American University Catalog 2024-2025 | | | Offered by the School of International Service , the International Economic Relations: Quantitative Methods (MA) offers advanced training in econometrics and quantitative methods and gives students an in-depth understanding of the economic, political, and social forces that shape relations among countries and their governments within the global economy, as well as of the private and official actors and institutions that shape international trade and financial developments and policies. Students learn the foundational debates and concepts in international trade and financial relations, as well as international political economy, global economic governance, and newly emergent issue areas. Students apply their training in econometrics and quantitative research methods to real-world policy problems in trade, finance, and other areas of international economic relations. Admission to the ProgramApplicants must hold an earned bachelor’s degree from an institution accredited by one of the six United States regional accreditation agencies or a degree equivalent to a four-year U.S. bachelor’s degree from an international institution with a similar level of accreditation or recognition by its home country. Assessment of a foreign degree will be based upon the characteristics of the national system of education, the type of institution attended, its accreditation, and the level of studies completed. Competitive candidates may demonstrate their potential to excel in diverse ways, including a strong academic record, demonstrated success in relevant coursework and/or professional experience, the presentation of a compelling motivation and objective for graduate work, or a combination of some of these factors. The application deadline for fall admission is January 15; for spring admission October 1 (September 15 for international students). Admitted students may defer matriculation for no longer than two semesters provided that a written request for deferment is submitted to and approved by the SIS Graduate Admissions Office. A Graduate Record Exam (GRE) is optional. Applicants who have completed the exam are welcome to submit their scores as part of their application. Non-native English speakers are required to submit results of the Test of English as a Foreign Language (TOEFL) or the International English Language Testing System (IELTS) unless they hold a degree from a U.S.-accredited institution before enrollment at SIS. The minimum TOEFL score for full admissions consideration is 100 on the Internet-based test (iBT) or 600 on the paper-based test (PBT). The minimum IELTS score is 7.0. LSAT scores will be accepted in place of the GRE for JD/MA applicants. Fall applicants should plan to take the appropriate test no later than December to ensure full consideration of the application by the January deadline. All applicants must submit two letters of reference evaluating undergraduate academic performance and suitability for graduate study in international affairs, at least one of which should be from an academic source. A resume should be included in the application. Applicants must submit transcripts from all institutions attended. Cultural factors are considered in evaluating transcripts and examination results. Requests for the transfer of a maximum of 6 graduate credit hours from other accredited institutions to be applied to a master’s degree must be completed in the student’s first semester in the graduate program at American University. A minimum grade of B in each course is required for transfer. Transfer courses must have been completed within five years of admission and must fulfill stated requirements of the degree program. Degree Requirements- 36 credit hours of approved graduate work
- Minimum 3.00 cumulative GPA required to remain in good academic standing and to earn the degree
- Practicum: 3 credit hours with grade of B or better
- Substantial Research Paper: 3 credit hours with grade of B or better
- Thesis: 6 credit hours of thesis credit and submission of the thesis
- Evidence of professional experience in the field: Relevant internship or work experience must be certified
- Proficiency in a modern foreign language: Research competence in English and another language relevant to the student’s career objectives must be certified
Course RequirementsCore (12 credit hours). - SIS-616 International Economics (3)
- SIS-665 International Trade Relations (3)
- SIS-666 International Financial Relations (3)
- SIS-751 International Political Economy (3)
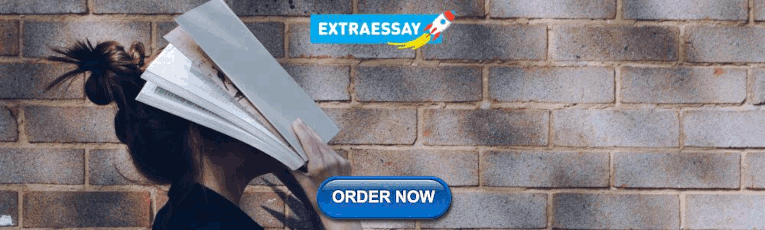 Quantitative Research Methods (9 credit hours)Complete one of the following options: Option I: Standard Track- ECON-623 Applied Econometrics I (3)
- ECON-624 Applied Econometrics II (3)
- SIS-600 Statistics, Research Design, and Research Methods for International Affairs (3)
Option II: Accelerated Track- 3 credit hours from the Approved International Economic Relations (IER) Third Methods Course List
Advanced Econometrics and Quantitative Methods Concentration (9 credit hours)Complete 9 credit hours from the following: - DATA-612 Statistical Programming in R (3)
- DATA-613 Data Science (3)
- ECON-665 Economic Experiments and Impact Evaluation (3)
- ENVS-654 Geographic Information Systems (3)
- ITEC-600 Programming Tools for Analytics: Python (3)
- ITEC-610 Applied Managerial Statistics (3)
- ITEC-670 Database and Big Data (3)
- SIS-750 Research and Professional Methods in International Affairs (3) (approved quantitative topics)
- STAT-520 Applied Multivariate Analysis (3)
- STAT-522 Time-Series Analysis (3)
- STAT-627 Statistical Machine Learning (3)
- STAT-628 Spatial Data Analysis (3)
Capstone (3 credit hours)Complete one of the following: Note: The thesis or substantial research paper must relate clearly to the field of international economic relations. Complete 3 credit hours from the following with grade of B or better: - SIS-793 Practicum in International Affairs (3) (topics)
Substantial Research PaperComplete 3 credit hours from one of the following with grade of B or better: - SIS-794 Substantial Research Paper with Coursework (3)
- SIS-795 Master’s Research Requirement (1-3)
- SIS-797 Master’s Thesis Supervision (1-6) (6 credit hours required; may include 3 elective credit hours)
Elective (3 credit hours)Complete 3 credit hours from the following, or other approved courses: - ECON-603 Introduction to Economic Theory (3)
- SIS-691 Internship in International Affairs (1-6)
- SIS-692 International Internship (1-6)
- SIS-730 Skills Institutes in International Affairs (1) (topics)
- SIS-797 Master’s Thesis Supervision (1-6)
Annual Bank Conference on Development Economics 2024—The Great Incoherence: Growth and Human Development in An Era of Stagnation- Google Calendar
- Yahoo! Calendar
- iCal Calendar
- Outlook Calendar
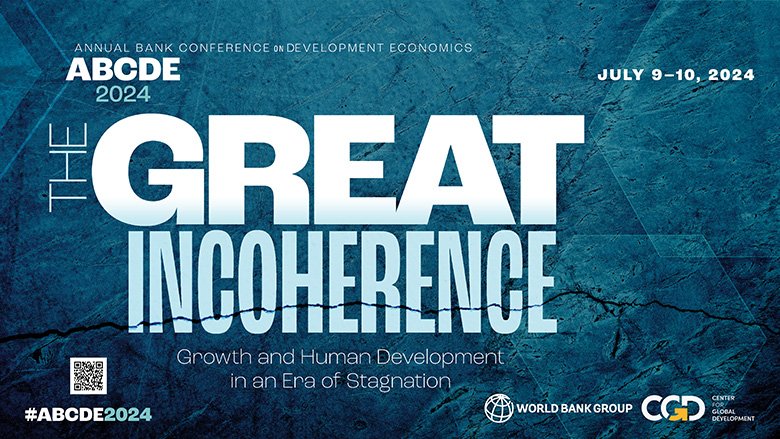 ** Please note that there is no open call for paper submissions for this year’s ABCDE conference **- KEYNOTE SPEAKER
- PROGRAM COMMITTEE
The World Bank Group’s Annual Conference on Development Economics —ABCDE—is a 35-year-old forum to stimulate an exchange of ideas between leaders in global policy discussions and researchers, policymakers, and development practitioners from the Bank’s member countries. Established in 1989 and organized by the World Bank’s Development Economics Vice Presidency (DEC), ABCDE became the premier venue for cutting-edge insights on how to tackle the most pressing challenges of development. Several younger researchers presenting papers went on to become Nobel Prize winners. The conference also played a role in shaping the global consensus on development policy. Beginning in 2024, ABCDE will be co-sponsored by partnership that rotates every two years. For the 2024 conference, the Center for Global Development will be the World Bank Group’s partner. A growing incoherence is clouding global debates over how to achieve the key development goals of the 2020s—a profound mismatch exists between international policy advocacy and the research-based solutions necessary to achieve the desired development outcomes. Policy advocates regularly remind us that “trillions” of dollars must be mobilized to tackle climate change, improve health and education, and rebuild war-torn countries. Yet amid decelerating economic growth and private investment, record public debt levels, and a growing tendency in many countries to prioritize national security over multilateral cooperation, there is precious little clarity on where the resources will come from. It’s time to reconcile ends and means. On July 9 and 10, the World Bank and the Center for Global Development will co-host the Annual Bank Conference on Development Economics (ABCDE). On the first day, the conference will aim to bring coherence to development policy debates by bringing together the world’s top economic minds to focus on four interrelated questions: 1) how can climate finance be increased, without deprioritizing other key development issues? 2) How should the world respond to the global debt crisis and promote future economic growth? 3) What are the implications of recent shifts in industrial policy for the future growth pathways of the Global South, and how can countries adapt? 4) how can private capital be mobilized for these issues and others? More inclusive and sustainable economic growth can help solve many of these challenges. The second day will focus on how to achieve this type of growth—specifically by overcoming the constraints that women and youth face in the labor market in low-and-middle-income-countries. The day will feature panel discussions on social safety nets and women’s labor-force participation; norms, and other constraints to the economic inclusion of women and young people. All times listed below are in Eastern Standard Time (EST) | 08:00 – 09:00 | | 09:00 – 09:05 | MODERATOR: , Editor-in-Chief, Central Bank Central | 09:05 | , World Bank President | 09:15 – 09:55 | former US Treasury Secretary DISCUSSANT: , Dean, Lee Kuan Yew School of Public Policy | 09:55 – 10:35 | SPEAKERS , Chief Economist of the World Bank Group and Senior Vice President for Development Economics (World Bank) , President of the Center for Global Development (CGD)DISCUSSANT , Dean, Lee Kuan Yew School of Public Policy MODERATOR: , Editor-in-Chief, Central Bank Central | 10:35 – 10:50 | Coffee Break | 10:50 – 12:05 | Is There Still a Role for Direct Government Support to Firms in Developing Countries? ( , World Bank) The Political Economy of Reviving Industrial Policy in Africa ( , University of Manchester) Industrial Policy for Developing Countries: Is There a Way to Pick Winners? ( , World Bank)DISCUSSANTS (World Bank); (World Bank) MODERATOR: (World Bank) | 12:05 – 13:15 | Lunch Break | 13:15 – 14:30 | The Financial Returns on China’s Belt and Road ( , Kiel University) Sovereign Defaults at Home and Abroad ( , Federal Reserve Board) Why do Some Countries Default More Often Than Others? The Role of Institutions ( , World Bank)DISCUSSANTS: (IMF); (World Bank) MODERATOR: (World Bank) | 14:30 – 14:45 | Coffee Break | 14:45 – 16:00 | Mobilizing Private Capital for Sustainable Development Goals ( , World Bank) Carbon Prices and Forest preservation Over Space and Time in the Brazilian Amazon ( , Columbia University) Climate Regulatory Risks and Corporate Bonds ( , Federal Reserve Bank of New York) Too-Big-to-Strand? Bond versus Bank Financing in the Transition to a Low-Carbon Economy ( , Audencia Business School, University of Ioannina)DISCUSSANTS: (University of Chile) and (World Bank) (TBC) MODERATOR: (President, Bezos Earth Fund) | 16:00 – 16:15 | Coffee Break | 16-15 – 16:45 | , University of Chicago | 16-45 – 18:00 | 7 Lessons from the Pandemic for Low and Middle-Income Countries ( , Yale University) Adjusting to Transitory Shocks: Worker Impact, Firm Channels, and (Lack of) Income Support ( , World Bank) How Much Learning was Lost during the Pandemic, and How Much has Been Made Up? ( , World Bank)MODERATOR: (Inter-American Development Bank) |
| 08:15 – 08:30 | , CGD (Incoming CGD President)
| 08:30 – 10:30 | SPEAKERS , Ashoka University. (Paris School of Economics) (NYU) MODERATOR: , World Bank | 10:30 – 11:00 | Coffee Break | 11:00 – 12:30 | Women’s Empowerment and the Efficacy of Social Safety Nets ( , University of Hawaii at Manoa) Shalini Roy, IFPRI Impacts of Safety Nets on Adolescent Girls’ Economic Inclusion ( , CGD) Cash Transfers and Women’s Economic Empowerment ( , CGD) MODERATOR , IDB | 12:30 – 14:15 | Lunch Break | 12:45 – 14:00 | CHAIR: (World Bank) Breaking the Mold: India’s Untraveled Path to Prosperity ( University of Chicago) The lunchtime keynote address will take place in the F L-101 at the IFC building. | 14:15 – 15:45 | Parental Leave Policies ( , Williams College) Social Norms and Networks ( , World Bank) Physical Safety and Workplace Harassment ( , Northeastern University) Marriage and the Female Labor Force Participation ( , University of Washington)
MODERATOR: (World Bank) | 15:45 – 16:00 | Coffee Break | 16:00 – 17:30 | Digitalization , World Bank) Youth Reskilling among Refugee Populations ( , CGD) ( , University of Virginia) ( , Williams College) MODERATOR: (Brookings Institution) | 17:30 | (Brookings Institution) | 18:00 – 19:00 | |
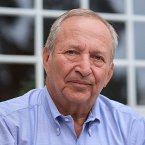 Former Treasury Secretary Lawrence H. Summers is one of America’s leading economists. In addition to serving as 71st Secretary of the Treasury in the Clinton Administration, Dr. Summers served as Director of the White House National Economic Council in the Obama Administration, as President of Harvard University , and as the Chief Economist of the World Bank. Dr. Summers’ tenure at the U.S. Treasury coincided with the longest period of sustained economic growth in U.S. history. He is the only Treasury Secretary in the last half century to have left office with the national budget in surplus. Dr. Summers has played a key role in addressing the major financial crisis for the last three decades. During the 1990s, he was a leader in crafting the U.S. response to international financial crises arising in Mexico, Brazil, Russia, Japan, and Asian emerging markets. As one of President Obama’s chief economic advisors, Dr. Summers’ thinking helped shape the U.S. response to the 2008 financial crisis, to the failure of the automobile industry, and to the pressures on the European monetary system. Upon Summers’ departure from the White House, President Obama said, “I will always be grateful that at a time of great peril for our country, a man of Larry’s brilliance, experience and judgment was willing to answer the call and lead our economic team.” The Economist recognized his influence when it defined the “Summers Doctrine,” an approach to economic policy during financial crises that fuses a microeconomic “laissez faire” mentality with macroeconomic activism. “Markets should allocate capital, labour and ideas without interference, but sometimes markets go haywire, and must be counteracted forcefully by government.” Summers’ five years as President of Harvard represented a time of major innovation for the University. He focused on equality of opportunity and removing all financial obligation from students with family incomes below $60,000 a year. He launched a major effort to make Boston, and Cambridge in particular, the global leader in life sciences research, with the formation of major programs for stem cell research and genomics. Perhaps most importantly, he led efforts to renew Harvard College with dramatic increases in study abroad programs, faculty-student contact, and collaboration across the University during his tenure. Currently, Dr. Summers is the President Emeritus and the Charles W. Eliot University Professor at Harvard University, where he became a full professor at age 28, one of the youngest in Harvard’s recent history. He directs the University’s Mossavar-Rahmani Center for Business and Government. Summers was the first social scientist to receive the National Science Foundation’s Alan Waterman Award for scientific achievement and, in 1993, he was awarded the John Bates Clark Medal, given to the most outstanding economist under 40 in the United States. He was elected to the National Academy of Sciences in 2002. He has published more than 150 papers in scholarly journals. 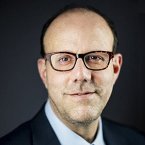 Michael Kremer is the University Professor in the Kenneth C. Griffin Department of Economics. He is the 2019 co-recipient of the Sveriges Riksbank Prize in Economic Sciences in Memory of Alfred Nobel. He is a Member of the National Academy of Sciences, a recipient of a MacArthur Fellowship and a Presidential Faculty Fellowship, and was named a Young Global Leader by the World Economic Forum. Kremer’s recent research examines education, health, water, and agriculture in developing countries. 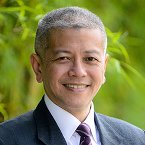 Danny Quah is Li Ka Shing Professor in Economics and Dean at the School. His research interests lie in income inequality, economic growth, and international economic relations. Quah’s work takes an economic approach to world order — focusing on global power shift and the rise of the east, and alternative models of global power relations. The economic approach emerges in that Quah’s work studies the supply and demand of world order: What international system do the world’s superpowers wish to provide; what world order does the global community need? Quah’s work on income inequality sets the challenge against a broader background of social mobility and cohesion, and in so doing suggests a single narrative on the challenge of income inequality is unlikely to be correct or helpful. Quah is Commissioner on the Spence-Stiglitz Commission on Global Economic Transformation ; and serves on the Executive Committee, International Economic Association ; the Executive Committee, Association of Professional Schools of International Affairs APSIA; the Advisory Council, Bennett Institute, Cambridge University; the Academic Advisory Board, Blavatnik School of Government, Oxford University; the Global Advisory Board, Tsinghua University School of Public Policy and Management; the Advisory Board, LSE IDEAS; the Advisory Council, OMFIF; the Eminent Advisory Council, UNDP Asia-Pacific; and the World Economic Forum’s Global Future Council for Geopolitics. He is Vice President, Economic Society of Singapore. Quah gave the third LSE-NUS lecture in 2013, TEDx talks in 2016 , 2014 , and 2012 , and the Inaugural LSE Big Questions Lecture in 2011. Quah’s research has been supported by the Khazanah Research Institute, the John D. and Catherine T. MacArthur Foundation, the British Academy, the UK’s Economic and Research Council, and the Andrew Mellon Foundation. Quah was previously Assistant Professor of Economics at MIT, and then at LSE Professor of Economics and International Development, and Director of the Saw Swee Hock Southeast Asia Centre. He served as LSE’s Head of Department for Economics, and Council Member on Malaysia’s National Economic Advisory Council. Quah studied at Princeton, Minnesota, and Harvard. Tuesday, July 9, 2024Plenary panel: the great incoherence. 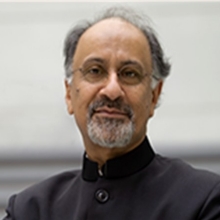 Indermit Gill is Chief Economist of the World Bank Group and Senior Vice President for Development Economics. He brings to the role a broad combination of leadership, expertise, and practical experience working with governments on macroeconomic imbalances, growth, poverty, institutions, conflict, and climate change. Before starting this position on September 1, 2022, Gill served as the World Bank’s Vice President for Equitable Growth, Finance, and Institutions, where he played a key role in shaping the Bank’s response to the extraordinary series of shocks that have hit developing economies since 2020. Between 2016 and 2021, he was a professor of public policy at Duke University and non-resident senior fellow at the Brookings Institution’s Global Economy and Development program. Gill is widely regarded for his contributions to development economics. He spearheaded the influential 2009 World Development Report on economic geography. His pioneering work includes introducing the concept of the “middle-income trap” to describe how countries stagnate after reaching a certain level of income. He has published extensively on key policy issues facing developing countries—among other things, sovereign debt vulnerabilities, green growth and natural-resource wealth, labor markets, and poverty and inequality. Gill has also taught at Georgetown University and the University of Chicago. He holds a Ph.D. in economics from the University of Chicago  Masood Ahmed is president of the Center for Global Development. He joined the Center in January 2017, capping a 35-year career driving economic development policy initiatives relating to debt, aid effectiveness, trade, and global economic prospects at major international institutions including the IMF, World Bank, and DFID. Ahmed joined CGD from the IMF, where he served for eight years as director, Middle East and Central Asia Department, earning praise from Managing Director Christine Lagarde as a “visionary leader.” In that role, he oversaw the Fund's operations in 32 countries, and managed relationships with key national and regional policy makers and stakeholders. Born and raised in Pakistan, Ahmed moved to London in 1971 to study at the LSE where he obtained a BSc Honors as well as an MSc Econ with distinction. Ahmed is a leading expert on Middle East economics, having served on the Advisory Board of the LSE Middle East Center, as well as on the World Economic Forum's Global Agenda Council on the Middle East and North Africa. 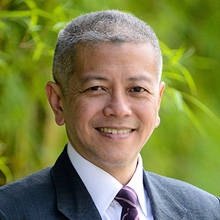 Danny Quah is Li Ka Shing Professor in Economics and Dean at the School. His research interests lie in income inequality, economic growth, and international economic relations. Quah’s work takes an economic approach to world order — focusing on global power shift and the rise of the east, and alternative models of global power relations. The economic approach emerges in that Quah’s work studies the supply and demand of world order: What international system do the world’s superpowers wish to provide; what world order does the global community need? Quah’s work on income inequality sets the challenge against a broader background of social mobility and cohesion, and in so doing suggests a single narrative on the challenge of income inequality is unlikely to be correct or helpful. 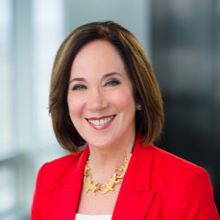 Kathleen Hays is Editor-in-Chief of Central Bank Central covering Federal Reserve policy and players, and central banks around the world that are driving global markets. Recognized as one of the top economics reporters and anchors in the country, Hays has covered the U.S. economy and the Federal Reserve for more than 30 years. For the past two years she has broadened out her coverage to include the economies and central banks of Asia, travelling regularly to Japan. Industrial Policy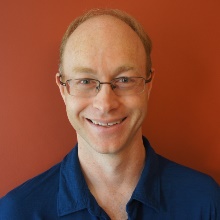 David McKenzie is a Lead Economist in the Development Research Group, Finance and Private Sector Development Unit. He received his B.Com.(Hons)/B.A. from the University of Auckland, New Zealand and his Ph.D. in Economics from Yale University. Prior to joining the World Bank, he spent four years as an assistant professor of Economics at Stanford University. His main research is on migration, enterprise development, and methodology for use with developing country data. He has published more than 150 articles in journals such as the Quarterly Journal of Economics , American Economic Review, Review of Economic Studies, Journal of Political Economy, Science, Review of Economics and Statistics , Journal of the European Economic Association , Economic Journal , American Economic Journal: Applied Micro , Journal of Econometrics , and all leading development journals. He is currently on the editorial boards of the Journal of Development Economics , the World Bank Economic Review , and Migration Studies . He is also a co-founder and regular contributor to the Development Impact blog . 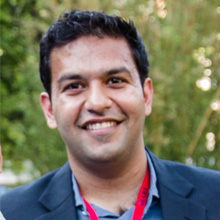 Pritish Behuria is a political economist with a PhD from SOAS, University of London, his work explores the intersection of development studies, comparative politics, and international political economy, focusing on late development challenges in the context of 21st-century globalization. He helds academic positions at SOAS, LSE, and currently at The University of Manchester, where he was promoted to Senior Lecturer in 2022. Pritish research spans the politics of financial integration, state-business relations in Africa, and India's solar energy sector. He has been recognized for his teaching and advising, and he actively contributes to academic groups and journals. Additionally, Pritish consultes for international organizations and his insights have been featured in global media. 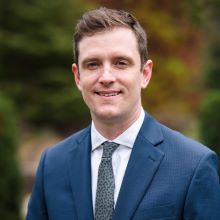 Tristan Reed is an applied economist at the World Bank's Development Research Group . His research documents how economic and political competition shape economic development. Alongside research, he provides advice to World Bank clients on sector development strategy and trade and competition policy. Prior to joining the Bank, Tristan was an associate at McKinsey & Company's Africa office. A native of California, he holds a Ph.D. in economics from Harvard University and a B.A. summa cum laude from UCLA. 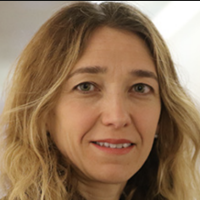 Ana Paula Cusolito is a Senior Economist currently working at the Markets, Competition & Technology Unit of the FCI Global Practice of the World Bank. Her research focuses on firm and aggregate productivity and its determinants, including foreign competition, digital-technology adoption, innovation, and corporate governance. Ana Paula has co-authored several academic papers, WBG flagship reports, and books. Her research has been published in international journals such as American Economic Review: Insights, Review of Economics and Statistics, Journal of Development Economics, World Bank Economic Review, Journal of Economics and Public Finance, IZA Journal of Labor and Development, and Journal of Development Effectiveness among others. She has been invited to present her work and be discussant at several international conferences, including Econometric Society, EEA, LACEA, RES, WEAI among others. Before joining the WBG, Ana Paula worked at the IADB as Country Economist for Costa Rica and the Ministry of Finance of Buenos Aires Province, UNDP program. She holds a Ph.D. in Economics from Universitat Pompeu Fabra, a Master from UCEMA, and a B.A, from Universidad Nacional de La Plata. 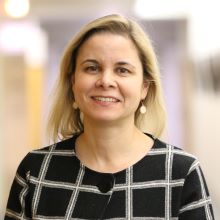 Ana Margarida Fernandes is a Lead Economist in the Trade and International Integration Unit of the Development Research Group at the World Bank. She joined the World Bank as a Young Economist in 2002. Her research examines the consequences of openness to trade and FDI for firm-level productivity, innovation and quality upgrading. Her work has also focused on the impact evaluation of trade-related policy interventions such as export promotion and customs reforms around the globe (Albania, Serbia, Madagascar, Tunisia). Since 2011 she has been managing the Exporter Dynamics Database project and studying the links between exporter growth and dynamics, development, policies, and shocks. She is currently working on deep trade agreements and on corruption in customs and tax evasion. 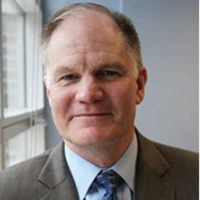 William F. Maloney is Chief Economist for the Latin America and the Caribbean region at the World Bank. Previously he was Chief Economist for Equitable Growth, Finance and Institutions, and Trade and Competitiveness; he was also Global Lead on Innovation and Productivity. Prior to the Bank, he was an assistant professor of Economics at the University of Illinois, Urbana-Champaign (1990-1997) and then Lead Economist in the Office of the Chief Economist for Latin America until 2009. From 2009 to 2014, he was Lead Economist in the Development Economics Research Group. From 2011 to 2014 he was visiting professor at the University of the Andes and worked closely with the Colombian government on innovation and firm upgrading issues. Mr. Maloney received his PhD in economics from the University of California Berkeley (1990), his BA from Harvard University (1981), and he studied at the University of the Andes in Bogota, Colombia (1982–83). Sovereign Debt and Default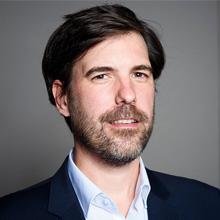 Christoph Trebesch is head of the Research Center International Finance and Macroeconomics at the Kiel Institute since April 2017, as well as Professor of Macroeconomics at Kiel University. Trebesch works at the intersection of international finance and macroeconomics, economic history, and political economy. He did his doctorate at the Free University Berlin and then moved to the University of Munich as assistant professor before joining the Kiel Institute. He has been a consultant and advisor for the IMF, the World Bank, the United Nations, the US Treasury as well as the German Ministry of Finance. His work is regularly cited in leading international media such as the New York Times, The Economist, or the Financial Times. A unifying theme of his work is a focus on rare events, meaning events that occur only every few years or decades, but, when they do occur, they have enormous economic consequences. This motivated his research on financial and debt crises, on the rise of extremist and populist political forces, on China’s financial ascent, and, more recently on geopolitics and great power rivalry. In 2022, he founded a new Research Initiative on Geopolitics and Economics at the Kiel Institute, which he now co-heads with Katrin Kamin, and created the widely covered Ukraine Support Tracker. 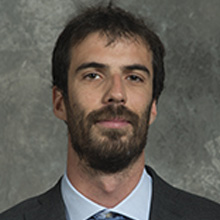 Enrico Mallucci principal economist at the Board of Governors of the Federal Reserve System. His main research interests are: International Economics and International Finance. His research focuses on: Sovereign Defaults and International Capital Flows 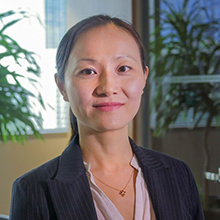 Rong Qian is a Senior Economist at the World Bank in the Philippines. She leads a team that develops programs to help improve the country’s global competitiveness and resilience to natural disasters. She is also the macroeconomics and fiscal management expert behind the Growth and Productivity Report and the Philippines Economic Update, which reviews salient socioeconomic issues and provides growth forecasts twice a year. Prior to joining the Philippine team, Rong was the country economist for Chile and Nicaragua based in Washington, DC. She specializes in fiscal policy, productivity, and growth—including green growth and its careful regard for the environment. Rong joined the bank in September 2011. Earlier in her career at the Bank, Rong was doing research on sovereign defaults and financial crises. She was also an intern at the United States Congressional Budget Office and the International Monetary Fund, while completing her Ph.D. in Economics from the University of Maryland. 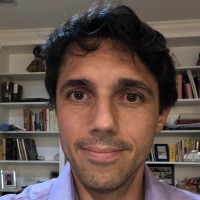 Luca Bandiera is a Lead Economist of the Global Macro and Debt Analytics in the Macroeconomics, Trade and Investment (MTI) Global Practice of the World Bank. He has worked on technical, analytical and operational tasks in low and middle-income countries in Sub-Saharan Africa, Middle East and North Africa, Europe and Central Asia and Latin America. He leads the analytical work on debt sustainability in the World Bank. He has published articles and contributed chapters in books on debt, infrastructure and growth. He holds a Doctorate degree from Catholic University in Milan. 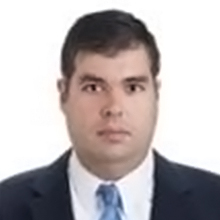 Marcos Chamon is a Deputy Division Chief at the International Monetary Fund. He has worked on a wide range of topics related to international finance, including problems related to sovereign debt structure, and restructuring, liability denomination, indexation of debt to GDP, the international financial architecture, country insurance, methodologies to assess vulnerabilities in emerging markets and advanced economies, consumption and savings in China, currency composition of reserves, the emerging market’s policy responses to the Global Financial Crisis, the design of capital controls and macro prudential policies. Prior to joining the Fund, he obtained a Ph.D. in Economics from Harvard University in 2003. 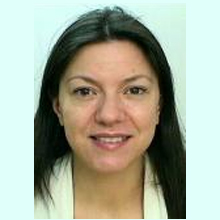 Manuela Francisco is the Global Director for the Macroeconomics, Trade and Investment (MTI) Global Practice in the Equitable Growth, Finance, and Institutions Practice Group (GGEVP), a position she started on 1 January 2023. Ms. Francisco, a Portuguese national, joined the World Bank in 2005 as Country Economist in the Africa Department. She has since held various positions of responsibility in the Bank. Prior to her current position, she was the Director of Credit Risk, in the Chief Risk Officer Vice Presidency, and before that, she was the Practice Manager for MTI in the South Asia Region. Before joining the Bank, Ms. Francisco was an Assistant Professor at Universidade do Minho, Portugal. Ms. Francisco has a PhD in Economics, International Macroeconomics from the University of Nottingham. Private Capital Mobilization for Sustainable Development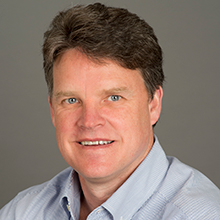 Robert Cull is Research Manager and Lead Economist in the Finance and Private Sector Development Team of the World Bank's Development Research Group. His most recent research is on the performance of microfinance institutions, African financial development, Chinese financial development and firm performance, and the effects of the global financial crisis on foreign banks and on bank regulation and supervision in developing economies. He has published numerous articles in peer-reviewed academic journals including in the Economic Journal , Journal of Development Economics , Journal of Economic Perspectives , Journal of Financial Economics , Journal of Law and Economics , and the Journal of Money, Credit, and Banking . The author or editor of multiple books, his most recent co-edited book, “Banking the World: Empirical Foundations of Financial Inclusion” was published by MIT Press January, 2013. He is also co-editor of the Interest Bearing Notes , a bi-monthly newsletter reporting on financial and private sector research. 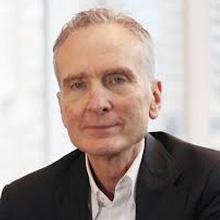 José Scheinkman is currently the Charles and Lynn Zhang Professor of Economics at Columbia University and the Theodore A. Wells '29 Professor of Economics Emeritus at Princeton University . He spent much of his career at the University of Chicago , where he served as department chair immediately prior to his departure for Princeton. He is best known for his work in mathematical economics (particularly dynamic optimization) and finance, oligopoly theory and the social economics of cities and crime; he also helped spur the development of work at the intersection of economics, finance and physics. Scheinkman also famously pioneered the now-ubiquitous application of academic financial theory to practical risk management of fixed incomes during a leave he took as Vice President in the Financial Strategies Group at Goldman, Sachs & Co. during the late 1980s. 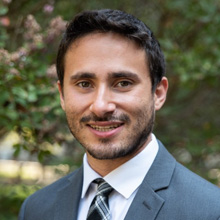 Lee Seltzer is a financial research economist in Climate Risk Studies within the Financial Intermediation Policy Research Division. His research interests include examining corporate finance issues in real estate markets, in particular the effects of firm corporate finance decision-making on rental housing markets. He also studies the implications of climate risk on financial markets. He holds a Ph.D. in Finance from the University of Texas at Austin and a B.A. in Economics and History from Rutgers University. 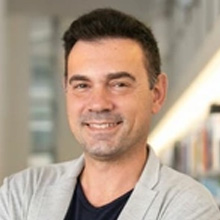 Manthos Delis is a Professor of Financial Economics at Audencia Business School and Member of the Board of Directors at the University of Ioannina. His current research emphasizes the role of sustainable finance (especially bank credit) in modern societies. More than 60 of his papers are published in top-tier journals, with 10 publications in FT-listed journals (Journal of Business Ethics, Journal of Financial Economics, Journal of Financial & Quantitative Analysis, Management Science, Operations Research, Review of Finance), 21 in ABS 4 journals, and many other in top-field journals (European Journal of Operational Research, Journal of Banking & Finance, Journal of Corporate Finance, Journal of Development Economics, Journal of Money, Credit & Banking, etc.). He has been a full professor since the age of 33 and IDEAS ranks his research among the top 1% economics researchers in the world. Among other awards, on March 2019, he was externally nominated for the USERN Prize 2019 and is included in the Stanford science-wide author databases of standardized citation indicators . He is a co-founder and member of the organizing committee of the CEPR Endless Summer Conference on Financial Intermediation and Corporate Finance . He is also a member of the program committee of the Swiss Winter Conference on Financial Intermediation , and a member of the scientific committee of the Hellenic Finance and Accounting Association (HFAA) . He serves as an Associate Editor of the Journal of Financial Stability and the International Review of Finance . 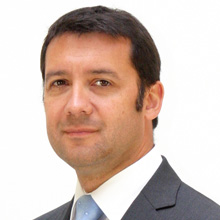 Claudio Raddatz is pofessor at the University of Chile. Previously, he was the Director of the Financial Policy Division at the Central Bank of Chile, he also worked at the World Bank, after earning a Ph.D. in economics from the Massachusetts Institute of Technology (MIT). Dr. Raddatz’s research focuses on the interactions between macroeconomics, finance, and development.  Sergio Schmukler is the Research Manager of Macroeconomics and Growth in the World Bank's Development Research Group. His research area is international finance and international financial markets and institutions. He obtained his Ph.D. in Economics from the University of California at Berkeley in 1997, when he joined the World Bank's Young Economist and Young Professionals Programs. He currently teaches financial development at Columbia University. He is a member of the Money and Finance Research (Mo.Fi.R) group and Treasurer of LACEA, the Latin America and Caribbean Economic Association (since 2004).  Dr. Andrew Steer is the President and CEO of the Bezos Earth Fund. Andrew joined the Bezos Earth Fund from the World Resources Institute, where he served as President & CEO for over eight years. Prior to this, he served as the World Bank’s Special Envoy for Climate Change from 2010 - 2012. From 2007 to 2010, he served as Director General at the UK Department of International Development. This followed 10 years in East Asia, where he was Head of the World Bank in Vietnam and Indonesia. Dr. Steer is a Global Agenda Trustee for the World Economic Forum, a Commissioner of the Energy Transitions Commission, a member of the China Council for International Cooperation on Environment and Development (CCICED), co-chair of the Greening the Belt and Road Coalition, and board member of the Global Energy Alliance for People and Planet. He is also a member of the Advisory Committees of the Asian Development Bank and on the leadership council of Concordia. Andrew was educated at St Andrews University, the University of Pennsylvania, and Cambridge University. He has a PhD in international economics and finance. He is married to Dr. Liesbet Steer and is the father of Charlotte and Benjamin. What should Developing Countries Do Differently in the Next Pandemic?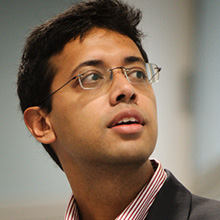 Ahmed Mushfiq Mobarak is the Jerome Kasoff ’54 Professor of Management and Economics at Yale University with concurrent appointments in the School of Management and in the Department of Economics. Mobarak is the founder and faculty director of the Yale Research Initiative on Innovation and Scale (Y-RISE) . Mobarak has several ongoing research projects in Bangladesh, Nepal, and Sierra Leone. He conducts field experiments exploring ways to induce people in developing countries to adopt technologies or behaviors that are likely to be welfare-improving. He also examines the complexities of scaling up development interventions that are proven effective in such trials. For example, he is scaling and testing strategies to address seasonal poverty using migration subsidies or consumption loans in Bangladesh, Nepal, and Indonesia. His research has been published in journals across disciplines, including Science, Nature, Econometrica, American Economic Review, Review of Economic Studies, BMJ, the American Political Science Review, PNAS, Marketing Science, and Demography , and covered by the New York Times, The Economist, NPR, BBC, NBC, The Washington Post, Wall Street Journal, Science, Nature, and other media outlets around the world. He received a Carnegie Fellowship in 2017 and was named to the inaugural Vox list of 50 “ scientists working to build a more perfect future ” in 2022.  Joana Silva is a Senior Economist at the Office of the Chief Economist for Latin America and the Caribbean. Since joining the World Bank in 2007 as a Young Professional, Joana published several books and articles on a broad set of issues related to economic development, including labor economics, education/skills, social safety nets, poverty, inequality, political economy of economic reforms, firm dynamics and international trade. Her research has been published in professional journals such as the Journal of International Economics , Economics Letters , Review of World Economics and IZA Journal of Labor Policy . Book titles authored or coauthored by Joana include “ Sustaining Employment and Wage Gains in Brazil: a Skills and Jobs Agenda ”, “ Inclusion and Resilience: The Way Forward for Social Safety Nets in the Middle East and North Africa ” and “ Striving for Better Jobs: The Challenge of Informality in the Middle East and North Africa ”. While at the Bank she authored thematic Flagship Reports (e.g. as Task Team Leader for the 2013 MENA Development Report, the Brazil Skills & Jobs report), managed cross-sectorial lending projects and advisory activities (e.g. Task Team Leader for innovative labor and social protection projects), and contributed to a range of analytical studies on design and evaluation of social welfare systems, labor markets, political economy, international integration and investment climate. She holds a Ph.D. in Economics from the University of Nottingham. Prior to joining the World Bank, she also worked for the Globalization and Economic Policy Research Center at the University of Nottingham and the Inter-American Development Bank. She is fluent in Portuguese, French, English, and Spanish. 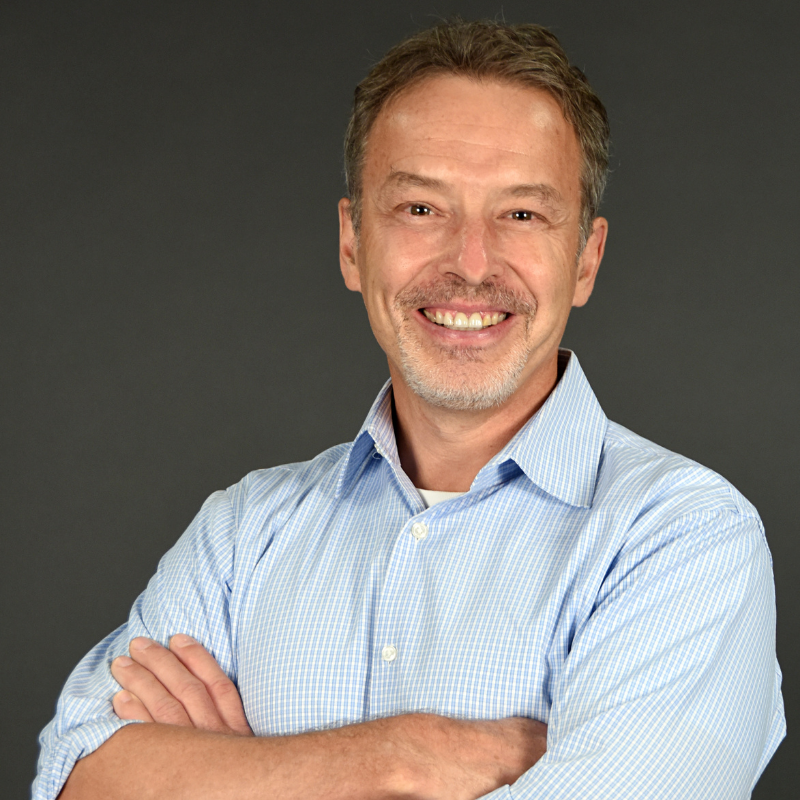 Norbert Schady, a German national, is Chief Economist for Human Development in the World Bank Group. Previously, he was Principal Economic Advisor, Social Sector, at the Inter-American Bank (2010-2021), Senior Economist in the Development Research Group (2003-2010), Economist in the Poverty Group of the Latin America region of the World Bank (2000-2003), and a Young Professional at the World Bank (1998-2000). Mr. Schady has also taught at Georgetown University and Princeton University. He received his PhD from Princeton University and his BA from Yale University. Mr. Schady has published extensively in academic journals, including in the Quarterly Journal of Economics , American Economic Journal: Applied , American Economic Journal: Policy , Review of Economics and Statistics , Journal of Human Resources , and Journal of Development Economics , among many others. He is also the author of numerous flagship reports, including The Early Years: Child Well-Being and the Role of Public Policy , Conditional Cash Transfers: Reducing Present and Future Poverty , and Closing the Gap in Education and Technology . Mr. Schady’s main research areas include early childhood development, teacher quality, cash transfer programs, and the effects of economic contractions on the accumulation of human capital. 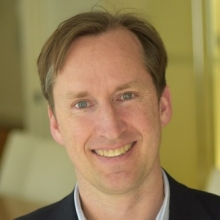 David Evans is the Principal Economic Advisor for the Social Sector at the Inter-American Development Bank . He was previously a senior fellow at the Center for Global Development , a visiting professor at the Center for Development Economics at Williams College , and a lead economist at the World Bank. Most of his research falls within the categories of education, health, gender, social protection, early childhood, and impact evaluation. David wants good research to inform policy. He has a Ph.D. in Economics from Harvard University (2005). Wednesday, July 10, 2024Welcome remarks. 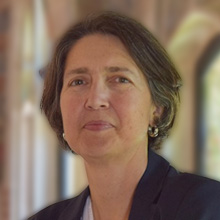 Dr. Rachel Glennerster has been appointed as the next President of CGD. She has distinguished background in international development; well-known for her groundbreaking work in academia and public service on both sides of the Atlantic. Her wealth of knowledge, innovative mindset, managerial acumen, and proven ability to secure funding will undoubtedly move CGD forward. Currently serving as an Associate Professor of Economics at the University of Chicago, Rachel has leveraged randomized trials to address critical issues spanning democracy, health, education, and women's empowerment, and pioneered ways to shape markets to promote innovation to address global challenges including pandemics and climate change. Her tenure as Chief Economist at the UK’s Foreign, Commonwealth & Development Office (FCDO) and its predecessor the Department for International Development, alongside her executive leadership at the Abdul Latif Jameel Poverty Action Lab (J-PAL), underscores her commitment to evidence-based policymaking and poverty reduction. Plenary Panel: Economic Inclusion of Women and Youth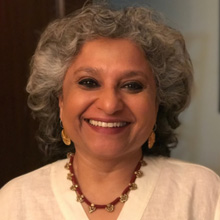 Ashwini Deshpande is Professor and Head, Department of Economics, and Founding Director, Centre for Economic Data and Analysis (CEDA) at Ashoka University, India. Her Ph.D. and early publications have been on the international debt crisis of the 1980s. Subsequently, she has been working on the economics of discrimination and affirmative action, with a focus on caste and gender in India. She has published extensively in leading scholarly journals. She is the author of “Grammar of Caste: economic discrimination in contemporary India”, Oxford University Press, New Delhi, 2011 (Hardcover) and 2017 (Paperback); and “Affirmative Action in India”, Oxford University Press, New Delhi, Oxford India Short Introductions series, 2013. She has edited several volumes, the latest of which is “Handbook on Economics of Discrimination and Affirmative Action” (Springer Major Reference Works). She is a Fellow of the International Economic Association. She received the EXIM Bank award for outstanding dissertation (now called the IERA Award) in 1994, the 2007 VKRV Rao Award for Indian economists under 45 and SKOCH Award for Gender Economics in 2022. 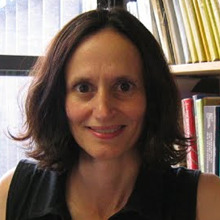 Raquel Fernández holds a Silver Professorship in the Department of Economics at NYU. She is a member of the NBER, the CEPR, IZA, BREAD, and of the Human Capital and Economic Opportunity Working Group. She has previously been a tenured professor at the London School of Economics, Boston University, and Oslo University. She was awarded the Carlos Diaz Alejandro Prize in 2024 and is a fellow of the Econometric Society and the recipient of numerous National Science Foundation grants. She has served as the Director of the Public Policy Program of the CEPR, as Vice President of the American Economic Association, and as President of the Latin American and Caribbean Economic Association (LACEA). She is the Founding Director of WELAC (Women Economists in LAC) — a standing subcommittee of LACEA that monitors and advances the status of women in the economics profession. She is co-director of the NBER’s Inequality and the Macroeconomy group and a member of the advisory and scientific committees of various institutions including the International Economics Association, the Barcelona School of Economics, UNU-WIDER, UBS Zurich, and RIDGE. Fernández has broad research interests that span sovereign debt, culture and economics, development and gender issues, macroeconomics and inequality, and political economy. She is a leading pioneer in the area of culture and economics and has published extensively in the major journals. 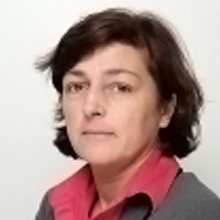 Karen Macours is a chaired professor at the Paris School of Economics (PSE), and senior researcher (directrice de recherche) at the French National Research Institute for Agriculture, Food and Environment (INRAE). Her research focusses on agricultural productivity and rural poverty reduction in developing countries, impact assessment related to agricultural R&D, the evaluation of programs addressing households’ productive and human capital investments (early childhood, health, nutrition, education) and related measurement and methodological questions. She is co-editor of the Journal of Development Economics, and associate editor of the American Journal of Agricultural Economics, and co-organizer of the Virtual Development Economics Seminar Series: VDEV/CEPR/BREAD . She is a board member of JPAL (Abdul Latif Jameel Poverty Action Lab) and serves as co-chair of JPAL's health sector and the Learning for All Initiative, is a member of the board of directors of BREAD (Bureau for Research and Economic Analysis of Development) and the Feed the Future Innovation Lab for Market Risk and Resilience, a member of the Weiss Fund committee, and until recently was chair of the CGIAR’s Standing Panel on Impact Assessment ( SPIA ). She is a research fellow of CEPR (Center for Economic Policy Research) and affiliate of EUDN (European Universities Development Network). 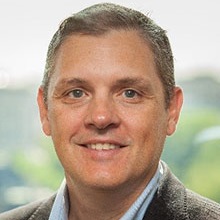 Deon Filmer is Director of the Research Group at the World Bank. He has previously served as Acting Research Manager in the Research Group, Co-Director of the World Development Report 2018: Learning to Realize Education’s Promise , and Lead Economist in the Human Development department of the Africa Region of the World Bank. He works on issues of human capital and skills, service delivery, and the impact of policies and programs to improve human development outcomes—with research spanning the areas of education, health, social protection, and poverty and inequality. He has published widely in refereed journals, including studies of the impact of demand-side programs on schooling and learning; the roles of poverty, gender, orphanhood, and disability in explaining education inequalities; and the determinants of effective service delivery. He has recently co-authored the following books: Making Schools Work: New Evidence from Accountability Reforms , Youth Employment in Sub-Saharan Africa , and From Mines and Wells to Well-Built Minds: Turning Sub-Saharan Africa's Natural Resource Wealth into Human Capital . He was a core team member of the World Bank's World Development Reports in 1995 Workers in an Integrating World and 2004 Making Services Work for Poor People , and a contributor to 2007’s report Development and the Next Generation . He holds a PhD and MA from Brown University and a BA from Tufts University. Social Safety Nets and Women’s Labor Force Participation in LMICs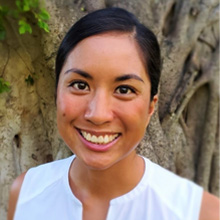 Teresa Molina is an Associate Professor of Economics at the University of Hawaii at Manoa, Research Affiliate at the IZA Institute of Labor Economics , Research Fellow at UHERO , and Non-Resident Fellow at the Center for Global Development . She currently serves as an Associate Editor for the Journal of Health Economics. Teressa received her Ph.D. in Economics from the University of Southern California and her undergraduate degree from Stanford University. Teresa works primarily on topics in development, health, and labor economics. Her research page contains a list of her publications and working papers. 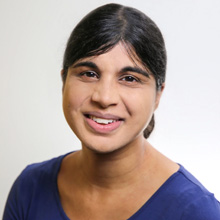 Shalini Roy is a Senior Research Fellow in the Poverty, Gender, and Inclusion Unit. Her research focuses on the relationship between households’ economic behavior and social protection, nutrition, agriculture, gender, and early childhood development. Much of her work is based on impact evaluations of development programming, with a particular interest in how programs both affect and are affected by intrahousehold dynamics. Her work spans South Asia (with a regional focus in Bangladesh), Africa, and Latin America. Shalini’s recent research includes comparing impacts of food and cash transfers on food security, nutrition, gender dynamics, and early childhood development in Bangladesh and Uganda; evaluating impacts of large-scale cash transfer projects in Mali, Brazil, and Mexico; and assessing gender- and nutrition-related impacts of agricultural projects in Bangladesh. Shalini has been at IFPRI since 2009 and holds a PhD in Economics from the University of Pennsylvania. 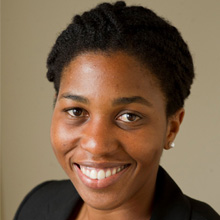 Kehinde Ajayi is a senior fellow at the Center for Global Development, working on gender equality, education, and social safety nets. Previously, Ajayi was at the World Bank, where she coordinated research initiatives on women’s economic empowerment, youth employment, social protection, and childcare in the Africa Gender Innovation Lab. Before joining the World Bank, she was an Assistant Professor of Economics at Boston University, a Faculty Research Fellow of the National Bureau of Economic Research, and a Fulbright Fellow. She holds a PhD in Economics from the University of California, Berkeley and a bachelor’s degree in Economics and International Relations from Stanford University. 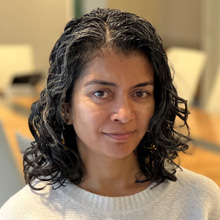 Eeshani is a Senior Fellow at the Center for Global Development; prior to this, she was a Senior Economist in the Development Research Group of the World Bank. She was born and raised in India and has a PhD from the University of Illinois and a BA from Macalester College. 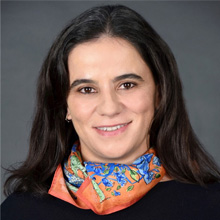 Maria Caridad Araujo is Chief of the Gender and Diversity Division at the IDB, where she leads efforts to improve access to quality services, economic opportunities, and strengthen the voice and representation of women, indigenous peoples, Afro-descendants, people with disabilities, and LGBTQ. + community. As chief economist in the IDB's Health and Social Protection Division, she worked on child development and poverty reduction programs. She was a professor at Georgetown University and worked at the World Bank. Maria Caridad has a Ph.D. in Agricultural and Natural Resource Economics from the University of California, Berkeley. Lunchtime Keynote Aaddress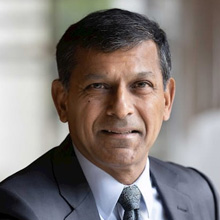 Raghuram Rajan is the Katherine Dusak Miller Distinguished Service Professor of Finance at Chicago Booth. He was the 23rd Governor of the Reserve Bank of India between September 2013 and September 2016. Between 2003 and 2006, Dr. Rajan was the Chief Economist and Director of Research at the International Monetary Fund. Dr. Rajan’s research interests are in banking, corporate finance, and economic development. The books he has written include Breaking the Mold: Reimagining India's Economic Future with Rohit Lamba, The Third Pillar: How the State and Markets hold the Community Behind 2019 which was a finalist for the Financial Times Business Book of the Year prize and Fault Lines: How Hidden Fractures Still Threaten the World Economy , for which he was awarded the Financial Times prize for Business Book of the Year in 2010. Norms and Other Constraints to Women’s Economic inclusion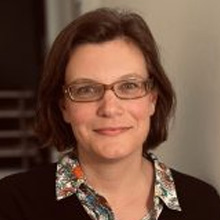 Pamela Jakiela is an Associate Professor in the Economics Department at Williams College and a Non-Resident Fellow at the Center for Global Development . She is also affiliated with BREAD , IPA , IZA , and J-PAL . 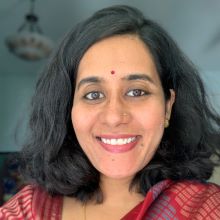 S Anukriti is a Senior Economist in the Development Research Group (Human Development Team) of the World Bank. She is an applied micro-economist, with interests in the fields of development economics, economics of gender and the family, and political economy. Her research examines the underlying causes of gender inequalities in developing societies, and explores mechanisms that can bring about gender equity. More broadly, she is interested in the role of social norms, formal and informal institutions, and public policy in affecting social change. Dr. Anukriti received her PhD in Economics from Columbia University, MA in Economics from the Delhi School of Economics, and BA (Honors) from St. Stephen’s College, Delhi. Prior to joining the World Bank in July 2020, she was an Assistant Professor of Economics at Boston College. She is also a Research Fellow at the Institute for Labor Economics (IZA). 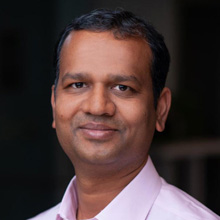 Nishith Prakash is a Research Fellow at CESifo, Centre for Research and Analysis of Migration (CReAM), The Institute for the Study of Labor (IZA), HiCN Households in Conflict Network (HiCN), Innovations for Poverty Action (IPA) Network Researcher, Global Labor Organization (GLO), and Member of Insights on Immigration and Development (INSIDE-SPAIN). He is also currently serving in the Editorial Board of the journal PLOS ONE and Associate Editor at the Journal of Development Economics. Born and raised in Bihar, India, he earned a B.A. (honors) in economics from Shivaji College, an M.A. in economics from Delhi School of Economics, Delhi University (India), and a Ph.D. in economics from University of Houston, TX. He was a post-doctoral research associate at Cornell University, NY from July 2010 – December 2011. He has been a Visiting Fellow at Yale, Columbia, Massachusetts Institute of Technology, Boston University, and Women and Public Policy Program at Harvard Kennedy School.  Rachel Heath is an associate professor in the department of Economics at the University of Washington. Her research interests are in development and labor economics. In particular, much of her research focuses on increased labor market opportunities for women in developing countries (such as the garment industry in Bangladesh). Rachel studies how these new job opportunities are changing women's lives, the factors that influence women's decisions to join the labor force, and how firms make hiring decisions. 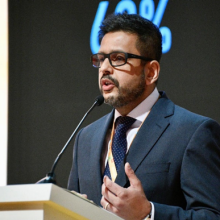 Somik Lall is Staff Director of the World Bank’s 2024 World Development Report, which examines the challenges of economic growth in middle-income countries. He is also an Economic Adviser in the Office of the World Bank Group Chief Economist. Previously, he headed the climate economics and policy team in the World Bank’s Equitable Growth, Finance and Institutions vice presidency, where he developed and supervised high-quality research programs on resilience and economic development, the macro-criticality of climate change, and innovations for the low-carbon transition. His other roles include Global Lead for territorial and spatial development and Lead Economist for the World Bank’s Urban, Disaster Risk, Resilience, and Land Global Practice. Dr. Lall also teaches at Johns Hopkins University and has been a Visiting Professor at the National Institute of Public Finance and Policy. He has advised senior policymakers in national and city governments on key policy issues in over 25 countries—including Brazil, China, India, Saudi Arabia, and South Africa. He is widely published in academic and policy journals. His 2021 book, Place, Productivity, and Prosperity , examines the spatial dimensions of productivity growth in developing nations, and provides a new analytic framework to help policy makers arrive at a disciplined view of a place’s economic potential. His 2017 book, “ Africa's Cities: Opening Doors to the World ,” has over 100,000 downloads and 3 million social media views. He has developed a novel data-driven approach to help city mayors rapidly respond to protect their citizens from the ravages of COVID 19. Listen to his Monocle podcast . Economic Inclusion of Youth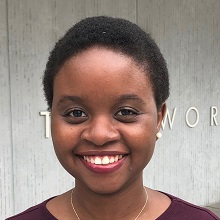 Oyebola Okunogbe is an Economist in the Human Development team of the World Bank Development Research Group. Her research interests are in governance and political economy, including policies on public finance, nation building, education, employment and gender. Oyebola obtained her PhD in Public Policy and MPA in International Development from Harvard University, and her B.A. in Economics from Dartmouth College. She was born and raised in Nigeria. 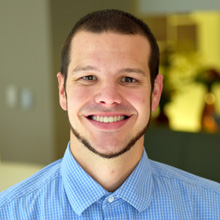 Thomas Ginn is a research fellow at the Center for Global Development, where he studies migration and displacement. His current research focuses on aid for refugees in lower-income countries, including projects on labor market access, housing, and host community attitudes. Prior to joining CGD, Thomas worked with the World Bank on a survey of Syrian refugees in Lebanon, Jordan, and Iraqi Kurdistan and with Innovations for Poverty Action in Kenya. He received his PhD in economics from Stanford University, where his dissertation evaluated camps for Syrian refugees, and his BA from the University of North Carolina at Chapel Hill.  Isaac M. Mbiti is an Associate Professor of Public Policy and Economics at the Frank Batten School of Leadership and Public Policy. Prior to his appointment at the Batten School, Mbiti was an Assistant Professor of Economics at Southern Methodist University and also served as a Martin Luther King Visiting Assistant Professor at the Massachusetts Institute of Technology. His research has focused broadly on African economic development with particular interests in examining the role of education policies such as free primary education and teacher performance pay programs, as well as the potential for new technologies (especially mobile phones) to spur the development process. His ongoing research projects in East and West Africa evaluate various policies that aim to improve the livelihoods of African youth through training programs. His research has been supported by numerous agencies including the National Science Foundation, The National Institutes of Health, the International Impact Evaluation Initiative, USAID and the World Bank. He is a research affiliate at the Abdul Latif Jameel Poverty Action Lab (J-PAL) at MIT and was previously selected as a National Academy of Education/Spencer Foundation Post-Doctoral Fellow. His publications have appeared in the American Economic Review , Journal of Economic Perspectives , American Economic Journal: Applied Economics , and Journal of African Economies . He has also authored several policy reports for the Kenyan Government, the World Bank and NGOs, such as the International Rescue Committee. He received his Ph.D. in economics from Brown University. 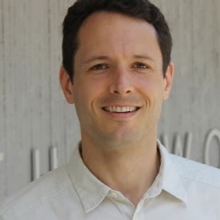 Owen Ozier is an Associate Professor in the Department of Economics at Williams College . He was previously a Senior Economist in the World Bank's Development Research Group, Human Development Team. He received his M.Eng. and B.S. degrees in Electrical Engineering and Computer Science and in Brain and Cognitive Sciences from the Massachusetts Institute of Technology in 1999, and his Ph.D. in Economics from the University of California at Berkeley in 2010. His current research projects focus on health, education, and economic decisions in Sub-Saharan Africa. 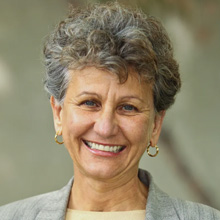 Tamar Manuelyan Atinc is a development professional with over 30 years of experience in the analysis and implementation of development policies and programs. She is currently a nonresident senior fellow at the Brookings Institution where she has worked since 2013. During her long career at the World Bank, Manuelyan Atinc was Vice President for Human Development and served in three regions, including Europe and Central Asia, East Asia and the Pacific, and Africa working to advance country policy and programs to foster human development, reduce poverty, and improve economic management. Her recent research has focused primarily on scaling up early childhood development, social impact bonds, and data and accountability for better education outcomes. Manuelyan Atinc is a member of the the Board of the Graduate Institute of International Relations and Development Studies in Geneva since October 2017, a member of the Board of ChildFund International, and a member of the Advisory Committee for the Queen Rania Foundation since October 2018. She started her career working in Geneva at the General Agreement on Trade and Tariffs and the United Nations Conference on Trade and Development. A Turkish national, Ms. Manuelyan Atinc has undergraduate and graduate degrees from Harvard University and the Kennedy School of Government at Harvard. Somik Lall is Senior Adviser to the Chief Economist of the World Bank Group. Lall has recently directed the World Bank's 2024 World Development Report on the “Middle Income Trap” that examines how middle-income countries can break into the ranks of the wealthiest economies. Previously, he headed the climate economics and policy team in the World Bank’s Equitable Growth, Finance, and Institutions vice presidency, where he developed and supervised policy research programs on resilience and economic development, the macro-criticality of climate change, and innovations for the low-carbon transition. His other roles include being the Global Lead for territorial development and Lead Economist for the World Bank’s Cities Practice where he developed a novel data-driven approach to help city mayors rapidly respond to protect their citizens from the ravages of COVID 19. Listen to his Monocle podcast . Lall is widely published in academic and policy journals. His 2021 book, Place, Productivity, and Prosperity , provides a new analytic framework to discipline policies targeting specific places within countries. His 2017 book, “ Africa's Cities: Opening Doors to the World ,” provides new evidence on institutional and regulatory constraints that hobble urbanization in Africa. Dr. Lall also teaches at Johns Hopkins University and has been a Visiting Professor at the National Institute of Public Finance and Policy in New Delhi. Eeshani is a Senior Fellow at the Center for Global Development; prior to this, she was a Senior Economist in the Development Research Group of the World Bank. She was born and raised in India and has a PhD from the University of Illinois and a BA from Macalester College. 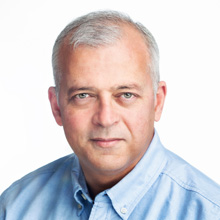 Joe Rebello is the World Bank’s External Affairs Lead for Development Economics. In this capacity, he advises the World Bank Group’s Chief Economist on external engagement and media relations. He also plays a key role in message development and external outreach on several flagship publications—including the World Development Report , Global Economic Prospects , and Women, Business, and the Law . He was previously a senior communications officer for the International Finance Corporation, where he advised senior management in crafting IFC’s highest-priority internal and external communications. Before joining the World Bank Group in 2008, he was a business journalist for 20 years, writing about US and international economic policy, debt distress, and banking for The Wall Street Journal , Dow Jones Newswires , and The Kansas City Star 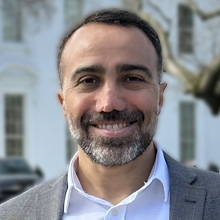 Kenan Karakülah is an Economist at the Development Economics Development Policy (DECDP) unit. He was a member of core team of 2024 World Development Report. His research interests cover various cross-cutting areas of macroeconomic policy, including economic growth, social protection, and sovereign debt. Prior to joining the Bank, he worked as the Head of Department at the Ministry of Treasury and Finance. Kenan holds a Ph.D. in Economics from the Hacettepe University, Türkiye, and a Master of International Development Policy from Duke University. 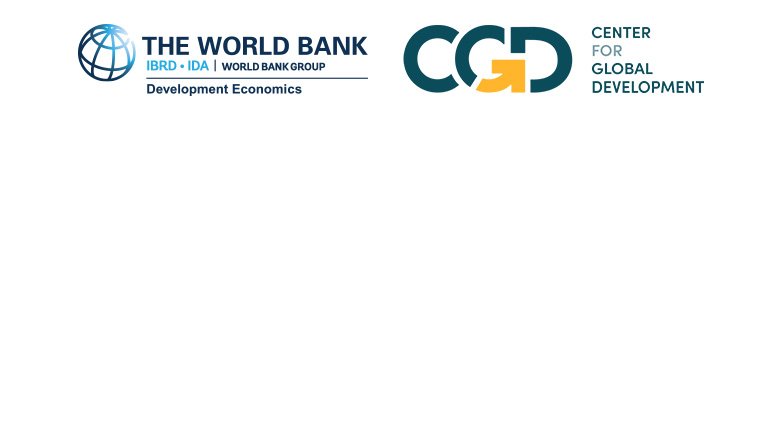 CONFERENCE DETAILS- DATE: July 9–10, 2024
- Venue (In-Person): Preston Auditorium, World Bank & Birdsall House Conference Center, Center for Global Development, Washington, DC
- Virtual: Zoom
➜ ABCDE 2024 PAST ABCDE CONFERENCES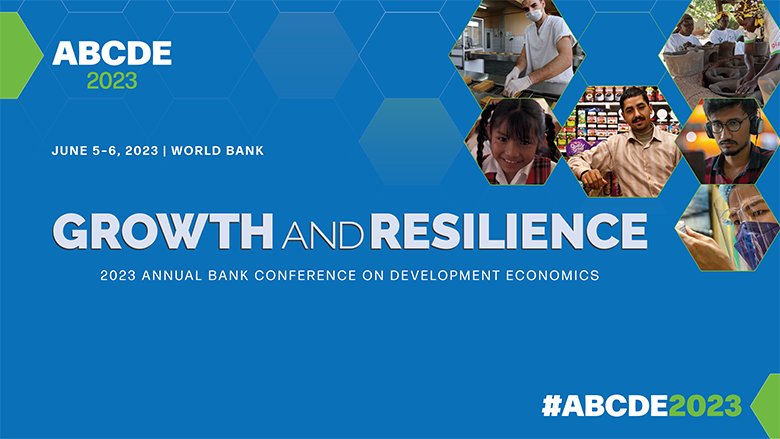 ➜ CGD Conference Site ➜ Development Economics This site uses cookies to optimize functionality and give you the best possible experience. If you continue to navigate this website beyond this page, cookies will be placed on your browser. To learn more about cookies, click here . - Work & Careers
- Life & Arts
The Chinese quant fund-turned-AI pioneerTry unlimited access only $1 for 4 weeks. Then $75 per month. Complete digital access to quality FT journalism on any device. Cancel anytime during your trial. - Global news & analysis
- Expert opinion
- Special features
- FirstFT newsletter
- Videos & Podcasts
- Android & iOS app
- FT Edit app
- 10 gift articles per month
Explore more offers.Standard digital. Premium DigitalPrint + premium digital, ft professional, weekend print + standard digital, weekend print + premium digital. Complete digital access to quality FT journalism with expert analysis from industry leaders. Pay a year upfront and save 20%. - Global news & analysis
- Exclusive FT analysis
- FT App on Android & iOS
- FirstFT: the day's biggest stories
- 20+ curated newsletters
- Follow topics & set alerts with myFT
- FT Videos & Podcasts
- 20 monthly gift articles to share
- Lex: FT's flagship investment column
- 15+ Premium newsletters by leading experts
- FT Digital Edition: our digitised print edition
- Weekday Print Edition
- Videos & Podcasts
- Premium newsletters
- 10 additional gift articles per month
- FT Weekend Print delivery
- Everything in Standard Digital
- Everything in Premium Digital
FT Weekend newspaper delivered Saturday plus complete digital access. - 10 monthly gift articles to share
- Everything in Print
- Make and share highlights
- FT Workspace
- Markets data widget
- Subscription Manager
- Workflow integrations
- Occasional readers go free
- Volume discount
Billed Quarterly at $199. Complete digital access plus the FT newspaper delivered Monday-Saturday. Terms & Conditions apply Explore our full range of subscriptions.Why the ft. See why over a million readers pay to read the Financial Times. International Edition Root out friction in every digital experience, super-charge conversion rates, and optimise digital self-service Uncover insights from any interaction, deliver AI-powered agent coaching, and reduce cost to serve Increase revenue and loyalty with real-time insights and recommendations delivered straight to teams on the ground Know how your people feel and empower managers to improve employee engagement, productivity, and retention Take action in the moments that matter most along the employee journey and drive bottom line growth Whatever they’re are saying, wherever they’re saying it, know exactly what’s going on with your people Get faster, richer insights with qual and quant tools that make powerful market research available to everyone Run concept tests, pricing studies, prototyping + more with fast, powerful studies designed by UX research experts Track your brand performance 24/7 and act quickly to respond to opportunities and challenges in your market Meet the operating system for experience management - Free Account
- Product Demos
- For Digital
- For Customer Care
- For Human Resources
- For Researchers
- Financial Services
- All Industries
Popular Use Cases - Customer Experience
- Employee Experience
- Employee Exit Interviews
- Net Promoter Score
- Voice of Customer
- Customer Success Hub
- Product Documentation
- Training & Certification
- XM Institute
- Popular Resources
- Customer Stories
- Artificial Intelligence
Market Research The annual gathering of the experience leaders at the world’s iconic brands building breakthrough business results. 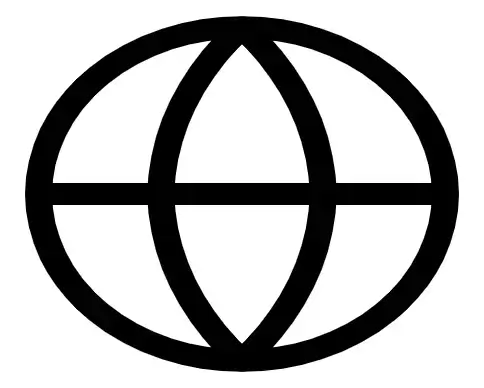 - English/AU & NZ
- Español/Europa
- Español/América Latina
- Português Brasileiro
- REQUEST DEMO
- Experience Management
- Ultimate Guide to Market Research
- Qualitative vs Quantitative Research
Try Qualtrics for freeQualitative vs quantitative research. 13 min read You’ll use both quantitative and qualitative research methods to gather survey data. What are they exactly, and how can you best use them to gain the most accurate insights? What is qualitative research?Qualitative research is all about language, expression, body language and other forms of human communication . That covers words, meanings and understanding. Qualitative research is used to describe WHY. Why do people feel the way they do, why do they act in a certain way, what opinions do they have and what motivates them? Qualitative data is used to understand phenomena – things that happen, situations that exist, and most importantly the meanings associated with them. It can help add a ‘why’ element to factual, objective data. Qualitative research gives breadth, depth and context to questions, although its linguistic subtleties and subjectivity can mean that results are trickier to analyse than quantitative data. This qualitative data is called unstructured data by researchers. This is because it has not traditionally had the type of structure that can be processed by computers, until today. It has, until recently at least, been exclusively accessible to human brains. And although our brains are highly sophisticated, they have limited processing power. What can help analyse this structured data to assist computers and the human brain? Discover the 2023 Market Research Trends Transforming the industry What is quantitative research?Quantitative data refers to numerical information. Quantitative research gathers information that can be counted, measured, or rated numerically – AKA quantitative data. Scores, measurements, financial records, temperature charts and receipts or ledgers are all examples of quantitative data. Quantitative data is often structured data, because it follows a consistent, predictable pattern that computers and calculating devices are able to process with ease. Humans can process it too, although we are now able to pass it over to machines to process on our behalf. This is partly what has made quantitative data so important historically, and why quantitative data – sometimes called ‘hard data’ – has dominated over qualitative data in fields like business, finance and economics. It’s easy to ‘crunch the numbers’ of quantitative data and produce results visually in graphs, tables and on data analysis dashboards. Thanks to today’s abundance and accessibility of processing power, combined with our ability to store huge amounts of information, quantitative data has fuelled the Big Data phenomenon, putting quantitative methods and vast amounts of quantitative data at our fingertips. As we’ve indicated, quantitative and qualitative data are entirely different and mutually exclusive categories. Here are a few of the differences between them. 1. Data collectionData collection methods for quantitative data and qualitative data vary, but there are also some places where they overlap. Qualitative data collection methods | Quantitative data collection methods | Gathered from focus groups, in-depth interviews, case studies, expert opinion, observation, audio recordings, and can also be collected using surveys. | Gathered from surveys, questionnaires, polls, or from secondary sources like census data, reports, records and historical business data. | Uses and open text survey questions | Intended to be as close to objective as possible. Understands the ‘human touch’ only through quantifying the OE data that only this type of research can code. | 2. Data analysisQuantitative data suits statistical analysis techniques like linear regression, T-tests and ANOVA. These are quite easy to automate, and large quantities of quantitative data can be analyzed quickly. Analyzing qualitative data needs a higher degree of human judgement, since unlike quantitative data, non numerical data of a subjective nature has certain characteristics that inferential statistics can’t perceive. Working at a human scale has historically meant that qualitative data is lower in volume – although it can be richer in insights. Qualitative data analysis | Quantitative data analysis | Results are categorised, summarised and interpreted using human language and perception, as well as logical reasoning | Results are analysed mathematically and statistically, without recourse to intuition or personal experience. | Fewer respondents needed, each providing more detail | Many respondents needed to achieve a representative result | 3. Strengths and weaknessesWhen weighing up qualitative vs quantitative research, it’s largely a matter of choosing the method appropriate to your research goals. If you’re in the position of having to choose one method over another, it’s worth knowing the strengths and limitations of each, so that you know what to expect from your results. Qualitative approach | Quantitative approach | Can be used to help formulate a theory to be researched by describing a present phenomenon | Can be used to test and confirm a formulated theory | Results typically expressed as text, in a report, presentation or journal article | Results expressed as numbers, tables and graphs, relying on numerical data to tell a story. | Less suitable for scientific research | More suitable for scientific research and compatible with most standard statistical analysis methods | Harder to replicate, since no two people are the same | Easy to replicate, since what is countable can be counted again | Less suitable for sensitive data: respondents may be biased or too familiar with the pro | Ideal for sensitive data as it can be anonymized and secured | Qualitative vs quantitative – the role of research questionsHow do you know whether you need qualitative or quantitative research techniques? By finding out what kind of data you’re going to be collecting. You’ll do this as you develop your research question, one of the first steps to any research program. It’s a single sentence that sums up the purpose of your research, who you’re going to gather data from, and what results you’re looking for. As you formulate your question, you’ll get a sense of the sort of answer you’re working towards, and whether it will be expressed in numerical data or qualitative data. For example, your research question might be “How often does a poor customer experience cause shoppers to abandon their shopping carts?” – this is a quantitative topic, as you’re looking for numerical values. Or it might be “What is the emotional impact of a poor customer experience on regular customers in our supermarket?” This is a qualitative topic, concerned with thoughts and feelings and answered in personal, subjective ways that vary between respondents. Here’s how to evaluate your research question and decide which method to use: Use this if your goal is to understand something – experiences, problems, ideas. For example, you may want to understand how poor experiences in a supermarket make your customers feel. You might carry out this research through focus groups or in depth interviews (IDI’s). For a larger scale research method you could start by surveying supermarket loyalty card holders, asking open text questions, like “How would you describe your experience today?” or “What could be improved about your experience?” This research will provide context and understanding that quantitative research will not. Use this if your goal is to test or confirm a hypothesis, or to study cause and effect relationships. For example, you want to find out what percentage of your returning customers are happy with the customer experience at your store. You can collect data to answer this via a survey. For example, you could recruit 1,000 loyalty card holders as participants, asking them, “On a scale of 1-5, how happy are you with our store?” You can then make simple mathematical calculations to find the average score. The larger sample size will help make sure your results aren’t skewed by anomalous data or outliers, so you can draw conclusions with confidence. Qualitative and quantitative research combined?Do you always have to choose between qualitative or quantitative data? 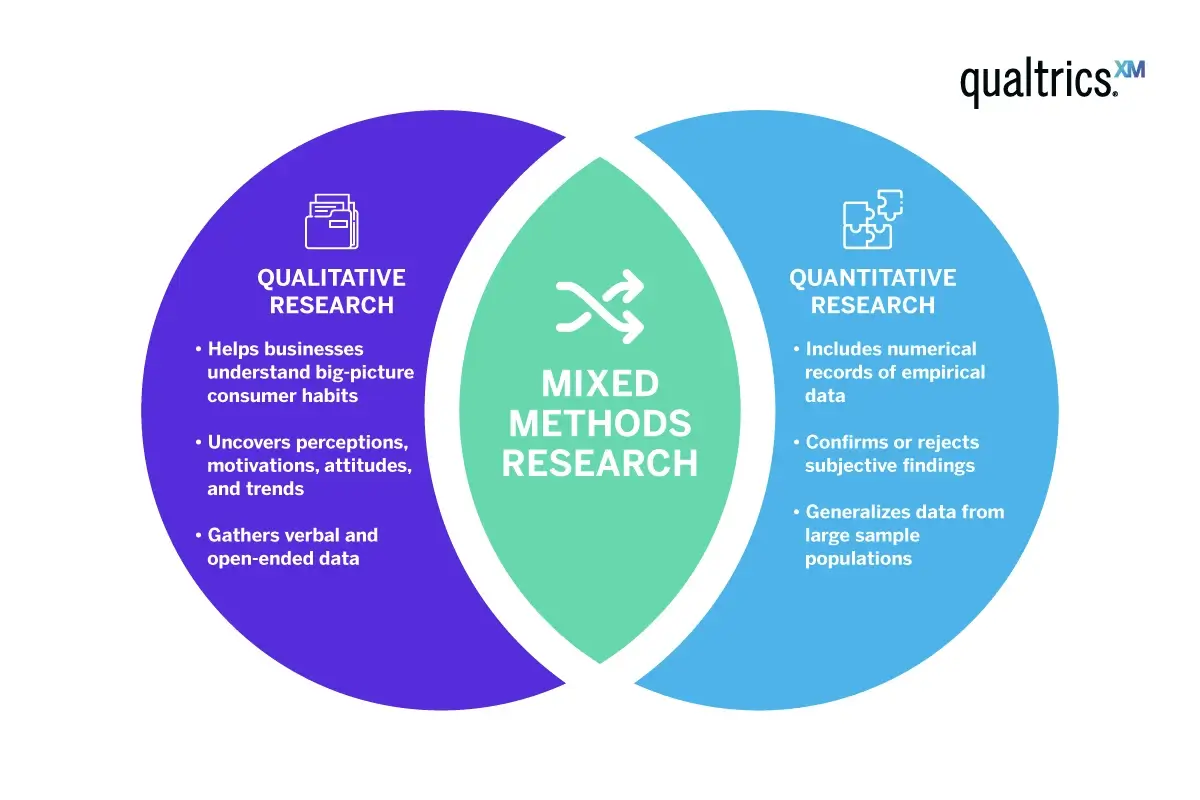 In some cases you can get the best of both worlds by combining both quantitative and qualitative data.You could use pre quantitative data to understand the landscape of your research. Here you can gain insights around a topic and propose a hypothesis. Then adopt a quantitative research method to test it out. Here you’ll discover where to focus your survey appropriately or to pre-test your survey, to ensure your questions are understood as you intended. Finally, using a round of qualitative research methods to bring your insights and story to life. This mixed methods approach is becoming increasingly popular with businesses who are looking for in depth insights. For example, in the supermarket scenario we’ve described, you could start out with a qualitative data collection phase where you use focus groups and conduct interviews with customers. You might find suggestions in your qualitative data that customers would like to be able to buy children’s clothes in the store. In response, the supermarket might pilot a children’s clothing range. Targeted quantitative research could then reveal whether or not those stores selling children’s clothes achieve higher customer satisfaction scores and a rise in profits for clothing. Together, qualitative and quantitative data, combined with statistical analysis, have provided important insights about customer experience, and have proven the effectiveness of a solution to business problems. Qualitative vs quantitative question typesAs we’ve noted, surveys are one of the data collection methods suitable for both quantitative and qualitative research. Depending on the types of questions you choose to include, you can generate qualitative and quantitative data. Here we have summarized some of the survey question types you can use for each purpose. Qualitative data survey questionsThere are fewer survey question options for collecting qualitative data, since they all essentially do the same thing – provide the respondent with space to enter information in their own words. Qualitative research is not typically done with surveys alone, and researchers may use a mix of qualitative methods. As well as a survey, they might conduct in depth interviews, use observational studies or hold focus groups. Open text ‘Other’ box (can be used with multiple choice questions) 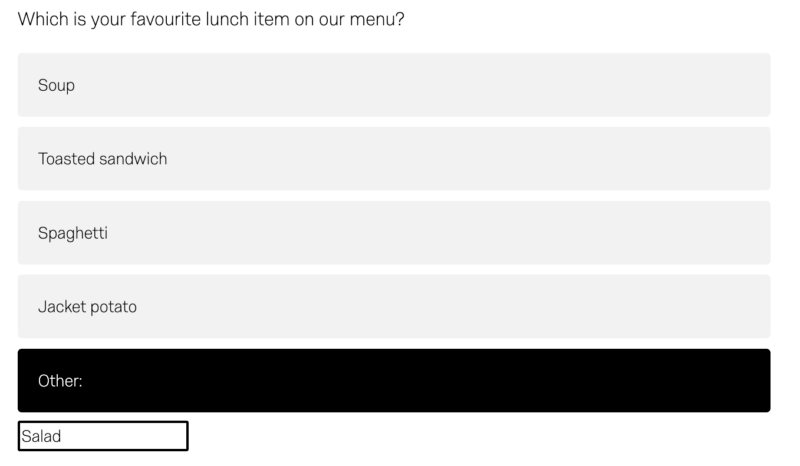 Text box (space for short written answer)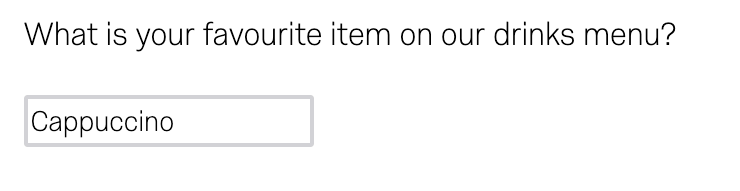 Essay box (space for longer, more detailed written answers)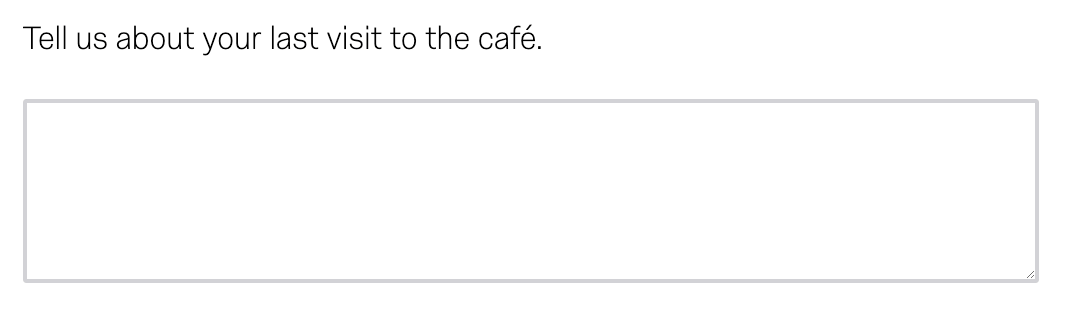 Quantitative data survey questionsThese questions will yield quantitative data – i.e. a numerical value. Net Promoter Score (NPS)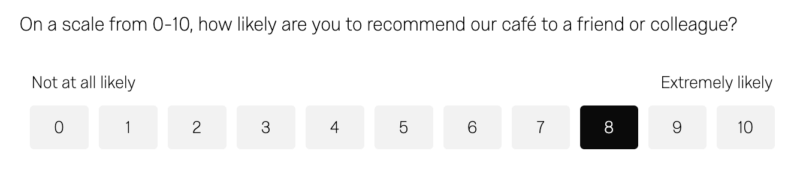 Likert Scale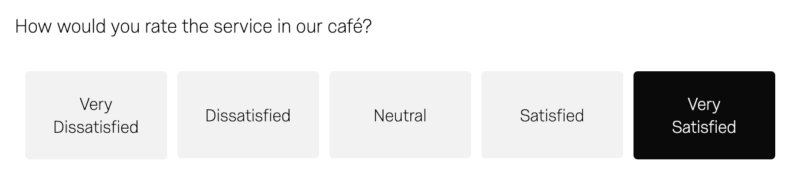 Radio buttons (respondents choose just one option)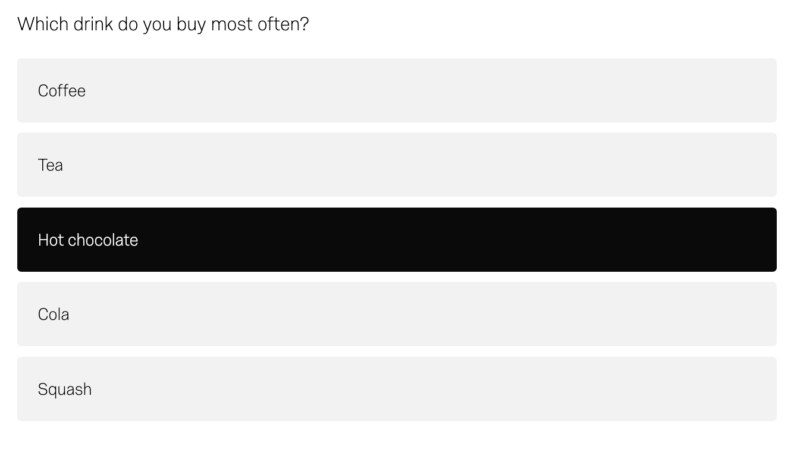 Check boxes (respondents can choose multiple options)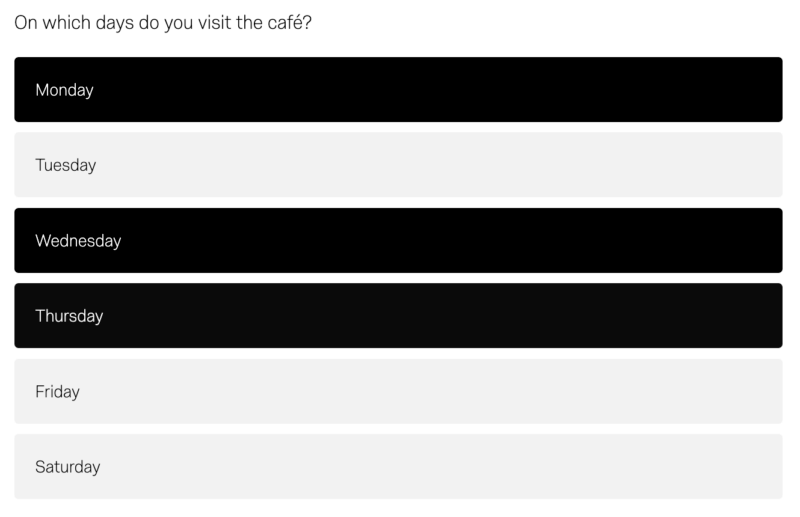 Sliding scale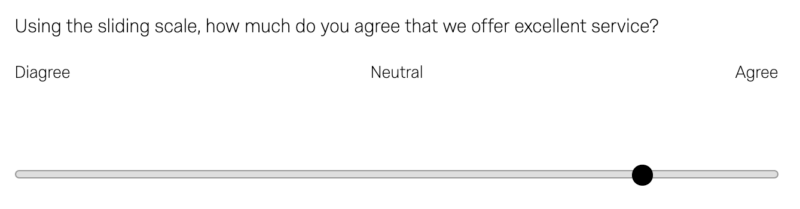 Star rating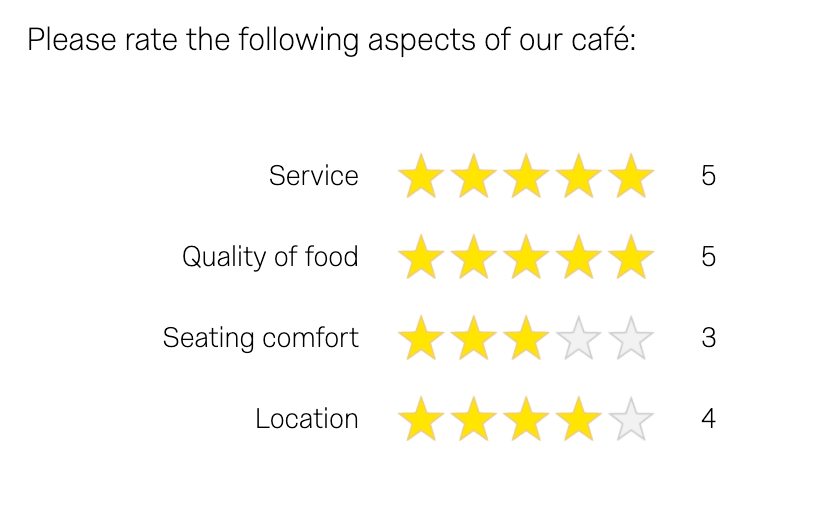 Analysing data (quantitative or qualitative) using technologyWe are currently at an exciting point in the history of qualitative analysis. Digital analysis and other methods that were formerly exclusively used for quantitative data are now used for interpreting non numerical data too. Artificial intelligence programs can now be used to analyse open text, and turn qualitative data into structured and semi structured quantitative data that relates to qualitative data topics such as emotion and sentiment, opinion and experience. Research that in the past would have meant qualitative researchers conducting time-intensive studies using analysis methods like thematic analysis can now be done in a very short space of time. This not only saves time and money, but opens up qualitative data analysis to a much wider range of businesses and organisations. The most advanced tools can even be used for real-time statistical analysis, forecasting and prediction, making them a powerful asset for businesses. Qualitative or quantitative – which is better for data analysis?Historically, quantitative data was much easier to analyse than qualitative data. But as we’ve seen, modern technology is helping qualitative analysis to catch up, making it quicker and less labor-intensive than before. That means the choice between qualitative and quantitative studies no longer needs to factor in ease of analysis, provided you have the right tools at your disposal. With an integrated platform like Qualtrics, which incorporates data collection, data cleaning, data coding and a powerful suite of analysis tools for both qualitative and quantitative data, you have a wide range of options at your fingertips. eBook: A guide to building agile research functions in-house Related resourcesMarket intelligence 9 min read, qualitative research questions 11 min read, ethnographic research 11 min read, business research methods 12 min read, qualitative research design 12 min read, business research 10 min read, qualitative research interviews 11 min read, request demo. Ready to learn more about Qualtrics? Numbers, Facts and Trends Shaping Your World Read our research on: Full Topic List Regions & Countries- Publications
- Our Methods
- Short Reads
- Tools & Resources
Read Our Research On: Globally, Biden Receives Higher Ratings Than TrumpStill, most disapprove of how biden has dealt with the israel-hamas war, table of contents. - Views of the U.S.
- Confidence in Biden, Trump and other world leaders
- Differences by ideology, age and gender
- 1. Views of the U.S.
- 2. Confidence in Joe Biden
- 3. Confidence in Donald Trump
- 4. Comparing confidence in Macron, Putin and Xi to ratings of Biden and Trump
- Biden’s handling of global economic problems
- Biden’s handling of climate change
- Biden’s handling of China
- Biden’s handling of the Russia-Ukraine war
- Biden’s handling of the Israel-Hamas war
- 6. Is U.S. democracy a good example to follow?
- Appendix A: Favorability of the United States since 2000
- Appendix B: Confidence in the U.S. president since 2001
- Acknowledgments
- About Pew Research Center’s Spring 2024 Global Attitudes Survey
This Pew Research Center analysis focuses on public opinion of the United States, President Joe Biden and other world leaders. It also explores what people think about Biden’s handling of international issues and their perceptions of American democracy. The study includes publics in 34 countries across the Asia-Pacific region, Europe, Latin America, the Middle East, North America and sub-Saharan Africa. This analysis draws on nationally representative surveys of 40,566 adults conducted from Jan. 5 to May 21, 2024. All surveys were conducted over the phone with adults in Canada, France, Germany, Greece, Italy, Japan, Malaysia, the Netherlands, Singapore, South Korea, Spain, Sweden and the United Kingdom. Surveys were conducted face to face in Argentina, Bangladesh, Brazil, Chile, Colombia, Ghana, Hungary, India, Israel, Kenya, Mexico, Nigeria, Peru, the Philippines, Poland, South Africa, Sri Lanka, Thailand, Tunisia and Turkey. In Australia, we used a mixed-mode probability-based online panel. 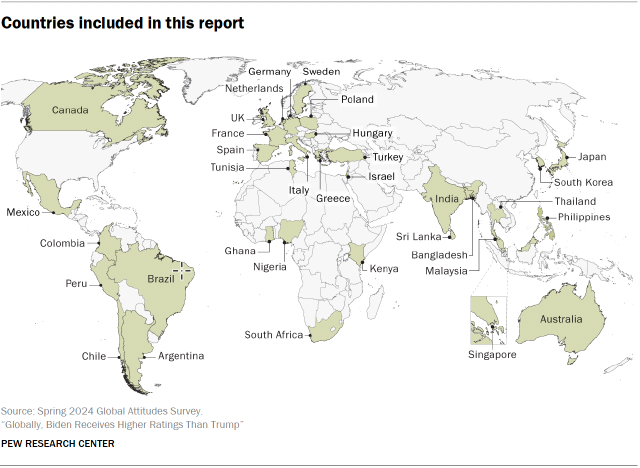 Throughout the report, we analyze respondents’ attitudes based on where they place themselves on an ideological scale. We asked about political ideology using several slightly different scales and categorized people as being on the ideological left, center or right. - In most countries, we asked people to place themselves on a scale ranging from “Extreme left” to “Extreme right.” The question was asked this way in Argentina, Bangladesh, Brazil, Canada, Chile, Colombia, France, Germany, Greece, Hungary, Israel, Italy, Mexico, the Netherlands, Nigeria, Peru, the Philippines, Poland, South Africa, Spain, Sweden, Turkey and the United Kingdom.
- In Australia, the scale ranged from “Left” to “Right”.
- In Japan, Singapore, South Korea and Thailand, ideology was measured on a scale from “Extremely progressive” to “Extremely conservative.”
- Ideology was not asked about in Ghana, India, Kenya, Malaysia, Sri Lanka or Tunisia.
Prior to 2024, combined totals were based on rounded topline figures. For all reports beginning in 2024, totals are based on unrounded topline figures, so combined totals might be different than in previous years. Refer to the 2024 topline to see our new rounding procedures applied to past years’ data. Here are the questions used for the report, along with responses, and the survey methodology . 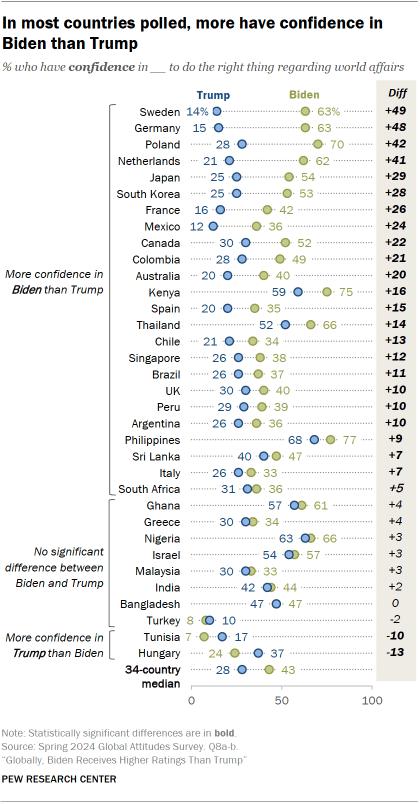 With many around the world closely following the fiercely contested rematch between U.S. President Joe Biden and former President Donald Trump, a new Pew Research Center survey finds that, internationally, Biden is viewed more positively than his rival. Across the 34 nations polled, a median of 43% have confidence in Biden to do the right thing regarding world affairs, while just 28% have confidence in Trump. The gap between ratings is quite wide in many countries, especially in Europe. Biden’s confidence rating is at least 40 percentage points higher than Trump’s in Germany, the Netherlands, Poland and Sweden. However, there are exceptions. There is no statistically significant difference in ratings of Biden and Trump in eight nations we surveyed. And people in Hungary and Tunisia give Trump more positive reviews than Biden, although neither leader gets especially high marks there. (The survey was conducted before Trump’s conviction in a state criminal trial in New York.) Even though Biden gets better assessments than Trump globally, ratings for the current U.S. president are down since last year in 14 of 21 countries where trends are available, including by double digits in Australia, Israel, Japan, Poland, South Africa, Spain, Sweden and the United Kingdom. 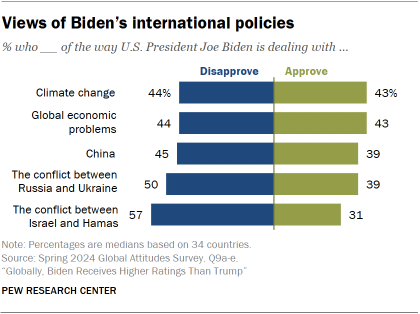 The survey included a series of questions about how Biden is handling major international issues. Overall, opinions are divided on how he is dealing with climate change and global economic problems. Across the 34 countries polled, a median of around four-in-ten approve of how Biden is dealing with China and with the war between Russia and Ukraine (39% each). The president gets his most negative reviews on his handling of the Israel-Hamas war: A median of just 31% approve of the way he is handling the conflict, while 57% disapprove. (The survey was conducted prior to Biden announcing a proposal to end the conflict .) Research in the West Bank and GazaPew Research Center has polled the Palestinian territories in previous years, but we were unable to conduct fieldwork in Gaza or the West Bank for our Spring 2024 survey due to security concerns. We are actively investigating possibilities for both qualitative and quantitative research on public opinion in the region and hope to be able to share data from the region in the coming months. Six-in-ten Israelis disapprove of how Biden is handling the war, including 53% of Jewish Israelis and 86% of Arab Israelis. (For more on how Israelis rate Biden, read “Israeli Views of the Israel-Hamas War.” ) Of the predominantly Muslim nations surveyed, large majorities in Malaysia, Tunisia and Turkey also disapprove of Biden’s handling of the Israel-Hamas war. Opinion is divided on this issue in Bangladesh. The new survey finds that overall attitudes toward the United States are generally positive: A median of 54% across the nations polled have a favorable view of the U.S., while 31% have a negative opinion. However, criticisms of American democracy are common in many nations. We asked respondents whether U.S. democracy is a good example for other countries to follow, used to be a good example but has not been in recent years, or has never been a good example. 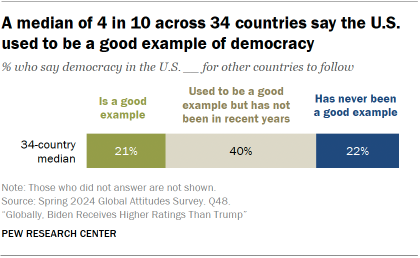 The predominant view in most countries is that the U.S. used to be a good model but has not been recently. Overall, a median of 21% believe it is currently a good example, while 22% say it has never been a good model for other countries. In eight of the 13 countries where trends are available, fewer people say American democracy is a good example than said so in spring 2021, when we last asked this question. For this report, we surveyed 40,566 people in 34 countries – not including the U.S. – from Jan. 5 to May 21, 2024. In addition to this overview, the report includes chapters on: - Attitudes toward the United States
- Ratings of Biden
- Ratings of Trump
- Views of Biden and Trump compared with other world leaders
- Views about Biden’s handling of major foreign policy issues
- Is the U.S. a good example of democracy?
Read some of the report’s key findings below. 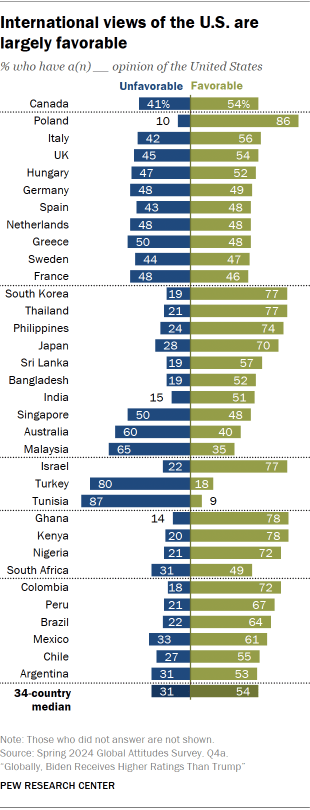 At least half of those in most countries surveyed express a favorable opinion of the U.S. Poles are the most positive, at 86% favorable. Of the European nations surveyed, ratings also lean positive in Italy, Hungary and the UK. Elsewhere in Europe, however, opinions tend to be closely divided. Attitudes toward the U.S. are largely favorable in the Asia-Pacific nations polled, especially Japan, the Philippines, South Korea and Thailand. However, most Australians and Malaysians give the U.S. poor marks. In the Middle East-North Africa region, a 77% majority of Israelis view the U.S. favorably, although this is down from 87% last year. Large majorities in Tunisia and Turkey offer an unfavorable opinion. The U.S. gets mostly positive ratings in the sub-Saharan African and Latin American nations surveyed. Two-thirds or more see the U.S. favorably in Colombia, Ghana, Kenya, Nigeria and Peru. Refer to Appendix A for long-term trends on views of the U.S. Pew Research Center has explored attitudes toward American presidents for over two decades, finding significant shifts in opinions over the years. Data from four Western European nations that we have surveyed consistently – France, Germany, Spain and the UK – shows long-term trends in views of recent presidents. George W. Bush received low and declining ratings during his time in the White House, while Barack Obama got mostly high marks. Attitudes toward Donald Trump were overwhelmingly negative throughout his presidency. Biden has consistently received more positive reviews than his predecessor, but his ratings have declined in these four countries during his time in office. 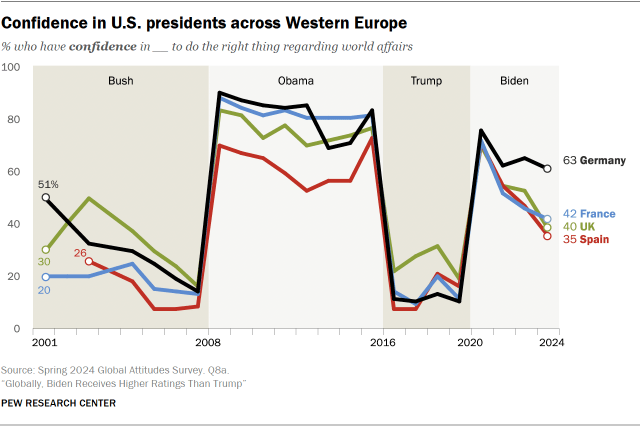 There are nine nations in this year’s survey where six-in-ten adults or more express confidence in Biden. Four are in Europe (Germany, the Netherlands, Poland and Sweden), two are in the Asia-Pacific region (the Philippines and Thailand) and three are in sub-Saharan Africa (Ghana, Kenya and Nigeria). Since last year, confidence in Biden has dropped significantly in 14 nations: Seven in Europe, plus Australia, Canada, Israel, Japan, Mexico, South Africa and South Korea. Biden gets his lowest ratings in Turkey and Tunisia, where only about one-in-ten express confidence in him. The two countries where at least six-in-ten adults have confidence in Trump are Nigeria and the Philippines. Like Biden, Trump gets one of his lowest ratings in Turkey, where just 10% view him favorably. Confidence in Trump has increased slightly in a few European countries since we last asked about him in 2020, although his ratings remain quite low in Europe. In contrast, Trump’s ratings have become more negative in Poland since 2019, which was the last year we asked about him there. Israeli views toward the former president have also become more negative over the past five years. Refer to Appendix B for long-term trends in confidence in U.S. presidents. 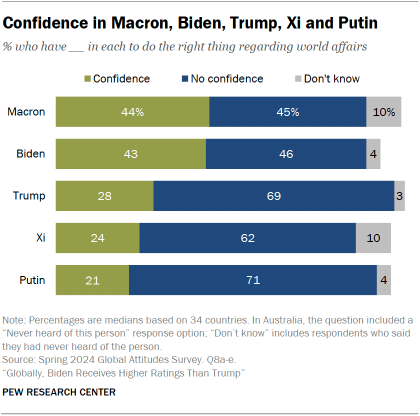 In addition to exploring confidence in Biden and Trump, the survey asked about trust in French President Emmanuel Macron, Russian President Vladimir Putin and Chinese President Xi Jinping. Overall, Macron receives the most positive ratings across the countries in the study, followed closely by Biden. The French president gets higher ratings than his U.S. counterpart in many of the European nations surveyed. Both Xi and Putin receive mostly poor marks across the countries in the study. 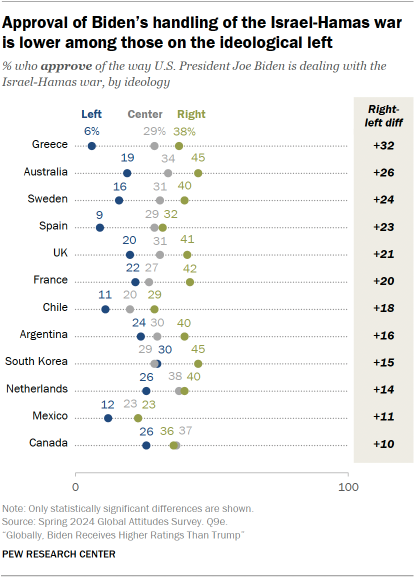 - In 17 of the 28 countries where political ideology is measured, people on the right are more likely to have a positive opinion of the U.S. than those on the left. For example, 65% of people on the right in Spain view the U.S. favorably, compared with 26% of people on the left.
- In 18 countries, people on the right are more likely to express confidence in Trump than those on the left. The gap is especially large in Israel, where 75% of those on the right have confidence in him, compared with just 23% of Israelis on the left.
- There are also some sizable ideological differences on views about Biden’s handling of the Israel-Hamas war. In several countries – including about half of the European countries surveyed – people on the right are more likely than those on the left to approve of how Biden is handling the conflict.
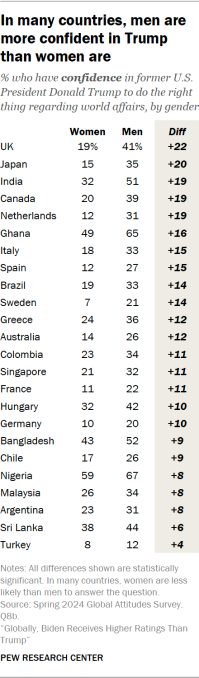 - In several countries – including Canada, all Latin American countries surveyed and several countries in the Asia-Pacific region – adults under 35 are more likely to have a positive opinion of the U.S. when compared with adults ages 50 and older. Australia, Israel and Sweden are the only countries where younger adults have a less favorable view of the U.S.
- In Canada, Australia and seven of the 10 European countries surveyed, young adults are less likely than older adults to approve of how Biden is dealing with the Israel-Hamas war .
- Men have more confidence in Trump than women do in many of the countries surveyed. The largest difference is in the UK, where men are about twice as likely as women to trust the former U.S. president. In many of the countries surveyed, women are less likely than men to answer this question at all.
Sign up for our weekly newsletterFresh data delivery Saturday mornings Sign up for The BriefingWeekly updates on the world of news & information - China Global Image
- Donald Trump
- Environment & Climate
- International Political Values
- Presidential Approval
- U.S. Global Image
- Vladimir Putin
- War & International Conflict
- World Leaders
How Americans and Israelis view one another and the U.S. role in the Israel-Hamas warIn east asia, many people see china’s power and influence as a major threat, how views of the u.s., china and their leaders have changed over time, comparing views of the u.s. and china in 24 countries, poles and hungarians differ over views of russia and the u.s., most popular, report materials. 1615 L St. NW, Suite 800 Washington, DC 20036 USA (+1) 202-419-4300 | Main (+1) 202-857-8562 | Fax (+1) 202-419-4372 | Media Inquiries Research TopicsABOUT PEW RESEARCH CENTER Pew Research Center is a nonpartisan fact tank that informs the public about the issues, attitudes and trends shaping the world. It conducts public opinion polling, demographic research, media content analysis and other empirical social science research. Pew Research Center does not take policy positions. It is a subsidiary of The Pew Charitable Trusts . © 2024 Pew Research Center 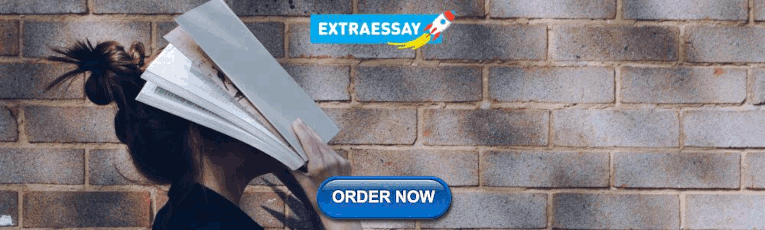 |
IMAGES
VIDEO
COMMENTS
The Philippines' economy was expected to increase gradually to 362.24 billion US dollars by 2020. By 2026, the Philippines' GDP should be $590.86. Philippines' real GDP would fall 9.5% by 2020.
Considering that GDP is one of the main indicators that influence the economic growth of a country, there are certain factors that. affect its increase or decrease. Some of the known factors are ...
observations about price movements in the Philippines: 1. Prices in the Philippines, on average, changed in about 75.6% of the months in the sample period (ie price increases and decreases). Between 1994 and M9 2019 (ie 308 months), there were 200 months when prices increased and 29 months when they decreased.
Abstract. At the start of its term, the Duterte administration reaped the benefits of the Philippines' momentum of economic growth and poverty reduction; the country's GDP continued to expand at above six percent during the 2016 to 2019 period while poverty incidence significantly declined to 16 percent in 2018.
Bank Officer V, Department of Economic Research, BSP. 3. Bank Officer V, Department of Economic Research, BSP. Price-setting Behaviour and Inflation Dynamics in ... dynamics in the Philippines, as the public have now become more forward-looking in their decision-making process. The ability of a central bank to anchor the public's inflation ...
202108 Growth During the Time of Covid19 by Renato E. Reside, Jr. ; 202107 Martial law and the Philippine economy by Emmanuel S. de Dios & Maria Socorro Gochoco-Bautista & Jan Carlo Punongbayan ; 202106 Virtues and institutions in Smith: a reconstruction by Emmanuel S. de Dios ; 202105 Accelerating Resilience and Climate Change Adaptation: Strengthening the Philippines' Contribution to Limit ...
As a response to the economic crisis brought by COVID-19, the Philippine government has started to implement its Social Amelioration Program (SAP) which aims to provide a monthly cash aid worth ...
The data used were acquired from the Philippine Statistics Authority, National Statistics Office, and Bangko Sentral ng Pilipinas. Following the Box-Jenkins method, the formulated model for forecasting the unemployment rate is SARIMA (6, 1, 5) × (0, 1, 1) 4 with a coefficient of determination of 0.79. The actual values are 99 percent identical ...
2002), Complementing use a quantitative model and micro-level data to disentangle the effects of the land reform legislation from the degree of imple-mentation, (ii) the functioning of the land market, and (iii) other changes occurring in the economy alongside the reform.
The Philippines is one of the fastest-growing economies of the past decade, averaging 6.4% growth per year over 2010-19. Indeed, an expanding and youthful population, combined with reforms and an ambitious infrastructure programme, have made it an enticing investment destination. Nevertheless, as is often the case in emerging markets, challenges regarding inequality - particularly the
agricultural productivity using a quantitative model and micro-level data before and after the reform. We study the 1988 land reform in the Philippines, known as the Comprehensive Agrarian Reform Program (CARP). CARP was an extensive land redistribution program that imposed a restrictive
THE PHILIPPINE REVIEW OF ECONOMICS Volume LIV No. 2 December 2017 June and December 2019 THE PHILIPPINE REVIEW OF ECONOMICS Volume LIV No. 2 December 2017 Volume LVI Nos. 1 and 2Volume LIV No. 2Volume LIV No. 2 ISSN 1655-1516 December 2017 A joint publication of the University of the Philippines School of Economics and the Philippine Economic ...
International Journal of Economics, Business and Management Research Vol. 2, No. 01; 2018 ISSN: 2456-7760 www.ijebmr.com Page 61 MICROFINANCE IN THE PHILIPPINES: A TOOL FOR ECONOMIC DEVELOPMENT, OR PERPETUAL DEBT? EVIDENCE OF ITS SUCCESS AND CHALLENGES IN THE PROVINCE Bryan W. Rich Research Fellow Palawan State University, Philippines Abstract
Abstract. Philippines is one of the fortunate countries that can generate viable coffee bean varieties. Local coffee output is declining, while market demand is growing. This research looks at the supply of coffee in both domestic and imported producers over a specified time period in order to develop a supply curve pattern that can be use as ...
Economic and Financial Data of the Philippines. ... The Philippines is using the "as relevant" flexibility for this data and will publish wage rates statistics with a periodicity of two years and a timeliness of 12 months. 4/ January - November 2005. The data include only the financing of the national government, and the 14 monitored non ...
This research uses annual time series data on inflation rates in the Philippines from 1960 to 2017, to model and forecast inflation using ARIMA models. Diagnostic tests indicate that P is I (1). The study presents the ARIMA (1, 1, 3). The diagnostic tests further imply that the presented optimal ARIMA (1, 1, 3) model is stable and acceptable ...
The Coronavirus Disease 2019 (COVID-19) is inevitably affecting remittance-dependent countries through economic downturns in the destination countries, and restrictions on travel and sending remittances to their home country. We explore the potential impacts of the COVID-19 pandemic on the welfare of remittance-dependent households using a dataset collected in the Philippines prior to the ...
An Analysis on the Economic Factors Affecting the Unemployment Rate in the Philippines from 1993-2018 Caligagan, Angelica Anne M. 1 , Ching, Roby Rafael O. 2 and Suin, Kristine April 3
Micro Business Enterprises (MBEs) is the lifeblood for economic development. Using a descriptive quantitative research design, the study aimed to investigate the impact of MBEs to economic development. As to impact, in general, it was of a moderate extent. Among the indicators, technology has the highest mean interpreted to a high extent, while infrastructure development scored the lowest mean ...
COVID-19 dynamics in the Philippines are driven by age, contact structure, mobility, and MHS adherence. Continued compliance with low-cost MHS should help the Philippines control the epidemic until vaccines are widely distributed, but disease resurgence may be occurring due to a combination of low population immunity and detection rates and new variants of concern.
Offered by the School of International Service , the International Economic Relations: Quantitative Methods (MA) offers advanced training in econometrics and quantitative methods and gives students an in-depth understanding of the economic, political, and social forces that shape relations among countries and their governments within the global economy, as well as of the private and official ...
She is also the macroeconomics and fiscal management expert behind the Growth and Productivity Report and the Philippines Economic Update, which reviews salient socioeconomic issues and provides growth forecasts twice a year. ... Journal of Financial & Quantitative Analysis, Management Science, Operations Research, Review of Finance), 21 in ABS ...
Practical Research II Final - Free download as Word Doc (.doc / .docx), PDF File (.pdf), Text File (.txt) or read online for free. This document outlines a research study on the perceptions of Grade 12 students at University of Luzon regarding inflation rates in the Philippines. The study aims to understand students' perceptions as well as gather recommendations on how to decrease inflation.
Flaim (1990) explained the growing population Journal of International Business, Economics andEntrepreneurship e-ISSN :2550-1429 Volume 4 (1) June 2019 in the United States during the 60s to 80s ...
The country's benchmark CSI 300 index, which tracks China's top 300 stocks, has risen 8 per cent over the same time period, according to research provider Simu Paipai.
Quantitative research gathers information that can be counted, measured, or rated numerically - AKA quantitative data. Scores, measurements, financial records, temperature charts and receipts or ledgers are all examples of quantitative data. ... finance and economics. It's easy to 'crunch the numbers' of quantitative data and produce ...
The research method followed a quantitative design and the 61-item survey included basic demographic questions, three validation check questions, and three scales: vanity, materialism, and general ...
Pew Research Center has polled the Palestinian territories in previous years, but we were unable to conduct fieldwork in Gaza or the West Bank for our Spring 2024 survey due to security concerns. We are actively investigating possibilities for both qualitative and quantitative research on public opinion in the region and hope to be able to ...