from 2016 to 2022. Using PRISMA principles and protocol, 138 articles were identified for a full examination. Using a priori, and grounded coding, the data from the 138 articles were extracted, analyzed, and coded. The findings of this study show that in 2021 ...
Artificial Intelligence (AI) has the potential to address some of the biggest challenges in education today, innovate teaching and learning practices, and accelerate progress towards SDG 4. However, rapid technological developments inevitably bring multiple risks and challenges, which have so far outpaced policy debates and regulatory frameworks.
The study shows that AI research in HE has centered around four themes - data as the catalyst of the digital transformation, the development of artificial intelligence, the implementation of artificial intelligence in higher education and the trends, work, and future of artificial intelligence in higher education.
In the past few decades, technology has completely transformed the world around us. Indeed, experts believe that the next big digital transformation in how we live, communicate, work, trade and learn will be driven by Artificial Intelligence (AI) [83]. This paper presents a high-level industrial and academic overview of AI in Education (AIEd). It presents the focus of latest research in AIEd ...
Applications of artificial intelligence in education (AIEd) are emerging and are new to researchers and practitioners alike. ... Impact of an artificial intelligence research frame on the perceived credibility of educational research evidence. International Journal of Artificial Intelligence in Education, 30 (2) (2020), pp. 205-235, 10.1007 ...
In this article, we discuss the role of generative artificial intelligence (AI) in education. The integration of AI in education has sparked a paradigm shift in teaching and learning, presenting both unparalleled opportunities and complex challenges. This paper explores critical aspects of implementing AI in education to advance educational goals, ethical considerations in scientific ...
The 2023 AI Index Report from the Stanford Institute for Human-Centered AI has documented notable acceleration of investment in AI as well as an increase of research on ethics, including issues of fairness and transparency.2 Of course, research on topics like ethics is increasing because problems are observed.
Gwo-Jen Hwang and Nian-Shing Chen, "Exploring the Potential of Generative Artificial Intelligence in Education: Applications, Challenges, and Future Research Directions," Educational Technology & Society 26, no. 2 (2023). Gwo-Jen Hwang and Nian-Shing Chen are enthusiastic about the potential benefits of incorporating generative AI into ...
The purpose of this study was to assess the impact of Artificial Intelligence (AI) on education. Premised on a narrative and framework for assessing AI identified from a preliminary analysis, the scope of the study was limited to the application and effects of AI in administration, instruction, and learning. A qualitative research approach, leveraging the use of literature review as a research ...
This is the Harvard EdCast. Chris Dede thinks we need to get smarter about using artificial intelligence and education. He has spent decades exploring emerging learning technologies as a Harvard researcher. The recent explosion of generative AI, like ChatGPT, has been met with mixed reactions in education.
Artificial intelligence (AI) applications in education are on the rise and have received a lot of attention in the last couple of years. AI and adaptive learning technologies are prominently featured as important developments in educational technology in the 2018 Horizon report (Educause, 2018), with a time to adoption of 2 or 3 years.According to the report, experts anticipate AI in education ...
Artificial Intelligence and the Future of Teaching and Learning. The U.S. Department of Education Office of Educational Technology's new policy report, Artificial Intelligence and the Future of Teaching and Learning: Insights and Recommendations, addresses the clear need for sharing knowledge, engaging educators, and refining technology plans and policies for artificial intelligence (AI) use ...
Today, the U.S. Department of Education's Office of Educational Technology (OET) released a new report, "Artificial Intelligence (AI) and the Future of Teaching and Learning: Insights and Recommendations" that summarizes the opportunities and risks for AI in teaching, learning, research, and assessment based on public input. This report is part of the Biden-Harris Administration's ongoing ...
When the Stanford Accelerator for Learning and the Stanford Institute for Human-Centered AI began planning the inaugural AI+Education Summit last year, the public furor around AI had not reached its current level. This was the time before ChatGPT. Even so, intensive research was already underway across Stanford University to understand the vast potential of AI, including generative AI, to ...
The abrupt emergence and rapid advancement of generative artificial intelligence (AI) technologies, transitioning from research labs to potentially all aspects of social life, has brought a profound impact on education, science, arts, journalism, and every facet of human life and communication. The purpose of this paper is to recapitulate the use of AI in education and examine potential ...
Submit proposals for research, implementation, and education projects involving multi-sector teams that focus on the responsible design, development, or deployment of technologies, including AI. ... Artificial Intelligence and the Future of Teaching and Learning. The Department of Education (ED) has released a report to guide educators in ...
Abstract: Artificial intelligence is the driving force of change focusing on the needs and demands of the student. The research explores Artificial Intelligence in Education (AIEd) for building personalised learning systems for students. The research investigates and proposes a framework for AIEd: social networking sites and chatbots, expert ...
1. Introduction. With the development of computing and information processing techniques, artificial intelligence (AI) has been widely applied in educational practices (Artificial Intelligence in Education; AIEd), such as intelligent tutoring systems, teaching robots, learning analytics dashboards, adaptive learning systems, human-computer interactions, etc. (Chen, Xie, & Hwang, 2020).
Abstract. Artificial intelligence (AI) is a field of study that combines the applications of machine learning, algorithm productions, and natural language processing. Applications of AI transform the tools of education. AI has a variety of educational applications, such as personalized learning platforms to promote students' learning ...
1. Personalize Education. Artificial Intelligence helps find out what a student does and does not know, building a personalized study schedule for each learner considering the knowledge gaps. In such a way, AI tailors studies according to student's specific needs, increasing their efficiency.
Artificial intelligence has captured the world's imagination, generating countless headlines and causing heated debates. These discussions are very live among those working in education - especially in English language teaching. What impact will AI have on how our learners gain knowledge and develop skills? What impact will it have on how ...
This guide explains the focus, rigor, and relevance of qualitative research, highlighting its role in dissecting complex social phenomena and providing in-depth, human-centered insights. ... Empowering qualitative research methods in education with artificial intelligence [Conference session]. World Conference on Qualitative Research (pp. 1 ...
Chengliang Wang is a Postgraduate in Department of Education Information Technology, Faculty of Education, East China Normal University. His research interests include social media learning, educational technology philosophy, human-computer interaction in education and artificial intelligence in education (AIED).
There is not a single, accepted explanation for artificial intelligence (AI). The phrase refers to computational techniques that are like mental functions such as thinking, comprehension, adjustment, sensory perception and collaboration but never fully replaces humans1. The most profitable branch of AI over the past decade has been the field of machine learning2
With the rapid advance of Artificial Intelligence (AI), higher education institutions must engage in thoughtful discussion about AI's impact on teaching, learning, curriculum, research, careers, and the broader societal implications. We have developed this page of resources, organized by themes, to keep our faculty and staff informed about AI.
Unfortunately, the same cannot be said about education. The U.S. has never adequately invested in R&D related to education, so persistent problems remain unsolved and the system is largely unable to handle unexpected emergencies, like COVID. Although strong research does exist, few education leaders use it to guide their decisions on behalf of ...
As Artificial Intelligence (AI) becomes pervasive in healthcare, including applications like robotic surgery and image analysis, the World Medical Association emphasises integrating AI education into medical curricula. This study evaluates medical students' perceptions of 'AI in medicine', their preferences for AI training in education, and their grasp of AI's ethical implications in ...