Advertisement
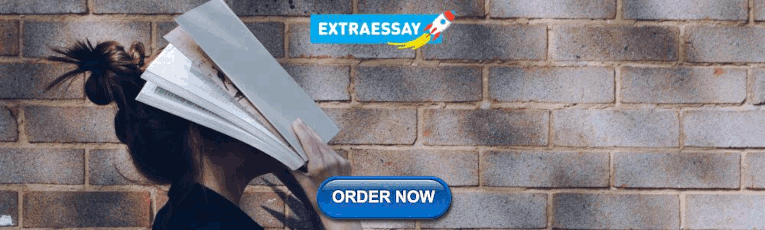
Empirical studies of factors associated with child malnutrition: highlighting the evidence about climate and conflict shocks
- Original Paper
- Open access
- Published: 21 May 2020
- Volume 12 , pages 1241–1252, ( 2020 )
Cite this article
You have full access to this open access article
- Molly E. Brown ORCID: orcid.org/0000-0001-7384-3314 1 ,
- David Backer 2 ,
- Trey Billing 2 ,
- Peter White 3 ,
- Kathryn Grace 4 ,
- Shannon Doocy 5 &
- Paul Huth 2
14k Accesses
26 Citations
37 Altmetric
Explore all metrics
Children who experience poor nutrition during the first 1000 days of life are more vulnerable to illness and death in the near term, as well as to lower work capacity and productivity as adults. These problems motivate research to identify basic and underlying factors that influence risks of child malnutrition. Based on a structured search of existing literature, we identified 90 studies that used statistical analyses to assess relationships between potential factors and major indicators of child malnutrition: stunting, wasting, and underweight. Our review determined that wasting, a measure of acute malnutrition, is substantially understudied compared to the other indicators. We summarize the evidence about relationships between child malnutrition and numerous factors at the individual, household, region/community, and country levels. Our results identify only select relationships that are statistically significant, with consistent signs, across multiple studies. Among the consistent predictors of child malnutrition are shocks due to variations in climate conditions (as measured with indicators of temperature, rainfall, and vegetation) and violent conflict. Limited research has been conducted on the relationship between violent conflict and wasting. Improved understanding of the variables associated with child malnutrition will aid advances in predictive modeling of the risks and severity of malnutrition crises and enhance the effectiveness of responses by the development and humanitarian communities.
Similar content being viewed by others
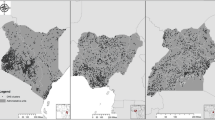
Conflict and Climate Factors and the Risk of Child Acute Malnutrition Among Children Aged 24–59 Months: A Comparative Analysis of Kenya, Nigeria, and Uganda
Kathryn Grace, Andrew Verdin, … Trey Billing
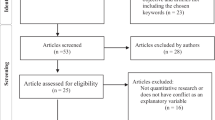
Conflict and Child Malnutrition: a Systematic Review of the Emerging Quantitative Literature
Maria Sassi & Harshita Thakare
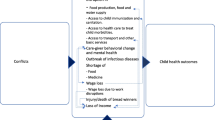
Exposure to conflict and child health outcomes: evidence from a large multi-country study
Srinivas Goli, Astghik Mavisakalyan, … Loan Vu
Avoid common mistakes on your manuscript.
1 Introduction
Malnutrition is preventable, yet remains a major public health challenge. This condition affects one in five children and contributes to nearly half of all deaths during childhood globally (Black et al. 2013 ). Children who have poor nutrition during their first 1000 days of life attain lower levels of education and have lower work capacity and productivity as adults. Malnourished children also face increased likelihoods of being overweight, of developing chronic illnesses such as cardiovascular disease, diabetes and cancer, and of suffering from mental health issues later in life (Haddad et al. 1994 ; Hoddinott et al. 2013 ). After having suffered of malnutrition during early childhood, girls face increased likelihoods of having children that are born too early or underweight (UNSCN 2010 ).
Given the serious repercussions for survival, health, and well-being, anticipating and addressing the circumstances under which children become malnourished is vital. Various development and humanitarian interventions focus on fostering healthy communities where children are better protected and able to recover from nutrient deficits (Collins et al. 2006 ). To facilitate those interventions, assessments of food security conducted by organizations such as the Famine Early Warning Systems Network (FEWS NET) and the Integrated Food Security Phase Classification (IPC) initiative have sought to project the future status of at-risk countries and issue alerts about impending and ongoing crises months in advance, aiming to ensure enough lead time for the coordination and implementation of appropriate responses (Brown et al. 2007 ; Funk et al. 2019 ; IPC 2012 ). Assessments that focus on early warning have advantages relative to relying on measuring the prevalence of malnutrition in a community, which can detect a crisis only after it emerges (Maxwell et al. 2020 ). Assessments such as FEWS NET and IPC, however, do not gauge, much less substantiate, associations between malnutrition at an individual level and relevant factors. Statistically modelling these empirical relationships is integral to detecting vulnerabilities, diagnosing their sources, and directing assistance.
In this article, we consolidate what has been learned from published studies that used statistical analysis of empirical data to examine relationships between malnutrition among children and a large array of individual-, household-, community-, regional- and national-level variables. Our literature review is guided by two main questions: (1) Which variables were consistently associated with child malnutrition? (2) What types of quantitative empirical data and statistical methods have been used to analyse the nature of the relationship between these variables and child malnutrition? Answering these questions results in a summary of drivers of malnutrition, clarifies the strengths and limitations of existing studies, and suggests potential directions for further research, which may include a formal meta-analysis.
Our review considers studies in which the outcomes of interest included at least one of three major indicators of malnutrition, formalized in international standards (WHO 2020 ). Wasting (low weight for height) indicates an acute decline in nutritional status experienced by a normally well-nourished child. This decline usually involves rapid and substantial weight loss. Stunting (low height for age), by contrast, indicates a chronic, long-term nutritional deficit, the effects of which are potentially irreversible (Kennedy et al. 2015 ). Children who suffer wasting regularly over time may also develop stunting (Hoddinott et al. 2008 ). Underweight (low weight for age), on the other hand, can reflect wasting, stunting, or both (WHO 2010 ).
The breadth of our review provides a more expansive picture of the findings from the empirical research about malnutrition, according to statistical modelling of quantitative data. In the process, we can compare the state of knowledge about factors associated with the different indicators of malnutrition. Our expectation is that the findings of the review will improve awareness of which factors yield consistent findings and emphasize how particular relationships can vary across different measures of malnutrition.
At the same time, we have a specific interest in the variables associated with wasting. The acute nature of this condition presents a distinct challenge in practice. Prompt, effective interventions, with the potential to mitigate the risk of wasting, depend on the existence of reliable guidance about factors that tend to be associated with changes in individuals’ nutritional status in the short term. Insights from such a review may help inform interventions that are focused on reversing weight loss trajectories in children before malnutrition becomes a persistent condition.
Finally, we spotlight the role of external shocks experienced by individuals, households and communities, especially those caused by exposures to environmental and societal forces. Disasters due to climate extremes (e.g., drought) and violent conflict (e.g., civil war) are regularly attributed as the primary causes – acting independently and in interaction – of crises such as famines resulting in prevalence spikes in the rate of acute malnutrition. The urgency of understanding the role of these shocks has been magnified as complex emergencies are becoming more common and lasting longer (Lautze and Raven-Roberts 2006 ; Young et al. 2004 ). Policies resulting in timely action are needed to reduce the impact of these crises on children, families, and the communities in which they live (Ghobarah et al. 2003 ; Hillbruner and Moloney 2012 ).
We start by presenting the methods we used to isolate and code relevant studies that were included in our review. Next, we summarize the results of our review. In the concluding section, we discuss the results in the context of the broader literature, with particular attention to studies about the relationship between malnutrition and climate extremes, conflict events, and their interactions.
We conducted a search of the American Economic Association’s EconLit database in January 2019. EconLit indexes six types of materials: journal articles, books, book chapters, dissertations, working papers, and book reviews. The coverage features nearly 1 million articles from over 1000 journals published in 74 countries, dating from 1969 to the present ( https://www.aeaweb.org/econlit/content ). Many of the topics covered by the material indexed in EconLit relate to child malnutrition, including Health and Economic Development (JEL code I15), Health and Inequality (JEL code I14), Health, Government Policy, and Regulation (JEL code I16), Welfare, Wellbeing and Poverty (JEL code I3), Fertility, Family Planning, Child Care, Children, and Youth (JEL code J13), Agricultural Economics (JEL code Q1), and Renewable Resources and Conservation (JEL code Q2) (see http://www.aeaweb.org/econlit/jelCodes.php for a complete list of topics covered by EconLit). We sought to identify articles indexed by EconLit that quantitatively assess potential factors, with explicit statistical tests, in relation to child malnutrition. We therefore conducted separate searches using “malnutrition,” “wasting,” “wasted,” “stunting,” “stunted,” “underweight,” and “undernourishment” as key words. In addition, we paired each of these key words with “child” when conducting searches. In total, the searches yielded a set of 688 articles.
Within this set, we then selected articles relevant to our review. We therefore searched for any mentions of “child wast*” (166 articles), “child stunt*” (104 articles), and “child under*” (39 articles) in the article titles and abstracts. Certain articles referenced multiple search terms. We thus selected 209 potentially relevant articles. Finally, we scanned the titles and abstracts of these remaining articles with the following criteria:
use of quantitative and/or numerically coded qualitative data within a statistical analysis.
dependent variable(s) in the analysis must be some variant(s) of child malnutrition.
testing of statistical relationship between child malnutrition and one or more independent variables.
Some of the reasons for excluding articles include:
article not published in an English-language academic journal (for reasons of feasibility in conducting the review).
title and/or abstract indicating that the study is unrelated to our review (e.g., food waste).
title and/or abstract indicating that the study is peripheral to our objective (e.g., global narratives regarding child health).
title and/or abstract not mentioning quantitative data analysis.
quantitative analysis referred to in abstract concerning adult malnutrition Articles evaluating adult nutrition (e.g., of the mother during pregnancy) as a factor for child malnutrition were retained.
quantitative analysis referred to in title and/or abstract concerning not concerning relationships between child nutrition prevalence and any of its factors.
quantitative analysis referred to in the title and/or abstract considering child malnutrition as an independent, and not a dependent variable.
Our approach using the inclusion and exclusion criteria yielded a sample of 61 articles from EconLit.
We further augmented the sample with 29 articles from beyond the EconLit database. These additional articles: (1) cited articles from the EconLit sample, (2) were cited by those articles, and/or (3) involved authors of those articles. All the additional articles satisfied the inclusion and exclusion criteria stated above. The final sample therefore consists of a total of 90 articles (see supplementary Table S 1 for details).
2.1 Coding of article variables
Information pertaining to each article was coded according to the following: the data used (location, timing, panel vs. cross-section, sample size); statistical methods of analysis; and the dependent and independent variables considered. Only the main statistical results in a given article were coded; other results (e.g., exploratory subgroup tests, robustness checks, and sensitivity analyses) were not included. Dependent variables were grouped into three categories: wasting (W, continuous weight-for-height z-score and/or binary indicator for wasted), stunting (S, continuous height-for-age z-score and/or binary indicator for stunted), and underweight (U, continuous weight-for-age z-score and/or binary indicator for underweight) (de Onis and Blössner 2003 ). Each combination of a dependent variable and an independent variable in a given article was coded with both the sign and the level of reported statistical significance of the relationship as evaluated in the analysis. Most of the reviewed studies focus on the sign of relationships; few studies pay close attention to the magnitude of effect sizes. P -values were not always reported in all articles, reflecting differences in standards across journals and fields. The coding categorized each relationship as significant if the p value was smaller than or equal to 0.05. These instances were marked as 1. All other instances were marked as 0. The coding also noted instances of p -values less than (or equal to) 0.01 and 0.001 (supplementary materials; (Finlay and Agresti 1986 )). Supplementary Table S 2 provides a list of all variables reported in all 90 reviewed articles, along with their reported statistical significance.
Across the sample of reviewed studies, more than 300 independent variables were found. Independent variables about the same factor, even if operationalized differently, were consolidated into a factor category to facilitate comparison across studies (Phalkey et al. 2015 ). Supplementary Table S 3 lists all variables analysed in the reviewed studies that comprise our factor categories, and which papers they came from.
The findings of the studies included in our review enabled each factor to be characterized as follows:
Risk factor – a majority of reviewed studies examining a given type of malnutrition report a significant ( p ≤ 0.05) positive relationship with the independent variables (i.e., a greater extent or probability of malnutrition as a function of increasing values of the independent variable).
Mitigating factor – a majority of reviewed studies examining a given type of malnutrition report a significant (p ≤ 0.05) negative relationship with the independent variables (i.e., a lower extent or probability of malnutrition as a function of increasing values of the independent variable).
Inconclusive factor – a majority of studies examining a given type of malnutrition report either an inconsistent sign of the relationship with the independent factor, or a relationship that is not statistically significant ( p > 0.05).
In order to facilitate comparison and the policy and other practical applications of the analysis, factors were grouped according to the scale they concern: child, household, region/community, or country (Smith et al. 2005 ).
Relationships between a given type of malnutrition and a given factor may have been evaluated in only one study. While all relationships appearing in the main statistical analysis of each study were coded and documented (Table S2 ), only factors evaluated in multiple studies were reported in the results. All studies were treated equally, regardless of scope, scale of the analysis, magnitude of effect sizes, and level of significance reported. The results of the analysis offer a general summary and mapping of results to capture patterns in the existing research. No statistical assessment of the importance of factors across publications (“effect size” in the meta-analysis literature) is provided in the interest of reflecting the broadest possible sample of studies.
Wasting and underweight have been studied less often than stunting (Table 1 ). Just over 34% of the reviewed studies modelled wasting. Slightly more studies operationalized this outcome with a binary variable (whether or not children were wasted, as a status based on exceeding a given threshold) than with a z-score (extent of deviation from international standards, along a continuous scale that captures a spectrum of outcomes in a process of becoming undernourished). Under 5% of the reviewed articles used both operationalizations of wasting in their analysis. Similarly, underweight appeared in 34% of the reviewed studies. In these studies, the operationalization was most often a z-score, rather than a binary variable. Meanwhile, 81% of the articles used stunting as a dependent variable; a z-score was most common for stunting as well.
3.1 Factors evaluated as affecting child nutrition
A total of 49 factors were evaluated in relation to wasting, stunting, and/or underweight by multiple studies (Fig. 1 ). This list includes 12 factors measured at the individual level; 25 factors measured at the household level (including five factors pertaining to mothers); eight factors measured at the region/community level; and four factors measured at the country level (Table S3 ). Analysis of disaggregated data at the individual and/or household level featured in 89% of the reviewed articles. Most analyses did not include any covariates measured at the regional/community or country levels (e.g., (Ekbrand and Halleröd 2018 )). Thus, fewer articles are available with which to evaluate the consistency of relationships of factors at the regional/community and country levels than at the individual and household levels. Of the 49 factors, 18 have been evaluated by multiple studies in relation to each of the three standard measures of child malnutrition (Fig. 1 ). The subsequent presentation of results is restricted to instances of prevailing evidence of statistically significant relationships indicating risk factors or mitigating factors, according to a majority of relevant reviewed studies.
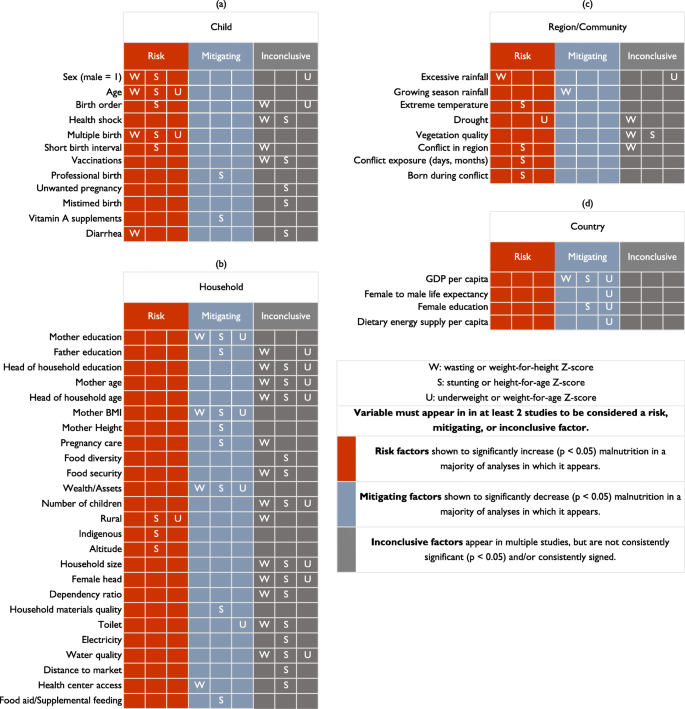
Summary of results from statistical analyses of relationships between indicators of child malnutrition and: a child-specific factors, b household-level factors, c region/community-level factors, and d country-level factors. Note: We limit the results reflected in this figure to factors that are evaluated in the main statistical analyses reported in at least two of the 90 reviewed articles. Details of which study was included in each factor can be found in Supplementary Table S 3
Eight of the 12 factors measured at the level of individual children exhibited statistically significant relationships for the following factors: child’s sex and age, if they were a multiple at birth (twin, triplet, etc.), and diarrhea status (Fig. 1a ). Seven of the 10 factors evaluated in relation to stunting exhibited statistically significant associations. These associations identified five risk factors: child’s sex and age, their birth order, if they were a multiple at birth, and short birth interval. Two mitigating factors were also identified: if a professionally trained assistant was present at the birth and if Vitamin A supplements had been used. The results indicated that two of the four factors evaluated in relation to underweight were statistically significant risk factors: child’s age and if they were a multiple at birth. According to our review, therefore, all three anthropometric measures of malnutrition were associated with two individual-level risk factors: age and multiple at birth.
Of the 25 household-level factors, just four of the 17 factors exhibited statistically significant associations: mother’s education, mother’s BMI, wealth/assets, and access to a health care center (Fig. 1b ). All were evaluated as being mitigating factors. Eleven of the 25 factors evaluated in relation to stunting yielded statistically significant associations. The relationships identified three risk factors: rural, indigenous, and altitude. In addition, eight mitigating factors were identified: mother’s education, father’s education, mother’s BMI, mother’s height, pregnancy care, wealth/assets, quality of household materials, and food aid or supplemental feeding. Five of the 13 factors evaluated in relation to underweight yielded statistically significant associations. Only one relationship identified a risk factor: rural residence. Four mitigating factors were also identified: mother’s education, mother’s BMI, wealth/assets, and quality of toilet. According to our review, therefore, all three anthropometric measures were associated with three household-level risk factors: mother’s education (either years of education or specific levels relative to no education), mother’s BMI, and wealth/assets (encompassing different indices).
The eight factors measured at the region/community level is split between measuring features of the environment, including climate conditions, and features related to conflict (Fig. 1c ). Wasting had a statistically significant association with excessive rainfall as a risk factor and growing season rainfall as a mitigating factor. Stunting had a statistically significant association with extreme temperatures as a risk factor. Underweight only exhibited a statistically significant association with drought as a risk factor. Several of the reviewed studies analysed vegetation quality, employing either the normalized difference vegetation index (NDVI) or the enhanced vegetation index (EVI), with varying operationalizations. In particular, vegetation quality during the previous growing season has been evaluated in multiple studies of both wasting and stunting, yielding findings that vary by context. Statistically significant associations were observed between stunting and three factors that reflect distinctive operationalizations of the role of conflict. Conflict in the surrounding region, conflict exposure (days or months), and whether a child was born during a conflict were all identified as risk factors for stunting.
At the country level, national per capita GDP was identified as a mitigating factor for wasting, stunting, and underweight (Fig. 1d ). Female education (encompassing national rates of female literacy and female secondary enrolment) was identified as a mitigating factor for stunting and underweight. Both the national average female-to-male life expectancy ratio and the dietary energy supply per capita were identified as mitigating factors for underweight.
3.2 Statistical methods
About 60% of the reviewed studies employed standard variations of multivariate regression techniques, such as linear, generalized linear (e.g., logit), or multilevel models. Only 5% of studies used explicit multilevel statistical techniques, modelling simultaneously the relationships between malnutrition and covariates at the individual, household, and regional/community levels (e.g., (Ekbrand and Halleröd 2018 )). Other studies that did not estimate multilevel models instead included covariates aggregated to higher levels, introduced dummy variables for geographic regions, or adjusted for within-spatial-unit correlation via clustered standard errors (e.g., (Rashad and Sharaf 2018 )). Five articles used quantile regression, which fits a model through quantiles of the dependent variable, rather than the mean (e.g., (Asfaw 2018 )). This approach has the advantage of allowing for heterogeneous treatment effects for different segments of the distribution of child malnutrition. For example, a given factor may exhibit a stronger association with weight-for-height z-scores for children who are undernourished (i.e., the left tail of the distribution), relative the association observed for children whose nutrition status is near the center of the distribution.
A majority of reviewed studies relied on cross-sectional analysis of either data from single surveys or a pooled dataset comprising multiple cross-sectional surveys. Just five of the studies capitalized on panel data involving repeated waves of data collection for the same children or households over time. The remaining studies employed a diversity of approaches, including time-series analysis of repeated cross-sections of countries or subnational regions. Among the reviewed studies, the most common source of malnutrition measures was Demographic and Health Survey (DHS) data (27 studies). Five of the reviewed studies used Living Standards and Measurements Survey (LSMS) data. The remaining studies employed other country-specific surveys, with India’s National Family Health Survey (4 studies) and Ethiopia’s Rural Household Survey (2 studies) featuring in multiple cases.
In terms of causal identification strategies, 17% of the reviewed studies directly leveraged the availability of data collected from repeated measurement over time, estimating either unit-level fixed effects or difference-in-differences models (e.g., (Lucas and Wilson 2013 )). A further 9% of articles featured an instrumental variables strategy (e.g., (Yamano et al. 2005 )) and another 6% of articles resorted to matching techniques (e.g., propensity score) to control for selection bias and minimize problems of sample imbalance. The remaining studies exhibited a variety of other approaches, including decomposition analysis (Block et al. 2004 ; Rodriguez 2016 ) and a regression discontinuity design (Ali and Elsayed 2018 ).
Among the reviewed studies, attention to the temporal relationship between malnutrition and potential factors was limited and uneven, constraining the ability to ascertain any general patterns. The lack of such examination of the impact of climate and conflict shocks is especially conspicuous. A common approach has been to measure deviations in conditions during the survey period relative to long-run average conditions, within a suitable sub-national geographic area surrounding the survey cluster. The implicit assumption is that the deviations in conditions exert a contemporaneous impact on malnutrition. Select studies used models specifying factors with time lags. For example, Johnson and Brown (Johnson and Brown 2014 ) tested one- and two-year lagged measures of shocks in vegetation, but the results of these estimations were not presented because the observed effects were not statistically significant. Kinyoki et al. ( 2016 ) tested lags measures of conflict during the three months prior to survey and the period from 3 to 12 months prior to the survey, finding that both variables have statistically significant associations with wasting and stunting. Howell et al. (Howell et al. 2018 ) tested yearly lagged values of conflict days and deaths in an analyses of stunting and wasting. Another approach in studies that have modelled the effects of conflict shocks on child malnutrition is cohort analysis. The effect of the shock is gauged based on birth timing relative to the shock, evaluating how the “during” shock cohort differs from the “before” shock and “after” shock cohorts (Grace et al. 2015 ).
3.3 Geographical coverage
Nearly 80% of the reviewed studies focused on a single country or even just one sub-national geographic area within a country. The country that features the most often was India, in 13% of the studies. Ethiopia was second (10%), followed by Guatemala and Kenya (6% each). Nineteen of the reviewed studies (21%) analysed data from multiple countries. The studies with the most extensive geographic scope covered 166 countries (Smith and Haddad 2015 ), 63 countries (Smith and Haddad 2001 ), and 41 countries (Kimenju and Qaim 2016 ). The analysis in each of these studies was conducted at the country level.
The shortage of comparative analysis within individual studies, the limited scope of geographic coverage among multiple studies that examined the same factors, and the lack of comparability of the studies that did examine the same factors in different country settings restricts understanding of the generalizability of observed relationships. Of interest, no comparative studies have been conducted to analyse the consistency of the relationship between child malnutrition and conflict across multiple country settings.
4 Discussion and conclusions
We conducted a review of 90 studies involving statistical analyses of empirical data to examine relationships of child malnutrition to factors measured at the individual, household, regional/community and national levels. Our main purpose was to consolidate understanding about the tendencies of findings to date and the design and extent of existing research. A main strength of our review was the wide scope, with respect to the number of studies included, the multiple measures of malnutrition reflected in the analyses reported in these studies, and the volume of factors evaluated in those analyses. The review by Phalkey et al. (Phalkey et al. 2015 ) takes a similar approach on fewer (15) studies and considered seven categories (agriculture, crops, weather, livelihood, demographics, morbidity, and diet). These categories were simply reported statistically significant, or not (Phalkey et al. 2015 ). The present review achieves a broader coverage of the literature, includes description of risk and mitigating factors, and highlights possible differences in relationships across the types of malnutrition. The approach used in the present review departs from a formal meta-analytical approach (Borenstein et al. 2011 ). Our approach allowed us to include a large number of studies, irrespective of statistical designs, choice of variables, and modelling approaches. Meta-analysis published elsewhere will be useful in confirming trends reported here.
Specifically, our review reveals that wasting is understudied as a measure of child malnutrition. Instead, far more attention has been paid to stunting. Another main observation is that many of the factors evaluated in relation to the different types of child malnutrition yielded inconclusive results or were not analysed in multiple studies. According to the prevailing evidence, select factors were associated with all three types of child malnutrition: age of child and multiple births are risk factors, while mother’s education, mother’s BMI, household wealth/assets, and national GDP per capita are mitigating factors. A single factor is associated with both wasting and stunting (child’s sex as a categorical variable) and two factors with both stunting and underweight (rural household, national female education level), while 23 factors are associated with only one of the types of malnutrition.
Previous research summarising determinants of child nutritional status identified factors similar to those in our review. For example, Smith et al. (Smith et al. 2005 ) list a number of individual- and household-level factors that seem important to nutritional status, including whether the child has had diarrhoea, mother’s education, mother’s nutritional status, feeding practices, sanitary conditions, wealth, and medical care. Many of these factors exhibit significant associations in the studies included in our review, which identifies other risk and mitigating factors as well.
The present review explicitly captures findings about the role of climate and conflict conditions, while other recent reviews about child malnutrition overlook these conditions (Jones et al. 2013 ; Leroy et al. 2015 ; (Wrottesley et al. 2015 )). Among the studies we reviewed, climate conditions are widely included in analyses, most often measured with indicators of precipitation, temperature, and vegetation. Measures of conflict conditions – activity in the surrounding region, extent of exposure, and birth during an affected period – are also included more selectively in analyses (Akresh et al. 2012 ; Delbiso et al. 2017 ). The prevailing evidence indicates that climate shocks involving excessive rainfall, extreme temperatures, and drought are among the risk factors for wasting, stunting, and underweight, respectively, while conflict emerged as a risk factor for stunting. Additional relationships between certain types of malnutrition and certain forms of external shocks may exist, but the evidence from our review is either inconclusive or only reflects single studies (Table S2 ).
Our results are consistent with existing research showing that climate-related shocks, such as droughts or floods, are detrimental to food security, especially of rural populations (Table S3 ) (Cooper et al. 2019 ; Douxchamps et al. 2016 ; Grace et al. 2014 ; Murali and Afifi 2014 ). Our review reveals that excessive rainfall is a risk factor and growing season rainfall is a mitigating factor. Excessive rainfall represents an extreme event, with the potential for natural disasters (e.g., floods) that can be damaging to health, well-being, and economic production. By comparison, growing season rainfall captures conditions during critical periods of agricultural productivity, when above-average precipitation logically tends to be beneficial to food security (Cooper et al. 2019 ; Funk et al. 2008 ). Another study found that households located in regions that experienced a drier-than-average year reported one more month of food insecurity than households experiencing wetter-than-average years (Niles and Brown 2017 ). Our review also found that stunting was associated with both extremely cold temperatures (Skoufias and Vinha 2012 ) and extremely hot temperatures (Jacoby et al. 2014 ) as risk factors. Other existing studies suggest that high temperatures and heat waves tend to be important for understanding food security (Phalkey et al. 2015 ; Bain et al. 2013 ; Grace et al. 2012 ). In addition, we found that the results for vegetation quality differ across countries. For example, Johnson and Brown (Johnson and Brown 2014 ) find vegetation quality during the previous growing season to be a statistically significant mitigating factor for wasting in Mali, but not Benin, Burkina Faso, or Guinea, while Shively et al. (Shively et al. 2015 ) did not find a statistically significant relationship between wasting and this factor in Nepal.
In comparison to the literature using climate variables, analyses of relationships between child malnutrition and conflict shocks are limited in number. Foundations for such studies exist in the literature about the effect of conflict on food security and public health. For example, Akresh et al. ( 2011 , (Akresh et al. 2012 )) and Bundervoet et al. ( 2009 ) showed that children in conflict-affected settings exhibit signs of stunting, with similar effects for children born before or during wartime. These results, however, have not translated into interventions that take advantage of data on climate and conflict, despite the increasing availability of sources with granular detail (Dunn 2018 ; Jones et al. 2010 ; Raleigh et al. 2010 ). Our review also highlights the lack of attention in existing research to the relationship between conflict and wasting. We view this gap as warranting attention given the acute nature of this type of malnutrition, which could plausibly be influenced by the sort of shock that conflict represents.
Another important consideration is that climate and conflict shocks can coincide – and influence one another. These potential intersections and interactions suggest possible causal pathways of child malnutrition. Multiple theories address the impact of climate shocks on the emergence of armed conflict, which cannot be decisively established (Hsiang and Burke 2014 ). Food security and nutrition may also be a key mediating factors in the nexus between climate and conflict. For example, Buhaug et al. (Buhaug et al. 2015 ) model a process in which climate shocks cause in a first stage to an increase of local food prices, which then lead to conflict during a second stage. They find evidence of a strong climate impact at the first stage, but a weak and inconsistent one at the second stage. Their argument is that the effect of food prices on conflict is likely conditional on local “socioeconomic and political contexts.” This explanation is consistent with findings of studies on the relationship between food prices and urban unrest (Berazneva and Lee 2013 ; Hendrix and Haggard 2015 ).
Our review indicates that empirical research evaluating the joint effects of environmental and political conditions on risks of malnutrition unfortunately remains the exception (Fig. 1 and Tables S 2 and S 3 ). Few studies on malnutrition have considered both climate and conflict simultaneously. A meta-analysis of nutrition surveys in Ethiopia from 2000 to 2017 concluded that droughts increase the prevalence of wasting, but the impact of conflict is less certain (Delbiso et al. 2017 ). Other research suggests that the effects of climate extremes and conflict should exacerbate one another, pushing conditions beyond a tipping point and contributing to complex emergencies. In the famines of the twentieth Century caused primarily by drought or flood, concurrent conflict often served to escalate the environmental crisis and exacerbate mortality (Devereux 2000 ). This finding is consistent with Sen’s (Sen 1981 ) seminal argument that no famines with exclusively natural causes have been observed in the modern era. Sen (Sen 1981 ) argues that contemporary societies should be able to respond more effectively to potential famines caused by droughts or other natural events, except when hampered by failures in social, economic, and political institutions. Crises arising with one type of shock may be eased by stored food or relief supplies, whereas the coincidence of both types of shocks undermines those responses. For example, conflict blocked aid from reaching populations at risk of malnutrition amid droughts in Ethiopia during the 1980s and in Somalia in 2011 (Hillbruner and Moloney 2012 ; Maxwell and Fitzpatrick 2012 ). More generally, conflict diminishes the capacity of households and communities to cope with other stresses and shocks (Raleigh et al. 2015 ).
Among the main challenges to achieving improvements in the detailed, rigorous analysis of relationships between child malnutrition and conflict is the availability of data. Surveys that serve as the source of data on nutrition are conducted in conflict-affected areas. The insecure nature of these conditions, however, can make data collection less frequent, extensive, and reliable, reducing their scope, scale, and quality. Also, the relevant surveys rarely collect information about direct conflict exposure at the individual or household level. Instead, studies that evaluate conflict as a factor usually resort to making inferences from analysis using event data. These data offer consistent precision of georeferencing of events only to the level of first- or at best second-order administrative divisions (Raleigh et al. 2010 ), rather than specific point locations or even small areas. Appropriately integrating these data on conflict into analysis requires a multilevel modelling approach (Gelman and Hill 2006 ), which can account for potential influences at the regional level, as well as other levels (individual, household, community). Such an approach can be complemented by reasonable theoretical arguments that children residing within regions experiencing conflict (and possibly affected neighbouring regions) are more vulnerable to suffering effects on malnutrition, through various causal pathways. Given that the direct exposure to conflict events is not measured, compounded by events being infrequent in most settings, the evaluated relationships are likely to be difficult to detect. Encountering such difficulties in the analysis of climate factors is less likely because of the greater geographic granularity of the available data, a more balanced distribution of conditions, and clearer, more direct pathways of influence of local conditions on individuals and households.
Ultimately, examining the state of knowledge about factors associated with acute and chronic child malnutrition holds the potential to help advance an ongoing agenda of scientific inquiry with practical applications that have important real-world consequences. Recent technological developments in mobile devices and remote sensing, communications coverage (including cell phone and Internet networks), and the ability to transmit large amounts of information rapidly improve the potential of designing and implementing more timely protective interventions (GSMA 2015 ). Considerable opportunities exist for identifying where, when, how, and why proven policy and public health interventions should be implemented (Collins et al. 2006 ), especially to gauge the local impact of climate and conflict shocks. Our review contributes to capabilities of isolating intervention points in ways that can improve strategies (Wrottesley et al. 2015 ; Walker et al. 2015 ). Further evidence from studies spanning multiple countries and time periods is needed to bolster the foundations for designing interventions (Dilley 2000 ). Pertinent data are increasingly available, including from sources (e.g., the World Food Program’s Food Aid Information System) that can be used to study the effectiveness of international aid and humanitarian assistance in relation to vulnerabilities to malnutrition.
Akresh, R., Lucchetti, L., & Thirumurthy, H. (2012). Wars and child health: Evidence from the Eritrean--Ethiopian conflict. Journal of Development Economics, 99 , 330–340.
Article Google Scholar
Akresh, R., Verwimp, P., & Bundervoet, T. (2011). Civil war, crop failure, and child stunting in Rwanda. Economic Development and Cultural Change, 59 , 777–810.
Ali, F. R. M., & Elsayed, M. A. A. (2018). The effect of parental education on child health: Quasi-experimental evidence from a reduction in the length of primary schooling in Egypt. Health Economics, 27 , 649–662.
Asfaw, A. A. (2018). The distributional effect of investment in early childhood nutrition: A panel quantile approach. World Development, 110 , 63–74.
Bain, L.E., Awah, P.K., Geraldine, N., Kindong, N.P., Siga, Y., Bernard, N., Tanjeko, A.T., 2013. Malnutrition in sub--Saharan Africa: Burden, causes and prospects. Pan Afr. Med. J. 15.
Berazneva, J., & Lee, D. R. (2013). Explaining the African food riots of 2007--2008: An empirical analysis. Food Policy, 39 , 28–39.
Black, R.E., Victora, C.G., Walker, S.P., Bhutta, Z.A., Christian, P., De Onis, M., Ezzati, M., Grantham-McGregor, S., Katz, J., Martorell, R., others, 2013. Maternal and child undernutrition and overweight in low-income and middle-income countries. Lancet, 382 : 427–451.
Block, S. A., Kiess, L., Webb, P., Kosen, S., Moench-Pfanner, R., Bloem, M. W., & Timmer, C. P. (2004). Macro shocks and micro outcomes: Child nutrition during Indonesia’s crisis. Economics and Human Biology, 2 , 21–44.
Borenstein, M., Hedges, L. V., Higgins, J. P., & Rothstein, H. R. (2011). Introduction to meta-analysis . Chichester: John Wiley & Sons.
Google Scholar
Brown, M. E., Funk, C., Galu, G., & Choularton, R. (2007). Earlier famine warning possible using remote sensing and models. EOS. Transactions of the American Geophysical Union, 88 , 381–382.
Buhaug, H., Benjaminsen, T. A., Sjaastad, E., & Theisen, O. M. (2015). Climate variability, food production shocks, and violent conflict in sub-Saharan Africa. Environmental Research Letters, 10 , 125015.
Bundervoet, T., Verwimp, P., & Akresh, R. (2009). Health and civil war in rural Burundi. Journal of Human Resources, 44 , 536–563.
Collins, S., Dent, N., Binns, P., Bahwere, P., Sadler, K., & Hallam, A. (2006). Management of severe acute malnutrition in children. Lancet, 368 , 1992–2000.
Cooper, M., Brown, M. E., Azzarri, C., & Meinzen-Dick, R. (2019). Hunger, nutrition, and precipitation: Evidence from Ghana and Bangladesh. Population and Environment, 41 , 151–208. https://doi.org/10.1007/s11111-019-00323-8 .
de Onis, M., & Blössner, M. (2003). The World Health Organization global database on child growth and malnutrition: Methodology and applications. International Journal of Epidemiology, 32 , 518–526.
Delbiso, T. D., Rodriguez-Llanes, J. M., Donneau, A.-F., Speybroeck, N., & Guha-Sapir, D. (2017). Drought, conflict and children’s undernutrition in Ethiopia 2000--2013: a meta-analysis. Bulletin of the World Health Organization, 95 , 94–102.
Devereux, S., 2000. Famine in the twentieth century. Institute of Development Studies.
Dilley, M. (2000). Warning and intervention: What kind of information does the response community need from the early warning community . Office of US Foreign Disaster Assistance, Washington DC: USAID.
Douxchamps, S., Van Wijk, M. T., Silvestri, S., Moussa, A. S., Quiros, C., Ndour, N. Y. B., Buah, S., Somé, L., Herrero, M., Kristjanson, P., Ouedraogo, M., Thornton, P. K., Van Asten, P., Zougmoré, R., & Rufino, M. C. (2016). Linking agricultural adaptation strategies, food security and vulnerability: Evidence from West Africa. Regional Environmental Change, 16 , 1305–1317. https://doi.org/10.1007/s10113-015-0838-6 .
Dunn, G. (2018). The impact of the Boko haram insurgency in Northeast Nigeria on childhood wasting: A double-difference study. Conflict and Health, 12 , 6.
Ekbrand, H., & Halleröd, B. (2018). The more gender equity, the less child poverty? A multilevel analysis of malnutrition and health deprivation in 49 low-and middle-income countries. World Development, 108 , 221–230.
Finlay, B., Agresti, A., 1986. Statistical methods for the social sciences. Dellen.
Funk, C. C., Dettinger, M. D., Michaelsen, J. C., Verdin, J. P., Brown, M. E., Barlow, M., & Hoell, A. (2008). The warm ocean dry Africa dipole threatens food insecure Africa, but could be mitigated by agricultural development. Proceedings of the National Academy of Sciences, 105 , 11081–11086. https://doi.org/10.1073/pnas.0708196105 .
Funk, C., Shukla, S., Thiaw, W.M., Rowland, J., Hoell, A., McNally, A., Husak, G., Novella, N., Budde, M., Peters-Lidard, C., others, 2019. Recognizing the famine early warning systems network: Over 30 years of drought early warning science advances and partnerships promoting global food security. Bulletin of the American Meteorological Society 100 : 1011–1027.
Gelman, A., & Hill, J. (2006). Data analysis using regression and multilevel/ hierarchical models . London: Cambridge University Press.
Book Google Scholar
Ghobarah, H. A., Huth, P., & Russett, B. (2003). Civil wars kill and maim people-long after the shooting stops. The American Political Science Review, 97 , 189–202. https://doi.org/10.1017/S0003055403000613 .
Grace, K., Davenport, F., Funk, C., & Lerner, A. M. (2012). Child malnutrition and climate in sub-Saharan Africa: An analysis of recent trends in Kenya. Applied Geography, 35 , 405–413.
Grace, K., Brown, M., & McNally, A. (2014). Examining the link between food prices and food insecurity: A multi-level analysis of maize price and birthweight in Kenya. Food Policy, 46 , 56–65. https://doi.org/10.1016/j.foodpol.2014.01.010 .
Grace, K., Davenport, F., Hanson, H., Funk, C., & Shukla, S. (2015). Linking climate change and health outcomes: Examining the relationship between temperature, precipitation and birth weight in Africa. Global Environmental Change, 35 , 125–137.
GSMA, 2015. The Mobile economy 2014, URL: http://www. gsmamobileeconomylatinamerica. com/GSMA_Mobile_Economy_ LatinAmerica_2014. pdf. London, UK.
Haddad, L., Kennedy, E., & Sullivan, J. (1994). Choice of indicators for food security and nutrition monitoring. Food Policy, 19 , 329–343.
Hendrix, C. S., & Haggard, S. (2015). Global food prices, regime type, and urban unrest in the developing world. Journal of Peace Research, 52 , 143–157.
Hillbruner, C., Moloney, G., 2012. When early warning is not enough - lessons learned from the 2011 Somalia famine. Glob. Food Sec. https://doi.org/10.1016/j.gfs.2012.08.001
Hoddinott, J., Maluccio, J. A., Behrman, J. R., Flores, R., & Martorell, R. (2008). Effect of a nutrition intervention during early childhood on economic productivity in Guatemalan adults. Lancet, 371 , 411–416.
Hoddinott, J., Alderman, H., Behrman, J. R., Haddad, L., & Horton, S. (2013). The economic rationale for investing in stunting reduction. Maternal & Child Nutrition, 9 , 69–82.
Howell, E., Waidmann, T., Holla, N., Birdsall, N., Jiang, K., 2018. The impact of civil conflict on child malnutrition and mortality, Nigeria, 2002–2013. Cent. Glob. Dev. Work. Pap.
Hsiang, S. M., & Burke, M. (2014). Climate, conflict, and social stability: What does the evidence say? Clim. Change, 123 , 39–55.
IPC (2012). Integrated food security phase classification technical manual version 2.0: evidence and standards for better food security decisions. . Food and Agriculture Organization (FAO), Rome.
Jacoby, H.G., Rabassa, M.J., Skoufias, E., 2014. Weather and child health in rural Nigeria.
Johnson, K. B., & Brown, M. E. (2014). Environmental risk factors and child nutritional status and survival in a context of climate variability and change. Applied Geography, 54 , 209–221. https://doi.org/10.1016/j.apgeog.2014.08.007 .
Jones, A. D., Ngure, F. M., Pelto, G., & Young,. S. L. (2013). What are we assessing when we measure food security? A compendium and review of current metrics. Advances in Nutrition, 4 , 481–505.
Jones, L., Jaspars, S., Pavanello, S., Ludi, E., Slater, R., Grist, N., Mtisi, S. (2010). Responding to a changing climate: Exploring how disaster risk reduction, social protection and livelihoods approaches promote features of adaptive capacity.
Kennedy, E., Branca, F., Webb, P., Bhutta, Z., & Brown, R. (2015). Setting the scene: An overview of issues related to policies and programs for moderate and severe acute malnutrition. Food and Nutrition Bulletin, 36 , S9–S14.
Kimenju, S. C., & Qaim, M. (2016). The nutrition transition and indicators of child malnutrition. Food Secur., 8 , 571–583.
Kinyoki, D. K., Berkley, J. A., Moloney, G. M., Odundo, E. O., Kandala, N,. & Noor, A. M. (2016). Space--time mapping of wasting among children under the age of five years in Somalia from 2007 to 2010. Spatial and Spatio-temporal Epidemiology, 16 , 77–87.
Lautze, S., & Raven-Roberts, A. (2006). Violence and complex humanitarian emergencies: Implications for livelihoods models. Disasters, 30 , 383–401.
Leroy, J. L., Ruel, M., Frongillo, E. A., Harris, J., & Ballard, T. J. (2015). Measuring the food access dimension of food security: a critical review and mapping of indicators. Food and Nutrition Bulletin, 36 , 167–195.
Lucas, A. M., & Wilson, N. L. (2013). Adult antiretroviral therapy and child health: Evidence from scale-up in Zambia. The American Economic Review, 103 , 456–461.
Maxwell, D., & Fitzpatrick, M. (2012). The 2011 Somalia famine: Context, causes, and complications. Global Food Security, 1 , 5–12.
Maxwell, D., Khalif, A., Hailey, P., Checchi, F., 2020. Determining famine: Multi-dimensional analysis for the twenty-first century. Food Policy in press.
Murali, J., & Afifi, T. (2014). Rainfall variability, food security and human mobility in the Janjgir-Champa district of Chhattisgarh state. India. Clim. Dev., 6 , 28–37. https://doi.org/10.1080/17565529.2013.867248 .
Niles, M., Brown, M.E., 2017. A multi-country assessment of factors related to smallholder food security in varying rainfall conditions. Sci. Rep.
Phalkey, R. K., Aranda-Jan, C., Marx, S., Höfle, B., & Sauerborn, R. (2015). Systematic review of current efforts to quantify the impacts of climate change on undernutrition. Proceedings of the National Academy of Sciences, 112 , E4522–E4529.
Article CAS Google Scholar
Raleigh, C., Linke, A., Hegre, H., & Karlsen, J. (2010). Introducing ACLED – Armed conflict location and event data. Journal of Peace Research, 47 , 651–660.
Raleigh, C., Choi, H. J., & Kniveton, D. (2015). The devil is in the details: An investigation of the relationships between conflict, food price and climate across Africa. Global Environmental Change, 32 , 187–199.
Rashad, A. S., & Sharaf, M. F. (2018). Economic growth and child malnutrition in Egypt: New evidence from national demographic and health survey. Social Indicators Research, 135 , 769–795.
Rodriguez, L. (2016). Intrahousehold inequalities in child rights and well-being. A barrier to progress? World Development, 83 , 111–134.
Sen, A. K. (1981). Poverty and famines: An essay on entitlements and deprivation . Oxford: Clarendon Press.
Shively, G., Sununtnasuk, C., & Brown, M. E. (2015). Environmental variability and child growth in Nepal. Health Place in press, 35 , 37–51. https://doi.org/10.1016/j.healthplace.2015.06.008 .
Skoufias, E., & Vinha, K. (2012). Climate variability and child height in rural Mexico. Economics and Human Biology, 10 , 54–73.
Smith, L. C., & Haddad, L. (2001). How important is improving food availability for reducing child malnutrition in developing countries? Agricultural Economics, 26 , 191–204.
Smith, L. C., & Haddad, L. (2015). Reducing child undernutrition: Past drivers and priorities for the post-MDG era. World Development, 68 , 180–204.
Smith, L. C., Ruel, M. T., & Ndiaye, A. (2005). Why is child malnutrition lower in urban than in rural areas? Evidence from 36 developing countries. World Development, 33 , 1285–1305.
UNSCN. (2010). Sixth report on the world nutrition situation . Italy: Rome.
Walker, S. P., Chang, S. M., Wright, A., Osmond, C., & Grantham-McGregor, S. M. (2015). Early childhood stunting is associated with lower developmental levels in the subsequent generation of children. The Journal of Nutrition, 145 , 823–828. https://doi.org/10.3945/jn.114.200261 .
Article CAS PubMed Google Scholar
WHO (2010). Nutrition Landscape Information System (NLIS) country profile indicators: interpretation guide.
WHO (2020) Severe acute malnutrition. Nutrition topics. United Nations World Health Organization.
Wrottesley, S. V., Lamper, C., & Pisa, P. T. (2015). Review of the importance of nutrition during the first 1000 days: Maternal nutritional status and its associations with fetal growth and birth, neonatal and infant outcomes among African women. Journal of Developmental Origins of Health and Disease, 7 , 144–162. https://doi.org/10.1017/S2040174415001439 .
Article PubMed Google Scholar
Yamano, T., Alderman, H., & Christiaensen, L. (2005). Child growth, shocks, and food aid in rural Ethiopia. American Journal of Agricultural Economics, 87 , 273–288.
Young, H., Borrel, A., Holland, D., & Salama, P. (2004). Public nutrition in complex emergencies. Lancet, 364 , 1899–1909.
Download references
Author information
Authors and affiliations.
Department of Geographical Sciences, University of Maryland, 7251 Preinkert Drive, 2181 LeFrak Hall, College Park, MD, 20742, USA
Molly E. Brown
Department of Government & Politics and Center for International Development and Conflict Management, University of Maryland, 2117 Chincoteague Hall, 7401 Preinkert Drive, College Park, MD, 20142, USA
David Backer, Trey Billing & Paul Huth
Department of Political Science, Auburn University, 7080 Haley Center, Auburn, AL, 36849, USA
Peter White
Department of Geography, Environment, and Society, Minnesota Population Center, University of Minnesota, Minneapolis, MN, 55455, USA
Kathryn Grace
International Health, Johns Hopkins University, 615 N. Wolfe Street, Room E8132, Baltimore, MD, 21205, USA
Shannon Doocy
You can also search for this author in PubMed Google Scholar
Corresponding author
Correspondence to Molly E. Brown .
Ethics declarations
Conflict of interest.
The authors declared that they have no conflict of interest.
Electronic supplementary material
(PDF 128 kb)
(PDF 69 kb)
(DOCX 16 kb)
Rights and permissions
Open Access This article is licensed under a Creative Commons Attribution 4.0 International License, which permits use, sharing, adaptation, distribution and reproduction in any medium or format, as long as you give appropriate credit to the original author(s) and the source, provide a link to the Creative Commons licence, and indicate if changes were made. The images or other third party material in this article are included in the article's Creative Commons licence, unless indicated otherwise in a credit line to the material. If material is not included in the article's Creative Commons licence and your intended use is not permitted by statutory regulation or exceeds the permitted use, you will need to obtain permission directly from the copyright holder. To view a copy of this licence, visit http://creativecommons.org/licenses/by/4.0/ .
Reprints and permissions
About this article
Brown, M.E., Backer, D., Billing, T. et al. Empirical studies of factors associated with child malnutrition: highlighting the evidence about climate and conflict shocks. Food Sec. 12 , 1241–1252 (2020). https://doi.org/10.1007/s12571-020-01041-y
Download citation
Received : 07 July 2019
Accepted : 30 April 2020
Published : 21 May 2020
Issue Date : December 2020
DOI : https://doi.org/10.1007/s12571-020-01041-y
Share this article
Anyone you share the following link with will be able to read this content:
Sorry, a shareable link is not currently available for this article.
Provided by the Springer Nature SharedIt content-sharing initiative
- Food security
- Undernutrition
- Literature review
- Find a journal
- Publish with us
- Track your research
- Research article
- Open access
- Published: 16 October 2020
Prevalence and determinants of undernutrition among under-five children residing in urban slums and rural area, Maharashtra, India: a community-based cross-sectional study
- Sujata Murarkar 1 ,
- Jayashree Gothankar ORCID: orcid.org/0000-0003-2088-677X 1 ,
- Prakash Doke 1 ,
- Prasad Pore 1 ,
- Sanjay Lalwani 2 ,
- Girish Dhumale 3 ,
- Sanjay Quraishi 3 ,
- Reshma Patil 4 ,
- Vivek Waghachavare 3 ,
- Randhir Dhobale 3 ,
- Kirti Rasote 3 ,
- Sonali Palkar 1 &
- Nandini Malshe 2
BMC Public Health volume 20 , Article number: 1559 ( 2020 ) Cite this article
35k Accesses
40 Citations
1 Altmetric
Metrics details
Undernutrition among under five children in India is a major public health problem. Despite India’s growth in the economy, the child mortality rate due to undernutrition is still high in both urban and rural areas. Studies that focus on urban slums are scarce. Hence the present study was carried out to assess the prevalence and determinants of undernutrition in children under five in Maharashtra, India.
A community-based cross-sectional study was conducted in 16 randomly selected clusters in two districts of Maharashtra state, India. Data were collected through house to house survey by interviewing mothers of under five children. Total 2929 mothers and their 3671 under five children were covered. Multivariate logistic regression analysis was carried out to identify the determinants of child nutritional status seperately in urban and rural areas.
The mean age of the children was 2.38 years (±SD 1.36) and mean age of mothers was 24.25 years (± SD 6.37). Overall prevalence of stunting among children under five was 45.9%, wasting was 17.1 and 35.4% children were underweight. Prevalence of wasting, stunting and underweight were more seen in an urban slum than a rural area. In the rural areas exclusive breast feeding ( p < 0.001) and acute diarrhea ( p = 0.001) were associated with wasting, children with birth order 2 or less than 2 were associated with stunting and exclusive breast feeding ( p < 0.05) and low maternal education were associated with underweight. Whereas in the urban slums exclusive breast feeding ( p < 0.05) was associated with wasting, sex of the child ( p < 0.05) and type of family ( p < 0.05) were associated with stunting,and low income of the family ( p < 0.05) was associated with underweight.
Conclusions
Factors like sex of the child, birth order,exclusive breast feeding,economic status of the family, type of family,acute diarrhea and maternal education have influence on nutritional status of the child. Improvement of maternal education will improve the nutritional status of the child. Strategies are needed to improve the economic status of the community.
Trial registration
Trial registration number: CTRI/2017/12/010881 ; Registration date:14/12/2017. Retrospectively registered.
Peer Review reports
Undernutrition among under five children in India is a major public health problem [ 1 ]. Its prevalence is highest in the world and is almost double that of Sub-Saharan Africa [ 2 , 3 ].
Out of the total world’s undernourished children, 80 % lives in 20 countries. In India almost 60 million children are underweight [ 4 ] .
UNICEF in the year 2006 reported the causes of childhood malnutrition as insufficient diet, frequent infections, poor breastfeeding practices, delayed introduction of complementary foods and inadequate protein in the diet. Other factors that influence food intake include health status, food taboos, growth and personal choice related to diet. Malnutrition can also develop due to neglect, abnormal mealtimes, insufficient quantities of food and insufficient parental knowledge [ 5 ].
Every year International Food Policy and Research Institute (IFPRI) publishes the Global Hunger Index (GHI). According to the report for 2019, India is ranked 102 out of a total of 119 countries [ 6 ]. Prevalence of undernutrition among under five children according to the National family health survey 4 (NFHS 4) in India shows that 35.7% under five children were underweight, 38.4% were stunted and 21% were wasted [ 7 ]. From National family health survey 1 to National family health survey 4, the prevalence of undernutrition has not declined as desired. According to the Comprehensive National Nutrition Survey report (2016–2018),35% of Indian children aged 0–4 years were stunted, 17% were wasted and 33% were underweight [ 8 ]. Malnutrition in the form of undernutrition, namely underweight, stunting and wasting has been coined as the “silent emergency” by the United Nations children’s fund [ 9 , 10 , 11 ].
The government of India has strongly committed to achieving the 2030 Sustainable Development Goals (SDGs). End hunger, achieve food security, and improved nutrition and promote sustainable agriculture, all these nutrition-related factors are included in sustainable development goals (SDGs) [ 12 ]. If undernutrition is not effectively reduced, the country will not meet its SDG target of child mortality reduction [ 8 ].
In developing countries, the nutritional status of children depends on socioeconomic status, awareness of diseases such as diarrhea and acute respiratory tract infection, educational status of mother and availability of safe drinking water [ 13 ]. Undernourished children are prone to infections. Statistically underweight children succumb to diseases such as diarrhea, measles, and malaria and lower respiratory tract infections. Undernutrition in young children has long-term negative effects on physical and cognitive development [ 14 ]. A global review on child stunting and economic outcomes revealed a 1 cm increase in height was associated with a 4% increase in wages for men and a 6% increase in wages for women. Investing in the reduction of child malnutrition is paramount for human and economic development [ 8 ]. Data from six longitudinal studies on the association between anthropometric status and mortality of children aged 6–59 months revealed a strong association between the severity of weight-for-age deficits and mortality rates. Indeed, out of the 11.6 million deaths among under-five children in 1995 in developing countries, it has been estimated that 6.3 million or 54% of young child mortality were associated with malnutrition [ 15 ].
Despite India’s growth in the economy, the child mortality rate due to undernutrition is still high in both urban and rural areas. Hence assessment of nutritional status among children is critical in framing health policies [ 16 ].
The majority of earlier studies on undernutrition were carried out either in the rural area or in the urban area, while few earlier studies focus only on chronic undernutrition i.e. stunting. To bridge this gap,the present study was conducted to assess the prevalence of undernutrition in children under five and its determinants in rural and urban areas of Maharashtra, India.
This study was a part of the baseline survey conducted in 16 clusters located in two districts of western Maharashtra, India, during cluster randomized control trial (CRCT) among children below 5 years of the rural area and urban slums. The trial was registered with clinical trial registry of India (CTRI).
Study design
A community-based cross-sectional study.
Study setting
The current study was carried out in rural areas and urban slums in two districts in Maharashtra state, India. Composite health index (Sangli = 0.66 & Pune = 0.65) of these two districts are similar as well as they are similar in health indicators [ 17 ]. The rural and urban areas have revenue villages and slums, respectively. These slums or revenue villages are referred to as clusters. There are total 45 clusters (Pune district = 20, Sangli district = 25). On average there are 250 under five children per cluster and a total population of 1, 89,504 [ 18 ].
Study duration
The main CRCT was conducted between 15th December 2015 to 14th March 2018. Benchmark data i.e. data of this study was collected between 15 Feb 2016 to 14 May 2016.
Sample size
Out of 45 clusters, 8 clusters each in intervention and comparison arm were selected. In selected clusters, all the under five children were included in the survey. However, we had tested the adequacy of the sample size. Considering the National prevalence of underweight (35.7%), stunting (38.4%) and wasting (21%) sample size was independently calculated for underweight, stunting and wasting [ 7 ]. With a 95% confidence interval and allowable difference of 10% of prevalence, sample size 1504 was calculated by using the following formula.
With cluster effect 2, the sample size came around 3008. By considering a 10% loss to follow up we need to study 3309 children, but we had included 3671 children which is an adequate sample size.
Study participants
All children below 5 years with their mothers in the selected areas.
Research tool
The questionnaire was first prepared in English . It was validated by a panel of experts and then translated into the local language(Marathi) and retranslated in English (Additional file 1 ). Inimitable identification code was given to each house, the mother of a child below 5 years and the child.
Data collection
Eight field supervisors (FS) appointed for the project collected data from house to house survey with the help of Accredited Social Health Activists (ASHAs) in all areas except Pune urban slum where anganwadi workers (AWWs) cooperation was sought. Designated site investigators (SI) monitored and supervised the activities of each field supervisor. These site investigators were faculty of the two medical colleges. In the selected clusters, all families residing for more than 6 months were included in the study. Households that were found to be locked during two consecutive visits were not included in the study. According to standard guidelines, weight measurements were recorded to the nearest 100 g using the standard weighing machine and the height of the children was recorded to the nearest 0.1 cm using a measuring tape. The exact age of the child was computed from the child’s date of birth. When data on the exact date of birth was not available, the age as told by the mother was used, to the nearest month.
Definitions used in the present study
Type of family: It was divided into two groups; the nuclear family consists of the husband, wife and unmarried children staying together, the joint family included all other families including three-generation family as well as extended family. Households, where its occupants were not blood- related i.e. household occupied by nonblood related members, were not considered as family and not included in the study.
Socio-economic status: In Maharashtra state, there are three types of ration cards, based on the income of the family. Accordingly, families were classified under three categories depending on income considering the color of the card as a proxy for income. The yellow ration card represents less than or up to Rs. 15,000 (205.97 USD), an orange ration card represents between Rs. 15,000 (205.97 USD) to Rs 100,000 (1373.15 USD) and white ration card represents more than Rs.100, 000 (1373.15 USD) income per annum [ 19 ].
Educational status including literacy status of mothers: A person who could not read and write was labeled as illiterate [ 20 ]. According to years of schooling educational status was classified as education up-to 6th standard,7th to 10th standard, 12th standard/Diploma, Graduate and above.
Birth order: Birth order of the child was considered as told by the mother.
Immunization status (for children between 12 and 23 months):
Fully immunized Children- Those who have received all vaccines recommended in National immunization schedule in infancy i.e. BCG, OPV zero dose and Hepatitis B at birth, DPT1 and OPV1 at 6 weeks, DPT2 and OPV 2 at 10 weeks, DPT 3 and OPV3 at 14 weeks, Measles and vitamin A at 9 months.
Non immunized children -Those who have not received a single vaccine.
Partially immunized- All other children were considered as partially immunized
Children with immunization card were considered for the analysis.
Exclusive breastfeeding: Child fed only breast milk except taking vitamins, mineral supplements, or medicines until 6 months of age was considered as exclusive breastfeeding [ 21 ].
Wasting in children: According to WHO standards child with weight for height z -score below − 2 Standard Deviations (SD) of the median of a reference standard [ 22 ].
Stunting in children: According to WHO standards child with length/height for age z -score below − 2 Standard Deviations (SD) of the median of a reference standard [ 22 ].
Underweight children: According to WHO standards child with weight for age z -score below − 2 Standard Deviations (SD) of the median of a reference standard [ 22 ].
CRCT - Cluster randomized control trial is a comparative study in which the units randomised are pre-existing (natural or self-selected) groups whose members have an identifiable feature in common, and in which outcomes are measured in all, or a representative sample of the individual members of the groups [ 23 ].
CTRI- Clinical Trial Registry of India is a online record system meant for registering all clinical trials conducted in India [ 24 ].
ASHA- Accredited social health activist works at village level under National health mission programme of India. She creates awareness on health in the community [ 25 ].
AWW- Under the Integrated child development services (ICDS) scheme anganwadi workers(part time workers) are appointed to render health services in the community [ 25 ].
SPSS-Statistical package for social sciences is a software package used for statistical analysis.
Data processing
All filled forms were entered into the software database. To check completeness and accuracy in the form, critical fields in the tool were identified. Along with critical fields, noncritical fields were also monitored. For critical data discrepancies up to 0.1% and and for non-critical data upto 1% were considered acceptable. For discrepancies related to data entry alternate forms were physically cross-checked. Complete cases were analyzed and missing data were not included because of low occurrence. The data were analyzed after cleaning the data and final report was prepared.
Data analysis
Data were analyzed using statistical package for social sciences (SPSS) (version 20). Variables adjusted during analysis were as follows- for maternal education illiterate and all categories less than 6th standard were merged together as ≤6th standard whereas all the categories above 6th standard were merged together as>6th standard. During analysis of type of family, families other than joint and nuclear were merged in joint family type. While analyzing exclusive breast feeding < 6 months and 6 months these to categories were merged together. The findings were reported in terms of frequency and percentage for qualitative variables and quantitative variable findings were shown by descriptive statistics. Multivariate logistic regression analysis was carried out to identify the determinants of child nutritional status seperately in urban and rural areas. Independent variables like sex of the child, type of family, income of the family, birth order, exclusive breastfeeding, immunization status, ARI, diarrhea, maternal age, maternal education were taken into consideration and the dependent variables were wasting,stunting and underweight in rural area and urban slum. As for immunization of children we had considered all doses within 1 year, the sample size for that variable got reduced. So due to the minimum sample size of this variable and multi-collinearity in covariables, this variable got removed from the multivariate logistic regression analysis. Results were reported by 95%confidence interval with a 5% level of significance. P -value of less than 0.05 ( p < 0.05) was considered significant.
In the present study, 2929 mothers with 3671 under five children were enumerated [ 18 ]. Out of the total population children below 5 years constituted 7.5%. There were 1834 under-five children in urban slum and 1837 in the rural area. There were 1939 boys and 1732 girls in the study area. The mean age of the children was 2.38 years (±SD 1.36). Out of these 3671 under five children, 752 were infants. The mean birth weight of these infants was 2.6 kg (±SD 0.61). Almost 80.1% women were in the age group between 20 and 29 years. The mean age of mothers was 24.25 years (±SD6.37). About 56.55% of mothers had education up-to high school.
Exclusive breastfeeding (EBF) was given to 46% of the children. It was seen higher in children from a rural area (78%) than in children from an urban slum (15%) ( P < 0.05).
There were a total of 768 children in the age group of 12 to 23 months but only 639 children possessed immunization card. Out of these 639 children, 94.6% were fully immunized. It was higher in rural clusters (97%) than urban clusters (91%) (P < 0.05). For 129 children, immunization information was missing as their status could not be confirmed by immunization card. We have studied the association of factors affecting undernutrition like the gender of the child, type of family, the income of the family, birth order, exclusive breastfeeding, immunization status, ARI, diarrhea, maternal age and maternal education.
Table 1 shows the types of under-nutrition in rural area and urban slums. Out of total 3671 under five children data of 3.5% of children were missing on the day of the survey, hence the total does not match. Overall, 17.1% under five children were wasted, 45.9% were stunted and 35.4% were underweight. It was observed that the prevalence of all three types of under-nutrition, wasting (OR = 0.836), stunting (OR = 0.737) and underweight (OR = 0.886) was less in rural areas. Stunting was the most common form of under-nutrition observed.
Exclusive breastfeeding in both rural area (Adj OR = 0.35, p < 0.001) and urban slum (Adj OR = 0.47, p < 0.05) and diarrhea in the rural area (Adj OR = 0.11, p = 0.001) are the determinants of wasting among under-five children (Table 2 ). In the computation of adjusted odds ratio of all the variables in table number 2, 3 and 4, following reference categories were considered – male sex of the child,exclusive breast feeding > 6 months, nuclear type of family,income of family≤1373.15 USD,birth order > 2,diarrhea -yes, ARI-yes,maternal age ≤ 20 and maternal education ≤6th standard.
It was observed that stunting among under-five children was significantly associated with the sex of the child in the urban slum(Adj. OR = 1.77, p < 0.05), birth order in the rural area (Adj OR = 2.11, p < 0.05) and type of family in the urban slum (Adj OR = 0.56, p < 0.05) (Table 3 ).
Important factors influencing underweight among children were exclusive breastfeeding up to 6 months in rural area (Adj OR = 0.50, p < 0.05), income of family in urban slum (Adj OR = 2.16, p < 0.05) and maternal education in rural area (Adj OR = 0.44, p < 0.05) (Table 4 ).
Thus multiple logistic regression analysis confirmed the factors such as sex of the child, exclusive breastfeeding, type of family, income of the family, birth order of the child, diarrhea (past 1 month) and maternal education as the determinants for undernutrition among under-five children.
The current study was a large comparative study as compared to most of the studies in India among under five children of urban slum and rural area [ 1 , 2 , 3 ]. This study is unique in providing information about undernutrition and it’s determinants at the community level. The data were collected by qualified and trained field supervisors and the quality checks for data collection was monitored by the site investigators from public health, community medicine and pediatrics specialty.
It was observed that overall prevalence of stunting among children under five was 45.9%, wasting was 17.1 and 35.4% children were underweight. Similar statistics emerge from several studies in India [ 8 , 26 , 27 ]. High prevalence of stunting (89.6%) and underweight (73.2%) were reported in a study among under-five children in Uttar Pradesh, India [ 28 ]. Whereas another study had revealed a low prevalence of both underweight (19.9%) and stunting (17.1%) and reversed the ranking [ 3 ].
Wasting may result from inadequate food intake or a recent episode of illness-causing weight loss. The highest prevalence of wasting is in South Asia, where approximately one in six children (16%) are moderately or severely wasted. The burden of wasting is highest in India, which has more than 25 million (20%) wasted children. This exceeds the combined burden of the next nine high-burden countries [ 29 ]. Overall, 17% of Indian children age 0–4 years were wasted [ 8 ], which is as per the present study finding.
Stunting reflects chronic undernutrition and hence UNICEF is also focusing on stunting among under five children [ 30 ]. In the present study, we found that stunting was more prevalent in the urban slum (49.7%) as compared to rural area (42.1) whereas according to Comprehensive National nutrition survey report (2016–2018) higher prevalence of stunting in under-fives was found in rural areas (37%) as compared to urban areas (27%) [ 8 ]. We feel that the availability of food grains are more or less uniform throughout the year in an urban slum. In rural area seasonal availability of food grains increases. There is a tendency to purchase only locally produced and available seasonal grains, vegetables, and fruits which are comparatively cheaper in rural areas. Hence stunting is more prevalent in the urban area.
Many socio-demographic characteristics of the child and family are associated with the presence of undernutrition. They include the gender of the child, birth weight, birth order, number of siblings, exclusive breastfeeding, immunization status, mother’s education and occupation, family income, mother’s knowledge about the timing of weaning and diet, etc. Out of these factors’ majority and particularly most critical we assessed in the present study.
It was seen that exclusive breastfeeding up to 6 months gives protection against wasting to children both from a rural area (Adj OR = 0.35, p < 0.001) and urban slum (Adj OR = 0.47, p < 0.05). Exclusive breastfeeding up to 6 months acts as a protective factor against infection because it is rich in anti-infective factors that prevent respiratory infections and diarrheal diseases. It enhances the immunity of the child. However, it should be accompanied by timely weaning. Continuing exclusive breastfeeding beyond 6 months implies the child is getting inadequate nutrition and become malnourished.
Childhood infections like diarrhea and acute respiratory tract infection are important causes of malnutrition among under-five children in developing countries. As these are acute episodes, it results in immediate weight loss. In the present study prevalence of wasting was found higher in under-five children with acute diarrhea in the rural area (Adj OR = 0.11, P = 0.001), a similar finding was supported by other studies [ 31 , 32 , 33 , 34 ].
Birth order has always been an important determinant of undernutrition. As compared to children with birth order 2 or more than 2, children with birth order less than two were more likely to be stunted in the rural area (Adj OR = 2.11, p < 0.05). This finding was opposite to findings from other studies in India [ 11 , 26 , 27 , 35 ]. It may be because in Maharashtra teenage marriages are common [ 7 ]. It triggers the link of early childbearing, low birthweight babies which results in developing long term undernutrition of the child. It was observed that the prevalence of stunting was more among boys as compared to girls (Adj OR = 1.77, P < 0.05) in the urban slum. This is in line with other studies [ 1 , 14 , 36 , 37 ]. Although the exact reason was not known, it may be due to the well-known fact that the male child is more affected by environmental stress than a female child. Contrary to this finding study from South West Rajasthan reported that stunting was 1.48 times more in females than in male children (OR = 1.48; Cl = 1.00–2.47) [ 38 ].
Family plays an important role in health and disease. It was seen that the joint family gives protection against stunting to under-five children of the urban slum (Adj OR = 0.56, p < 0.05). This emphasizes the importance of a joint family in society. The Indians understand the importance of a joint family system since time immemorial. Sharing resources and responsibilities amongst family members can help parents reduce the economical and physical stress. Children also get more attention in the joint family. Association between the type of family and undernutrition was not found significant by other studies [ 38 , 39 ].
As per the recommendation of global public health, for achieving optimum growth, development, and health a child should be breastfed exclusively during the first 6 months of life. To evolve as a healthy individual, the infant should be continued with adequate and appropriate safe complimentary food along with breast milk up to 2 years of age or beyond [ 5 ]. In the present study also, similar finding was observed i.e. exclusive breastfeeding up to 6 months acts as a protective factor against underweight among under five children from a rural area (Adj OR = 0.50, p < 0.05). This finding was supported by other studies [ 9 , 40 ].
It is well known that socioeconomic status is one of the important determinants of the wellbeing of children and health [ 41 ]. Lower the socioeconomic status higher is the risk of undernutrition. A supportive study done in India and Africa reveals that families with low economic status have a significant association with undernutrition [ 6 ]. With the improvement in socioeconomic status undernutrition proportionately declines [ 42 ]. It was observed that the low income of the family had resulted in underweight among children from an urban slum (Adj OR = 2.16, p < 0.05). A similar finding was also revealed by a study in urban slums of Delhi [ 43 ].
Another important factor affecting underweight was maternal education. It was observed that low maternal education was a risk factor for undernutrition among under five children of rural areas (Adj OR = 0.44, p < 0.05). Undernutrition decreases with an increase in the educational qualification of the mothers. This was in line with other studies [ 9 , 44 , 45 ]. Mother is a universally first caregiver for the child and hence mother’s education matters. Educated mothers are more aware of the health services available and the acceptance to utilize the same is better among them. Mother is also the first teacher of the child and hence mother and child are treated as one unit. Educated girls marry at slightly higher age comparative to less educated girls and accordingly late childbearing and have a fewer number of children.
Study results indicate that under nutrition is still an important health concern among under five children. Undernutrition in the form of underweight and stunting is more prevalent than wasting in the urban slum and rural area. Factors like sex of the child, birth order,exclusive breast feeding,economic status of the family, type of family,acute diarrhea and maternal education have influence on nutritional status of the child. Improvement of maternal education will improve the nutritional status of the child. Strategies are needed to improve the economic status of the community.
Limitations
As immunization status was based on immunization card, children who had received some immunizations but lost their immunization card would be misclassified.
We have not taken dietary survey of under five children.
Availability of data and materials
Corresponding author Dr. Jayashree Gothankar has the datasets analysed during the current study. Data can be accessed by requesting her.
Abbreviations
Cluster Randomized Control Trial
Clinical Trial Registry of India
Acute respiratory infections
Exclusive breastfeeding
Accredited social health activist
Anganwadi worker
Field supervisor
Site Investigator
Statistical package for social sciences
Confidence interval
United Nations Children’s Fund
World Health Organization
Qadri HA, et al. Under-nutrition more in male children: A new study. Int J Res Med Sci. 2015;3(11):3363–6.
Article Google Scholar
Sahu SK, Ganesh Kumar S. Malnutrition among under five children in India and strategies for control. J Nat Sci Biol Med. 2015;6(1):18–23.
Sundari S, et al. A Study on the prevalence and pattern of malnutrition among children under five years in Chennai. Int J Pharm Bio Sci. 2017;8(3): (B):135–9.
Google Scholar
Bryce J, Coitinho D, Darnton H, Pinsturp I, Anderson P. Maternal and child undernutrition, effective action at national level. Lancet. 2008 Feb 9;371(9611):510–26.
Ansuya et al. Risk factors for malnutrition among preschool children in rural Karnataka: a case-control study. BMC Public Health. (2018); 18:283.
Global hunger index 2019:India. https://www.globalhungerindex.org/pdf/en/2019/India.pdf. Accessed 25th Apr 2020.
NFHS 4(2015–16) Fact sheet. https://rchiips.org/NFHS/pdf/NFHS4/India.pdf . Accessed 13th Aug 2018.
Comprehensive National Nutrition survey report 2016–2018. https://nhm.gov.in/index1.php?lang=1&level=2&sublinkid=1332&lid=713 . Accessed 15th Oct 2019.
Priyanka R, Vincent V, et al. An assessment of the nutritional status of underfive children in rural area of Thrissur district, Kerala India. Int J Community Med Public Health. 2016;3(12):3479–86.
Yadav SS, Yadav ST, Mishra P, Mittal A, Kumar R, Singh J. An epidemiological study of malnutrition among under five children of rural and urban Haryana. J Clin Diagn Res. 2016 Feb;10(2):LC07.
CAS PubMed PubMed Central Google Scholar
Shukla N, Toppo NA, Thakur A, Kasar PK, Sharma B. A study of malnutrition and associated risk factors among children of age 06-59 months in rural area of Jabalpur district, Madhya Pradesh. Indian J Community Health. 2018;30(1):24–9.
Sustainable development –knowledge platform by WHO. https://sustainabledevelopment.un.org/memberstates/india .Accessed 20th Sept 2018.
Mittal A, Singh J, Ahluwalia SK. Effect of maternal factors on nutritional status of 1-5-year old children in urban slum population. Indian J Community Med. 2007;32:264–7.
Dhone A, et al. Epidemiological study of under nutrition among under five years children in an urban slum. Medical Journal of Dr. D.Y. Patil University. 2012;5(2):110–3.
Vinod N, et al. Nutritional status and dietary pattern of underfive children in urban slum area. National J Community Med. 2011;2(1):143–8.
Pal A, Pari AK, Sinha A, Dhara PC. Prevalence of undernutrition and associated factors: a cross-sectional study among rural adolescents in West Bengal, India. Int J Pediatr Adolesc Med. 2017;4(1):9–18.
Doke PP. Comprehensive index for community health assessment of typical district administrative units in Maharashtra state, India. Indian J Community Med. 2016 Oct;41(4):288.
Gothankar J, Doke P, Dhumale G, Pore P, Lalwani S, Quraishi S, Murarkar S, Patil R, Baliram WV, Vishnupant DR, Hemant PS. Reported incidence and risk factors of childhood pneumonia in India: a community-based cross-sectional study. BMC Public Health. 2018;18:1111.
Government of Maharashtra food civil supplies and consumer protection department, public distribution system. http://mahafood.gov.in/website/englishpds.aspx .Accessed 28th Aug 2018.
Directorate of Census operations. Census of India Maharashtra District census handbook2011. http://www.censusindia.gov.in/2011census/dchb_A/27/2785_PART_A_DCHB_PUNE.pdf . Accessed 23rd Aug 2018.
Hunegnaw MT, et al. Breastfeeding and associated factors among mothers in Gozamin district, northwest Ethiopia: a community-based cross- sectional study. Int Breastfeeding J. 2017;12:30.
WHO Child growth Standards Length/height for age, weight for age , weight for length/weight and body mass index for age methods and development. http://www.who.int/childgrowth/publications/tecnical reportpub/en/indexhtml . Accessed 28th Aug 2018.
Moberg J, Kramer M. A brief history of the cluster randomised trial design. J R Soc Med. 2015;108(5):192–8.
Dixit JV. Principals and practice of medical research. First ed. Banarasidas Bhanot publishers; 2013. p. 61.
Park K. Park’s textbook of Preventive and Social Medicine. 24th ed. Jabalpur: Banarasidas Bhanot publishers; 2017. p. 936.
Sarkar S. Cross-sectional study of child malnutrition and associated risk factors among children aged under five in West Bengal, India. Int J Population Stud. 2016;7:2(1).
Purohit L, et al. Nutritional status of underfive children in a city of Maharashtra:a community based study. Int J Community Med Public Health. 2017;4(4):1171–8.
Awasthi S, Das R, Verma T, Vir S. Anemia, and undernutrition among preschool children in Uttar Pradesh. India Indian Pediatrics. 2003;40:985–90.
PubMed Google Scholar
Goutam SK, Verma M, et al. Nutritional status and its corelates in under five slum children of Kanpur Nagar, India. Int J Contemporary Pediatr. 2018;5(2):584–90.
Reducing stunting in children under five years of age: a comprehensive evaluation of UNICEF’s strategies and programme performance – India Country Case Study. 2017. https://www.unicef.org/evaldatabase/index_100696.html . Accessed 20th Sept 2018.
Singh JP, et al. Study of nutritional status among under five children attending outpatient department at a primary care rural hospital, Bareilly (UP). Sch. J. App Med Sci. 2013;1(6):769–73.
Rice AL, Sacco L, Hyder A, Black RE. Malnutrition as an underlying cause of childhood deaths associated with infectious diseases in developing countries. Bull World Health Organ. 2000;78:1207–21.
Gupta A. Study of the prevalence of diarrhea in children under age of five years: it’s association with wasting .2014. Indian J Sci Res. 2014;7:1315–8.
Suri S, Kumar D. Nutritional status and the factors associated with it among children aged 1-5 years in a rural area of Jammu. Int J Sci Stud. 2015 Jun;3(3):60–4.
Meena S, et al. Nutritional status of children under five years of age in anganwadi centers in Kolar area of Madhya Pradesh. National Journal of Community Medicine Apr. Jun 2015;6(2):247–52.
Kavita Baranwal V. M Gupta et al. factors influencing the nutritional status of under-five (1-5years) children in urban slum area of Varansi. Indian J Community Health. July 2009;21(2):13–7.
Meshram II, Mallikharjun Rao et al. Prevalence of undernutrition and its predictors among under five children in surat region, Gujarat India. J Clin Nutrition Dietetics. 2016;2(1,2).
Sharma AK, et al. Prevalence and risk factors for stunting among triable under-five children at south West Rajasthan India. National J Community Med. 2016;7(6):461–7.
Sengupta P, Philip N, et al. Epidemiological correlates of undernutrition in under-five children in an urban slum of Ludhiana. Health and population perspectives and issues. 2010;33(1):1–9.
Pant B, Vaish A, Jain S, Ahmad S, Varshney AM. Rupesh. Malnutrition among under five children in the peri-urban area of Meerut city: impact of socio demographic factors. Asian J Pharm Hea Sci. 2013;3(3):769–73.
Damor RD, et al. A study on assessment of nutritional and immunization status of under-five children in urban slums of Jamnagar city, Gujarat. Healthline. 2013;4:2.
Viramgami AP, et al. Influence of maternal factors on nutritional status of urban slum children. Int J Sci Res. 2018;7:6.
Bhadoria AS, et al. Prevalence of underweight, stunting and wasting among children in urban slums of Delhi. Int J Nutr Pharmacology, Neurological diseases. 2013;3(3):323–4.
Popat CN, et al. A cross sectional study to measure the prevalence of malnutrition and factors associated with malnutrition among under five children of an urban slum of Vadodara city. Journal of research in medical and dental science. July –September. 2014;2(3):59–64.
Gupta R, Chakrabarti S, Chatterjee SG. A study to evaluate the effect of various maternal factors on the nutritional status of under-five children. Indian J Nutrition. 2016;3:2.
Download references
Acknowledgments
We are grateful to mothers of under five children for their participation, field supervisors and government stakeholders for their cooperation. Statisticians, Mrs. Aruna Deshpande, Mrs. S G Garad, Mr. S P Sane, Mr. Rupesh Deshmukh for Statistical analysis; Project coordinators, Mrs. Mahima Dwivedi and Dr. Supriya Phadnis, for their significant inputs in implementation of project and preparation of report; Dr. Manoj Das, INCLEN trust international for guiding on technical issues.
This study was funded by The Bill and Melinda Gates Foundation (OPP 1084307) through The INCLEN Trust International, New-Delhi, India (Project ID; INC2015GNT006). The Bill and Melinda Gates Foundation and The INCLEN Trust International had no role in study design, data collection,data analysis data interpretation,or writing of the report.
Author information
Authors and affiliations.
Department of Community Medicine, Bharati Vidyapeeth Deemed to be University Medical College, Off Pune Satara Road, Pune, 411043, India
Sujata Murarkar, Jayashree Gothankar, Prakash Doke, Prasad Pore & Sonali Palkar
Department of Pediatrics, Bharati Vidyapeeth Deemed to be University Medical College, Pune, India
Sanjay Lalwani & Nandini Malshe
Department of Community Medicine, Bharati Vidyapeeth Deemed to be University Medical College, Sangli, India
Girish Dhumale, Sanjay Quraishi, Vivek Waghachavare, Randhir Dhobale & Kirti Rasote
Department of Community Medicine, Symbiosis Medical College for women, Pune, Maharashtra, India
Reshma Patil
You can also search for this author in PubMed Google Scholar
Contributions
JG, PD, PP, GD, SL contributed in the framing of design of the study; SQ, SM, RP, SP, VW, RD, KR, NM were responsible for the data collection. JG, PP, GD, PD analyzed and interpreted the data, with inputs from SL, SQ, SL, SM, RP, NM, SP, VW, RD, KR. SM drafted the manuscript and all authors were involved in revising it critically. All authors have read and approved the final manuscript.
Corresponding author
Correspondence to Jayashree Gothankar .
Ethics declarations
Ethics approval and consent to participate.
Ethics approval was sought from Bharati Vidyapeeth Deemed University Institutional Ethics Committee (ECR/313/Inst/MH/2013/RR-16) and Department of Pharmacology Bharati Vidyapeeth Deemed University Medical College and Hospital Sangli (ECR/276/Inst/MH/2013/RR-16) before the start of the study [ 18 ]. Written informed consent was taken from mothers of under-five children to participate in the study before the start of the study.
Consent for publication
Not applicable.
Competing interests
None declared.
Additional information
Publisher’s note.
Springer Nature remains neutral with regard to jurisdictional claims in published maps and institutional affiliations.
Supplementary information
Additional file 1..
Study questionnaire. Study questionnaire used for data collection.
Rights and permissions
Open Access This article is licensed under a Creative Commons Attribution 4.0 International License, which permits use, sharing, adaptation, distribution and reproduction in any medium or format, as long as you give appropriate credit to the original author(s) and the source, provide a link to the Creative Commons licence, and indicate if changes were made. The images or other third party material in this article are included in the article's Creative Commons licence, unless indicated otherwise in a credit line to the material. If material is not included in the article's Creative Commons licence and your intended use is not permitted by statutory regulation or exceeds the permitted use, you will need to obtain permission directly from the copyright holder. To view a copy of this licence, visit http://creativecommons.org/licenses/by/4.0/ . The Creative Commons Public Domain Dedication waiver ( http://creativecommons.org/publicdomain/zero/1.0/ ) applies to the data made available in this article, unless otherwise stated in a credit line to the data.
Reprints and permissions
About this article
Cite this article.
Murarkar, S., Gothankar, J., Doke, P. et al. Prevalence and determinants of undernutrition among under-five children residing in urban slums and rural area, Maharashtra, India: a community-based cross-sectional study. BMC Public Health 20 , 1559 (2020). https://doi.org/10.1186/s12889-020-09642-0
Download citation
Received : 03 November 2019
Accepted : 04 October 2020
Published : 16 October 2020
DOI : https://doi.org/10.1186/s12889-020-09642-0
Share this article
Anyone you share the following link with will be able to read this content:
Sorry, a shareable link is not currently available for this article.
Provided by the Springer Nature SharedIt content-sharing initiative
- Undernutrition
- Under-five children
BMC Public Health
ISSN: 1471-2458
- Submission enquiries: [email protected]
- General enquiries: [email protected]
- Open access
- Published: 14 November 2023
Knowledge, attitude and practice towards malnutrition and micronutrient deficiency among male and female farmers in Ethiopia
- Girma Gezimu Gebre 1 , 2 ,
- Derebe Ermias Chefebo 3 &
- Deribe Kaske Kacharo 1
BMC Nutrition volume 9 , Article number: 130 ( 2023 ) Cite this article
1492 Accesses
2 Altmetric
Metrics details
Despite a large body of literature on the nexus between knowledge, attitude and practice towards nutrition and gender, this nexus is likely to vary and is not clear in many societies, such as Ethiopia.
The study aimed to analyze the level of gender-based knowledge, attitude, and practice towards malnutrition and micronutrient deficiency using primary data collected from two regional states in Ethiopia.
Qualitative and quantitative data collection approaches were used. Qualitative data were analyzed using a narrative and content approach. Quantitative data were analyzed using descriptive statistics.
Results indicate that female are generally more adept than male at identifying the symptoms of malnutrition. However, concerning vitamin A and iodine food types and its deficiency, male respondents had relatively better knowledge and consumption practice than female. Results show that there is very little awareness about biofortified and fortified foods. When we rate respondents, male had a relatively better understanding about fortified foods than their female counterparts.
Findings can support development agents working to improve nutrition in Ethiopia to focus on improving community knowledge and perception of biofortified and fortified foods to improve diet quality through increased micronutrient intake. The majority of the respondents were aware of the importance of consuming micronutrient rich foods and had a positive attitude towards them. However, there is still a gap in practice. It may therefore require more targeted campaigns to increase the ability of community members to adopt best practices while reducing barriers to consumption of nutritious diet.
Peer Review reports
Introduction
Global statistics show that more than two billion individuals, or one in three people globally, suffering from hidden hunger. Hidden hunger is the presence of multiple micronutrient deficiencies such as iron, iodine and vitamin A; which can occur without a deficit in energy intake as a result of consuming an energy-dense, but nutrient-poor diet [ 1 ]. When we are deficient in a specific micronutrient, say vitamin A, iron, and iodine, our brain does not get or trigger the same signal as our body's needs for more food. This form of hunger is known as hidden hunger or micronutrient deficiency [ 1 ].
Malnutrition is an abnormal physiological condition, typically due to deficiencies or excesses in nutrient intake, or imbalances of essential nutrients in the body. Malnutrition, in all its forms (under nutrition or over nutrition) affects almost every country in the world, leading to serious public health risks and incurring high economic costs [ 2 ]. Micronutrient deficiencies, particularly those in folic acid, iodine, iron, and vitamin A, have a long-lasting impact on growth and development and are thus a national priority [ 3 ]. Improvements in nutrition will significantly contribute to reducing poverty and achieving the health, education, and employment goals outlined in the United Nations [ 2 ]. Nutrition stimulates economic growth, which improves the physical productivity and mental health of the labor force. The Inter-Agency Standing Committee (IASC) noted that girls and boys—and men and women—have different nutritional needs at different life stages. They face different risks and challenges in accessing sufficient nutrition. Gender inequality exacerbates food insecurity, malnutrition and poverty in humanitarian crises [ 4 ]. The socially constructed gender roles of men and women interact with their biological roles to affect the nutrition status of the entire family and of each gender [ 5 , 6 ]. All gender and age groups have the right to equal access to nutrition services and the foods they need to live a healthy life [ 4 ]. Women are the main food producers, yet they are disproportionally affected by hunger and malnutrition. Evidence suggests that when women have more control over how and how much time they spend feeding their children, and when women have better access to healthcare, the prevalence of undernutrition decreases (Royal Tropical Institute [KIT] and Netherlands Development Organization [ 7 , 8 , 9 ]. The nutritional status of women (before, during, and after pregnancy) is intimately linked with the nutritional status of their children [ 5 , 7 , 8 , 10 ]. However, despite global efforts to address under-nutrition among women and children, the prevalence of under-nutrition remains high [ 7 , 8 ].
Even though it was at a slower pace, Ethiopia has made progress in the reduction of child stunting and maternal undernutrition in the last two decades. For example, the prevalence of stunting has decreased from 58% in 2000 to 37% in 2019, depicting an average decline of 1.25 percentage points a year [ 11 ]. On July 15 th , 2015, the Government of Ethiopia made a declaration to end child malnutrition by 2030, reaffirming its commitment to nutrition as a foundation for economic development. Accelerating progress towards this goal, set out in the Seqota Declaration , will require coordinated multisectoral efforts to increase nutrition, smart investments in infrastructure and technology, behavior change, and empowering communities to innovate and identify localized solutions to address malnutrition [ 12 ]. Eliminating undernutrition in Ethiopia would prevent losses of 8–11% per year from the gross national product [ 3 ].
Women are among the most at-risk for poor nutrition, particularly in Ethiopia, where economic and social disparities tend to be greater [ 13 ]. Even when food is available at home, women tend to be malnourished because of their gender status. Women shoulder “triple roles”, including their reproductive, productive, and social (community) responsibilities [ 14 , 15 , 16 ]. These roles place a significant burden on women, increasing their risk of malnutrition [ 13 ]. A study by [ 17 ] in Ethiopia indicated that women have a lower decision-making authority than men within households regarding decisions on the proportion of produced food consumed at home. Sociocultural and traditional norms often result in women consuming smaller amounts of food or foods with less nutritional diversity, as well as prioritizing the more nutritious food items for men. Effectively understanding sociocultural structures and gender dynamics has served to strengthen results from interventions for improved nutrition practices or enforcing nutrition programmes with education on rights and advocacy skills [ 18 ].
Despite a large body of literature on the nexus between nutrition and gender, this nexus is likely to vary and is not clear in many societies, such as Ethiopia. Hence, this paper contributes to literature on the nexus between nutrition and gender using primary data collected from Ethiopia. The objectives of this study were (i) to analyze the level of male and female farmers’ knowledge, attitude, and practice towards malnutrition and micronutrient (e.g. vitamin A, iron, and iodine) deficiency; and (ii) to assess the status of consumption of micronutrient-rich foods among farming households in the study area.
Materials and methods
Study area and data.
The study is based on primary data collected in December 2021 in three woredas (Angecha woreda in the South Nation Nationalities and People Region (SNNPR), Arsi Negelle, and Anna Sora woredas in the Oromia region) of Ethiopia. Angacha woredas are located in Kembata, Tembaro zone of the SNNPR in Ethiopia. Anna Sora and Arsi Negelle woredas are located in the Guji and West Arsi zones of the Oromia region in Ethiopia, respectively (Fig. 1 ). The study applied a mixed methods approach consisting of qualitative data collection through focus group discussion (FGD) and key informant interview (KII) and quantitative data collection through concisely formed questionnaires. The study used a convergence research design through which the qualitative and quantitative data are collected and analyzed during a similar timeframe. The KII and FGD served as the basis for including local context on key KAP issues found in the study. These were done by letting the FGD participants and key informants explain the reasons behind the KAP findings of this study.
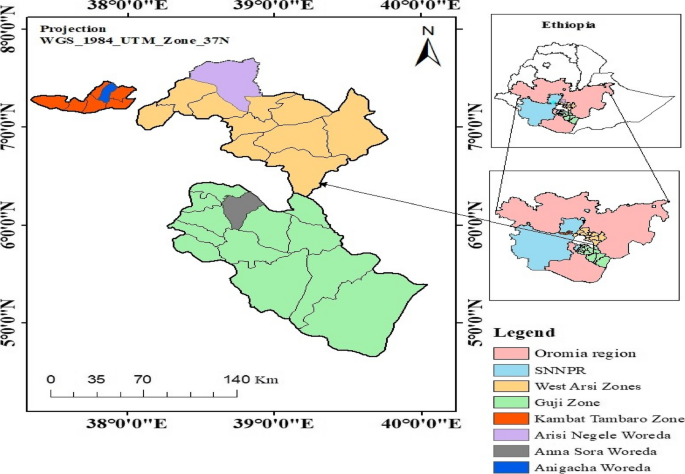
Location of the study area, Source: Authors
Sample and data collection methods
Sampling techniques.
Detailed FGD guides and questions were developed in close coordination with the quantitative data collectors. The discussions were conducted by the researcher to verify the self-reported information by the household respondents and to provide an in-depth understanding of the reported nutrition KAP. Discussions were held in the local language, which was a translation from English by professional local language interpreters. Illustrations related to the topics were used to stimulate discussion and engage the participants. On average, FGD consisted of six participants each. In order to avoid mutual influence in the responses, only one member of each household was randomly selected. A total of 21 groups, with a total of 126 participants, were formed separately in accordance with age, gender, and characteristics to allow the participants to speak freely. The farmer's training center was used as a venue for conducting FGD. Each discussion lasted no more than one hour. The FGDs were conducted with the following groups (Table 1 ).
A KII guide was carefully developed based on broader lines of inquiry to further explore gender and nutrition issues. The KIIs consisted of woreda agricultural extension coordinators, agricultural development agents working at kebele level, and health extension service providers. In each kebele, 1–3, key informants were invited to talk to the qualitative team to provid detailed information concerning household knowledge, attitudes, and practices towards malnutrition, vitamin "A", iron, and iodine deficiencies (Table 1 ). Moreover, the interview focused on issues related to gender and nutrition in the community. The interviews took place at their homes or offices separately in order to create a safe space for them to talk about this sensitive topic. Explicit consent to interview each person was collected and data from these interviews containing sensitive issues on gender and nutrition-related issues is kept under the strictest confidence. Data collected from KII were transcribed by the lead researcher who clearly understand the local language.
A multi-stage sampling procedure was applied to collect quantitative data. First, woreda was selected, followed by kebele, household, and individual respondent in each household. At the household level, the sample size of 311 respondents (185 males and 126 females) was calculated using Fisher’s formula [ 19 , 20 ]. Accordingly, from each household, one respondent was randomly selected, totaling 50 to 54 interviews per kebele (Table 2 ). The quantitative data collectors consisted of six locally based enumerators and two nutrition-sensitive research supervisors. Each interview took one hour per person on average. The interviews were conducted by trained enumerators at a farmer's home using a mobile data collection tool called Kobo Collect. Kobo Collect is a smart phone application applicable for field survey data collection. Of course, there are different smart phone applications useful for field survey data collection, such as Survey Solution. But Kobo Collect is easy to use in any rural area with no access to internet service. We used this tool for very large survey data collection, including the USAID. This tool has applied in several studies been including our previous published works (e.g. [ 16 , 17 ]).
Training of enumerators took place over four days, where three days occurred in a classroom setting, and one day in a selected community for pre-testing. The training schedule included the basics of a KAP study overall, interviewing methodology by enumerators, review of questionnaires, translation from English (orally) to local languages, and pre-testing of questionnaires in the field (see questionnaire attached as online Supplementary material ).
The pre-testing involved reviewing the qualitative and quantitative instruments to ensure the questions were clear, understandable, relevant to the intended topics, effective in providing useful information and, more importantly, to avoid redundant or unnecessary questions. Pre-testing also allowed enumerators and qualitative researchers to verify the correct local words and phrases of some of the complex ideas to which the study team wanted to gain insights on nutrition and gender. Pre-testing was conducted immediately prior to data collection in Kofele woreda of West Arsi zone, close to the study area.
Regarding components of the questionnaire used for quantitative data collection, the first part of the questionnaire comprised of demographic details such as age, marital status, sex of the household head, education level, household size, and farming experience. The latter part consisted of nutrition-related KAP questions. In total, the questionnaire is comprised of 41 KAP questions that encompass 16 knowledge questions, 7 practice questions, and 18 attitude-related questions.
The household interviews were conducted in the local language (Kambaatissa and Afan Oromo). Also, the questionnaire on the data collection platform, Kobo Collect, was in both Kambaatissa and Oromifa. This enables the enumerators to be intimately familiar with the questionnaire and be comfortable during interview time.
The participation of all research subjects in this study was voluntary and collected in a written form. Participants were informed before an interview or discussion took place about the purpose and were given the opportunity to refuse upon understanding the purpose. No exercise of undue inducement or any other form of coercion to participate in the study was permitted or accepted.
Data analysis
Qualitative data were analyzed using a narrative and content approach. Quantitative data were analyzed using descriptive statistics such as frequency distribution, mean comparison, percentages, and chi-square test. Moreover, a Likert scale was used to establish the respondent’s attitude towards malnutrition. SPSS software was used to analyze quantitative data.
Respondents socio-demographic characteristics
Table 3 presents the socio-demographic characteristics of the surveyed respondents. Male and female respondents account for 59.5% and 40.5% of the total (311) surveyed respondents, respectively. The study focused on men and women to get their opinion as well as gauge their nutrition knowledge, attitude, and practices. The same knowledge would facilitate an in-depth understanding of how men and women get involved and participate in decisions pertaining to the household. The overall average age of sampled respondents was 38 years old . The mean age of male and female respondents was 39.3 and 35.1, respectively. As a result of the T-test, the mean difference between males and females was statistically significant at 1% ( p = 0.001). This indicates that, male respondents become more aware of malnutrition as their age increases, contrary to female respondents. This implied that higher level of understanding and deeper experience in household nutritional issues come with age.
The household heads with longer farming experience are supposed to have better understanding of malnutrition of the household than the household heads with shorter farming experience. The mean year of farming experience of male and female respondents was 22.448 and 18.539, respectively. As a result of T-test, the mean difference between males and females was statistically significant at 1% ( p = 0.001). This indicates that, male respondents become more aware of malnutrition as their year of farming increases, contrary to female respondents. This implied that, household head with longer farming experience were to be more knowledgeable and practicable regarding household malnutrition.
More than half of the respondents (54%) had only primary level education. The importance of the level of education in gender equality is also underscored by the World Bank, which notes that the low levels of education, especially among women, represent a very serious constraint on development in most of the sub-Sahara African countries, Ethiopia not being exceptional. At the individual level, for example, education is perceived to be the ultimate liberator, which empowers a person to make personal and social choices [ 21 ]. The World Bank argues that education is also perceived to be the ultimate equalizer, particularly in promoting greater gender equity for women. Education is very important for farmers to understand malnutrition. Farmers who have high formal education are expected to be aware of malnutrition earlier than uneducated; because farmers with higher education levels were able to get information from different sources. The study results also revealed that the education level of the household head has a positive relationship and is statistically significant (chi 2 = 32.431, p = 0.000) at 1% of level (Table 3 ).
Nearly all (92%) respondents were married and the total average household size was 7, which is higher than the national average of 5. The mean household size for male and female-headed households was 7.2 and 7.0 respectively. The statistical analysis also, revealed that there is no significant difference (0.652) in the mean household size between male and female household head.
Knowledge and attitude towards malnutrition
Knowledge about malnutrition.
The results of the study on Table 4 show that, the majority of male (96%) and female (92%) can recognize if someone in their household is malnourished. The results of chi-square analysis indicate that recognition of malnutrition in the household has positive relationship but statistically not significant (x 2 = 2.5013; p = 0.114) (Table 4 ). About 85% of respondents know that lack of energy or weakness, are the main symptoms of being malnourished, while 58% and 84% of respondents know that weakness of the body's immune system and loss of weight/thinness, respectively, are the main symptoms of being malnourished in their respective households. FGD and KII participants also stated that, weakness, less immunity, chest pain, and headache were the most common symptoms of malnutrition at household and community level in the study area.
It was found that 87% of study participants had insufficient money to buy food, and 71% were unable to access multiple food groups. A majority of respondents (72%) suggested that eating foods enriched with micronutrients such as iron and vitamin "A" would prevent malnutrition problems in their household. Around 77% of respondents said that raising awareness among household members about making healthy food choices would help prevent malnutrition, while 75% and 49% said that increasing household income to afford nutritious food in the market and distributing food fairly among family members in the household would help prevent malnutrition (Table 4 ).
Attitude towards malnutrition
A Likert scale was used to establish the respondent’s attitude towards malnutrition. Nearly three-quarters (74%) of the total respondents were not likely to think that their household may have malnourished members, while 22% of the respondents were likely to think that there would be malnourished members in their household. The results of chi-square test indicate that attitude towards malnutrition in the household has positive relationship but statistically not significant (x 2 = 3.1058; p = 0.376). More than half of the respondents (57%) did not think malnutrition was a serious problem for household members' health and only 11% of respondents thought malnutrition was a very serious issue in their household. According to the results of the chi-square test, attitudes towards malnutrition in the household have a positive relationship, but the relationship is not statistically significant (× 2 = 3.1058; p = 0.376) (Table 4 ).
Consumption of iron-rich foods and iron -deficiency”
Knowledge about iron-rich foods and iron deficiency.
Table 5 presents respondent knowledge, attitude, and practice about iron-rich foods in the household. Regarding the sources of iron-rich foods, 78% of the respondents chose red meat as their major source of iron-rich foods, while 66%, 59%, and 34% chose teff (injera - a flat spongy Ethiopian bread mostly made of fermented teff flou r), butter, and pumpkin, respectively. 9% of the respondents had no knowledge about the sources of iron-rich food. A majority of the respondents (93%) knew about the benefits of eating iron-rich foods.
Body weakness, paleness, and headache were the most common symptoms of inadequate intake of iron-rich foods reported by the respondents. The majority of the respondents (88%) reported that they had heard about iron-deficiency anemia. Moreover, FGD and KII participants reported that there are incidences of anemia in the community.
Attitude towards consumption of iron-rich foods and iron-deficiency
More than half of the respondents (60%) think that it is a serious problem when their household members do not eat iron-rich foods. Nearly 7 out of 10 respondents (68%) think it is good to prepare meals with iron-rich foods such as red meat, chicken, liver, and dark green vegetables. Approximately 34% of respondents said it is extremely difficult for their households to prepare meals rich in iron, while less than 18% said it is not difficult. Almost three-quarters (73%) of the respondents are not confident in preparing meals with iron-rich foods, indicating a perceived ability to prepare iron-rich foods is a major barrier, yet most of them (88%) like the taste of iron-rich foods such as red meat, liver, injera, and chicken.
About 4 out of every 10 respondents (40%) think that it is not likely to have iron-deficient household members. Those with less than 10% think it would be most likely to have a household member who is iron deficient.
Consumption practices of iron-rich foods
About half (53%) of survey respondents consumed iron-rich foods in the last 24 h prior to the survey. The most commonly consumed iron-rich foods reported by the surveyed respondents were Teff (injera), legumes (mixed beans, baked beans, lentils, chickpeas), and dark leafy green vegetables.
Consumption of vitamin “A” rich foods
Knowledge about vitamin a-rich foods.
Table 6 presents respondents’ knowledge, attitude, and practice regarding vitamin A-rich food consumption in the household. Most surveyed respondents (89%) had heard about human health problems such as night blindness or inability to see in dim light in their community. About 83% had heard about Vitamin "A" deficiency or diseases caused by not consuming vitamin A-rich foods such as eggs, carrots, cheese, orange-fleshed sweet potatoes (OFSP), milk, or yoghurt (full cream dairy). In open-ended questions, respondents were asked to list vitamin "A" rich foods they are consuming in their households. Their responses were summarized as butter, milk, carrot, OFSP, and don't know for the purpose of presentation. Accordingly, most of the respondents (88%) reported carrots as a major source of vitamin "A", while 75%, 58%, and 39% noted milk, butter, and OFSP, respectively, as the major sources of vitamin "A". About 6% of the respondents were not aware of the major sources of vitamin "A" rich foods. Respondents were also asked about their knowledge about the benefits of eating vitamin "A" rich foods such as biofortified foods (e.g., orange-fleshed sweet potatoes) and fortified foods (e.g., fortified oil, wheat flour, and iodized salt). The majority of them, 90%, knew about the benefits of eating vitamin "A" rich foods.
Attitude towards vitamin A-rich foods
The respondents were asked how likely they thought it was that any of their household members lacked vitamin "A". Thus, nearly half (46%) believe that it is likely Over half (52%) of respondents said vitamin A deficiency is serious. Seventy-one percent of respondents feel confident that they can prepare meals containing vitamin-A-rich foods. About 50% of respondents thought it was somewhat difficult to prepare foods rich in Vitamin A. About 54% of respondents were feel less confident in preparing meals with vitamin-A-rich foods in the household. The majority (95%) of the respondents like the taste of vitamin-A-rich foods (Table 6 ). These results indicate that respondents perceive a positive attitude towards eating vitamin A-rich foods.
Consumption practices of vitamin A-rich foods
A majority (83%) of the respondents consumed vitamin "A" rich foods in the past 24 h prior to this survey. More than half (57%) of the respondents stated that all family members have equal access to vitamin A-rich foods. About 58% of the respondent’s household uses biofortified and fortified foods, such as orange-fleshed sweet potatoes. Whereas, 79% of their households use fortified foods such as fortified edible oil or wheat flour (Table 6 ).
Consumption of iodized salt
Knowledge about consumption of iodized salt.
Survey results indicate that a majority of respondents (69%) had information about human health problems related to iodine deficiency, such as goiter, apathy, and muscle weakness (Table 7 ). All of the respondents stated that their household uses salt to cook meals.
Attitude towards consumption of iodized salt
Results show that 32% of respondents think "it is likely " and 31% think "it is not likely " to lack iodized salt at home (Table 7 ). About (42%) think that the lack of iodized salt is a serious issue. About half (47%) were confident that they could prepare meals with iodized salt, and a slight majority (57%) stated that it was easy for their household to buy and use iodized salt. Only 14% said it was very difficult for their household to buy and use iodized salt.
Consumption practices of iodized salt
All surveyed respondents responded that they use salt to cook meals, with 39% using iodized salt and 45% using non-iodized salt (Table 7 ). All FGD and KII participants stated that the local community usually consumes non-iodized salt and on very rare occasions they consume packed and iodized salt. Some of the reasons given were lack of awareness about the existence of so-called iodized salt; they only knew about common or regular salt. Affordability (high price compared to the normal one) was also stated as a reason for not using iodized salt; even though they were aware of its existence, it was not available for purchase in local markets and shops. The results confirm that there is a knowledge and practice gap in the consumption of iodized salt in the study area.
This study was intended to assess the level of KAP among male and female farmers towards malnutrition and micronutrient deficiency; and the status of consumption of micronutrient-rich foods. The study findings revealed that, in general, the majority of respondents had poor knowledge, attitudes, and practice towards reducing malnutrition. This is in line with studies that revealed in all communities, regardless of differences in socioeconomic class and educational level, knowledge, attitudes, and practices about vitamin A were low [ 22 , 23 ]. However, this result contradicts the study`s finding that the majority of caregivers had knowledge of important baby and young child feeding practices [ 24 ].
39% and 71% of the respondents knew about the benefits of eating orange-fleshed sweet potatoes and fortified foods, respectively. Moreover, there were no such knowledge differences in the two group' about micronutrients. However, FGD and KII participants reflect contrary to the study findings regarding community knowledge of biofortified and fortified foods. They believe that, non-fortified oil is consumed more than fortified oil, as fortified oil was sold at a higher price in the study area. Also, there is a lack of knowledge on fortified oils' health benefits and types of nutrients added in the fortified oil. In SNNPR (Angecha woreda), fortified wheat flour is not known to the community. Farmers in Angecha woreda used to go to the "grinding mill house" and prepare the wheat flour locally. Even when they buy wheat flour from the market, it is the same and there is no access to fortified wheat flour. When they buy the processed and packed wheat flour, they don’t care whether it is fortified or not. Unlike survey participants, FGD and KII participants were reported that, Orange-fleshed sweet potato (OFSP) was not being produced in their community and people were not aware of the health benefits of eating OFSP. Even more educated professionals, such as development agents, health extension workers, and kebele coordinators who participated in KII, are not aware of the orange-fleshed sweet potato. In general, consumption of regular sweet potatoes is also quite rare in the study area. This misalignment between FGD and KII participants and survey respondents may be attributed to the misunderstanding of what fortified and biofortified foods are when asked in the survey, and the closed nature of a questionnaire does not allow enumerators to verify with follow-up questions. Similar findings were reported by WFP [ 25 ] in the KAP study on maternal nutrition, infant and young child feeding, sanitation and hygiene, and sexual and reproductive health, including obstetric fistula, in Chemba District, Sofala in Mozambique. In the [ 25 ] study, all FGD participants stated that they are unable to identify fortified foods, so they cannot distinguish fortified and non-fortified foods in the market, and they cannot make an informed choice when it comes to buying micronutrient supplements or fortified products. They indicated that they have never been told about the existence of these products by any communication channels in the communities in Mozambique. However, approximately 70% of survey respondents in the WFP study stated that they are aware of fortified foods. Moreover, 64% of survey respondents (66% of men and 62% of women), indicated they purchase fortified foods in the market for their children, and only a mere 3% indicated they do not.
Females are generally more adept than males at identifying the symptoms of malnutrition. However, concerning vitamin A and iodine food types and its deficiency male respondents had relatively better knowledge and consumption practice than female. Compared to female, male respondents had better understanding about fortified food in the study area.
According to the findings, a lack of income (87%) was the primary cause of a lack of a balanced diet in the study locations. As reported in Table 4 , the most crucial elements in preventing malnutrition are increasing sources of income so that people can purchase healthy food and raising awareness so that people may make wise food choices. Malnutrition was stated by both the FGD and KII respondents as the central problem in the study community/area. In terms of nutrition, the majority of the families under investigation continue to struggle with hunger and food insecurity in general, especially in Angecha woreda.
Conclusion and recommendations
The aim of this study was to assess nutrition-related knowledge, attitudes, and practice among male and female farmers in the Oromia and SNNP regions of Ethiopia. The findings from this study will inform the nutrition and gender-related development project implementation with a focus on its social behavior change communication (SBC) plan. Results indicate that female are generally more adept than male at identifying the symptoms of malnutrition. However, concerning vitamin A and iodine food types and its deficiency male respondents had relatively better knowledge and consumption practice than female.
Findings also show that the benefits of biofortified and fortified foods are still largely unknown among community members. Even more educated professionals such as development agents, health extension workers, and kebele coordinators who participated in KII are not aware of biofortified foods such as orange-fleshed sweet potatoes. To supplement the government of Ethiopia's efforts to increase consumption of micronutrient rich foods such as orange-fleshed sweet potatoes, high iron pearl millet, and fortified foods such as iron fortified wheat flour, iodized salt, and vitamin A fortified oils; non-governmental organizations such as Sasakawa Africa Association need to raise awareness about the benefits of consuming biofortified and fortified foods. Therefore, awareness creation is needed for extension experts and community members on the benefits of producing and consuming biofortified and fortified foods.
The findings show that the majority of the respondents are aware of the importance of consuming vitamin A and iron-rich foods, iodized salt, as well as production and consumption of fruits and vegetables and have a positive attitude towards them. However, there is still a gap in practice. As a result, more targeted campaigns may be required to increase community members' ability to adopt best practices while reducing barriers to consumption of nutritious diet. The current SBC strategy should change from an awareness creation campaign to a behavior change campaign, focusing on perceptions of the benefits and practices of the production and consumption of vitamin A and iron-rich foods as well as the consumption of iodized salt.
Availability of data and materials
Data used in this study are confidential. Data would be available up on reasonable request from the corresponding author.
Lowe NM. (2021) The global challenge of hidden hunger: perspectives from the field. Proc Nutr Soc. 2021;80(3):283–9. https://doi.org/10.1017/S0029665121000902 . Epub 2021 Apr 26 PMID: 33896431.
Article PubMed Google Scholar
Research Institute (IFPRI), I. F. P. Global nutrition report 2015: Actions and accountability to advance nutrition and sustainable development. 2015. https://ebrary.ifpri.org/digital/collection/p15738coll2/id/129443 . https://doi.org/10.2499/9780896298835 .
Nair MK, Augustine LF, Konapur A. Food-based interventions to modify diet quality and diversity to address multiple micronutrient deficiency. Front Public Health. 2016;3:277.
Article PubMed PubMed Central Google Scholar
The Inter-Agency Standing Committee (IASC). Gender equality measures in nutrition. 2018.
Google Scholar
Oniang’o, R, Mukudi, E. Nutrition and Gender. In Nutrition. 2002. https://www.unscn.org/files/Publications/Briefs_on_Nutrition/Brief7_EN.pdf .
Vlassoff C. Gender differences in determinants and consequences of health and illness. J Health Popul Nutr. 2007;25:47–61.
PubMed PubMed Central Google Scholar
Swiss Agency for Development and Cooperation (SDC). Gender analysis of maize post-harvest management in Zimbabwe: a case study of Shamva district. 2015.
KIT, SNV, CDI, and SDC. Intra-household dynamics and dietary diversity. Insights from Sustainable Nutrition for All in Uganda and Zambia. 2018.
Bundala N, et al. Gaps in knowledge and practice on dietary consumption among rural farming households; a call for nutrition education training in Tanzania. Int J Food Sci Nutr. 2020;71:341–51.
Black RE, et al. Maternal and child undernutrition: global and regional exposures and health consequences. The Lancet. 2008;371:243–60.
Article Google Scholar
Demographic and Health Survey. Addis Ababa: Central Statistical Agency; 2021.
Ministry of Health of Ethiopia, Seqota declaration. Ending undernutrition in Ethiopia by 2030. (2015).
Ersino G, Zello GA, Henry CJ, Regassa N. Gender and household structure factors associated with maternal and child undernutrition in rural communities in Ethiopia. PLoS ONE. 2018;13:e0203914.
Moser CON. Gender planning in the third world: Meeting practical and strategic gender needs. World Dev. 1989;17:1799–825.
Moser C. Gender planning and development: theory, practice and training. Routledge: 2012. https://doi.org/10.4324/9780203411940 .
Gebre GG, Legesse T, Fikadu AA. Food safety knowledge, attitude, and practice among male and female food handlers: evidence from fruit and vegetable producers in Ethiopia. Heliyon. 2023;9(6):e17301.
Gebre GG. Intra-household decision-making and their effects on women dietary diversity: evidence from Ethiopia. Ecol Food Nutr. 2022;61(6):705–27. https://doi.org/10.1080/03670244.2022.2135509 .
Root R. How to better integrate gender equality and nutrition. 2019.
Fisher RA. Statistical methods and scientific inference. New York: Hafner Press; 1973.
Orlet Fisher J, Mitchell DC, Wright HS, Birch LL. Parental influences on young girls’ fruit and vegetable, micronutrient, and fat intakes. J Am Diet Assoc. 2002;102:58–64.
World Bank. The World Bank Annual Report 2016. 2016.
Book Google Scholar
Charles Shapu R, Ismail S, Ahmad N, Ying LP, Abubakar Njodi I. Knowledge, Attitude, and Practice of Adolescent Girls towards Reducing Malnutrition in Maiduguri Metropolitan Council, Borno State, Nigeria: Cross-Sectional Study. Nutrients. 2020;12:1681.
Mills JP, Mills TA, Reicks M. Caregiver knowledge, attitudes and practices regarding vitamin A intake by Dominican children. Matern Child Nutr. 2007;3:58–68.
Asiimwe JK, et al. Knowledge, Attitudes and Practices of Caregivers of Children 0–23 Months in Eastern and Central Uganda. Food Nutr Sci. 2021;12:494–508.
CAS Google Scholar
World Food Program (WFP). On maternal nutrition, infant and young child feeding, sanitation and hygiene, and sexual and reproductive health, including obstetric fistula, in Chemba District, Sofala. 2021.
Download references
Acknowledgements
We would like to express our sincere gratitude to Hawassa University for supporting our study. We are also grateful to the two anonymous reviewers for their helpful comments to improve the paper.
Not applicable.
Author information
Authors and affiliations.
Faculty of Environment, Gender and Development Studies, Hawassa University, Hawassa, Ethiopia
Girma Gezimu Gebre & Deribe Kaske Kacharo
The Japan Society for the Promotion of Science (JSPS) Postdoctoral Research Fellowship Program, Ritsumeikan University, Kyoto, 603-8577, Japan
Girma Gezimu Gebre
Department of Agricultural Economics, College of Agriculture and Natural Resource, Werabe University, Werabe, Ethiopia
Derebe Ermias Chefebo
You can also search for this author in PubMed Google Scholar
Contributions
Girma Gezimu Gebre (PhD): Conceptualization, Methodology, Validation, Writing—original draft, Writing—review & editing. Derebe Ermias Chefebo (Mr): Data collection and manuscript revision. Deribe Kaske Kacharo (PhD): Data collection and manuscript revision.
Corresponding author
Correspondence to Girma Gezimu Gebre .
Ethics declarations
Ethics approval and consent to participate.
This study is based on survey methods involving interviewing farmers to answer questions about their socioeconomic and farming activities, knowledge, attitude and practices towards nutrition. The participation of all research subjects was voluntary. Participants were informed before an interview about the purpose of the study and given the opportunity to refuse upon understanding the purpose. Informed consent was obtained from all the literate participants and legal guardians of the illiterate participants. All procedures were performed in accordance with relevant guidelines.
Consent for publication
Competing interests.
The authors declare no competing interests.
Additional information
Publisher’s note.
Springer Nature remains neutral with regard to jurisdictional claims in published maps and institutional affiliations.
Supplementary Information
Additional file 1..
Household survey questionnaire.
Rights and permissions
Open Access This article is licensed under a Creative Commons Attribution 4.0 International License, which permits use, sharing, adaptation, distribution and reproduction in any medium or format, as long as you give appropriate credit to the original author(s) and the source, provide a link to the Creative Commons licence, and indicate if changes were made. The images or other third party material in this article are included in the article's Creative Commons licence, unless indicated otherwise in a credit line to the material. If material is not included in the article's Creative Commons licence and your intended use is not permitted by statutory regulation or exceeds the permitted use, you will need to obtain permission directly from the copyright holder. To view a copy of this licence, visit http://creativecommons.org/licenses/by/4.0/ . The Creative Commons Public Domain Dedication waiver ( http://creativecommons.org/publicdomain/zero/1.0/ ) applies to the data made available in this article, unless otherwise stated in a credit line to the data.
Reprints and permissions
About this article
Cite this article.
Gebre, G.G., Chefebo, D.E. & Kacharo, D.K. Knowledge, attitude and practice towards malnutrition and micronutrient deficiency among male and female farmers in Ethiopia. BMC Nutr 9 , 130 (2023). https://doi.org/10.1186/s40795-023-00791-0
Download citation
Received : 29 July 2022
Accepted : 02 November 2023
Published : 14 November 2023
DOI : https://doi.org/10.1186/s40795-023-00791-0
Share this article
Anyone you share the following link with will be able to read this content:
Sorry, a shareable link is not currently available for this article.
Provided by the Springer Nature SharedIt content-sharing initiative
- Malnutrition
- Micronutrient deficiency
BMC Nutrition
ISSN: 2055-0928
- General enquiries: [email protected]
Academia.edu no longer supports Internet Explorer.
To browse Academia.edu and the wider internet faster and more securely, please take a few seconds to upgrade your browser .
Enter the email address you signed up with and we'll email you a reset link.
- We're Hiring!
- Help Center
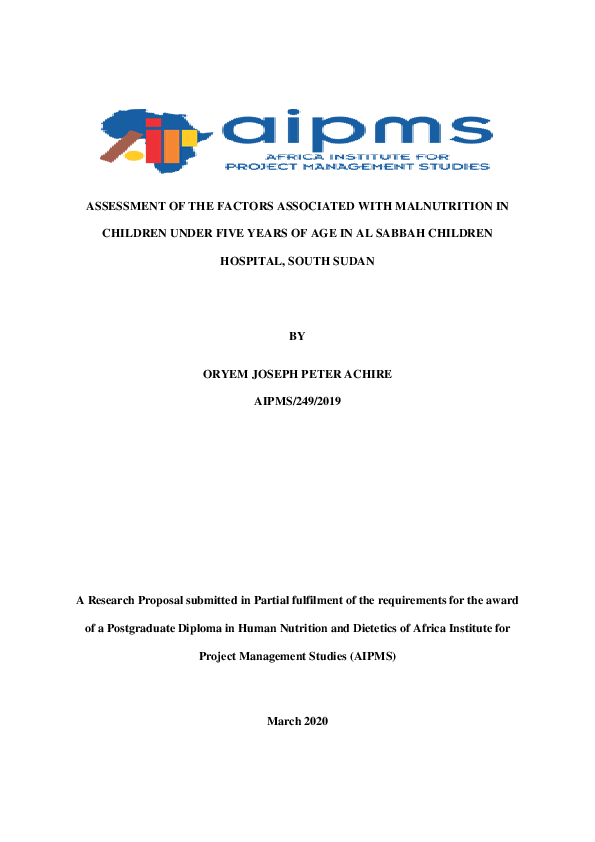
MALNUTRITION RESEARCH BY ORYEM JOSEPH

Related Papers
Polish Annals of Medicine
Taha H Musa
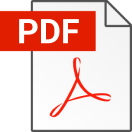
JUNAID ABID
Malnutrition shows a decline in health due to the disproportion of nutrients and energy in the body. Malnutrition is one of the main risk factors related to children's morbidity and mortality. It is estimated that about 52.50% of child mortality is linked to malnutrition and its associated diseases. 1 Malnutrition is a crucial medical problem in approximately every region of the globe and particularly in Southern Asia and Sub-Saharan Africa. In several developing nations, stunting, underweight, and micronutrient deficiencies among children are common due to insufficient nutrition and ABSTRACT Background: Malnutrition is one of the main health issues among children. Malnutrition is more prevalent in developing countries. Malnutrition among children is affected by many factors. These factors are studied in many parts of the world but they are understudied in most the areas of Pakistan. This study aimed to assess the incidence of malnutrition and its associated factors among children in Murree, Rawalpindi, Pakistan. Methods: This descriptive cross-sectional study was carried out among children of Murree, Rawalpindi for about 6 months from August 2021 to January 2022. Simple random sampling along with an established inclusion and exclusion criteria was applied to enroll 316 participants. A self-adapted questionnaire was applied to take data after taking ethical approval from the institutional research board and informed consent from the participants. Results: The incidence of underweight, normal weight, overweight and obesity among school children of study population was 22.80%, 35.40%, 26.90%, and 14.90% respectively. The association between malnutrition and gender (p=0.001), birth weight (p=0.01), supplementation intake (p=0.03), filtered water use (p=0.02), hygiene (p=0.01), vaccination status (p=0.04), recurrent infection history (p=0.02), socioeconomic status (p=0.04), mother education (p=0.04), mother occupation (p=0.03), awareness of parents about balanced diet (p=0.02), and family size (p=0.04) was statistically significant, whereas association between nutritional status and age group (p=0.05) was insignificant. Conclusions: The incidence of underweight, overweight, and obesity was remarkable among children. Many factors such as gender, birth weight, supplementation intake, filtered water use, hygiene, vaccination status, recurrent infection, socioeconomic status, mother education, mother occupation, parental awareness about a balanced diet, and family size were found to associate with malnutrition among children.
Abdul Tauqeer
Eng Abdirahman
Abstract Background: Malnutrition is a severe problem that affects a child’s cognitive and physical development. An adequate, balanced nutrition is crucial for a proper physical, emotional, and mental development. Malnutrition remains one of the most common causes of morbidity and mortality among children under 5 children throughout the World. It is the most important risk factor for the burden of disease causing about 300, 000 deaths per year directly and indirectly responsible for more than half of all deaths in children. Child malnutrition is one of the most serious public health problems in the developing world. Objective: To assess prevalence of malnutrition and associated factors among children aged 5 years at Lasanod district, Sool region, October 2014. Methods: An institution based cross sectional study was done among MCHs under five children found in Lasanod town on October 2014. The sample size of the study was 113. A pretested structured questionnaire and interview guide were employed to obtain the necessary information for this study. The structured questionnaire were originally prepared in English language and then translated into the Somali language and then back to English. The collected data was analyzing using SPSS Version 20.0 and triangulated. Bivariate and multivariate logistic regressions were carried out. Result: The analysis this study revealed that, 57.5%, 25.6.9% and 33.7% of children were underweight ,wasted, and stunted respectively and also revealed that 3.5%, 10.6% and 13.3% of children were severe underweight , severe wasted and severe stunted. The main associated factors of malnutrition were found to be mother’s age at first birth, place of delivery and duration of breastfeeding. Conclusion and recommendation: - This study revealed that, prevalence of malnutrition was high and it was the top list among the health problems in Lasanod district. In Somalia where most mothers do not know nutritional status and nutrient foods, a lot should be done by different sectors. We suggest that under nutrition prevention programs and strategies in the region as well as other regions should target Nutritional status among mothers and their children in extensive way to bring further positive changes related to diet.
Journal of Nutritional Science and Vitaminology
Francis Nkrumah
American Journal of Public Health Research
Irene Sumbele
International Journal Foundation
This is cross-sectional community based study conducted in Angola area in Khartoum State of Sudan during period of 2015-2017. The aim of the study was to assess nutritional status of under five years old children and its associated risk factors using anthropometric measurements, interview of childcare givers, and observation on nutrition status indicators and socioeconomic profile of families. 282 children and their caregivers were selected and investigated using cluster sampling techniques and predesigned questionnaires and checklist. The results revealed that 19.1 of the studied children were severely malnourished, and 4.7 were moderately malnourished with children in age of one to two years were mostly affective with P value of < 0.05. Family size and parent education level also were reported among the major risk factors of malnutrition with P value of < 0.05. 96.6% of the children had episode of diarrhea at least once, and 81.1% had respiratory tract infection at least once. Few were exposed to frequently to those infectious diseases. The study concluded that severe and moderate malnutrition affect almost quarter of the children in the area especially in the age group of one to two years. Poor education and awareness on how to maintain children health generally is the main risk factor especially knowledge and skills on the causes of malnutrition, proper young children food and feeding practices, breastfeeding, and utilization of available health services. The study recommended extensive health education program along with family support through provision of nutrients high density food. Study Area: The area has a total population of the area 56,534 with 10,386 under five year old children according to the area popular committees. Household with children aged 6 to 59 months were selected for the study along with their mothers. Diarrheal diseases, malaria and acute respiratory infections were the major health problem among young children in the areas. There are five health centers providing PHC services and 5 private clinics. Diarrheal diseases, malaria and acute respiratory infections were the major health problem among young children in the areas. There are five health centers providing PHC services and 5 private clinics. Sample size: 282 children and their mother were selected using the following formula and based on prevalence rate of nutritional deficiency diseases in Khartoum State of which was estimated to be 10% according Khartoum State Ministry of Health, 2009 n = z 2 pq* design defect (d) 2 Where: n = sample size, Z = 1.96, P = prevalence rate of nutritional deficiency diseases= (10%), q = 1-p, d = 0.05, Design defect=2 n =(1.96) 2 X 0.9 X0.X 2 = 138.2976 X 2 = 276.59 (0.05) 2 (14) The number was rounded to 282 children taken into account the refusal which was estimated to be 9%. Cluster sampling techniques was used by dividing the area into 6 clusters, in each 47 children's and their mothers/caretakers were selected randomly (15). Data were collected in predesigned questionnaire and check list through interview with mothers and measuring weight and high of their children. Indicators used during this study were: height –for –age (for chronic malnutrition), weight for weight (for acute malnutrition) and edema (16,17). Weight: The Staler 25kg hanging spring scale marked out in steps 0.1 kg, was used instrument was adjusted to zero before used, the child freed from heavy clothing (16,17). Height: Children up to 2years (23 months 85 cm length) of age were measured on horizontal measuring board. Children over two years of age (or over 85cm) were measured standing on horizontal surface against vertical measuring device. The height was read out as before, to nearest 0.1cm (29) Age: The birth data was entered on the recording form from birth certificates where this document was not available we used date of birth given by mothers Edema: Presence of edema also was recording after examination of children using finger press on the abdomen and legs.
Geleta Asebe
Abdul-Rasheed L Sulaiman , Ahmed Olusi
The road to good health is through good food which depends on the socioeconomic condition of the giver of the food. Numerous studies had been conducted on the causes of child malnutrition among children less than 5 years, that of children between 8 and 16 years with keen interest on the socioeconomic context of the giver has not been well documented. This lacuna is what this paper filled. Cross-sectional household survey was used for the study. 322 respondents were selected using a multi stage cluster sampling design. A well-structured pretested questionnaire was used to elicit the socio-demographic data from the respondents, while the respondents' nutritional status was calculated using the Body Mass Index (B.M.I) method. Chi-square and bivariate logistics regression were used to test the hypotheses. The study discovered that parental education and parental income were the fundamental factors affecting child malnutrition in the study location. Hence, government should ensure that education is made compulsory and affordable to everyone. Also, the menace of poverty should be adequately addressed.
RELATED PAPERS
Mathematics and Computers in Simulation
Jose Acevedo
Physical Review Letters
Joseph Lyding
Applied Sciences
Pavol Labun
Pensando - Revista de Filosofia
Vanessa Sievers de Almeida
Scientific Reports
Mariana Cruz Campos
Gregory Gatlin
Administracao De Empresas Em Revista
Cláudio José Luchesa
Cost Effectiveness and Resource Allocation
SHAKIL AHMED
Journal of entomology and zoology studies
Lakshya Dutta
Archives of Microbiology
Lubbert Dijkhuizen
Emerging Trends in Research Methodology: An Overview
Kaustuv Bhattacharyya, Ph.D.
Ciência & Educação (Bauru)
Everton Bedin
Atom Indonesia
Mukh Syaifudin
Mineralogia
Alif Athalla Putra
Procedia Computer Science
KARINA GUADALUPE CENOBIO ROJAS
Journal of Cerebral Blood Flow & Metabolism
Lorraine McSweeney
Ahmad Zaenuri
Journal of vestibular research
James Zacher
Buletinul Muzeului Științei și Tehnicii ”Ștefan Procopiu” Iași
Ana Lacramioara Leon
David Vopřada
Claire Pagetti
See More Documents Like This
- We're Hiring!
- Help Center
- Find new research papers in:
- Health Sciences
- Earth Sciences
- Cognitive Science
- Mathematics
- Computer Science
- Academia ©2024
- Research note
- Open access
- Published: 09 November 2018
Nutritional status and correlation with academic performance among primary school children, northwest Ethiopia
- Biachew Asmare 1 ,
- Mekuanint Taddele 1 ,
- Sileshi Berihun 1 &
- Fasil Wagnew 1
BMC Research Notes volume 11 , Article number: 805 ( 2018 ) Cite this article
72k Accesses
27 Citations
9 Altmetric
Metrics details
This study aimed to determine the association between nutritional status and academic performance among primary school children in Debre Markos Town, northwest Ethiopia, 2017.
The prevalence of stunting, underweight and wasting were 27.5% (95% CI 23.2–31.9%), 20.4% (95% CI 16.5–24.3%) and 8.7% (95% CI 6.2–11.5%), correspondingly. The low level of educational performance was significantly higher (p < 0.05) among the stunted, underweight and wasted children than that of the normal children. In multivariable logistic regression, age of the child (Adjusted Odds Ratio (AOR) = 0.177, 95% CI 0.07, 0.4), monthly income less < 1000.00 birr (AOR = 0.05, 95% Cl 0.02, 0.15), stunted children (AOR = 0.21, 95% CI 0.10, 0.43) and under-weight (AOR = 0.63, 95% CI 0.26, 0.84) were associated with academic performance. This study revealed that indicators of undernutrition were prevalent among school-age children. Thus, collaboration between the health and education sectors is required to alleviate the problem.
Introduction
Quality education plays a pivotal role in the economic, social and political development. Currently, getting children into schools is not enough; government ensure that children attain the basic knowledge and skills needed for personal well-being [ 1 ]. Primary school is an important stage in the development of consciousness and personality of the child [ 1 , 2 ].
Nutrition is also a vital component of human health, life, and brain development through the entire lifespan [ 3 ]. Balanced nutritious is crucial for endurance, physical growth, cognitive development and productivity [ 4 ]. As well, malnutrition is considered a pressing problem that affects the ability of children to learn and causes them to perform at a lower level in school [ 5 , 6 , 7 ]. Undernutrition is a major public health challenging affecting academic school achievement [ 8 ]. Ethiopia is one of the sub-Saharan African countries basically affected by child malnutrition. Previous studies conducted in different areas have shown that under nutrition is common among school-age children; it was reported in the form of stunting range from 11 to 48.7% and underweight from 7.2 to 59.7% [ 9 ]. A study conducted in eastern Ethiopia reported that the prevalence of stunting was 8.9%, of which, 2% had severe stunted among school-aged children [ 10 ]. Though evidence about the prevalence of malnutrition is well studied in Ethiopia, there is insufficient evidence regarding nutritional status allied with academic performance among school-age children [ 9 ]. The association between nutritional status and educational achievement among school-age children in developing countries have not been recognized well [ 9 , 11 ]. Stunting is referred as the best indicator for a chronic type of under nutrition [ 9 ]. Children who are stunted have low ability to learn at school and poor scholastic achievement [ 12 ]. Furthermore, poor feeding practices are associated with stunted and impaired brain development [ 6 , 13 ].
On this background, there is a necessity to overlook the relationship between nutritional status and educational performance among school-age children in the Debre Markos town. This study was aimed to determine nutritional status and correlation with academic performance among first cycle governmental primary school in Debre Markos Town, northwest Ethiopia.
Study area, setting and period
The study was conducted at Debre Markos town primary school. In the town, there were a total of 7473 population. Of them, 3831 were females studying in the school. Debre Markos is a city of East Gojjam Zone which is located 299 km away from Addis Ababa in the North. It had 15 governmental and 8 private primary schools. The study was conducted between January15 to March 17/2018.
Study design and population: An institutional-based, cross-sectional study was employed at primary school in Debre Markos town.
Sample size and sampling techniques: The sample size was determined using double population proportion by considering the following statistical assumptions: prevalence of stunting among school children (p 1 ) is 48% and p 2 is 29% and level of significance (α) = 5%, at 95% level of confidence, power of the study 90% and design effect 1.5. Finally, the overall sample size was found 442.
Sampling procedure: Participants were carefully chosen using a multi- stage sampling technique. Out of 15 primary schools, 4 schools were selected randomly by lottery method at stage one. Students were allocated proportionally at stage two. Then participants from selected schools were selected by systematic random sampling method using students’ name list by calculating ‘k’ value for each class.
Data collection methods: Data were collected using a pre-tested structured questionnaire and translated into the local language (Amharic version) by trained and experienced data collectors. Respondents were parents/caregivers of the children identified in the study schools. After students were systematically selected from the schools, their household address was traced in the students’ parent database. Then data collectors went to the children’s house to interview parents/caretakers. Training on the standard procedures and technique how to collect data were given for the data collectors and supervisors for 2 consecutive days. The contents on questionnaires were briefly described to reduce interviewer bias.
Data processing and analysis: Data were entered into Epi-Data version 3.1 and then exported to SPSS version 20 for further analysis. Emergency Nutrition Assessment (ENA) for SMART software was used to calculate the Z-score of weight-for-age, height-for-age and weight -for-height of the children. Variables which were significant at p-value < 0.2 in the bivariable analyses were candidate for entering into the multivariable logistic regression model to identify the independent predictors for academic performance. Before inclusion of factors, we checked multicollinearity using variance inflation factor (VIF) < 10.
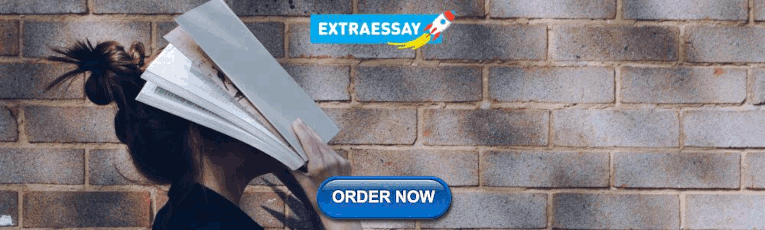
Definition of academic performance
The overall subjects the students were given in the academic year 2017/18 were considered to examine the academic achievements of the students. The annual average score was computed by taking the result of two consecutive semesters of the year. To verify the relationship between nutritional status and academic performance, average marks of the overall subjects the students received were either poor academic achievement or good academic achievement, in accordance with an average mark of 50%. This cut off average point was decided by considering the pass mark set by Ethiopian ministry of education.
Socio-demographic characteristics
A total of 436 children were included in the study with a response rate of 98.6%. Of them, 245 (56.2%) were males. The mean age of the study participants was 8.57 (± 1.12) ranging from 7 to 10 years. Majority of the study participants 398 (90.8%) were located in urban, 389 (89.2%) orthodox and 153 (35.1%) from grade one. Educational status of parents of the study participants showed that 81 (19.5%) mothers and 67 (17.7%) fathers had no formal education (Table 1 ).
Out of the 436 children, 37 (8.5%) were being sick in the last semester and only 140 (32.1%) of school-age children were attending preschool. Majority of study participants 403 (92.44) were traveled to school with in 2.18 km (Additional file 1 : Table S1).
Level of nutritional status in study participants: The overall prevalence of stunting, underweight and wasting were 27.5% (95% CI 23.2–31.9%), 20.4% (95% CI 16.5–24.3%) and 8.7% (95% CI 6.2–11.5%) respectively. The percentage of children having any kind of under nutrition (stunting, wasting and underweight) was 56.2%. The prevalence of stunting was significantly higher in males than females (Fig. 1 ).
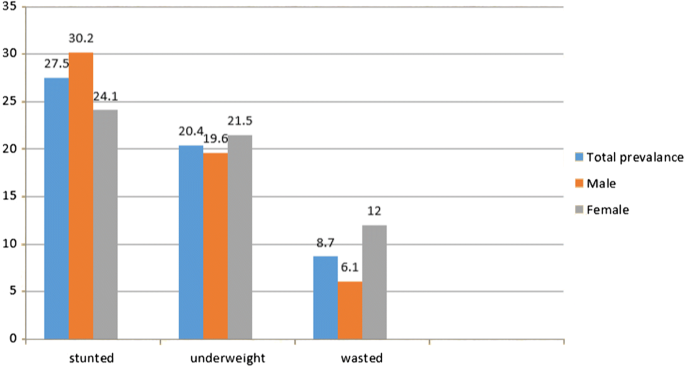
Prevalence of under nutrition by gender among children in Debre Markos town, Northwest, Ethiopia, 2017 (n = 436)
Nutritional status and academic performance: In comparison of median t-test analysis revealed that there was significant mean difference in all subject average score between children who were having any kind of undernutrition (Additional file 2 : Table S2).
After adjusting factors, age, family income, nutritional indicators (WAZ and HAZ) had significant positive associations with academic achievement of students ( p < 0.05). Age of the child (AOR = 0.177, 95% CI 0.07, 0.4), Monthly income less < 1000.00 birr (AOR = 0.05, 95% CI 0.02, 0.15), stunted children (AOR = 0.21, 95% CI 0.10, 0.43) and under-weight (AOR = 0.63, 95% CI 0.26, 0.84) were significantly associated with academic performance. Students those nutritional status had stunted were 79% less likely to score high academic performance as compared with normal. Students whose nutritional status had under-weight were 37% less likely to score high academic performance compared with their counterparts (Table 2 ).
Discussions
The aim of this study was to determine the relationship between nutritional status and academic performance among governmental primary school children. In this study, the prevalence of stunting, underweight and wasting were 27.5%, 20.4%, and 8.7% respectively. This finding was comparable with a study done in Zambia reported that 28.9% of stunted, 14.5% of underweight and 3. 9% of wasted [ 14 ]. In addition, the prevalence of stunting and wasting in this study was also in line with the findings of Sri Lanka among school-age children which indicated that the prevalence of under-nutrition in the central province was 26.6% stunted and 8.5% wasted [ 15 ], and in northwest Ethiopia, 27.1% stunted [ 16 ]. In contrast, this finding was higher as compared to other previous studies conducted in Brazil was found (14.9% stunted and 9.7% wasted) [ 9 ], in Kenya (24% stunted, 14.9% underweight, 9.7% wasted) [ 17 ], in Nicaragua (5% wasted) [ 18 ], in eastern Ethiopia (8.9% stunted) [ 19 ]. The reason for this observed discrepancy might be due to sociodemographic characteristics, area of sampling and study period.
Regarding factors, the present study revealed that age and monthly income were significant factors for academic performance among primary school children. This finding was consistent with a systematic review and meta-analysis showed that there is a strong association between academic performance and socio-economic status including age [ 20 ]. Compromised socio-economic status of a family was statistically associated with poor academic performance in children [ 21 ]. Similarly, other studies done in Southeast Ethiopia [ 22 ] and in Malaysia [ 23 ] reported that minimum wealth indexed score of the family were a positive association with poor academic performance. This might be due to balanced nutritional intake is required for adequate biological functioning affect such complex brain functions as the cognitive processes related academic performance [ 24 ].
Moreover, in developing countries macronutrient and micronutrient deficiencies are a devastating problem. Consequently, this obstacle has been either direct or indirect influence on children future of life [ 25 ]. Improved nutritional status has been exposed to have a positive and direct impact on academic performance of children [ 4 ]. In the current study, under-weight and stunting were associated factors for good academic performance among school-age children. This finding is in line with a study done in Sri Lanka [ 26 ] and in Uganda [ 27 ] which revealed that child with a high score of WHZ and HAZ had good academic performance as compared to their counterparts. Also, marasmic-kwashiorkor children may acquire delay brain development. Chronic types of malnutrition (stunting) had a negative impact on child cognitive development [ 20 ]. Similarly, a study done in Southeast Ethiopia revealed that higher score of HAZ was significantly associated with a higher academic score [ 20 ]. In this study, wasting (WHZ) was not statistical association with child academic performance. This non-significance effect might be due to the fact that wasting is acute malnutrition which implies a temporary nutritional disorder that may not negative substantial impact on academic performance [ 28 , 29 ].
The study revealed that indicators of undernutrition were prevalent among Debre Markos town primary school children. Age, income, HAZ and WAZ scores showed significant association with academic performance. Therefore, the government should paid attention to implement nutrition screening program and intervention strategy to improve academic performance at primary school children.
Limitation of the study
Finally, some important limitations of this study was cross-sectional nature of the study could not establish a cause and effect relationship between the dependent and independent variables. The other limitation of the study is that it was done in an urban areas which may inadequate representative for rural area.
Aregaw E. Multilevel analysis of factors affecting academic achievement of primary school students, Ethiopia, Gondar. Imperial J Interdiscip Res. 2016;2(2):1362–2454.
Google Scholar
Rastogi P, Nagesh K, Yoganarasimha K. Estimation of stature from hand dimensions of north and south Indians. Leg Med. 2008;10(4):185–9.
Article Google Scholar
Chambers R. Back matter-challenging the professions. Challenging the professions: frontiers for rural development. Rugby: Practical Action Publishing; 1993. p. 122–43.
Opoola F, Adebisi SS, Ibegbu AO. The Study of nutritional status and academic performance of primary school children in zaria, Kaduna state, Nigeria. Annal Bioanthropol. 2016;4(2):96.
Endalew B, Muche M, Tadesse S. Assessment of food security situation in Ethiopia: a review. Asian J Agric Res. 2015;9(2):55–68.
Grantham-McGregor SM, Fernald LC, Sethuraman K. Effects of health and nutrition on cognitive and behavioural development in children in the first three years of life Part 2: infections and micronutrient deficiencies: iodine, iron, and zinc. Food Nutr Bull. 1999;20(1):76–99.
Senbanjo IO, Oshikoya KA, Odusanya OO, Njokanma OF. Prevalence of and risk factors for stunting among school children and adolescents in Abeokuta, Southwest Nigeria. J Health Popul Nutr. 2011;29(4):364.
Duyar İ, Pelin C. Estimating body height from ulna length: need of a population-specific formula. Eur J Anthropol. 2010;1(1):11–7.
Sarma MSWD, Sivananthawerl T. The effects of nutritional status on educational performance of primary school children in the plantation sector in Nuwara Eliya Educational Zone. Trop Agric Res. 2013;24(3):14–203.
Anuar MLC, Low W, Harun F. Effects of nutritional status on academic performance of Malaysian primary school children. Asia Pac J Public Health. 2005;17:7–81.
Galal OHJ. The relationship between nutrition and children’s educational performance. Br J Nutr. 2003;25:11–20.
Shariff ZMBJ, Johnson NE. Nutrition and educational achievement of urban primary school children in Malaysia. Asia Pac J Clin Nutr. 2000;9(4):73–264.
Mendez MA, Adair LS. Severity and timing of stunting in the first two years of life affect performance on cognitive tests in late childhood. J Nutr. 1999;129(8):1555–62.
Article CAS Google Scholar
al GMe: Iodine deficiency, anthropometric status cognitive function of school age children in Hawassa town, Southern Ethiopia. Awassa: Hawassa University; 2009.
Herrador Z. Cross-sectional study of malnutrition and associated factors among school aged children in rural and urban settings of Fogera and LiboKemkem Districts. Ethiopia. PLoS ONE. 2014;9:9.
McClung IP. Growth deficits in school age children in Brazil. Am J Clin Nutr. 2008;50:687–96.
Degarege D, Degarege A, Animut A. Undernutrition and associated risk factors among school age children in Addis Ababa, Ethiopia. BMC Public Health. 2015;15(1):375.
Mesfin FBY, Worku A. Prevalence and associated factors of stunting among primary school children in Eastern Ethiopia. Nutr Diet Suppl. 2015;7:7–61.
Kar BRS, Chandramouli BA. Cognitive development in children with chronic protein energy malnutrition. Behav Brain Funct Nicaregu. 2008;4:31.
Jhanji S, Thomas B, Ely A, Watson D, Hinds C, Pearse R. Mortality and utilisation of critical care resources amongst high-risk surgical patients in a large NHS trust. Anaesthesia. 2008;63(7):695–700.
Jamison DT. Child malnutrition and school performance in China. J Dev Econ. 1986;20(2):299–309.
MoH. United Nations International Children’s Education Fund (UNICEF), author Inc The 1999 National micronutrient survey report. UNICEF. 2001;2001:93.
Hodgkin E. Nutrition and academic achievement: Are they related?. An international journal of faith, thought and action 2007.
Aturupane HGP, Wisniewski S. The impact of School Quality, Socio-Economic Factors and Child Health on Students’ Academic Performance. Evidence from Sri Lankan Primary Schools . 2002.
Mian RM. The nutritional status of school-aged children in an urban squatter settlement in Pakistan. Pak J Nutr. 2011;1(3):121–3.
McKnelly: nutrition and school performance. livestrongcom 2015.
Katona P. The interaction between nutrition and infection. Clin Infect Dis. 2008;46:8–1582.
Kleinman RE. Diet, breakfast, and academic performance in children. Ann NutrMetab. 2002;46(1):24–30.
CAS Google Scholar
Frongillo EA. Food stamp program participation is associated with better academic learning among school children. J Nutr. 2006;136:80–1077.
Download references
Authors’ contributions
BA, MT, SB and FW were participated in proposal writing, analyzing the data, and drafting the paper. MT and FW prepared the manuscript for publication. All authors read and approved the final manuscript.
Acknowledgements
The author’s deep gratitude goes to Debre Markos University, college of medicine and health sciences for proper review and approval of this paper. The authors would also like to extend their gratitude to Debre Markos town educational office and primary schools in the town, data collectors, and supervisors for valuable contribution for the success of this study.
Competing interests
The authors declare that they have no competing interests.
Availability of data and materials
Additional file: data collection tool.
Data set: The datasets used and/or analyzed during the current study are available from the corresponding author on reasonable request.
Consent to publication
Not applicable.
Ethics approval and consent to participate
The study was carried out after getting approval from Debre Markos University institutional review board (IRB). Written permission was obtained from educational office and administrative leaders of respective schools. The verbal (non-written) consent was obtained because written consent needed a certain level of education to read and sign the consent. Participants had the right to refrain from answering some questions or withdraw from the study process at any time. To maintain confidentiality, each and every one collected data were coded and locked in a separate room prior to enter into the computer. Following entered into the computer all data were protected by password.
Publisher’s Note
Springer Nature remains neutral with regard to jurisdictional claims in published maps and institutional affiliations.
Author information
Authors and affiliations.
College of Health Sciences, Debre Markos University, PO. Box: +251-269, Debre Markos, Ethiopia
Biachew Asmare, Mekuanint Taddele, Sileshi Berihun & Fasil Wagnew
You can also search for this author in PubMed Google Scholar
Corresponding authors
Correspondence to Biachew Asmare or Fasil Wagnew .
Additional files
Additional file 1: table s1..
General characteristics of study participants in Debre Markos town, March, 2017 (n = 436).
Additional file 2: Table S2.
Prevalence of low educational performance (marks < median of student result of nutritional status of children, Debre Markos, 2017 (n = 436).
Rights and permissions
Open Access This article is distributed under the terms of the Creative Commons Attribution 4.0 International License ( http://creativecommons.org/licenses/by/4.0/ ), which permits unrestricted use, distribution, and reproduction in any medium, provided you give appropriate credit to the original author(s) and the source, provide a link to the Creative Commons license, and indicate if changes were made. The Creative Commons Public Domain Dedication waiver ( http://creativecommons.org/publicdomain/zero/1.0/ ) applies to the data made available in this article, unless otherwise stated.
Reprints and permissions
About this article
Cite this article.
Asmare, B., Taddele, M., Berihun, S. et al. Nutritional status and correlation with academic performance among primary school children, northwest Ethiopia. BMC Res Notes 11 , 805 (2018). https://doi.org/10.1186/s13104-018-3909-1
Download citation
Received : 24 July 2018
Accepted : 01 November 2018
Published : 09 November 2018
DOI : https://doi.org/10.1186/s13104-018-3909-1
Share this article
Anyone you share the following link with will be able to read this content:
Sorry, a shareable link is not currently available for this article.
Provided by the Springer Nature SharedIt content-sharing initiative
- Academic performance
- Nutritional status
- School age children
BMC Research Notes
ISSN: 1756-0500
- Submission enquiries: [email protected]
- General enquiries: [email protected]
Click through the PLOS taxonomy to find articles in your field.
For more information about PLOS Subject Areas, click here .
Loading metrics
Open Access
Peer-reviewed
Research Article
The double burden of malnutrition in India: Trends and inequalities (2006–2016)
Roles Conceptualization, Data curation, Formal analysis, Investigation, Methodology, Visualization, Writing – original draft, Writing – review & editing
* E-mail: [email protected]
Affiliation Poverty, Health and Nutrition Division, International Food Policy Research Institute, Washington, DC, United States of America

Roles Conceptualization, Visualization, Writing – original draft, Writing – review & editing
Roles Methodology, Validation, Writing – review & editing
Roles Data curation, Formal analysis, Methodology, Visualization
Roles Data curation, Formal analysis, Methodology, Validation, Visualization
Affiliation FHI360, Hanoi, Vietnam
Roles Conceptualization, Funding acquisition, Investigation, Writing – review & editing
Roles Conceptualization, Data curation, Investigation, Methodology, Supervision, Writing – review & editing
- Phuong Hong Nguyen,
- Samuel Scott,
- Derek Headey,
- Nishmeet Singh,
- Lan Mai Tran,
- Purnima Menon,
- Marie T. Ruel
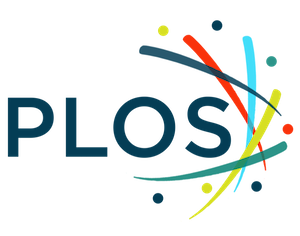
- Published: February 25, 2021
- https://doi.org/10.1371/journal.pone.0247856
- Reader Comments
Rapid urban expansion has important health implications. This study examines trends and inequalities in undernutrition and overnutrition by gender, residence (rural, urban slum, urban non-slum), and wealth among children and adults in India. We used National Family Health Survey data from 2006 and 2016 (n = 311,182 children 0-5y and 972,192 adults 15-54y in total). We calculated differences, slope index of inequality (SII) and concentration index to examine changes over time and inequalities in outcomes by gender, residence, and wealth quintile. Between 2006 and 2016, child stunting prevalence dropped from 48% to 38%, with no gender differences in trends, whereas child overweight/obesity remained at ~7–8%. In both years, stunting prevalence was higher in rural and urban slum households compared to urban non-slum households. Within-residence, wealth inequalities were large for stunting (SII: -33 to -19 percentage points, pp) and declined over time only in urban non-slum households. Among adults, underweight prevalence decreased by ~13 pp but overweight/obesity doubled (10% to 21%) between 2006 and 2016. Rises in overweight/obesity among women were greater in rural and urban slum than urban non-slum households. Within-residence, wealth inequalities were large for both underweight (SII -35 to -12pp) and overweight/obesity (+16 to +29pp) for adults, with the former being more concentrated among poorer households and the latter among wealthier households. In conclusion, India experienced a rapid decline in child and adult undernutrition between 2006 and 2016 across genders and areas of residence. Of great concern, however, is the doubling of adult overweight/obesity in all areas during this period and the rise in wealth inequalities in both rural and urban slum households. With the second largest urban population globally, India needs to aggressively tackle the multiple burdens of malnutrition, especially among rural and urban slum households and develop actions to maintain trends in undernutrition reduction without exacerbating the rapidly rising problems of overweight/obesity.
Citation: Nguyen PH, Scott S, Headey D, Singh N, Tran LM, Menon P, et al. (2021) The double burden of malnutrition in India: Trends and inequalities (2006–2016). PLoS ONE 16(2): e0247856. https://doi.org/10.1371/journal.pone.0247856
Editor: Srinivas Goli, University of Western Australia, AUSTRALIA
Received: August 18, 2020; Accepted: February 15, 2021; Published: February 25, 2021
Copyright: © 2021 Nguyen et al. This is an open access article distributed under the terms of the Creative Commons Attribution License , which permits unrestricted use, distribution, and reproduction in any medium, provided the original author and source are credited.
Data Availability: All relevant data are within the manuscript and its Supporting Information files.
Funding: This research is funded by the Bill & Melinda Gates Foundation through POSHAN, led by International Food Policy Research Institute. The funders had no role in study design, data collection and analysis, decision to publish, or preparation of the manuscript.
Competing interests: The authors have declared that no competing interests exist.
Introduction
Urban expansion is occurring rapidly in many parts of the world. Whereas approximately 55% of the world’s population currently lives in urban areas, this figure is expected to rise to 60% in 2030 and 68% by 2050 [ 1 ]. Urban populations often grow faster than the capacity of cities to support them, with slum populations increasing as a result, particularly in South Asia. An estimated 881 million people worldwide lived in slums in 2014, and this number is projected to increase to two billion in 2030 [ 2 ]. India has the second largest urban population in the world; the proportion of India’s population living in urban areas is expected increased from 34% (460 million) in 2018 to 40% (607 million) in 2030 and 53% (876 million) in 2050 [ 1 ]. Several large cities in India currently have more than 40% of their population residing in slums [ 3 ].
As the world’s population becomes more urbanized, many challenges related to the rapid expansion of urban areas and the explosion of slums arise, including overcrowding, lack of basic services, substandard housing, limited access to healthcare, unsafe water, and inadequate sanitation [ 4 ]. Among the many health and nutrition challenges faced by urban dwellers, the double burden of malnutrition–i.e. the coexistence of undernutrition and micronutrient deficiencies and of overweight/obesity–is becoming a major public health concern globally and in urban areas in particular [ 5 , 6 ]. Urbanization is a strong driver of the double burden of malnutrition because it causes remarkable shifts in urban food systems (particularly the availability of cheap ultra-processed food and beverages), in urban diets (convenient and processed foods), and in lifestyles (lower levels of physical activity related to the labor-saving technologies, more sedentary work, more leisure, and motorized transportation) [ 5 ].
While many studies from low- and middle- income countries (LMICs) have reported malnutrition disparities between socioeconomic groups or by urban–rural residence, very few have examined the intersection between wealth and area of residence, and none have done so in India (S1 Table in S1 File ). Understanding inequalities in different forms of malnutrition related to wealth status and residence is vital to identifying solutions to achieving equality, a core cross-cutting theme of the Sustainable Development Goals.
In India, health has improved in recent decades, but progress has been uneven and inequitable. Maternal and child undernutrition disproportionately burdens the poor [ 7 , 8 ], especially the urban poor where undernutrition and other health conditions are worse than among their wealthier counterparts [ 9 , 10 ], and sometimes even worse than in rural areas [ 11 ]. Urban slum-dwellers are among the most vulnerable population groups and face high mortality and undernutrition, as well as limited access to maternal and child health care services [ 11 , 12 ]. Furthermore, slum-dwellers are burdened by the low-quality of public service provision such as access to water, sanitation, decent housing, and health insurance [ 13 – 15 ]. Despite these widening gaps, understanding inequalities in the different forms of malnutrition in India has not received sufficient attention. Most health inequality studies used previous National Family Health Survey (before 2006) and some used data on some select states, but no study to our knowledge has examined trends and wealth inequalities within place of residence over time. Our study aimed to fill this gap by analyzing trends and inequalities in child and adult undernutrition and overweight/obesity by gender, area of residence, and wealth in India from 2006 to 2016.
Data sources
We used data from two rounds of nationally representative surveys—the National Family Health Surveys in 2005–2006 (NFHS-3) [ 16 ] and 2015–2016 (NFHS-4) [ 17 ]. Both surveys follow a systematic, multi-stage stratified sampling design used in Demographic and Health Surveys in many other countries. The first stage involved selection of primary sampling units (i.e. villages in rural areas and Census Enumeration Blocks in urban areas) using probability proportional to population size. The second stage involved the random selection of 22 households from each primary sampling unit. These surveys gathered demographic, health and nutrition data on 109,041 and 601,509 sample households, respectively. While NFHS-3 is representative at the state level, NHFS-4 is representative at both state and district levels, and both surveys are also representative at urban/rural levels. In this study, we used data from girls 0–59 mo (n = 24,756 for 2006, 124,525 for 2016), boys 0–59 mo (n = 26,799 for 2006, 135,102 for 2016), women 15–49 y (n = 118,474 for 2006, 667,258 for 2016) and men 15-54y (n = 74,338 for 2006, 112,122 for 2016) with available anthropometric measurements.
The outcomes of interest were anthropometric measures for girls, boys, women and men. Children’s weight and length/height measurements were used to derive z-scores by comparing each child’s anthropometric measurements to the WHO age- and gender-appropriate child growth standards. Two indicators were calculated: length/height-for-age z-score (HAZ) and body mass index-for-age z-score (BMIZ, using children’s weight and height for age). Stunting was defined as HAZ < -2 and overweight as BMIZ >1. The height and weight measures of women and men were used to calculate body mass index (BMI) in kg/m 2 . Underweight was defined as BMI <18.5 kg/m 2 and overweight as BMI ≥25 kg/m 2 . We use a combination of overweight and obesity given extensive epidemiological research highlighting the risks of noncommunicable diseases associated with BMI ≥25 kg/m 2 [ 18 ].
Definition of rural, urban slum and urban non-slum households
We compared outcomes in rural, urban slum and urban non-slum households. The urban and rural clusters in NFHS were chosen using the classifications of the Census of India 2011 [ 19 ]. Urban areas were defined as Statutory Towns (including all places with a municipality, corporation, cantonment board or notified town area committee) and Census Town (including all other places with a minimum population of 5,000; at least 75% of the male working population engaged in non-agricultural pursuits; and a density of population of at least 400 persons/square kilometer).
There is no agreement on how to define and hence identify a slum (S2 Table in S1 File ). After reviewing literature on slum definitions, we applied the UN-HABITAT definition [ 20 , 21 ] which ascribes a household to a slum based on satisfying at least two of the following four criteria: 1) overcrowding/insufficient living space (three or more people sharing a room), 2) non-durable housing (if the wall, roof or floor of the house is built with unimproved materials), 3) lack of access to improved and adequate safe drinking water, and 4) lack of access to adequate sanitation facility. All these criteria applied for households defined as urban in NFHS only. We compared the percentage of slum households using our definition to the percentage of identified slums reported in NFHS-3 and NFHS-4 in 8 large cities and found similar results.
Wealth index
A wealth index was constructed using pooled data from 2006 and 2016 to compare wealth over time. A principal component analysis was used to construct the index, which included household ownership of 17 assets (car, motorbike, bicycle, television, radio, computer, refrigerator, mobile phone, watch, fan, bed, mattress, table, chair, pressure cooker, sewing machine, water pump), livestock (cow, goat, chicken), house and land [ 22 ]. The first component derived from the component scores explained ~80% of the variance and was then used to categorize wealth into quintiles for urban slum, non-slum and rural separately; the lowest quintile (Q1) represents the poorest 20% and the highest quintile (Q5) represents the richest 20% of the pooled population.
Data analysis
Descriptive analyses were used to summarize sample characteristics. Graphical methods were used to visualize how stunting in children, underweight in adults, and overweight in both children and adults differed by residential areas over time. These changes were examined using absolute change, the percentage point (pp) difference between the two surveys. The statistical significance of changes between 2006 and 2016 was tested using an adjusted Wald test.
To examine inequalities in the burden of malnutrition by wealth index within residential areas, we first used equity plots disaggregated by residence and wealth quintile for each age/sex group, to visualize the prevalence of outcomes in 2006 and 2016. We then examined absolute and relative wealth inequalities for each outcome by residential area using the slope index of inequality (SII) and the concentration index (CIX) [ 23 , 24 ]. These two complex measures are widely used to summarize health inequality in a series of subgroups such as wealth quintiles, taking into account the entire distribution of outcomes over the five quintiles, and weighted by the sample size of each quintile. SII represents the absolute difference (percentage points, pp) in predicted values of outcomes between the two extremes of the wealth distribution (lowest and highest wealth quintile). CIX is a relative measure of inequality; it is related to the Gini coefficient and can be expressed in the form of a curve that ranks the sample wealth index on the x-axis and plots cumulative health outcomes on the y-axis. Both SII and CIX take values from -100 to +100, where positive values indicate the outcome is more prevalent among the rich and negative values indicate the outcome is more prevalent among the poor. These two inequality measures were also used to assess changes in inequalities over time. All analyses were performed using Stata version 15.1. All models were adjusted for age, cluster sampling design and survey sampling weights. Two-sided P<0.05 was used for statistical significance.
Ethics statement
This study was a secondary data analysis of NFHS data, which was approved by the institutional review board of the International Institute of Population Sciences, India. All respondents in the NFHS undergo an informed consent process for participation in the survey. The enumerators read a detailed informed consent statement to the respondents, informing about the survey, describing the procedure, and emphasizing the voluntary nature of participation. Once the respondent agreed to participate in the survey, the interviewer signed the questionnaire to indicate that the informed consent statement had been read to the respondent.
Sample characteristics
In 2006, two thirds of households lived in rural, 26% in non-slum and 7% in slum areas ( Table 1 ). As per survey design, the share of households in each residential area was similar between 2006 and 2016. Most houses in rural clusters were built of non-durable material (71% in 2006 and 57% in 2016), were crowded (42% in 2006 and 35% in 2016) and lacked an adequate sanitation facility (77% in 2006 and 57% in 2016). The prevalence of insufficient living space was highest for urban slum dwellers (~72% in both years). Wealth and living conditions improved over time for all three residential areas.
- PPT PowerPoint slide
- PNG larger image
- TIFF original image
https://doi.org/10.1371/journal.pone.0247856.t001
Differences in stunting, underweight, and overweight/obesity by residential area and time
Child stunting dropped by 10 percentage points (from 48% to 38%) over the 10-year period from 2006 to 2016 ( Table 2 ), with no statistically significant differences between boys and girls. The reduction in stunting was significantly higher among children from rural and urban slum households (-9 to -12 pp, depending on area and sex) than among those in urban non-slum households (-6 pp) ( Fig 1 and Table 2 ). At both time points, stunting was similarly high among children from rural and urban slum households (~50% in 2006 and ~40% in 2016), but much lower among those from urban non-slums (33% in 2006 and 27% in 2016 for girls, 34% in 2006 and 29% in 2016 for boys).
(A) Girls (0-5y) stunting, (B) Boys (0-5y) stunting, (C) Girls (0-5y) overweight/obese, (D) Boys (0-5y) overweight/obese, (E) Women (15-49y) underweight, (F) Men (15-54y) underweight, (G) Women (15-49y) overweight/obese, (H) Men (15-54y) overweight/obese.
https://doi.org/10.1371/journal.pone.0247856.g001
https://doi.org/10.1371/journal.pone.0247856.t002
Overweight/obesity affected ~8% of children and did not change over time. Overweight/obesity prevalence was slightly lower (by ~3–4 pp) in rural compared to slum and non-slum households for both girls and boys.
The prevalence of underweight also declined markedly between 2006 and 2016, from 36% to 23% among women and 34% to 20% among men ( Fig 1 and Table 2 ). Larger declines were observed among adults living in rural (14–16 pp), followed by urban slum (12–14 pp) and non-slum dwellers (8–10 pp). By contrast, the prevalence of overweight/obesity in adults almost doubled between 2006 and 2016 (from 10 to 21%) and the relative increases were higher in adults from rural households (103–136%) compared to those from slum (83–88%) and non-slum households (24–49%) ( Table 2 ).
Wealth inequality in stunting, underweight and overweight/obesity by gender and residential area
Child stunting prevalence was higher among poorer compared to wealthier households across all residential areas and survey years, as shown by the equity plots and negative SII and CIX ( Fig 2 and Table 3 ). Large wealth gaps (Q5 vs. Q1) were found among girls and boys in all residential areas and in both years (SII ranged from -33 to -19 pp). The inequality gaps in stunting decreased between 2006 and 2016 in urban non-slum households but did not statistically significant change over time for rural or urban slum households. Regarding overweight/ obese, wealth gaps were small for children in both rural or urban slum households (SII: 0.8 to 7pp) but increased slightly in urban non-slum households between 2006 and 2016.
(A) Girls (0-5y), (B) Boys (0-5y), (C) Women (15-49y), (D) Men (15-54y). 1 Within each residential area in a given year, each dot represents the prevalence of a given burden for a quintile subgroup and the spread of the dots indicates the inequality in the burden of malnutrition; 2 Negative values mean that the burden is more concentrated in the poor and positive values mean that the burden is more concentrated in the wealthy.
https://doi.org/10.1371/journal.pone.0247856.g002
https://doi.org/10.1371/journal.pone.0247856.t003
Adult underweight affects the poor more than the rich across all residential areas and survey years, as shown by the equity plots and negative SII and CIX ( Fig 2 and Table 3 ). The wealth gaps in underweight among adults were large for all residential areas and in both years (SII -22 to -35 pp in 2006 and -12 to -23 pp in 2016). The wealth gaps in adult underweight were narrower in 2016 than in 2006 (p< 0.05) for both women and men in rural and non-slum households, mainly due to improvements among the poor in these residential areas.
Both women and men across all wealth quintiles and residential areas are becoming more overweight/obese over time, with the only exception of the highest quintile in urban non slums who have stabilized at an alarmingly high prevalence of about 40% ( Fig 2 ). The CIX values for adult overweight/ obesity were all positive, indicating that the problem was more concentrated in the high wealth quintile households. The differences between wealth groups were large in all residential areas (SII: +16 to +29 pp) and increased between 2006 and 2016 in rural (SII: +21 to +28 pp) and urban slum areas (SII: +22 to +29 pp) between 2006 and 2016 but decreased in non-slum areas for both women and men (SII: +29 to +16 pp) ( Table 3 ).
Although India has made progress in reducing undernutrition in the past decade, wealth inequalities within residential areas and the rapid rise in adult overweight/obesity are major challenges. India still has a large and relatively impoverished rural population, but also a rapidly growing urban population, of which a large proportion lives in urban slum areas. We have shown that the prevalence of stunting among children and underweight among adults (and the wealth inequalities within area of residence) have declined over time but remain significantly higher in rural and urban slum compared to urban non-slum households. By contrast, overweight/obesity among both men and women doubled over time in rural and urban slum households, reaching 15% and 22%, respectively, and 33% in urban non slums. Overweight/obesity are highly concentrated in upper wealth quintiles across all three areas of residence. Overweight/ obesity in children, however, remained at a relatively low 8% between 2006 and 2016.
India’s progress in reducing stunting among children aged 0–59 months (48% to 38%) likely reflects improvements in income and socioeconomic status, parental education, access to health services and improvements in water and sanitation [ 25 ]. We now show that stunting is almost as prevalent in urban slums as in rural households and, relative to these two areas, lower in urban non-slum households. Between 2006 and 2016, wealth inequalities in stunting declined in urban non-slum households but persisted in rural and urban slum households. Our findings highlight the precarious health and nutrition conditions of children living in poor urban households and call for renewed efforts to reduce inequalities in access to social and health programs for both urban slum and rural households [ 11 ]. Strategies to improve health and nutrition for urban slum households should be tailored to the specific needs of these populations and should be mainstreamed in the implementation of the Sustainable Development Goals and the New Urban Agenda [ 4 ].
Among adult men and women, we found large reductions in underweight from 2006 to 2016, especially in rural and urban slum areas, but a doubling in overweight/obesity over the same period. This is consistent with the global trend observed in LMICs, where the prevalence of underweight has declined substantially in the past few decades whereas rates of overweight/ obesity have increased [ 6 ]. We found an 8 pp increase in prevalence of overweight/obesity among Indian women (from 12.5% to 20.5%), compared to 5.6 pp among adult women in LMICs (from 24.4% to 30%) or 4.8 pp among adult women worldwide (from 34.4% to 39.2%) [ 26 ]. In our study, the relative increase in overweight/obesity was greater in rural compared to urban slum or urban non-slum households, a finding consistent with results from a previous report for women in LMICs [ 27 ]. The highest prevalence of adult overweight/obesity in India is currently found in urban non-slum households (at 33% for women and 29% for men) but, if past trends continue, rural and urban slum dwellers will quickly catch up to their urban counterparts. These findings point to the emergence of a significant double-burden of undernutrition and overnutrition in both rural and urban slum areas.
We also found large but narrowing wealth inequalities over time in underweight prevalence in all three residential areas, with similar patterns across adult men and women. India is unusual, compared to other countries, in having a high prevalence of underweight among both men and women, and a similar pattern emerging for overweight/obesity in 2016 which is only slightly lower among men (e.g. 22% and 19% in women and men living in urban slums and 33% and 29% respectively among those living in urban non-slums). Typically, overweight prevalence is higher among women compared to men at early stages of the nutrition transition [ 28 , 29 ]; for example, women’s overweight prevalence in 2016 exceeds that of men’s by 16pp in Africa (39% vs 23%) and by 13pp in LMICs (32% vs.19%) [ 26 ]. These differences are mainly due to different contextual factors that drive gender differences in food consumption, physical activity, sedentary lifestyles, and sociocultural preferences and beliefs [ 28 , 29 ]. We do not find any hypothesis to explain why women are not more overweight/obese than men in India.
While improvements in income and SES are key economic drivers of reductions in underweight among adults, these improvements are also contributing to the rapid rises in overweight/obesity [ 8 , 30 , 31 ]. A recent study in India showed that improvements in socio-economic status between 2006 and 2016 explain 29% of the reduction in underweight and 46% of the increase in overweight/obesity [ 8 ]. As incomes rise, people consume more diverse foods, a positive change, but also increase their consumption of processed foods and meals consumed away from home, thereby increasing their intake of energy, saturated fats and oils, added sugars, and salt [ 6 ]. Urbanization and economic growth are also typically associated with reductions in physical activity at work, in daily commute, and in leisure [ 6 ], which exacerbate the effects of increased energy consumption on overweight/obesity and diet-related non-communicable diseases.
India has a robust policy framework to address malnutrition in all forms [ 32 ]. Several evidence-based nutrition interventions in the first 1000 days are delivered through a wide range of national programs, including the Integrated Child Development Services, National Health Mission, Mid-Day Meal Scheme, Targeted Public Distribution System, and National Food Security Mission, among others. The 2017 National Nutrition Strategy [ 33 ] and POSHAN Abhiyaan, India’s national nutrition mission launched in early 2018, provide an updated strategic framework for action to improve nutritional outcomes by holistically addressing the multiple determinants of malnutrition through cross-sectoral convergence and contextualized planning at each level of the implementation process. Many policies and programs are heavily focused on undernutrition and on the rural poor given the historically high rates of maternal and child undernutrition especially in rural areas and the generally low prevalence of overnutrition. For example, early expansion of the National Rural Health Mission created more opportunities in rural areas than in urban areas [ 34 ] and the ICDS platform has expanded more in rural than urban areas between 2006 and 2016 [ 35 ]. It is now time for India to focus on all forms of malnutrition and on tackling the unique nutrition and health problems and inequalities of urban populations, especially those living in urban slums. New strategies need to shift to a focus on all forms of malnutrition, including undernutrition and micronutrient deficiencies as well as overnutrition (overweight and obesity and diet-related non-communicable diseases). Such interventions–referred to as “double-duty actions” [ 36 , 37 ] have the potential to simultaneously tackle multiple forms of malnutrition and also prevent unintended negative consequences on overweight and obesity that has been shown to arise from programs aimed at reducing food insecurity and undernutrition in countries undergoing a rapid nutrition transition [ 38 ].
One potential limitation of this study relates to the definition of slums. Because there is no widespread consensus on the definition of slums, we used a definition that bears similarities to many others used in the empirical literature on slums, but we cannot rule out the possibility that our results may be sensitive to the choice of definition. With our definition, we found that 5% of the sample was classified as living in urban slum areas, which is consistent with the government estimates of 5.4% [ 3 ]. However, there is still uncertainty surrounding the true population-level prevalence of slum dwellers, making it difficult to validate our definition. One advantage of the NHFS is that it over-sampled slum areas of eight major cities, but it may have under-sampled slums in other urban centers of India. Another limitation is that our child-level analysis is restricted to children under five years old, whereas overweight prevalence may emerge in later childhood.
Due to the widespread burden of malnutrition and its importance for public health, research in India has increasingly focused on this topic, but studies have typically examined the effects of residential area [ 11 , 39 , 40 ] or socioeconomic status [ 30 , 41 , 42 ] separately. Our study is novel in examining the evolution of the double burden of malnutrition across distinct areas of residence and wealth quintiles within area. We use two rounds of large nationally representative data sets to explore trends and inequalities for both undernutrition and overnutrition for both children and adults. NFHS has several strengths in this regard, including standard measures of anthropometry and consistent scope for SES measures through well-established asset indices [ 22 ]. For inequality analyses, we used complex measures (SII and CIX) that account for the overall frequency and change in the outcomes over time and include weighting by sample size.
The profile of malnutrition in India is changing rapidly, with progress on several indicators of undernutrition but rapidly rising rates of overweight/obesity, especially among adults. In addition to this double burden of malnutrition, India is characterized by significant health inequalities across socioeconomic groups and areas of residence and has made very limited progress in redressing these inequalities. The persistence of undernutrition alongside rising overweight/obesity suggests that India needs to develop new nutrition strategies that prioritize double-duty actions [ 36 ]. Potential interventions to consider in the Indian context include measures to promote positive changes in diets and physical activity, interventions to stimulate the demand for nutritious foods complemented by agri-food system innovation to increase their affordability, and regulatory approaches to discourage production, marketing and consumption of unhealthy, ultra-processed foods, beverages, and snacks. Future research should consider the efficacy of double duty actions to address both undernutrition and overweight/obesity and contextualize these actions to India’s diverse socioeconomic and environmental circumstances.
Supporting information
https://doi.org/10.1371/journal.pone.0247856.s001
- 1. United Nations. Department of Economic and Social Affairs, Population Division. World Urbanization Prospects: The 2018 Revision, Online Edition. 2018.
- View Article
- PubMed/NCBI
- Google Scholar
- 3. Government of India: Slums in India. A statistical Compendium 2015. In. New Delhi, India: Government of India. Ministry of Housing and Urban Porverty Alleviation. National Building Organisation; 2015.
- 5. Ruel MT, Garrett J, Yosef S, Olivier M: Urbanization, Food Security and Nutrition. In: Nutrition and Health in a Developing World . Edited by de Pee S, Taren D, Bloem MW. Humana Press, Cham 2017.
- 16. IIPS. International Institute for Population Sciences (IIPS) and Macro International. National Family Health Survey (NFHS-3), 2005–06: India. Mumbai: IIPS. 2007.
- 17. IIPS. International Institute for Population Sciences and ICF. National Family Health Survey (NFHS-4), 2015–16: India. Mumbai: IIPS. 2017.
- 19. Census of India. Office of the Registrar General and Census Commissioner, Ministry of Home Affairs, Government of India. Census of India. http://censusindia.gov.in/2011-Common/CensusData2011.html 2011.
- 20. UN-Habitat: The challenge of slums: global report on human settlements, 2003 / United Nations Human Settlements Programme. Earthscan Publications Ltd. London and Sterling, VA; 2003.
- 23. WHO: Handbook on health inequality monitoring with a special focus on low-and middle-income countries. Geneva: World Health Organization; 2013.
- 26. WHO: Global Health Observatory data repository. Prevalence of overweight among adults, BMI ≥ 25, age-standardized. In. https://apps.who.int/gho/data/view.main.BMI25AWBv?lang=en . Accessed April 12, 2020: World Health Organization; 2020.
- 33. NITI Aayog. Nourishing Iindia. National Nutrition Strategy. Government of India. https://niti.gov.in/writereaddata/files/document_publication/Nutrition_Strategy_Booklet.pdf . 2017.
- 37. WHO. Double-duty actions for nutrition. Policy Brief. https://apps.who.int/iris/bitstream/handle/10665/255414/WHO-NMH-NHD-17.2-eng.pdf . Accessed July 19, 2020. 2020.
Thank you for visiting nature.com. You are using a browser version with limited support for CSS. To obtain the best experience, we recommend you use a more up to date browser (or turn off compatibility mode in Internet Explorer). In the meantime, to ensure continued support, we are displaying the site without styles and JavaScript.
- View all journals
- My Account Login
- Explore content
- About the journal
- Publish with us
- Sign up for alerts
- Open access
- Published: 19 March 2024
TacticAI: an AI assistant for football tactics
- Zhe Wang ORCID: orcid.org/0000-0002-0748-5376 1 na1 ,
- Petar Veličković ORCID: orcid.org/0000-0002-2820-4692 1 na1 ,
- Daniel Hennes ORCID: orcid.org/0000-0002-3646-5286 1 na1 ,
- Nenad Tomašev ORCID: orcid.org/0000-0003-1624-0220 1 ,
- Laurel Prince 1 ,
- Michael Kaisers 1 ,
- Yoram Bachrach 1 ,
- Romuald Elie 1 ,
- Li Kevin Wenliang 1 ,
- Federico Piccinini 1 ,
- William Spearman 2 ,
- Ian Graham 3 ,
- Jerome Connor 1 ,
- Yi Yang 1 ,
- Adrià Recasens 1 ,
- Mina Khan 1 ,
- Nathalie Beauguerlange 1 ,
- Pablo Sprechmann 1 ,
- Pol Moreno 1 ,
- Nicolas Heess ORCID: orcid.org/0000-0001-7876-9256 1 ,
- Michael Bowling ORCID: orcid.org/0000-0003-2960-8418 4 ,
- Demis Hassabis 1 &
- Karl Tuyls ORCID: orcid.org/0000-0001-7929-1944 5
Nature Communications volume 15 , Article number: 1906 ( 2024 ) Cite this article
20k Accesses
241 Altmetric
Metrics details
- Computational science
- Information technology
Identifying key patterns of tactics implemented by rival teams, and developing effective responses, lies at the heart of modern football. However, doing so algorithmically remains an open research challenge. To address this unmet need, we propose TacticAI, an AI football tactics assistant developed and evaluated in close collaboration with domain experts from Liverpool FC. We focus on analysing corner kicks, as they offer coaches the most direct opportunities for interventions and improvements. TacticAI incorporates both a predictive and a generative component, allowing the coaches to effectively sample and explore alternative player setups for each corner kick routine and to select those with the highest predicted likelihood of success. We validate TacticAI on a number of relevant benchmark tasks: predicting receivers and shot attempts and recommending player position adjustments. The utility of TacticAI is validated by a qualitative study conducted with football domain experts at Liverpool FC. We show that TacticAI’s model suggestions are not only indistinguishable from real tactics, but also favoured over existing tactics 90% of the time, and that TacticAI offers an effective corner kick retrieval system. TacticAI achieves these results despite the limited availability of gold-standard data, achieving data efficiency through geometric deep learning.
Similar content being viewed by others
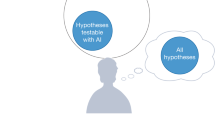
Artificial intelligence and illusions of understanding in scientific research
Lisa Messeri & M. J. Crockett
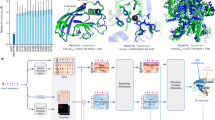
Highly accurate protein structure prediction with AlphaFold
John Jumper, Richard Evans, … Demis Hassabis
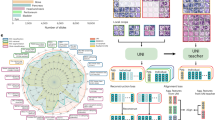
Towards a general-purpose foundation model for computational pathology
Richard J. Chen, Tong Ding, … Faisal Mahmood
Introduction
Association football, or simply football or soccer, is a widely popular and highly professionalised sport, in which two teams compete to score goals against each other. As each football team comprises up to 11 active players at all times and takes place on a very large pitch (also known as a soccer field), scoring goals tends to require a significant degree of strategic team-play. Under the rules codified in the Laws of the Game 1 , this competition has nurtured an evolution of nuanced strategies and tactics, culminating in modern professional football leagues. In today’s play, data-driven insights are a key driver in determining the optimal player setups for each game and developing counter-tactics to maximise the chances of success 2 .
When competing at the highest level the margins are incredibly tight, and it is increasingly important to be able to capitalise on any opportunity for creating an advantage on the pitch. To that end, top-tier clubs employ diverse teams of coaches, analysts and experts, tasked with studying and devising (counter-)tactics before each game. Several recent methods attempt to improve tactical coaching and player decision-making through artificial intelligence (AI) tools, using a wide variety of data types from videos to tracking sensors and applying diverse algorithms ranging from simple logistic regression to elaborate neural network architectures. Such methods have been employed to help predict shot events from videos 3 , forecast off-screen movement from spatio-temporal data 4 , determine whether a match is in-play or interrupted 5 , or identify player actions 6 .
The execution of agreed-upon plans by players on the pitch is highly dynamic and imperfect, depending on numerous factors including player fitness and fatigue, variations in player movement and positioning, weather, the state of the pitch, and the reaction of the opposing team. In contrast, set pieces provide an opportunity to exert more control on the outcome, as the brief interruption in play allows the players to reposition according to one of the practiced and pre-agreed patterns, and make a deliberate attempt towards the goal. Examples of such set pieces include free kicks, corner kicks, goal kicks, throw-ins, and penalties 2 .
Among set pieces, corner kicks are of particular importance, as an improvement in corner kick execution may substantially modify game outcomes, and they lend themselves to principled, tactical and detailed analysis. This is because corner kicks tend to occur frequently in football matches (with ~10 corners on average taking place in each match 7 ), they are taken from a fixed, rigid position, and they offer an immediate opportunity for scoring a goal—no other set piece simultaneously satisfies all of the above. In practice, corner kick routines are determined well ahead of each match, taking into account the strengths and weaknesses of the opposing team and their typical tactical deployment. It is for this reason that we focus on corner kick analysis in particular, and propose TacticAI, an AI football assistant for supporting the human expert with set piece analysis, and the development and improvement of corner kick routines.
TacticAI is rooted in learning efficient representations of corner kick tactics from raw, spatio-temporal player tracking data. It makes efficient use of this data by representing each corner kick situation as a graph—a natural representation for modelling relationships between players (Fig. 1 A, Table 2 ), and these player relationships may be of higher importance than the absolute distances between them on the pitch 8 . Such a graph input is a natural candidate for graph machine learning models 9 , which we employ within TacticAI to obtain high-dimensional latent player representations. In the Supplementary Discussion section, we carefully contrast TacticAI against prior art in the area.
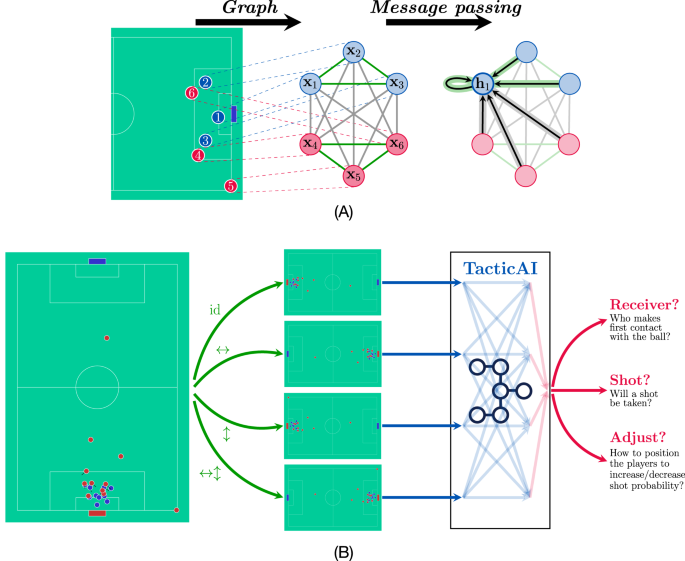
A How corner kick situations are converted to a graph representation. Each player is treated as a node in a graph, with node, edge and graph features extracted as detailed in the main text. Then, a graph neural network operates over this graph by performing message passing; each node’s representation is updated using the messages sent to it from its neighbouring nodes. B How TacticAI processes a given corner kick. To ensure that TacticAI’s answers are robust in the face of horizontal or vertical reflections, all possible combinations of reflections are applied to the input corner, and these four views are then fed to the core TacticAI model, where they are able to interact with each other to compute the final player representations—each internal blue arrow corresponds to a single message passing layer from ( A ). Once player representations are computed, they can be used to predict the corner’s receiver, whether a shot has been taken, as well as assistive adjustments to player positions and velocities, which increase or decrease the probability of a shot being taken.
Uniquely, TacticAI takes advantage of geometric deep learning 10 to explicitly produce player representations that respect several symmetries of the football pitch (Fig. 1 B). As an illustrative example, we can usually safely assume that under a horizontal or vertical reflection of the pitch state, the game situation is equivalent. Geometric deep learning ensures that TacticAI’s player representations will be identically computed under such reflections, such that this symmetry does not have to be learnt from data. This proves to be a valuable addition, as high-quality tracking data is often limited—with only a few hundred matches played each year in every league. We provide an in-depth overview of how we employ geometric deep learning in TacticAI in the “Methods” section.
From these representations, TacticAI is then able to answer various predictive questions about the outcomes of a corner—for example, which player is most likely to make first contact with the ball, or whether a shot will take place. TacticAI can also be used as a retrieval system—for mining similar corner kick situations based on the similarity of player representations—and a generative recommendation system, suggesting adjustments to player positions and velocities to maximise or minimise the estimated shot probability. Through several experiments within a case study with domain expert coaches and analysts from Liverpool FC, the results of which we present in the next section, we obtain clear statistical evidence that TacticAI readily provides useful, realistic and accurate tactical suggestions.
To demonstrate the diverse qualities of our approach, we design TacticAI with three distinct predictive and generative components: receiver prediction, shot prediction, and tactic recommendation through guided generation, which also correspond to the benchmark tasks for quantitatively evaluating TacticAI. In addition to providing accurate quantitative insights for corner kick analysis with its predictive components, the interplay between TacticAI’s predictive and generative components allows coaches to sample alternative player setups for each routine of interest, and directly evaluate the possible outcomes of such alternatives.
We will first describe our quantitative analysis, which demonstrates that TacticAI’s predictive components are accurate at predicting corner kick receivers and shot situations on held-out test corners and that the proposed player adjustments do not strongly deviate from ground-truth situations. However, such an analysis only gives an indirect insight into how useful TacticAI would be once deployed. We tackle this question of utility head-on and conduct a comprehensive case study in collaboration with our partners at Liverpool FC—where we directly ask human expert raters to judge the utility of TacticAI’s predictions and player adjustments. The following sections expand on the specific results and analysis we have performed.
In what follows, we will describe TacticAI’s components at a minimal level necessary to understand our evaluation. We defer detailed descriptions of TacticAI’s components to the “Methods” section. Note that, all our error bars reported in this research are standard deviations.
Benchmarking TacticAI
We evaluate the three components of TacticAI on a relevant benchmark dataset of corner kicks. Our dataset consists of 7176 corner kicks from the 2020 to 2021 Premier League seasons, which we randomly shuffle and split into a training (80%) and a test set (20%). As previously mentioned, TacticAI operates on graphs. Accordingly, we represent each corner kick situation as a graph, where each node corresponds to a player. The features associated with each node encode the movements (velocities and positions) and simple profiles (heights and weights) of on-pitch players at the timestamp when the corresponding corner kick was being taken by the attacking kicker (see the “Methods” section), and no information of ball movement was encoded. The graphs are fully connected; that is, for every pair of players, we will include the edge connecting them in the graph. Each of these edges encodes a binary feature, indicating whether the two players are on opposing teams or not. For each task, we generated the relevant dataset of node/edge/graph features and corresponding labels (Tables 1 and 2 , see the “Methods” section). The components were then trained separately with their corresponding corner kick graphs. In particular, we only employ a minimal set of features to construct the corner kick graphs, without encoding the movements of the ball nor explicitly encoding the distances between players into the graphs. We used a consistent training-test split for all benchmark tasks, as this made it possible to benchmark not only the individual components but also their interactions.
Accurate receiver and shot prediction through geometric deep learning
One of TacticAI’s key predictive models forecasts the receiver out of the 22 on-pitch players. The receiver is defined as the first player touching the ball after the corner is taken. In our evaluation, all methods used the same set of features (see the “Receiver prediction” entry in Table 1 and the “Methods” section). We leveraged the receiver prediction task to benchmark several different TacticAI base models. Our best-performing model—achieving 0.782 ± 0.039 in top-3 test accuracy after 50,000 training steps—was a deep graph attention network 11 , 12 , leveraging geometric deep learning 10 through the use of D 2 group convolutions 13 . We supplement this result with a detailed ablation study, verifying that both our choice of base architecture and group convolution yielded significant improvements in the receiver prediction task (Supplementary Table 2 , see the subsection “Ablation study” in the “Methods” section). Considering that corner kick receiver prediction is a highly challenging task with many factors that are unseen by our model—including fatigue and fitness levels, and actual ball trajectory—we consider TacticAI’s top-3 accuracy to reflect a high level of predictive power, and keep the base TacticAI architecture fixed for subsequent studies. In addition to this quantitative evaluation with the evaluation dataset, we also evaluate the performance of TacticAI’s receiver prediction component in a case study with human raters. Please see the “Case study with expert raters” section for more details.
For shot prediction, we observe that reusing the base TacticAI architecture to directly predict shot events—i.e., directly modelling the probability \({\mathbb{P}}(\,{{\mbox{shot}}}| {{\mbox{corner}}}\,)\) —proved challenging, only yielding a test F 1 score of 0.52 ± 0.03, for a GATv2 base model. Note that here we use the F 1 score—the harmonic mean of precision and recall—as it is commonly used in binary classification problems over imbalanced datasets, such as shot prediction. However, given that we already have a potent receiver predictor, we decided to use its output to give us additional insight into whether or not a shot had been taken. Hence, we opted to decompose the probability of taking a shot as
where \({\mathbb{P}}(\,{{\mbox{receiver}}}| {{\mbox{corner}}}\,)\) are the probabilities computed by TacticAI’s receiver prediction system, and \({\mathbb{P}}(\,{{\mbox{shot}}}| {{\mbox{receiver}}},{{\mbox{corner}}}\,)\) models the conditional shot probability after a specific player makes first contact with the ball. This was implemented through providing an additional global feature to indicate the receiver in the corresponding corner kick (Table 1 ) while the architecture otherwise remained the same as that of receiver prediction (Supplementary Fig. 2 , see the “Methods” section). At training time, we feed the ground-truth receiver as input to the model—at inference time, we attempt every possible receiver, weighing their contributions using the probabilities given by TacticAI’s receiver predictor, as per Eq. ( 1 ). This two-phased approach yielded a final test F 1 score of 0.68 ± 0.04 for shot prediction, which encodes significantly more signal than the unconditional shot predictor, especially considering the many unobservables associated with predicting shot events. Just as for receiver prediction, this performance can be further improved using geometric deep learning; a conditional GATv2 shot predictor with D 2 group convolutions achieves an F 1 score of 0.71 ± 0.01.
Moreover, we also observe that, even just through predicting the receivers, without explicitly classifying any other salient features of corners, TacticAI learned generalisable representations of the data. Specifically, team setups with similar tactical patterns tend to cluster together in TacticAI’s latent space (Fig. 2 ). However, no clear clusters are observed in the raw input space (Supplementary Fig. 1 ). This indicates that TacticAI can be leveraged as a useful corner kick retrieval system, and we will present our evaluation of this hypothesis in the “Case study with expert raters” section.
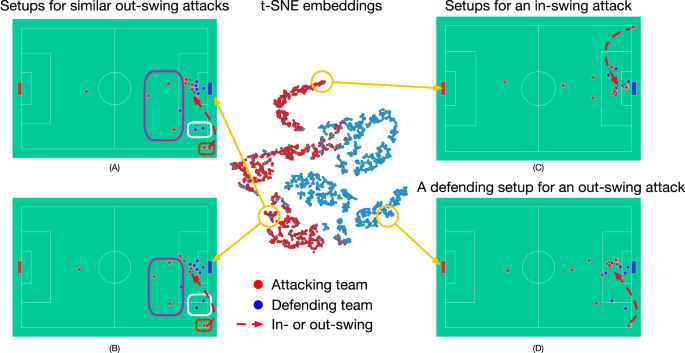
We visualise the latent representations of attacking and defending teams in 1024 corner kicks using t -SNE. A latent team embedding in one corner kick sample is the mean of the latent player representations on the same attacking ( A – C ) or defending ( D ) team. Given the reference corner kick sample ( A ), we retrieve another corner kick sample ( B ) with respect to the closest distance of their representations in the latent space. We observe that ( A ) and ( B ) are both out-swing corner kicks and share similar patterns of their attacking tactics, which are highlighted with rectangles having the same colours, although they bear differences with respect to the absolute positions and velocities of the players. All the while, the latent representation of an in-swing attack ( C ) is distant from both ( A ) and ( B ) in the latent space. The red arrows are only used to demonstrate the difference between in- and out-swing corner kicks, not the actual ball trajectories.
Lastly, it is worth emphasising that the utility of the shot predictor likely does not come from forecasting whether a shot event will occur—a challenging problem with many imponderables—but from analysing the difference in predicted shot probability across multiple corners. Indeed, in the following section, we will show how TacticAI’s generative tactic refinements can directly influence the predicted shot probabilities, which will then corresponds to highly favourable evaluation by our expert raters in the “Case study with expert raters” section.
Controlled tactic refinement using class-conditional generative models
Equipped with components that are able to potently relate corner kicks with their various outcomes (e.g. receivers and shot events), we can explore the use of TacticAI to suggest adjustments of tactics, in order to amplify or reduce the likelihood of certain outcomes.
Specifically, we aim to produce adjustments to the movements of players on one of the two teams, including their positions and velocities, which would maximise or minimise the probability of a shot event, conditioned on the initial corner setup, consisting of the movements of players on both teams and their heights and weights. In particular, although in real-world scenarios both teams may react simultaneously to the movements of each other, in our study, we focus on moderate adjustments to player movements, which help to detect players that are not responding to a tactic properly. Due to this reason, we simplify the process of tactic refinement through generating the adjustments for only one team while keeping the other fixed. The way we train a model for this task is through an auto-encoding objective: we feed the ground-truth shot outcome (a binary indicator) as an additional graph-level feature to TacticAI’s model (Table 1 ), and then have it learn to reconstruct a probability distribution of the input player coordinates (Fig. 1 B, also see the “Methods” section). As a consequence, our tactic adjustment system does not depend on the previously discussed shot predictor—although we can use the shot predictor to evaluate whether the adjustments make a measurable difference in shot probability.
This autoencoder-based generative model is an individual component that separates from TacticAI’s predictive systems. All three systems share the encoder architecture (without sharing parameters), but use different decoders (see the “Methods” section). At inference time, we can instead feed in a desired shot outcome for the given corner setup, and then sample new positions and velocities for players on one team using this probability distribution. This setup, in principle, allows for flexible downstream use, as human coaches can optimise corner kick setups through generating adjustments conditioned on the specific outcomes of their interest—e.g., increasing shot probability for the attacking team, decreasing it for the defending team (Fig. 3 ) or amplifying the chance that a particular striker receives the ball.
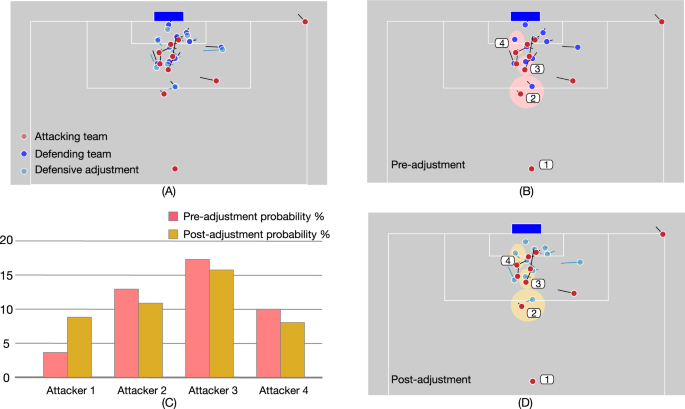
TacticAI makes it possible for human coaches to redesign corner kick tactics in ways that help maximise the probability of a positive outcome for either the attacking or the defending team by identifying key players, as well as by providing temporally coordinated tactic recommendations that take all players into consideration. As demonstrated in the present example ( A ), for a corner kick in which there was a shot attempt in reality ( B ), TacticAI can generate a tactically-adjusted setting in which the shot probability has been reduced, by adjusting the positioning of the defenders ( D ). The suggested defender positions result in reduced receiver probability for attacking players 2–5 (see bottom row), while the receiver probability of Attacker 1, who is distant from the goalpost, has been increased ( C ). The model is capable of generating multiple such scenarios. Coaches can inspect the different options visually and additionally consult TacticAI’s quantitative analysis of the presented tactics.
We first evaluate the generated adjustments quantitatively, by verifying that they are indistinguishable from the original corner kick distribution using a classifier. To do this, we synthesised a dataset consisting of 200 corner kick samples and their corresponding conditionally generated adjustments. Specifically, for corners without a shot event, we generated adjustments for the attacking team by setting the shot event feature to 1, and vice-versa for the defending team when a shot event did happen. We found that the real and generated samples were not distinguishable by an MLP classifier, with an F 1 score of 0.53 ± 0.05, indicating random chance level accuracy. This result indicates that the adjustments produced by TacticAI are likely similar enough to real corner kicks that the MLP is unable to tell them apart. Note that, in spite of this similarity, TacticAI recommends player-level adjustments that are not negligible—in the following section we will illustrate several salient examples of this. To more realistically validate the practical indistinguishability of TacticAI’s adjustments from realistic corners, we also evaluated the realism of the adjustments in a case study with human experts, which we will present in the following section.
In addition, we leveraged our TacticAI shot predictor to estimate whether the proposed adjustments were effective. We did this by analysing 100 corner kick samples in which threatening shots occurred, and then, for each sample, generated one defensive refinement through setting the shot event feature to 0. We observed that the average shot probability significantly decreased, from 0.75 ± 0.14 for ground-truth corners to 0.69 ± 0.16 for adjustments ( z = 2.62, p < 0.001). This observation was consistent when testing for attacking team refinements (shot probability increased from 0.18 ± 0.16 to 0.31 ± 0.26 ( z = −4.46, p < 0.001)). Moving beyond this result, we also asked human raters to assess the utility of TacticAI’s proposed adjustments within our case study, which we detail next.
Case study with expert raters
Although quantitative evaluation with well-defined benchmark datasets was critical for the technical development of TacticAI, the ultimate test of TacticAI as a football tactic assistant is its practical downstream utility being recognised by professionals in the industry. To this end, we evaluated TacticAI through a case study with our partners at Liverpool FC (LFC). Specifically, we invited a group of five football experts: three data scientists, one video analyst, and one coaching assistant. Each of them completed four tasks in the case study, which evaluated the utility of TacticAI’s components from several perspectives; these include (1) the realism of TacticAI’s generated adjustments, (2) the plausibility of TacticAI’s receiver predictions, (3) effectiveness of TacticAI’s embeddings for retrieving similar corners, and (4) usefulness of TacticAI’s recommended adjustments. We provide an overview of our study’s results here and refer the interested reader to Supplementary Figs. 3 – 5 and the Supplementary Methods for additional details.
We first simultaneously evaluated the realism of the adjusted corner kicks generated by TacticAI, and the plausibility of its receiver predictions. Going through a collection of 50 corner kick samples, we first asked the raters to classify whether a given sample was real or generated by TacticAI, and then they were asked to identify the most likely receivers in the corner kick sample (Supplementary Fig. 3 ).
On the task of classifying real and generated samples, first, we found that the raters’ average F 1 score of classifying the real vs. generated samples was only 0.60 ± 0.04, with individual F 1 scores ( \({F}_{1}^{A}=0.54,{F}_{1}^{B}=0.64,{F}_{1}^{C}=0.65,{F}_{1}^{D}=0.62,{F}_{1}^{E}=0.56\) ), indicating that the raters were, in many situations, unable to distinguish TacticAI’s adjustments from real corners.
The previous evaluation focused on analysing realism detection performance across raters. We also conduct a study that analyses realism detection across samples. Specifically, we assigned ratings for each sample—assigning +1 to a sample if it was identified as real by a human rater, and 0 otherwise—and computed the average rating for each sample across the five raters. Importantly, by studying the distribution of ratings, we found that there was no significant difference between the average ratings assigned to real and generated corners ( z = −0.34, p > 0.05) (Fig. 4 A). Hence, the real and generated samples were assigned statistically indistinguishable average ratings by human raters.
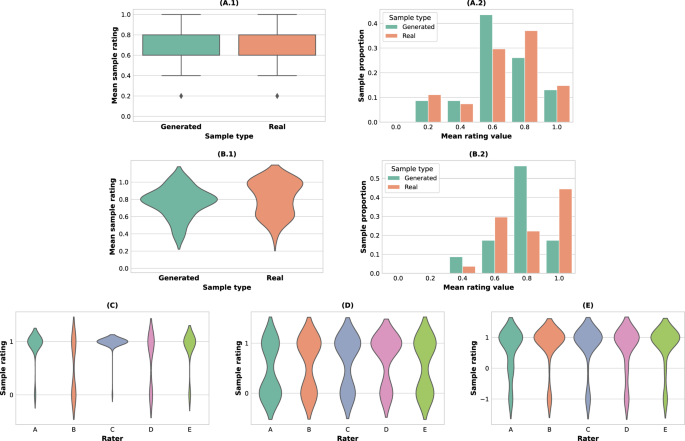
In task 1, we tested the statistical difference between the real corner kick samples and the synthetic ones generated by TacticAI from two aspects: ( A.1 ) the distributions of their assigned ratings, and ( A.2 ) the corresponding histograms of the rating values. Analogously, in task 2 (receiver prediction), ( B.1 ) we track the distributions of the top-3 accuracy of receiver prediction using those samples, and ( B.2 ) the corresponding histogram of the mean rating per sample. No statistical difference in the mean was observed in either cases (( A.1 ) ( z = −0.34, p > 0.05), and ( B.1 ) ( z = 0.97, p > 0.05)). Additionally, we observed a statistically significant difference between the ratings of different raters on receiver prediction, with three clear clusters emerging ( C ). Specifically, Raters A and E had similar ratings ( z = 0.66, p > 0.05), and Raters B and D also rated in similar ways ( z = −1.84, p > 0.05), while Rater C responded differently from all other raters. This suggests a good level of variety of the human raters with respect to their perceptions of corner kicks. In task 3—identifying similar corners retrieved in terms of salient strategic setups—there were no significant differences among the distributions of the ratings by different raters ( D ), suggesting a high level of agreement on the usefulness of TacticAI’s capability of retrieving similar corners ( F 1,4 = 1.01, p > 0.1). Finally, in task 4, we compared the ratings of TacticAI’s strategic refinements across the human raters ( E ) and found that the raters also agreed on the general effectiveness of the refinements recommended by TacticAI ( F 1,4 = 0.45, p > 0.05). Note that the violin plots used in B.1 and C – E model a continuous probability distribution and hence assign nonzero probabilities to values outside of the allowed ranges. We only label y -axis ticks for the possible set of ratings.
For the task of identifying receivers, we rated TacticAI’s predictions with respect to a rater as +1 if at least one of the receivers identified by the rater appeared in TacticAI’s top-3 predictions, and 0 otherwise. The average top-3 accuracy among the human raters was 0.79 ± 0.18; specifically, 0.81 ± 0.17 for the real samples, and 0.77 ± 0.21 for the generated ones. These scores closely line up with the accuracy of TacticAI in predicting receivers for held-out test corners, validating our quantitative study. Further, after averaging the ratings for receiver prediction sample-wise, we found no statistically significant difference between the average ratings of predicting receivers over the real and generated samples ( z = 0.97, p > 0.05) (Fig. 4 B). This indicates that TacticAI was equally performant in predicting the receivers of real corners and TacticAI-generated adjustments, and hence may be leveraged for this purpose even in simulated scenarios.
There is a notably high variance in the average receiver prediction rating of TacticAI. We hypothesise that this is due to the fact that different raters may choose to focus on different salient features when evaluating the likely receivers (or even the amount of likely receivers). We set out to validate this hypothesis by testing the pair-wise similarity of the predictions by the human raters through running a one-away analysis of variance (ANOVA), followed by a Tukey test. We found that the distributions of the five raters’ predictions were significantly different ( F 1,4 = 14.46, p < 0.001) forming three clusters (Fig. 4 C). This result indicates that different human raters—as suggested by their various titles at LFC—may often use very different leads when suggesting plausible receivers. The fact that TacticAI manages to retain a high top-3 accuracy in such a setting suggests that it was able to capture the salient patterns of corner kick strategies, which broadly align with human raters’ preferences. We will further test this hypothesis in the third task—identifying similar corners.
For the third task, we asked the human raters to judge 50 pairs of corners for their similarity. Each pair consisted of a reference corner and a retrieved corner, where the retrieved corner was chosen either as the nearest-neighbour of the reference in terms of their TacticAI latent space representations, or—as a feature-level heuristic—the cosine similarities of their raw features (Supplementary Fig. 4 ) in our corner kick dataset. We score the raters’ judgement of a pair as +1 if they considered the corners presented in the case to be usefully similar, otherwise, the pair is scored with 0. We first computed, for each rater, the recall with which they have judged a baseline- or TacticAI-retrieved pair as usefully similar—see description of Task 3 in the Supplementary Methods . For TacticAI retrievals, the average recall across all raters was 0.59 ± 0.09, and for the baseline system, the recall was 0.36 ± 0.10. Secondly, we assess the statistical difference between the results of the two methods by averaging the ratings for each reference–retrieval pair, finding that the average rating of TacticAI retrievals is significantly higher than the average rating of baseline method retrievals ( z = 2.34, p < 0.05). These two results suggest that TacticAI significantly outperforms the feature-space baseline as a method for mining similar corners. This indicates that TacticAI is able to extract salient features from corners that are not trivial to extract from the input data alone, reinforcing it as a potent tool for discovering opposing team tactics from available data. Finally, we observed that this task exhibited a high level of inter-rater agreement for TacticAI-retrieved pairs ( F 1,4 = 1.01, p > 0.1) (Fig. 4 D), suggesting that human raters were largely in agreement with respect to their assessment of TacticAI’s performance.
Finally, we evaluated TacticAI’s player adjustment recommendations for their practical utility. Specifically, each rater was given 50 tactical refinements together with the corresponding real corner kick setups—see Supplementary Fig. 5 , and the “Case study design” section in the Supplementary Methods . The raters were then asked to rate each refinement as saliently improving the tactics (+1), saliently making them worse (−1), or offering no salient differences (0). We calculated the average rating assigned by each of the raters (giving us a value in the range [− 1, 1] for each rater). The average of these values across all five raters was 0.7 ± 0.1. Further, for 45 of the 50 situations (90%), the human raters found TacticAI’s suggestion to be favourable on average (by majority voting). Both of these results indicate that TacticAI’s recommendations are salient and useful to a downstream football club practitioner, and we set out to validate this with statistical tests.
We performed statistical significance testing of the observed positive ratings. First, for each of the 50 situations, we averaged its ratings across all five raters and then ran a t -test to assess whether the mean rating was significantly larger than zero. Indeed, the statistical test indicated that the tactical adjustments recommended by TacticAI were constructive overall ( \({t}_{49}^{{{{{{{{\rm{avg}}}}}}}}}=9.20,\, p \, < \, 0.001\) ). Secondly, we verified that each of the five raters individually found TacticAI’s recommendations to be constructive, running a t -test on each of their ratings individually. For all of the five raters, their average ratings were found to be above zero with statistical significance ( \({t}_{49}^{A}=5.84,\, {p}^{A} \, < \, 0.001;{t}_{49}^{B}=7.88,\; {p}^{B} \, < \, 0.001;{t}_{49}^{C}=7.00,\; {p}^{C} \, < \, 0.001;{t}_{49}^{D}=6.04,\; {p}^{D} \, < \, 0.001;{t}_{49}^{E}=7.30,\, {p}^{E} \, < \, 0.001\) ). In addition, their ratings also shared a high level of inter-agreement ( F 1,4 = 0.45, p > 0.05) (Fig. 4 E), suggesting a level of practical usefulness that is generally recognised by human experts, even though they represent different backgrounds.
Taking all of these results together, we find TacticAI to possess strong components for prediction, retrieval, and tactical adjustments on corner kicks. To illustrate the kinds of salient recommendations by TacticAI, in Fig. 5 we present four examples with a high degree of inter-rater agreement.
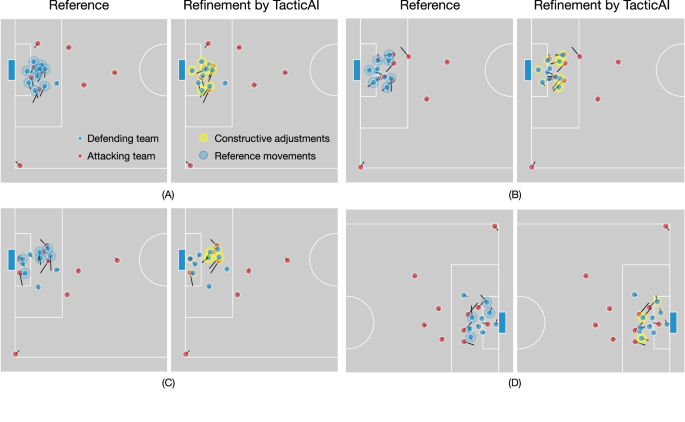
These examples are selected from our case study with human experts, to illustrate the breadth of tactical adjustments that TacticAI suggests to teams defending a corner. The density of the yellow circles coincides with the number of times that the corresponding change is recognised as constructive by human experts. Instead of optimising the movement of one specific player, TacticAI can recommend improvements for multiple players in one generation step through suggesting better positions to block the opposing players, or better orientations to track them more efficiently. Some specific comments from expert raters follow. In A , according to raters, TacticAI suggests more favourable positions for several defenders, and improved tracking runs for several others—further, the goalkeeper is positioned more deeply, which is also beneficial. In B , TacticAI suggests that the defenders furthest away from the corner make improved covering runs, which was unanimously deemed useful, with several other defenders also positioned more favourably. In C , TacticAI recommends improved covering runs for a central group of defenders in the penalty box, which was unanimously considered salient by our raters. And in D , TacticAI suggests substantially better tracking runs for two central defenders, along with a better positioning for two other defenders in the goal area.
We have demonstrated an AI assistant for football tactics and provided statistical evidence of its efficacy through a comprehensive case study with expert human raters from Liverpool FC. First, TacticAI is able to accurately predict the first receiver after a corner kick is taken as well as the probability of a shot as the direct result of the corner. Second, TacticAI has been shown to produce plausible tactical variations that improve outcomes in a salient way, while being indistinguishable from real scenarios by domain experts. And finally, the system’s latent player representations are a powerful means to retrieve similar set-piece tactics, allowing coaches to analyse relevant tactics and counter-tactics that have been successful in the past.
The broader scope of strategy modelling in football has previously been addressed from various individual angles, such as pass prediction 14 , 15 , 16 , shot prediction 3 or corner kick tactical classification 7 . However, to the best of our knowledge, our work stands out by combining and evaluating predictive and generative modelling of corner kicks for tactic development. It also stands out in its method of applying geometric deep learning, allowing for efficiently incorporating various symmetries of the football pitch for improved data efficiency. Our method incorporates minimal domain knowledge and does not rely on intricate feature engineering—though its factorised design naturally allows for more intricate feature engineering approaches when such features are available.
Our methodology requires the position and velocity estimates of all players at the time of execution of the corner and subsequent events. Here, we derive these from high-quality tracking and event data, with data availability from tracking providers limited to top leagues. Player tracking based on broadcast video would increase the reach and training data substantially, but would also likely result in noisier model inputs. While the attention mechanism of GATs would allow us to perform introspection of the most salient factors contributing to the model outcome, our method does not explicitly model exogenous (aleatoric) uncertainty, which would be valuable context for the football analyst.
While the empirical study of our method’s efficacy has been focused on corner kicks in association football, it readily generalises to other set pieces (such as throw-ins, which similarly benefit from similarity retrieval, pass and/or shot prediction) and other team sports with suspended play situations. The learned representations and overall framing of TacticAI also lay the ground for future research to integrate a natural language interface that enables domain-grounded conversations with the assistant, with the aim to retrieve particular situations of interest, make predictions for a given tactical variant, compare and contrast, and guide through an interactive process to derive tactical suggestions. It is thus our belief that TacticAI lays the groundwork for the next-generation AI assistant for football.
We devised TacticAI as a geometric deep learning pipeline, further expanded in this section. We process labelled spatio-temporal football data into graph representations, and train and evaluate on benchmarking tasks cast as classification or regression. These steps are presented in sequence, followed by details on the employed computational architecture.
Raw corner kick data
The raw dataset consisted of 9693 corner kicks collected from the 2020–21, 2021–22, and 2022–23 (up to January 2023) Premier League seasons. The dataset was provided by Liverpool FC and comprises four separate data sources, described below.
Our primary data source is spatio-temporal trajectory frames (tracking data), which tracked all on-pitch players and the ball, for each match, at 25 frames per second. In addition to player positions, their velocities are derived from position data through filtering. For each corner kick, we only used the frame in which the kick is being taken as input information.
Secondly, we also leverage event stream data, which annotated the events or actions (e.g., passes, shots and goals) that have occurred in the corresponding tracking frames.
Thirdly, the line-up data for the corresponding games, which recorded the players’ profiles, including their heights, weights and roles, is also used.
Lastly, we have access to miscellaneous game data, which contains the game days, stadium information, and pitch length and width in meters.
Graph representation and construction
We assumed that we were provided with an input graph \({{{{{{{\mathcal{G}}}}}}}}=({{{{{{{\mathcal{V}}}}}}}},\,{{{{{{{\mathcal{E}}}}}}}})\) with a set of nodes \({{{{{{{\mathcal{V}}}}}}}}\) and edges \({{{{{{{\mathcal{E}}}}}}}}\subseteq {{{{{{{\mathcal{V}}}}}}}}\times {{{{{{{\mathcal{V}}}}}}}}\) . Within the context of football games, we took \({{{{{{{\mathcal{V}}}}}}}}\) to be the set of 22 players currently on the pitch for both teams, and we set \({{{{{{{\mathcal{E}}}}}}}}={{{{{{{\mathcal{V}}}}}}}}\times {{{{{{{\mathcal{V}}}}}}}}\) ; that is, we assumed all pairs of players have the potential to interact. Further analyses, leveraging more specific choices of \({{{{{{{\mathcal{E}}}}}}}}\) , would be an interesting avenue for future work.
Additionally, we assume that the graph is appropriately featurised. Specifically, we provide a node feature matrix, \({{{{{{{\bf{X}}}}}}}}\in {{\mathbb{R}}}^{| {{{{{{{\mathcal{V}}}}}}}}| \times k}\) , an edge feature tensor, \({{{{{{{\bf{E}}}}}}}}\in {{\mathbb{R}}}^{| {{{{{{{\mathcal{V}}}}}}}}| \times | {{{{{{{\mathcal{V}}}}}}}}| \times l}\) , and a graph feature vector, \({{{{{{{\bf{g}}}}}}}}\in {{\mathbb{R}}}^{m}\) . The appropriate entries of these objects provide us with the input features for each node, edge, and graph. For example, \({{{{{{{{\bf{x}}}}}}}}}_{u}\in {{\mathbb{R}}}^{k}\) would provide attributes of an individual player \(u\in {{{{{{{\mathcal{V}}}}}}}}\) , such as position, height and weight, and \({{{{{{{{\bf{e}}}}}}}}}_{uv}\in {{\mathbb{R}}}^{l}\) would provide the attributes of a particular pair of players \((u,\, v)\in {{{{{{{\mathcal{E}}}}}}}}\) , such as their distance, and whether they belong to the same team. The graph feature vector, g , can be used to store global attributes of interest to the corner kick, such as the game time, current score, or ball position. For a simplified visualisation of how a graph neural network would process such an input, refer to Fig. 1 A.
To construct the input graphs, we first aligned the four data sources with respect to their game IDs and timestamps and filtered out 2517 invalid corner kicks, for which the alignment failed due to missing data, e.g., missing tracking frames or event labels. This filtering yielded 7176 valid corner kicks for training and evaluation. We summarised the exact information that was used to construct the input graphs in Table 2 . In particular, other than player heights (measured in centimeters (cm)) and weights (measured in kilograms (kg)), the players were anonymous in the model. For the cases in which the player profiles were missing, we set their heights and weights to 180 cm and 75 kg, respectively, as defaults. In total, we had 385 such occurrences out of a total of 213,246( = 22 × 9693) during data preprocessing. We downscaled the heights and weights by a factor of 100. Moreover, for each corner kick, we zero-centred the positions of on-pitch players and normalised them onto a 10 m × 10 m pitch, and their velocities were re-scaled accordingly. For the cases in which the pitch dimensions were missing, we used a standard pitch dimension of 110 m × 63 m as default.
We summarised the grouping of the features in Table 1 . The actual features used in different benchmark tasks may differ, and we will describe this in more detail in the next section. To focus on modelling the high-level tactics played by the attacking and defending teams, other than a binary indicator for ball possession—which is 1 for the corner kick taker and 0 for all other players—no information of ball movement, neither positions nor velocities, was used to construct the input graphs. Additionally, we do not have access to the player’s vertical movement, therefore only information on the two-dimensional movements of each player is provided in the data. We do however acknowledge that such information, when available, would be interesting to consider in a corner kick outcome predictor, considering the prevalence of aerial battles in corners.
Benchmark tasks construction
TacticAI consists of three predictive and generative models, which also correspond to three benchmark tasks implemented in this study. Specifically, (1) Receiver prediction, (2) Threatening shot prediction, and (3) Guided generation of team positions and velocities (Table 1 ). The graphs of all the benchmark tasks used the same feature space of nodes and edges, differing only in the global features.
For all three tasks, our models first transform the node features to a latent node feature matrix, \({{{{{{{\bf{H}}}}}}}}={f}_{{{{{{{{\mathcal{G}}}}}}}}}({{{{{{{\bf{X}}}}}}}},\, {{{{{{{\bf{E}}}}}}}},\, {{{{{{{\bf{g}}}}}}}})\) , from which we could answer queries: either about individual players—in which case we learned a relevant classifier or regressor over the h u vectors (the rows of H )—or about the occurrence of a global event (e.g. shot taken)—in which case we classified or regressed over the aggregated player vectors, ∑ u h u . In both cases, the classifiers were trained using stochastic gradient descent over an appropriately chosen loss function, such as categorical cross-entropy for classifiers, and mean squared error for regressors.
For different tasks, we extracted the corresponding ground-truth labels from either the event stream data or the tracking data. Specifically, (1) We modelled receiver prediction as a node classification task and labelled the first player to touch the ball after the corner was taken as the target node. This player could be either an attacking or defensive player. (2) Shot prediction was modelled as graph classification. In particular, we considered a next-ball-touch action by the attacking team as a shot if it was a direct corner, a goal, an aerial, hit on the goalposts, a shot attempt saved by the goalkeeper, or missing target. This yielded 1736 corners labelled as a shot being taken, and 5440 corners labelled as a shot not being taken. (3) For guided generation of player position and velocities, no additional label was needed, as this model relied on a self-supervised reconstruction objective.
The entire dataset was split into training and evaluation sets with an 80:20 ratio through random sampling, and the same splits were used for all tasks.
Graph neural networks
The central model of TacticAI is the graph neural network (GNN) 9 , which computes latent representations on a graph by repeatedly combining them within each node’s neighbourhood. Here we define a node’s neighbourhood, \({{{{{{{{\mathcal{N}}}}}}}}}_{u}\) , as the set of all first-order neighbours of node u , that is, \({{{{{{{{\mathcal{N}}}}}}}}}_{u}=\{v\,| \,(v,\, u)\in {{{{{{{\mathcal{E}}}}}}}}\}\) . A single GNN layer then transforms the node features by passing messages between neighbouring nodes 17 , following the notation of related work 10 , and the implementation of the CLRS-30 benchmark baselines 18 :
where \(\psi :{{\mathbb{R}}}^{k}\times {{\mathbb{R}}}^{k}\times {{\mathbb{R}}}^{l}\times {{\mathbb{R}}}^{m}\to {{\mathbb{R}}}^{{k}^{{\prime} }}\) and \(\phi :{{\mathbb{R}}}^{k}\times {{\mathbb{R}}}^{{k}^{{\prime} }}\to {{\mathbb{R}}}^{{k}^{{\prime} }}\) are two learnable functions (e.g. multilayer perceptrons), \({{{{{{{{\bf{h}}}}}}}}}_{u}^{(t)}\) are the features of node u after t GNN layers, and ⨁ is any permutation-invariant aggregator, such as sum, max, or average. By definition, we set \({{{{{{{{\bf{h}}}}}}}}}_{u}^{(0)}={{{{{{{{\bf{x}}}}}}}}}_{u}\) , and iterate Eq. ( 2 ) for T steps, where T is a hyperparameter. Then, we let \({{{{{{{\bf{H}}}}}}}}={f}_{{{{{{{{\mathcal{G}}}}}}}}}({{{{{{{\bf{X}}}}}}}},\, {{{{{{{\bf{E}}}}}}}},\, {{{{{{{\bf{g}}}}}}}})={{{{{{{{\bf{H}}}}}}}}}^{(T)}\) be the final node embeddings coming out of the GNN.
It is well known that Eq. ( 2 ) is remarkably general; it can be used to express popular models such as Transformers 19 as a special case, and it has been argued that all discrete deep learning models can be expressed in this form 20 , 21 . This makes GNNs a perfect framework for benchmarking various approaches to modelling player–player interactions in the context of football.
Different choices of ψ , ϕ and ⨁ yield different architectures. In our case, we utilise a message function that factorises into an attentional mechanism, \(a:{{\mathbb{R}}}^{k}\times {{\mathbb{R}}}^{k}\times {{\mathbb{R}}}^{l}\times {{\mathbb{R}}}^{m}\to {\mathbb{R}}\) :
yielding the graph attention network (GAT) architecture 12 . In our work, specifically, we use a two-layer multilayer perceptron for the attentional mechanism, as proposed by GATv2 11 :
where \({{{{{{{{\bf{W}}}}}}}}}_{1},\, {{{{{{{{\bf{W}}}}}}}}}_{2}\in {{\mathbb{R}}}^{k\times h}\) , \({{{{{{{{\bf{W}}}}}}}}}_{e}\in {{\mathbb{R}}}^{l\times h}\) , \({{{{{{{{\bf{W}}}}}}}}}_{g}\in {{\mathbb{R}}}^{m\times h}\) and \({{{{{{{\bf{a}}}}}}}}\in {{\mathbb{R}}}^{h}\) are the learnable parameters of the attentional mechanism, and LeakyReLU is the leaky rectified linear activation function. This mechanism computes coefficients of interaction (a single scalar value) for each pair of connected nodes ( u , v ), which are then normalised across all neighbours of u using the \({{{{{{{\rm{softmax}}}}}}}}\) function.
Through early-stage experimentation, we have ascertained that GATs are capable of matching the performance of more generic choices of ψ (such as the MPNN 17 ) while being more scalable. Hence, we focus our study on the GAT model in this work. More details can be found in the subsection “Ablation study” section.
Geometric deep learning
In spite of the power of Eq. ( 2 ), using it in its full generality is often prone to overfitting, given the large number of parameters contained in ψ and ϕ . This problem is exacerbated in the football analytics domain, where gold-standard data is generally very scarce—for example, in the English Premier League, only a few hundred games are played every season.
In order to tackle this issue, we can exploit the immense regularity of data arising from football games. Strategically equivalent game states are also called transpositions, and symmetries such as arriving at the same chess position through different move sequences have been exploited computationally since the 1960s 22 . Similarly, game rotations and reflections may yield equivalent strategic situations 23 . Using the blueprint of geometric deep learning (GDL) 10 , we can design specialised GNN architectures that exploit this regularity.
That is, geometric deep learning is a generic methodology for deriving mathematical constraints on neural networks, such that they will behave predictably when inputs are transformed in certain ways. In several important cases, these constraints can be directly resolved, directly informing neural network architecture design. For a comprehensive example of point clouds under 3D rotational symmetry, see Fuchs et al. 24 .
To elucidate several aspects of the GDL framework on a high level, let us assume that there exists a group of input data transformations (symmetries), \({\mathfrak{G}}\) under which the ground-truth label remains unchanged. Specifically, if we let y ( X , E , g ) be the label given to the graph featurised with X , E , g , then for every transformation \({\mathfrak{g}}\in {\mathfrak{G}}\) , the following property holds:
This condition is also referred to as \({\mathfrak{G}}\) -invariance. Here, by \({\mathfrak{g}}({{{{{{{\bf{X}}}}}}}})\) we denote the result of transforming X by \({\mathfrak{g}}\) —a concept also known as a group action. More generally, it is a function of the form \({\mathfrak{G}}\times {{{{{{{\mathcal{S}}}}}}}}\to {{{{{{{\mathcal{S}}}}}}}}\) for some state set \({{{{{{{\mathcal{S}}}}}}}}\) . Note that a single group element, \({\mathfrak{g}}\in {\mathfrak{G}}\) can easily produce different actions on different \({{{{{{{\mathcal{S}}}}}}}}\) —in this case, \({{{{{{{\mathcal{S}}}}}}}}\) could be \({{\mathbb{R}}}^{| {{{{{{{\mathcal{V}}}}}}}}| \times k}\) ( X ), \({{\mathbb{R}}}^{| {{{{{{{\mathcal{V}}}}}}}}| \times | {{{{{{{\mathcal{V}}}}}}}}| \times l}\) ( E ) and \({{\mathbb{R}}}^{m}\) ( g ).
It is worth noting that GNNs may also be derived using a GDL perspective if we set the symmetry group \({\mathfrak{G}}\) to \({S}_{| {{{{{{{\mathcal{V}}}}}}}}}|\) , the permutation group of \(| {{{{{{{\mathcal{V}}}}}}}}|\) objects. Owing to the design of Eq. ( 2 ), its outputs will not be dependent on the exact permutation of nodes in the input graph.
Frame averaging
A simple mechanism to enforce \({\mathfrak{G}}\) -invariance, given any predictor \({f}_{{{{{{{{\mathcal{G}}}}}}}}}({{{{{{{\bf{X}}}}}}}},\, {{{{{{{\bf{E}}}}}}}},\, {{{{{{{\bf{g}}}}}}}})\) , performs frame averaging across all \({\mathfrak{G}}\) -transformed inputs:
This ensures that all \({\mathfrak{G}}\) -transformed versions of a particular input (also known as that input’s orbit) will have exactly the same output, satisfying Eq. ( 5 ). A variant of this approach has also been applied in the AlphaGo architecture 25 to encode symmetries of a Go board.
In our specific implementation, we set \({\mathfrak{G}}={D}_{2}=\{{{{{{{{\rm{id}}}}}}}},\leftrightarrow,\updownarrow,\leftrightarrow \updownarrow \}\) , the dihedral group. Exploiting D 2 -invariance allows us to encode quadrant symmetries. Each element of the D 2 group encodes the presence of vertical or horizontal reflections of the input football pitch. Under these transformations, the pitch is assumed completely symmetric, and hence many predictions, such as which player receives the corner kick, or takes a shot from it, can be safely assumed unchanged. As an example of how to compute transformed features in Eq. ( 6 ), ↔( X ) horizontally reflects all positional features of players in X (e.g. the coordinates of the player), and negates the x -axis component of their velocity.
Group convolutions
While the frame averaging approach of Eq. ( 6 ) is a powerful way to restrict GNNs to respect input symmetries, it arguably misses an opportunity for the different \({\mathfrak{G}}\) -transformed views to interact while their computations are being performed. For small groups such as D 2 , a more fine-grained approach can be assumed, operating over a single GNN layer in Eq. ( 2 ), which we will write shortly as \({{{{{{{{\bf{H}}}}}}}}}^{(t)}={g}_{{{{{{{{\mathcal{G}}}}}}}}}({{{{{{{{\bf{H}}}}}}}}}^{(t-1)},\, {{{{{{{\bf{E}}}}}}}},\, {{{{{{{\bf{g}}}}}}}})\) . The condition that we need a symmetry-respecting GNN layer to satisfy is as follows, for all transformations \({\mathfrak{g}}\in {\mathfrak{G}}\) :
that is, it does not matter if we apply \({\mathfrak{g}}\) it to the input or the output of the function \({g}_{{{{{{{{\mathcal{G}}}}}}}}}\) —the final answer is the same. This condition is also referred to as \({\mathfrak{G}}\) -equivariance, and it has recently proved to be a potent paradigm for developing powerful GNNs over biochemical data 24 , 26 .
To satisfy D 2 -equivariance, we apply the group convolution approach 13 . Therein, views of the input are allowed to directly interact with their \({\mathfrak{G}}\) -transformed variants, in a manner very similar to grid convolutions (which is, indeed, a special case of group convolutions, setting \({\mathfrak{G}}\) to be the translation group). We use \({{{{{{{{\bf{H}}}}}}}}}_{{\mathfrak{g}}}^{(t)}\) to denote the \({\mathfrak{g}}\) -transformed view of the latent node features at layer t . Omitting E and g inputs for brevity, and using our previously designed layer \({g}_{{{{{{{{\mathcal{G}}}}}}}}}\) as a building block, we can perform a group convolution as follows:
Here, ∥ is the concatenation operation, joining the two node feature matrices column-wise; \({{\mathfrak{g}}}^{-1}\) is the inverse transformation to \({\mathfrak{g}}\) (which must exist as \({\mathfrak{G}}\) is a group); and \({{\mathfrak{g}}}^{-1}{\mathfrak{h}}\) is the composition of the two transformations.
Effectively, Eq. ( 8 ) implies our D 2 -equivariant GNN needs to maintain a node feature matrix \({{{{{{{{\bf{H}}}}}}}}}_{{\mathfrak{g}}}^{(t)}\) for every \({\mathfrak{G}}\) -transformation of the current input, and these views are recombined by invoking \({g}_{{{{{{{{\mathcal{G}}}}}}}}}\) on all pairs related together by applying a transformation \({\mathfrak{h}}\) . Note that all reflections are self-inverses, hence, in D 2 , \({\mathfrak{g}}={{\mathfrak{g}}}^{-1}\) .
It is worth noting that both the frame averaging in Eq. ( 6 ) and group convolution in Eq. ( 8 ) are similar in spirit to data augmentation. However, whereas standard data augmentation would only show one view at a time to the model, a frame averaging/group convolution architecture exhaustively generates all views and feeds them to the model all at once. Further, group convolutions allow these views to explicitly interact in a way that does not break symmetries. Here lies the key difference between the two approaches: frame averaging and group convolutions rigorously enforce the symmetries in \({\mathfrak{G}}\) , whereas data augmentation only provides implicit hints to the model about satisfying them. As a consequence of the exhaustive generation, Eqs. ( 6 ) and ( 8 ) are only feasible for small groups like D 2 . For larger groups, approaches like Steerable CNNs 27 may be employed.
Network architectures
While the three benchmark tasks we are performing have minor differences in the global features available to the model, the neural network models designed for them all have the same encoder–decoder architecture. The encoder has the same structure in all tasks, while the decoder model is tailored to produce appropriately shaped outputs for each benchmark task.
Given an input graph, TacticAI’s model first generates all relevant D 2 -transformed versions of it, by appropriately reflecting the player coordinates and velocities. We refer to the original input graph as the identity view, and the remaining three D 2 -transformed graphs as reflected views.
Once the views are prepared, we apply four group convolutional layers (Eq. ( 8 )) with a GATv2 base model (Eqs. ( 3 ) and ( 4 )) as the \({g}_{{{{{{{{\mathcal{G}}}}}}}}}\) function. Specifically, this means that, in Eqs. ( 3 ) and ( 4 ), every instance of \({{{{{{{{\bf{h}}}}}}}}}_{u}^{(t-1)}\) is replaced by the concatenation of \({({{{{{{{{\bf{h}}}}}}}}}_{{\mathfrak{h}}}^{(t-1)})}_{u}\parallel {({{{{{{{{\bf{h}}}}}}}}}_{{{\mathfrak{g}}}^{-1}{\mathfrak{h}}}^{(t-1)})}_{u}\) . Each GATv2 layer has eight attention heads and computes four latent features overall per player. Accordingly, once the four group convolutions are performed, we have a representation of \({{{{{{{\bf{H}}}}}}}}\in {{\mathbb{R}}}^{4\times 22\times 4}\) , where the first dimension corresponds to the four views ( \({{{{{{{{\bf{H}}}}}}}}}_{{{{{{{{\rm{id}}}}}}}}},\, {{{{{{{{\bf{H}}}}}}}}}_{\leftrightarrow },\, {{{{{{{{\bf{H}}}}}}}}}_{\updownarrow },\, {{{{{{{{\bf{H}}}}}}}}}_{\leftrightarrow \updownarrow }\in {{\mathbb{R}}}^{22\times 4}\) ), the second dimension corresponds to the players (eleven on each team), and the third corresponds to the 4-dimensional latent vector for each player node in this particular view. How this representation is used by the decoder depends on the specific downstream task, as we detail below.
For receiver prediction, which is a fully invariant function (i.e. reflections do not change the receiver), we perform simple frame averaging across all views, arriving at
and then learn a node-wise classifier over the rows of \({{{{{{{{\bf{H}}}}}}}}}^{{{{{{{{\rm{node}}}}}}}}}\in {{\mathbb{R}}}^{22\times 4}\) . We further decode H node into a logit vector \({{{{{{{\bf{O}}}}}}}}\in {{\mathbb{R}}}^{22}\) with a linear layer before computing the corresponding softmax cross entropy loss.
For shot prediction, which is once again fully invariant (i.e. reflections do not change the probability of a shot), we can further average the frame-averaged features across all players to get a global graph representation:
and then learn a binary classifier over \({{{{{{{{\bf{h}}}}}}}}}^{{{{{{{{\rm{graph}}}}}}}}}\in {{\mathbb{R}}}^{4}\) . Specifically, we decode the hidden vector into a single logit with a linear layer and compute the sigmoid binary cross-entropy loss with the corresponding label.
For guided generation (position/velocity adjustments), we generate the player positions and velocities with respect to a particular outcome of interest for the human coaches, predicted over the rows of the hidden feature matrix. For example, the model may adjust the defensive setup to decrease the shot probability by the attacking team. The model output is now equivariant rather than invariant—reflecting the pitch appropriately reflects the predicted positions and velocity vectors. As such, we cannot perform frame averaging, and take only the identity view’s features, \({{{{{{{{\bf{H}}}}}}}}}_{{{{{{{{\rm{id}}}}}}}}}\in {{\mathbb{R}}}^{22\times 4}\) . From this latent feature matrix, we can then learn a conditional distribution from each row, which models the positions or velocities of the corresponding player. To do this, we extend the backbone encoder with conditional variational autoencoder (CVAE 28 , 29 ). Specifically, for the u -th row of H id , h u , we first map its latent embedding to the parameters of a two-dimensional Gaussian distribution \({{{{{{{\mathcal{N}}}}}}}}({\mu }_{u}| {\sigma }_{u})\) , and then sample the coordinates and velocities from this distribution. At training time, we can efficiently propagate gradients through this sampling operation using the reparameterisation trick 28 : sample a random value \({\epsilon }_{u} \sim {{{{{{{\mathcal{N}}}}}}}}(0,1)\) for each player from the unit Gaussian distribution, and then treat μ u + σ u ϵ u as the sample for this player. In what follows, we omit edge features for brevity. For each corner kick sample X with the corresponding outcome o (e.g. a binary value indicating a shot event), we extend the standard VAE loss 28 , 29 to our case of outcome-conditional guided generation as
where h u is the player embedding corresponding to the u th row of H id , and \({\mathbb{KL}}\) is Kullback–Leibler (KL) divergence. Specifically, the first term is the generation loss between the real player input x u and the reconstructed sample decoded from h u with the decoder p ϕ . Using the KL term, the distribution of the latent embedding h u is regularised towards p ( h u ∣ o ), which is a multivariate Gaussian in our case.
A complete high-level summary of the generic encoder–decoder equivariant architecture employed by TacticAI can be summarised in Supplementary Fig. 2 . In the following section, we will provide empirical evidence for justifying these architectural decisions. This will be done through targeted ablation studies on our predictive benchmarks (receiver prediction and shot prediction).
Ablation study
We leveraged the receiver prediction task as a way to evaluate various base model architectures, and directly quantitatively assess the contributions of geometric deep learning in this context. We already see that the raw corner kick data can be better represented through geometric deep learning, yielding separable clusters in the latent space that could correspond to different attacking or defending tactics (Fig. 2 ). In addition, we hypothesise that these representations can also yield better performance on the task of receiver prediction. Accordingly, we ablate several design choices using deep learning on this task, as illustrated by the following four questions:
Does a factorised graph representation help? To assess this, we compare it against a convolutional neural network (CNN 30 ) baseline, which does not leverage a graph representation.
Does a graph structure help? To assess this, we compare against a Deep Sets 31 baseline, which only models each node in isolation without considering adjacency information—equivalently, setting each neighbourhood \({{{{{{{{\mathcal{N}}}}}}}}}_{u}\) to a singleton set { u }.
Are attentional GNNs a good strategy? To assess this, we compare against a message passing neural network 32 , MPNN baseline, which uses the fully potent GNN layer from Eq. ( 2 ) instead of the GATv2.
Does accounting for symmetries help? To assess this, we compare our geometric GATv2 baseline against one which does not utilise D 2 group convolutions but utilises D 2 frame averaging, and one which does not explicitly utilise any aspect of D 2 symmetries at all.
Each of these models has been trained for a fixed budget of 50,000 training steps. The test top- k receiver prediction accuracies of the trained models are provided in Supplementary Table 2 . As already discussed in the section “Results”, there is a clear advantage to using a full graph structure, as well as directly accounting for reflection symmetry. Further, the usage of the MPNN layer leads to slight overfitting compared to the GATv2, illustrating how attentional GNNs strike a good balance of expressivity and data efficiency for this task. Our analysis highlights the quantitative benefits of both graph representation learning and geometric deep learning for football analytics from tracking data. We also provide a brief ablation study for the shot prediction task in Supplementary Table 3 .
Training details
We train each of TacticAI’s models in isolation, using NVIDIA Tesla P100 GPUs. To minimise overfitting, each model’s learning objective is regularised with an L 2 norm penalty with respect to the network parameters. During training, we use the Adam stochastic gradient descent optimiser 33 over the regularised loss.
All models, including baselines, have been given an equal hyperparameter tuning budget, spanning the number of message passing steps ({1, 2, 4}), initial learning rate ({0.0001, 0.00005}), batch size ({128, 256}) and L 2 regularisation coefficient ({0.01, 0.005, 0.001, 0.0001, 0}). We summarise the chosen hyperparameters of each TacticAI model in Supplementary Table 1 .
Data availability
The data collected in the human experiments in this study have been deposited in the Zenodo database under accession code https://zenodo.org/records/10557063 , and the processed data which is used in the statistical analysis and to generate the relevant figures in the main text are available under the same accession code. The input and output data generated and/or analysed during the current study are protected and are not available due to data privacy laws and licensing restrictions. However, contact details of the input data providers are available from the corresponding authors on reasonable request.
Code availability
All the core models described in this research were built with the Graph Neural Network processors provided by the CLRS Algorithmic Reasoning Benchmark 18 , and their source code is available at https://github.com/google-deepmind/clrs . We are unable to release our code for this work as it was developed in a proprietary context; however, the corresponding authors are open to answer specific questions concerning re-implementations on request. For general data analysis, we used the following freely available packages: numpy v1.25.2 , pandas v1.5.3 , matplotlib v3.6.1 , seaborn v0.12.2 and scipy v1.9.3 . Specifically, the code of the statistical analysis conducted in this study is available at https://zenodo.org/records/10557063 .
The International Football Association Board (IFAB). Laws of the Game (The International Football Association Board, 2023).
Tuyls, K. et al. Game plan: what AI can do for football, and what football can do for AI. J. Artif. Intell. Res. 71 , 41–88 (2021).
Article Google Scholar
Goka, R., Moroto, Y., Maeda, K., Ogawa, T. & Haseyama, M. Prediction of shooting events in soccer videos using complete bipartite graphs and players’ spatial–temporal relations. Sensors 23 , 4506 (2023).
Article ADS PubMed PubMed Central Google Scholar
Omidshafiei, S. et al. Multiagent off-screen behavior prediction in football. Sci. Rep. 12 , 8638 (2022).
Article ADS CAS PubMed PubMed Central Google Scholar
Lang, S., Wild, R., Isenko, A. & Link, D. Predicting the in-game status in soccer with machine learning using spatiotemporal player tracking data. Sci. Rep. 12 , 16291 (2022).
Baccouche, M., Mamalet, F., Wolf, C., Garcia, C. & Baskurt, A. Action classification in soccer videos with long short-term memory recurrent neural networks. In International Conference on Artificial Neural Networks (eds Diamantaras, K., Duch, W. & Iliadis, L. S.) pages 154–159 (Springer, 2010).
Shaw, L. & Gopaladesikan, S. Routine inspection: a playbook for corner kicks. In Machine Learning and Data Mining for Sports Analytics: 7th International Workshop, MLSA 2020, Co-located with ECML/PKDD 2020 , Proceedings, Ghent, Belgium, September 14–18, 2020, Vol . 7 , 3–16 (Springer, 2020).
Araújo, D. & Davids, K. Team synergies in sport: theory and measures. Front. Psychol. 7 , 1449 (2016).
Article PubMed PubMed Central Google Scholar
Veličković, P. Everything is connected: graph neural networks. Curr. Opin. Struct. Biol. 79 , 102538 (2023).
Article PubMed Google Scholar
Bronstein, M. M., Bruna, J., Cohen, T. & Veličković, P. Geometric deep learning: grids, groups, graphs, geodesics, and gauges. arXiv preprint arXiv:2104.13478 (2021).
Brody, S., Alon, U. & Yahav, E. How attentive are graph attention networks? In International Conference on Learning Representations (ICLR, 2022). https://openreview.net/forum?id=F72ximsx7C1 .
Veličković, P. et al. Graph attention networks. In International Conference on Learning Representations (ICLR, 2018). https://openreview.net/forum?id=rJXMpikCZ .
Cohen, T. & Welling, M. Group equivariant convolutional networks. In International Conference on Machine Learning (eds Balcan, M. F. & Weinberger, K. Q.) 2990–2999 (PMLR, 2016).
Honda, Y., Kawakami, R., Yoshihashi, R., Kato, K. & Naemura, T. Pass receiver prediction in soccer using video and players’ trajectories. In 2022 IEEE/CVF Conference on Computer Vision and Pattern Recognition Workshops (CVPRW) 3502–3511 (2022). https://ieeexplore.ieee.org/document/9857310 .
Hubáček, O., Sourek, G. & Železný, F. Deep learning from spatial relations for soccer pass prediction. In MLSA@PKDD/ECML (eds Brefeld, U., Davis, J., Van Haaren, J. & Zimmermann, A.) Vol. 11330, (Lecture Notes in Computer Science, Springer, Cham, 2018).
Sanyal, S. Who will receive the ball? Predicting pass recipient in soccer videos. J Visual Commun. Image Represent. 78 , 103190 (2021).
Gilmer, J., Schoenholz, S. S., Riley, P. F., Vinyals, O. & Dahl, G. E. Neural message passing for quantum chemistry. In International Conference on Machine Learning (Precup, D. & Teh, Y. W.) 1263–1272 (PMLR, 2017).
Veličković, P. et al. The CLRS algorithmic reasoning benchmark. In International Conference on Machine Learning (eds Chaudhuri, K. et al.) 22084–22102 (PMLR, 2022).
Vaswani, A. et al. Attention is all you need. In Advances in Neural Information Processing Systems (eds Guyon, I. et al.) Vol. 30 (Curran Associates, Inc., 2017).
Veličković, P. Message passing all the way up. In ICLR 2022 Workshop on Geometrical and Topological Representation Learning (GTRL, 2022). https://openreview.net/forum?id=Bc8GiEZkTe5 .
Baranwal, A., Kimon, F. & Aukosh, J. Optimality of message-passing architectures for sparse graphs. In Thirty-seventh Conference on Neural Information Processing Systems (2023). https://papers.nips.cc/paper_files/paper/2023/hash/7e991aa4cd2fdf0014fba2f000f542d0-Abstract-Conference.html .
Greenblatt, R. D., Eastlake III, D. E. & Crocker, S. D. The Greenblatt chess program. In Proc. Fall Joint Computer Conference , 14–16 , 801–810 (Association for Computing Machinery, 1967). https://dl.acm.org/doi/10.1145/1465611.1465715 .
Schijf, M., Allis, L. V. & Uiterwijk, J. W. Proof-number search and transpositions. ICGA J. 17 , 63–74 (1994).
Fuchs, F., Worrall, D., Fischer, V. & Welling, M. SE(3)-transformers: 3D roto-translation equivariant attention networks. Adv. Neural Inf. Process. Syst. 33 , 1970–1981 (2020).
Google Scholar
Silver, D. et al. Mastering the game of Go with deep neural networks and tree search. Nature 529 , 484–489 (2016).
Article ADS CAS PubMed Google Scholar
Satorras, V. G., Hoogeboom, E. & Welling, M. E ( n ) equivariant graph neural networks. In International Conference on Machine Learning (eds Meila, M. & Zhang, T.) 9323–9332 (PMLR, 2021).
Cohen, T. S. & Welling, M. Steerable CNNs. In International Conference on Learning Representations (ICLR, 2017). https://openreview.net/forum?id=rJQKYt5ll .
Kingma, D. P. & Welling, M. Auto-encoding variational Bayes. In 2nd International Conference on Learning Representations, ICLR 2014, Banff, AB, Canada, April 14–16, 2014, Conference Track Proceedings (ICLR, 2014). https://openreview.net/forum?id=33X9fd2-9FyZd .
Sohn, K., Lee, H. & Yan, X. Learning structured output representation using deep conditional generative models. In Advances in Neural Information Processing Systems (eds Cortes, C, Lawrence, N., Lee, D., Sugiyama, M. & Garnett, R.) Vol. 28 (Curran Associates, Inc., 2015).
Fernández, J. & Bornn, L. Soccermap: a deep learning architecture for visually-interpretable analysis in soccer. In Machine Learning and Knowledge Discovery in Databases. Applied Data Science and Demo Track: European Conference, ECML PKDD 2020, Ghent, Belgium, September 14–18, 2020, Proceedings, Part V (eds Dong, Y., Ifrim, G., Mladenić, D., Saunders, C. & Van Hoecke, S.) 491–506 (Springer, 2021).
Zaheer, M. et al. Deep sets. In Advances in Neural Information Processing Systems Vol. 30 (eds Guyon, I., et al.) (Curran Associates, Inc., 2017).
Gilmer, J., Schoenholz, S. S., Riley, P. F., Vinyals, O. & Dahl, G. E. Neural message passing for quantum chemistry. In Proc. 34th International Conference on Machine Learning , Vol. 70 of Proceedings of Machine Learning Research, 6–11 Aug 2017 (eds Precup, D. & Whye Teh, Y) 1263–1272 (PMLR, 2017).
Kingma, G. E. & Ba, J. Adam: a method for stochastic optimization. In ICLR (Poster) , (eds Bengio, Y. & LeCun, Y.) (International Conference of Learning Representations (ICLR), 2015). https://openreview.net/forum?id=8gmWwjFyLj .
Download references
Acknowledgements
We gratefully acknowledge the support of James French, Timothy Waskett, Hans Leitert and Benjamin Hervey for their extensive efforts in analysing TacticAI’s outputs. Further, we are thankful to Kevin McKee, Sherjil Ozair and Beatrice Bevilacqua for useful technical discussions, and Marc Lanctôt and Satinder Singh for reviewing the paper prior to submission.
Author information
These authors contributed equally: Zhe Wang, Petar Veličković, Daniel Hennes.
Authors and Affiliations
Google DeepMind, 6-8 Handyside Street, London, N1C 4UZ, UK
Zhe Wang, Petar Veličković, Daniel Hennes, Nenad Tomašev, Laurel Prince, Michael Kaisers, Yoram Bachrach, Romuald Elie, Li Kevin Wenliang, Federico Piccinini, Jerome Connor, Yi Yang, Adrià Recasens, Mina Khan, Nathalie Beauguerlange, Pablo Sprechmann, Pol Moreno, Nicolas Heess & Demis Hassabis
Liverpool FC, AXA Training Centre, Simonswood Lane, Kirkby, Liverpool, L33 5XB, UK
William Spearman
Liverpool FC, Kirkby, UK
University of Alberta, Amii, Edmonton, AB, T6G 2E8, Canada
Michael Bowling
Google DeepMind, London, UK
You can also search for this author in PubMed Google Scholar
Contributions
Z.W., D. Hennes, L.P. and K.T. coordinated and organised the research effort leading to this paper. P.V. and Z.W. developed the core TacticAI models. Z.W., W.S. and I.G. prepared the Premier League corner kick dataset used for training and evaluating these models. P.V., Z.W., D. Hennes and N.T. designed the case study with human experts and Z.W. and P.V. performed the qualitative evaluation and statistical analysis of its outcomes. Z.W., P.V., D. Hennes, N.T., L.P., M. Kaisers, Y.B., R.E., L.K.W., F.P., W.S., I.G., N.H., M.B., D. Hassabis and K.T. contributed to writing the paper and providing feedback on the final manuscript. J.C., Y.Y., A.R., M. Khan, N.B., P.S. and P.M. contributed valuable technical and implementation discussions throughout the work’s development.
Corresponding authors
Correspondence to Zhe Wang , Petar Veličković or Karl Tuyls .
Ethics declarations
Competing interests.
The authors declare no competing interests but the following competing interests: TacticAI was developed during the course of the Authors’ employment at Google DeepMind and Liverpool Football Club, as applicable to each Author.
Peer review
Peer review information.
Nature Communications thanks Rui Luo and the other anonymous reviewer(s) for their contribution to the peer review of this work. A peer review file is available.
Additional information
Publisher’s note Springer Nature remains neutral with regard to jurisdictional claims in published maps and institutional affiliations.
Supplementary information
Supplementary information, peer review file, rights and permissions.
Open Access This article is licensed under a Creative Commons Attribution 4.0 International License, which permits use, sharing, adaptation, distribution and reproduction in any medium or format, as long as you give appropriate credit to the original author(s) and the source, provide a link to the Creative Commons licence, and indicate if changes were made. The images or other third party material in this article are included in the article’s Creative Commons licence, unless indicated otherwise in a credit line to the material. If material is not included in the article’s Creative Commons licence and your intended use is not permitted by statutory regulation or exceeds the permitted use, you will need to obtain permission directly from the copyright holder. To view a copy of this licence, visit http://creativecommons.org/licenses/by/4.0/ .
Reprints and permissions
About this article
Cite this article.
Wang, Z., Veličković, P., Hennes, D. et al. TacticAI: an AI assistant for football tactics. Nat Commun 15 , 1906 (2024). https://doi.org/10.1038/s41467-024-45965-x
Download citation
Received : 13 October 2023
Accepted : 08 February 2024
Published : 19 March 2024
DOI : https://doi.org/10.1038/s41467-024-45965-x
Share this article
Anyone you share the following link with will be able to read this content:
Sorry, a shareable link is not currently available for this article.
Provided by the Springer Nature SharedIt content-sharing initiative
By submitting a comment you agree to abide by our Terms and Community Guidelines . If you find something abusive or that does not comply with our terms or guidelines please flag it as inappropriate.
Quick links
- Explore articles by subject
- Guide to authors
- Editorial policies
Sign up for the Nature Briefing newsletter — what matters in science, free to your inbox daily.


An official website of the United States government
The .gov means it’s official. Federal government websites often end in .gov or .mil. Before sharing sensitive information, make sure you’re on a federal government site.
The site is secure. The https:// ensures that you are connecting to the official website and that any information you provide is encrypted and transmitted securely.
- Publications
- Account settings
Preview improvements coming to the PMC website in October 2024. Learn More or Try it out now .
- Advanced Search
- Journal List
- Children (Basel)

Child Malnutrition in Pakistan: Evidence from Literature
Muhammad asim.
1 Population Research Center, University of Texas, Austin, TX 78712, USA
2 Department of Sociology, University of Sargodha, Sargodha, Punjab 40100, Pakistan; [email protected]
Yasir Nawaz
Pakistan has one of the highest prevalences of child malnutrition as compared to other developing countries. This narrative review was accomplished to examine the published empirical literature on children’s nutritional status in Pakistan. The objectives of this review were to know about the methodological approaches used in previous studies, to assess the overall situation of childhood malnutrition, and to identify the areas that have not yet been studied. This study was carried out to collect and synthesize the relevant data from previously published papers through different scholarly database search engines. The most relevant and current published papers between 2000–2016 were included in this study. The research papers that contain the data related to child malnutrition in Pakistan were assessed. A total of 28 articles was reviewed and almost similar methodologies were used in all of them. Most of the researchers conducted the cross sectional quantitative and descriptive studies, through structured interviews for identifying the causes of child malnutrition. Only one study used the mix method technique for acquiring data from the respondents. For the assessment of malnutrition among children, out of 28 papers, 20 used the World Health Organization (WHO) weight for age, age for height, and height for weight Z-score method. Early marriages, large family size, high fertility rates with a lack of birth spacing, low income, the lack of breast feeding, and exclusive breastfeeding were found to be the themes that repeatedly emerged in the reviewed literature. There is a dire need of qualitative and mixed method researches to understand and have an insight into the underlying factors of child malnutrition in Pakistan.
1. Introduction
There are 165 million malnourished children under five years around the globe [ 1 ]. Malnutrition accounts for at least half of all childhood deaths worldwide [ 2 , 3 ]. Child malnutrition is generally only a problem of developing and underdeveloped countries [ 4 ]. Malnutrition is the fundamental cause of morbidity and mortality among the children [ 5 ]. Almost half of the mortality in children around the globe is attributed to undernutrition [ 6 ]. It also poses a risk to children’s physical and mental development, which results in poor academic achievement [ 7 ]. Adequate nutrition is indispensable to ensure a strong immune system and proper physical and intellectual development in early childhood [ 8 , 9 ].
It has been estimated that 170 million (30%) of children under the age of five in the world are moderately or severely stunted, and 110 million (19%) are moderately or severely underweight [ 10 ]. Almost half of all stunned children reside in Asia, 51 million (8%) children under five years of age are wasted, and two thirds of all wasted children live in Asia [ 11 ]. Malnutrition affects the future health and socioeconomic development of children and the dynamic prospective of the society. Pakistan has been reported to have one of the highest levels of prevalence of child malnutrition compared to other developing counties [ 12 ]. According to the National Nutrition Survey, 33%of all children were underweight, nearly 44% were stunted, 15% are wasted, 50%were anemic, and 33%were anemic (iron deficiency). In the last two decades, there has been a little reduction in the prevalence of child malnutrition in Pakistan compared to other developing countries [ 13 ].
Despite economic and social development, childhood malnutrition still remains a major public health and social problem in less developed countries [ 3 , 14 , 15 ]. The contributing factors in childhood malnutrition are low birth weight, inadequate breast feeding and exclusive breastfeeding, inappropriate complementary feeding, maternal education, lack of proper knowledge of nutrition, micronutrient intake, parity, birth spacing, household socioeconomic status, food insecurity, poor sanitation, vaccination, and infectious diseases [ 16 , 17 , 18 , 19 , 20 ]. Pakistan is among the countries in the world with the highest rates of child malnutrition, and its progress in child nutrition and health remains slower than in other South Asian countries [ 21 , 22 , 23 ].
2. Aim and Objectives of the Review
Malnutrition is the underlying cause of child morbidity and mortality in Pakistan. It has not been the priority issue of government of Pakistan to overcome the malnutrition in children. The aim of this narrative review is to collate and synthesize the published data to understand the problem as a whole. The dearth of studies on child malnutrition in Pakistan has evoked the researcher to search for and review the existing relevant literature. The specific objectives of this review article were:
- (i) to identify about the range of methodologies and methods to access the children malnutrition;
- (ii) to highlight the emerging themes to address the children malnutrition;
- (iii) to identify the geographical areas that are neglected in this research in Pakistan; and
- (iv) to provide recommendations for future work.
3. Methodology
Malnutrition is widely known as under-nutrition until and unless it is not specified [ 24 ]. Malnutrition in children is assessed through stunting, wasting, and underweight in children less than five years of age [ 25 ]. Stunting is chronic malnutrition, underweight is acute malnutrition, and wasting is the combination of acute and chronic malnutrition. Pakistan has been divided into four administrative provinces: Punjab, Sindh, Khyber Pakhtunkhwa (KPK), and Baluchistan. More than two-thirds of the population of Pakistan live in rural areas, where the prevalence of poverty is more common. Most of the rural people are engaged in agriculture and agricultural labor, taking care of livestock, and informal business and have the highest prevalence of malnutrition among their children.
As the topic is public health and anthropological relevance, various databases were utilized, including PubMed, Medline, NCBI, CINAHL, Scopus, and social science citation index. A number of keywords and combination of keywords were used to locate the relevant literature, such as “child malnutrition in Pakistan”, “child undernutrition in Pakistan”, “nutritional status of children in Pakistan”, “child health and nutritional status in Pakistan”, and “child health situation in Pakistan”. Furthermore, the list of references of relevant papers was also reviewed to access for more relevant literature on child malnutrition.
Inclusion and Exclusion Criteria
The most relevant and current published papers in between 2000–2016 were included in this methodological review article. The research papers that contain the data related to Pakistan were assessed. The review papers, case studies, and letters to the editors were not entertained in this study. At the first stage, through different keywords, 178 papers were searched through different search engines using medical subject heading. At the second stage, titles and abstracts were screened for inclusion in the current study, and duplicate and irrelevant papers (review papers, opinion, and letter to editor) were discarded. At the third stage, 69 papers were selected to review the title of the papers. At the fourth stage, the whole abstracts of the selected papers were reviewed and screened, and at the fifth stage, the decision was made to either include or exclude to review the whole article on the basis of full text papers. At the final stage, 28 papers were considered relevant and included for accomplishing this study. Narrative review is a method used to describe trends in the research field by calculating how many studies have used certain research methodologies, where they were carried out and what are the associated factors with problem under investigation. In a narrative review, the statistical analysis is not possible because the previously published studies were diverse in their objectives and questions. Narrative reviews do connect information into themes. The meta-analysis is only suitable for statistical analysis. Meta-analysis can only be undertaken when studies address the same question and administer the intervention in a similar manner or measure the same outcomes [ 26 ]. There was no any disagreement between the authors in the whole procedure of inclusion and exclusion of the papers in this study. The systematic method of selecting the relevant papers is presented in Figure 1 .

The process of selection of relevant papers is given criteria.
4.1. Methodological Findings
It was observed that in four papers ( Table 1 ), body mass index (BMI) was used to access the malnutrition status of children. Two authors used weight and height through Jelliffe’s classification, one paper used Harvard standard, and two papers used the rapid assessment approach mid upper arm circumference (MUAC) for assessing the malnutrition among children.
Description of the studies included in the review.
MUAC, mid upper arm circumference; NCHS, National Center for Health Sciences; WHO, World Health Organization; Z score, standard score; KPK, Khyber Pakhtunkhwa. *VHHD (very high human development), HHD (high human development), MHD (medium human development), LHD (low human development), VLHD (very low human development).
In 12 papers, the target population of the children was school-age (more than five years), and 15 papers focused on less than five years’ children in the assessment of malnutrition. Different national and international reports have warned that first 1000 days are the window of the opportunity to strengthen the nutritional status of the children [ 1 , 26 , 27 , 28 , 29 ]. Only two papers focused on the most vulnerable population of less than two years for the assessment of the malnutrition. Out of 28 studies, 13 studies were community-based, nine were school-based, four were hospital-based, and one was from secondary data analysis. Mostly, the studies were community-based and school-based for the assessment of the child nutrition in Pakistan. Out of 28 papers, 10 papers were published in international journals, and the rest of them were published in different Pakistani journals.
Different other cross-sectional research and regional studies presented varied results in term of stunting, wasting, and underweight according to their methodology and geographical area. The prevalence of child malnutrition according to the studies under reviewed is discussed in Table 2 . In KPK, district Nowshera, Ali [ 30 ] found that 12.5% children were stunted, 15% were underweight, and 7% were wasted. Another study from KPK, Afridi accounted that 14% children are underweight, 8% were underweight and stunned in Swat [ 31 ]. In a study from Quetta, Baluchistan [ 32 ], it was reported that one out of two (48%) children were stunned and 10% were wasted. In another study Ansari [ 33 ] highlighted that 22% children were stunned, 10% were wasted, and 24% were underweight in urban areas of Pakistan. Similar finding was also presented by the Batool [ 34 ] that the prevalence of stunting was 46% and underweight was 25% and 18% was under weight. In Sindh province, the situation of children in term of stunting, wasting, and underweight is alarming according to different studies. Nisar reported that 61% of children were stunned, 54% were underweight, and 47% were wasted in rural Sindh [ 35 ]. Similar findings were observed by Shah, who found that 26% children were wasted, 55% were stunned, and 15% were both wasted and stunned in hospitalized children [ 36 ].
Major findings of studies included in the review.
4.2. Coverage of Geographical Area
Figure 2 shows a map of Pakistan and geographic areas under review in this study. Most of the studies on child malnutrition focused on Sind and Punjab provinces. In Sind province, most of the studies focused in the rural areas. In the Punjab, most of the studies were conducted in central Punjab, and only one study covered the area of southern Punjab. Punjab has more than half of the population of Pakistan but still has many districts and divisions that have not yet to be studied, to know about the severity of the problem. In KPK, six researches were found on child malnutrition, two from Peshawar, and two from Swat. Two studies were conducted in the capital city (Islamabad). However, only one study has been carried out in the provincial capital Quetta, Baluchistan, and none of the research on child malnutrition was carried out in Gilgit Baltistan, Azad Jammu Kashmir, and Federally Administrated Tribal Areas (FATA).

Coverage of geographical areas in the studies reviewed. The total number does not equal the total number of studies as certain studies focused on more than one area.
4.3. Child Age
Different studies have highlighted that the severity of malnutrition occurs in a particular age group of children. Laghari [ 37 ] and Gul and Kibria [ 38 ] pointed out that the prevalence of malnutrition is considerably higher in children less than two years of age. Studies from Sindh, Tharparkar, and Umerkot also exhibited similar finding that the highest prevalence of malnutrition was found in children less than two years of age [ 39 ]. Nisar assessed the nutritional status of hospitalized children and found that children less than two years of age had a higher prevalence of nutritional anemia and poor nutritional status in the capital city of Pakistan [ 35 ]. Nuruddin [ 40 ] also pointed out that the prevalence of wasting, stunting, and underweight were higher in the age group of those less than two years of age.
4.4. Rural Urban Disparities
There is rural and urban disparity in the prevalence of child malnutrition across the world. Mushtaq pointed out that in rural areas the prevalence of child malnutrition was higher compared to urban areas [ 41 ]. The burden of malnutrition was lower in urban areas as compared to rural areas in Pakistan. Similar finding was presented by Anwer, who stated that children belong to rural areas are highly vulnerable of all types of malnutrition. In rural areas of Faisalabad, 65% of children were underweight, 41% were stunned, and 33% were wasted [ 42 ]. According to the national nutrition survey of Pakistan, acute and chronic child malnutrition were significantly higher in rural areas as compared to urban areas across the country [ 13 ].
4.5. Gender
Gender is an important indicator of child malnutrition. Khattak found there was no significant difference in stunting and wasting in male and female children [ 43 ]. Many other studies, including Khan [ 44 ], Mushtaq [ 41 ], and Shah [ 36 ], also ascertained that stunting and thinness was not associated with gender. Laghari [ 37 ] stated that severe malnutrition was reported higher in females than male children. Similar findings were also presented by Ansari [ 33 ], who stated that female children were three times more likely to be stunned than male children. Khuwaja [ 45 ] and Batool [ 34 ] also pointed out that female children were found more likely to be stunned as compared to male children. Another perspective was also presented in some research where the highest prevalence of malnutrition was found in boys as compared to girls. Fryarand and Ogden determined that boys were 1.5 times more likely to be underweight than girls [ 46 ]. There was a greater likelihood ratio of undernutrition in boys as compared to girls [ 47 ]. According to the Pakistan Demographic and Health Survey [ 48 ], the prevalence of stunting was slightly higher (51%) in boys than in girls (45%).
5. Discussion
5.1. methodological issues.
Table 1 depicted that similar methodology had been used to collect data on child malnutrition in previously published papers in Pakistan. However, most of the researchers have conducted the cross sectional quantitative and descriptive studies, through structured interviews for assessment of child malnutrition. Kroger pointed out that this is the best technique to overcome the interviewer’s influences [ 60 ]. Various medical and social indicators are being used to examine the nutritional status of children i.e., MUAC, anthropometry, calories intake method, hemoglobin test, and through skin fold thickness [ 61 ]. It was found that most of the research used anthropometric assessments include wasting (weight-for-height), stunting (height-forage), and underweight (weight-for-age) by the National Center for Health Sciences (NCHS)/World Health Organization (WHO) for assessment of child nutritional status [ 62 , 63 ]. The WHO classification is widely used as the benchmark for assessing the malnutrition children under the age of five across the globe. WHO classification is currently the most accepted method for assessing malnutrition in children [ 64 ]. This criterion was formulated and updated, keeping in view the growth standard of the six courtiers across the world i.e., Ghana, Brazil, India, Oman, the United States of America (USA), and Norway through a multicenter growth reference study in 2006 [ 65 ]. WHO classification for child malnutrition provides comprehensive study of all types of child malnutrition. This is why most of the researchers used WHO classification to assess the child malnutrition in their studies in Pakistan. However, the MUAC technique is used as a quick and easy method to identify the malnutrition in under five years children [ 66 , 67 , 68 ]. MUAC is a better indicator to observe the nutritional status of children in emergency situations [ 69 ]. Some other anthropometric indicators have been used for the assessment of child’s nutritional status are Jelliffe’s classification, Gomez’s classification, and weight for age using Harvard standard. Another social indicator dietary diversity or calories intake method and hemoglobin level biomedical method are also used for the nutritional assessment of children. However, in different papers, BMI was also used for nutritional assessment especially for children of more than five years of age. Dietary diversity or calories intake method is a complex method to access the malnutrition status among children [ 70 ]. Therefore, the researchers could not find any study for the assessment of nutritional status among children through calories in take method in previously published papers in Pakistan from 2000–2016. The nutritional status of infants and children has proved almost impossible to estimate accurately through the calorie intake approach. For these age groups, it is generally agreed that anthropometric method provides more reliable estimates [ 71 ]. Therefore, most of the researchers utilized the anthropometric methods to access the nutritional status of children.
5.2. Prevalence of Malnutrition among Children
According to the latest national survey, 31.5% children were stunned, 45% were underweight, and 10.5% were wasted [ 47 ]. Similar results were presented in another national study that 15% children were wasted, 43% were stunting, and 31% were underweight [ 13 ]. According to United Nations International Children’s Fund (UNICEF), the global stunting rate is 23%, underweight is 16%, and wasting is 7%. Keeping in view the global prevalence of undernutrition, Pakistan has a higher burden of all the types of undernutrition in all the previously published research reviewed in this study. The government should launch policy measures to reduce the burden of all types of malnutrition in Pakistan. The prevalence of all types of child malnutrition is varied in different research under review in this study due to different sample size, geographical areas, type of studies, and different measuring techniques of child malnutrition. The prevalence of child malnutrition according to the studies under review is presented in Table 2 .
5.3. Associated Factors with Malnutrition
Most of the researchers highlighted that socioeconomic, demographic, and lifestyle factors are responsible of malnutrition in children. Associated factors with child malnutrition according to the studies under review are briefly explained in Table 2 . According to Khattak et al. (2010) [ 43 ], there is a strong association of malnourished children with family size, household income and number of children in a family. Gul and Kibria [ 38 ] stated that socioeconomic factors leading towards malnutrition were mother’s illiteracy, younger mothers, and multiple parities. Batool [ 34 ] and Mushtaq [ 55 ] pointed out that poverty, lower education, low income, and overcrowded houses were the associated factors of children malnutrition. Malnutrition is directly associated with large family size, mother’s illiteracy, and poverty. In Pakistan, the typical family size is almost seven members per household, indicating one member is fulfilling the social, economic, and biological needs of the whole family. Almost 40% of the Pakistani population lives under the poverty line. Ali pointed out that illiteracy, large family size, lack of or exclusive breastfeeding, early weaning, and poverty were the associated factors for child under nutrition [ 30 ]. Mahmood [ 49 ] pointed out that malnutrition is significantly associated with maternal literacy and the presence of family members with special needs. Households with uneducated parents tend to have low income and have large families where household head not able to spend more on food, which leads to growth failure in children. The uneducated parents also have not enough awareness and knowledge about the importance of child exclusive breastfeeding and initiation of complementary feedings that are prerequisites for child health and nutrition.
Ansari [ 33 ] pointed out that, in those houses where knowledge of childcare practices are insufficient, food insecurity exists, lack of mother’s education, and large family size were the major determinants of child stunting. Ullah [ 53 ] found that teenage pregnancy, large family size, and lack of child vaccination were major associated factors of child malnutrition in Swat. Shah [ 36 ] pointed out that stunned children were more likely to live in a house with more than three people sharing one room, mud houses, have incomplete child vaccinations, less income, and no formal education in the rural areas of Pakistan. Hasnain et al. (2010) [ 56 ] found that a birth interval of less than two years was the leading factor of child stunting in Dadu Sindh. The prevalence of stunting and wasting among children was more common in a nuclear family [ 52 ].
5.4. Child Age
Different national and international reports have warned that the first 1000 days are the window of the opportunity to strengthen the nutritional status of children [ 58 , 59 , 60 ]. Different published studies have clearly depicted that the higher burden of child malnutrition can be traced in children less than two years of age. The first phase of malnutrition starts in the gestational period due to lack of proper diet of women during pregnancy. Low birth-weight babies have higher risk of morbidity, mortality, and being malnourished. The second phase of malnutrition starts in infancy or the growth faltering stage, where children remain highly dependent on breastfeeding and complementary feeding. Therefore, it is suggested that more focus should be given on children less than two years of age to combat the early stages of severe malnutrition.
6. Conclusions
There is dearth of qualitative and mixed-method studies on the causes of child malnutrition in Pakistan. In terms of geographical area covered, most of the studies were conducted in the provinces of Punjab, Sindh, and Khyber Pakhtunkhwa, whereas Baluchistan, Azad Kashmir, Gilgit Baltistan, and Federally Administrated Tribal Areas (FATA) were largely neglected by researchers. The prevalence of all types of malnutrition in Pakistan was found to be higher than the global threshold value. It was found that malnutrition starts at an early age and remains persistent at later stages. Most of the researchers employed the anthropometry technique i.e., wasting (weight-for-height), stunting (height-forage), and underweight (weight-for-age) by the NCHS/WHO for assessment of child nutritional status. The most vulnerable age group of children (6–23 months) was entirely neglected to exclusively study infant and young child feeding practices. It was clearly evident that higher intensity of child malnutrition prevails in the rural areas of Pakistan. Early marriages, large family size, high fertility rates with a lack of birth spacing, low income, and the lack of breastfeeding and exclusive breastfeeding were found to be major determinants of child malnutrition.
7. Strength and Limitation of Study
This is the first study to review the range of methodologies and identify the neglected geographical areas and widely used methods of previously published papers assessing child malnutrition in Pakistan. This research will be helpful for further researchers to design their methodology and target population before conducting their research. This study highlighted methodological gaps: preferred techniques for assessing the child malnutrition, varied study designs, and tagged the geographical areas with human development index rating. However, there are some limitations in this study because the previously published papers were published in different time spans, varied types of populations were under-studied, the varied age groups of the children, the small sample sizes, and different natures of the studies. One-fourth of the studies reviewed in this research had sample sizes of less than 200, and it is very difficult to generalize the result of these small-scale studies. The studies reviewed in this research had the three types of the population groups i.e., community-based, hospital-based, and school-based studies. There was much variation in results of all three types of population, and it was difficult to draw conclusion about the causes of child malnutrition. Therefore, it is suggested that the future studies must be clear in the methodological stage for the selection of the homogeneous studies in term of target population, type and nature of the study, and sample size for better understanding the problem under investigation. There is a need for more research with a more homogeneous approach to know the current real situation of child malnutrition in a socio-cultural and demographic context.
8. Recommendation for Future Studies
It is suggested that further studies should focus on the causes of malnutrition in the infancy stage to strengthen the nutritional status of children and that the neglected areas should also be studied to highlight this problem. The major causes of child malnutrition are also associated with the mother’s reproductive health care behavior, maternal diet, and autonomy. It is therefore suggested that further research also study the maternal factor while exploring the causes of child malnutrition. There is also a need to know about the diet and type of food that mothers prefer to give their children while exploring the causes of child malnutrition. Therefore, it is also suggested that the dietary diversity (DD) recall method should also be utilized to know about the preferred food that mothers give to their children. The component of micronutrients deficiency was entirely omitted under discussion of the previously published research. It is recommended that the micronutrient assessment should be a component of future research for a better understanding of the type of malnutrition. There is also the dire need of qualitative and mixed-method research to understand and highlight the cultural and lifestyle factors that cause child malnutrition in Pakistan.
Acknowledgments
This paper is the part of a Ph.D. dissertation entitled “Mother Child Malnutrition: Causes and Consequences in Punjab, Pakistan” of the first author. The authors neither get any funding to accomplish this research project nor claim the costs from any agency to publish in open access.
Author Contributions
M.A. was responsible for content, literature review, and manuscript preparation. M.A. conceived and designed the study; M.A. emerged the themes of the study; M.A. designed the methodology; M.A. wrote the paper and Y.N. supervised the whole project.
Conflicts of Interest
The authors declare no potential conflicts of interest with respect to research, financial relationships, authorship, and/or publication of this article.
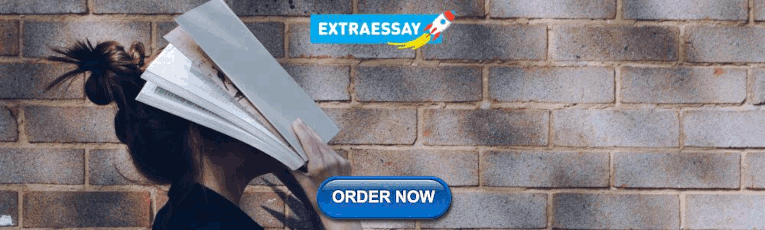
IMAGES
VIDEO
COMMENTS
Show abstract. ... Malnutrition can manifest as stunting, being underweight, wasting in women or adolescent girls, poor hygiene, and inadequate support for breastfeeding. Even mild malnutrition ...
Introduction. As studies show, malnutrition is one of the risk factors responsible for non-communicable diseases (NCDs) globally. 1 2 About one-third of people in any community have at least one form of malnutrition, which includes disorders caused by excessive and/or imbalanced intake, leading to obesity and overweight, and disorders caused by deficient intake of energy or nutrients, leading ...
Background. Malnutrition is a health condition resulting from eating food that contains either insufficient or too many calories, carbohydrates, vitamins, proteins or minerals. 1,2 It is a state of under- or overnutrition, evidenced by a deficiency or an excess of essential nutrients. 3 Good nutrition is the basic need for children to thrive, grow, learn, play and participate.
Introduction. Malnutrition refers to deficiency, excess, or an impaired utilization of one or more essential nutrients. 1 It consists of both under and over-nutrition. 2 Undernutrition includes wasting, stunting, underweight, and micronutrient deficiencies. 3 Malnutrition in any of its forms is a significant public health problem. 4 Globally, 29.8% of school-age children have insufficient ...
malnutrition in the greater familial and household contexts [40. Ghana addressed the concurrent occurrence of obesity and stunting in children. aged under 5 years, providing data for the first ...
Malnutrition is also a major resource issue for public expenditure. BAPEN has calculated that the costs associated with disease-related malnutrition in 2011-2012 in the UK were £19.6 billion (far greater than those associated with obesity), or about 15% of the total expenditure on health and social care. These costs represent an increase of ...
Children who experience poor nutrition during the first 1000 days of life are more vulnerable to illness and death in the near term, as well as to lower work capacity and productivity as adults. These problems motivate research to identify basic and underlying factors that influence risks of child malnutrition. Based on a structured search of existing literature, we identified 90 studies that ...
Today's publication of the WHO Series on the Double Burden of Malnutrition comes after 12 months of Lancet articles exploring nutrition in all its forms. In January, we published two complementary Commissions—Food in the Anthropocene: the EAT-Lancet Commission on healthy diets from sustainable food systems and The Global Syndemic of Obesity, Undernutrition, and Climate Change. In May and ...
Malnutrition remains prevalent and existing health problem globally 1,2,3.Particularly Undernutrition is a major public health issue in low and middle income countries 2,4,5,6.Across regions there ...
Shukla et al. [14] conducted a cross-sectional research on malnutrition and risk factors in children aged 6 to 59 months in the Jabalpur district's urban region (M.P.). 720 children between the ...
Background Globally, under-nutrition accounts for > 3 million deaths annually among children < 5 years, with Kenya having ~ 35,000 deaths. This study aimed to identify factors associated with malnutrition in children aged < 5 years in western Kenya. Methods We conducted a hospital-based unmatched case-control study between May and June 2017. Cases were defined as children aged 6-59 months ...
Background The prevalence of child malnutrition in Angola is still very high, and little is known about its associated factors. The aim of this study was to identify these factors in children under 2 years in a suburban area of the country's capital city. Methods We used data from a cross-sectional population-based study conducted in 2010. The outcomes studied were stunting and underweight ...
This study includes 67 published papers on malnutrition between 1998 and 2013, covering nine countries in Africa and four in Asia. Standard systematic review ... its determinants, its effects, and intervention research on malnutrition. One hundred and forty eight papers were identified and independently reviewed by the authors and 67 were ...
Popat CN, et al. A cross sectional study to measure the prevalence of malnutrition and factors associated with malnutrition among under five children of an urban slum of Vadodara city. Journal of research in medical and dental science. July -September. 2014;2(3):59-64. Google Scholar Gupta R, Chakrabarti S, Chatterjee SG.
Background Despite a large body of literature on the nexus between knowledge, attitude and practice towards nutrition and gender, this nexus is likely to vary and is not clear in many societies, such as Ethiopia. Objectives The study aimed to analyze the level of gender-based knowledge, attitude, and practice towards malnutrition and micronutrient deficiency using primary data collected from ...
All the data was collected on a proforma prepared for the said purpose. Results: The patients between 1-6 years of age were 53% (n=53); while 47 % ( n=47) were between 7-14 years of age. The mean ...
Malnutrition shows a decline in health due to the disproportion of nutrients and energy in the body. Malnutrition is one of the main risk factors related to children's morbidity and mortality. It is estimated that about 52.50% of child mortality is linked to malnutrition and its associated diseases. 1 Malnutrition is a crucial medical problem ...
This study aimed to determine the association between nutritional status and academic performance among primary school children in Debre Markos Town, northwest Ethiopia, 2017. The prevalence of stunting, underweight and wasting were 27.5% (95% CI 23.2-31.9%), 20.4% (95% CI 16.5-24.3%) and 8.7% (95% CI 6.2-11.5%), correspondingly. The low level of educational performance was significantly ...
Malnutrition is a serious problem with a negative impact on the quality of life and the evolution of patients, contributing to an increase in morbidity, length of hospital stay, mortality, and health spending. ... Over time, different nutritional assessment methods have been used, some complicated and expensive, used mainly in research, and ...
Rapid urban expansion has important health implications. This study examines trends and inequalities in undernutrition and overnutrition by gender, residence (rural, urban slum, urban non-slum), and wealth among children and adults in India. We used National Family Health Survey data from 2006 and 2016 (n = 311,182 children 0-5y and 972,192 adults 15-54y in total). We calculated differences ...
2017. TLDR. Although there has been a downward trend in the malnutrition rates of children 0-5 years old since 1990, the prevalence of malnutrition in the Philippines is still above the Millennium Development Goal (MDG) target and the Global Hunger Index (GHI) scored the Philippines at 20.1, indicating serious levels of hunger.
Malnutrition is one of the most critical areas which needs government focus as it plays a crucial role in a country's economic and social development. In recent times, India is proving to be one ...
Identifying key patterns of tactics implemented by rival teams, and developing effective responses, lies at the heart of modern football. However, doing so algorithmically remains an open research ...
The research papers that contain the data related to child malnutrition in Pakistan were assessed. A total of 28 articles was reviewed and almost similar methodologies were used in all of them. Most of the researchers conducted the cross sectional quantitative and descriptive studies, through structured interviews for identifying the causes of ...