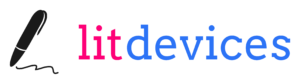
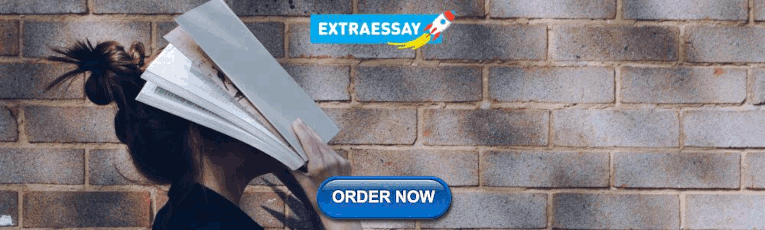
The Importance of Being Earnest
Introduction.
Welcome to a delightful journey through Oscar Wilde’s masterpiece, “The Importance of Being Earnest.” 🎠This play, first performed in 1895, is a brilliant satire of the Victorian era’s social mores and attitudes, especially concerning marriage and the pursuit of love. Wilde, known for his sharp wit and flamboyant style, was one of the most famous playwrights of his time. 📚
“The Importance of Being Earnest” falls under the genre of comedy , more specifically, a farce and a comedy of manners. It ridicules the societal norms of the late Victorian London, cleverly exposing the superficiality and pretentiousness of the upper class. Through its ingenious plot, memorable characters, and witty dialogue , Wilde critiques the triviality with which society treats institutions as serious as marriage, all while entertaining his audience with relentless humor and irony.
So, let’s dive into the world of mistaken identities, double lives, and the ultimate quest for love and earnestness, all served with a generous side of Oscar Wilde’s incomparable wit . 🌹🎩
Plot Summary
“The Importance of Being Earnest” is a whirlwind of romance , mistaken identities, and witty banter, all wrapped up in Oscar Wilde’s critique of Victorian society. Here’s a closer look at the main events that shape this unforgettable comedy :
Exposition — The play opens in Algernon Moncrieff’s luxurious London flat, where Algernon discovers that his friend, Jack Worthing, leads a double life. Jack is known as “Ernest” in London, where he often escapes to enjoy himself away from his country estate and his ward, Cecily Cardew.
Rising Action — Jack wishes to propose to Algernon’s cousin, Gwendolen Fairfax, but faces obstacles, including Gwendolen’s mother, Lady Bracknell, who disapproves of Jack’s unknown parentage. Meanwhile, Algernon, intrigued by Jack’s description of his young and pretty ward Cecily, decides to visit Jack’s country home, posing as the fictitious brother, “Ernest.”
Climax — Gwendolen and Cecily both believe themselves to be engaged to “Ernest.” When they meet at Jack’s country home and discover the confusion, tensions rise. Jack and Algernon are forced to confess their deceptions, leaving both relationships in jeopardy.
Falling Action — As the couples reconcile, Lady Bracknell arrives to confront the situation. Her objections to Jack’s proposal to Gwendolen remain, but she is swayed when she discovers Cecily is wealthy. However, Cecily’s fortune is conditional on her marrying someone named Ernest, adding to the complexity.
Resolution — The play reaches its resolution when it’s revealed that Jack is actually Algernon’s older brother, making him a suitable match for Gwendolen by birth and wealth. Furthermore, Jack discovers that his real name is indeed Ernest, satisfying the conditions of Cecily’s inheritance and fulfilling the earnest desires of both couples for love and marriage.
Through this series of misunderstandings and revelations, Wilde critiques the superficial values of Victorian society while entertaining the audience with the characters’ elaborate schemes to live up to societal expectations and find true love.
Character Analysis
In “The Importance of Being Earnest,” Oscar Wilde introduces us to a cast of characters who are both delightfully absurd and deeply reflective of the societal norms of his time. Let’s dive into the main characters:
- Jack Worthing — Jack is the play’s protagonist , who leads a double life as “Ernest” in London. He is responsible and respectable in the country but seeks escape and excitement in the city. Throughout the play, Jack’s challenge is to reconcile his dual identities and find happiness in love and truth.
- Algernon Moncrieff — Algernon is Jack’s friend, characterized by his witty, cynical outlook on life and a penchant for creating trouble. He also adopts an alter ego , visiting Jack’s country home as the fictitious “Ernest.” Algernon represents Wilde’s critique of the idle upper class, showing both the charm and the superficiality of high society.
- Gwendolen Fairfax — Gwendolen is Algernon’s cousin and Jack’s love interest. She embodies the Victorian ideal of a sophisticated, somewhat shallow, society lady. Gwendolen is fixated on the name Ernest, symbolizing the absurdity of societal expectations. Her character arc shows growth towards valuing sincerity over superficial qualities.
- Cecily Cardew — Cecily is Jack’s ward, a young and imaginative girl who becomes engaged to Algernon (as “Ernest”). Her fanciful nature contrasts with Gwendolen’s more worldly persona , but both women are similarly obsessed with the name Ernest. Cecily represents innocence and the romantic idealism that Wilde both admired and satirized.
- Lady Bracknell — Lady Bracknell is Gwendolen’s mother and the embodiment of Victorian societal norms. Authoritative, snobbish, and utterly unyielding, she serves as the main antagonist , blocking the young lovers’ paths to happiness with her concerns over social status and propriety.
- Miss Prism — Miss Prism is Cecily’s governess, whose past mistake with a baby carriage is a pivotal plot point. She represents the Victorian moral compass, but with her own quirks and a hidden past that adds depth to her character.
- Rev. Canon Chasuble, D.D. — The rector of Jack’s parish, Chasuble, provides comic relief through his interactions with Miss Prism and the young couples. He represents the Church’s presence in society, but his character is drawn with a light touch, avoiding deep religious commentary.
Character Analysis Summary
Through these characters, Wilde explores themes of identity, societal expectations, and the pursuit of happiness, all while entertaining us with their quirks and foibles.
Themes and Symbols
Oscar Wilde’s “The Importance of Being Earnest” is rich with themes and symbols that critique Victorian society while entertaining the audience with its wit and irony. Here are the major themes and symbols explored in the play:
- Dual Identity — The theme of living a double life is central to the play, illustrated by Jack’s and Algernon’s alter egos. This duality satirizes the hypocrisy and superficiality of the Victorian upper class, showing how societal pressures lead individuals to lead secret lives.
- The Critique of Marriage — Wilde uses the play to critique the Victorian attitudes towards marriage, portraying it as a social contract motivated by status and wealth rather than love. Lady Bracknell’s interrogation of Jack and her concerns over Cecily’s dowry underscore this theme .
- The Importance of Being “Earnest” — The play’s title itself is a pun on the double meaning of “earnest” as both a name and a trait of sincerity. Wilde plays with the idea that being earnest (sincere) is less important in society than having the right appearances or connections. The resolution suggests a reconciliation between the two, as Jack discovers his true identity and learns the value of being sincere.
- Food as a Symbol — Food in the play often symbolizes the characters’ indulgence and the excesses of the upper class. Algernon’s voracious appetite, particularly for cucumbers sandwiches, serves as a comedic element that also critiques the trivial concerns of the aristocracy.
- The Misplaced Manuscript — The manuscript that Miss Prism accidentally leaves in the perambulator is a pivotal symbol in the play. It represents the hidden truths and mistakes that underlie the characters’ public personas, leading to the ultimate unraveling of the plot and resolution of the characters’ various dilemmas.
- Gardens — The gardens in the play, especially in the country estate, symbolize a natural, idyllic space away from the constraints of society. They are settings for romantic pursuits and misunderstandings, contrasting with the more stifling atmosphere of London society.
These themes and symbols work together to create a layered critique of Wilde’s society, questioning the values and norms of the Victorian era with humor and insight. Through “The Importance of Being Earnest,” Wilde invites the audience to reflect on the absurdity of societal expectations and the importance of authenticity, all within the framework of a highly entertaining theatrical experience.
Style and Tone
Oscar Wilde’s writing style in “The Importance of Being Earnest” is distinguished by its wit , irony, and satirical edge, all of which contribute significantly to the mood and atmosphere of the play. Here’s how Wilde’s style and tone serve as the backbone of this comedic masterpiece:
- Wit and Wordplay — Wilde’s dialogue sparkles with wit and clever wordplay , making the most of puns , double entendres, and paradoxes. This linguistic playfulness not only entertains but also sharpens the critique of societal norms, highlighting the absurdity and hypocrisy of Victorian attitudes.
- Satire and Irony — The play is a satirical masterpiece, using irony to expose the superficiality and triviality of upper-class society. Wilde’s tone often oscillates between light-hearted jest and biting critique, allowing him to comment on serious social issues without losing the play’s comedic edge.
- Aestheticism — Wilde was a proponent of the aesthetic movement, which held that art should be pursued for its beauty alone rather than for its moral or political message. This philosophy is reflected in the play’s emphasis on clever dialogue and a plot that prioritizes entertainment and irony over moralizing.
- Epigrams — Wilde’s use of epigrams, or memorable, succinct sayings, is a hallmark of his writing style. These witty observations, often delivered by Algernon and Lady Bracknell, encapsulate Wilde’s views on society, marriage, and human nature in a way that is both humorous and thought-provoking.
- Farce and Comedy of Manners — The play is structured as a farce , featuring improbable situations, mistaken identities, and slapstick elements, all serving to exaggerate the follies of the characters and their situations. As a comedy of manners, it also focuses on satirizing the behaviors and customs of the aristocratic society, particularly in matters of marriage and courtship.
The interplay of these stylistic elements creates a distinctive tone that is at once light and serious, frivolous and profound. Wilde masterfully balances humor with social commentary, making “The Importance of Being Earnest” not only a delightful theatrical experience but also a sharp critique of the mores of his time.
Literary Devices used in The Importance of Being Earnest
Oscar Wilde’s “The Importance of Being Earnest” is a treasure trove of literary devices that enhance its satirical tone and comedic effect. Here are the top 10 literary devices Wilde employs, each serving to enrich the play’s texture and depth:
- Irony — The use of irony is pervasive, with Wilde often saying the opposite of what is meant, especially to highlight the absurdity of societal norms and expectations. The very title is ironic, considering the play’s exploration of deceit and dual identities.
- Satire — Wilde satirizes the Victorian society’s superficial values, particularly around marriage, social status, and the importance of appearances. Through exaggerated characters and situations, he critiques the moral hypocrisy of the era.
- Epigrams — Wilde’s epigrams are witty, concise statements that convey his observations on life, society, and human nature. These memorable lines are both humorous and insightful, adding a layer of sophistication to the dialogue .
- Paradox — The play is filled with paradoxical statements that challenge conventional wisdom and societal norms, encouraging the audience to see the absurdity in everyday assumptions and behaviors.
- Hyperbole — Exaggeration is used for comedic effect, especially in the characters’ reactions to situations and in their descriptions of each other. This device amplifies the play’s critique of the excesses and frivolity of upper-class society.
- Puns and Wordplay — Wilde’s love for puns and clever wordplay is evident throughout the play, with characters often engaging in verbal sparring that entertains while subtly revealing their personalities and relationships.
- Dramatic Irony — The audience is often in on secrets that the characters are not, creating humorous situations where characters speak or act in ignorance of the full context , enhancing the comedic effect.
- Foreshadowing — Subtle hints at future events or revelations are woven into the dialogue and action , building anticipation and adding layers to the narrative .
- Symbolism — Objects and actions in the play often symbolize larger themes or societal critiques, such as the importance of names, food as a symbol of excess, and the handbag which plays a pivotal role in the plot’s resolution .
- Repetition — Repetition is used for emphasis and comic effect, especially in the characters’ obsessions (like the name “Ernest”) and in the play’s running gags, reinforcing the critique of society’s absurdities.
These literary devices are not just stylistic flourishes; they are integral to the play’s themes and its effectiveness as a satirical comedy . Wilde’s masterful use of these tools engages the audience on multiple levels, making “The Importance of Being Earnest” a rich, multi-dimensional work that continues to entertain and provoke thought long after the curtain falls.
Literary Devices Examples
Let’s explore examples and explanations for each of the top 10 literary devices used in “The Importance of Being Earnest” by Oscar Wilde, showcasing how these devices contribute to the play’s wit , humor, and satirical commentary.
- Jack’s statement that he has “lost” his brother Ernest, which in reality means he is ceasing to pretend he has a brother.
- Lady Bracknell’s various pronouncements, such as her disapproval of Jack for not knowing his parents, which is ironic considering her obsession with social status.
- The entire concept of “Bunburying,” which is a fabrication but is treated with utmost seriousness by Algernon.
- Lady Bracknell’s interrogation of Jack about his suitability as a husband for Gwendolen, focusing on trivial matters rather than character.
- Algernon’s views on marriage and the importance of not being married, which satirize societal views on the institution.
- Miss Prism’s romantic novel , which she considers to be a serious work, satirizing the seriousness with which society takes itself.
- “The truth is rarely pure and never simple.”
- “All women become like their mothers. That is their tragedy . No man does. That’s his.”
- “To lose one parent may be regarded as a misfortune; to lose both looks like carelessness.”
- The concept of being earnest in name but not in behavior, as seen with both Jack and Algernon.
- Lady Bracknell’s assertion that ignorance is a delicate exotic fruit; touch it, and the bloom is gone, which contradicts the value of knowledge.
- Miss Prism’s statement that the good end happily, and the bad unhappily, which is the very definition of fiction , highlighting the paradox of societal expectations versus reality.
- Lady Bracknell’s shock at Jack being found in a handbag, exaggerating the importance of one’s origins to absurdity.
- Algernon’s excessive consumption of cucumber sandwiches intended for Lady Bracknell.
- Gwendolen’s declaration that she could never love a man named anything but Ernest, exaggerating the importance of a name.
Puns and Wordplay
- The play on the name Ernest/earnest throughout the play.
- Algernon’s statement about “Bunburying” as an invaluable permanent invalid, which plays on the words to critique social excuses.
- Jack’s declaration that he plans to “kill” his brother Ernest, playing with the concept of ending his double life.
Dramatic Irony
- The audience knows Jack’s and Algernon’s deceptions from the start, while the other characters do not.
- Lady Bracknell does not know that her own nephew is posing as Ernest.
- The audience is aware of the mix-up with the handbag before the characters realize its significance.
Foreshadowing
- Miss Prism’s uncomfortable reaction to the mention of a handbag hints at her involvement in the mix-up.
- Algernon’s interest in Cecily before he meets her, suggesting their future relationship.
- Jack’s disdain for his imaginary brother Ernest foreshadows his own troubles with his double identity.
- The handbag symbolizes Jack’s mysterious origins and his true identity.
- Food, particularly cucumber sandwiches and muffins, symbolizes the characters’ indulgence and the trivialities of their concerns.
- The name Ernest symbolizes the importance of sincerity and the absurdity of societal emphasis on appearances.
- The repeated insistence on the name Ernest by both Gwendolen and Cecily, emphasizing the absurdity of societal expectations.
- Lady Bracknell’s repeated concerns about social status and connections, underscoring her character’s superficial values.
- The repeated act of “Bunburying,” highlighting the characters’ duplicity and the lengths to which they will go to escape societal pressures.
Through these examples, it’s clear how Wilde skillfully uses literary devices to enhance the humor, develop characters, and critique societal norms, making “The Importance of Being Earnest” a rich and enduring work of literature.
The Importance of Being Earnest – FAQs
What is the main plot of “The Importance of Being Earnest”? The main plot revolves around two friends, Jack Worthing and Algernon Moncrieff, who use alter egos to escape their daily lives. Jack, who goes by Ernest in London, falls in love with Gwendolen Fairfax, while Algernon, posing as Ernest, falls for Cecily Cardew. The play unfolds with a series of comedic misunderstandings and revelations, ultimately exploring themes of identity, social hypocrisy, and the importance of being earnest.
Who is the author of “The Importance of Being Earnest”? Oscar Wilde, an Irish playwright, poet, and author, wrote “The Importance of Being Earnest.” Wilde is renowned for his wit , literary skills, and critique of Victorian society, which are prominently displayed in this play.
What are the main themes in “The Importance of Being Earnest”? The play explores several themes, including the triviality of societal norms, the duality of public and private selves, the absurdity of romantic and social conventions, and the critique of marriage as a social tool rather than an expression of love.
Why is the title “The Importance of Being Earnest” considered a pun? The title is a pun on the dual meaning of the word “earnest”—as a name (Ernest) and as a quality of sincerity or seriousness. The play humorously contrasts the characters’ lack of sincerity with their obsession with the name Ernest, highlighting the superficiality of societal values.
How does Oscar Wilde critique Victorian society in the play? Wilde critiques Victorian society through satire and irony, targeting its rigid social codes, hypocrisy, and the importance placed on appearances and propriety over genuine feelings and moral integrity. Characters such as Lady Bracknell epitomize the extreme and often absurd standards of the era.
Can “The Importance of Being Earnest” be considered a comedy of manners? Yes, it is a prime example of a comedy of manners, a genre that satirizes the behaviors, customs, and etiquette of a particular social class, often through witty dialogue and an intricate plot. The play mocks the Victorian upper class’s pretensions and moral values.
What literary devices does Oscar Wilde use in the play? Wilde employs numerous literary devices, including irony, satire, epigrams (witty, concise statements), paradoxes, and wordplay , to enhance the humor and critique of social norms.
What is the significance of the characters’ dual identities? The dual identities of Jack and Algernon symbolize the duality of Victorian society, where individuals often led double lives to escape the constraints of respectability and societal expectations. This theme questions the authenticity of social identities and the value of earnestness.
How does “The Importance of Being Earnest” reflect Oscar Wilde’s personal views? The play reflects Wilde’s views on art, society, and morality, showcasing his belief in aestheticism (art for art’s sake), his disdain for the superficiality of social conventions, and his advocacy for individuality and sincerity.
What impact has “The Importance of Being Earnest” had on modern theater? “The Importance of Being Earnest” remains one of the most enduring and frequently performed plays in the English language, admired for its wit , masterful language, and insightful critique of society. It has influenced countless playwrights and continues to be relevant in discussions of social hypocrisy, identity, and the nature of love and marriage.
This quiz is designed to test comprehension of “The Importance of Being Earnest” by Oscar Wilde, touching on key plot points, characters, themes, and literary devices.
Identify the literary devices used in the following excerpt from “The Importance of Being Earnest” by Oscar Wilde:
“ALGERNON: The only way to behave to a woman is to make love to her, if she is pretty, and to someone else, if she is plain.” “JACK: That is nonsense.” “ALGERNON: What about your brother? What about the profligate Ernest?” “JACK: Oh, that is all nonsense. Ernest is a very different person indeed.”
- Irony – Algernon’s statement about how to behave to a woman is ironic, given the play’s exploration of superficiality in relationships.
- Epigram – Algernon’s line is a witty, memorable saying that encapsulates a view of the world with humor and brevity.
- Satire – This exchange satirizes the way Victorian society approached courtship and marriage, with a focus on appearances and convenience rather than genuine connection.
- Characterization – Through this dialogue , Wilde further develops Algernon and Jack’s characters, showcasing Algernon’s cynical view on love and Jack’s more pragmatic and skeptical perspective .
- Foreshadowing – Mentioning the “profligate Ernest” foreshadows the later revelations about Jack’s own double life and the consequences of his deception.
The Importance of Being Earnest
A trivial comedy for serious people, the persons in the play.
John Worthing, J.P. Algernon Moncrieff Rev. Canon Chasuble, D.D. Merriman, Butler Lane, Manservant Lady Bracknell Hon. Gwendolen Fairfax Cecily Cardew Miss Prism, Governess
THE SCENES OF THE PLAY
ACT I. Algernon Moncrieff’s Flat in Half-Moon Street, W.
ACT II. The Garden at the Manor House, Woolton.
ACT III. Drawing-Room at the Manor House, Woolton.
TIME: The Present.
LONDON: ST. JAMES’S THEATRE
Lessee and Manager: Mr. George Alexander
February 14th, 1895
John Worthing, J.P.: Mr. George Alexander. Algernon Moncrieff: Mr. Allen Aynesworth. Rev. Canon Chasuble, D.D.: Mr. H. H. Vincent. Merriman: Mr. Frank Dyall. Lane: Mr. F. Kinsey Peile. Lady Bracknell: Miss Rose Leclercq. Hon. Gwendolen Fairfax: Miss Irene Vanbrugh. Cecily Cardew: Miss Evelyn Millard. Miss Prism: Mrs. George Canninge.
Morning-room in Algernon’s flat in Half-Moon Street. The room is luxuriously and artistically furnished. The sound of a piano is heard in the adjoining room.
[ Lane is arranging afternoon tea on the table, and after the music has ceased, Algernon enters.]
ALGERNON. Did you hear what I was playing, Lane?
LANE. I didn’t think it polite to listen, sir.
ALGERNON. I’m sorry for that, for your sake. I don’t play accurately—any one can play accurately—but I play with wonderful expression. As far as the piano is concerned, sentiment is my forte. I keep science for Life.
LANE. Yes, sir.
ALGERNON. And, speaking of the science of Life, have you got the cucumber sandwiches cut for Lady Bracknell?
LANE. Yes, sir. [Hands them on a salver.]
ALGERNON. [Inspects them, takes two, and sits down on the sofa.] Oh! . . . by the way, Lane, I see from your book that on Thursday night, when Lord Shoreman and Mr. Worthing were dining with me, eight bottles of champagne are entered as having been consumed.
LANE. Yes, sir; eight bottles and a pint.
ALGERNON. Why is it that at a bachelor’s establishment the servants invariably drink the champagne? I ask merely for information.
LANE. I attribute it to the superior quality of the wine, sir. I have often observed that in married households the champagne is rarely of a first-rate brand.
ALGERNON. Good heavens! Is marriage so demoralising as that?
LANE. I believe it is a very pleasant state, sir. I have had very little experience of it myself up to the present. I have only been married once. That was in consequence of a misunderstanding between myself and a young person.
ALGERNON. [Languidly . ] I don’t know that I am much interested in your family life, Lane.
LANE. No, sir; it is not a very interesting subject. I never think of it myself.
ALGERNON. Very natural, I am sure. That will do, Lane, thank you.
LANE. Thank you, sir. [ Lane goes out.]
ALGERNON. Lane’s views on marriage seem somewhat lax. Really, if the lower orders don’t set us a good example, what on earth is the use of them? They seem, as a class, to have absolutely no sense of moral responsibility.
[Enter Lane .]
LANE. Mr. Ernest Worthing.
[Enter Jack .]
[ Lane goes out . ]
ALGERNON. How are you, my dear Ernest? What brings you up to town?
JACK. Oh, pleasure, pleasure! What else should bring one anywhere? Eating as usual, I see, Algy!
ALGERNON. [Stiffly . ] I believe it is customary in good society to take some slight refreshment at five o’clock. Where have you been since last Thursday?
JACK. [Sitting down on the sofa.] In the country.
ALGERNON. What on earth do you do there?
JACK. [Pulling off his gloves . ] When one is in town one amuses oneself. When one is in the country one amuses other people. It is excessively boring.
ALGERNON. And who are the people you amuse?
JACK. [Airily . ] Oh, neighbours, neighbours.
ALGERNON. Got nice neighbours in your part of Shropshire?
JACK. Perfectly horrid! Never speak to one of them.
ALGERNON. How immensely you must amuse them! [Goes over and takes sandwich.] By the way, Shropshire is your county, is it not?
JACK. Eh? Shropshire? Yes, of course. Hallo! Why all these cups? Why cucumber sandwiches? Why such reckless extravagance in one so young? Who is coming to tea?
ALGERNON. Oh! merely Aunt Augusta and Gwendolen.
JACK. How perfectly delightful!
ALGERNON. Yes, that is all very well; but I am afraid Aunt Augusta won’t quite approve of your being here.
JACK. May I ask why?
ALGERNON. My dear fellow, the way you flirt with Gwendolen is perfectly disgraceful. It is almost as bad as the way Gwendolen flirts with you.
JACK. I am in love with Gwendolen. I have come up to town expressly to propose to her.
ALGERNON. I thought you had come up for pleasure? . . . I call that business.
JACK. How utterly unromantic you are!
ALGERNON. I really don’t see anything romantic in proposing. It is very romantic to be in love. But there is nothing romantic about a definite proposal. Why, one may be accepted. One usually is, I believe. Then the excitement is all over. The very essence of romance is uncertainty. If ever I get married, I’ll certainly try to forget the fact.
JACK. I have no doubt about that, dear Algy. The Divorce Court was specially invented for people whose memories are so curiously constituted.
ALGERNON. Oh! there is no use speculating on that subject. Divorces are made in Heaven—[ Jack puts out his hand to take a sandwich. Algernon at once interferes.] Please don’t touch the cucumber sandwiches. They are ordered specially for Aunt Augusta. [Takes one and eats it.]
JACK. Well, you have been eating them all the time.
ALGERNON. That is quite a different matter. She is my aunt. [Takes plate from below.] Have some bread and butter. The bread and butter is for Gwendolen. Gwendolen is devoted to bread and butter.
JACK. [Advancing to table and helping himself.] And very good bread and butter it is too.
ALGERNON. Well, my dear fellow, you need not eat as if you were going to eat it all. You behave as if you were married to her already. You are not married to her already, and I don’t think you ever will be.
JACK. Why on earth do you say that?
ALGERNON. Well, in the first place girls never marry the men they flirt with. Girls don’t think it right.
JACK. Oh, that is nonsense!
ALGERNON. It isn’t. It is a great truth. It accounts for the extraordinary number of bachelors that one sees all over the place. In the second place, I don’t give my consent.
JACK. Your consent!
ALGERNON. My dear fellow, Gwendolen is my first cousin. And before I allow you to marry her, you will have to clear up the whole question of Cecily. [Rings bell.]
JACK. Cecily! What on earth do you mean? What do you mean, Algy, by Cecily! I don’t know any one of the name of Cecily.
ALGERNON. Bring me that cigarette case Mr. Worthing left in the smoking-room the last time he dined here.
LANE. Yes, sir. [ Lane goes out.]
JACK. Do you mean to say you have had my cigarette case all this time? I wish to goodness you had let me know. I have been writing frantic letters to Scotland Yard about it. I was very nearly offering a large reward.
ALGERNON. Well, I wish you would offer one. I happen to be more than usually hard up.
JACK. There is no good offering a large reward now that the thing is found.
[Enter Lane with the cigarette case on a salver. Algernon takes it at once. Lane goes out.]
ALGERNON. I think that is rather mean of you, Ernest, I must say. [Opens case and examines it.] However, it makes no matter, for, now that I look at the inscription inside, I find that the thing isn’t yours after all.
JACK. Of course it’s mine. [Moving to him.] You have seen me with it a hundred times, and you have no right whatsoever to read what is written inside. It is a very ungentlemanly thing to read a private cigarette case.
ALGERNON. Oh! it is absurd to have a hard and fast rule about what one should read and what one shouldn’t. More than half of modern culture depends on what one shouldn’t read.
JACK. I am quite aware of the fact, and I don’t propose to discuss modern culture. It isn’t the sort of thing one should talk of in private. I simply want my cigarette case back.
ALGERNON. Yes; but this isn’t your cigarette case. This cigarette case is a present from some one of the name of Cecily, and you said you didn’t know any one of that name.
JACK. Well, if you want to know, Cecily happens to be my aunt.
ALGERNON. Your aunt!
JACK. Yes. Charming old lady she is, too. Lives at Tunbridge Wells. Just give it back to me, Algy.
ALGERNON. [Retreating to back of sofa.] But why does she call herself little Cecily if she is your aunt and lives at Tunbridge Wells? [Reading.] ‘From little Cecily with her fondest love.’
JACK. [Moving to sofa and kneeling upon it.] My dear fellow, what on earth is there in that? Some aunts are tall, some aunts are not tall. That is a matter that surely an aunt may be allowed to decide for herself. You seem to think that every aunt should be exactly like your aunt! That is absurd! For Heaven’s sake give me back my cigarette case. [Follows Algernon round the room.]
ALGERNON. Yes. But why does your aunt call you her uncle? ‘From little Cecily, with her fondest love to her dear Uncle Jack.’ There is no objection, I admit, to an aunt being a small aunt, but why an aunt, no matter what her size may be, should call her own nephew her uncle, I can’t quite make out. Besides, your name isn’t Jack at all; it is Ernest.
JACK. It isn’t Ernest; it’s Jack.
ALGERNON. You have always told me it was Ernest. I have introduced you to every one as Ernest. You answer to the name of Ernest. You look as if your name was Ernest. You are the most earnest-looking person I ever saw in my life. It is perfectly absurd your saying that your name isn’t Ernest. It’s on your cards. Here is one of them. [Taking it from case.] ‘Mr. Ernest Worthing, B. 4, The Albany.’ I’ll keep this as a proof that your name is Ernest if ever you attempt to deny it to me, or to Gwendolen, or to any one else. [Puts the card in his pocket.]
JACK. Well, my name is Ernest in town and Jack in the country, and the cigarette case was given to me in the country.
ALGERNON. Yes, but that does not account for the fact that your small Aunt Cecily, who lives at Tunbridge Wells, calls you her dear uncle. Come, old boy, you had much better have the thing out at once.
JACK. My dear Algy, you talk exactly as if you were a dentist. It is very vulgar to talk like a dentist when one isn’t a dentist. It produces a false impression.
ALGERNON. Well, that is exactly what dentists always do. Now, go on! Tell me the whole thing. I may mention that I have always suspected you of being a confirmed and secret Bunburyist; and I am quite sure of it now.
JACK. Bunburyist? What on earth do you mean by a Bunburyist?
ALGERNON. I’ll reveal to you the meaning of that incomparable expression as soon as you are kind enough to inform me why you are Ernest in town and Jack in the country.
JACK. Well, produce my cigarette case first.
ALGERNON. Here it is. [Hands cigarette case.] Now produce your explanation, and pray make it improbable. [Sits on sofa.]
JACK. My dear fellow, there is nothing improbable about my explanation at all. In fact it’s perfectly ordinary. Old Mr. Thomas Cardew, who adopted me when I was a little boy, made me in his will guardian to his grand-daughter, Miss Cecily Cardew. Cecily, who addresses me as her uncle from motives of respect that you could not possibly appreciate, lives at my place in the country under the charge of her admirable governess, Miss Prism.
ALGERNON. Where is that place in the country, by the way?
JACK. That is nothing to you, dear boy. You are not going to be invited . . . I may tell you candidly that the place is not in Shropshire.
ALGERNON. I suspected that, my dear fellow! I have Bunburyed all over Shropshire on two separate occasions. Now, go on. Why are you Ernest in town and Jack in the country?
JACK. My dear Algy, I don’t know whether you will be able to understand my real motives. You are hardly serious enough. When one is placed in the position of guardian, one has to adopt a very high moral tone on all subjects. It’s one’s duty to do so. And as a high moral tone can hardly be said to conduce very much to either one’s health or one’s happiness, in order to get up to town I have always pretended to have a younger brother of the name of Ernest, who lives in the Albany, and gets into the most dreadful scrapes. That, my dear Algy, is the whole truth pure and simple.
ALGERNON. The truth is rarely pure and never simple. Modern life would be very tedious if it were either, and modern literature a complete impossibility!
JACK. That wouldn’t be at all a bad thing.
ALGERNON. Literary criticism is not your forte, my dear fellow. Don’t try it. You should leave that to people who haven’t been at a University. They do it so well in the daily papers. What you really are is a Bunburyist. I was quite right in saying you were a Bunburyist. You are one of the most advanced Bunburyists I know.
JACK. What on earth do you mean?
ALGERNON. You have invented a very useful younger brother called Ernest, in order that you may be able to come up to town as often as you like. I have invented an invaluable permanent invalid called Bunbury, in order that I may be able to go down into the country whenever I choose. Bunbury is perfectly invaluable. If it wasn’t for Bunbury’s extraordinary bad health, for instance, I wouldn’t be able to dine with you at Willis’s to-night, for I have been really engaged to Aunt Augusta for more than a week.
JACK. I haven’t asked you to dine with me anywhere to-night.
ALGERNON. I know. You are absurdly careless about sending out invitations. It is very foolish of you. Nothing annoys people so much as not receiving invitations.
JACK. You had much better dine with your Aunt Augusta.
ALGERNON. I haven’t the smallest intention of doing anything of the kind. To begin with, I dined there on Monday, and once a week is quite enough to dine with one’s own relations. In the second place, whenever I do dine there I am always treated as a member of the family, and sent down with either no woman at all, or two. In the third place, I know perfectly well whom she will place me next to, to-night. She will place me next Mary Farquhar, who always flirts with her own husband across the dinner-table. That is not very pleasant. Indeed, it is not even decent . . . and that sort of thing is enormously on the increase. The amount of women in London who flirt with their own husbands is perfectly scandalous. It looks so bad. It is simply washing one’s clean linen in public. Besides, now that I know you to be a confirmed Bunburyist I naturally want to talk to you about Bunburying. I want to tell you the rules.
JACK. I’m not a Bunburyist at all. If Gwendolen accepts me, I am going to kill my brother, indeed I think I’ll kill him in any case. Cecily is a little too much interested in him. It is rather a bore. So I am going to get rid of Ernest. And I strongly advise you to do the same with Mr. . . . with your invalid friend who has the absurd name.
ALGERNON. Nothing will induce me to part with Bunbury, and if you ever get married, which seems to me extremely problematic, you will be very glad to know Bunbury. A man who marries without knowing Bunbury has a very tedious time of it.
JACK. That is nonsense. If I marry a charming girl like Gwendolen, and she is the only girl I ever saw in my life that I would marry, I certainly won’t want to know Bunbury.
ALGERNON. Then your wife will. You don’t seem to realise, that in married life three is company and two is none.
JACK. [Sententiously.] That, my dear young friend, is the theory that the corrupt French Drama has been propounding for the last fifty years.
ALGERNON. Yes; and that the happy English home has proved in half the time.
JACK. For heaven’s sake, don’t try to be cynical. It’s perfectly easy to be cynical.
ALGERNON. My dear fellow, it isn’t easy to be anything nowadays. There’s such a lot of beastly competition about. [The sound of an electric bell is heard.] Ah! that must be Aunt Augusta. Only relatives, or creditors, ever ring in that Wagnerian manner. Now, if I get her out of the way for ten minutes, so that you can have an opportunity for proposing to Gwendolen, may I dine with you to-night at Willis’s?
JACK. I suppose so, if you want to.
ALGERNON. Yes, but you must be serious about it. I hate people who are not serious about meals. It is so shallow of them.
LANE. Lady Bracknell and Miss Fairfax.
[ Algernon goes forward to meet them. Enter Lady Bracknell and Gwendolen .]
LADY BRACKNELL. Good afternoon, dear Algernon, I hope you are behaving very well.
ALGERNON. I’m feeling very well, Aunt Augusta.
LADY BRACKNELL. That’s not quite the same thing. In fact the two things rarely go together. [Sees Jack and bows to him with icy coldness.]
ALGERNON. [To Gwendolen .] Dear me, you are smart!
GWENDOLEN. I am always smart! Am I not, Mr. Worthing?
JACK. You’re quite perfect, Miss Fairfax.
GWENDOLEN. Oh! I hope I am not that. It would leave no room for developments, and I intend to develop in many directions. [ Gwendolen and Jack sit down together in the corner.]
LADY BRACKNELL. I’m sorry if we are a little late, Algernon, but I was obliged to call on dear Lady Harbury. I hadn’t been there since her poor husband’s death. I never saw a woman so altered; she looks quite twenty years younger. And now I’ll have a cup of tea, and one of those nice cucumber sandwiches you promised me.
ALGERNON. Certainly, Aunt Augusta. [Goes over to tea-table.]
LADY BRACKNELL. Won’t you come and sit here, Gwendolen?
GWENDOLEN. Thanks, mamma, I’m quite comfortable where I am.
ALGERNON. [Picking up empty plate in horror.] Good heavens! Lane! Why are there no cucumber sandwiches? I ordered them specially.
LANE. [Gravely.] There were no cucumbers in the market this morning, sir. I went down twice.
ALGERNON. No cucumbers!
LANE. No, sir. Not even for ready money.
ALGERNON. That will do, Lane, thank you.
LANE. Thank you, sir. [Goes out.]
ALGERNON. I am greatly distressed, Aunt Augusta, about there being no cucumbers, not even for ready money.
LADY BRACKNELL. It really makes no matter, Algernon. I had some crumpets with Lady Harbury, who seems to me to be living entirely for pleasure now.
ALGERNON. I hear her hair has turned quite gold from grief.
LADY BRACKNELL. It certainly has changed its colour. From what cause I, of course, cannot say. [ Algernon crosses and hands tea.] Thank you. I’ve quite a treat for you to-night, Algernon. I am going to send you down with Mary Farquhar. She is such a nice woman, and so attentive to her husband. It’s delightful to watch them.
ALGERNON. I am afraid, Aunt Augusta, I shall have to give up the pleasure of dining with you to-night after all.
LADY BRACKNELL. [Frowning.] I hope not, Algernon. It would put my table completely out. Your uncle would have to dine upstairs. Fortunately he is accustomed to that.
ALGERNON. It is a great bore, and, I need hardly say, a terrible disappointment to me, but the fact is I have just had a telegram to say that my poor friend Bunbury is very ill again. [Exchanges glances with Jack .] They seem to think I should be with him.
LADY BRACKNELL. It is very strange. This Mr. Bunbury seems to suffer from curiously bad health.
ALGERNON. Yes; poor Bunbury is a dreadful invalid.
LADY BRACKNELL. Well, I must say, Algernon, that I think it is high time that Mr. Bunbury made up his mind whether he was going to live or to die. This shilly-shallying with the question is absurd. Nor do I in any way approve of the modern sympathy with invalids. I consider it morbid. Illness of any kind is hardly a thing to be encouraged in others. Health is the primary duty of life. I am always telling that to your poor uncle, but he never seems to take much notice . . . as far as any improvement in his ailment goes. I should be much obliged if you would ask Mr. Bunbury, from me, to be kind enough not to have a relapse on Saturday, for I rely on you to arrange my music for me. It is my last reception, and one wants something that will encourage conversation, particularly at the end of the season when every one has practically said whatever they had to say, which, in most cases, was probably not much.
ALGERNON. I’ll speak to Bunbury, Aunt Augusta, if he is still conscious, and I think I can promise you he’ll be all right by Saturday. Of course the music is a great difficulty. You see, if one plays good music, people don’t listen, and if one plays bad music people don’t talk. But I’ll run over the programme I’ve drawn out, if you will kindly come into the next room for a moment.
LADY BRACKNELL. Thank you, Algernon. It is very thoughtful of you. [Rising, and following Algernon .] I’m sure the programme will be delightful, after a few expurgations. French songs I cannot possibly allow. People always seem to think that they are improper, and either look shocked, which is vulgar, or laugh, which is worse. But German sounds a thoroughly respectable language, and indeed, I believe is so. Gwendolen, you will accompany me.
GWENDOLEN. Certainly, mamma.
[ Lady Bracknell and Algernon go into the music-room, Gwendolen remains behind.]
JACK. Charming day it has been, Miss Fairfax.
GWENDOLEN. Pray don’t talk to me about the weather, Mr. Worthing. Whenever people talk to me about the weather, I always feel quite certain that they mean something else. And that makes me so nervous.
JACK. I do mean something else.
GWENDOLEN. I thought so. In fact, I am never wrong.
JACK. And I would like to be allowed to take advantage of Lady Bracknell’s temporary absence . . .
GWENDOLEN. I would certainly advise you to do so. Mamma has a way of coming back suddenly into a room that I have often had to speak to her about.
JACK. [Nervously.] Miss Fairfax, ever since I met you I have admired you more than any girl . . . I have ever met since . . . I met you.
GWENDOLEN. Yes, I am quite well aware of the fact. And I often wish that in public, at any rate, you had been more demonstrative. For me you have always had an irresistible fascination. Even before I met you I was far from indifferent to you. [ Jack looks at her in amazement.] We live, as I hope you know, Mr. Worthing, in an age of ideals. The fact is constantly mentioned in the more expensive monthly magazines, and has reached the provincial pulpits, I am told; and my ideal has always been to love some one of the name of Ernest. There is something in that name that inspires absolute confidence. The moment Algernon first mentioned to me that he had a friend called Ernest, I knew I was destined to love you.
JACK. You really love me, Gwendolen?
GWENDOLEN. Passionately!
JACK. Darling! You don’t know how happy you’ve made me.
GWENDOLEN. My own Ernest!
JACK. But you don’t really mean to say that you couldn’t love me if my name wasn’t Ernest?
GWENDOLEN. But your name is Ernest.
JACK. Yes, I know it is. But supposing it was something else? Do you mean to say you couldn’t love me then?
GWENDOLEN. [Glibly.] Ah! that is clearly a metaphysical speculation, and like most metaphysical speculations has very little reference at all to the actual facts of real life, as we know them.
JACK. Personally, darling, to speak quite candidly, I don’t much care about the name of Ernest . . . I don’t think the name suits me at all.
GWENDOLEN. It suits you perfectly. It is a divine name. It has a music of its own. It produces vibrations.
JACK. Well, really, Gwendolen, I must say that I think there are lots of other much nicer names. I think Jack, for instance, a charming name.
GWENDOLEN. Jack? . . . No, there is very little music in the name Jack, if any at all, indeed. It does not thrill. It produces absolutely no vibrations . . . I have known several Jacks, and they all, without exception, were more than usually plain. Besides, Jack is a notorious domesticity for John! And I pity any woman who is married to a man called John. She would probably never be allowed to know the entrancing pleasure of a single moment’s solitude. The only really safe name is Ernest.
JACK. Gwendolen, I must get christened at once—I mean we must get married at once. There is no time to be lost.
GWENDOLEN. Married, Mr. Worthing?
JACK. [Astounded.] Well . . . surely. You know that I love you, and you led me to believe, Miss Fairfax, that you were not absolutely indifferent to me.
GWENDOLEN. I adore you. But you haven’t proposed to me yet. Nothing has been said at all about marriage. The subject has not even been touched on.
JACK. Well . . . may I propose to you now?
GWENDOLEN. I think it would be an admirable opportunity. And to spare you any possible disappointment, Mr. Worthing, I think it only fair to tell you quite frankly before-hand that I am fully determined to accept you.
JACK. Gwendolen!
GWENDOLEN. Yes, Mr. Worthing, what have you got to say to me?
JACK. You know what I have got to say to you.
GWENDOLEN. Yes, but you don’t say it.
JACK. Gwendolen, will you marry me? [Goes on his knees.]
GWENDOLEN. Of course I will, darling. How long you have been about it! I am afraid you have had very little experience in how to propose.
JACK. My own one, I have never loved any one in the world but you.
GWENDOLEN. Yes, but men often propose for practice. I know my brother Gerald does. All my girl-friends tell me so. What wonderfully blue eyes you have, Ernest! They are quite, quite, blue. I hope you will always look at me just like that, especially when there are other people present. [Enter Lady Bracknell .]
LADY BRACKNELL. Mr. Worthing! Rise, sir, from this semi-recumbent posture. It is most indecorous.
GWENDOLEN. Mamma! [He tries to rise; she restrains him.] I must beg you to retire. This is no place for you. Besides, Mr. Worthing has not quite finished yet.
LADY BRACKNELL. Finished what, may I ask?
GWENDOLEN. I am engaged to Mr. Worthing, mamma. [They rise together.]
LADY BRACKNELL. Pardon me, you are not engaged to any one. When you do become engaged to some one, I, or your father, should his health permit him, will inform you of the fact. An engagement should come on a young girl as a surprise, pleasant or unpleasant, as the case may be. It is hardly a matter that she could be allowed to arrange for herself . . . And now I have a few questions to put to you, Mr. Worthing. While I am making these inquiries, you, Gwendolen, will wait for me below in the carriage.
GWENDOLEN. [Reproachfully.] Mamma!
LADY BRACKNELL. In the carriage, Gwendolen! [ Gwendolen goes to the door. She and Jack blow kisses to each other behind Lady Bracknell’s back. Lady Bracknell looks vaguely about as if she could not understand what the noise was. Finally turns round.] Gwendolen, the carriage!
GWENDOLEN. Yes, mamma. [Goes out, looking back at Jack .]
LADY BRACKNELL. [Sitting down.] You can take a seat, Mr. Worthing.
[Looks in her pocket for note-book and pencil.]
JACK. Thank you, Lady Bracknell, I prefer standing.
LADY BRACKNELL. [Pencil and note-book in hand.] I feel bound to tell you that you are not down on my list of eligible young men, although I have the same list as the dear Duchess of Bolton has. We work together, in fact. However, I am quite ready to enter your name, should your answers be what a really affectionate mother requires. Do you smoke?
JACK. Well, yes, I must admit I smoke.
LADY BRACKNELL. I am glad to hear it. A man should always have an occupation of some kind. There are far too many idle men in London as it is. How old are you?
JACK. Twenty-nine.
LADY BRACKNELL. A very good age to be married at. I have always been of opinion that a man who desires to get married should know either everything or nothing. Which do you know?
JACK. [After some hesitation.] I know nothing, Lady Bracknell.
LADY BRACKNELL. I am pleased to hear it. I do not approve of anything that tampers with natural ignorance. Ignorance is like a delicate exotic fruit; touch it and the bloom is gone. The whole theory of modern education is radically unsound. Fortunately in England, at any rate, education produces no effect whatsoever. If it did, it would prove a serious danger to the upper classes, and probably lead to acts of violence in Grosvenor Square. What is your income?
JACK. Between seven and eight thousand a year.
LADY BRACKNELL. [Makes a note in her book.] In land, or in investments?
JACK. In investments, chiefly.
LADY BRACKNELL. That is satisfactory. What between the duties expected of one during one’s lifetime, and the duties exacted from one after one’s death, land has ceased to be either a profit or a pleasure. It gives one position, and prevents one from keeping it up. That’s all that can be said about land.
JACK. I have a country house with some land, of course, attached to it, about fifteen hundred acres, I believe; but I don’t depend on that for my real income. In fact, as far as I can make out, the poachers are the only people who make anything out of it.
LADY BRACKNELL. A country house! How many bedrooms? Well, that point can be cleared up afterwards. You have a town house, I hope? A girl with a simple, unspoiled nature, like Gwendolen, could hardly be expected to reside in the country.
JACK. Well, I own a house in Belgrave Square, but it is let by the year to Lady Bloxham. Of course, I can get it back whenever I like, at six months’ notice.
LADY BRACKNELL. Lady Bloxham? I don’t know her.
JACK. Oh, she goes about very little. She is a lady considerably advanced in years.
LADY BRACKNELL. Ah, nowadays that is no guarantee of respectability of character. What number in Belgrave Square?
LADY BRACKNELL. [Shaking her head.] The unfashionable side. I thought there was something. However, that could easily be altered.
JACK. Do you mean the fashion, or the side?
LADY BRACKNELL. [Sternly.] Both, if necessary, I presume. What are your politics?
JACK. Well, I am afraid I really have none. I am a Liberal Unionist.
LADY BRACKNELL. Oh, they count as Tories. They dine with us. Or come in the evening, at any rate. Now to minor matters. Are your parents living?
JACK. I have lost both my parents.
LADY BRACKNELL. To lose one parent, Mr. Worthing, may be regarded as a misfortune; to lose both looks like carelessness. Who was your father? He was evidently a man of some wealth. Was he born in what the Radical papers call the purple of commerce, or did he rise from the ranks of the aristocracy?
JACK. I am afraid I really don’t know. The fact is, Lady Bracknell, I said I had lost my parents. It would be nearer the truth to say that my parents seem to have lost me . . . I don’t actually know who I am by birth. I was . . . well, I was found.
LADY BRACKNELL. Found!
JACK. The late Mr. Thomas Cardew, an old gentleman of a very charitable and kindly disposition, found me, and gave me the name of Worthing, because he happened to have a first-class ticket for Worthing in his pocket at the time. Worthing is a place in Sussex. It is a seaside resort.
LADY BRACKNELL. Where did the charitable gentleman who had a first-class ticket for this seaside resort find you?
JACK. [Gravely.] In a hand-bag.
LADY BRACKNELL. A hand-bag?
JACK. [Very seriously.] Yes, Lady Bracknell. I was in a hand-bag—a somewhat large, black leather hand-bag, with handles to it—an ordinary hand-bag in fact.
LADY BRACKNELL. In what locality did this Mr. James, or Thomas, Cardew come across this ordinary hand-bag?
JACK. In the cloak-room at Victoria Station. It was given to him in mistake for his own.
LADY BRACKNELL. The cloak-room at Victoria Station?
JACK. Yes. The Brighton line.
LADY BRACKNELL. The line is immaterial. Mr. Worthing, I confess I feel somewhat bewildered by what you have just told me. To be born, or at any rate bred, in a hand-bag, whether it had handles or not, seems to me to display a contempt for the ordinary decencies of family life that reminds one of the worst excesses of the French Revolution. And I presume you know what that unfortunate movement led to? As for the particular locality in which the hand-bag was found, a cloak-room at a railway station might serve to conceal a social indiscretion—has probably, indeed, been used for that purpose before now—but it could hardly be regarded as an assured basis for a recognised position in good society.
JACK. May I ask you then what you would advise me to do? I need hardly say I would do anything in the world to ensure Gwendolen’s happiness.
LADY BRACKNELL. I would strongly advise you, Mr. Worthing, to try and acquire some relations as soon as possible, and to make a definite effort to produce at any rate one parent, of either sex, before the season is quite over.
JACK. Well, I don’t see how I could possibly manage to do that. I can produce the hand-bag at any moment. It is in my dressing-room at home. I really think that should satisfy you, Lady Bracknell.
LADY BRACKNELL. Me, sir! What has it to do with me? You can hardly imagine that I and Lord Bracknell would dream of allowing our only daughter—a girl brought up with the utmost care—to marry into a cloak-room, and form an alliance with a parcel? Good morning, Mr. Worthing!
[ Lady Bracknell sweeps out in majestic indignation.]
JACK. Good morning! [ Algernon , from the other room, strikes up the Wedding March. Jack looks perfectly furious, and goes to the door.] For goodness’ sake don’t play that ghastly tune, Algy. How idiotic you are!
[The music stops and Algernon enters cheerily.]
ALGERNON. Didn’t it go off all right, old boy? You don’t mean to say Gwendolen refused you? I know it is a way she has. She is always refusing people. I think it is most ill-natured of her.
JACK. Oh, Gwendolen is as right as a trivet. As far as she is concerned, we are engaged. Her mother is perfectly unbearable. Never met such a Gorgon . . . I don’t really know what a Gorgon is like, but I am quite sure that Lady Bracknell is one. In any case, she is a monster, without being a myth, which is rather unfair . . . I beg your pardon, Algy, I suppose I shouldn’t talk about your own aunt in that way before you.
ALGERNON. My dear boy, I love hearing my relations abused. It is the only thing that makes me put up with them at all. Relations are simply a tedious pack of people, who haven’t got the remotest knowledge of how to live, nor the smallest instinct about when to die.
ALGERNON. It isn’t!
JACK. Well, I won’t argue about the matter. You always want to argue about things.
ALGERNON. That is exactly what things were originally made for.
JACK. Upon my word, if I thought that, I’d shoot myself . . . [A pause.] You don’t think there is any chance of Gwendolen becoming like her mother in about a hundred and fifty years, do you, Algy?
ALGERNON. All women become like their mothers. That is their tragedy. No man does. That’s his.
JACK. Is that clever?
ALGERNON. It is perfectly phrased! and quite as true as any observation in civilised life should be.
JACK. I am sick to death of cleverness. Everybody is clever nowadays. You can’t go anywhere without meeting clever people. The thing has become an absolute public nuisance. I wish to goodness we had a few fools left.
ALGERNON. We have.
JACK. I should extremely like to meet them. What do they talk about?
ALGERNON. The fools? Oh! about the clever people, of course.
JACK. What fools!
ALGERNON. By the way, did you tell Gwendolen the truth about your being Ernest in town, and Jack in the country?
JACK. [In a very patronising manner.] My dear fellow, the truth isn’t quite the sort of thing one tells to a nice, sweet, refined girl. What extraordinary ideas you have about the way to behave to a woman!
ALGERNON. The only way to behave to a woman is to make love to her, if she is pretty, and to some one else, if she is plain.
JACK. Oh, that is nonsense.
ALGERNON. What about your brother? What about the profligate Ernest?
JACK. Oh, before the end of the week I shall have got rid of him. I’ll say he died in Paris of apoplexy. Lots of people die of apoplexy, quite suddenly, don’t they?
ALGERNON. Yes, but it’s hereditary, my dear fellow. It’s a sort of thing that runs in families. You had much better say a severe chill.
JACK. You are sure a severe chill isn’t hereditary, or anything of that kind?
ALGERNON. Of course it isn’t!
JACK. Very well, then. My poor brother Ernest is carried off suddenly, in Paris, by a severe chill. That gets rid of him.
ALGERNON. But I thought you said that . . . Miss Cardew was a little too much interested in your poor brother Ernest? Won’t she feel his loss a good deal?
JACK. Oh, that is all right. Cecily is not a silly romantic girl, I am glad to say. She has got a capital appetite, goes long walks, and pays no attention at all to her lessons.
ALGERNON. I would rather like to see Cecily.
JACK. I will take very good care you never do. She is excessively pretty, and she is only just eighteen.
ALGERNON. Have you told Gwendolen yet that you have an excessively pretty ward who is only just eighteen?
JACK. Oh! one doesn’t blurt these things out to people. Cecily and Gwendolen are perfectly certain to be extremely great friends. I’ll bet you anything you like that half an hour after they have met, they will be calling each other sister.
ALGERNON. Women only do that when they have called each other a lot of other things first. Now, my dear boy, if we want to get a good table at Willis’s, we really must go and dress. Do you know it is nearly seven?
JACK. [Irritably.] Oh! It always is nearly seven.
ALGERNON. Well, I’m hungry.
JACK. I never knew you when you weren’t . . .
ALGERNON. What shall we do after dinner? Go to a theatre?
JACK. Oh no! I loathe listening.
ALGERNON. Well, let us go to the Club?
JACK. Oh, no! I hate talking.
ALGERNON. Well, we might trot round to the Empire at ten?
JACK. Oh, no! I can’t bear looking at things. It is so silly.
ALGERNON. Well, what shall we do?
JACK. Nothing!
ALGERNON. It is awfully hard work doing nothing. However, I don’t mind hard work where there is no definite object of any kind.
LANE. Miss Fairfax.
[Enter Gwendolen . Lane goes out.]
ALGERNON. Gwendolen, upon my word!
GWENDOLEN. Algy, kindly turn your back. I have something very particular to say to Mr. Worthing.
ALGERNON. Really, Gwendolen, I don’t think I can allow this at all.
GWENDOLEN. Algy, you always adopt a strictly immoral attitude towards life. You are not quite old enough to do that. [ Algernon retires to the fireplace.]
JACK. My own darling!
GWENDOLEN. Ernest, we may never be married. From the expression on mamma’s face I fear we never shall. Few parents nowadays pay any regard to what their children say to them. The old-fashioned respect for the young is fast dying out. Whatever influence I ever had over mamma, I lost at the age of three. But although she may prevent us from becoming man and wife, and I may marry some one else, and marry often, nothing that she can possibly do can alter my eternal devotion to you.
JACK. Dear Gwendolen!
GWENDOLEN. The story of your romantic origin, as related to me by mamma, with unpleasing comments, has naturally stirred the deeper fibres of my nature. Your Christian name has an irresistible fascination. The simplicity of your character makes you exquisitely incomprehensible to me. Your town address at the Albany I have. What is your address in the country?
JACK. The Manor House, Woolton, Hertfordshire.
[ Algernon , who has been carefully listening, smiles to himself, and writes the address on his shirt-cuff. Then picks up the Railway Guide.]
GWENDOLEN. There is a good postal service, I suppose? It may be necessary to do something desperate. That of course will require serious consideration. I will communicate with you daily.
JACK. My own one!
GWENDOLEN. How long do you remain in town?
JACK. Till Monday.
GWENDOLEN. Good! Algy, you may turn round now.
ALGERNON. Thanks, I’ve turned round already.
GWENDOLEN. You may also ring the bell.
JACK. You will let me see you to your carriage, my own darling?
GWENDOLEN. Certainly.
JACK. [To Lane , who now enters.] I will see Miss Fairfax out.
LANE. Yes, sir. [ Jack and Gwendolen go off.]
[ Lane presents several letters on a salver to Algernon . It is to be surmised that they are bills, as Algernon , after looking at the envelopes, tears them up.]
ALGERNON. A glass of sherry, Lane.
ALGERNON. To-morrow, Lane, I’m going Bunburying.
ALGERNON. I shall probably not be back till Monday. You can put up my dress clothes, my smoking jacket, and all the Bunbury suits . . .
LANE. Yes, sir. [Handing sherry.]
ALGERNON. I hope to-morrow will be a fine day, Lane.
LANE. It never is, sir.
ALGERNON. Lane, you’re a perfect pessimist.
LANE. I do my best to give satisfaction, sir.
[Enter Jack . Lane goes off.]
JACK. There’s a sensible, intellectual girl! the only girl I ever cared for in my life. [ Algernon is laughing immoderately.] What on earth are you so amused at?
ALGERNON. Oh, I’m a little anxious about poor Bunbury, that is all.
JACK. If you don’t take care, your friend Bunbury will get you into a serious scrape some day.
ALGERNON. I love scrapes. They are the only things that are never serious.
JACK. Oh, that’s nonsense, Algy. You never talk anything but nonsense.
ALGERNON. Nobody ever does.
[ Jack looks indignantly at him, and leaves the room. Algernon lights a cigarette, reads his shirt-cuff, and smiles.]
Garden at the Manor House. A flight of grey stone steps leads up to the house. The garden, an old-fashioned one, full of roses. Time of year, July. Basket chairs, and a table covered with books, are set under a large yew-tree.
[ Miss Prism discovered seated at the table. Cecily is at the back watering flowers.]
MISS PRISM. [Calling.] Cecily, Cecily! Surely such a utilitarian occupation as the watering of flowers is rather Moulton’s duty than yours? Especially at a moment when intellectual pleasures await you. Your German grammar is on the table. Pray open it at page fifteen. We will repeat yesterday’s lesson.
CECILY. [Coming over very slowly.] But I don’t like German. It isn’t at all a becoming language. I know perfectly well that I look quite plain after my German lesson.
MISS PRISM. Child, you know how anxious your guardian is that you should improve yourself in every way. He laid particular stress on your German, as he was leaving for town yesterday. Indeed, he always lays stress on your German when he is leaving for town.
CECILY. Dear Uncle Jack is so very serious! Sometimes he is so serious that I think he cannot be quite well.
MISS PRISM. [Drawing herself up.] Your guardian enjoys the best of health, and his gravity of demeanour is especially to be commended in one so comparatively young as he is. I know no one who has a higher sense of duty and responsibility.
CECILY. I suppose that is why he often looks a little bored when we three are together.
MISS PRISM. Cecily! I am surprised at you. Mr. Worthing has many troubles in his life. Idle merriment and triviality would be out of place in his conversation. You must remember his constant anxiety about that unfortunate young man his brother.
CECILY. I wish Uncle Jack would allow that unfortunate young man, his brother, to come down here sometimes. We might have a good influence over him, Miss Prism. I am sure you certainly would. You know German, and geology, and things of that kind influence a man very much. [ Cecily begins to write in her diary.]
MISS PRISM. [Shaking her head.] I do not think that even I could produce any effect on a character that according to his own brother’s admission is irretrievably weak and vacillating. Indeed I am not sure that I would desire to reclaim him. I am not in favour of this modern mania for turning bad people into good people at a moment’s notice. As a man sows so let him reap. You must put away your diary, Cecily. I really don’t see why you should keep a diary at all.
CECILY. I keep a diary in order to enter the wonderful secrets of my life. If I didn’t write them down, I should probably forget all about them.
MISS PRISM. Memory, my dear Cecily, is the diary that we all carry about with us.
CECILY. Yes, but it usually chronicles the things that have never happened, and couldn’t possibly have happened. I believe that Memory is responsible for nearly all the three-volume novels that Mudie sends us.
MISS PRISM. Do not speak slightingly of the three-volume novel, Cecily. I wrote one myself in earlier days.
CECILY. Did you really, Miss Prism? How wonderfully clever you are! I hope it did not end happily? I don’t like novels that end happily. They depress me so much.
MISS PRISM. The good ended happily, and the bad unhappily. That is what Fiction means.
CECILY. I suppose so. But it seems very unfair. And was your novel ever published?
MISS PRISM. Alas! no. The manuscript unfortunately was abandoned. [ Cecily starts.] I use the word in the sense of lost or mislaid. To your work, child, these speculations are profitless.
CECILY. [Smiling.] But I see dear Dr. Chasuble coming up through the garden.
MISS PRISM. [Rising and advancing.] Dr. Chasuble! This is indeed a pleasure.
[Enter Canon Chasuble .]
CHASUBLE. And how are we this morning? Miss Prism, you are, I trust, well?
CECILY. Miss Prism has just been complaining of a slight headache. I think it would do her so much good to have a short stroll with you in the Park, Dr. Chasuble.
MISS PRISM. Cecily, I have not mentioned anything about a headache.
CECILY. No, dear Miss Prism, I know that, but I felt instinctively that you had a headache. Indeed I was thinking about that, and not about my German lesson, when the Rector came in.
CHASUBLE. I hope, Cecily, you are not inattentive.
CECILY. Oh, I am afraid I am.
CHASUBLE. That is strange. Were I fortunate enough to be Miss Prism’s pupil, I would hang upon her lips. [ Miss Prism glares.] I spoke metaphorically.—My metaphor was drawn from bees. Ahem! Mr. Worthing, I suppose, has not returned from town yet?
MISS PRISM. We do not expect him till Monday afternoon.
CHASUBLE. Ah yes, he usually likes to spend his Sunday in London. He is not one of those whose sole aim is enjoyment, as, by all accounts, that unfortunate young man his brother seems to be. But I must not disturb Egeria and her pupil any longer.
MISS PRISM. Egeria? My name is Lætitia, Doctor.
CHASUBLE. [Bowing.] A classical allusion merely, drawn from the Pagan authors. I shall see you both no doubt at Evensong?
MISS PRISM. I think, dear Doctor, I will have a stroll with you. I find I have a headache after all, and a walk might do it good.
CHASUBLE. With pleasure, Miss Prism, with pleasure. We might go as far as the schools and back.
MISS PRISM. That would be delightful. Cecily, you will read your Political Economy in my absence. The chapter on the Fall of the Rupee you may omit. It is somewhat too sensational. Even these metallic problems have their melodramatic side.
[Goes down the garden with Dr. Chasuble .]
CECILY. [Picks up books and throws them back on table.] Horrid Political Economy! Horrid Geography! Horrid, horrid German!
[Enter Merriman with a card on a salver.]
MERRIMAN. Mr. Ernest Worthing has just driven over from the station. He has brought his luggage with him.
CECILY. [Takes the card and reads it.] ‘Mr. Ernest Worthing, B. 4, The Albany, W.’ Uncle Jack’s brother! Did you tell him Mr. Worthing was in town?
MERRIMAN. Yes, Miss. He seemed very much disappointed. I mentioned that you and Miss Prism were in the garden. He said he was anxious to speak to you privately for a moment.
CECILY. Ask Mr. Ernest Worthing to come here. I suppose you had better talk to the housekeeper about a room for him.
MERRIMAN. Yes, Miss.
[ Merriman goes off.]
CECILY. I have never met any really wicked person before. I feel rather frightened. I am so afraid he will look just like every one else.
[Enter Algernon , very gay and debonnair.] He does!
ALGERNON. [Raising his hat.] You are my little cousin Cecily, I’m sure.
CECILY. You are under some strange mistake. I am not little. In fact, I believe I am more than usually tall for my age. [ Algernon is rather taken aback.] But I am your cousin Cecily. You, I see from your card, are Uncle Jack’s brother, my cousin Ernest, my wicked cousin Ernest.
ALGERNON. Oh! I am not really wicked at all, cousin Cecily. You mustn’t think that I am wicked.
CECILY. If you are not, then you have certainly been deceiving us all in a very inexcusable manner. I hope you have not been leading a double life, pretending to be wicked and being really good all the time. That would be hypocrisy.
ALGERNON. [Looks at her in amazement.] Oh! Of course I have been rather reckless.
CECILY. I am glad to hear it.
ALGERNON. In fact, now you mention the subject, I have been very bad in my own small way.
CECILY. I don’t think you should be so proud of that, though I am sure it must have been very pleasant.
ALGERNON. It is much pleasanter being here with you.
CECILY. I can’t understand how you are here at all. Uncle Jack won’t be back till Monday afternoon.
ALGERNON. That is a great disappointment. I am obliged to go up by the first train on Monday morning. I have a business appointment that I am anxious . . . to miss?
CECILY. Couldn’t you miss it anywhere but in London?
ALGERNON. No: the appointment is in London.
CECILY. Well, I know, of course, how important it is not to keep a business engagement, if one wants to retain any sense of the beauty of life, but still I think you had better wait till Uncle Jack arrives. I know he wants to speak to you about your emigrating.
ALGERNON. About my what?
CECILY. Your emigrating. He has gone up to buy your outfit.
ALGERNON. I certainly wouldn’t let Jack buy my outfit. He has no taste in neckties at all.
CECILY. I don’t think you will require neckties. Uncle Jack is sending you to Australia.
ALGERNON. Australia! I’d sooner die.
CECILY. Well, he said at dinner on Wednesday night, that you would have to choose between this world, the next world, and Australia.
ALGERNON. Oh, well! The accounts I have received of Australia and the next world, are not particularly encouraging. This world is good enough for me, cousin Cecily.
CECILY. Yes, but are you good enough for it?
ALGERNON. I’m afraid I’m not that. That is why I want you to reform me. You might make that your mission, if you don’t mind, cousin Cecily.
CECILY. I’m afraid I’ve no time, this afternoon.
ALGERNON. Well, would you mind my reforming myself this afternoon?
CECILY. It is rather Quixotic of you. But I think you should try.
ALGERNON. I will. I feel better already.
CECILY. You are looking a little worse.
ALGERNON. That is because I am hungry.
CECILY. How thoughtless of me. I should have remembered that when one is going to lead an entirely new life, one requires regular and wholesome meals. Won’t you come in?
ALGERNON. Thank you. Might I have a buttonhole first? I never have any appetite unless I have a buttonhole first.
CECILY. A Marechal Niel? [Picks up scissors.]
ALGERNON. No, I’d sooner have a pink rose.
CECILY. Why? [Cuts a flower.]
ALGERNON. Because you are like a pink rose, Cousin Cecily.
CECILY. I don’t think it can be right for you to talk to me like that. Miss Prism never says such things to me.
ALGERNON. Then Miss Prism is a short-sighted old lady. [ Cecily puts the rose in his buttonhole.] You are the prettiest girl I ever saw.
CECILY. Miss Prism says that all good looks are a snare.
ALGERNON. They are a snare that every sensible man would like to be caught in.
CECILY. Oh, I don’t think I would care to catch a sensible man. I shouldn’t know what to talk to him about.
[They pass into the house. Miss Prism and Dr. Chasuble return.]
MISS PRISM. You are too much alone, dear Dr. Chasuble. You should get married. A misanthrope I can understand—a womanthrope, never!
CHASUBLE. [With a scholar’s shudder.] Believe me, I do not deserve so neologistic a phrase. The precept as well as the practice of the Primitive Church was distinctly against matrimony.
MISS PRISM. [Sententiously.] That is obviously the reason why the Primitive Church has not lasted up to the present day. And you do not seem to realise, dear Doctor, that by persistently remaining single, a man converts himself into a permanent public temptation. Men should be more careful; this very celibacy leads weaker vessels astray.
CHASUBLE. But is a man not equally attractive when married?
MISS PRISM. No married man is ever attractive except to his wife.
CHASUBLE. And often, I’ve been told, not even to her.
MISS PRISM. That depends on the intellectual sympathies of the woman. Maturity can always be depended on. Ripeness can be trusted. Young women are green. [ Dr. Chasuble starts.] I spoke horticulturally. My metaphor was drawn from fruits. But where is Cecily?
CHASUBLE. Perhaps she followed us to the schools.
[Enter Jack slowly from the back of the garden. He is dressed in the deepest mourning, with crape hatband and black gloves.]
MISS PRISM. Mr. Worthing!
CHASUBLE. Mr. Worthing?
MISS PRISM. This is indeed a surprise. We did not look for you till Monday afternoon.
JACK. [Shakes Miss Prism’s hand in a tragic manner.] I have returned sooner than I expected. Dr. Chasuble, I hope you are well?
CHASUBLE. Dear Mr. Worthing, I trust this garb of woe does not betoken some terrible calamity?
JACK. My brother.
MISS PRISM. More shameful debts and extravagance?
CHASUBLE. Still leading his life of pleasure?
JACK. [Shaking his head.] Dead!
CHASUBLE. Your brother Ernest dead?
JACK. Quite dead.
MISS PRISM. What a lesson for him! I trust he will profit by it.
CHASUBLE. Mr. Worthing, I offer you my sincere condolence. You have at least the consolation of knowing that you were always the most generous and forgiving of brothers.
JACK. Poor Ernest! He had many faults, but it is a sad, sad blow.
CHASUBLE. Very sad indeed. Were you with him at the end?
JACK. No. He died abroad; in Paris, in fact. I had a telegram last night from the manager of the Grand Hotel.
CHASUBLE. Was the cause of death mentioned?
JACK. A severe chill, it seems.
MISS PRISM. As a man sows, so shall he reap.
CHASUBLE. [Raising his hand.] Charity, dear Miss Prism, charity! None of us are perfect. I myself am peculiarly susceptible to draughts. Will the interment take place here?
JACK. No. He seems to have expressed a desire to be buried in Paris.
CHASUBLE. In Paris! [Shakes his head.] I fear that hardly points to any very serious state of mind at the last. You would no doubt wish me to make some slight allusion to this tragic domestic affliction next Sunday. [ Jack presses his hand convulsively.] My sermon on the meaning of the manna in the wilderness can be adapted to almost any occasion, joyful, or, as in the present case, distressing. [All sigh.] I have preached it at harvest celebrations, christenings, confirmations, on days of humiliation and festal days. The last time I delivered it was in the Cathedral, as a charity sermon on behalf of the Society for the Prevention of Discontent among the Upper Orders. The Bishop, who was present, was much struck by some of the analogies I drew.
JACK. Ah! that reminds me, you mentioned christenings I think, Dr. Chasuble? I suppose you know how to christen all right? [ Dr. Chasuble looks astounded.] I mean, of course, you are continually christening, aren’t you?
MISS PRISM. It is, I regret to say, one of the Rector’s most constant duties in this parish. I have often spoken to the poorer classes on the subject. But they don’t seem to know what thrift is.
CHASUBLE. But is there any particular infant in whom you are interested, Mr. Worthing? Your brother was, I believe, unmarried, was he not?
JACK. Oh yes.
MISS PRISM. [Bitterly.] People who live entirely for pleasure usually are.
JACK. But it is not for any child, dear Doctor. I am very fond of children. No! the fact is, I would like to be christened myself, this afternoon, if you have nothing better to do.
CHASUBLE. But surely, Mr. Worthing, you have been christened already?
JACK. I don’t remember anything about it.
CHASUBLE. But have you any grave doubts on the subject?
JACK. I certainly intend to have. Of course I don’t know if the thing would bother you in any way, or if you think I am a little too old now.
CHASUBLE. Not at all. The sprinkling, and, indeed, the immersion of adults is a perfectly canonical practice.
JACK. Immersion!
CHASUBLE. You need have no apprehensions. Sprinkling is all that is necessary, or indeed I think advisable. Our weather is so changeable. At what hour would you wish the ceremony performed?
JACK. Oh, I might trot round about five if that would suit you.
CHASUBLE. Perfectly, perfectly! In fact I have two similar ceremonies to perform at that time. A case of twins that occurred recently in one of the outlying cottages on your own estate. Poor Jenkins the carter, a most hard-working man.
JACK. Oh! I don’t see much fun in being christened along with other babies. It would be childish. Would half-past five do?
CHASUBLE. Admirably! Admirably! [Takes out watch.] And now, dear Mr. Worthing, I will not intrude any longer into a house of sorrow. I would merely beg you not to be too much bowed down by grief. What seem to us bitter trials are often blessings in disguise.
MISS PRISM. This seems to me a blessing of an extremely obvious kind.
[Enter Cecily from the house.]
CECILY. Uncle Jack! Oh, I am pleased to see you back. But what horrid clothes you have got on! Do go and change them.
MISS PRISM. Cecily!
CHASUBLE. My child! my child! [ Cecily goes towards Jack ; he kisses her brow in a melancholy manner.]
CECILY. What is the matter, Uncle Jack? Do look happy! You look as if you had toothache, and I have got such a surprise for you. Who do you think is in the dining-room? Your brother!
CECILY. Your brother Ernest. He arrived about half an hour ago.
JACK. What nonsense! I haven’t got a brother.
CECILY. Oh, don’t say that. However badly he may have behaved to you in the past he is still your brother. You couldn’t be so heartless as to disown him. I’ll tell him to come out. And you will shake hands with him, won’t you, Uncle Jack? [Runs back into the house.]
CHASUBLE. These are very joyful tidings.
MISS PRISM. After we had all been resigned to his loss, his sudden return seems to me peculiarly distressing.
JACK. My brother is in the dining-room? I don’t know what it all means. I think it is perfectly absurd.
[Enter Algernon and Cecily hand in hand. They come slowly up to Jack .]
JACK. Good heavens! [Motions Algernon away.]
ALGERNON. Brother John, I have come down from town to tell you that I am very sorry for all the trouble I have given you, and that I intend to lead a better life in the future. [ Jack glares at him and does not take his hand.]
CECILY. Uncle Jack, you are not going to refuse your own brother’s hand?
JACK. Nothing will induce me to take his hand. I think his coming down here disgraceful. He knows perfectly well why.
CECILY. Uncle Jack, do be nice. There is some good in every one. Ernest has just been telling me about his poor invalid friend Mr. Bunbury whom he goes to visit so often. And surely there must be much good in one who is kind to an invalid, and leaves the pleasures of London to sit by a bed of pain.
JACK. Oh! he has been talking about Bunbury, has he?
CECILY. Yes, he has told me all about poor Mr. Bunbury, and his terrible state of health.
JACK. Bunbury! Well, I won’t have him talk to you about Bunbury or about anything else. It is enough to drive one perfectly frantic.
ALGERNON. Of course I admit that the faults were all on my side. But I must say that I think that Brother John’s coldness to me is peculiarly painful. I expected a more enthusiastic welcome, especially considering it is the first time I have come here.
CECILY. Uncle Jack, if you don’t shake hands with Ernest I will never forgive you.
JACK. Never forgive me?
CECILY. Never, never, never!
JACK. Well, this is the last time I shall ever do it. [Shakes with Algernon and glares.]
CHASUBLE. It’s pleasant, is it not, to see so perfect a reconciliation? I think we might leave the two brothers together.
MISS PRISM. Cecily, you will come with us.
CECILY. Certainly, Miss Prism. My little task of reconciliation is over.
CHASUBLE. You have done a beautiful action to-day, dear child.
MISS PRISM. We must not be premature in our judgments.
CECILY. I feel very happy. [They all go off except Jack and Algernon .]
JACK. You young scoundrel, Algy, you must get out of this place as soon as possible. I don’t allow any Bunburying here.
[Enter Merriman .]
MERRIMAN. I have put Mr. Ernest’s things in the room next to yours, sir. I suppose that is all right?
JACK. What?
MERRIMAN. Mr. Ernest’s luggage, sir. I have unpacked it and put it in the room next to your own.
JACK. His luggage?
MERRIMAN. Yes, sir. Three portmanteaus, a dressing-case, two hat-boxes, and a large luncheon-basket.
ALGERNON. I am afraid I can’t stay more than a week this time.
JACK. Merriman, order the dog-cart at once. Mr. Ernest has been suddenly called back to town.
MERRIMAN. Yes, sir. [Goes back into the house.]
ALGERNON. What a fearful liar you are, Jack. I have not been called back to town at all.
JACK. Yes, you have.
ALGERNON. I haven’t heard any one call me.
JACK. Your duty as a gentleman calls you back.
ALGERNON. My duty as a gentleman has never interfered with my pleasures in the smallest degree.
JACK. I can quite understand that.
ALGERNON. Well, Cecily is a darling.
JACK. You are not to talk of Miss Cardew like that. I don’t like it.
ALGERNON. Well, I don’t like your clothes. You look perfectly ridiculous in them. Why on earth don’t you go up and change? It is perfectly childish to be in deep mourning for a man who is actually staying for a whole week with you in your house as a guest. I call it grotesque.
JACK. You are certainly not staying with me for a whole week as a guest or anything else. You have got to leave . . . by the four-five train.
ALGERNON. I certainly won’t leave you so long as you are in mourning. It would be most unfriendly. If I were in mourning you would stay with me, I suppose. I should think it very unkind if you didn’t.
JACK. Well, will you go if I change my clothes?
ALGERNON. Yes, if you are not too long. I never saw anybody take so long to dress, and with such little result.
JACK. Well, at any rate, that is better than being always over-dressed as you are.
ALGERNON. If I am occasionally a little over-dressed, I make up for it by being always immensely over-educated.
JACK. Your vanity is ridiculous, your conduct an outrage, and your presence in my garden utterly absurd. However, you have got to catch the four-five, and I hope you will have a pleasant journey back to town. This Bunburying, as you call it, has not been a great success for you.
[Goes into the house.]
ALGERNON. I think it has been a great success. I’m in love with Cecily, and that is everything.
[Enter Cecily at the back of the garden. She picks up the can and begins to water the flowers.] But I must see her before I go, and make arrangements for another Bunbury. Ah, there she is.
CECILY. Oh, I merely came back to water the roses. I thought you were with Uncle Jack.
ALGERNON. He’s gone to order the dog-cart for me.
CECILY. Oh, is he going to take you for a nice drive?
ALGERNON. He’s going to send me away.
CECILY. Then have we got to part?
ALGERNON. I am afraid so. It’s a very painful parting.
CECILY. It is always painful to part from people whom one has known for a very brief space of time. The absence of old friends one can endure with equanimity. But even a momentary separation from anyone to whom one has just been introduced is almost unbearable.
ALGERNON. Thank you.
MERRIMAN. The dog-cart is at the door, sir. [ Algernon looks appealingly at Cecily .]
CECILY. It can wait, Merriman for . . . five minutes.
MERRIMAN. Yes, Miss. [Exit Merriman .]
ALGERNON. I hope, Cecily, I shall not offend you if I state quite frankly and openly that you seem to me to be in every way the visible personification of absolute perfection.
CECILY. I think your frankness does you great credit, Ernest. If you will allow me, I will copy your remarks into my diary. [Goes over to table and begins writing in diary.]
ALGERNON. Do you really keep a diary? I’d give anything to look at it. May I?
CECILY. Oh no. [Puts her hand over it.] You see, it is simply a very young girl’s record of her own thoughts and impressions, and consequently meant for publication. When it appears in volume form I hope you will order a copy. But pray, Ernest, don’t stop. I delight in taking down from dictation. I have reached ‘absolute perfection’. You can go on. I am quite ready for more.
ALGERNON. [Somewhat taken aback.] Ahem! Ahem!
CECILY. Oh, don’t cough, Ernest. When one is dictating one should speak fluently and not cough. Besides, I don’t know how to spell a cough. [Writes as Algernon speaks.]
ALGERNON. [Speaking very rapidly.] Cecily, ever since I first looked upon your wonderful and incomparable beauty, I have dared to love you wildly, passionately, devotedly, hopelessly.
CECILY. I don’t think that you should tell me that you love me wildly, passionately, devotedly, hopelessly. Hopelessly doesn’t seem to make much sense, does it?
ALGERNON. Cecily!
MERRIMAN. The dog-cart is waiting, sir.
ALGERNON. Tell it to come round next week, at the same hour.
MERRIMAN. [Looks at Cecily , who makes no sign.] Yes, sir.
[ Merriman retires.]
CECILY. Uncle Jack would be very much annoyed if he knew you were staying on till next week, at the same hour.
ALGERNON. Oh, I don’t care about Jack. I don’t care for anybody in the whole world but you. I love you, Cecily. You will marry me, won’t you?
CECILY. You silly boy! Of course. Why, we have been engaged for the last three months.
ALGERNON. For the last three months?
CECILY. Yes, it will be exactly three months on Thursday.
ALGERNON. But how did we become engaged?
CECILY. Well, ever since dear Uncle Jack first confessed to us that he had a younger brother who was very wicked and bad, you of course have formed the chief topic of conversation between myself and Miss Prism. And of course a man who is much talked about is always very attractive. One feels there must be something in him, after all. I daresay it was foolish of me, but I fell in love with you, Ernest.
ALGERNON. Darling! And when was the engagement actually settled?
CECILY. On the 14th of February last. Worn out by your entire ignorance of my existence, I determined to end the matter one way or the other, and after a long struggle with myself I accepted you under this dear old tree here. The next day I bought this little ring in your name, and this is the little bangle with the true lover’s knot I promised you always to wear.
ALGERNON. Did I give you this? It’s very pretty, isn’t it?
CECILY. Yes, you’ve wonderfully good taste, Ernest. It’s the excuse I’ve always given for your leading such a bad life. And this is the box in which I keep all your dear letters. [Kneels at table, opens box, and produces letters tied up with blue ribbon.]
ALGERNON. My letters! But, my own sweet Cecily, I have never written you any letters.
CECILY. You need hardly remind me of that, Ernest. I remember only too well that I was forced to write your letters for you. I wrote always three times a week, and sometimes oftener.
ALGERNON. Oh, do let me read them, Cecily?
CECILY. Oh, I couldn’t possibly. They would make you far too conceited. [Replaces box.] The three you wrote me after I had broken off the engagement are so beautiful, and so badly spelled, that even now I can hardly read them without crying a little.
ALGERNON. But was our engagement ever broken off?
CECILY. Of course it was. On the 22nd of last March. You can see the entry if you like. [Shows diary.] ‘To-day I broke off my engagement with Ernest. I feel it is better to do so. The weather still continues charming.’
ALGERNON. But why on earth did you break it off? What had I done? I had done nothing at all. Cecily, I am very much hurt indeed to hear you broke it off. Particularly when the weather was so charming.
CECILY. It would hardly have been a really serious engagement if it hadn’t been broken off at least once. But I forgave you before the week was out.
ALGERNON. [Crossing to her, and kneeling.] What a perfect angel you are, Cecily.
CECILY. You dear romantic boy. [He kisses her, she puts her fingers through his hair.] I hope your hair curls naturally, does it?
ALGERNON. Yes, darling, with a little help from others.
CECILY. I am so glad.
ALGERNON. You’ll never break off our engagement again, Cecily?
CECILY. I don’t think I could break it off now that I have actually met you. Besides, of course, there is the question of your name.
ALGERNON. Yes, of course. [Nervously.]
CECILY. You must not laugh at me, darling, but it had always been a girlish dream of mine to love some one whose name was Ernest. [ Algernon rises, Cecily also.] There is something in that name that seems to inspire absolute confidence. I pity any poor married woman whose husband is not called Ernest.
ALGERNON. But, my dear child, do you mean to say you could not love me if I had some other name?
CECILY. But what name?
ALGERNON. Oh, any name you like—Algernon—for instance . . .
CECILY. But I don’t like the name of Algernon.
ALGERNON. Well, my own dear, sweet, loving little darling, I really can’t see why you should object to the name of Algernon. It is not at all a bad name. In fact, it is rather an aristocratic name. Half of the chaps who get into the Bankruptcy Court are called Algernon. But seriously, Cecily . . . [Moving to her] . . . if my name was Algy, couldn’t you love me?
CECILY. [Rising.] I might respect you, Ernest, I might admire your character, but I fear that I should not be able to give you my undivided attention.
ALGERNON. Ahem! Cecily! [Picking up hat.] Your Rector here is, I suppose, thoroughly experienced in the practice of all the rites and ceremonials of the Church?
CECILY. Oh, yes. Dr. Chasuble is a most learned man. He has never written a single book, so you can imagine how much he knows.
ALGERNON. I must see him at once on a most important christening—I mean on most important business.
CECILY. Oh!
ALGERNON. I shan’t be away more than half an hour.
CECILY. Considering that we have been engaged since February the 14th, and that I only met you to-day for the first time, I think it is rather hard that you should leave me for so long a period as half an hour. Couldn’t you make it twenty minutes?
ALGERNON. I’ll be back in no time.
[Kisses her and rushes down the garden.]
CECILY. What an impetuous boy he is! I like his hair so much. I must enter his proposal in my diary.
MERRIMAN. A Miss Fairfax has just called to see Mr. Worthing. On very important business, Miss Fairfax states.
CECILY. Isn’t Mr. Worthing in his library?
MERRIMAN. Mr. Worthing went over in the direction of the Rectory some time ago.
CECILY. Pray ask the lady to come out here; Mr. Worthing is sure to be back soon. And you can bring tea.
MERRIMAN. Yes, Miss. [Goes out.]
CECILY. Miss Fairfax! I suppose one of the many good elderly women who are associated with Uncle Jack in some of his philanthropic work in London. I don’t quite like women who are interested in philanthropic work. I think it is so forward of them.
MERRIMAN. Miss Fairfax.
[Enter Gwendolen .]
[Exit Merriman .]
CECILY. [Advancing to meet her.] Pray let me introduce myself to you. My name is Cecily Cardew.
GWENDOLEN. Cecily Cardew? [Moving to her and shaking hands.] What a very sweet name! Something tells me that we are going to be great friends. I like you already more than I can say. My first impressions of people are never wrong.
CECILY. How nice of you to like me so much after we have known each other such a comparatively short time. Pray sit down.
GWENDOLEN. [Still standing up.] I may call you Cecily, may I not?
CECILY. With pleasure!
GWENDOLEN. And you will always call me Gwendolen, won’t you?
CECILY. If you wish.
GWENDOLEN. Then that is all quite settled, is it not?
CECILY. I hope so. [A pause. They both sit down together.]
GWENDOLEN. Perhaps this might be a favourable opportunity for my mentioning who I am. My father is Lord Bracknell. You have never heard of papa, I suppose?
CECILY. I don’t think so.
GWENDOLEN. Outside the family circle, papa, I am glad to say, is entirely unknown. I think that is quite as it should be. The home seems to me to be the proper sphere for the man. And certainly once a man begins to neglect his domestic duties he becomes painfully effeminate, does he not? And I don’t like that. It makes men so very attractive. Cecily, mamma, whose views on education are remarkably strict, has brought me up to be extremely short-sighted; it is part of her system; so do you mind my looking at you through my glasses?
CECILY. Oh! not at all, Gwendolen. I am very fond of being looked at.
GWENDOLEN. [After examining Cecily carefully through a lorgnette.] You are here on a short visit, I suppose.
CECILY. Oh no! I live here.
GWENDOLEN. [Severely.] Really? Your mother, no doubt, or some female relative of advanced years, resides here also?
CECILY. Oh no! I have no mother, nor, in fact, any relations.
GWENDOLEN. Indeed?
CECILY. My dear guardian, with the assistance of Miss Prism, has the arduous task of looking after me.
GWENDOLEN. Your guardian?
CECILY. Yes, I am Mr. Worthing’s ward.
GWENDOLEN. Oh! It is strange he never mentioned to me that he had a ward. How secretive of him! He grows more interesting hourly. I am not sure, however, that the news inspires me with feelings of unmixed delight. [Rising and going to her.] I am very fond of you, Cecily; I have liked you ever since I met you! But I am bound to state that now that I know that you are Mr. Worthing’s ward, I cannot help expressing a wish you were—well, just a little older than you seem to be—and not quite so very alluring in appearance. In fact, if I may speak candidly—
CECILY. Pray do! I think that whenever one has anything unpleasant to say, one should always be quite candid.
GWENDOLEN. Well, to speak with perfect candour, Cecily, I wish that you were fully forty-two, and more than usually plain for your age. Ernest has a strong upright nature. He is the very soul of truth and honour. Disloyalty would be as impossible to him as deception. But even men of the noblest possible moral character are extremely susceptible to the influence of the physical charms of others. Modern, no less than Ancient History, supplies us with many most painful examples of what I refer to. If it were not so, indeed, History would be quite unreadable.
CECILY. I beg your pardon, Gwendolen, did you say Ernest?
GWENDOLEN. Yes.
CECILY. Oh, but it is not Mr. Ernest Worthing who is my guardian. It is his brother—his elder brother.
GWENDOLEN. [Sitting down again.] Ernest never mentioned to me that he had a brother.
CECILY. I am sorry to say they have not been on good terms for a long time.
GWENDOLEN. Ah! that accounts for it. And now that I think of it I have never heard any man mention his brother. The subject seems distasteful to most men. Cecily, you have lifted a load from my mind. I was growing almost anxious. It would have been terrible if any cloud had come across a friendship like ours, would it not? Of course you are quite, quite sure that it is not Mr. Ernest Worthing who is your guardian?
CECILY. Quite sure. [A pause.] In fact, I am going to be his.
GWENDOLEN. [Inquiringly.] I beg your pardon?
CECILY. [Rather shy and confidingly.] Dearest Gwendolen, there is no reason why I should make a secret of it to you. Our little county newspaper is sure to chronicle the fact next week. Mr. Ernest Worthing and I are engaged to be married.
GWENDOLEN. [Quite politely, rising.] My darling Cecily, I think there must be some slight error. Mr. Ernest Worthing is engaged to me. The announcement will appear in the Morning Post on Saturday at the latest.
CECILY. [Very politely, rising.] I am afraid you must be under some misconception. Ernest proposed to me exactly ten minutes ago. [Shows diary.]
GWENDOLEN. [Examines diary through her lorgnettte carefully.] It is certainly very curious, for he asked me to be his wife yesterday afternoon at 5.30. If you would care to verify the incident, pray do so. [Produces diary of her own.] I never travel without my diary. One should always have something sensational to read in the train. I am so sorry, dear Cecily, if it is any disappointment to you, but I am afraid I have the prior claim.
CECILY. It would distress me more than I can tell you, dear Gwendolen, if it caused you any mental or physical anguish, but I feel bound to point out that since Ernest proposed to you he clearly has changed his mind.
GWENDOLEN. [Meditatively.] If the poor fellow has been entrapped into any foolish promise I shall consider it my duty to rescue him at once, and with a firm hand.
CECILY. [Thoughtfully and sadly.] Whatever unfortunate entanglement my dear boy may have got into, I will never reproach him with it after we are married.
GWENDOLEN. Do you allude to me, Miss Cardew, as an entanglement? You are presumptuous. On an occasion of this kind it becomes more than a moral duty to speak one’s mind. It becomes a pleasure.
CECILY. Do you suggest, Miss Fairfax, that I entrapped Ernest into an engagement? How dare you? This is no time for wearing the shallow mask of manners. When I see a spade I call it a spade.
GWENDOLEN. [Satirically.] I am glad to say that I have never seen a spade. It is obvious that our social spheres have been widely different.
[Enter Merriman , followed by the footman. He carries a salver, table cloth, and plate stand. Cecily is about to retort. The presence of the servants exercises a restraining influence, under which both girls chafe.]
MERRIMAN. Shall I lay tea here as usual, Miss?
CECILY. [Sternly, in a calm voice.] Yes, as usual. [ Merriman begins to clear table and lay cloth. A long pause. Cecily and Gwendolen glare at each other.]
GWENDOLEN. Are there many interesting walks in the vicinity, Miss Cardew?
CECILY. Oh! yes! a great many. From the top of one of the hills quite close one can see five counties.
GWENDOLEN. Five counties! I don’t think I should like that; I hate crowds.
CECILY. [Sweetly.] I suppose that is why you live in town? [ Gwendolen bites her lip, and beats her foot nervously with her parasol.]
GWENDOLEN. [Looking round.] Quite a well-kept garden this is, Miss Cardew.
CECILY. So glad you like it, Miss Fairfax.
GWENDOLEN. I had no idea there were any flowers in the country.
CECILY. Oh, flowers are as common here, Miss Fairfax, as people are in London.
GWENDOLEN. Personally I cannot understand how anybody manages to exist in the country, if anybody who is anybody does. The country always bores me to death.
CECILY. Ah! This is what the newspapers call agricultural depression, is it not? I believe the aristocracy are suffering very much from it just at present. It is almost an epidemic amongst them, I have been told. May I offer you some tea, Miss Fairfax?
GWENDOLEN. [With elaborate politeness.] Thank you. [Aside.] Detestable girl! But I require tea!
CECILY. [Sweetly.] Sugar?
GWENDOLEN. [Superciliously.] No, thank you. Sugar is not fashionable any more. [ Cecily looks angrily at her, takes up the tongs and puts four lumps of sugar into the cup.]
CECILY. [Severely.] Cake or bread and butter?
GWENDOLEN. [In a bored manner.] Bread and butter, please. Cake is rarely seen at the best houses nowadays.
CECILY. [Cuts a very large slice of cake, and puts it on the tray.] Hand that to Miss Fairfax.
[ Merriman does so, and goes out with footman. Gwendolen drinks the tea and makes a grimace. Puts down cup at once, reaches out her hand to the bread and butter, looks at it, and finds it is cake. Rises in indignation.]
GWENDOLEN. You have filled my tea with lumps of sugar, and though I asked most distinctly for bread and butter, you have given me cake. I am known for the gentleness of my disposition, and the extraordinary sweetness of my nature, but I warn you, Miss Cardew, you may go too far.
CECILY. [Rising.] To save my poor, innocent, trusting boy from the machinations of any other girl there are no lengths to which I would not go.
GWENDOLEN. From the moment I saw you I distrusted you. I felt that you were false and deceitful. I am never deceived in such matters. My first impressions of people are invariably right.
CECILY. It seems to me, Miss Fairfax, that I am trespassing on your valuable time. No doubt you have many other calls of a similar character to make in the neighbourhood.
GWENDOLEN. [Catching sight of him.] Ernest! My own Ernest!
JACK. Gwendolen! Darling! [Offers to kiss her.]
GWENDOLEN. [Draws back.] A moment! May I ask if you are engaged to be married to this young lady? [Points to Cecily .]
JACK. [Laughing.] To dear little Cecily! Of course not! What could have put such an idea into your pretty little head?
GWENDOLEN. Thank you. You may! [Offers her cheek.]
CECILY. [Very sweetly.] I knew there must be some misunderstanding, Miss Fairfax. The gentleman whose arm is at present round your waist is my guardian, Mr. John Worthing.
GWENDOLEN. I beg your pardon?
CECILY. This is Uncle Jack.
GWENDOLEN. [Receding.] Jack! Oh!
[Enter Algernon .]
CECILY. Here is Ernest.
ALGERNON. [Goes straight over to Cecily without noticing any one else.] My own love! [Offers to kiss her.]
CECILY. [Drawing back.] A moment, Ernest! May I ask you—are you engaged to be married to this young lady?
ALGERNON. [Looking round.] To what young lady? Good heavens! Gwendolen!
CECILY. Yes! to good heavens, Gwendolen, I mean to Gwendolen.
ALGERNON. [Laughing.] Of course not! What could have put such an idea into your pretty little head?
CECILY. Thank you. [Presenting her cheek to be kissed.] You may. [ Algernon kisses her.]
GWENDOLEN. I felt there was some slight error, Miss Cardew. The gentleman who is now embracing you is my cousin, Mr. Algernon Moncrieff.
CECILY. [Breaking away from Algernon .] Algernon Moncrieff! Oh! [The two girls move towards each other and put their arms round each other’s waists as if for protection.]
CECILY. Are you called Algernon?
ALGERNON. I cannot deny it.
GWENDOLEN. Is your name really John?
JACK. [Standing rather proudly.] I could deny it if I liked. I could deny anything if I liked. But my name certainly is John. It has been John for years.
CECILY. [To Gwendolen .] A gross deception has been practised on both of us.
GWENDOLEN. My poor wounded Cecily!
CECILY. My sweet wronged Gwendolen!
GWENDOLEN. [Slowly and seriously.] You will call me sister, will you not? [They embrace. Jack and Algernon groan and walk up and down.]
CECILY. [Rather brightly.] There is just one question I would like to be allowed to ask my guardian.
GWENDOLEN. An admirable idea! Mr. Worthing, there is just one question I would like to be permitted to put to you. Where is your brother Ernest? We are both engaged to be married to your brother Ernest, so it is a matter of some importance to us to know where your brother Ernest is at present.
JACK. [Slowly and hesitatingly.] Gwendolen—Cecily—it is very painful for me to be forced to speak the truth. It is the first time in my life that I have ever been reduced to such a painful position, and I am really quite inexperienced in doing anything of the kind. However, I will tell you quite frankly that I have no brother Ernest. I have no brother at all. I never had a brother in my life, and I certainly have not the smallest intention of ever having one in the future.
CECILY. [Surprised.] No brother at all?
JACK. [Cheerily.] None!
GWENDOLEN. [Severely.] Had you never a brother of any kind?
JACK. [Pleasantly.] Never. Not even of any kind.
GWENDOLEN. I am afraid it is quite clear, Cecily, that neither of us is engaged to be married to any one.
CECILY. It is not a very pleasant position for a young girl suddenly to find herself in. Is it?
GWENDOLEN. Let us go into the house. They will hardly venture to come after us there.
CECILY. No, men are so cowardly, aren’t they?
[They retire into the house with scornful looks.]
JACK. This ghastly state of things is what you call Bunburying, I suppose?
ALGERNON. Yes, and a perfectly wonderful Bunbury it is. The most wonderful Bunbury I have ever had in my life.
JACK. Well, you’ve no right whatsoever to Bunbury here.
ALGERNON. That is absurd. One has a right to Bunbury anywhere one chooses. Every serious Bunburyist knows that.
JACK. Serious Bunburyist! Good heavens!
ALGERNON. Well, one must be serious about something, if one wants to have any amusement in life. I happen to be serious about Bunburying. What on earth you are serious about I haven’t got the remotest idea. About everything, I should fancy. You have such an absolutely trivial nature.
JACK. Well, the only small satisfaction I have in the whole of this wretched business is that your friend Bunbury is quite exploded. You won’t be able to run down to the country quite so often as you used to do, dear Algy. And a very good thing too.
ALGERNON. Your brother is a little off colour, isn’t he, dear Jack? You won’t be able to disappear to London quite so frequently as your wicked custom was. And not a bad thing either.
JACK. As for your conduct towards Miss Cardew, I must say that your taking in a sweet, simple, innocent girl like that is quite inexcusable. To say nothing of the fact that she is my ward.
ALGERNON. I can see no possible defence at all for your deceiving a brilliant, clever, thoroughly experienced young lady like Miss Fairfax. To say nothing of the fact that she is my cousin.
JACK. I wanted to be engaged to Gwendolen, that is all. I love her.
ALGERNON. Well, I simply wanted to be engaged to Cecily. I adore her.
JACK. There is certainly no chance of your marrying Miss Cardew.
ALGERNON. I don’t think there is much likelihood, Jack, of you and Miss Fairfax being united.
JACK. Well, that is no business of yours.
ALGERNON. If it was my business, I wouldn’t talk about it. [Begins to eat muffins.] It is very vulgar to talk about one’s business. Only people like stock-brokers do that, and then merely at dinner parties.
JACK. How can you sit there, calmly eating muffins when we are in this horrible trouble, I can’t make out. You seem to me to be perfectly heartless.
ALGERNON. Well, I can’t eat muffins in an agitated manner. The butter would probably get on my cuffs. One should always eat muffins quite calmly. It is the only way to eat them.
JACK. I say it’s perfectly heartless your eating muffins at all, under the circumstances.
ALGERNON. When I am in trouble, eating is the only thing that consoles me. Indeed, when I am in really great trouble, as any one who knows me intimately will tell you, I refuse everything except food and drink. At the present moment I am eating muffins because I am unhappy. Besides, I am particularly fond of muffins. [Rising.]
JACK. [Rising.] Well, that is no reason why you should eat them all in that greedy way. [Takes muffins from Algernon .]
ALGERNON. [Offering tea-cake.] I wish you would have tea-cake instead. I don’t like tea-cake.
JACK. Good heavens! I suppose a man may eat his own muffins in his own garden.
ALGERNON. But you have just said it was perfectly heartless to eat muffins.
JACK. I said it was perfectly heartless of you, under the circumstances. That is a very different thing.
ALGERNON. That may be. But the muffins are the same. [He seizes the muffin-dish from Jack .]
JACK. Algy, I wish to goodness you would go.
ALGERNON. You can’t possibly ask me to go without having some dinner. It’s absurd. I never go without my dinner. No one ever does, except vegetarians and people like that. Besides I have just made arrangements with Dr. Chasuble to be christened at a quarter to six under the name of Ernest.
JACK. My dear fellow, the sooner you give up that nonsense the better. I made arrangements this morning with Dr. Chasuble to be christened myself at 5.30, and I naturally will take the name of Ernest. Gwendolen would wish it. We can’t both be christened Ernest. It’s absurd. Besides, I have a perfect right to be christened if I like. There is no evidence at all that I have ever been christened by anybody. I should think it extremely probable I never was, and so does Dr. Chasuble. It is entirely different in your case. You have been christened already.
ALGERNON. Yes, but I have not been christened for years.
JACK. Yes, but you have been christened. That is the important thing.
ALGERNON. Quite so. So I know my constitution can stand it. If you are not quite sure about your ever having been christened, I must say I think it rather dangerous your venturing on it now. It might make you very unwell. You can hardly have forgotten that some one very closely connected with you was very nearly carried off this week in Paris by a severe chill.
JACK. Yes, but you said yourself that a severe chill was not hereditary.
ALGERNON. It usen’t to be, I know—but I daresay it is now. Science is always making wonderful improvements in things.
JACK. [Picking up the muffin-dish.] Oh, that is nonsense; you are always talking nonsense.
ALGERNON. Jack, you are at the muffins again! I wish you wouldn’t. There are only two left. [Takes them.] I told you I was particularly fond of muffins.
JACK. But I hate tea-cake.
ALGERNON. Why on earth then do you allow tea-cake to be served up for your guests? What ideas you have of hospitality!
JACK. Algernon! I have already told you to go. I don’t want you here. Why don’t you go!
ALGERNON. I haven’t quite finished my tea yet! and there is still one muffin left. [ Jack groans, and sinks into a chair. Algernon still continues eating.]
Morning-room at the Manor House.
[ Gwendolen and Cecily are at the window, looking out into the garden.]
GWENDOLEN. The fact that they did not follow us at once into the house, as any one else would have done, seems to me to show that they have some sense of shame left.
CECILY. They have been eating muffins. That looks like repentance.
GWENDOLEN. [After a pause.] They don’t seem to notice us at all. Couldn’t you cough?
CECILY. But I haven’t got a cough.
GWENDOLEN. They’re looking at us. What effrontery!
CECILY. They’re approaching. That’s very forward of them.
GWENDOLEN. Let us preserve a dignified silence.
CECILY. Certainly. It’s the only thing to do now. [Enter Jack followed by Algernon . They whistle some dreadful popular air from a British Opera.]
GWENDOLEN. This dignified silence seems to produce an unpleasant effect.
CECILY. A most distasteful one.
GWENDOLEN. But we will not be the first to speak.
CECILY. Certainly not.
GWENDOLEN. Mr. Worthing, I have something very particular to ask you. Much depends on your reply.
CECILY. Gwendolen, your common sense is invaluable. Mr. Moncrieff, kindly answer me the following question. Why did you pretend to be my guardian’s brother?
ALGERNON. In order that I might have an opportunity of meeting you.
CECILY. [To Gwendolen .] That certainly seems a satisfactory explanation, does it not?
GWENDOLEN. Yes, dear, if you can believe him.
CECILY. I don’t. But that does not affect the wonderful beauty of his answer.
GWENDOLEN. True. In matters of grave importance, style, not sincerity is the vital thing. Mr. Worthing, what explanation can you offer to me for pretending to have a brother? Was it in order that you might have an opportunity of coming up to town to see me as often as possible?
JACK. Can you doubt it, Miss Fairfax?
GWENDOLEN. I have the gravest doubts upon the subject. But I intend to crush them. This is not the moment for German scepticism. [Moving to Cecily .] Their explanations appear to be quite satisfactory, especially Mr. Worthing’s. That seems to me to have the stamp of truth upon it.
CECILY. I am more than content with what Mr. Moncrieff said. His voice alone inspires one with absolute credulity.
GWENDOLEN. Then you think we should forgive them?
CECILY. Yes. I mean no.
GWENDOLEN. True! I had forgotten. There are principles at stake that one cannot surrender. Which of us should tell them? The task is not a pleasant one.
CECILY. Could we not both speak at the same time?
GWENDOLEN. An excellent idea! I nearly always speak at the same time as other people. Will you take the time from me?
CECILY. Certainly. [ Gwendolen beats time with uplifted finger.]
GWENDOLEN and CECILY [Speaking together.] Your Christian names are still an insuperable barrier. That is all!
JACK and ALGERNON [Speaking together.] Our Christian names! Is that all? But we are going to be christened this afternoon.
GWENDOLEN. [To Jack .] For my sake you are prepared to do this terrible thing?
JACK. I am.
CECILY. [To Algernon .] To please me you are ready to face this fearful ordeal?
ALGERNON. I am!
GWENDOLEN. How absurd to talk of the equality of the sexes! Where questions of self-sacrifice are concerned, men are infinitely beyond us.
JACK. We are. [Clasps hands with Algernon .]
CECILY. They have moments of physical courage of which we women know absolutely nothing.
GWENDOLEN. [To Jack .] Darling!
ALGERNON. [To Cecily .] Darling! [They fall into each other’s arms.]
[Enter Merriman . When he enters he coughs loudly, seeing the situation.]
MERRIMAN. Ahem! Ahem! Lady Bracknell!
JACK. Good heavens!
[Enter Lady Bracknell . The couples separate in alarm. Exit Merriman .]
LADY BRACKNELL. Gwendolen! What does this mean?
GWENDOLEN. Merely that I am engaged to be married to Mr. Worthing, mamma.
LADY BRACKNELL. Come here. Sit down. Sit down immediately. Hesitation of any kind is a sign of mental decay in the young, of physical weakness in the old. [Turns to Jack .] Apprised, sir, of my daughter’s sudden flight by her trusty maid, whose confidence I purchased by means of a small coin, I followed her at once by a luggage train. Her unhappy father is, I am glad to say, under the impression that she is attending a more than usually lengthy lecture by the University Extension Scheme on the Influence of a permanent income on Thought. I do not propose to undeceive him. Indeed I have never undeceived him on any question. I would consider it wrong. But of course, you will clearly understand that all communication between yourself and my daughter must cease immediately from this moment. On this point, as indeed on all points, I am firm.
JACK. I am engaged to be married to Gwendolen, Lady Bracknell!
LADY BRACKNELL. You are nothing of the kind, sir. And now, as regards Algernon! . . . Algernon!
ALGERNON. Yes, Aunt Augusta.
LADY BRACKNELL. May I ask if it is in this house that your invalid friend Mr. Bunbury resides?
ALGERNON. [Stammering.] Oh! No! Bunbury doesn’t live here. Bunbury is somewhere else at present. In fact, Bunbury is dead.
LADY BRACKNELL. Dead! When did Mr. Bunbury die? His death must have been extremely sudden.
ALGERNON. [Airily.] Oh! I killed Bunbury this afternoon. I mean poor Bunbury died this afternoon.
LADY BRACKNELL. What did he die of?
ALGERNON. Bunbury? Oh, he was quite exploded.
LADY BRACKNELL. Exploded! Was he the victim of a revolutionary outrage? I was not aware that Mr. Bunbury was interested in social legislation. If so, he is well punished for his morbidity.
ALGERNON. My dear Aunt Augusta, I mean he was found out! The doctors found out that Bunbury could not live, that is what I mean—so Bunbury died.
LADY BRACKNELL. He seems to have had great confidence in the opinion of his physicians. I am glad, however, that he made up his mind at the last to some definite course of action, and acted under proper medical advice. And now that we have finally got rid of this Mr. Bunbury, may I ask, Mr. Worthing, who is that young person whose hand my nephew Algernon is now holding in what seems to me a peculiarly unnecessary manner?
JACK. That lady is Miss Cecily Cardew, my ward. [ Lady Bracknell bows coldly to Cecily .]
ALGERNON. I am engaged to be married to Cecily, Aunt Augusta.
LADY BRACKNELL. I beg your pardon?
CECILY. Mr. Moncrieff and I are engaged to be married, Lady Bracknell.
LADY BRACKNELL. [With a shiver, crossing to the sofa and sitting down.] I do not know whether there is anything peculiarly exciting in the air of this particular part of Hertfordshire, but the number of engagements that go on seems to me considerably above the proper average that statistics have laid down for our guidance. I think some preliminary inquiry on my part would not be out of place. Mr. Worthing, is Miss Cardew at all connected with any of the larger railway stations in London? I merely desire information. Until yesterday I had no idea that there were any families or persons whose origin was a Terminus. [ Jack looks perfectly furious, but restrains himself.]
JACK. [In a clear, cold voice.] Miss Cardew is the grand-daughter of the late Mr. Thomas Cardew of 149 Belgrave Square, S.W.; Gervase Park, Dorking, Surrey; and the Sporran, Fifeshire, N.B.
LADY BRACKNELL. That sounds not unsatisfactory. Three addresses always inspire confidence, even in tradesmen. But what proof have I of their authenticity?
JACK. I have carefully preserved the Court Guides of the period. They are open to your inspection, Lady Bracknell.
LADY BRACKNELL. [Grimly.] I have known strange errors in that publication.
JACK. Miss Cardew’s family solicitors are Messrs. Markby, Markby, and Markby.
LADY BRACKNELL. Markby, Markby, and Markby? A firm of the very highest position in their profession. Indeed I am told that one of the Mr. Markby’s is occasionally to be seen at dinner parties. So far I am satisfied.
JACK. [Very irritably.] How extremely kind of you, Lady Bracknell! I have also in my possession, you will be pleased to hear, certificates of Miss Cardew’s birth, baptism, whooping cough, registration, vaccination, confirmation, and the measles; both the German and the English variety.
LADY BRACKNELL. Ah! A life crowded with incident, I see; though perhaps somewhat too exciting for a young girl. I am not myself in favour of premature experiences. [Rises, looks at her watch.] Gwendolen! the time approaches for our departure. We have not a moment to lose. As a matter of form, Mr. Worthing, I had better ask you if Miss Cardew has any little fortune?
JACK. Oh! about a hundred and thirty thousand pounds in the Funds. That is all. Goodbye, Lady Bracknell. So pleased to have seen you.
LADY BRACKNELL. [Sitting down again.] A moment, Mr. Worthing. A hundred and thirty thousand pounds! And in the Funds! Miss Cardew seems to me a most attractive young lady, now that I look at her. Few girls of the present day have any really solid qualities, any of the qualities that last, and improve with time. We live, I regret to say, in an age of surfaces. [To Cecily .] Come over here, dear. [ Cecily goes across.] Pretty child! your dress is sadly simple, and your hair seems almost as Nature might have left it. But we can soon alter all that. A thoroughly experienced French maid produces a really marvellous result in a very brief space of time. I remember recommending one to young Lady Lancing, and after three months her own husband did not know her.
JACK. And after six months nobody knew her.
LADY BRACKNELL. [Glares at Jack for a few moments. Then bends, with a practised smile, to Cecily .] Kindly turn round, sweet child. [ Cecily turns completely round.] No, the side view is what I want. [ Cecily presents her profile.] Yes, quite as I expected. There are distinct social possibilities in your profile. The two weak points in our age are its want of principle and its want of profile. The chin a little higher, dear. Style largely depends on the way the chin is worn. They are worn very high, just at present. Algernon!
ALGERNON. Yes, Aunt Augusta!
LADY BRACKNELL. There are distinct social possibilities in Miss Cardew’s profile.
ALGERNON. Cecily is the sweetest, dearest, prettiest girl in the whole world. And I don’t care twopence about social possibilities.
LADY BRACKNELL. Never speak disrespectfully of Society, Algernon. Only people who can’t get into it do that. [To Cecily .] Dear child, of course you know that Algernon has nothing but his debts to depend upon. But I do not approve of mercenary marriages. When I married Lord Bracknell I had no fortune of any kind. But I never dreamed for a moment of allowing that to stand in my way. Well, I suppose I must give my consent.
ALGERNON. Thank you, Aunt Augusta.
LADY BRACKNELL. Cecily, you may kiss me!
CECILY. [Kisses her.] Thank you, Lady Bracknell.
LADY BRACKNELL. You may also address me as Aunt Augusta for the future.
CECILY. Thank you, Aunt Augusta.
LADY BRACKNELL. The marriage, I think, had better take place quite soon.
LADY BRACKNELL. To speak frankly, I am not in favour of long engagements. They give people the opportunity of finding out each other’s character before marriage, which I think is never advisable.
JACK. I beg your pardon for interrupting you, Lady Bracknell, but this engagement is quite out of the question. I am Miss Cardew’s guardian, and she cannot marry without my consent until she comes of age. That consent I absolutely decline to give.
LADY BRACKNELL. Upon what grounds may I ask? Algernon is an extremely, I may almost say an ostentatiously, eligible young man. He has nothing, but he looks everything. What more can one desire?
JACK. It pains me very much to have to speak frankly to you, Lady Bracknell, about your nephew, but the fact is that I do not approve at all of his moral character. I suspect him of being untruthful. [ Algernon and Cecily look at him in indignant amazement.]
LADY BRACKNELL. Untruthful! My nephew Algernon? Impossible! He is an Oxonian.
JACK. I fear there can be no possible doubt about the matter. This afternoon during my temporary absence in London on an important question of romance, he obtained admission to my house by means of the false pretence of being my brother. Under an assumed name he drank, I’ve just been informed by my butler, an entire pint bottle of my Perrier-Jouet, Brut, ’89; wine I was specially reserving for myself. Continuing his disgraceful deception, he succeeded in the course of the afternoon in alienating the affections of my only ward. He subsequently stayed to tea, and devoured every single muffin. And what makes his conduct all the more heartless is, that he was perfectly well aware from the first that I have no brother, that I never had a brother, and that I don’t intend to have a brother, not even of any kind. I distinctly told him so myself yesterday afternoon.
LADY BRACKNELL. Ahem! Mr. Worthing, after careful consideration I have decided entirely to overlook my nephew’s conduct to you.
JACK. That is very generous of you, Lady Bracknell. My own decision, however, is unalterable. I decline to give my consent.
LADY BRACKNELL. [To Cecily .] Come here, sweet child. [ Cecily goes over.] How old are you, dear?
CECILY. Well, I am really only eighteen, but I always admit to twenty when I go to evening parties.
LADY BRACKNELL. You are perfectly right in making some slight alteration. Indeed, no woman should ever be quite accurate about her age. It looks so calculating . . . [In a meditative manner.] Eighteen, but admitting to twenty at evening parties. Well, it will not be very long before you are of age and free from the restraints of tutelage. So I don’t think your guardian’s consent is, after all, a matter of any importance.
JACK. Pray excuse me, Lady Bracknell, for interrupting you again, but it is only fair to tell you that according to the terms of her grandfather’s will Miss Cardew does not come legally of age till she is thirty-five.
LADY BRACKNELL. That does not seem to me to be a grave objection. Thirty-five is a very attractive age. London society is full of women of the very highest birth who have, of their own free choice, remained thirty-five for years. Lady Dumbleton is an instance in point. To my own knowledge she has been thirty-five ever since she arrived at the age of forty, which was many years ago now. I see no reason why our dear Cecily should not be even still more attractive at the age you mention than she is at present. There will be a large accumulation of property.
CECILY. Algy, could you wait for me till I was thirty-five?
ALGERNON. Of course I could, Cecily. You know I could.
CECILY. Yes, I felt it instinctively, but I couldn’t wait all that time. I hate waiting even five minutes for anybody. It always makes me rather cross. I am not punctual myself, I know, but I do like punctuality in others, and waiting, even to be married, is quite out of the question.
ALGERNON. Then what is to be done, Cecily?
CECILY. I don’t know, Mr. Moncrieff.
LADY BRACKNELL. My dear Mr. Worthing, as Miss Cardew states positively that she cannot wait till she is thirty-five—a remark which I am bound to say seems to me to show a somewhat impatient nature—I would beg of you to reconsider your decision.
JACK. But my dear Lady Bracknell, the matter is entirely in your own hands. The moment you consent to my marriage with Gwendolen, I will most gladly allow your nephew to form an alliance with my ward.
LADY BRACKNELL. [Rising and drawing herself up.] You must be quite aware that what you propose is out of the question.
JACK. Then a passionate celibacy is all that any of us can look forward to.
LADY BRACKNELL. That is not the destiny I propose for Gwendolen. Algernon, of course, can choose for himself. [Pulls out her watch.] Come, dear, [ Gwendolen rises] we have already missed five, if not six, trains. To miss any more might expose us to comment on the platform.
[Enter Dr. Chasuble .]
CHASUBLE. Everything is quite ready for the christenings.
LADY BRACKNELL. The christenings, sir! Is not that somewhat premature?
CHASUBLE. [Looking rather puzzled, and pointing to Jack and Algernon .] Both these gentlemen have expressed a desire for immediate baptism.
LADY BRACKNELL. At their age? The idea is grotesque and irreligious! Algernon, I forbid you to be baptized. I will not hear of such excesses. Lord Bracknell would be highly displeased if he learned that that was the way in which you wasted your time and money.
CHASUBLE. Am I to understand then that there are to be no christenings at all this afternoon?
JACK. I don’t think that, as things are now, it would be of much practical value to either of us, Dr. Chasuble.
CHASUBLE. I am grieved to hear such sentiments from you, Mr. Worthing. They savour of the heretical views of the Anabaptists, views that I have completely refuted in four of my unpublished sermons. However, as your present mood seems to be one peculiarly secular, I will return to the church at once. Indeed, I have just been informed by the pew-opener that for the last hour and a half Miss Prism has been waiting for me in the vestry.
LADY BRACKNELL. [Starting.] Miss Prism! Did I hear you mention a Miss Prism?
CHASUBLE. Yes, Lady Bracknell. I am on my way to join her.
LADY BRACKNELL. Pray allow me to detain you for a moment. This matter may prove to be one of vital importance to Lord Bracknell and myself. Is this Miss Prism a female of repellent aspect, remotely connected with education?
CHASUBLE. [Somewhat indignantly.] She is the most cultivated of ladies, and the very picture of respectability.
LADY BRACKNELL. It is obviously the same person. May I ask what position she holds in your household?
CHASUBLE. [Severely.] I am a celibate, madam.
JACK. [Interposing.] Miss Prism, Lady Bracknell, has been for the last three years Miss Cardew’s esteemed governess and valued companion.
LADY BRACKNELL. In spite of what I hear of her, I must see her at once. Let her be sent for.
CHASUBLE. [Looking off.] She approaches; she is nigh.
[Enter Miss Prism hurriedly.]
MISS PRISM. I was told you expected me in the vestry, dear Canon. I have been waiting for you there for an hour and three-quarters. [Catches sight of Lady Bracknell , who has fixed her with a stony glare. Miss Prism grows pale and quails. She looks anxiously round as if desirous to escape.]
LADY BRACKNELL. [In a severe, judicial voice.] Prism! [ Miss Prism bows her head in shame.] Come here, Prism! [ Miss Prism approaches in a humble manner.] Prism! Where is that baby? [General consternation. The Canon starts back in horror. Algernon and Jack pretend to be anxious to shield Cecily and Gwendolen from hearing the details of a terrible public scandal.] Twenty-eight years ago, Prism, you left Lord Bracknell’s house, Number 104, Upper Grosvenor Street, in charge of a perambulator that contained a baby of the male sex. You never returned. A few weeks later, through the elaborate investigations of the Metropolitan police, the perambulator was discovered at midnight, standing by itself in a remote corner of Bayswater. It contained the manuscript of a three-volume novel of more than usually revolting sentimentality. [ Miss Prism starts in involuntary indignation.] But the baby was not there! [Every one looks at Miss Prism .] Prism! Where is that baby? [A pause.]
MISS PRISM. Lady Bracknell, I admit with shame that I do not know. I only wish I did. The plain facts of the case are these. On the morning of the day you mention, a day that is for ever branded on my memory, I prepared as usual to take the baby out in its perambulator. I had also with me a somewhat old, but capacious hand-bag in which I had intended to place the manuscript of a work of fiction that I had written during my few unoccupied hours. In a moment of mental abstraction, for which I never can forgive myself, I deposited the manuscript in the basinette, and placed the baby in the hand-bag.
JACK. [Who has been listening attentively.] But where did you deposit the hand-bag?
MISS PRISM. Do not ask me, Mr. Worthing.
JACK. Miss Prism, this is a matter of no small importance to me. I insist on knowing where you deposited the hand-bag that contained that infant.
MISS PRISM. I left it in the cloak-room of one of the larger railway stations in London.
JACK. What railway station?
MISS PRISM. [Quite crushed.] Victoria. The Brighton line. [Sinks into a chair.]
JACK. I must retire to my room for a moment. Gwendolen, wait here for me.
GWENDOLEN. If you are not too long, I will wait here for you all my life. [Exit Jack in great excitement.]
CHASUBLE. What do you think this means, Lady Bracknell?
LADY BRACKNELL. I dare not even suspect, Dr. Chasuble. I need hardly tell you that in families of high position strange coincidences are not supposed to occur. They are hardly considered the thing.
[Noises heard overhead as if some one was throwing trunks about. Every one looks up.]
CECILY. Uncle Jack seems strangely agitated.
CHASUBLE. Your guardian has a very emotional nature.
LADY BRACKNELL. This noise is extremely unpleasant. It sounds as if he was having an argument. I dislike arguments of any kind. They are always vulgar, and often convincing.
CHASUBLE. [Looking up.] It has stopped now. [The noise is redoubled.]
LADY BRACKNELL. I wish he would arrive at some conclusion.
GWENDOLEN. This suspense is terrible. I hope it will last. [Enter Jack with a hand-bag of black leather in his hand.]
JACK. [Rushing over to Miss Prism .] Is this the hand-bag, Miss Prism? Examine it carefully before you speak. The happiness of more than one life depends on your answer.
MISS PRISM. [Calmly.] It seems to be mine. Yes, here is the injury it received through the upsetting of a Gower Street omnibus in younger and happier days. Here is the stain on the lining caused by the explosion of a temperance beverage, an incident that occurred at Leamington. And here, on the lock, are my initials. I had forgotten that in an extravagant mood I had had them placed there. The bag is undoubtedly mine. I am delighted to have it so unexpectedly restored to me. It has been a great inconvenience being without it all these years.
JACK. [In a pathetic voice.] Miss Prism, more is restored to you than this hand-bag. I was the baby you placed in it.
MISS PRISM. [Amazed.] You?
JACK. [Embracing her.] Yes . . . mother!
MISS PRISM. [Recoiling in indignant astonishment.] Mr. Worthing! I am unmarried!
JACK. Unmarried! I do not deny that is a serious blow. But after all, who has the right to cast a stone against one who has suffered? Cannot repentance wipe out an act of folly? Why should there be one law for men, and another for women? Mother, I forgive you. [Tries to embrace her again.]
MISS PRISM. [Still more indignant.] Mr. Worthing, there is some error. [Pointing to Lady Bracknell .] There is the lady who can tell you who you really are.
JACK. [After a pause.] Lady Bracknell, I hate to seem inquisitive, but would you kindly inform me who I am?
LADY BRACKNELL. I am afraid that the news I have to give you will not altogether please you. You are the son of my poor sister, Mrs. Moncrieff, and consequently Algernon’s elder brother.
JACK. Algy’s elder brother! Then I have a brother after all. I knew I had a brother! I always said I had a brother! Cecily,—how could you have ever doubted that I had a brother? [Seizes hold of Algernon .] Dr. Chasuble, my unfortunate brother. Miss Prism, my unfortunate brother. Gwendolen, my unfortunate brother. Algy, you young scoundrel, you will have to treat me with more respect in the future. You have never behaved to me like a brother in all your life.
ALGERNON. Well, not till to-day, old boy, I admit. I did my best, however, though I was out of practice.
[Shakes hands.]
GWENDOLEN. [To Jack .] My own! But what own are you? What is your Christian name, now that you have become some one else?
JACK. Good heavens! . . . I had quite forgotten that point. Your decision on the subject of my name is irrevocable, I suppose?
GWENDOLEN. I never change, except in my affections.
CECILY. What a noble nature you have, Gwendolen!
JACK. Then the question had better be cleared up at once. Aunt Augusta, a moment. At the time when Miss Prism left me in the hand-bag, had I been christened already?
LADY BRACKNELL. Every luxury that money could buy, including christening, had been lavished on you by your fond and doting parents.
JACK. Then I was christened! That is settled. Now, what name was I given? Let me know the worst.
LADY BRACKNELL. Being the eldest son you were naturally christened after your father.
JACK. [Irritably.] Yes, but what was my father’s Christian name?
LADY BRACKNELL. [Meditatively.] I cannot at the present moment recall what the General’s Christian name was. But I have no doubt he had one. He was eccentric, I admit. But only in later years. And that was the result of the Indian climate, and marriage, and indigestion, and other things of that kind.
JACK. Algy! Can’t you recollect what our father’s Christian name was?
ALGERNON. My dear boy, we were never even on speaking terms. He died before I was a year old.
JACK. His name would appear in the Army Lists of the period, I suppose, Aunt Augusta?
LADY BRACKNELL. The General was essentially a man of peace, except in his domestic life. But I have no doubt his name would appear in any military directory.
JACK. The Army Lists of the last forty years are here. These delightful records should have been my constant study. [Rushes to bookcase and tears the books out.] M. Generals . . . Mallam, Maxbohm, Magley, what ghastly names they have—Markby, Migsby, Mobbs, Moncrieff! Lieutenant 1840, Captain, Lieutenant-Colonel, Colonel, General 1869, Christian names, Ernest John. [Puts book very quietly down and speaks quite calmly.] I always told you, Gwendolen, my name was Ernest, didn’t I? Well, it is Ernest after all. I mean it naturally is Ernest.
LADY BRACKNELL. Yes, I remember now that the General was called Ernest, I knew I had some particular reason for disliking the name.
GWENDOLEN. Ernest! My own Ernest! I felt from the first that you could have no other name!
JACK. Gwendolen, it is a terrible thing for a man to find out suddenly that all his life he has been speaking nothing but the truth. Can you forgive me?
GWENDOLEN. I can. For I feel that you are sure to change.
CHASUBLE. [To Miss Prism .] Lætitia! [Embraces her]
MISS PRISM. [Enthusiastically.] Frederick! At last!
ALGERNON. Cecily! [Embraces her.] At last!
JACK. Gwendolen! [Embraces her.] At last!
LADY BRACKNELL. My nephew, you seem to be displaying signs of triviality.
JACK. On the contrary, Aunt Augusta, I’ve now realised for the first time in my life the vital Importance of Being Earnest.
This eBook is for the use of anyone anywhere in the United States and most other parts of the world at no cost and with almost no restrictions whatsoever. You may copy it, give it away or re-use it under the terms of the Project Gutenberg License included with this eBook or online at www.gutenberg.org . If you are not located in the United States, you will have to check the laws of the country where you are located before using this eBook.
The Importance of Being Earnest: Brief Overview and Thorough Analysis
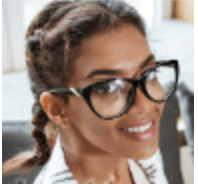
If you're intrigued by the wit and whimsy of 'The Importance of Being Earnest,' then settle in for a delightful journey through this literary gem. In an era when seriousness reigned supreme in literature, Oscar Wilde's eccentric comedy breathed fresh life into British society of the late 1800s.
At first glance, one might dismiss it as mere frivolity, but that would be a mistake. Behind every comedic flourish lies a profound layer of meaning waiting to be unearthed. Let this article be your guide to The Importance of Being Earnest analysis as we delve into the depths of Wilde's wit, offering a unique perspective and perhaps even a touch of inspiration for your own scholarly pursuits and custom research paper .
Shortly about Oscar Wilde
Born on October 16, 1854, in Dublin, Ireland, Oscar Wilde was destined for literary greatness from the start. With a razor-sharp wit and a penchant for drama, he dazzled those around him from an early age.
After receiving an education at Trinity College, Dublin, Wilde set sail for England, where he quickly became a literary sensation. His plays, novels, and essays captured the essence of Victorian society with a sharpness and humor unparalleled in his time.
Wilde's magnum opus, 'The Importance of Being Earnest,' stands as a testament to his comedic genius and satirical prowess. With its biting wit and clever wordplay, the play remains a staple of English literature, captivating audiences with its timeless humor.
However, behind Wilde's public persona lay a life rife with scandal and controversy. His relationship with Lord Alfred Douglas, known as 'Bosie,' sparked outrage and condemnation in Victorian society, ultimately leading to Wilde's downfall.
In 1895, Wilde faced a series of trials that culminated in his conviction for 'gross indecency' and subsequent imprisonment. Despite the adversity he faced, he maintained his irrepressible spirit, penning one of his most famous works, 'De Profundis,' during his time in prison.
The author's legacy endures not only in his literary works but also in his unapologetic embrace of individualism and defiance of societal norms. His famous aphorisms, such as 'I can resist anything except temptation' and 'We are all in the gutter, but some of us are looking at the stars,' continue to resonate with readers around the world, reminding us to live life with wit, style, and unyielding authenticity.
Do You Struggle Writing an Essay?
Ask our professional writers to get help.
Before we dive into the The Importance of Being Earnest analysis, let's take a moment to familiarize ourselves with the play and briefly review the main characters.
Jack Worthing
A gentleman of wealth and property, Jack leads a double life. In the city, he goes by the name Ernest, while in the countryside, he is Jack. He is responsible, well-meaning, and deeply in love with Gwendolen Fairfax. However, his tangled web of lies threatens to unravel as the play progresses.
Algernon Moncrieff
Jack's close friend and charming bachelor, Algernon, is known for his wit and love of pleasure. He adopts the identity of Ernest to pursue his romantic interests, leading to humorous misunderstandings and complications. Algernon's carefree demeanor contrasts sharply with Jack's more serious nature.
Cecily Cardew
Jack's ward and a charming young woman, Cecily, is sheltered yet spirited, with a penchant for romantic fantasies. She falls deeply in love with the idea of Ernest before even meeting him, setting the stage for a series of comedic misunderstandings with Algernon.
Gwendolen Fairfax
The sophisticated and determined love interest of Jack, Gwendolen, is enamored with the name Ernest and believes it to be a vital prerequisite for a husband. She is headstrong, witty, and unapologetically romantic, determined to marry a man by that name regardless of any obstacles.
Lady Bracknell
Gwendolen's formidable and snobbish mother, Lady Bracknell, is the epitome of Victorian societal expectations. She is determined to secure a suitable match for her daughter and is highly critical of anyone who does not meet her exacting standards, including Jack.
Cecily's governess, Miss Prism, is a well-intentioned but absent-minded character with a mysterious past. She becomes entangled in the romantic entanglements of the other characters, inadvertently revealing secrets that have long been buried.
Rev. Canon Chasuble, D.D.
The local rector, Canon Chasuble, is a somewhat oblivious clergyman who becomes embroiled in the romantic escapades of the other characters. He provides a source of comic relief with his peculiarities and misunderstandings.
First performed in 1895, the play revolves around mistaken identities, societal conventions, and the absurdities of romance in Victorian England.
The story begins with Algernon Moncrieff, a charming bachelor, and his friend Jack Worthing. Jack leads a double life, presenting himself as Ernest in the city and Jack in the country. Algernon, intrigued by Jack's mysterious past and his beautiful young ward, Cecily Cardew, decides to visit Jack's country estate.
Meanwhile, Jack is in love with Gwendolen Fairfax, Lady Bracknell's sophisticated and determined daughter. Gwendolen shares Jack's passion, particularly for the name 'Ernest, believing it to be the epitome of masculine charm.
As the plot unfolds, mistaken identities abound. Algernon masquerades as Jack's fictitious brother, Ernest, to court Cecily, who quickly falls in love with the idea of being engaged to someone named Ernest.
The arrival of Gwendolen at Jack's country estate further complicates matters when she discovers that Jack's real name is not Ernest, as she had believed. Nevertheless, both Gwendolen and Cecily remain determined to marry a man named Ernest.
Amidst the chaos, Lady Bracknell arrives, determined to ensure that her daughter marries into a suitable family. However, she is appalled by Jack's mysterious parentage and refuses to consent to his marriage to Gwendolen.
In the end, secrets are revealed, misunderstandings are resolved, and true love triumphs. Jack learns of his true parentage and his real name, Ernest. Lady Bracknell relents, giving her blessing to the marriages of both Jack and Algernon to Gwendolen and Cecily, respectively. The play concludes with a humorous twist as Jack reflects on the absurdity of his own story and the importance of being earnest in matters of both love and social etiquette.
The Importance of Being Earnest Essay Sample
Here's a glimpse into The Importance of Being Earnest essay sample exploring the role of honesty. If you'd like a custom one, don't forget you can always pay someone to do my essay and receive an expertly crafted paper from us.
The Importance of Being Earnest Analysis
The playwright masterfully dissects the hypocrisies and absurdities of the Victorian upper class through a lens of satire and humor. Let's delve deeper into the analysis of The Importance of Being Earnest and examine the key elements of this timeless play:
.webp)
- Dual Identities and Deception: Wilde explores the theme of dual identities and deception through the characters of Jack and Algernon, who adopt false personas ('Ernest') to navigate social expectations. This theme highlights the disconnect between appearance and reality, exposing the superficiality of societal norms.
- Social Class and Marriage: The play satirizes the rigid social hierarchies and expectations surrounding marriage in Victorian society. Characters like Lady Bracknell embody the aristocratic disdain for those deemed beneath their station, while the pursuit of marriage becomes a farcical endeavor driven by wealth and status rather than genuine affection.
- Morality and Hypocrisy: Wilde exposes the hypocrisy and moral decay lurking beneath the veneer of respectability. The characters engage in deceitful behaviors and moral relativism, challenging conventional notions of virtue and propriety.
2. Characters:
- Jack Worthing (Ernest): Jack serves as a representative of the upper-middle class grappling with societal expectations and personal desires. His adoption of the persona 'Ernest' reflects his desire to escape the constraints of his social identity while also highlighting the absurdity of societal conventions.
- Algernon Moncrieff: Algernon embodies the dandyish charm and hedonistic tendencies prevalent among the aristocracy. His pursuit of pleasure and romantic conquests masks a deeper sense of ennui and disillusionment with societal norms.
- Gwendolen Fairfax: Gwendolen represents the prototypical Victorian woman constrained by societal expectations of femininity and marriage. Her obsession with the name 'Ernest' symbolizes her desire for romantic fulfillment and escape from her stifling existence.
- Cecily Cardew: Cecily embodies youthful innocence and romantic idealism, eagerly awaiting her own romantic hero in the form of 'Ernest.' Her sheltered upbringing and penchant for melodrama serve as a foil to the cynicism of the adult characters.
- Lady Bracknell: Lady Bracknell personifies the aristocratic disdain for social mobility and the pursuit of personal happiness. Her interrogation of potential suitors highlights the absurdity of marriage as a transactional arrangement driven by wealth and lineage.
3. Satirical Techniques:
- Epigrams and Paradoxes: Wilde's use of epigrams and paradoxes infuses the dialogue with wit and irony, challenging conventional wisdom and exposing the contradictions inherent in Victorian society.
- Exaggeration and Farce: The play employs exaggeration and farce to heighten the absurdity of its characters and situations, eliciting laughter while also provoking reflection on deeper societal issues.
4. Resolution:
- Revelations and Irony: The resolution of the play sees the unraveling of deception and the revelation of the characters' true identities. However, the irony lies in the fact that despite the chaos and absurdity, the characters ultimately conform to societal expectations, underscoring the entrenched nature of Victorian values.
As we continue Importance of Being Earnest analysis, let's examine some of the central themes explored in the play that offer insight into the Victorian upper class while challenging traditional norms.
Earnestness
At the heart of the play lies the theme of earnestness, or the lack thereof, among the characters. Wilde examines the contrast between those who take life seriously and those who adopt a more carefree attitude. Characters like Jack and Algernon grapple with their responsibilities, while others, such as Algernon's butler, Lane, exhibit a nonchalant approach to life's obligations.
Responsibility
Wilde explores the notion of responsibility through the actions of his characters. While some, like Jack, exhibit a strong sense of duty towards their societal obligations, others, like Algernon, shirk their responsibilities in favor of pleasure-seeking. This theme highlights the tension between individual desires and societal expectations.
Religion serves as a backdrop against which Wilde critiques the superficiality and hypocrisy of the upper class. The characters' shallow attitude towards religious rituals reflects a broader skepticism towards traditional institutions and moral values. Wilde suggests that religion is often used as a facade to maintain appearances rather than a genuine expression of faith.
Marriage emerges as a central theme in the play, with Wilde satirizing the institution and the societal expectations surrounding it. Despite the characters' aversion to the idea of matrimony, they find themselves entangled in a web of engagements and proposals. Wilde highlights the tension between societal pressure to marry and the desire for personal freedom, particularly among the male protagonists.
Freedom and Individuality
Wilde subtly critiques the constraints placed on individuals by Victorian upper classes, particularly in terms of gender roles and social expectations. Characters like Cecily and Gwendolen assert their independence and agency in pursuing their romantic interests, challenging traditional notions of femininity and passivity.
Social Class
Social class plays a significant role in the play, with characters like Lady Bracknell embodying the aristocratic disdain for those deemed beneath their station. Wilde exposes the absurdity of class distinctions and the superficiality of societal hierarchies, suggesting that one's worth should not be determined by birth or wealth.
If you're eager to delve deeper into the themes, consider the option to buy a essay from our team of expert analysts.
The Importance of Being Earnest Symbols
What are some symbols in The Importance of Being Earnest? They appear throughout the play, bringing additional meaning to the scenes. Let's examine the major figures:
.webp)
Ernest and Bunbury
Undeniably, both 'brother Ernest' and 'friend Bunbury' are fundamental to the play. 'Ernest' is the fictional alter ego created by Jack and Algernon to escape the constraints of their everyday lives. He represents the desire to break free from societal expectations and indulge in frivolity. Similarly, 'Bunbury' symbolizes the fabricated excuses and deceitful practices employed by the characters to evade responsibility and pursue their desires.
Big city and countryside
The contrast between the bustling city life of London and the tranquil countryside serves as a symbolic reflection of societal attitudes and class distinctions. The characters' discussions about their preferences for city living versus country living highlight their aspirations for social status and cultural refinement. The city represents sophistication and wealth, while the countryside is associated with simplicity and lower social standing. Wilde uses this symbolism to critique the superficiality of societal judgments based on geography and class, challenging the notion that one's worth is determined by one's surroundings.
Food and Dining
Scenes set around the dining table are often accompanied by witty banter and social commentary, highlighting the performative nature of Victorian etiquette. Food becomes a symbol of status and refinement, with characters using elaborate dinner parties and tea ceremonies to assert their social superiority. However, beneath the veneer of civility lies a world of deception and hypocrisy, as characters engage in verbal sparring and manipulation over tea and cucumber sandwiches.
The Importance of Being Earnest Movie
While you can relish Oscar Wilde's play on stage, you can also savor 'The Importance of Being Earnest' movie from the comfort of your home. Unlike the works of George Bernard Shaw, Charles Dickens, or Jane Austen, Wilde's comedic genius creates an atmosphere that is both cozy and humorous, a vibe that shines through in the 2002 film adaptation.
The movie boasts high-quality set decorations and costumes that transport viewers to the England of Queen Victoria. The attention to detail helps recreate the opulence and elegance of the Victorian era, providing a visually stunning backdrop for the witty banter and comedic antics of the characters.
In terms of success, the film achieved impressive financial returns, raking in over seventeen million dollars in revenue worldwide. This widespread success speaks to the enduring appeal of Wilde's timeless humor and the universal themes explored in the play.
Are you working on a war essay assignment, or perhaps you're curious about who is Daisy in The Great Gatsby ? Our blog offers a variety of intriguing articles that may pique your interest.
The Importance of Being Earnest Quotes
Oscar Wilde is renowned for his unforgettable quotes, and 'The Importance of Being Earnest' is brimming with brilliant lines that capture the essence of its characters.
Take, for example, Lady Bracknell's infamous line, 'To lose one parent may be regarded as a misfortune… to lose both seems like carelessness.' This quip not only showcases Wilde's razor-sharp humor but also characterizes Lady Bracknell as a woman lacking in empathy and understanding.
Similarly, Cecily Cardew's remark, 'I never travel without my diary. One should always have something sensational to read on the train,' speaks volumes about her shallow and melodramatic nature. It's a witty observation that perfectly encapsulates Cecily's penchant for romantic fantasy and self-absorption.
Throughout the play, Wilde's dialogue is peppered with clever and incisive lines, each tailored to the character uttering them. Whether it's Algernon's irreverent wit or Jack's earnest sincerity, every character's voice rings true, adding depth and humor to the narrative.
As we conclude The Importance of Being Earnest analysis, the phenomenal comedy by Oscar Wilde shook the conservative empire that got used to Charles Dickens, George Bernard Shaw, and Jane Austen. Comedic elements, exaggerations, and different literary devices entertain the audience while reminding them of an important trait like honesty. This author was truly ahead of his time, as the play still wins people's hearts today.
And before you go, don't forget our team of writers is always here to assist you! Whether you need math or physics help , simply submit your ' help me with my math homework ' request, and we'll ensure it's completed to the highest standard!
Curious about Oscar Wilde's Masterpiece?
Let us guide you through its wonders with our thorough analysis.Â
What Literary Devices Does Oscar Wilde Use in The Importance of Being Earnest?
What makes the importance of being earnest a comedy of manners, what is the main message of the importance of being earnest, what is the book the importance of being earnest about, what is the significance of the repetition of the word absurd in the importance of being earnest, related articles.

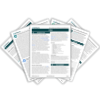
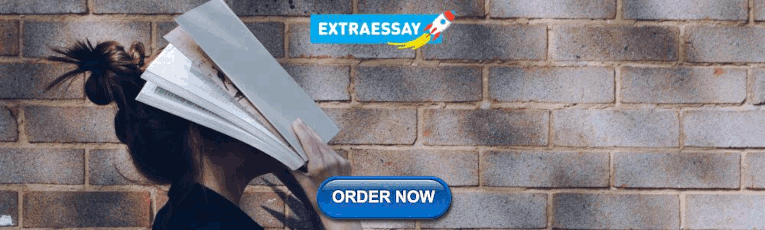
The Importance of Being Earnest
Oscar wilde, ask litcharts ai: the answer to your questions.

The Art of Deception: Fact v. Fiction
As a leader of the Aesthetic movement, Wilde was especially interested in the relationship between life and art, pondering the eternal question, “Does art imitate life, or life imitate art?” Wilde explores this relationship in The Importance of Being Earnest through the conflict that arises when fact collides with fiction. The conflict between fact and fiction is driven by Algernon and Jack’s lies about their respective identities, specifically the fictional personas they create in order…

The Pursuit of Marriage
The pursuit of marriage is a driving force behind much of the play’s action. Similar to many Victorian novels of the period, the play reads as a marriage plot, documenting the errors in social etiquette and romantic upheavals that come about as Jack and Algernon stumble towards the altar. Jack pursues Gwendolen’s hand, while Algernon pursues Cecily . Because Jack and Algernon are willing to go to such outlandish lengths to appease Gwendolen and Cecily’s…

Cash, Class, and Character
The Victorian society in which Wilde lived was concerned with wealth, family status, and moral character, especially when it came to marriage. Lady Bracknell’s interrogation of Jack’s proposal to marry Gwendolen demonstrates the three “Cs”—cash, class, and character. First she asks him about his finances and then his family relations, a measure of his class. That Jack has none—no family relations, or family name, reflects poorly on his character. Upon finding that Jack has no…

Name and Identity
Through Jack’s search for his origins and family name, Wilde satirizes the Victorian Era’s intense scrutiny of cash, class, and character. Wilde subversively prods this question through the name of “ Ernest ,” a Christian name, or given name, as opposed to a family name. The name of “Ernest” comes to symbolize different things for different people. For Gwendolen and Cecily it “inspires absolute confidence” but also symbolizes the ideal husband/ lover. For Jack, “Ernest”…

Hypocrisy, Folly, and Victorian Morality
A witty wordsmith, Wilde exposes the hypocrisy of the Victorians’ strict social mores through puns, paradoxes, epigrams, and inversions in the characters’ actions and dialogue. For instance the characters often say and do the opposite of what they mean, or intend. Gwendolen flips “style” and “sincerity” when she says, “In matters of grave importance, style, not sincerity is the vital thing.” One would expect that “sincerity” should take precedence over “style” in “matters of grave…

Men and Women in Love
In the game of love that Wilde plays throughout The Importance of Being Earnest , Jack and Algernon , who strive for love, are pitted against the fickleness of the women they desire. Even though Wilde assigns stereotypical gender roles to each sex—Jack and Algernon are suave dandies , while Cecily and Gwendolen are vapid beauties—when it comes to marriage and love, he places women in a position of power because they are able to…

“The Importance of Being Earnest” by Oscar Wilde: Critical Analysis Essay
For some people, maintaining a good public appearance is more important than being honest with oneself and others. The play “The Importance of Being Earnest” was written by Oscar Wilde. The author intends to demonstrate the consequences of being two-faced. The main character, Jack Worthing, leads a double life because he pretends to be Earnest when escaping to London and indulging in behavior that Jack himself disapproves of. The theme of morality and earnestness is the core element of this play. This paper will critically analyze “The Importance of Being Earnest.”
Wilde depicts a Victorian society where upholding oneself to a high moral standard was a must. However, the author mocks this idea by showing that people find ways to overcome the restrictions that these moral standards place on them. For instance, Jack tells people that he has a younger brother, Earnest, who behaves immorally. Notably, Jack thinks that once he is married, he will no longer need an excuse in the form of Earnest. This detail may be a reference to viewing marriage as something that transforms an individual and makes them happy, although, in reality, marriage requires commitment and work. Hence, Wilde also uses the depiction of marriage as a way to show that societal standards and what society promotes are also not earnest because they create unrealistic expectations for marriage.
The author’s use of the name Earnest is another way of conveying the issue of moral standards and behavior. The word itself should characterize a person who is sincere and truthful. However, Wild creates a moral paradox because Jack, as Earnest, does things that others would judge. But Jack is not the only person who uses excuses to behave the way they want to without violating societal standards. His friend, Algernon, says, “a man who marries without knowing Bunbury has a very tedious time of it” (Wild 10). Bunbury is an imaginary friend whom Algernon uses as an excuse to avoid meetings that he does not want to attend.
Morality is an important topic that Wilde discloses through the lens of deception. The double life that Jack lives appears to be commonplace for the era. The presence of Bunbury suggests that appearance is more important than sincerity in the society that Wild depicts. Moreover, Gwendolen highlights the importance of a correct marriage proposal, and her brother practices it. For the aristocratic society, it is vital to ensure that a person maintains a good appearance, even though they may lead a double life.
The consequences of the conflict between earnestness and double life are shown in Act II. The two women, Gwendolen and Cecily, are fighting during a tea ceremony because both believe they are engaged to a man named Earnest. They talk about two men, each of whom called himself Earnest to hide their true identity. Although this play has a happy ending, both Jack and Algernon could have lost their fiances due to their lack of earnestness.
Overall, in this play, Wilde emphasizes the need, to be honest and sincere. The name of this play leads one to think about the feature of earnestness. The main character is not sincere or serious because he uses any excuse to behave the way he wants to and blames everything on his younger brother. Other characters, apart from Jack, convey the issue of morality and earnestness as well, for example, his best friend Algernon uses an excuse of a dying friend named Bunbury to get out of social occasions he does not want to be a part of.
Wilde, Oscar. The Importance of Being Earnest. Dover Publications, 1990.
- Chicago (A-D)
- Chicago (N-B)
IvyPanda. (2022, February 27). "The Importance of Being Earnest" by Oscar Wilde: Critical Analysis. https://ivypanda.com/essays/the-importance-of-being-earnest-by-oscar-wilde-critical-analysis/
""The Importance of Being Earnest" by Oscar Wilde: Critical Analysis." IvyPanda , 27 Feb. 2022, ivypanda.com/essays/the-importance-of-being-earnest-by-oscar-wilde-critical-analysis/.
IvyPanda . (2022) '"The Importance of Being Earnest" by Oscar Wilde: Critical Analysis'. 27 February.
IvyPanda . 2022. ""The Importance of Being Earnest" by Oscar Wilde: Critical Analysis." February 27, 2022. https://ivypanda.com/essays/the-importance-of-being-earnest-by-oscar-wilde-critical-analysis/.
1. IvyPanda . ""The Importance of Being Earnest" by Oscar Wilde: Critical Analysis." February 27, 2022. https://ivypanda.com/essays/the-importance-of-being-earnest-by-oscar-wilde-critical-analysis/.
Bibliography
IvyPanda . ""The Importance of Being Earnest" by Oscar Wilde: Critical Analysis." February 27, 2022. https://ivypanda.com/essays/the-importance-of-being-earnest-by-oscar-wilde-critical-analysis/.
- Representation of Food in the Importance of Being Earnest
- Marriage in the Importance of Being Earnest: Analysis
- Oscar Wilde’s “The Importance of Being Earnest”: Function of Conflict
- Analysis of "The Glass Menagerie" and "The Taming of the Shrew"
- Dorian Gray and His Downfall
- The Impact of Improper Conflict Resolution
- The Use of Symbolic Meaning in "A Rose for Emily" by Faulkner
- Yunior's Character in 'This Is How You Lose Her' by Junot DĂaz
The Importance of Being Earnest
By oscar wilde, the importance of being earnest essay questions.
Explain the pun of the title. Who is being Earnest in this play? Do Gwendolen and Cecily prefer having husbands named Ernest to having earnest husbands?
Analyze the gender reversals in this text. Does the feminized Lord Bracknell foreshadow what Jack and Algernon may become? Are males or females more passive/dominated in this play?
How does dramatic irony create humor in The Importance of Being Earnest? Identify a handful of instances in which the audience members know more about what is going on than the characters on stage. Why does this create humor?
What is the role of textuality in this play-do letters and diaries have a stronger reliability or ring of truth than conversation?
Analyze the various times that the characters eat in this play, primarily the cucumber sandwiches and the muffins. Does eating serve a primarily social or anti-social function?
Does Wilde prevent any version of true love? Does the extent to which Gwendolen and Cecily are self-centered affect your analysis?
Algernon observes that: "Women only call each other sister when they have called each other a lot of other things first." How does the development of the relationship between Cecily and Gwendolen bear out this remark? What causes them to bond together? What causes them to behave competitively?
What does the common classification of The Importance of being Earnest as a "comedy of manners" refer to? Could this play operate in a classless, non-hierarchical society?
How do words take on a life of their own in this play? How does this relate to why it is it so easy for Prism to substitute her manuscript for a baby? Analyze diaries as a source of power and truth-making.
Analyze the role of class in Lady Bracknell's worldview. If she more impressed by land, by nobility, or by wealth?
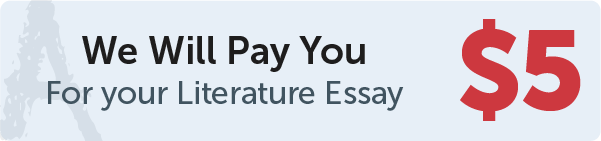
The Importance of Being Earnest Questions and Answers
The Question and Answer section for The Importance of Being Earnest is a great resource to ask questions, find answers, and discuss the novel.
What happened as a result of the following situation?
When Jack tells Lady Bracknell that Cecily will be a rich woman, Lady Bracknell immediately changes her mind about Cecily and decides that she will be a suitable wife for Algernon.
The Importance of Being Earnest by Oscar Wilde
Algernon's throwaway quip to Lane that "anyone can play [piano] accurately but I play with wonderful expression" is a good thumbnail of Wilde's philosophy of art. Wilde was heavily influenced by Walter Pater and the other aesthetes of the...
What can I say about the NARRATIVE TECHNIQUE ?
The play, The Importance of Being Earnest , does not have a narrator.
Study Guide for The Importance of Being Earnest
The Importance of Being Earnest study guide contains a biography of Oscar Wilde, literature essays, a complete e-text, quiz questions, major themes, characters, and a full summary and analysis.
- About The Importance of Being Earnest
- The Importance of Being Earnest Summary
- Character List
Essays for The Importance of Being Earnest
The Importance of Being Earnest essays are academic essays for citation. These papers were written primarily by students and provide critical analysis of The Importance of Being Earnest.
- Maxims and Masks: The Epigram in "The Importance of Being Earnest"
- Paradox through Pacing in Oscar Wilde's "The Importance of Being Earnest"
- Sincere Triviality: The Comedy of Oscar Wilde
- Structural Stereotypes of the Characters in The Importance of Being Earnest
- The Institution of Marriage in Oscar Wilde’s “The Importance of Being Earnest” and Susan Glaspell’s “Trifles”
Lesson Plan for The Importance of Being Earnest
- About the Author
- Study Objectives
- Common Core Standards
- Introduction to The Importance of Being Earnest
- Relationship to Other Books
- Bringing in Technology
- Notes to the Teacher
- Related Links
- The Importance of Being Earnest Bibliography
E-Text of The Importance of Being Earnest
The Importance of Being Earnest e-text contains the full text of The Importance of Being Earnest by Oscar Wilde.
Wikipedia Entries for The Importance of Being Earnest
- Introduction
- Composition
- Productions
“The Importance of Being Earnest” by Oscar Wilde
Welcome to our The Importance of Being Earnest essay sample! Here, you’ll find the analysis of the story’s main themes and comedy elements. Get ideas for your essay on The Importance of Being Earnest with our essay sample.
The Importance of Being Earnest Essay Thesis Statement
The importance of being earnest essay introduction, morality and marriage, why a comedy, the importance of being earnest essay conclusion, works cited.
Oscar Wilde had written during the Victorian time which was an era that laid much emphasis on moral values. It can be contended that The Importance of Being Earnest is in essence a play on morality since the major argument surfacing after its reading relates to honesty as being the best policy.
Although the learning from the play strengthens the values as prevailing during the period, there is quite a lot in the play that is not as per convention. The primary reason for Wilde’s success was that he was able to narrate an appealing story that further strengthened the prevailing social values. This he was able to do by making use of the untraditional relationships and images.
The Importance of Being Earnest was the last play written by Oscar Wilde and it undoubtedly became the most celebrated. George Bernard Shaw and H G Wells considered the play amongst the funniest that were ever written and to this day the play continues to absorb and entertain theatre lovers through out the world.
The play makes fun of the literary world, the aristocratic society and the customs and mannerisms of the British, while at the same time questions the concept of identity. The plans of the different characters in the play are seen to be going topsy-turvy due to the occurrence of unexpected developments. Wilde has skilfully taken up the issues of romantic gamesmanship, social ambitions and class pretensions through wit sharpened dialogues.
A major reason for the play’s success is the large number of spicy epigrams used by Wilde. Although some of the succinct and inconsistent statements relate to contemporary happenings, most of them are general manifestations of beauty, classes, women and men.
Most of the statements are being quoted to this day and keep on delighting the audience with their mix of absurdities and sophistication. Other than revealing the beauty, the play is a masterpiece in depicting Victorian styles as prevalent during the time, especially in relation to morality and marriage.
For long, marriage had been a significant issue and Wilde had depicted its scheming use as a social instrument of progression. Other than Miss Prism, all the ladies in the play are seen as having hidden motives in regard to romance. Wilde has convincingly criticized the superficial ways of politeness as practiced by society and has outlined the nature of the shallow masks that were worn by aristocratic Victorians.
A major source of humour in the play is the confused source of values as displayed by the characters. In this regard, Wilde had commented about the play as being “exquisitely trivial, a delicate bubble of fancy, and it has its philosophy that we should treat all the trivial things of life seriously, and all the serious things of life with sincere and studied triviality,” (Oscar Wilde, 2005).
Wilde had impressed upon his actors to speak out their words very seriously so that the audience did not think that they were joking. Although in essence the play is a comedy that relates to protocol, it has openly used ridiculous means to minimize its significance. Fortunately the audience is ever willing to ignore the inconsistency and indiscretions in the play.
Within the structure of the play one can feel the allusions of homosexuality implied in the male characters. It is known that while he was writing this play, Wilde was leading a twin life of a married man as also of a homosexual.
The original audiences of the play were utterly shocked at the reference of such a culture in the play and unfortunately for Wilde, the success of the play was not carried too further as his well known trial began after the opening night of the play and his career began to get loose.
There are two major issues forming the critique of The Importance of Being Earnest. Firstly, although the play was very well received by audiences when it opened for the first time, critics during the time openly questioned the moral aspects pertinent in the play.
The play was attacked by George Bernard Shaw for its “real degeneracy” (Bob Nelson, 1993), and described the playwright’s word play as being rather hateful and sinister. The second issue relates to the dramatic framework of the play in exhibiting aspects of parody, comedy of manners and mockery. Critics have been unable to come to a conclusion in regard to what category the play belongs to.
Critics are divided on the issue of morality in The Importance of Being Earnest. According to Edouard Roditi, who wrote the book Oscar Wilde, the playwright’s comedy did not rise higher than “the incomplete or the trivial” (Edouard Roditi, 1947).
Roditi felt that the play did not have ethical perspectives since no character saw through other characters nor criticized their values. Eric Bentley also felt the same way and concluded that “because of its ridiculous action, the play fails to break… into bitter criticism of serious issues” (Eric Bentley, 1987).
Otto Reinert has opined that Wilde’s comedy has had the effect of “an exposure both of hypocrisy and of the unnatural convention that necessitates hypocrisy” (Otto Reinert, 1956). Consequently there was a superficial cover up of the white lies that maintained politeness in the so called polite society, which alone was able to give the plot a moral meaning.
This is exemplified by the instance in the play when Algernon is criticized by Lady Bracknell for having taken care of his make believe friend, Bunberry who was supposed to make a decision whether he was going to die or live. In criticizing him she voices her conservative belief that “illness in others is always faked [and]… consequently sympathy with invalids is faked also” (Oscar Wilde, 2005)
Although Lady Bracknell is portrayed as respecting convention she is believed to have had no illusions about “the reality her professed convention is supposed to conceal” (Otto Reinert, 1956). She presumes that both Bunberry and Algeron are “bubburying” and she behaves in a way that “exposes the polite cynicism that negates all values save personal convenience and salon decorum” (Otto Reinert, 1956).
The lady’s behaviour is in the nature of exposing the polite cynicism in negating all desired values except salon decorum and personal convenience. Lady Bracknell is not protected from her own shortcomings in being extra earnest.
She disapproves of marriages amongst mercenaries and admits that when she had married Lord Bracknell she did not have any fortune, which implied that she was opposed to marrying for money, and that she was not in possession of much wealth at the time she married a wealthy man.
According to Reinert, “this position is neither cynical nor funny. It represents… [a] compromise between practical hardheadedness and conventional morality” (Otto Reinert, 1956).
In all, the play has not endorsed social dishonesty and for some time it makes a mockery of respectability. The use of paradoxical morality by Wilde has served as an evaluation of the “the problem of manners.”
This is so because Algeron, in trying to escape the pretence of conventions, becomes a hypocrite himself when he pretends to be a person that he actually is not. Wilde has conveyed that the so called Victorian morality forced people to lead a life of double standards, one that was frolicsome and another that was respectable, none of them being solemn.
A critical issue in the play relates to the categorization of the play. It has been described as a “farce that represents the reality that Victorian convention pretends to ignore” (Otto Reinert, 1956). The characters have not been ironic enough by way of saying something but meaning something else.
In fact they really mean what ever they state, which is evident from the fact that Algernon does not wish to attend lady Bracknell’s dinner party since she will invariably make him sit near Mary Farquhar who is in the habit of flirting while sitting with her own husband.
Reinert has written in this regard that, “Algernon is indignant with a woman who spoils the fun of extramarital flirtation and who parades her virtue. He is shocked at convention. And his tone implies that he is elevating break of convention into a moral norm,” (Otto Reinert, 1956). This makes things conventional out of unconventional situations.
Wilde’s comedy is seen as working through a caricature in transforming the techniques of comedy, plot situations and the characters.
The play has been defended against the charge that it was just a mockery because mockery “depends for its effects upon extremely simplified characters tangling themselves up in incongruous situations, as in Shakespeare’s The Comedy of Errors or Goldsmith’s She Stoops to Conquer.
Instead, the comedy of Earnest subsists, for the most part, not in action or situation but in dialogue, which is too witty and intellectual to be described simply as a farce,”(Forster, 1956).
Instead of being a comedy of manners or a mockery, Forster believed that Wilde used characters and familiar plot devices satirizing the Victorian community. The relationship that Jack has with Gwendolen symbolizes the problems faced by lovers in being forced to stay away from getting married due to class differences.
Wilde found a novel solution by establishing Jack’s patrimony in being the child at the railway station. A common feature of romantic literature pertains to falling in love at first sight which too is demonstrated by Wilde in total contrast when Cecily falls in love with Algernon, not at first sight but simply because she is under the impression that his name is Everest.
Although Algernon is depicted as being cynical, but there is evidence in indicating that such cynicism is shallow since after he met Cecily, “Algernon is engaged to be married and reconciled to getting christened,” (Forster, 1956).
In appearing to be innocent and protected, Cecily conveys that it would become a hypocritical situation if Algernon tries to be good while trying to project himself as being fiendish. According to Forster, “The moral of Wilde’s parody: the rake is a fake, girlish innocence is the bait of a monstrous mantrap, the wages of sin in matrimony,” (Forster, 1956).
In essence the dramatic troubles as identified by some critics in the play, are seen as being its strengths. Forster emphasizes that the whole point of the play lies in the machinations of its plot and the convenience outlined behind the numerous coincidences that are neatly placed in its resolutions.
Bob Nelson, The Importance of Being Earnest , A study Guide.
Edouard Roditi, Oscar Wilde, 1947, Norfolk: New Directions
Eric Bentley, The Playwright as Thinker, 1987, Harvest Books Foster, Richard. “Wilde as Parodist: A Second Look at The Importance Of Being Earnest.” October, 1956, College English
Oscar Wilde, The Importance of Being Earnest, 2005, Prestwick House Inc
Reinert, Otto. “Satiric Strategy in The Importance Of Being Earnest.” October, 1956, College English
Cite this paper
- Chicago (N-B)
- Chicago (A-D)
StudyCorgi. (2020, January 8). “The Importance of Being Earnest” by Oscar Wilde. https://studycorgi.com/the-importance-of-being-earnest-by-oscar-wilde/
"“The Importance of Being Earnest” by Oscar Wilde." StudyCorgi , 8 Jan. 2020, studycorgi.com/the-importance-of-being-earnest-by-oscar-wilde/.
StudyCorgi . (2020) '“The Importance of Being Earnest” by Oscar Wilde'. 8 January.
1. StudyCorgi . "“The Importance of Being Earnest” by Oscar Wilde." January 8, 2020. https://studycorgi.com/the-importance-of-being-earnest-by-oscar-wilde/.
Bibliography
StudyCorgi . "“The Importance of Being Earnest” by Oscar Wilde." January 8, 2020. https://studycorgi.com/the-importance-of-being-earnest-by-oscar-wilde/.
StudyCorgi . 2020. "“The Importance of Being Earnest” by Oscar Wilde." January 8, 2020. https://studycorgi.com/the-importance-of-being-earnest-by-oscar-wilde/.
This paper, ““The Importance of Being Earnest” by Oscar Wilde”, was written and voluntary submitted to our free essay database by a straight-A student. Please ensure you properly reference the paper if you're using it to write your assignment.
Before publication, the StudyCorgi editorial team proofread and checked the paper to make sure it meets the highest standards in terms of grammar, punctuation, style, fact accuracy, copyright issues, and inclusive language. Last updated: June 12, 2022 .
If you are the author of this paper and no longer wish to have it published on StudyCorgi, request the removal . Please use the “ Donate your paper ” form to submit an essay.
Plot Summary for the Importance of being Earnest
This essay about Oscar Wilde’s “The Importance of Being Earnest” explores the satire and themes of identity, sincerity, and social hypocrisy in Victorian society. It highlights the characters’ comedic misadventures and deceit as a critique of the superficial values of the time. The essay also connects the play’s message about authenticity to contemporary issues around personal integrity and the pressure of societal expectations.
How it works
In a world where sincerity often feels like a scarce commodity, the enduring relevance of Oscar Wilde’s “The Importance of Being Earnest” shines through like a beacon of authenticity amidst a sea of pretense. With its razor-sharp wit and clever satire, Wilde’s masterpiece not only entertains but also provokes profound reflection on the significance of honesty and genuineness in a society often obsessed with appearances.
Set against the backdrop of Victorian England, a time marked by strict social norms and rigid expectations, “The Importance of Being Earnest” follows the escapades of two bachelors, Jack Worthing and Algernon Moncrieff, as they navigate the complexities of love, identity, and societal conventions.
Through their hilarious misadventures and tangled web of deceit, Wilde invites us to question the facade of respectability and the consequences of living a life built on falsehoods.
At the heart of the play lies the theme of identity, as Jack assumes the alter ego of Ernest in order to escape the responsibilities of his rural life and indulge in the pleasures of London society. However, it is only through the unraveling of his deception and the discovery of his true parentage that Jack comes to understand the true importance of being earnest.
Wilde’s characters are larger than life, each a caricature of the hypocrisy and superficiality prevalent in Victorian society. From the formidable Lady Bracknell, whose obsession with social status and lineage knows no bounds, to the vivacious Gwendolen Fairfax, whose romantic ideals are shattered by the revelation of Jack’s true identity, the characters in “The Importance of Being Earnest” serve as both comic foils and cautionary tales.
Through his trademark wit and biting satire, Wilde exposes the absurdity of societal conventions and the folly of placing undue importance on appearances. The play’s famous one-liners and witty repartee are not merely comedic devices but potent critiques of the hypocrisy and pretension that plagued Wilde’s society.
Yet, amidst the laughter and absurdity, “The Importance of Being Earnest” imparts a timeless message about the transformative power of honesty and authenticity. It is only when Jack embraces his true self and declares, “I’ve now realized for the first time in my life the vital Importance of Being Earnest,” that he is able to find true happiness and fulfillment.
The importance of being earnest extends far beyond the confines of Wilde’s play, resonating deeply in today’s world where authenticity is often overshadowed by artifice and pretense. In a society dominated by social media and the pressure to present a carefully curated image of oneself, the value of sincerity and genuineness cannot be overstated.
Being earnest is more than just telling the truth; it is a commitment to living with integrity and authenticity in all aspects of life. It means being true to oneself and others, even when it may be difficult or inconvenient. It is about forging genuine connections based on trust and mutual respect, rather than shallow appearances or superficiality.
“The Importance of Being Earnest” serves as a timeless reminder of the importance of authenticity and sincerity in a world that often prizes conformity over individuality. Through its sharp wit and memorable characters, Wilde’s play challenges us to embrace the vital importance of being earnest in our own lives, for it is only by being true to ourselves and others that we can truly find happiness and fulfillment.

Cite this page
Plot Summary for The Importance of Being Earnest. (2024, May 01). Retrieved from https://papersowl.com/examples/plot-summary-for-the-importance-of-being-earnest/
"Plot Summary for The Importance of Being Earnest." PapersOwl.com , 1 May 2024, https://papersowl.com/examples/plot-summary-for-the-importance-of-being-earnest/
PapersOwl.com. (2024). Plot Summary for The Importance of Being Earnest . [Online]. Available at: https://papersowl.com/examples/plot-summary-for-the-importance-of-being-earnest/ [Accessed: 12 May. 2024]
"Plot Summary for The Importance of Being Earnest." PapersOwl.com, May 01, 2024. Accessed May 12, 2024. https://papersowl.com/examples/plot-summary-for-the-importance-of-being-earnest/
"Plot Summary for The Importance of Being Earnest," PapersOwl.com , 01-May-2024. [Online]. Available: https://papersowl.com/examples/plot-summary-for-the-importance-of-being-earnest/. [Accessed: 12-May-2024]
PapersOwl.com. (2024). Plot Summary for The Importance of Being Earnest . [Online]. Available at: https://papersowl.com/examples/plot-summary-for-the-importance-of-being-earnest/ [Accessed: 12-May-2024]
Don't let plagiarism ruin your grade
Hire a writer to get a unique paper crafted to your needs.
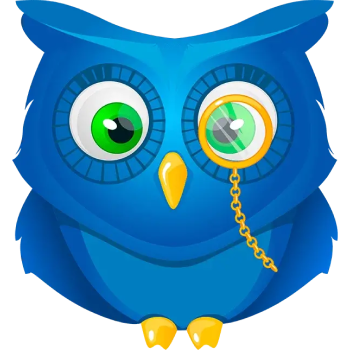
Our writers will help you fix any mistakes and get an A+!
Please check your inbox.
You can order an original essay written according to your instructions.
Trusted by over 1 million students worldwide
1. Tell Us Your Requirements
2. Pick your perfect writer
3. Get Your Paper and Pay
Hi! I'm Amy, your personal assistant!
Don't know where to start? Give me your paper requirements and I connect you to an academic expert.
short deadlines
100% Plagiarism-Free
Certified writers
Academia.edu no longer supports Internet Explorer.
To browse Academia.edu and the wider internet faster and more securely, please take a few seconds to upgrade your browser .
Enter the email address you signed up with and we'll email you a reset link.
- We're Hiring!
- Help Center
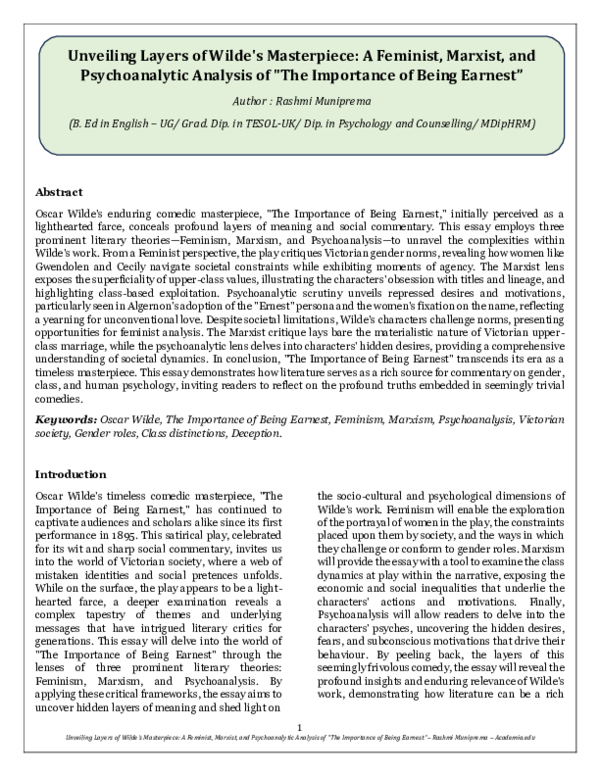
A Feminist Marxist and Psychoanalytic Analysis of The Importance of Being Earnest

2023, Unveiling Layers of Wilde's Masterpiece: A Feminist, Marxist, and Psychoanalytic Analysis of "The Importance of Being Earnest”
Oscar Wilde's enduring comedic masterpiece, "The Importance of Being Earnest," initially perceived as a lighthearted farce, conceals profound layers of meaning and social commentary. This essay employs three prominent literary theories-Feminism, Marxism, and Psychoanalysis-to unravel the complexities within Wilde's work. From a Feminist perspective, the play critiques Victorian gender norms, revealing how women like Gwendolen and Cecily navigate societal constraints while exhibiting moments of agency. The Marxist lens exposes the superficiality of upper-class values, illustrating the characters' obsession with titles and lineage, and highlighting class-based exploitation. Psychoanalytic scrutiny unveils repressed desires and motivations, particularly seen in Algernon's adoption of the "Ernest" persona and the women's fixation on the name, reflecting a yearning for unconventional love. Despite societal limitations, Wilde's characters challenge norms, presenting opportunities for feminist analysis. The Marxist critique lays bare the materialistic nature of Victorian upperclass marriage, while the psychoanalytic lens delves into characters' hidden desires, providing a comprehensive understanding of societal dynamics. In conclusion, "The Importance of Being Earnest" transcends its era as a timeless masterpiece. This essay demonstrates how literature serves as a rich source for commentary on gender, class, and human psychology, inviting readers to reflect on the profound truths embedded in seemingly trivial comedies.
Related Papers
Maroof Ahmed
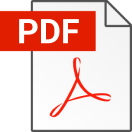
This paper attempts to present how reality and fiction intersect in Wilde's The Importance of Being Earnest to challenge, if not subvert, social obligations and perception of identity in Victorian society. In so doing, the paper critically attempts to touch upon the concepts of duality and appearance as they possess the utmost importance for the Victorian sense of morality. The article also strives to show how Wilde undermines the basis of the truthful representation of gender identity instead of the Victorian perception of the term. In the play, as the paper argues, Wilde hints at the idea that there is a difference if we can call it a duality of identity between the appearance and what is hidden beneath. In the case of the fictional characters, they wear fake identities or imagine a view of identity to suit the public's expectations, challenging the perception of stable autonomous identity that the Victorian believed. However, the inner and outer worlds of the characters are pretty different from inside and outside, so that they constantly +vacillate in-between these identities. The paper concludes that, as Wilde hints, it is impossible to define a person fully when they display various identities simultaneously as in the modern sense.
This essay discusses how Wilde addresses the very nature of being. In repressive Victorian society, he chronicles the emergence of a self that is hidden and double and thus must exist at the margin, if not in the shadows. The result in Wilde’s private life is an identity in flux that reveals him as an identity migrant, who at one moment is the Victorian father and husband, and at another, the homosexual lover of Lord Alfred Douglas.
Modern Drama
Sarah Balkin
Oscar Wilde’s “The Portrait of Mr. W.H.” (1889) and The Importance of Being Earnest (1895) both centrally feature imaginary persons. In “The Portrait of Mr. W.H.,” Wilde’s narrator says that “all Art” is “to a certain degree a mode of acting, an attempt to realise one’s own personality.” The Importance of Being Earnest assigns actors’ bodies to the imaginary person of the title. My essay examines what it meant to realize a personality on the late-nineteenth-century stage in light of recent scholarship on character, stage properties, and materiality. I argue that – because theatre shows the constructedness of material and corporeal being, because farce renders male identity a matter of genre, and because Wilde unifies the characters’ desires under one name – The Importance of Being Earnest uniquely locates personality in a living human body.
Yakaiah Kathy
Caroline Lee
Facta Universitatis (Series Linguistics and …
Magdalena Nigoevic
Akshay Kumar Roy
Adilah Zabir
HAL (Le Centre pour la Communication Scientifique Directe)
Svetlana Bochman
RELATED PAPERS
Fernando Blanco Vaca
Proceedings of XXIX International Symposium on Lattice Field Theory — PoS(Lattice 2011)
Piero Vicini
Acta Biologica Colombiana
Gabriel Pinilla Agudelo
Journal of Oncology Pharmacy Practice
Christopher Negrete
Jurnal Analisa
Yayu Rahayu
Annals of cardiothoracic surgery
Michael Vallely
Journal of Geophysical Research: Planets
Biochemical Society Transactions
Anders Grubb
PROCEEDINGS OF THE II INTERNATIONAL SCIENTIFIC CONFERENCE ON ADVANCES IN SCIENCE, ENGINEERING AND DIGITAL EDUCATION: (ASEDU-II 2021)
Noor Hidayat
Balıkesir Üniversitesi Sosyal Bilimler Enstitüsü
Nedim Ă–zer HoĹźol
LĂgia Almeida
Angela K Murray
Journal of Organometallic Chemistry
Wolfgang Petz
The Journal of the Acoustical Society of America
Arden Huang
Maaike Dooper
Scientia Iranica
Joseph Ribeiro
Journal of Cardiology Cases
Shokoufeh Hajsadeghi
Jurnal Madani: Ilmu Pengetahuan, Teknologi, dan Humaniora
ivan putranto
CALL GIRL IN Kalkaji,, DELHI NCR
delhi munirka
Call Girls In Ashok Nagar
Antimicrobial Agents and Chemotherapy
Patricia Roblin
The Poznań University of Economics Review
Radoslaw Wolniak
실시간카지노 í† í† ě‚¬ěť´íŠ¸
RELATED TOPICS
- We're Hiring!
- Help Center
- Find new research papers in:
- Health Sciences
- Earth Sciences
- Cognitive Science
- Mathematics
- Computer Science
- Academia ©2024
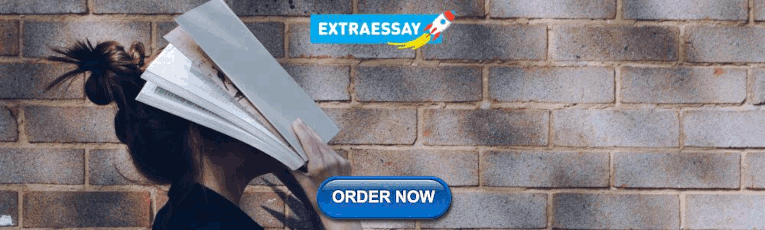
IMAGES
VIDEO
COMMENTS
The Importance of Being Earnest has a good deal to say about the nature of deceptive or superficial appearances, including the illusion of virtue that Jack projects and the signs of elegance, status, and propriety that Gwendolen, Lady Bracknell, and Miss Prism covet, as well as the phenomenon of hypocrisy, a word that derives from the ancient ...
The Importance of Being Earnest by Oscar Wilde, first performed in 1895, is a comedic play that satirizes the conventions and manners of Victorian society.The subtitle of the play, A Trivial Comedy for Serious People, aptly captures Wilde's tongue-in-cheek take on the cultural milieu to which he was subject.Set in England during the late 19th century, the play follows the lives of two young ...
Welcome to a delightful journey through Oscar Wilde's masterpiece, "The Importance of Being Earnest." 🎠This play, first performed in 1895, is a brilliant satire of the Victorian era's social mores and attitudes, especially concerning marriage and the pursuit of love. Wilde, known for his sharp wit and flamboyant style, was one of ...
During the initial run of The Importance of Being Earnest, Lord Alfred's father, the Marquess of Queensberry, accused Wilde of being a "somdomite" (sic). Under his lover's influence, Wilde countered by suing the Marquess for libel. Queensberry was acquitted, but enough evidence of Wilde's homosexuality surfaced during the first trial that Wilde was charged with "gross indecency."
The Project Gutenberg eBook of The Importance of Being Earnest, by Oscar Wilde. This eBook is for the use of anyone anywhere in the United States and most other parts of the world at no cost and with almost no restrictions whatsoever. You may copy it, give it away or re-use it under the terms of the Project Gutenberg License included with this ...
To modern theatre audiences, the title of Oscar Wilde's most popular play, The Importance of Being Earnest, seems a clever play on words. After all, the plot hinges on the telling of little—and ...
New York: New York University Press, 1980. Includes two excellent essays on The Importance of Being Earnest, as well as many allusions to it. Discusses the play as a conduit for self-discovery for ...
Karl Beckson. The Importance of Being Earnest, play in three acts by Oscar Wilde, performed in 1895 and published in 1899. A satire of Victorian social hypocrisy, the witty play is considered Wilde's greatest dramatic achievement. Jack Worthing is a fashionable young man who lives in the country with his ward,
Critical Overview. Two major issues predominate much of The Importance of Being Earnest 's criticism. First, while audiences from the play's opening have warmly received it, Wilde's contemporaries ...
The thought of meeting someone who lives outside the bounds of prudery and rules is exciting to naïve Cecily. Even using the name Ernest for his secret life is ironic because Algernon is not being dutiful — earnest — in living a secret life. Various characters in the play allude to passion, sex and moral looseness.
The Importance of Being Earnest Analysis. The playwright masterfully dissects the hypocrisies and absurdities of the Victorian upper class through a lens of satire and humor. Let's delve deeper into the analysis of The Importance of Being Earnest and examine the key elements of this timeless play: 1. Themes:
The Importance of Being Earnest. Composed by Oscar Wilde to be first played at the St. James Theatre of London in the year of 1895, The Importance of Being Ernest has since named itself one notable satire among the blooming era of comedies that dwell on the hypocrisy of the upper... The Importance of Being Earnest essays are academic essays for ...
LitCharts assigns a color and icon to each theme in The Importance of Being Earnest, which you can use to track the themes throughout the work. The Art of Deception: Fact v. Fiction. As a leader of the Aesthetic movement, Wilde was especially interested in the relationship between life and art, pondering the eternal question, "Does art ...
Suggested Essay Topics. 1. What is the overall effect of the play's references to death? How is death, as a theme, dealt with in the play? 2. Is Cecily a more realistic character than Gwendolen? Why or why not? 3. Which union—Jack's with Gwendolen or Algernon's with Cecily—seems more likely to succeed?
The play "The Importance of Being Earnest" was written by Oscar Wilde. The author intends to demonstrate the consequences of being two-faced. The main character, Jack Worthing, leads a double life because he pretends to be Earnest when escaping to London and indulging in behavior that Jack himself disapproves of.
The Importance of Being Earnest Full Play Summary. Jack Worthing, the play's protagonist, is a pillar of the community in Hertfordshire, where he is guardian to Cecily Cardew, the pretty, eighteen-year-old granddaughter of the late Thomas Cardew, who found and adopted Jack when he was a baby. In Hertfordshire, Jack has responsibilities: he is ...
The Importance of Being Earnest Questions and Answers. The Question and Answer section for The Importance of Being Earnest is a great resource to ask questions, find answers, and discuss the novel. What happened as a result of the following situation? When Jack tells Lady Bracknell that Cecily will be a rich woman, Lady Bracknell immediately ...
Essays and criticism on Oscar Wilde's The Importance of Being Earnest - Critical Essays. Select an area of the website to search. Search this site Go Start an essay Ask a question Join Sign ...
25 essay samples found. The Importance of Being Earnest is a comedic play by Oscar Wilde that satirizes the Victorian social norms and aristocratic pretenses. Essays on this play might explore Wilde's witty dialogue, the social commentary embedded within the humor, or the characters' quest for personal freedom amidst societal expectations.
Manuscripts are used by various characters — diaries, sermons, and a three-volume novel. What function does each have in the play? 5. What attitudes of the aristocracy can be seen in Lady Bracknell's dialogue? 6. How is conflict developed in the play? 7. How does Wilde turn around well-known proverbs or epigrams to comment on Victorian attitudes?
The Importance of Being Earnest Essay Introduction The Importance of Being Earnest was the last play written by Oscar Wilde and it undoubtedly became the most celebrated. George Bernard Shaw and H G Wells considered the play amongst the funniest that were ever written and to this day the play continues to absorb and entertain theatre lovers ...
Importance of Being Earnest Essay: Importance of being earnest is a very famous play by Oscar Wilde, a comedy for primer readers. St. James theatre in London witnessed the first performance of the story. The drama is a farcical comedy in which the hero maintains fictitious personae. There are short and long essays written to help you in studies ...
Essay Example: In a world where sincerity often feels like a scarce commodity, the enduring relevance of Oscar Wilde's "The Importance of Being Earnest" shines through like a beacon of authenticity amidst a sea of pretense. With its razor-sharp wit and clever satire, Wilde's masterpiece not
Oscar Wilde's "The Portrait of Mr. W.H." (1889) and The Importance of Being Earnest (1895) both centrally feature imaginary persons. In "The Portrait of Mr. W.H.," Wilde's narrator says that "all Art" is "to a certain degree a mode of acting, an attempt to realise one's own personality."